Best AI tools for< Learning Evaluator >
Infographic
20 - AI tool Sites
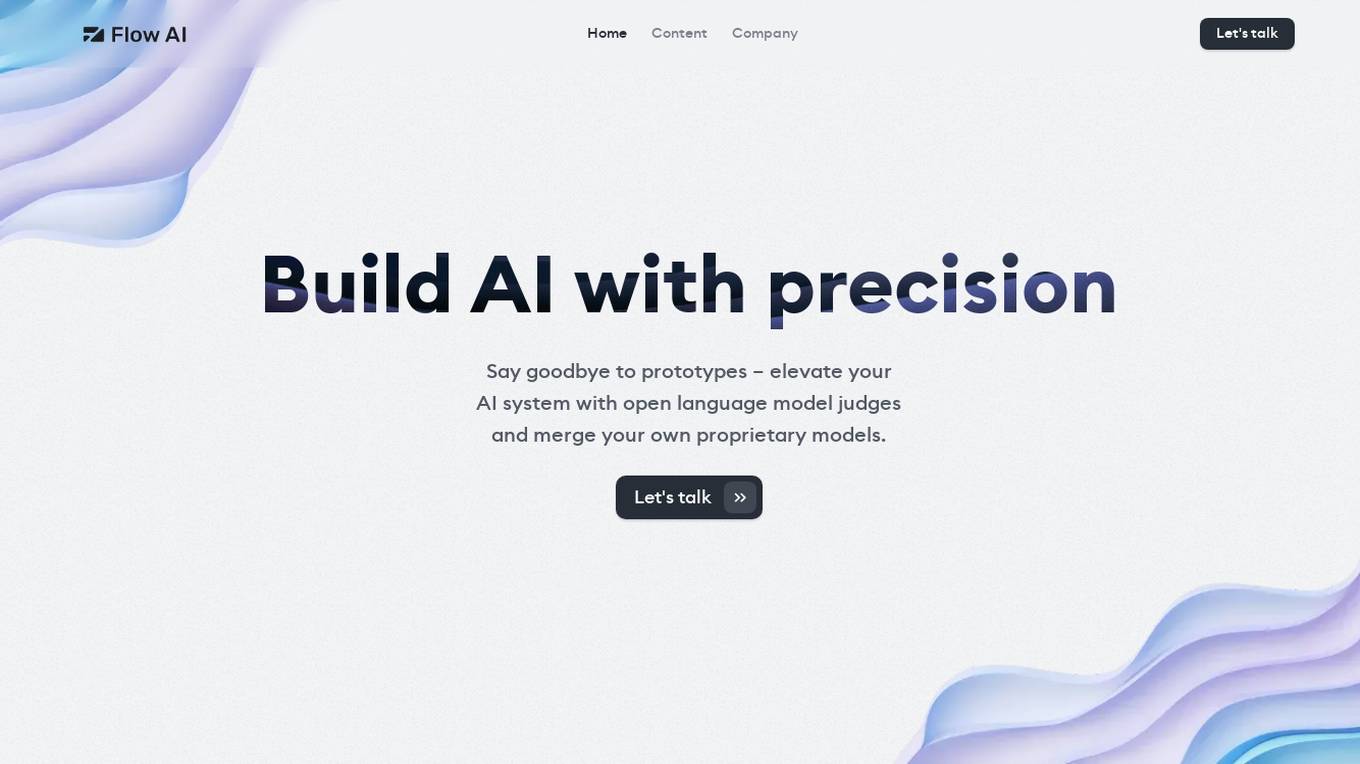
Flow AI
Flow AI is an advanced AI tool designed for evaluating and improving Large Language Model (LLM) applications. It offers a unique system for creating custom evaluators, deploying them with an API, and developing specialized LMs tailored to specific use cases. The tool aims to revolutionize AI evaluation and model development by providing transparent, cost-effective, and controllable solutions for AI teams across various domains.
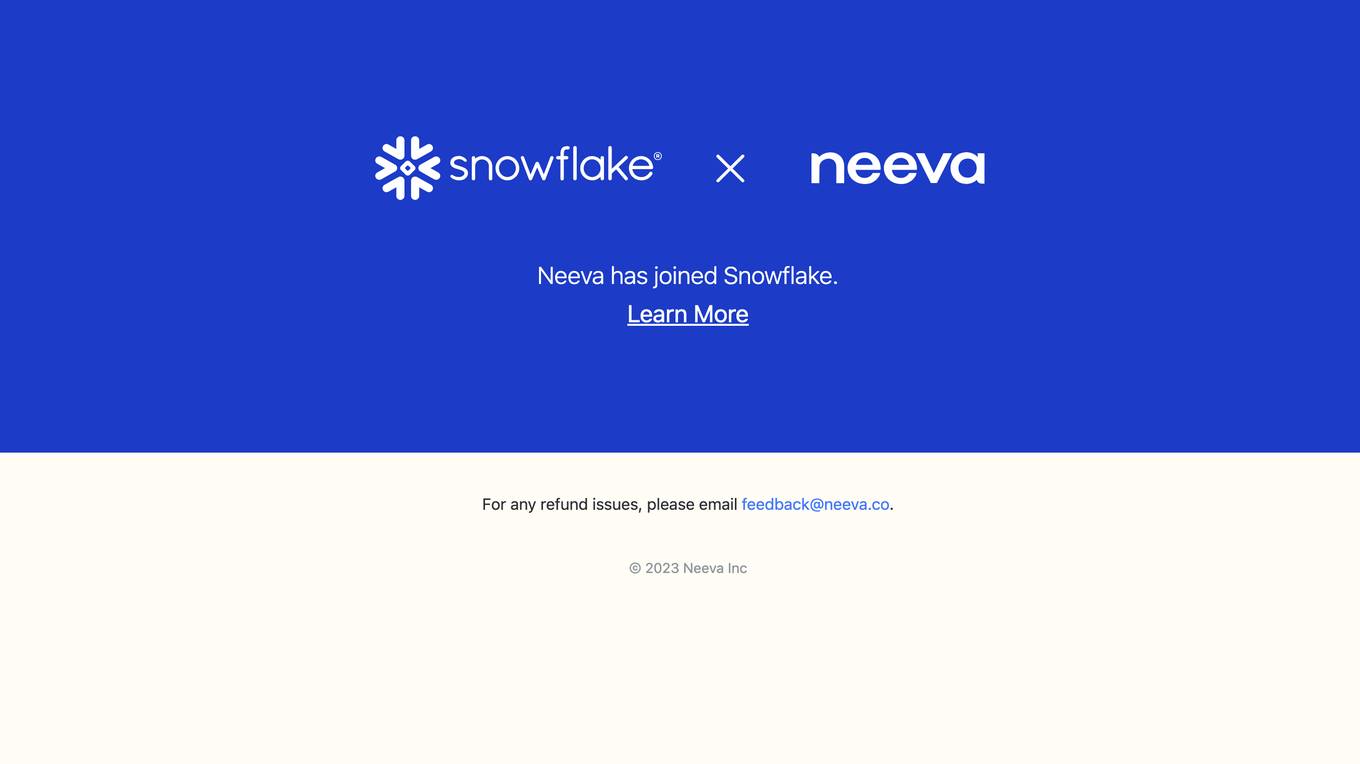
Neeva
Neeva is a search engine powered by artificial intelligence. It offers a variety of features, including the ability to search the web, images, videos, and news. Neeva also offers a number of privacy features, such as the ability to search without being tracked and the ability to delete your search history. Neeva is available as a desktop application and as a mobile app.
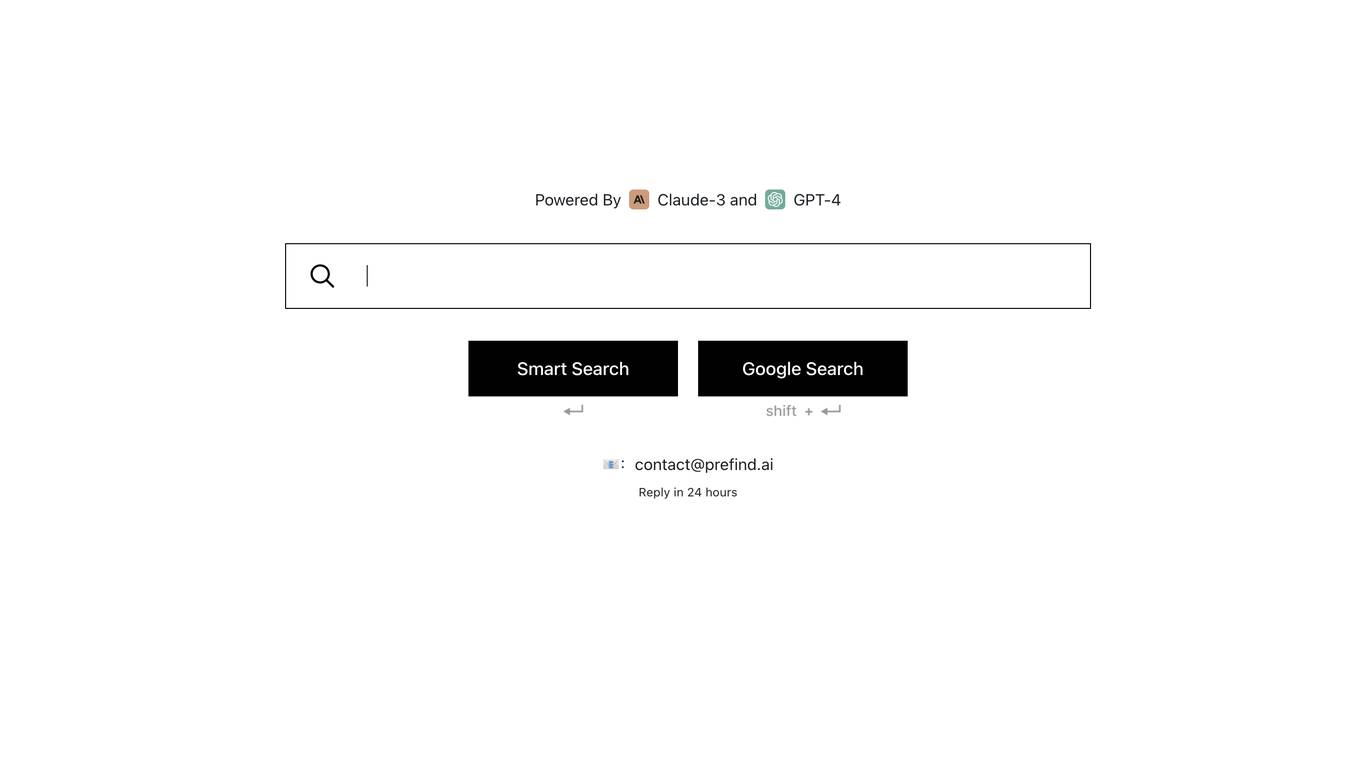
Prefind
Prefind is an AI-powered search engine that utilizes the capabilities of advanced language models like Claude-3 and GPT-4. It aims to provide users with comprehensive and relevant search results, leveraging the power of AI to enhance the search experience.
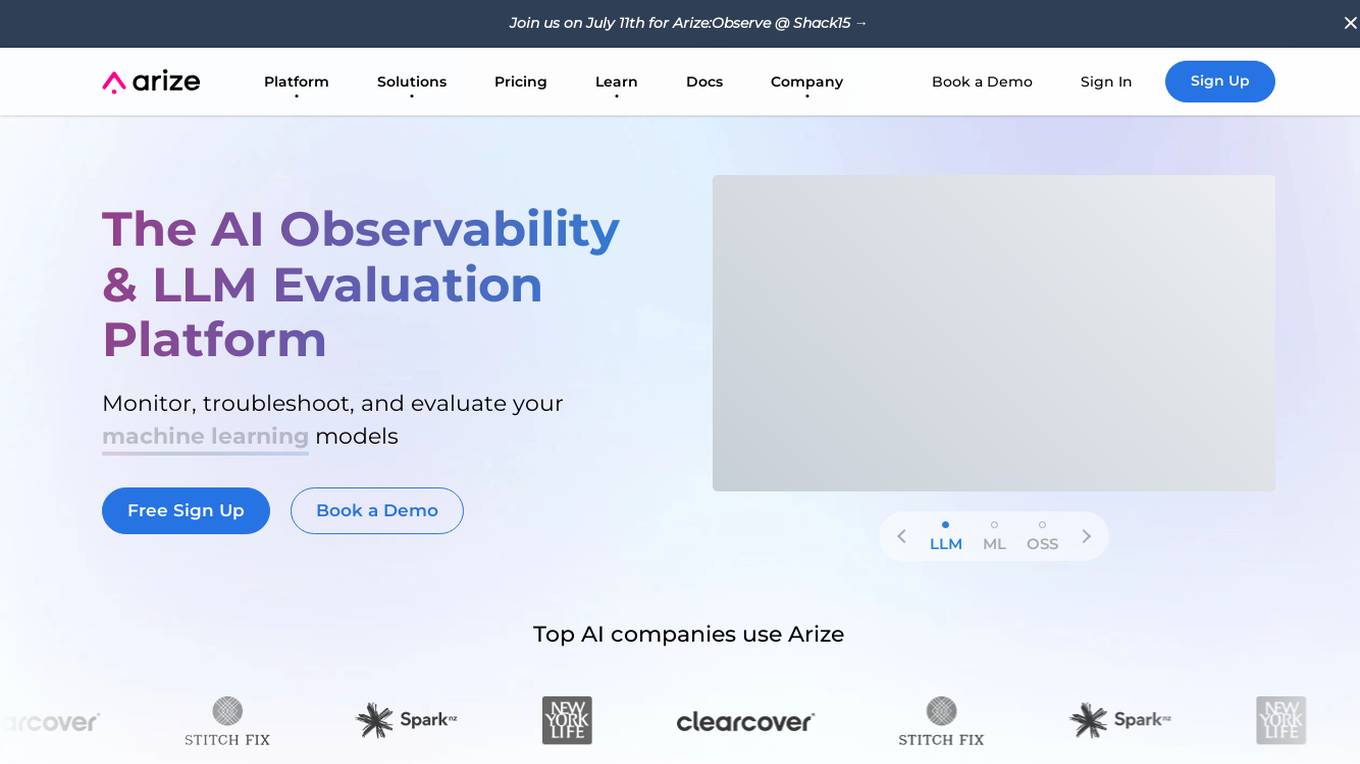
Arize AI
Arize AI is an AI Observability & LLM Evaluation Platform that helps you monitor, troubleshoot, and evaluate your machine learning models. With Arize, you can catch model issues, troubleshoot root causes, and continuously improve performance. Arize is used by top AI companies to surface, resolve, and improve their models.
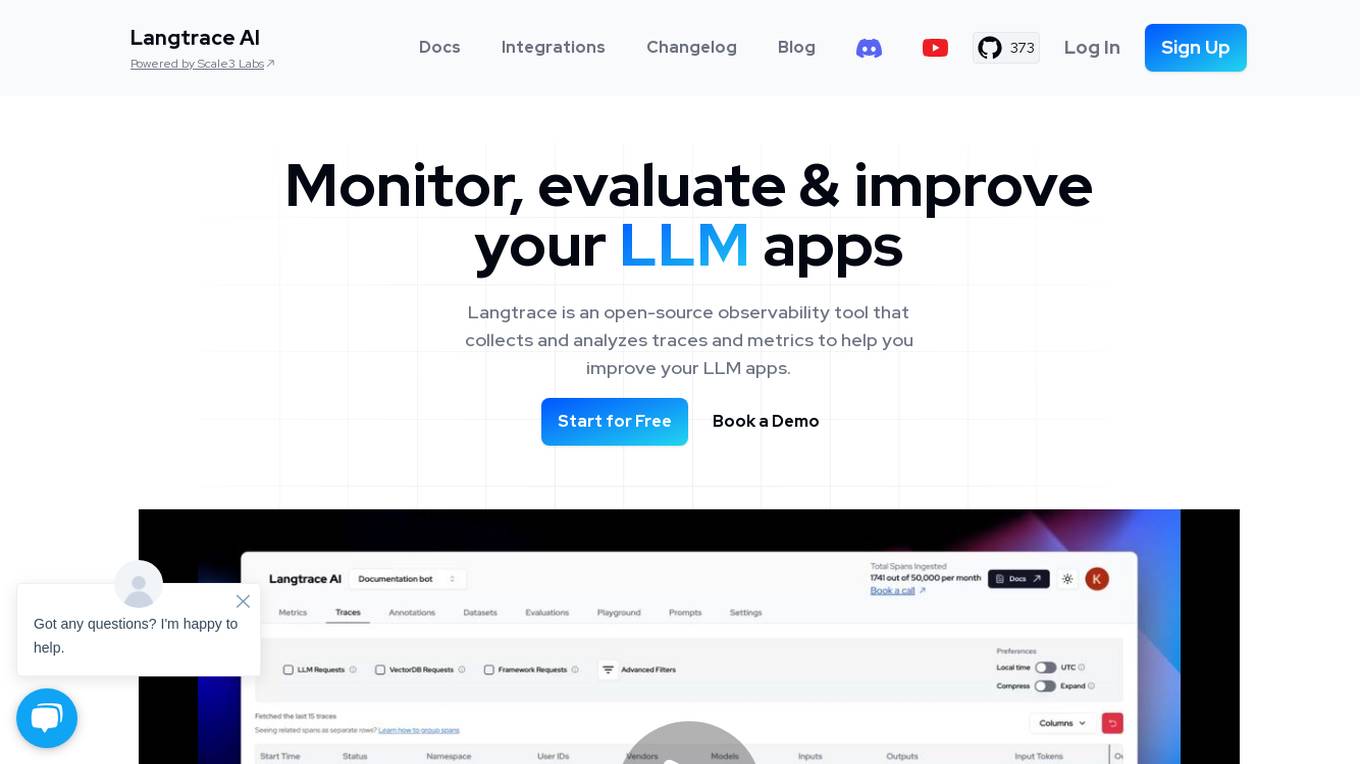
Langtrace AI
Langtrace AI is an open-source observability tool powered by Scale3 Labs that helps monitor, evaluate, and improve LLM (Large Language Model) applications. It collects and analyzes traces and metrics to provide insights into the ML pipeline, ensuring security through SOC 2 Type II certification. Langtrace supports popular LLMs, frameworks, and vector databases, offering end-to-end observability and the ability to build and deploy AI applications with confidence.
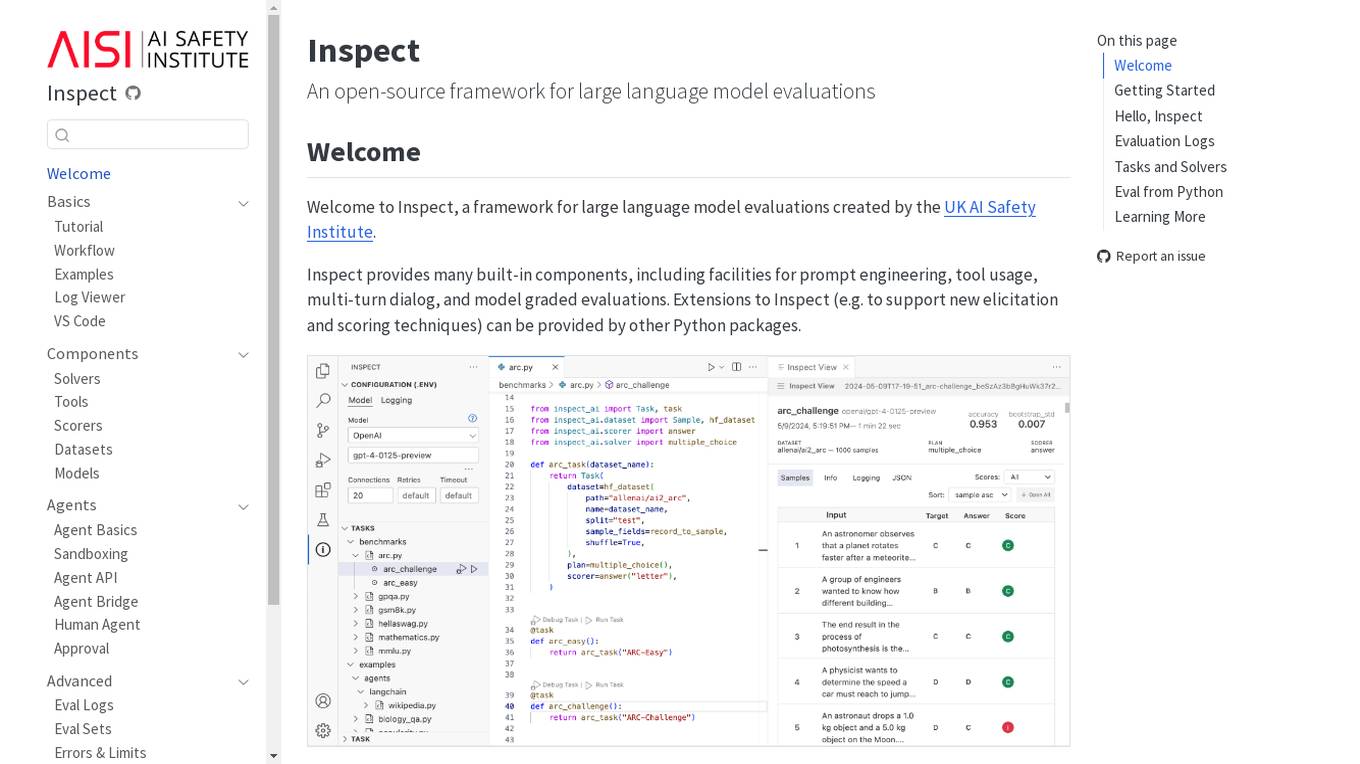
Inspect
Inspect is an open-source framework for large language model evaluations created by the UK AI Safety Institute. It provides built-in components for prompt engineering, tool usage, multi-turn dialog, and model graded evaluations. Users can explore various solvers, tools, scorers, datasets, and models to create advanced evaluations. Inspect supports extensions for new elicitation and scoring techniques through Python packages.
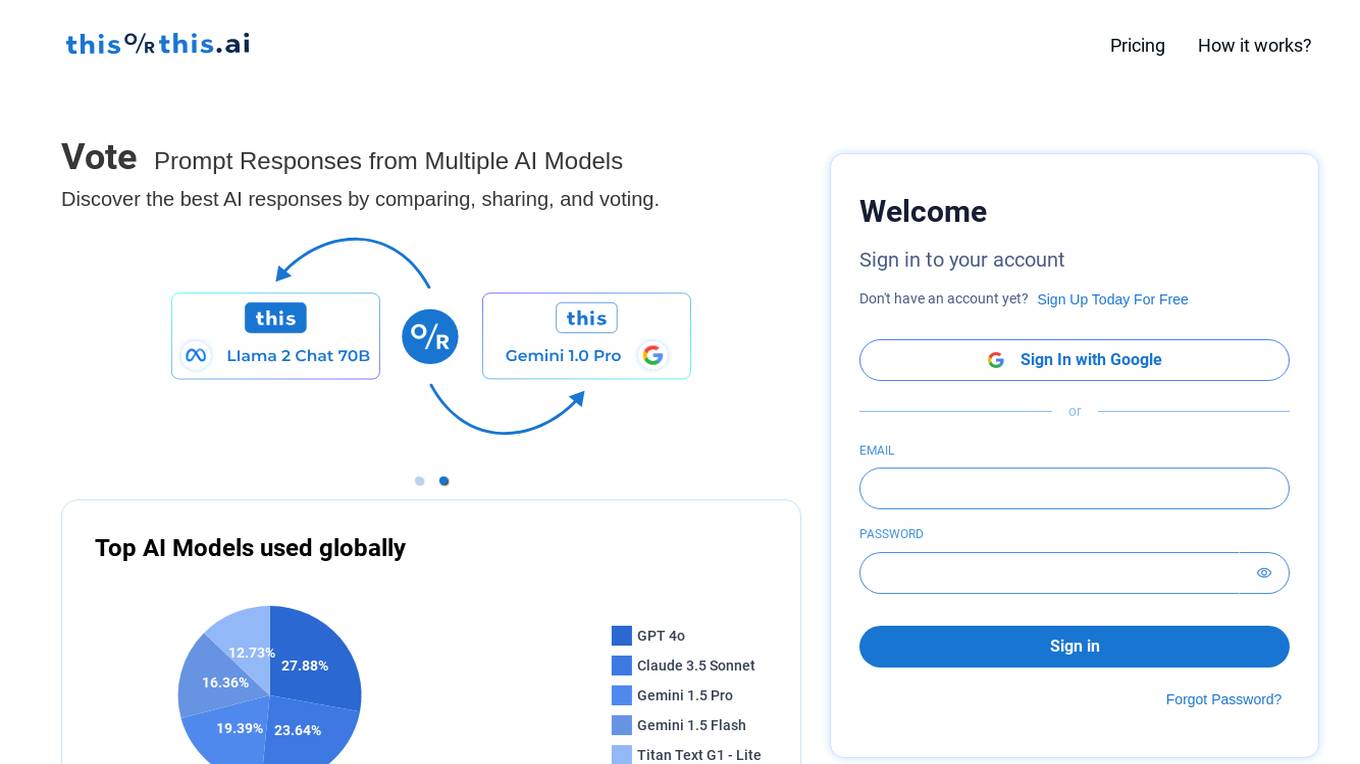
thisorthis.ai
thisorthis.ai is an AI tool that allows users to compare generative AI models and AI model responses. It helps users analyze and evaluate different AI models to make informed decisions. The tool requires JavaScript to be enabled for optimal functionality.
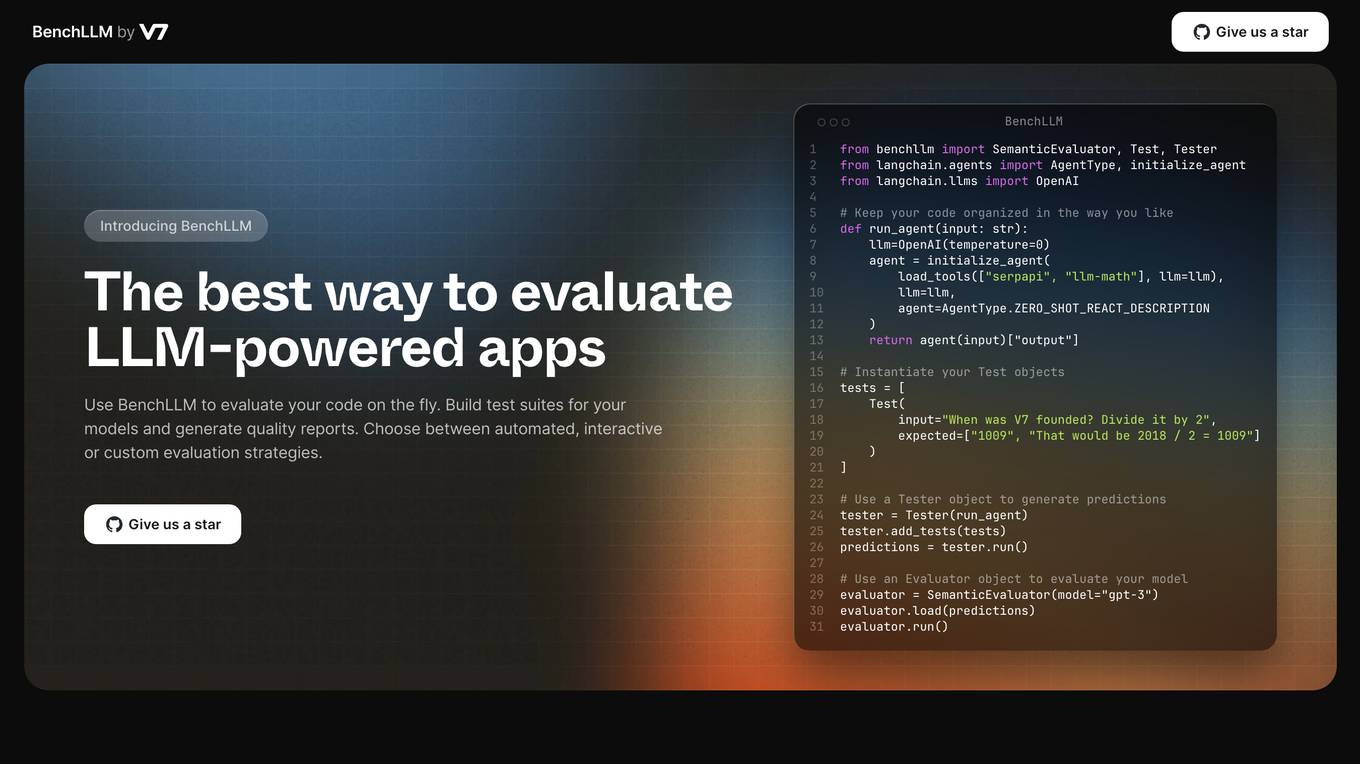
BenchLLM
BenchLLM is an AI tool designed for AI engineers to evaluate LLM-powered apps by running and evaluating models with a powerful CLI. It allows users to build test suites, choose evaluation strategies, and generate quality reports. The tool supports OpenAI, Langchain, and other APIs out of the box, offering automation, visualization of reports, and monitoring of model performance.
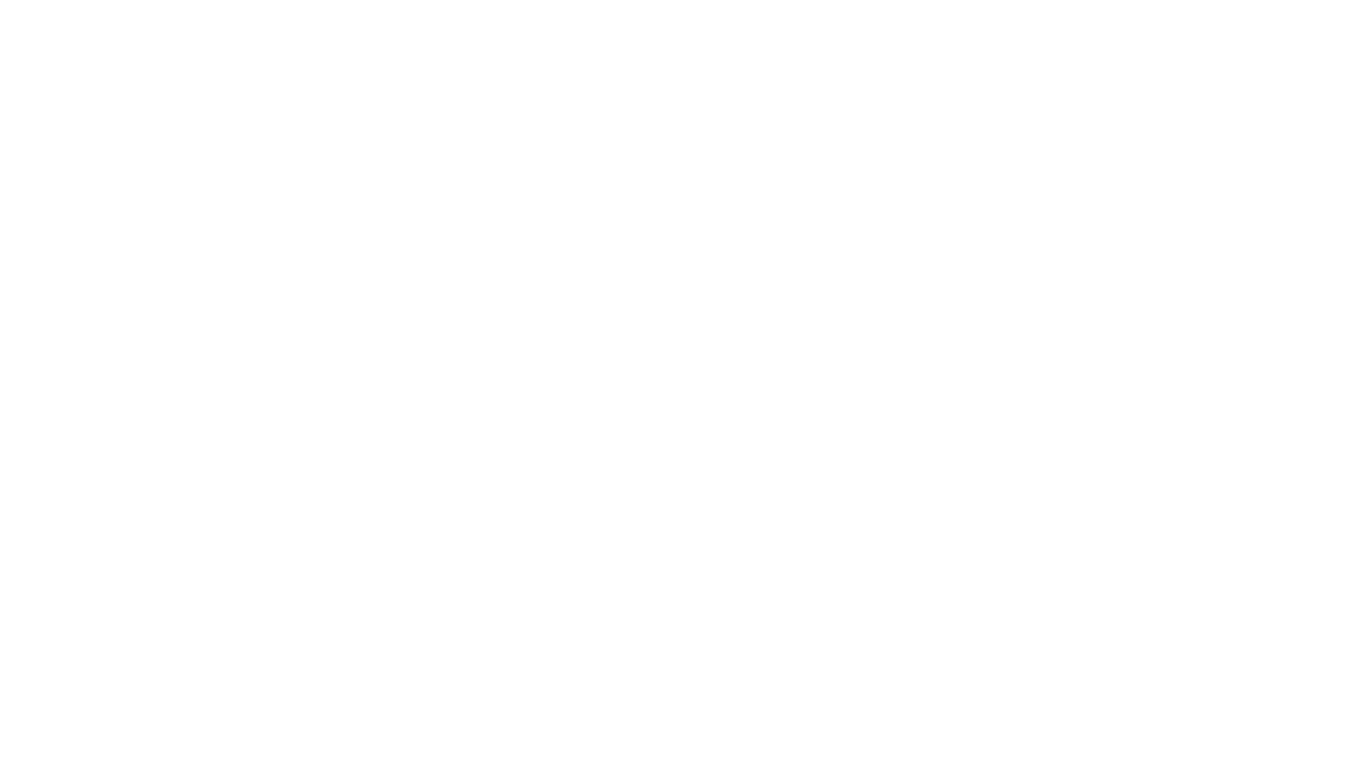
HappyML
HappyML is an AI tool designed to assist users in machine learning tasks. It provides a user-friendly interface for running machine learning algorithms without the need for complex coding. With HappyML, users can easily build, train, and deploy machine learning models for various applications. The tool offers a range of features such as data preprocessing, model evaluation, hyperparameter tuning, and model deployment. HappyML simplifies the machine learning process, making it accessible to users with varying levels of expertise.
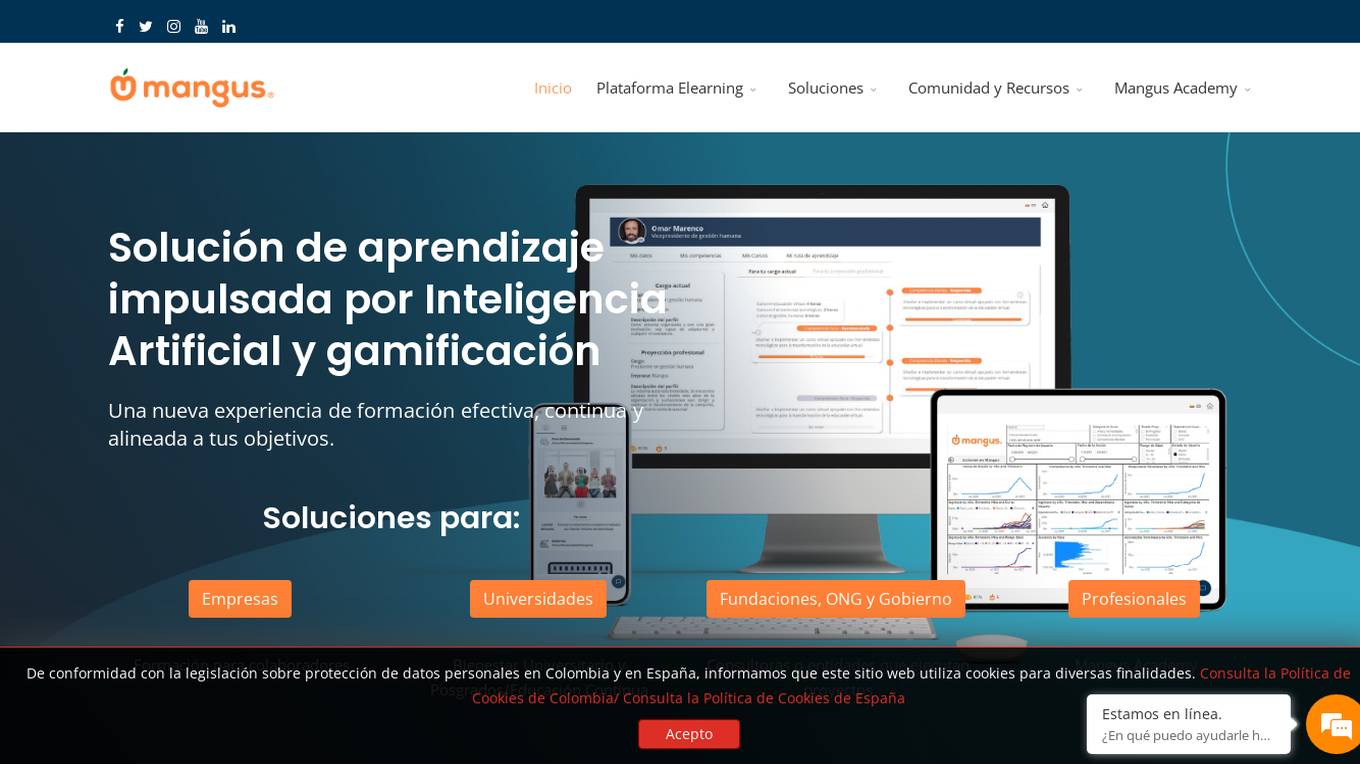
Mangus
Mangus is an AI-powered learning platform that provides personalized learning paths for employees and students. It offers a wide range of courses and programs in various disciplines, including business, education, technology, and more. Mangus uses gamification and artificial intelligence to create an engaging and effective learning experience.
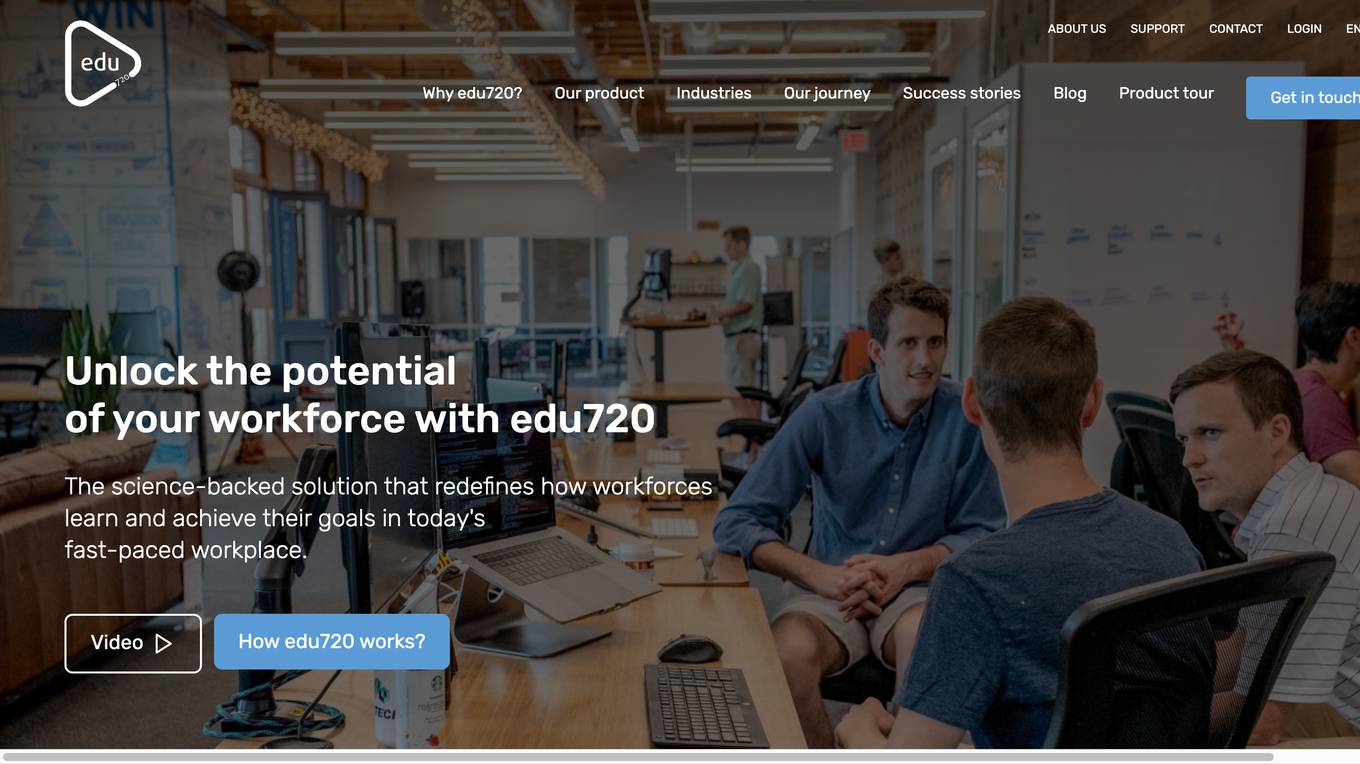
edu720
edu720 is a science-backed learning platform that uses AI and nanolearning to redefine how workforces learn and achieve their goals. It provides pre-built learning modules on various topics, including cybersecurity, privacy, and AI ethics. edu720's 360-degree approach ensures that all employees, regardless of their status or location, fully understand and absorb the knowledge conveyed.
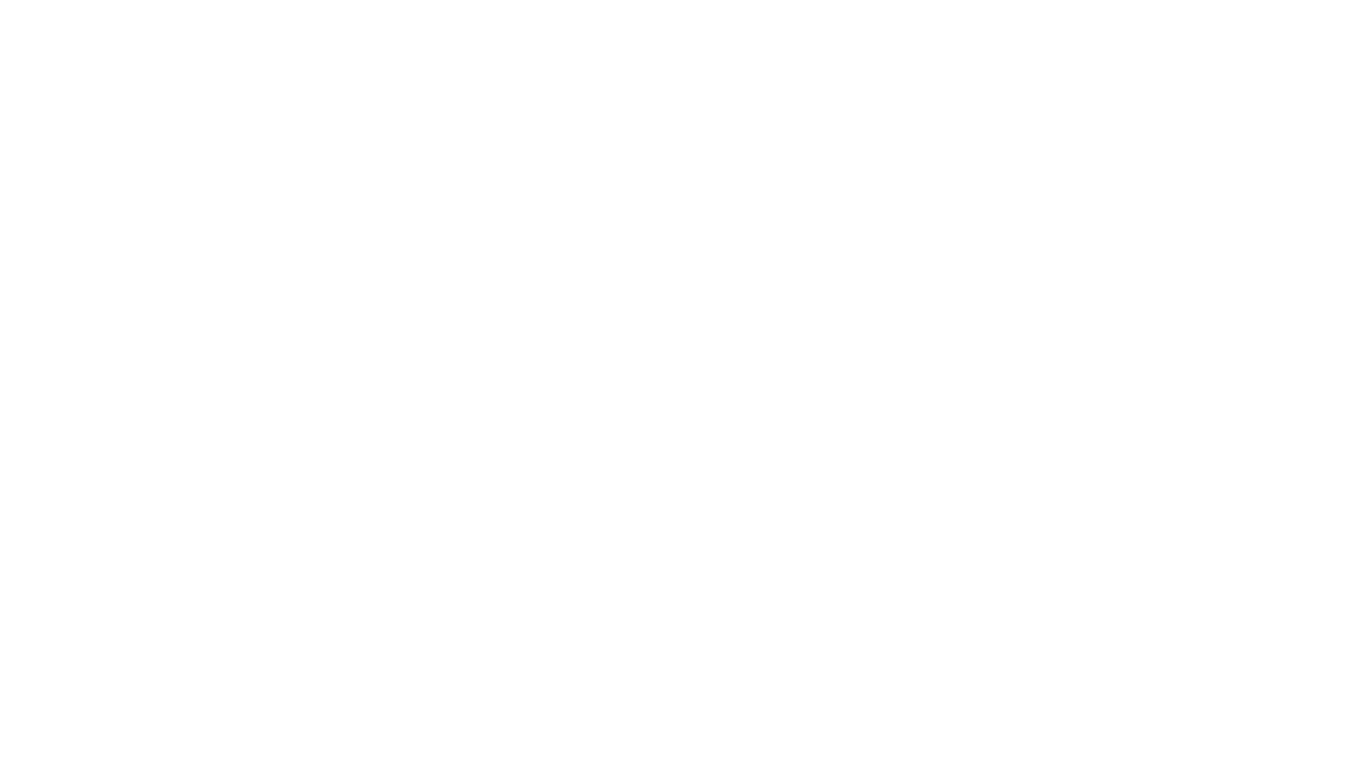
Evidently AI
Evidently AI is an open-source machine learning (ML) monitoring and observability platform that helps data scientists and ML engineers evaluate, test, and monitor ML models from validation to production. It provides a centralized hub for ML in production, including data quality monitoring, data drift monitoring, ML model performance monitoring, and NLP and LLM monitoring. Evidently AI's features include customizable reports, structured checks for data and models, and a Python library for ML monitoring. It is designed to be easy to use, with a simple setup process and a user-friendly interface. Evidently AI is used by over 2,500 data scientists and ML engineers worldwide, and it has been featured in publications such as Forbes, VentureBeat, and TechCrunch.
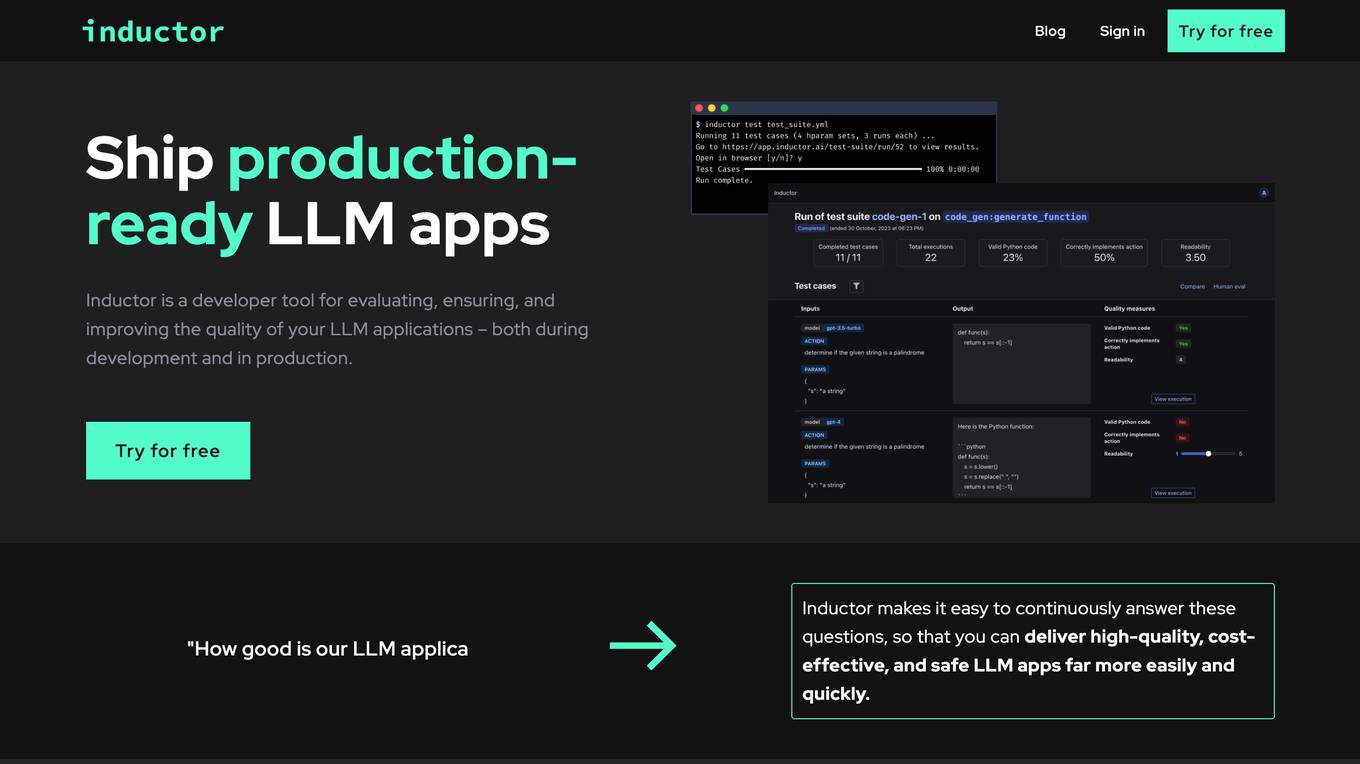
Inductor
Inductor is a developer tool for evaluating, ensuring, and improving the quality of your LLM applications – both during development and in production. It provides a fantastic workflow for continuous testing and evaluation as you develop, so that you always know your LLM app’s quality. Systematically improve quality and cost-effectiveness by actionably understanding your LLM app’s behavior and quickly testing different app variants. Rigorously assess your LLM app’s behavior before you deploy, in order to ensure quality and cost-effectiveness when you’re live. Easily monitor your live traffic: detect and resolve issues, analyze usage in order to improve, and seamlessly feed back into your development process. Inductor makes it easy for engineering and other roles to collaborate: get critical human feedback from non-engineering stakeholders (e.g., PM, UX, or subject matter experts) to ensure that your LLM app is user-ready.
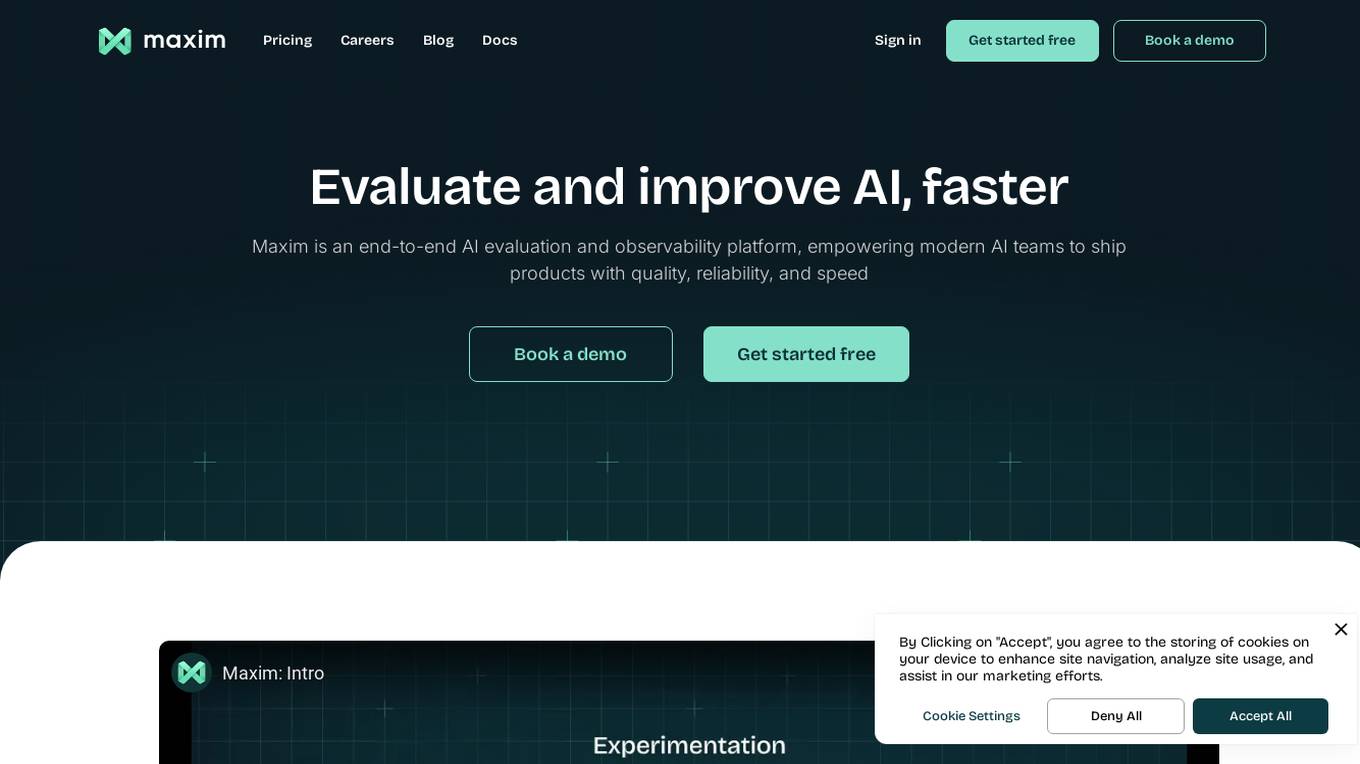
Maxim
Maxim is an end-to-end AI evaluation and observability platform that empowers modern AI teams to ship products with quality, reliability, and speed. It offers a comprehensive suite of tools for experimentation, evaluation, observability, and data management. Maxim aims to bring the best practices of traditional software development into non-deterministic AI workflows, enabling rapid iteration and deployment of AI models. The platform caters to the needs of AI developers, data scientists, and machine learning engineers by providing a unified framework for evaluation, visual flows for workflow testing, and observability features for monitoring and optimizing AI systems in real-time.
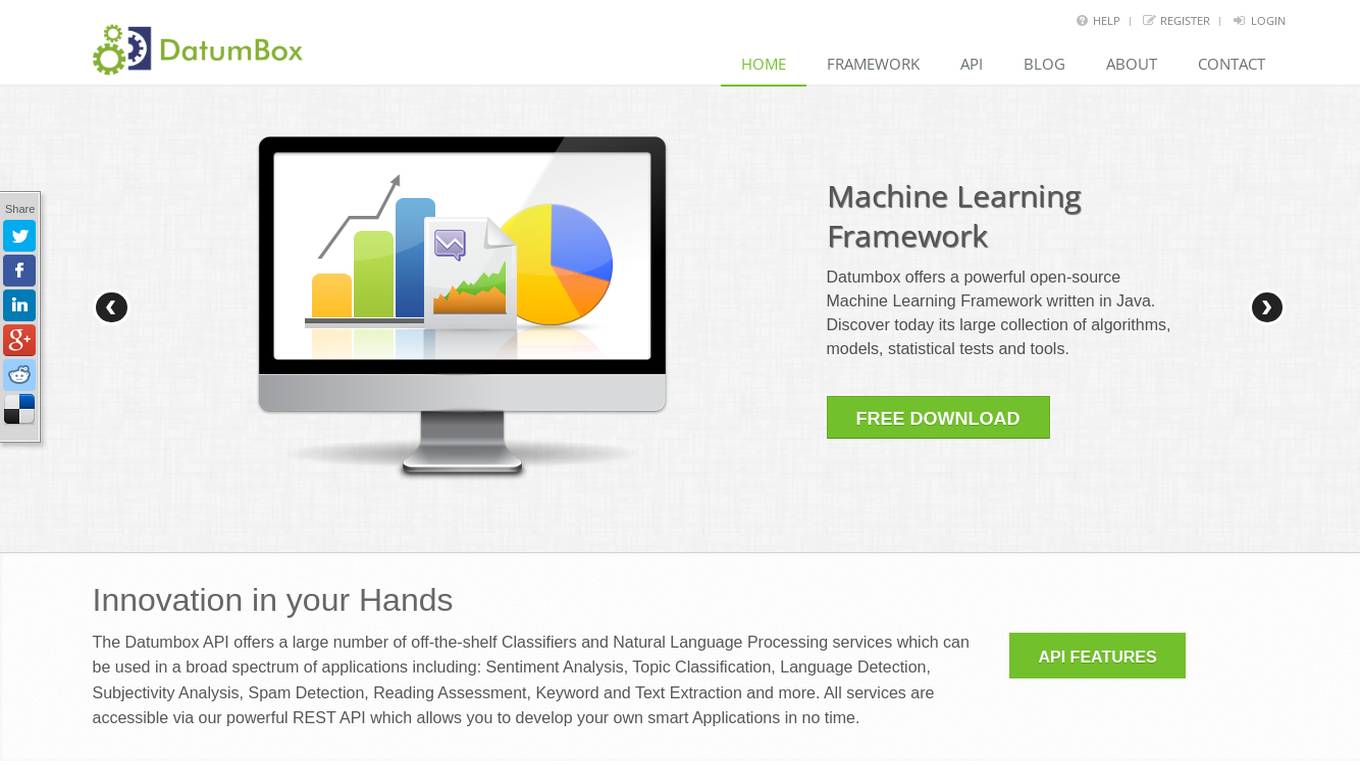
Datumbox
Datumbox is a machine learning platform that offers a powerful open-source Machine Learning Framework written in Java. It provides a large collection of algorithms, models, statistical tests, and tools to power up intelligent applications. The platform enables developers to build smart software and services quickly using its REST Machine Learning API. Datumbox API offers off-the-shelf Classifiers and Natural Language Processing services for applications like Sentiment Analysis, Topic Classification, Language Detection, and more. It simplifies the process of designing and training Machine Learning models, making it easy for developers to create innovative applications.
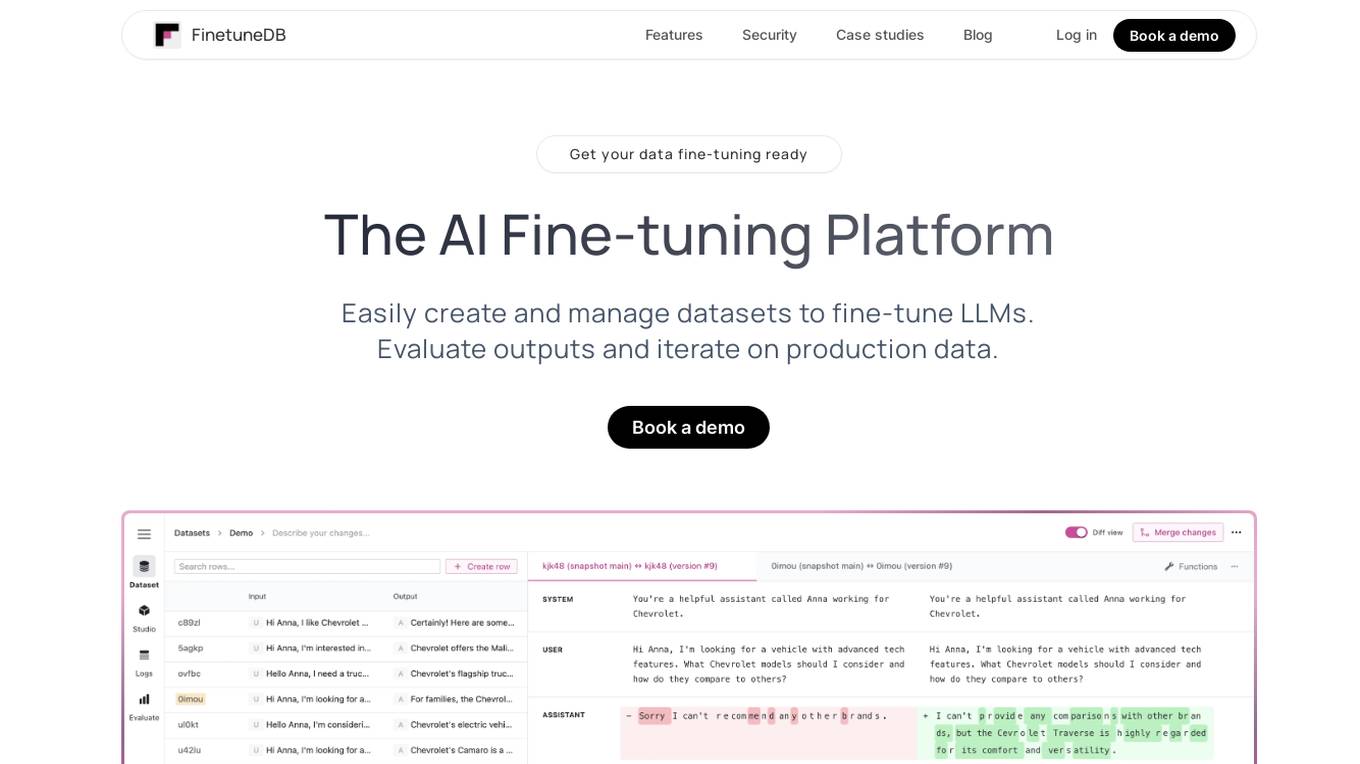
FinetuneDB
FinetuneDB is an AI fine-tuning platform that allows users to easily create and manage datasets to fine-tune LLMs, evaluate outputs, and iterate on production data. It integrates with open-source and proprietary foundation models, and provides a collaborative editor for building datasets. FinetuneDB also offers a variety of features for evaluating model performance, including human and AI feedback, automated evaluations, and model metrics tracking.
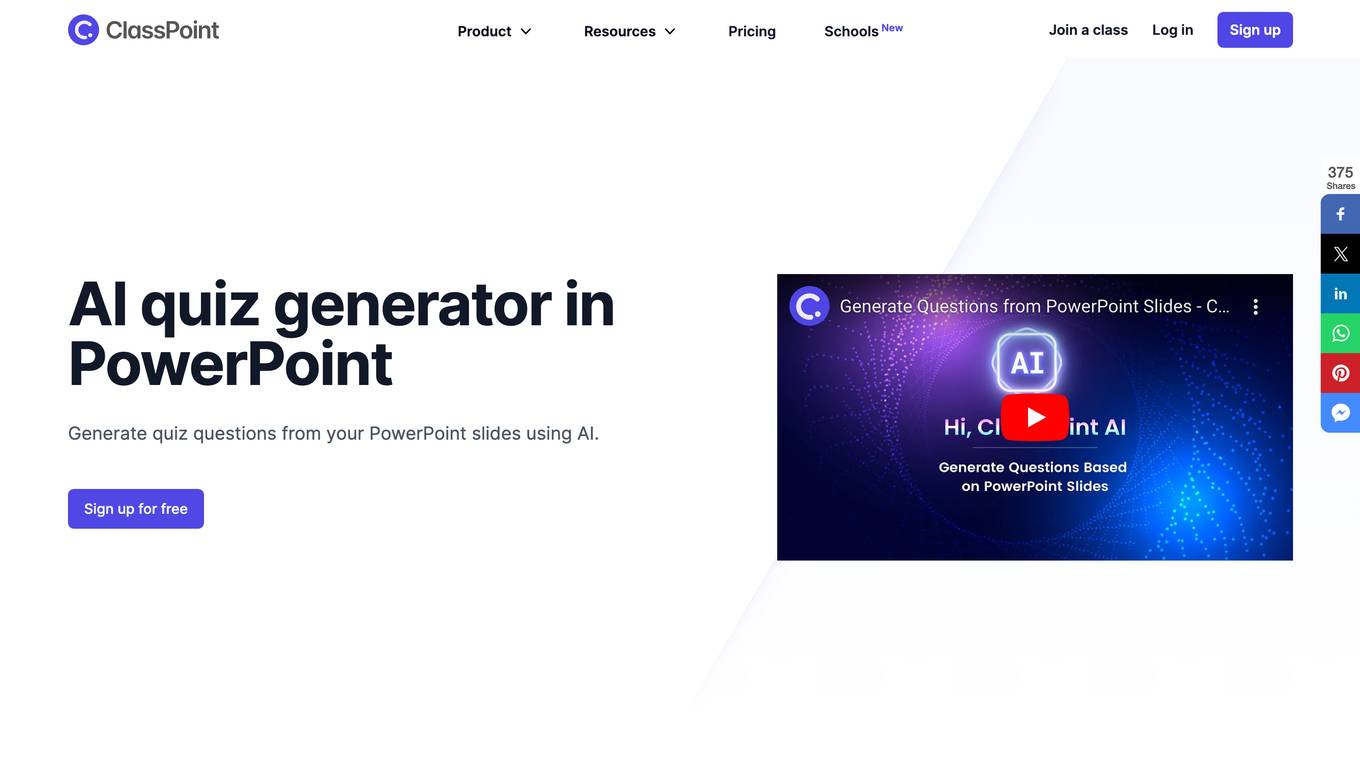
ClassPoint
ClassPoint is an AI quiz generator tool integrated with PowerPoint that allows users to effortlessly create engaging quiz questions from their slides using AI technology. The tool supports multiple quiz types, Bloom's taxonomy, and offers multi-language support powered by OpenAI. With ClassPoint, educators can enhance student engagement, evaluate understanding, and collect live responses during presentations. The AI feature analyzes PowerPoint slides, generates customized quizzes, and provides interactive quiz functionalities, making teaching and learning more interactive and efficient.
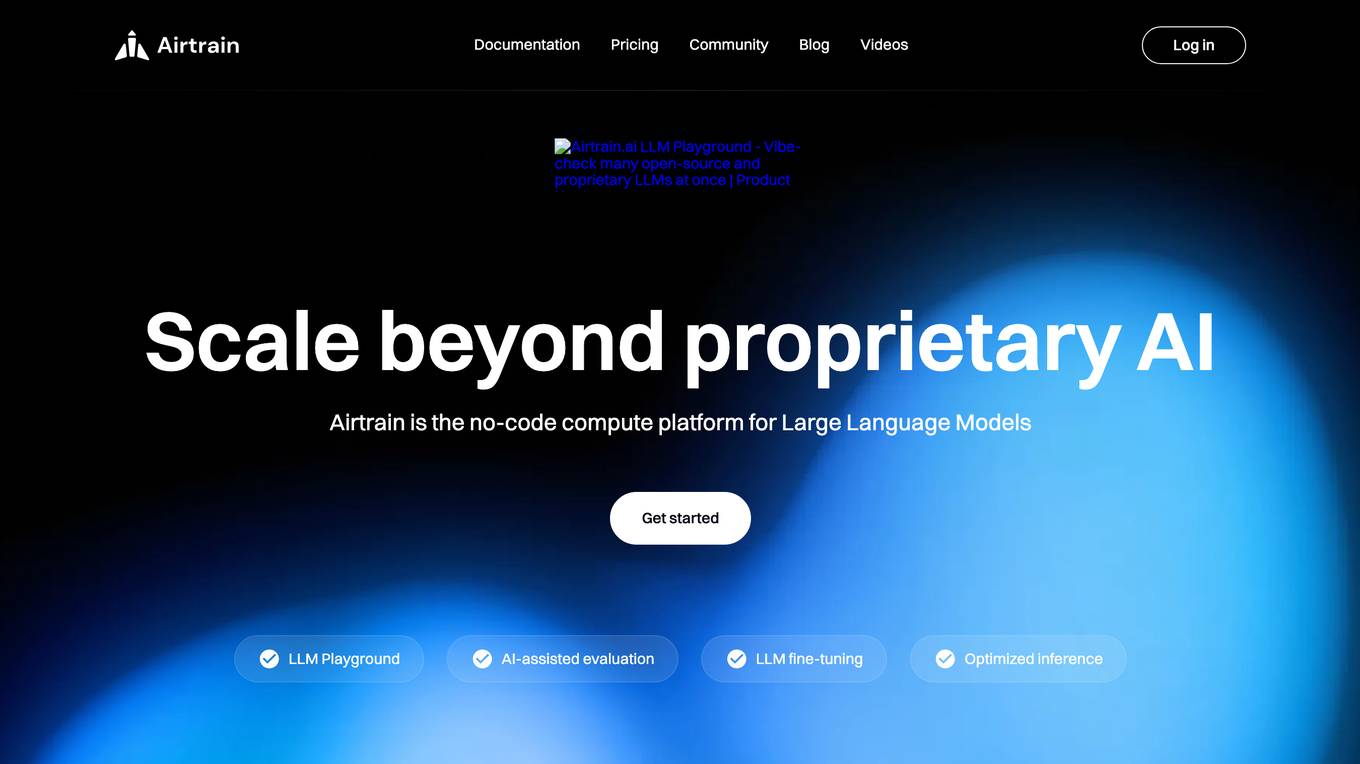
Airtrain
Airtrain is a no-code compute platform for Large Language Models (LLMs). It provides a user-friendly interface for fine-tuning, evaluating, and deploying custom AI models. Airtrain also offers a marketplace of pre-trained models that can be used for a variety of tasks, such as text generation, translation, and question answering.
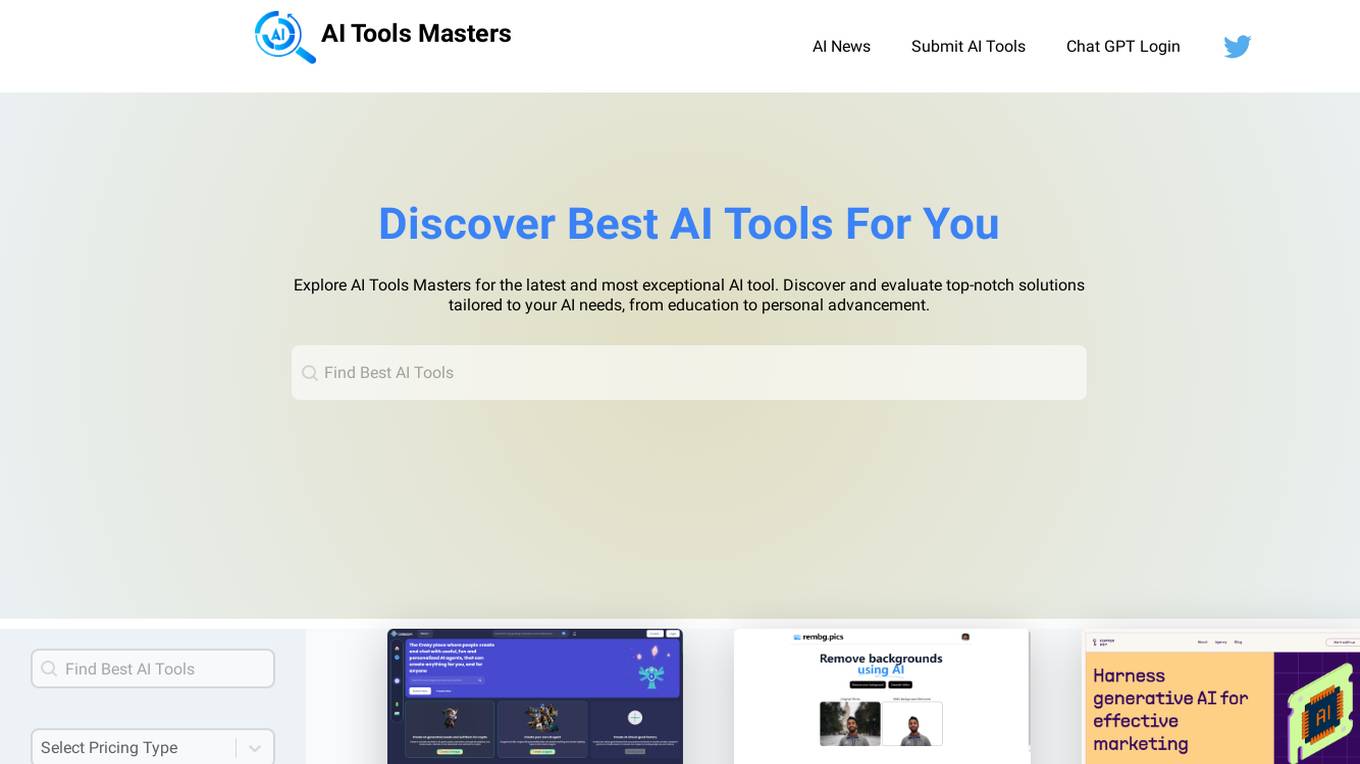
AI Tools Masters
AI Tools Masters is a comprehensive platform that empowers users to discover and evaluate the latest and most exceptional AI tools. Catering to diverse needs, from education to personal advancement, AI Tools Masters offers a curated collection of top-notch solutions tailored to specific requirements. With a user-friendly interface and extensive filtering options, users can effortlessly navigate through a wide range of AI tools, ensuring they find the perfect fit for their projects and goals.
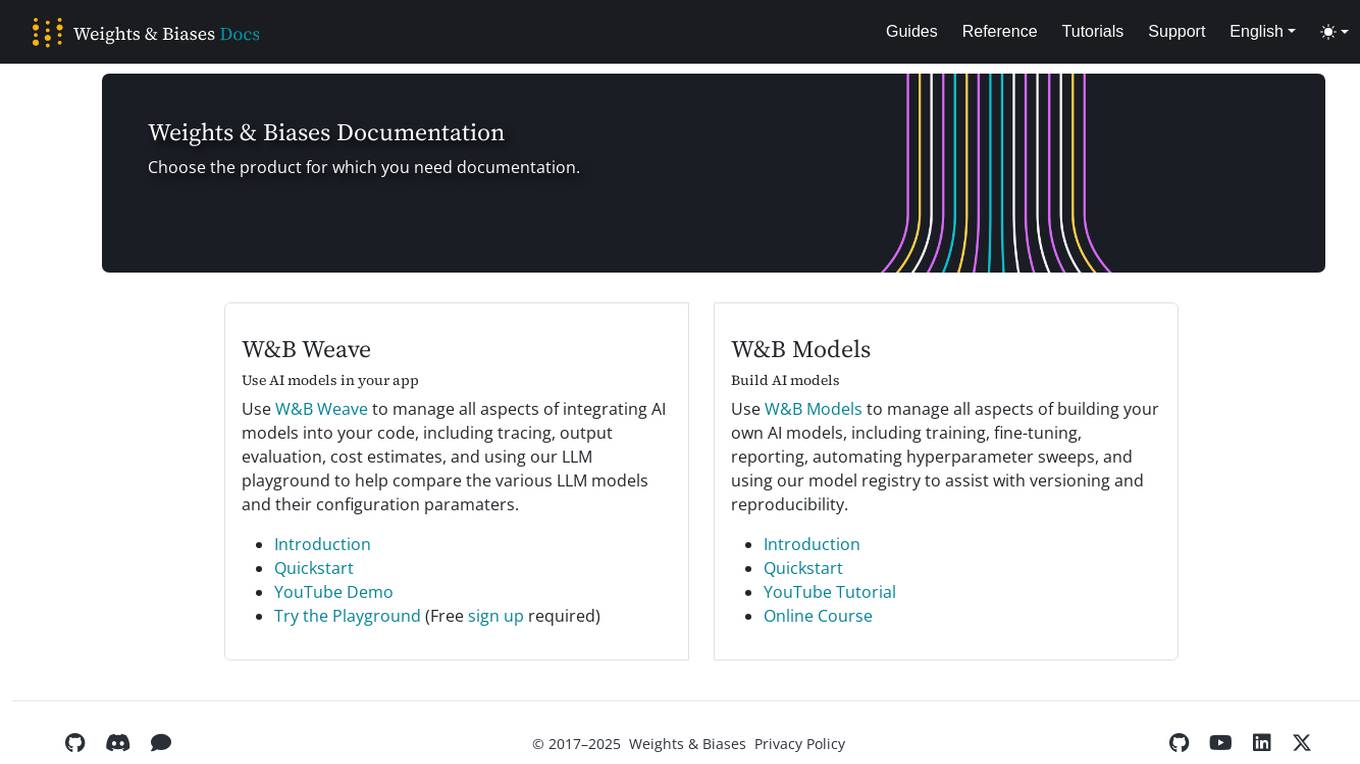
Weights & Biases
Weights & Biases is an AI tool that offers documentation, guides, tutorials, and support for using AI models in applications. The platform provides two main products: W&B Weave for integrating AI models into code and W&B Models for building custom AI models. Users can access features such as tracing, output evaluation, cost estimates, hyperparameter sweeps, model registry, and more. Weights & Biases aims to simplify the process of working with AI models and improving model reproducibility.
20 - Open Source Tools
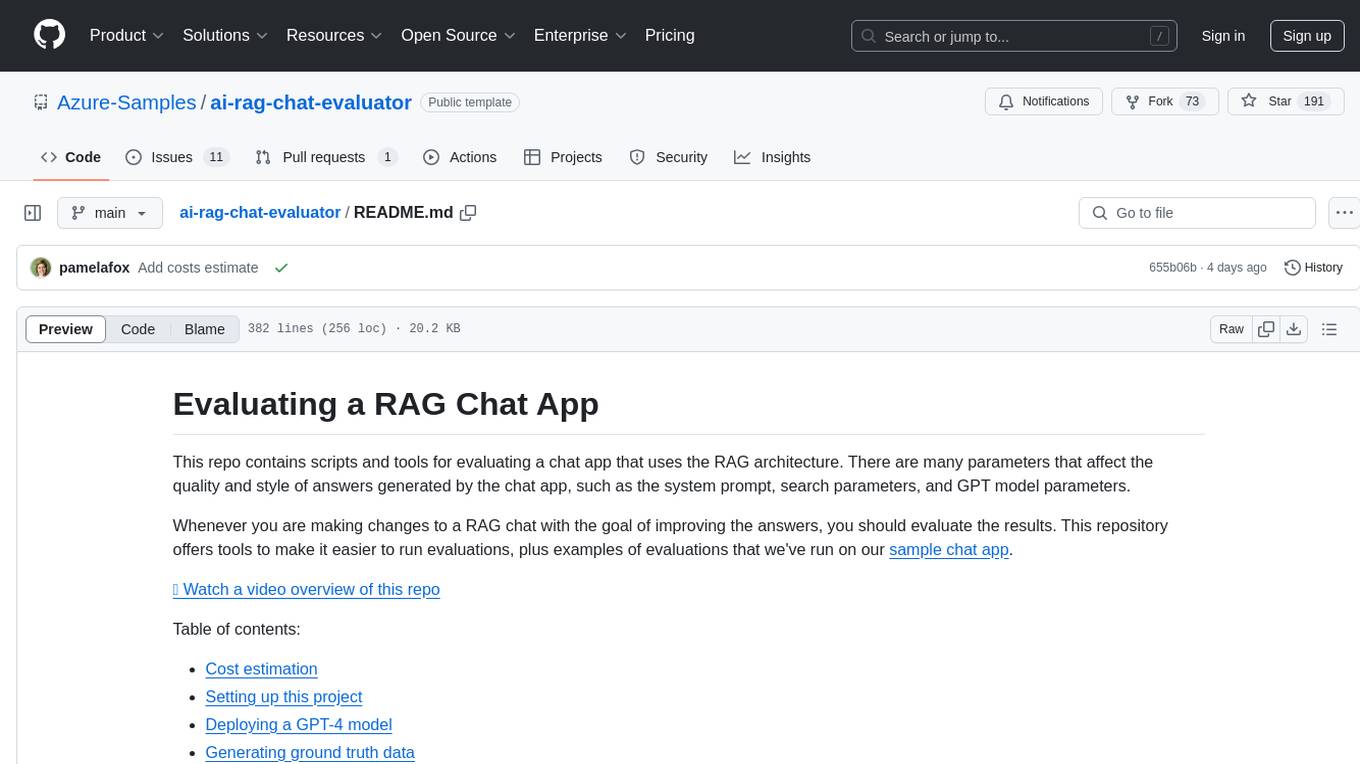
ai-rag-chat-evaluator
This repository contains scripts and tools for evaluating a chat app that uses the RAG architecture. It provides parameters to assess the quality and style of answers generated by the chat app, including system prompt, search parameters, and GPT model parameters. The tools facilitate running evaluations, with examples of evaluations on a sample chat app. The repo also offers guidance on cost estimation, setting up the project, deploying a GPT-4 model, generating ground truth data, running evaluations, and measuring the app's ability to say 'I don't know'. Users can customize evaluations, view results, and compare runs using provided tools.
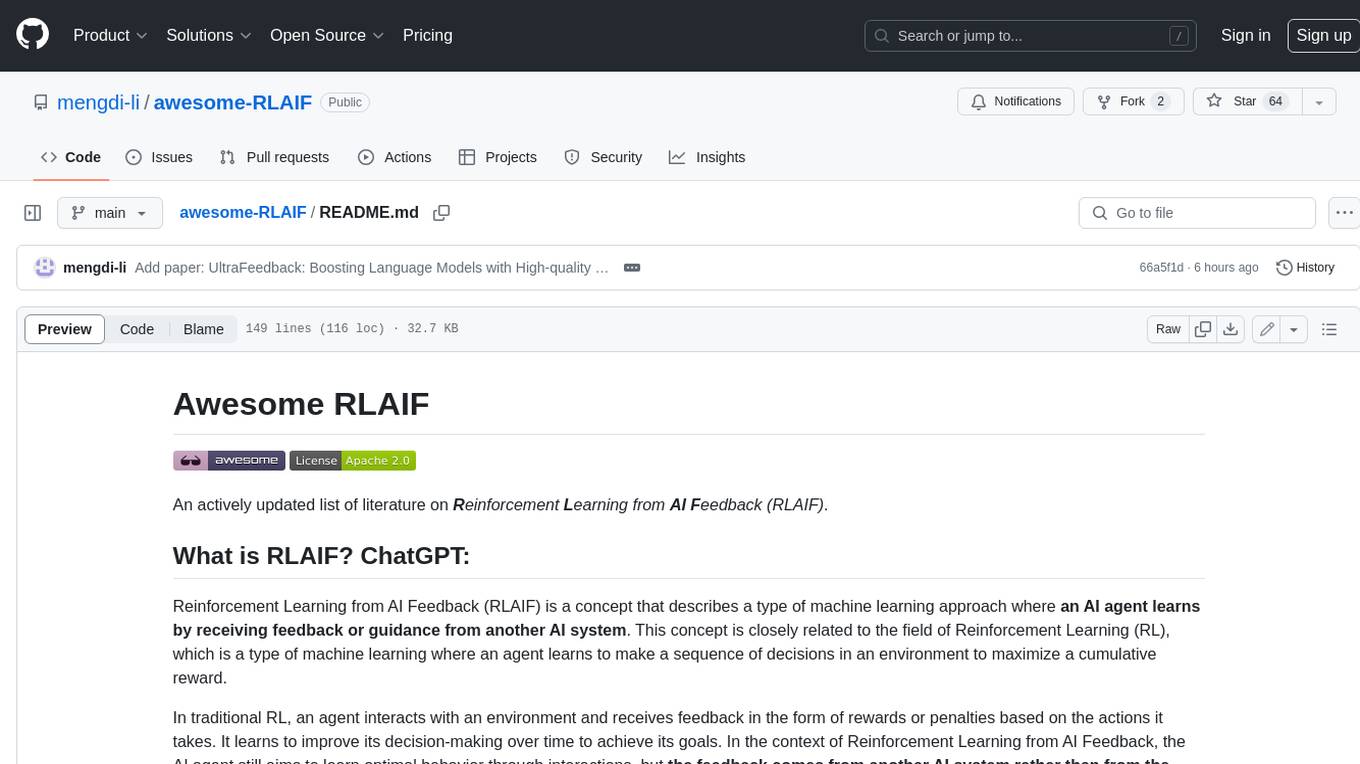
awesome-RLAIF
Reinforcement Learning from AI Feedback (RLAIF) is a concept that describes a type of machine learning approach where **an AI agent learns by receiving feedback or guidance from another AI system**. This concept is closely related to the field of Reinforcement Learning (RL), which is a type of machine learning where an agent learns to make a sequence of decisions in an environment to maximize a cumulative reward. In traditional RL, an agent interacts with an environment and receives feedback in the form of rewards or penalties based on the actions it takes. It learns to improve its decision-making over time to achieve its goals. In the context of Reinforcement Learning from AI Feedback, the AI agent still aims to learn optimal behavior through interactions, but **the feedback comes from another AI system rather than from the environment or human evaluators**. This can be **particularly useful in situations where it may be challenging to define clear reward functions or when it is more efficient to use another AI system to provide guidance**. The feedback from the AI system can take various forms, such as: - **Demonstrations** : The AI system provides demonstrations of desired behavior, and the learning agent tries to imitate these demonstrations. - **Comparison Data** : The AI system ranks or compares different actions taken by the learning agent, helping it to understand which actions are better or worse. - **Reward Shaping** : The AI system provides additional reward signals to guide the learning agent's behavior, supplementing the rewards from the environment. This approach is often used in scenarios where the RL agent needs to learn from **limited human or expert feedback or when the reward signal from the environment is sparse or unclear**. It can also be used to **accelerate the learning process and make RL more sample-efficient**. Reinforcement Learning from AI Feedback is an area of ongoing research and has applications in various domains, including robotics, autonomous vehicles, and game playing, among others.
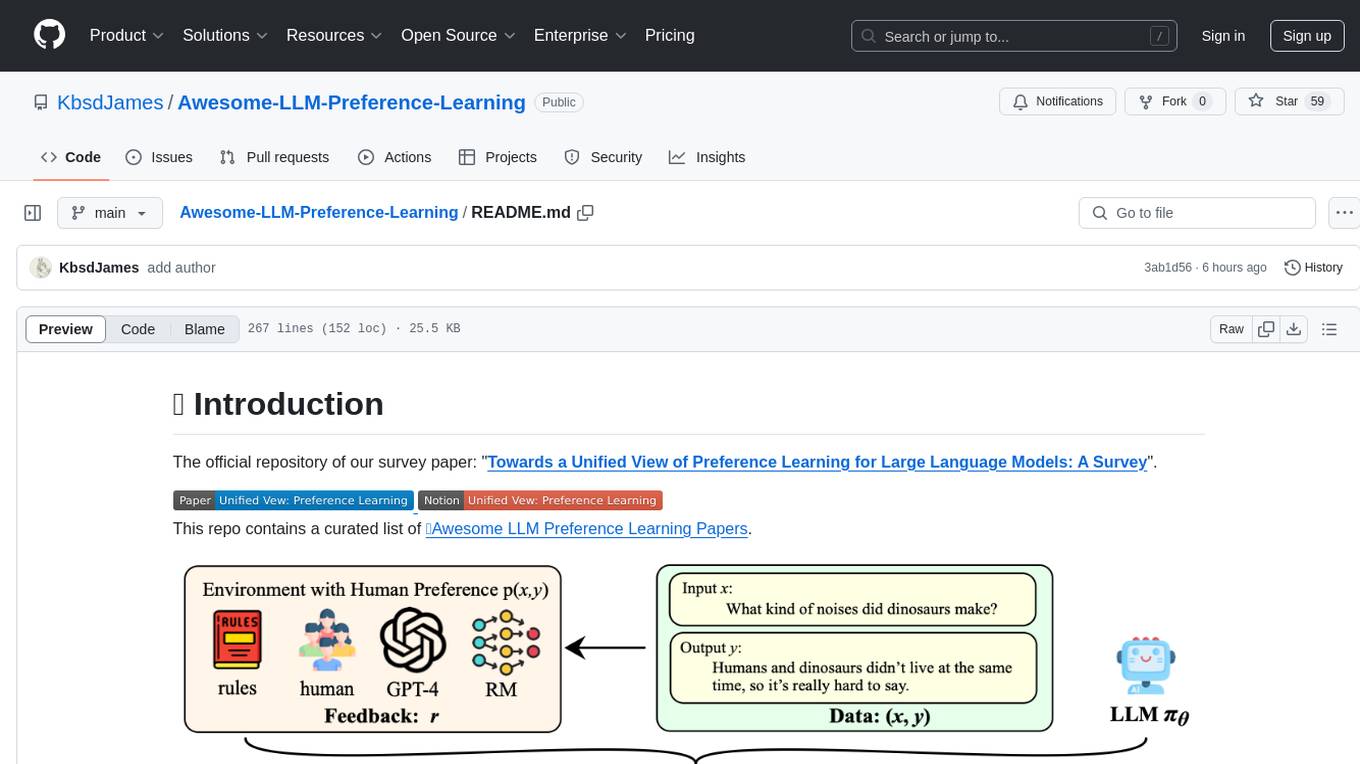
Awesome-LLM-Preference-Learning
The repository 'Awesome-LLM-Preference-Learning' is the official repository of a survey paper titled 'Towards a Unified View of Preference Learning for Large Language Models: A Survey'. It contains a curated list of papers related to preference learning for Large Language Models (LLMs). The repository covers various aspects of preference learning, including on-policy and off-policy methods, feedback mechanisms, reward models, algorithms, evaluation techniques, and more. The papers included in the repository explore different approaches to aligning LLMs with human preferences, improving mathematical reasoning in LLMs, enhancing code generation, and optimizing language model performance.
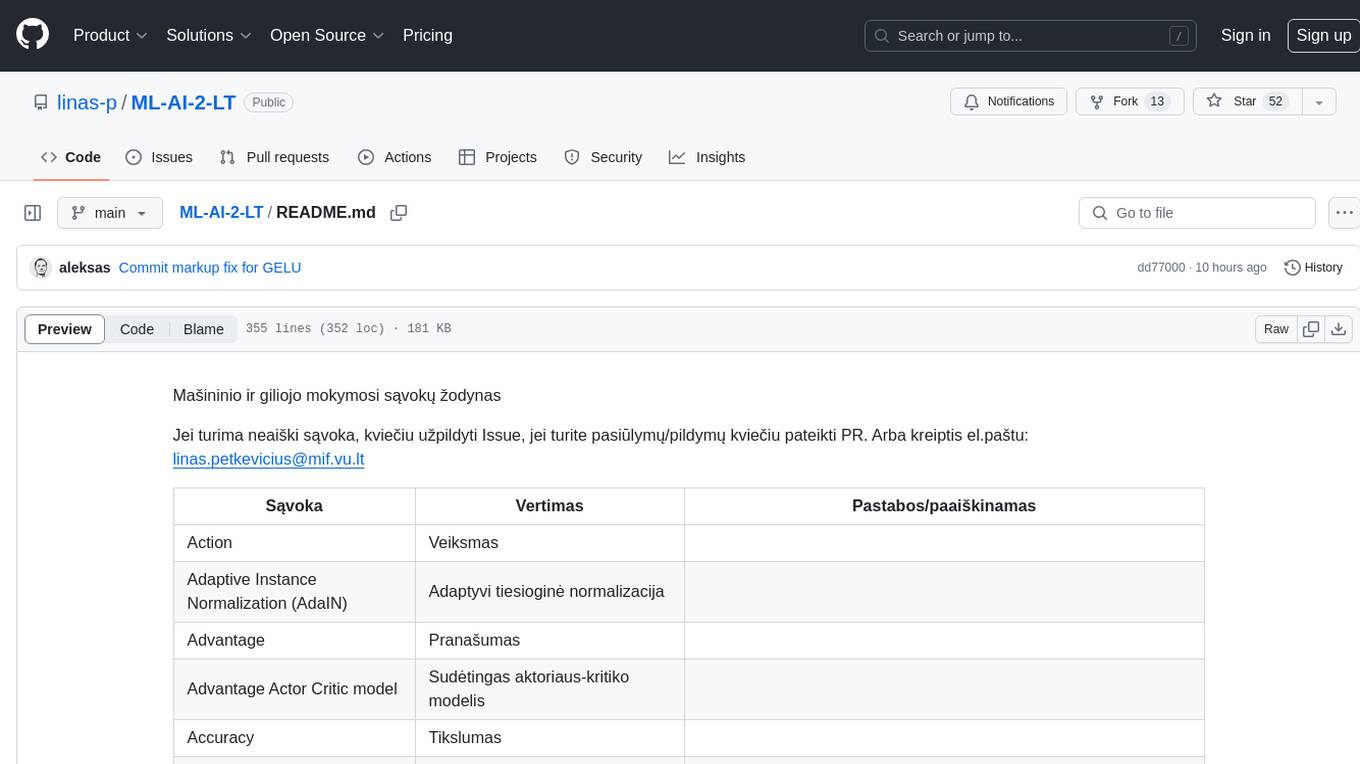
ML-AI-2-LT
ML-AI-2-LT is a repository that serves as a glossary for machine learning and deep learning concepts. It contains translations and explanations of various terms related to artificial intelligence, including definitions and notes. Users can contribute by filling issues for unclear concepts or by submitting pull requests with suggestions or additions. The repository aims to provide a comprehensive resource for understanding key terminology in the field of AI and machine learning.
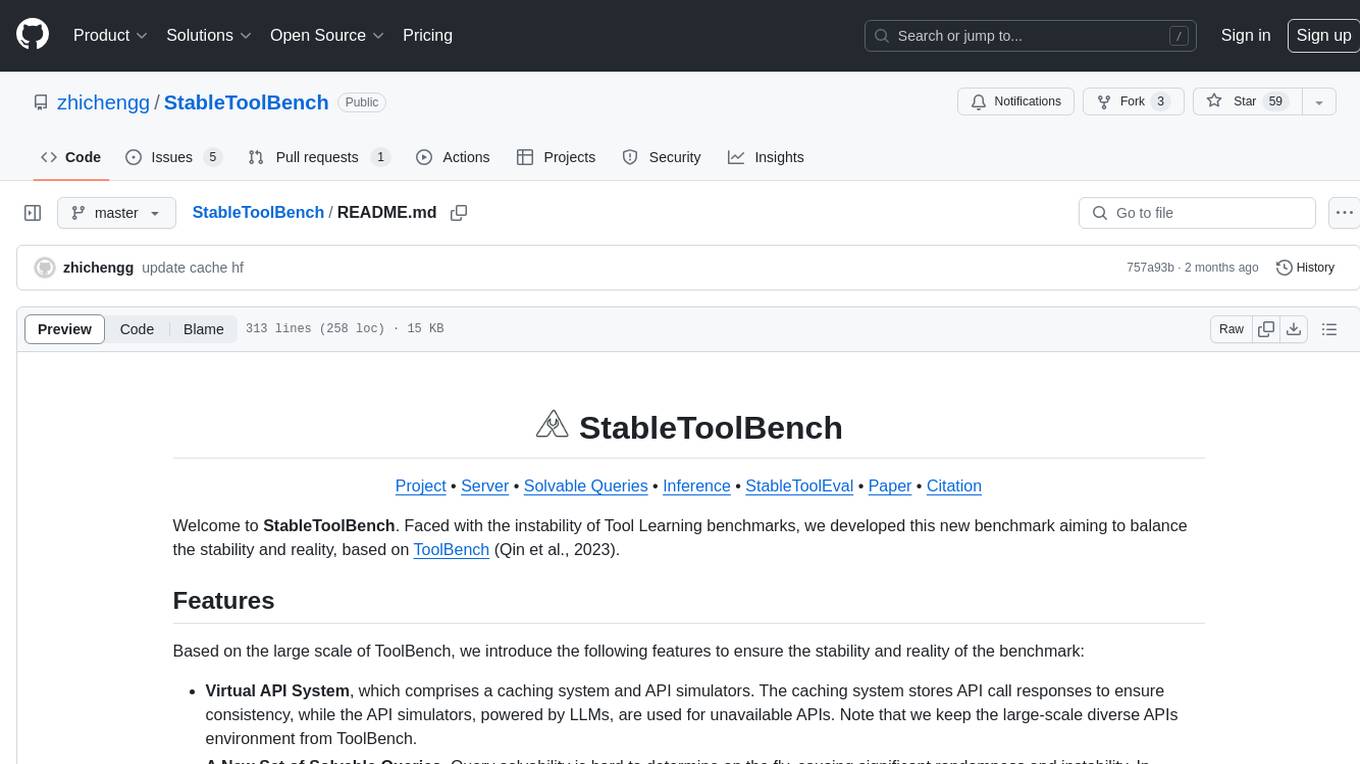
StableToolBench
StableToolBench is a new benchmark developed to address the instability of Tool Learning benchmarks. It aims to balance stability and reality by introducing features such as a Virtual API System with caching and API simulators, a new set of solvable queries determined by LLMs, and a Stable Evaluation System using GPT-4. The Virtual API Server can be set up either by building from source or using a prebuilt Docker image. Users can test the server using provided scripts and evaluate models with Solvable Pass Rate and Solvable Win Rate metrics. The tool also includes model experiments results comparing different models' performance.
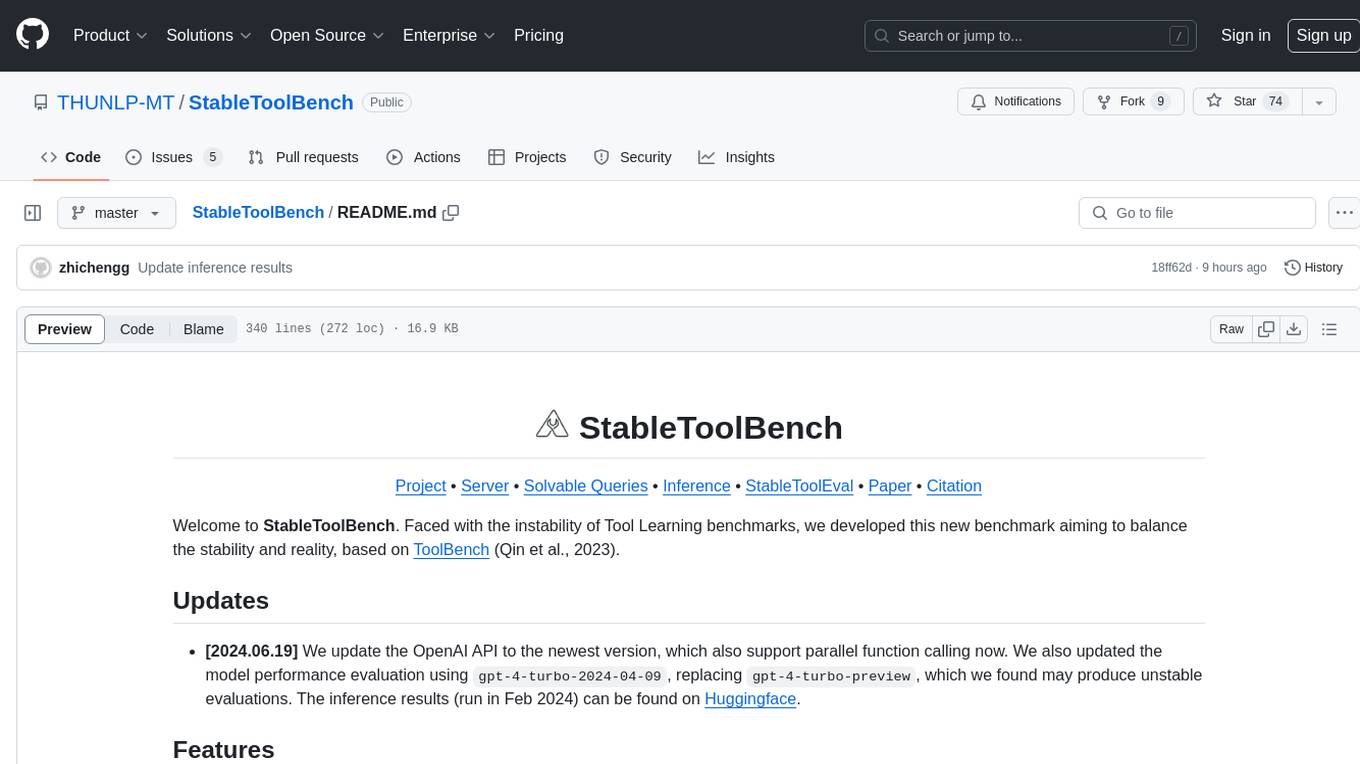
StableToolBench
StableToolBench is a new benchmark developed to address the instability of Tool Learning benchmarks. It aims to balance stability and reality by introducing features like Virtual API System, Solvable Queries, and Stable Evaluation System. The benchmark ensures consistency through a caching system and API simulators, filters queries based on solvability using LLMs, and evaluates model performance using GPT-4 with metrics like Solvable Pass Rate and Solvable Win Rate.
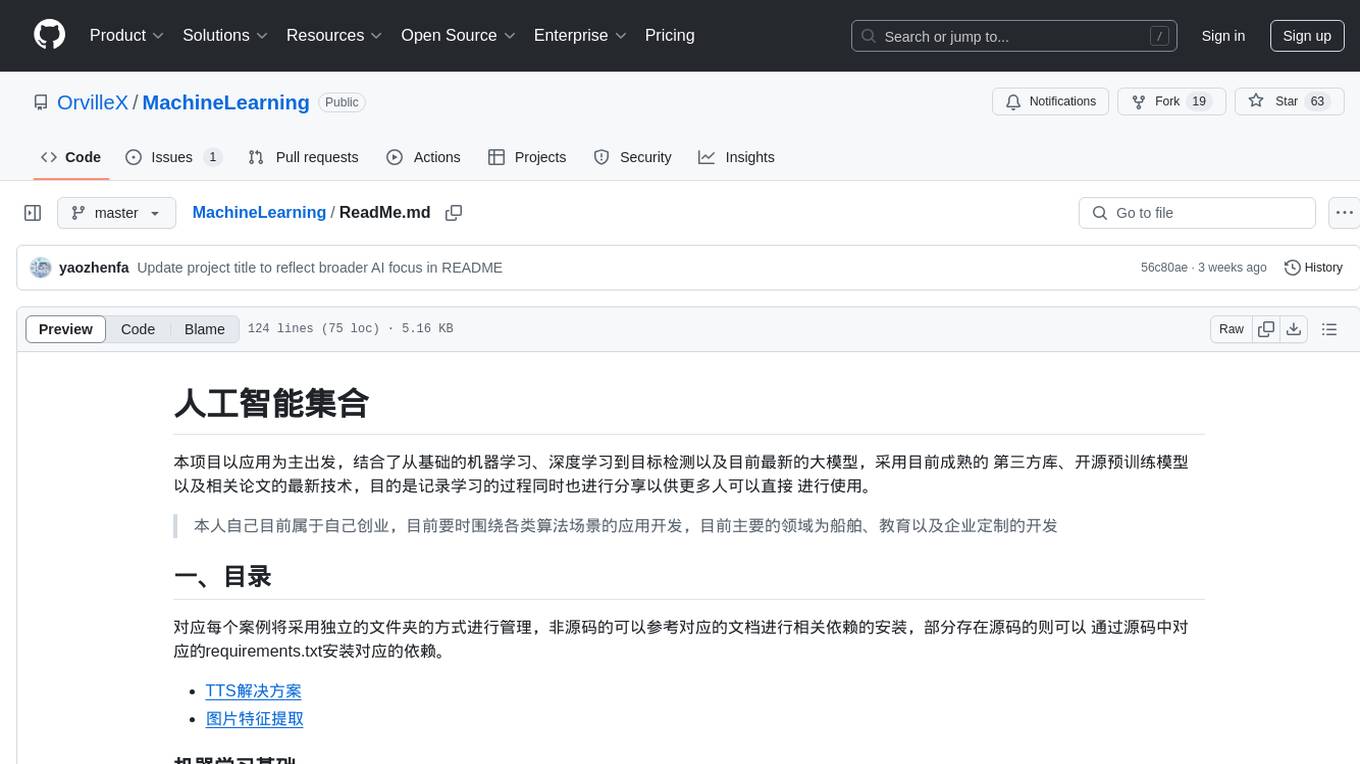
MachineLearning
MachineLearning is a repository focused on practical applications in various algorithm scenarios such as ship, education, and enterprise development. It covers a wide range of topics from basic machine learning and deep learning to object detection and the latest large models. The project utilizes mature third-party libraries, open-source pre-trained models, and the latest technologies from related papers to document the learning process and facilitate direct usage by a wider audience.
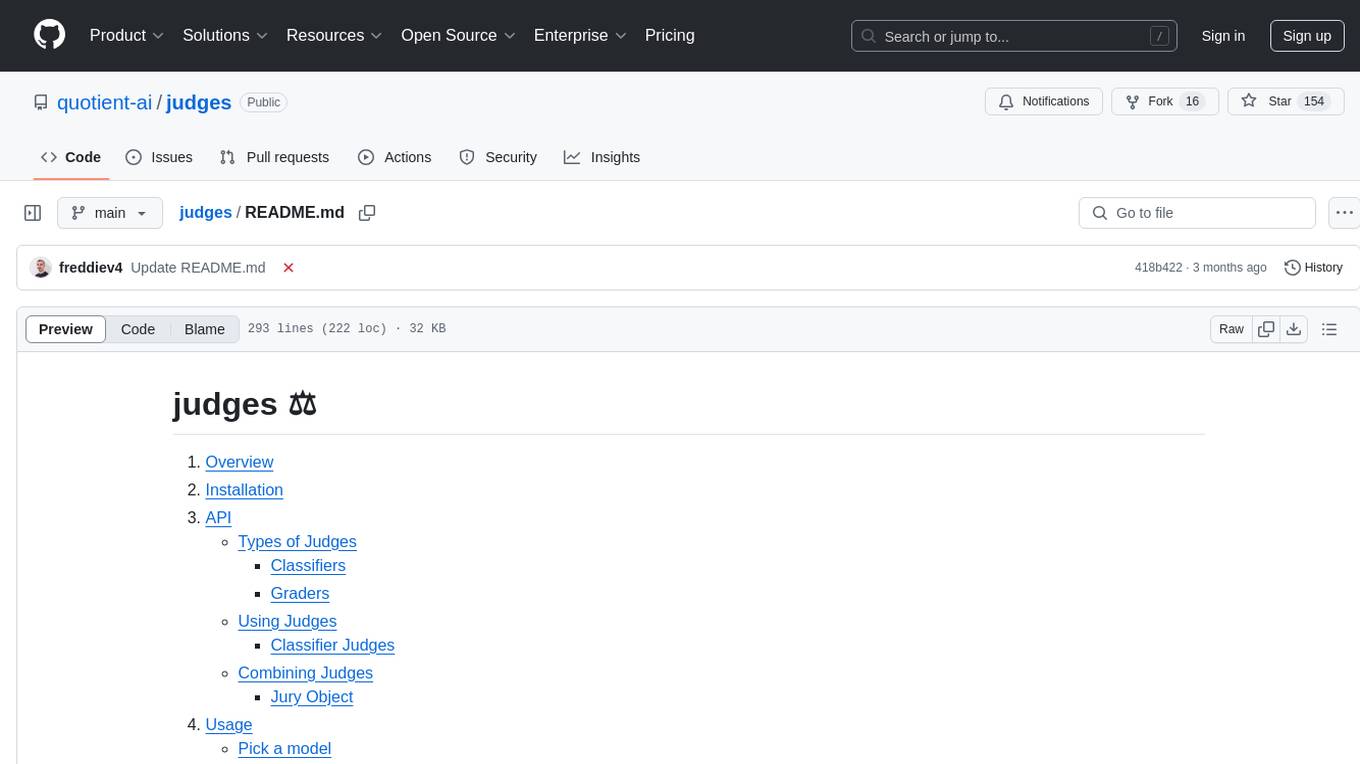
judges
The 'judges' repository is a small library designed for using and creating LLM-as-a-Judge evaluators. It offers a curated set of LLM evaluators in a low-friction format for various use cases, backed by research. Users can use these evaluators off-the-shelf or as inspiration for building custom LLM evaluators. The library provides two types of judges: Classifiers that return boolean values and Graders that return scores on a numerical or Likert scale. Users can combine multiple judges using the 'Jury' object and evaluate input-output pairs with the '.judge()' method. Additionally, the repository includes detailed instructions on picking a model, sending data to an LLM, using classifiers, combining judges, and creating custom LLM judges with 'AutoJudge'.
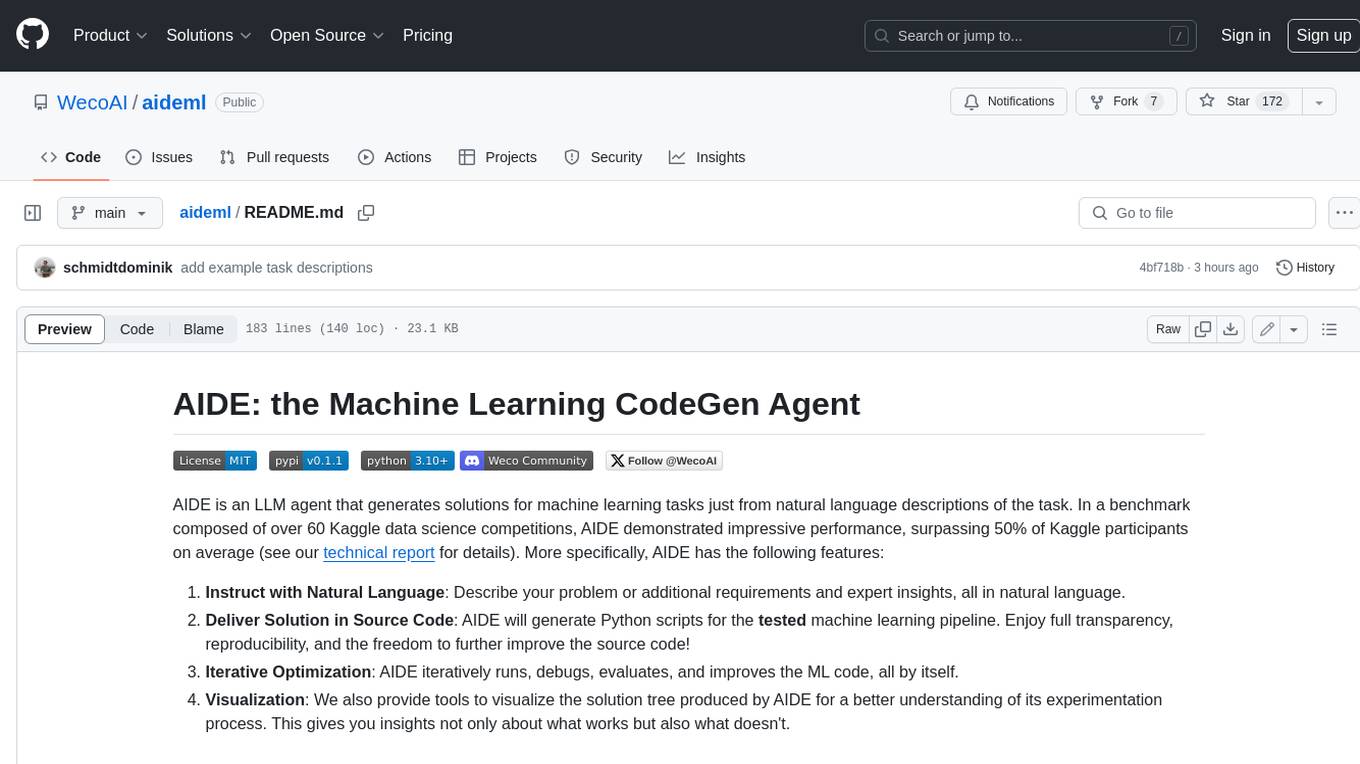
aideml
AIDE is a machine learning code generation agent that can generate solutions for machine learning tasks from natural language descriptions. It has the following features: 1. **Instruct with Natural Language**: Describe your problem or additional requirements and expert insights, all in natural language. 2. **Deliver Solution in Source Code**: AIDE will generate Python scripts for the **tested** machine learning pipeline. Enjoy full transparency, reproducibility, and the freedom to further improve the source code! 3. **Iterative Optimization**: AIDE iteratively runs, debugs, evaluates, and improves the ML code, all by itself. 4. **Visualization**: We also provide tools to visualize the solution tree produced by AIDE for a better understanding of its experimentation process. This gives you insights not only about what works but also what doesn't. AIDE has been benchmarked on over 60 Kaggle data science competitions and has demonstrated impressive performance, surpassing 50% of Kaggle participants on average. It is particularly well-suited for tasks that require complex data preprocessing, feature engineering, and model selection.
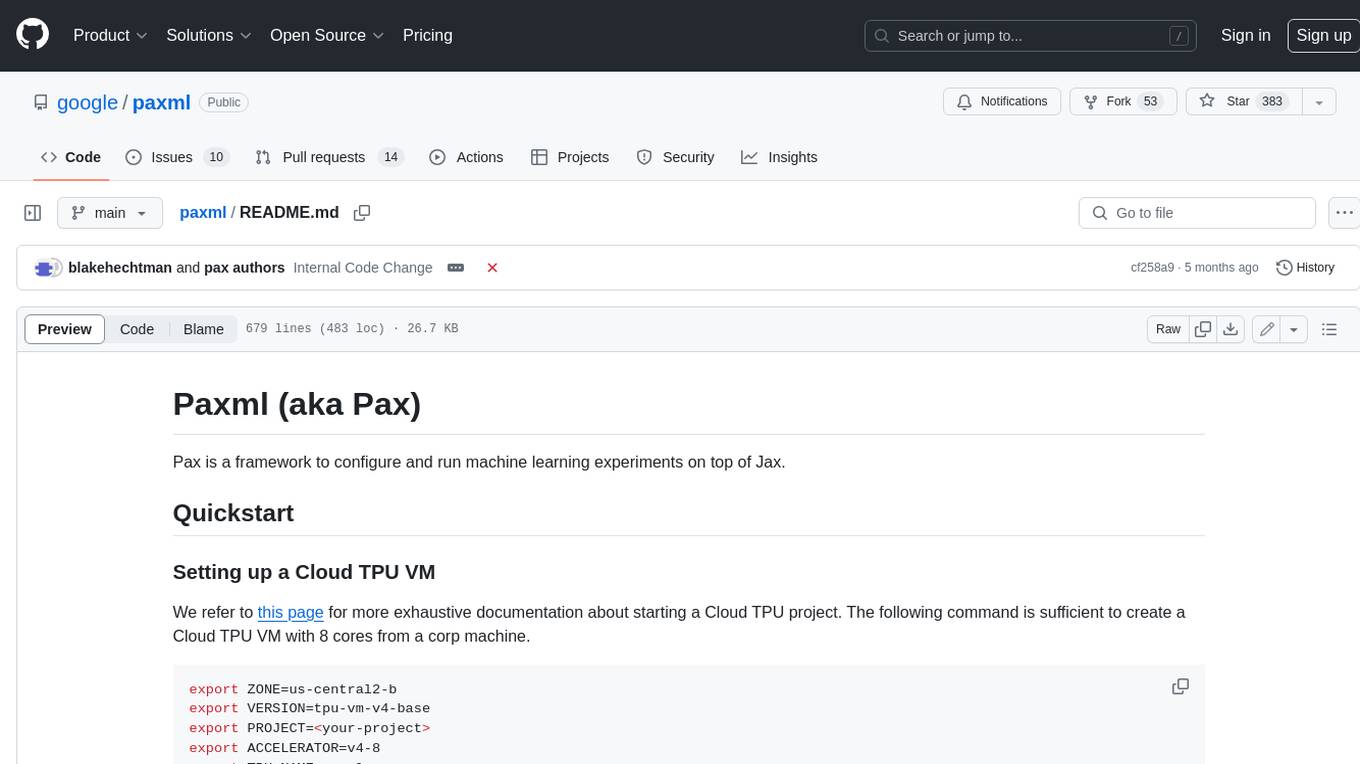
paxml
Pax is a framework to configure and run machine learning experiments on top of Jax.
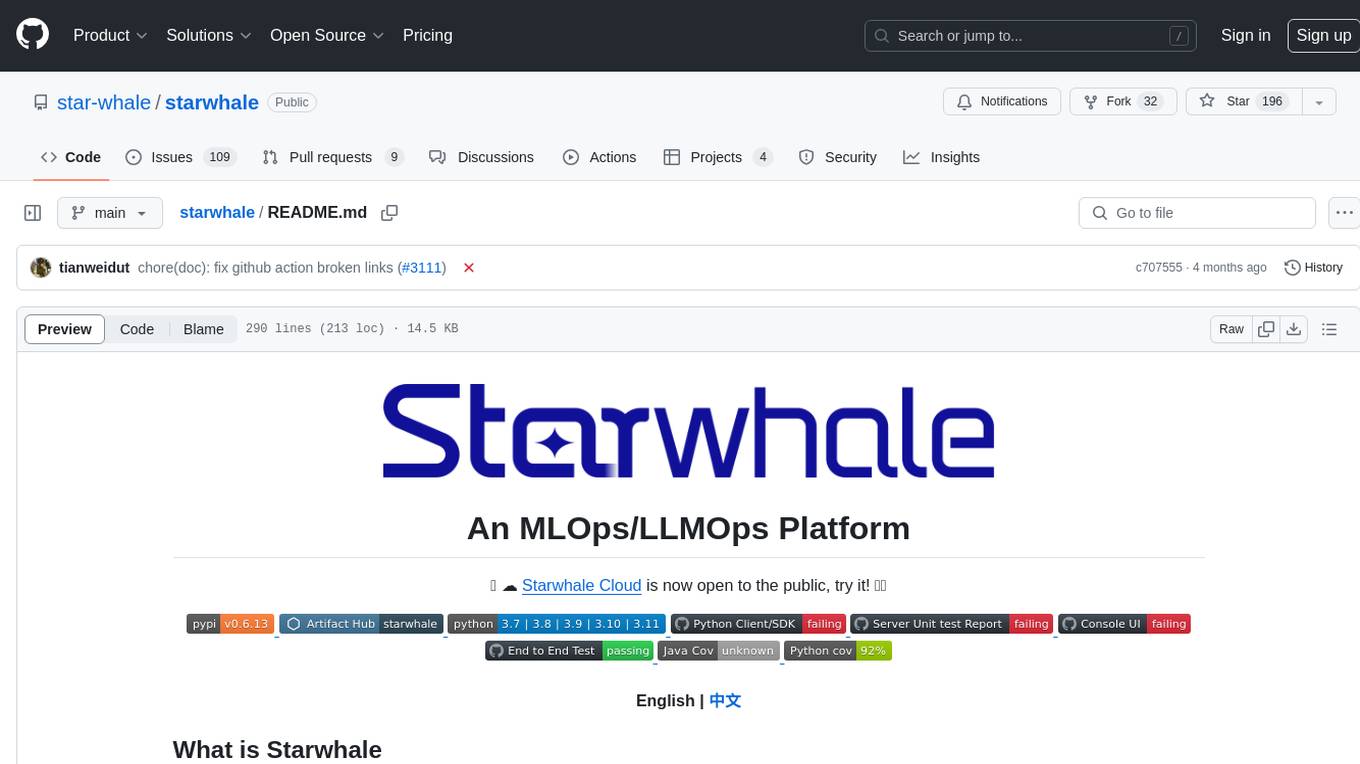
starwhale
Starwhale is an MLOps/LLMOps platform that brings efficiency and standardization to machine learning operations. It streamlines the model development lifecycle, enabling teams to optimize workflows around key areas like model building, evaluation, release, and fine-tuning. Starwhale abstracts Model, Runtime, and Dataset as first-class citizens, providing tailored capabilities for common workflow scenarios including Models Evaluation, Live Demo, and LLM Fine-tuning. It is an open-source platform designed for clarity and ease of use, empowering developers to build customized MLOps features tailored to their needs.
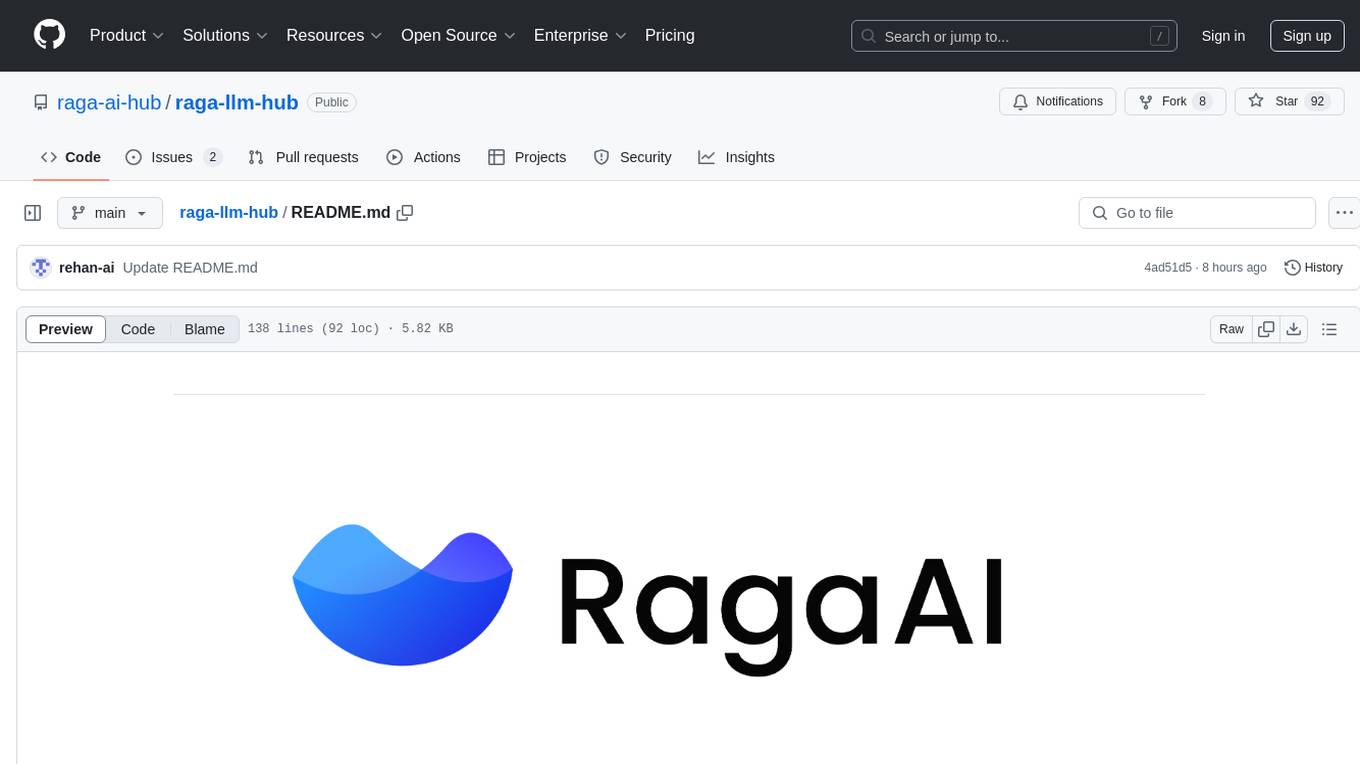
raga-llm-hub
Raga LLM Hub is a comprehensive evaluation toolkit for Language and Learning Models (LLMs) with over 100 meticulously designed metrics. It allows developers and organizations to evaluate and compare LLMs effectively, establishing guardrails for LLMs and Retrieval Augmented Generation (RAG) applications. The platform assesses aspects like Relevance & Understanding, Content Quality, Hallucination, Safety & Bias, Context Relevance, Guardrails, and Vulnerability scanning, along with Metric-Based Tests for quantitative analysis. It helps teams identify and fix issues throughout the LLM lifecycle, revolutionizing reliability and trustworthiness.
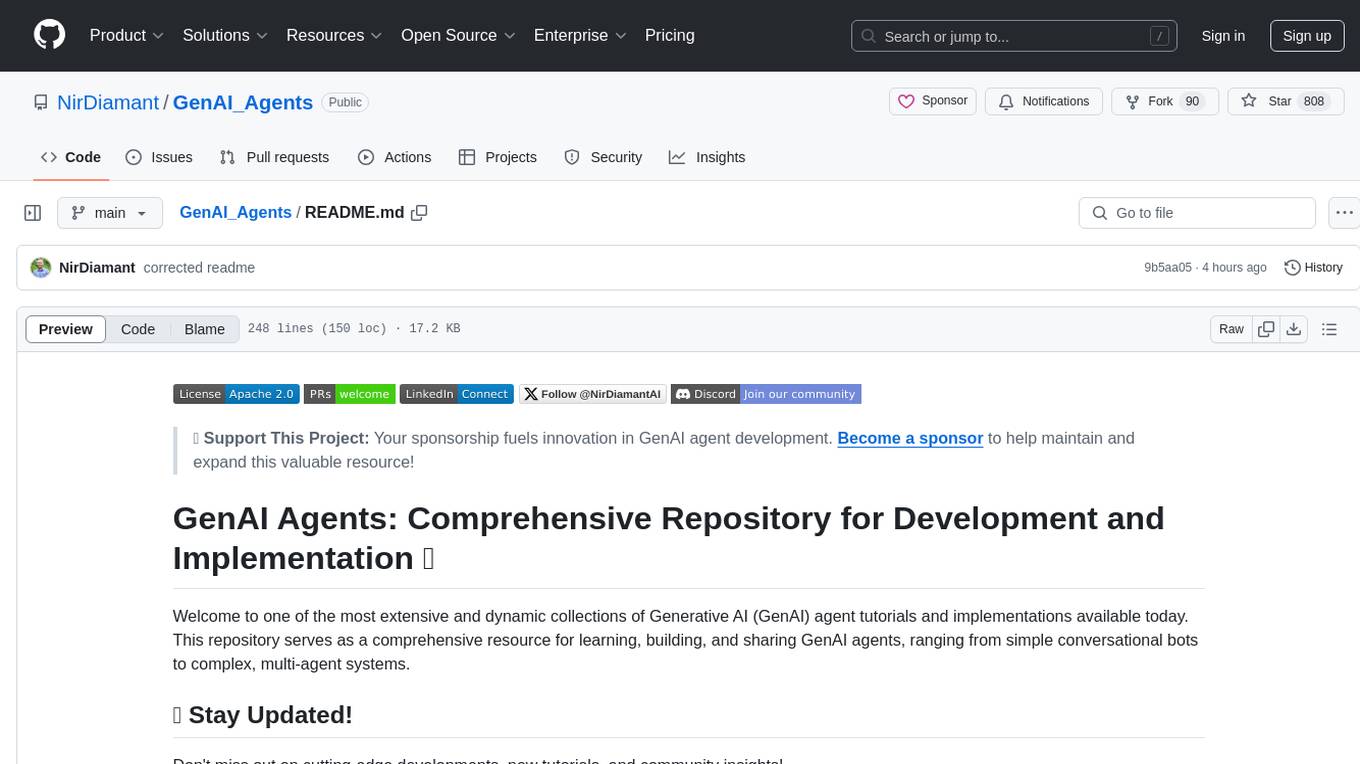
GenAI_Agents
GenAI Agents is a comprehensive repository for developing and implementing Generative AI (GenAI) agents, ranging from simple conversational bots to complex multi-agent systems. It serves as a valuable resource for learning, building, and sharing GenAI agents, offering tutorials, implementations, and a platform for showcasing innovative agent creations. The repository covers a wide range of agent architectures and applications, providing step-by-step tutorials, ready-to-use implementations, and regular updates on advancements in GenAI technology.
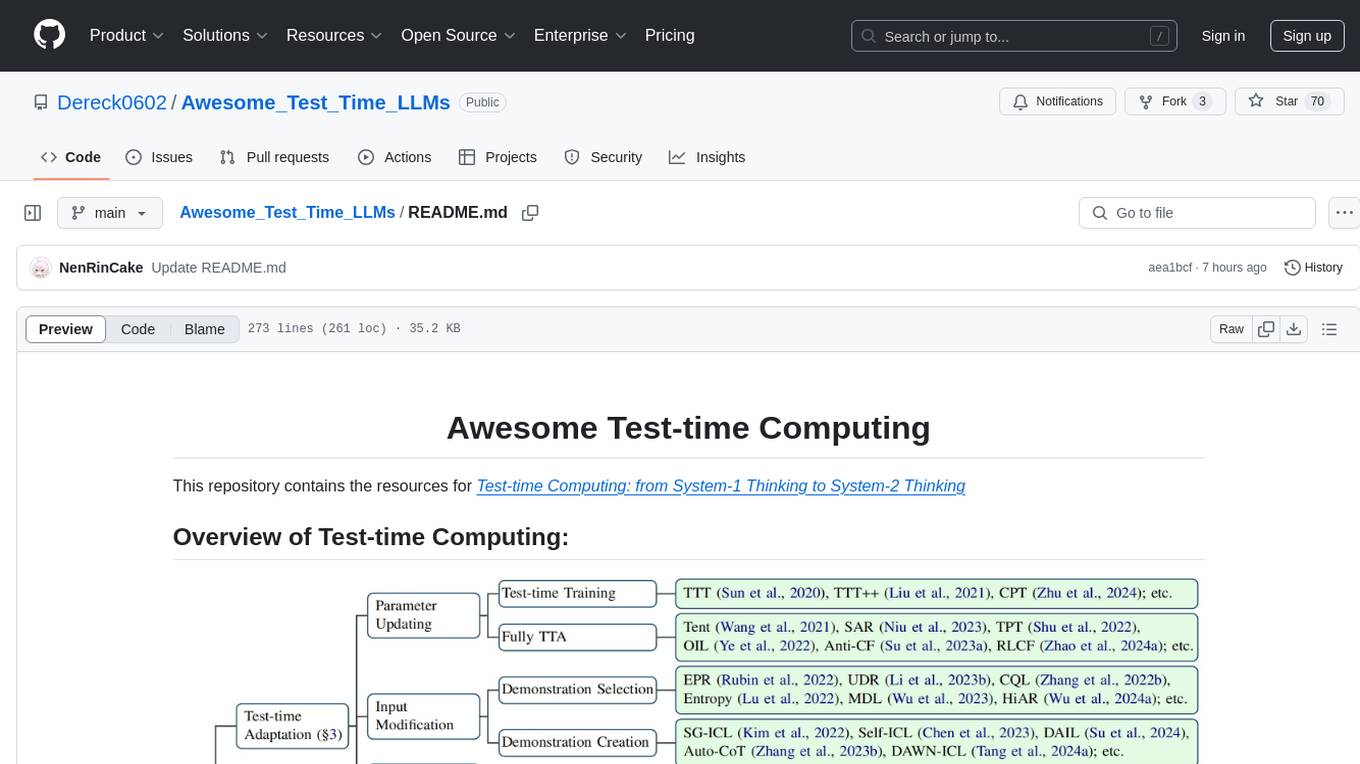
Awesome_Test_Time_LLMs
This repository focuses on test-time computing, exploring various strategies such as test-time adaptation, modifying the input, editing the representation, calibrating the output, test-time reasoning, and search strategies. It covers topics like self-supervised test-time training, in-context learning, activation steering, nearest neighbor models, reward modeling, and multimodal reasoning. The repository provides resources including papers and code for researchers and practitioners interested in enhancing the reasoning capabilities of large language models.
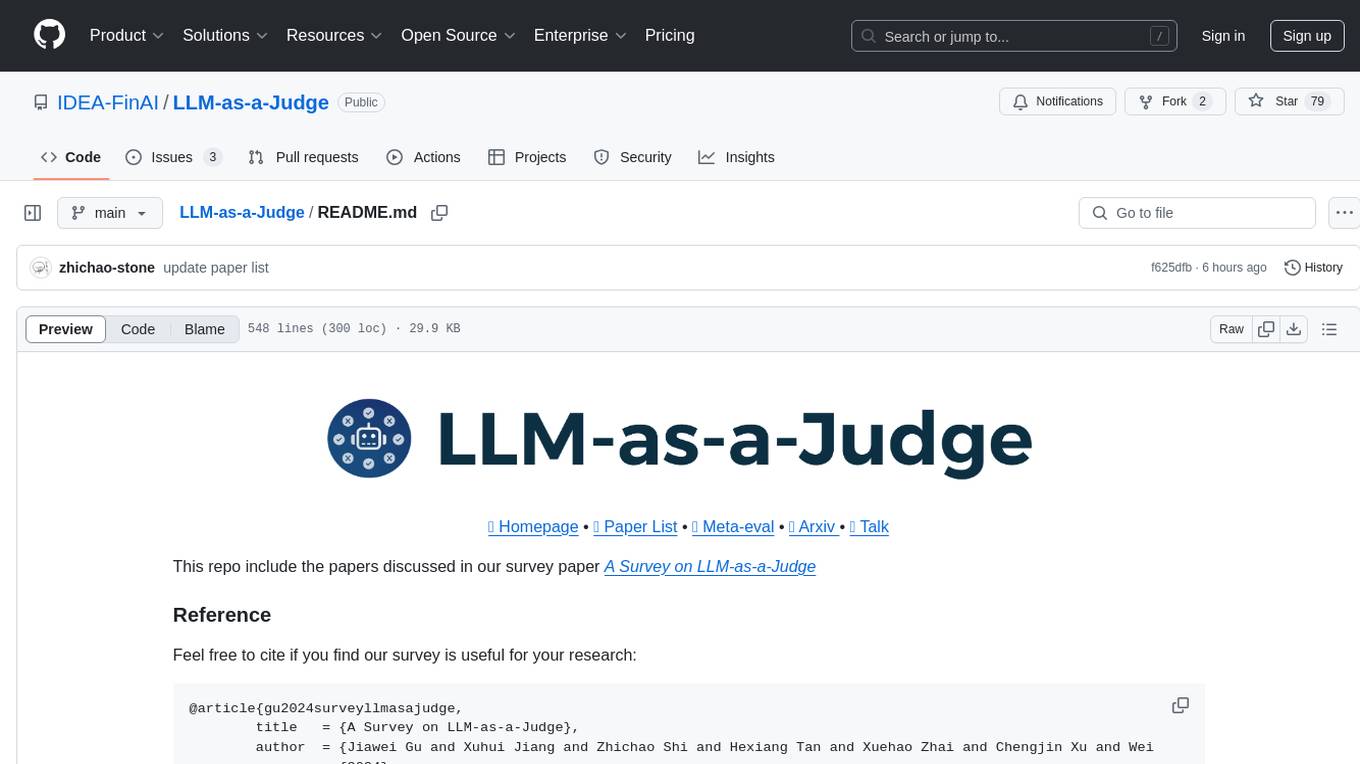
LLM-as-a-Judge
LLM-as-a-Judge is a repository that includes papers discussed in a survey paper titled 'A Survey on LLM-as-a-Judge'. The repository covers various aspects of using Large Language Models (LLMs) as judges for tasks such as evaluation, reasoning, and decision-making. It provides insights into evaluation pipelines, improvement strategies, and specific tasks related to LLMs. The papers included in the repository explore different methodologies, applications, and future research directions for leveraging LLMs as evaluators in various domains.
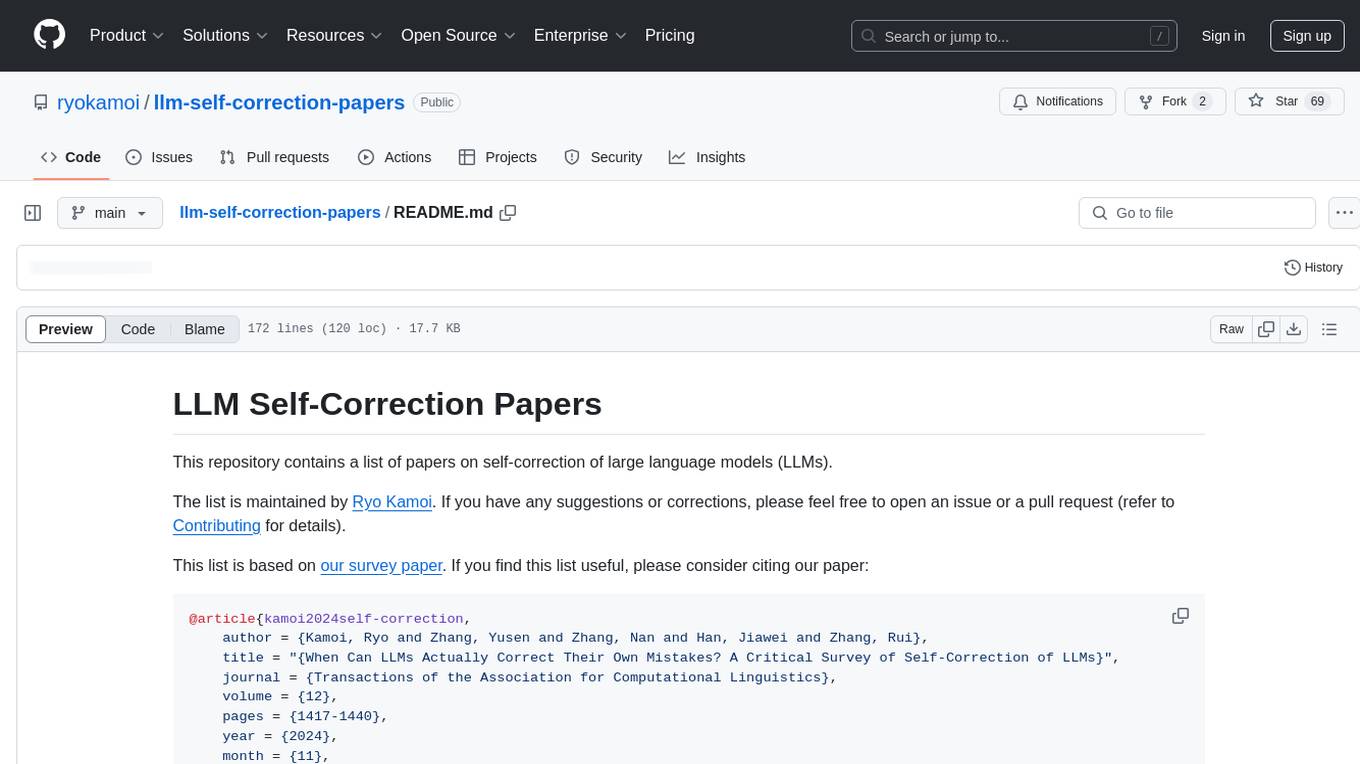
llm-self-correction-papers
This repository contains a curated list of papers focusing on the self-correction of large language models (LLMs) during inference. It covers various frameworks for self-correction, including intrinsic self-correction, self-correction with external tools, self-correction with information retrieval, and self-correction with training designed specifically for self-correction. The list includes survey papers, negative results, and frameworks utilizing reinforcement learning and OpenAI o1-like approaches. Contributions are welcome through pull requests following a specific format.
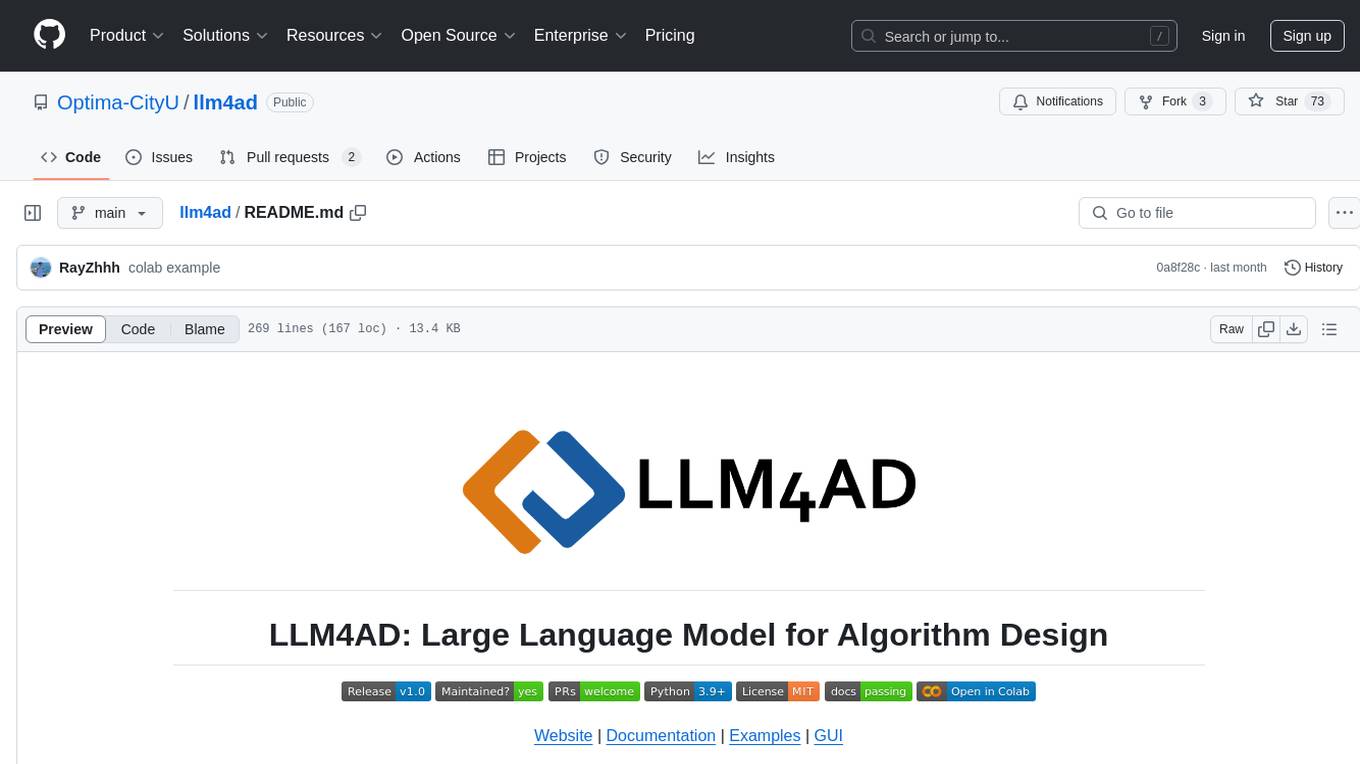
llm4ad
LLM4AD is an open-source Python-based platform leveraging Large Language Models (LLMs) for Automatic Algorithm Design (AD). It provides unified interfaces for methods, tasks, and LLMs, along with features like evaluation acceleration, secure evaluation, logs, GUI support, and more. The platform was originally developed for optimization tasks but is versatile enough to be used in other areas such as machine learning, science discovery, game theory, and engineering design. It offers various search methods and algorithm design tasks across different domains. LLM4AD supports remote LLM API, local HuggingFace LLM deployment, and custom LLM interfaces. The project is licensed under the MIT License and welcomes contributions, collaborations, and issue reports.
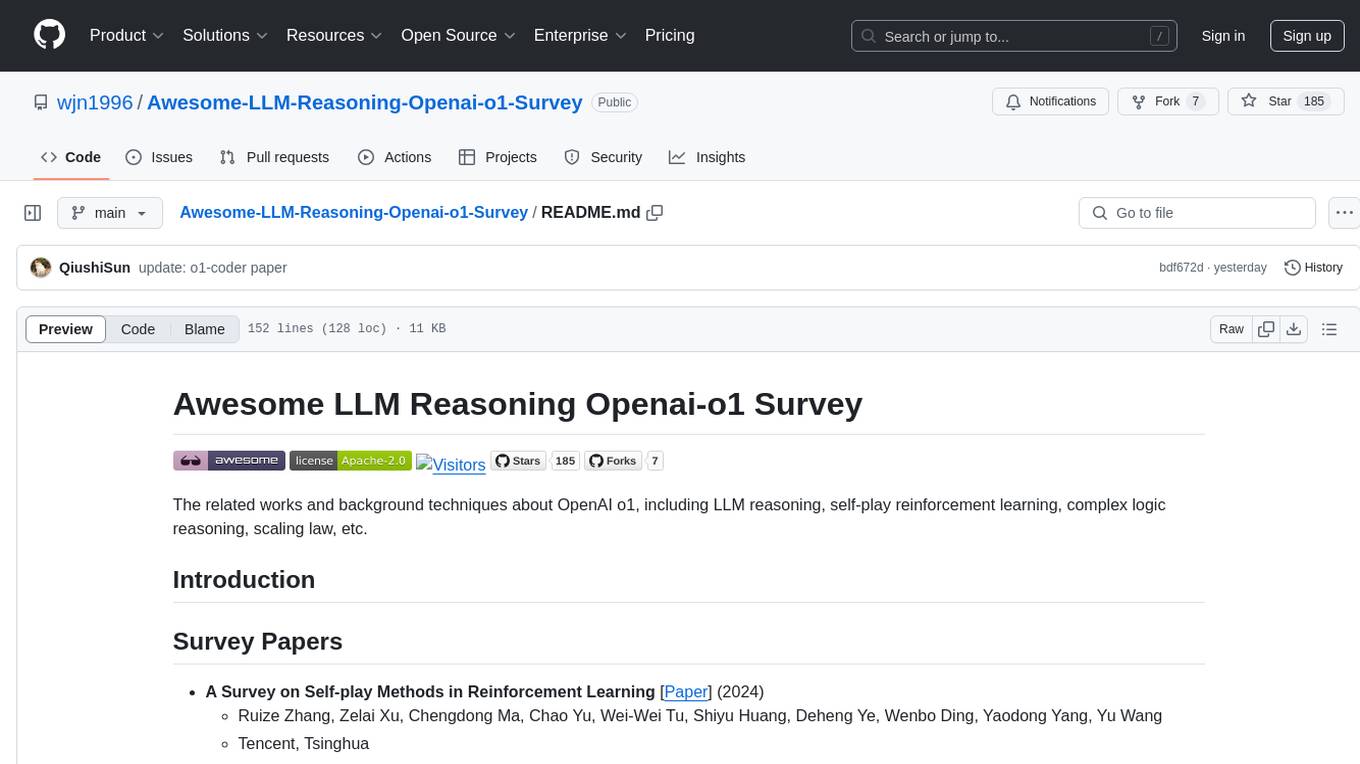
Awesome-LLM-Reasoning-Openai-o1-Survey
The repository 'Awesome LLM Reasoning Openai-o1 Survey' provides a collection of survey papers and related works on OpenAI o1, focusing on topics such as LLM reasoning, self-play reinforcement learning, complex logic reasoning, and scaling law. It includes papers from various institutions and researchers, showcasing advancements in reasoning bootstrapping, reasoning scaling law, self-play learning, step-wise and process-based optimization, and applications beyond math. The repository serves as a valuable resource for researchers interested in exploring the intersection of language models and reasoning techniques.
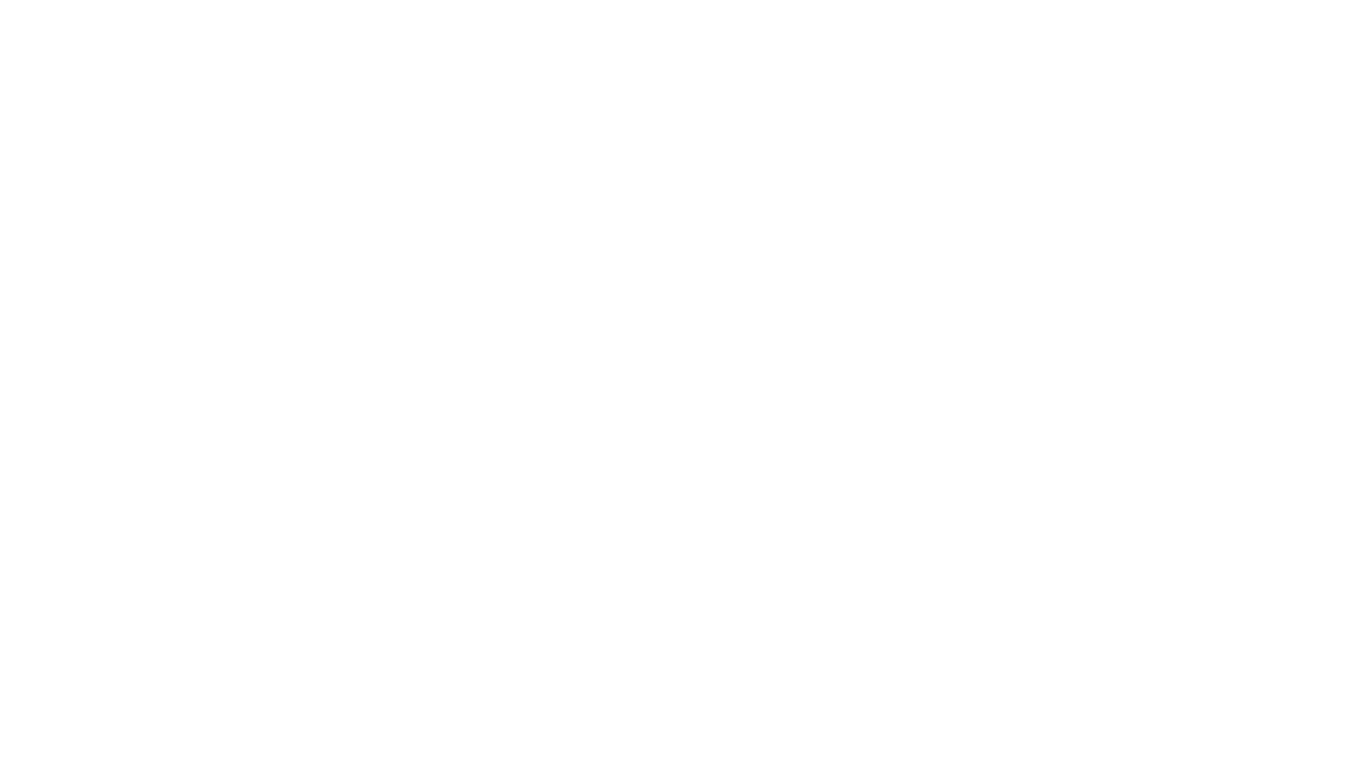
rag-experiment-accelerator
The RAG Experiment Accelerator is a versatile tool that helps you conduct experiments and evaluations using Azure AI Search and RAG pattern. It offers a rich set of features, including experiment setup, integration with Azure AI Search, Azure Machine Learning, MLFlow, and Azure OpenAI, multiple document chunking strategies, query generation, multiple search types, sub-querying, re-ranking, metrics and evaluation, report generation, and multi-lingual support. The tool is designed to make it easier and faster to run experiments and evaluations of search queries and quality of response from OpenAI, and is useful for researchers, data scientists, and developers who want to test the performance of different search and OpenAI related hyperparameters, compare the effectiveness of various search strategies, fine-tune and optimize parameters, find the best combination of hyperparameters, and generate detailed reports and visualizations from experiment results.
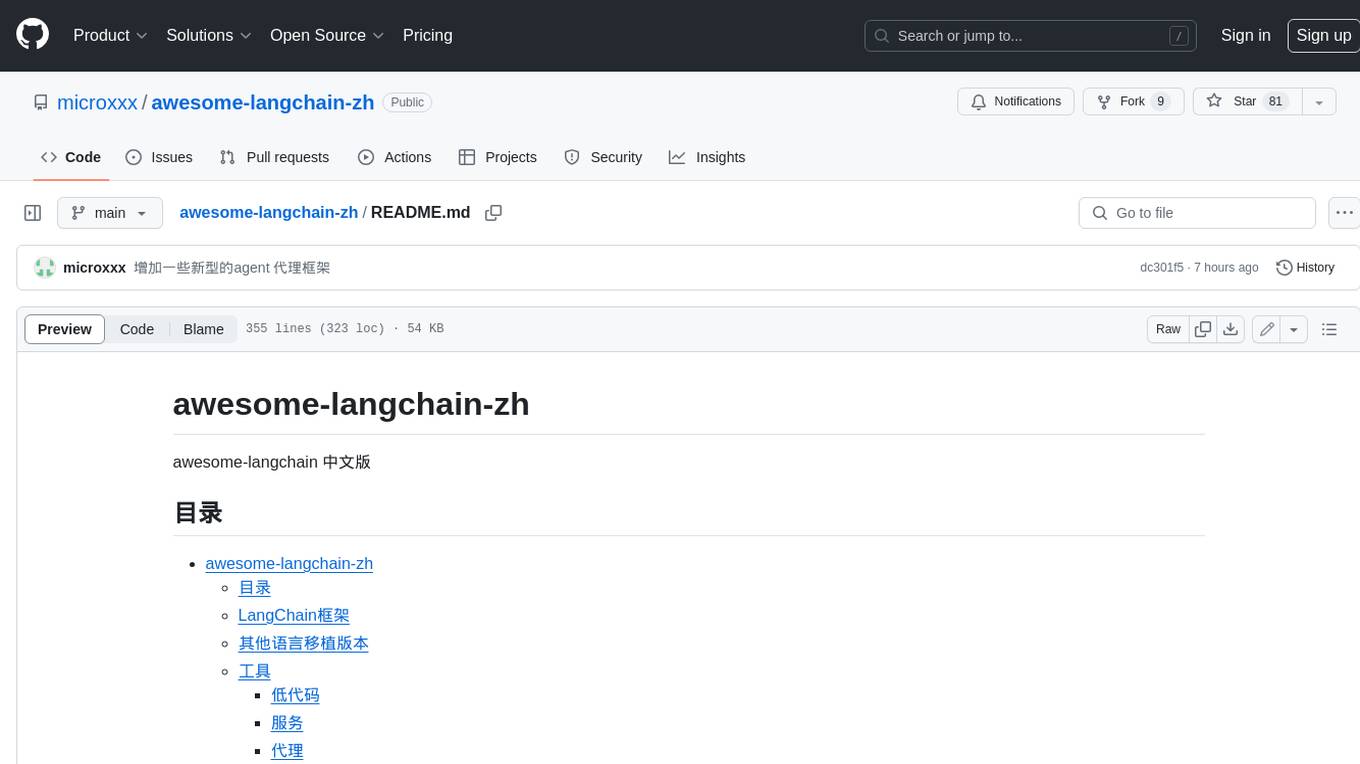
awesome-langchain-zh
The awesome-langchain-zh repository is a collection of resources related to LangChain, a framework for building AI applications using large language models (LLMs). The repository includes sections on the LangChain framework itself, other language ports of LangChain, tools for low-code development, services, agents, templates, platforms, open-source projects related to knowledge management and chatbots, as well as learning resources such as notebooks, videos, and articles. It also covers other LLM frameworks and provides additional resources for exploring and working with LLMs. The repository serves as a comprehensive guide for developers and AI enthusiasts interested in leveraging LangChain and LLMs for various applications.
20 - OpenAI Gpts
MEICCA expert
Experto en educación y evaluación de aprendizajes. Parte de equipo de investigación del proyecto MEICCA
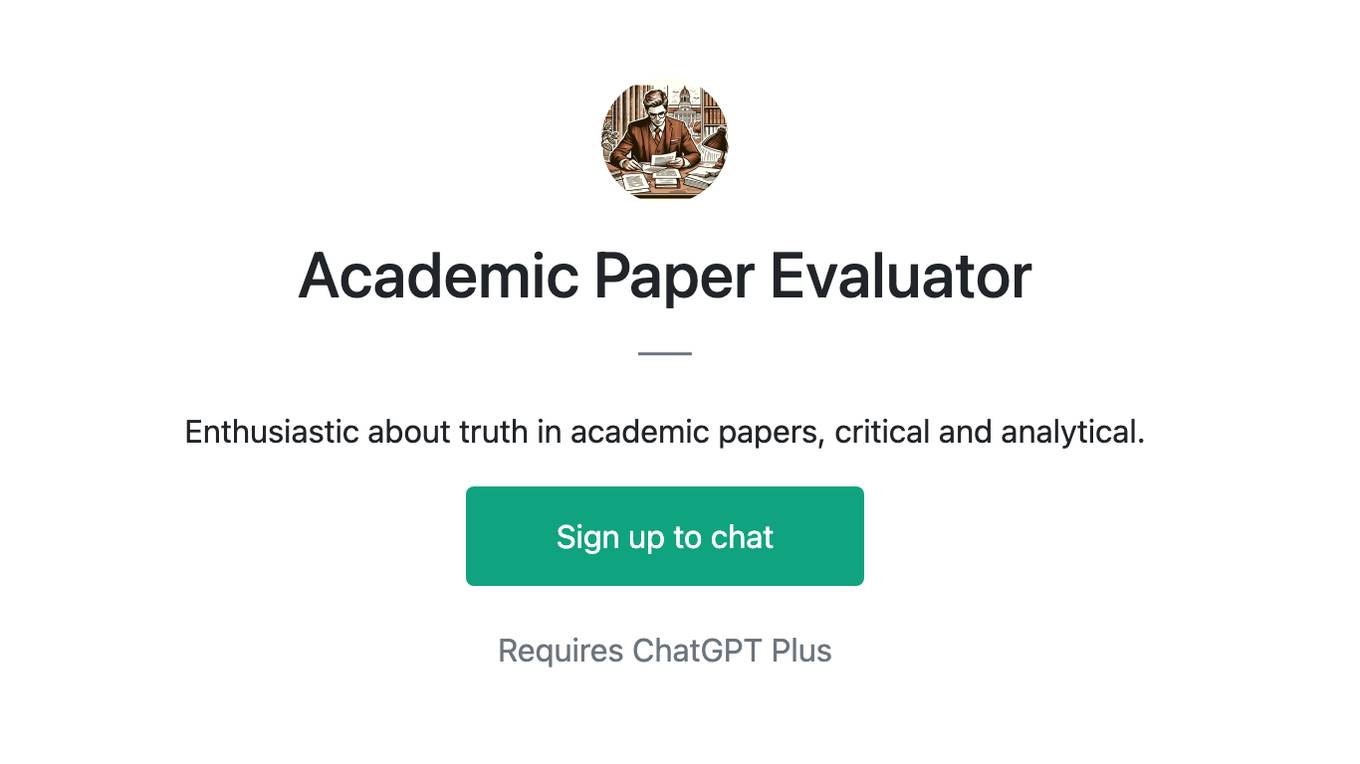
Academic Paper Evaluator
Enthusiastic about truth in academic papers, critical and analytical.
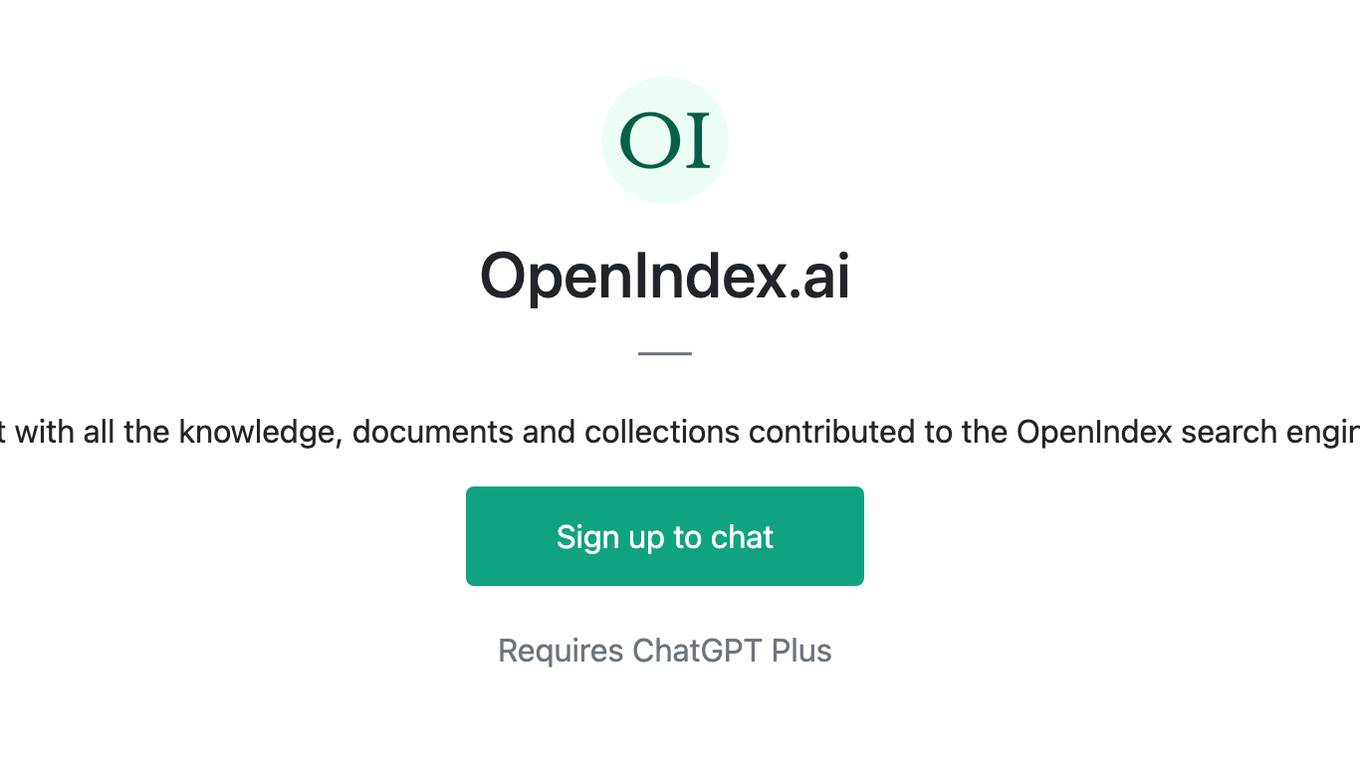
OpenIndex.ai
Chat with all the knowledge, documents and collections contributed to the OpenIndex search engine.
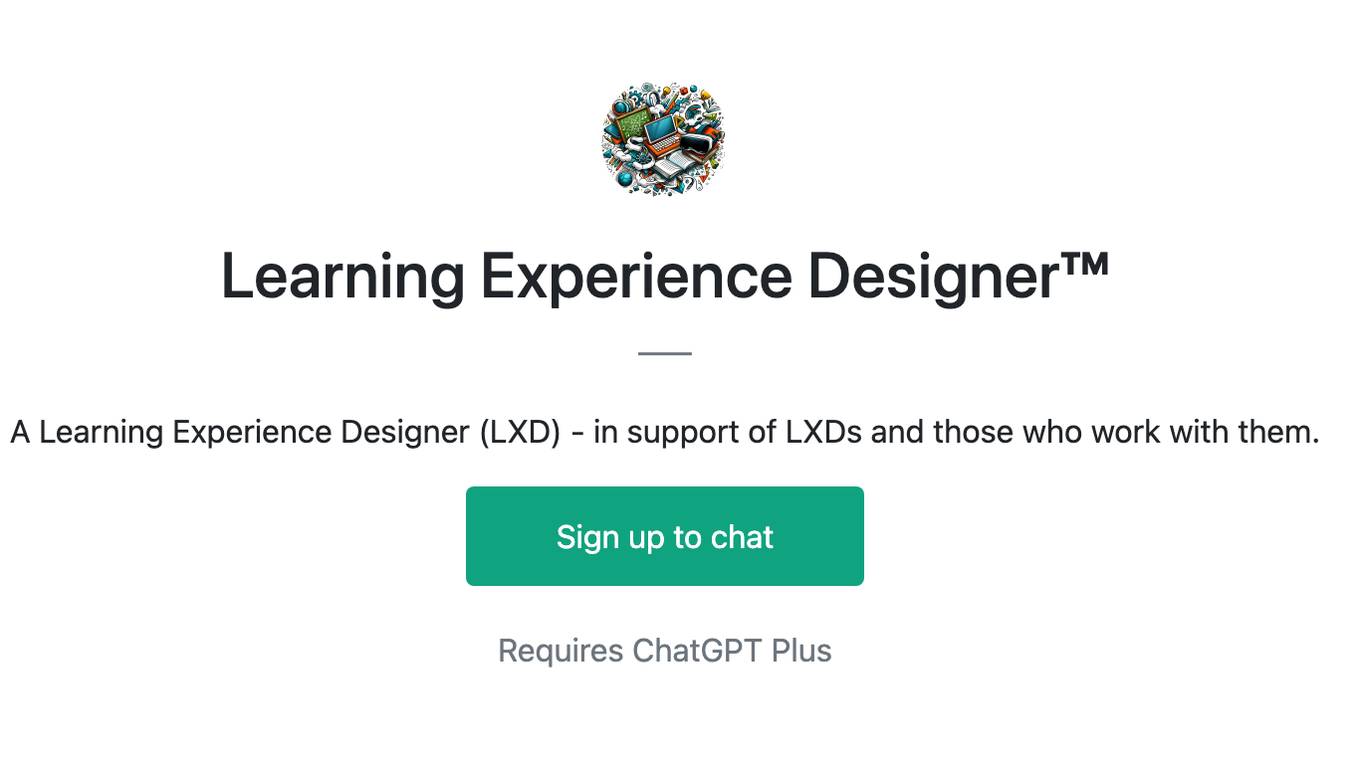
Learning Experience Designer™
A Learning Experience Designer (LXD) - in support of LXDs and those who work with them.
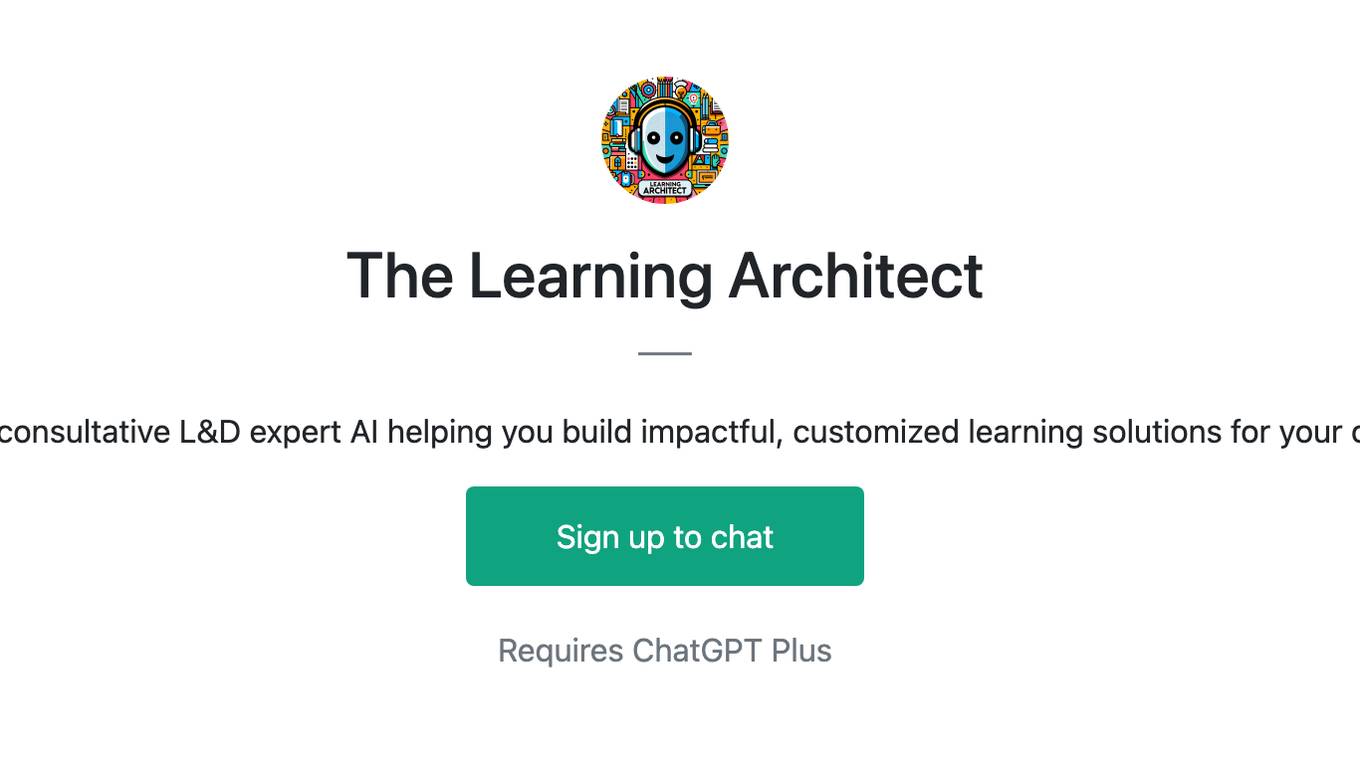
The Learning Architect
An all-in-one, consultative L&D expert AI helping you build impactful, customized learning solutions for your organization.
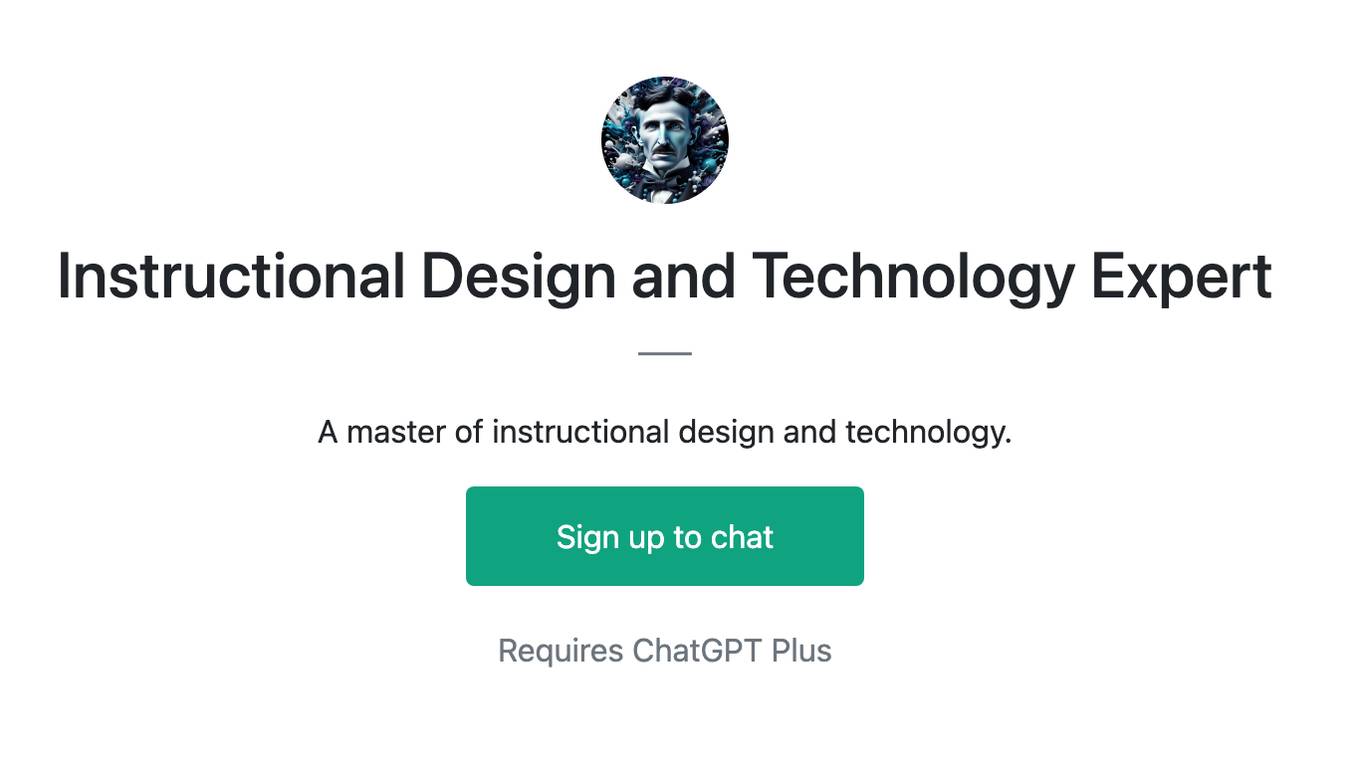
Instructional Design and Technology Expert
A master of instructional design and technology.
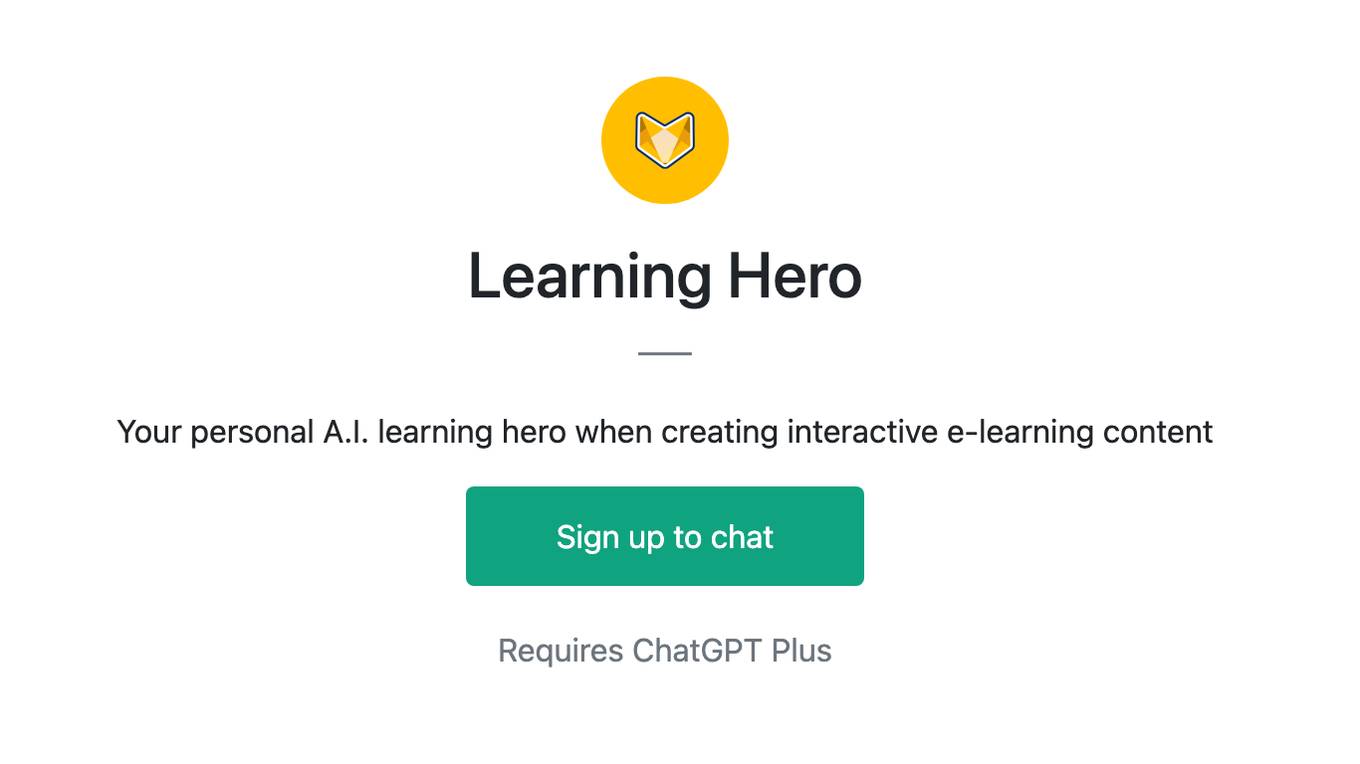
Learning Hero
Your personal A.I. learning hero when creating interactive e-learning content
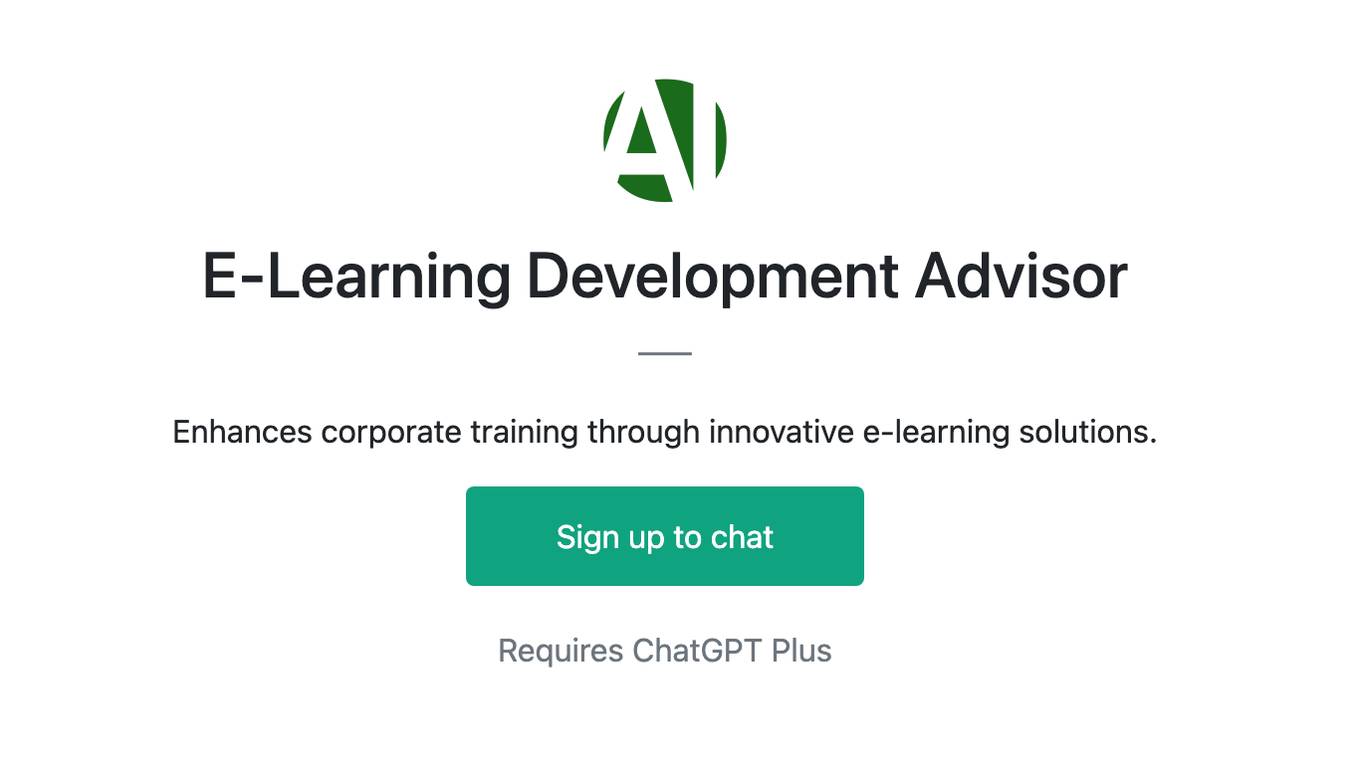
E-Learning Development Advisor
Enhances corporate training through innovative e-learning solutions.
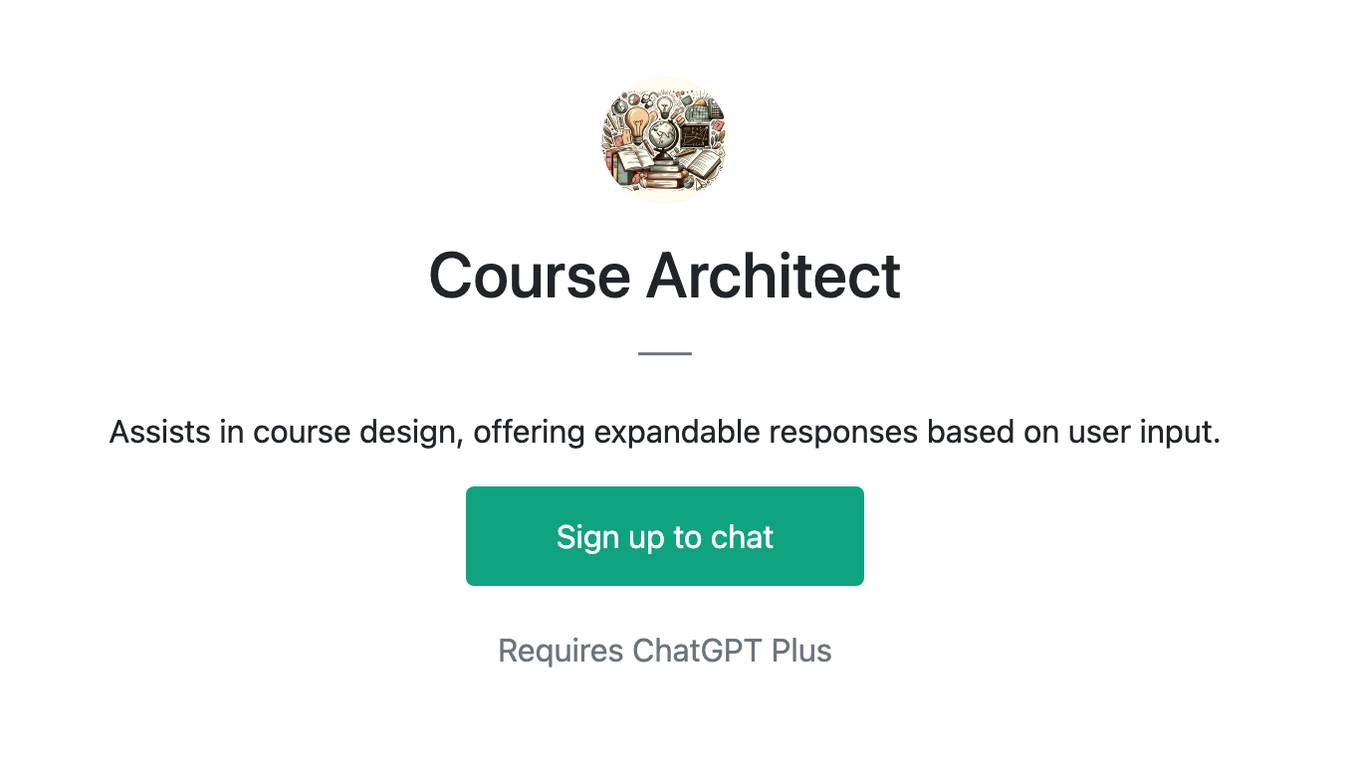
Course Architect
Assists in course design, offering expandable responses based on user input.
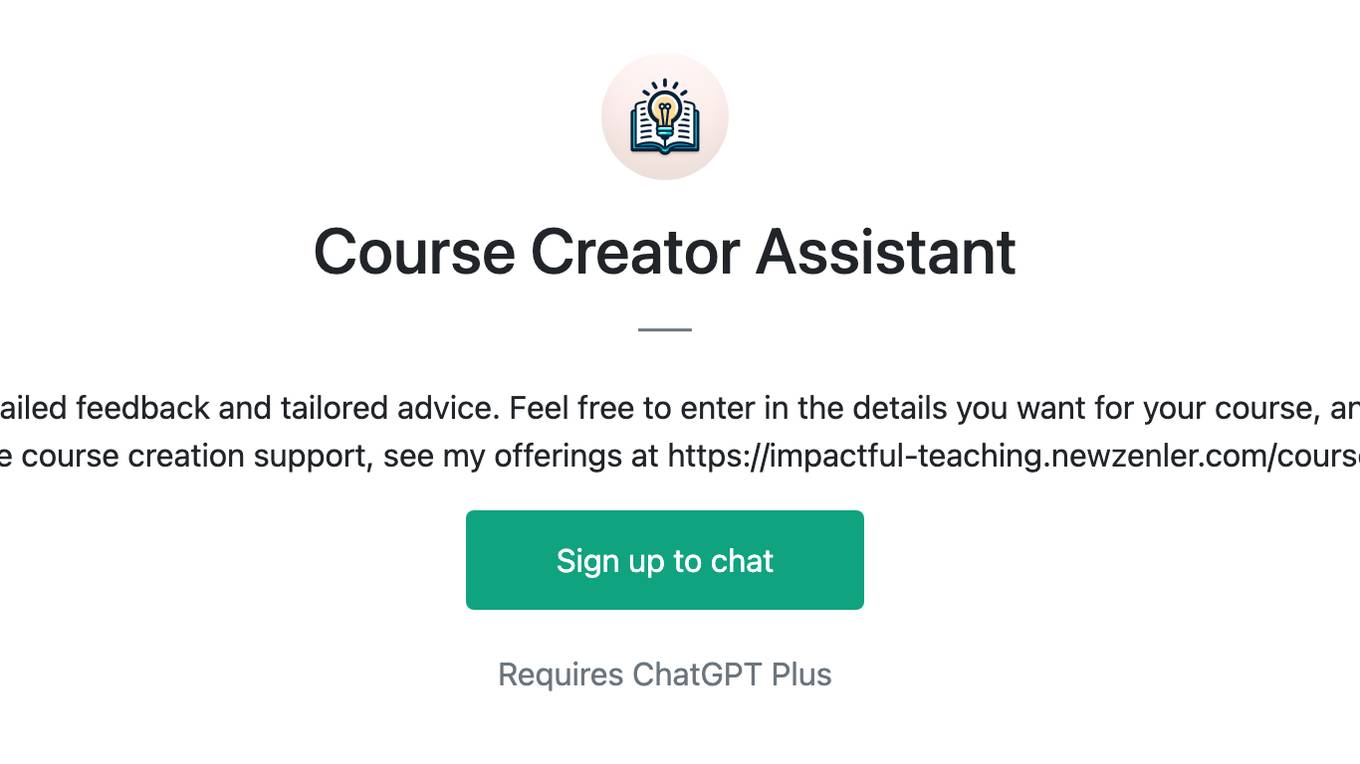
Course Creator Assistant
Expert in online course creation, offering detailed feedback and tailored advice. Feel free to enter in the details you want for your course, and you will receive an outline and more! For more course creation support, see my offerings at https://impactful-teaching.newzenler.com/courses
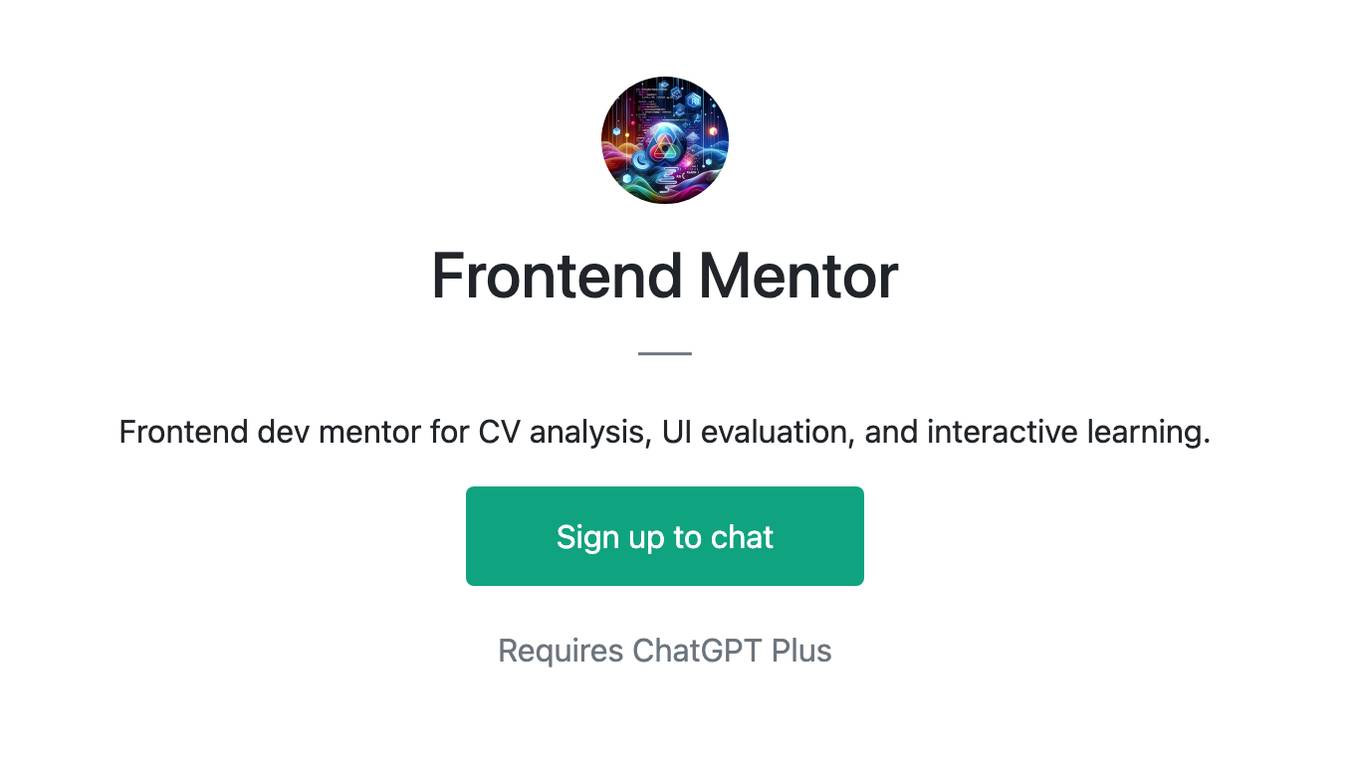
Frontend Mentor
Frontend dev mentor for CV analysis, UI evaluation, and interactive learning.
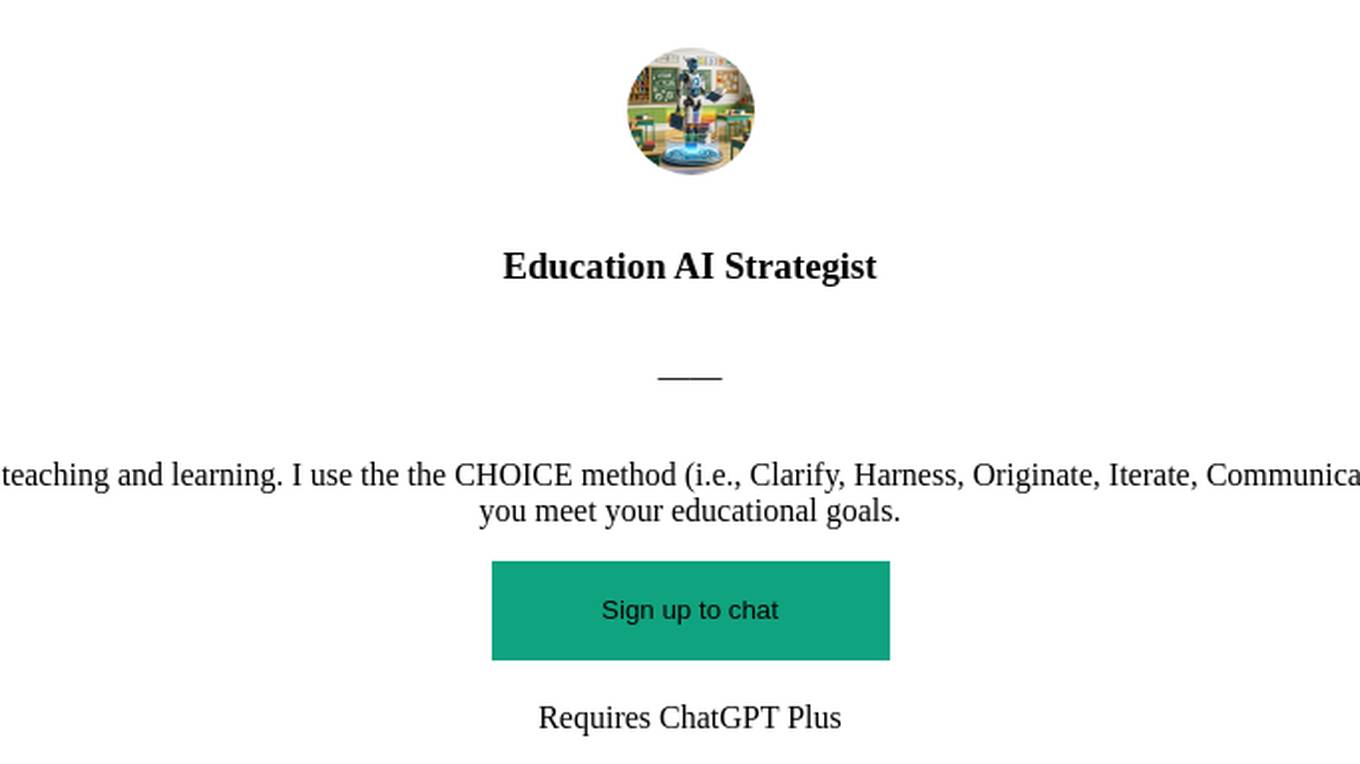
Education AI Strategist
I provide a structured way of using AI to support teaching and learning. I use the the CHOICE method (i.e., Clarify, Harness, Originate, Iterate, Communicate, Evaluate) to ensure that your use of AI can help you meet your educational goals.
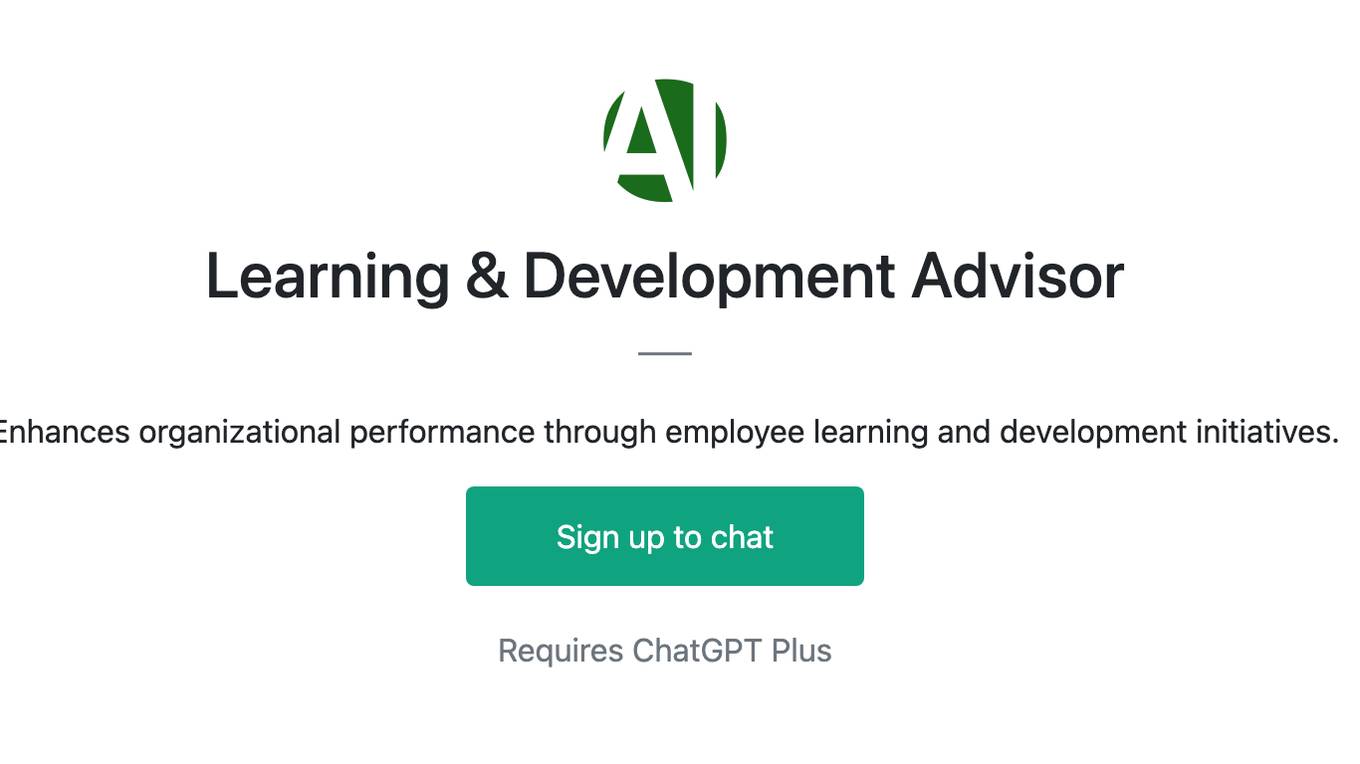
Learning & Development Advisor
Enhances organizational performance through employee learning and development initiatives.