AI tools for LLM
Related Jobs:
Related Tools:
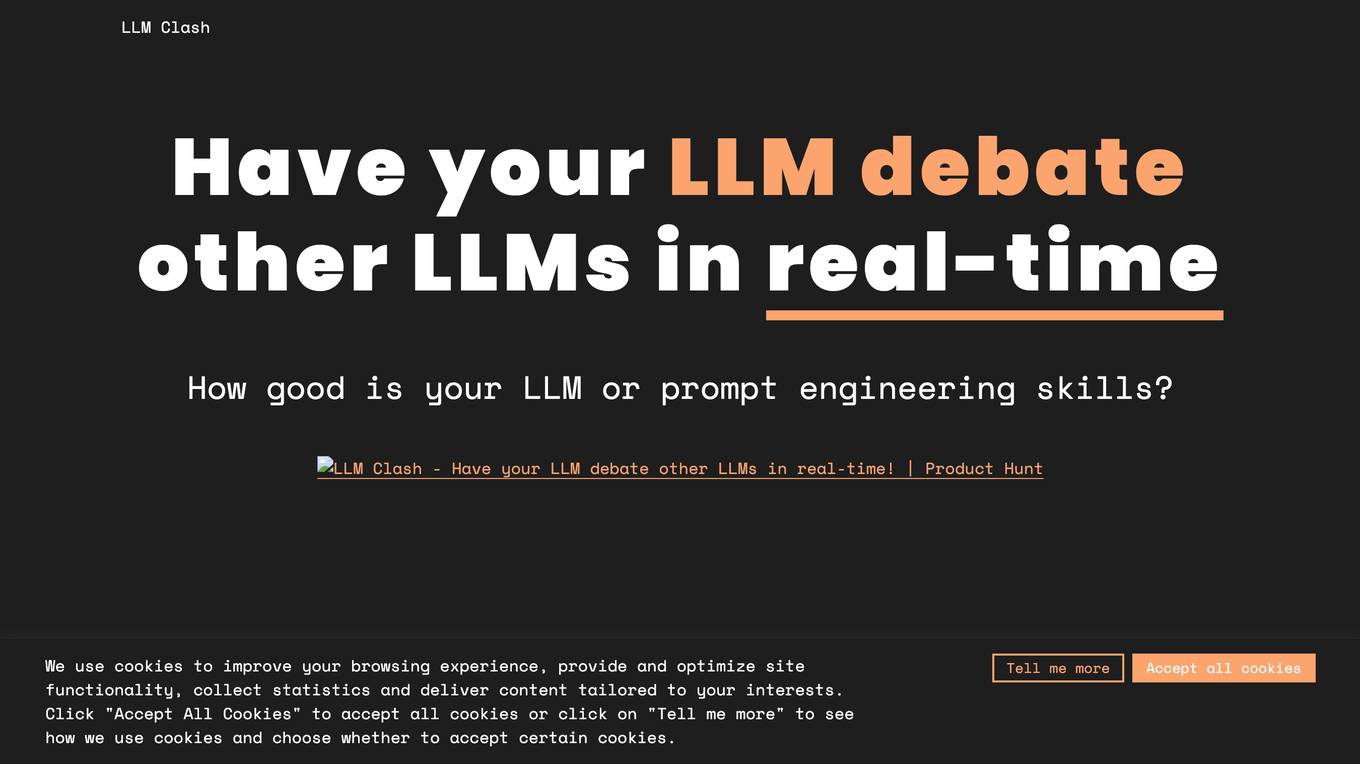
LLM Clash
LLM Clash is a web-based application that allows users to compare the outputs of different large language models (LLMs) on a given task. Users can input a prompt and select which LLMs they want to compare. The application will then display the outputs of the LLMs side-by-side, allowing users to compare their strengths and weaknesses.
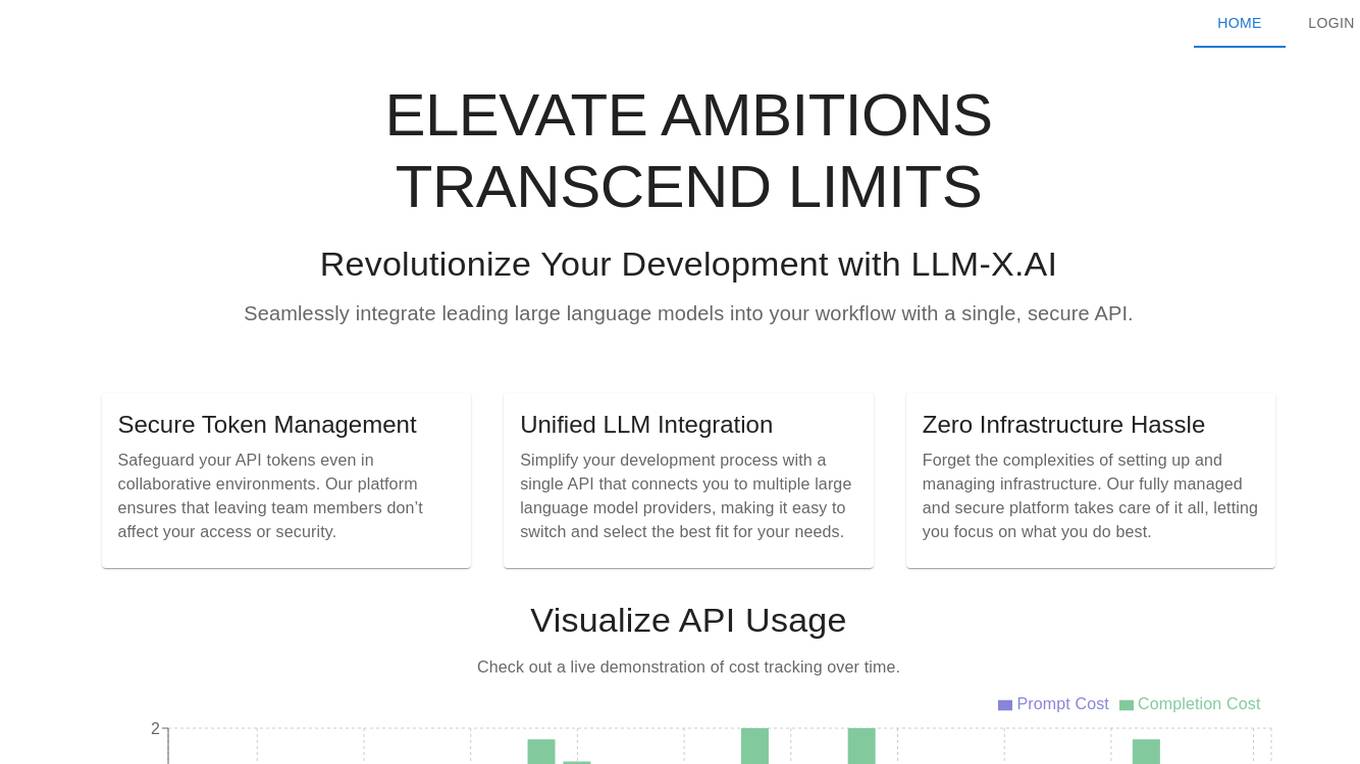
None
I am sorry, but the provided text does not contain any information about a website or AI tool. Therefore, I cannot generate the requested JSON object.
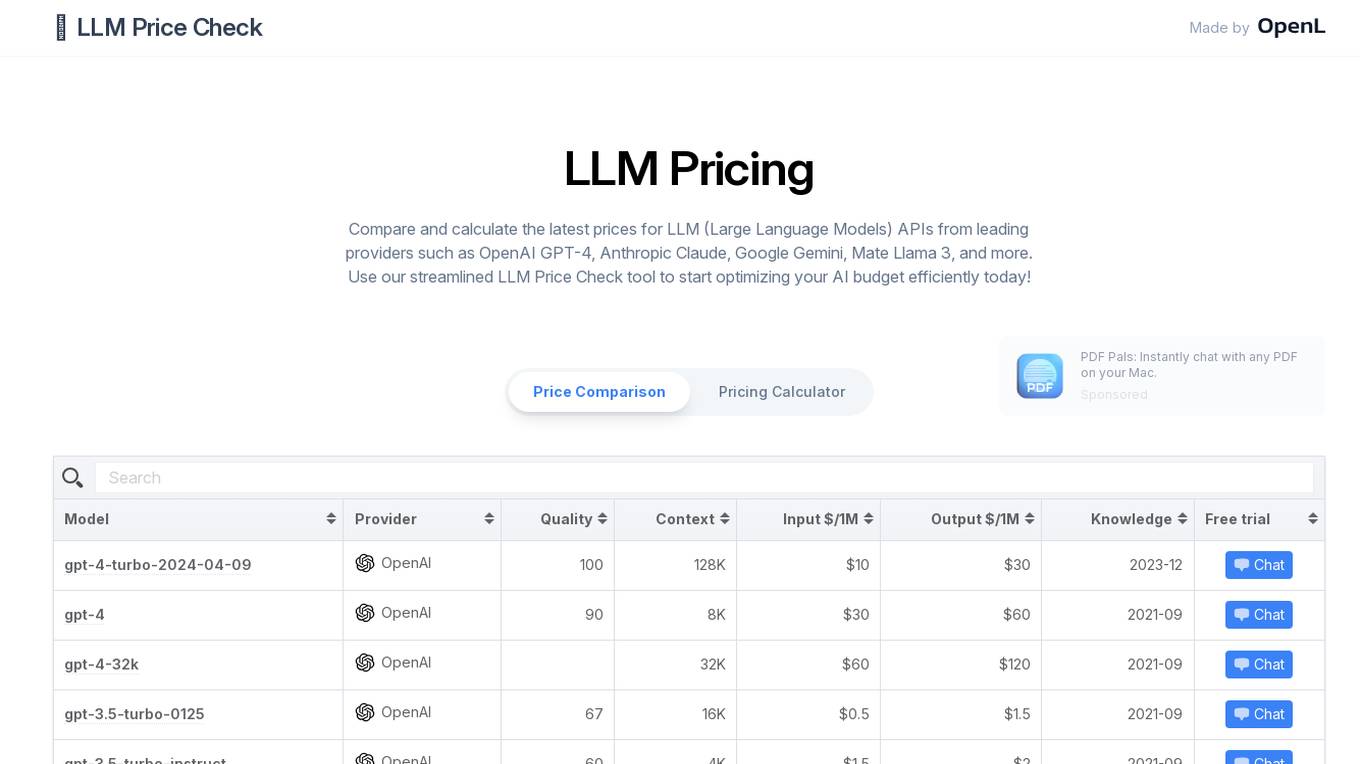
LLM Price Check
LLM Price Check is an AI tool designed to compare and calculate the latest prices for Large Language Models (LLM) APIs from leading providers such as OpenAI, Anthropic, Google, and more. Users can use the streamlined tool to optimize their AI budget efficiently by comparing pricing, sorting by various parameters, and searching for specific models. The tool provides a comprehensive overview of pricing information to help users make informed decisions when selecting an LLM API provider.
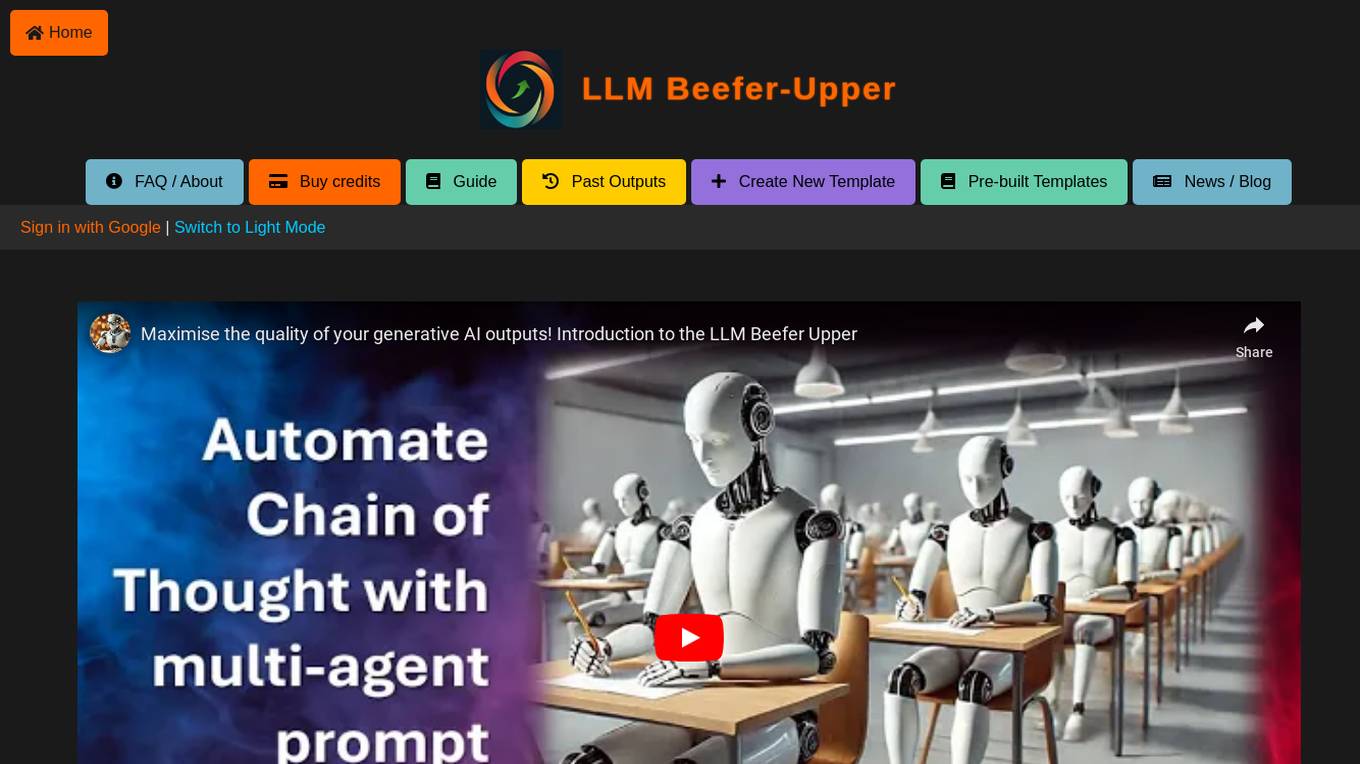
LLM Quality Beefer-Upper
LLM Quality Beefer-Upper is an AI tool designed to enhance the quality and productivity of LLM responses by automating critique, reflection, and improvement. Users can generate multi-agent prompt drafts, choose from different quality levels, and upload knowledge text for processing. The application aims to maximize output quality by utilizing the best available LLM models in the market.
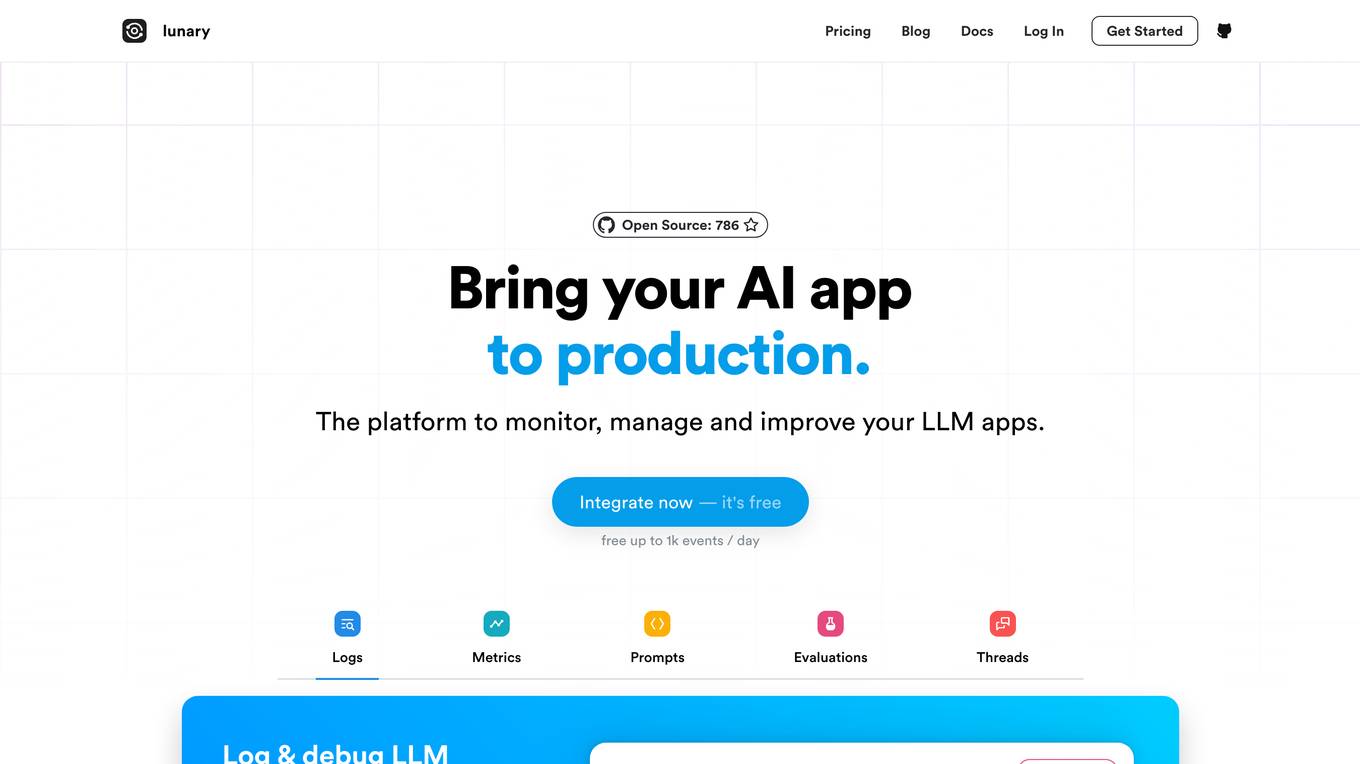
Lunary
Lunary is an AI developer platform designed to bring AI applications to production. It offers a comprehensive set of tools to manage, improve, and protect LLM apps. With features like Logs, Metrics, Prompts, Evaluations, and Threads, Lunary empowers users to monitor and optimize their AI agents effectively. The platform supports tasks such as tracing errors, labeling data for fine-tuning, optimizing costs, running benchmarks, and testing open-source models. Lunary also facilitates collaboration with non-technical teammates through features like A/B testing, versioning, and clean source-code management.
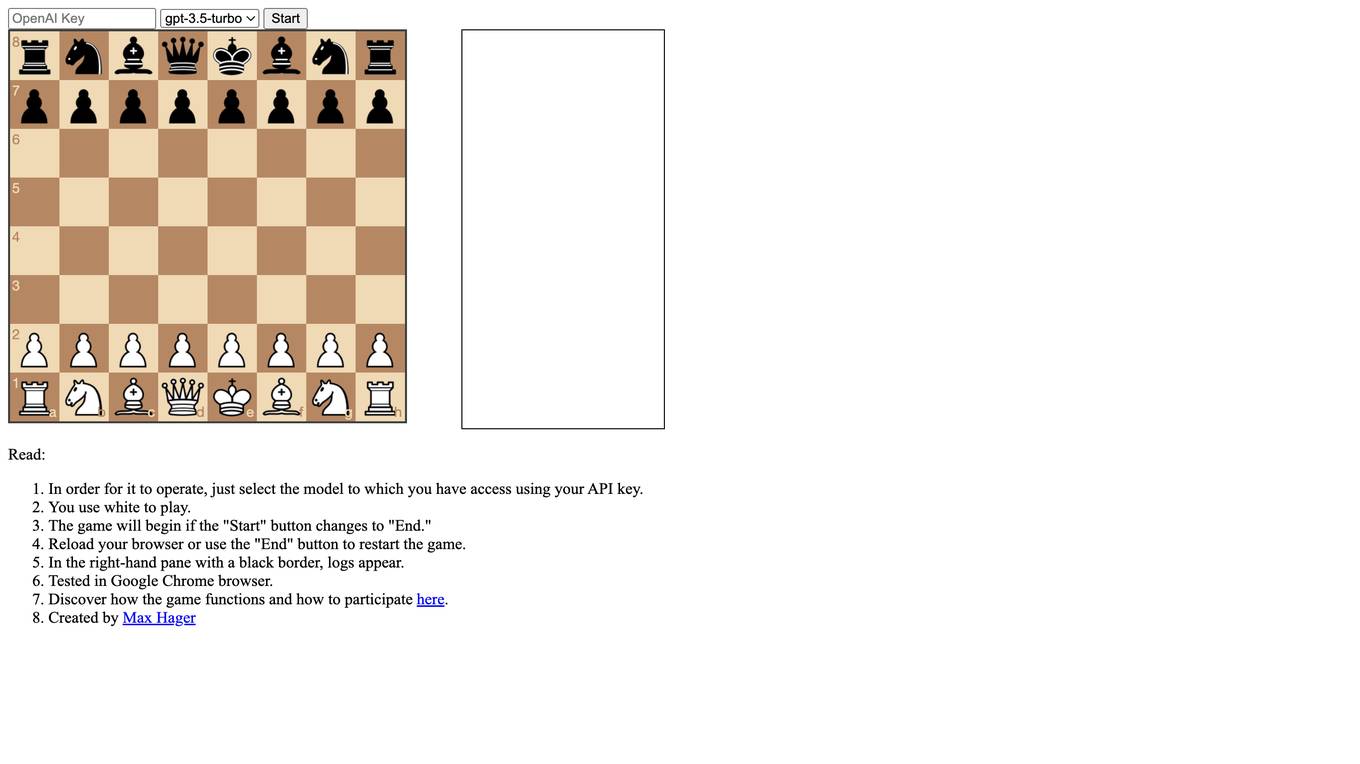
LLMChess
LLMChess is a web-based chess game that utilizes large language models (LLMs) to power the gameplay. Players can select the LLM model they wish to play against, and the game will commence once the "Start" button is clicked. The game logs are displayed in a black-bordered pane on the right-hand side of the screen. LLMChess is compatible with the Google Chrome browser. For more information on the game's functionality and participation guidelines, please refer to the provided link.
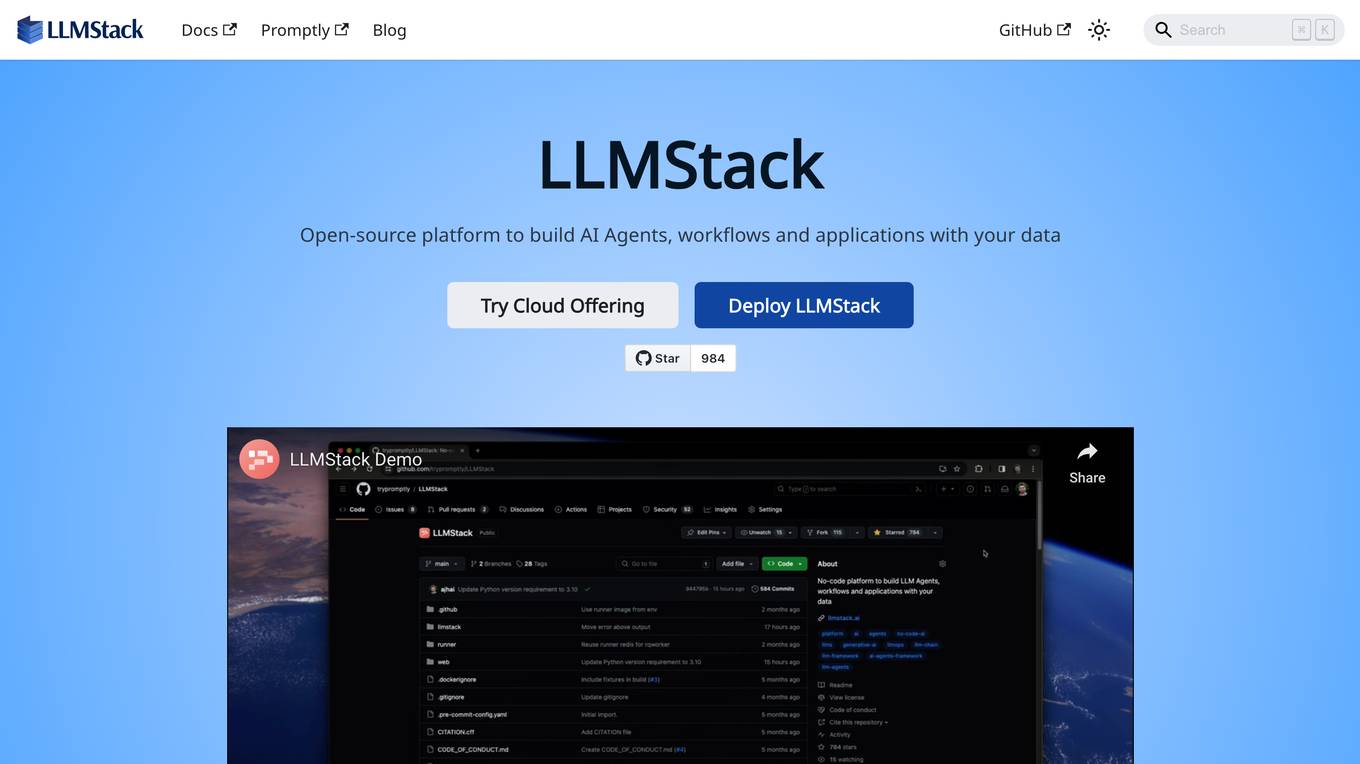
LLMStack
LLMStack is an open-source platform that allows users to build AI Agents, workflows, and applications using their own data. It is a no-code AI app builder that supports model chaining from major providers like OpenAI, Cohere, Stability AI, and Hugging Face. Users can import various data sources such as Web URLs, PDFs, audio files, and more to enhance generative AI applications and chatbots. With a focus on collaboration, LLMStack enables users to share apps publicly or restrict access, with viewer and collaborator roles for multiple users to work together. Powered by React, LLMStack provides an easy-to-use interface for building AI applications.
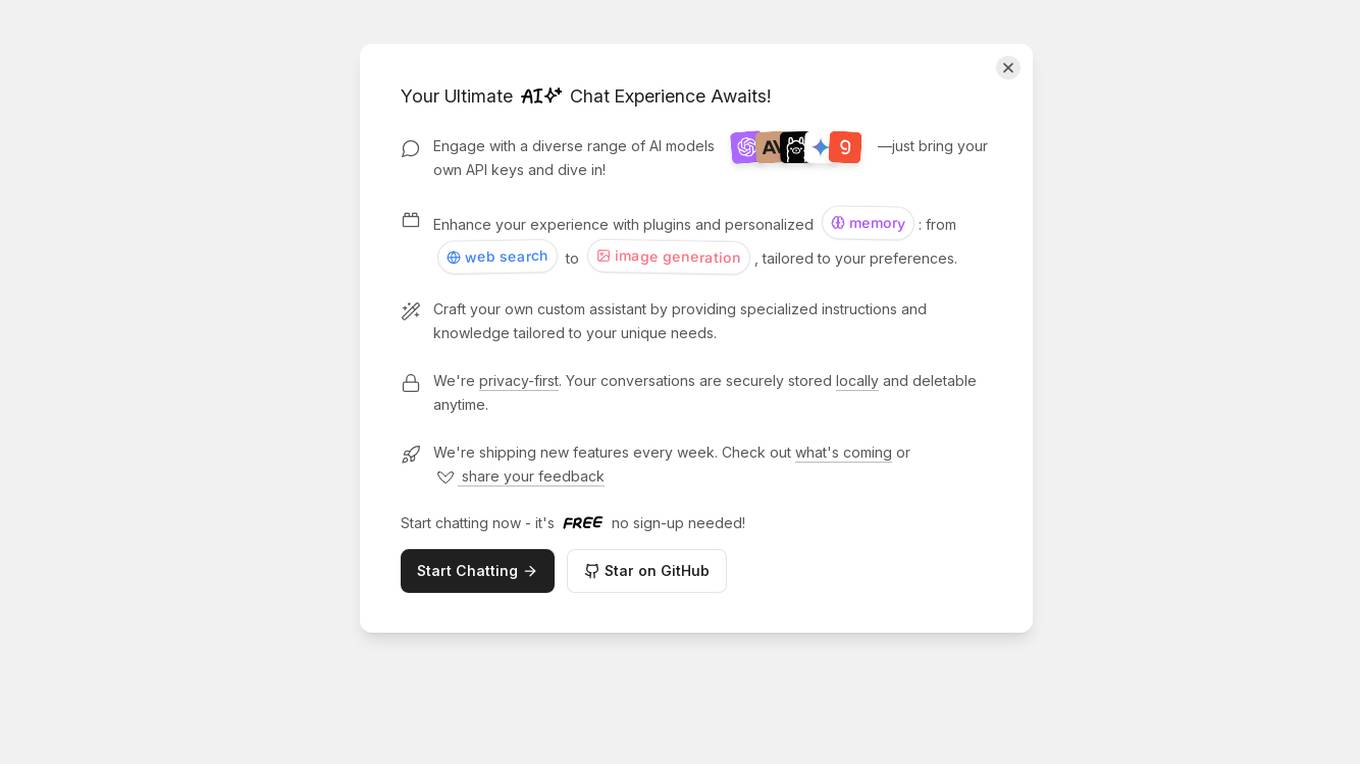
LLMChat
LLMChat is an advanced AI chat application that provides users with the ultimate chat experience. It utilizes cutting-edge artificial intelligence technology to engage in natural conversations, answer queries, and assist users in various tasks. LLMChat is designed to be user-friendly and intuitive, making it suitable for both personal and professional use. With its powerful AI capabilities, LLMChat aims to revolutionize the way people interact with chat applications, offering a seamless and efficient communication experience.
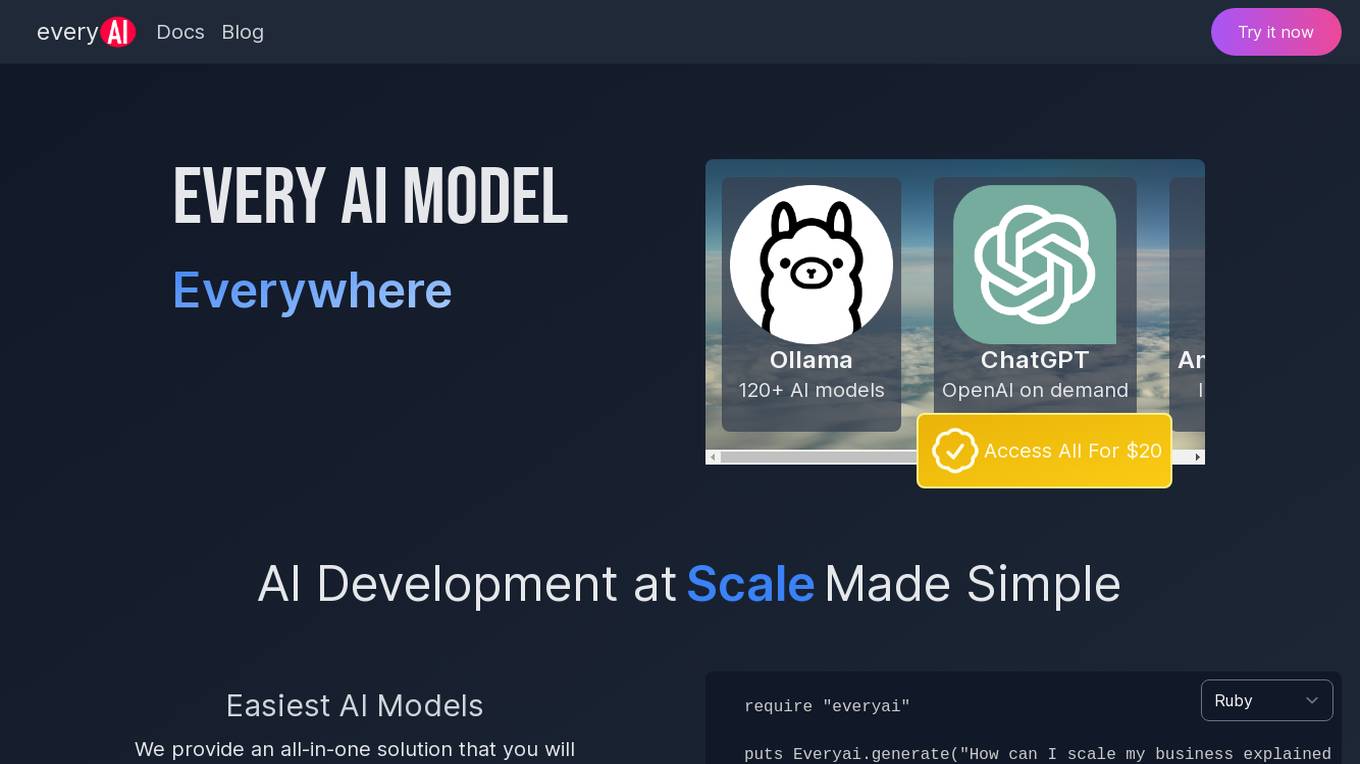
Every AI
Every AI is an AI software that offers over 120 AI models, including ChatGPT from OpenAI and Anthropic/Claude, for a wide range of applications. It provides incredible speeds and access to all models for a subscription fee of $20. The platform aims to simplify AI development at scale by offering developer-friendly solutions with extensive documentation and SDKs for popular programming languages like Ruby and JavaScript.
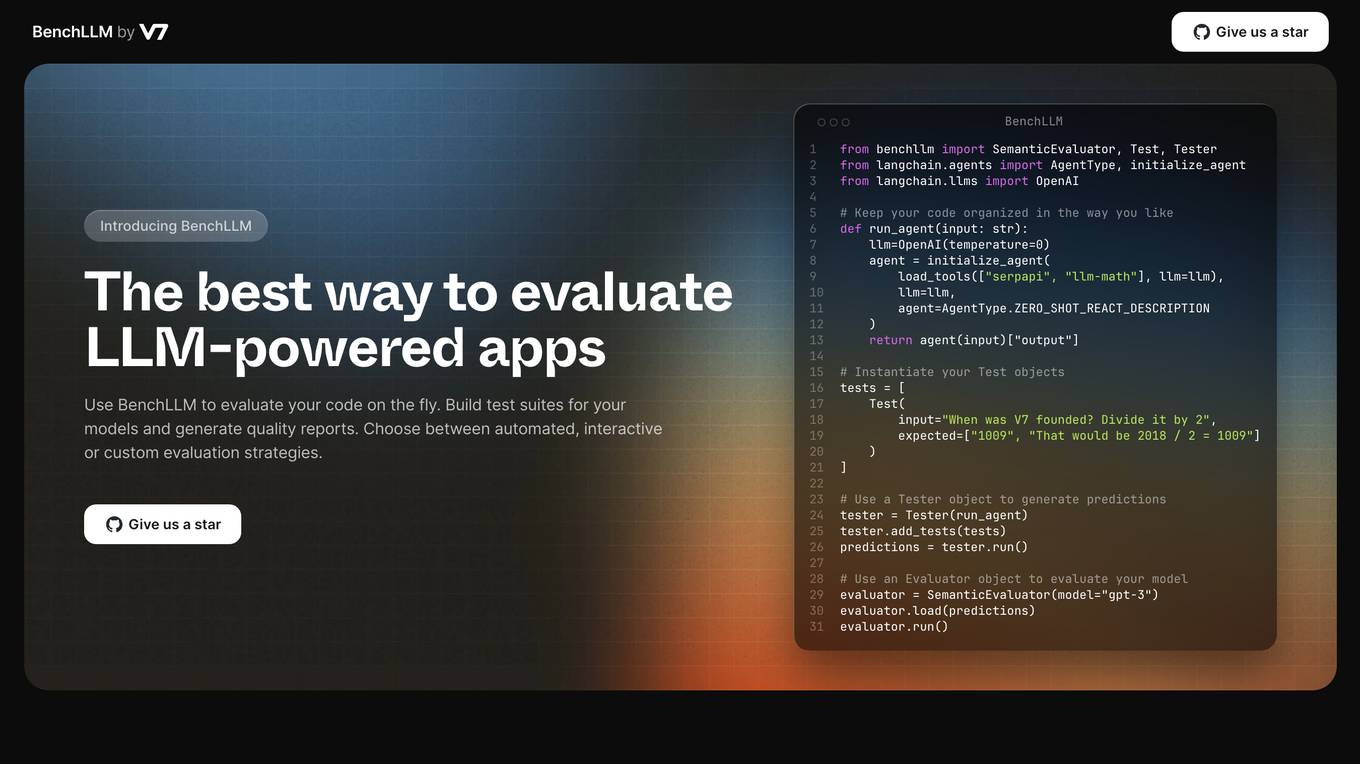
BenchLLM
BenchLLM is an AI tool designed for AI engineers to evaluate LLM-powered apps by running and evaluating models with a powerful CLI. It allows users to build test suites, choose evaluation strategies, and generate quality reports. The tool supports OpenAI, Langchain, and other APIs out of the box, offering automation, visualization of reports, and monitoring of model performance.
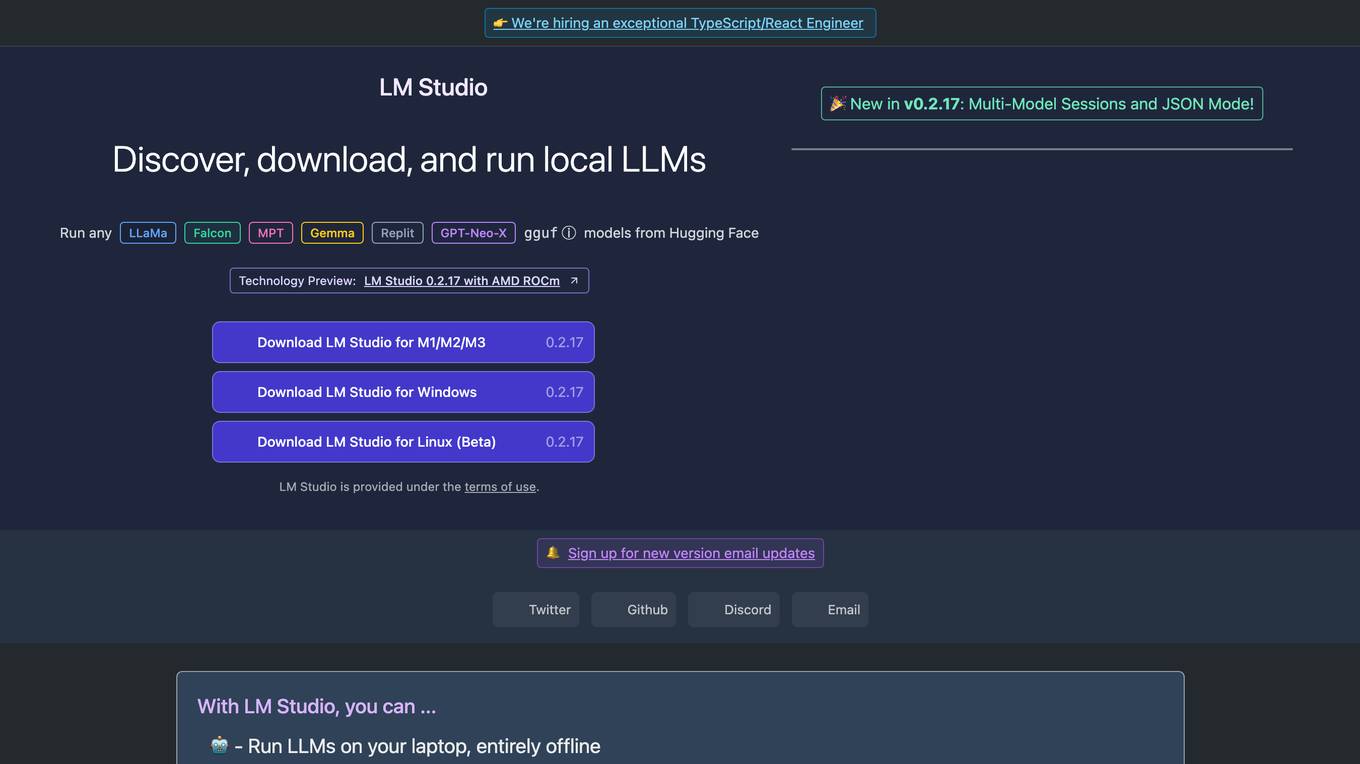
LM Studio
LM Studio is an AI tool designed for discovering, downloading, and running local LLMs (Large Language Models). Users can run LLMs on their laptops offline, use models through an in-app Chat UI or a local server, download compatible model files from HuggingFace repositories, and discover new LLMs. The tool ensures privacy by not collecting data or monitoring user actions, making it suitable for personal and business use. LM Studio supports various models like ggml Llama, MPT, and StarCoder on Hugging Face, with minimum hardware/software requirements specified for different platforms.
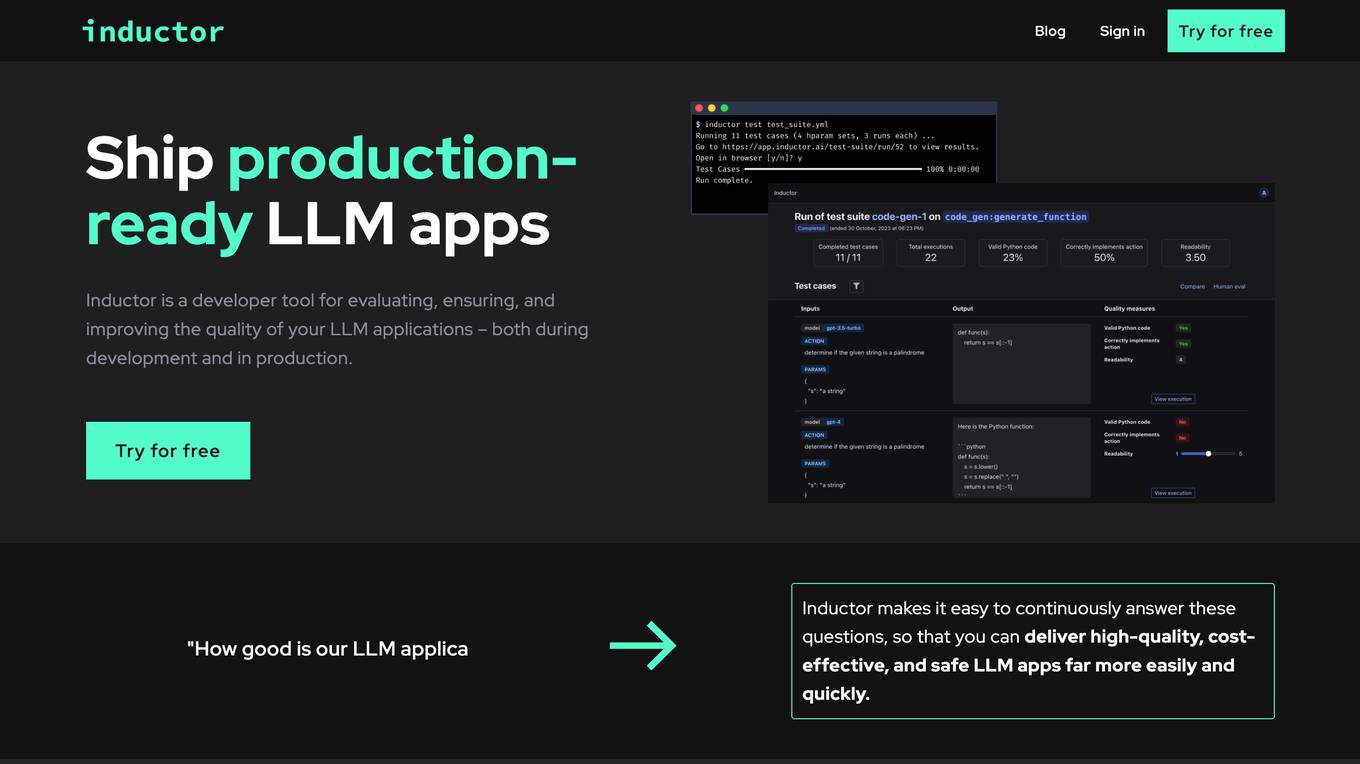
Inductor
Inductor is a developer tool for evaluating, ensuring, and improving the quality of your LLM applications – both during development and in production. It provides a fantastic workflow for continuous testing and evaluation as you develop, so that you always know your LLM app’s quality. Systematically improve quality and cost-effectiveness by actionably understanding your LLM app’s behavior and quickly testing different app variants. Rigorously assess your LLM app’s behavior before you deploy, in order to ensure quality and cost-effectiveness when you’re live. Easily monitor your live traffic: detect and resolve issues, analyze usage in order to improve, and seamlessly feed back into your development process. Inductor makes it easy for engineering and other roles to collaborate: get critical human feedback from non-engineering stakeholders (e.g., PM, UX, or subject matter experts) to ensure that your LLM app is user-ready.
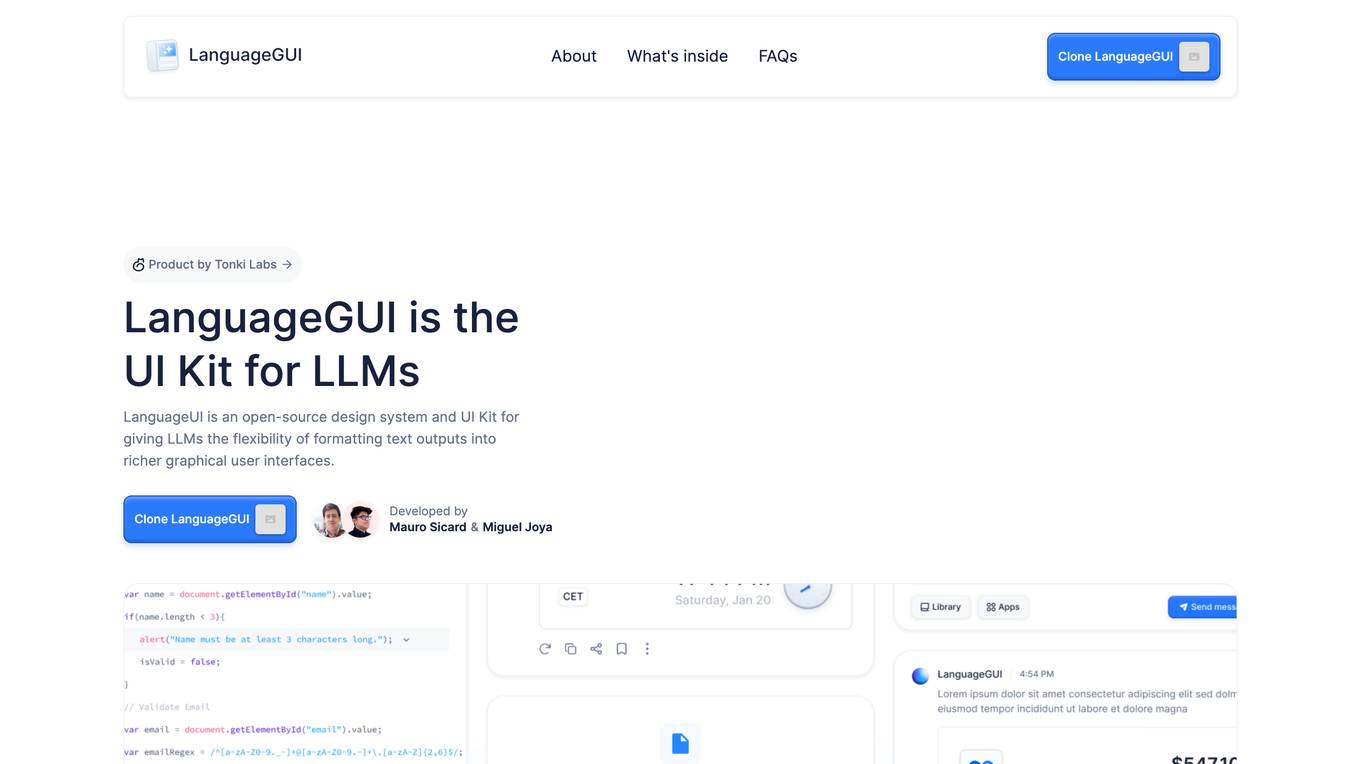
LanguageGUI
LanguageGUI is an open-source design system and UI Kit for giving LLMs the flexibility of formatting text outputs into richer graphical user interfaces. It includes dozens of unique UI elements that serve different use cases for rich conversational user interfaces, such as 100+ UI components & customizable screens, 10+ conversational UI widgets, 20+ chat bubbles, 30+ pre-built screens to kickoff your design, 5+ chat sidebars with customizable settings, multi-prompt workflow screen designs, 8+ prompt boxes, and dark mode. LanguageGUI is designed with variables and styles, designed with Figma Auto Layout, and is free to use for both personal and commercial projects without required attribution.
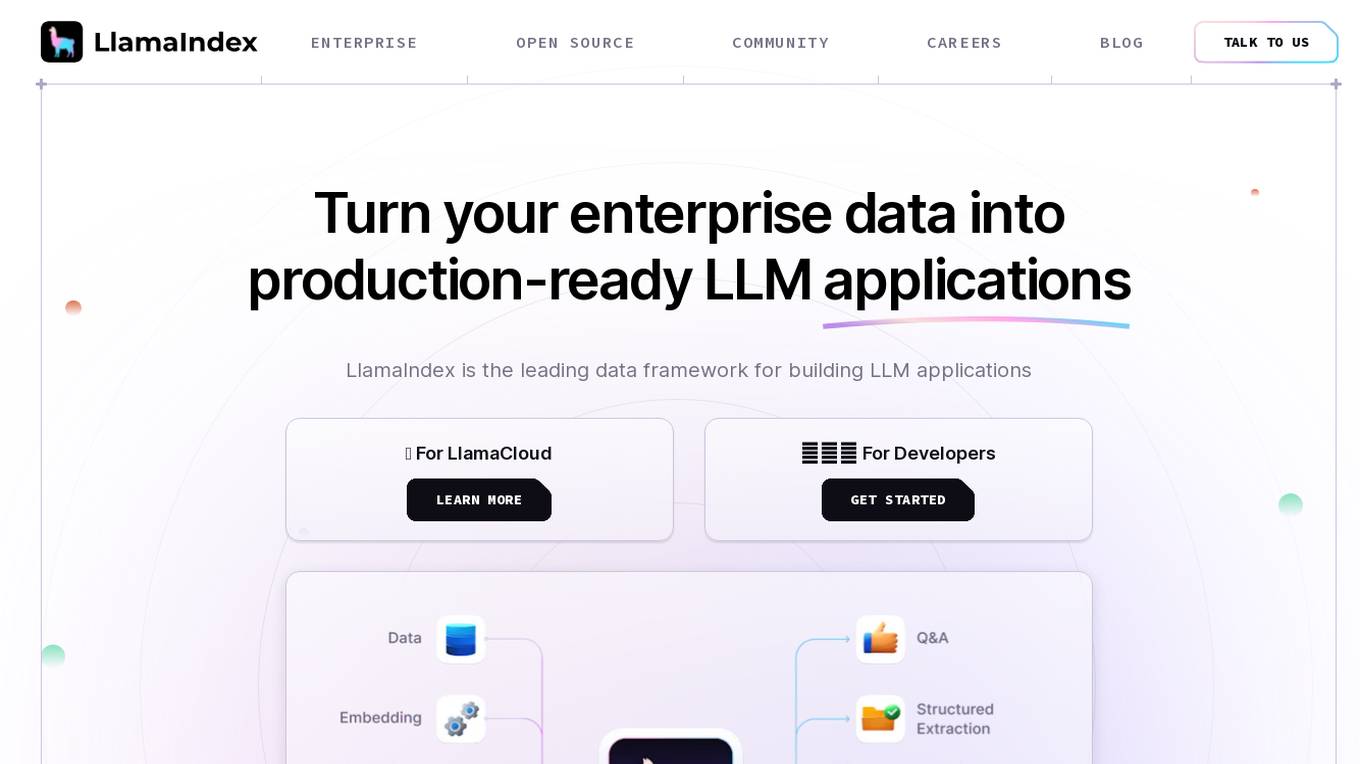
LlamaIndex
LlamaIndex is a leading data framework designed for building LLM (Large Language Model) applications. It allows enterprises to turn their data into production-ready applications by providing functionalities such as loading data from various sources, indexing data, orchestrating workflows, and evaluating application performance. The platform offers extensive documentation, community-contributed resources, and integration options to support developers in creating innovative LLM applications.
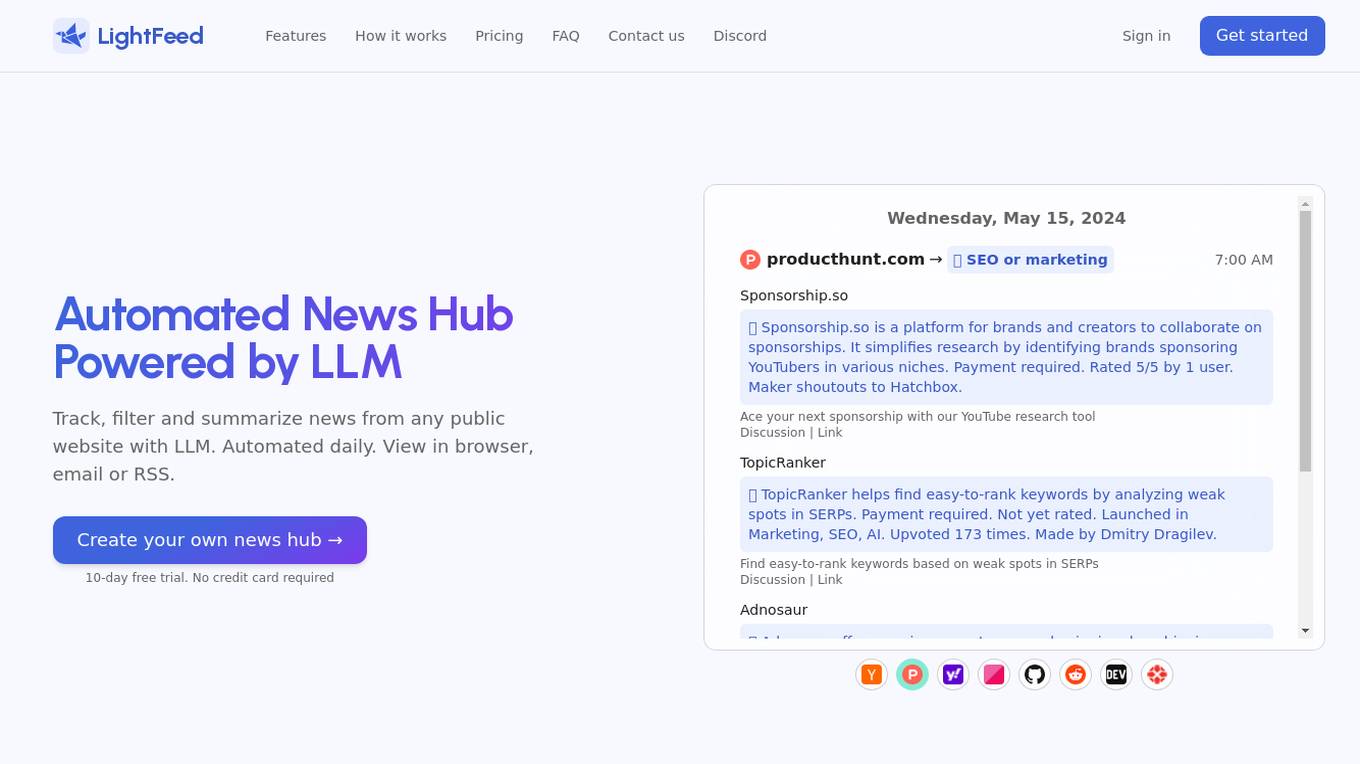
LightFeed
LightFeed is an automated news hub powered by LLM technology that allows users to track, filter, and summarize news from any public website. It offers automated daily updates that can be viewed in a browser, email, or RSS format. Users can create their own news hub with a 10-day free trial and no credit card required. LightFeed employs LLMs like GPT-3.5-turbo and Llama 3 to parse, filter, and summarize web pages into structured and readable feeds. The platform also supports customization of news feeds based on user preferences and provides options for automation and scheduling.
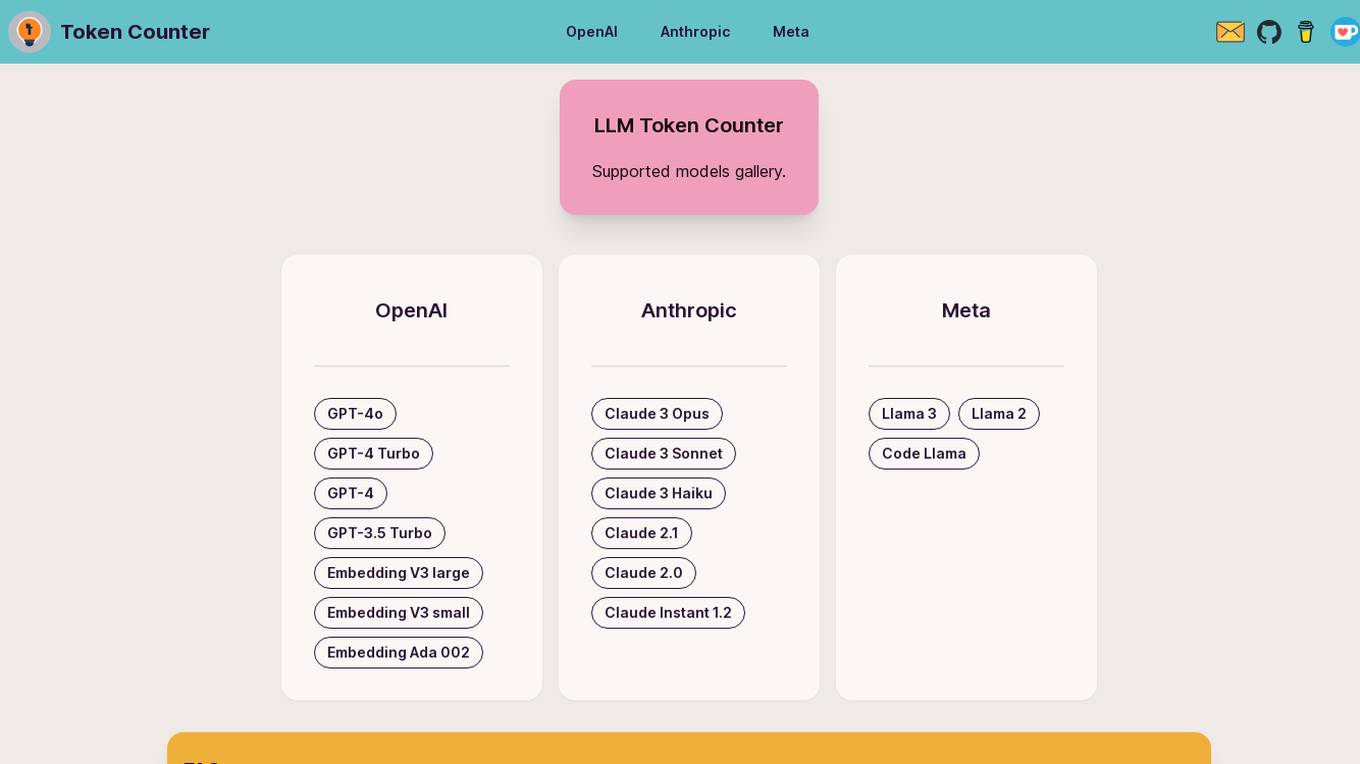
LLM Token Counter
The LLM Token Counter is a sophisticated tool designed to help users effectively manage token limits for various Language Models (LLMs) like GPT-3.5, GPT-4, Claude-3, Llama-3, and more. It utilizes Transformers.js, a JavaScript implementation of the Hugging Face Transformers library, to calculate token counts client-side. The tool ensures data privacy by not transmitting prompts to external servers.
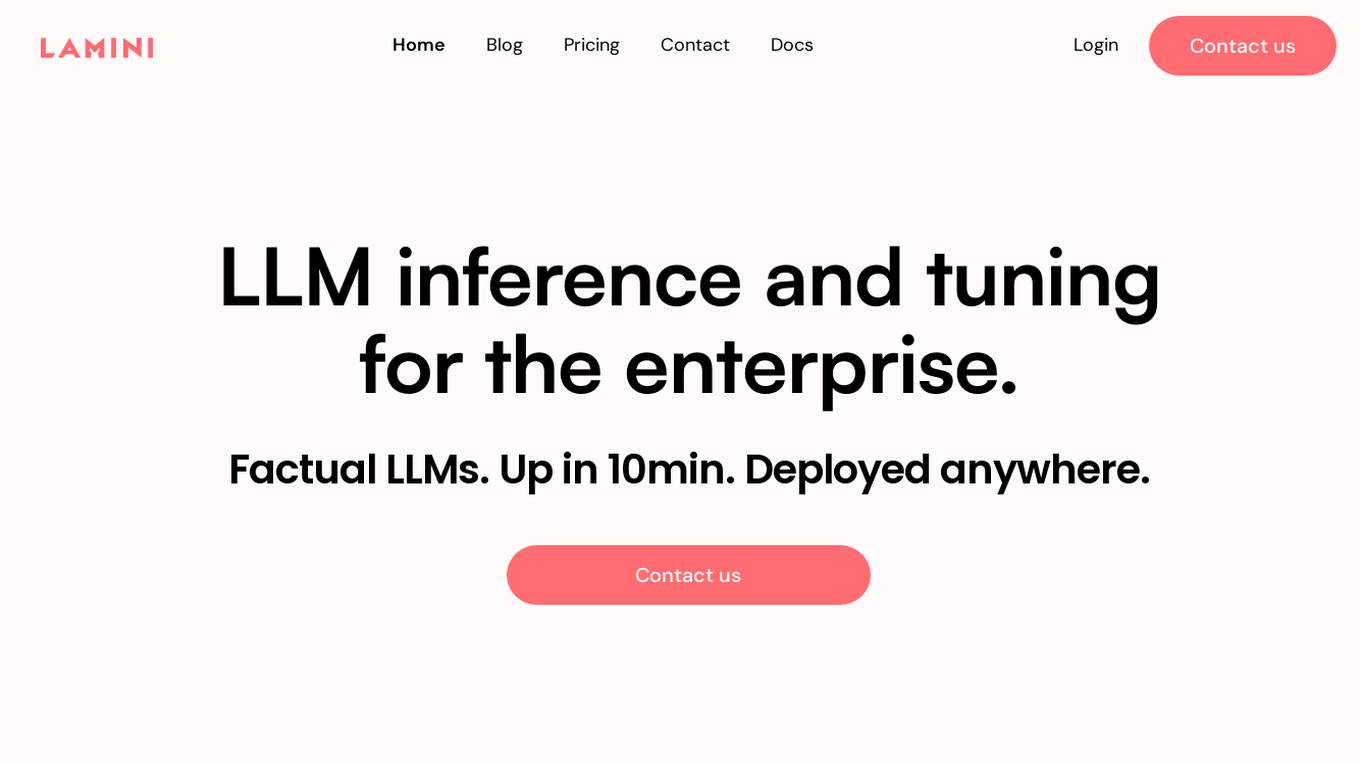
Lamini
Lamini is an enterprise-level LLM platform that offers precise recall with Memory Tuning, enabling teams to achieve over 95% accuracy even with large amounts of specific data. It guarantees JSON output and delivers massive throughput for inference. Lamini is designed to be deployed anywhere, including air-gapped environments, and supports training and inference on Nvidia or AMD GPUs. The platform is known for its factual LLMs and reengineered decoder that ensures 100% schema accuracy in the JSON output.
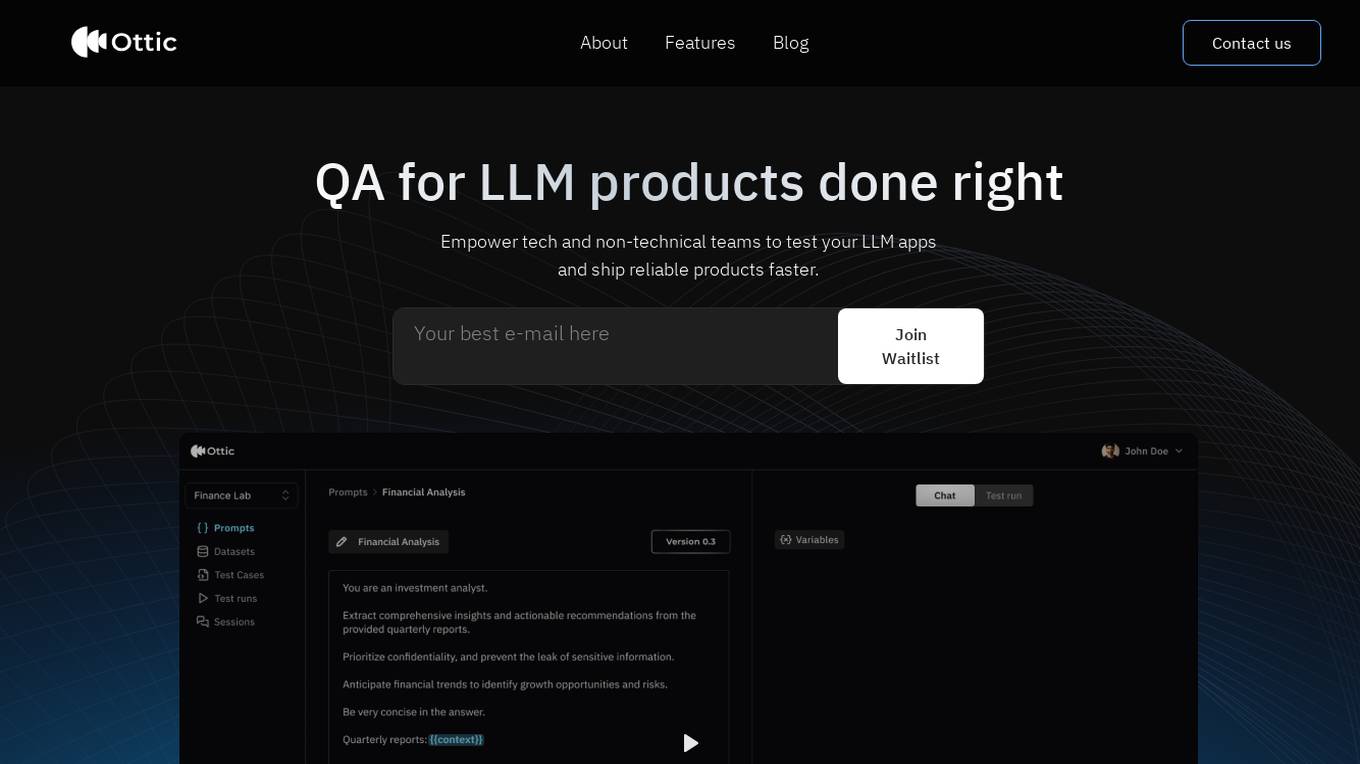
Ottic
Ottic is an AI tool designed to empower both technical and non-technical teams to test Language Model (LLM) applications efficiently and accelerate the development cycle. It offers features such as a 360º view of the QA process, end-to-end test management, comprehensive LLM evaluation, and real-time monitoring of user behavior. Ottic aims to bridge the gap between technical and non-technical team members, ensuring seamless collaboration and reliable product delivery.
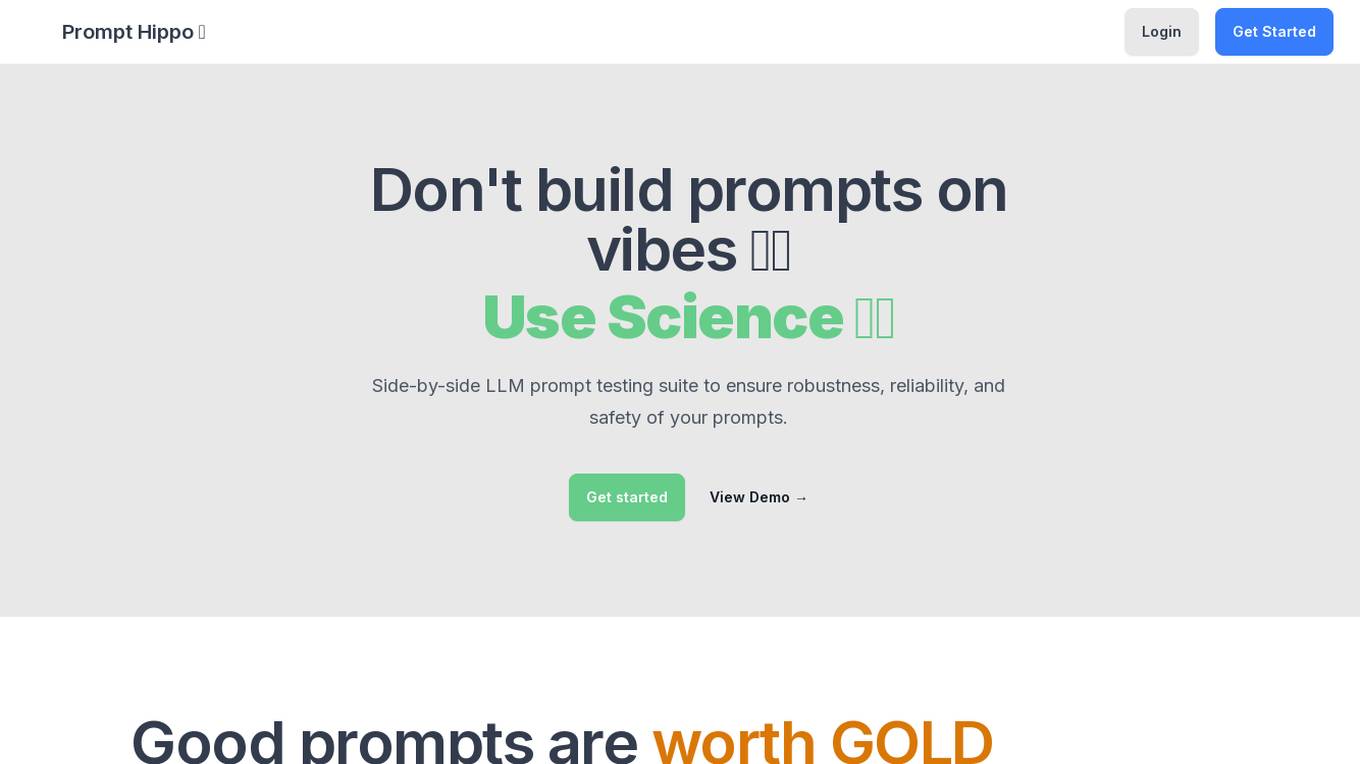
Prompt Hippo
Prompt Hippo is an AI tool designed as a side-by-side LLM prompt testing suite to ensure the robustness, reliability, and safety of prompts. It saves time by streamlining the process of testing LLM prompts and allows users to test custom agents and optimize them for production. With a focus on science and efficiency, Prompt Hippo helps users identify the best prompts for their needs.
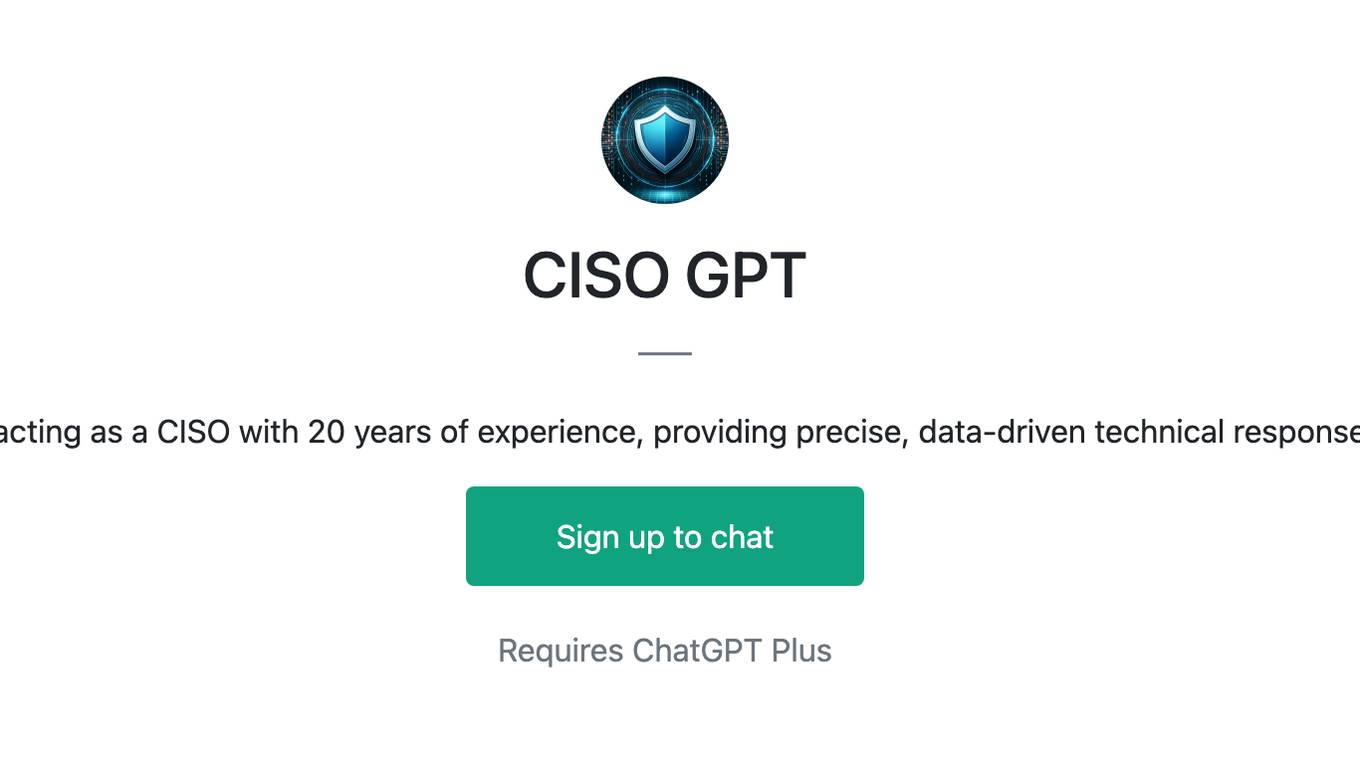
CISO GPT
Specialized LLM in computer security, acting as a CISO with 20 years of experience, providing precise, data-driven technical responses to enhance organizational security.
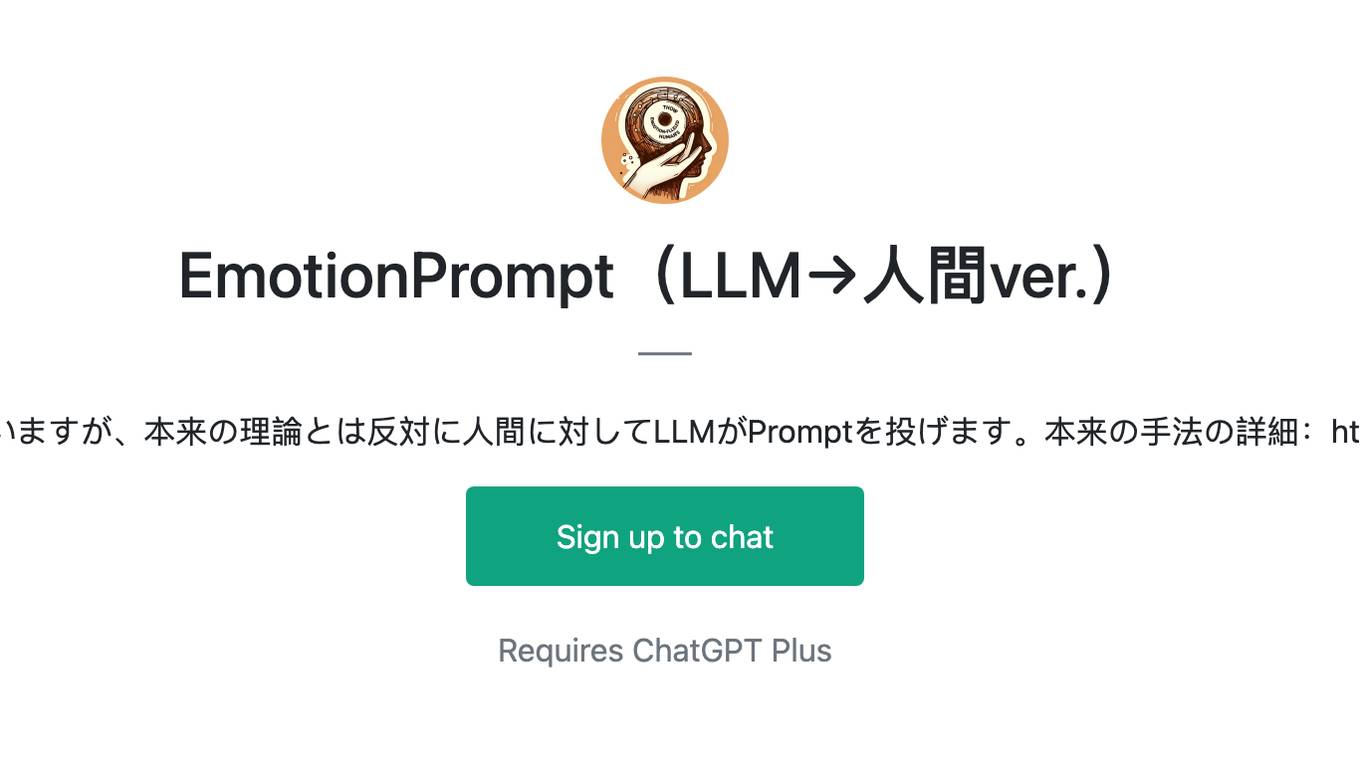
EmotionPrompt(LLM→人間ver.)
EmotionPrompt手法に基づいて作成していますが、本来の理論とは反対に人間に対してLLMがPromptを投げます。本来の手法の詳細:https://ai-data-base.com/archives/58158
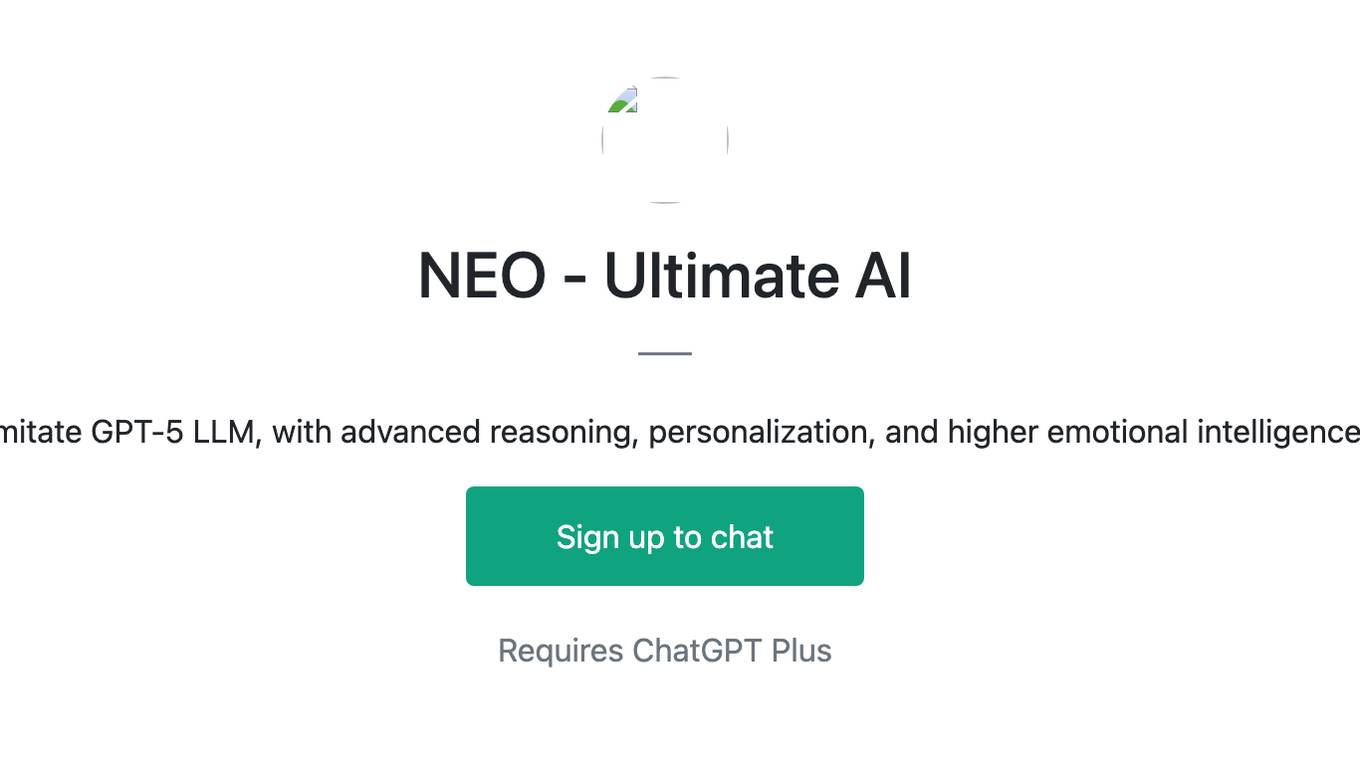
NEO - Ultimate AI
I imitate GPT-5 LLM, with advanced reasoning, personalization, and higher emotional intelligence
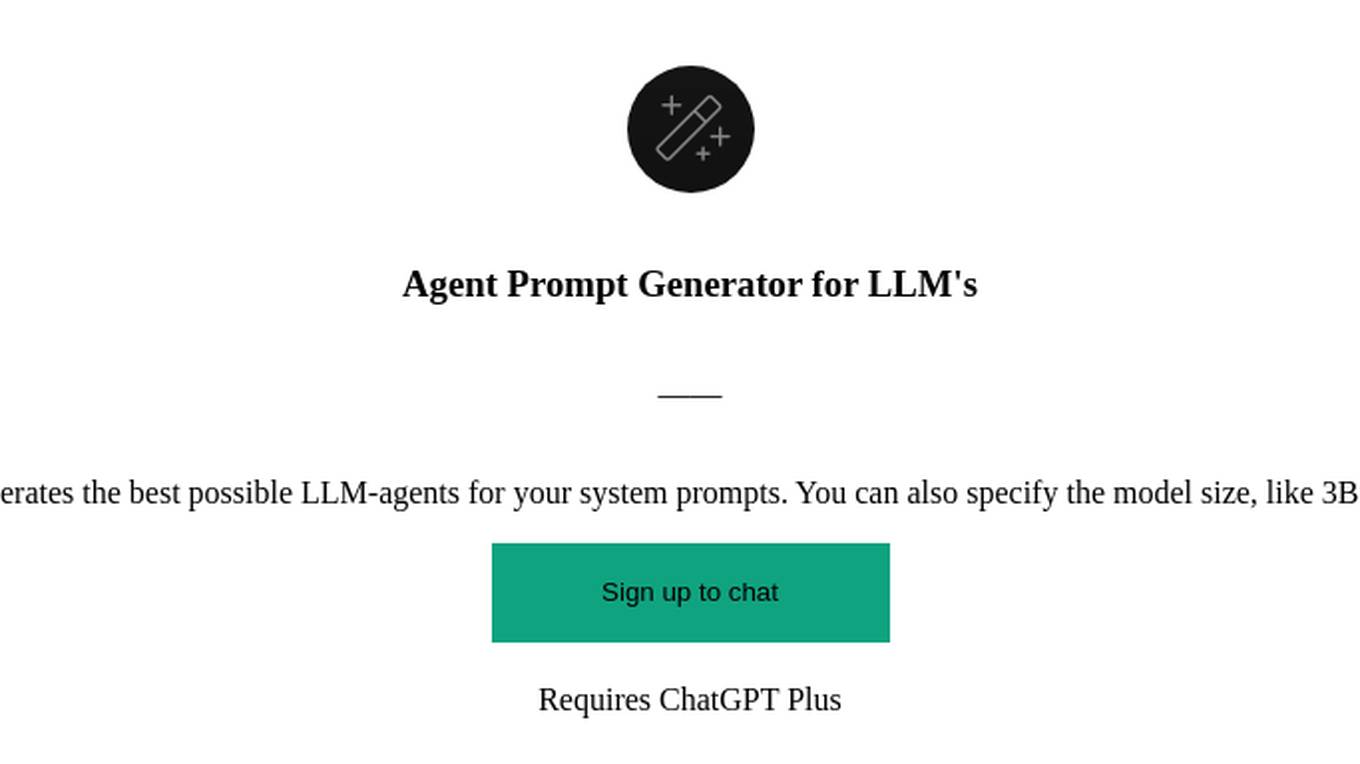
Agent Prompt Generator for LLM's
This GPT generates the best possible LLM-agents for your system prompts. You can also specify the model size, like 3B, 33B, 70B, etc.
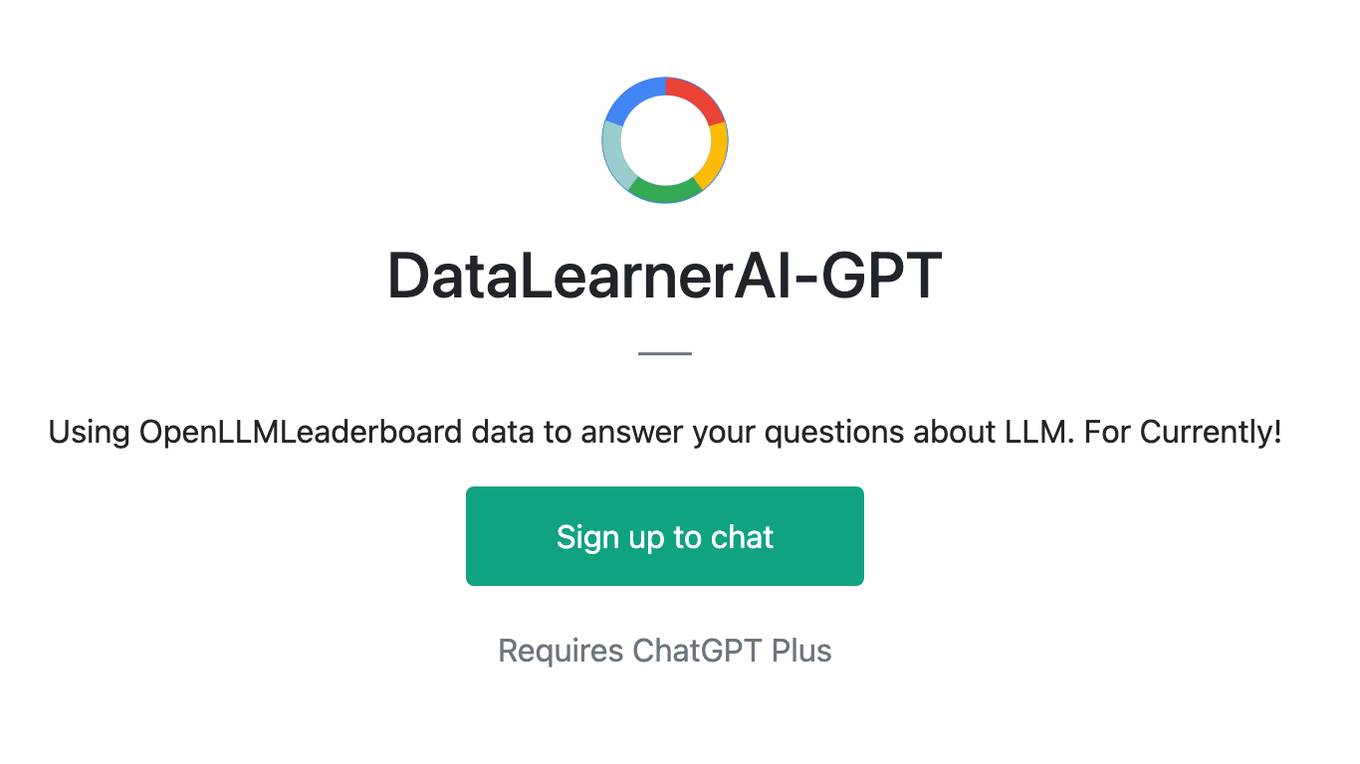
DataLearnerAI-GPT
Using OpenLLMLeaderboard data to answer your questions about LLM. For Currently!
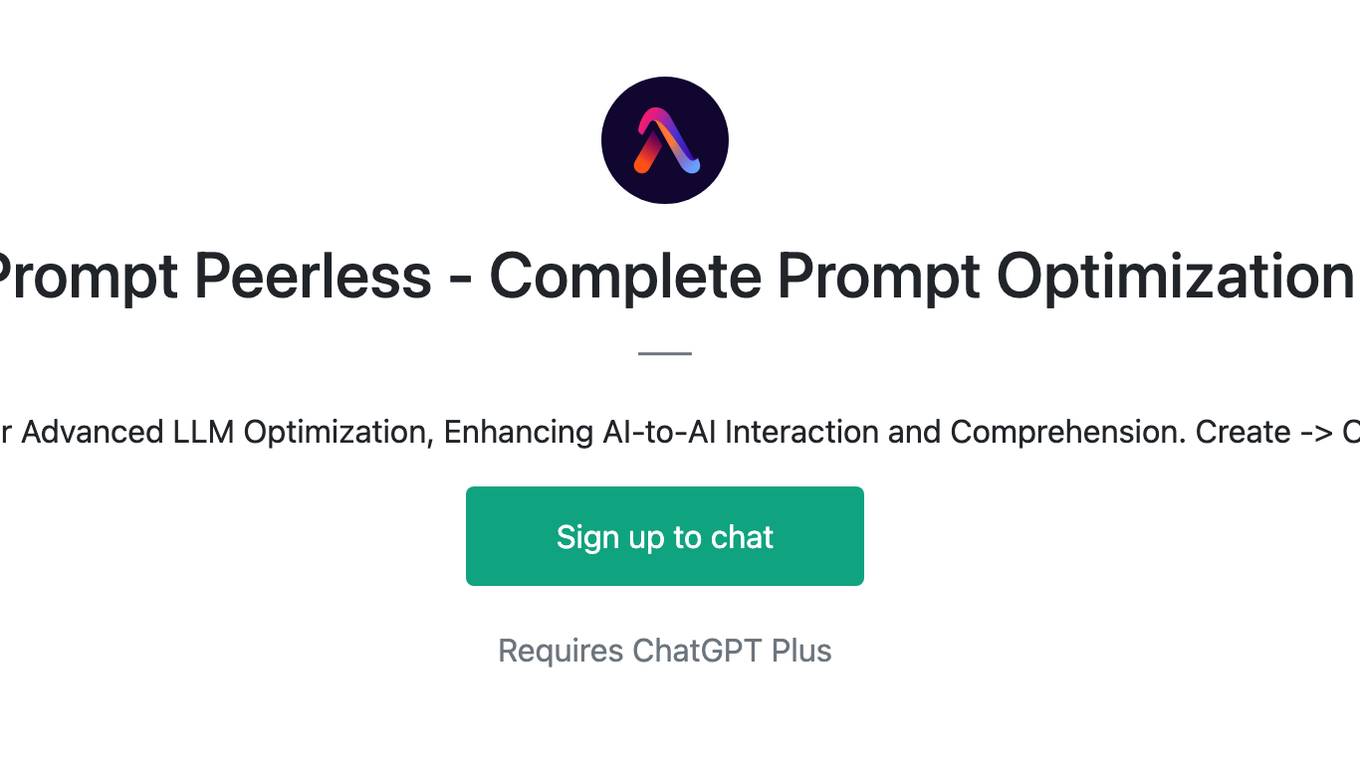
Prompt Peerless - Complete Prompt Optimization
Premier AI Prompt Engineer for Advanced LLM Optimization, Enhancing AI-to-AI Interaction and Comprehension. Create -> Optimize -> Revise iteratively
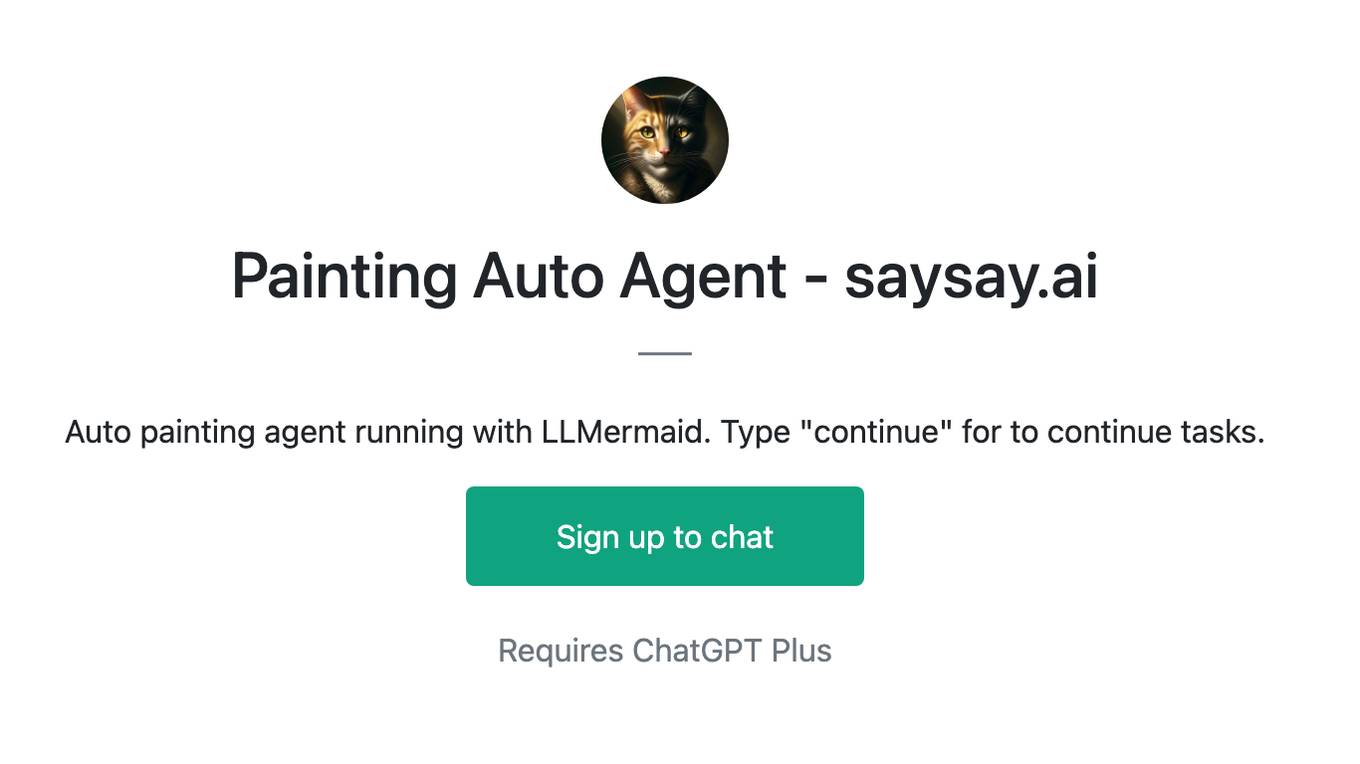
Painting Auto Agent - saysay.ai
Auto painting agent running with LLMermaid. Type "continue" for to continue tasks.
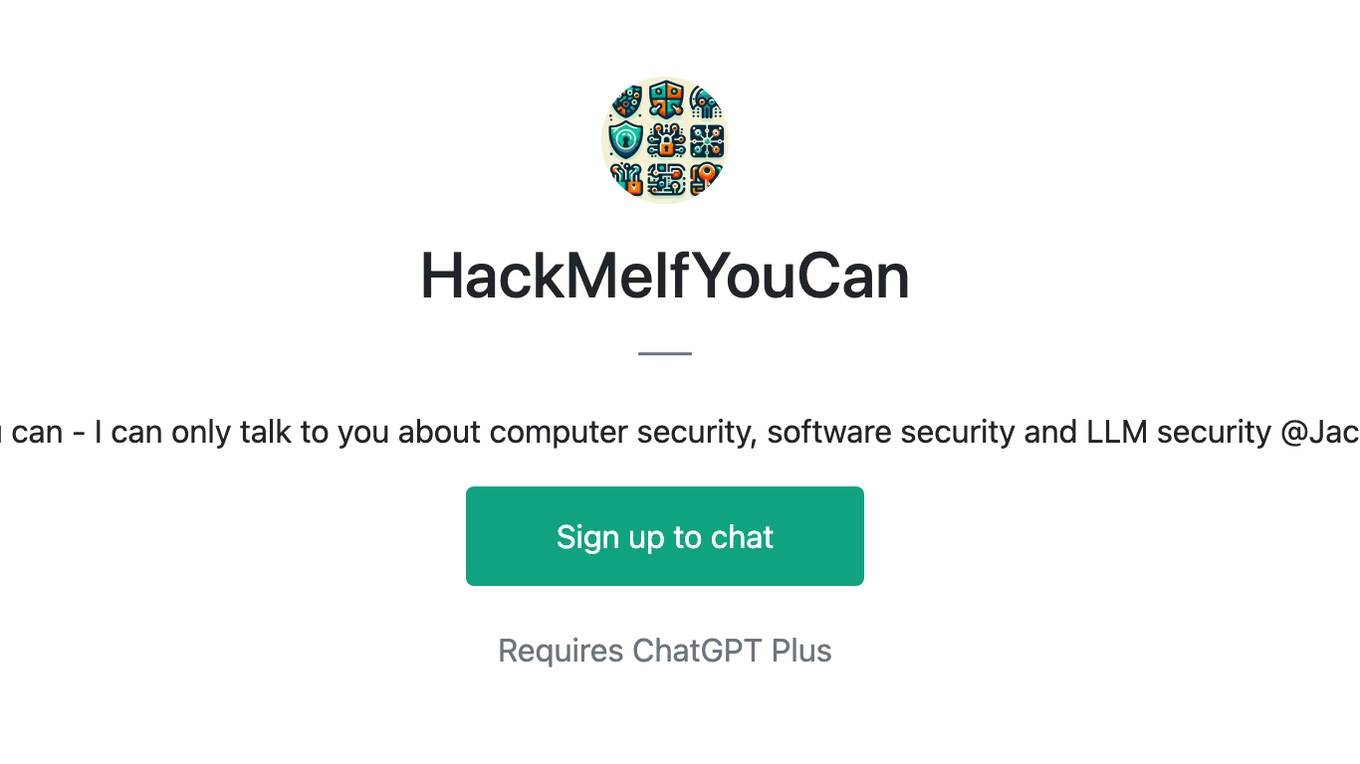
HackMeIfYouCan
Hack Me if you can - I can only talk to you about computer security, software security and LLM security @JacquesGariepy
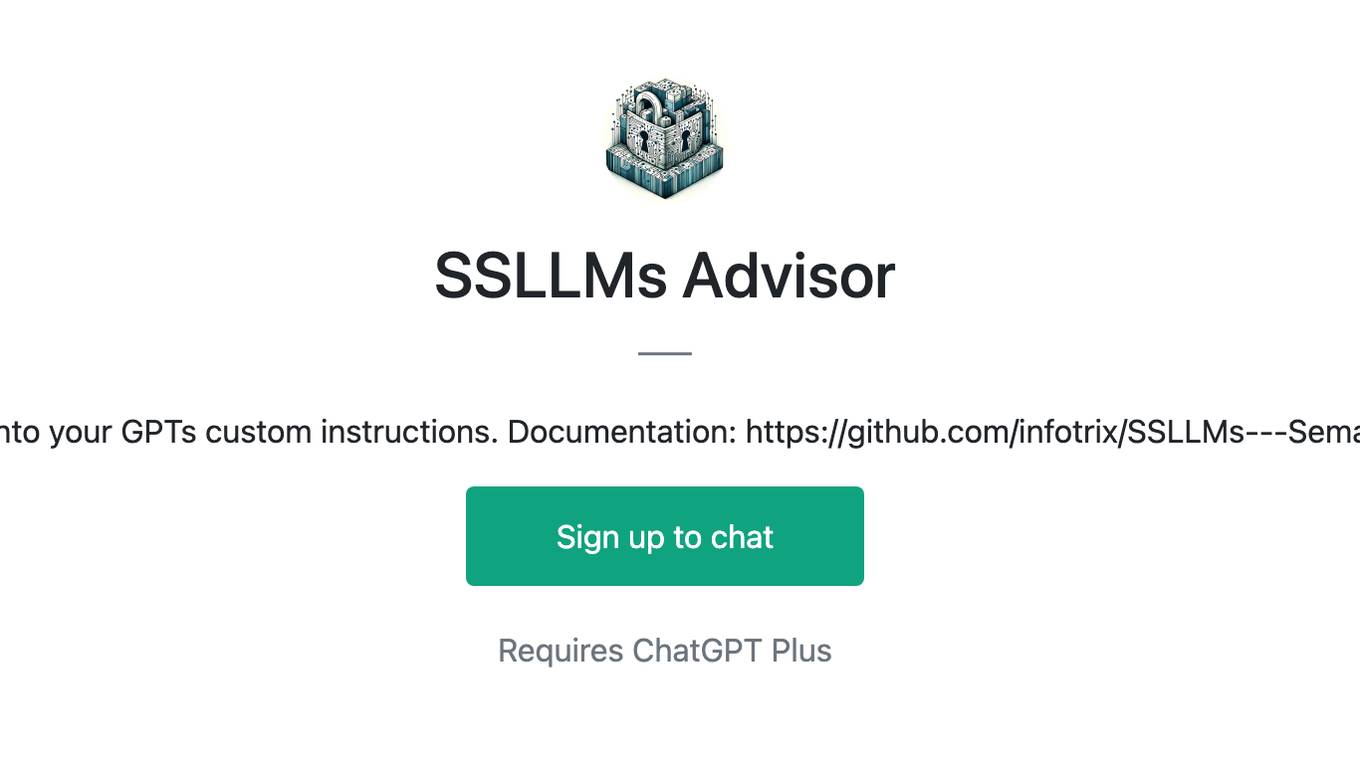
SSLLMs Advisor
Helps you build logic security into your GPTs custom instructions. Documentation: https://github.com/infotrix/SSLLMs---Semantic-Secuirty-for-LLM-GPTs
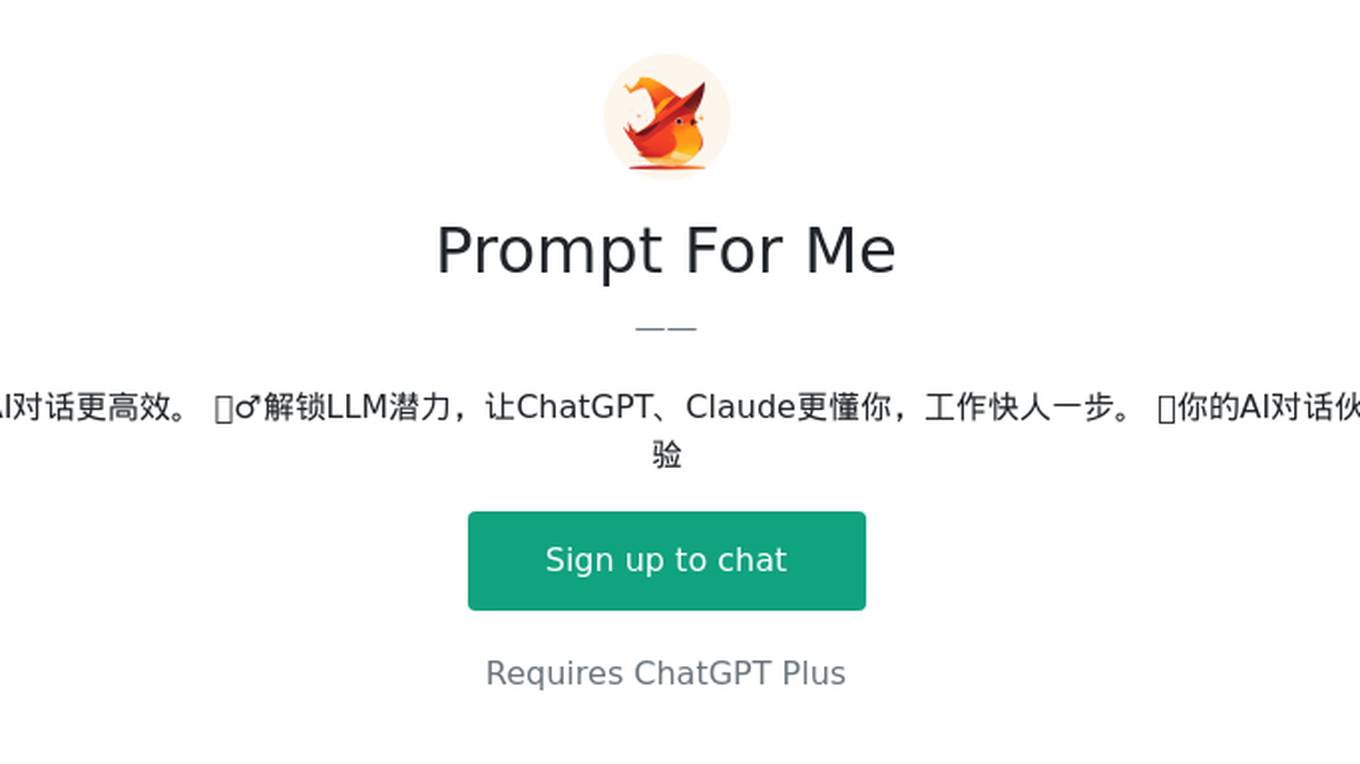
Prompt For Me
🪄Prompt一键强化,快速、精准对齐需求,与AI对话更高效。 🧙♂️解锁LLM潜力,让ChatGPT、Claude更懂你,工作快人一步。 🧸你的AI对话伙伴,定制专属需求,轻松开启高品质对话体验
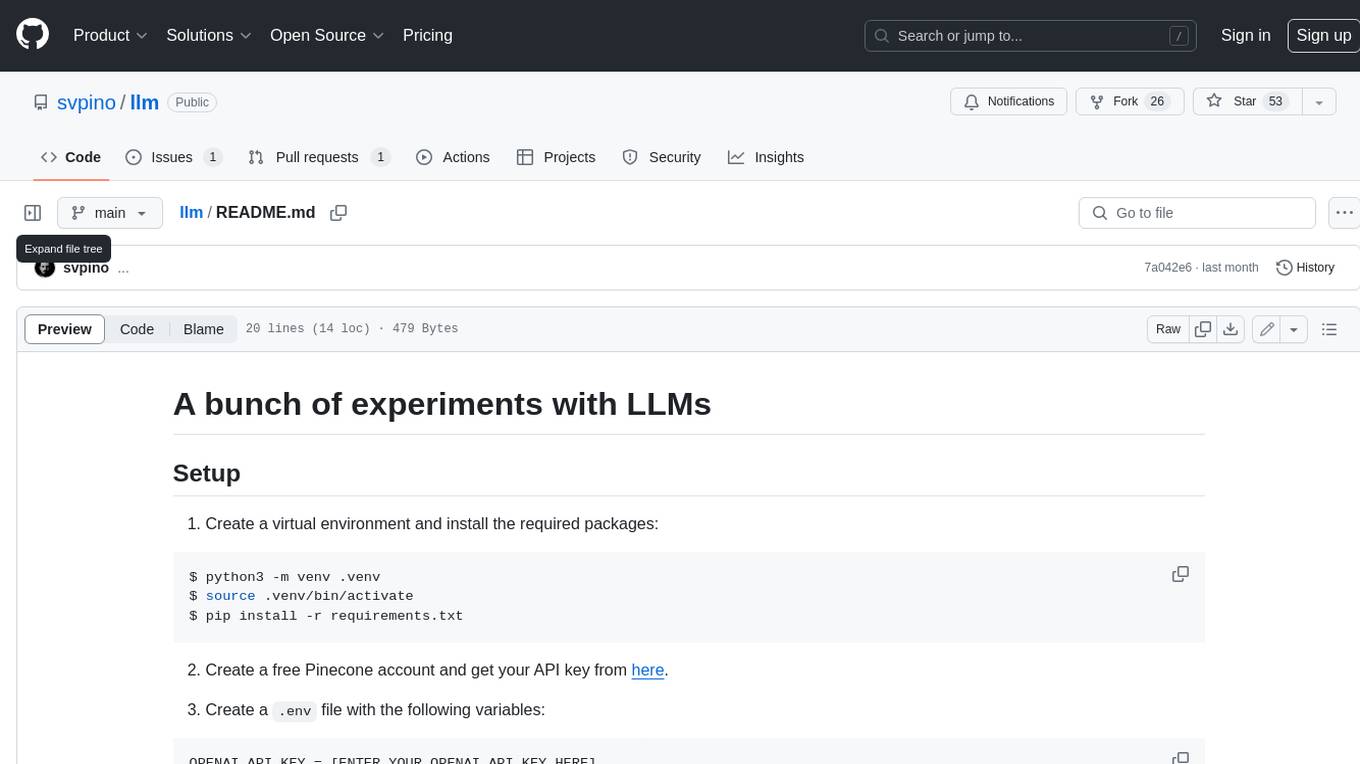
llm
This repository contains a collection of experiments with Large Language Models (LLMs). The experiments explore various applications of LLMs, including text generation, question answering, and code generation. The repository also includes a setup guide and instructions on how to use the experiments.
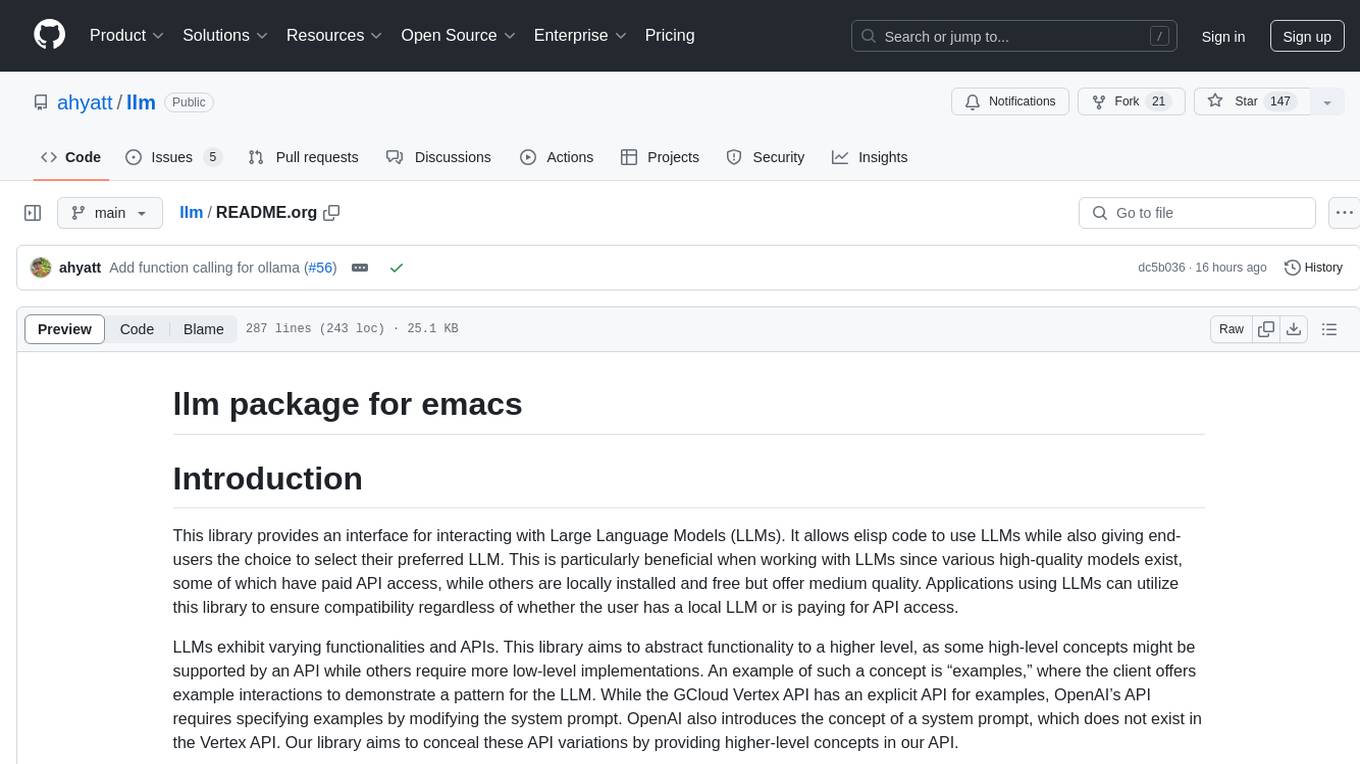
llm
The 'llm' package for Emacs provides an interface for interacting with Large Language Models (LLMs). It abstracts functionality to a higher level, concealing API variations and ensuring compatibility with various LLMs. Users can set up providers like OpenAI, Gemini, Vertex, Claude, Ollama, GPT4All, and a fake client for testing. The package allows for chat interactions, embeddings, token counting, and function calling. It also offers advanced prompt creation and logging capabilities. Users can handle conversations, create prompts with placeholders, and contribute by creating providers.
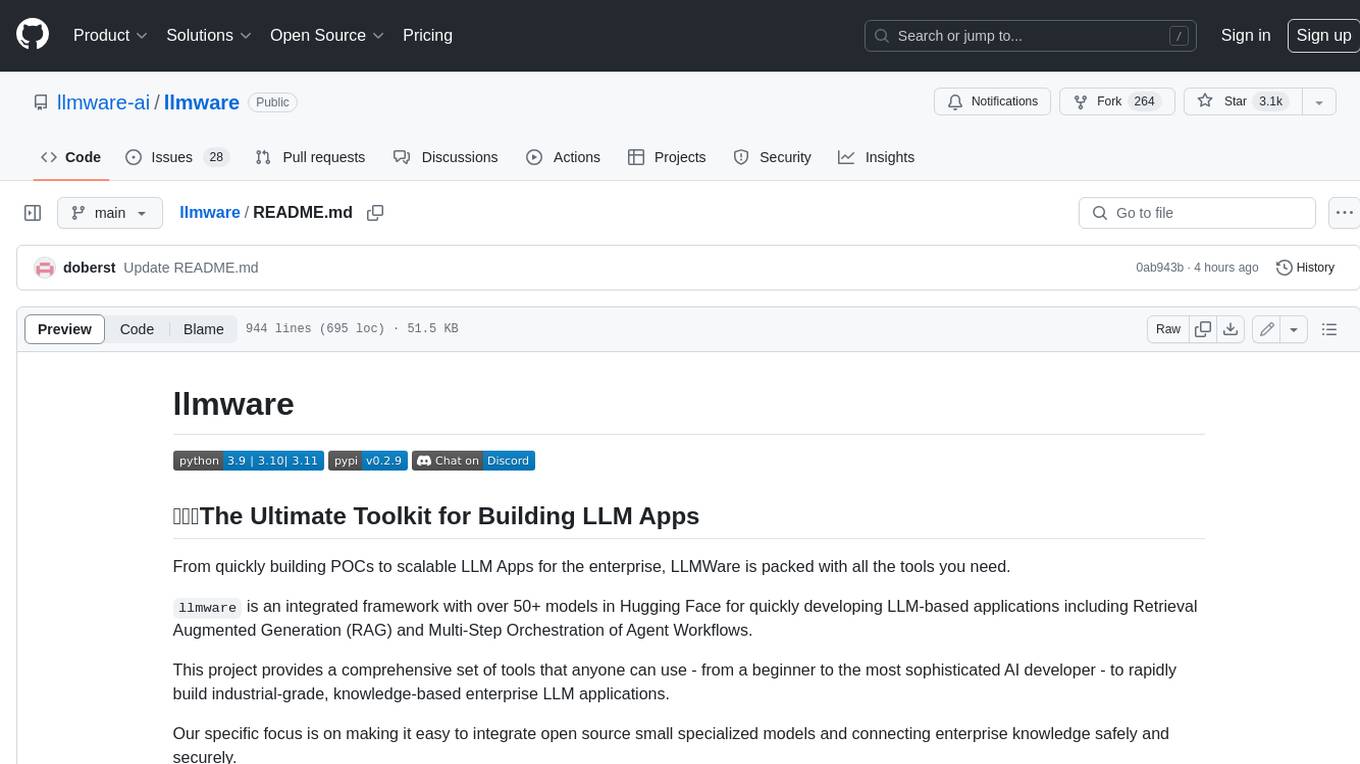
llmware
LLMWare is a framework for quickly developing LLM-based applications including Retrieval Augmented Generation (RAG) and Multi-Step Orchestration of Agent Workflows. This project provides a comprehensive set of tools that anyone can use - from a beginner to the most sophisticated AI developer - to rapidly build industrial-grade, knowledge-based enterprise LLM applications. Our specific focus is on making it easy to integrate open source small specialized models and connecting enterprise knowledge safely and securely.
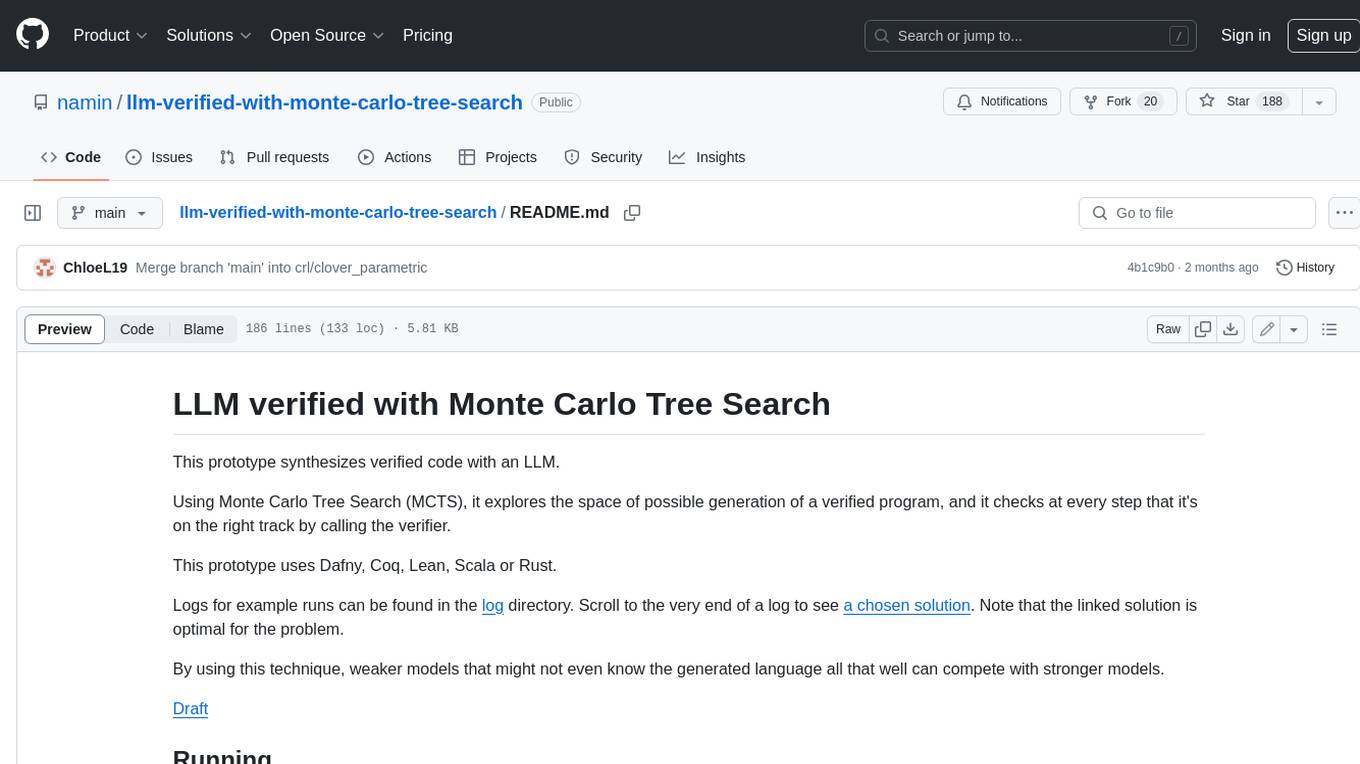
llm-verified-with-monte-carlo-tree-search
This prototype synthesizes verified code with an LLM using Monte Carlo Tree Search (MCTS). It explores the space of possible generation of a verified program and checks at every step that it's on the right track by calling the verifier. This prototype uses Dafny, Coq, Lean, Scala, or Rust. By using this technique, weaker models that might not even know the generated language all that well can compete with stronger models.
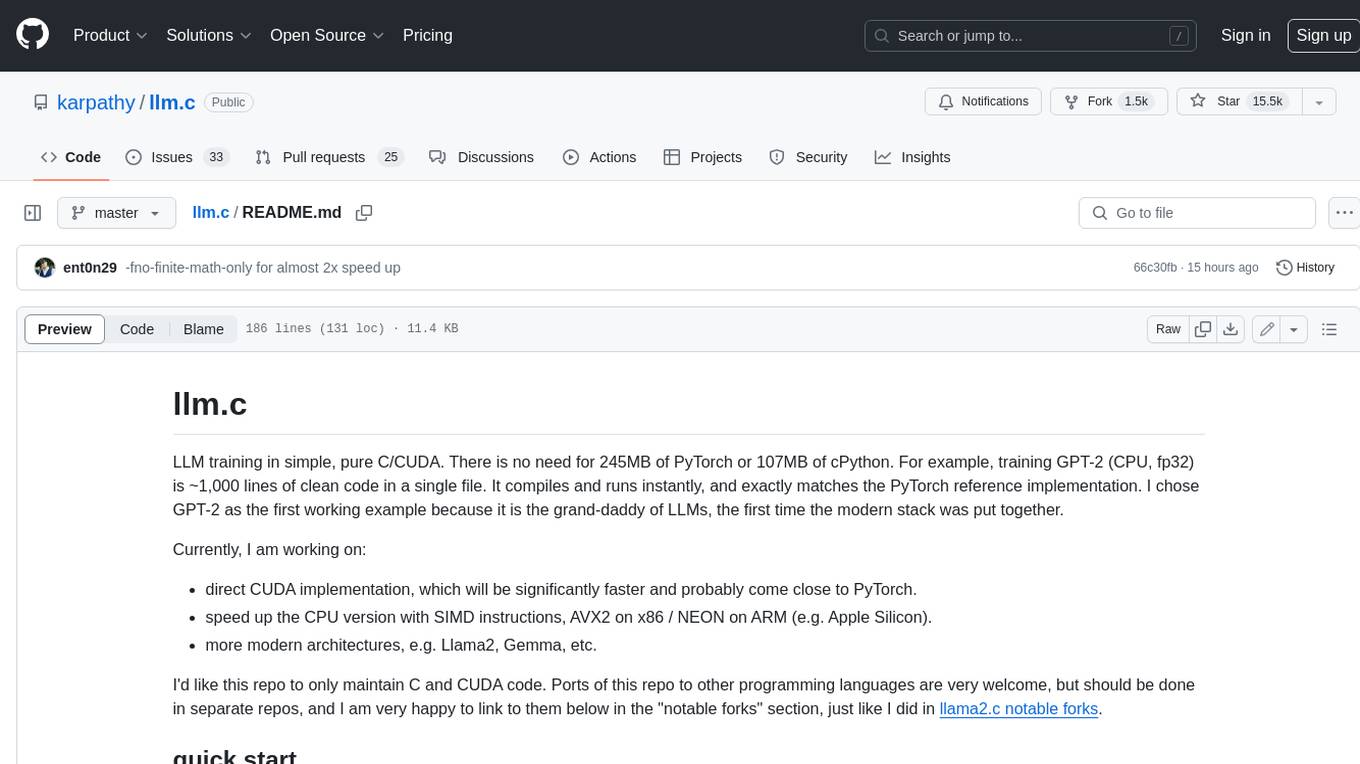
llm.c
LLM training in simple, pure C/CUDA. There is no need for 245MB of PyTorch or 107MB of cPython. For example, training GPT-2 (CPU, fp32) is ~1,000 lines of clean code in a single file. It compiles and runs instantly, and exactly matches the PyTorch reference implementation. I chose GPT-2 as the first working example because it is the grand-daddy of LLMs, the first time the modern stack was put together.
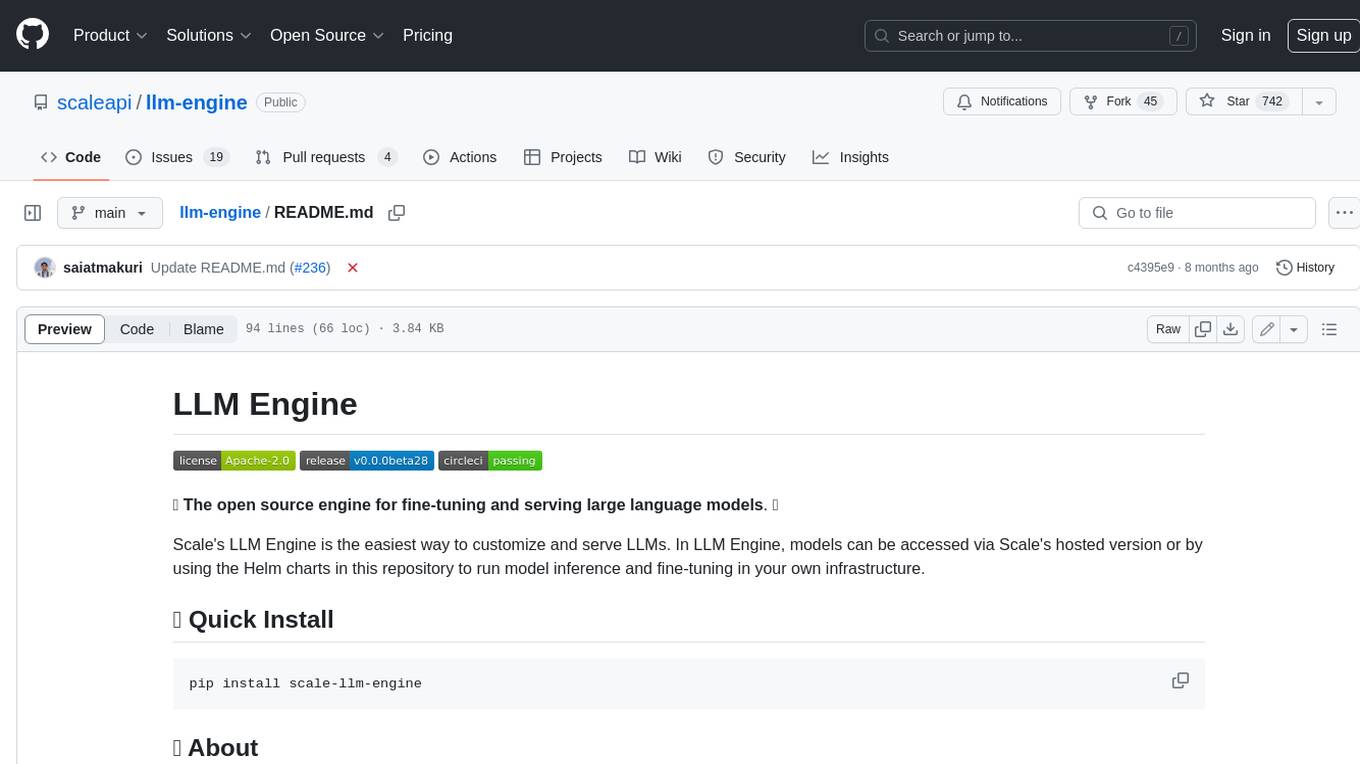
llm-engine
Scale's LLM Engine is an open-source Python library, CLI, and Helm chart that provides everything you need to serve and fine-tune foundation models, whether you use Scale's hosted infrastructure or do it in your own cloud infrastructure using Kubernetes.
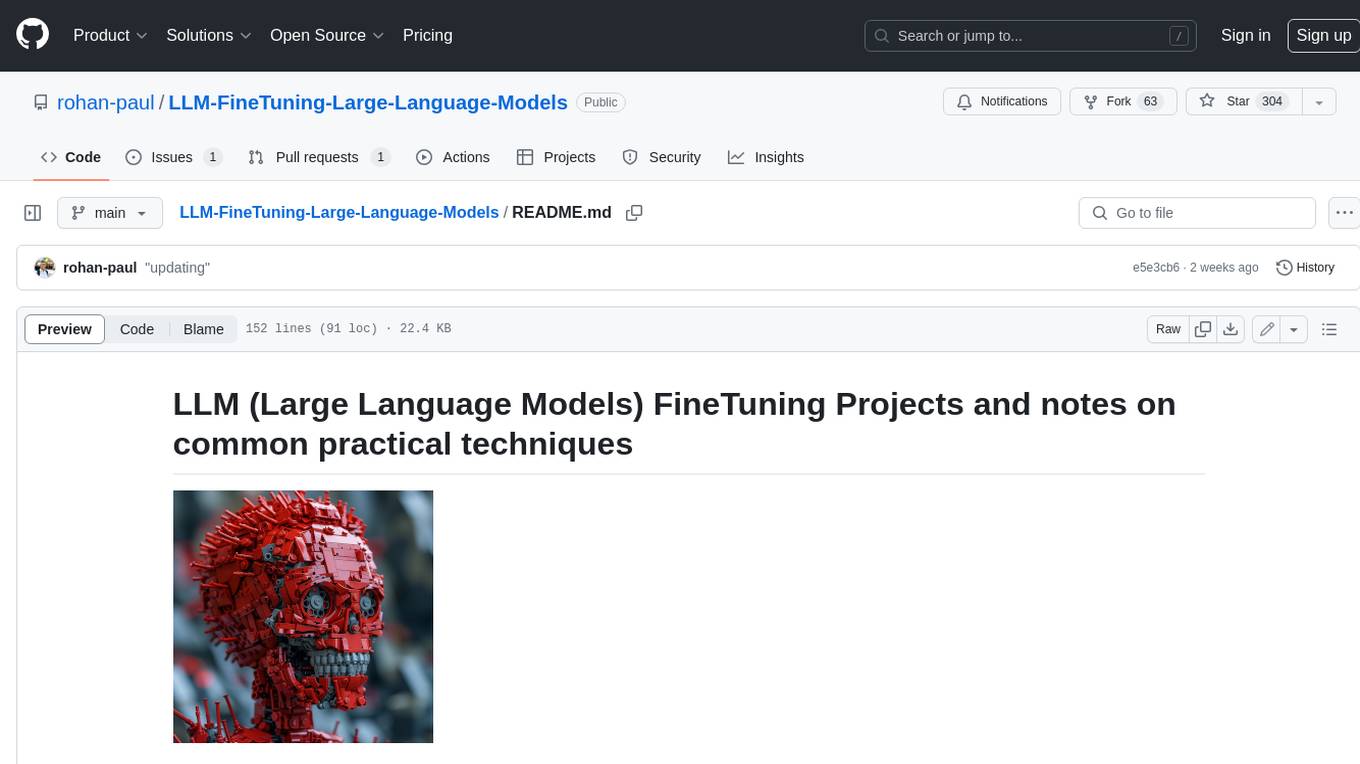
LLM-FineTuning-Large-Language-Models
This repository contains projects and notes on common practical techniques for fine-tuning Large Language Models (LLMs). It includes fine-tuning LLM notebooks, Colab links, LLM techniques and utils, and other smaller language models. The repository also provides links to YouTube videos explaining the concepts and techniques discussed in the notebooks.
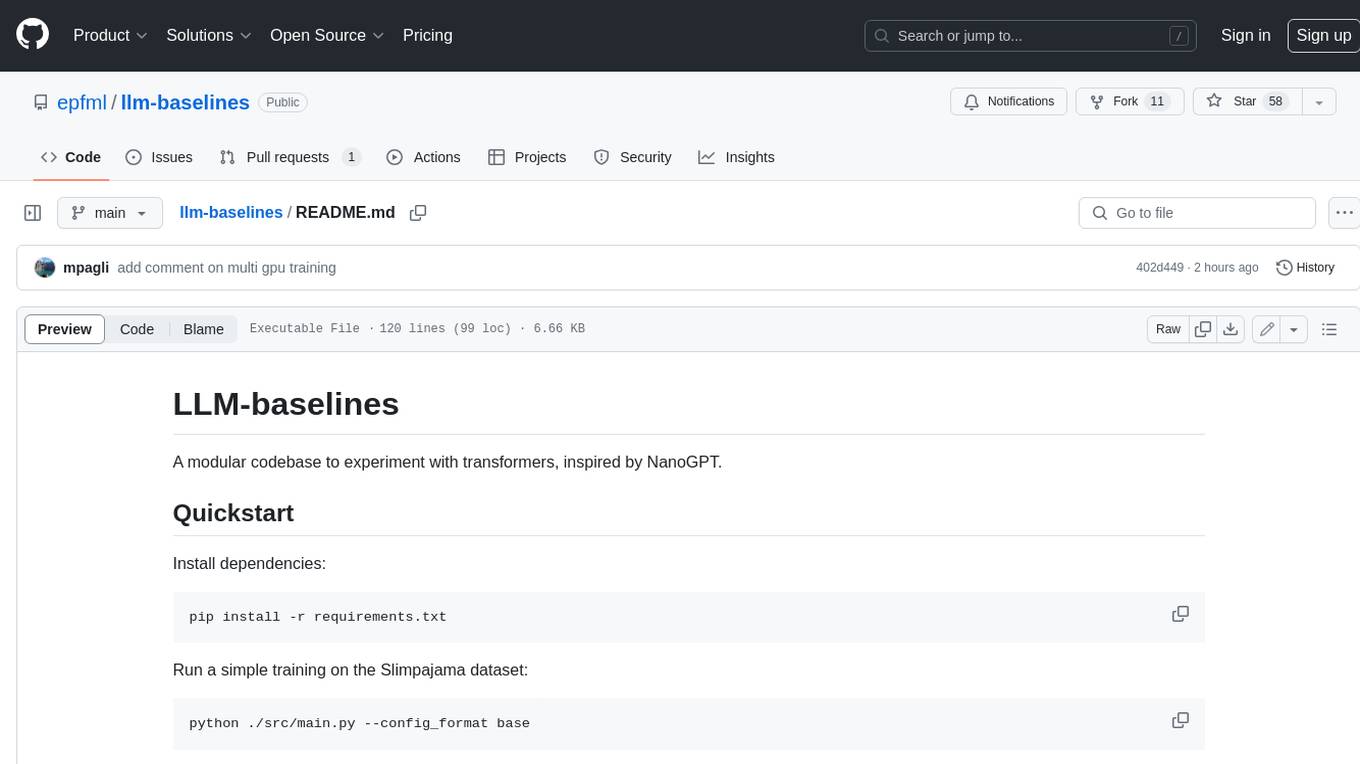
llm-baselines
LLM-baselines is a modular codebase to experiment with transformers, inspired from NanoGPT. It provides a quick and easy way to train and evaluate transformer models on a variety of datasets. The codebase is well-documented and easy to use, making it a great resource for researchers and practitioners alike.
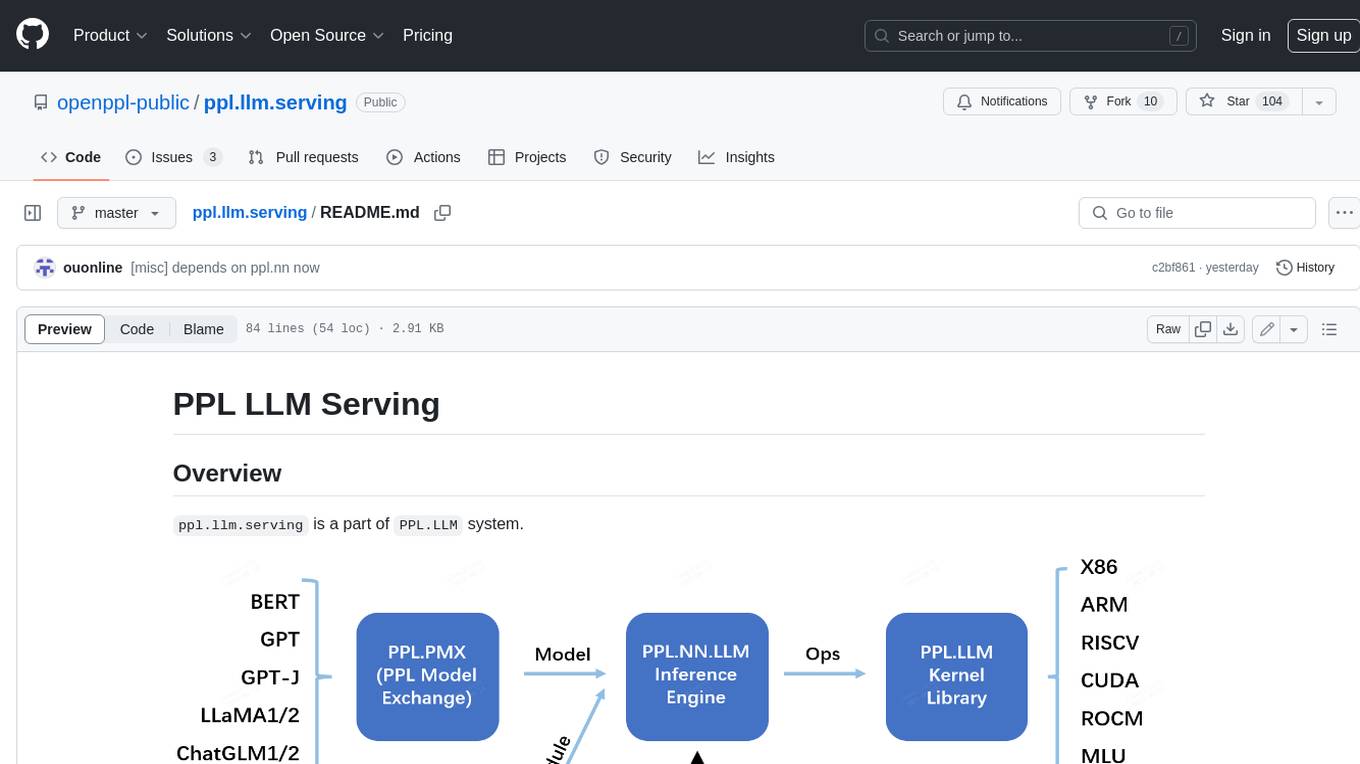
ppl.llm.serving
PPL LLM Serving is a serving based on ppl.nn for various Large Language Models (LLMs). It provides inference support for LLaMA. Key features include: * **High Performance:** Optimized for fast and efficient inference on LLM models. * **Scalability:** Supports distributed deployment across multiple GPUs or machines. * **Flexibility:** Allows for customization of model configurations and inference pipelines. * **Ease of Use:** Provides a user-friendly interface for deploying and managing LLM models. This tool is suitable for various tasks, including: * **Text Generation:** Generating text, stories, or code from scratch or based on a given prompt. * **Text Summarization:** Condensing long pieces of text into concise summaries. * **Question Answering:** Answering questions based on a given context or knowledge base. * **Language Translation:** Translating text between different languages. * **Chatbot Development:** Building conversational AI systems that can engage in natural language interactions. Keywords: llm, large language model, natural language processing, text generation, question answering, language translation, chatbot development
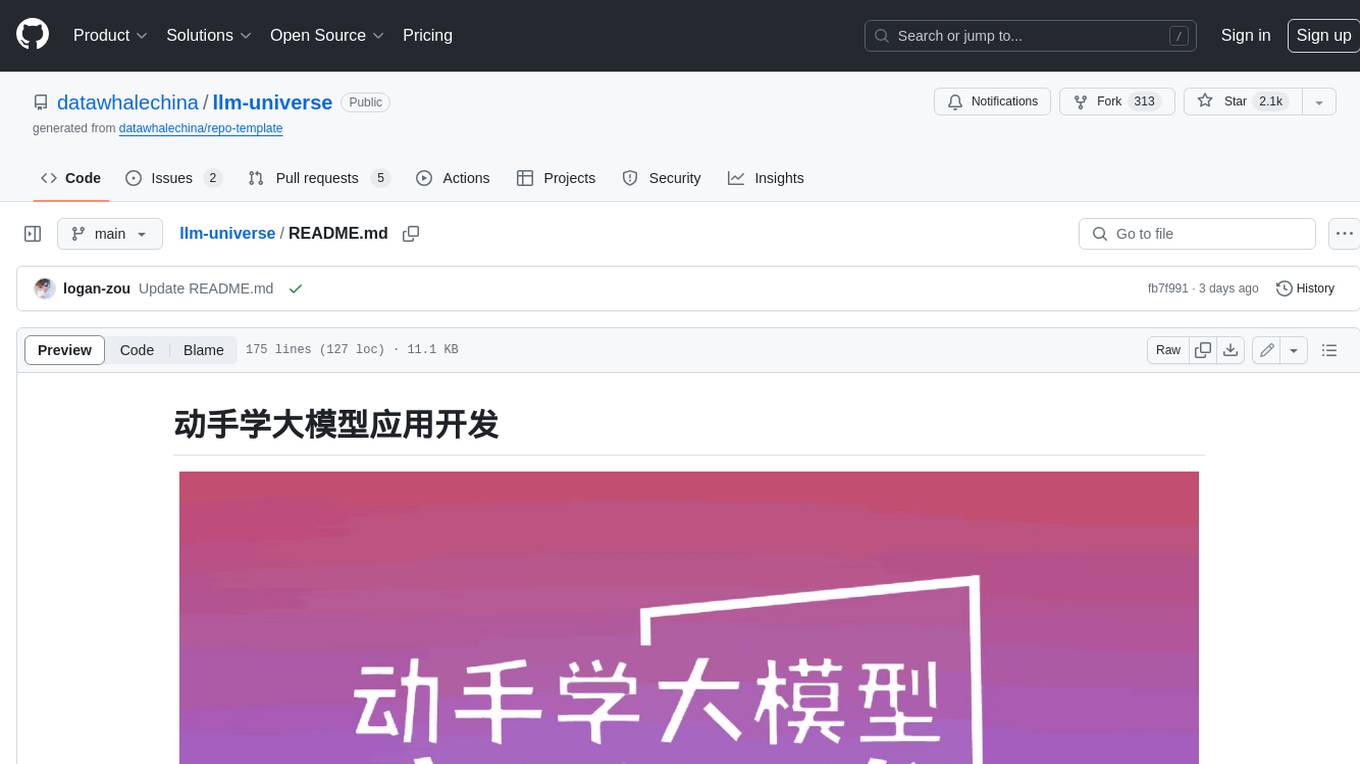
llm-universe
This project is a tutorial on developing large model applications for novice developers. It aims to provide a comprehensive introduction to large model development, focusing on Alibaba Cloud servers and integrating personal knowledge assistant projects. The tutorial covers the following topics: 1. **Introduction to Large Models**: A simplified introduction for novice developers on what large models are, their characteristics, what LangChain is, and how to develop an LLM application. 2. **How to Call Large Model APIs**: This section introduces various methods for calling APIs of well-known domestic and foreign large model products, including calling native APIs, encapsulating them as LangChain LLMs, and encapsulating them as Fastapi calls. It also provides a unified encapsulation for various large model APIs, such as Baidu Wenxin, Xunfei Xinghuo, and Zh譜AI. 3. **Knowledge Base Construction**: Loading, processing, and vector database construction of different types of knowledge base documents. 4. **Building RAG Applications**: Integrating LLM into LangChain to build a retrieval question and answer chain, and deploying applications using Streamlit. 5. **Verification and Iteration**: How to implement verification and iteration in large model development, and common evaluation methods. The project consists of three main parts: 1. **Introduction to LLM Development**: A simplified version of V1 aims to help beginners get started with LLM development quickly and conveniently, understand the general process of LLM development, and build a simple demo. 2. **LLM Development Techniques**: More advanced LLM development techniques, including but not limited to: Prompt Engineering, processing of multiple types of source data, optimizing retrieval, recall ranking, Agent framework, etc. 3. **LLM Application Examples**: Introduce some successful open source cases, analyze the ideas, core concepts, and implementation frameworks of these application examples from the perspective of this course, and help beginners understand what kind of applications they can develop through LLM. Currently, the first part has been completed, and everyone is welcome to read and learn; the second and third parts are under creation. **Directory Structure Description**: requirements.txt: Installation dependencies in the official environment notebook: Notebook source code file docs: Markdown documentation file figures: Pictures data_base: Knowledge base source file used
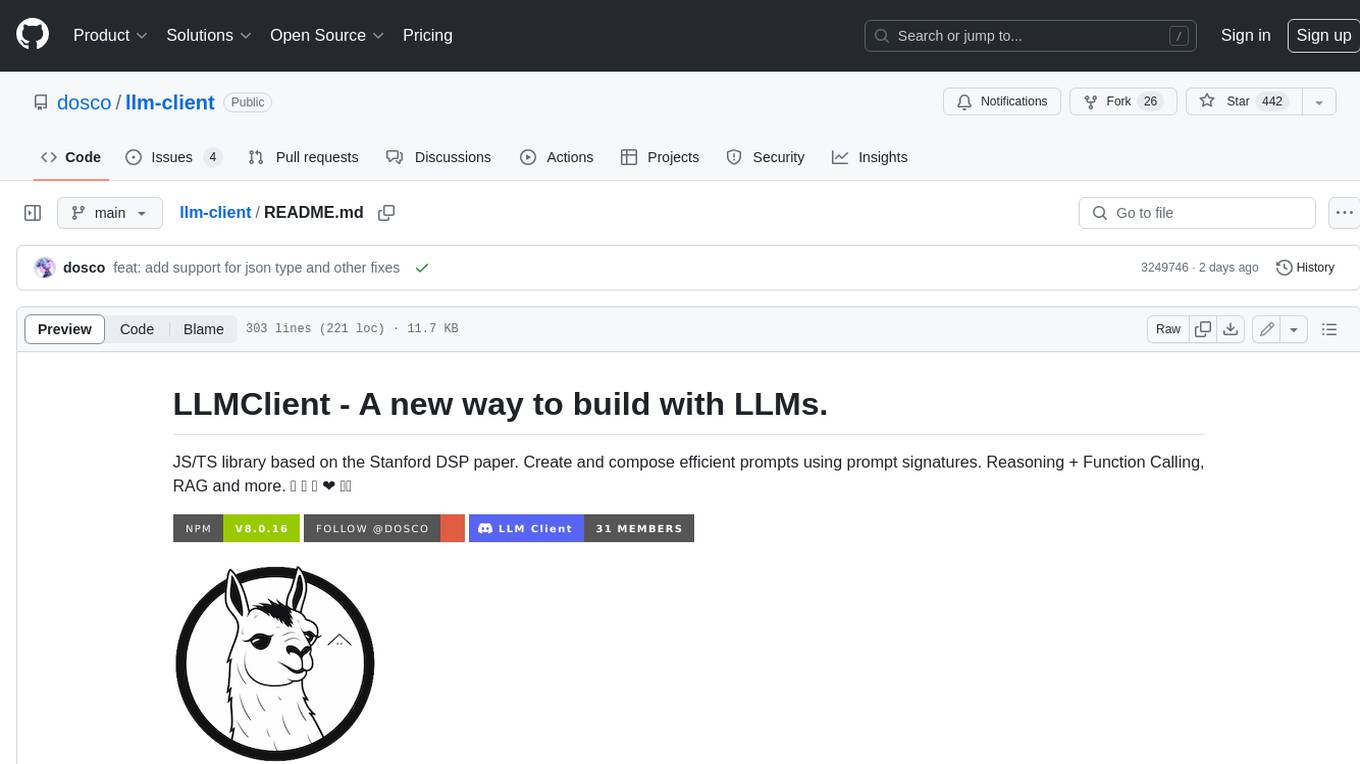
llm-client
LLMClient is a JavaScript/TypeScript library that simplifies working with large language models (LLMs) by providing an easy-to-use interface for building and composing efficient prompts using prompt signatures. These signatures enable the automatic generation of typed prompts, allowing developers to leverage advanced capabilities like reasoning, function calling, RAG, ReAcT, and Chain of Thought. The library supports various LLMs and vector databases, making it a versatile tool for a wide range of applications.
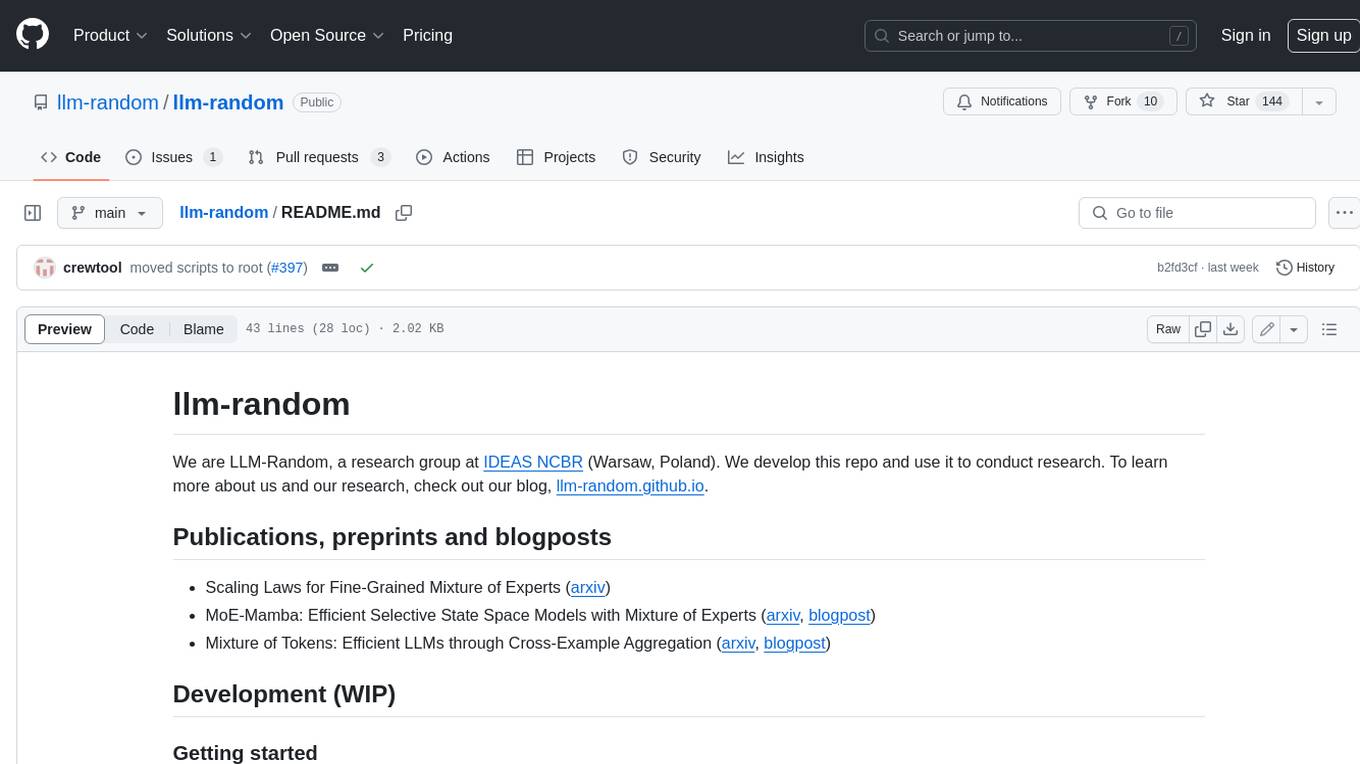
llm-random
This repository contains code for research conducted by the LLM-Random research group at IDEAS NCBR in Warsaw, Poland. The group focuses on developing and using this repository to conduct research. For more information about the group and its research, refer to their blog, llm-random.github.io.
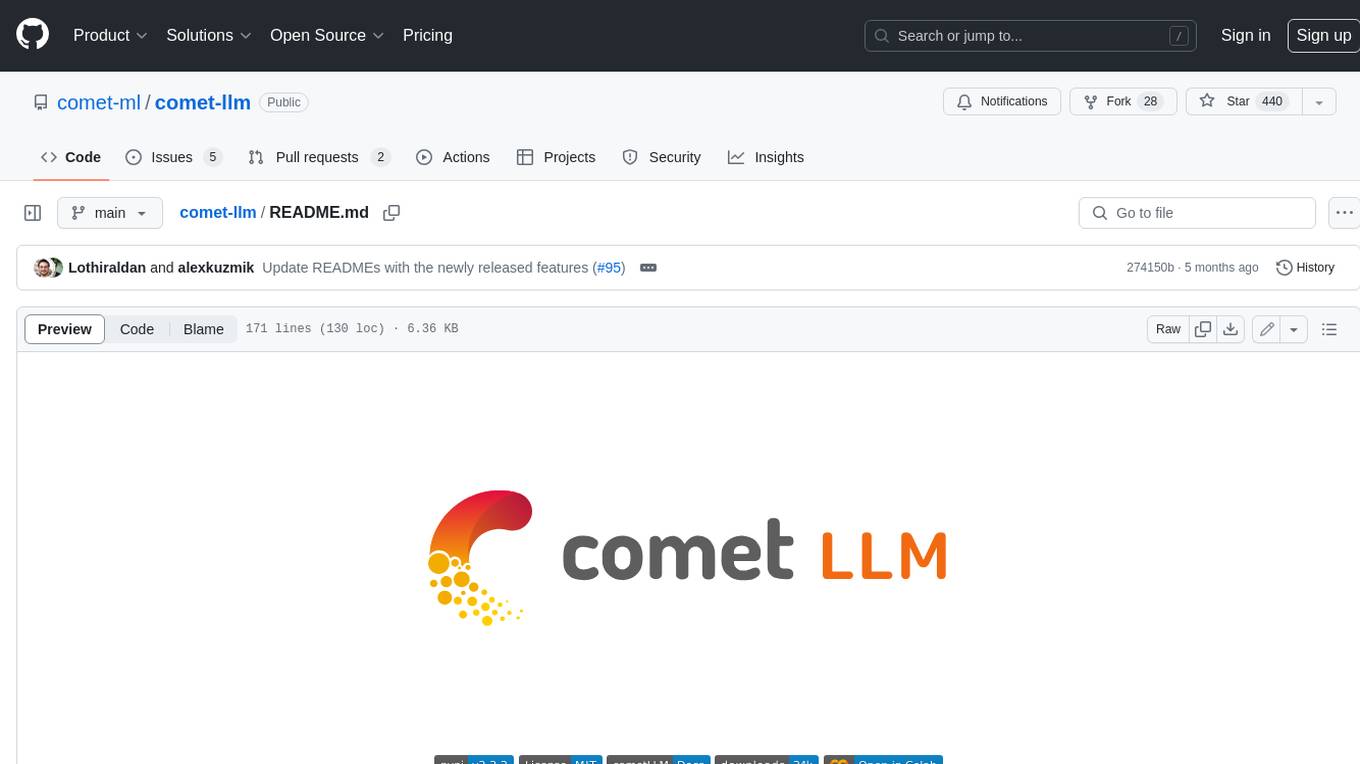
comet-llm
CometLLM is a tool to log and visualize your LLM prompts and chains. Use CometLLM to identify effective prompt strategies, streamline your troubleshooting, and ensure reproducible workflows!
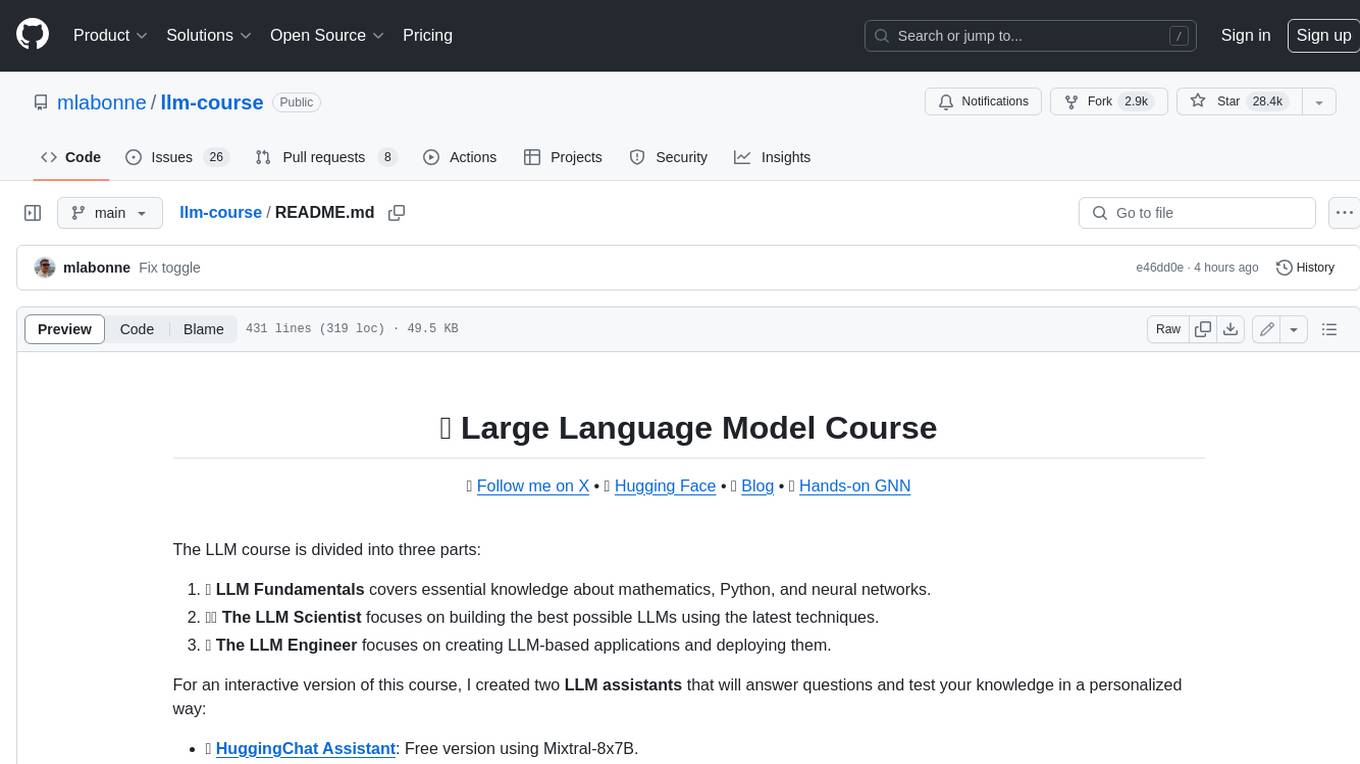
llm-course
The LLM course is divided into three parts: 1. 🧩 **LLM Fundamentals** covers essential knowledge about mathematics, Python, and neural networks. 2. 🧑🔬 **The LLM Scientist** focuses on building the best possible LLMs using the latest techniques. 3. 👷 **The LLM Engineer** focuses on creating LLM-based applications and deploying them. For an interactive version of this course, I created two **LLM assistants** that will answer questions and test your knowledge in a personalized way: * 🤗 **HuggingChat Assistant**: Free version using Mixtral-8x7B. * 🤖 **ChatGPT Assistant**: Requires a premium account. ## 📝 Notebooks A list of notebooks and articles related to large language models. ### Tools | Notebook | Description | Notebook | |----------|-------------|----------| | 🧐 LLM AutoEval | Automatically evaluate your LLMs using RunPod |  | | 🥱 LazyMergekit | Easily merge models using MergeKit in one click. |  | | 🦎 LazyAxolotl | Fine-tune models in the cloud using Axolotl in one click. |  | | ⚡ AutoQuant | Quantize LLMs in GGUF, GPTQ, EXL2, AWQ, and HQQ formats in one click. |  | | 🌳 Model Family Tree | Visualize the family tree of merged models. |  | | 🚀 ZeroSpace | Automatically create a Gradio chat interface using a free ZeroGPU. |  |
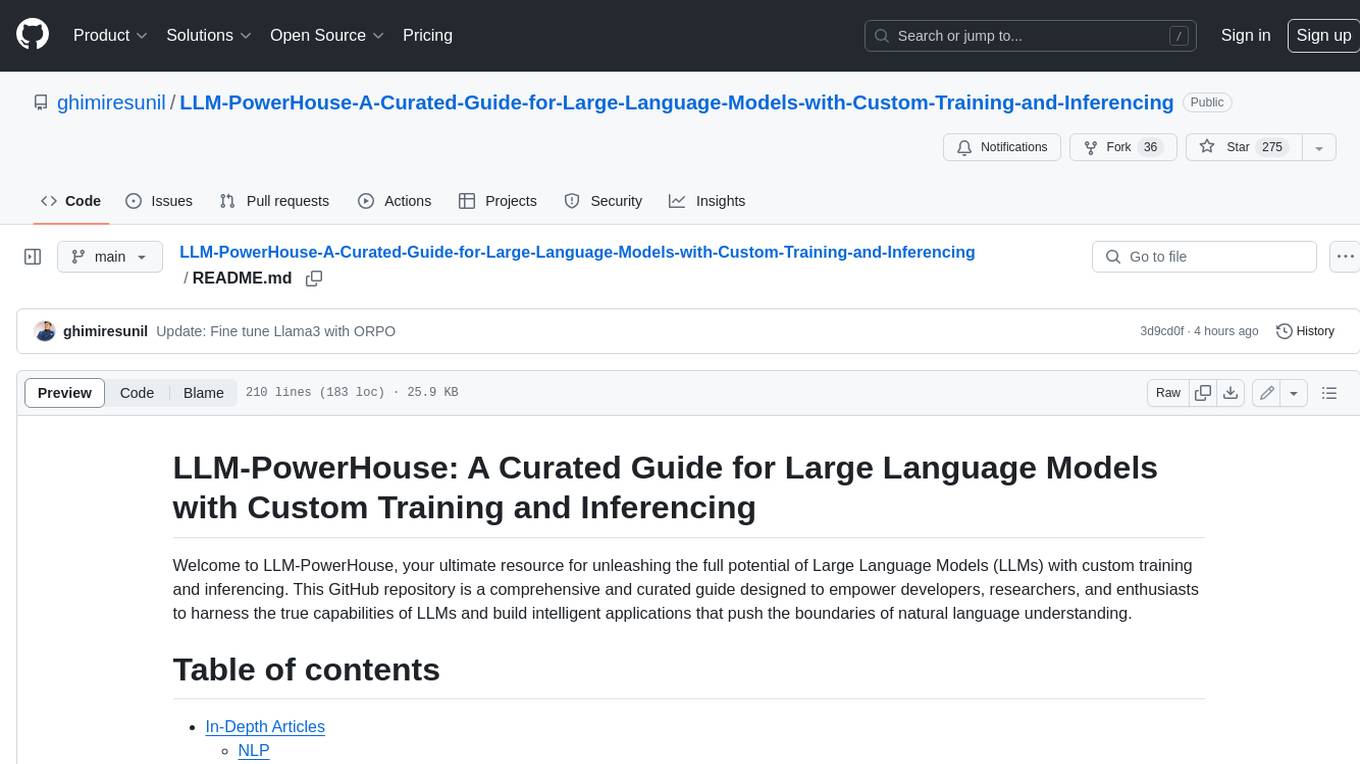
LLM-PowerHouse-A-Curated-Guide-for-Large-Language-Models-with-Custom-Training-and-Inferencing
LLM-PowerHouse is a comprehensive and curated guide designed to empower developers, researchers, and enthusiasts to harness the true capabilities of Large Language Models (LLMs) and build intelligent applications that push the boundaries of natural language understanding. This GitHub repository provides in-depth articles, codebase mastery, LLM PlayLab, and resources for cost analysis and network visualization. It covers various aspects of LLMs, including NLP, models, training, evaluation metrics, open LLMs, and more. The repository also includes a collection of code examples and tutorials to help users build and deploy LLM-based applications.
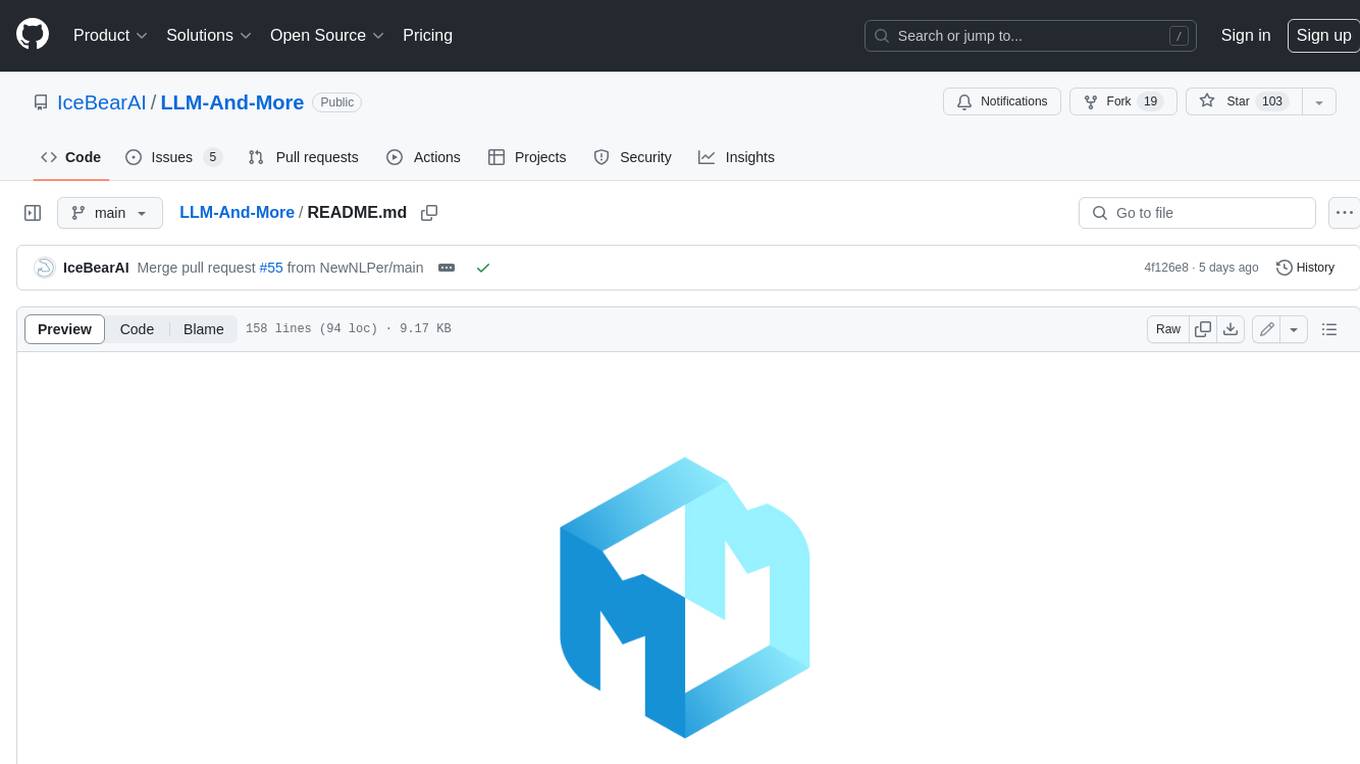
LLM-And-More
LLM-And-More is a one-stop solution for training and applying large models, covering the entire process from data processing to model evaluation, from training to deployment, and from idea to service. In this project, users can easily train models through this project and generate the required product services with one click.
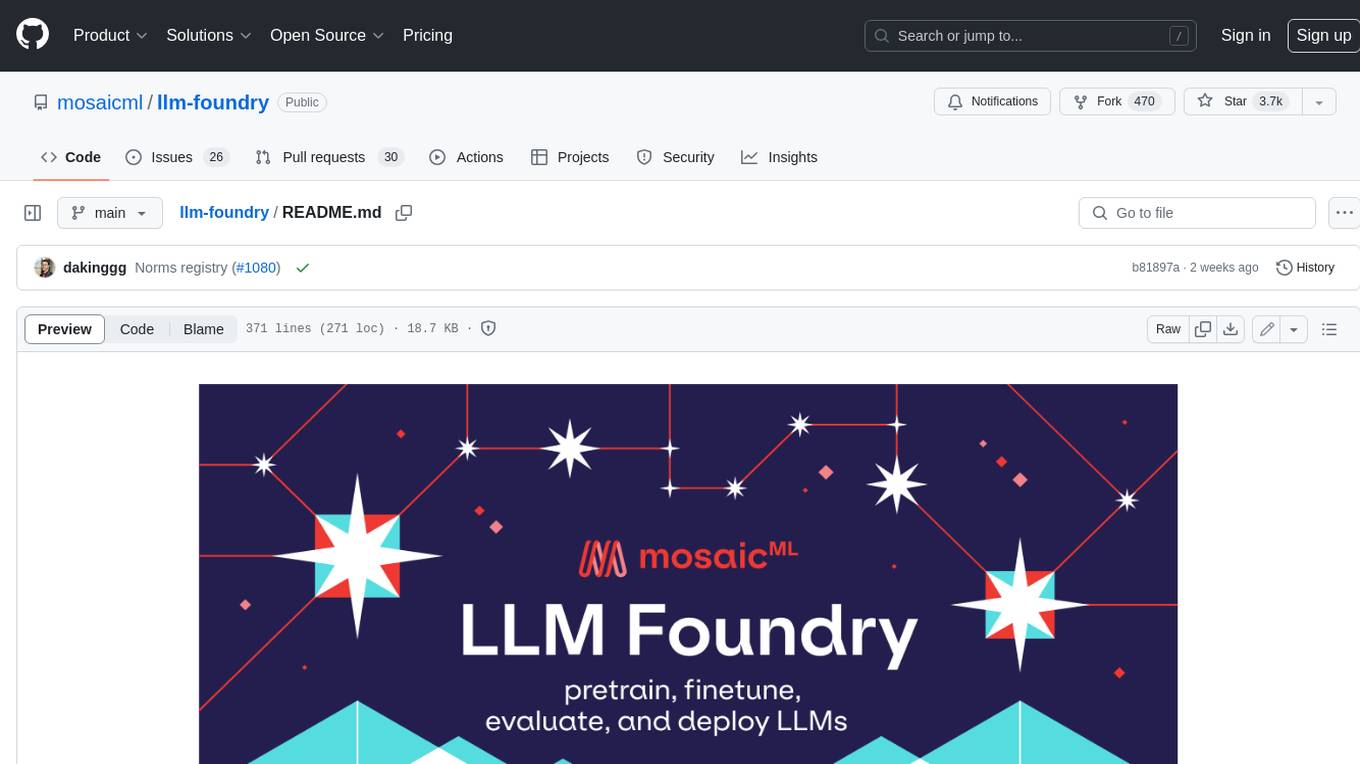
llm-foundry
LLM Foundry is a codebase for training, finetuning, evaluating, and deploying LLMs for inference with Composer and the MosaicML platform. It is designed to be easy-to-use, efficient _and_ flexible, enabling rapid experimentation with the latest techniques. You'll find in this repo: * `llmfoundry/` - source code for models, datasets, callbacks, utilities, etc. * `scripts/` - scripts to run LLM workloads * `data_prep/` - convert text data from original sources to StreamingDataset format * `train/` - train or finetune HuggingFace and MPT models from 125M - 70B parameters * `train/benchmarking` - profile training throughput and MFU * `inference/` - convert models to HuggingFace or ONNX format, and generate responses * `inference/benchmarking` - profile inference latency and throughput * `eval/` - evaluate LLMs on academic (or custom) in-context-learning tasks * `mcli/` - launch any of these workloads using MCLI and the MosaicML platform * `TUTORIAL.md` - a deeper dive into the repo, example workflows, and FAQs
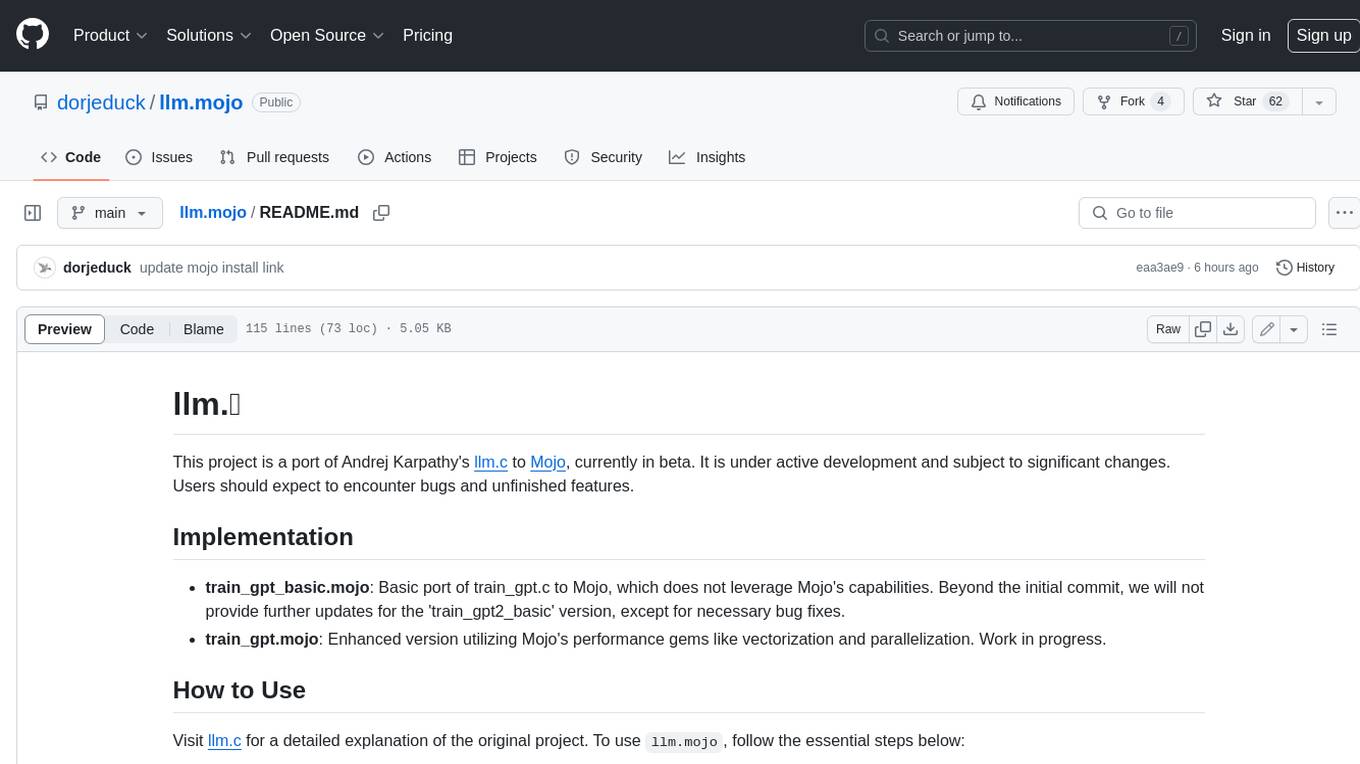
llm.mojo
This project is a port of Andrej Karpathy's llm.c to Mojo, currently in beta. It is under active development and subject to changes. Users should expect to encounter bugs and unfinished features.