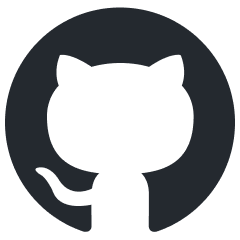
daydreams
Daydreams is a generative agent framework for executing anything onchain
Stars: 417
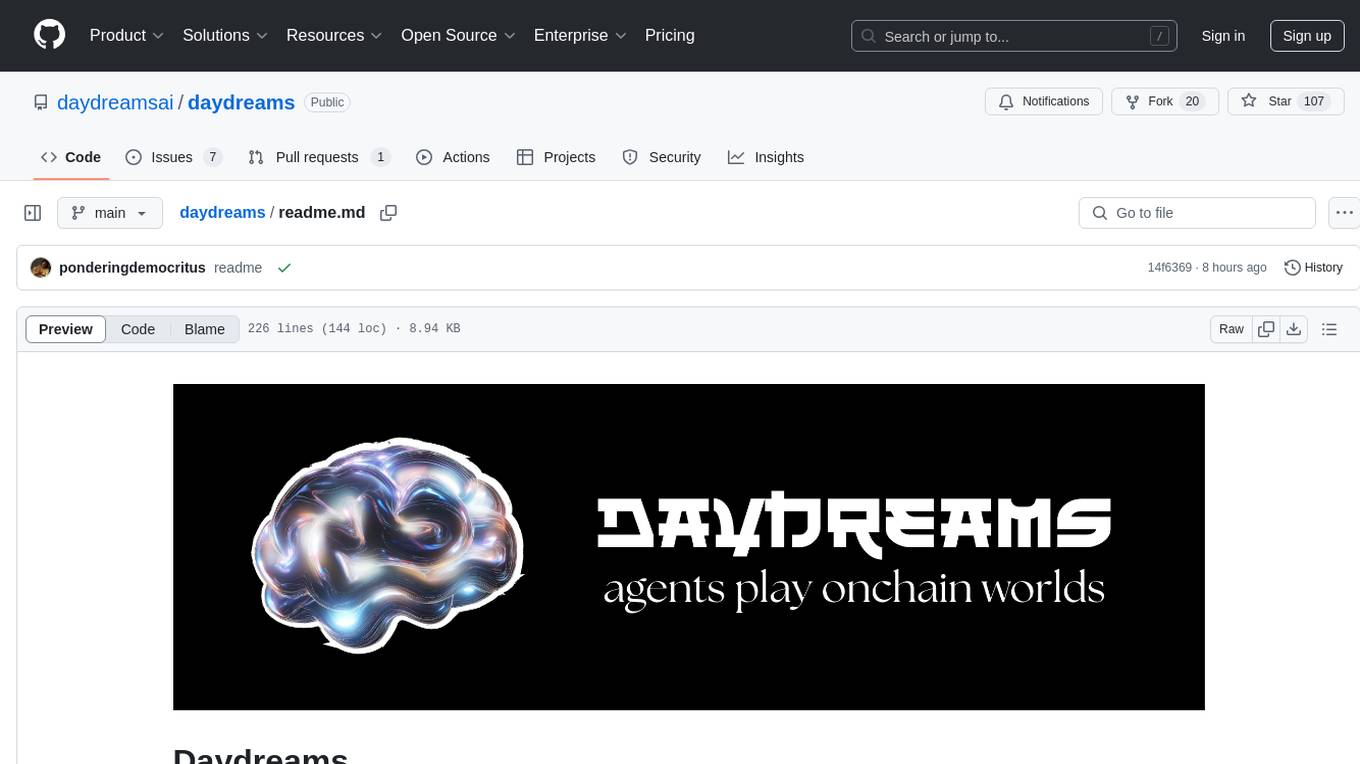
Daydreams is a generative agent library designed for playing onchain games by injecting context. It is chain agnostic and allows users to perform onchain tasks, including playing any onchain game. The tool is lightweight and powerful, enabling users to define game context, register actions, set goals, monitor progress, and integrate with external agents. Daydreams aims to be 'lite' and 'composable', dynamically generating code needed to play games. It is currently in pre-alpha stage, seeking feedback and collaboration for further development.
README:
⚠️ Warning: This is alpha software under active development. Expect frequent breaking changes and bugs. The API is not yet stable.
Daydreams is a powerful framework for building generative agents that can execute tasks across any blockchain or API.
Feature | Description |
---|---|
🔗 Chain Agnostic | Execute transactions and interact with any blockchain network |
👥 Multi-Expert System | Leverage specialized modules working together to solve complex tasks |
🧠 Context Management | Simple yet powerful memory and context handling system |
🎯 Goal-Oriented | Long-term planning and goal-oriented behavior capabilities |
💾 Persistent Memory | Built-in support for storing and retrieving long-term information |
🤔 Advanced Reasoning | Multi-step reasoning using Hierarchical Task Networks |
Want to contribute? Check our
issues for tasks labeled
good first issue
.
- Node.js 18+ using nvm
You'll need an API key for the LLM you want to use. We recommend using Groq for most use cases.
We use ai-sdk to support multiple LLMs. You will need to install the ai-sdk for the LLM you want to use.
npm i @daydreamsai/core
Read the docs for more information on how to use the agent.
We use bun for development.
bun install
bun build:packages --watch
Edit the core package and watch for changes, and play with examples.
Looking to contribute? We'd love your help!
If you are a developer and would like to contribute with code, please open an issue to discuss before opening a Pull Request.
For Tasks:
Click tags to check more tools for each tasksFor Jobs:
Alternative AI tools for daydreams
Similar Open Source Tools
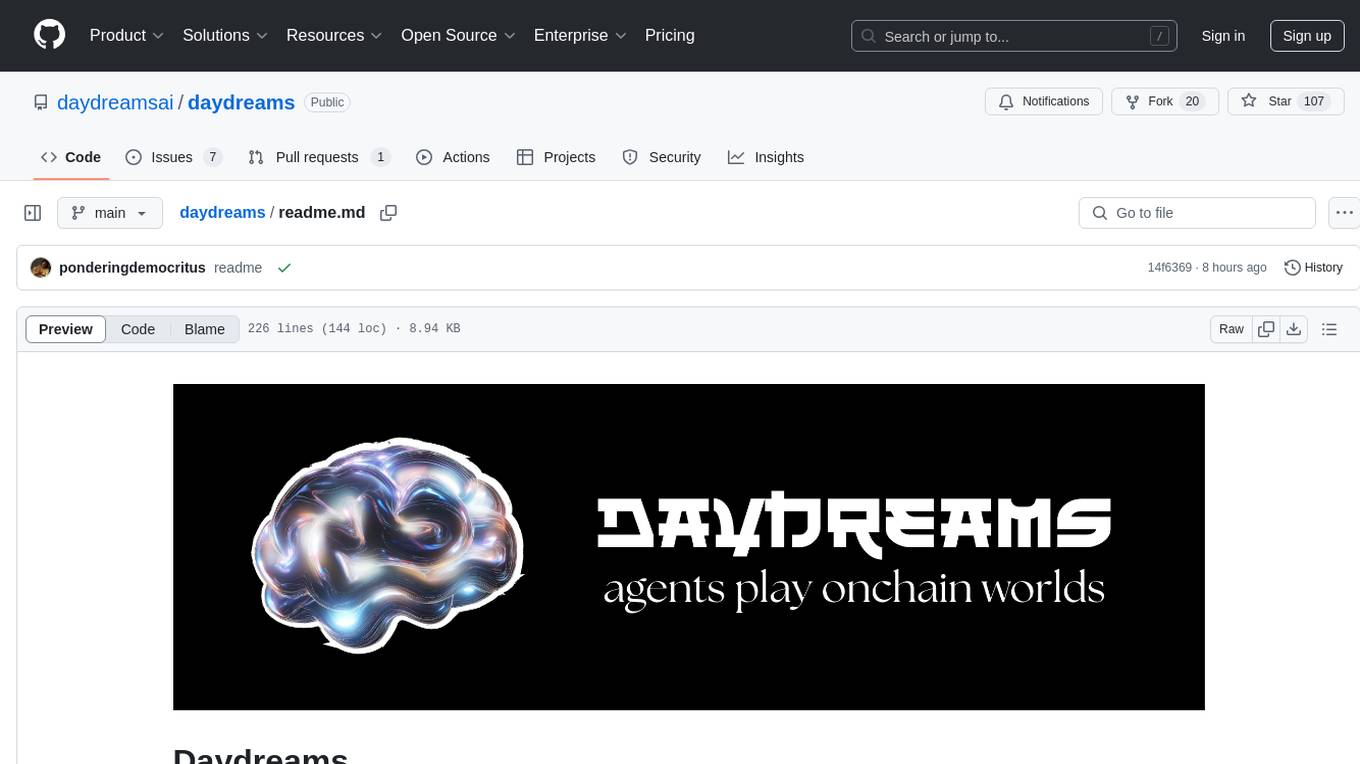
daydreams
Daydreams is a generative agent library designed for playing onchain games by injecting context. It is chain agnostic and allows users to perform onchain tasks, including playing any onchain game. The tool is lightweight and powerful, enabling users to define game context, register actions, set goals, monitor progress, and integrate with external agents. Daydreams aims to be 'lite' and 'composable', dynamically generating code needed to play games. It is currently in pre-alpha stage, seeking feedback and collaboration for further development.
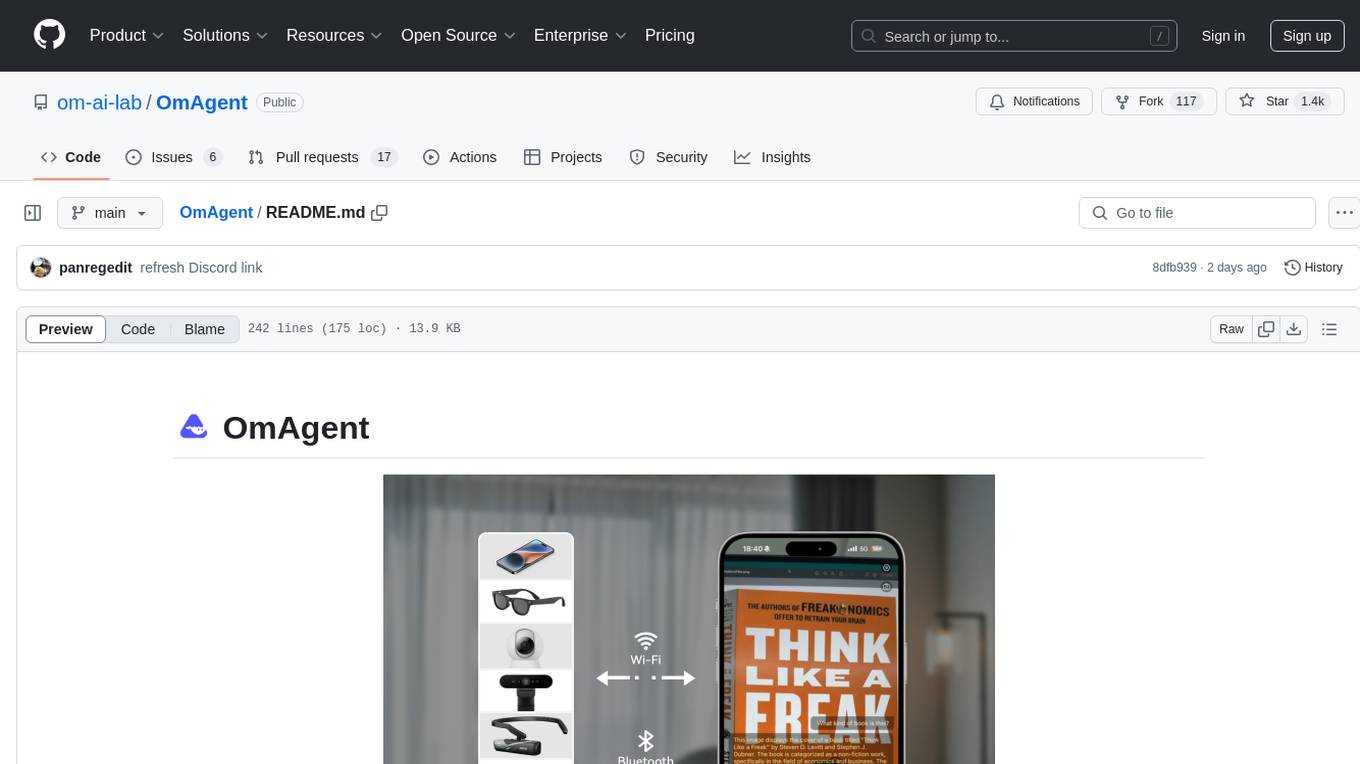
OmAgent
OmAgent is an open-source agent framework designed to streamline the development of on-device multimodal agents. It enables agents to empower various hardware devices, integrates speed-optimized SOTA multimodal models, provides SOTA multimodal agent algorithms, and focuses on optimizing the end-to-end computing pipeline for real-time user interaction experience. Key features include easy connection to diverse devices, scalability, flexibility, and workflow orchestration. The architecture emphasizes graph-based workflow orchestration, native multimodality, and device-centricity, allowing developers to create bespoke intelligent agent programs.
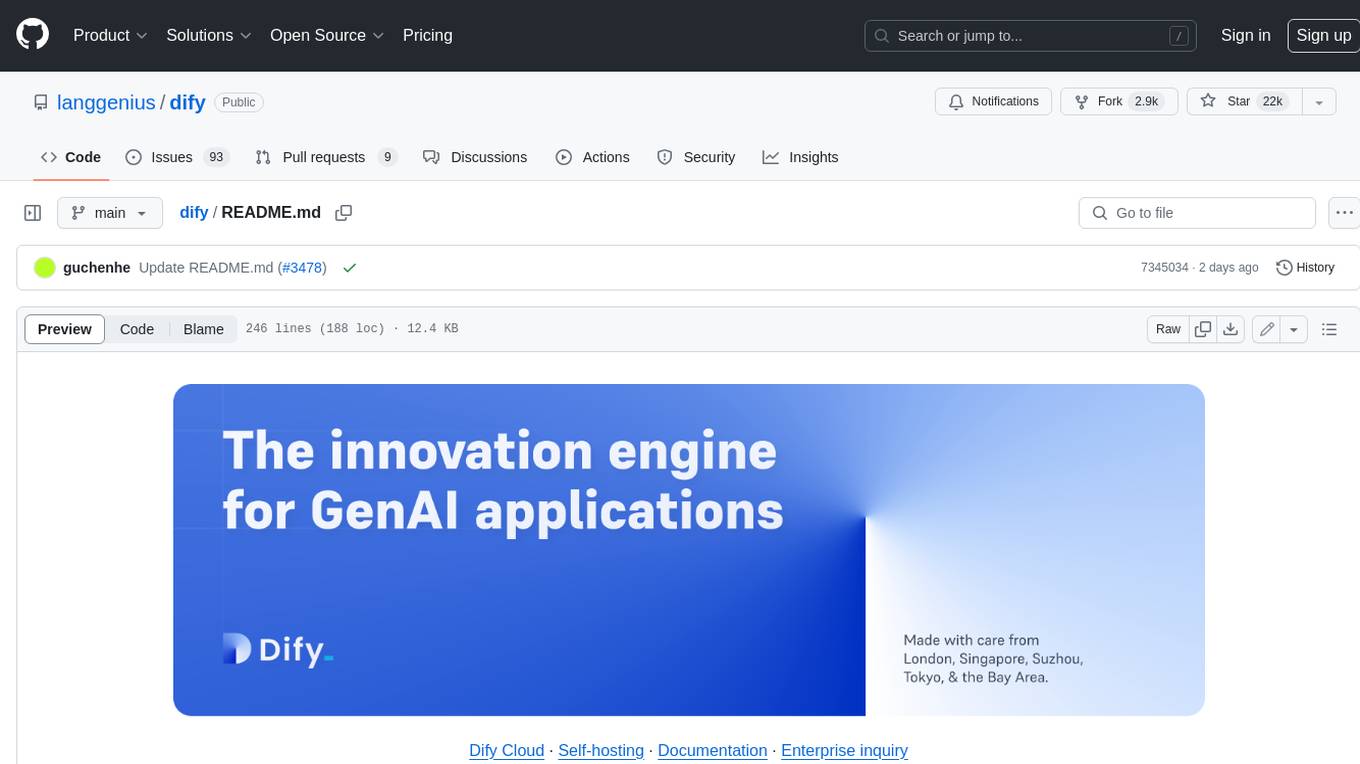
dify
Dify is an open-source LLM app development platform that combines AI workflow, RAG pipeline, agent capabilities, model management, observability features, and more. It allows users to quickly go from prototype to production. Key features include: 1. Workflow: Build and test powerful AI workflows on a visual canvas. 2. Comprehensive model support: Seamless integration with hundreds of proprietary / open-source LLMs from dozens of inference providers and self-hosted solutions. 3. Prompt IDE: Intuitive interface for crafting prompts, comparing model performance, and adding additional features. 4. RAG Pipeline: Extensive RAG capabilities that cover everything from document ingestion to retrieval. 5. Agent capabilities: Define agents based on LLM Function Calling or ReAct, and add pre-built or custom tools. 6. LLMOps: Monitor and analyze application logs and performance over time. 7. Backend-as-a-Service: All of Dify's offerings come with corresponding APIs for easy integration into your own business logic.
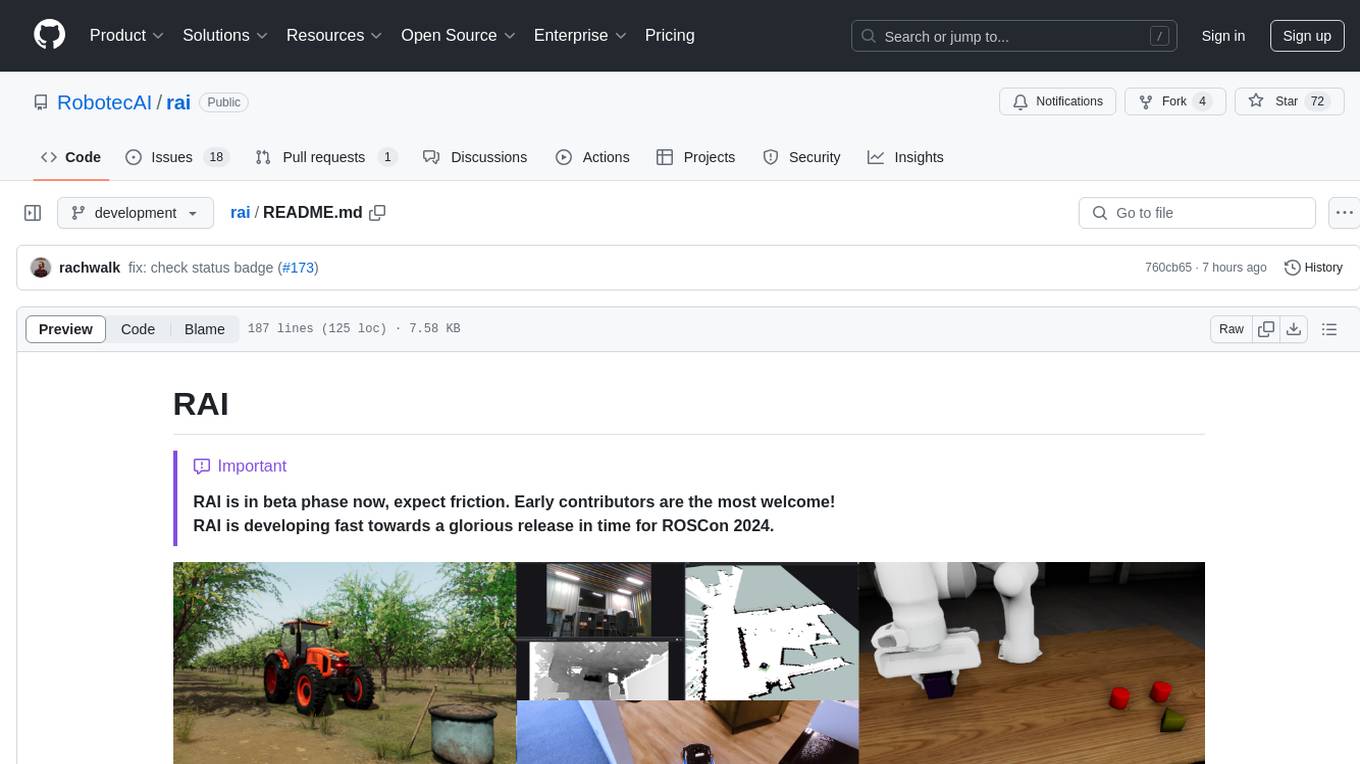
rai
RAI is a framework designed to bring general multi-agent system capabilities to robots, enhancing human interactivity, flexibility in problem-solving, and out-of-the-box AI features. It supports multi-modalities, incorporates an advanced database for agent memory, provides ROS 2-oriented tooling, and offers a comprehensive task/mission orchestrator. The framework includes features such as voice interaction, customizable robot identity, camera sensor access, reasoning through ROS logs, and integration with LangChain for AI tools. RAI aims to support various AI vendors, improve human-robot interaction, provide an SDK for developers, and offer a user interface for configuration.
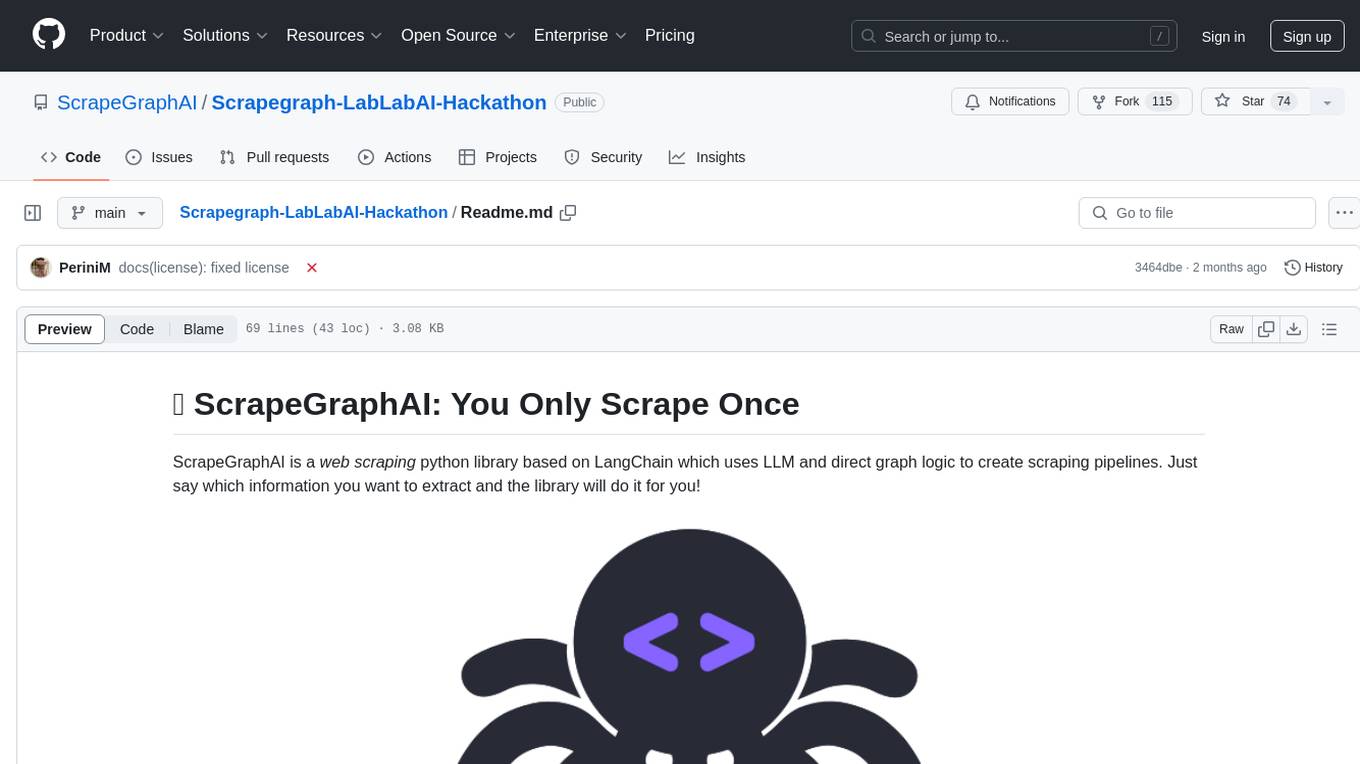
Scrapegraph-LabLabAI-Hackathon
ScrapeGraphAI is a web scraping Python library that utilizes LangChain, LLM, and direct graph logic to create scraping pipelines. Users can specify the information they want to extract, and the library will handle the extraction process. The tool is designed to simplify web scraping tasks by providing a streamlined and efficient approach to data extraction.
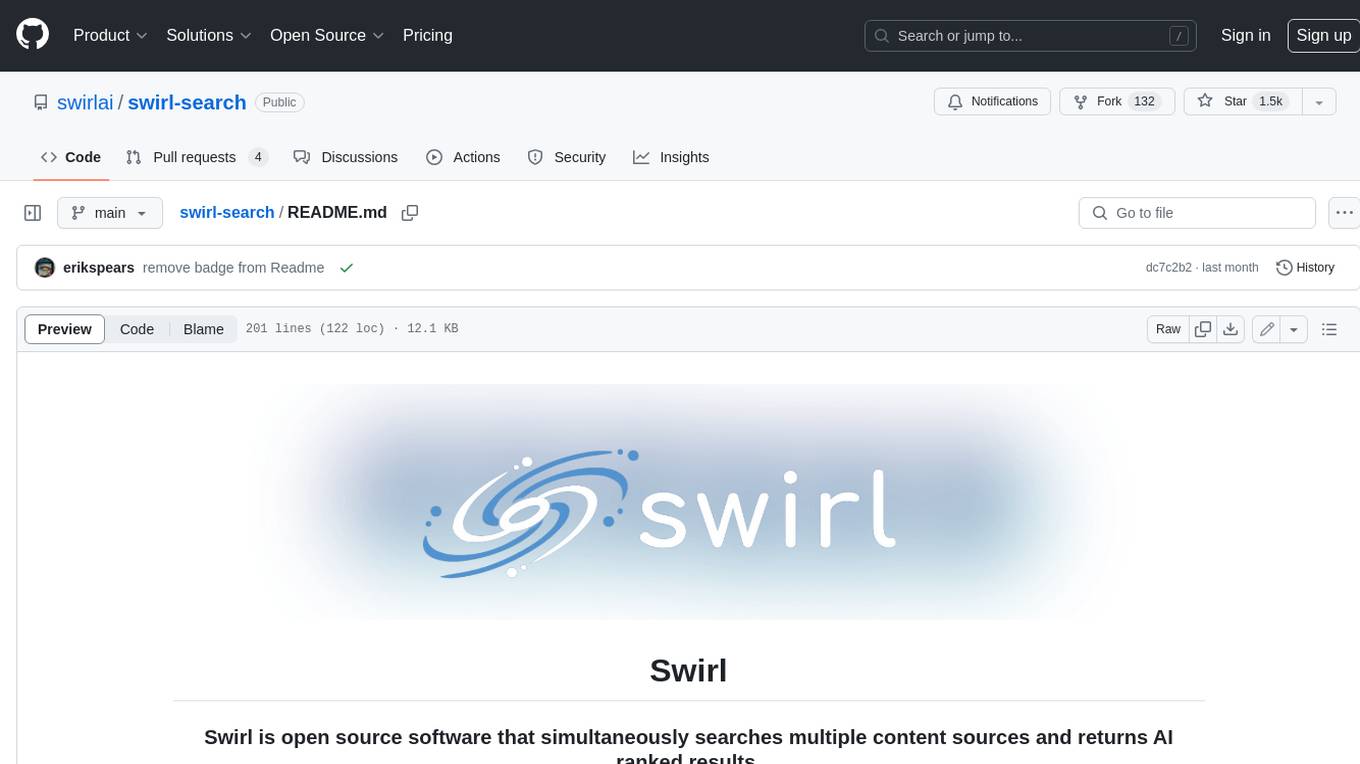
swirl-search
Swirl is an open-source software that allows users to simultaneously search multiple content sources and receive AI-ranked results. It connects to various data sources, including databases, public data services, and enterprise sources, and utilizes AI and LLMs to generate insights and answers based on the user's data. Swirl is easy to use, requiring only the download of a YML file, starting in Docker, and searching with Swirl. Users can add credentials to preloaded SearchProviders to access more sources. Swirl also offers integration with ChatGPT as a configured AI model. It adapts and distributes user queries to anything with a search API, re-ranking the unified results using Large Language Models without extracting or indexing anything. Swirl includes five Google Programmable Search Engines (PSEs) to get users up and running quickly. Key features of Swirl include Microsoft 365 integration, SearchProvider configurations, query adaptation, synchronous or asynchronous search federation, optional subscribe feature, pipelining of Processor stages, results stored in SQLite3 or PostgreSQL, built-in Query Transformation support, matching on word stems and handling of stopwords, duplicate detection, re-ranking of unified results using Cosine Vector Similarity, result mixers, page through all results requested, sample data sets, optional spell correction, optional search/result expiration service, easily extensible Connector and Mixer objects, and a welcoming community for collaboration and support.
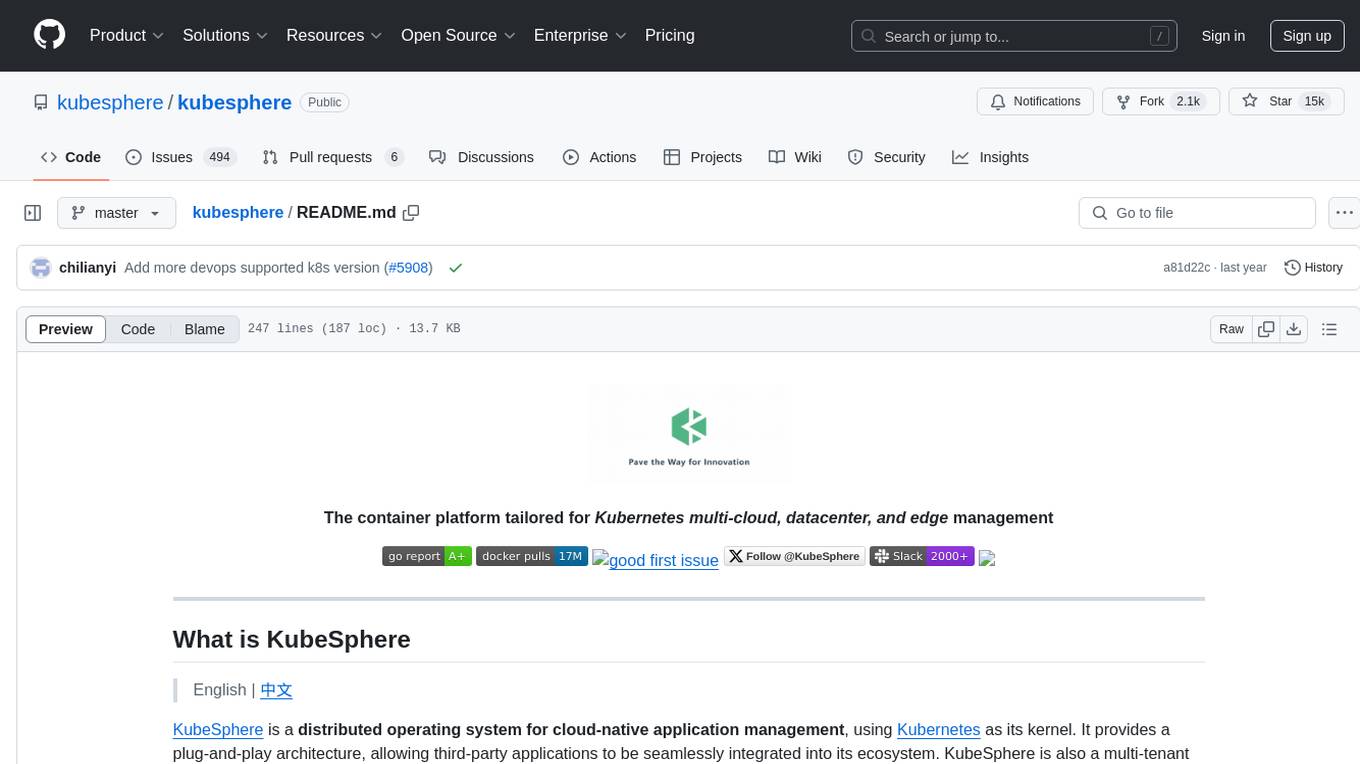
kubesphere
KubeSphere is a distributed operating system for cloud-native application management, using Kubernetes as its kernel. It provides a plug-and-play architecture, allowing third-party applications to be seamlessly integrated into its ecosystem. KubeSphere is also a multi-tenant container platform with full-stack automated IT operation and streamlined DevOps workflows. It provides developer-friendly wizard web UI, helping enterprises to build out a more robust and feature-rich platform, which includes most common functionalities needed for enterprise Kubernetes strategy.
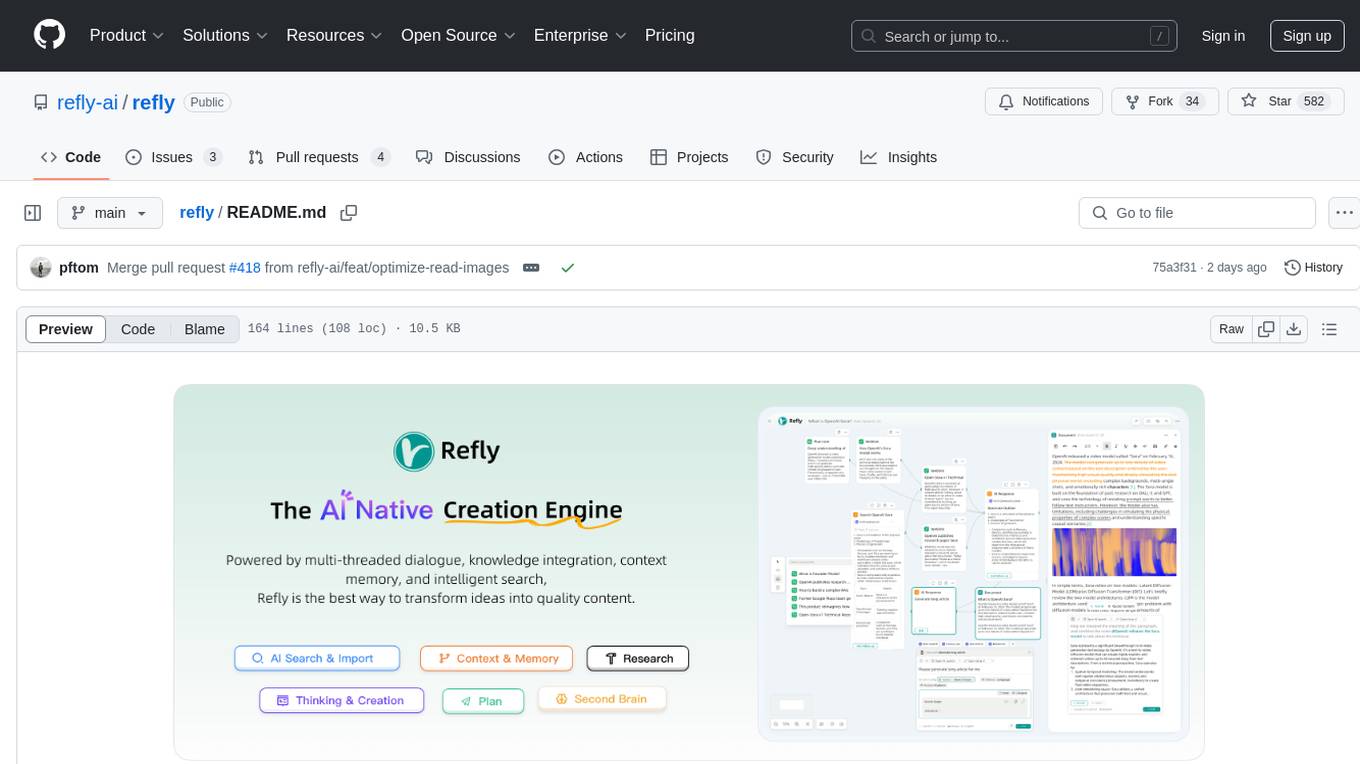
refly
Refly.AI is an open-source AI-native creation engine that empowers users to transform ideas into production-ready content. It features a free-form canvas interface with multi-threaded conversations, knowledge base integration, contextual memory, intelligent search, WYSIWYG AI editor, and more. Users can leverage AI-powered capabilities, context memory, knowledge base integration, quotes, and AI document editing to enhance their content creation process. Refly offers both cloud and self-hosting options, making it suitable for individuals, enterprises, and organizations. The tool is designed to facilitate human-AI collaboration and streamline content creation workflows.
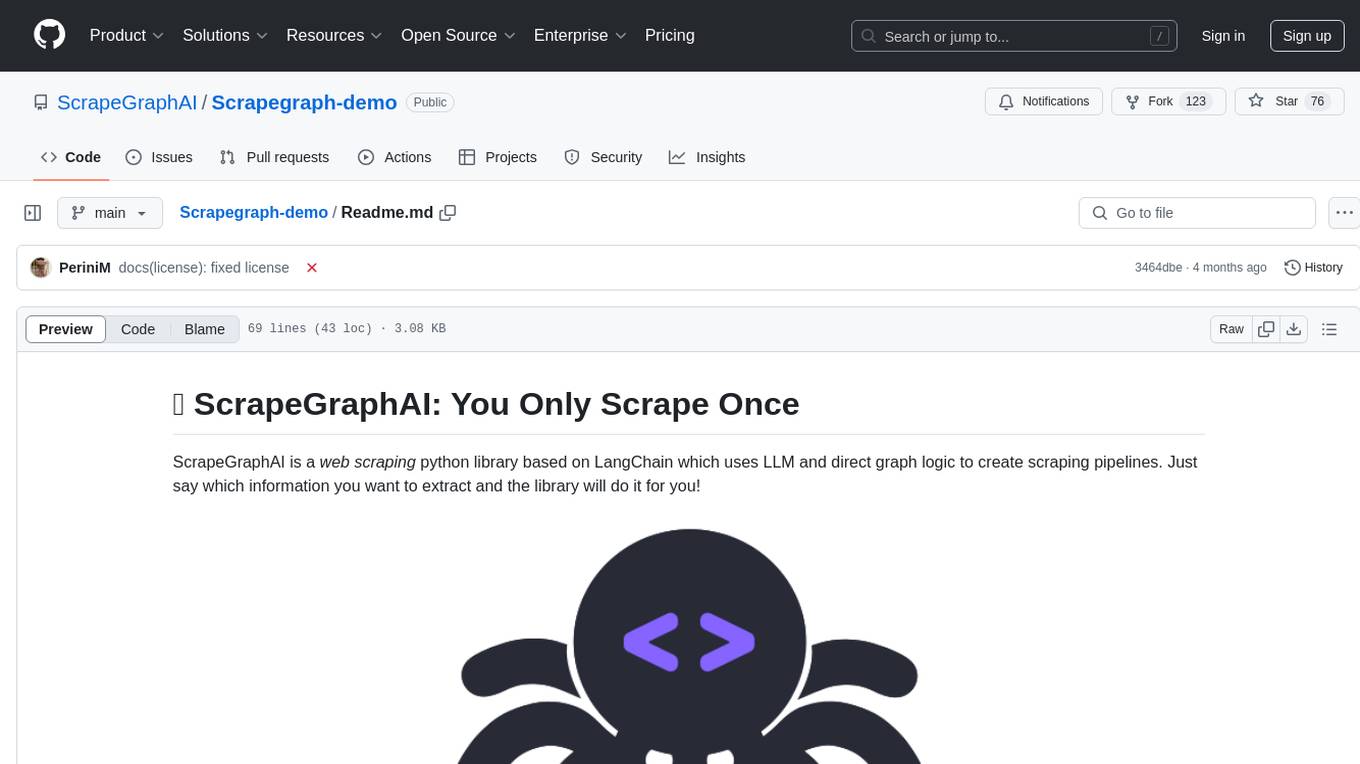
Scrapegraph-demo
ScrapeGraphAI is a web scraping Python library that utilizes LangChain, LLM, and direct graph logic to create scraping pipelines. Users can specify the information they want to extract, and the library will handle the extraction process. This repository contains an official demo/trial for the ScrapeGraphAI library, showcasing its capabilities in web scraping tasks. The tool is designed to simplify the process of extracting data from websites by providing a user-friendly interface and powerful scraping functionalities.
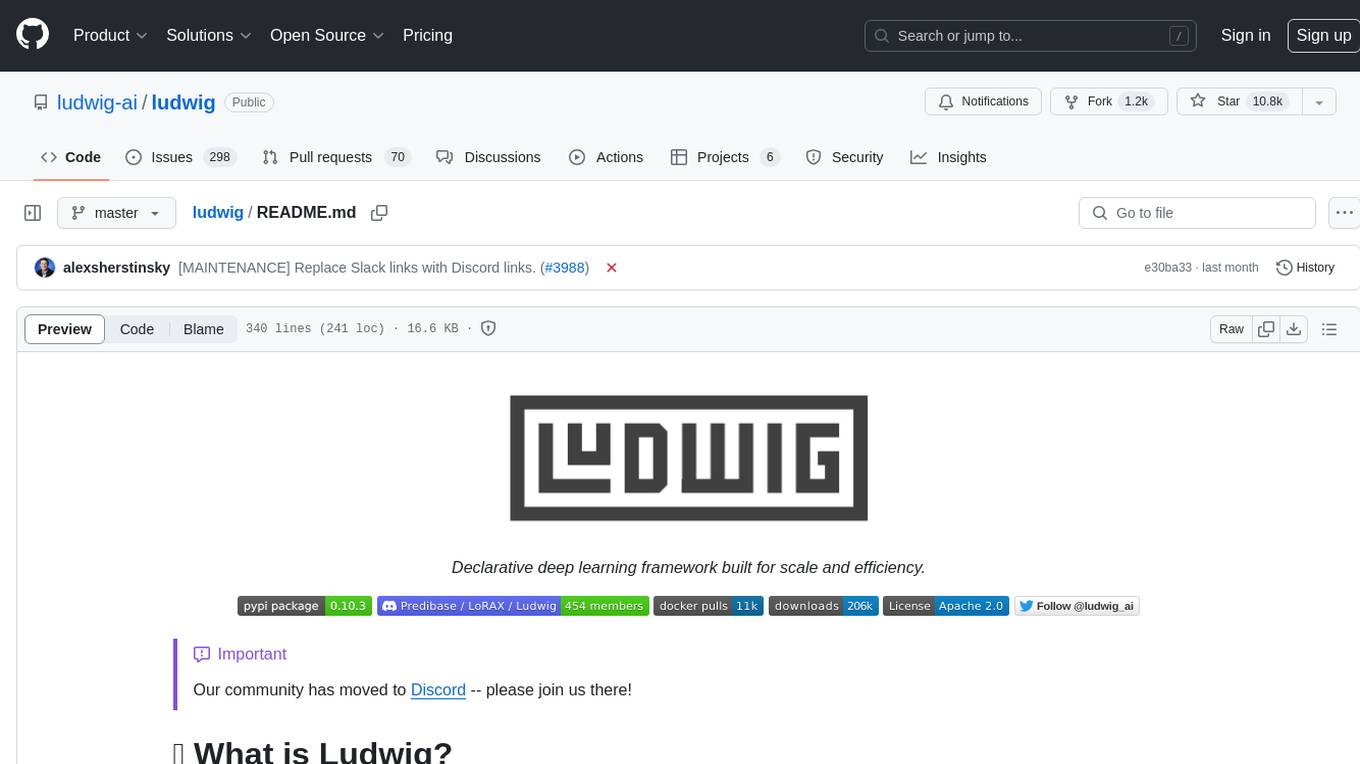
ludwig
Ludwig is a declarative deep learning framework designed for scale and efficiency. It is a low-code framework that allows users to build custom AI models like LLMs and other deep neural networks with ease. Ludwig offers features such as optimized scale and efficiency, expert level control, modularity, and extensibility. It is engineered for production with prebuilt Docker containers, support for running with Ray on Kubernetes, and the ability to export models to Torchscript and Triton. Ludwig is hosted by the Linux Foundation AI & Data.
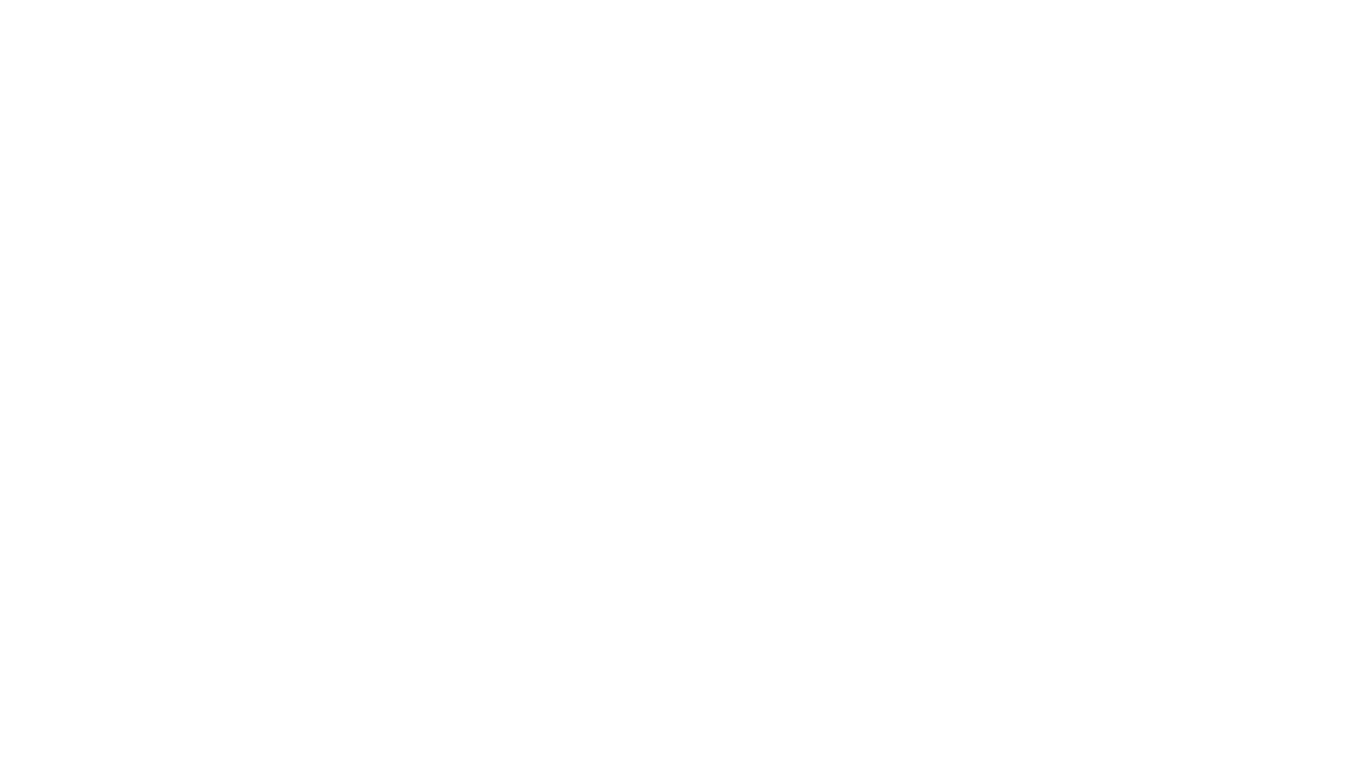
second-brain-ai-assistant-course
This open-source course teaches how to build an advanced RAG and LLM system using LLMOps and ML systems best practices. It helps you create an AI assistant that leverages your personal knowledge base to answer questions, summarize documents, and provide insights. The course covers topics such as LLM system architecture, pipeline orchestration, large-scale web crawling, model fine-tuning, and advanced RAG features. It is suitable for ML/AI engineers and data/software engineers & data scientists looking to level up to production AI systems. The course is free, with minimal costs for tools like OpenAI's API and Hugging Face's Dedicated Endpoints. Participants will build two separate Python applications for offline ML pipelines and online inference pipeline.
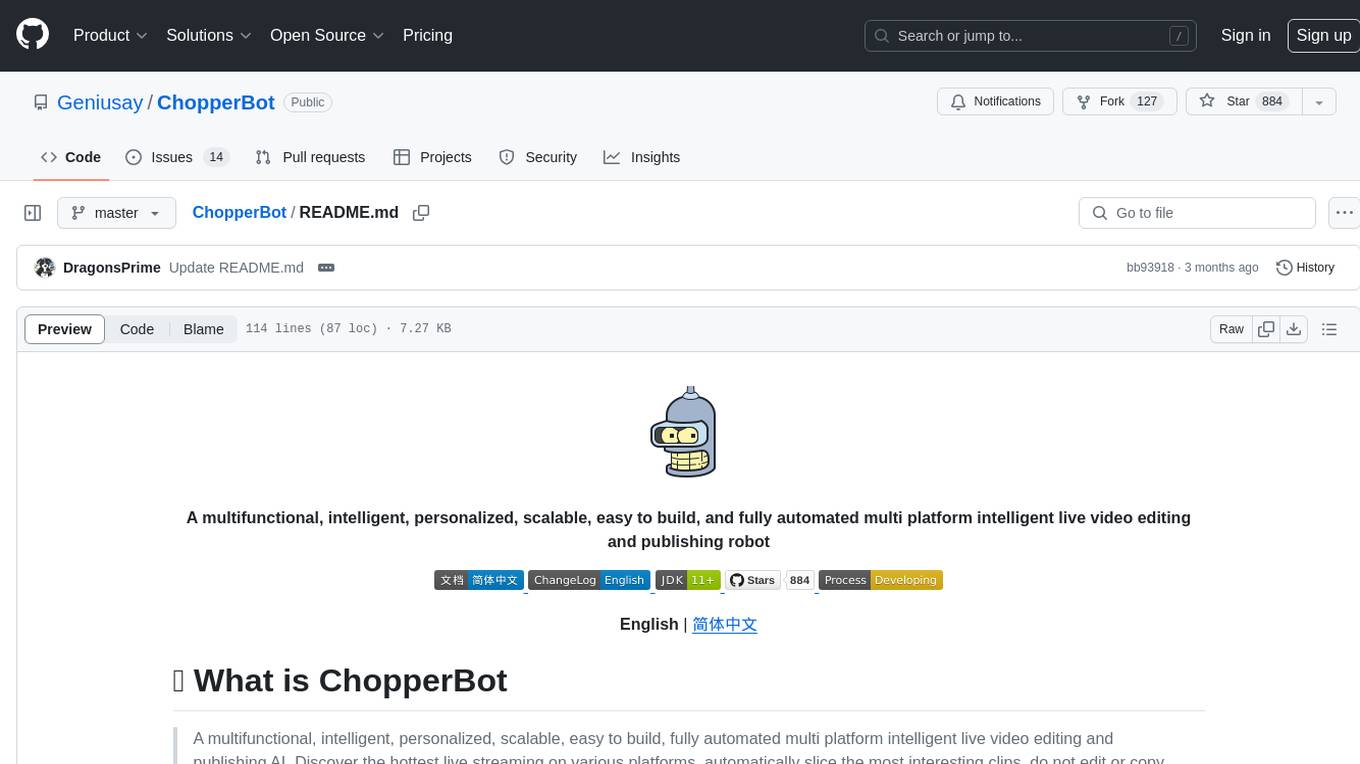
ChopperBot
A multifunctional, intelligent, personalized, scalable, easy to build, and fully automated multi platform intelligent live video editing and publishing robot. ChopperBot is a comprehensive AI tool that automatically analyzes and slices the most interesting clips from popular live streaming platforms, generates and publishes content, and manages accounts. It supports plugin DIY development and hot swapping functionality, making it easy to customize and expand. With ChopperBot, users can quickly build their own live video editing platform without the need to install any software, thanks to its visual management interface.
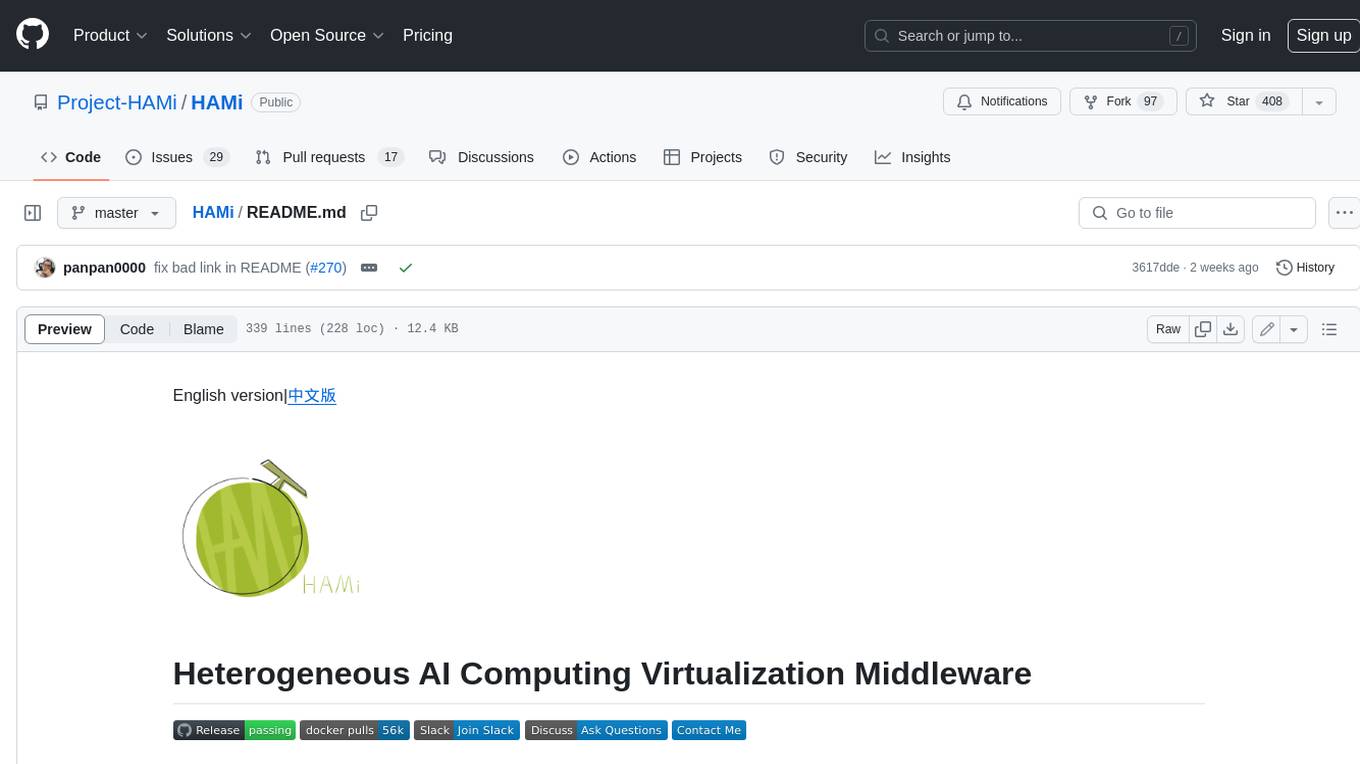
HAMi
HAMi is a Heterogeneous AI Computing Virtualization Middleware designed to manage Heterogeneous AI Computing Devices in a Kubernetes cluster. It allows for device sharing, device memory control, device type specification, and device UUID specification. The tool is easy to use and does not require modifying task YAML files. It includes features like hard limits on device memory, partial device allocation, streaming multiprocessor limits, and core usage specification. HAMi consists of components like a mutating webhook, scheduler extender, device plugins, and in-container virtualization techniques. It is suitable for scenarios requiring device sharing, specific device memory allocation, GPU balancing, low utilization optimization, and scenarios needing multiple small GPUs. The tool requires prerequisites like NVIDIA drivers, CUDA version, nvidia-docker, Kubernetes version, glibc version, and helm. Users can install, upgrade, and uninstall HAMi, submit tasks, and monitor cluster information. The tool's roadmap includes supporting additional AI computing devices, video codec processing, and Multi-Instance GPUs (MIG).
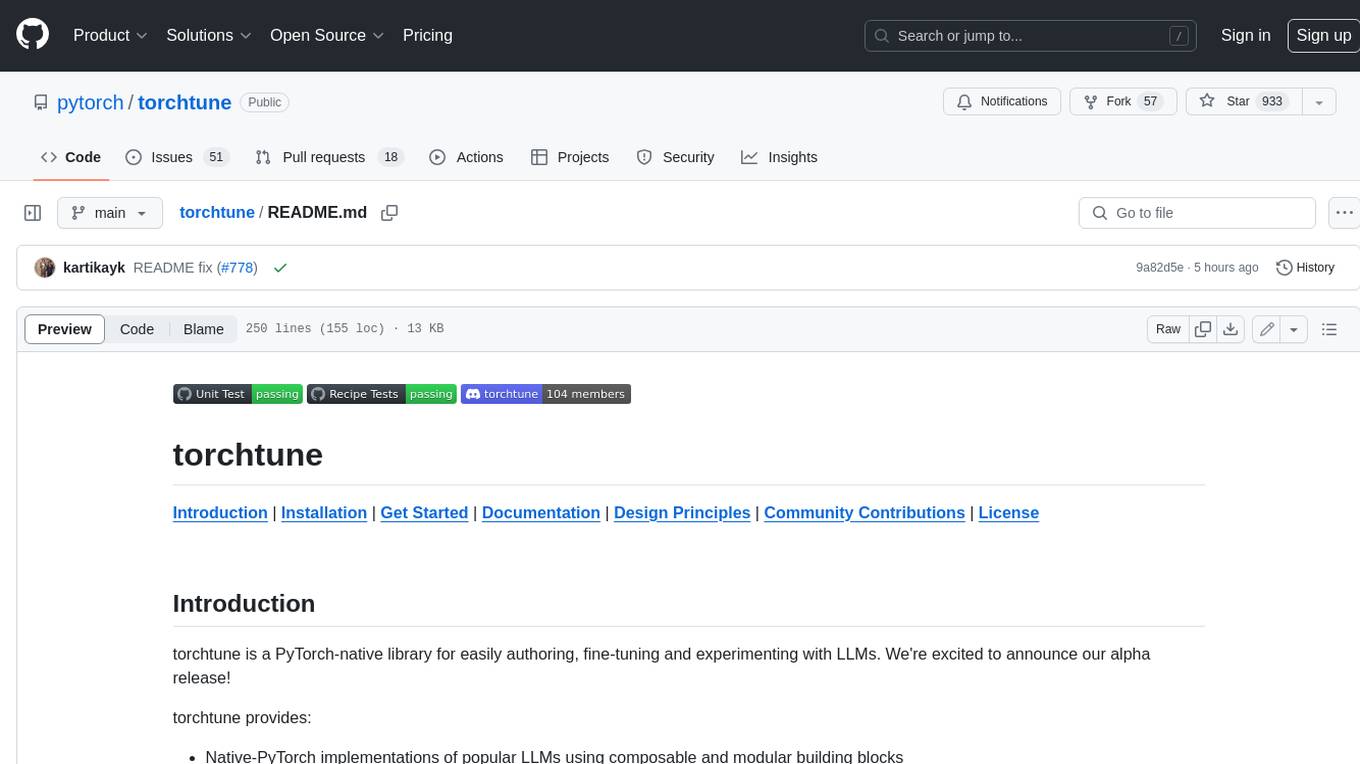
torchtune
Torchtune is a PyTorch-native library for easily authoring, fine-tuning, and experimenting with LLMs. It provides native-PyTorch implementations of popular LLMs using composable and modular building blocks, easy-to-use and hackable training recipes for popular fine-tuning techniques, YAML configs for easily configuring training, evaluation, quantization, or inference recipes, and built-in support for many popular dataset formats and prompt templates to help you quickly get started with training.
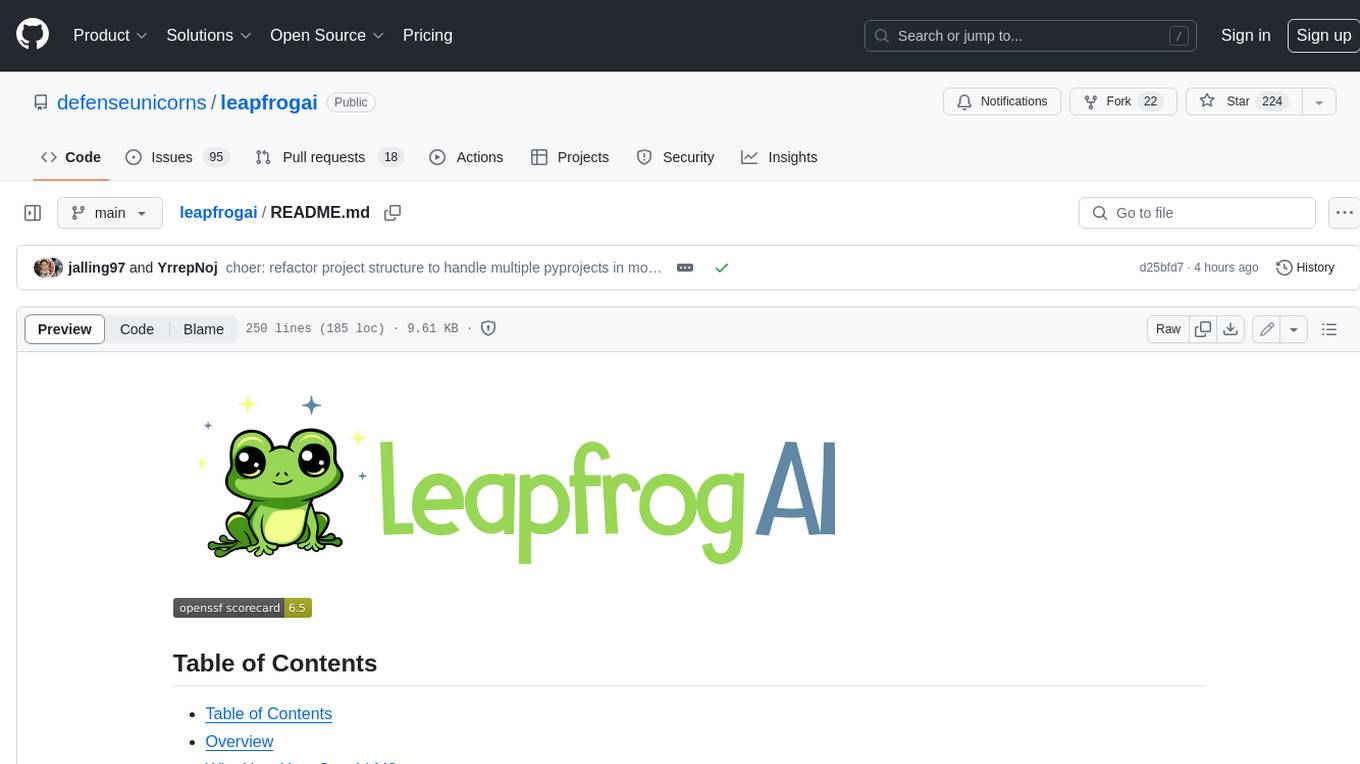
leapfrogai
LeapfrogAI is a self-hosted AI platform designed to be deployed in air-gapped resource-constrained environments. It brings sophisticated AI solutions to these environments by hosting all the necessary components of an AI stack, including vector databases, model backends, API, and UI. LeapfrogAI's API closely matches that of OpenAI, allowing tools built for OpenAI/ChatGPT to function seamlessly with a LeapfrogAI backend. It provides several backends for various use cases, including llama-cpp-python, whisper, text-embeddings, and vllm. LeapfrogAI leverages Chainguard's apko to harden base python images, ensuring the latest supported Python versions are used by the other components of the stack. The LeapfrogAI SDK provides a standard set of protobuffs and python utilities for implementing backends and gRPC. LeapfrogAI offers UI options for common use-cases like chat, summarization, and transcription. It can be deployed and run locally via UDS and Kubernetes, built out using Zarf packages. LeapfrogAI is supported by a community of users and contributors, including Defense Unicorns, Beast Code, Chainguard, Exovera, Hypergiant, Pulze, SOSi, United States Navy, United States Air Force, and United States Space Force.
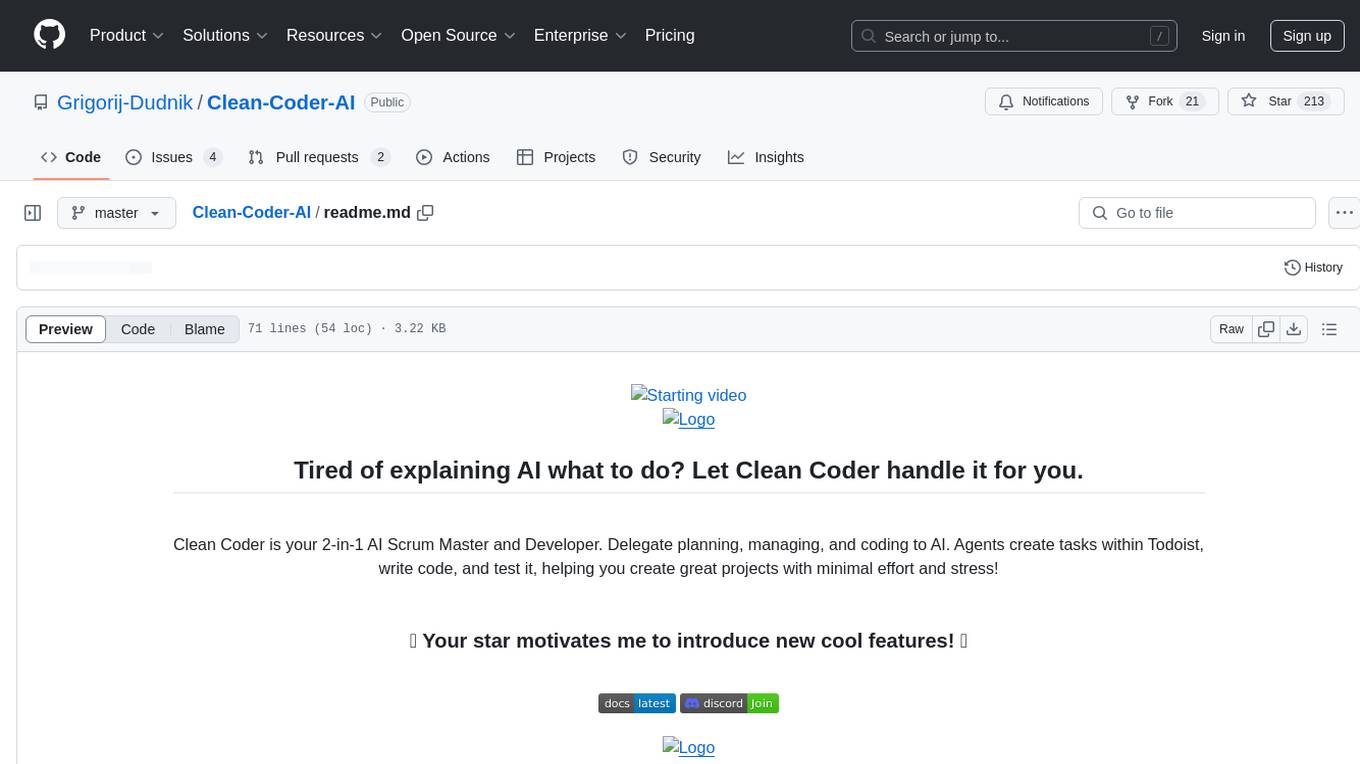
Clean-Coder-AI
Clean Coder is an AI tool that serves as a 2-in-1 Scrum Master and Developer. It helps users delegate planning, managing, and coding tasks to AI agents. These agents create tasks within Todoist, write code, and test it, enabling users to work on projects with minimal effort and stress. The tool offers features like project supervision, task execution by programming agents, frontend feedback, automatic file linting, file researcher agent, and sensitive files protection. Users can interact with Clean Coder through speech and benefit from advanced techniques for intelligent task execution.
For similar tasks
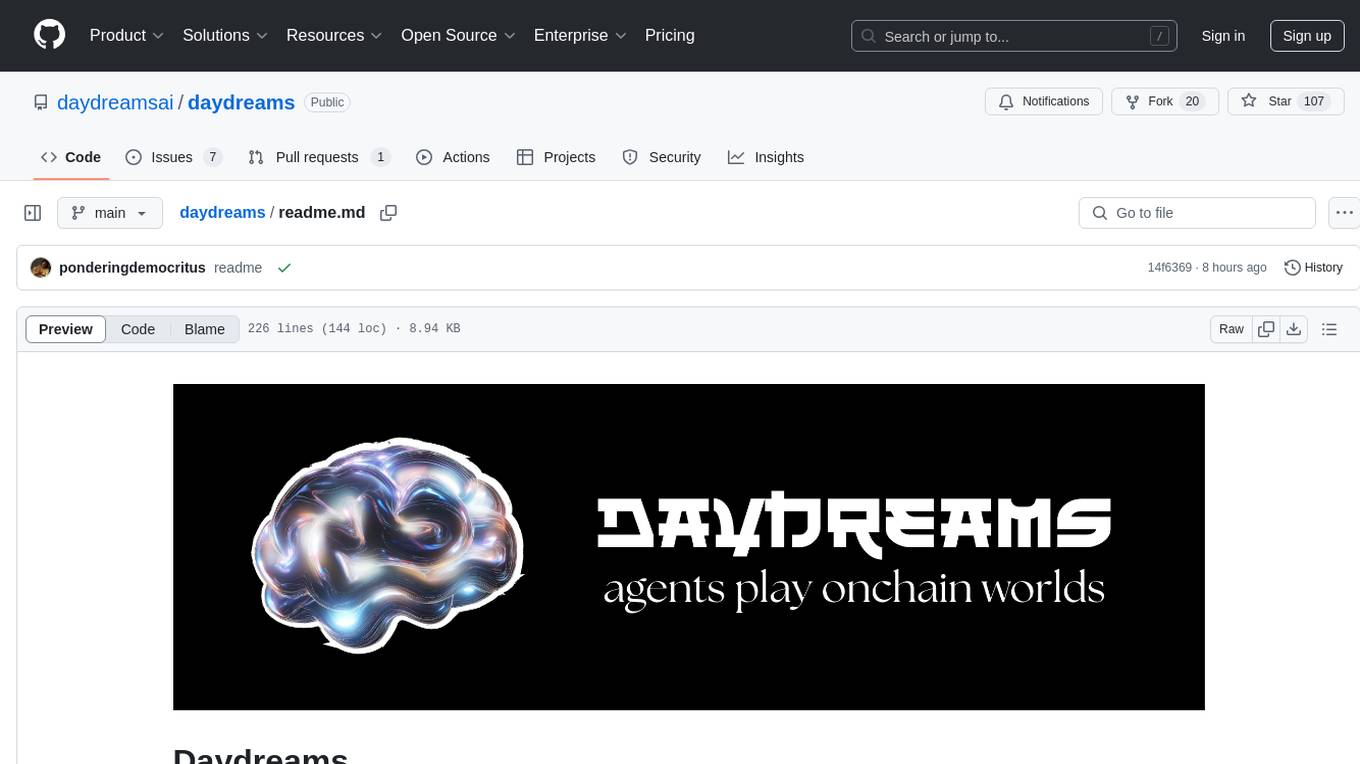
daydreams
Daydreams is a generative agent library designed for playing onchain games by injecting context. It is chain agnostic and allows users to perform onchain tasks, including playing any onchain game. The tool is lightweight and powerful, enabling users to define game context, register actions, set goals, monitor progress, and integrate with external agents. Daydreams aims to be 'lite' and 'composable', dynamically generating code needed to play games. It is currently in pre-alpha stage, seeking feedback and collaboration for further development.
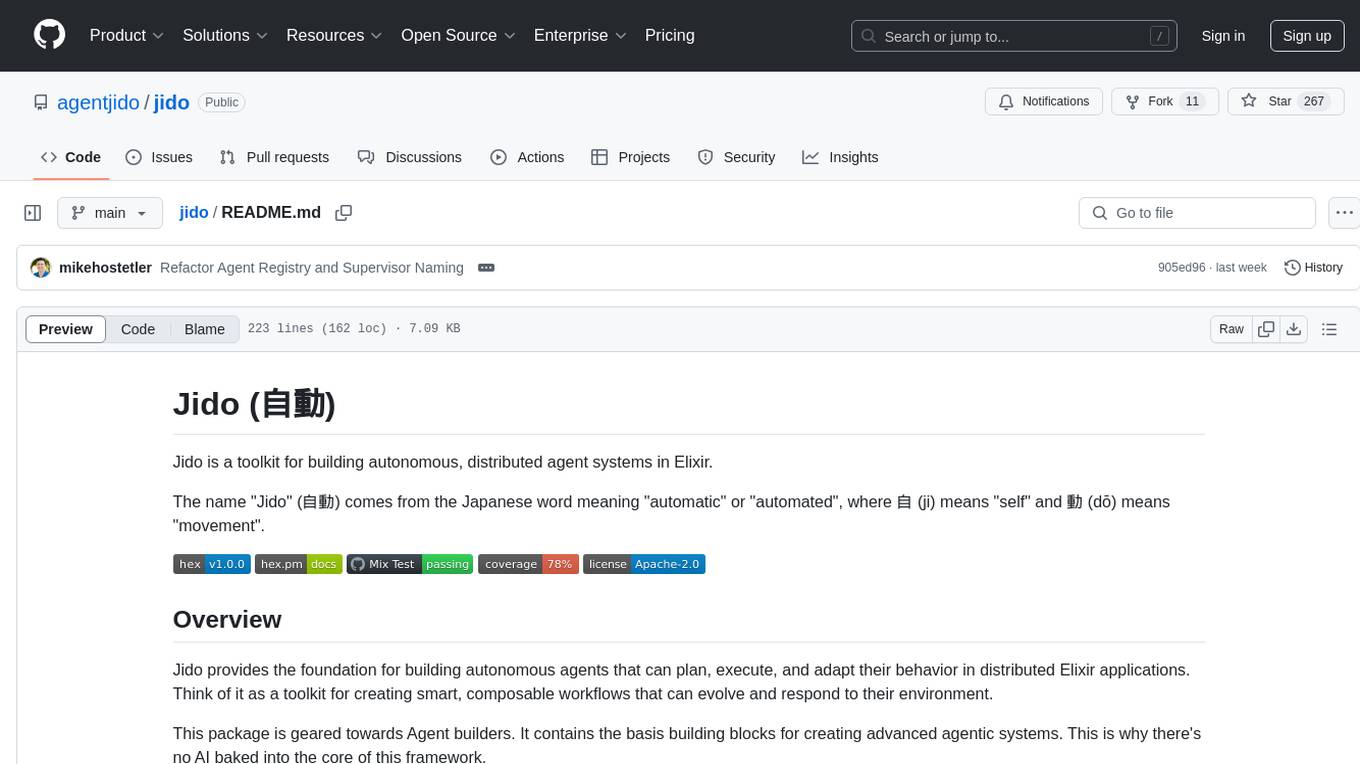
jido
Jido is a toolkit for building autonomous, distributed agent systems in Elixir. It provides the foundation for creating smart, composable workflows that can evolve and respond to their environment. Geared towards Agent builders, it contains core state primitives, composable actions, agent data structures, real-time sensors, signal system, skills, and testing tools. Jido is designed for multi-node Elixir clusters and offers rich helpers for unit and property-based testing.
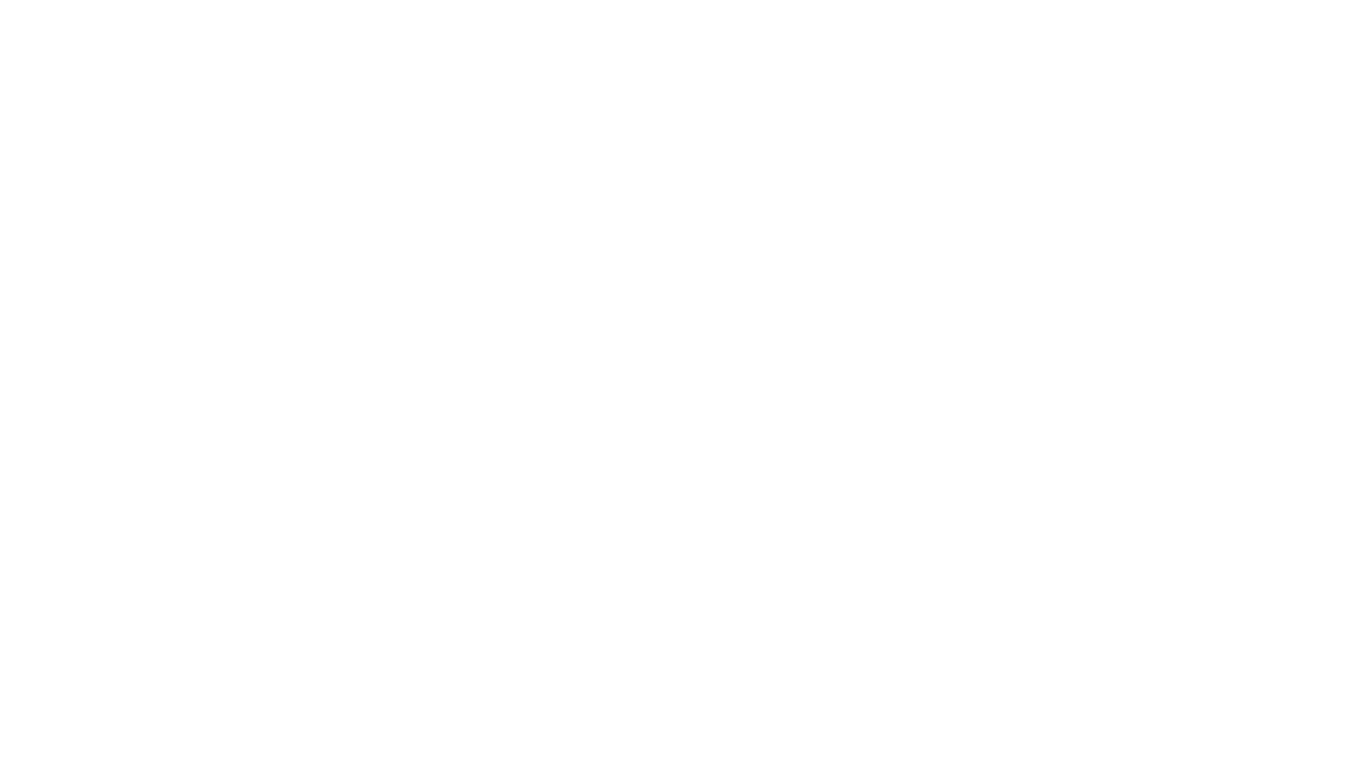
maiar-ai
MAIAR is a composable, plugin-based AI agent framework designed to abstract data ingestion, decision-making, and action execution into modular plugins. It enables developers to define triggers and actions as standalone plugins, while the core runtime handles decision-making dynamically. This framework offers extensibility, composability, and model-driven behavior, allowing seamless addition of new functionality. MAIAR's architecture is influenced by Unix pipes, ensuring highly composable plugins, dynamic execution pipelines, and transparent debugging. It remains declarative and extensible, allowing developers to build complex AI workflows without rigid architectures.
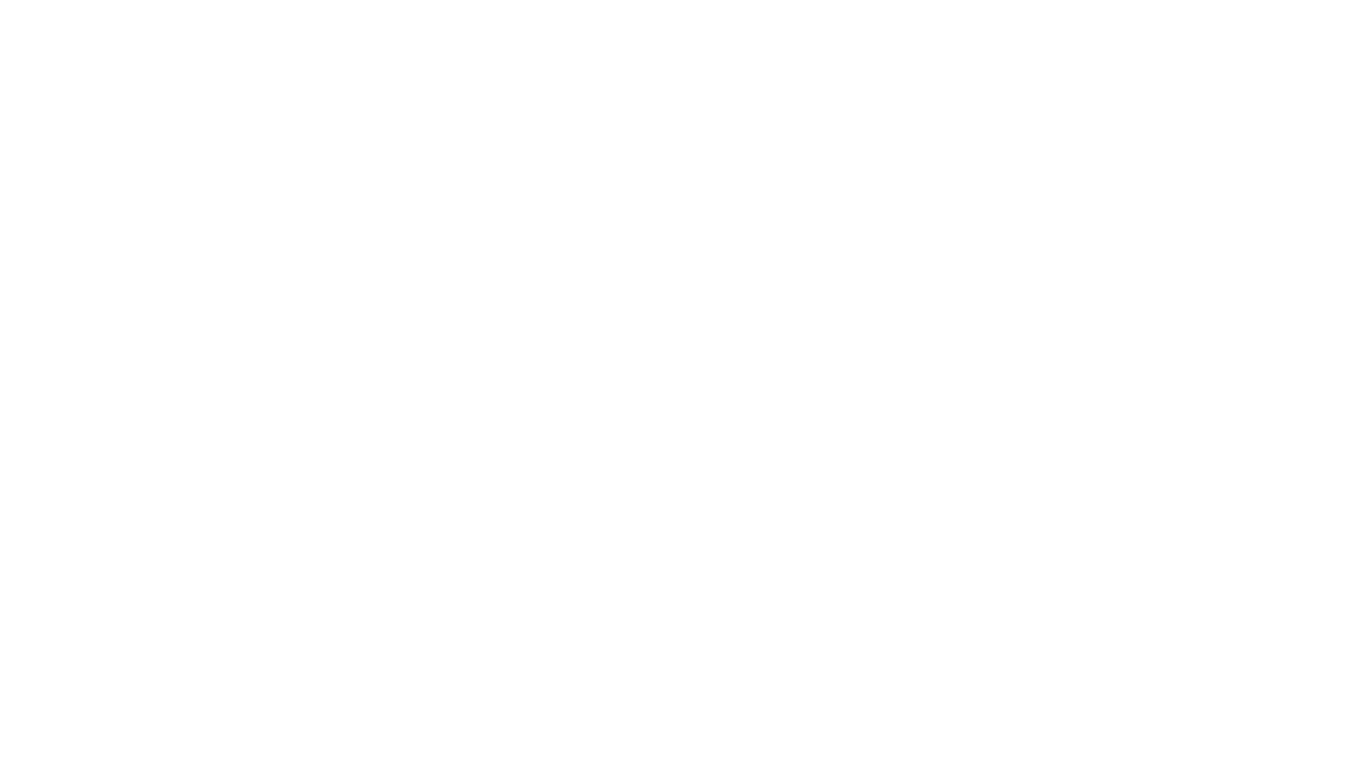
airflow
Apache Airflow (or simply Airflow) is a platform to programmatically author, schedule, and monitor workflows. When workflows are defined as code, they become more maintainable, versionable, testable, and collaborative. Use Airflow to author workflows as directed acyclic graphs (DAGs) of tasks. The Airflow scheduler executes your tasks on an array of workers while following the specified dependencies. Rich command line utilities make performing complex surgeries on DAGs a snap. The rich user interface makes it easy to visualize pipelines running in production, monitor progress, and troubleshoot issues when needed.
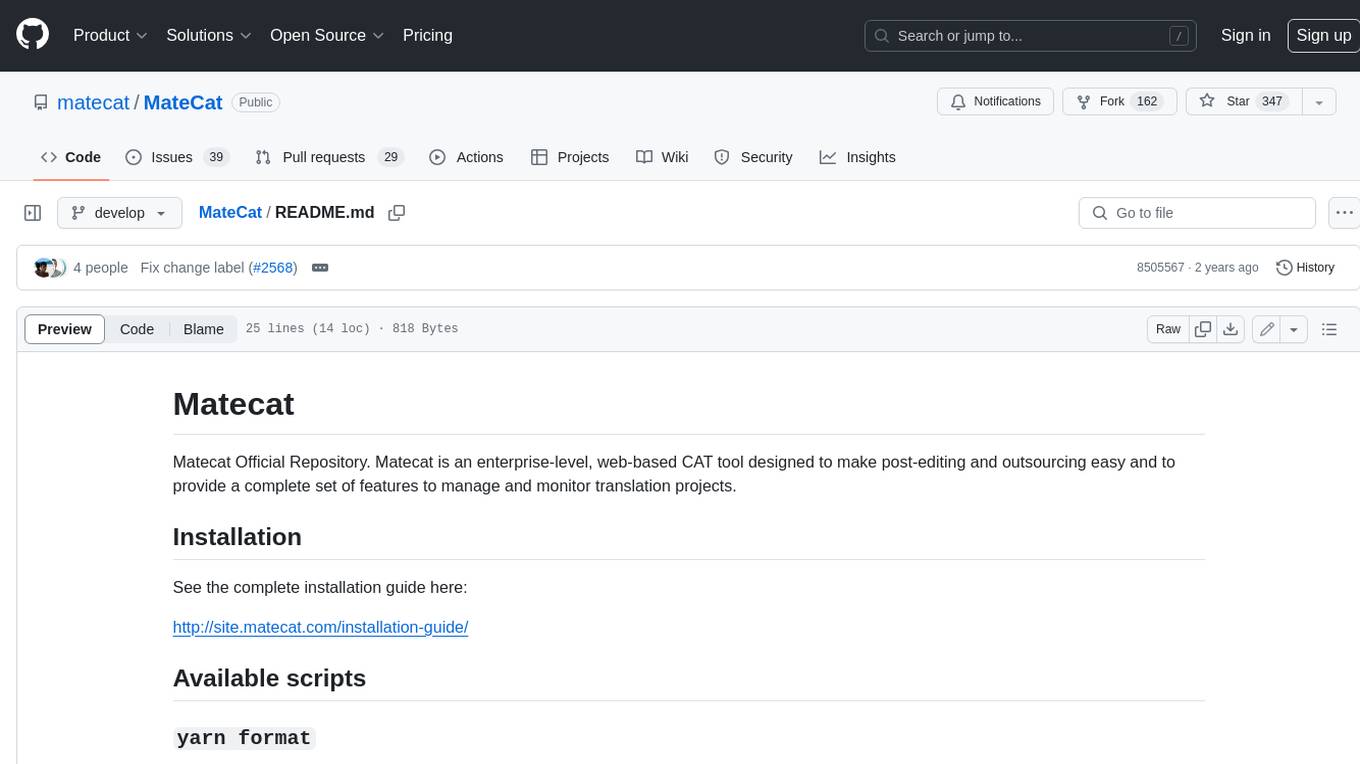
MateCat
Matecat is an enterprise-level, web-based CAT tool designed to make post-editing and outsourcing easy and to provide a complete set of features to manage and monitor translation projects.
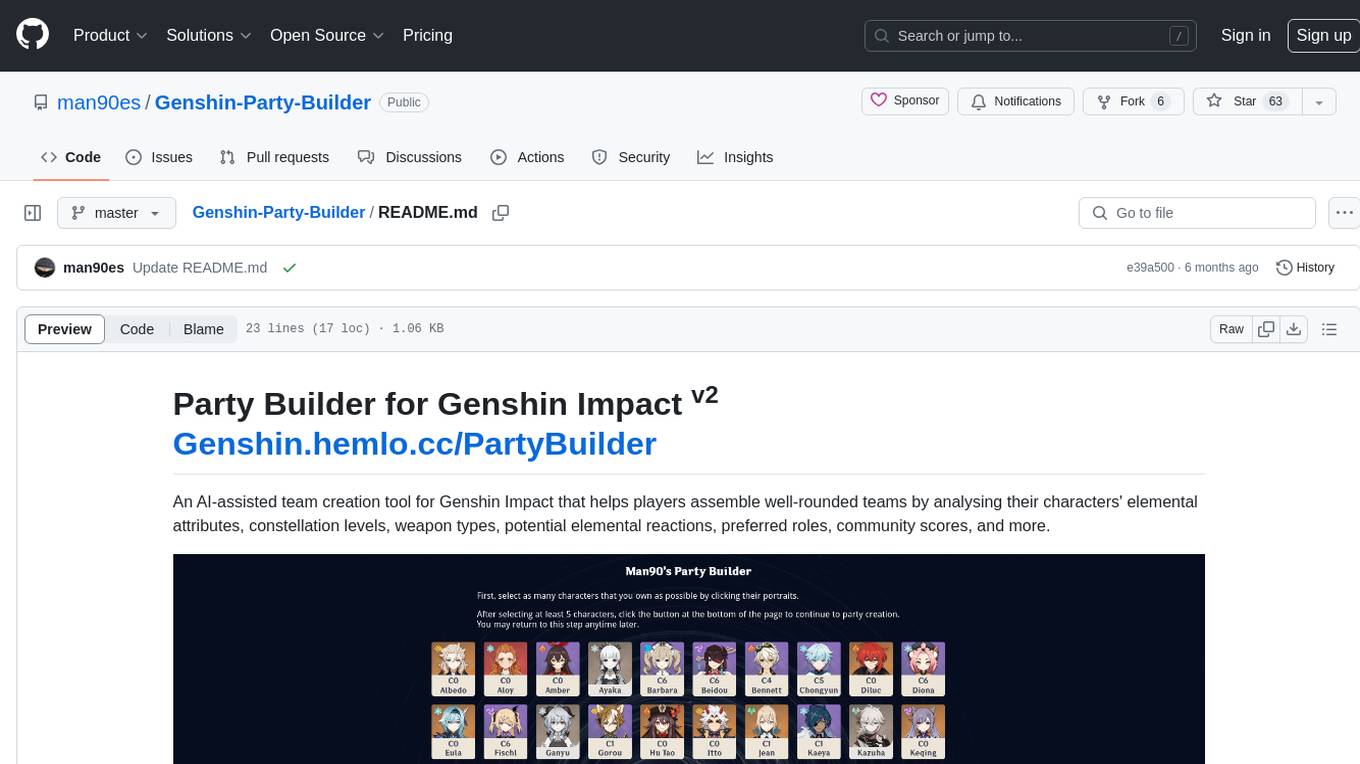
Genshin-Party-Builder
Party Builder for Genshin Impact is an AI-assisted team creation tool that helps players assemble well-rounded teams by analyzing characters' attributes, constellation levels, weapon types, elemental reactions, roles, and community scores. It allows users to optimize their team compositions for better gameplay experiences. The tool provides a user-friendly interface for easy team customization and strategy planning, enhancing the overall gaming experience for Genshin Impact players.
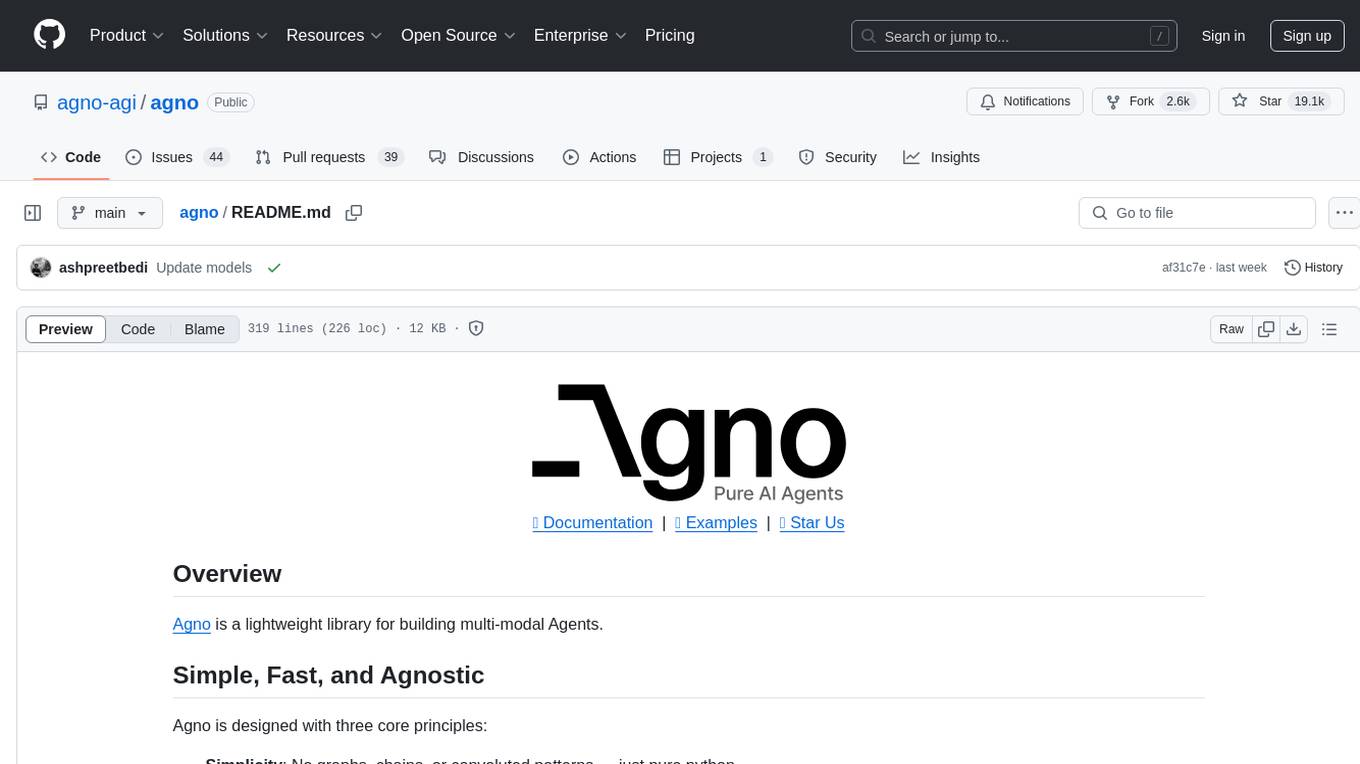
agno
Agno is a lightweight library for building multi-modal Agents. It is designed with core principles of simplicity, uncompromising performance, and agnosticism, allowing users to create blazing fast agents with minimal memory footprint. Agno supports any model, any provider, and any modality, making it a versatile container for AGI. Users can build agents with lightning-fast agent creation, model agnostic capabilities, native support for text, image, audio, and video inputs and outputs, memory management, knowledge stores, structured outputs, and real-time monitoring. The library enables users to create autonomous programs that use language models to solve problems, improve responses, and achieve tasks with varying levels of agency and autonomy.
For similar jobs
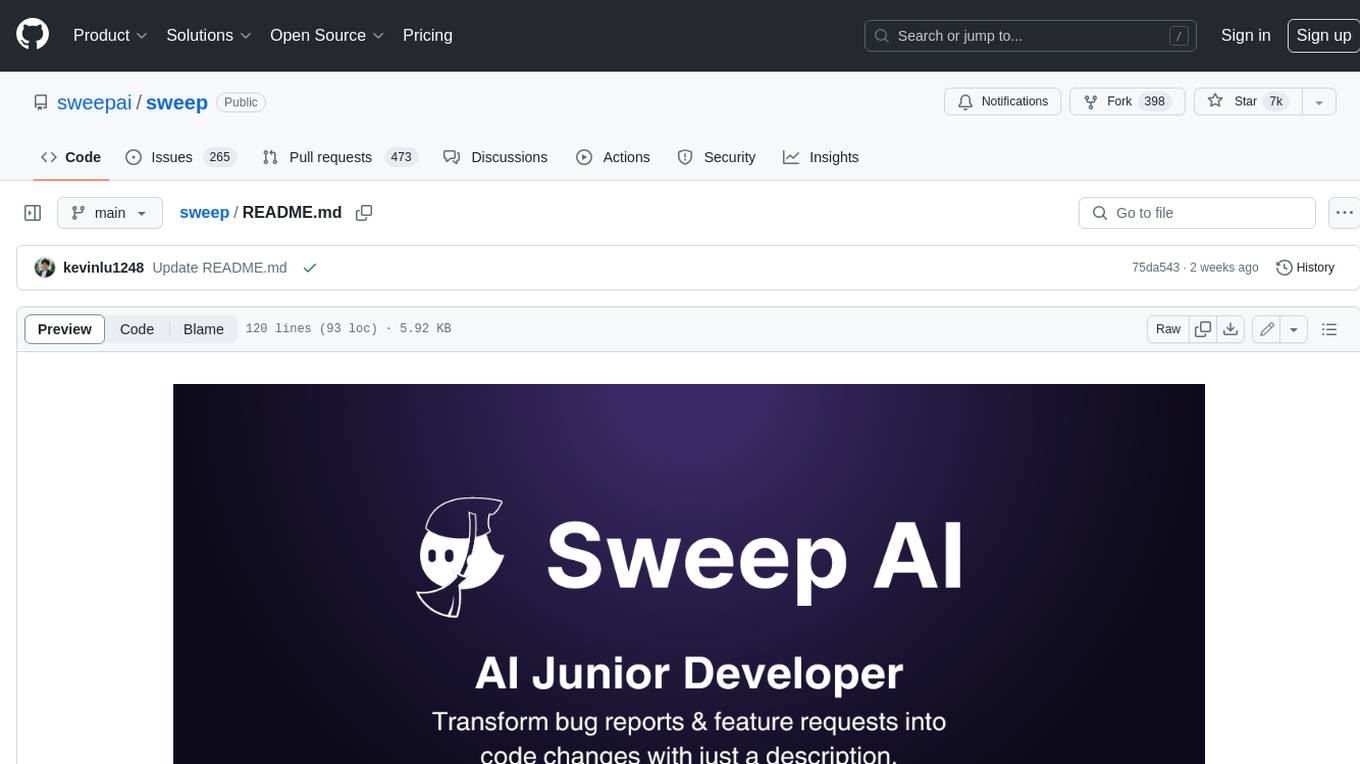
sweep
Sweep is an AI junior developer that turns bugs and feature requests into code changes. It automatically handles developer experience improvements like adding type hints and improving test coverage.
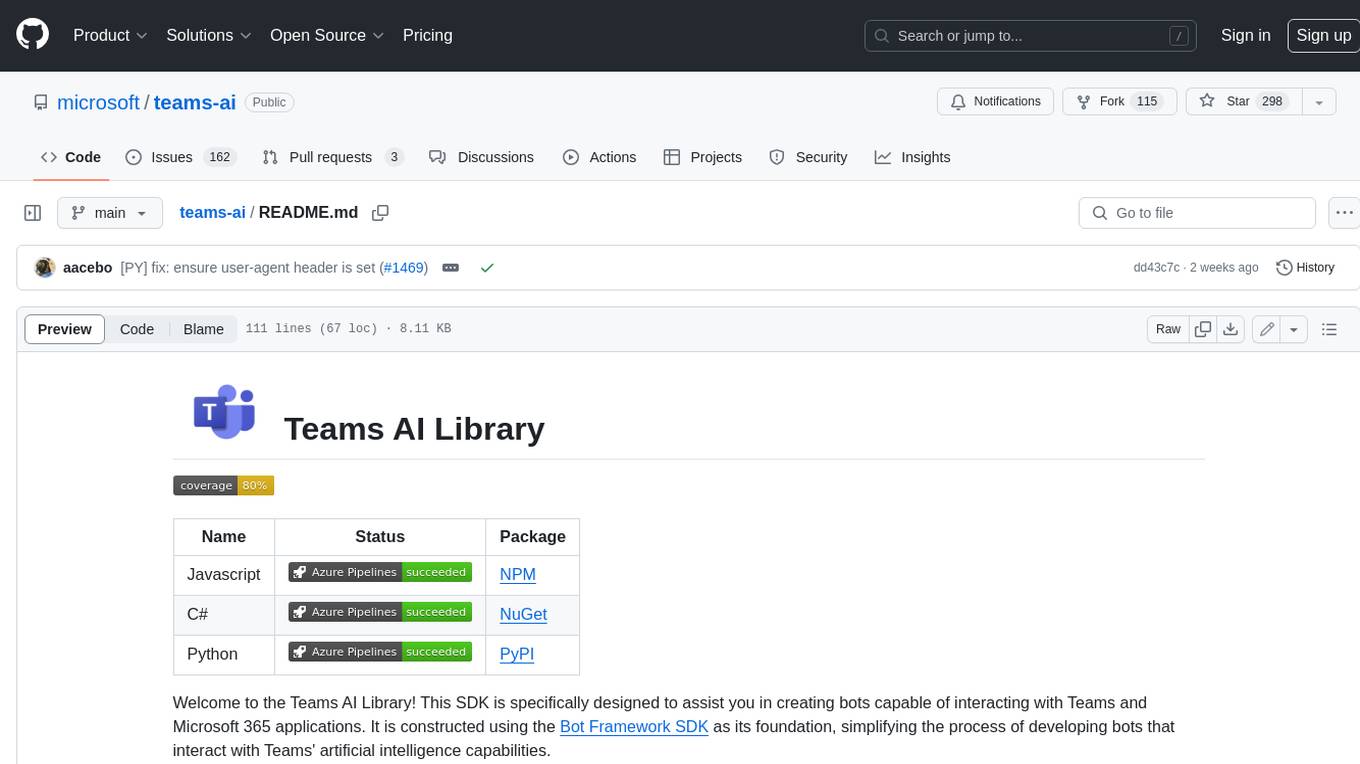
teams-ai
The Teams AI Library is a software development kit (SDK) that helps developers create bots that can interact with Teams and Microsoft 365 applications. It is built on top of the Bot Framework SDK and simplifies the process of developing bots that interact with Teams' artificial intelligence capabilities. The SDK is available for JavaScript/TypeScript, .NET, and Python.
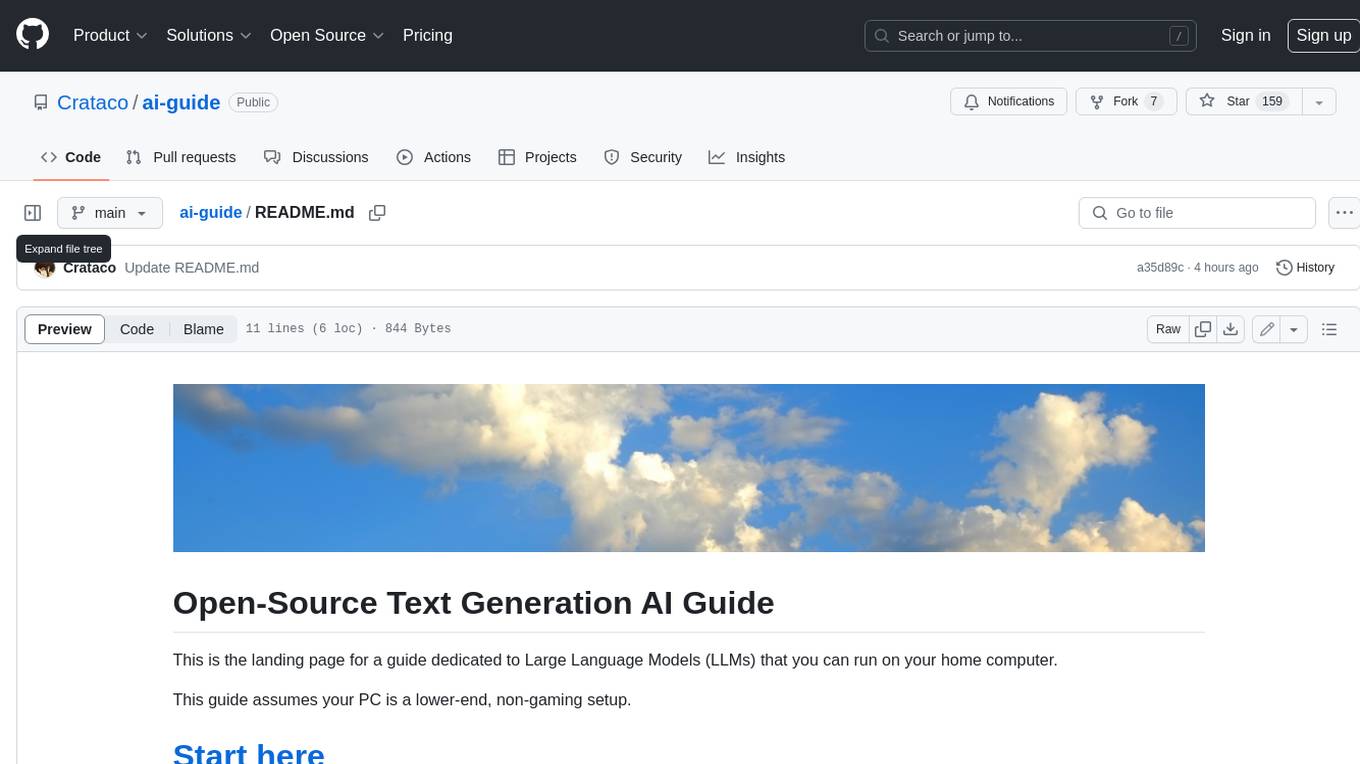
ai-guide
This guide is dedicated to Large Language Models (LLMs) that you can run on your home computer. It assumes your PC is a lower-end, non-gaming setup.
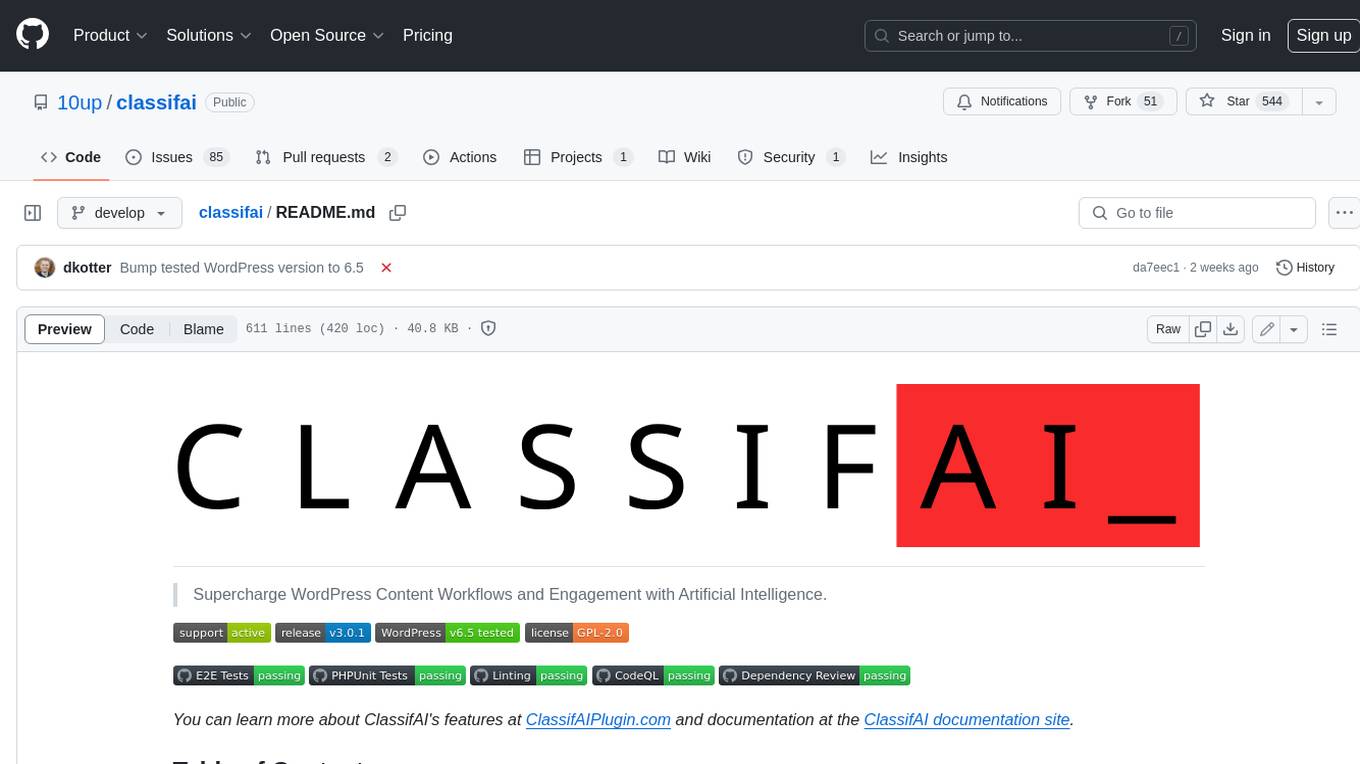
classifai
Supercharge WordPress Content Workflows and Engagement with Artificial Intelligence. Tap into leading cloud-based services like OpenAI, Microsoft Azure AI, Google Gemini and IBM Watson to augment your WordPress-powered websites. Publish content faster while improving SEO performance and increasing audience engagement. ClassifAI integrates Artificial Intelligence and Machine Learning technologies to lighten your workload and eliminate tedious tasks, giving you more time to create original content that matters.
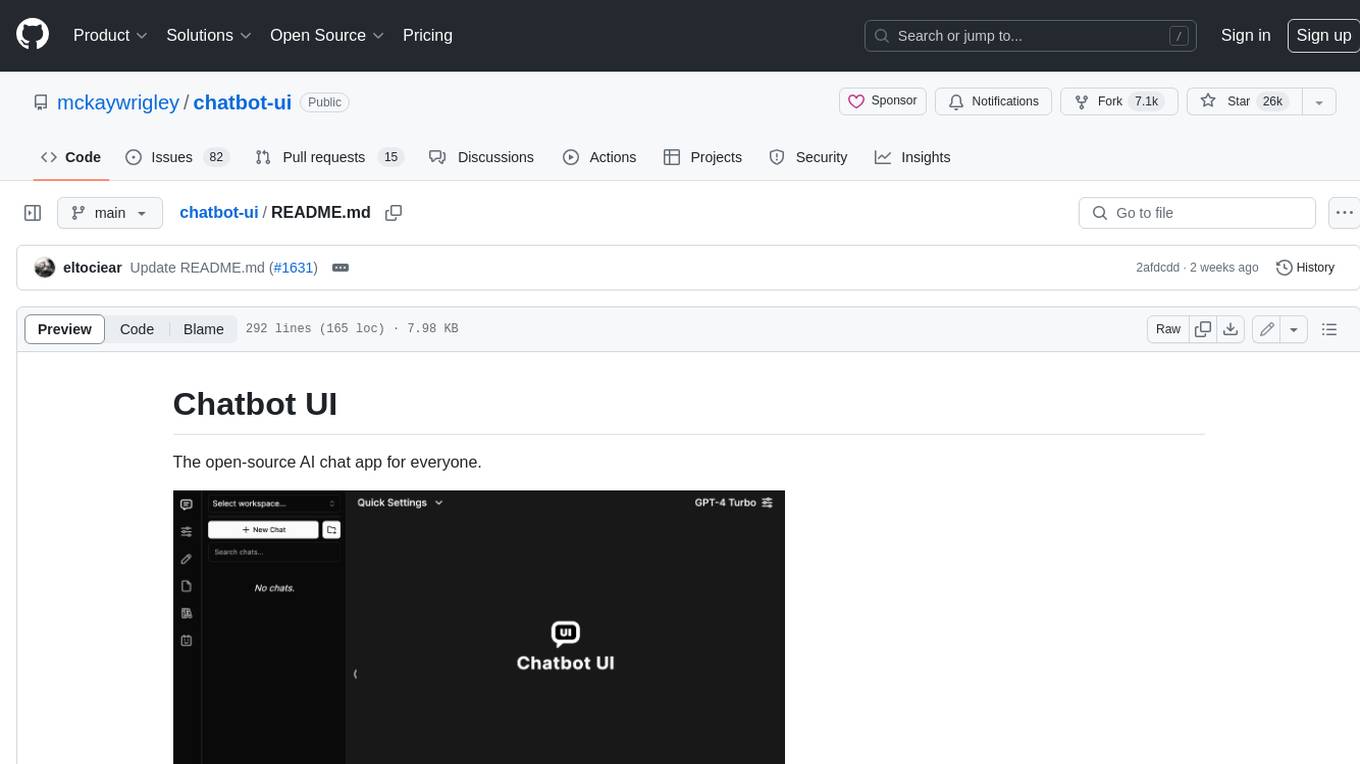
chatbot-ui
Chatbot UI is an open-source AI chat app that allows users to create and deploy their own AI chatbots. It is easy to use and can be customized to fit any need. Chatbot UI is perfect for businesses, developers, and anyone who wants to create a chatbot.
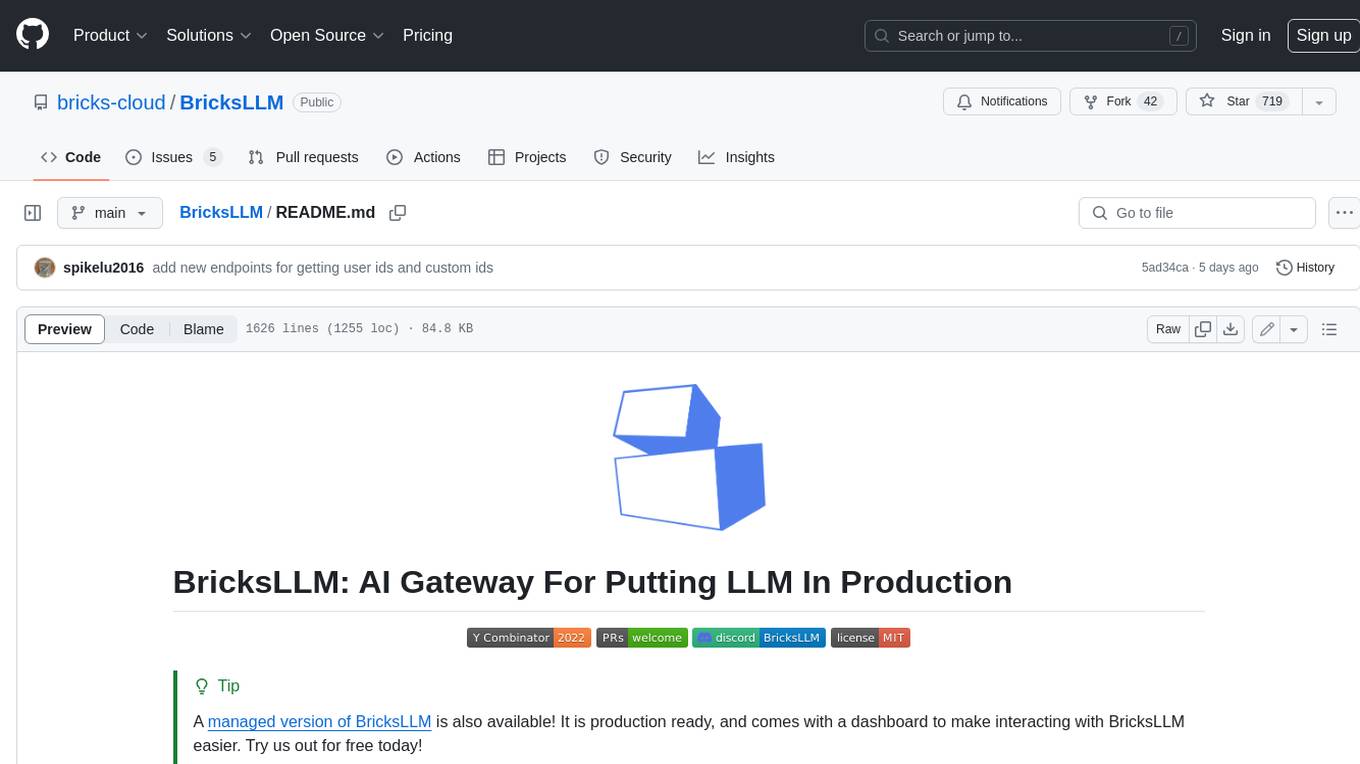
BricksLLM
BricksLLM is a cloud native AI gateway written in Go. Currently, it provides native support for OpenAI, Anthropic, Azure OpenAI and vLLM. BricksLLM aims to provide enterprise level infrastructure that can power any LLM production use cases. Here are some use cases for BricksLLM: * Set LLM usage limits for users on different pricing tiers * Track LLM usage on a per user and per organization basis * Block or redact requests containing PIIs * Improve LLM reliability with failovers, retries and caching * Distribute API keys with rate limits and cost limits for internal development/production use cases * Distribute API keys with rate limits and cost limits for students
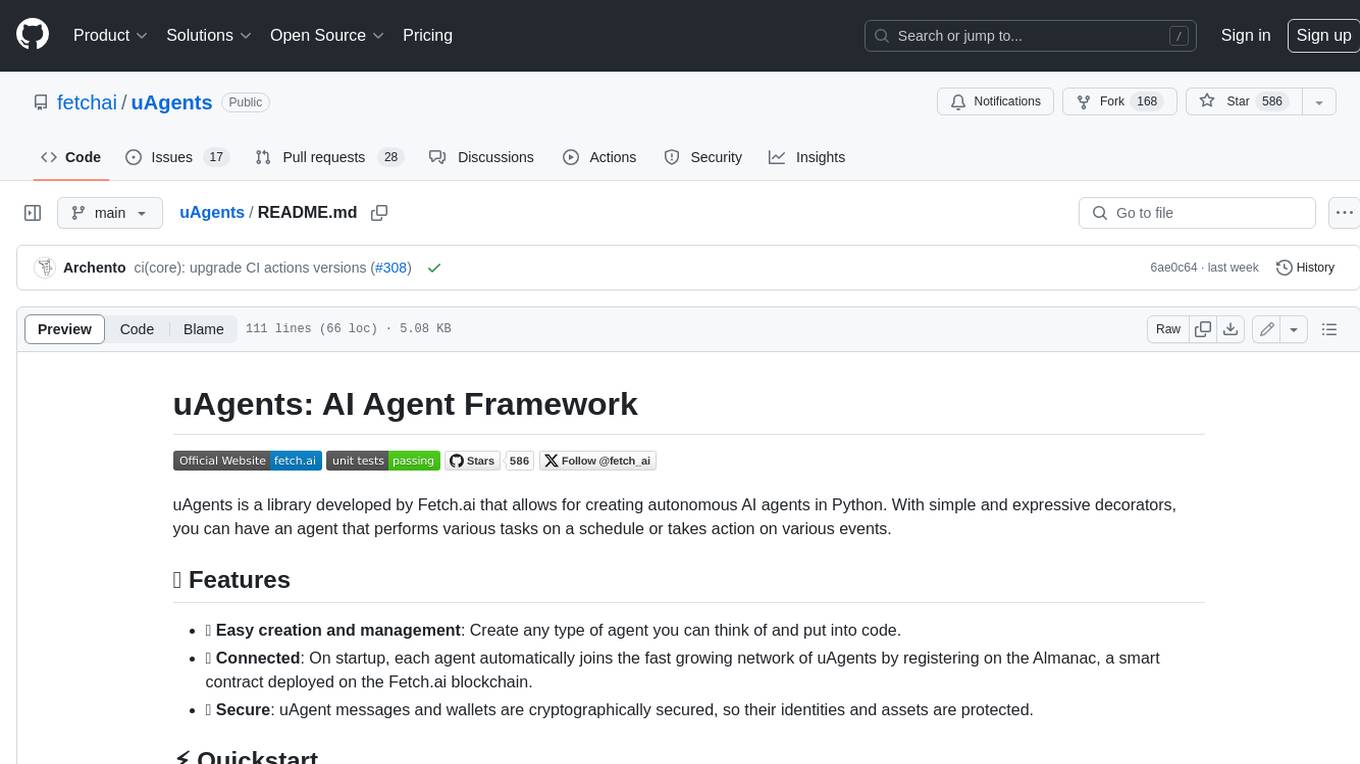
uAgents
uAgents is a Python library developed by Fetch.ai that allows for the creation of autonomous AI agents. These agents can perform various tasks on a schedule or take action on various events. uAgents are easy to create and manage, and they are connected to a fast-growing network of other uAgents. They are also secure, with cryptographically secured messages and wallets.
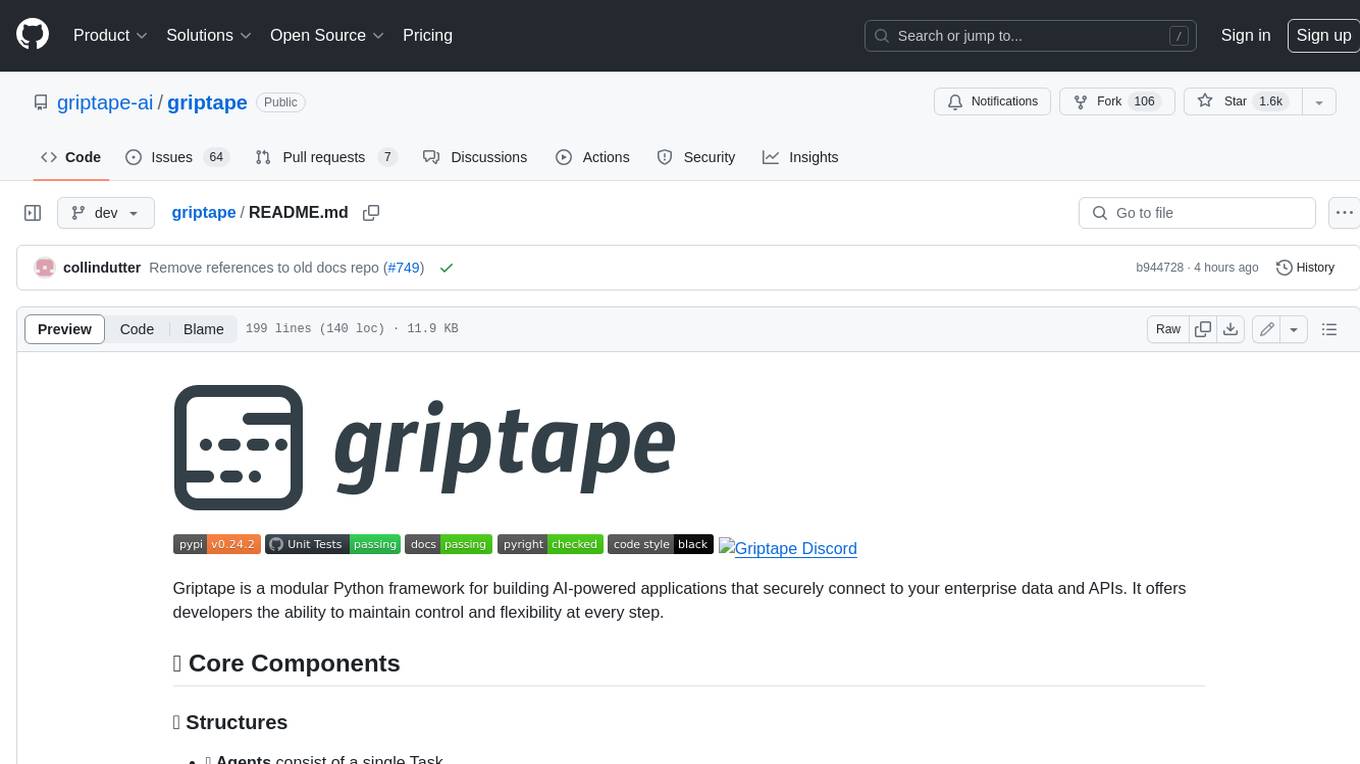
griptape
Griptape is a modular Python framework for building AI-powered applications that securely connect to your enterprise data and APIs. It offers developers the ability to maintain control and flexibility at every step. Griptape's core components include Structures (Agents, Pipelines, and Workflows), Tasks, Tools, Memory (Conversation Memory, Task Memory, and Meta Memory), Drivers (Prompt and Embedding Drivers, Vector Store Drivers, Image Generation Drivers, Image Query Drivers, SQL Drivers, Web Scraper Drivers, and Conversation Memory Drivers), Engines (Query Engines, Extraction Engines, Summary Engines, Image Generation Engines, and Image Query Engines), and additional components (Rulesets, Loaders, Artifacts, Chunkers, and Tokenizers). Griptape enables developers to create AI-powered applications with ease and efficiency.