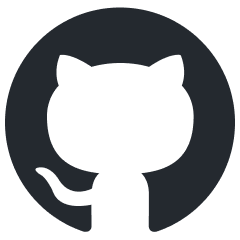
generative-ai-cdk-constructs
AWS Generative AI CDK Constructs are sample implementations of AWS CDK for common generative AI patterns.
Stars: 403
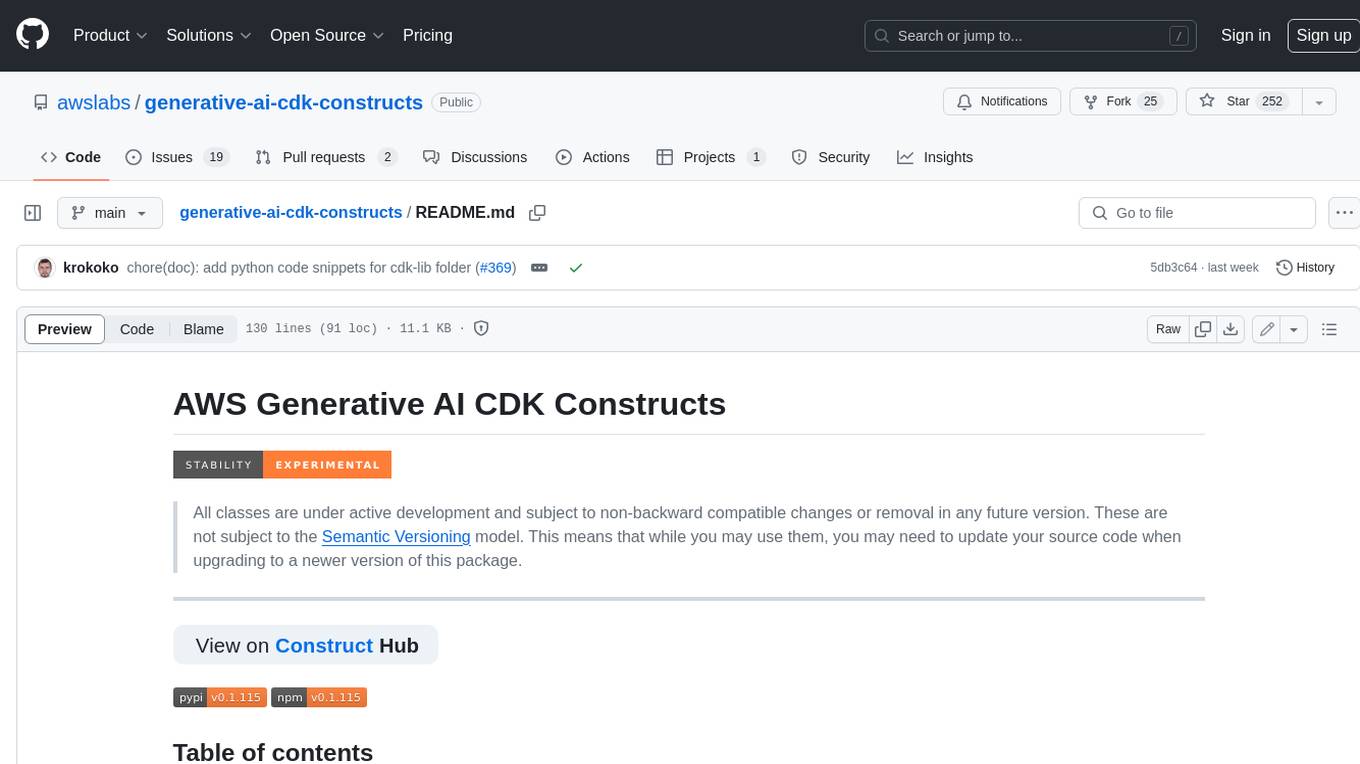
The AWS Generative AI Constructs Library is an open-source extension of the AWS Cloud Development Kit (AWS CDK) that provides multi-service, well-architected patterns for quickly defining solutions in code to create predictable and repeatable infrastructure, called constructs. The goal of AWS Generative AI CDK Constructs is to help developers build generative AI solutions using pattern-based definitions for their architecture. The patterns defined in AWS Generative AI CDK Constructs are high level, multi-service abstractions of AWS CDK constructs that have default configurations based on well-architected best practices. The library is organized into logical modules using object-oriented techniques to create each architectural pattern model.
README:
All classes are under active development and subject to non-backward compatible changes or removal in any future version. These are not subject to the Semantic Versioning model. This means that while you may use them, you may need to update your source code when upgrading to a newer version of this package.
- Introduction
- CDK Versions
- Contributing
- Design guidelines and Development guide
- Getting Started
- Catalog
- Sample Use Cases
- Additional Resources
- Contributors
- Operational Metrics Collection
- Roadmap
- License
- Legal Disclaimer
The AWS Generative AI Constructs Library is an open-source extension of the AWS Cloud Development Kit (AWS CDK) that provides multi-service, well-architected patterns for quickly defining solutions in code to create predictable and repeatable infrastructure, called constructs. The goal of AWS Generative AI CDK Constructs is to help developers build generative AI solutions using pattern-based definitions for their architecture.
The patterns defined in AWS Generative AI CDK Constructs are high level, multi-service abstractions of AWS CDK constructs that have default configurations based on well-architected best practices. The library is organized into logical modules using object-oriented techniques to create each architectural pattern model.
AWS Generative AI CDK Constructs and the AWS CDK are independent teams and have different release schedules. Each release of AWS Generative AI CDK Constructs is built against a specific version of the AWS CDK. The CHANGELOG.md file lists the CDK version associated with each AWS Generative AI Constructs release. For instance, AWS Generative AI CDK Constructs v0.0.0 was built against AWS CDK v2.96.2. This means that to use AWS Generative AI CDK Constructs v0.0.0, your application must include AWS CDK v2.96.2 or later. You can continue to use the latest AWS CDK versions and upgrade the your AWS Generative AI CDK Constructs version when new releases become available.
Contributions of all kinds are welcome! Check out our contributor guide
If you want to add a new construct to the library, check out our design guidelines, then follow the development guide
TypeScript
- Create or use an existing CDK application in TypeScript.
cdk init app --language typescript
- Run
npm install @cdklabs/generative-ai-cdk-constructs
- The package should be added to your package.json.
- Import the library:
import * as genai from '@cdklabs/generative-ai-cdk-constructs';
Python
- Create or use an existing CDK application in Python
cdk init app --language python
- Install the package:
pip install cdklabs.generative-ai-cdk-constructs
- Import the library:
import cdklabs.generative_ai_cdk_constructs
NuGet
- Create or use an existing CDK application in Python
cdk init app --language csharp
- Install the package while in the Visual Studio project:
dotnet add package CdkLabs.GenerativeAICdkConstructs
- Use the namespace:
using Cdklabs.GenerativeAiCdkConstructs;
Go
- Create or use an existing CDK application in Python
cdk init app --language go
- Get the module:
go get github.com/cdklabs/generative-ai-cdk-constructs-go/generative-ai-cdk-constructs
- Import the library:
import "github.com/cdklabs/generative-ai-cdk-constructs-go/generative-ai-cdk-constructs"
NOTE: The Go distribution repository, distributes the JSII tar gzipped versioned source from the source repository
Java
- Create or use an existing CDK application in Java
cdk init app --language java
- Add the dependency into the
pom.xml
<dependency>
<groupId>io.github.cdklabs</groupId>
<artifactId>generative-ai-cdk-constructs</artifactId>
<version>Get the latest version and insert it here</version>
</dependency>
Refer to the documentation for additional guidance on a particular construct: Catalog
The following constructs are available in the library:
Construct | Description | AWS Services used |
---|---|---|
Data ingestion pipeline - OpenSearch | Ingestion pipeline providing a RAG (retrieval augmented generation) source for storing documents in a knowledge base. | Amazon OpenSearch, AWS Step Functions, Amazon Bedrock, AWS AppSync, AWS Lambda |
Question answering | Utilizing Large Language Models (Anthropic Claude V2.1.) for Question Answering on PDF documents with RAG (retrieval augmented generation) source and/or long context. Additionally, leveraging Anthropic Claude 3 for visual question answering on images. | Amazon OpenSearch, AWS Lambda, Amazon Bedrock, AWS AppSync |
Summarization | Document summarization with a large language model (Anthropic Claude V2.1). | AWS Lambda, Amazon Bedrock, AWS AppSync and Amazon ElastiCache for Redis. |
SageMaker model deployment (JumpStart) | Deploy a foundation model from Amazon SageMaker JumpStart to an Amazon SageMaker endpoint. | Amazon SageMaker |
SageMaker model deployment (Hugging Face) | Deploy a foundation model from Hugging Face to an Amazon SageMaker endpoint. | Amazon SageMaker |
SageMaker model deployment (Custom) | Deploy a foundation model from an S3 location to an Amazon SageMaker endpoint. | Amazon SageMaker |
Content Generation | Generate images from text using Amazon titan-image-generator-v1 or stability.stable-diffusion-xl-v1 model. | AWS Lambda, Amazon Bedrock, AWS AppSync |
Amazon Bedrock Monitoring (Amazon CloudWatch Dashboard) | Amazon CloudWatch dashboard to monitor model usage from Amazon Bedrock. | Amazon CloudWatch |
TXT to SQL | Leverages generative AI capabilities to facilitate natural language-based SQL query generation. | Amazon Event Bridge, Amazon Bedrock, AWS Lambda, Amazon SQS, AWS Secrets, and database of choice |
LlamaIndex Data Loading | Use LlamaIndex to load data in preparation for generative AI workloads | Amazon ECS Fargate, Amazon SQS, and AWS Systems Manager Parameters |
Construct | Description | AWS Services used |
---|---|---|
Amazon Bedrock | CDK L2 Constructs for Amazon Bedrock. | Amazon Bedrock, Amazon OpenSearch Serverless, AWS Lambda |
Amazon OpenSearch Serverless Vector Collection | CDK L2 Constructs to create a vector collection. | Amazon OpenSearch Vector Index |
Amazon OpenSearch Vector Index | CDK L1 Custom Resource to create a vector index. | Amazon OpenSearch Serverless, AWS Lambda |
The official samples repository includes a collection of functional use case implementations to demonstrate the usage of AWS Generative AI CDK Constructs. These can be used in the same way as architectural patterns, and can be conceptualized as an additional "higher-level" abstraction of those patterns. Those patterns (constructs) are composed together into stacks, forming a "CDK app".
Resource | Type | Description |
---|---|---|
AWS re:Invent 2023 - Keynote with Dr. Werner Vogels | Keynote | Dr. Werner Vogels, Amazon.com's VP and CTO, announces the AWS Generative AI CDK Constructs during his AWS re:Invent 2023 keynote. |
Workshop - Building Generative AI Apps on AWS with CDK | Workshop | In this workshop, you will explore how to build a sample generative AI app on AWS using CDK and Generative AI CDK Constructs. |
Workshop - Hands on AWS CDK Generative AI Constructs | Workshop | In this workshop you will deploy projects that use CDK constructs from this library. Projects are from the amazon-bedrock-samples Github Repository. |
Build generative AI applications with Amazon Titan Text Premier, Amazon Bedrock, and AWS CDK | Blog post + Code sample | Blog post exploring building and deploying two sample applications powered by Amazon Titan Text Premier using the Generative AI CDK constructs. |
aws-cdk-stack-builder-tool | Code sample | AWS CDK Builder is a browser-based tool designed to streamline bootstrapping of Infrastructure as Code (IaC) projects using the AWS Cloud Development Kit (CDK). |
CDK Live! Building generative AI applications and architectures leveraging AWS CDK Constructs! | Video | CDK Live! episode focused on building and deploying generative AI applications and architectures on AWS using the AWS Cloud Development Kit (CDK) and the AWS Generative AI CDK Constructs. |
Announcing AWS Generative AI CDK Constructs! | Blog post | Blog post announcing the release of the AWS Generative AI CDK Constructs. |
Streamline insurance underwriting with generative AI using Amazon Bedrock | Blog post + Code sample | Blog post and code sample discussing how to use AWS generative artificial intelligence (AI) solutions like Amazon Bedrock to improve the underwriting process, including rule validation, underwriting guidelines adherence, and decision justification. |
aws-genai-llm-chatbot | Code sample | Multi-Model and Multi-RAG Powered Chatbot Using AWS CDK on AWS allowing you to experiment with a variety of Large Language Models and Multimodal Language Models, settings and prompts in your own AWS account. |
bedrock-claude-chat | Code sample | AWS-native chatbot using Bedrock + Claude (+Mistral). |
amazon-bedrock-rag | Code sample | Fully managed RAG solution using Knowledge Bases for Amazon Bedrock. |
Amazon Bedrock Multimodal Search | Code sample | Multimodal product search app built using Amazon Titan Multimodal Embeddings model. |
Amazon Bedrock Knowledge Bases with Private Data | Blog post + Code sample | Blog post and associated code sample demonstrating how to integrate Knowledge Bases into Amazon Bedrock to provide foundational models with contextual data from private data sources. |
Automating tasks using Amazon Bedrock Agents and AI | Blog post + Code sample | Blog post and associated code sample demonstrating how to deploy an Amazon Bedrock Agent and a Knowledge Base through a hotel and spa use case. |
Agents for Amazon Bedrock - Powertools for AWS Lambda (Python) | Code sample | Create Agents for Amazon Bedrock using event handlers and auto generation of OpenAPI schemas. |
Text to SQL Bedrock Agent | Code sample | Harnessing the power of natural language processing, the "Text to SQL Bedrock Agent" facilitates the automatic transformation of natural language questions into executable SQL queries. |
Generative AI CDK Constructs may collect anonymous operational metrics, including: the region a construct is deployed, the name and version of the construct deployed, and related information. We may use the metrics to maintain, provide, develop, and improve the constructs and AWS services.
Roadmap is available through the GitHub Project
Apache-2.0
You should consider doing your own independent assessment before using the content in this library for production purposes. This may include (amongst other things) testing, securing, and optimizing the CDK constructs and other content, provided in this library, based on your specific quality control practices and standards.
© Copyright Amazon.com, Inc. or its affiliates. All Rights Reserved.
For Tasks:
Click tags to check more tools for each tasksFor Jobs:
Alternative AI tools for generative-ai-cdk-constructs
Similar Open Source Tools
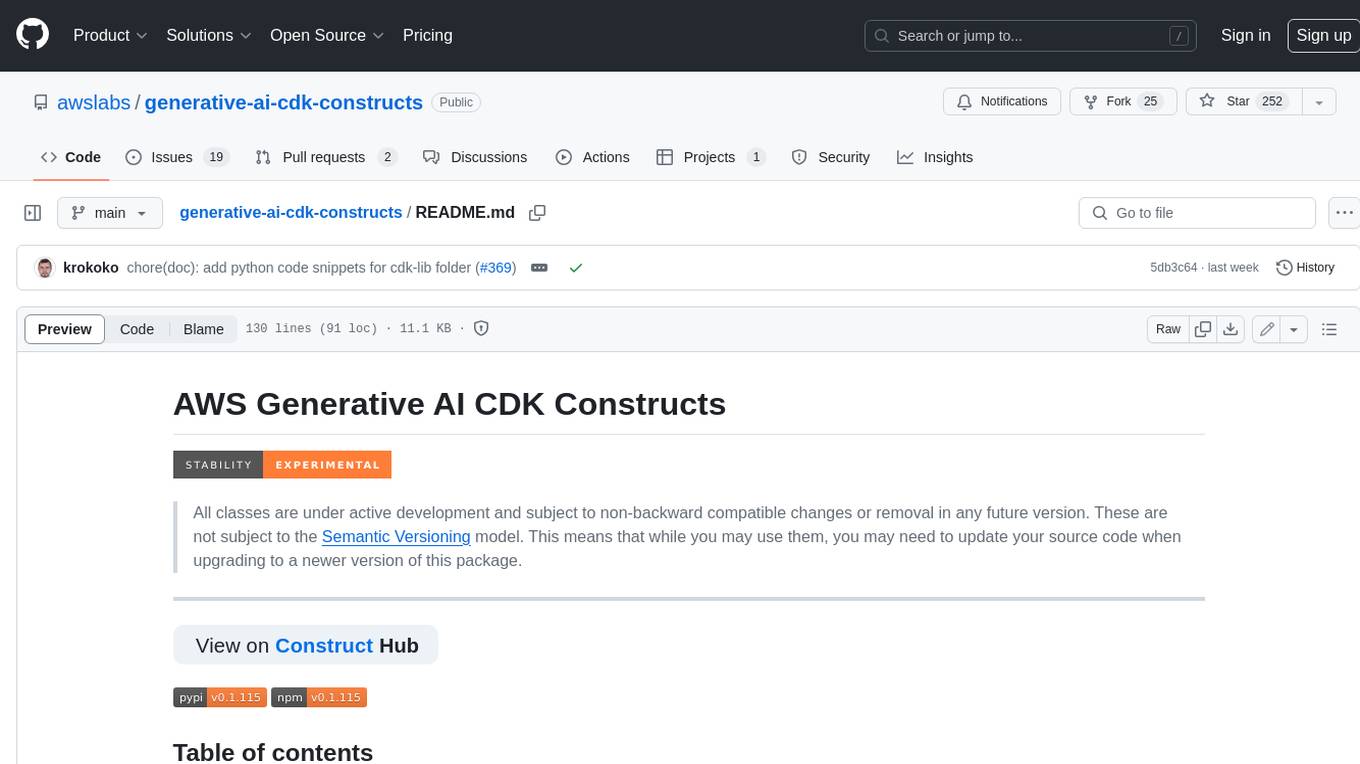
generative-ai-cdk-constructs
The AWS Generative AI Constructs Library is an open-source extension of the AWS Cloud Development Kit (AWS CDK) that provides multi-service, well-architected patterns for quickly defining solutions in code to create predictable and repeatable infrastructure, called constructs. The goal of AWS Generative AI CDK Constructs is to help developers build generative AI solutions using pattern-based definitions for their architecture. The patterns defined in AWS Generative AI CDK Constructs are high level, multi-service abstractions of AWS CDK constructs that have default configurations based on well-architected best practices. The library is organized into logical modules using object-oriented techniques to create each architectural pattern model.
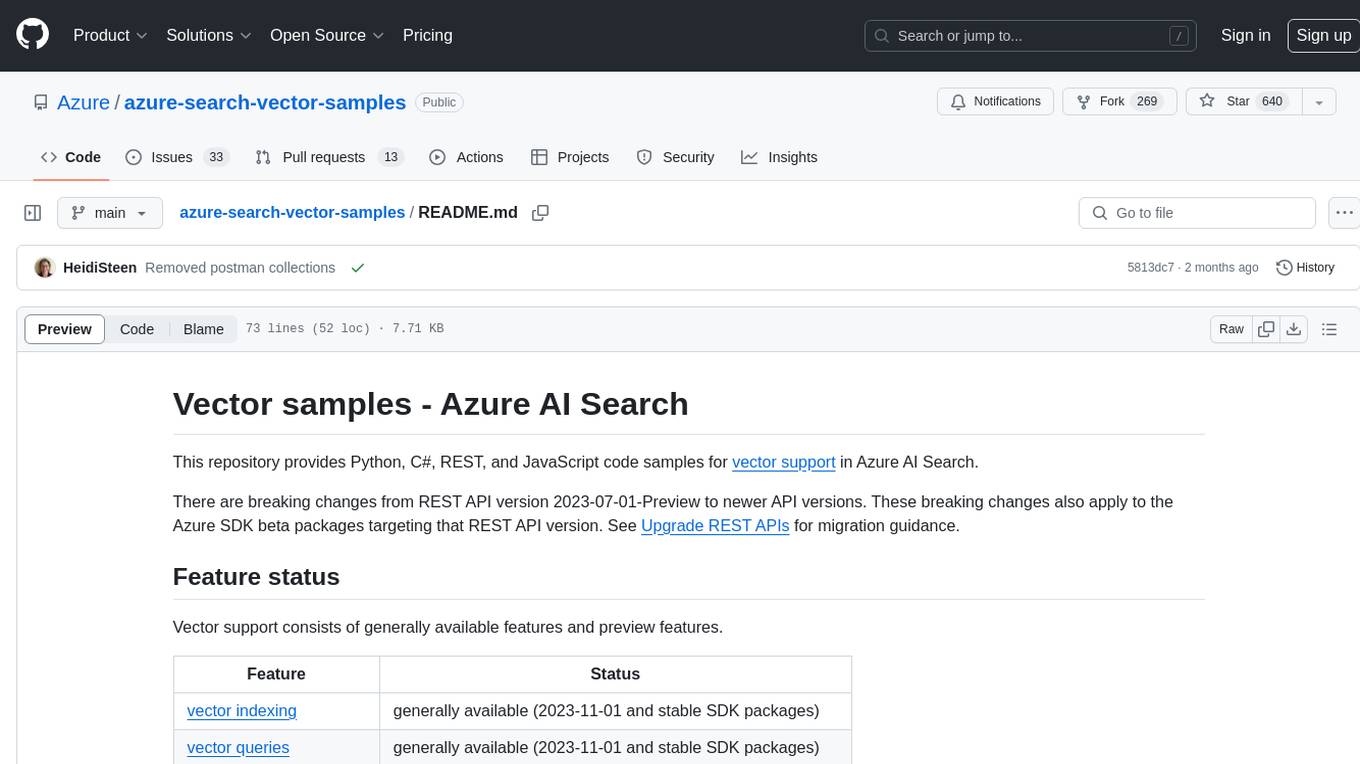
azure-search-vector-samples
This repository provides code samples in Python, C#, REST, and JavaScript for vector support in Azure AI Search. It includes demos for various languages showcasing vectorization of data, creating indexes, and querying vector data. Additionally, it offers tools like Azure AI Search Lab for experimenting with AI-enabled search scenarios in Azure and templates for deploying custom chat-with-your-data solutions. The repository also features documentation on vector search, hybrid search, creating and querying vector indexes, and REST API references for Azure AI Search and Azure OpenAI Service.
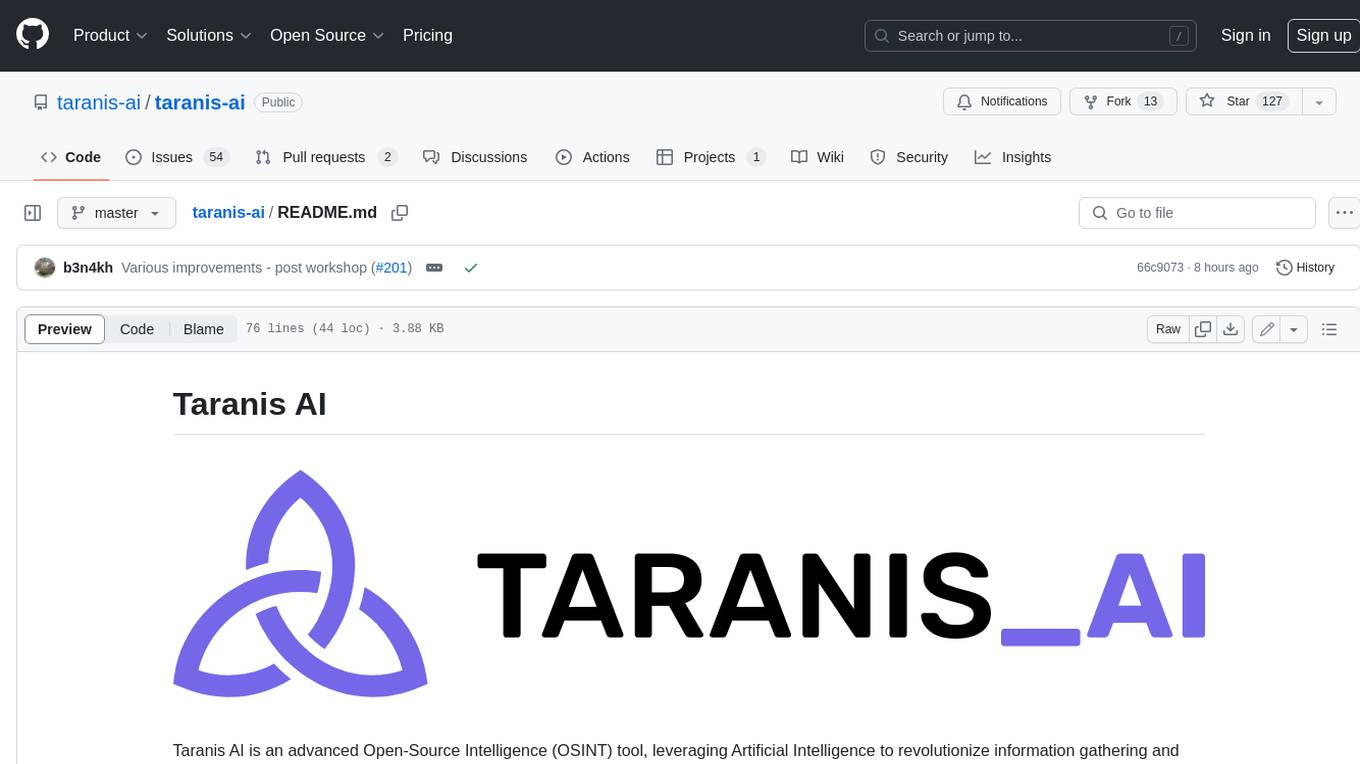
taranis-ai
Taranis AI is an advanced Open-Source Intelligence (OSINT) tool that leverages Artificial Intelligence to revolutionize information gathering and situational analysis. It navigates through diverse data sources like websites to collect unstructured news articles, utilizing Natural Language Processing and Artificial Intelligence to enhance content quality. Analysts then refine these AI-augmented articles into structured reports that serve as the foundation for deliverables such as PDF files, which are ultimately published.
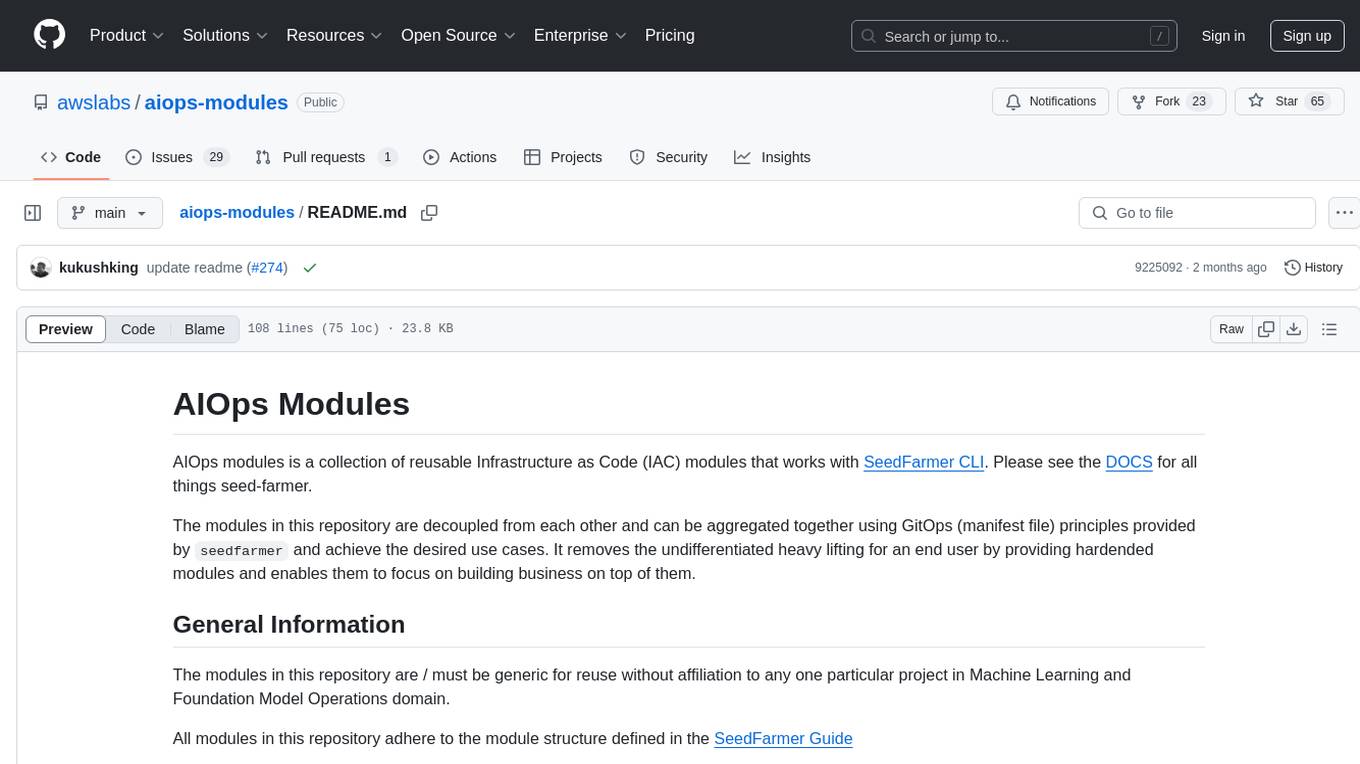
aiops-modules
AIOps Modules is a collection of reusable Infrastructure as Code (IAC) modules that work with SeedFarmer CLI. The modules are decoupled and can be aggregated using GitOps principles to achieve desired use cases, removing heavy lifting for end users. They must be generic for reuse in Machine Learning and Foundation Model Operations domain, adhering to SeedFarmer Guide structure. The repository includes deployment steps, project manifests, and various modules for SageMaker, Mlflow, FMOps/LLMOps, MWAA, Step Functions, EKS, and example use cases. It also supports Industry Data Framework (IDF) and Autonomous Driving Data Framework (ADDF) Modules.
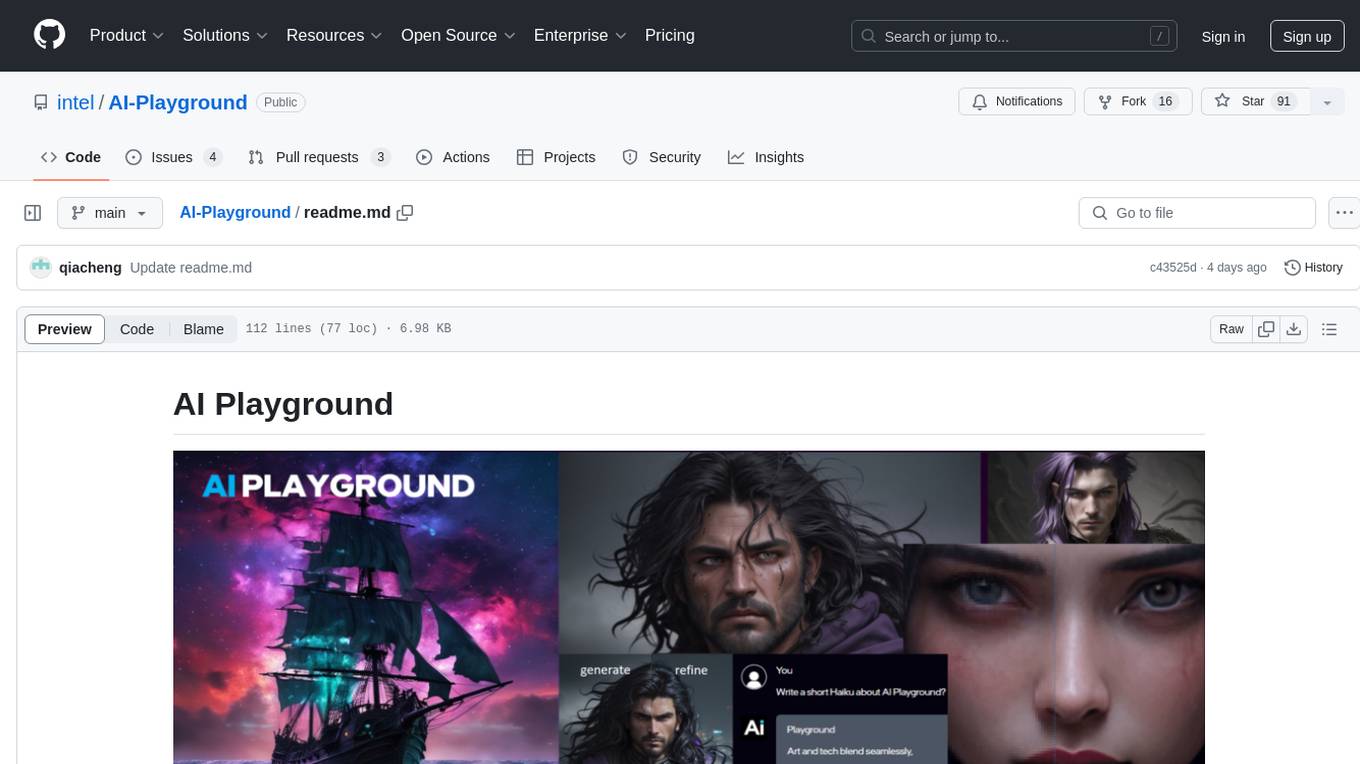
AI-Playground
AI Playground is an open-source project and AI PC starter app designed for AI image creation, image stylizing, and chatbot functionalities on a PC powered by an Intel Arc GPU. It leverages libraries from GitHub and Huggingface, providing users with the ability to create AI-generated content and interact with chatbots. The tool requires specific hardware specifications and offers packaged installers for ease of setup. Users can also develop the project environment, link it to the development environment, and utilize alternative models for different AI tasks.
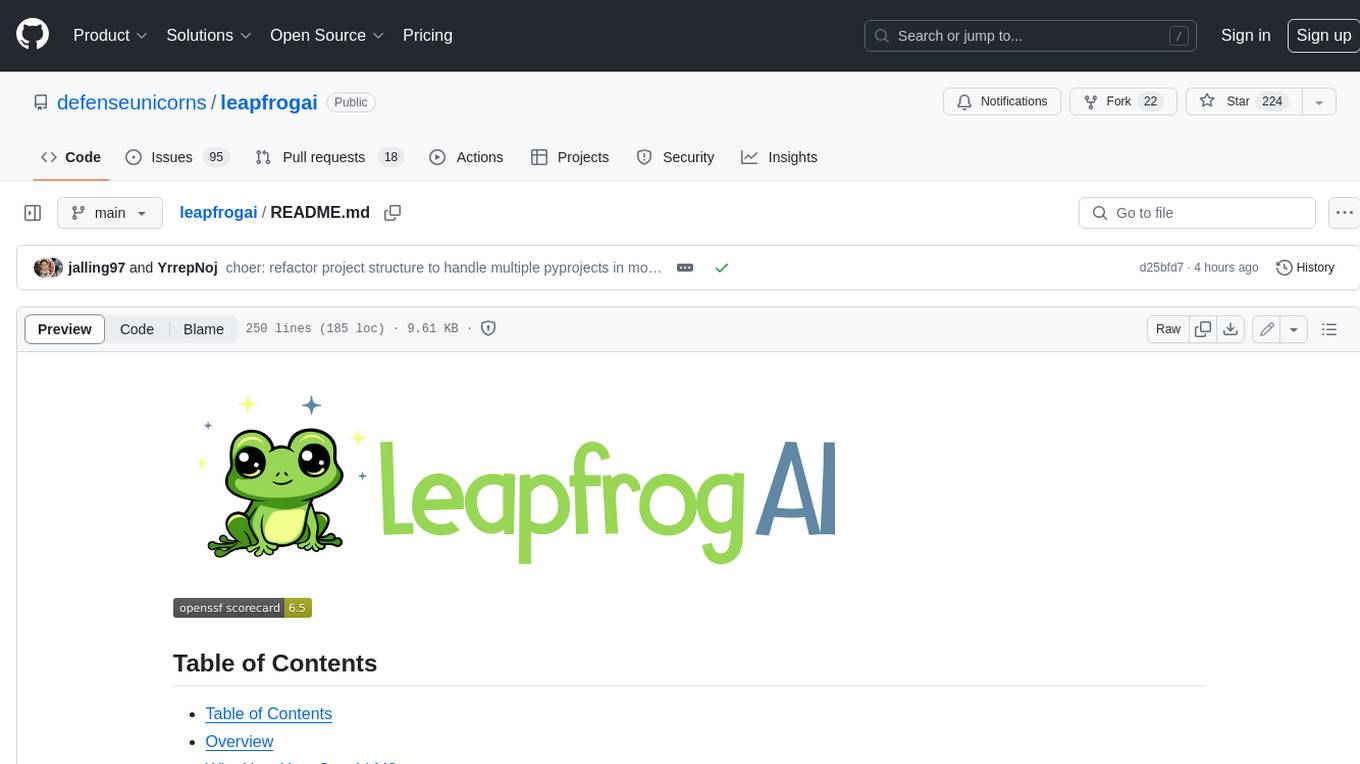
leapfrogai
LeapfrogAI is a self-hosted AI platform designed to be deployed in air-gapped resource-constrained environments. It brings sophisticated AI solutions to these environments by hosting all the necessary components of an AI stack, including vector databases, model backends, API, and UI. LeapfrogAI's API closely matches that of OpenAI, allowing tools built for OpenAI/ChatGPT to function seamlessly with a LeapfrogAI backend. It provides several backends for various use cases, including llama-cpp-python, whisper, text-embeddings, and vllm. LeapfrogAI leverages Chainguard's apko to harden base python images, ensuring the latest supported Python versions are used by the other components of the stack. The LeapfrogAI SDK provides a standard set of protobuffs and python utilities for implementing backends and gRPC. LeapfrogAI offers UI options for common use-cases like chat, summarization, and transcription. It can be deployed and run locally via UDS and Kubernetes, built out using Zarf packages. LeapfrogAI is supported by a community of users and contributors, including Defense Unicorns, Beast Code, Chainguard, Exovera, Hypergiant, Pulze, SOSi, United States Navy, United States Air Force, and United States Space Force.
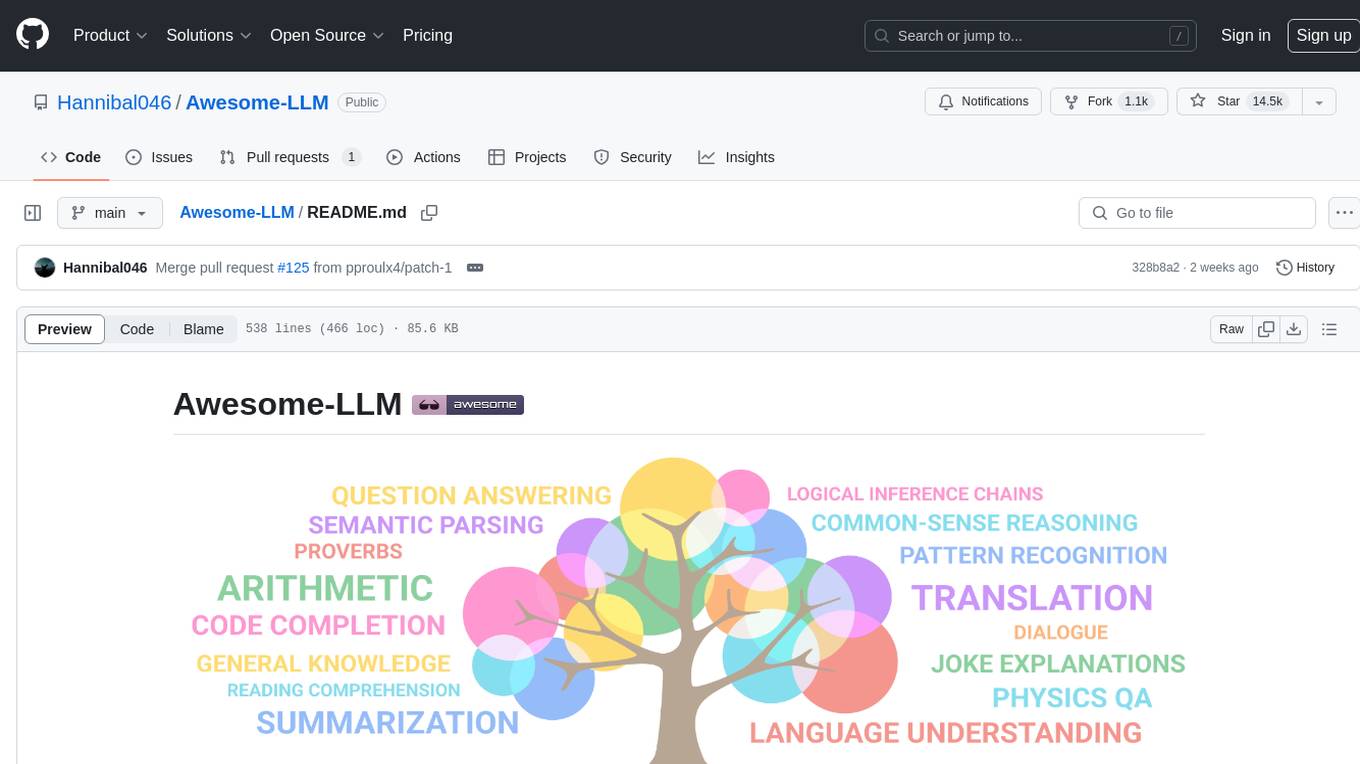
Awesome-LLM
Awesome-LLM is a curated list of resources related to large language models, focusing on papers, projects, frameworks, tools, tutorials, courses, opinions, and other useful resources in the field. It covers trending LLM projects, milestone papers, other papers, open LLM projects, LLM training frameworks, LLM evaluation frameworks, tools for deploying LLM, prompting libraries & tools, tutorials, courses, books, and opinions. The repository provides a comprehensive overview of the latest advancements and resources in the field of large language models.
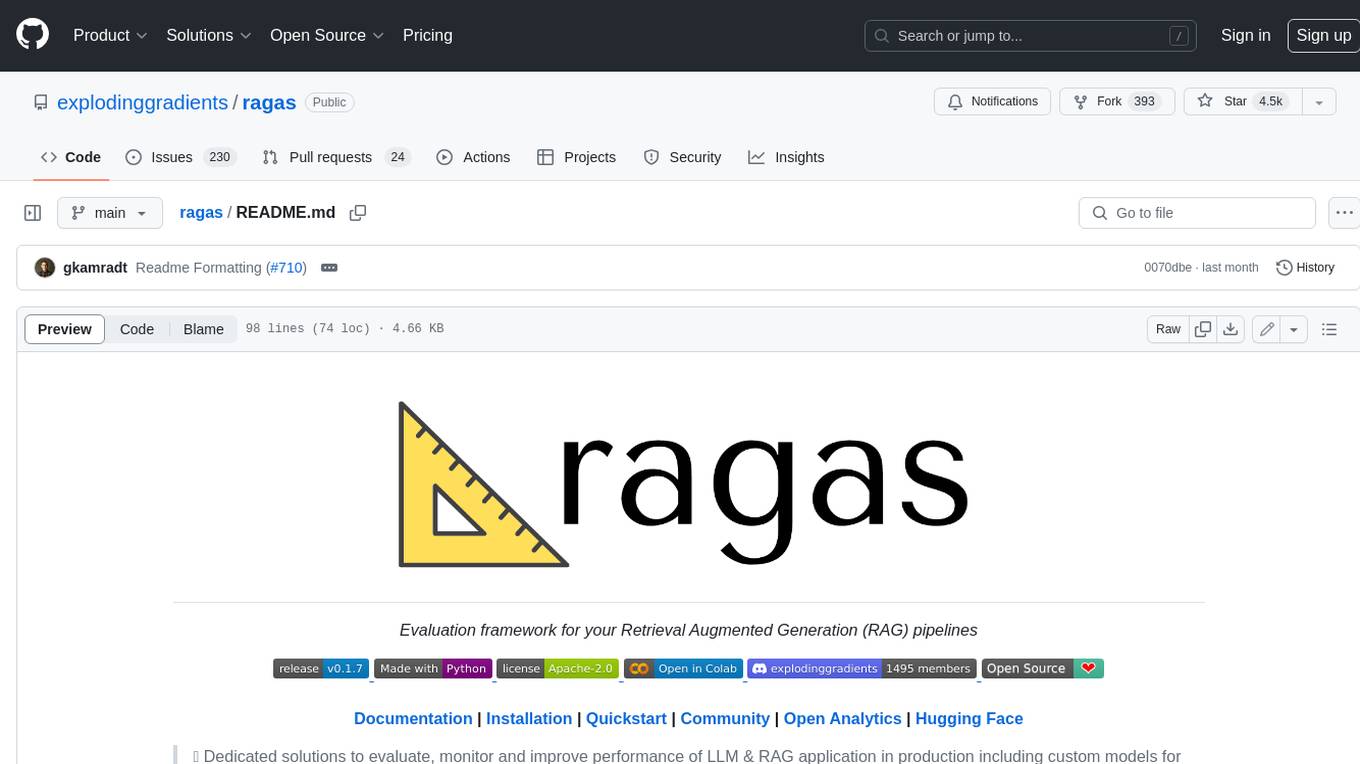
ragas
Ragas is a framework that helps you evaluate your Retrieval Augmented Generation (RAG) pipelines. RAG denotes a class of LLM applications that use external data to augment the LLM’s context. There are existing tools and frameworks that help you build these pipelines but evaluating it and quantifying your pipeline performance can be hard. This is where Ragas (RAG Assessment) comes in. Ragas provides you with the tools based on the latest research for evaluating LLM-generated text to give you insights about your RAG pipeline. Ragas can be integrated with your CI/CD to provide continuous checks to ensure performance.
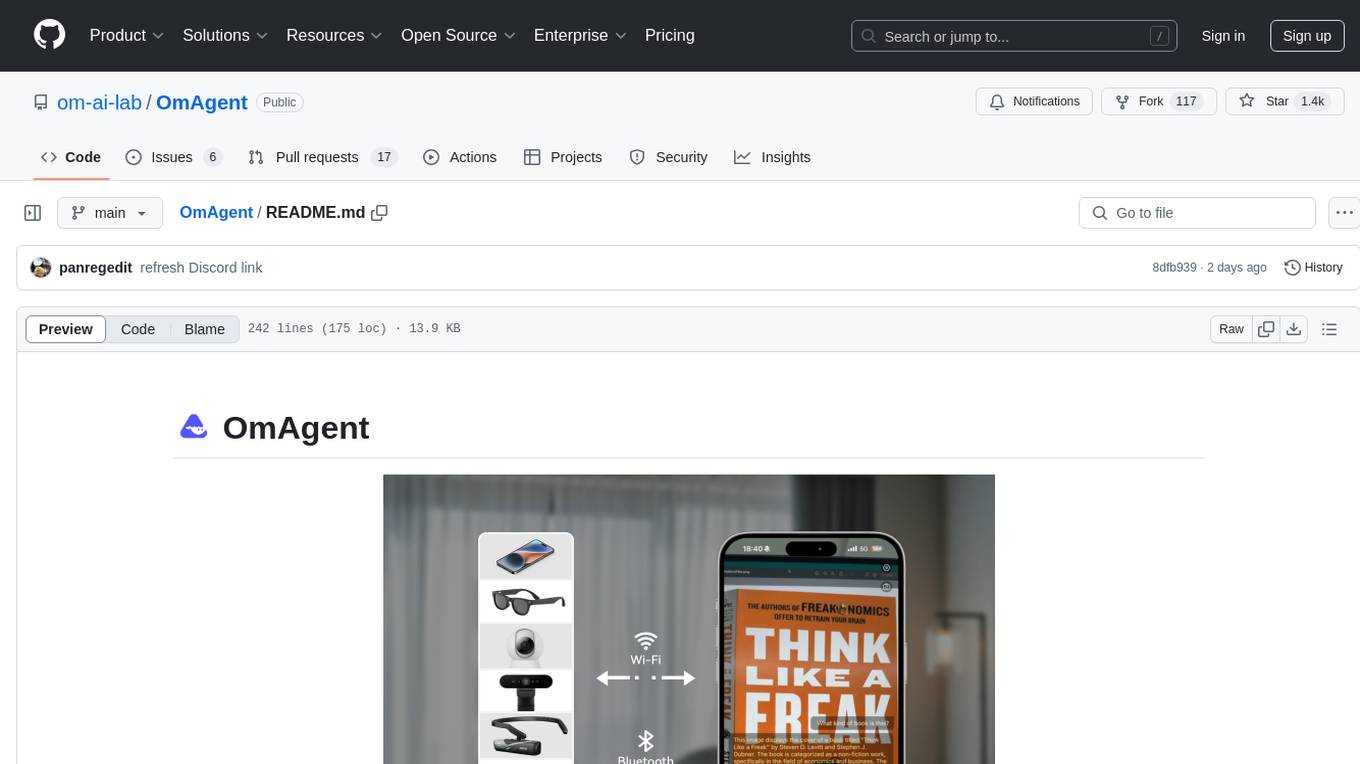
OmAgent
OmAgent is an open-source agent framework designed to streamline the development of on-device multimodal agents. It enables agents to empower various hardware devices, integrates speed-optimized SOTA multimodal models, provides SOTA multimodal agent algorithms, and focuses on optimizing the end-to-end computing pipeline for real-time user interaction experience. Key features include easy connection to diverse devices, scalability, flexibility, and workflow orchestration. The architecture emphasizes graph-based workflow orchestration, native multimodality, and device-centricity, allowing developers to create bespoke intelligent agent programs.
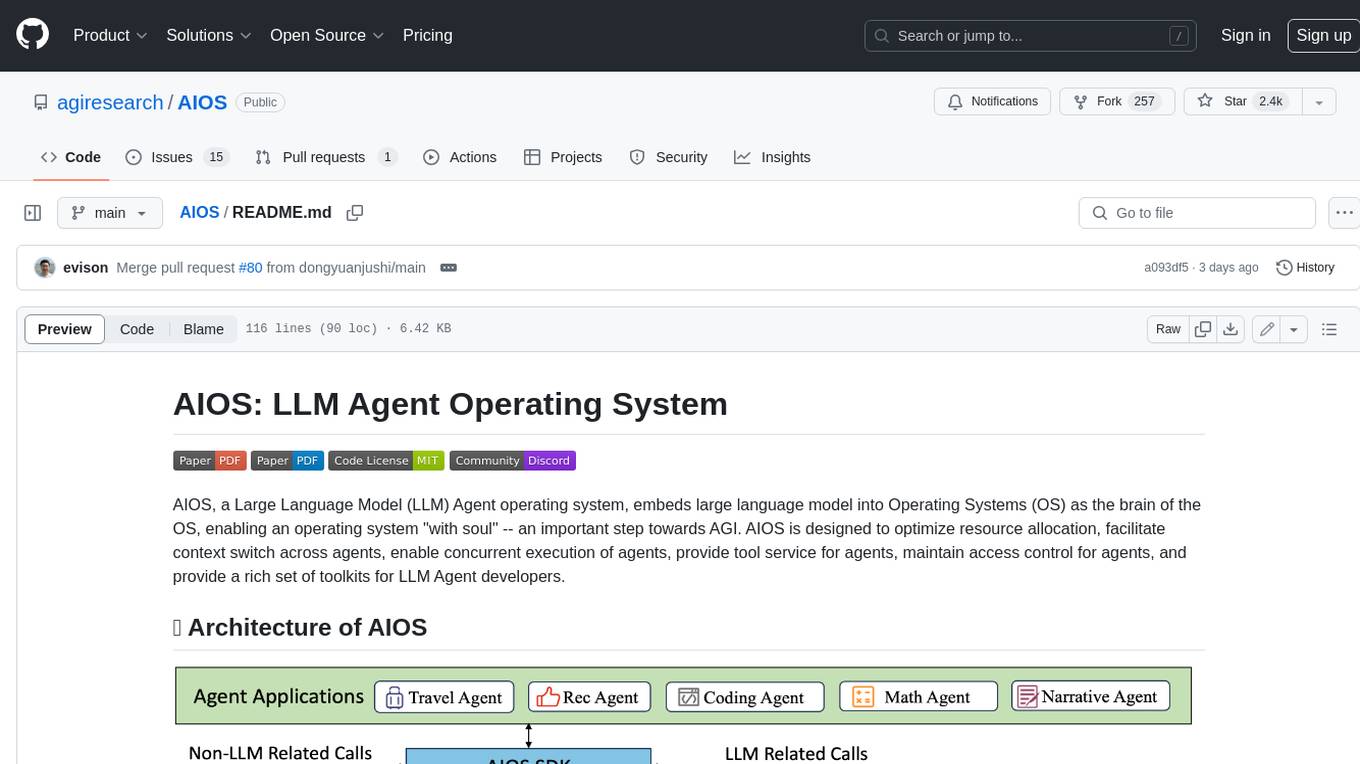
AIOS
AIOS, a Large Language Model (LLM) Agent operating system, embeds large language model into Operating Systems (OS) as the brain of the OS, enabling an operating system "with soul" -- an important step towards AGI. AIOS is designed to optimize resource allocation, facilitate context switch across agents, enable concurrent execution of agents, provide tool service for agents, maintain access control for agents, and provide a rich set of toolkits for LLM Agent developers.
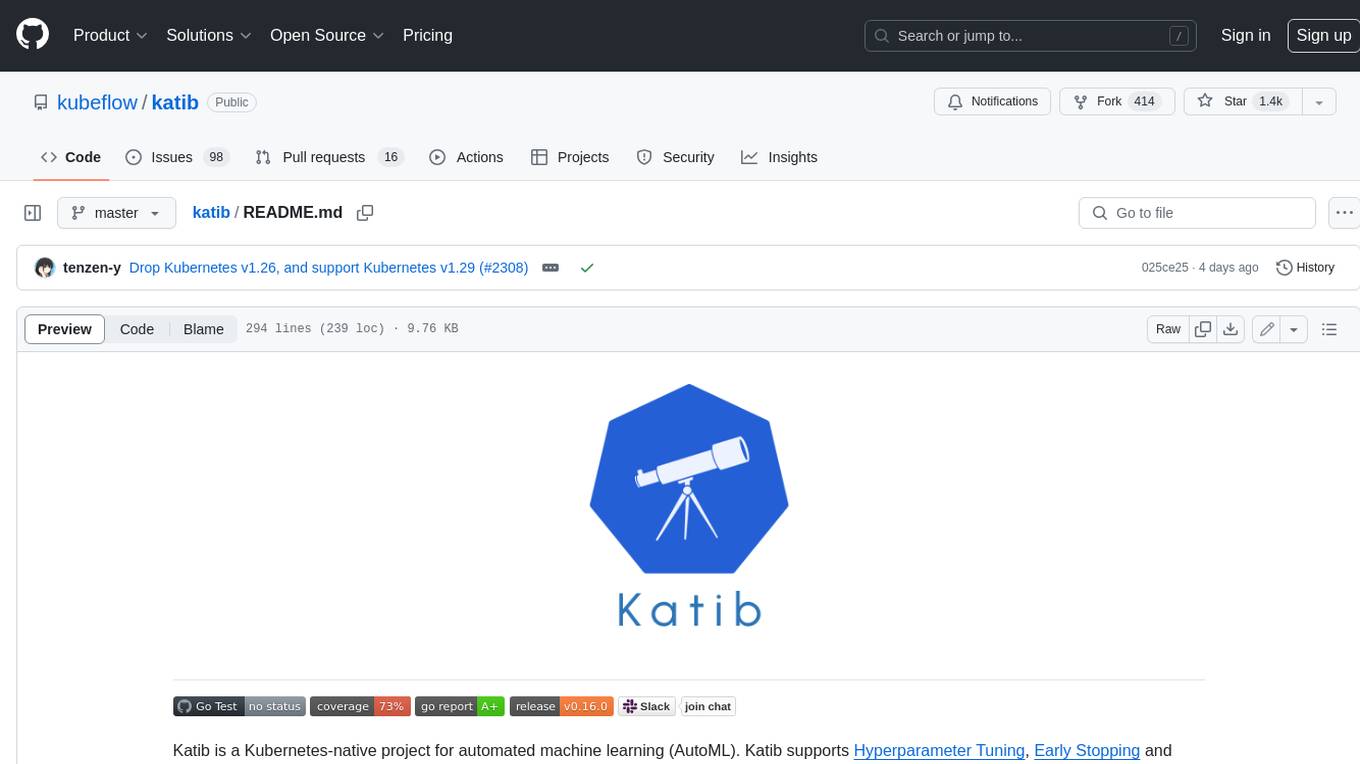
katib
Katib is a Kubernetes-native project for automated machine learning (AutoML). Katib supports Hyperparameter Tuning, Early Stopping and Neural Architecture Search. Katib is the project which is agnostic to machine learning (ML) frameworks. It can tune hyperparameters of applications written in any language of the users’ choice and natively supports many ML frameworks, such as TensorFlow, Apache MXNet, PyTorch, XGBoost, and others. Katib can perform training jobs using any Kubernetes Custom Resources with out of the box support for Kubeflow Training Operator, Argo Workflows, Tekton Pipelines and many more.
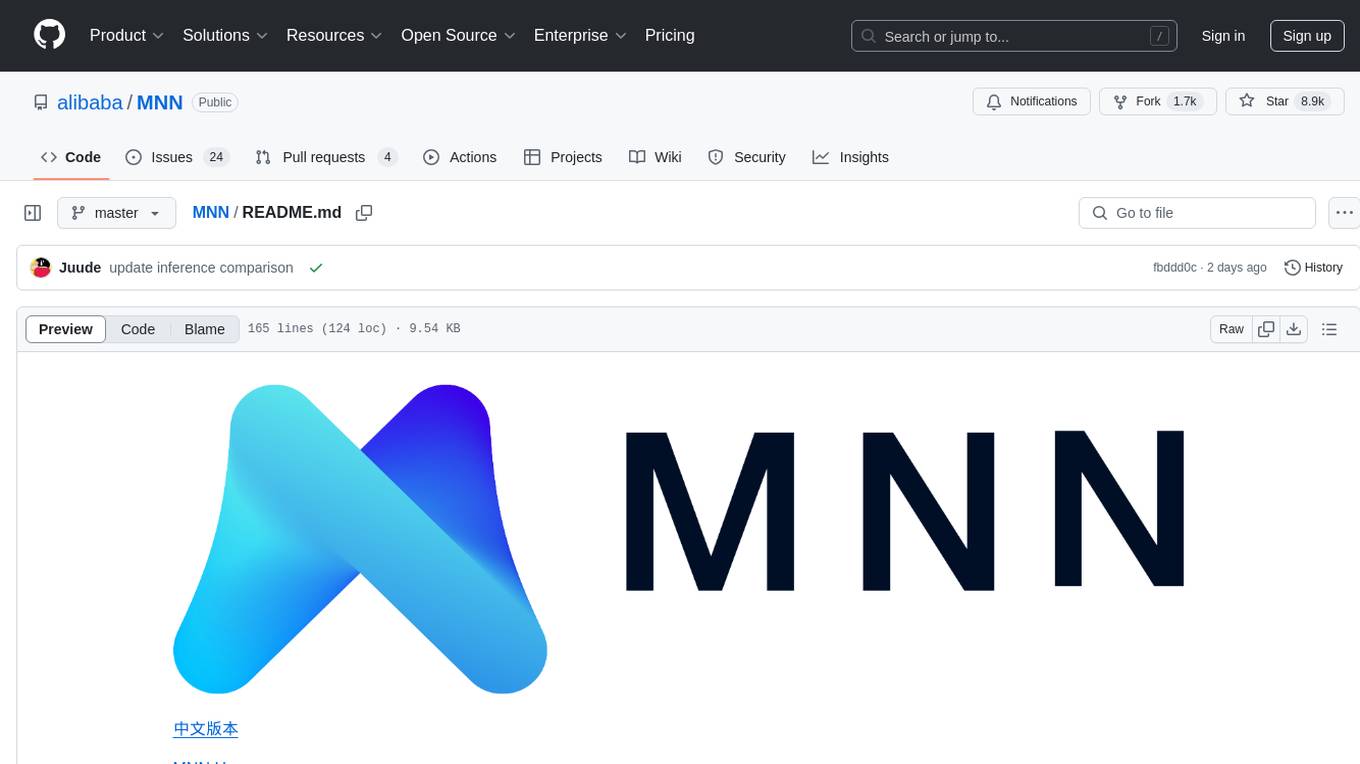
MNN
MNN is a highly efficient and lightweight deep learning framework that supports inference and training of deep learning models. It has industry-leading performance for on-device inference and training. MNN has been integrated into various Alibaba Inc. apps and is used in scenarios like live broadcast, short video capture, search recommendation, and product searching by image. It is also utilized on embedded devices such as IoT. MNN-LLM and MNN-Diffusion are specific runtime solutions developed based on the MNN engine for deploying language models and diffusion models locally on different platforms. The framework is optimized for devices, supports various neural networks, and offers high performance with optimized assembly code and GPU support. MNN is versatile, easy to use, and supports hybrid computing on multiple devices.
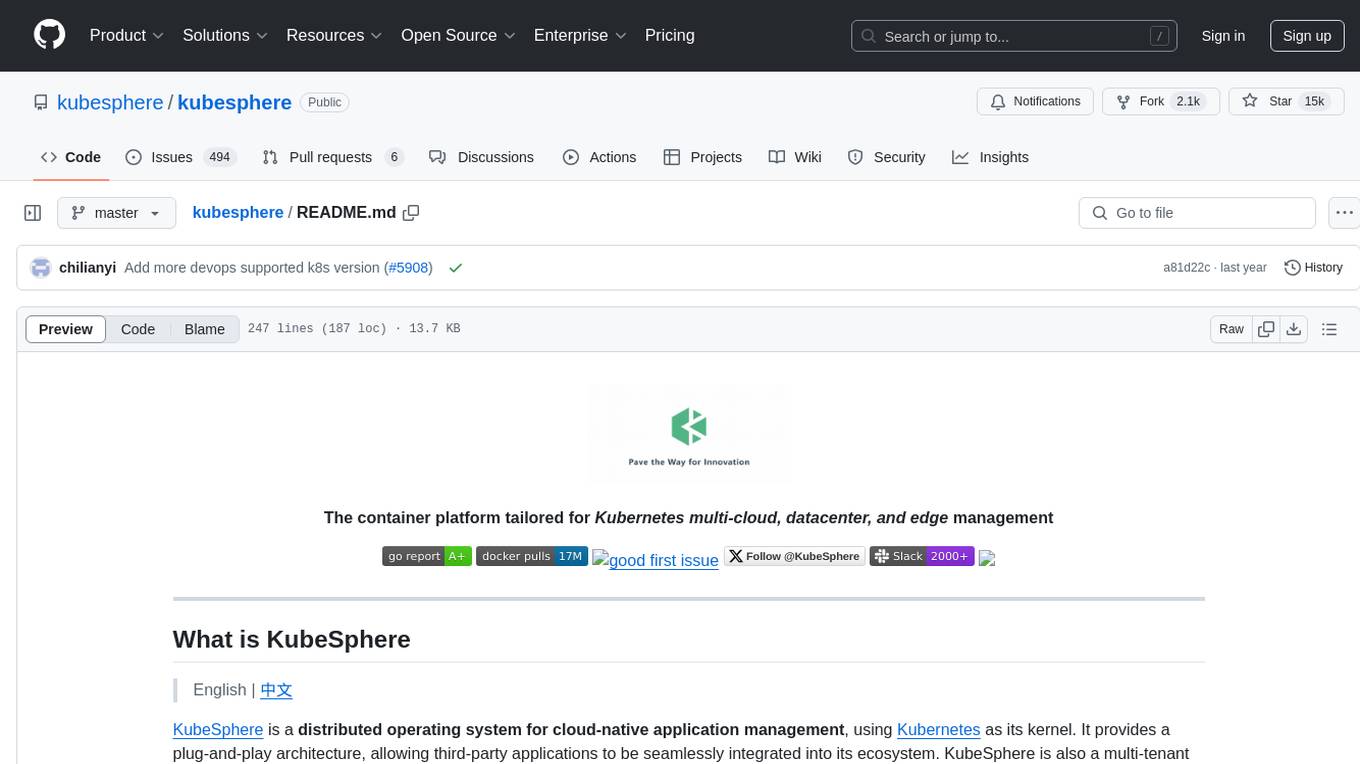
kubesphere
KubeSphere is a distributed operating system for cloud-native application management, using Kubernetes as its kernel. It provides a plug-and-play architecture, allowing third-party applications to be seamlessly integrated into its ecosystem. KubeSphere is also a multi-tenant container platform with full-stack automated IT operation and streamlined DevOps workflows. It provides developer-friendly wizard web UI, helping enterprises to build out a more robust and feature-rich platform, which includes most common functionalities needed for enterprise Kubernetes strategy.
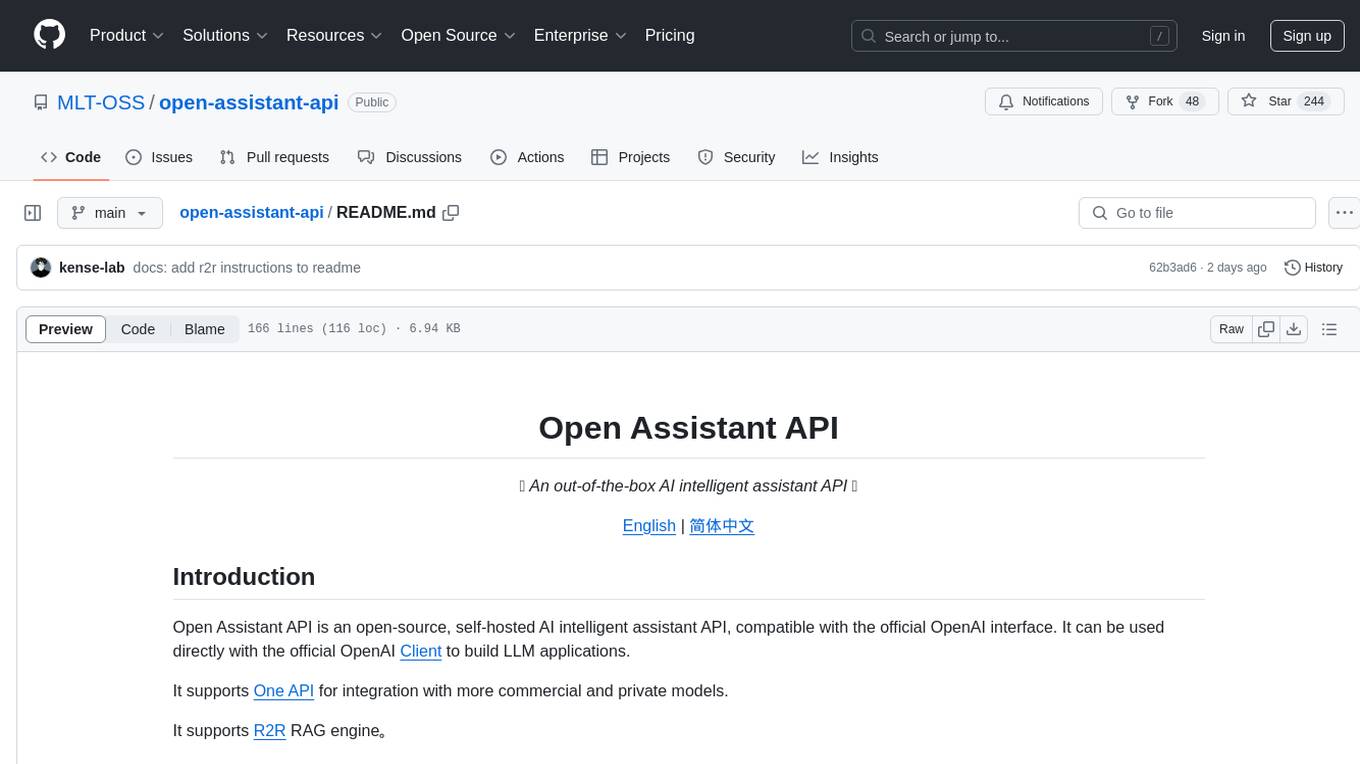
open-assistant-api
Open Assistant API is an open-source, self-hosted AI intelligent assistant API compatible with the official OpenAI interface. It supports integration with more commercial and private models, R2R RAG engine, internet search, custom functions, built-in tools, code interpreter, multimodal support, LLM support, and message streaming output. Users can deploy the service locally and expand existing features. The API provides user isolation based on tokens for SaaS deployment requirements and allows integration of various tools to enhance its capability to connect with the external world.
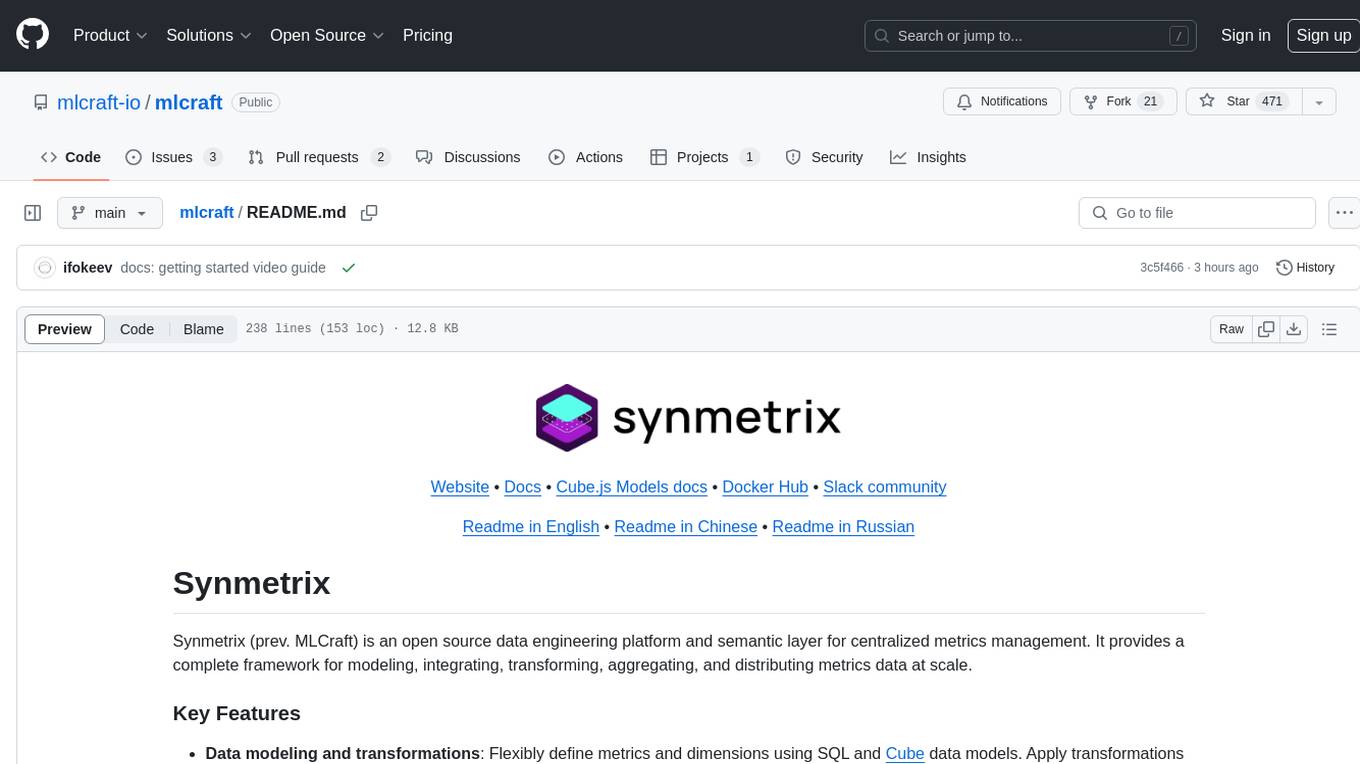
mlcraft
Synmetrix (prev. MLCraft) is an open source data engineering platform and semantic layer for centralized metrics management. It provides a complete framework for modeling, integrating, transforming, aggregating, and distributing metrics data at scale. Key features include data modeling and transformations, semantic layer for unified data model, scheduled reports and alerts, versioning, role-based access control, data exploration, caching, and collaboration on metrics modeling. Synmetrix leverages Cube (Cube.js) for flexible data models that consolidate metrics from various sources, enabling downstream distribution via a SQL API for integration into BI tools, reporting, dashboards, and data science. Use cases include data democratization, business intelligence, embedded analytics, and enhancing accuracy in data handling and queries. The tool speeds up data-driven workflows from metrics definition to consumption by combining data engineering best practices with self-service analytics capabilities.
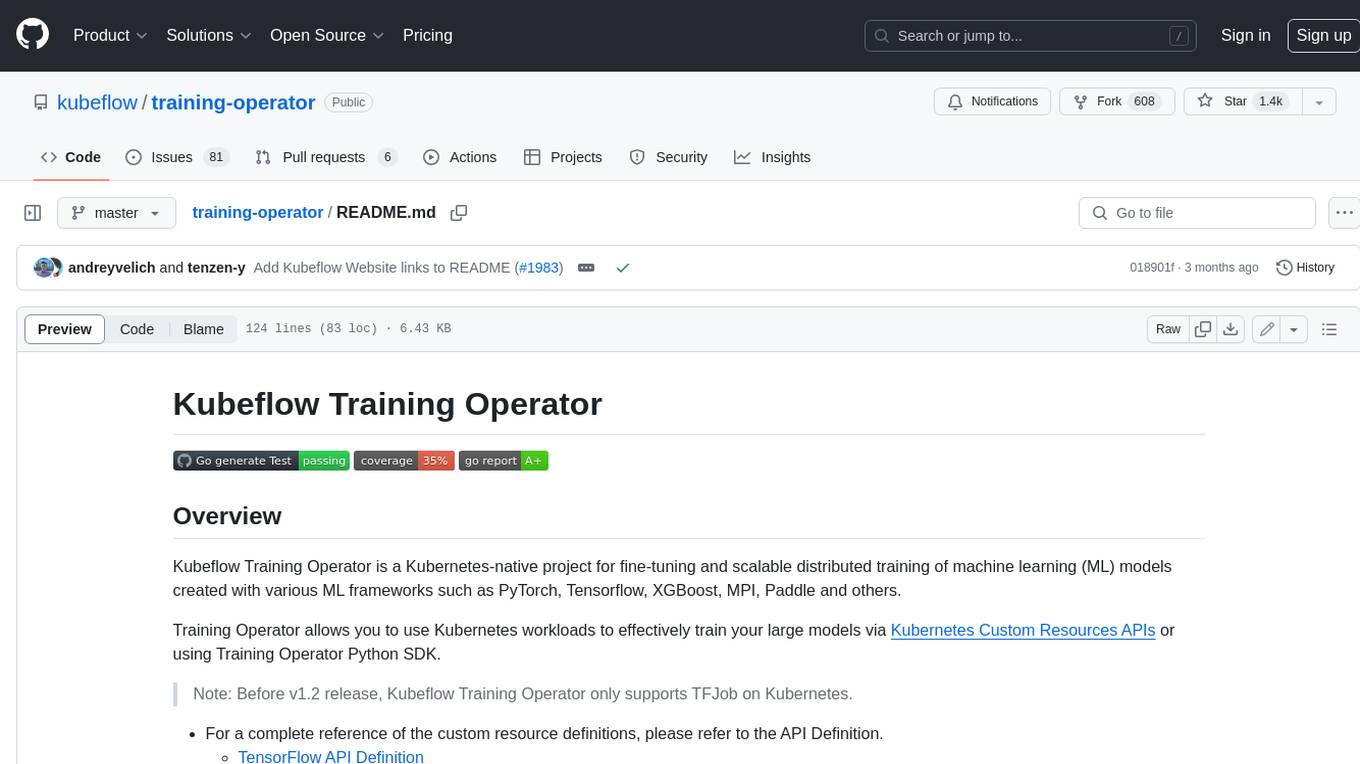
training-operator
Kubeflow Training Operator is a Kubernetes-native project for fine-tuning and scalable distributed training of machine learning (ML) models created with various ML frameworks such as PyTorch, Tensorflow, XGBoost, MPI, Paddle and others. Training Operator allows you to use Kubernetes workloads to effectively train your large models via Kubernetes Custom Resources APIs or using Training Operator Python SDK. > Note: Before v1.2 release, Kubeflow Training Operator only supports TFJob on Kubernetes. * For a complete reference of the custom resource definitions, please refer to the API Definition. * TensorFlow API Definition * PyTorch API Definition * Apache MXNet API Definition * XGBoost API Definition * MPI API Definition * PaddlePaddle API Definition * For details of all-in-one operator design, please refer to the All-in-one Kubeflow Training Operator * For details on its observability, please refer to the monitoring design doc.
For similar tasks
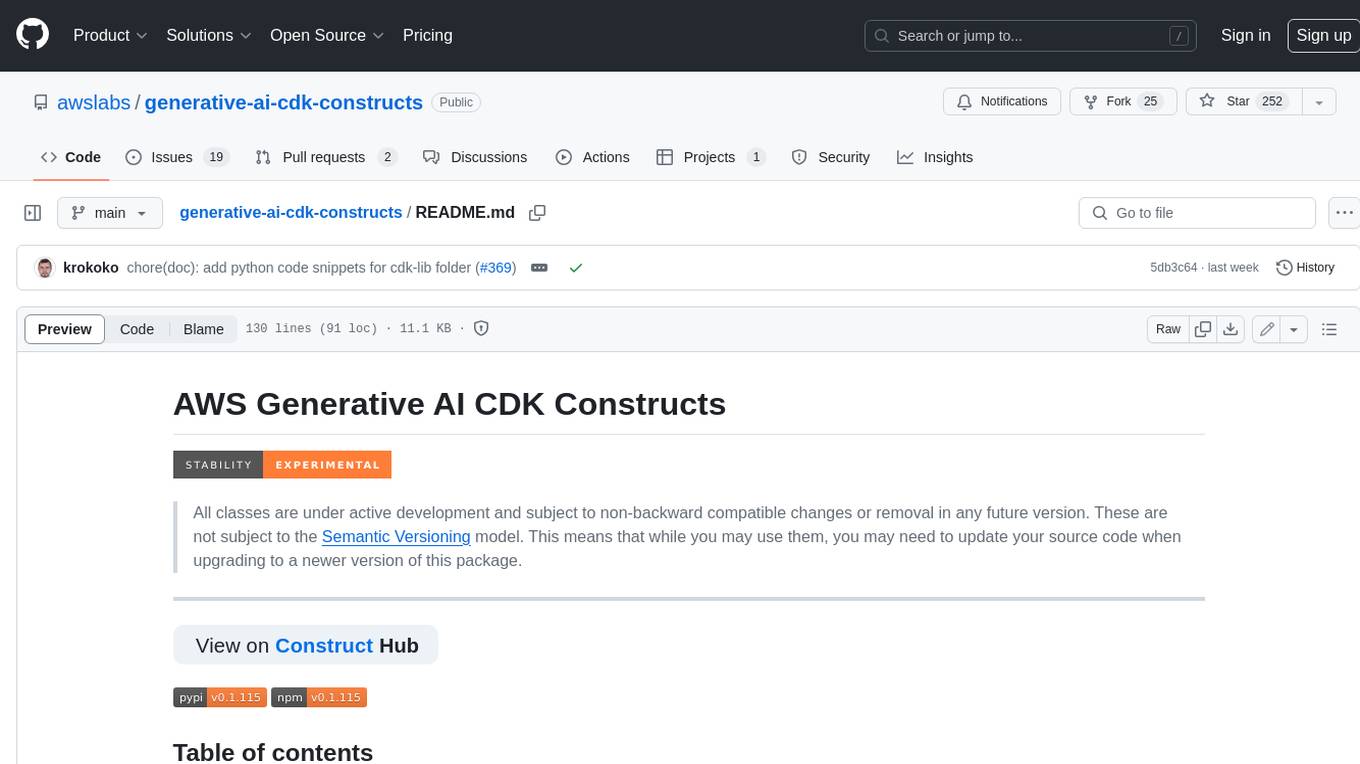
generative-ai-cdk-constructs
The AWS Generative AI Constructs Library is an open-source extension of the AWS Cloud Development Kit (AWS CDK) that provides multi-service, well-architected patterns for quickly defining solutions in code to create predictable and repeatable infrastructure, called constructs. The goal of AWS Generative AI CDK Constructs is to help developers build generative AI solutions using pattern-based definitions for their architecture. The patterns defined in AWS Generative AI CDK Constructs are high level, multi-service abstractions of AWS CDK constructs that have default configurations based on well-architected best practices. The library is organized into logical modules using object-oriented techniques to create each architectural pattern model.
For similar jobs
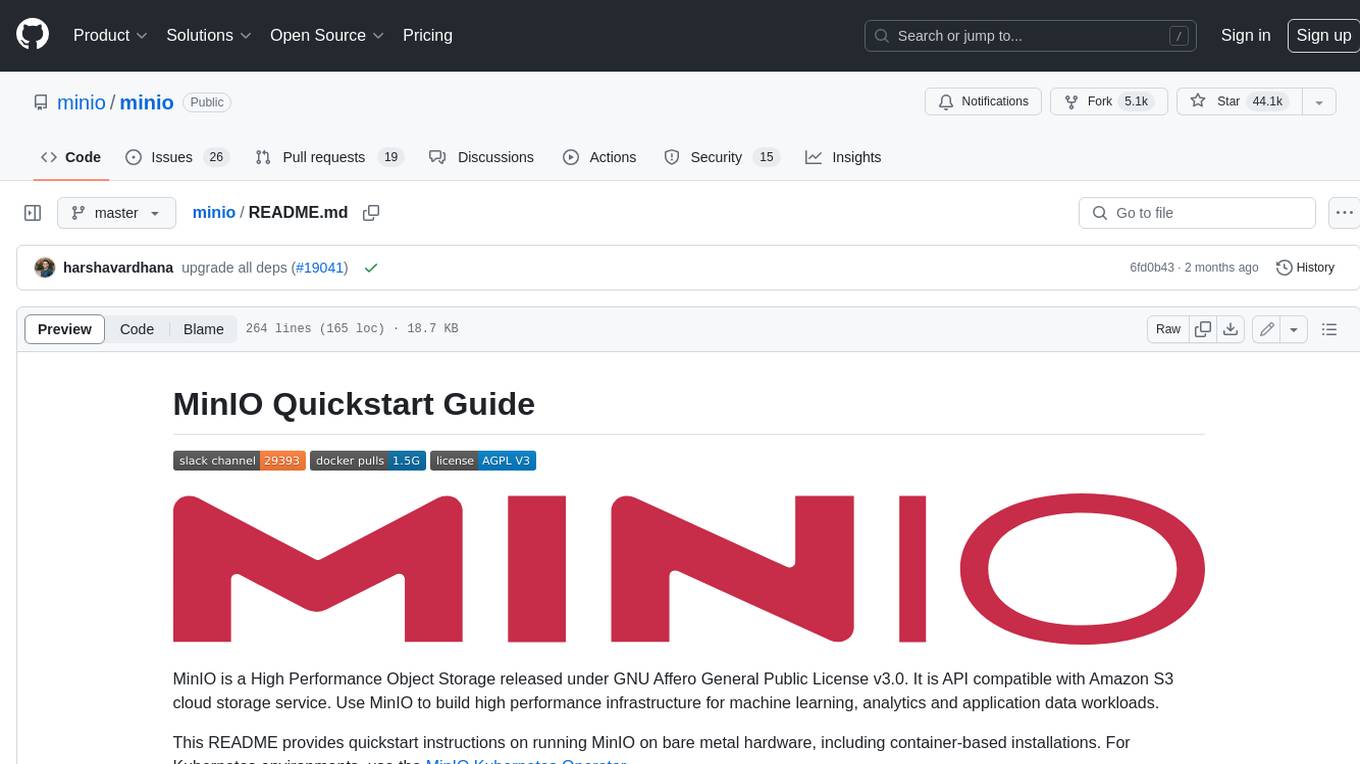
minio
MinIO is a High Performance Object Storage released under GNU Affero General Public License v3.0. It is API compatible with Amazon S3 cloud storage service. Use MinIO to build high performance infrastructure for machine learning, analytics and application data workloads.
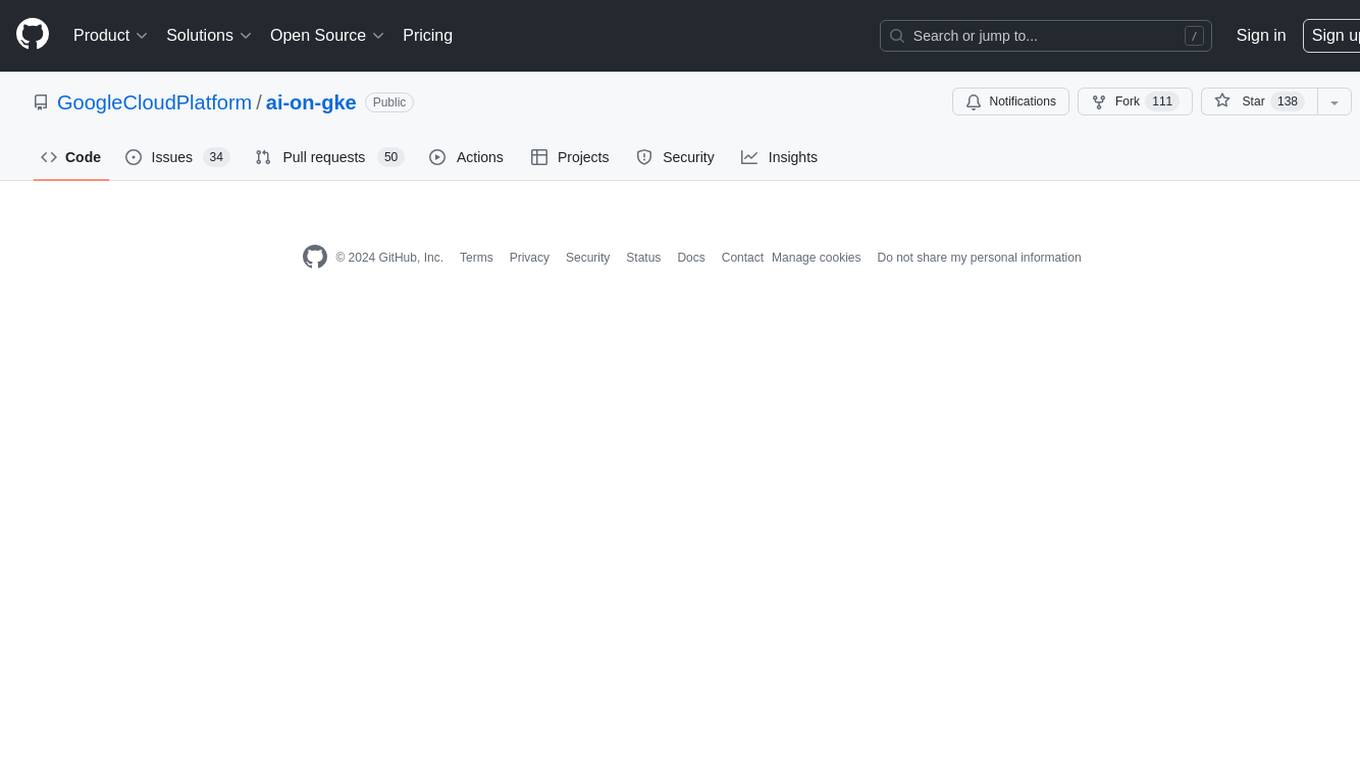
ai-on-gke
This repository contains assets related to AI/ML workloads on Google Kubernetes Engine (GKE). Run optimized AI/ML workloads with Google Kubernetes Engine (GKE) platform orchestration capabilities. A robust AI/ML platform considers the following layers: Infrastructure orchestration that support GPUs and TPUs for training and serving workloads at scale Flexible integration with distributed computing and data processing frameworks Support for multiple teams on the same infrastructure to maximize utilization of resources
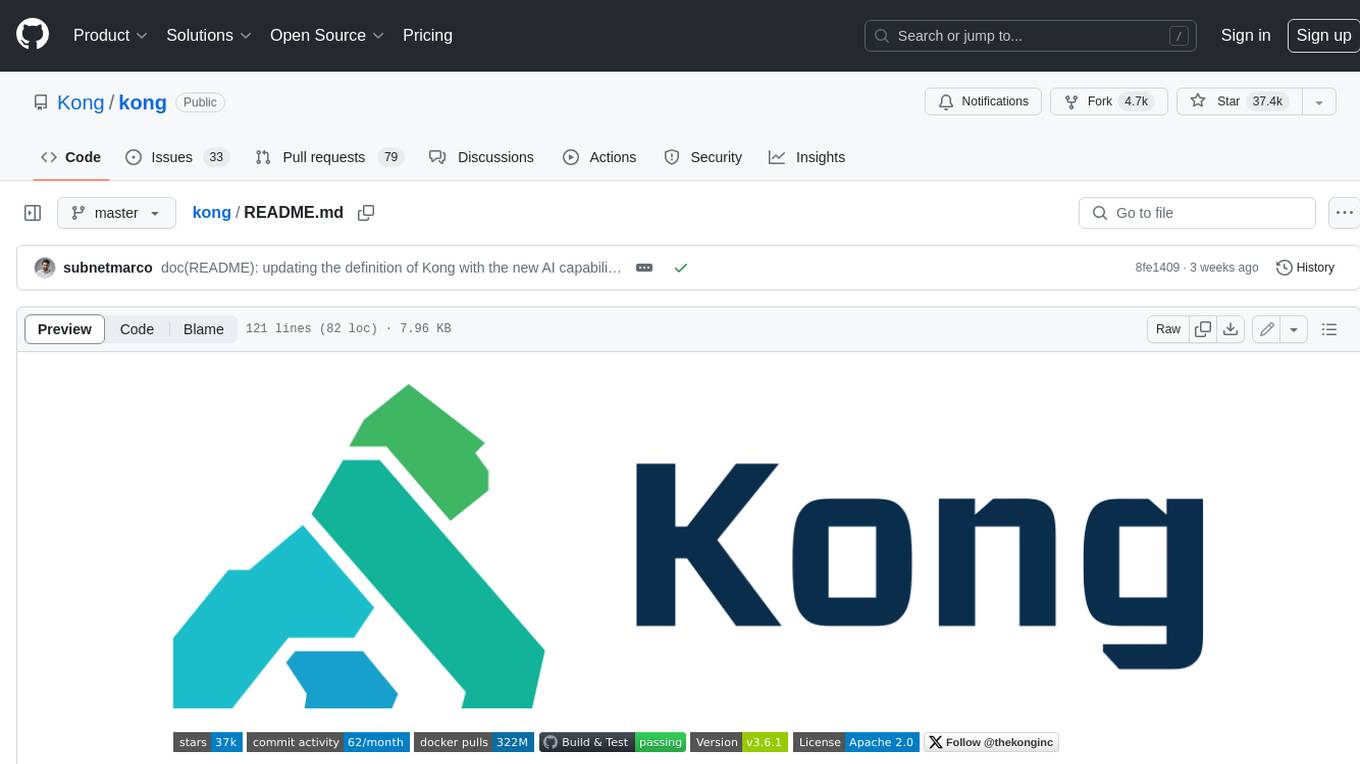
kong
Kong, or Kong API Gateway, is a cloud-native, platform-agnostic, scalable API Gateway distinguished for its high performance and extensibility via plugins. It also provides advanced AI capabilities with multi-LLM support. By providing functionality for proxying, routing, load balancing, health checking, authentication (and more), Kong serves as the central layer for orchestrating microservices or conventional API traffic with ease. Kong runs natively on Kubernetes thanks to its official Kubernetes Ingress Controller.
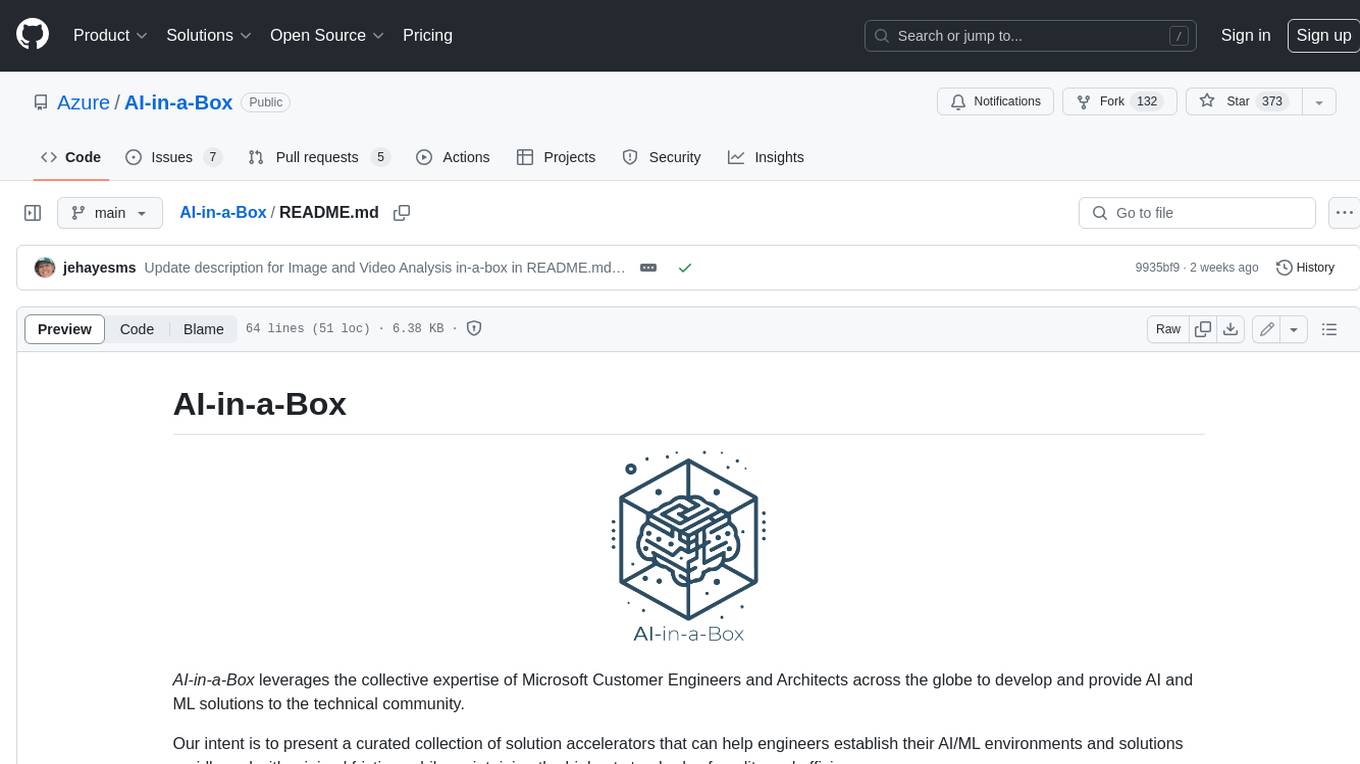
AI-in-a-Box
AI-in-a-Box is a curated collection of solution accelerators that can help engineers establish their AI/ML environments and solutions rapidly and with minimal friction, while maintaining the highest standards of quality and efficiency. It provides essential guidance on the responsible use of AI and LLM technologies, specific security guidance for Generative AI (GenAI) applications, and best practices for scaling OpenAI applications within Azure. The available accelerators include: Azure ML Operationalization in-a-box, Edge AI in-a-box, Doc Intelligence in-a-box, Image and Video Analysis in-a-box, Cognitive Services Landing Zone in-a-box, Semantic Kernel Bot in-a-box, NLP to SQL in-a-box, Assistants API in-a-box, and Assistants API Bot in-a-box.
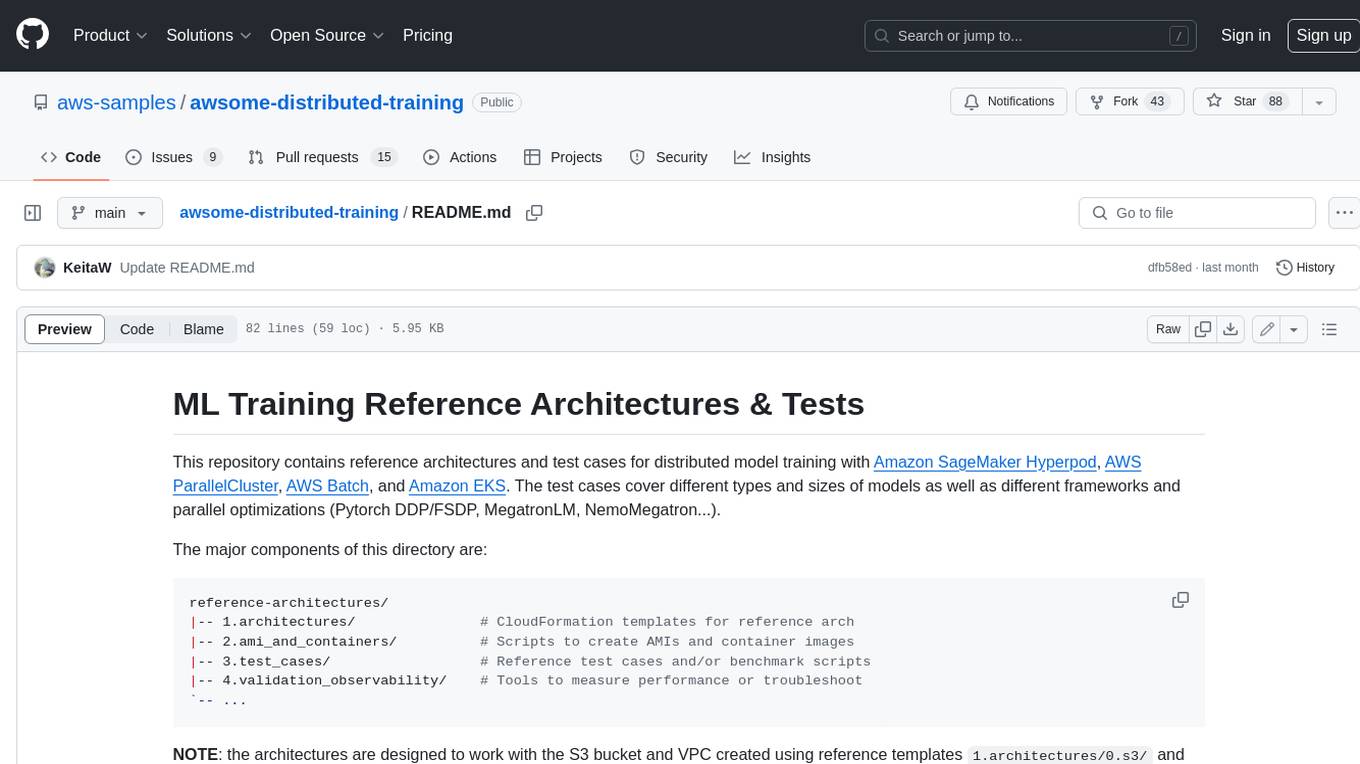
awsome-distributed-training
This repository contains reference architectures and test cases for distributed model training with Amazon SageMaker Hyperpod, AWS ParallelCluster, AWS Batch, and Amazon EKS. The test cases cover different types and sizes of models as well as different frameworks and parallel optimizations (Pytorch DDP/FSDP, MegatronLM, NemoMegatron...).
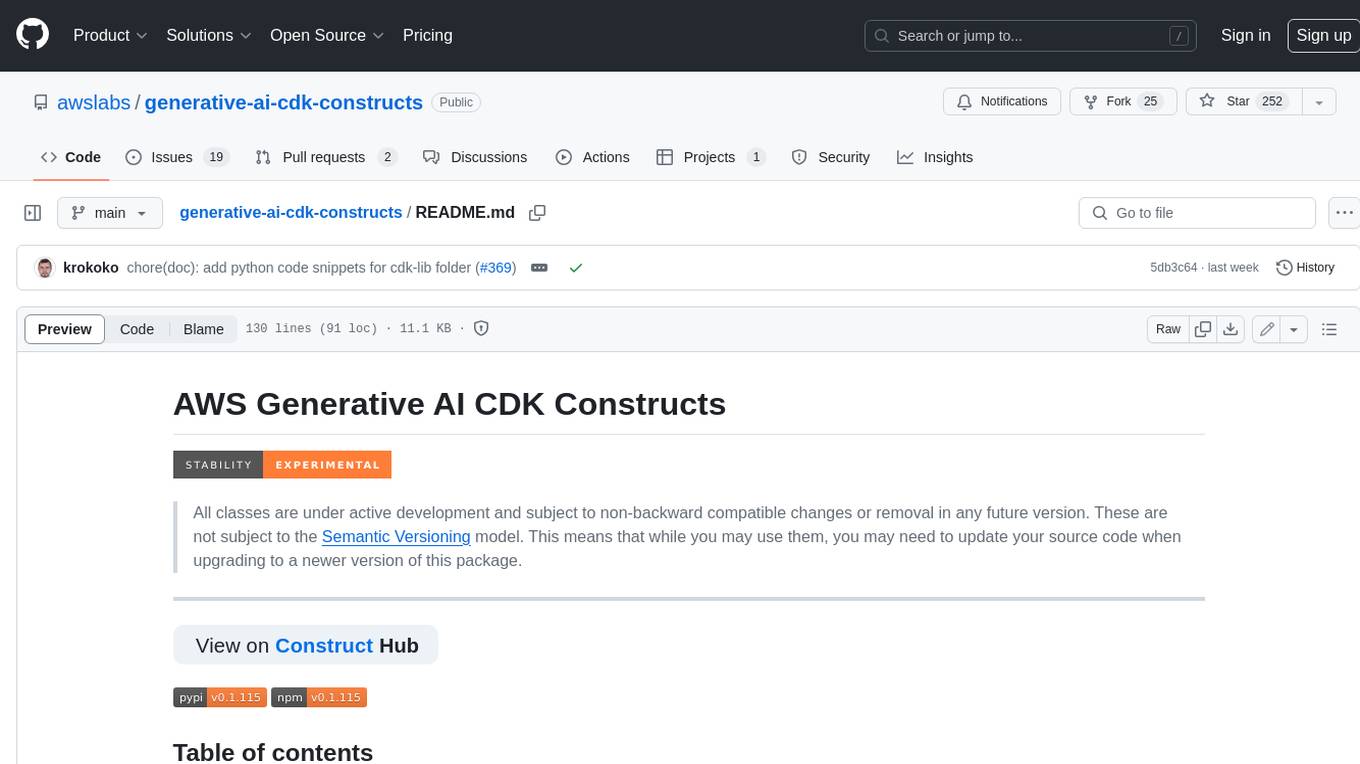
generative-ai-cdk-constructs
The AWS Generative AI Constructs Library is an open-source extension of the AWS Cloud Development Kit (AWS CDK) that provides multi-service, well-architected patterns for quickly defining solutions in code to create predictable and repeatable infrastructure, called constructs. The goal of AWS Generative AI CDK Constructs is to help developers build generative AI solutions using pattern-based definitions for their architecture. The patterns defined in AWS Generative AI CDK Constructs are high level, multi-service abstractions of AWS CDK constructs that have default configurations based on well-architected best practices. The library is organized into logical modules using object-oriented techniques to create each architectural pattern model.
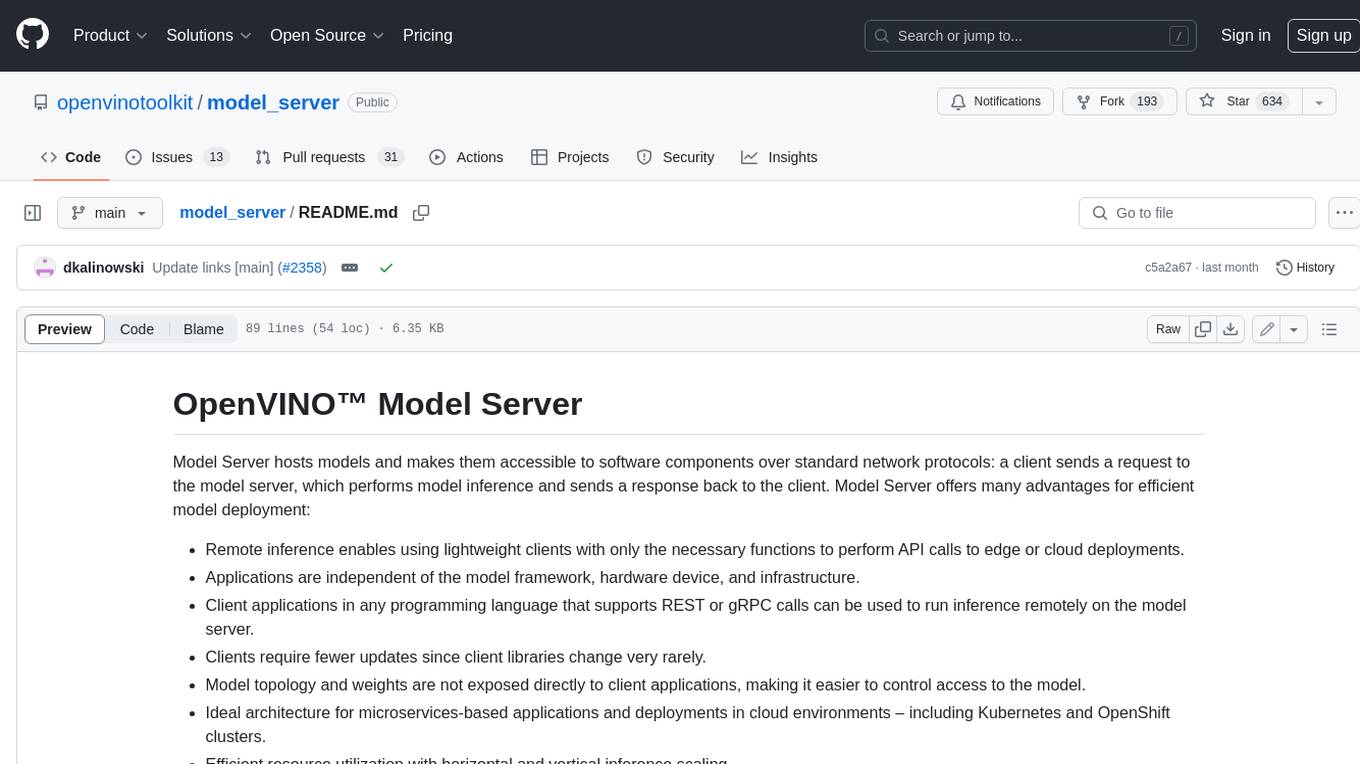
model_server
OpenVINO™ Model Server (OVMS) is a high-performance system for serving models. Implemented in C++ for scalability and optimized for deployment on Intel architectures, the model server uses the same architecture and API as TensorFlow Serving and KServe while applying OpenVINO for inference execution. Inference service is provided via gRPC or REST API, making deploying new algorithms and AI experiments easy.
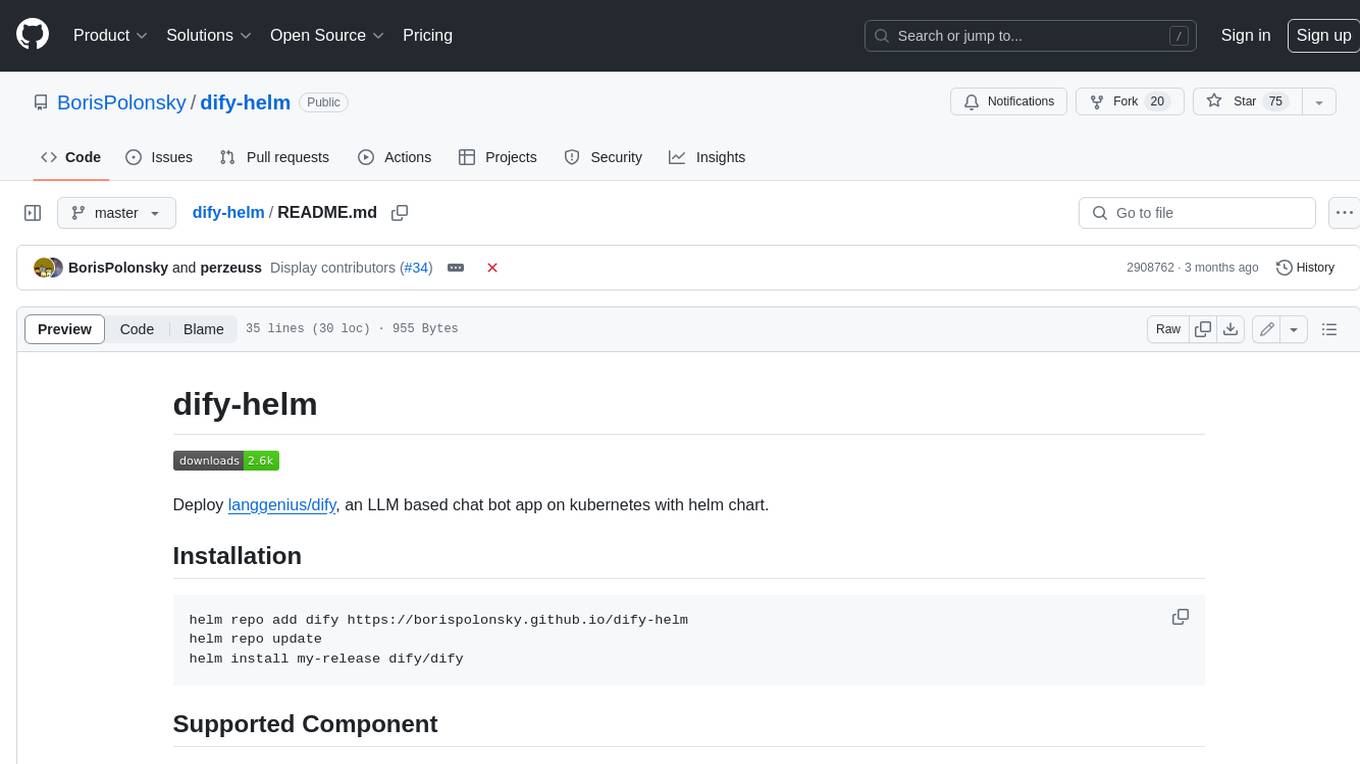
dify-helm
Deploy langgenius/dify, an LLM based chat bot app on kubernetes with helm chart.