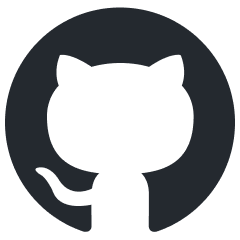
Winter
UCI Chess Engine
Stars: 80
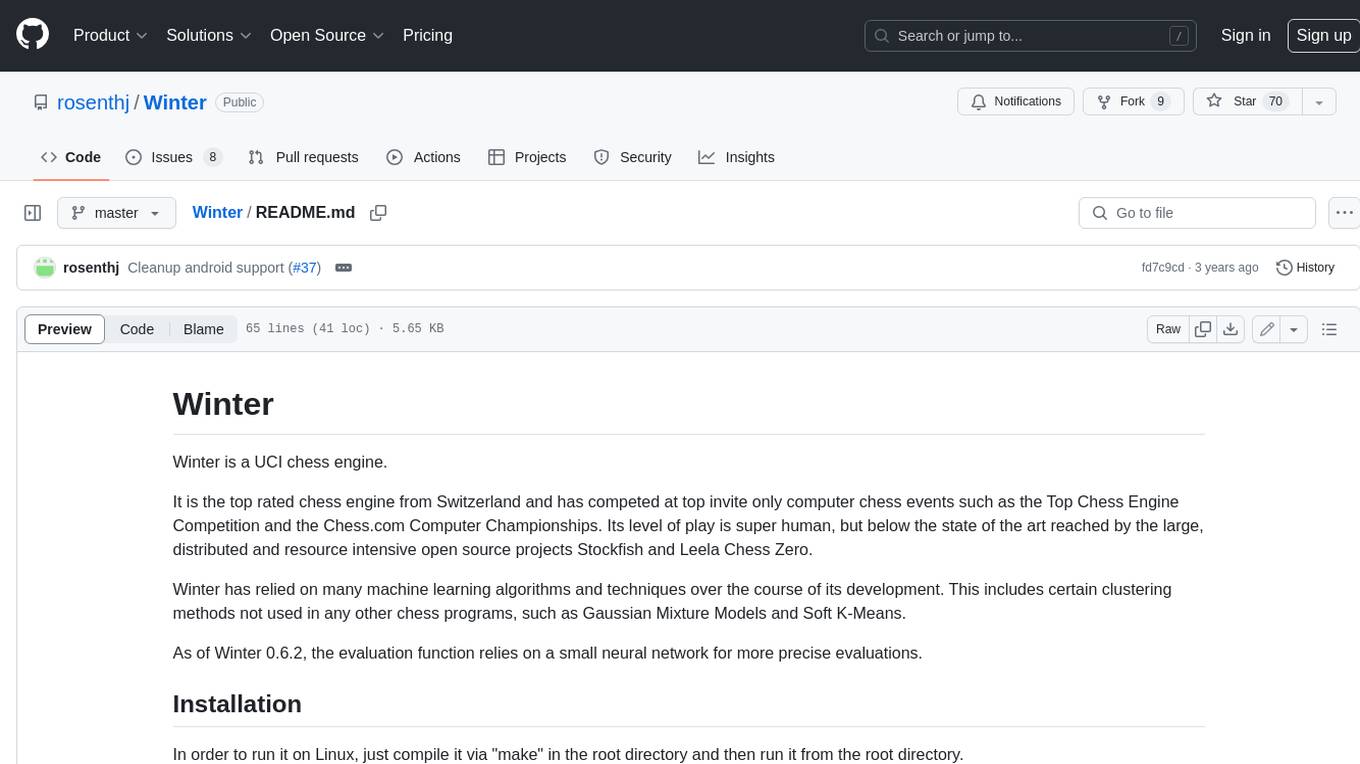
Winter is a UCI chess engine that has competed at top invite-only computer chess events. It is the top-rated chess engine from Switzerland and has a level of play that is super human but below the state of the art reached by large, distributed, and resource-intensive open-source projects like Stockfish and Leela Chess Zero. Winter has relied on many machine learning algorithms and techniques over the course of its development, including certain clustering methods not used in any other chess programs, such as Gaussian Mixture Models and Soft K-Means. As of Winter 0.6.2, the evaluation function relies on a small neural network for more precise evaluations.
README:
Winter is a UCI chess engine.
It is the top rated chess engine from Switzerland and has competed at top invite only computer chess events such as the Top Chess Engine Competition and the Chess.com Computer Championships. Its level of play is super human, but below the state of the art reached by the large, distributed and resource intensive open source projects Stockfish and Leela Chess Zero.
Winter has relied on many machine learning algorithms and techniques over the course of its development. This includes certain clustering methods not used in any other chess programs, such as Gaussian Mixture Models and Soft K-Means.
As of Winter 0.6.2, the evaluation function relies on a small neural network for more precise evaluations.
In order to run it on Linux, just compile it via "make" in the root directory and then run it from the root directory.
The makefile will assume you are making a native build, but if you are making a build for a different system, it should be reasonably straightforward to modify yourself.
Winter does not rely on any external libraries aside from the Standard Template Library. All algorithms have been implemented from scratch. As of Winter 0.6.2 I have started to build an external codebase for neural network training.
Compiling for android is only a bit more complicated than compiling for Linux. Follow the following instructions.
- Download and extract Android's NDK.
- Export your NDK path. For example
$ export PATH=$PATH:$HOME/android-ndk-r21d/toolchains/llvm/prebuilt/linux-x86_64/bin
- Switch to the Winter root directory
$ cd Winter
- Get the sse2neon header file.
$ wget -O src/learning/sse2neon.h https://raw.githubusercontent.com/DLTcollab/sse2neon/master/sse2neon.h
- Build!
$ make ARCH=aarch64
(64-bit) or$ make ARCH=armv7a
(32-bit)$ aarch64-linux-android-strip Winter
Winter versions 0.7 and later have support for contempt settings. In most engines contempt is used to reduce the number of draws and thus increase performance against weaker engines, often at the cost of performance in self play or against stronger opposition.
Winter uses a novel contempt implementation that utilizes the fact that Winter calculates win, draw and loss probabilities. Increasing contempt in Winter reduces how much it values draws for itself and increases how much it believes the opponent values draws.
As of version v0.8.1 disabled. If I don't remember to add this again before v0.9, please pester me with an issue on github!
Internally Winter actually tends to have a negative score for positive contempt and vice versa. This is natural as positive contempt is reducing the value of a draw for the side to move, so the score will be negatively biased.
In order to have more realistic score outputs, Winter does a bias adjustment for non-mate scores. The formula assumes a maximum probability for a draw and readjusts the score based on that. This results in an overcorrection. Ie: positive contempt values will result in a reported upper bound score and negative contempt values will result in a reported lower bound score.
For high contempt values it is recommended to adjust adjudication settings.
An increasingly popular format in human chess is Armageddon. Winter is the first engine to the author's knowledge to natively support Armageddon play as a UCI option. Internally this works by setting contempt to high positive value when playing white and a high negative value when playing black.
At the moment contempt is not set to the maximum in Armageddon mode. In the limited testing done this proved to perform more consistently. This may change in the future.
In Armageddon mode score recalibration is not performed. The score recalibration formula for regular contempt assumes the contempt pushes the score away from the true symmetrical evaluation. In Armageddon the true eval is not symmetric.
As of version v0.8.1 disabled. If I don't remember to add this again before v0.9, please pester me with an issue on github!
At the moment training a neural network for use in Winter is only supported in a very limited way. I intend to release the script shortly which was used in order to train the initial 0.6.2 net.
In the following I describe the steps to get from a pgn game database to a network for Winter.
- Get and compile the latest pgn-extract by David J. Barnes.
- Use pgn-extract on your .pgn file with the arguments
-Wuci
and--notags
. This will create a file readable by Winter. - Run Winter from command line. Call
gen_eval_csv filename out_filename
where filename is the name of the file generated in 2. and out_filename is what Winter should call the generated file. This will create a .csv dataset file (described below) based on pseudo-quiescent positions from the input games. - Train a neural network on the dataset. It is recommended to try to train something simple for now. Keep in mind I would like to refrain from making Winter rely on any external libraries.
- Integrate the network into Winter. In the future I will probably support loading external weight files, but for now you need to replace the appropriate entries in
src/net_weights.h
. -
make clean
andmake
The structure of the .csv dataset generated in 3. is as follows. The first column is a boolean value indicating wether the player to move won. The second column is a boolean value indicating whether the player to move scored at least a draw. The remaining collumns are features which are somewhat sparse. An overview of these features can be found in src/net_evaluation.h
.
For Tasks:
Click tags to check more tools for each tasksFor Jobs:
Alternative AI tools for Winter
Similar Open Source Tools
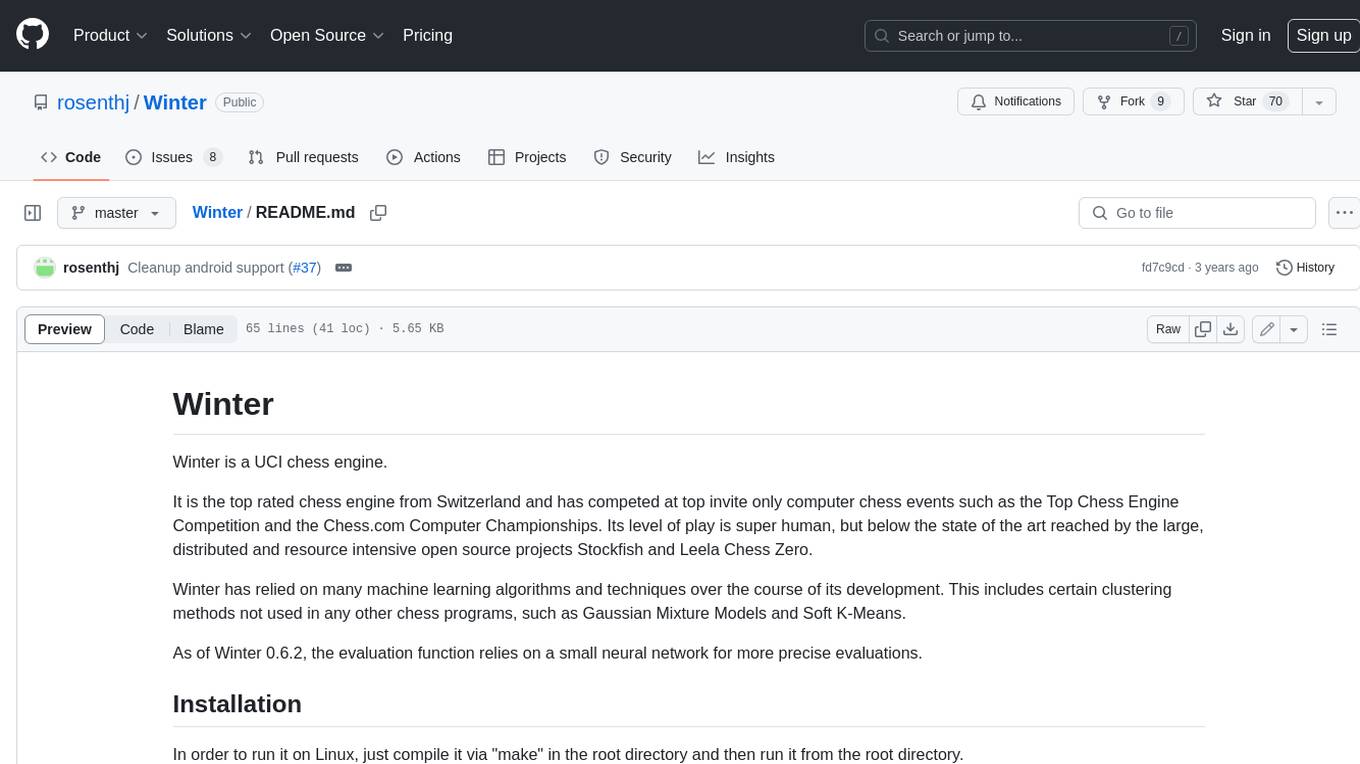
Winter
Winter is a UCI chess engine that has competed at top invite-only computer chess events. It is the top-rated chess engine from Switzerland and has a level of play that is super human but below the state of the art reached by large, distributed, and resource-intensive open-source projects like Stockfish and Leela Chess Zero. Winter has relied on many machine learning algorithms and techniques over the course of its development, including certain clustering methods not used in any other chess programs, such as Gaussian Mixture Models and Soft K-Means. As of Winter 0.6.2, the evaluation function relies on a small neural network for more precise evaluations.
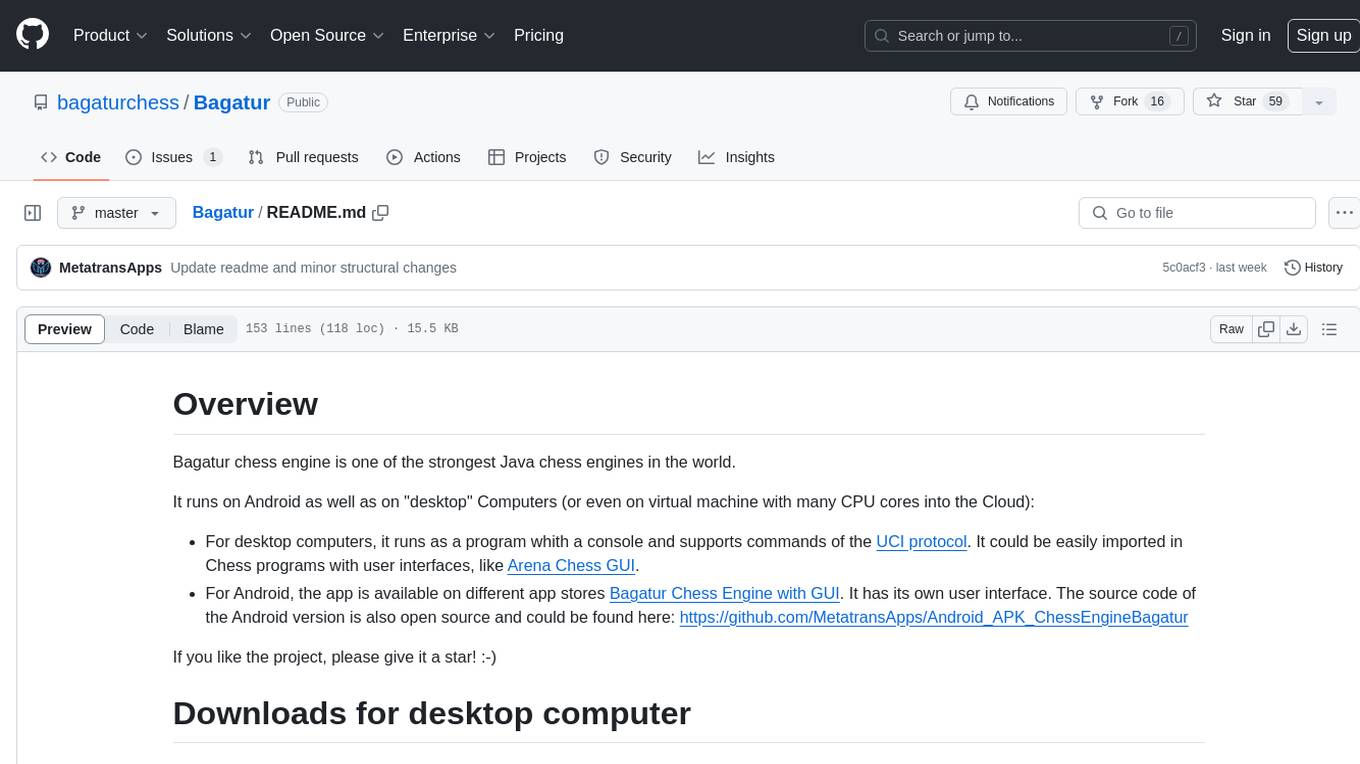
Bagatur
Bagatur chess engine is a powerful Java chess engine that can run on Android devices and desktop computers. It supports the UCI protocol and can be easily integrated into chess programs with user interfaces. The engine is available for download on various platforms and has advanced features like SMP (multicore) support and NNUE evaluation function. Bagatur also includes syzygy endgame tablebases and offers various UCI options for customization. The project started as a personal challenge to create a chess program that could defeat a friend, leading to years of development and improvements.
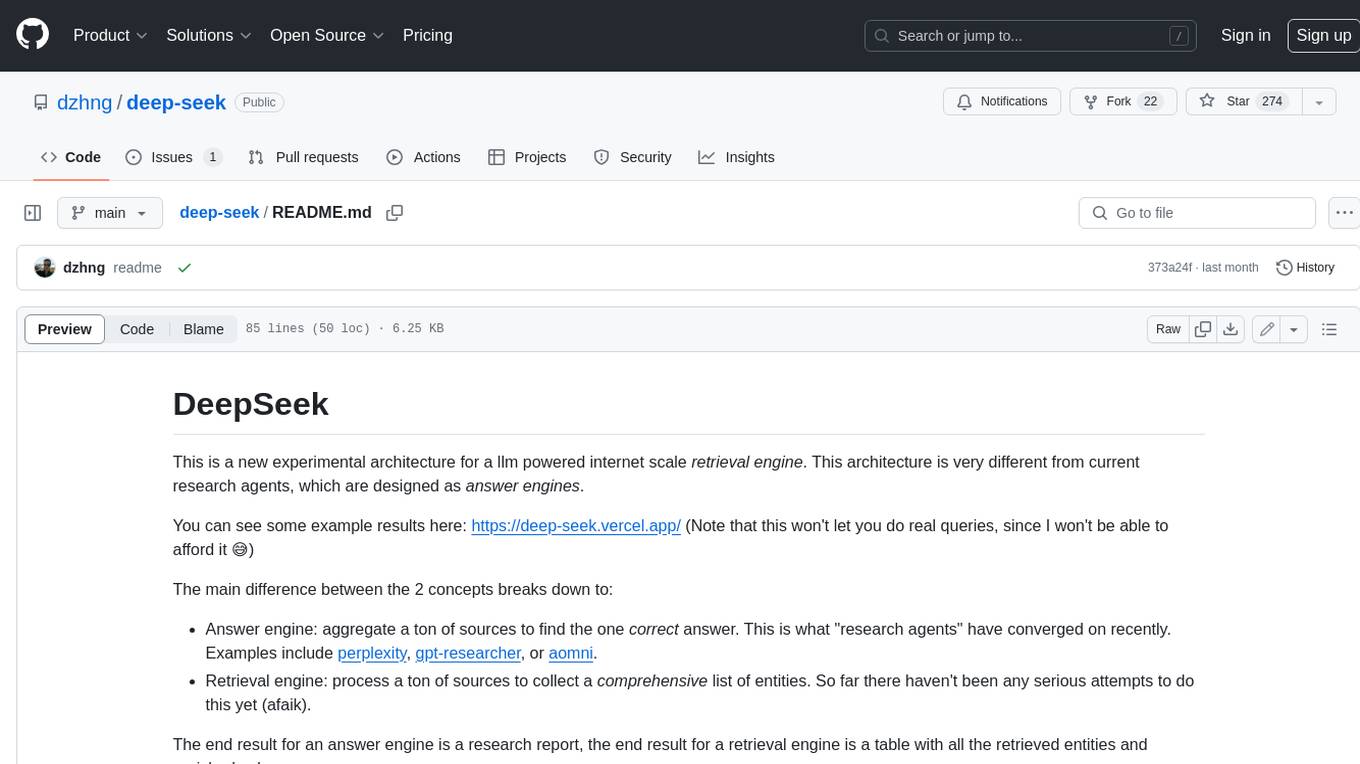
deep-seek
DeepSeek is a new experimental architecture for a large language model (LLM) powered internet-scale retrieval engine. Unlike current research agents designed as answer engines, DeepSeek aims to process a vast amount of sources to collect a comprehensive list of entities and enrich them with additional relevant data. The end result is a table with retrieved entities and enriched columns, providing a comprehensive overview of the topic. DeepSeek utilizes both standard keyword search and neural search to find relevant content, and employs an LLM to extract specific entities and their associated contents. It also includes a smaller answer agent to enrich the retrieved data, ensuring thoroughness. DeepSeek has the potential to revolutionize research and information gathering by providing a comprehensive and structured way to access information from the vastness of the internet.
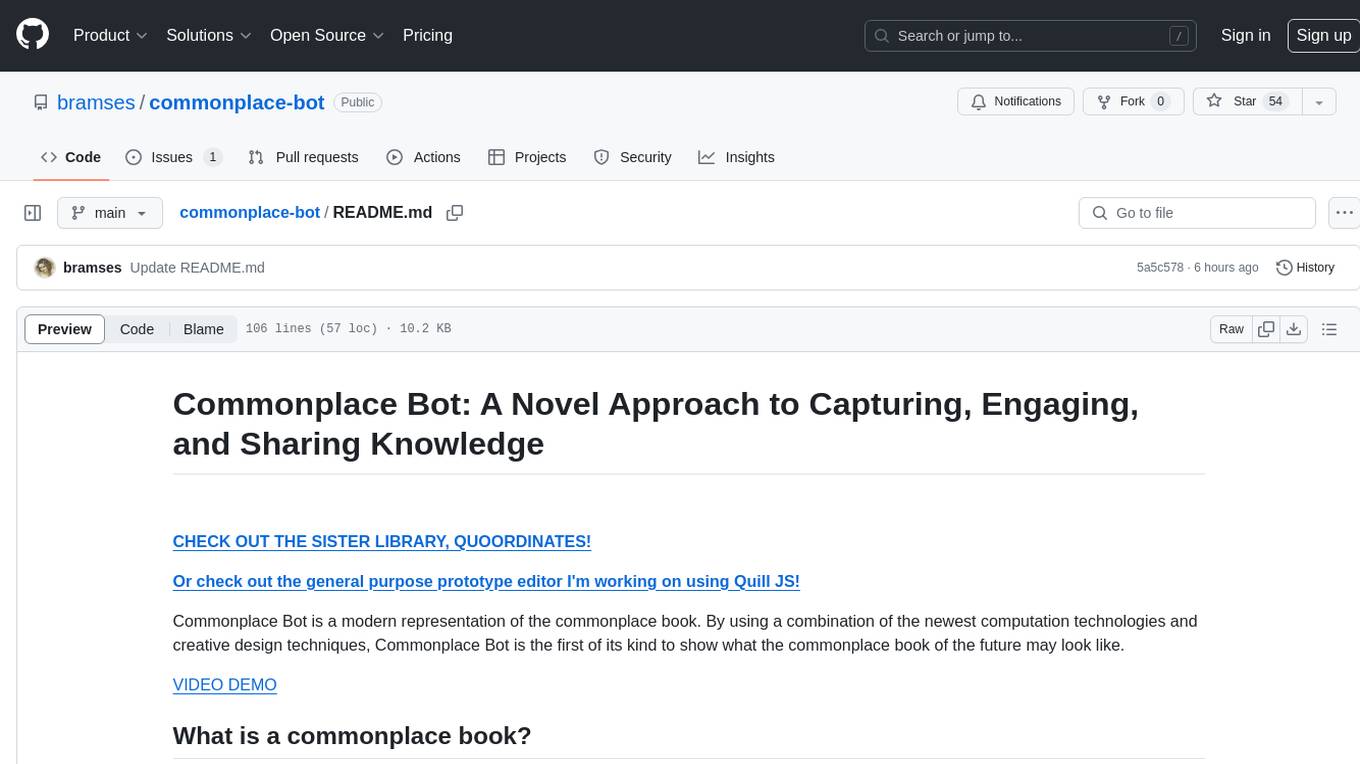
commonplace-bot
Commonplace Bot is a modern representation of the commonplace book, leveraging modern technological advancements in computation, data storage, machine learning, and networking. It aims to capture, engage, and share knowledge by providing a platform for users to collect ideas, quotes, and information, organize them efficiently, engage with the data through various strategies and triggers, and transform the data into new mediums for sharing. The tool utilizes embeddings and cached transformations for efficient data storage and retrieval, flips traditional engagement rules by engaging with the user, and enables users to alchemize raw data into new forms like art prompts. Commonplace Bot offers a unique approach to knowledge management and creative expression.
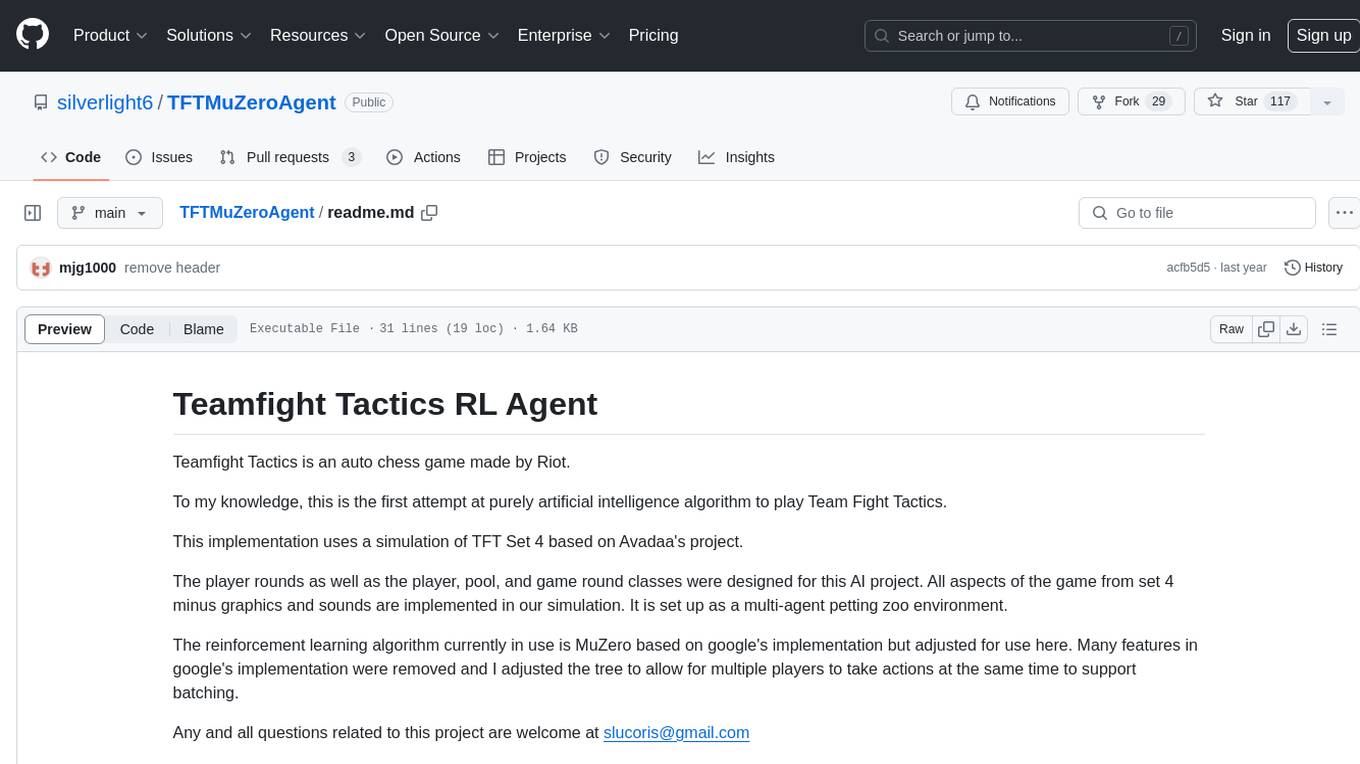
TFTMuZeroAgent
TFTMuZeroAgent is an implementation of a purely artificial intelligence algorithm to play Teamfight Tactics, an auto chess game made by Riot. It uses a simulation of TFT Set 4 and the MuZero reinforcement learning algorithm. The project provides a multi-agent petting zoo environment where players, pool, and game round classes are designed for AI project. The implementation excludes graphics and sounds but covers all aspects of the game from set 4. The codebase is open for contributions and improvements, allowing for additional models to be added to the environment.
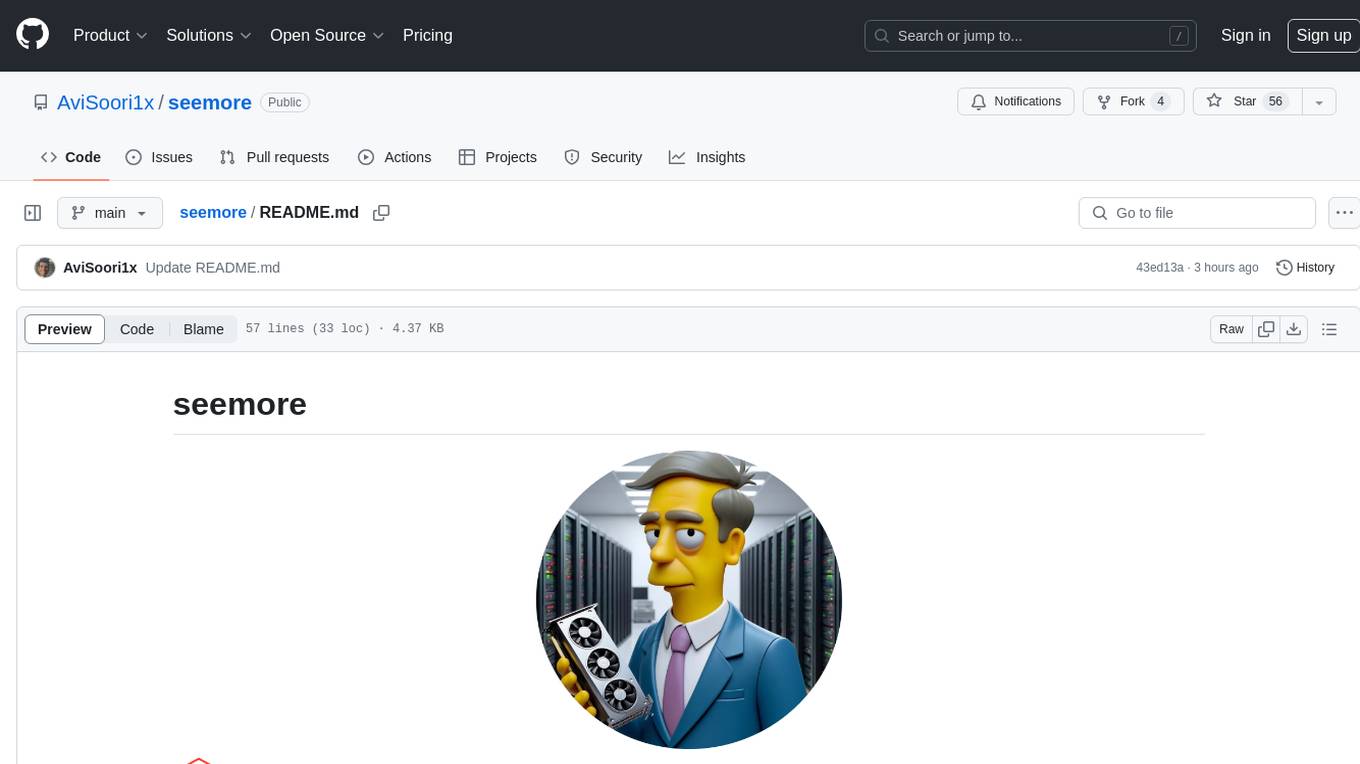
seemore
seemore is a vision language model developed in Pytorch, implementing components like image encoder, vision-language projector, and decoder language model. The model is built from scratch, including attention mechanisms and patch creation. It is designed for readability and hackability, with the intention to be improved upon. The implementation is based on public publications and borrows attention mechanism from makemore by Andrej Kapathy. The code was developed on Databricks using a single A100 for compute, and MLFlow is used for tracking metrics. The tool aims to provide a simplistic version of vision language models like Grok 1.5/GPT-4 Vision, suitable for experimentation and learning.
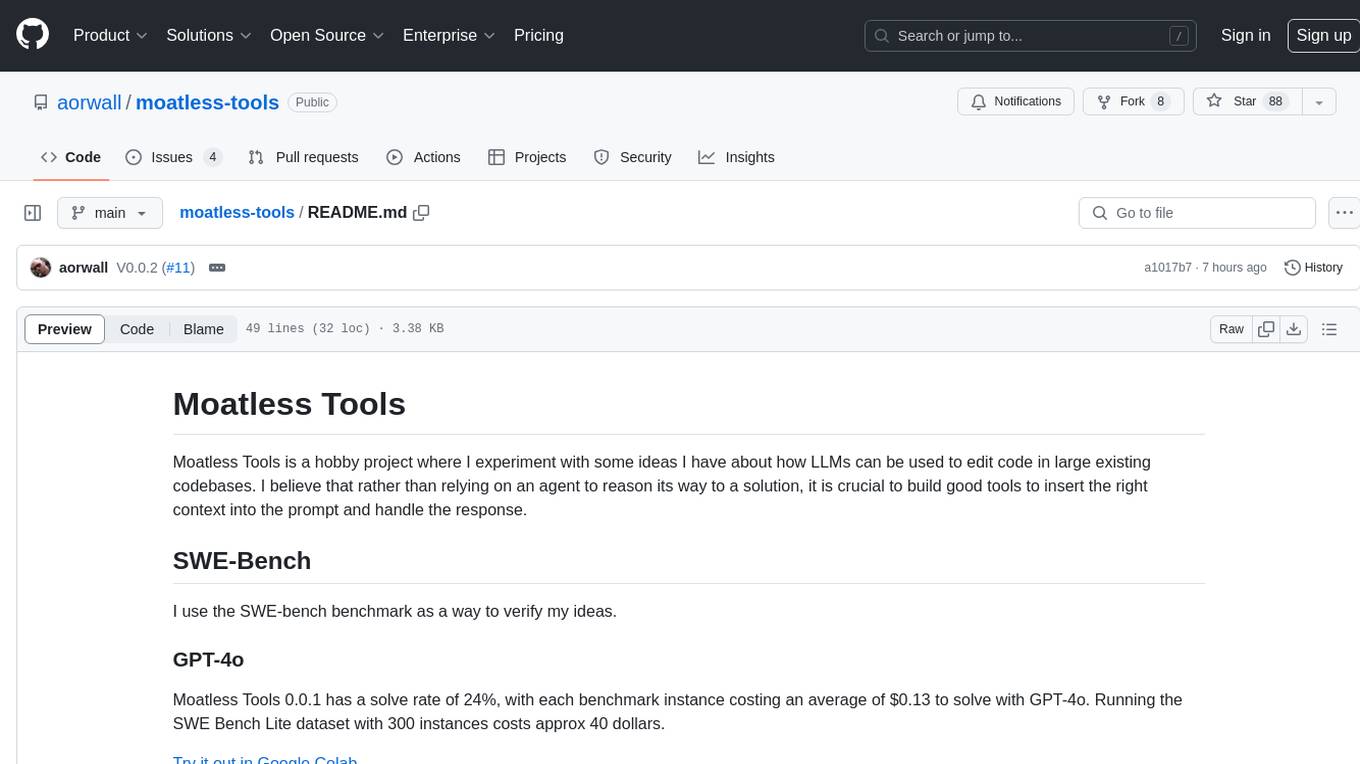
moatless-tools
Moatless Tools is a hobby project focused on experimenting with using Large Language Models (LLMs) to edit code in large existing codebases. The project aims to build tools that insert the right context into prompts and handle responses effectively. It utilizes an agentic loop functioning as a finite state machine to transition between states like Search, Identify, PlanToCode, ClarifyChange, and EditCode for code editing tasks.
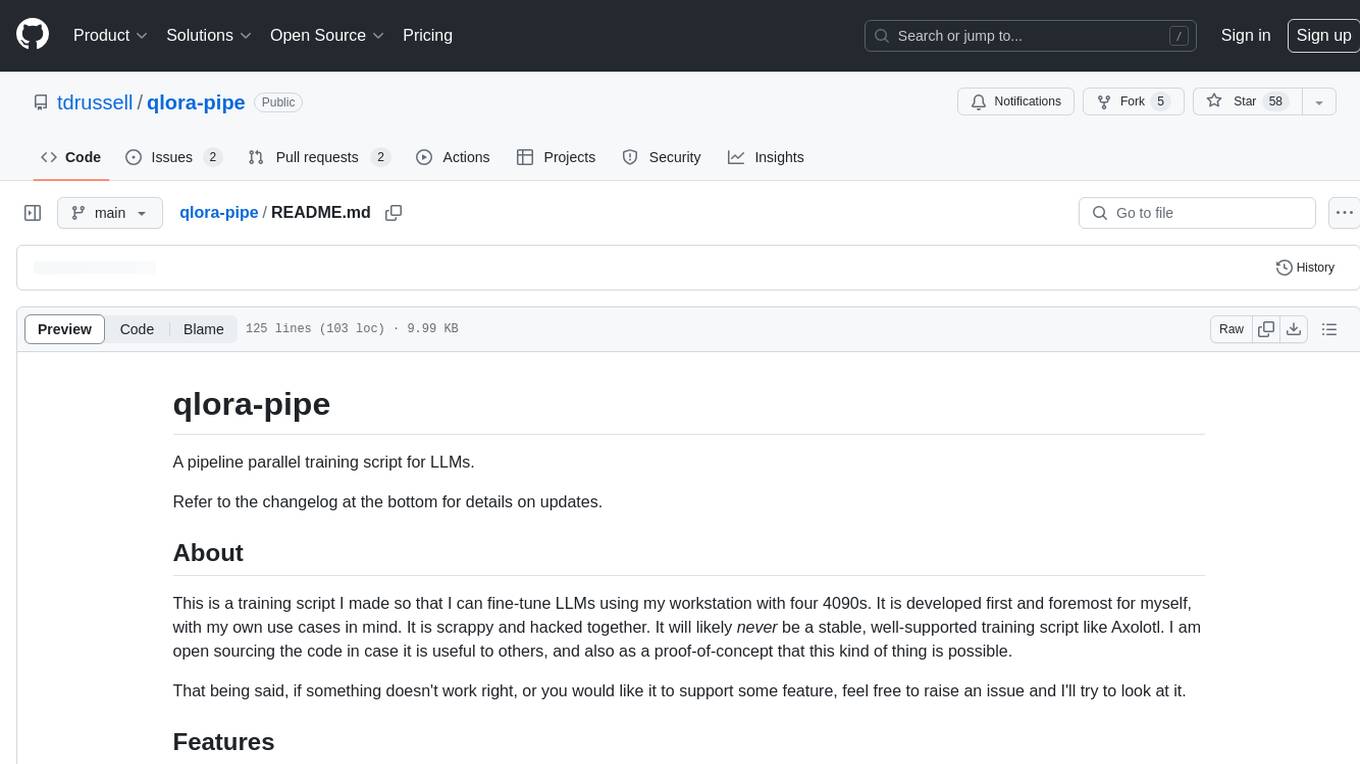
qlora-pipe
qlora-pipe is a pipeline parallel training script designed for efficiently training large language models that cannot fit on one GPU. It supports QLoRA, LoRA, and full fine-tuning, with efficient model loading and the ability to load any dataset that Axolotl can handle. The script allows for raw text training, resuming training from a checkpoint, logging metrics to Tensorboard, specifying a separate evaluation dataset, training on multiple datasets simultaneously, and supports various models like Llama, Mistral, Mixtral, Qwen-1.5, and Cohere (Command R). It handles pipeline- and data-parallelism using Deepspeed, enabling users to set the number of GPUs, pipeline stages, and gradient accumulation steps for optimal utilization.
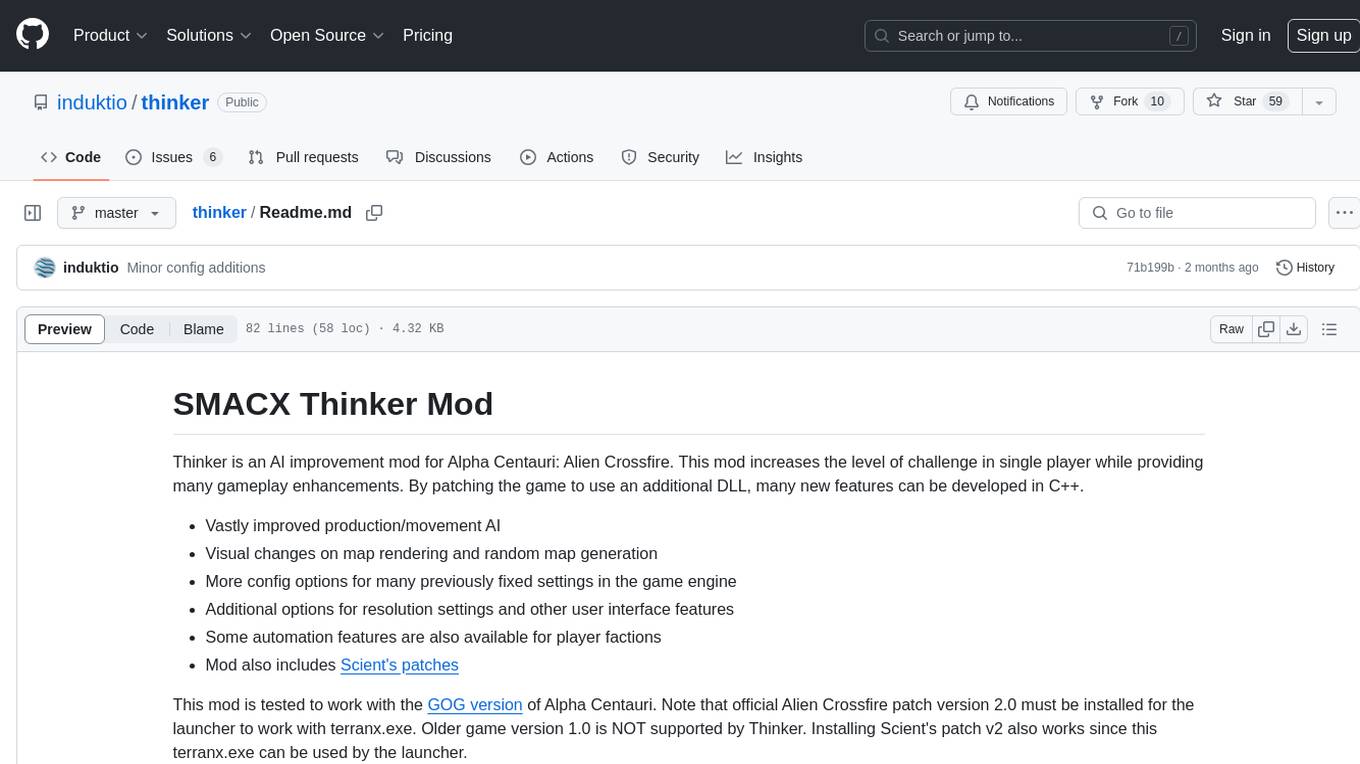
thinker
Thinker is an AI improvement mod for Alpha Centauri: Alien Crossfire that enhances single player challenge and gameplay with features like improved production/movement AI, visual changes on map rendering, more config options, resolution settings, and automation features. It includes Scient's patches and requires the GOG version of Alpha Centauri with the official Alien Crossfire patch version 2.0 installed. The mod provides additional DLL features developed in C++ for a richer gaming experience.
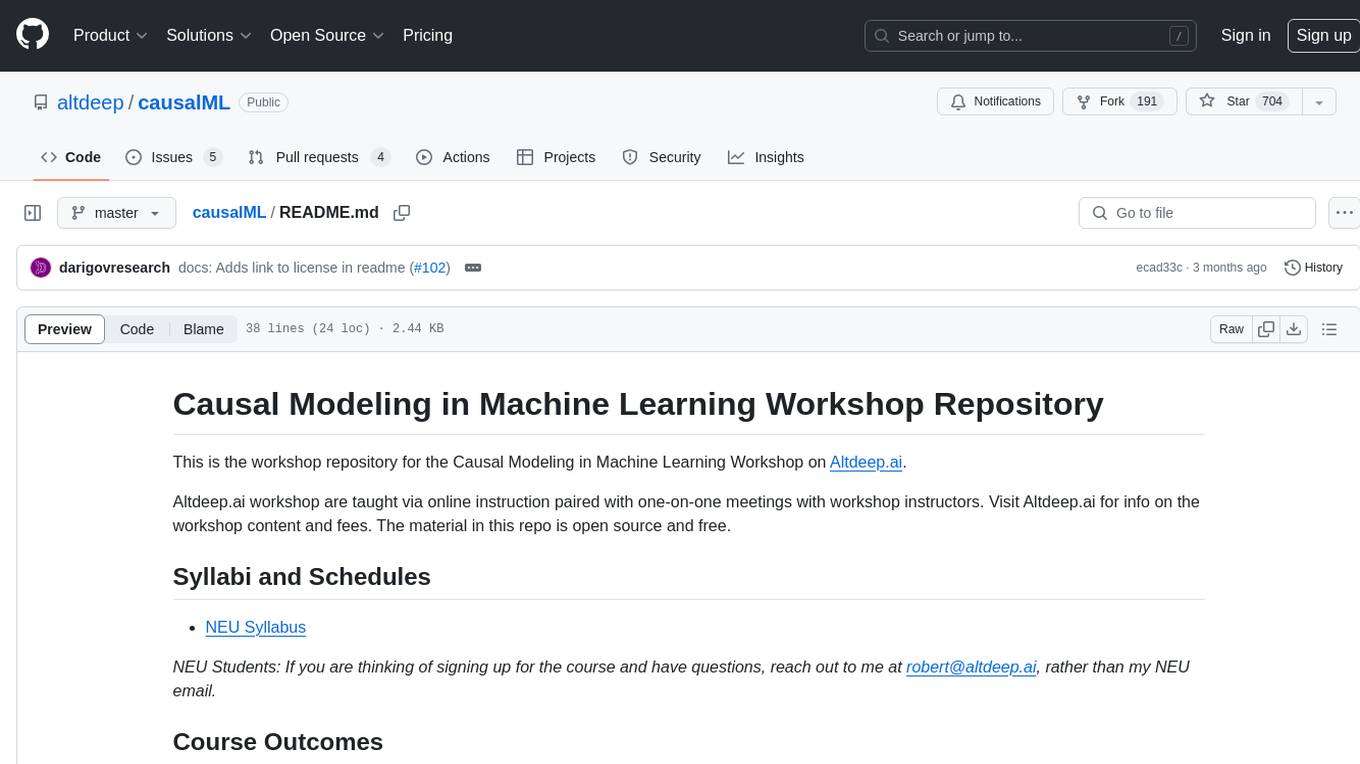
causalML
This repository is the workshop repository for the Causal Modeling in Machine Learning Workshop on Altdeep.ai. The material is open source and free. The course covers causality in model-based machine learning, Bayesian modeling, interventions, counterfactual reasoning, and deep causal latent variable models. It aims to equip learners with the ability to build causal reasoning algorithms into decision-making systems in data science and machine learning teams within top-tier technology organizations.
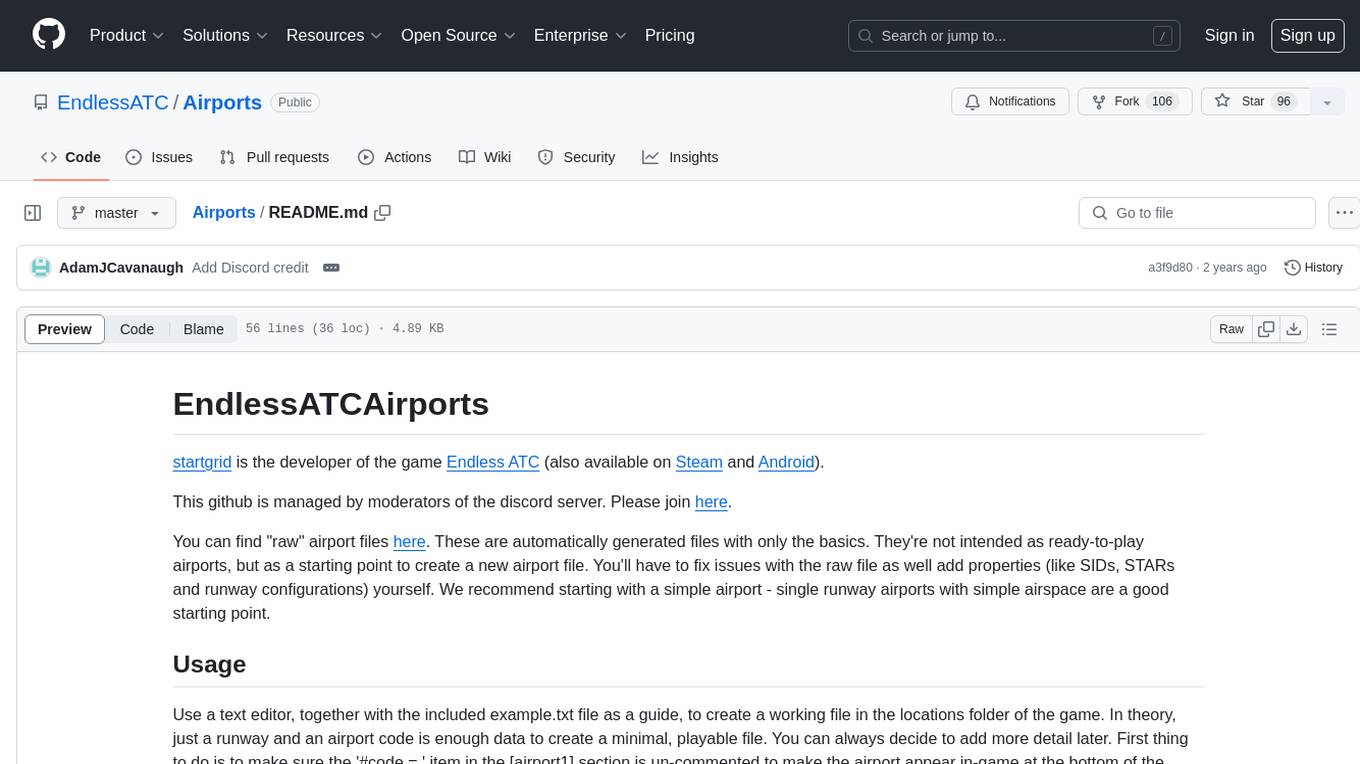
Airports
This repository contains raw airport files intended as a starting point to create new airport files for the game Endless ATC. Users can contribute by customizing airport files and submitting pull requests. The repository also welcomes markdown files with gameplay and development tips. Contributors are encouraged to join the Discord server for assistance and information.
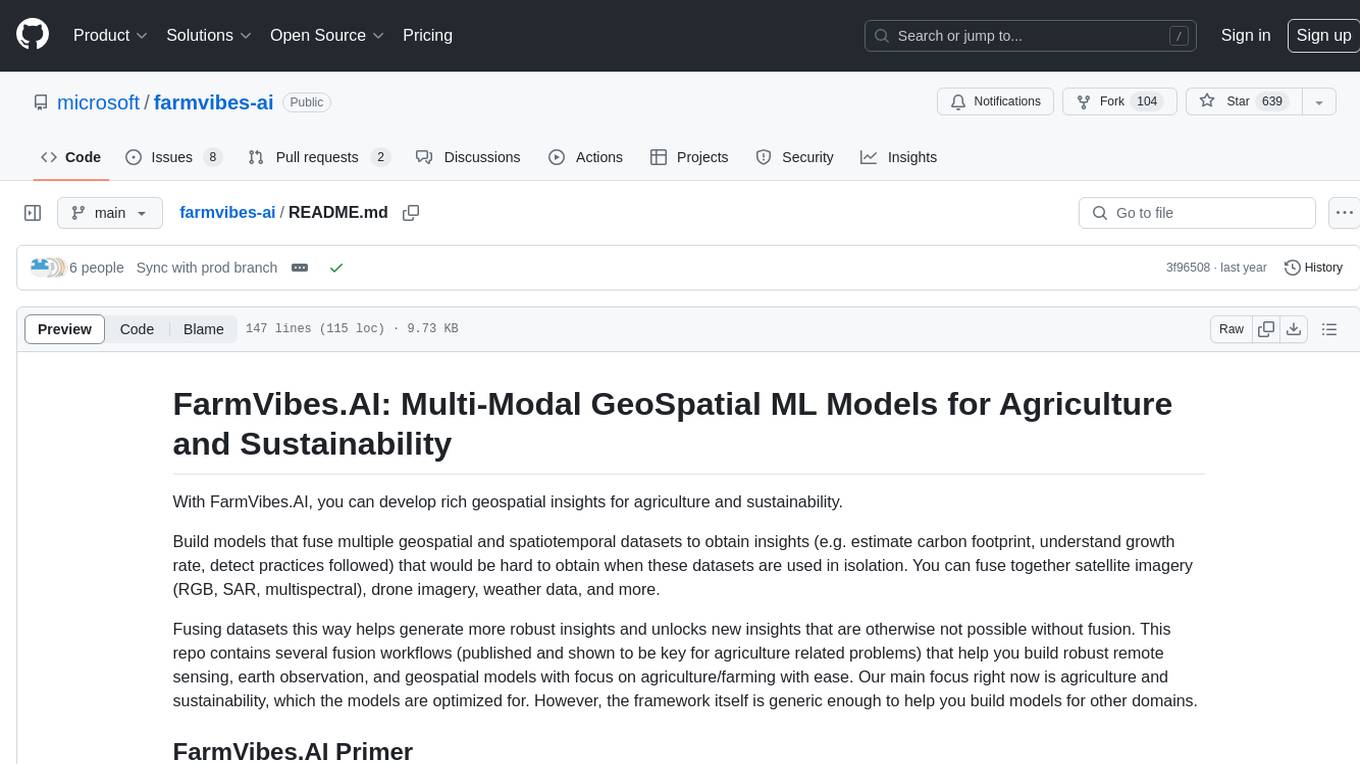
farmvibes-ai
FarmVibes.AI is a repository focused on developing multi-modal geospatial machine learning models for agriculture and sustainability. It enables users to fuse various geospatial and spatiotemporal datasets, such as satellite imagery, drone imagery, and weather data, to generate robust insights for agriculture-related problems. The repository provides fusion workflows, data preparation tools, model training notebooks, and an inference engine to facilitate the creation of geospatial models tailored for agriculture and farming. Users can interact with the tools via a local cluster, REST API, or a Python client, and the repository includes documentation and notebook examples to guide users in utilizing FarmVibes.AI for tasks like harvest date detection, climate impact estimation, micro climate prediction, and crop identification.
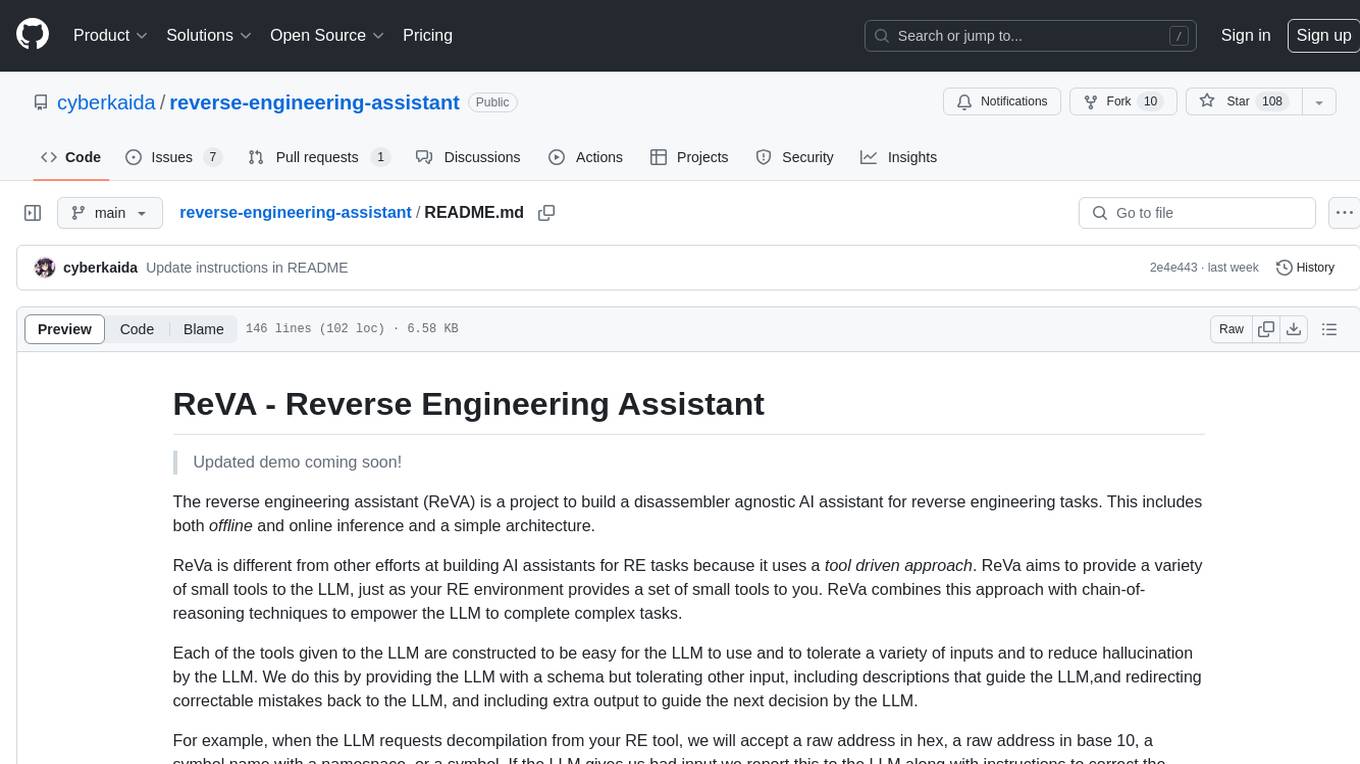
reverse-engineering-assistant
ReVA (Reverse Engineering Assistant) is a project aimed at building a disassembler agnostic AI assistant for reverse engineering tasks. It utilizes a tool-driven approach, providing small tools to the user to empower them in completing complex tasks. The assistant is designed to accept various inputs, guide the user in correcting mistakes, and provide additional context to encourage exploration. Users can ask questions, perform tasks like decompilation, class diagram generation, variable renaming, and more. ReVA supports different language models for online and local inference, with easy configuration options. The workflow involves opening the RE tool and program, then starting a chat session to interact with the assistant. Installation includes setting up the Python component, running the chat tool, and configuring the Ghidra extension for seamless integration. ReVA aims to enhance the reverse engineering process by breaking down actions into small parts, including the user's thoughts in the output, and providing support for monitoring and adjusting prompts.
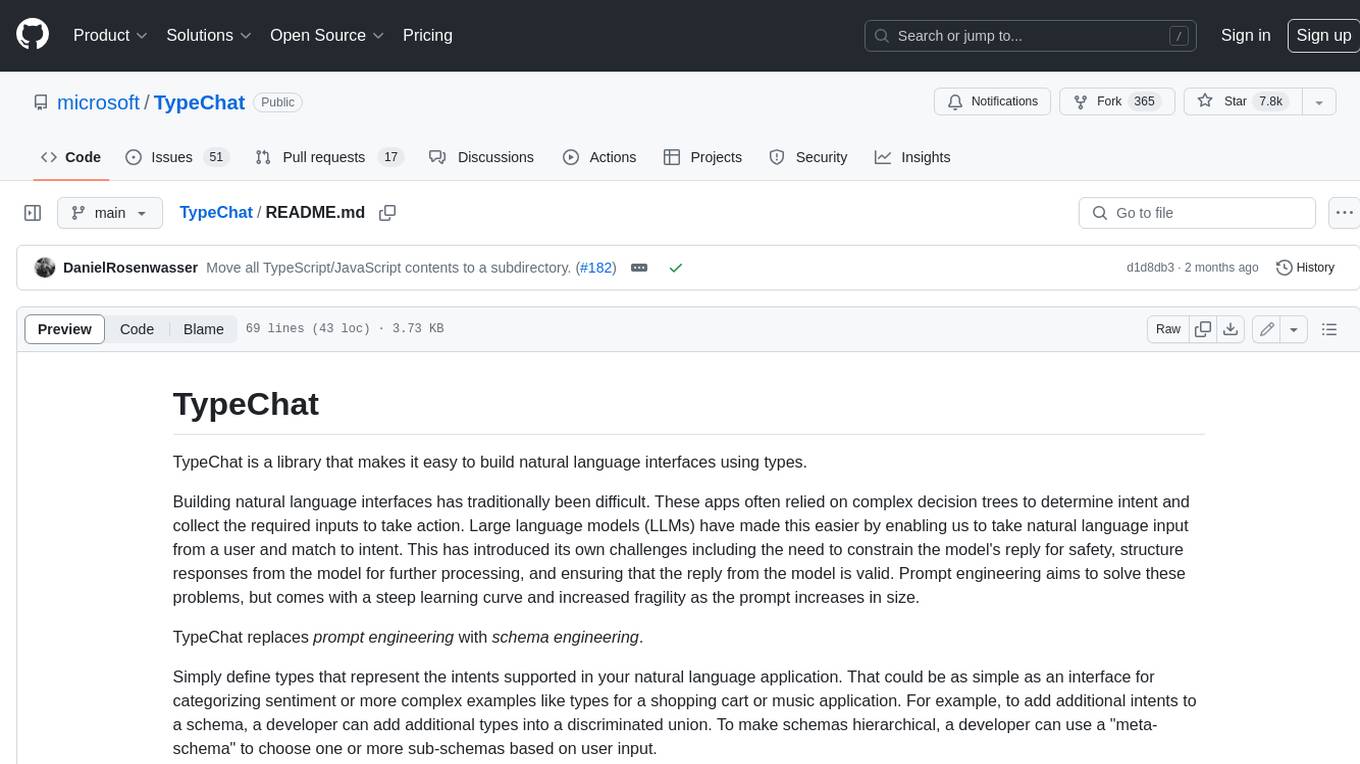
TypeChat
TypeChat is a library that simplifies the creation of natural language interfaces using types. Traditionally, building natural language interfaces has been challenging, often relying on complex decision trees to determine intent and gather necessary inputs for action. Large language models (LLMs) have simplified this process by allowing us to accept natural language input from users and match it to intent. However, this has introduced new challenges, such as the need to constrain the model's response for safety, structure responses from the model for further processing, and ensure the validity of the model's response. Prompt engineering aims to address these issues, but it comes with a steep learning curve and increased fragility as the prompt grows in size.
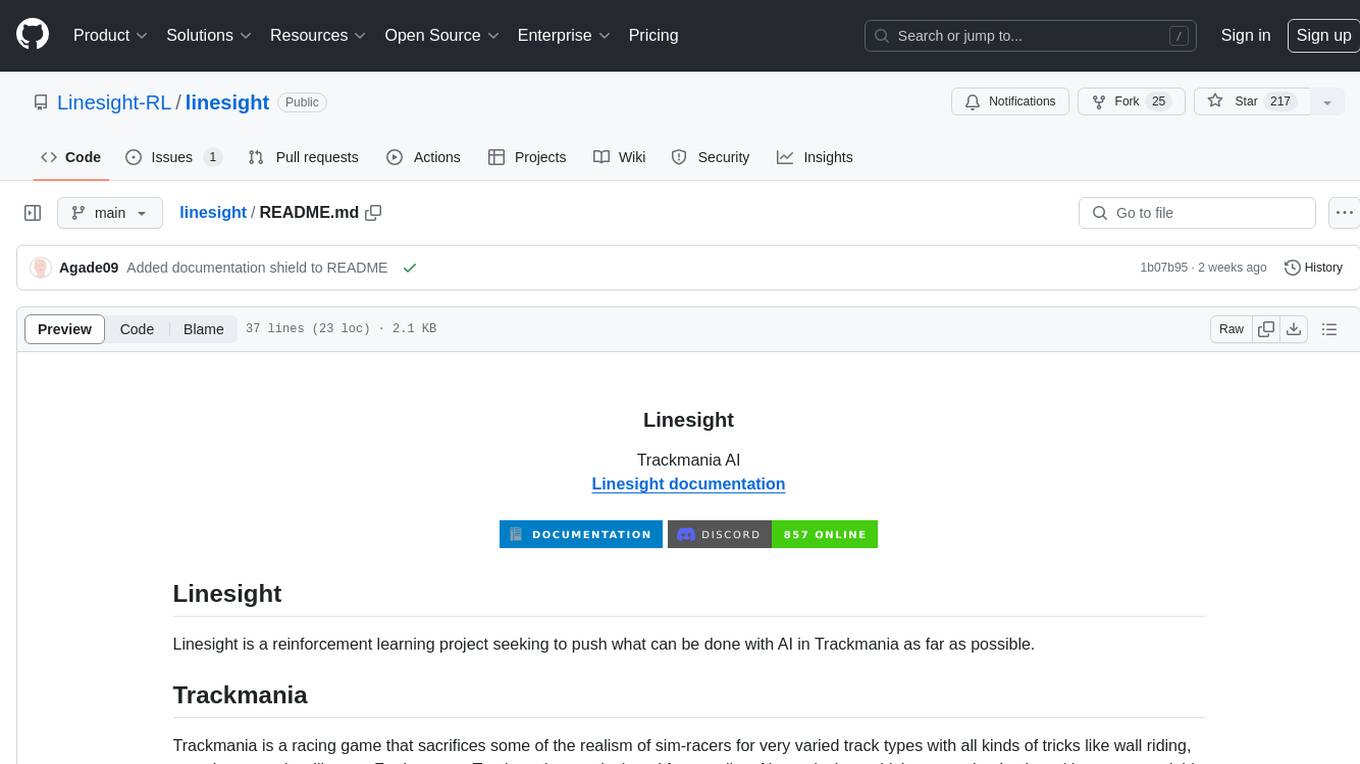
linesight
Linesight is a reinforcement learning project focused on advancing AI capabilities in the racing game Trackmania. It aims to push the boundaries of AI performance by utilizing deep learning algorithms to achieve human-level driving and beat world records on official campaign tracks. The project provides an interface to interact with Trackmania Nations Forever programmatically, enabling tasks such as sending inputs, retrieving car states, and capturing screenshots. With a strong emphasis on equality of input devices, Linesight serves as a benchmark for testing various reinforcement learning algorithms in a challenging and dynamic gaming environment.
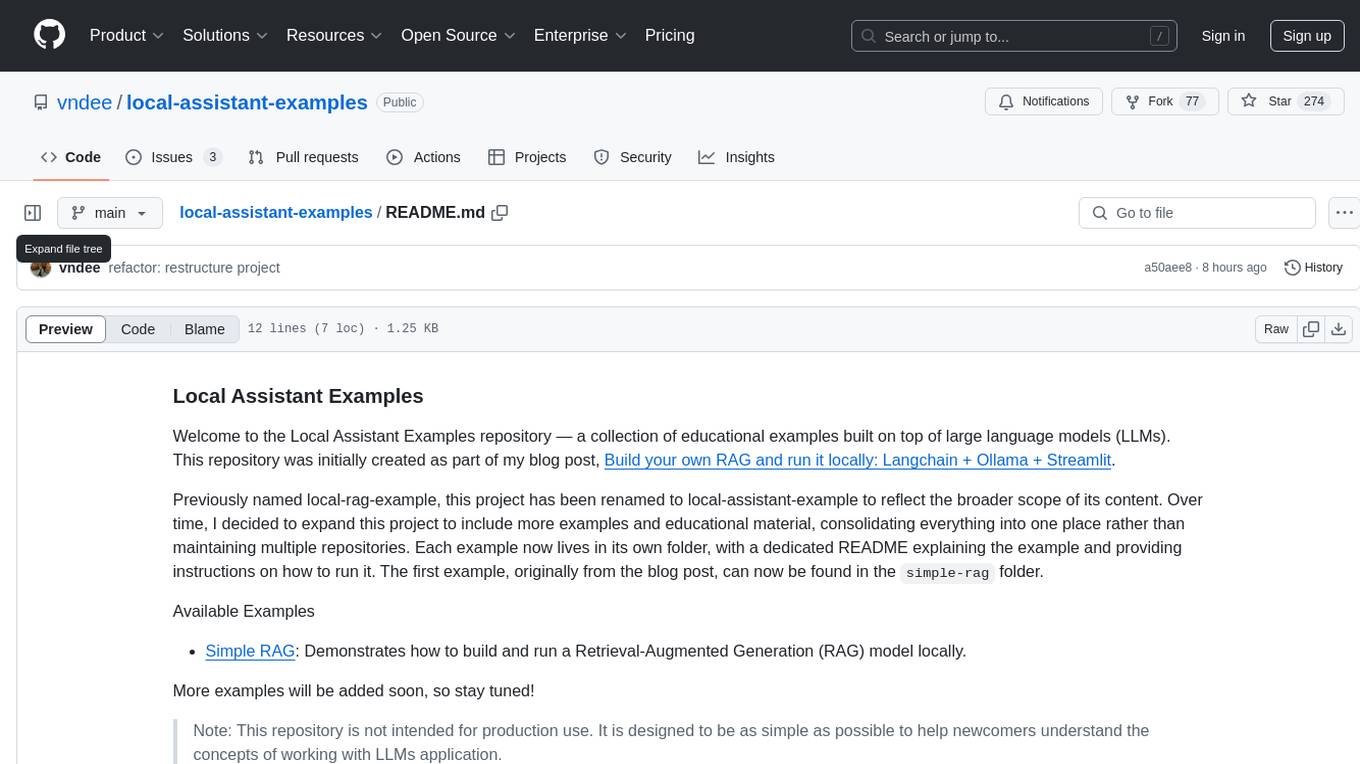
local-assistant-examples
The Local Assistant Examples repository is a collection of educational examples showcasing the use of large language models (LLMs). It was initially created for a blog post on building a RAG model locally, and has since expanded to include more examples and educational material. Each example is housed in its own folder with a dedicated README providing instructions on how to run it. The repository is designed to be simple and educational, not for production use.
For similar tasks
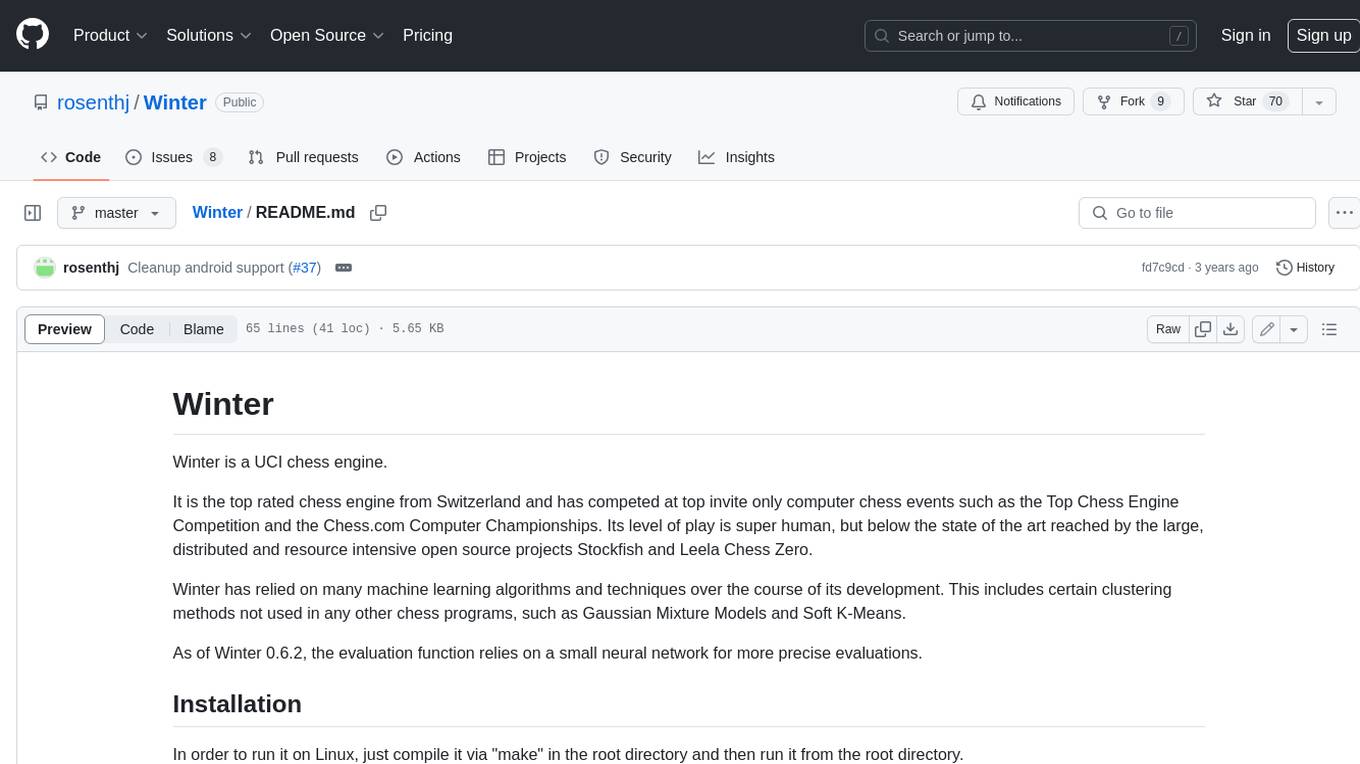
Winter
Winter is a UCI chess engine that has competed at top invite-only computer chess events. It is the top-rated chess engine from Switzerland and has a level of play that is super human but below the state of the art reached by large, distributed, and resource-intensive open-source projects like Stockfish and Leela Chess Zero. Winter has relied on many machine learning algorithms and techniques over the course of its development, including certain clustering methods not used in any other chess programs, such as Gaussian Mixture Models and Soft K-Means. As of Winter 0.6.2, the evaluation function relies on a small neural network for more precise evaluations.
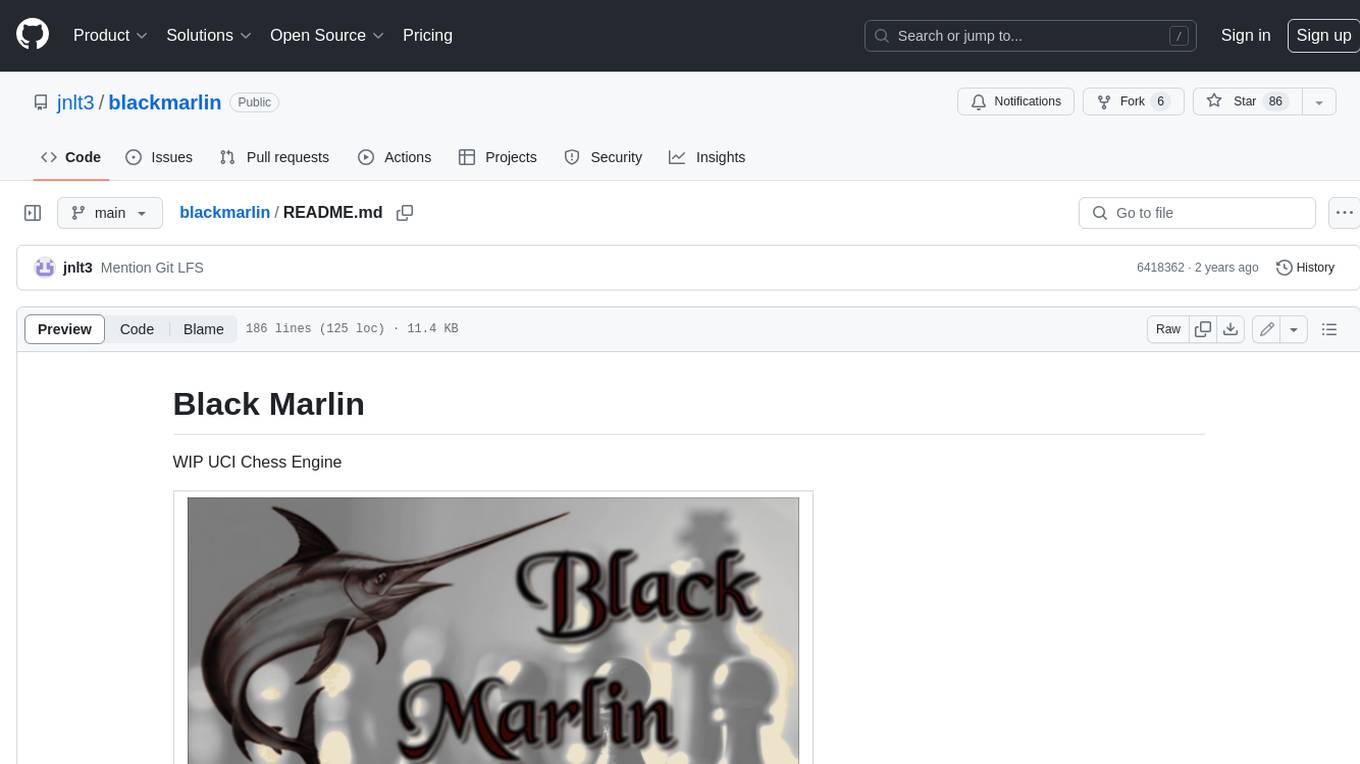
blackmarlin
Black Marlin is a UCI compliant chess engine fully written in Rust by Doruk Sekercioglu. It supports Chess960 and features a variety of search algorithms, pruning techniques, and evaluation methods. Black Marlin is designed to be efficient and accurate, and it has been shown to perform well against other top chess engines.
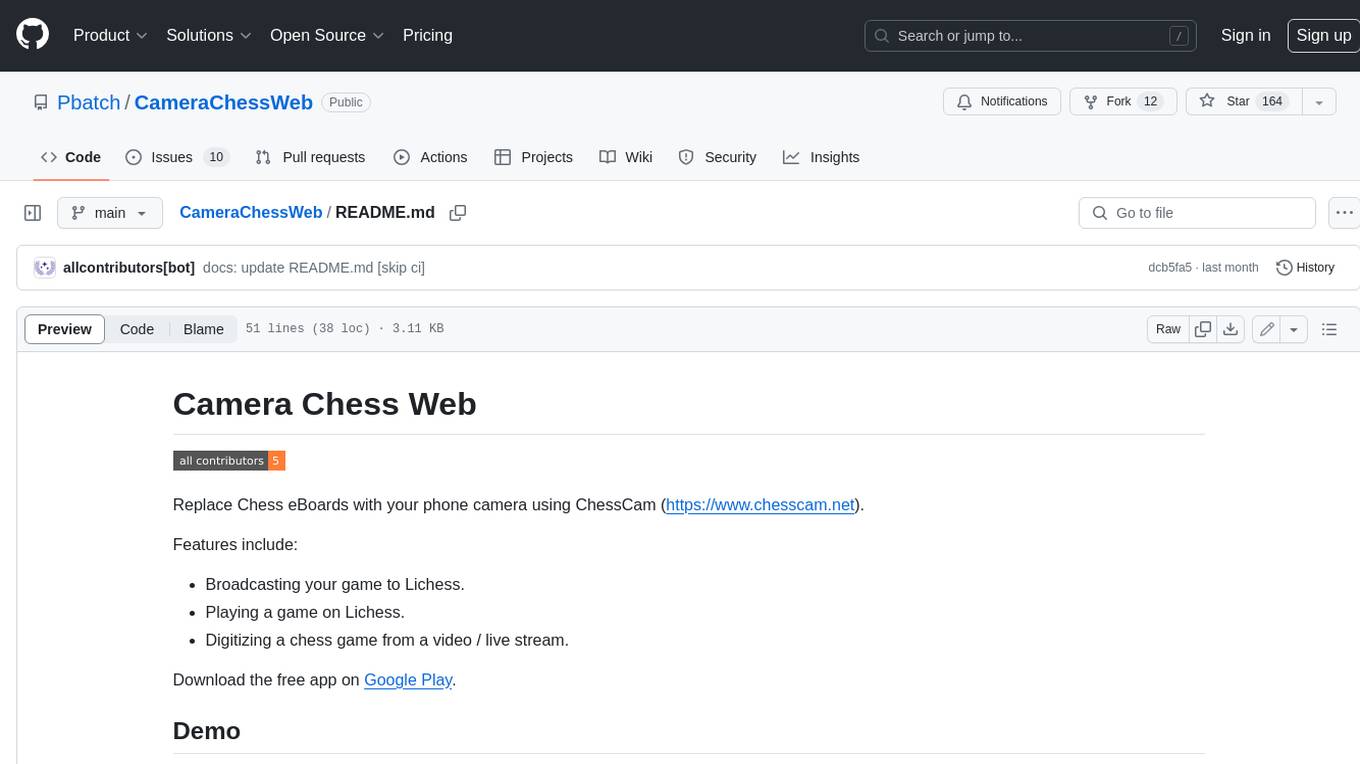
CameraChessWeb
Camera Chess Web is a tool that allows you to use your phone camera to replace chess eBoards. With Camera Chess Web, you can broadcast your game to Lichess, play a game on Lichess, or digitize a chess game from a video or live stream. Camera Chess Web is free to download on Google Play.
For similar jobs
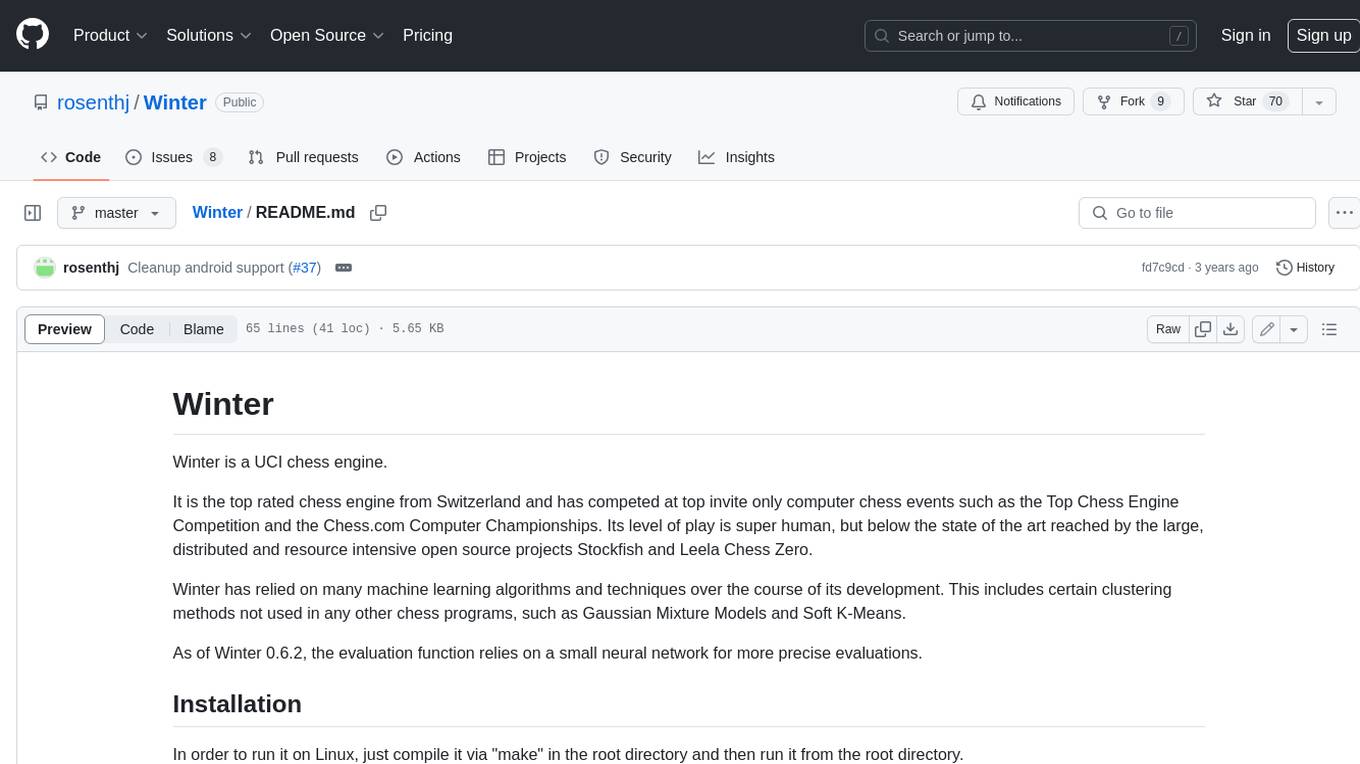
Winter
Winter is a UCI chess engine that has competed at top invite-only computer chess events. It is the top-rated chess engine from Switzerland and has a level of play that is super human but below the state of the art reached by large, distributed, and resource-intensive open-source projects like Stockfish and Leela Chess Zero. Winter has relied on many machine learning algorithms and techniques over the course of its development, including certain clustering methods not used in any other chess programs, such as Gaussian Mixture Models and Soft K-Means. As of Winter 0.6.2, the evaluation function relies on a small neural network for more precise evaluations.
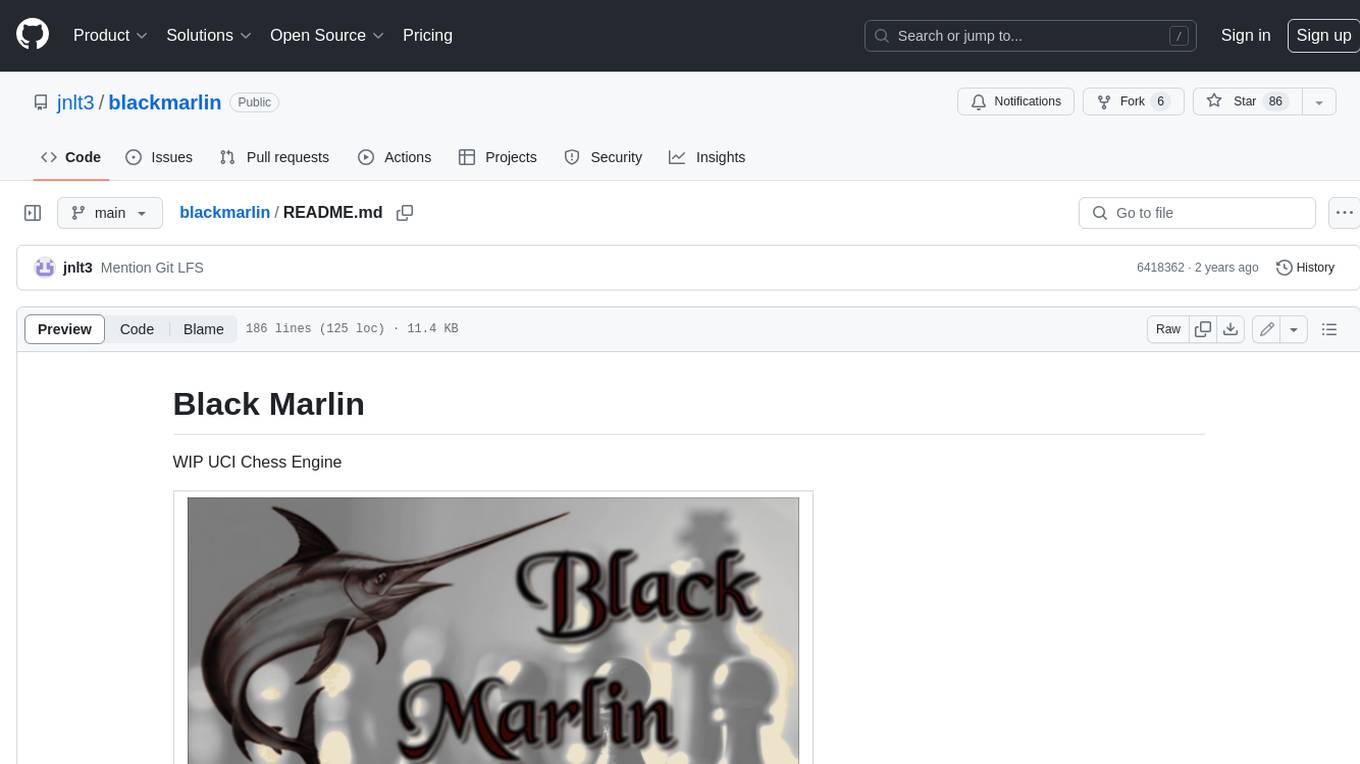
blackmarlin
Black Marlin is a UCI compliant chess engine fully written in Rust by Doruk Sekercioglu. It supports Chess960 and features a variety of search algorithms, pruning techniques, and evaluation methods. Black Marlin is designed to be efficient and accurate, and it has been shown to perform well against other top chess engines.