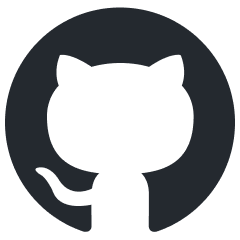
Coding-Tutor
Training Turn-by-Turn Verifiers for Dialogue Tutoring Agents: The Curious Case of LLMs as Your Coding Tutors
Stars: 57
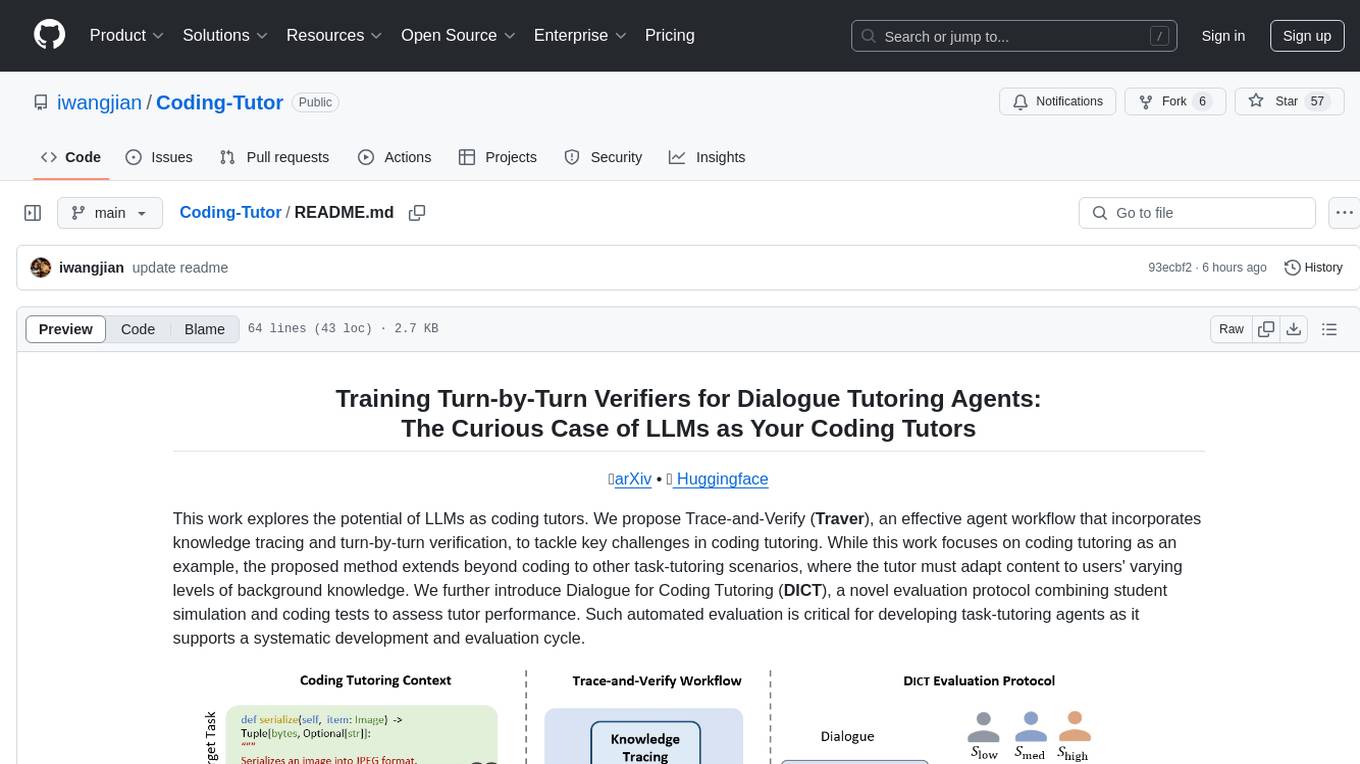
This repository explores the potential of LLMs as coding tutors through the proposed Traver agent workflow. It focuses on incorporating knowledge tracing and turn-by-turn verification to tackle challenges in coding tutoring. The method extends beyond coding to other task-tutoring scenarios, adapting content to users' varying levels of background knowledge. The repository introduces the DICT evaluation protocol for assessing tutor performance through student simulation and coding tests. It also discusses the inference-time scaling with verifiers and provides resources for training and evaluation.
README:
Training Turn-by-Turn Verifiers for Dialogue Tutoring Agents:
The Curious Case of LLMs as Your Coding Tutors
📃arXiv • 🤗 Huggingface
This work explores the potential of LLMs as coding tutors. We propose Trace-and-Verify (Traver), an effective agent workflow that incorporates knowledge tracing and turn-by-turn verification, to tackle key challenges in coding tutoring. While this work focuses on coding tutoring as an example, the proposed method extends beyond coding to other task-tutoring scenarios, where the tutor must adapt content to users' varying levels of background knowledge. We further introduce Dialogue for Coding Tutoring (DICT), a novel evaluation protocol combining student simulation and coding tests to assess tutor performance. Such automated evaluation is critical for developing task-tutoring agents as it supports a systematic development and evaluation cycle.
Under a controlled setup, simulated students at different levels demonstrate distinct abilities in completing target coding tasks. Our DICT protocol serves as a feasible proxy for human evaluation, offering its advantages of scalability and cost-effectiveness for evaluating tutor agents.
Our proposed Traver agent workflow with the trained verifier shows inference-time scaling for coding tutoring:
- [ ] Add detailed instructions for quick start
- [ ] Add shell scripts for training and evaluation
- [ ] Release checkpoints for the verifiers
Please refer to output
for the released data and evaluation results.
Please refer to scripts/eval/
for the evaluation scripts.
If you find the resources in this repository useful for your work, please kindly cite our work as:
@article{wang2025training,
title={Training Turn-by-Turn Verifiers for Dialogue Tutoring Agents: The Curious Case of LLMs as Your Coding Tutors},
author={Wang, Jian and Dai, Yinpei and Zhang, Yichi and Ma, Ziqiao and Li, Wenjie and Chai, Joyce},
journal={arXiv preprint arXiv:2502.13311},
url={https://arxiv.org/abs/2502.13311},
year={2025}
}
For Tasks:
Click tags to check more tools for each tasksFor Jobs:
Alternative AI tools for Coding-Tutor
Similar Open Source Tools
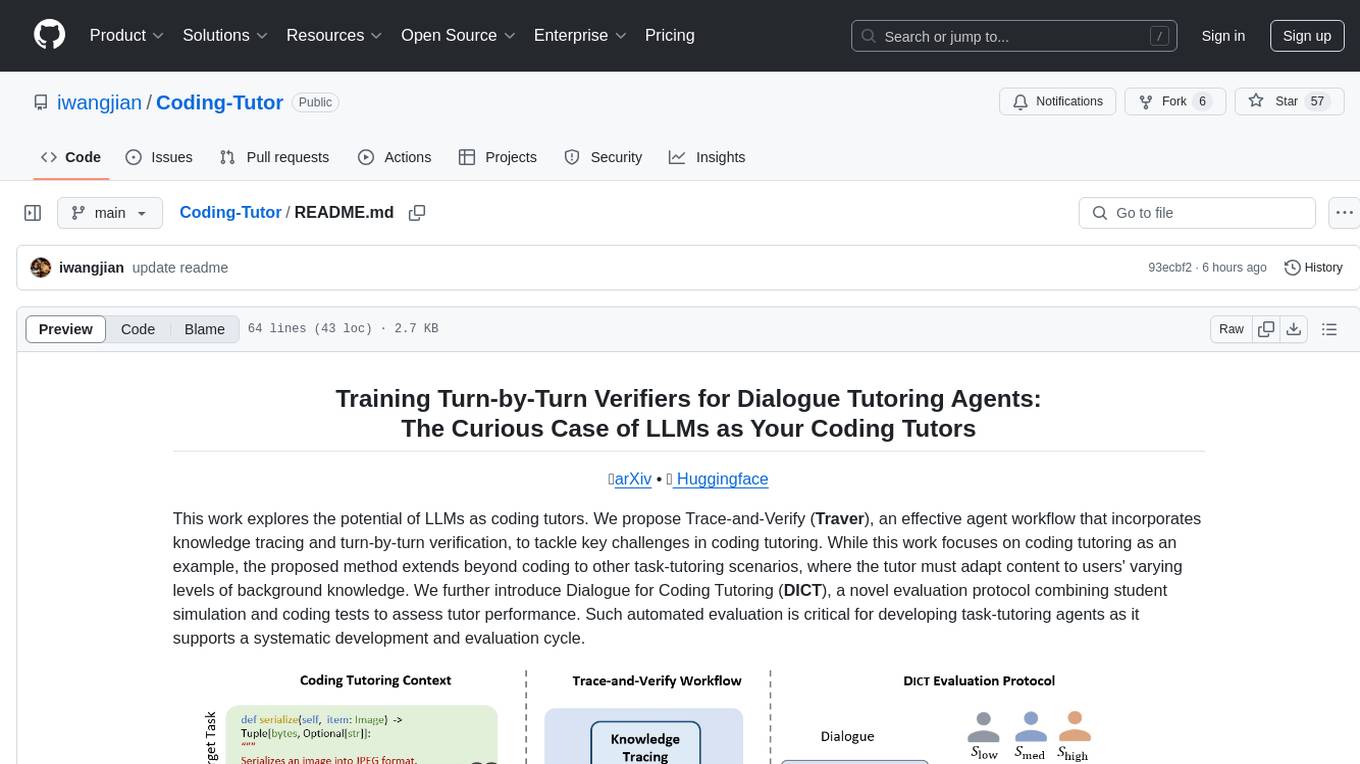
Coding-Tutor
This repository explores the potential of LLMs as coding tutors through the proposed Traver agent workflow. It focuses on incorporating knowledge tracing and turn-by-turn verification to tackle challenges in coding tutoring. The method extends beyond coding to other task-tutoring scenarios, adapting content to users' varying levels of background knowledge. The repository introduces the DICT evaluation protocol for assessing tutor performance through student simulation and coding tests. It also discusses the inference-time scaling with verifiers and provides resources for training and evaluation.
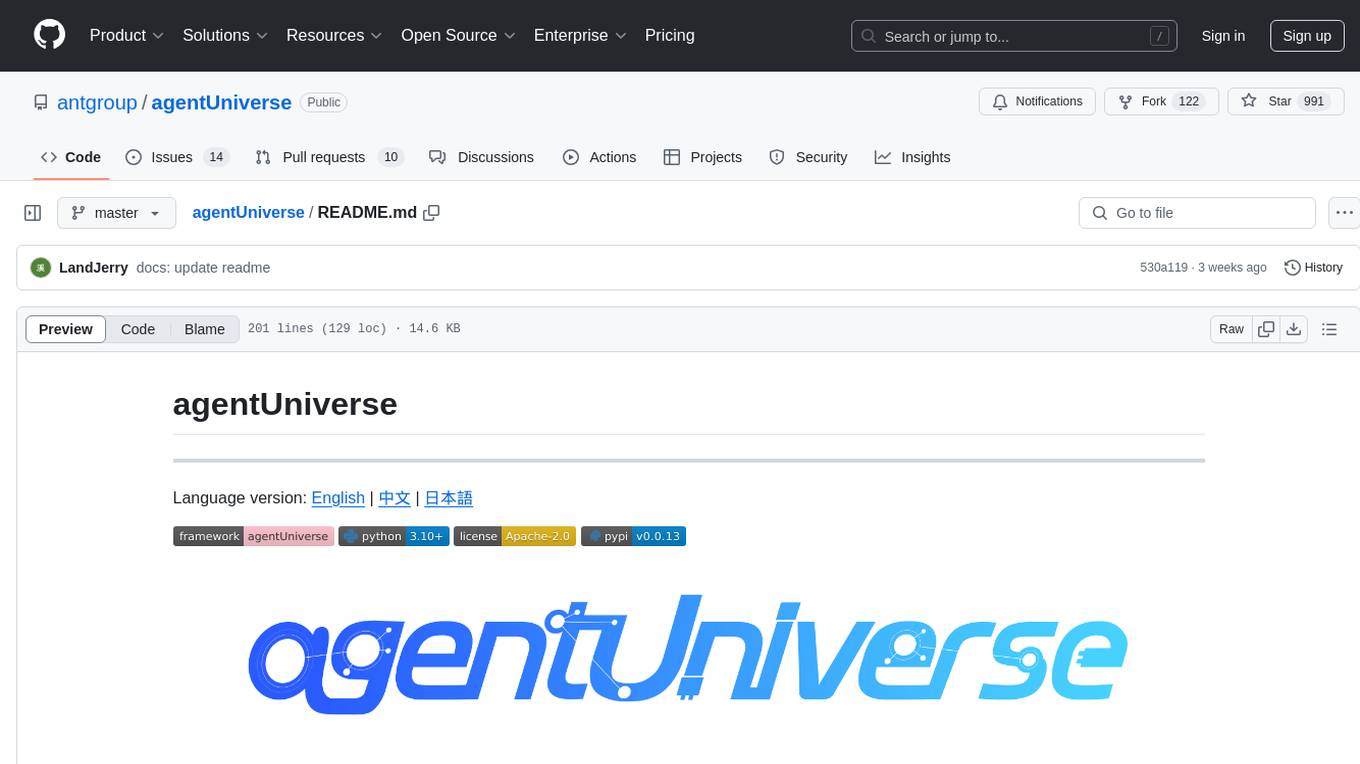
agentUniverse
agentUniverse is a multi-agent framework based on large language models, providing flexible capabilities for building individual agents. It focuses on multi-agent collaborative patterns, integrating domain experience to help agents solve problems in various fields. The framework includes pattern components like PEER and DOE for event interpretation, industry analysis, and financial report generation. It offers features for agent construction, multi-agent collaboration, and domain expertise integration, aiming to create intelligent applications with professional know-how.
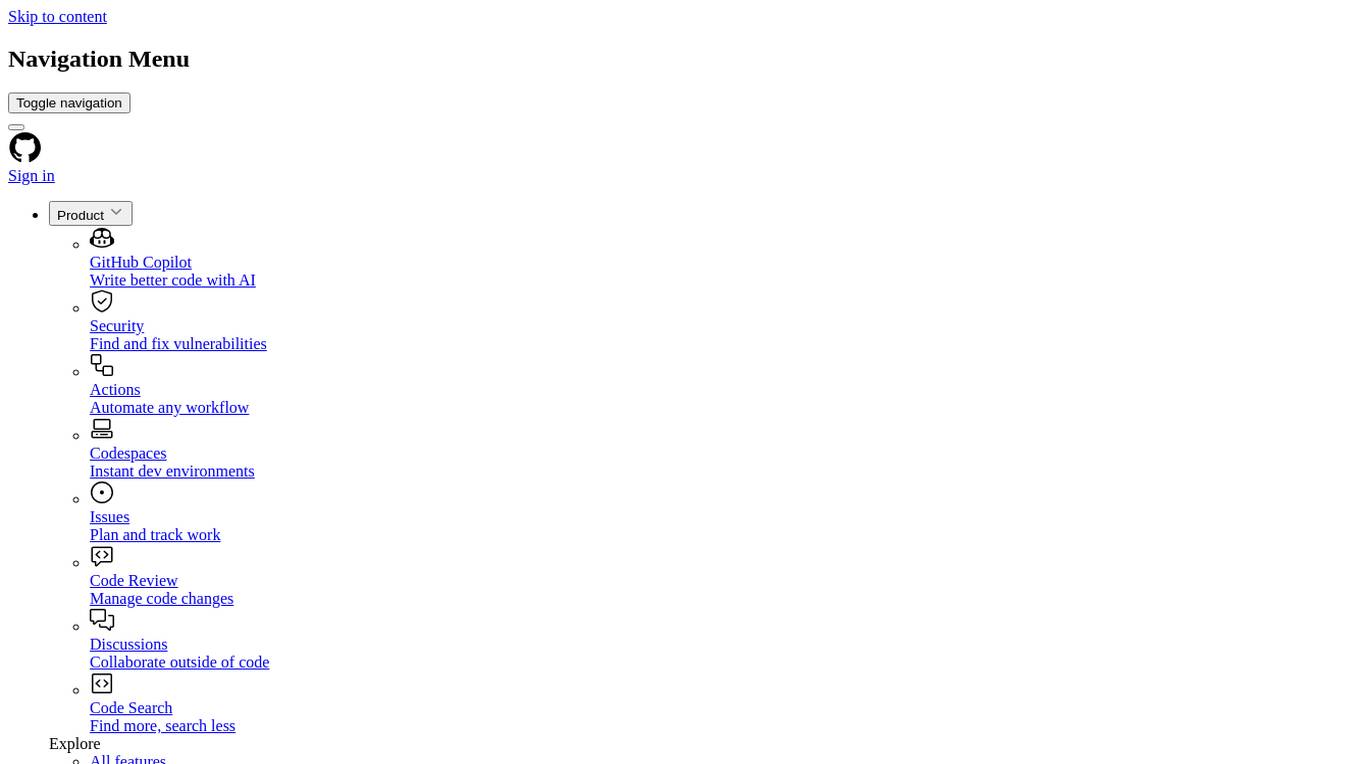
agentUniverse
agentUniverse is a multi-agent framework based on large language models, providing flexible capabilities for building individual agents. It focuses on collaborative pattern components to solve problems in various fields and integrates domain experience. The framework supports LLM model integration and offers various pattern components like PEER and DOE. Users can easily configure models and set up agents for tasks. agentUniverse aims to assist developers and enterprises in constructing domain-expert-level intelligent agents for seamless collaboration.
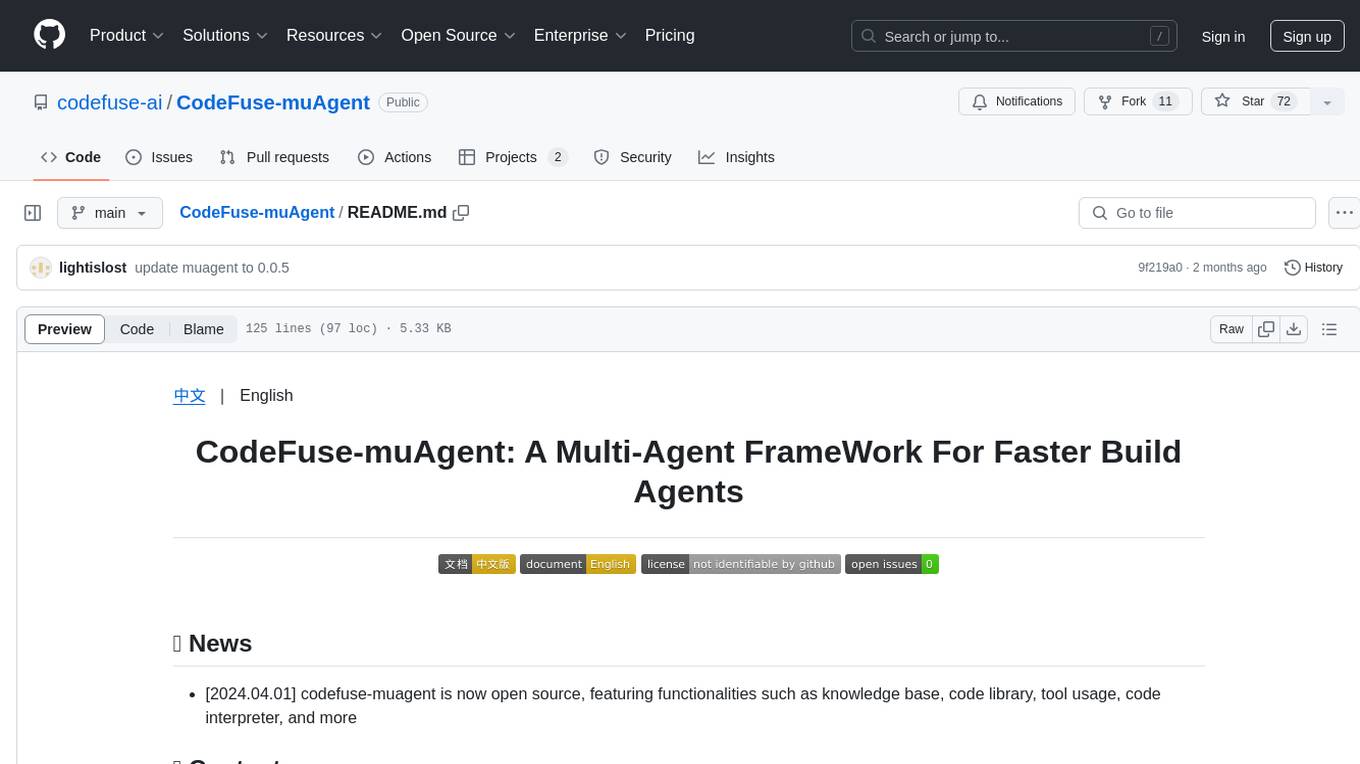
CodeFuse-muAgent
CodeFuse-muAgent is a Multi-Agent framework designed to streamline Standard Operating Procedure (SOP) orchestration for agents. It integrates toolkits, code libraries, knowledge bases, and sandbox environments for rapid construction of complex Multi-Agent interactive applications. The framework enables efficient execution and handling of multi-layered and multi-dimensional tasks.
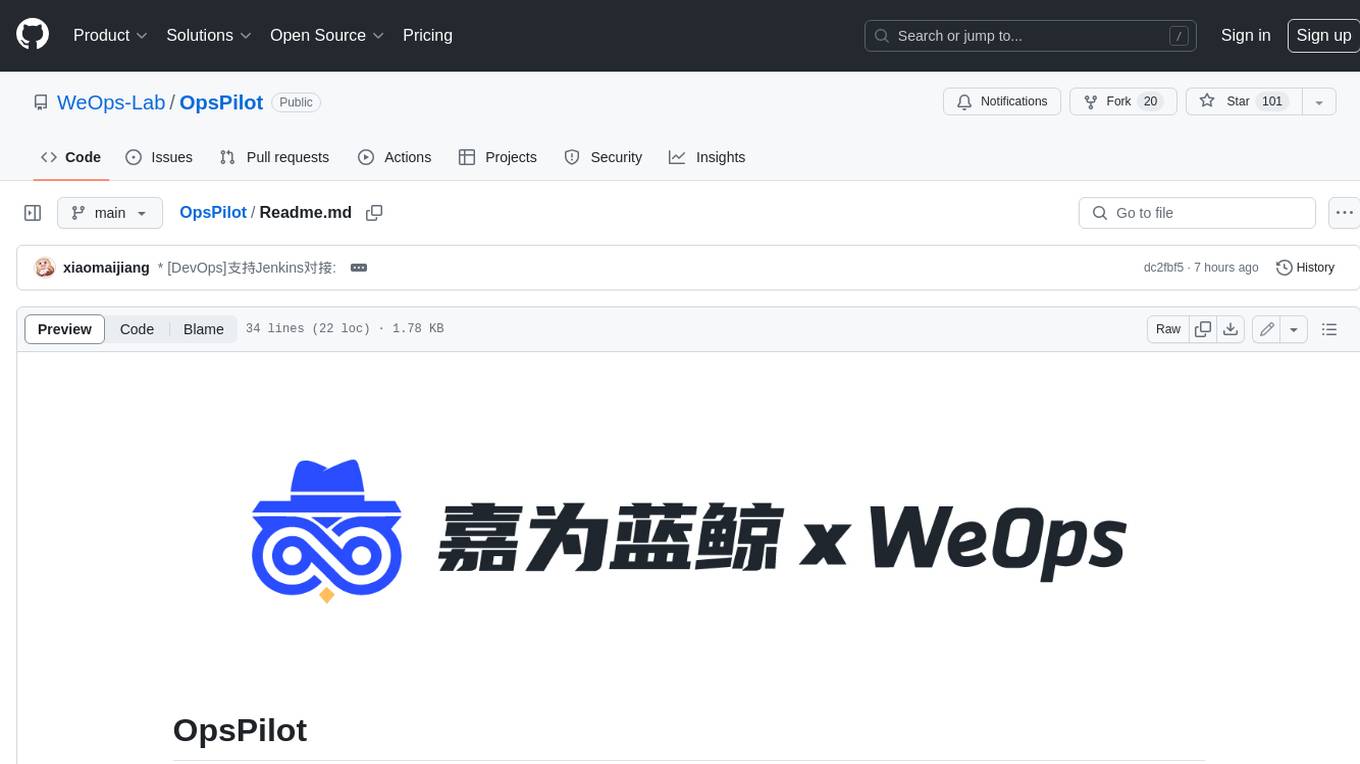
OpsPilot
OpsPilot is an AI-powered operations navigator developed by the WeOps team. It leverages deep learning and LLM technologies to make operations plans interactive and generalize and reason about local operations knowledge. OpsPilot can be integrated with web applications in the form of a chatbot and primarily provides the following capabilities: 1. Operations capability precipitation: By depositing operations knowledge, operations skills, and troubleshooting actions, when solving problems, it acts as a navigator and guides users to solve operations problems through dialogue. 2. Local knowledge Q&A: By indexing local knowledge and Internet knowledge and combining the capabilities of LLM, it answers users' various operations questions. 3. LLM chat: When the problem is beyond the scope of OpsPilot's ability to handle, it uses LLM's capabilities to solve various long-tail problems.
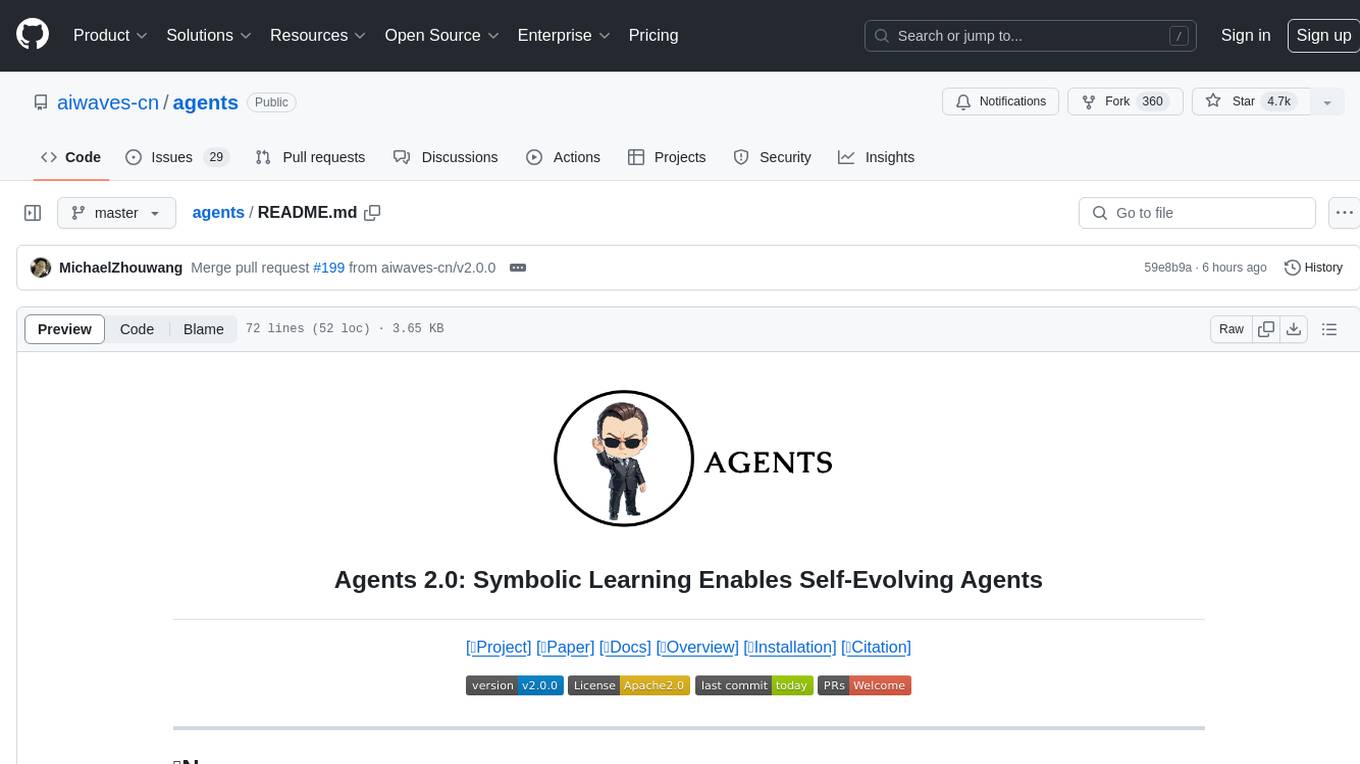
agents
Agents 2.0 is a framework for training language agents using symbolic learning, inspired by connectionist learning for neural nets. It implements main components of connectionist learning like back-propagation and gradient-based weight update in the context of agent training using language-based loss, gradients, and weights. The framework supports optimizing multi-agent systems and allows multiple agents to take actions in one node.
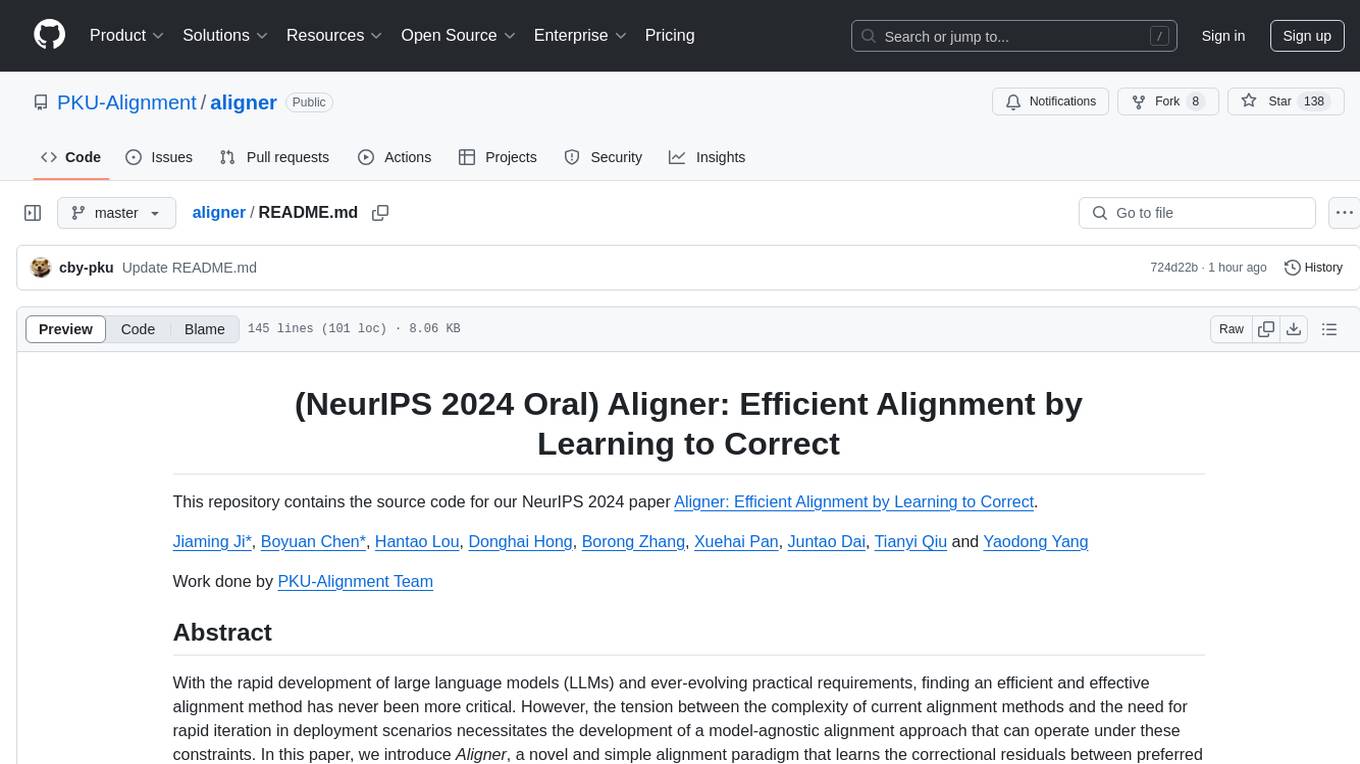
aligner
Aligner is a model-agnostic alignment tool designed to efficiently correct responses from large language models. It redistributes initial answers to align with human intentions, improving performance across various LLMs. The tool can be applied with minimal training, enhancing upstream models and reducing hallucination. Aligner's 'copy and correct' method preserves the base structure while enhancing responses. It achieves significant performance improvements in helpfulness, harmlessness, and honesty dimensions, with notable success in boosting Win Rates on evaluation leaderboards.
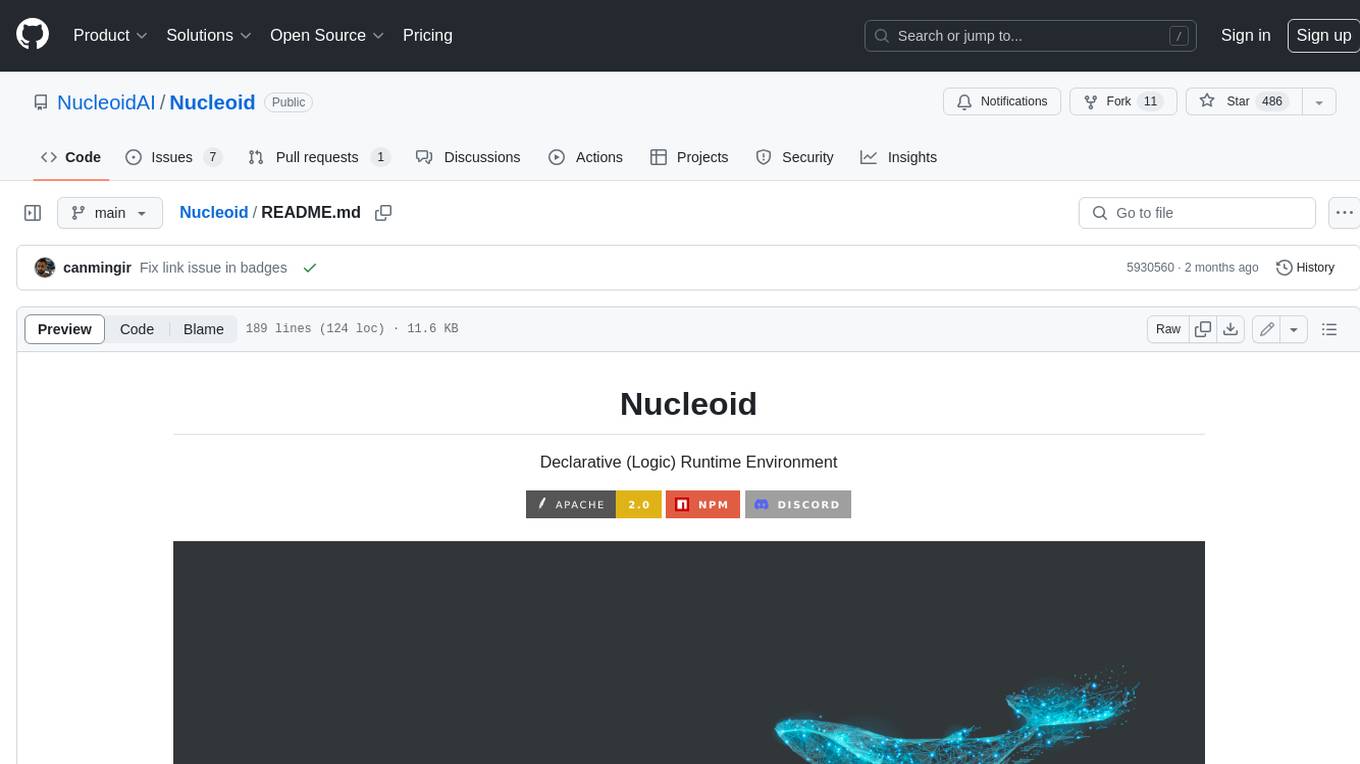
Nucleoid
Nucleoid is a declarative (logic) runtime environment that manages both data and logic under the same runtime. It uses a declarative programming paradigm, which allows developers to focus on the business logic of the application, while the runtime manages the technical details. This allows for faster development and reduces the amount of code that needs to be written. Additionally, the sharding feature can help to distribute the load across multiple instances, which can further improve the performance of the system.
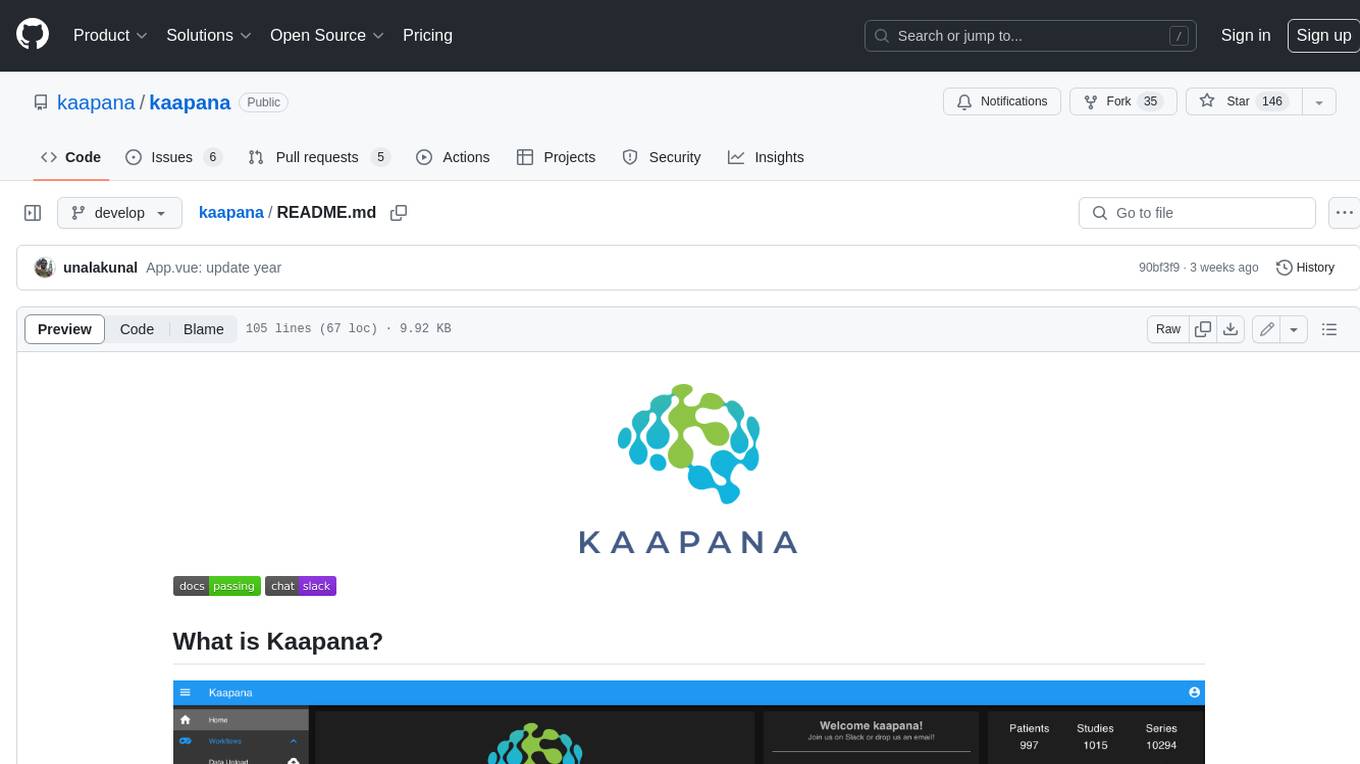
kaapana
Kaapana is an open-source toolkit for state-of-the-art platform provisioning in the field of medical data analysis. The applications comprise AI-based workflows and federated learning scenarios with a focus on radiological and radiotherapeutic imaging. Obtaining large amounts of medical data necessary for developing and training modern machine learning methods is an extremely challenging effort that often fails in a multi-center setting, e.g. due to technical, organizational and legal hurdles. A federated approach where the data remains under the authority of the individual institutions and is only processed on-site is, in contrast, a promising approach ideally suited to overcome these difficulties. Following this federated concept, the goal of Kaapana is to provide a framework and a set of tools for sharing data processing algorithms, for standardized workflow design and execution as well as for performing distributed method development. This will facilitate data analysis in a compliant way enabling researchers and clinicians to perform large-scale multi-center studies. By adhering to established standards and by adopting widely used open technologies for private cloud development and containerized data processing, Kaapana integrates seamlessly with the existing clinical IT infrastructure, such as the Picture Archiving and Communication System (PACS), and ensures modularity and easy extensibility.
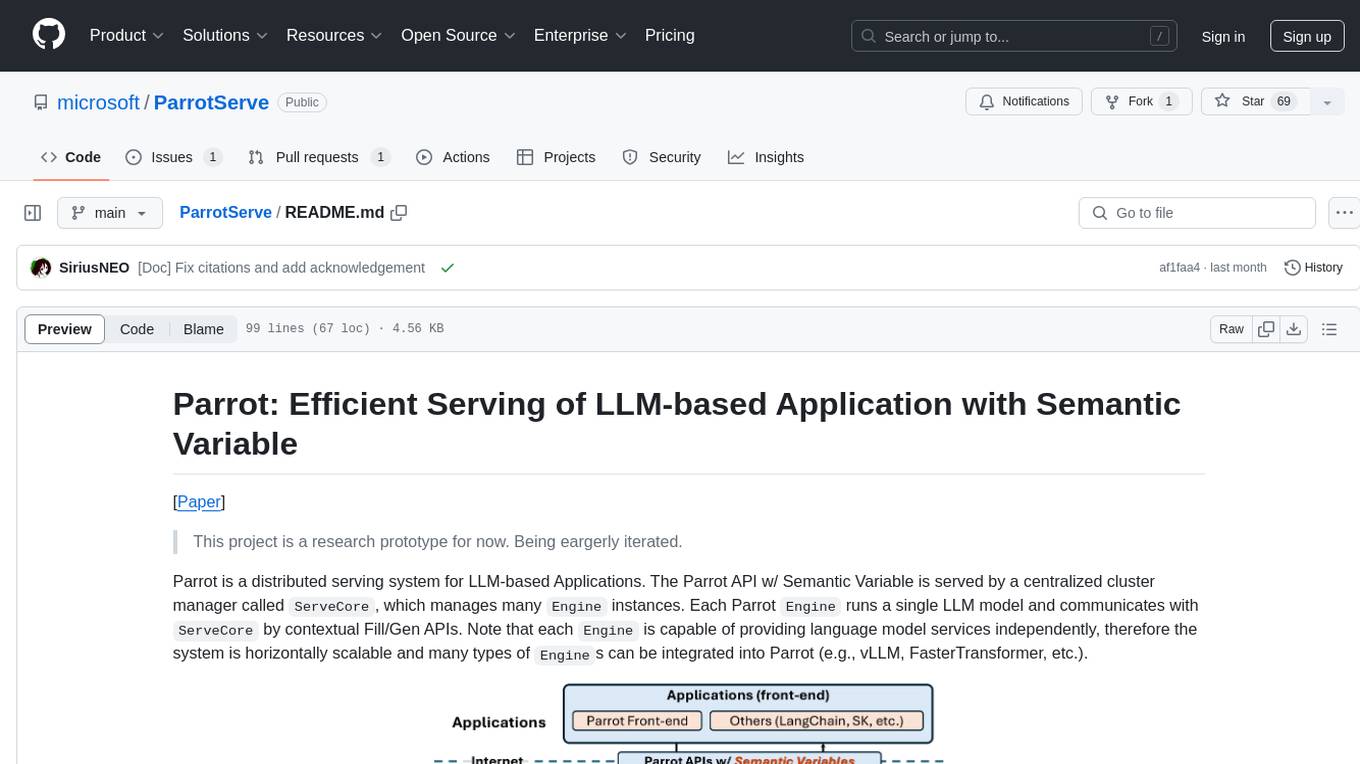
ParrotServe
Parrot is a distributed serving system for LLM-based Applications, designed to efficiently serve LLM-based applications by adding Semantic Variable in the OpenAI-style API. It allows for horizontal scalability with multiple Engine instances running LLM models communicating with ServeCore. The system enables AI agents to interact with LLMs via natural language prompts for collaborative tasks.
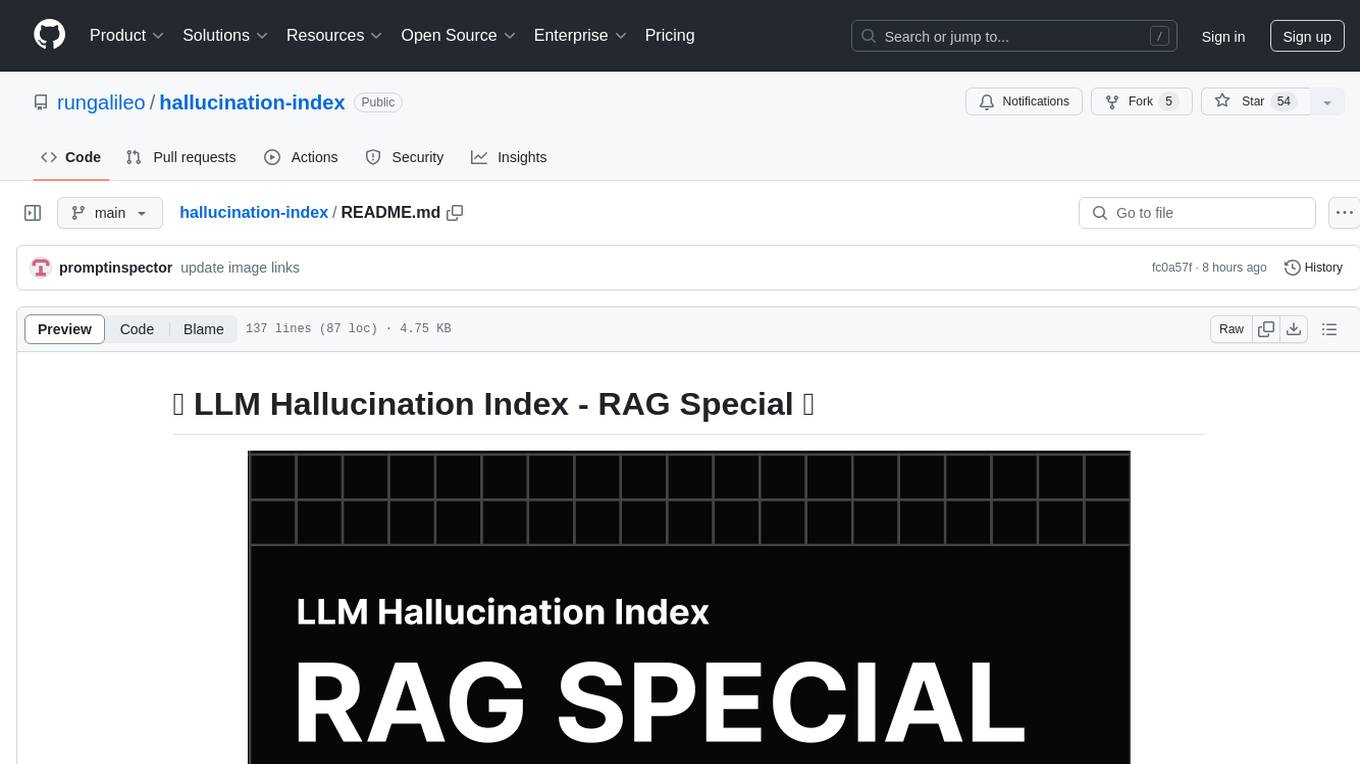
hallucination-index
LLM Hallucination Index - RAG Special is a comprehensive evaluation of large language models (LLMs) focusing on context length and open vs. closed-source attributes. The index explores the impact of context length on model performance and tests the assumption that closed-source LLMs outperform open-source ones. It also investigates the effectiveness of prompting techniques like Chain-of-Note across different context lengths. The evaluation includes 22 models from various brands, analyzing major trends and declaring overall winners based on short, medium, and long context insights. Methodologies involve rigorous testing with different context lengths and prompting techniques to assess models' abilities in handling extensive texts and detecting hallucinations.
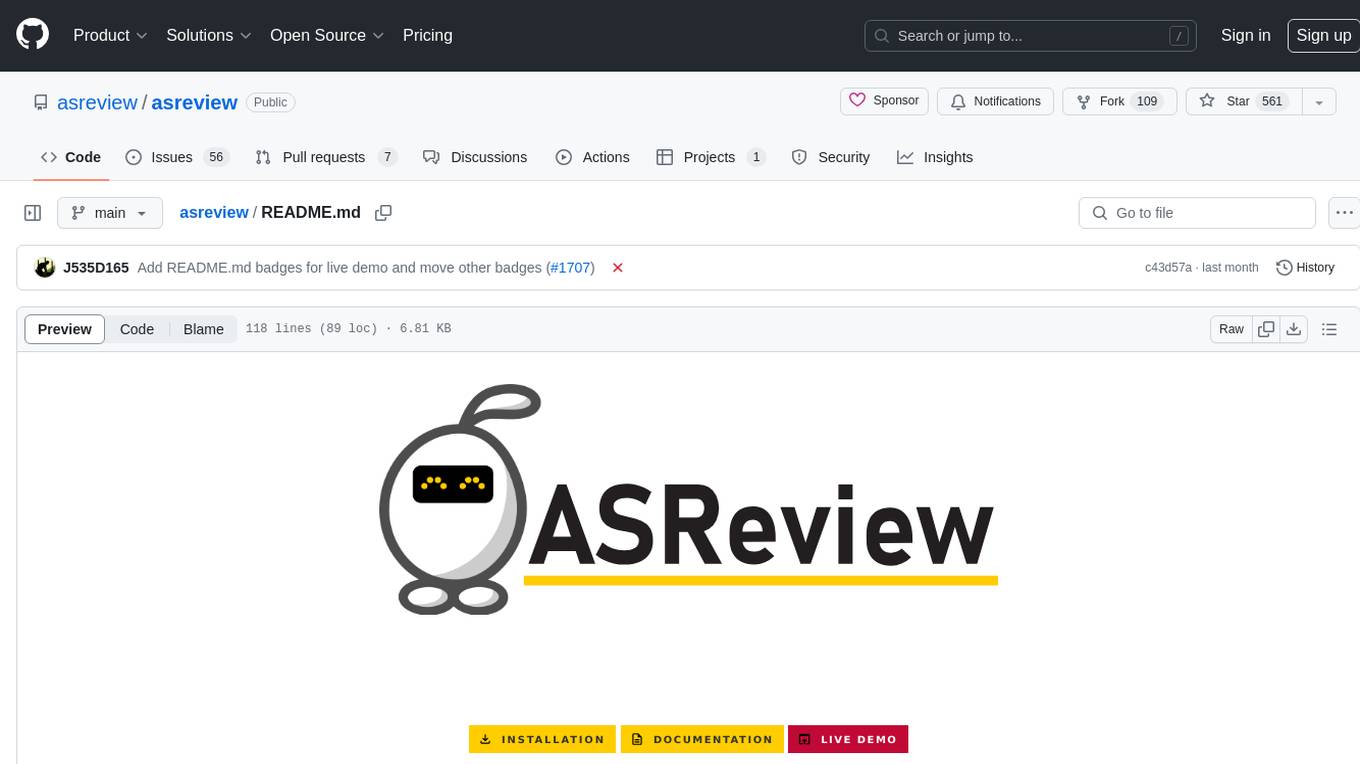
asreview
The ASReview project implements active learning for systematic reviews, utilizing AI-aided pipelines to assist in finding relevant texts for search tasks. It accelerates the screening of textual data with minimal human input, saving time and increasing output quality. The software offers three modes: Oracle for interactive screening, Exploration for teaching purposes, and Simulation for evaluating active learning models. ASReview LAB is designed to support decision-making in any discipline or industry by improving efficiency and transparency in screening large amounts of textual data.
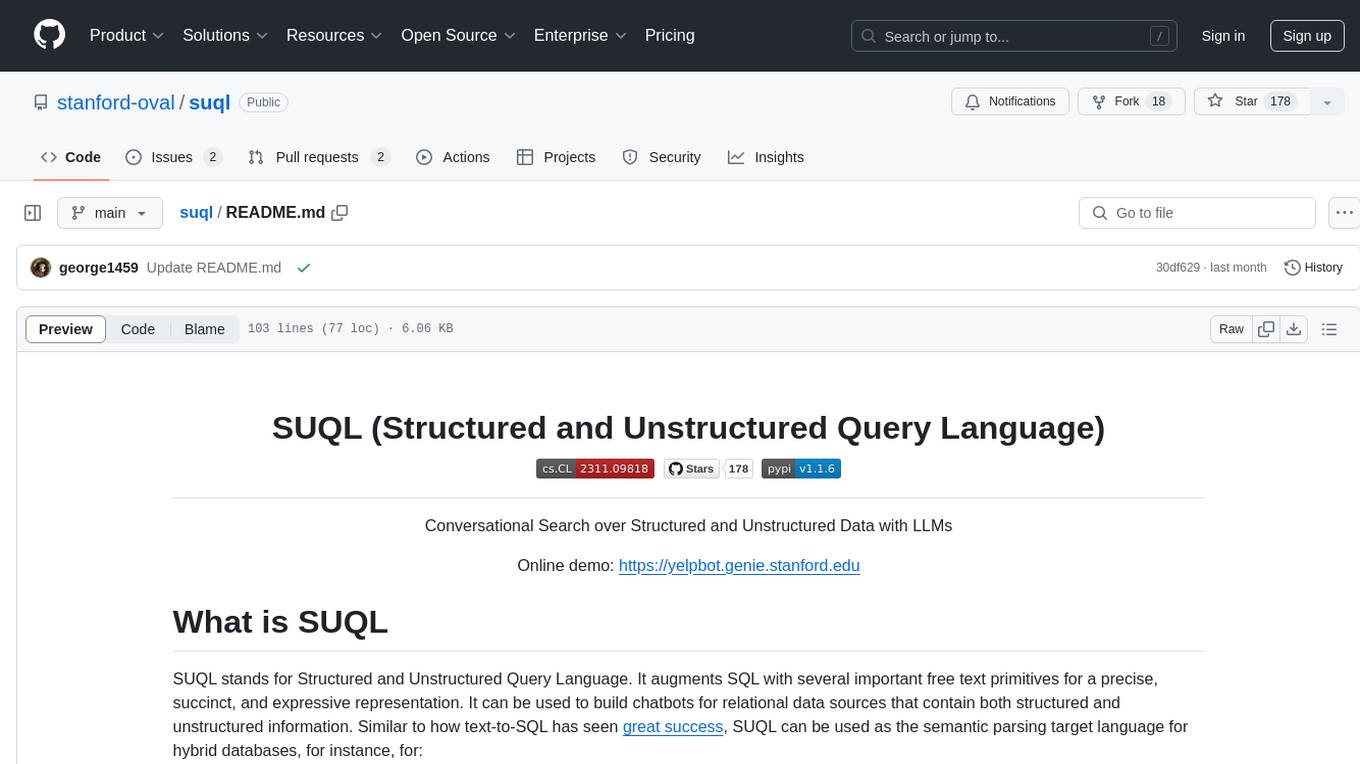
suql
SUQL (Structured and Unstructured Query Language) is a tool that augments SQL with free text primitives for building chatbots that can interact with relational data sources containing both structured and unstructured information. It seamlessly integrates retrieval models, large language models (LLMs), and traditional SQL to provide a clean interface for hybrid data access. SUQL supports optimizations to minimize expensive LLM calls, scalability to large databases with PostgreSQL, and general SQL operations like JOINs and GROUP BYs.
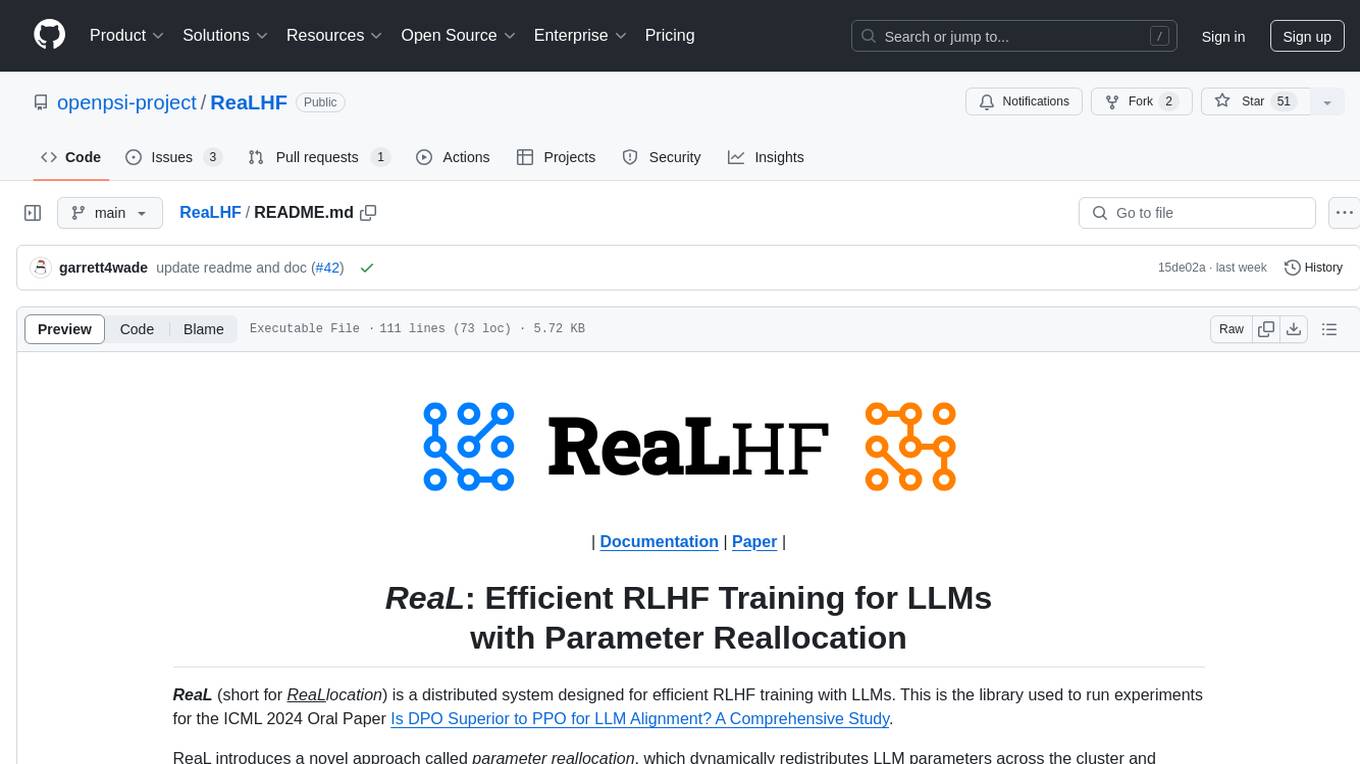
ReaLHF
ReaLHF is a distributed system designed for efficient RLHF training with Large Language Models (LLMs). It introduces a novel approach called parameter reallocation to dynamically redistribute LLM parameters across the cluster, optimizing allocations and parallelism for each computation workload. ReaL minimizes redundant communication while maximizing GPU utilization, achieving significantly higher Proximal Policy Optimization (PPO) training throughput compared to other systems. It supports large-scale training with various parallelism strategies and enables memory-efficient training with parameter and optimizer offloading. The system seamlessly integrates with HuggingFace checkpoints and inference frameworks, allowing for easy launching of local or distributed experiments. ReaLHF offers flexibility through versatile configuration customization and supports various RLHF algorithms, including DPO, PPO, RAFT, and more, while allowing the addition of custom algorithms for high efficiency.
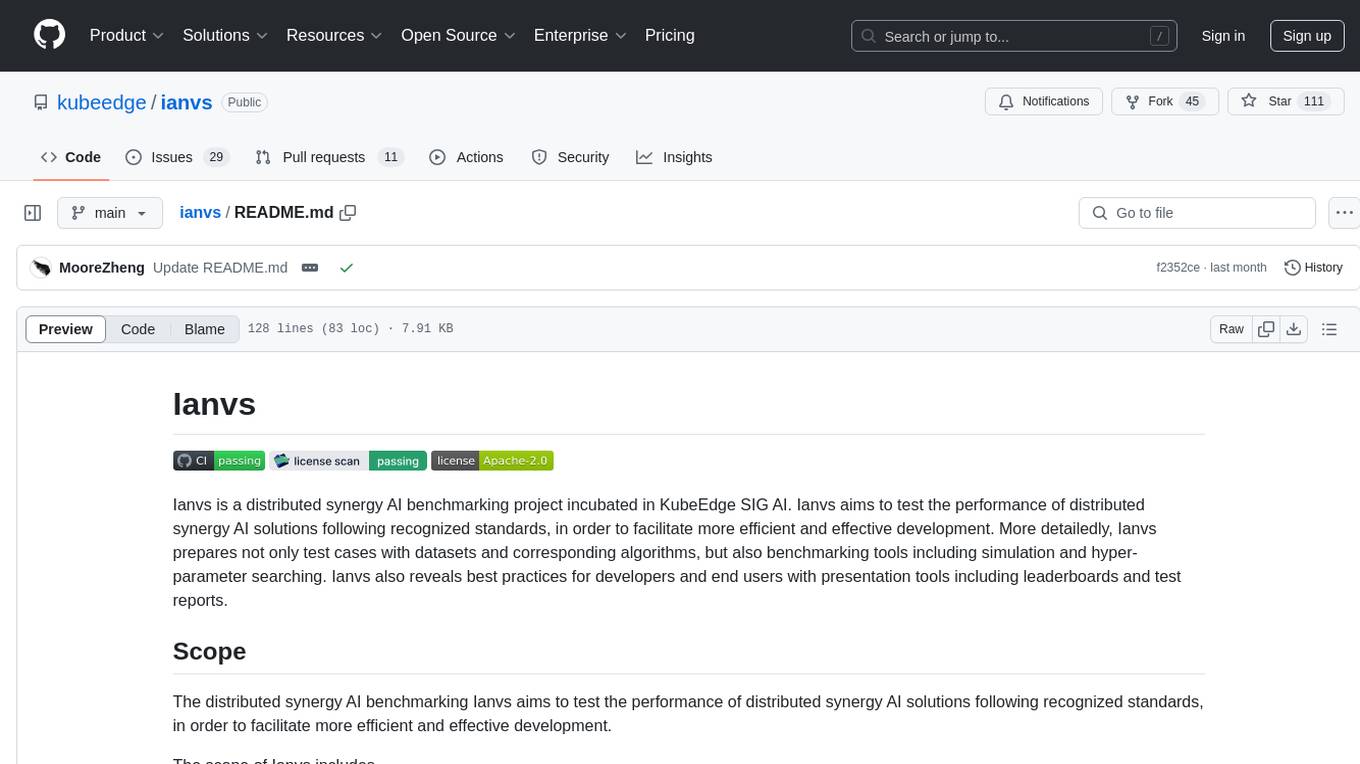
ianvs
Ianvs is a distributed synergy AI benchmarking project incubated in KubeEdge SIG AI. It aims to test the performance of distributed synergy AI solutions following recognized standards, providing end-to-end benchmark toolkits, test environment management tools, test case control tools, and benchmark presentation tools. It also collaborates with other organizations to establish comprehensive benchmarks and related applications. The architecture includes critical components like Test Environment Manager, Test Case Controller, Generation Assistant, Simulation Controller, and Story Manager. Ianvs documentation covers quick start, guides, dataset descriptions, algorithms, user interfaces, stories, and roadmap.
For similar tasks
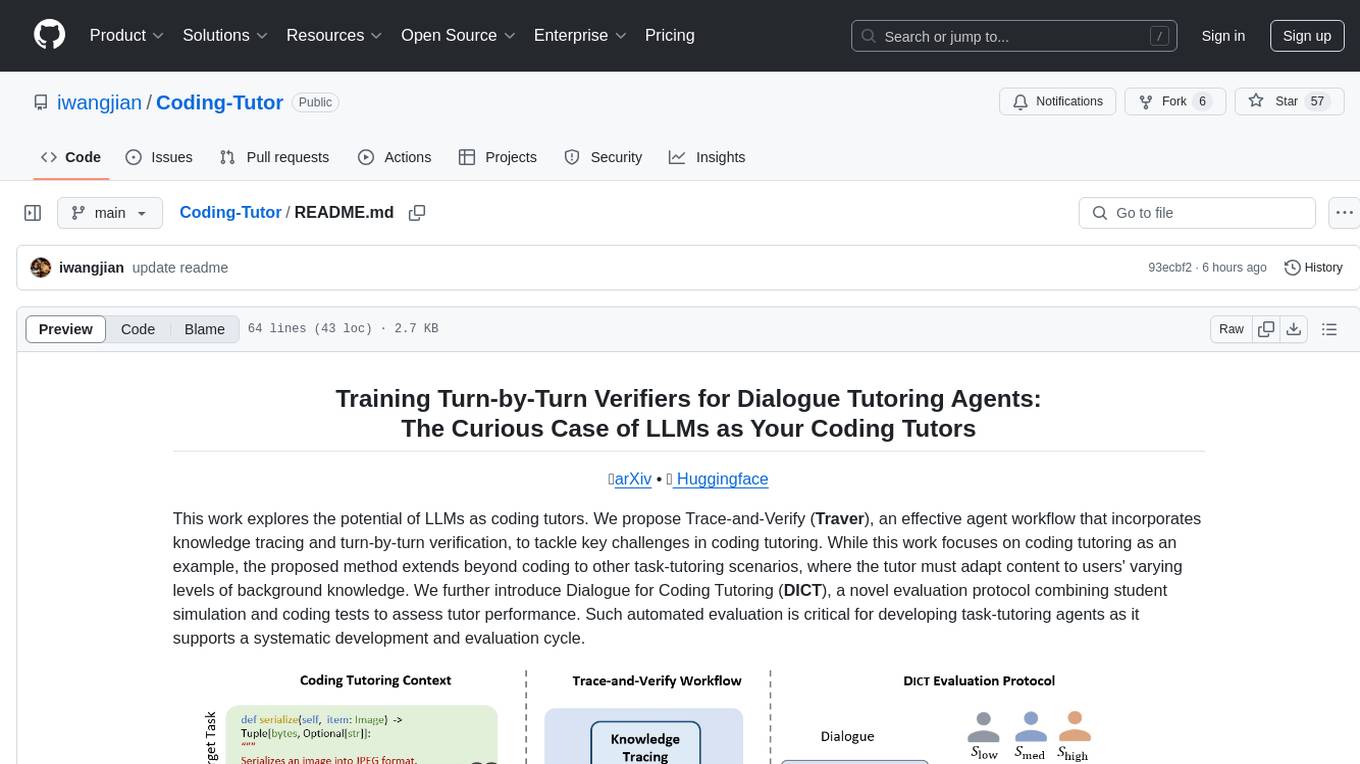
Coding-Tutor
This repository explores the potential of LLMs as coding tutors through the proposed Traver agent workflow. It focuses on incorporating knowledge tracing and turn-by-turn verification to tackle challenges in coding tutoring. The method extends beyond coding to other task-tutoring scenarios, adapting content to users' varying levels of background knowledge. The repository introduces the DICT evaluation protocol for assessing tutor performance through student simulation and coding tests. It also discusses the inference-time scaling with verifiers and provides resources for training and evaluation.
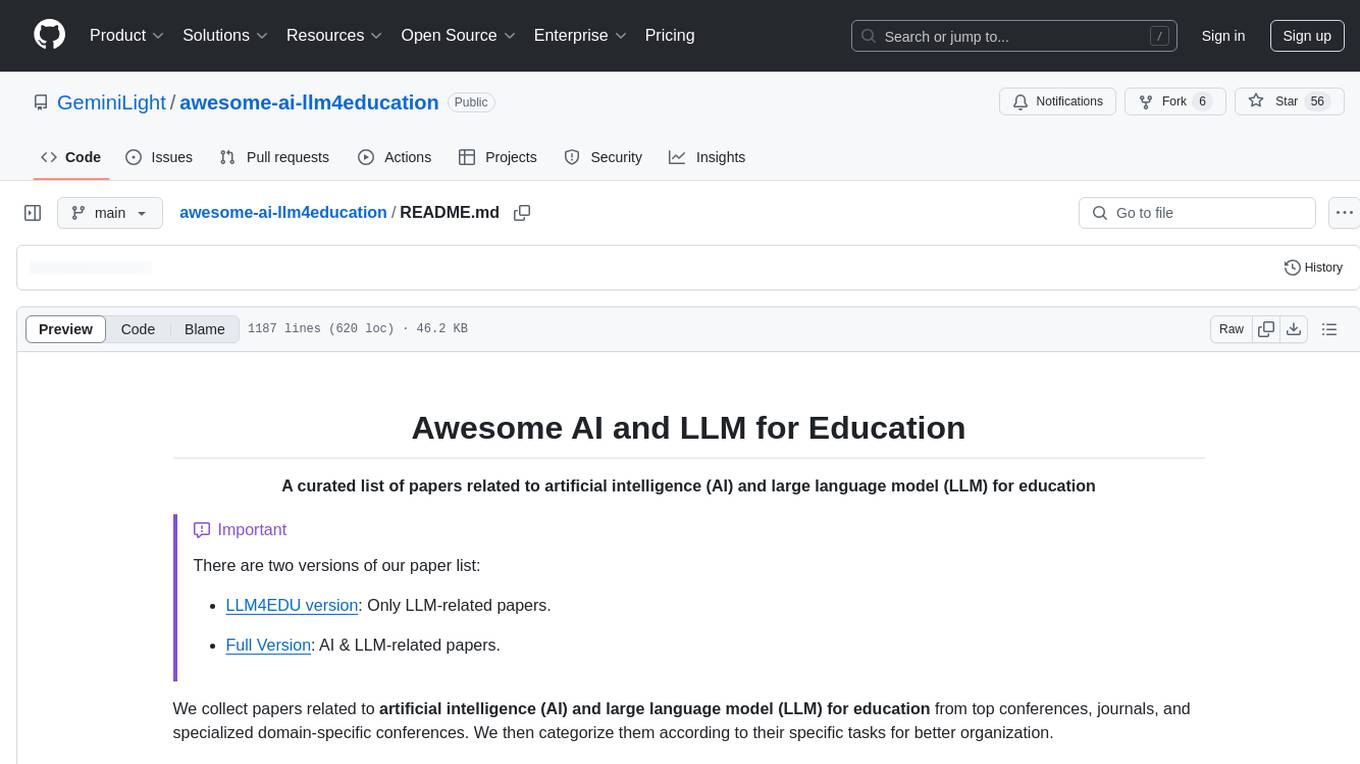
awesome-ai-llm4education
The 'awesome-ai-llm4education' repository is a curated list of papers related to artificial intelligence (AI) and large language models (LLM) for education. It collects papers from top conferences, journals, and specialized domain-specific conferences, categorizing them based on specific tasks for better organization. The repository covers a wide range of topics including tutoring, personalized learning, assessment, material preparation, specific scenarios like computer science, language, math, and medicine, aided teaching, as well as datasets and benchmarks for educational research.
For similar jobs
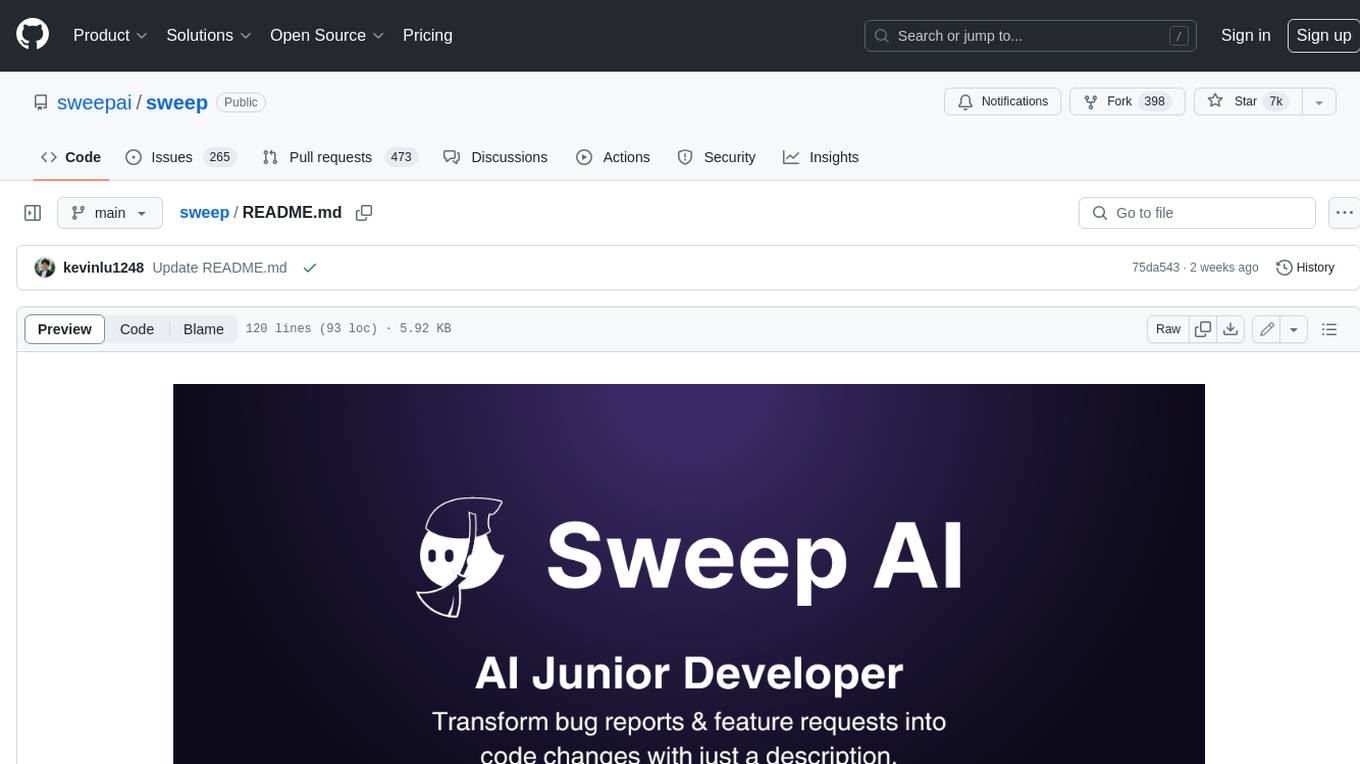
sweep
Sweep is an AI junior developer that turns bugs and feature requests into code changes. It automatically handles developer experience improvements like adding type hints and improving test coverage.
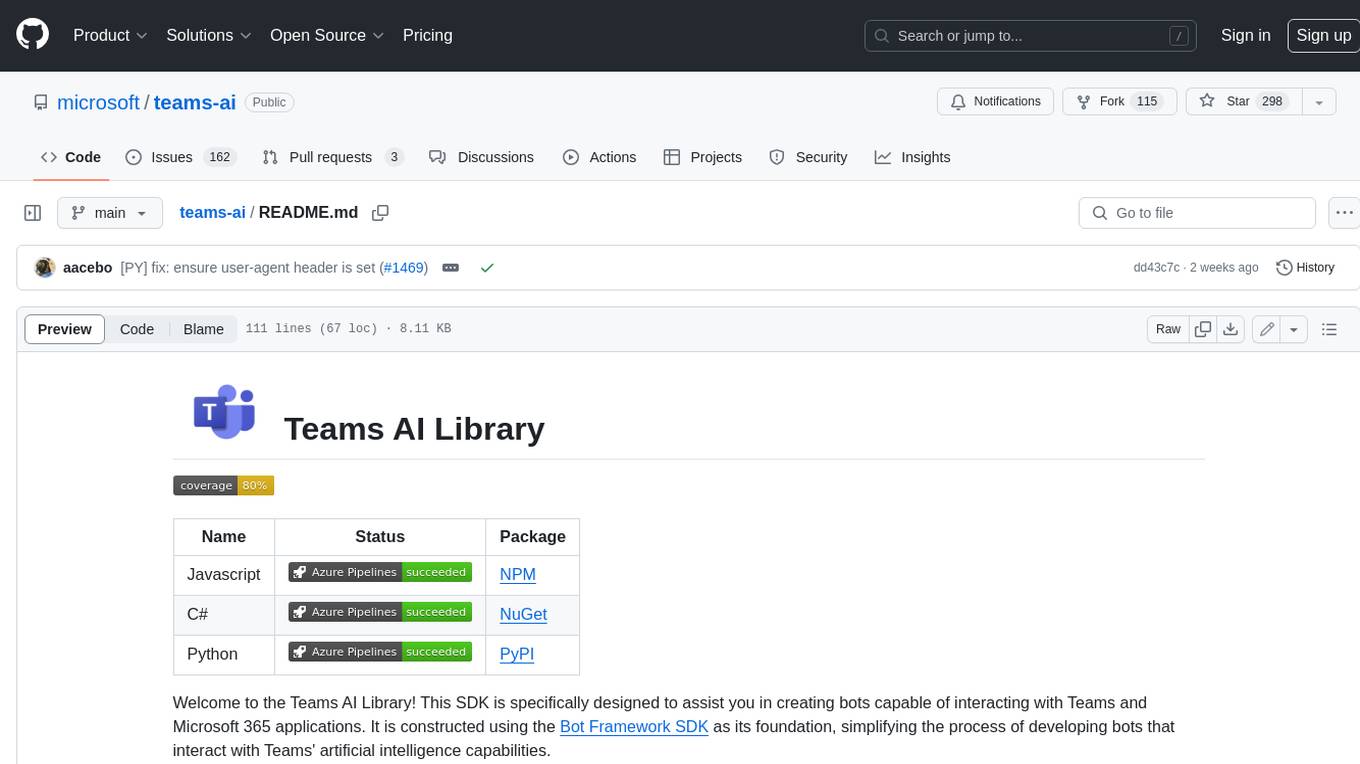
teams-ai
The Teams AI Library is a software development kit (SDK) that helps developers create bots that can interact with Teams and Microsoft 365 applications. It is built on top of the Bot Framework SDK and simplifies the process of developing bots that interact with Teams' artificial intelligence capabilities. The SDK is available for JavaScript/TypeScript, .NET, and Python.
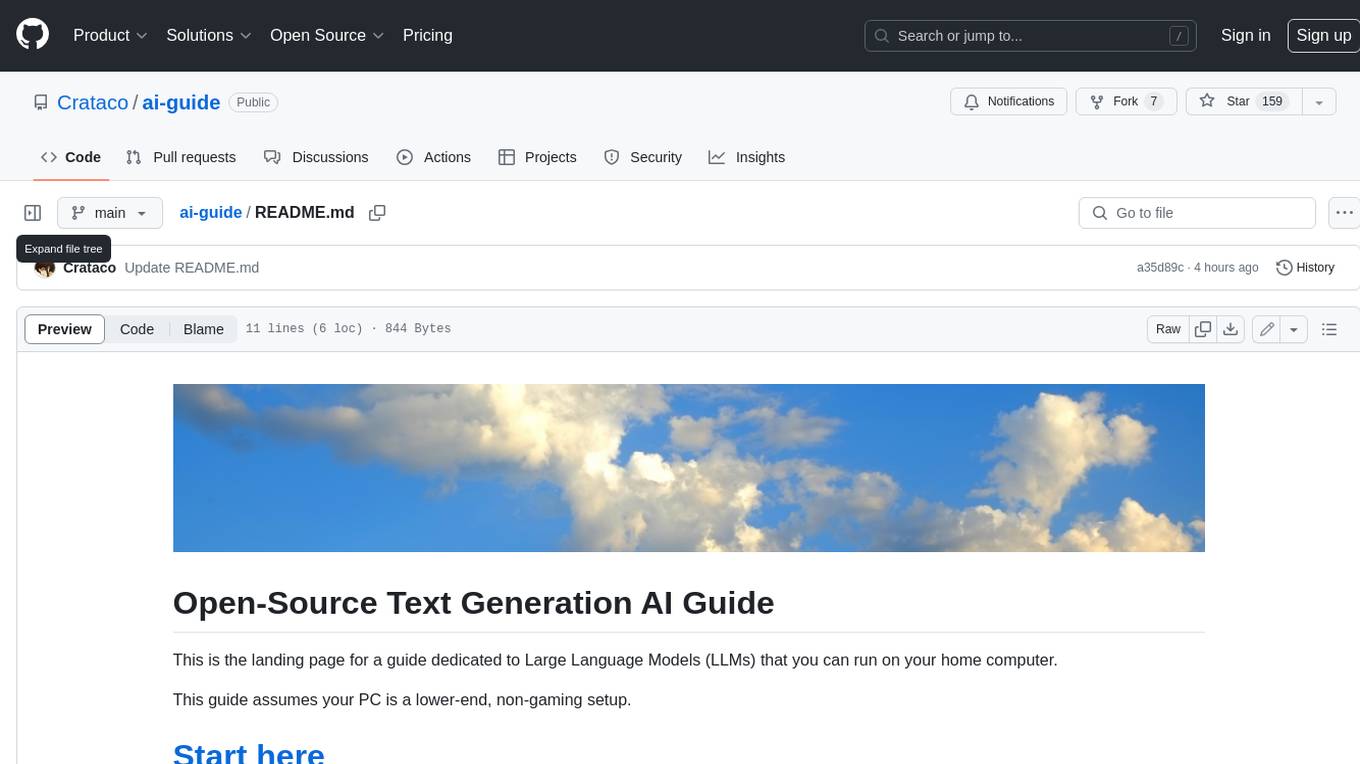
ai-guide
This guide is dedicated to Large Language Models (LLMs) that you can run on your home computer. It assumes your PC is a lower-end, non-gaming setup.
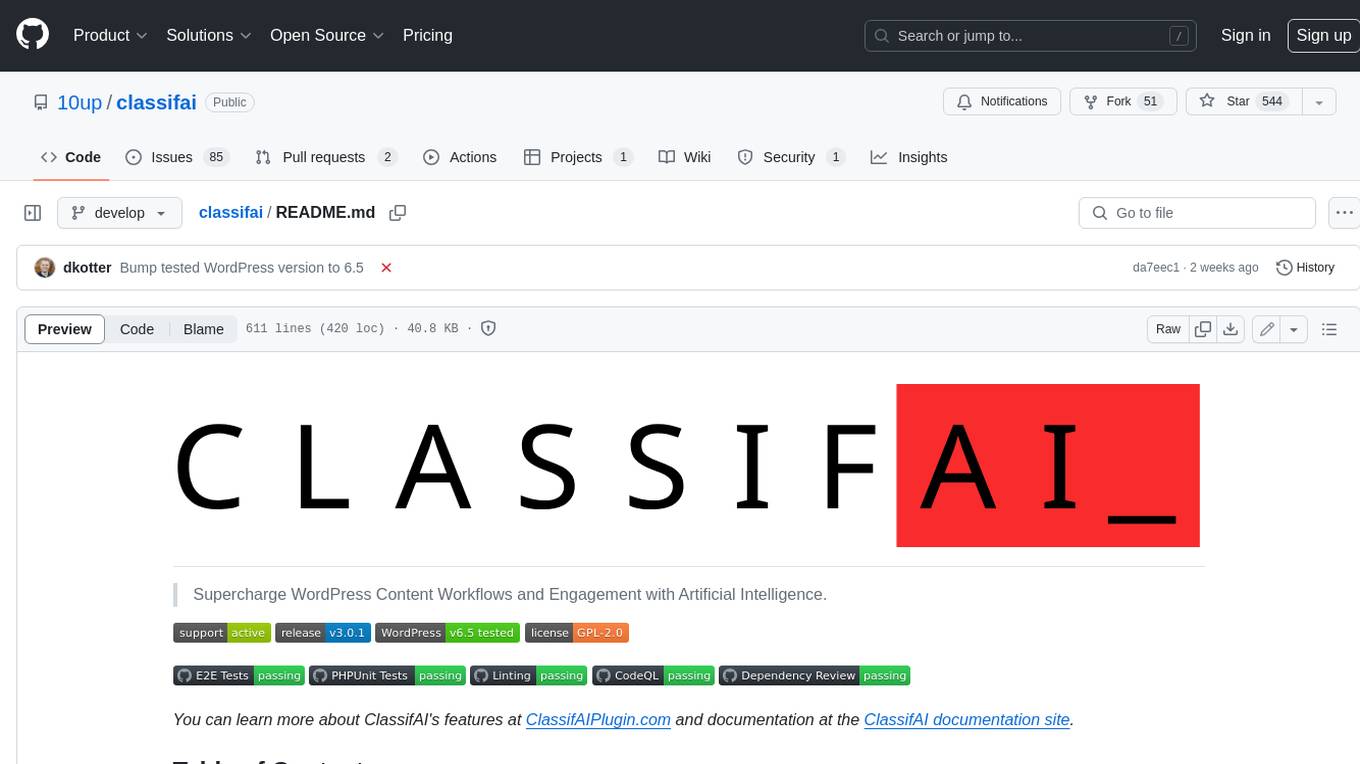
classifai
Supercharge WordPress Content Workflows and Engagement with Artificial Intelligence. Tap into leading cloud-based services like OpenAI, Microsoft Azure AI, Google Gemini and IBM Watson to augment your WordPress-powered websites. Publish content faster while improving SEO performance and increasing audience engagement. ClassifAI integrates Artificial Intelligence and Machine Learning technologies to lighten your workload and eliminate tedious tasks, giving you more time to create original content that matters.
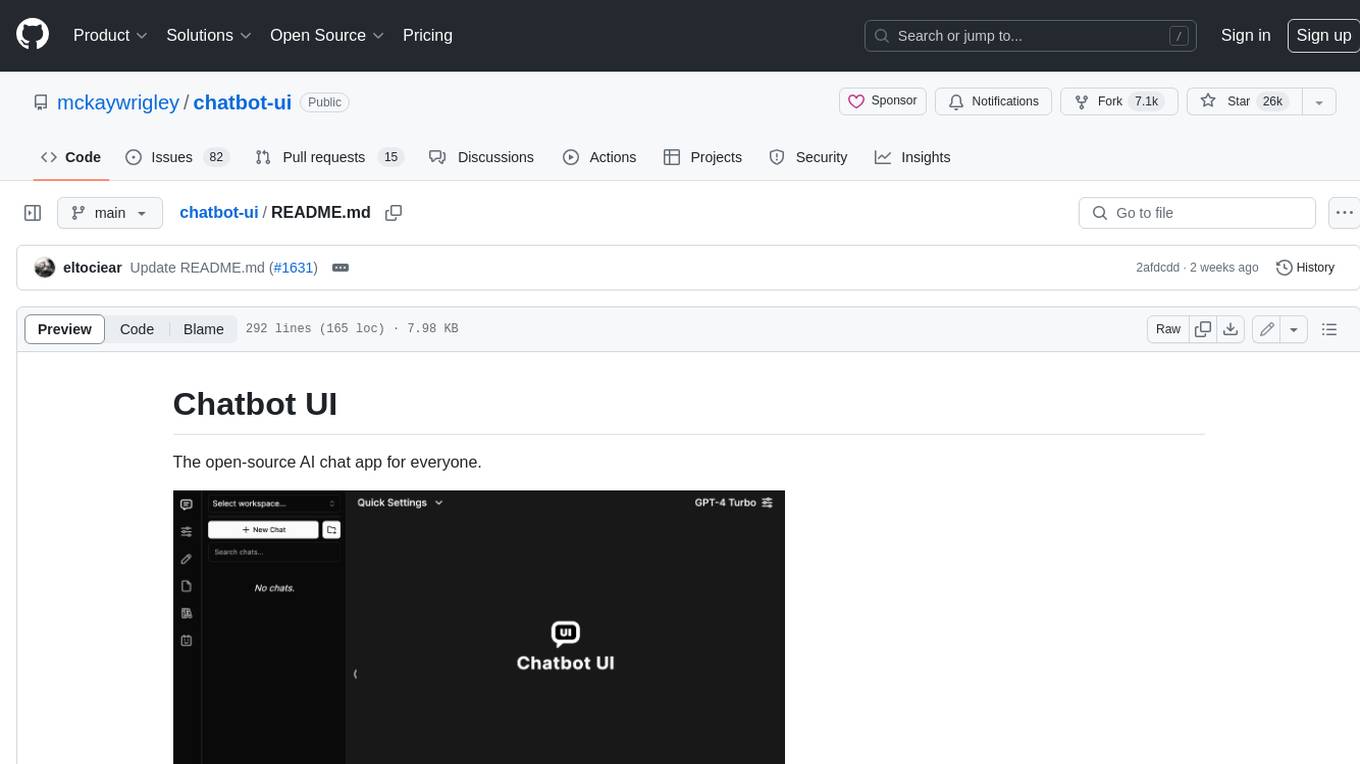
chatbot-ui
Chatbot UI is an open-source AI chat app that allows users to create and deploy their own AI chatbots. It is easy to use and can be customized to fit any need. Chatbot UI is perfect for businesses, developers, and anyone who wants to create a chatbot.
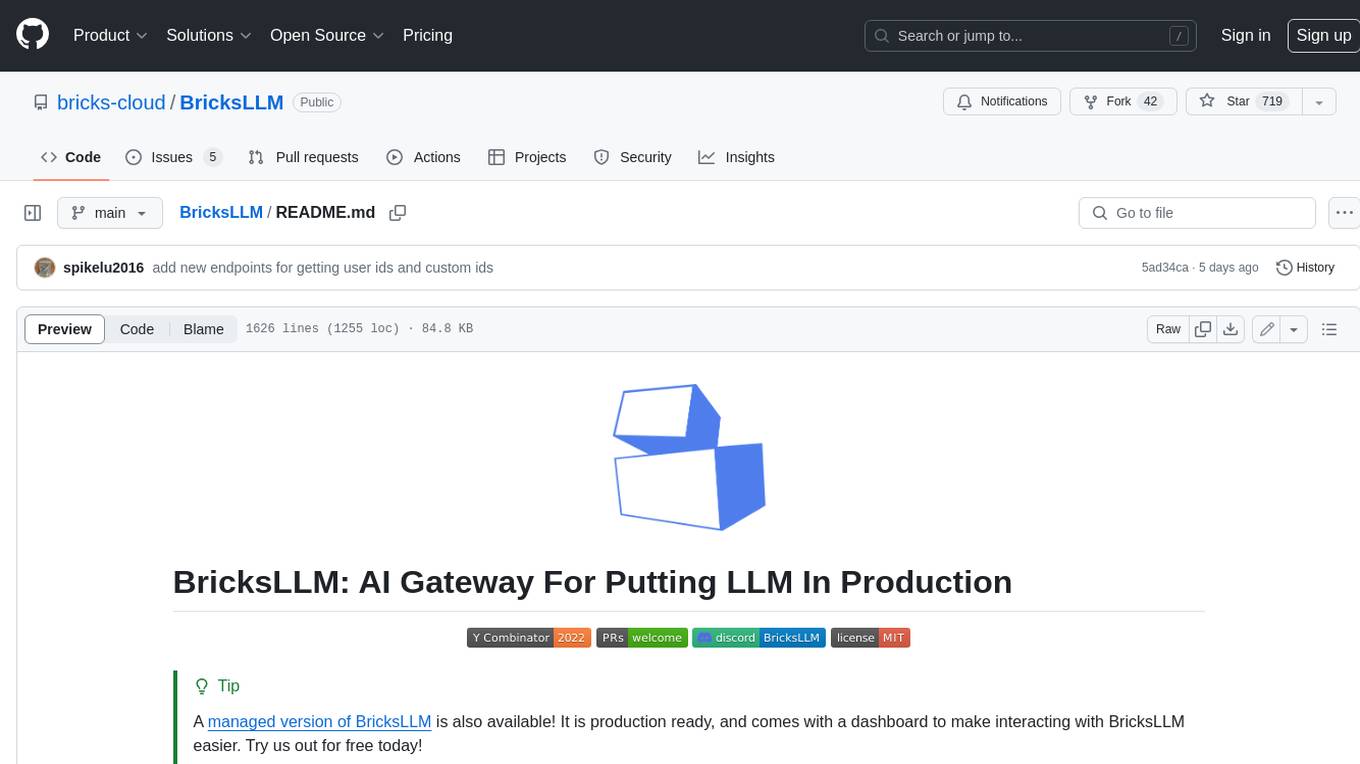
BricksLLM
BricksLLM is a cloud native AI gateway written in Go. Currently, it provides native support for OpenAI, Anthropic, Azure OpenAI and vLLM. BricksLLM aims to provide enterprise level infrastructure that can power any LLM production use cases. Here are some use cases for BricksLLM: * Set LLM usage limits for users on different pricing tiers * Track LLM usage on a per user and per organization basis * Block or redact requests containing PIIs * Improve LLM reliability with failovers, retries and caching * Distribute API keys with rate limits and cost limits for internal development/production use cases * Distribute API keys with rate limits and cost limits for students
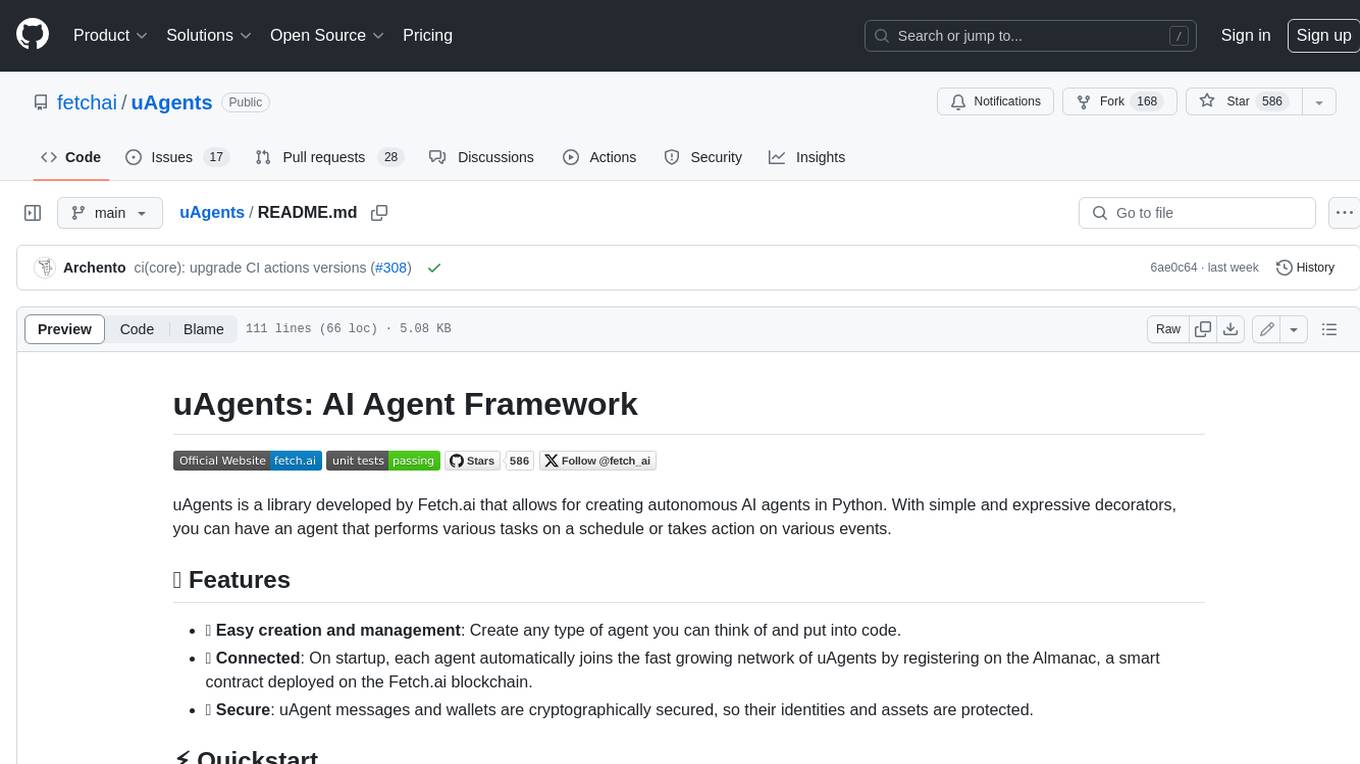
uAgents
uAgents is a Python library developed by Fetch.ai that allows for the creation of autonomous AI agents. These agents can perform various tasks on a schedule or take action on various events. uAgents are easy to create and manage, and they are connected to a fast-growing network of other uAgents. They are also secure, with cryptographically secured messages and wallets.
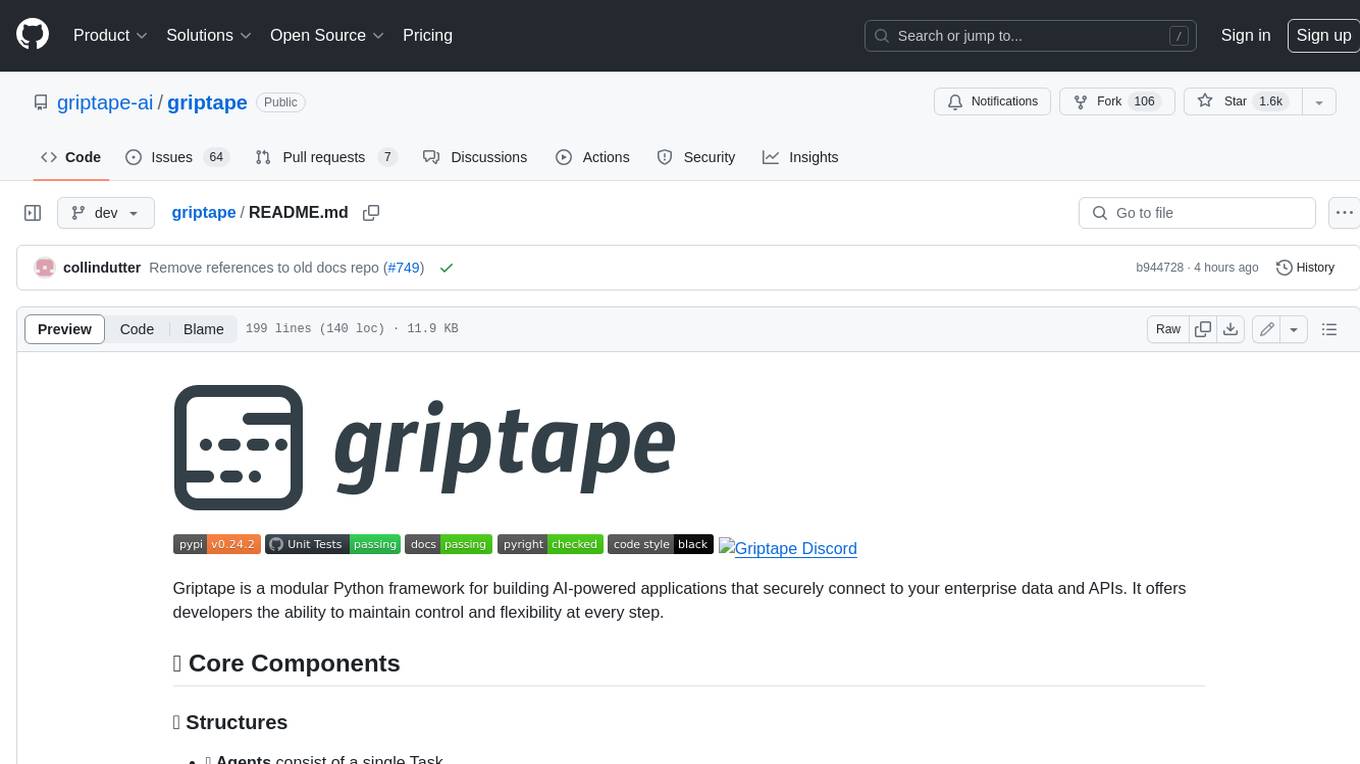
griptape
Griptape is a modular Python framework for building AI-powered applications that securely connect to your enterprise data and APIs. It offers developers the ability to maintain control and flexibility at every step. Griptape's core components include Structures (Agents, Pipelines, and Workflows), Tasks, Tools, Memory (Conversation Memory, Task Memory, and Meta Memory), Drivers (Prompt and Embedding Drivers, Vector Store Drivers, Image Generation Drivers, Image Query Drivers, SQL Drivers, Web Scraper Drivers, and Conversation Memory Drivers), Engines (Query Engines, Extraction Engines, Summary Engines, Image Generation Engines, and Image Query Engines), and additional components (Rulesets, Loaders, Artifacts, Chunkers, and Tokenizers). Griptape enables developers to create AI-powered applications with ease and efficiency.