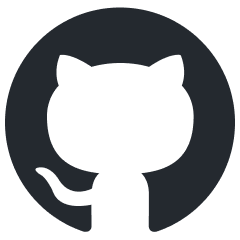
llm-apps-java-spring-ai
Samples showing how to build Java applications powered by Generative AI and LLMs using Spring AI and Spring Boot.
Stars: 294
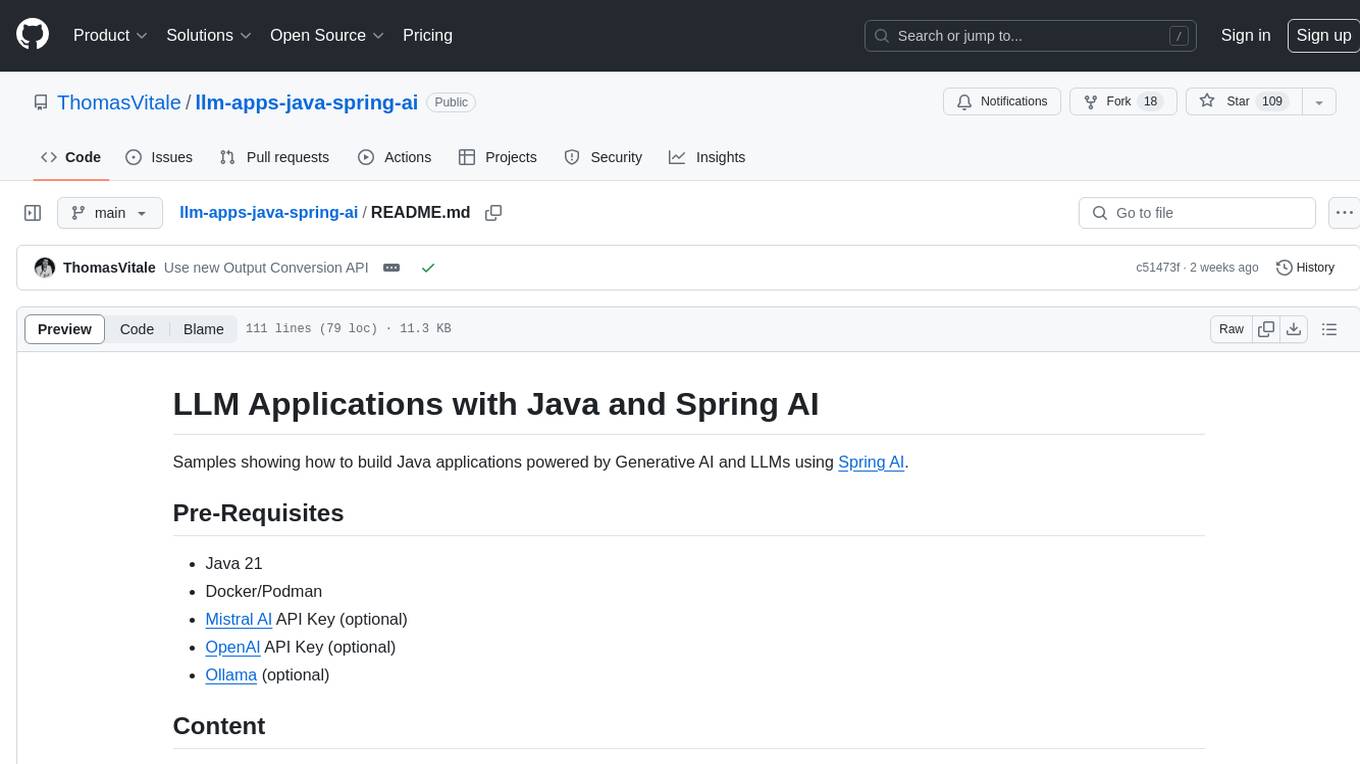
The 'LLM Applications with Java and Spring AI' repository provides samples demonstrating how to build Java applications powered by Generative AI and Large Language Models (LLMs) using Spring AI. It includes projects for question answering, chat completion models, prompts, templates, multimodality, output converters, embedding models, document ETL pipeline, function calling, image models, and audio models. The repository also lists prerequisites such as Java 21, Docker/Podman, Mistral AI API Key, OpenAI API Key, and Ollama. Users can explore various use cases and projects to leverage LLMs for text generation, vector transformation, document processing, and more.
README:
Samples showing how to build Java applications powered by Generative AI and Large Language Models (LLMs) using Spring AI.
- Java 23
- Docker/Podman
Project | Description |
---|---|
chatbot | Chatbot using LLMs via Ollama. |
question-answering | Question answering with documents (RAG) using LLMs via Ollama and PGVector. |
semantic-search | Semantic search using LLMs via Ollama and PGVector. |
structured-data-extraction | Structured data extraction using LLMs via Ollama. |
text-classification | Text classification using LLMs via Ollama. |
Project | Description |
---|---|
chat-models-mistral-ai | Text generation with LLMs via Mistral AI. |
chat-models-ollama | Text generation with LLMs via Ollama. |
chat-models-openai | Text generation with LLMs via OpenAI. |
chat-models-multiple-providers | Text generation with LLMs via multiple providers. |
Project | Description |
---|---|
prompts-basics-ollama | Prompting using simple text with LLMs via Ollama. |
prompts-basics-openai | Prompting using simple text with LLMs via OpenAI. |
prompts-messages-ollama | Prompting using structured messages and roles with LLMs via Ollama. |
prompts-messages-openai | Prompting using structured messages and roles with LLMs via OpenAI. |
prompts-templates-ollama | Prompting using templates with LLMs via Ollama. |
prompts-templates-openai | Prompting using templates with LLMs via OpenAI. |
Project | Description |
---|---|
structured-output-ollama | Converting the LLM output to structured JSON and Java objects via Ollama. |
structured-output-openai | Converting the LLM output to structured JSON and Java objects via Open AI. |
Project | Description |
---|---|
multimodality-ollama | Multimodality to include various media in a prompt with LLMs via Ollama. |
multimodality-openai | Multimodality to include various media in a prompt with LLMs via OpenAI. |
Project | Description |
---|---|
function-calling-mistral-ai | Function calling with LLMs via Mistral AI. |
function-calling-ollama | Function calling with LLMs via Ollama. |
function-calling-openai | Function calling with LLMs via OpenAI. |
Project | Description |
---|---|
embedding-models-mistral-ai | Vector transformation (embeddings) with LLMs via Mistral AI. |
embedding-models-ollama | Vector transformation (embeddings) with LLMs via Ollama. |
embedding-models-openai | Vector transformation (embeddings) with LLMs via OpenAI. |
embedding-models-transformers | Vector transformation (embeddings) with LLMs via ONNX Sentence Transformers. |
Project | Description |
---|---|
document-readers-json-ollama | Reading and vectorizing JSON documents with LLMs via Ollama. |
document-readers-pdf-ollama | Reading and vectorizing PDF documents with LLMs via Ollama. |
document-readers-text-ollama | Reading and vectorizing text documents with LLMs via Ollama. |
document-transformers-metadata-ollama | Enrich documents with keywords and summary metadata for enhanced retrieval via Ollama. |
document-transformers-splitters-ollama | Divide documents into chunks to fit the LLM context window via Ollama. |
Coming soon
Coming soon
Coming soon
Project | Description |
---|---|
image-models-openai | Image generation with LLMs via OpenAI. |
Project | Description |
---|---|
audio-models-speech-openai | Speech generation with LLMs via OpenAI. |
audio-models-transcription-openai | Speech transcription with LLMs via OpenAI. |
Coming soon
Project | Description |
---|---|
observability-models-mistral-ai | LLM Observability for Mistral AI. |
observability-models-ollama | LLM Observability for Ollama. |
observability-models-openai | LLM Observability for OpenAI. |
observability-vector-stores-pgvector | Vector Store Observability for PGVector. |
Coming soon
Coming soon
- Introducing Spring AI by Christian Tzolov and Mark Pollack (Spring I/O 2024)
- Spring AI Is All You Need by Christian Tzolov (GOTO Amsterdam 2024)
- Concerto for Java and AI - Building Production-Ready LLM Applications by Thomas Vitale (Spring I/O 2024)
- Building Intelligent Applications With Spring AI by Dan Vega (JetBrains Live Stream)
- Spring AI Series by Dan Vega
- Spring AI Series by Craig Walls
- Spring AI Series by Josh Long
For Tasks:
Click tags to check more tools for each tasksFor Jobs:
Alternative AI tools for llm-apps-java-spring-ai
Similar Open Source Tools
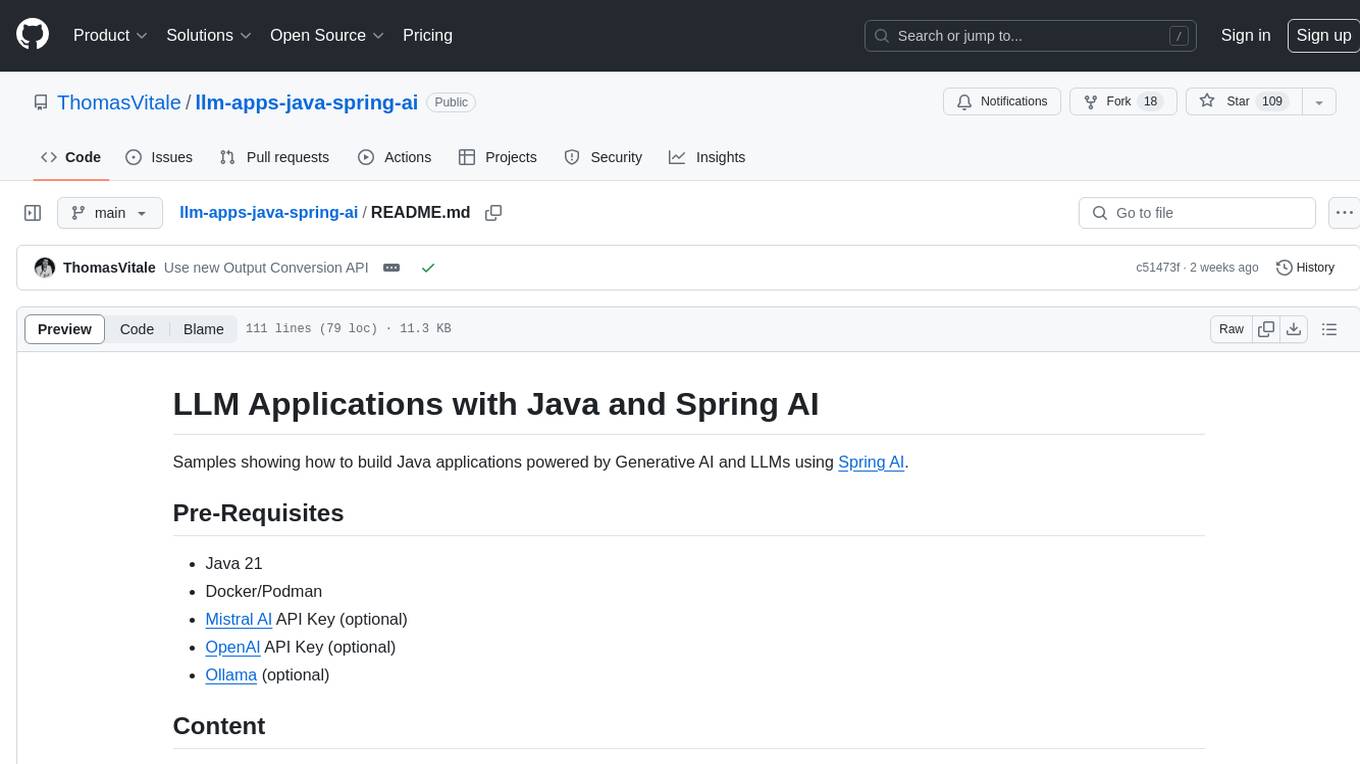
llm-apps-java-spring-ai
The 'LLM Applications with Java and Spring AI' repository provides samples demonstrating how to build Java applications powered by Generative AI and Large Language Models (LLMs) using Spring AI. It includes projects for question answering, chat completion models, prompts, templates, multimodality, output converters, embedding models, document ETL pipeline, function calling, image models, and audio models. The repository also lists prerequisites such as Java 21, Docker/Podman, Mistral AI API Key, OpenAI API Key, and Ollama. Users can explore various use cases and projects to leverage LLMs for text generation, vector transformation, document processing, and more.
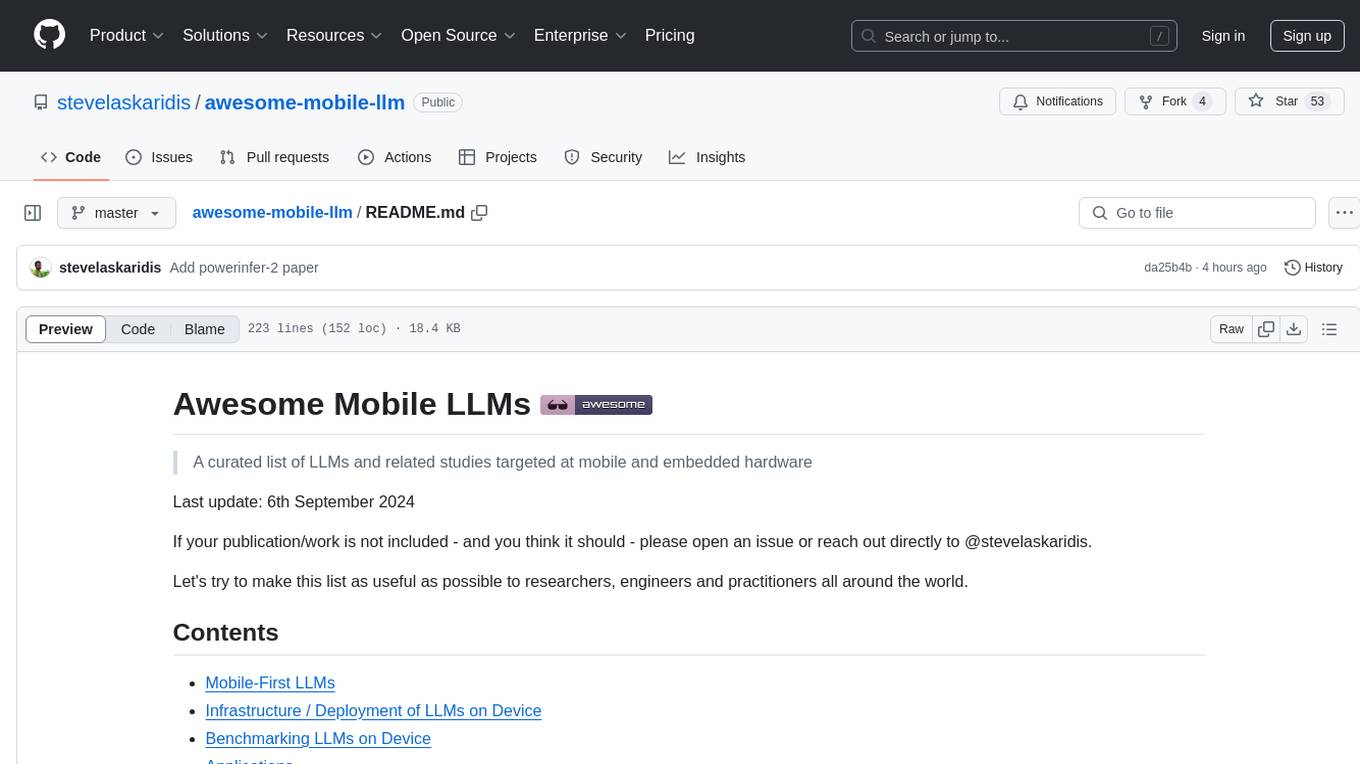
awesome-mobile-llm
Awesome Mobile LLMs is a curated list of Large Language Models (LLMs) and related studies focused on mobile and embedded hardware. The repository includes information on various LLM models, deployment frameworks, benchmarking efforts, applications, multimodal LLMs, surveys on efficient LLMs, training LLMs on device, mobile-related use-cases, industry announcements, and related repositories. It aims to be a valuable resource for researchers, engineers, and practitioners interested in mobile LLMs.
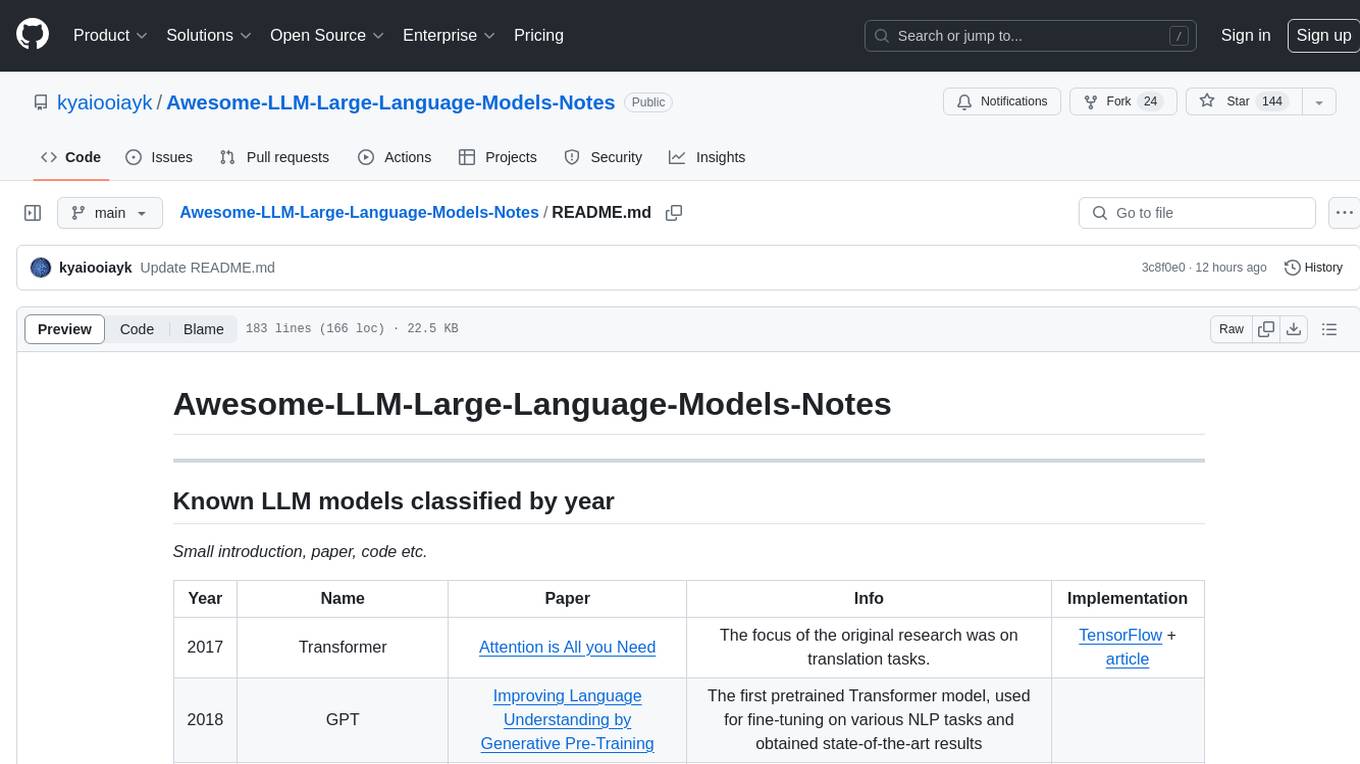
Awesome-LLM-Large-Language-Models-Notes
Awesome-LLM-Large-Language-Models-Notes is a repository that provides a comprehensive collection of information on various Large Language Models (LLMs) classified by year, size, and name. It includes details on known LLM models, their papers, implementations, and specific characteristics. The repository also covers LLM models classified by architecture, must-read papers, blog articles, tutorials, and implementations from scratch. It serves as a valuable resource for individuals interested in understanding and working with LLMs in the field of Natural Language Processing (NLP).
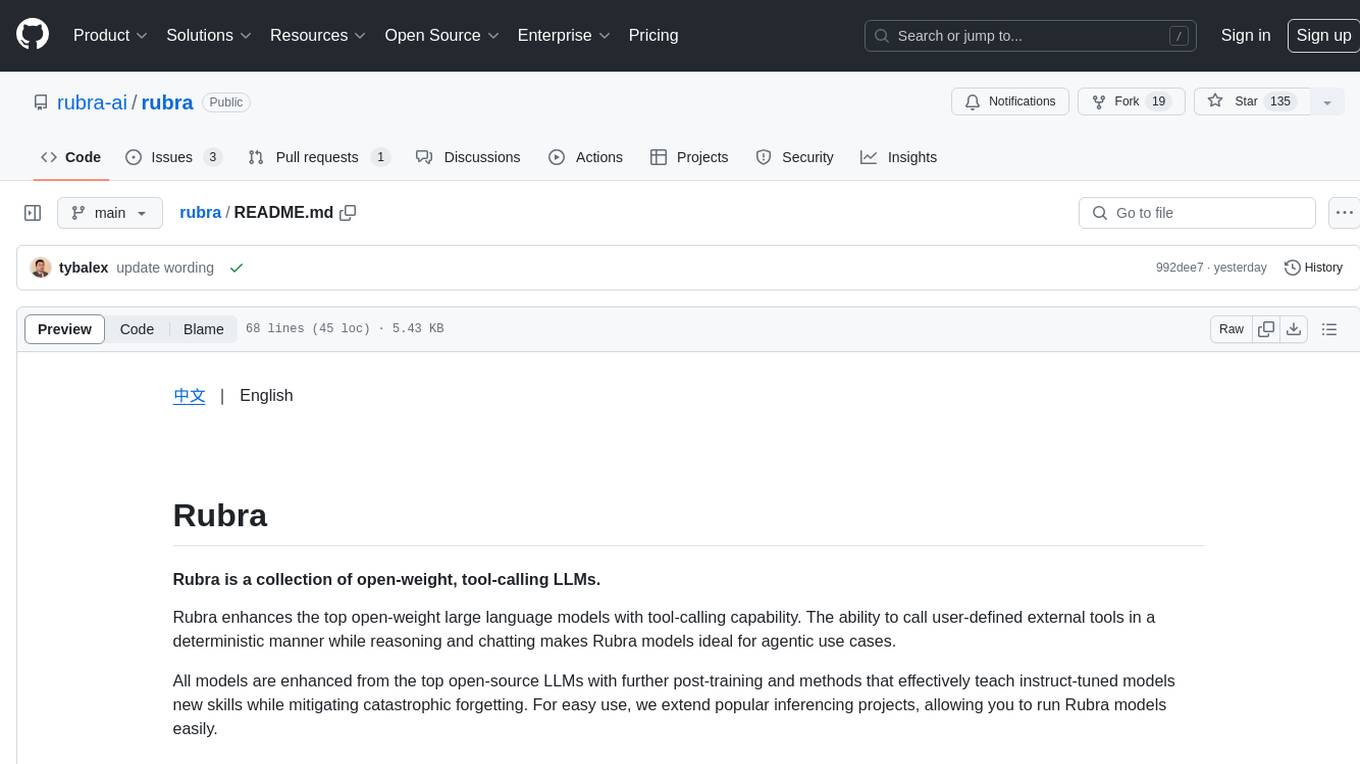
rubra
Rubra is a collection of open-weight large language models enhanced with tool-calling capability. It allows users to call user-defined external tools in a deterministic manner while reasoning and chatting, making it ideal for agentic use cases. The models are further post-trained to teach instruct-tuned models new skills and mitigate catastrophic forgetting. Rubra extends popular inferencing projects for easy use, enabling users to run the models easily.
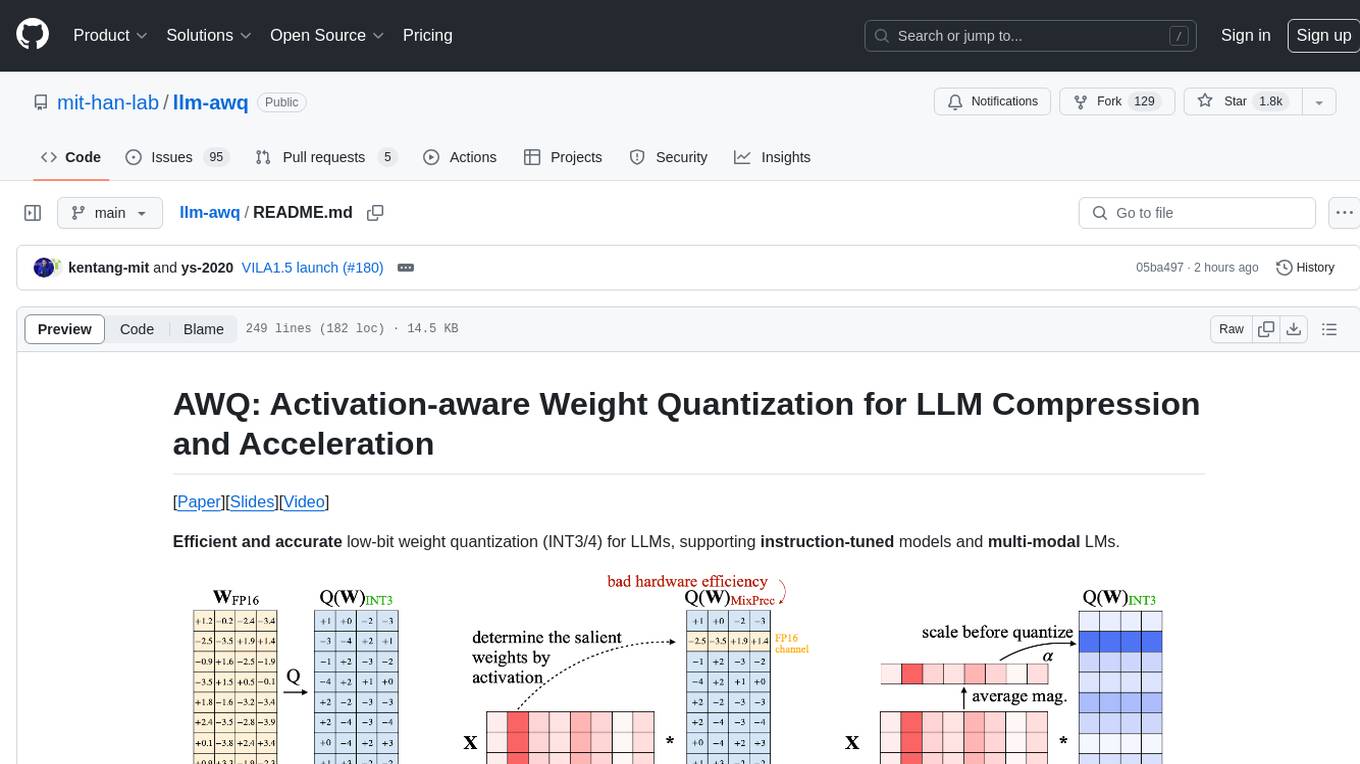
llm-awq
AWQ (Activation-aware Weight Quantization) is a tool designed for efficient and accurate low-bit weight quantization (INT3/4) for Large Language Models (LLMs). It supports instruction-tuned models and multi-modal LMs, providing features such as AWQ search for accurate quantization, pre-computed AWQ model zoo for various LLMs, memory-efficient 4-bit linear in PyTorch, and efficient CUDA kernel implementation for fast inference. The tool enables users to run large models on resource-constrained edge platforms, delivering more efficient responses with LLM/VLM chatbots through 4-bit inference.
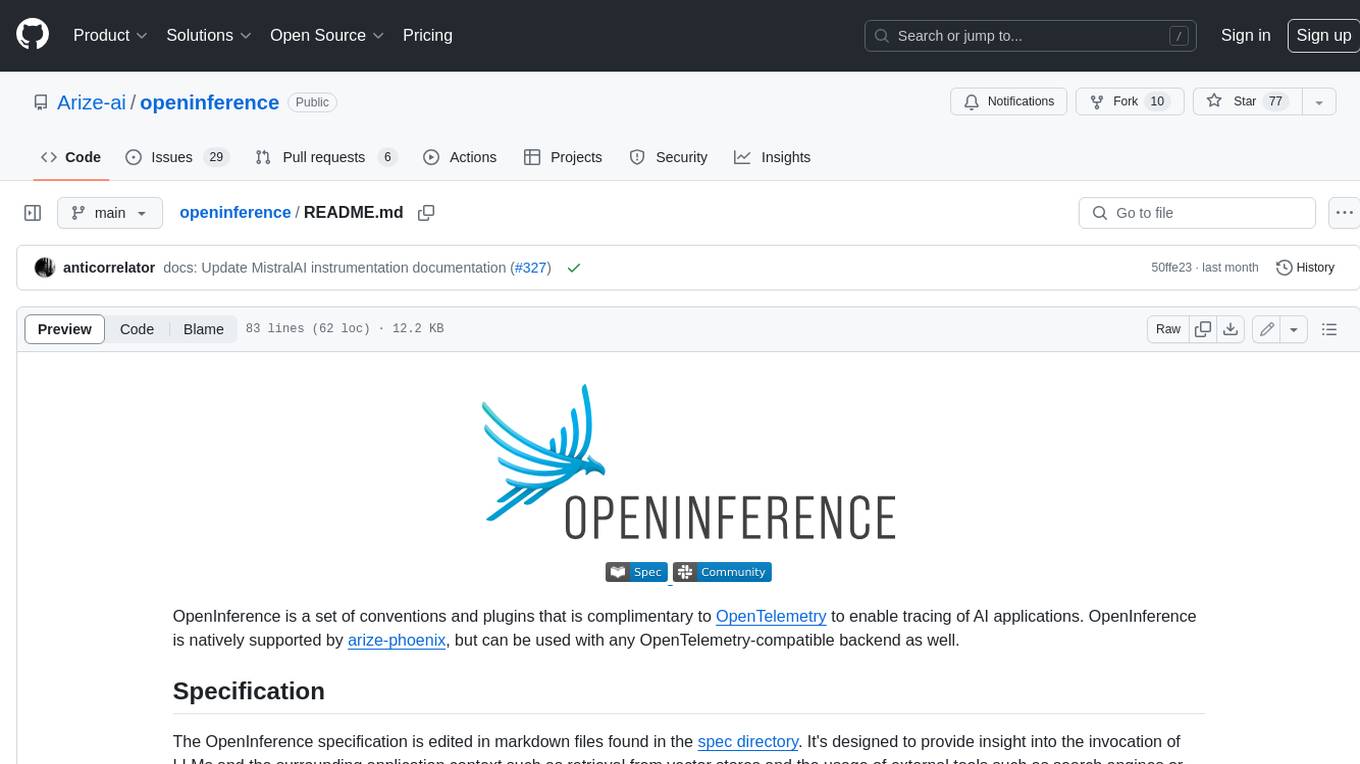
openinference
OpenInference is a set of conventions and plugins that complement OpenTelemetry to enable tracing of AI applications. It provides a way to capture and analyze the performance and behavior of AI models, including their interactions with other components of the application. OpenInference is designed to be language-agnostic and can be used with any OpenTelemetry-compatible backend. It includes a set of instrumentations for popular machine learning SDKs and frameworks, making it easy to add tracing to your AI applications.
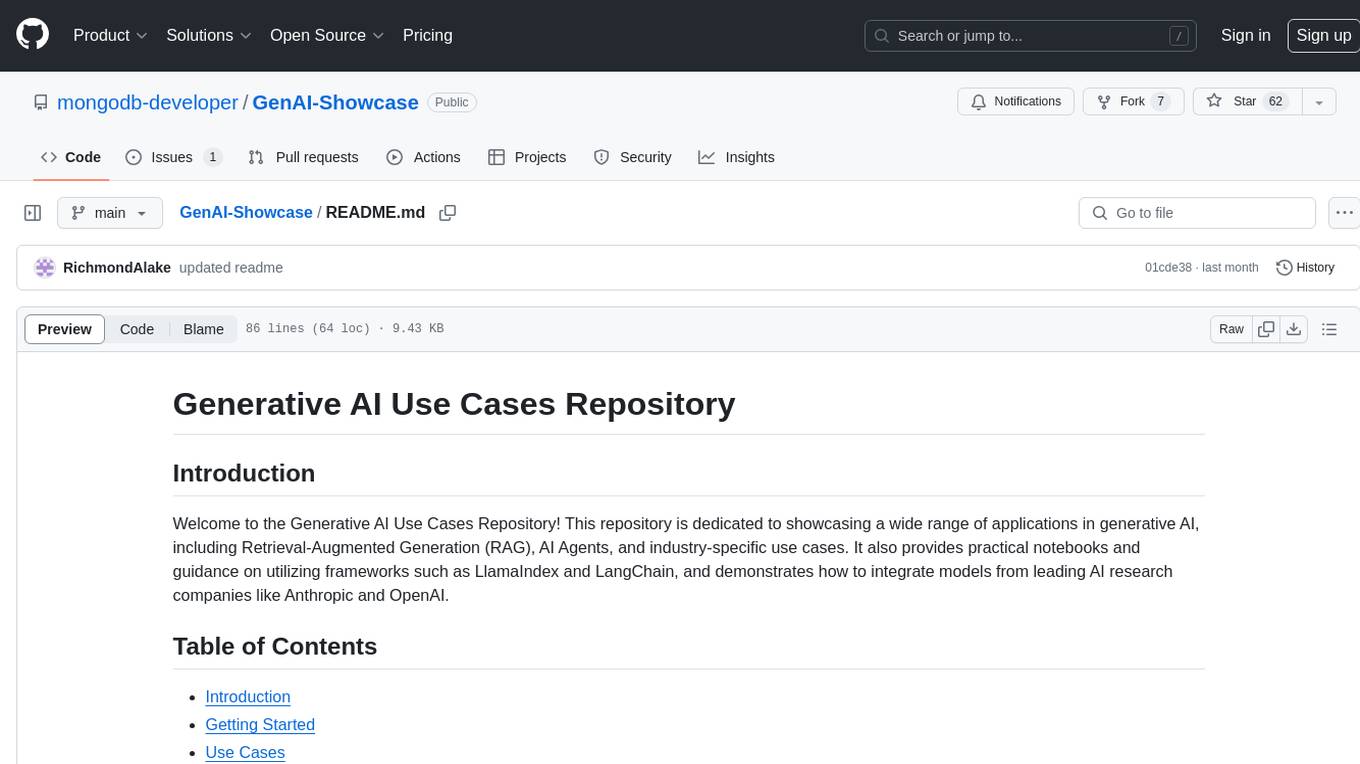
GenAI-Showcase
The Generative AI Use Cases Repository showcases a wide range of applications in generative AI, including Retrieval-Augmented Generation (RAG), AI Agents, and industry-specific use cases. It provides practical notebooks and guidance on utilizing frameworks such as LlamaIndex and LangChain, and demonstrates how to integrate models from leading AI research companies like Anthropic and OpenAI.
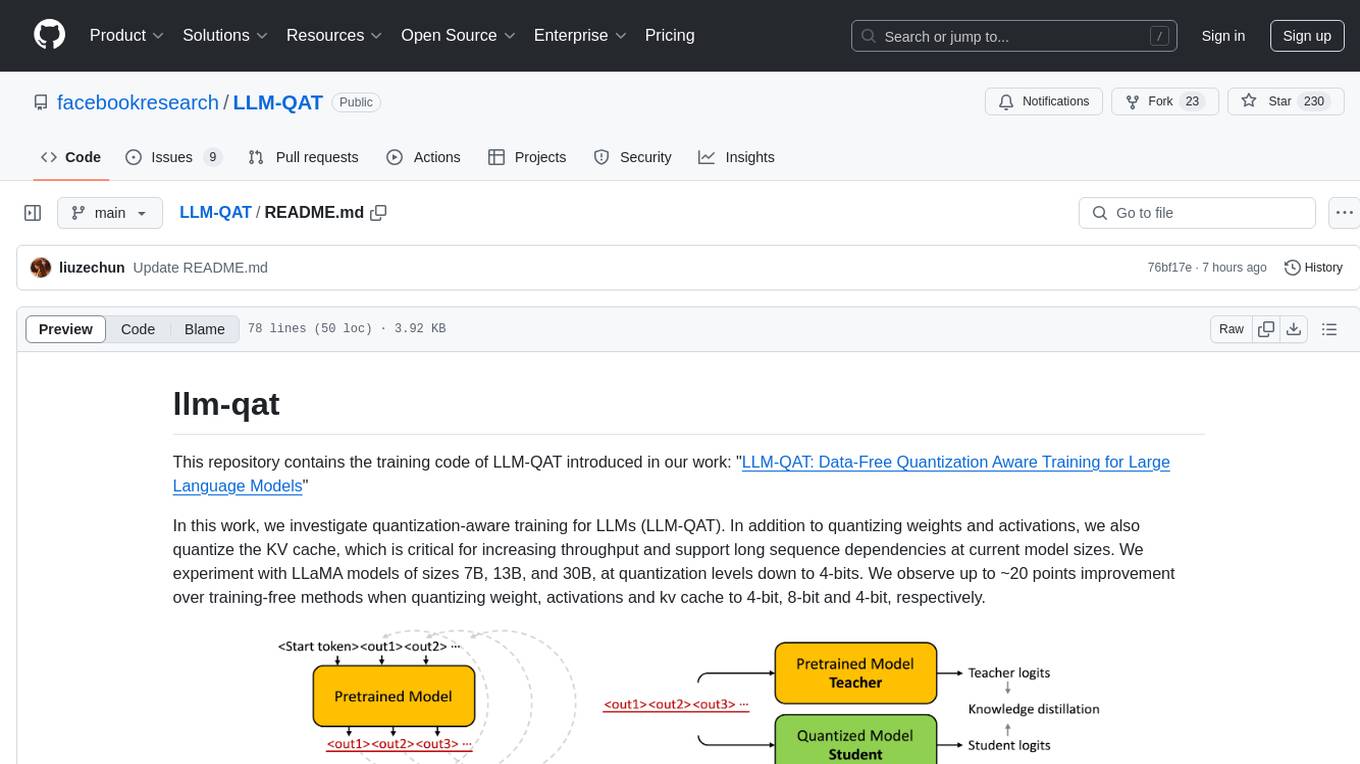
LLM-QAT
This repository contains the training code of LLM-QAT for large language models. The work investigates quantization-aware training for LLMs, including quantizing weights, activations, and the KV cache. Experiments were conducted on LLaMA models of sizes 7B, 13B, and 30B, at quantization levels down to 4-bits. Significant improvements were observed when quantizing weight, activations, and kv cache to 4-bit, 8-bit, and 4-bit, respectively.
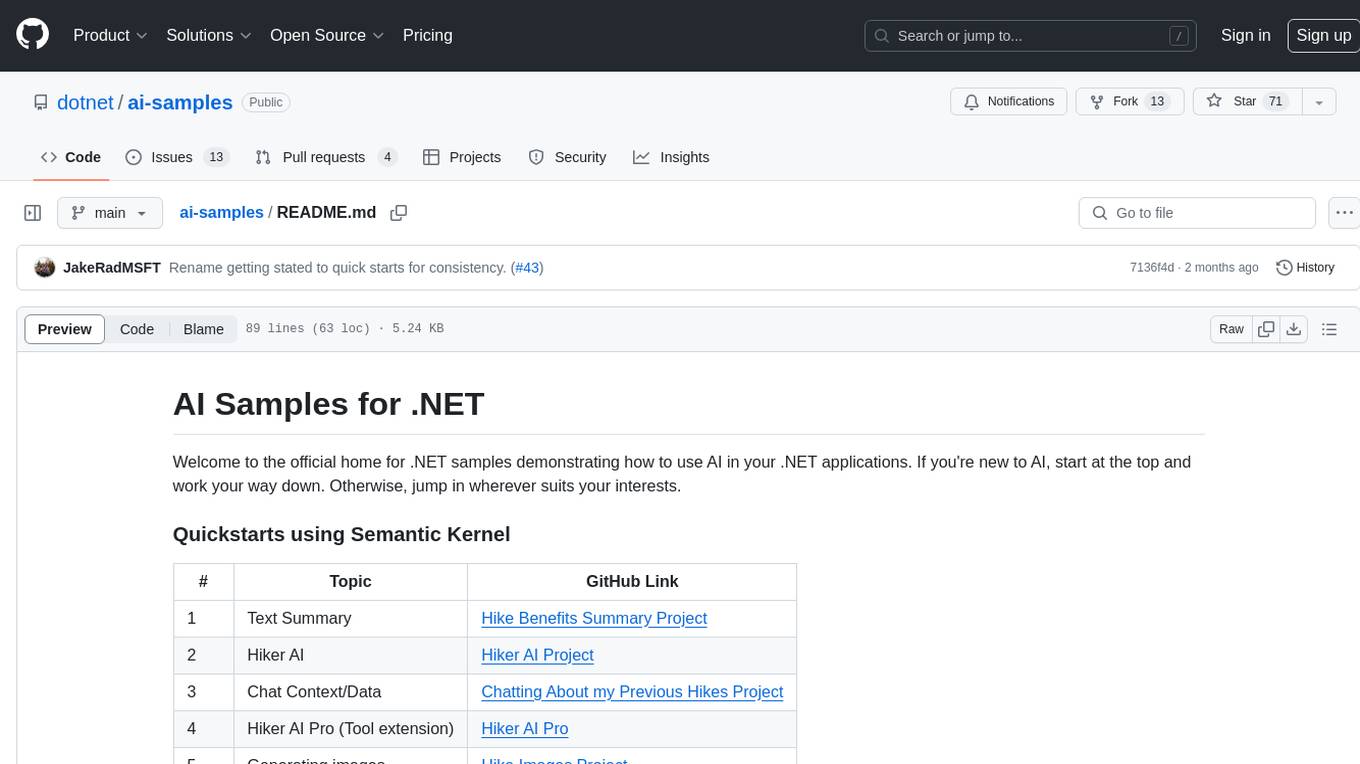
ai-samples
AI Samples for .NET is a repository containing various samples demonstrating how to use AI in .NET applications. It provides quickstarts using Semantic Kernel and Azure OpenAI SDK, covers LLM Core Concepts, End to End Examples, Local Models, Local Embedding Models, Tokenizers, Vector Databases, and Reference Examples. The repository showcases different AI-related projects and tools for developers to explore and learn from.
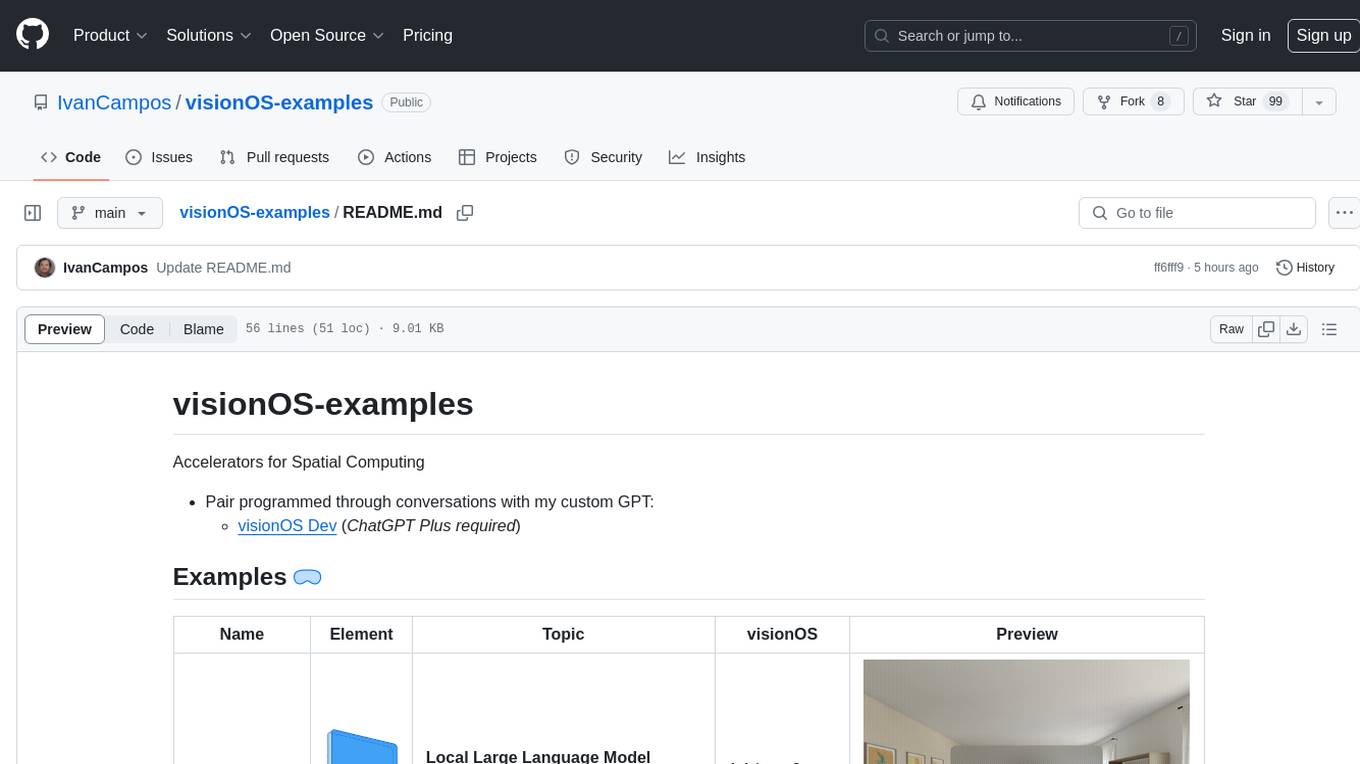
visionOS-examples
visionOS-examples is a repository containing accelerators for Spatial Computing. It includes examples such as Local Large Language Model, Chat Apple Vision Pro, WebSockets, Anchor To Head, Hand Tracking, Battery Life, Countdown, Plane Detection, Timer Vision, and PencilKit for visionOS. The repository showcases various functionalities and features for Apple Vision Pro, offering tools for developers to enhance their visionOS apps with capabilities like hand tracking, plane detection, and real-time cryptocurrency prices.
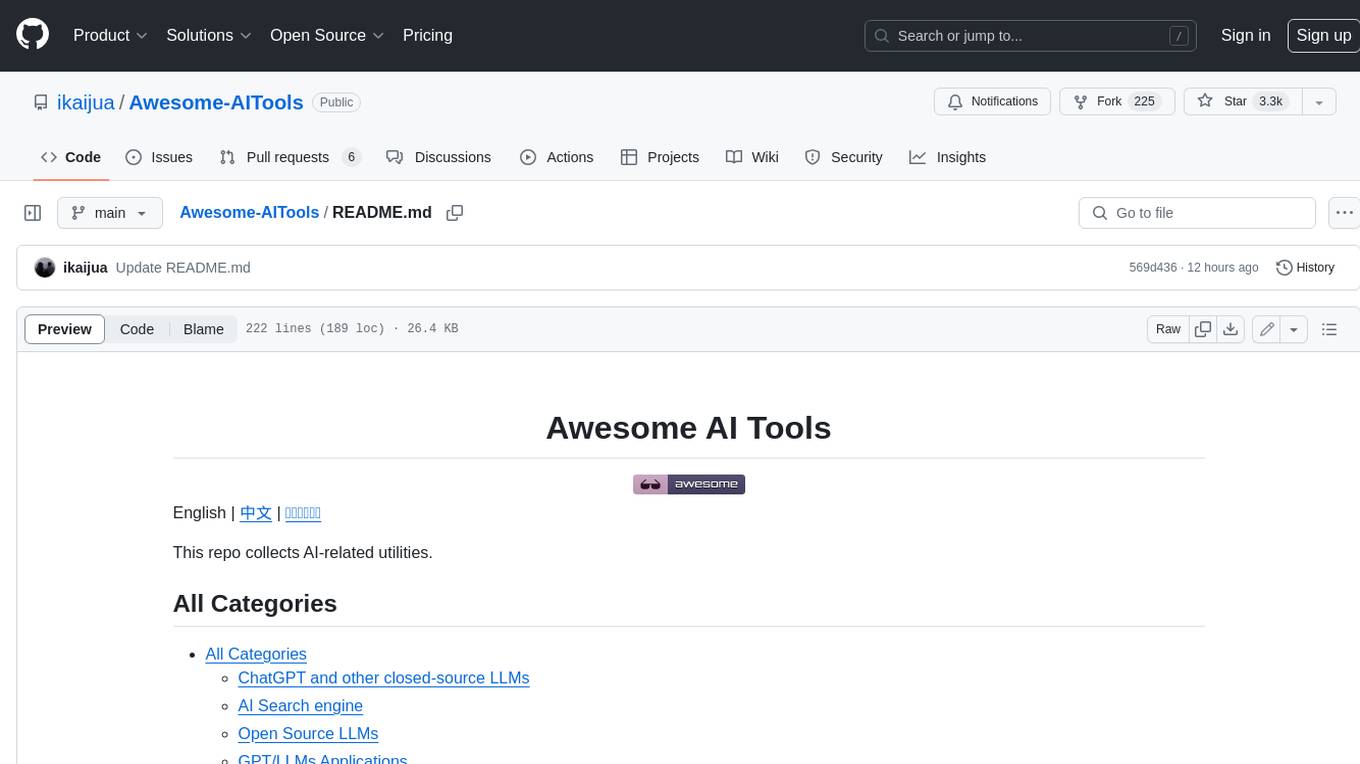
Awesome-AITools
This repo collects AI-related utilities. ## All Categories * All Categories * ChatGPT and other closed-source LLMs * AI Search engine * Open Source LLMs * GPT/LLMs Applications * LLM training platform * Applications that integrate multiple LLMs * AI Agent * Writing * Programming Development * Translation * AI Conversation or AI Voice Conversation * Image Creation * Speech Recognition * Text To Speech * Voice Processing * AI generated music or sound effects * Speech translation * Video Creation * Video Content Summary * OCR(Optical Character Recognition)
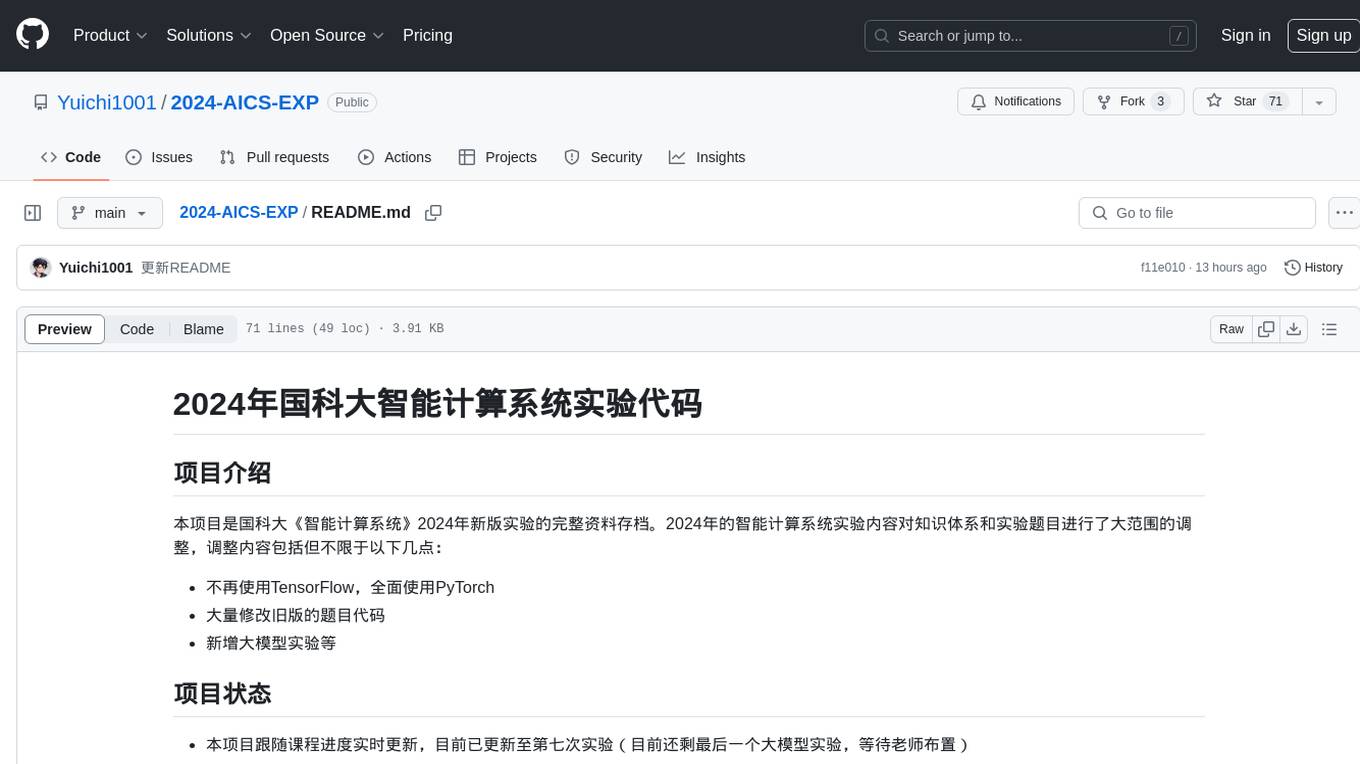
2024-AICS-EXP
This repository contains the complete archive of the 2024 version of the 'Intelligent Computing System' experiment at the University of Chinese Academy of Sciences. The experiment content for 2024 has undergone extensive adjustments to the knowledge system and experimental topics, including the transition from TensorFlow to PyTorch, significant modifications to previous code, and the addition of experiments with large models. The project is continuously updated in line with the course progress, currently up to the seventh experiment. Updates include the addition of experiments like YOLOv5 in Experiment 5-3, updates to theoretical teaching materials, and fixes for bugs in Experiment 6 code. The repository also includes experiment manuals, questions, and answers for various experiments, with some data sets hosted on Baidu Cloud due to size limitations on GitHub.
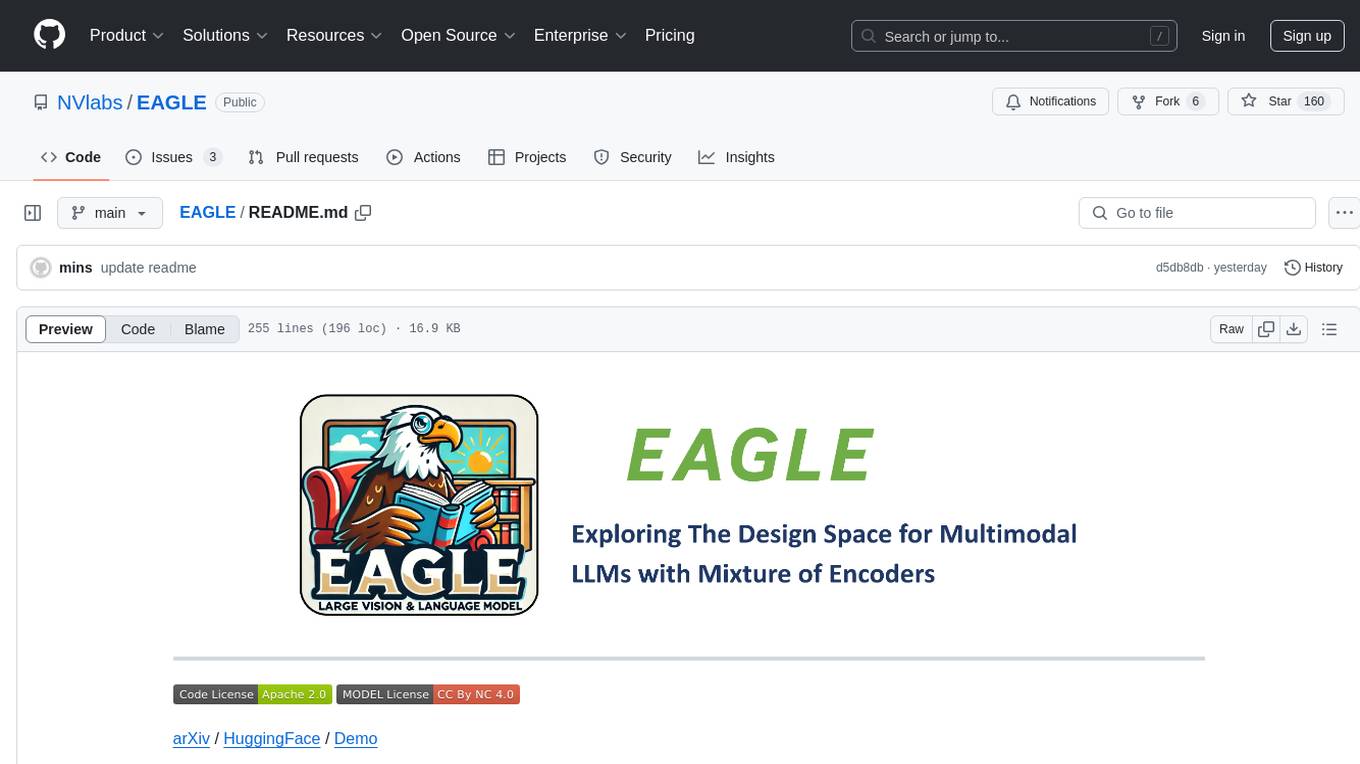
EAGLE
Eagle is a family of Vision-Centric High-Resolution Multimodal LLMs that enhance multimodal LLM perception using a mix of vision encoders and various input resolutions. The model features a channel-concatenation-based fusion for vision experts with different architectures and knowledge, supporting up to over 1K input resolution. It excels in resolution-sensitive tasks like optical character recognition and document understanding.
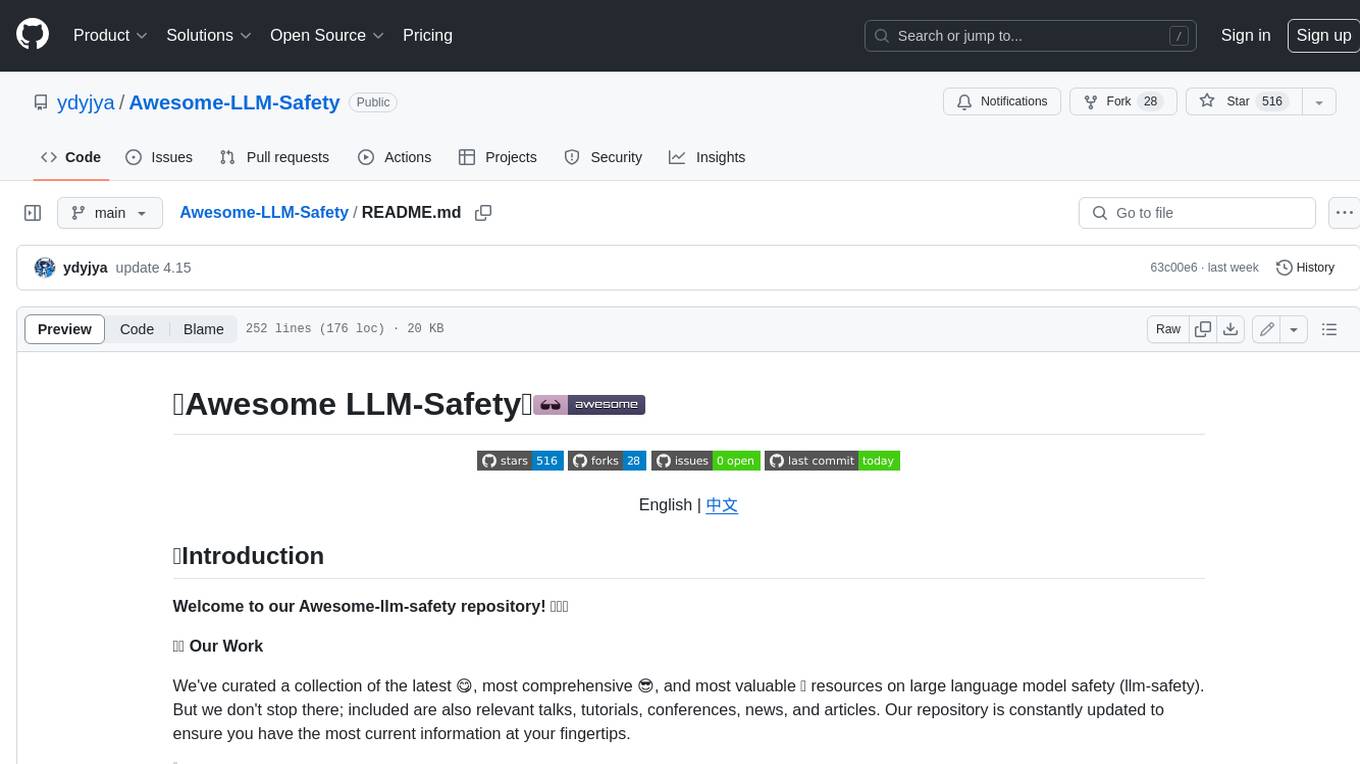
Awesome-LLM-Safety
Welcome to our Awesome-llm-safety repository! We've curated a collection of the latest, most comprehensive, and most valuable resources on large language model safety (llm-safety). But we don't stop there; included are also relevant talks, tutorials, conferences, news, and articles. Our repository is constantly updated to ensure you have the most current information at your fingertips.
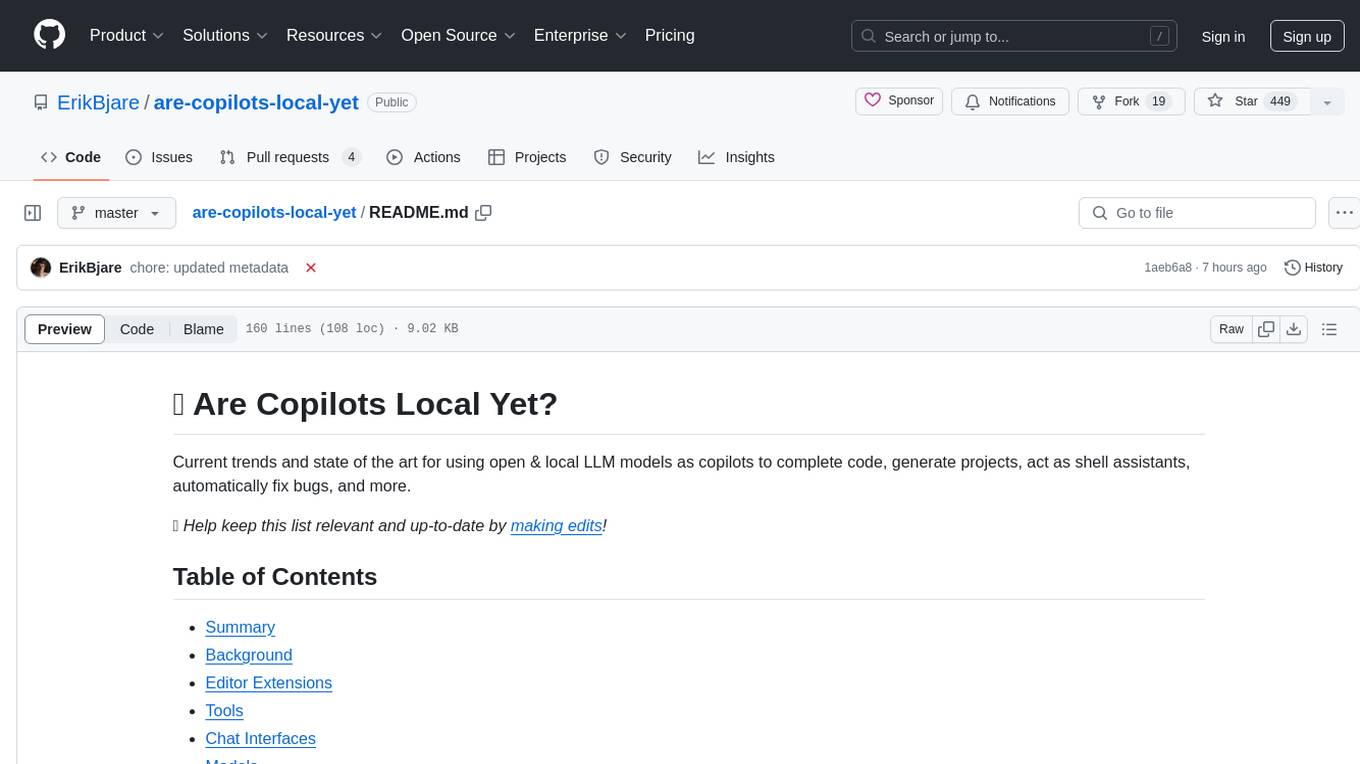
are-copilots-local-yet
Current trends and state of the art for using open & local LLM models as copilots to complete code, generate projects, act as shell assistants, automatically fix bugs, and more. This document is a curated list of local Copilots, shell assistants, and related projects, intended to be a resource for those interested in a survey of the existing tools and to help developers discover the state of the art for projects like these.
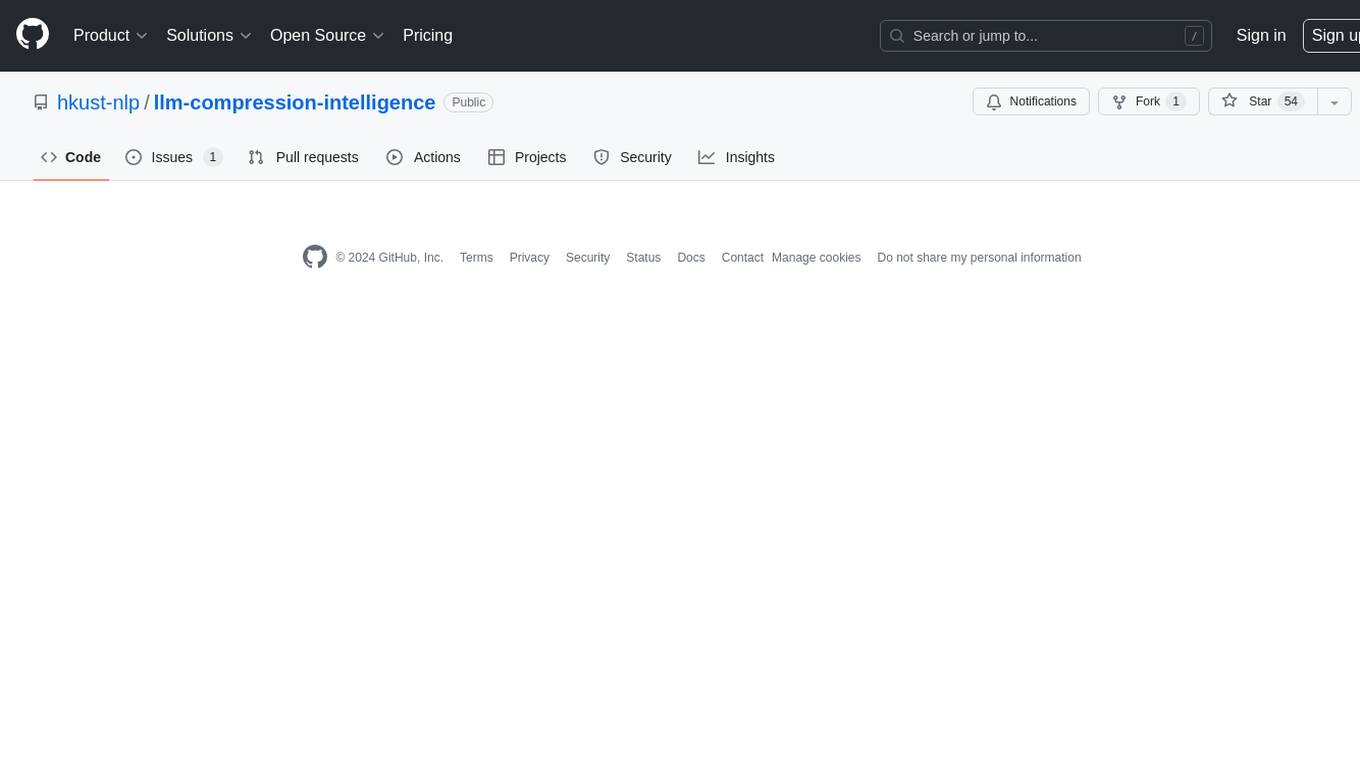
llm-compression-intelligence
This repository presents the findings of the paper "Compression Represents Intelligence Linearly". The study reveals a strong linear correlation between the intelligence of LLMs, as measured by benchmark scores, and their ability to compress external text corpora. Compression efficiency, derived from raw text corpora, serves as a reliable evaluation metric that is linearly associated with model capabilities. The repository includes the compression corpora used in the paper, code for computing compression efficiency, and data collection and processing pipelines.
For similar tasks
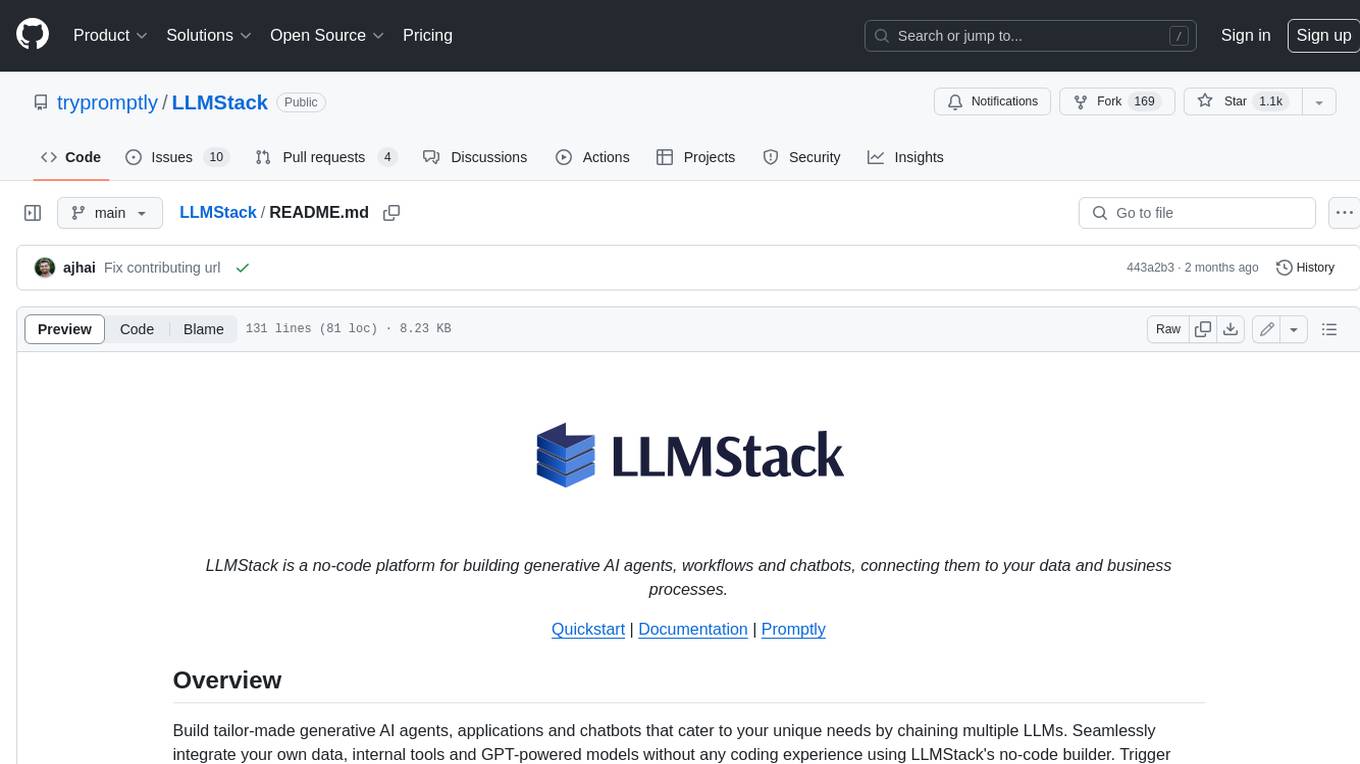
LLMStack
LLMStack is a no-code platform for building generative AI agents, workflows, and chatbots. It allows users to connect their own data, internal tools, and GPT-powered models without any coding experience. LLMStack can be deployed to the cloud or on-premise and can be accessed via HTTP API or triggered from Slack or Discord.
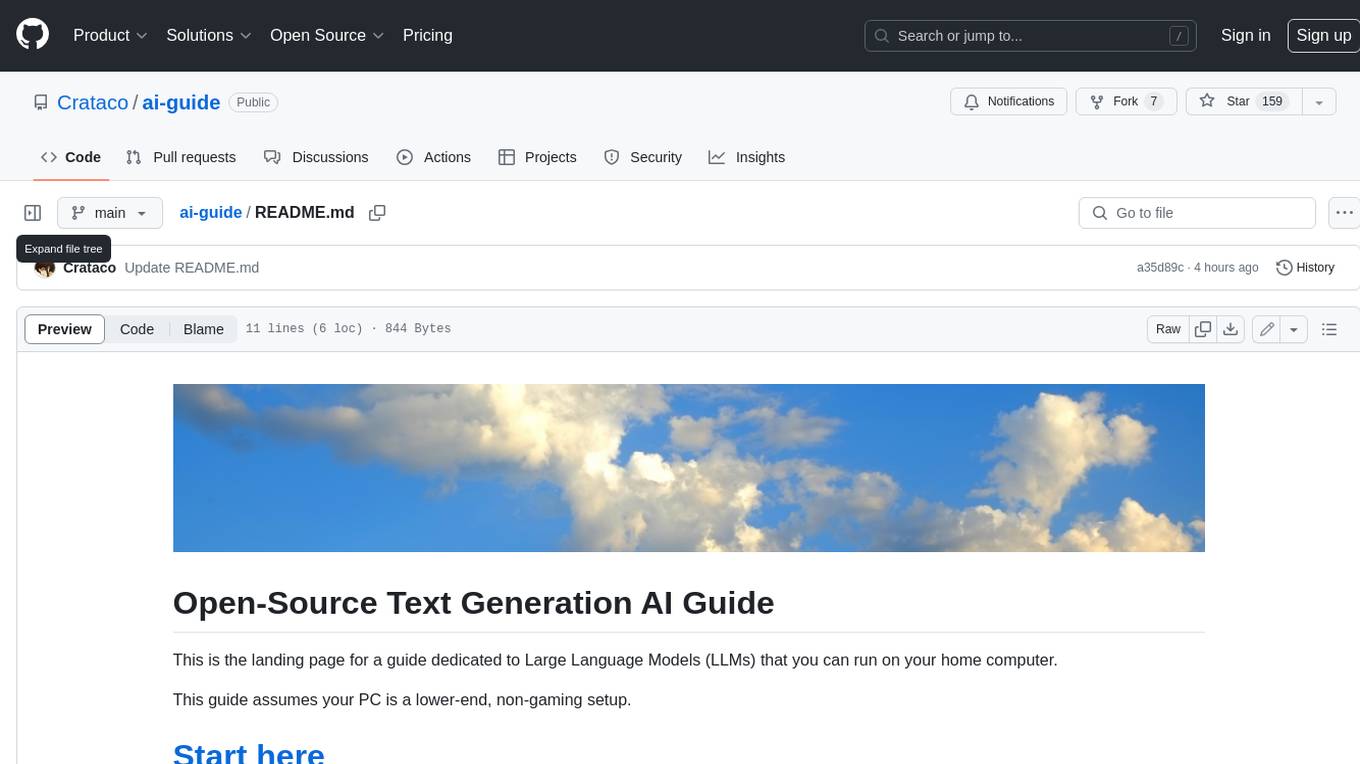
ai-guide
This guide is dedicated to Large Language Models (LLMs) that you can run on your home computer. It assumes your PC is a lower-end, non-gaming setup.
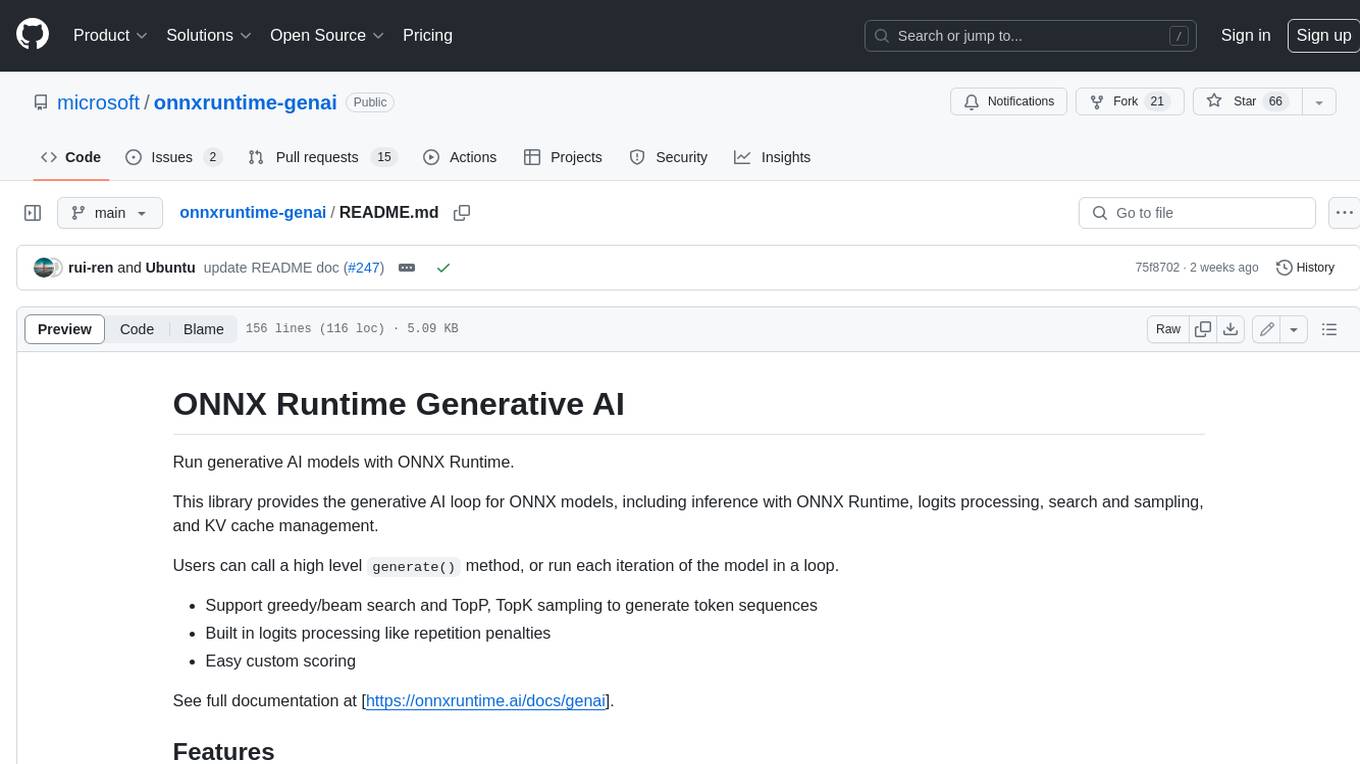
onnxruntime-genai
ONNX Runtime Generative AI is a library that provides the generative AI loop for ONNX models, including inference with ONNX Runtime, logits processing, search and sampling, and KV cache management. Users can call a high level `generate()` method, or run each iteration of the model in a loop. It supports greedy/beam search and TopP, TopK sampling to generate token sequences, has built in logits processing like repetition penalties, and allows for easy custom scoring.
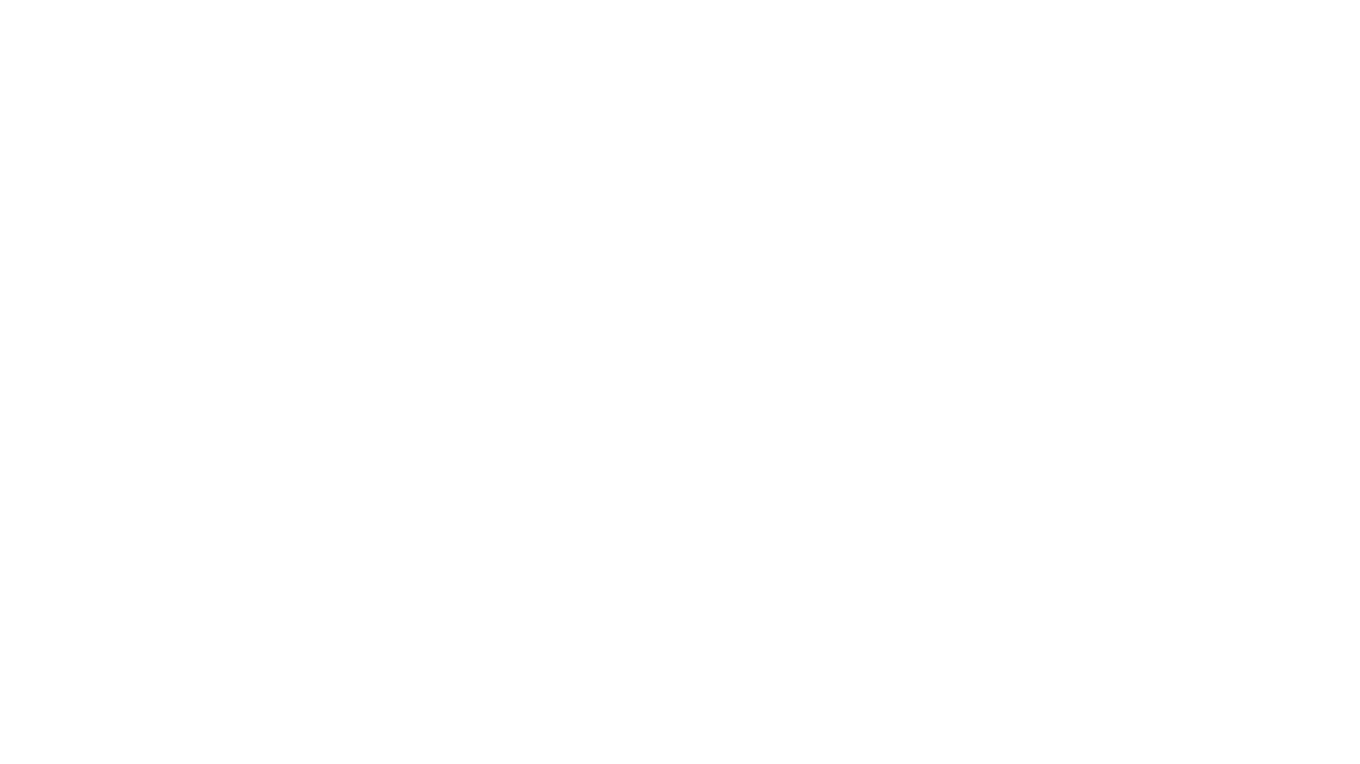
jupyter-ai
Jupyter AI connects generative AI with Jupyter notebooks. It provides a user-friendly and powerful way to explore generative AI models in notebooks and improve your productivity in JupyterLab and the Jupyter Notebook. Specifically, Jupyter AI offers: * An `%%ai` magic that turns the Jupyter notebook into a reproducible generative AI playground. This works anywhere the IPython kernel runs (JupyterLab, Jupyter Notebook, Google Colab, Kaggle, VSCode, etc.). * A native chat UI in JupyterLab that enables you to work with generative AI as a conversational assistant. * Support for a wide range of generative model providers, including AI21, Anthropic, AWS, Cohere, Gemini, Hugging Face, NVIDIA, and OpenAI. * Local model support through GPT4All, enabling use of generative AI models on consumer grade machines with ease and privacy.
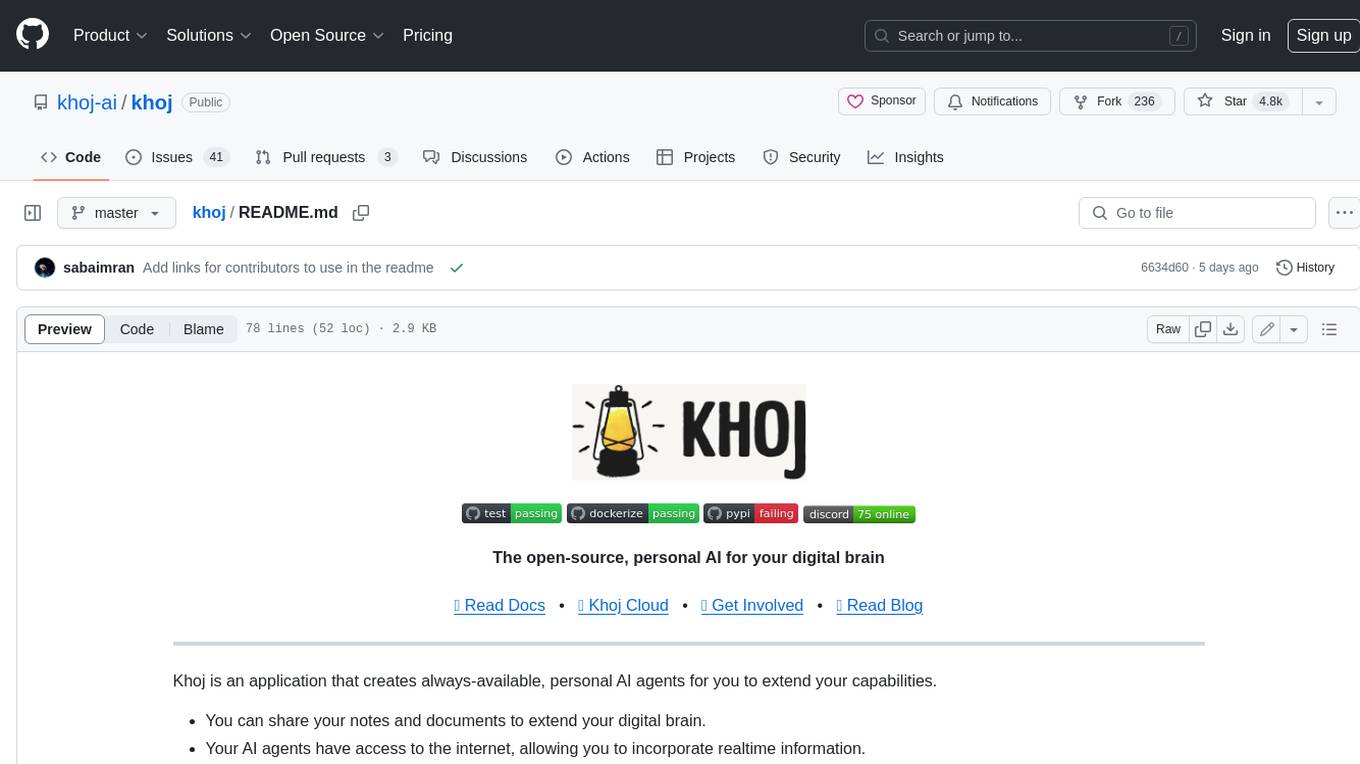
khoj
Khoj is an open-source, personal AI assistant that extends your capabilities by creating always-available AI agents. You can share your notes and documents to extend your digital brain, and your AI agents have access to the internet, allowing you to incorporate real-time information. Khoj is accessible on Desktop, Emacs, Obsidian, Web, and Whatsapp, and you can share PDF, markdown, org-mode, notion files, and GitHub repositories. You'll get fast, accurate semantic search on top of your docs, and your agents can create deeply personal images and understand your speech. Khoj is self-hostable and always will be.
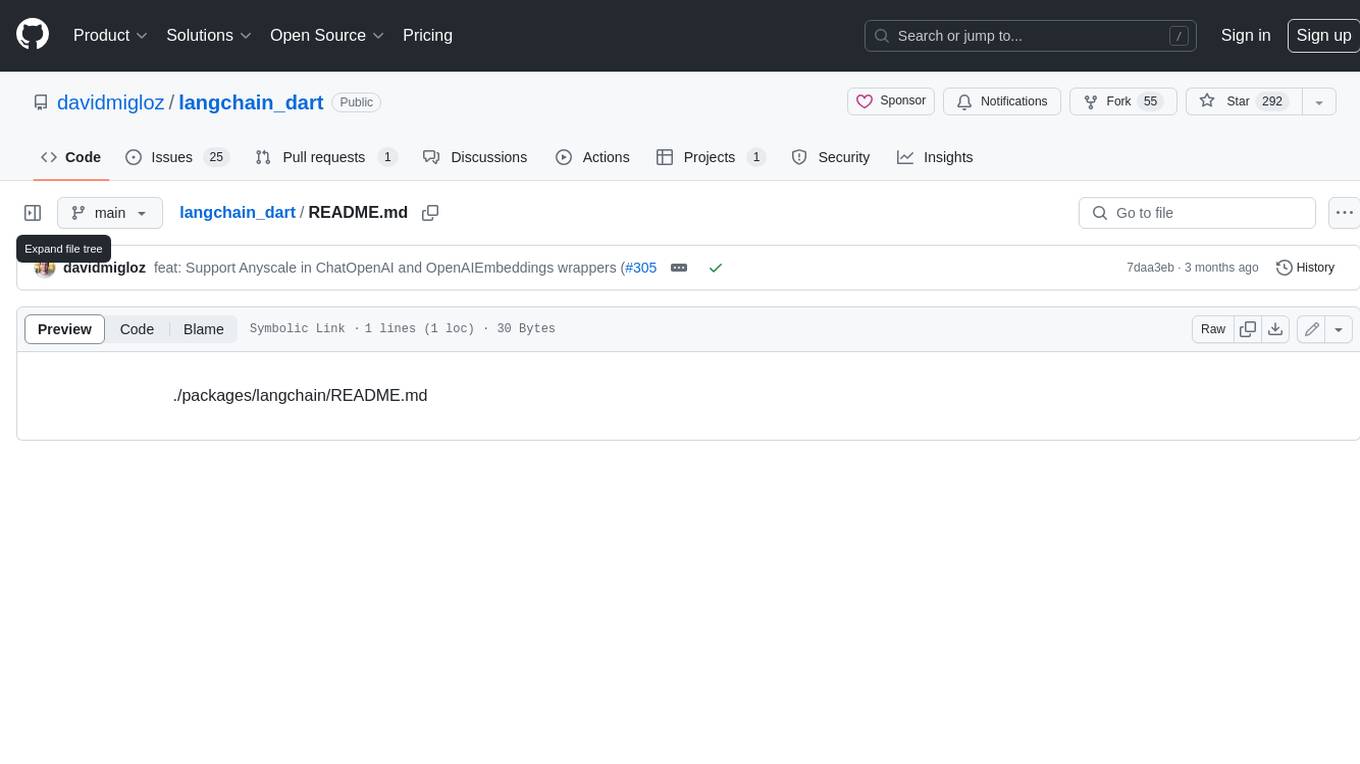
langchain_dart
LangChain.dart is a Dart port of the popular LangChain Python framework created by Harrison Chase. LangChain provides a set of ready-to-use components for working with language models and a standard interface for chaining them together to formulate more advanced use cases (e.g. chatbots, Q&A with RAG, agents, summarization, extraction, etc.). The components can be grouped into a few core modules: * **Model I/O:** LangChain offers a unified API for interacting with various LLM providers (e.g. OpenAI, Google, Mistral, Ollama, etc.), allowing developers to switch between them with ease. Additionally, it provides tools for managing model inputs (prompt templates and example selectors) and parsing the resulting model outputs (output parsers). * **Retrieval:** assists in loading user data (via document loaders), transforming it (with text splitters), extracting its meaning (using embedding models), storing (in vector stores) and retrieving it (through retrievers) so that it can be used to ground the model's responses (i.e. Retrieval-Augmented Generation or RAG). * **Agents:** "bots" that leverage LLMs to make informed decisions about which available tools (such as web search, calculators, database lookup, etc.) to use to accomplish the designated task. The different components can be composed together using the LangChain Expression Language (LCEL).
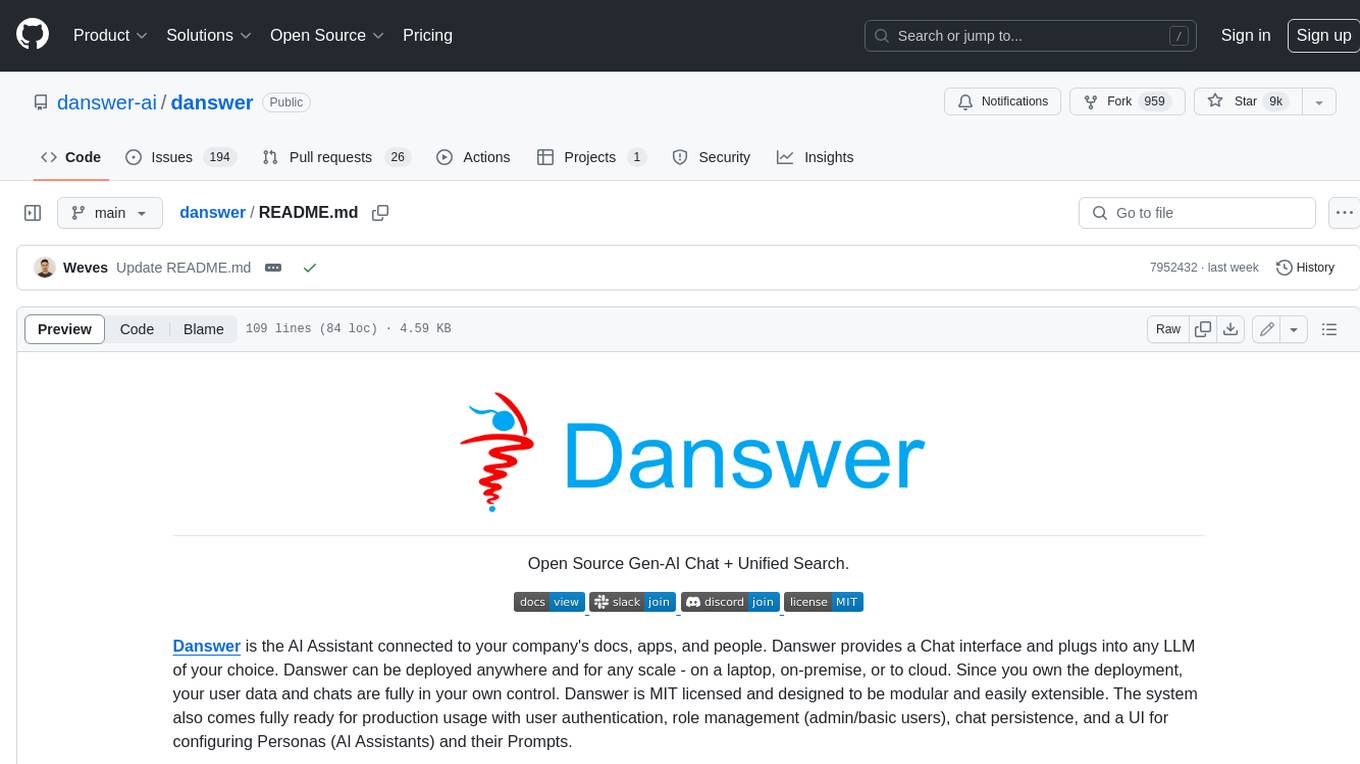
danswer
Danswer is an open-source Gen-AI Chat and Unified Search tool that connects to your company's docs, apps, and people. It provides a Chat interface and plugs into any LLM of your choice. Danswer can be deployed anywhere and for any scale - on a laptop, on-premise, or to cloud. Since you own the deployment, your user data and chats are fully in your own control. Danswer is MIT licensed and designed to be modular and easily extensible. The system also comes fully ready for production usage with user authentication, role management (admin/basic users), chat persistence, and a UI for configuring Personas (AI Assistants) and their Prompts. Danswer also serves as a Unified Search across all common workplace tools such as Slack, Google Drive, Confluence, etc. By combining LLMs and team specific knowledge, Danswer becomes a subject matter expert for the team. Imagine ChatGPT if it had access to your team's unique knowledge! It enables questions such as "A customer wants feature X, is this already supported?" or "Where's the pull request for feature Y?"
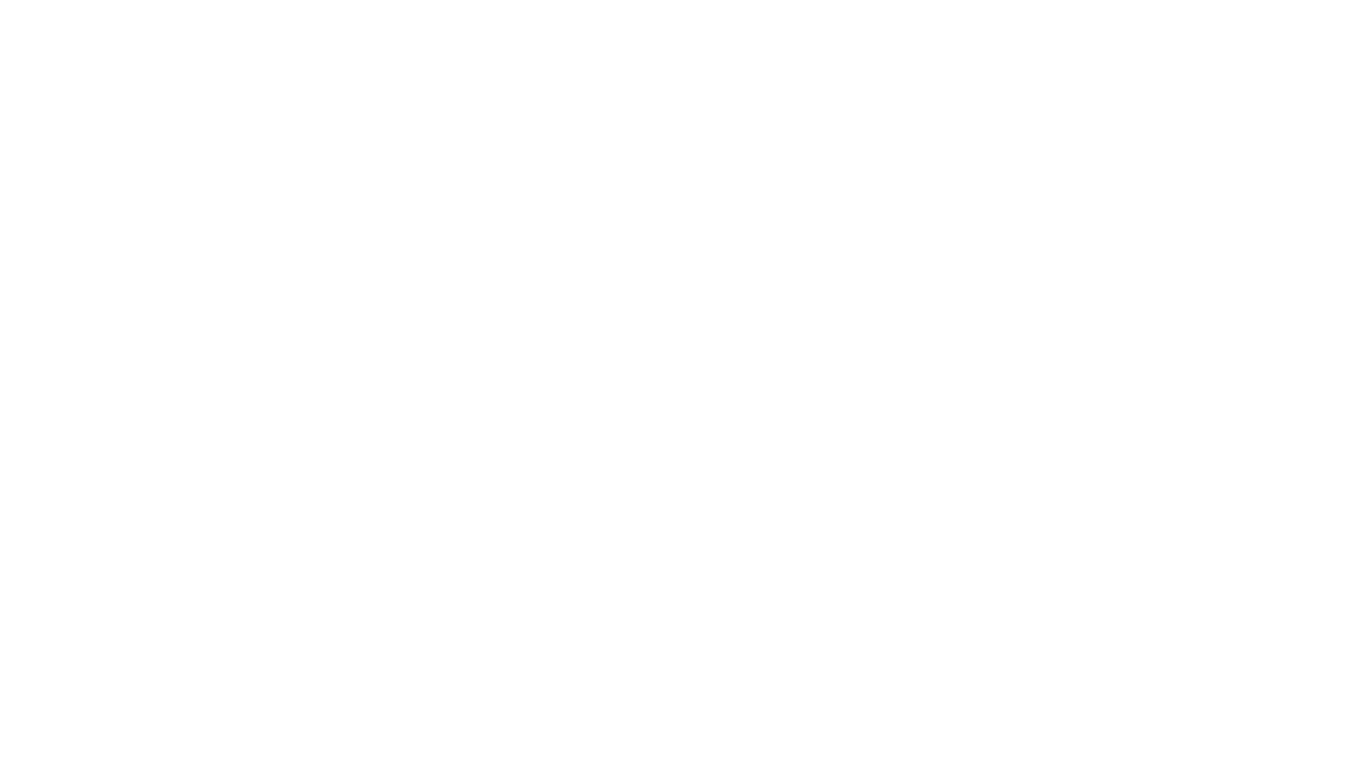
infinity
Infinity is an AI-native database designed for LLM applications, providing incredibly fast full-text and vector search capabilities. It supports a wide range of data types, including vectors, full-text, and structured data, and offers a fused search feature that combines multiple embeddings and full text. Infinity is easy to use, with an intuitive Python API and a single-binary architecture that simplifies deployment. It achieves high performance, with 0.1 milliseconds query latency on million-scale vector datasets and up to 15K QPS.
For similar jobs
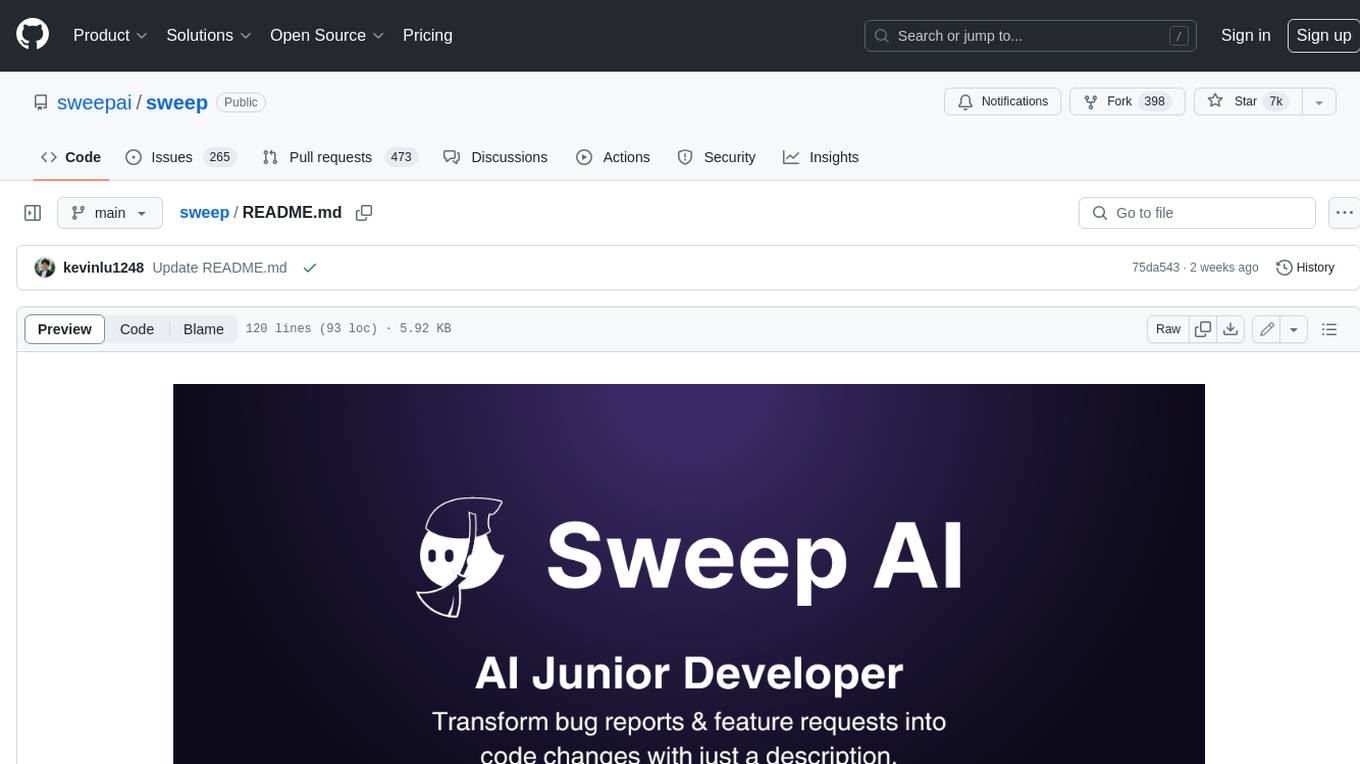
sweep
Sweep is an AI junior developer that turns bugs and feature requests into code changes. It automatically handles developer experience improvements like adding type hints and improving test coverage.
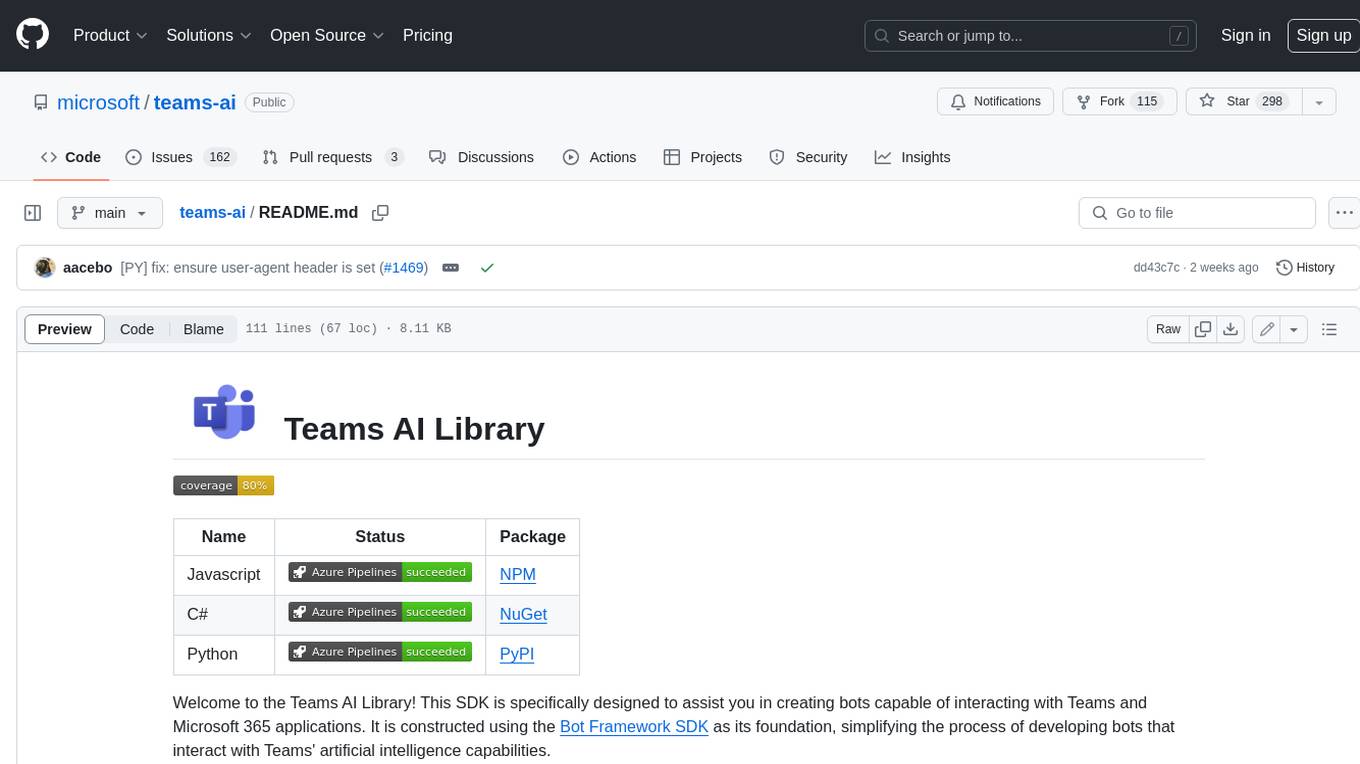
teams-ai
The Teams AI Library is a software development kit (SDK) that helps developers create bots that can interact with Teams and Microsoft 365 applications. It is built on top of the Bot Framework SDK and simplifies the process of developing bots that interact with Teams' artificial intelligence capabilities. The SDK is available for JavaScript/TypeScript, .NET, and Python.
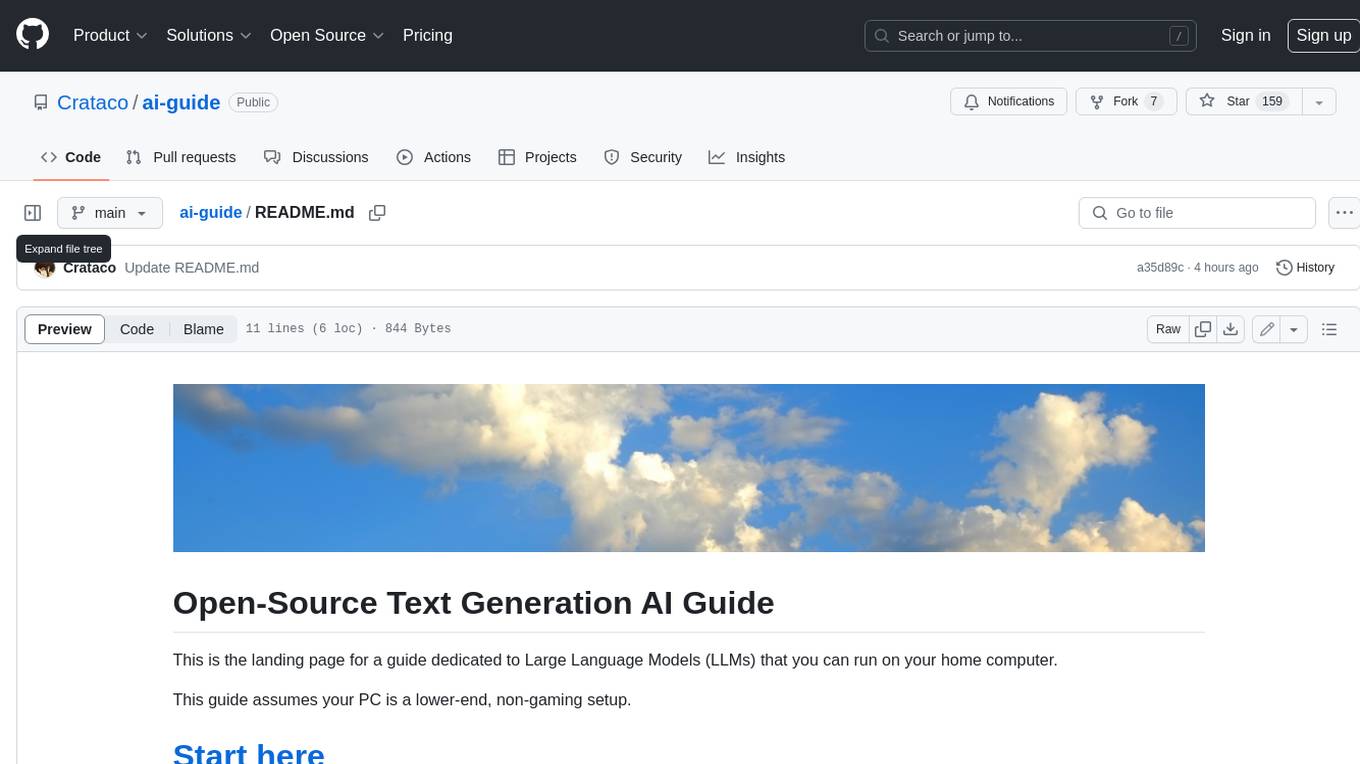
ai-guide
This guide is dedicated to Large Language Models (LLMs) that you can run on your home computer. It assumes your PC is a lower-end, non-gaming setup.
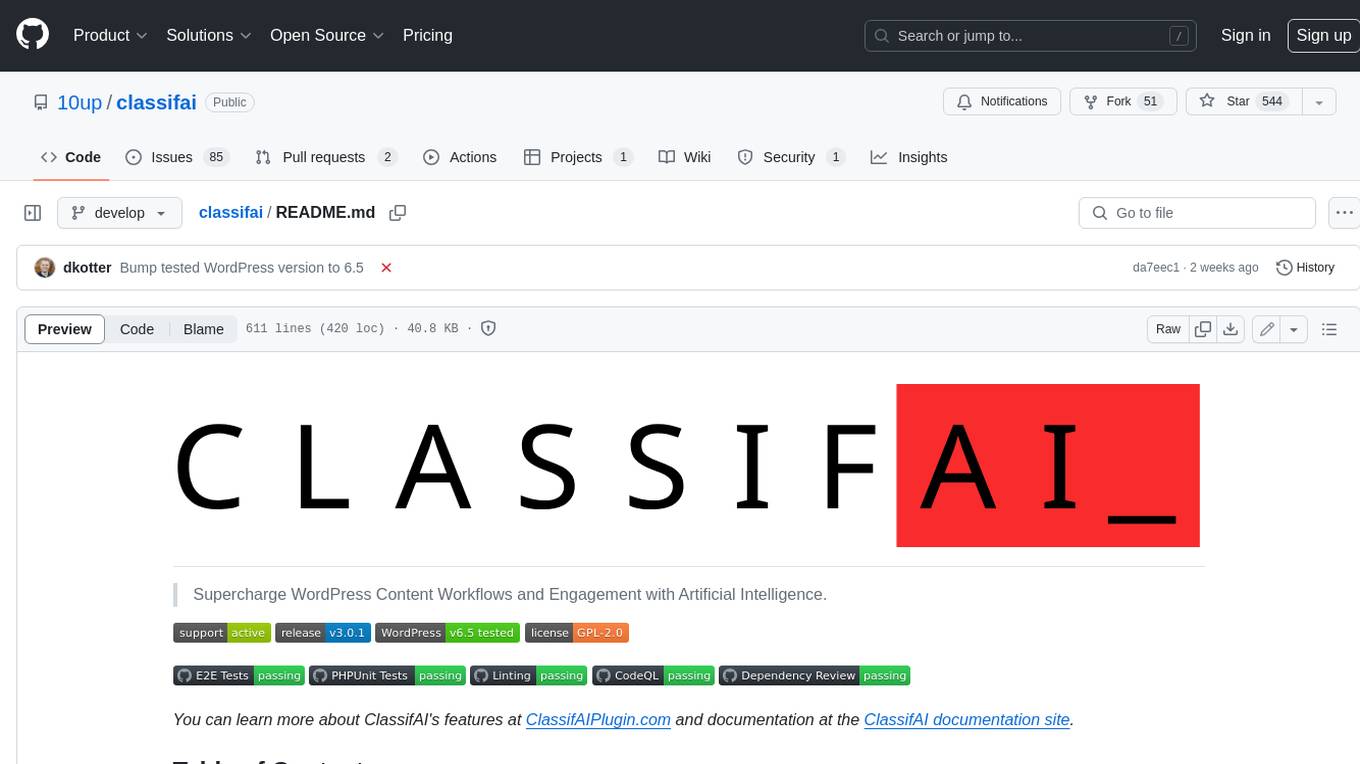
classifai
Supercharge WordPress Content Workflows and Engagement with Artificial Intelligence. Tap into leading cloud-based services like OpenAI, Microsoft Azure AI, Google Gemini and IBM Watson to augment your WordPress-powered websites. Publish content faster while improving SEO performance and increasing audience engagement. ClassifAI integrates Artificial Intelligence and Machine Learning technologies to lighten your workload and eliminate tedious tasks, giving you more time to create original content that matters.
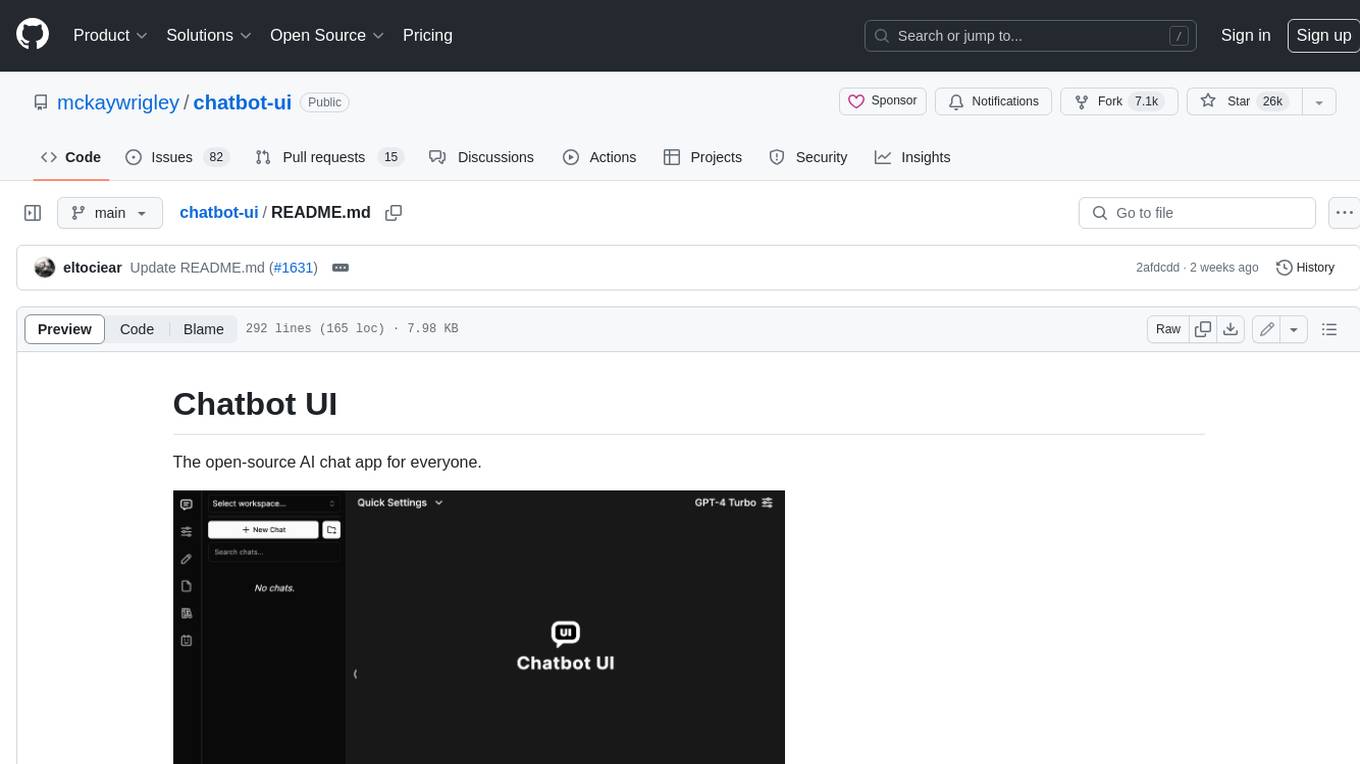
chatbot-ui
Chatbot UI is an open-source AI chat app that allows users to create and deploy their own AI chatbots. It is easy to use and can be customized to fit any need. Chatbot UI is perfect for businesses, developers, and anyone who wants to create a chatbot.
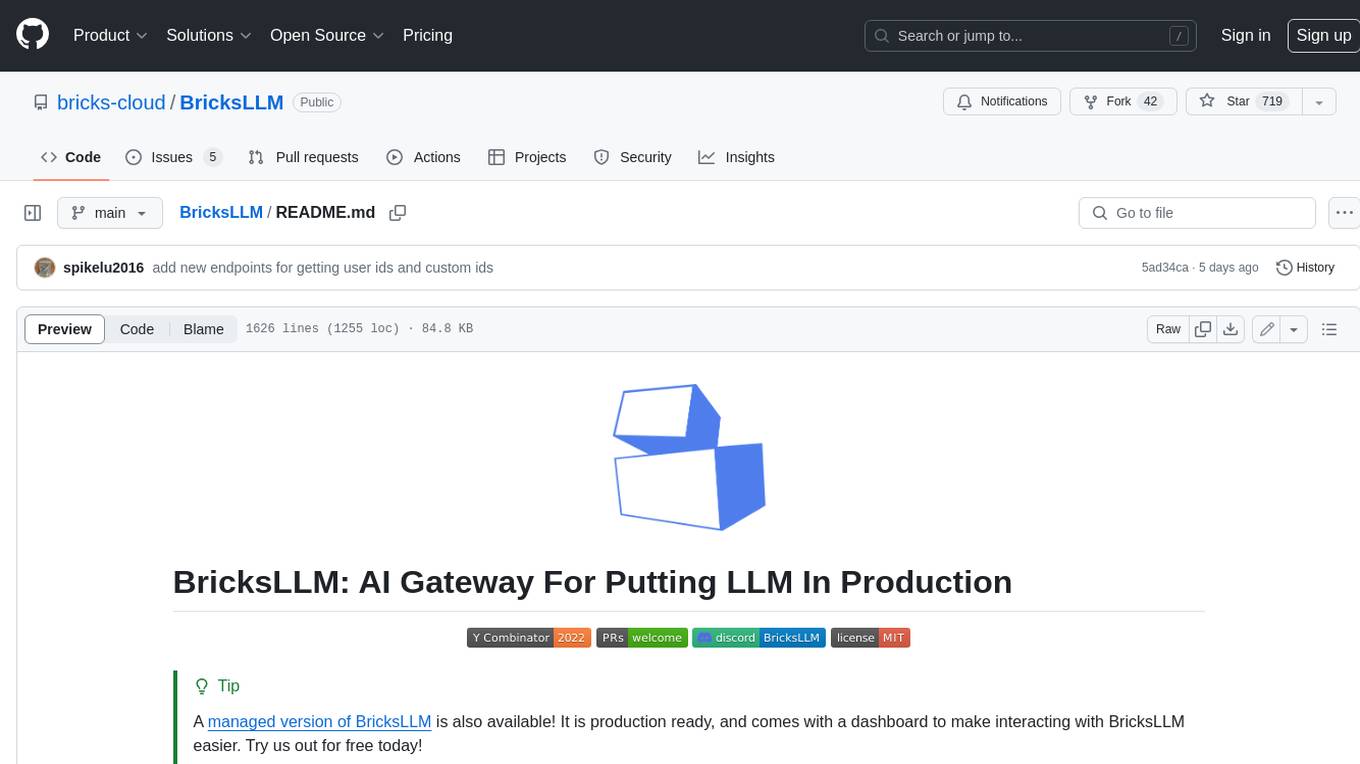
BricksLLM
BricksLLM is a cloud native AI gateway written in Go. Currently, it provides native support for OpenAI, Anthropic, Azure OpenAI and vLLM. BricksLLM aims to provide enterprise level infrastructure that can power any LLM production use cases. Here are some use cases for BricksLLM: * Set LLM usage limits for users on different pricing tiers * Track LLM usage on a per user and per organization basis * Block or redact requests containing PIIs * Improve LLM reliability with failovers, retries and caching * Distribute API keys with rate limits and cost limits for internal development/production use cases * Distribute API keys with rate limits and cost limits for students
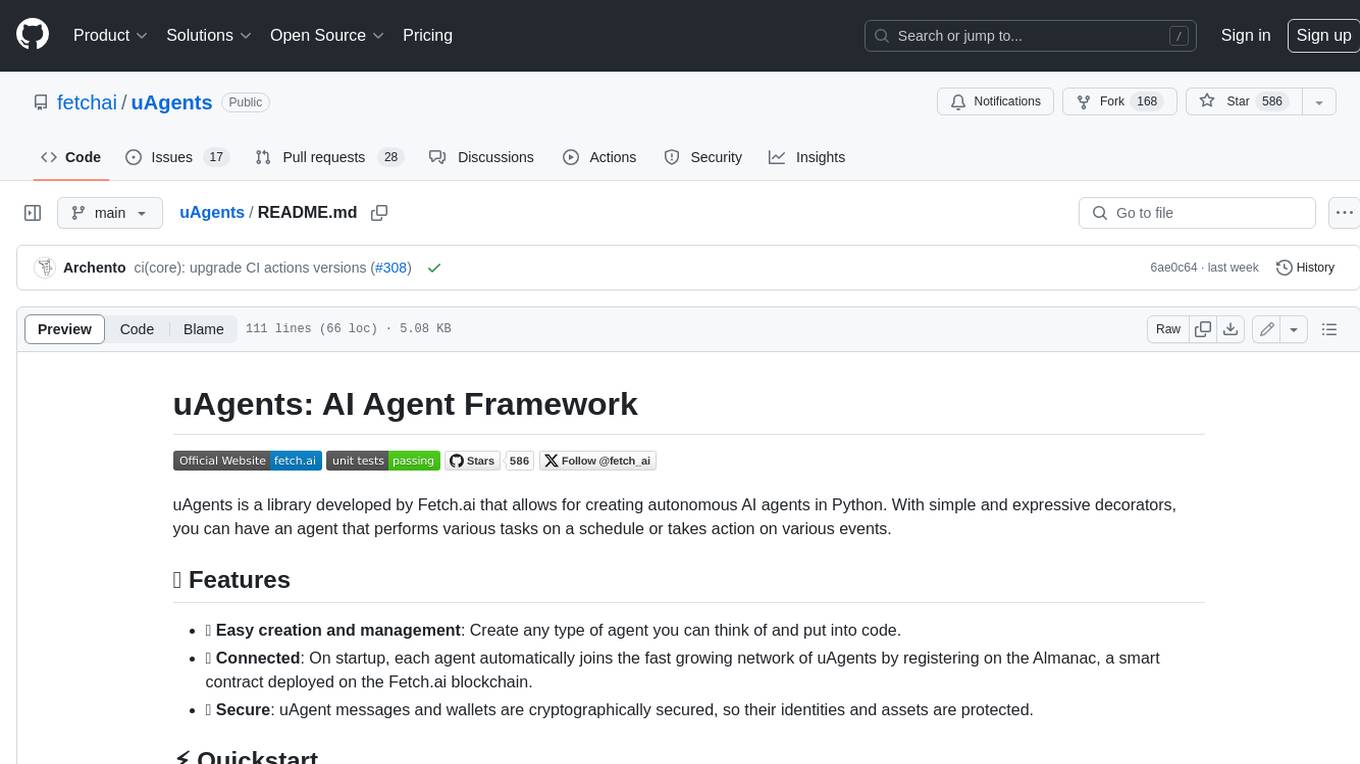
uAgents
uAgents is a Python library developed by Fetch.ai that allows for the creation of autonomous AI agents. These agents can perform various tasks on a schedule or take action on various events. uAgents are easy to create and manage, and they are connected to a fast-growing network of other uAgents. They are also secure, with cryptographically secured messages and wallets.
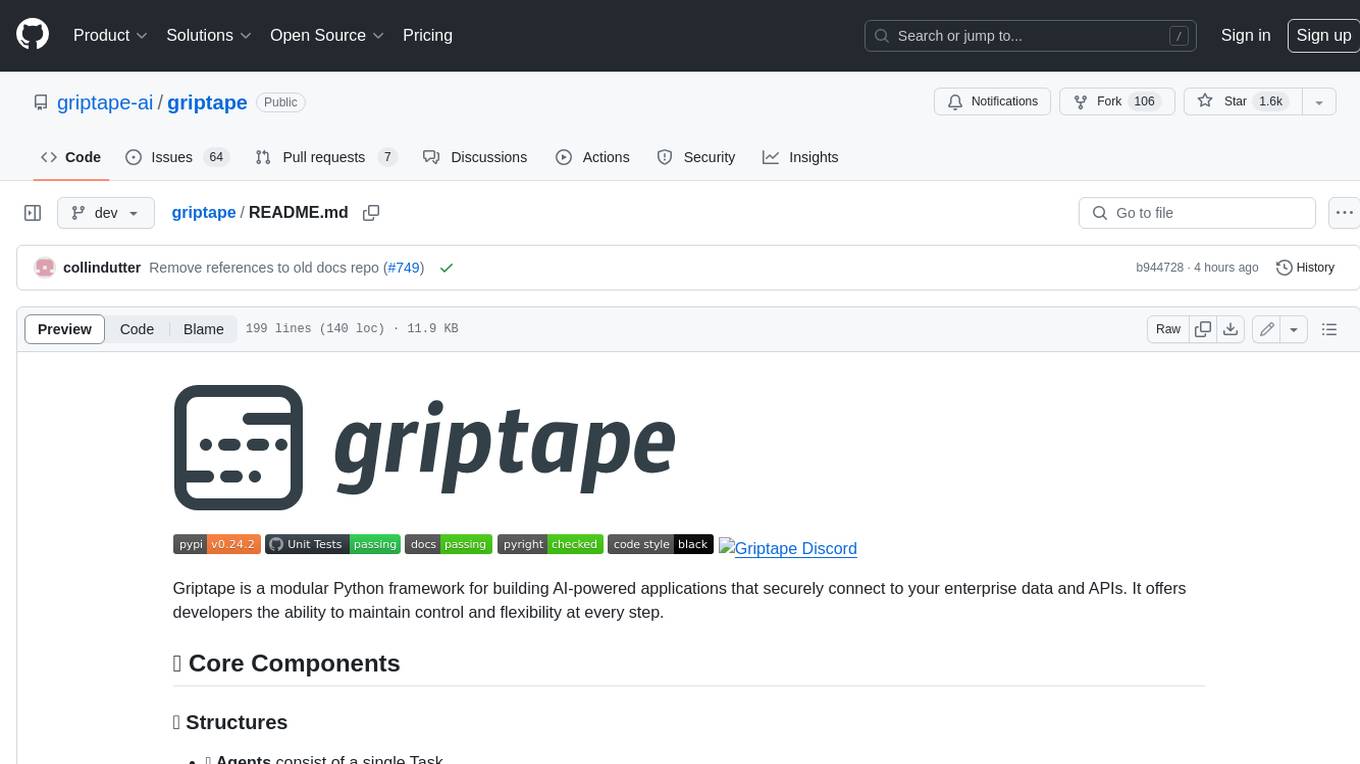
griptape
Griptape is a modular Python framework for building AI-powered applications that securely connect to your enterprise data and APIs. It offers developers the ability to maintain control and flexibility at every step. Griptape's core components include Structures (Agents, Pipelines, and Workflows), Tasks, Tools, Memory (Conversation Memory, Task Memory, and Meta Memory), Drivers (Prompt and Embedding Drivers, Vector Store Drivers, Image Generation Drivers, Image Query Drivers, SQL Drivers, Web Scraper Drivers, and Conversation Memory Drivers), Engines (Query Engines, Extraction Engines, Summary Engines, Image Generation Engines, and Image Query Engines), and additional components (Rulesets, Loaders, Artifacts, Chunkers, and Tokenizers). Griptape enables developers to create AI-powered applications with ease and efficiency.