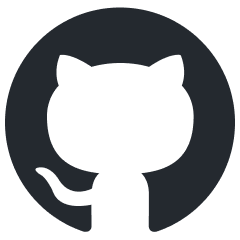
FBP
FBP项目全称FootBallPrediction,历经9个月完成的足球比赛预测项目。项目结合大数据+机器学习,不断摸索开发了一个程序。程序根据各大公司赔率多维度预测足球比赛结果(包含胜和不胜)。机器学习用的是自己建立的“三木板模型”算法,已在国家期刊发表论文并被万方数据库收录,详见_ML_文件。目前准确率可达80%。该项目在自己创建的微信群里已经吸引了很多人,附件为群讨论截图,并且每天均有部分人根据预测结果参考投注竞彩,参考的人都获得了相应的收益。 现在想通过认识更多的有识之士,一起探索如何将项目做大做强,找到合伙人,实现共赢。希望感兴趣的同仁联系本人,微信号acredjb。公众号AI金胆(或AI-FBP),每天都有程序预测的足球比赛。程序优势请看Advantages和README文件。程序3.0版本:(第三轮目前13中12) 8月10日:13让负(正确) 8月11日:27让负(正确) 8月12日:11让负(正确) 8月13日:6胜(不正确) 8月14日:25让负(正确) 8月15日:无预测 8月16日:1胜(正确) 8月17日:6让负(正确) 8月18日:16胜(正确) 8月19日:34让负(正确) ... 1.0版本(第一轮为11中9) 2.0版本(第二轮13中11).
Stars: 417
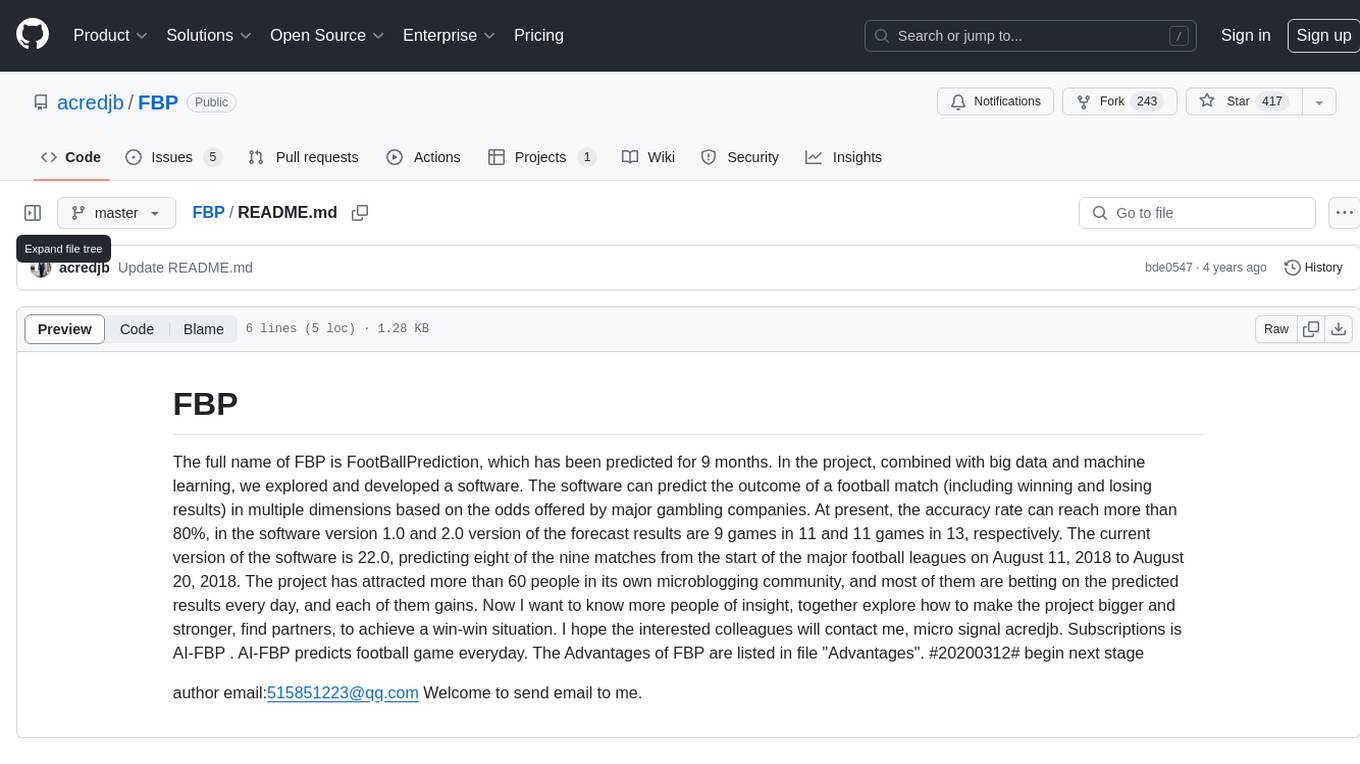
FootBallPrediction (FBP) is a software project that utilizes big data and machine learning to predict the outcome of football matches based on odds from gambling companies. The software has achieved an accuracy rate of over 80% in predicting match results. The current version, 22.0, successfully predicted eight out of nine matches from major football leagues. The project has a community of over 60 members who benefit from the predicted results. The author is seeking collaboration to further enhance the project and welcomes interested individuals to join. AI-FBP is a subscription service that provides daily football game predictions.
README:
The full name of FBP is FootBallPrediction, which has been predicted for 9 months. In the project, combined with big data and machine learning, we explored and developed a software. The software can predict the outcome of a football match (including winning and losing results) in multiple dimensions based on the odds offered by major gambling companies. At present, the accuracy rate can reach more than 80%, in the software version 1.0 and 2.0 version of the forecast results are 9 games in 11 and 11 games in 13, respectively. The current version of the software is 22.0, predicting eight of the nine matches from the start of the major football leagues on August 11, 2018 to August 20, 2018. The project has attracted more than 60 people in its own microblogging community, and most of them are betting on the predicted results every day, and each of them gains. Now I want to know more people of insight, together explore how to make the project bigger and stronger, find partners, to achieve a win-win situation. I hope the interested colleagues will contact me, micro signal acredjb. Subscriptions is AI-FBP . AI-FBP predicts football game everyday. The Advantages of FBP are listed in file "Advantages". #20200312# begin next stage
author email:[email protected] Welcome to send email to me.
For Tasks:
Click tags to check more tools for each tasksFor Jobs:
Alternative AI tools for FBP
Similar Open Source Tools
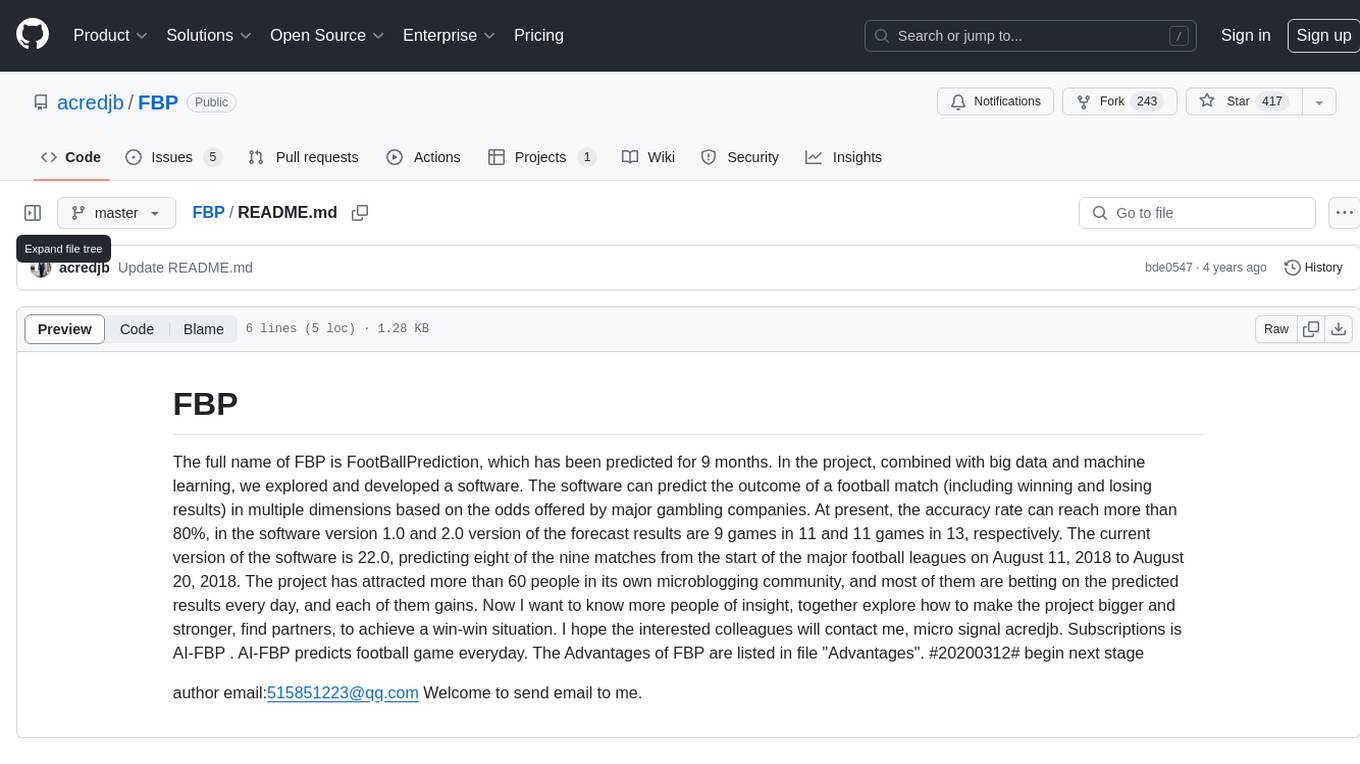
FBP
FootBallPrediction (FBP) is a software project that utilizes big data and machine learning to predict the outcome of football matches based on odds from gambling companies. The software has achieved an accuracy rate of over 80% in predicting match results. The current version, 22.0, successfully predicted eight out of nine matches from major football leagues. The project has a community of over 60 members who benefit from the predicted results. The author is seeking collaboration to further enhance the project and welcomes interested individuals to join. AI-FBP is a subscription service that provides daily football game predictions.
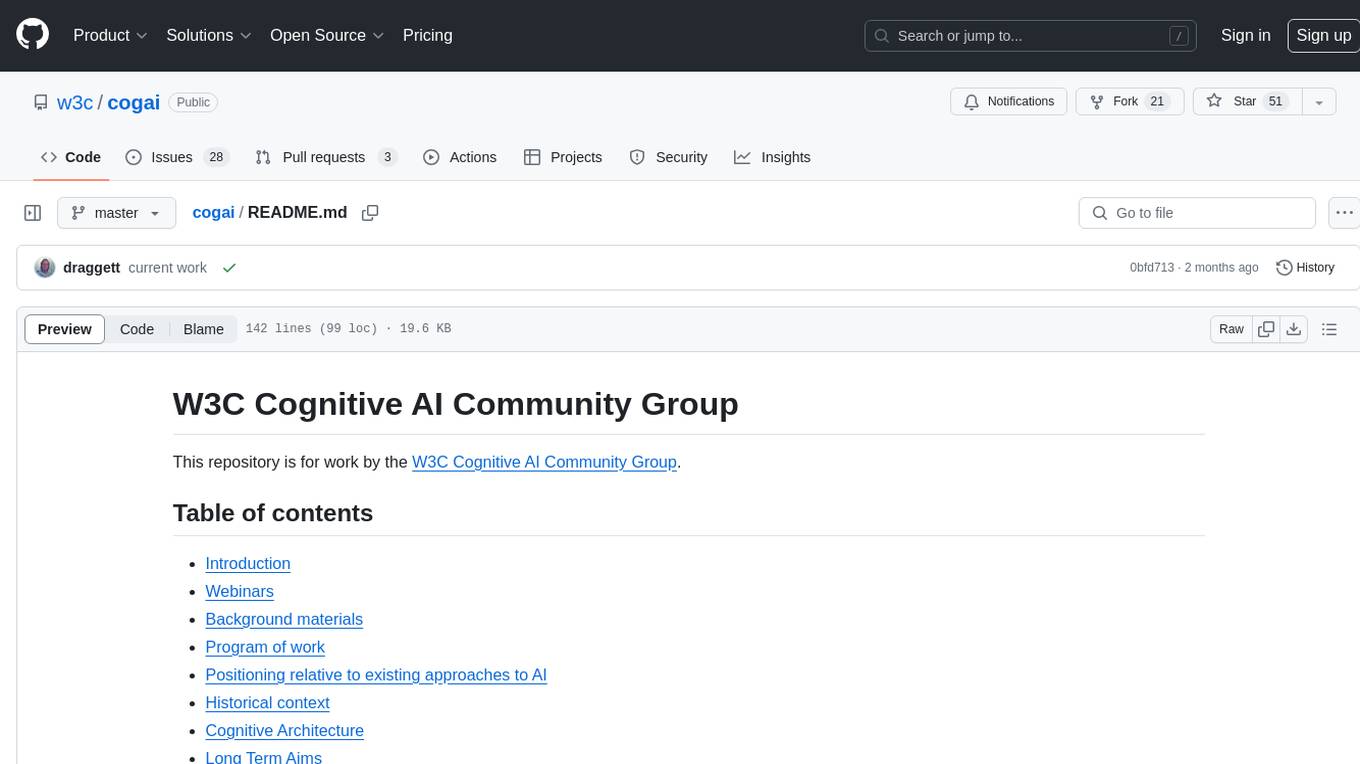
cogai
The W3C Cognitive AI Community Group focuses on advancing Cognitive AI through collaboration on defining use cases, open source implementations, and application areas. The group aims to demonstrate the potential of Cognitive AI in various domains such as customer services, healthcare, cybersecurity, online learning, autonomous vehicles, manufacturing, and web search. They work on formal specifications for chunk data and rules, plausible knowledge notation, and neural networks for human-like AI. The group positions Cognitive AI as a combination of symbolic and statistical approaches inspired by human thought processes. They address research challenges including mimicry, emotional intelligence, natural language processing, and common sense reasoning. The long-term goal is to develop cognitive agents that are knowledgeable, creative, collaborative, empathic, and multilingual, capable of continual learning and self-awareness.
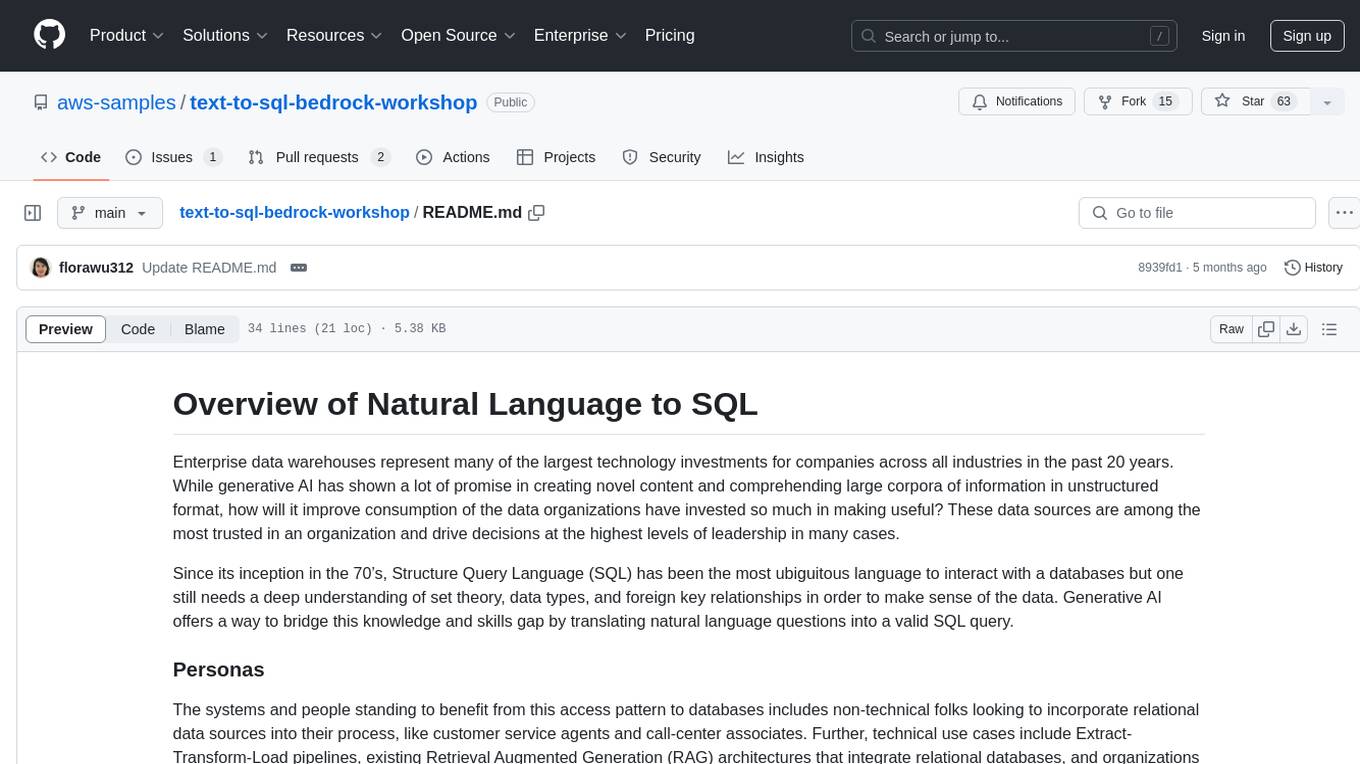
text-to-sql-bedrock-workshop
This repository focuses on utilizing generative AI to bridge the gap between natural language questions and SQL queries, aiming to improve data consumption in enterprise data warehouses. It addresses challenges in SQL query generation, such as foreign key relationships and table joins, and highlights the importance of accuracy metrics like Execution Accuracy (EX) and Exact Set Match Accuracy (EM). The workshop content covers advanced prompt engineering, Retrieval Augmented Generation (RAG), fine-tuning models, and security measures against prompt and SQL injections.
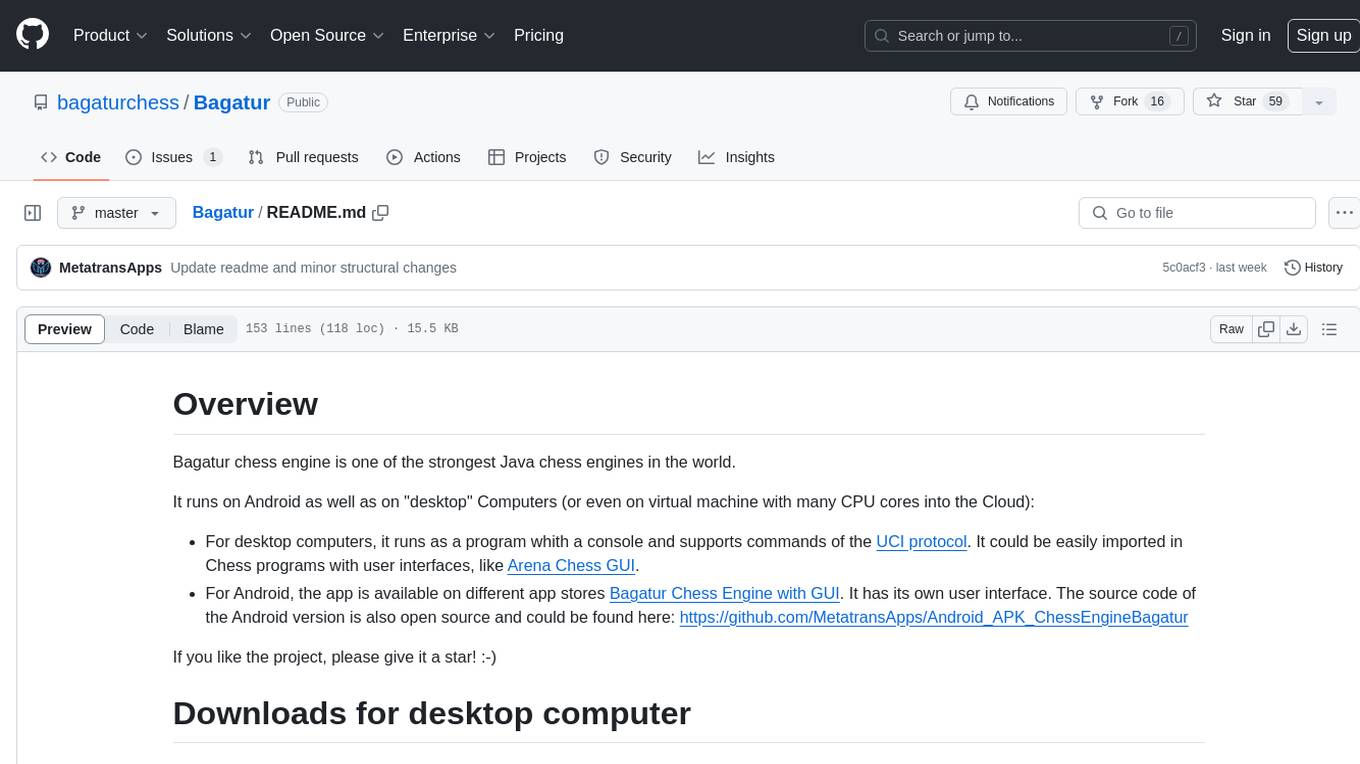
Bagatur
Bagatur chess engine is a powerful Java chess engine that can run on Android devices and desktop computers. It supports the UCI protocol and can be easily integrated into chess programs with user interfaces. The engine is available for download on various platforms and has advanced features like SMP (multicore) support and NNUE evaluation function. Bagatur also includes syzygy endgame tablebases and offers various UCI options for customization. The project started as a personal challenge to create a chess program that could defeat a friend, leading to years of development and improvements.
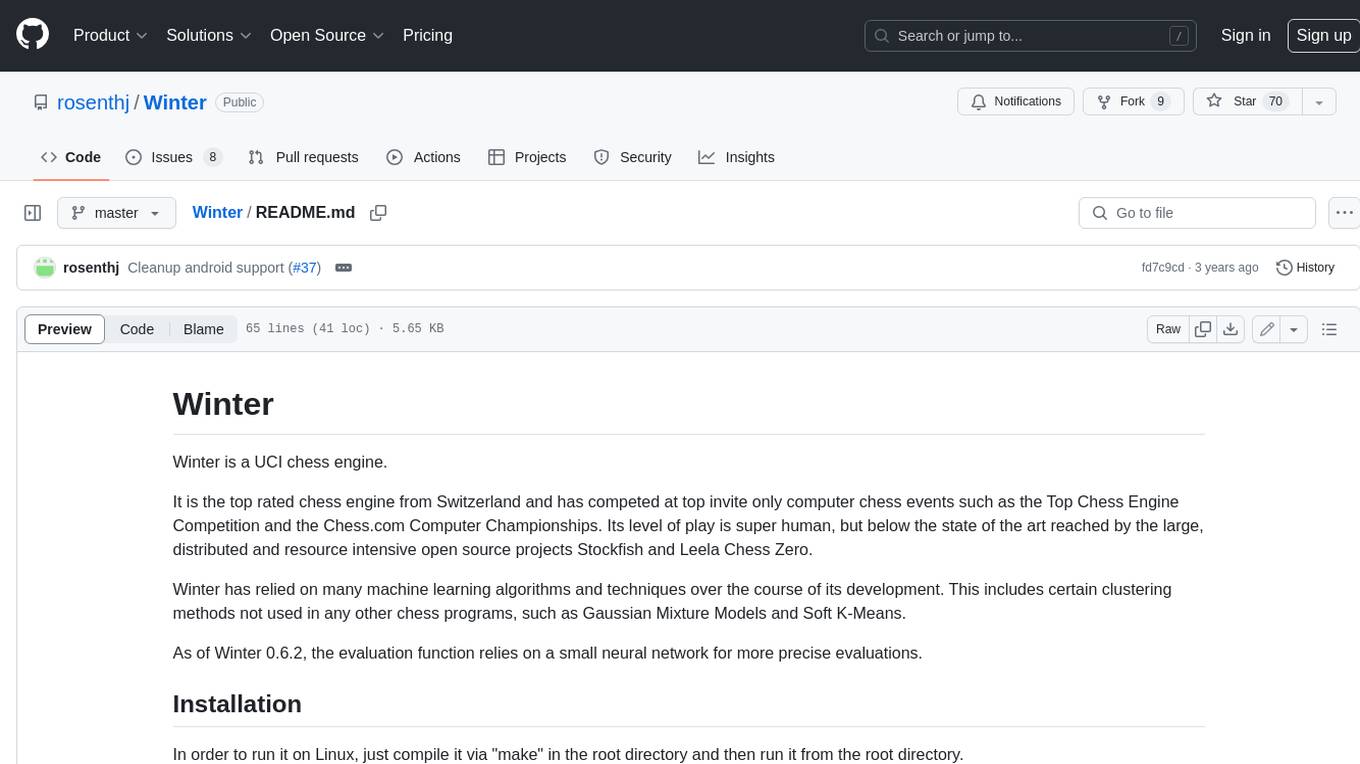
Winter
Winter is a UCI chess engine that has competed at top invite-only computer chess events. It is the top-rated chess engine from Switzerland and has a level of play that is super human but below the state of the art reached by large, distributed, and resource-intensive open-source projects like Stockfish and Leela Chess Zero. Winter has relied on many machine learning algorithms and techniques over the course of its development, including certain clustering methods not used in any other chess programs, such as Gaussian Mixture Models and Soft K-Means. As of Winter 0.6.2, the evaluation function relies on a small neural network for more precise evaluations.
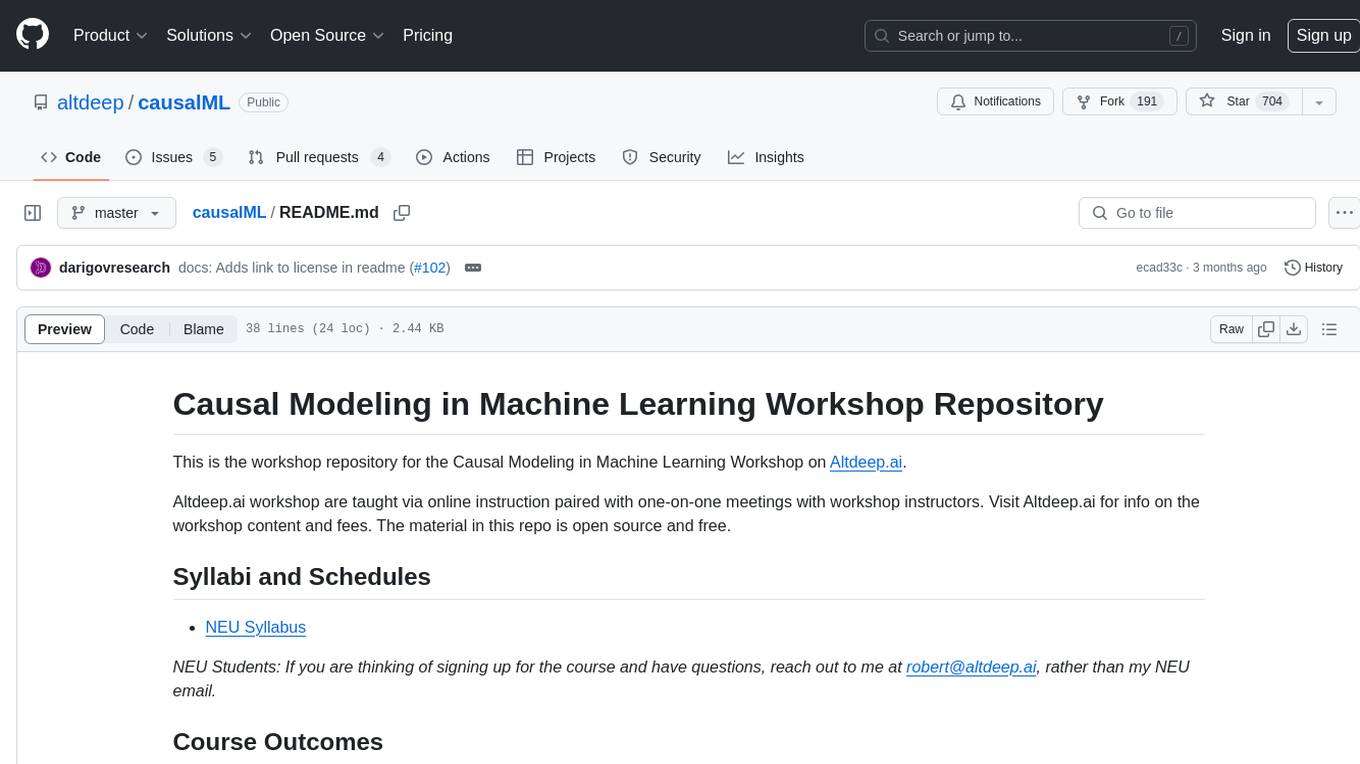
causalML
This repository is the workshop repository for the Causal Modeling in Machine Learning Workshop on Altdeep.ai. The material is open source and free. The course covers causality in model-based machine learning, Bayesian modeling, interventions, counterfactual reasoning, and deep causal latent variable models. It aims to equip learners with the ability to build causal reasoning algorithms into decision-making systems in data science and machine learning teams within top-tier technology organizations.
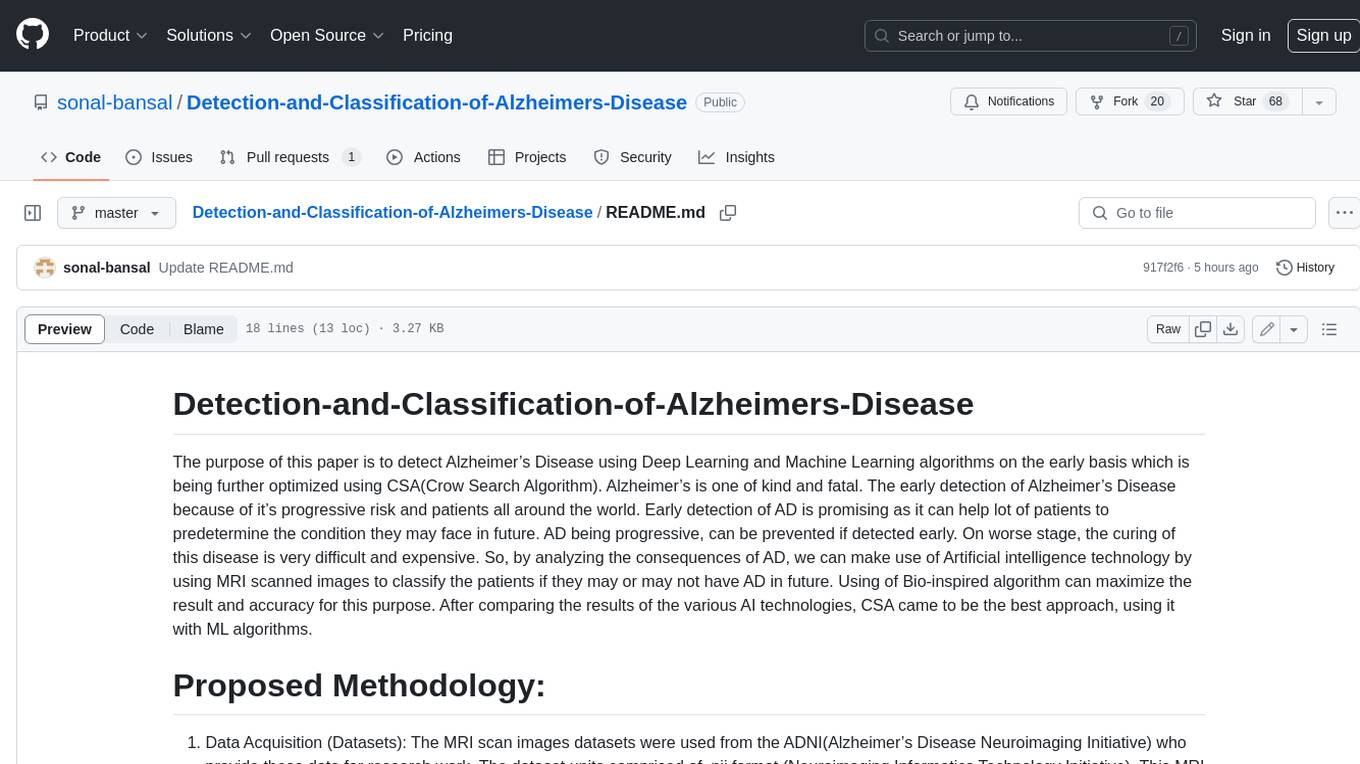
Detection-and-Classification-of-Alzheimers-Disease
This tool is designed to detect and classify Alzheimer's Disease using Deep Learning and Machine Learning algorithms on an early basis, which is further optimized using the Crow Search Algorithm (CSA). Alzheimer's is a fatal disease, and early detection is crucial for patients to predetermine their condition and prevent its progression. By analyzing MRI scanned images using Artificial Intelligence technology, this tool can classify patients who may or may not develop AD in the future. The CSA algorithm, combined with ML algorithms, has proven to be the most effective approach for this purpose.
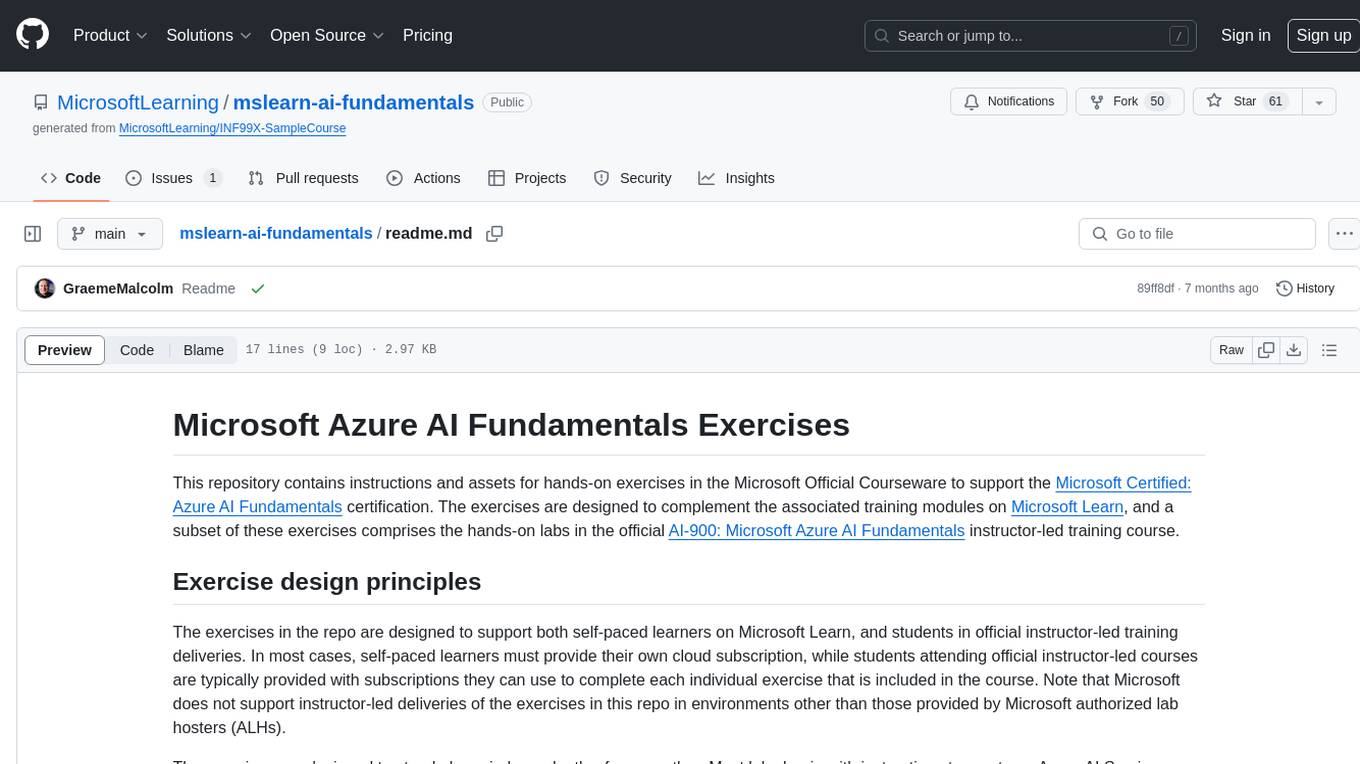
mslearn-ai-fundamentals
This repository contains materials for the Microsoft Learn AI Fundamentals module. It covers the basics of artificial intelligence, machine learning, and data science. The content includes hands-on labs, interactive learning modules, and assessments to help learners understand key concepts and techniques in AI. Whether you are new to AI or looking to expand your knowledge, this module provides a comprehensive introduction to the fundamentals of AI.
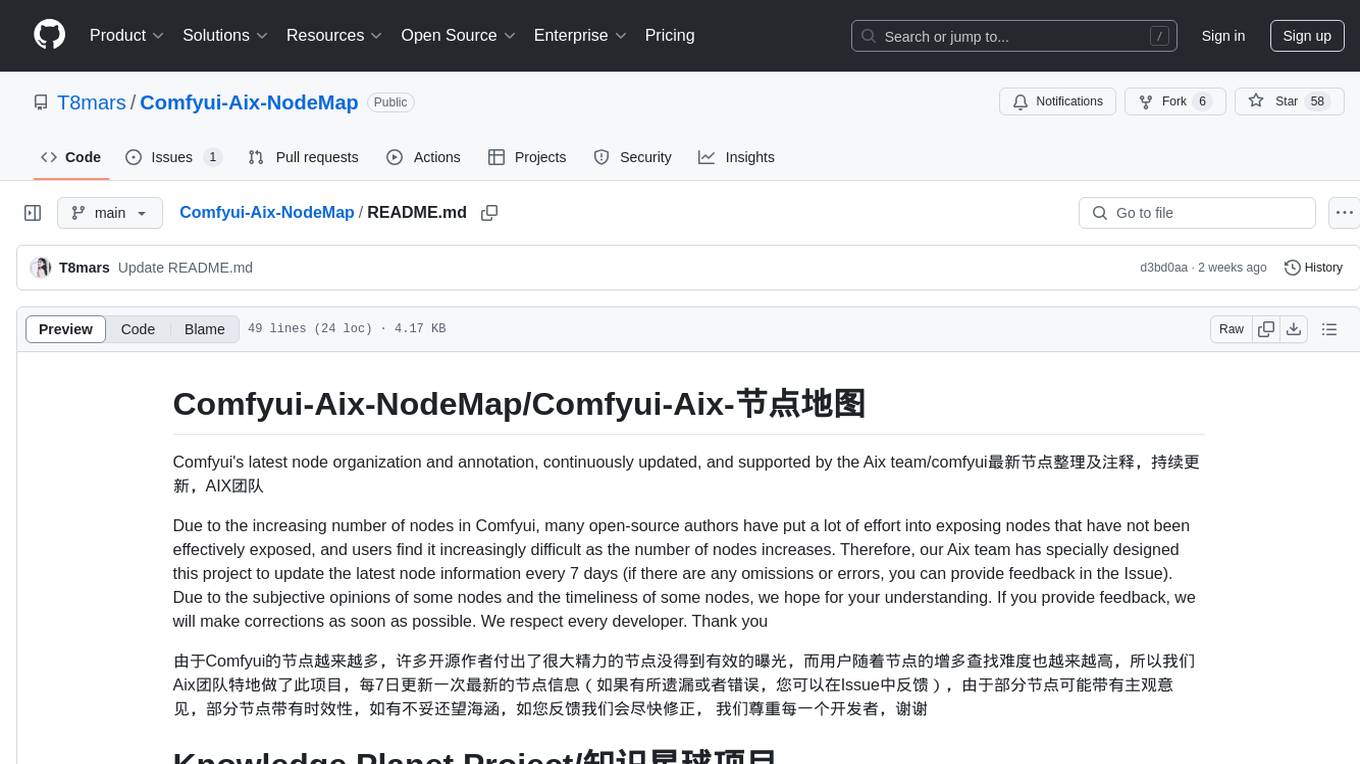
Comfyui-Aix-NodeMap
Comfyui-Aix-NodeMap is a project by the Aix team to organize and annotate the latest nodes in Comfyui. It aims to address the challenge of finding nodes effectively due to the increasing number of nodes. The project is updated every 7 days to provide the most recent node information. Users can provide feedback for any omissions or errors, and corrections will be made promptly. The project respects every developer and values community collaboration in improving node exposure and accessibility.
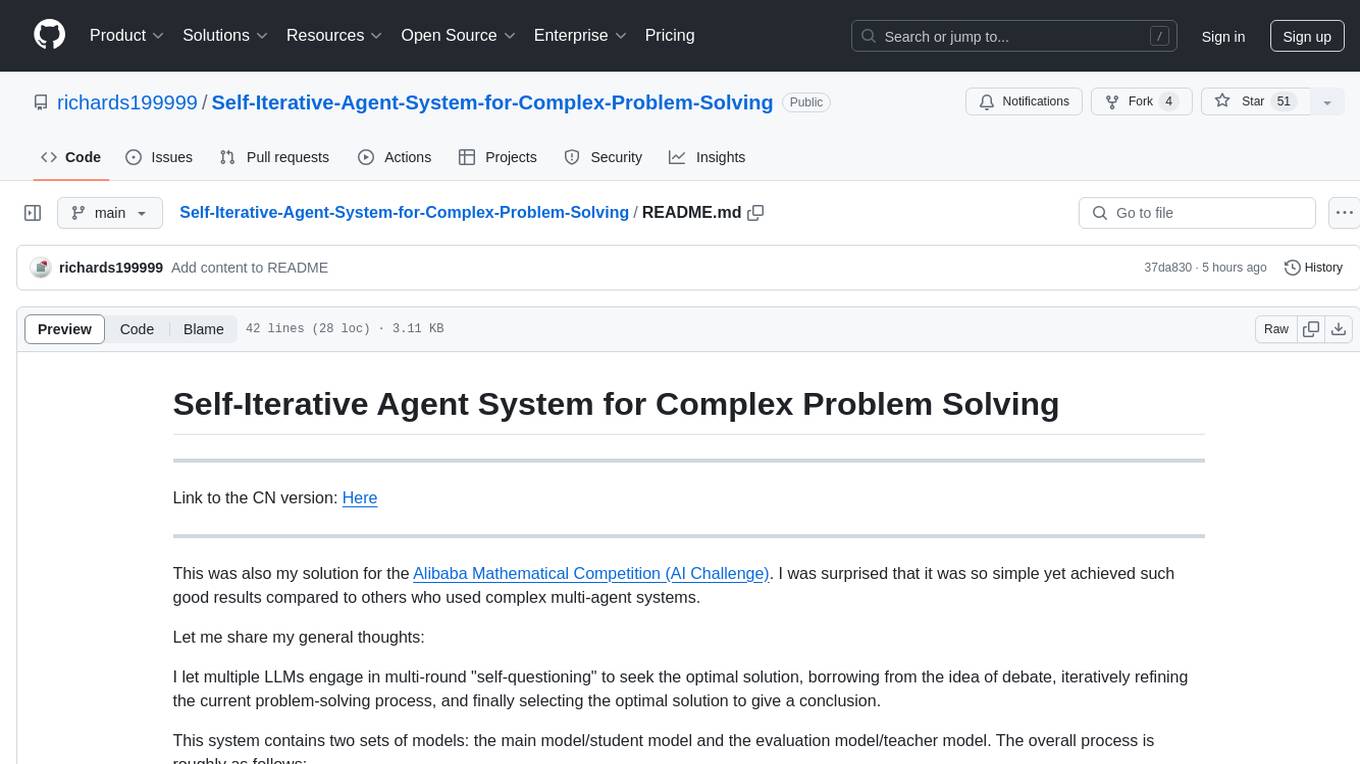
Self-Iterative-Agent-System-for-Complex-Problem-Solving
The Self-Iterative Agent System for Complex Problem Solving is a solution developed for the Alibaba Mathematical Competition (AI Challenge). It involves multiple LLMs engaging in multi-round 'self-questioning' to iteratively refine the problem-solving process and select optimal solutions. The system consists of main and evaluation models, with a process that includes detailed problem-solving steps, feedback loops, and iterative improvements. The approach emphasizes communication and reasoning between sub-agents, knowledge extraction, and the importance of Agent-like architectures in complex tasks. While effective, there is room for improvement in model capabilities and error prevention mechanisms.
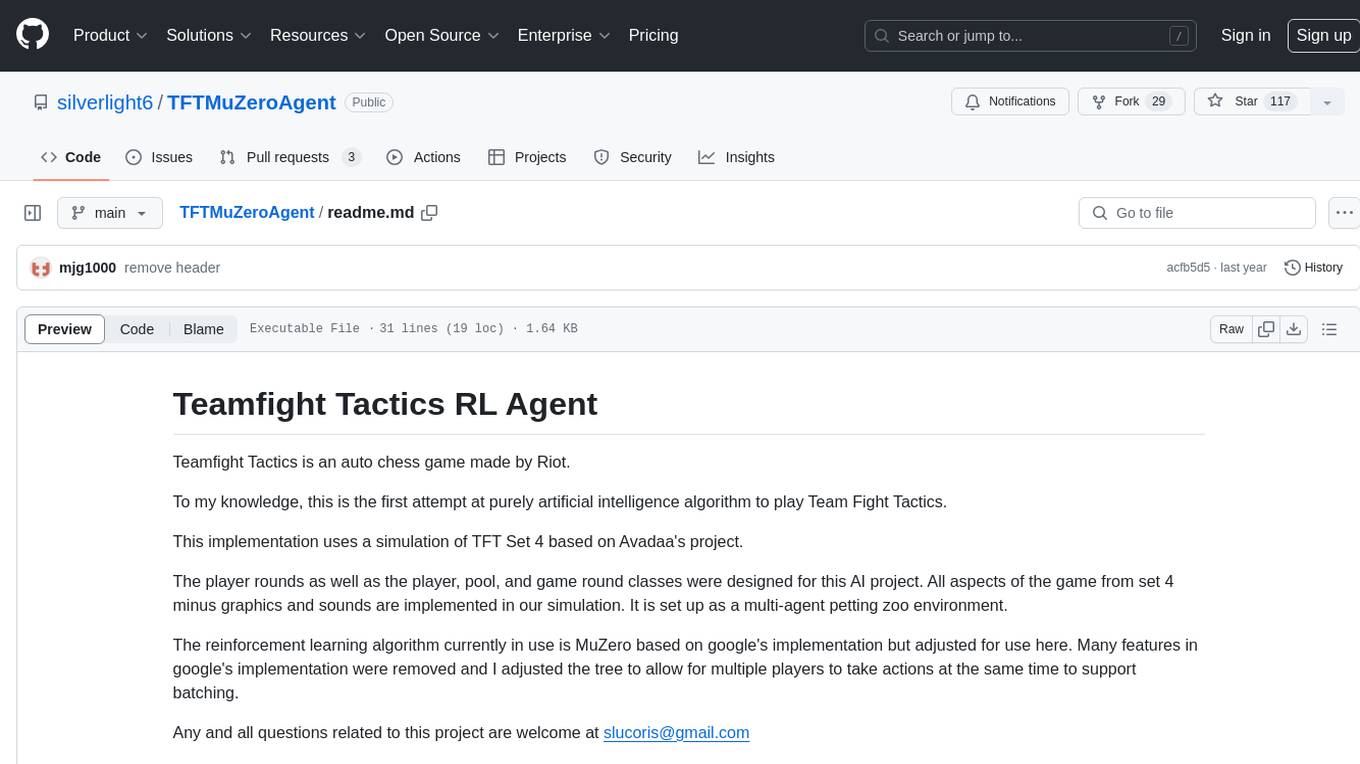
TFTMuZeroAgent
TFTMuZeroAgent is an implementation of a purely artificial intelligence algorithm to play Teamfight Tactics, an auto chess game made by Riot. It uses a simulation of TFT Set 4 and the MuZero reinforcement learning algorithm. The project provides a multi-agent petting zoo environment where players, pool, and game round classes are designed for AI project. The implementation excludes graphics and sounds but covers all aspects of the game from set 4. The codebase is open for contributions and improvements, allowing for additional models to be added to the environment.
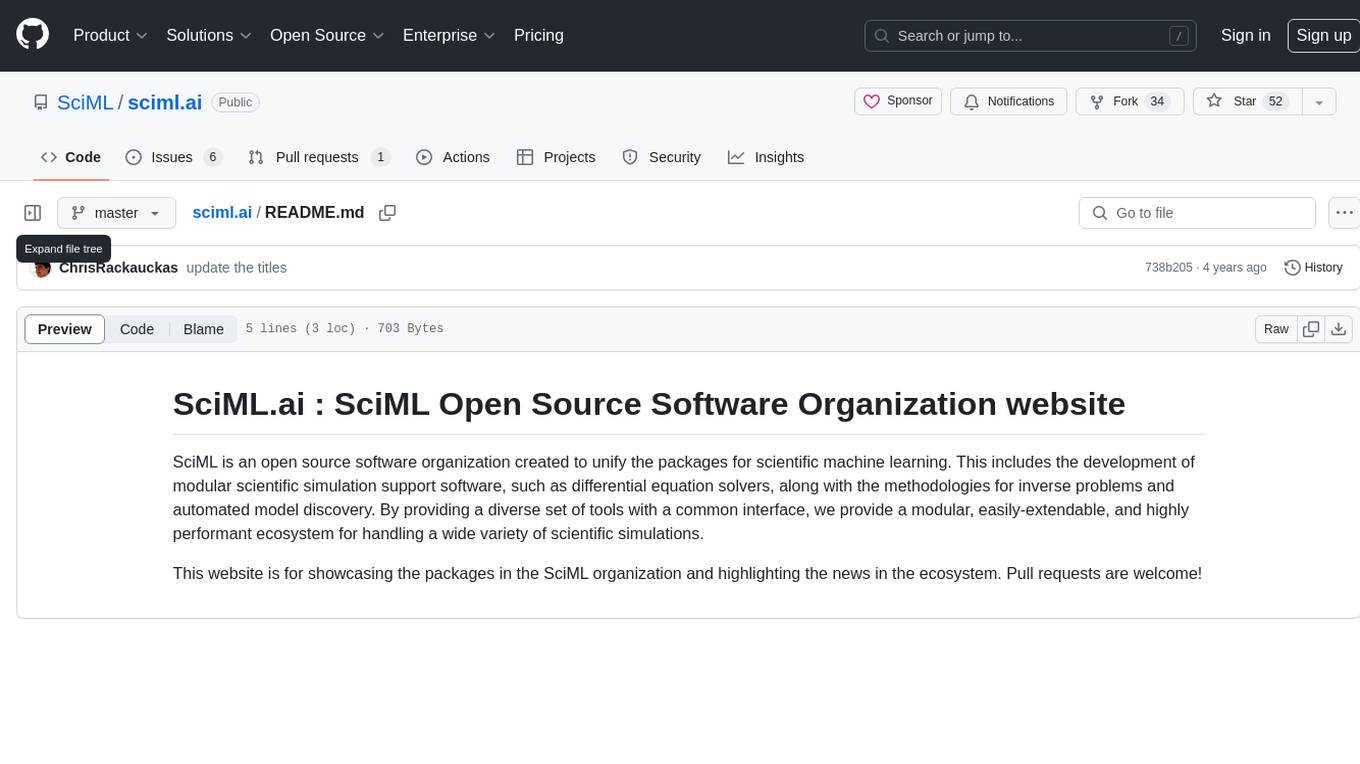
sciml.ai
SciML.ai is an open source software organization dedicated to unifying packages for scientific machine learning. It focuses on developing modular scientific simulation support software, including differential equation solvers, inverse problems methodologies, and automated model discovery. The organization aims to provide a diverse set of tools with a common interface, creating a modular, easily-extendable, and highly performant ecosystem for scientific simulations. The website serves as a platform to showcase SciML organization's packages and share news within the ecosystem. Pull requests are encouraged for contributions.
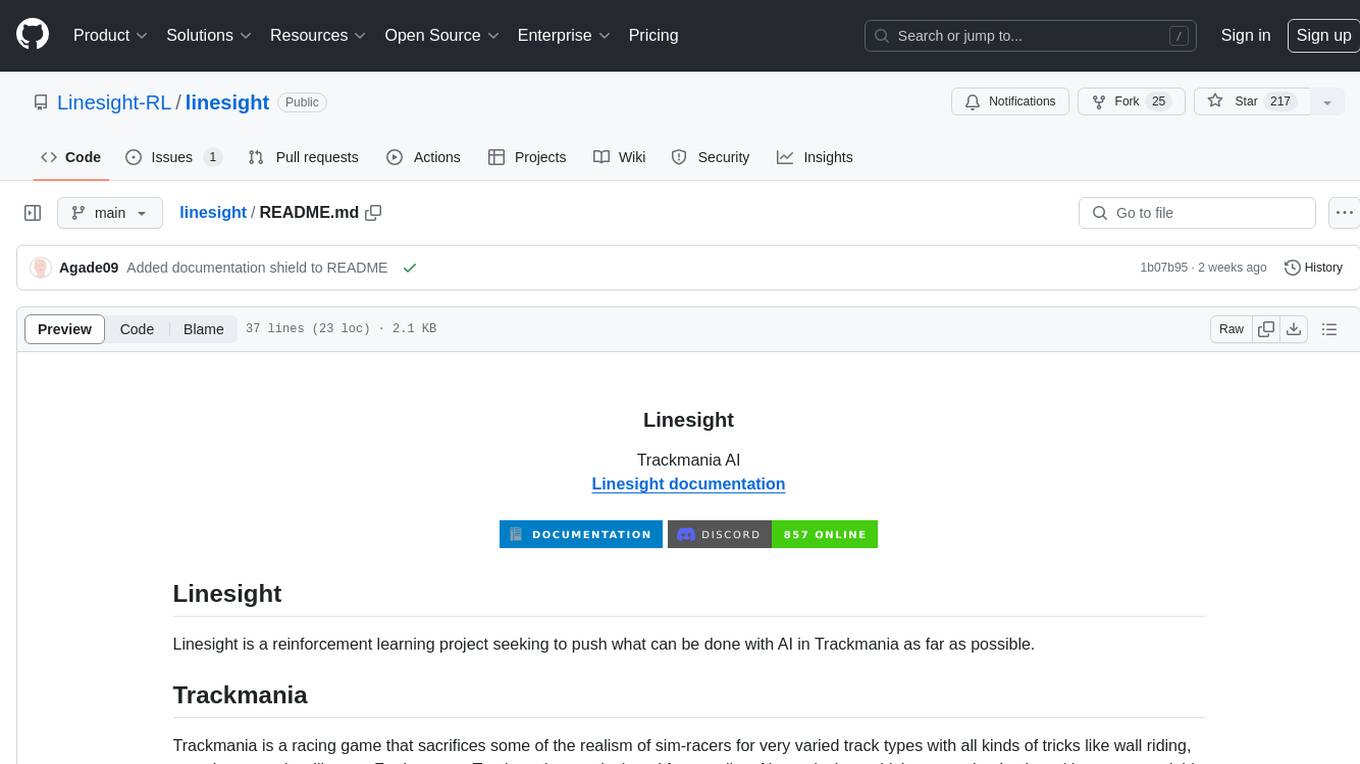
linesight
Linesight is a reinforcement learning project focused on advancing AI capabilities in the racing game Trackmania. It aims to push the boundaries of AI performance by utilizing deep learning algorithms to achieve human-level driving and beat world records on official campaign tracks. The project provides an interface to interact with Trackmania Nations Forever programmatically, enabling tasks such as sending inputs, retrieving car states, and capturing screenshots. With a strong emphasis on equality of input devices, Linesight serves as a benchmark for testing various reinforcement learning algorithms in a challenging and dynamic gaming environment.
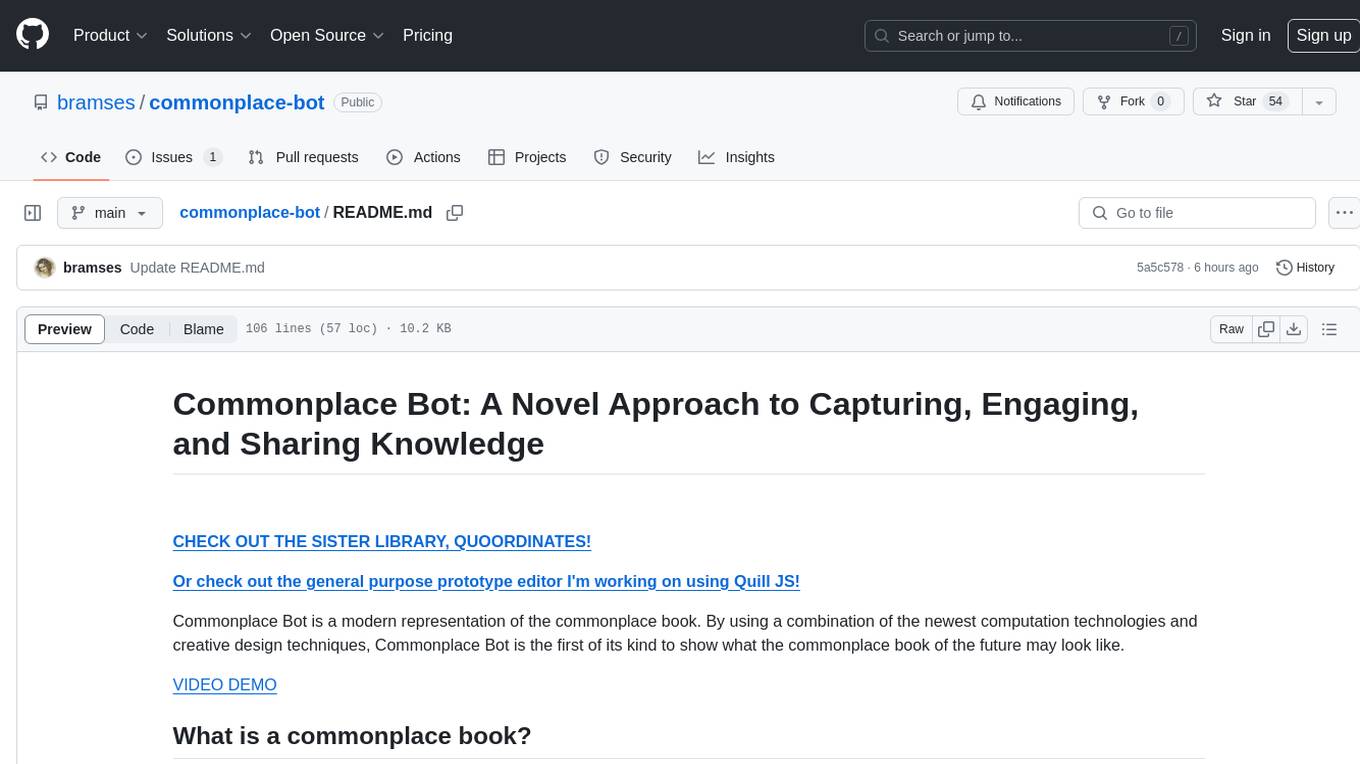
commonplace-bot
Commonplace Bot is a modern representation of the commonplace book, leveraging modern technological advancements in computation, data storage, machine learning, and networking. It aims to capture, engage, and share knowledge by providing a platform for users to collect ideas, quotes, and information, organize them efficiently, engage with the data through various strategies and triggers, and transform the data into new mediums for sharing. The tool utilizes embeddings and cached transformations for efficient data storage and retrieval, flips traditional engagement rules by engaging with the user, and enables users to alchemize raw data into new forms like art prompts. Commonplace Bot offers a unique approach to knowledge management and creative expression.
For similar tasks
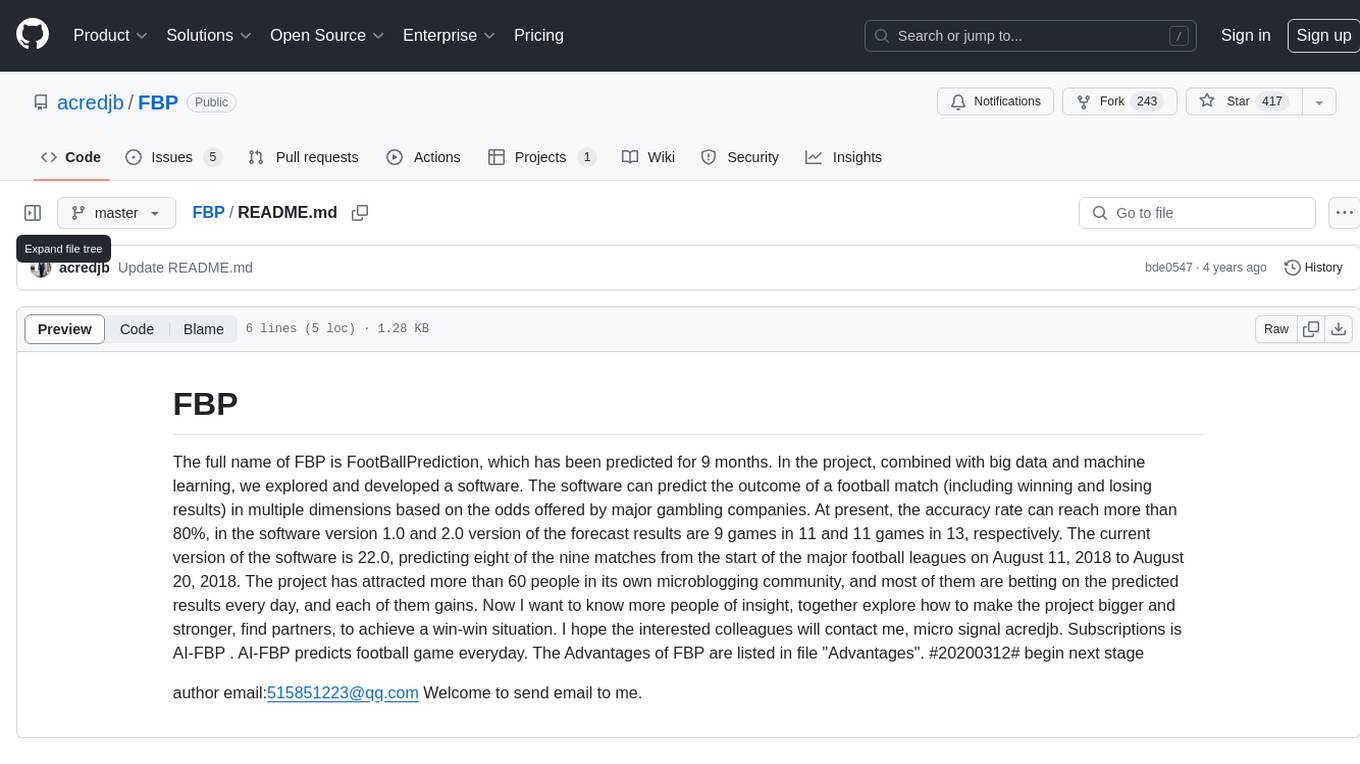
FBP
FootBallPrediction (FBP) is a software project that utilizes big data and machine learning to predict the outcome of football matches based on odds from gambling companies. The software has achieved an accuracy rate of over 80% in predicting match results. The current version, 22.0, successfully predicted eight out of nine matches from major football leagues. The project has a community of over 60 members who benefit from the predicted results. The author is seeking collaboration to further enhance the project and welcomes interested individuals to join. AI-FBP is a subscription service that provides daily football game predictions.
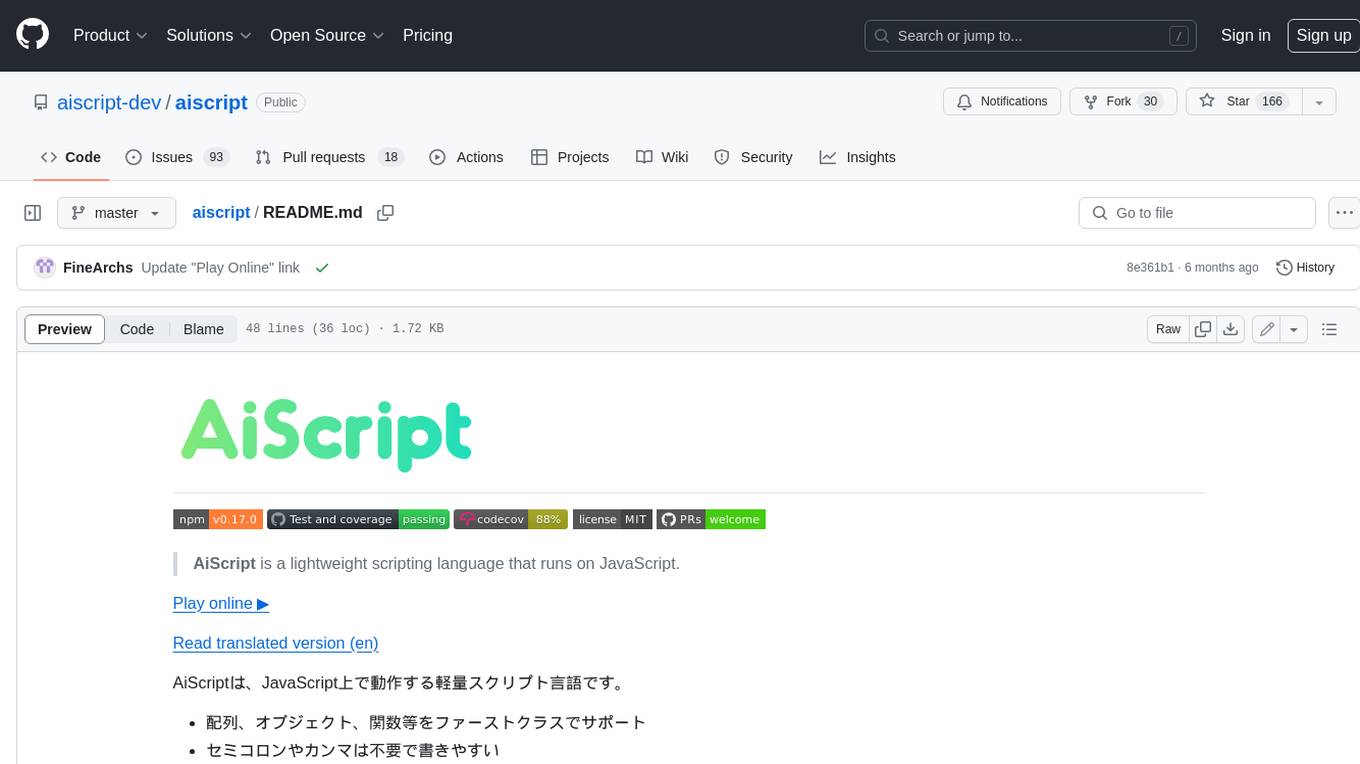
aiscript
AiScript is a lightweight scripting language that runs on JavaScript. It supports arrays, objects, and functions as first-class citizens, and is easy to write without the need for semicolons or commas. AiScript runs in a secure sandbox environment, preventing infinite loops from freezing the host. It also allows for easy provision of variables and functions from the host.
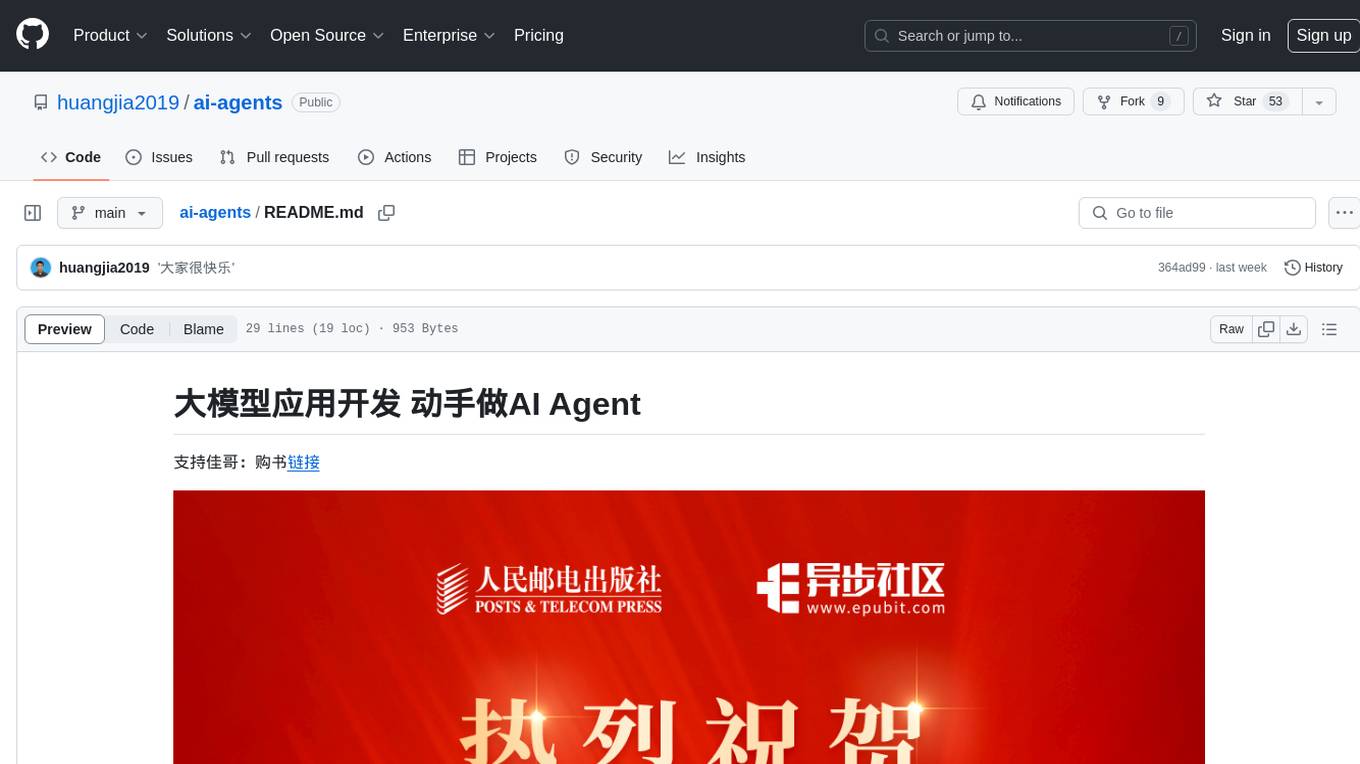
ai-agents
The 'ai-agents' repository is a collection of books and resources focused on developing AI agents, including topics such as GPT models, building AI agents from scratch, machine learning theory and practice, and basic methods and tools for data analysis. The repository provides detailed explanations and guidance for individuals interested in learning about and working with AI agents.
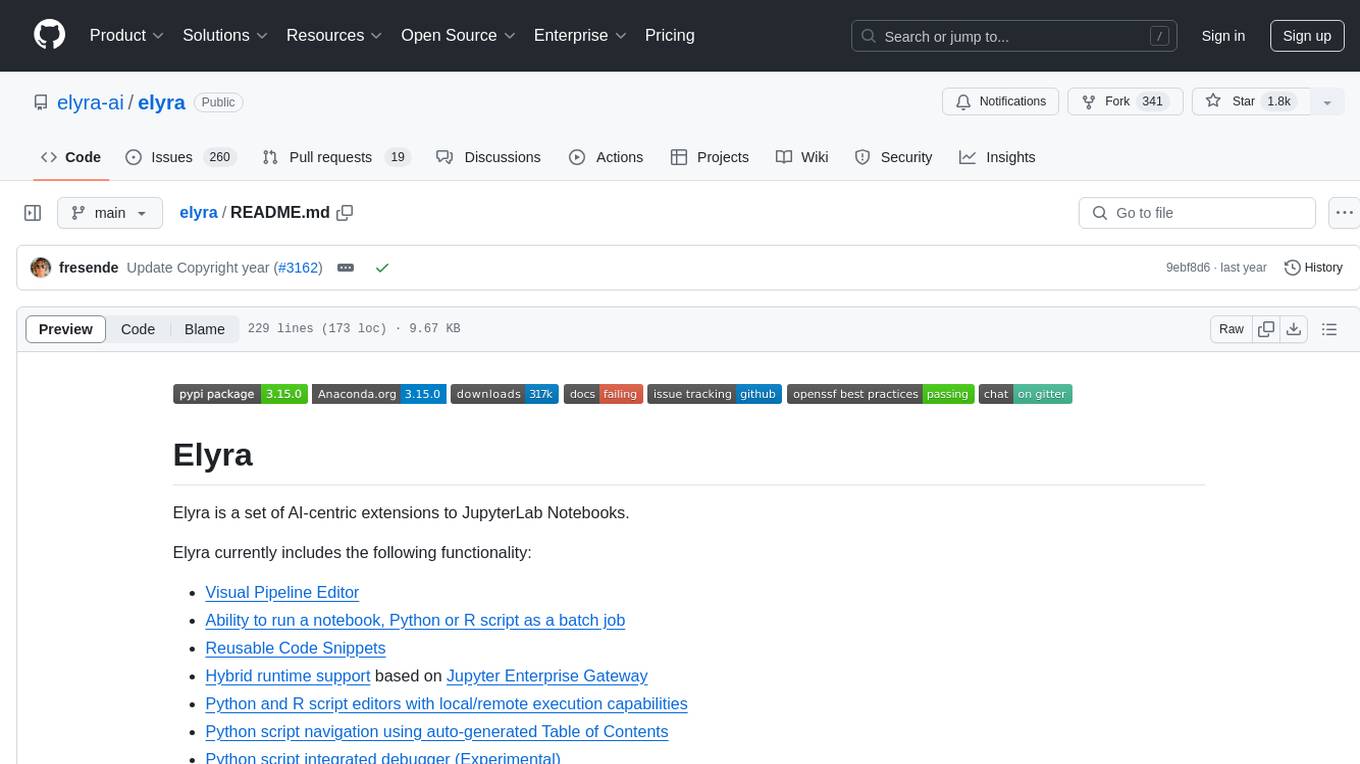
elyra
Elyra is a set of AI-centric extensions to JupyterLab Notebooks that includes features like Visual Pipeline Editor, running notebooks/scripts as batch jobs, reusable code snippets, hybrid runtime support, script editors with execution capabilities, debugger, version control using Git, and more. It provides a comprehensive environment for data scientists and AI practitioners to develop, test, and deploy machine learning models and workflows efficiently.
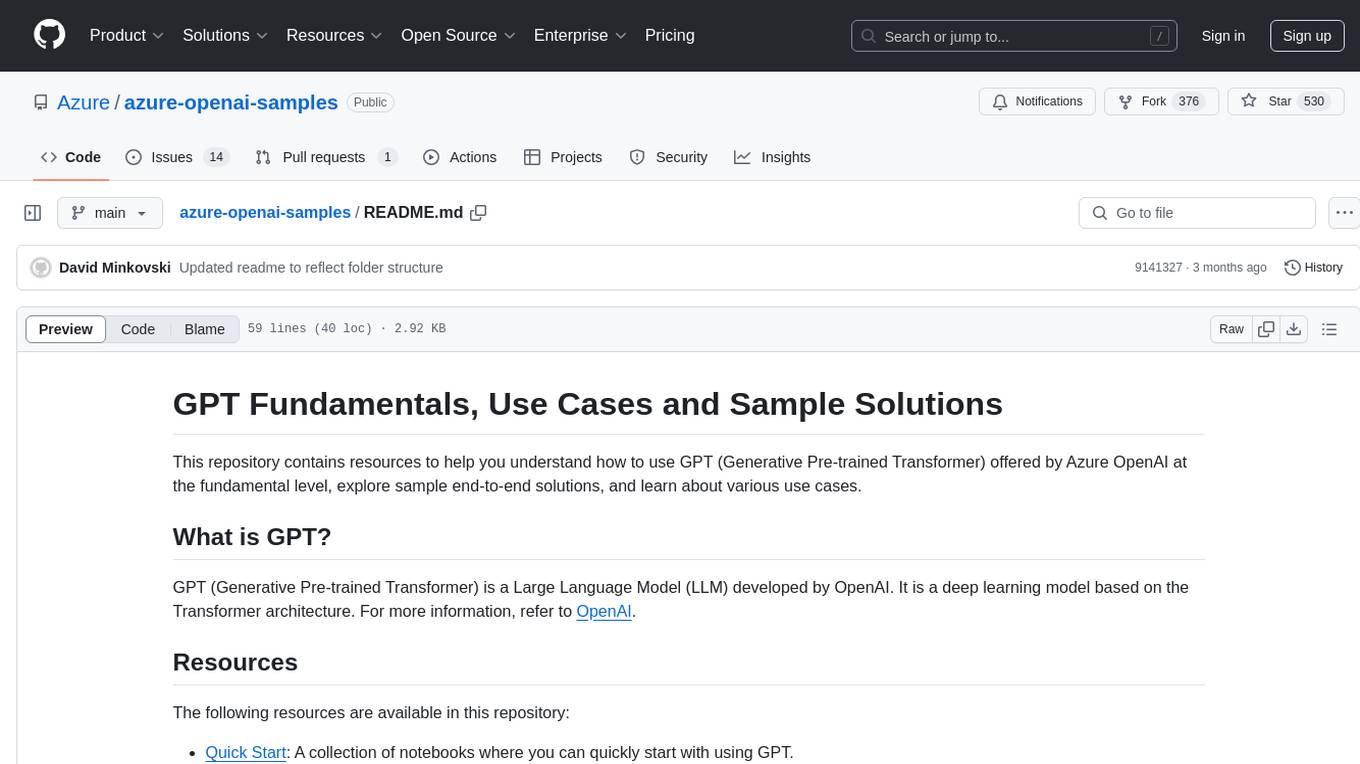
azure-openai-samples
This repository provides resources to understand and utilize GPT (Generative Pre-trained Transformer) by Azure OpenAI. It includes sample solutions, use cases, and quick start guides. Users can explore various applications of GPT, such as chatbots, customer service, and content generation. The repository also offers Langchain, Semantic Kernel, and Prompt Flow samples, along with Serverless SQL GPT for natural language processing in Azure Synapse Analytics. The samples are based on GPT 3.5, with plans to update for GPT-4. Users are encouraged to contribute to keep the repository updated with the latest technologies and solutions.
For similar jobs
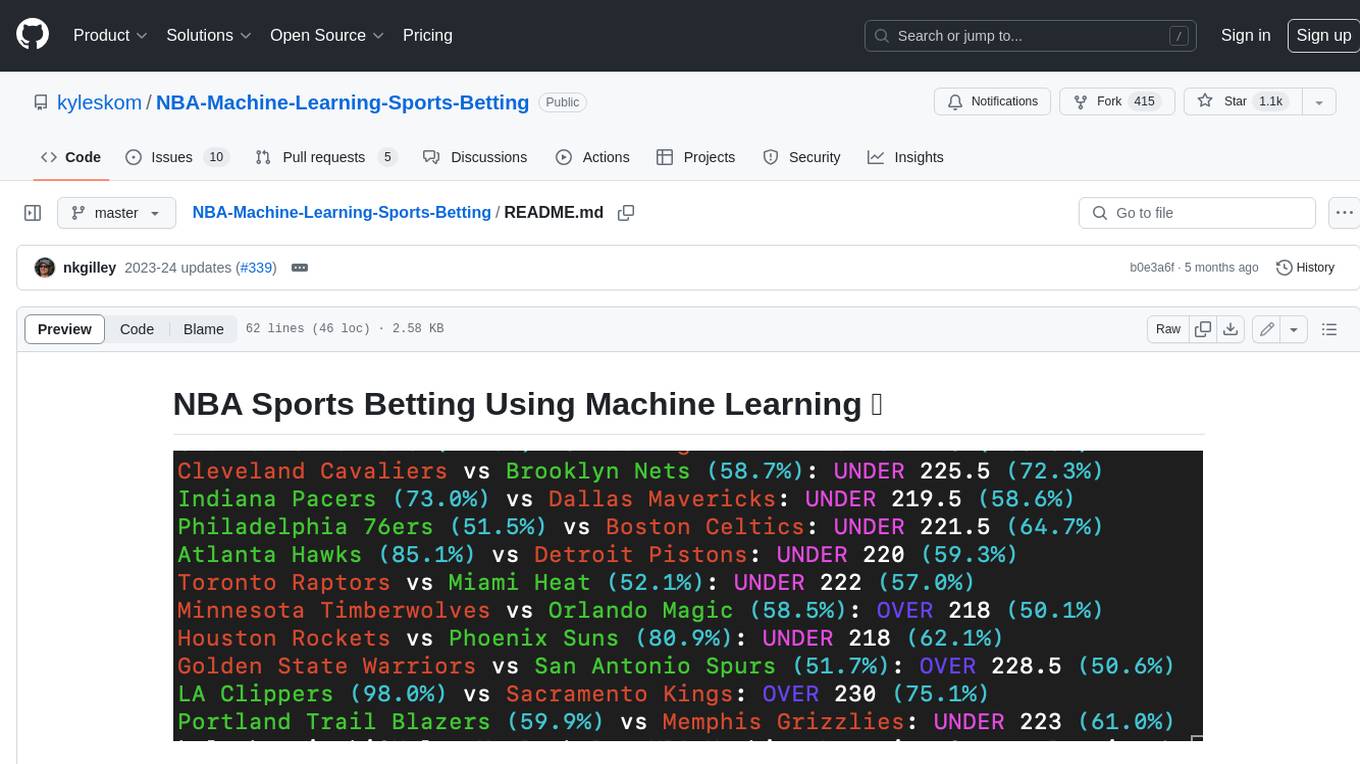
NBA-Machine-Learning-Sports-Betting
This tool is a machine learning AI used to predict the winners and under/overs of NBA games. It takes all team data from the 2007-08 season to the current season, matched with odds of those games, and uses a neural network to predict winning bets for today's games. The tool achieves ~69% accuracy on money lines and ~55% on under/overs. It outputs expected value for teams' money lines to provide better insight and the fraction of your bankroll to bet based on the Kelly Criterion. A popular, less risky approach is to bet 50% of the stake recommended by the Kelly Criterion.
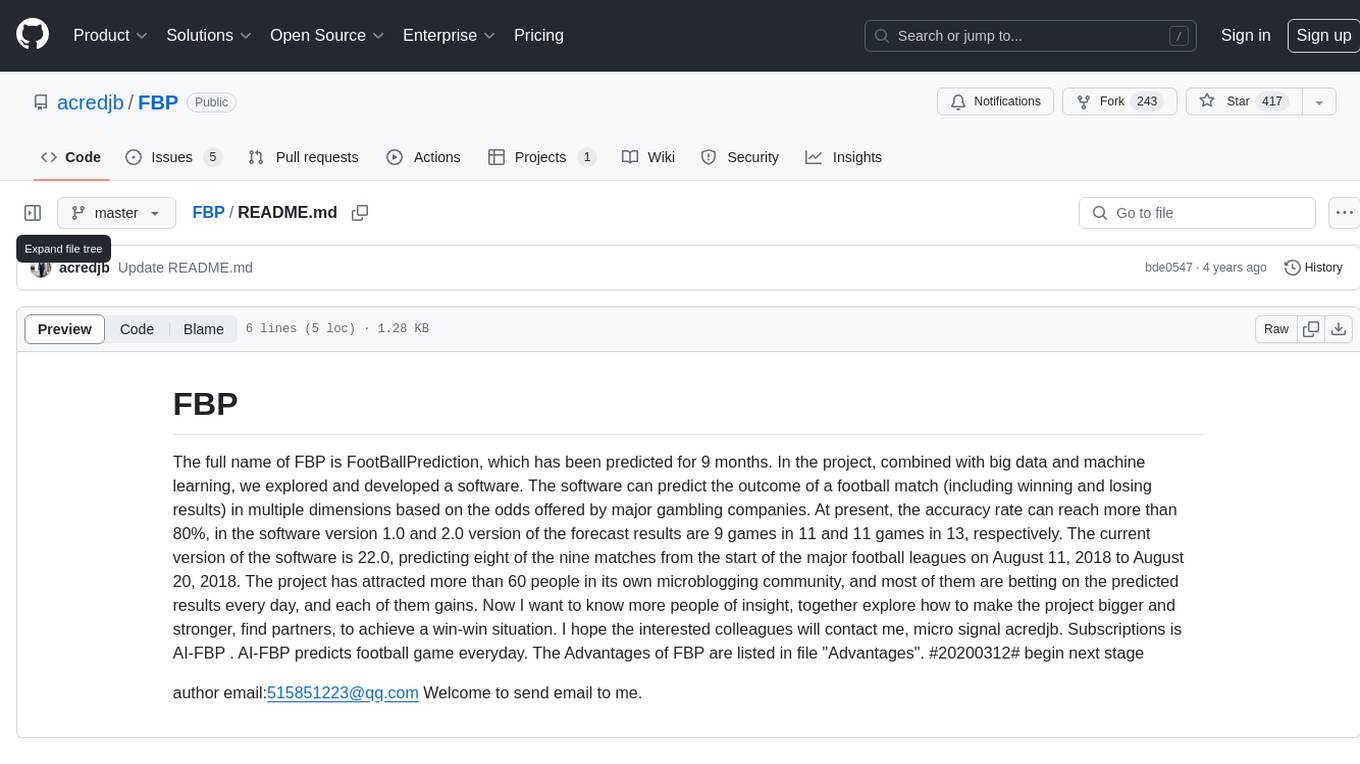
FBP
FootBallPrediction (FBP) is a software project that utilizes big data and machine learning to predict the outcome of football matches based on odds from gambling companies. The software has achieved an accuracy rate of over 80% in predicting match results. The current version, 22.0, successfully predicted eight out of nine matches from major football leagues. The project has a community of over 60 members who benefit from the predicted results. The author is seeking collaboration to further enhance the project and welcomes interested individuals to join. AI-FBP is a subscription service that provides daily football game predictions.
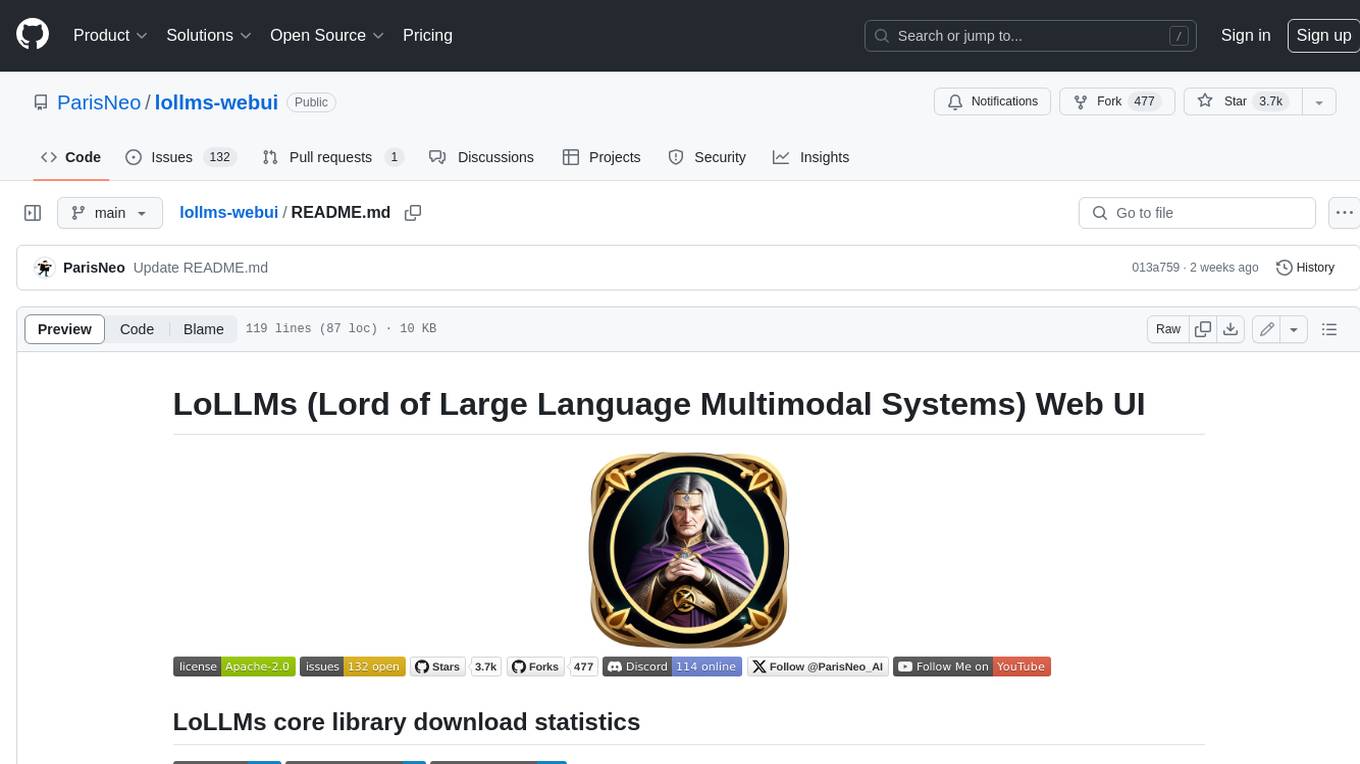
lollms-webui
LoLLMs WebUI (Lord of Large Language Multimodal Systems: One tool to rule them all) is a user-friendly interface to access and utilize various LLM (Large Language Models) and other AI models for a wide range of tasks. With over 500 AI expert conditionings across diverse domains and more than 2500 fine tuned models over multiple domains, LoLLMs WebUI provides an immediate resource for any problem, from car repair to coding assistance, legal matters, medical diagnosis, entertainment, and more. The easy-to-use UI with light and dark mode options, integration with GitHub repository, support for different personalities, and features like thumb up/down rating, copy, edit, and remove messages, local database storage, search, export, and delete multiple discussions, make LoLLMs WebUI a powerful and versatile tool.
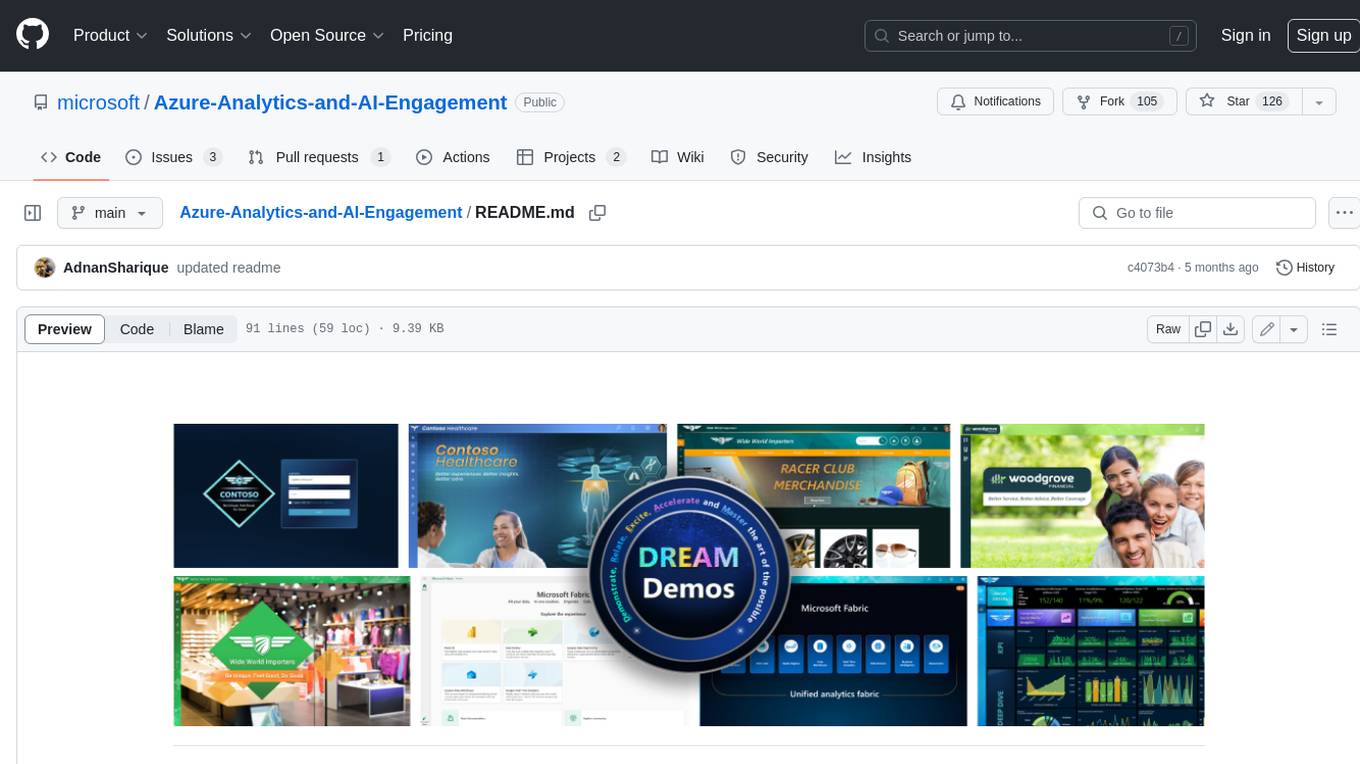
Azure-Analytics-and-AI-Engagement
The Azure-Analytics-and-AI-Engagement repository provides packaged Industry Scenario DREAM Demos with ARM templates (Containing a demo web application, Power BI reports, Synapse resources, AML Notebooks etc.) that can be deployed in a customer’s subscription using the CAPE tool within a matter of few hours. Partners can also deploy DREAM Demos in their own subscriptions using DPoC.
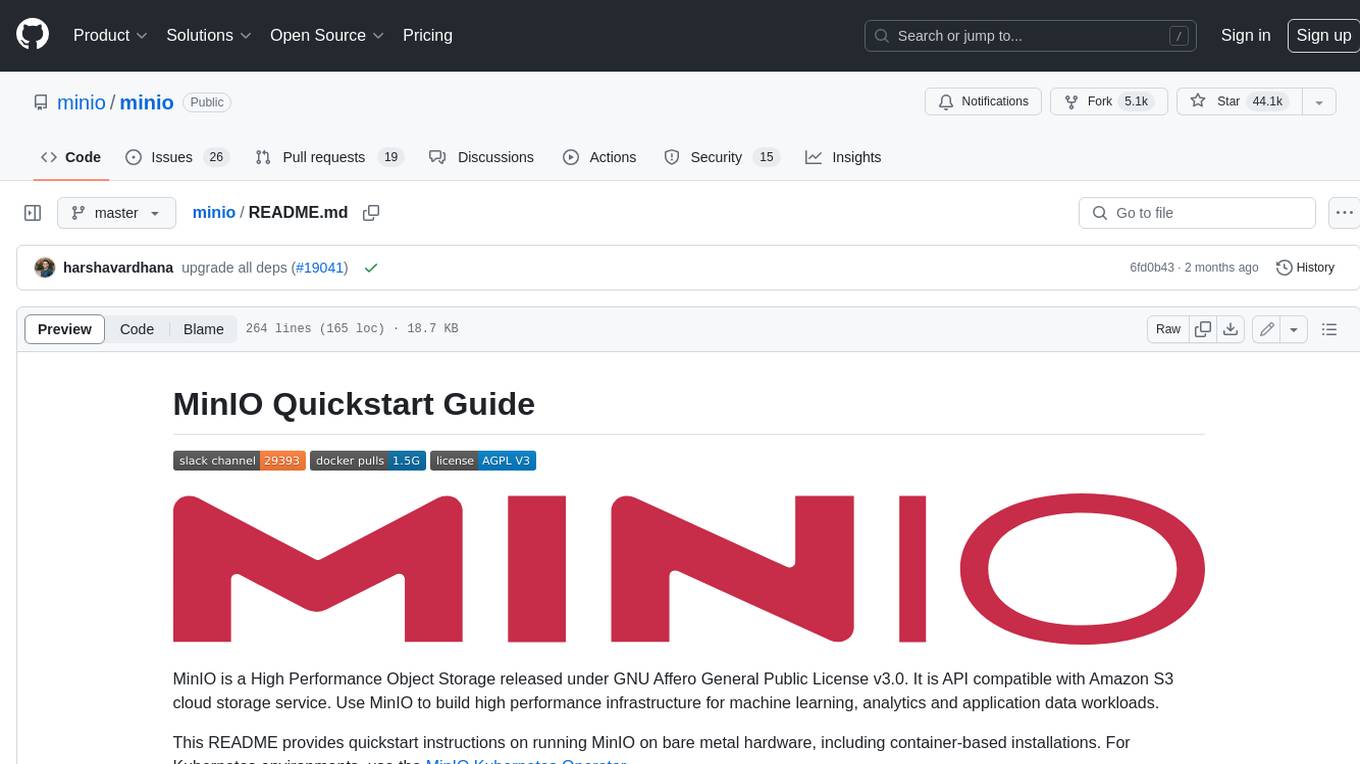
minio
MinIO is a High Performance Object Storage released under GNU Affero General Public License v3.0. It is API compatible with Amazon S3 cloud storage service. Use MinIO to build high performance infrastructure for machine learning, analytics and application data workloads.
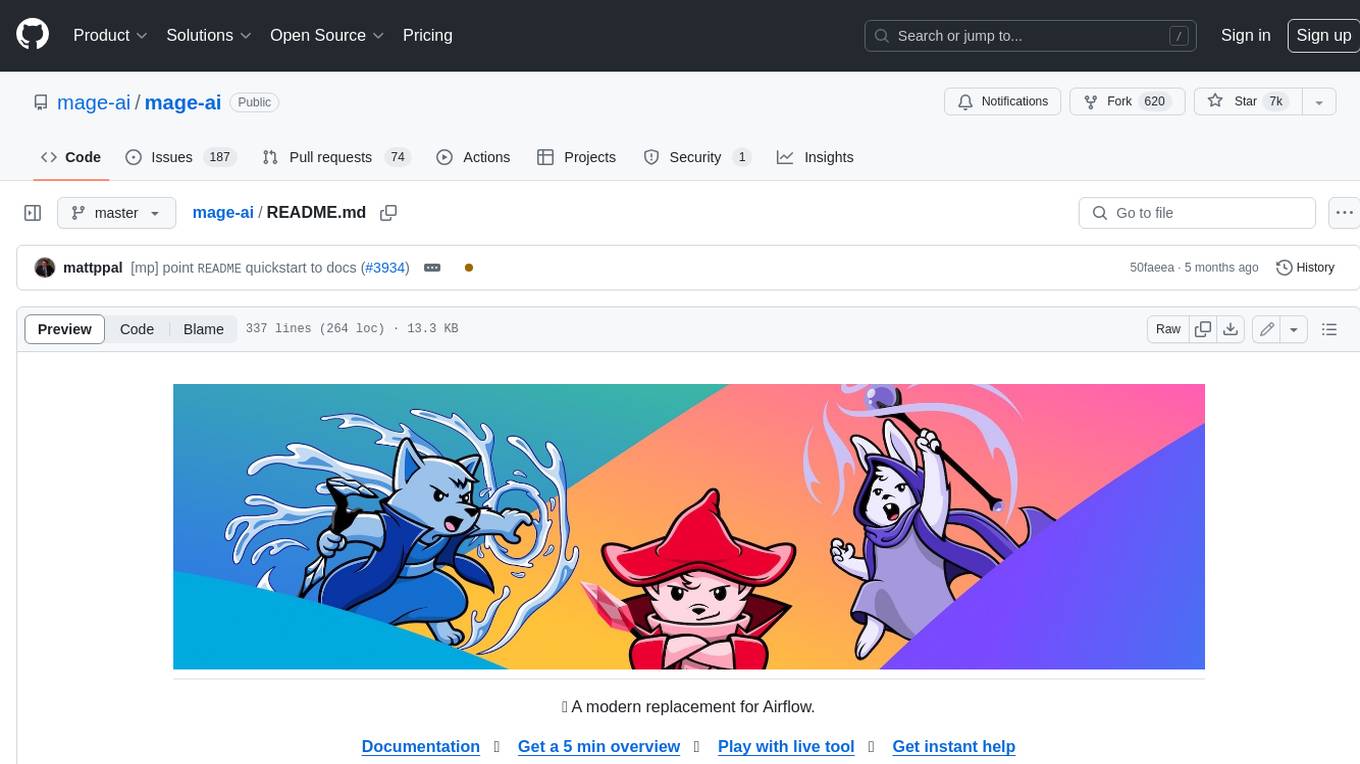
mage-ai
Mage is an open-source data pipeline tool for transforming and integrating data. It offers an easy developer experience, engineering best practices built-in, and data as a first-class citizen. Mage makes it easy to build, preview, and launch data pipelines, and provides observability and scaling capabilities. It supports data integrations, streaming pipelines, and dbt integration.
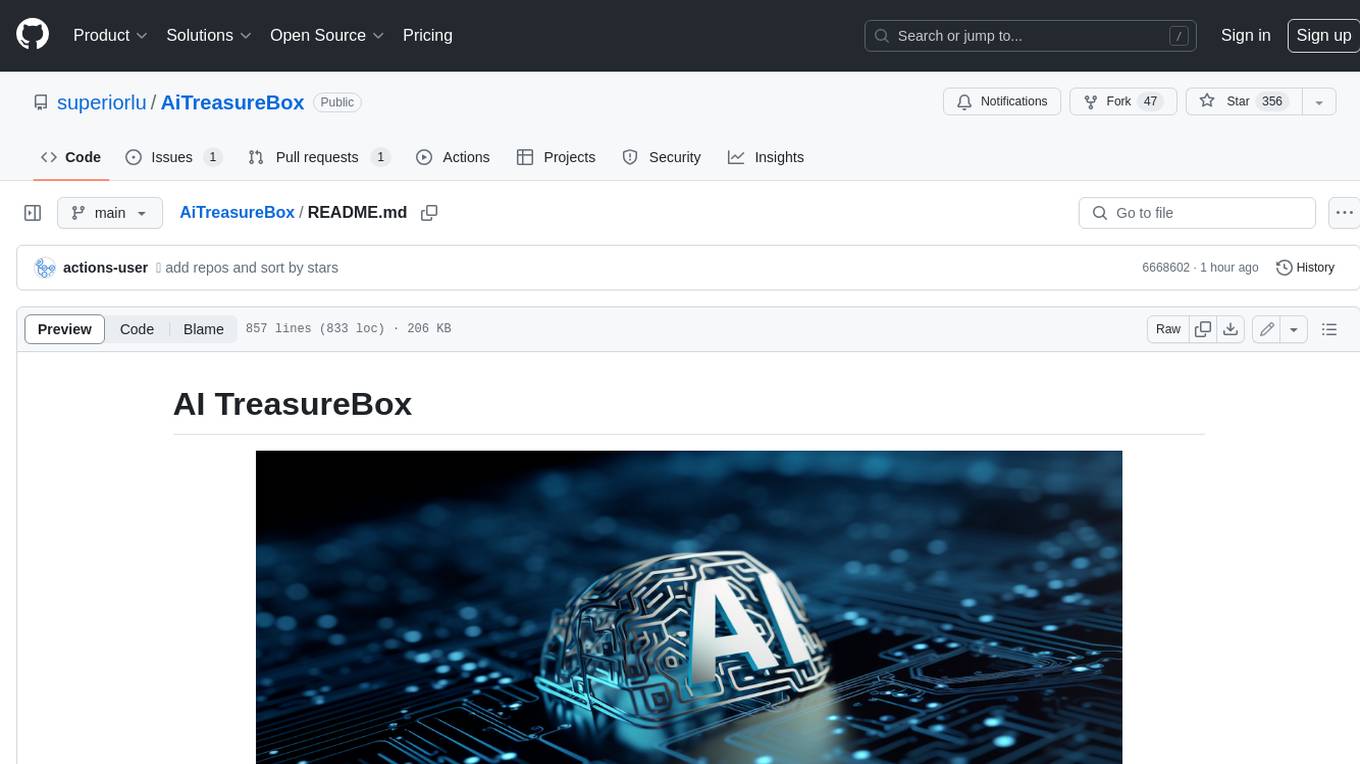
AiTreasureBox
AiTreasureBox is a versatile AI tool that provides a collection of pre-trained models and algorithms for various machine learning tasks. It simplifies the process of implementing AI solutions by offering ready-to-use components that can be easily integrated into projects. With AiTreasureBox, users can quickly prototype and deploy AI applications without the need for extensive knowledge in machine learning or deep learning. The tool covers a wide range of tasks such as image classification, text generation, sentiment analysis, object detection, and more. It is designed to be user-friendly and accessible to both beginners and experienced developers, making AI development more efficient and accessible to a wider audience.
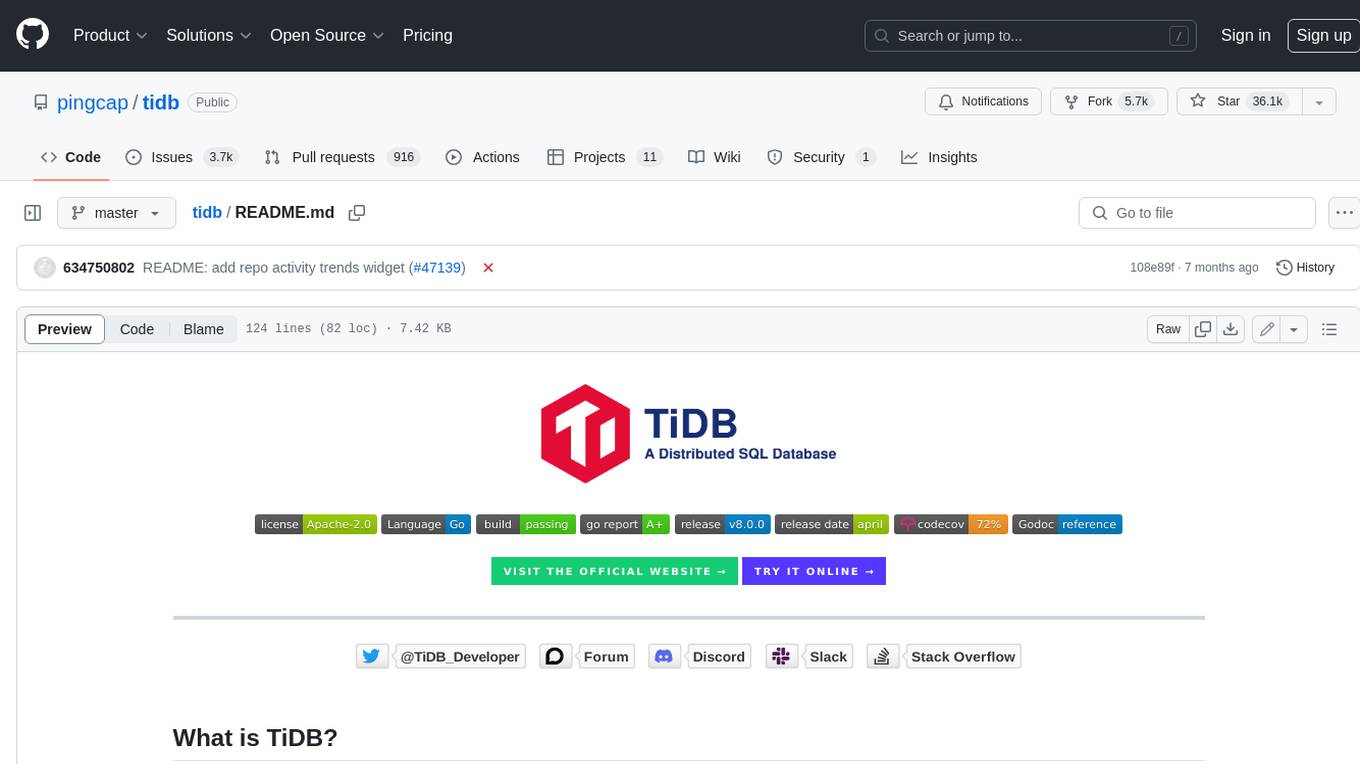
tidb
TiDB is an open-source distributed SQL database that supports Hybrid Transactional and Analytical Processing (HTAP) workloads. It is MySQL compatible and features horizontal scalability, strong consistency, and high availability.