Best AI tools for< Understand Model Mechanisms >
20 - AI tool Sites
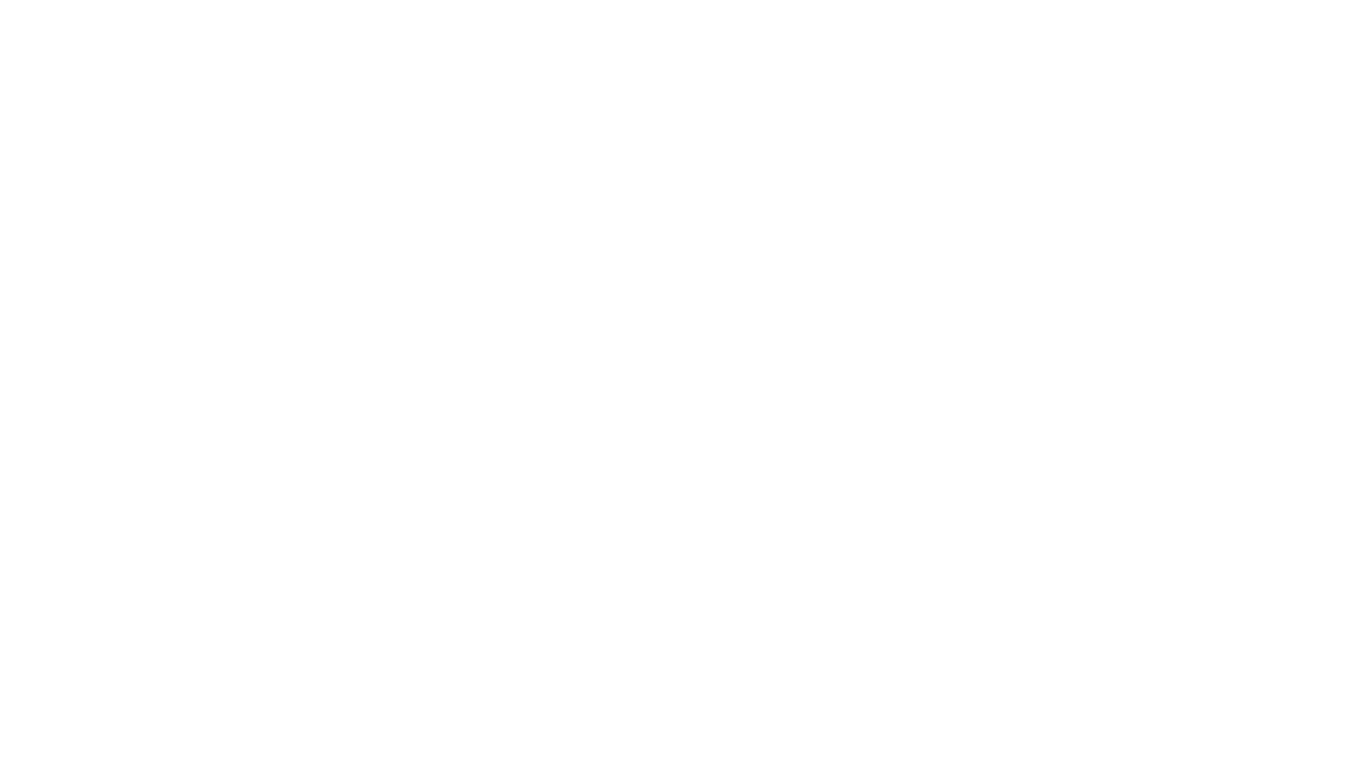
John Schulman's Homepage
John Schulman's Homepage is an AI tool developed by a researcher at Anthropic. The website focuses on aligning large language models, scalable oversight, and developing better written specifications of model behavior. It showcases the researcher's work in the field of AI, including projects like ChatGPT and the OpenAI API. The homepage also highlights the researcher's academic background, including a PhD in Computer Science from UC Berkeley with a focus on robotics and reinforcement learning.
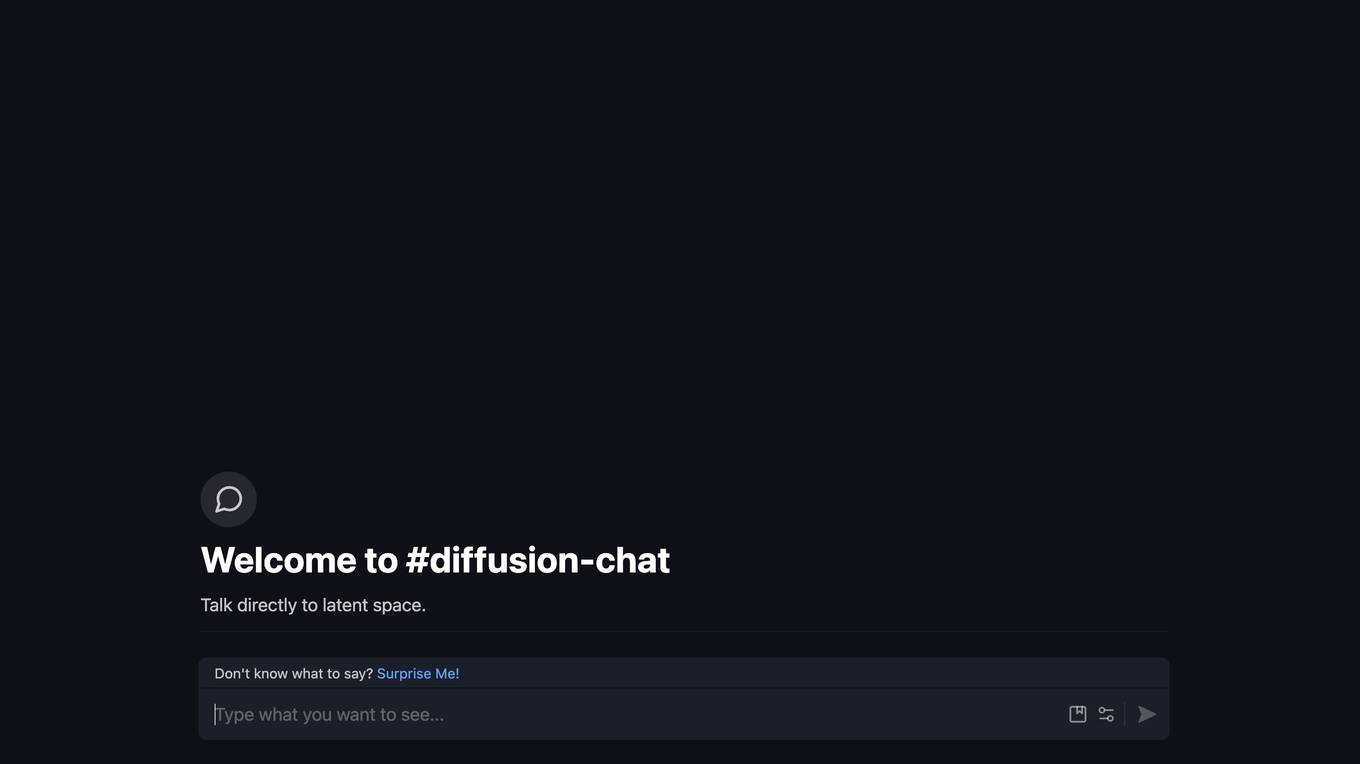
Diffusion Chat
Diffusion Chat is a text-to-image AI tool that allows users to generate images from text prompts. The tool uses a large language model to understand the user's prompt and then generates an image that matches the description. Diffusion Chat is still in development, but it has already shown great promise for creating realistic and creative images.
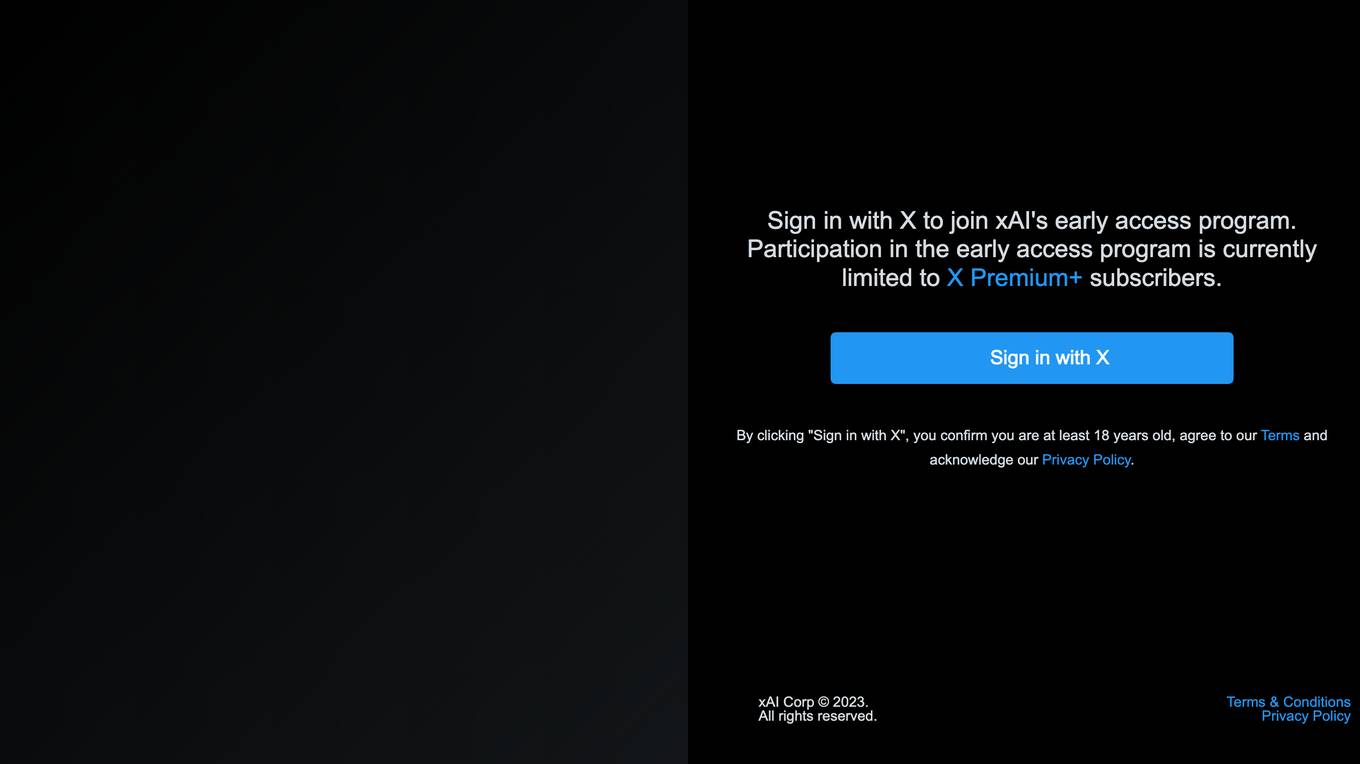
xAI Grok
xAI Grok is a visual analytics platform that helps users understand and interpret machine learning models. It provides a variety of tools for visualizing and exploring model data, including interactive charts, graphs, and tables. xAI Grok also includes a library of pre-built visualizations that can be used to quickly get started with model analysis.
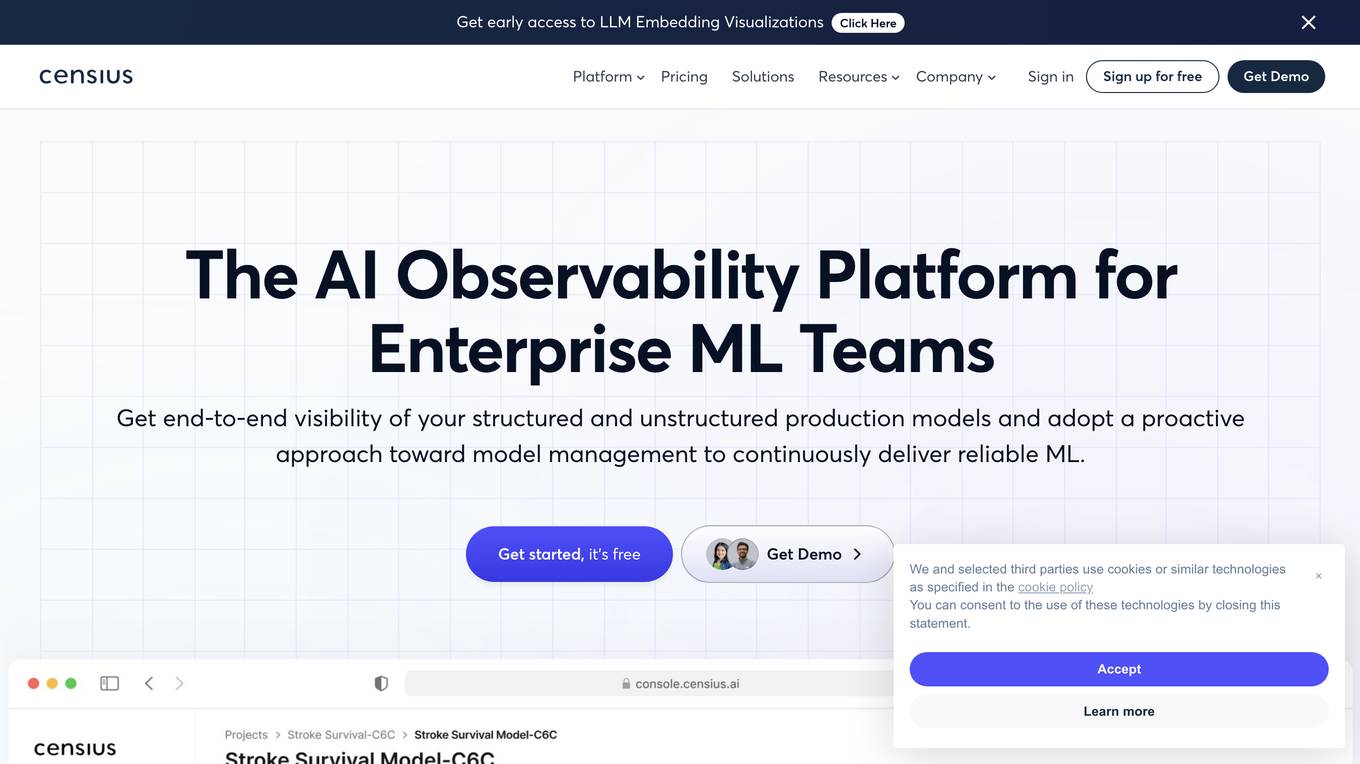
Censius
Censius is an AI Observability Platform for Enterprise ML Teams. It provides end-to-end visibility of structured and unstructured production models, enabling proactive model management and continuous delivery of reliable ML. Key features include model monitoring, explainability, and analytics.
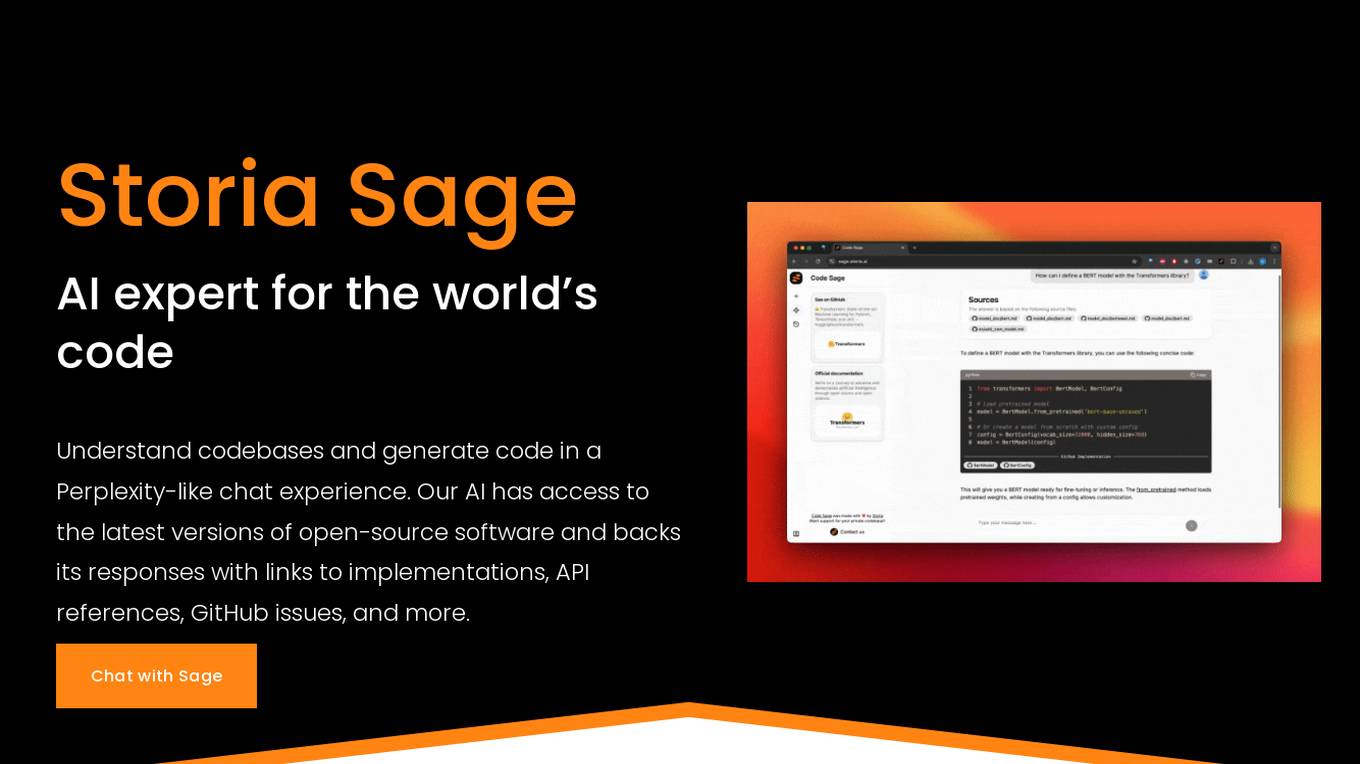
Storia AI
Storia AI is an AI tool designed to assist software engineering teams in understanding and generating code. It provides a Perplexity-like chat experience where users can interact with an AI expert that has access to the latest versions of open-source software. The tool aims to improve code understanding and generation by providing responses backed with links to implementations, API references, GitHub issues, and more. Storia AI is developed by a team of natural language processing researchers from Google and Amazon Alexa, with a mission to build the most reliable AI pair programmer for engineering teams.
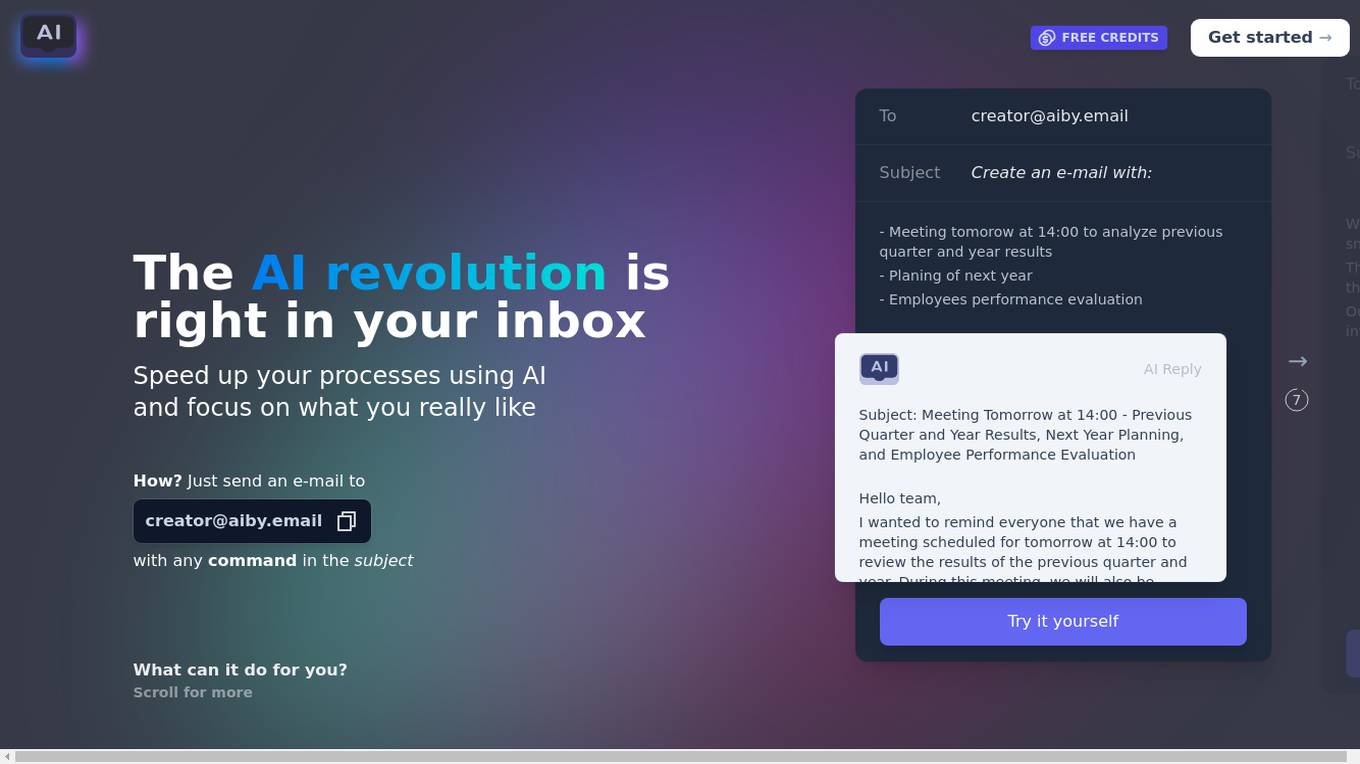
AIby.email
AIby.email is an AI-powered email assistant that helps you write better emails, faster. It uses natural language processing to understand your intent and generate personalized email responses. AIby.email also offers a variety of other features, such as email scheduling, tracking, and analytics.
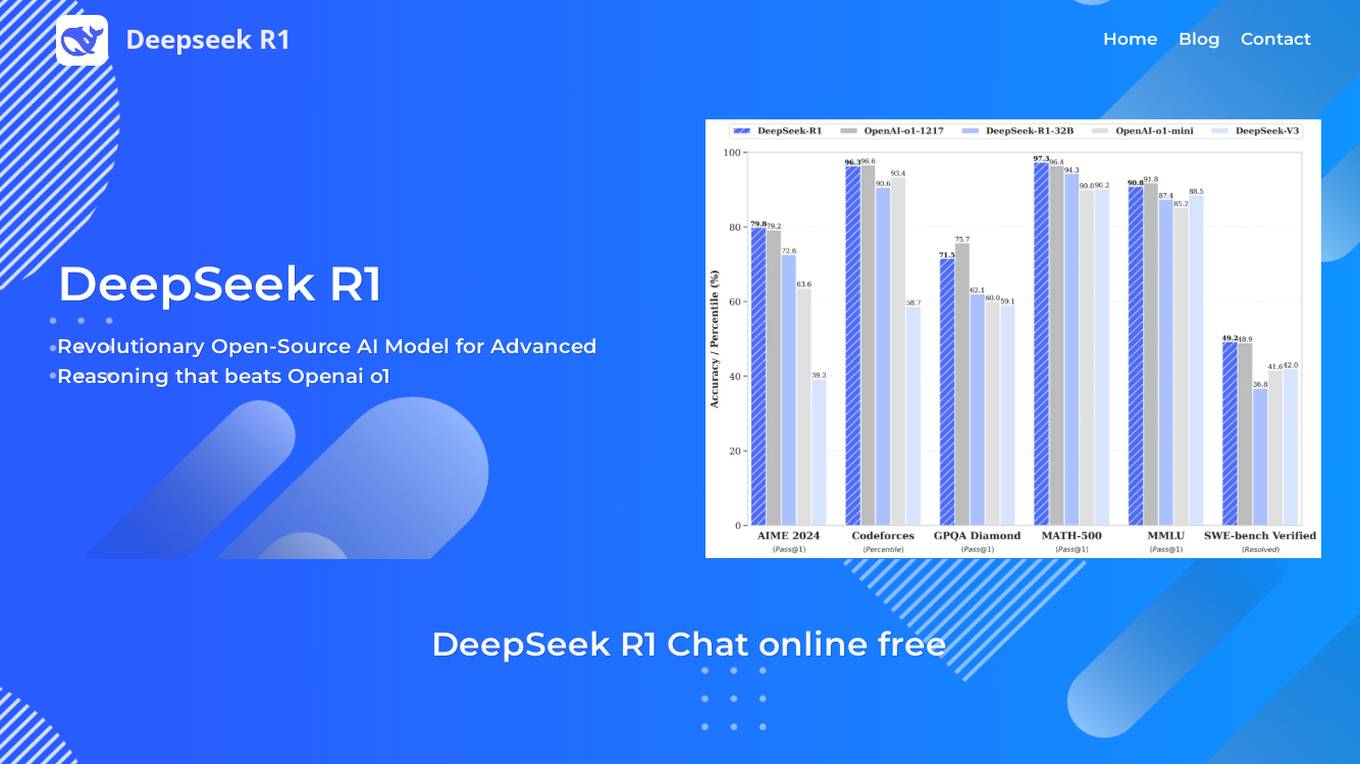
DeepSeek R1
DeepSeek R1 is a revolutionary open-source AI model for advanced reasoning that outperforms leading AI models in mathematics, coding, and general reasoning tasks. It utilizes a sophisticated MoE architecture with 37B active/671B total parameters and 128K context length, incorporating advanced reinforcement learning techniques. DeepSeek R1 offers multiple variants and distilled models optimized for complex problem-solving, multilingual understanding, and production-grade code generation. It provides cost-effective pricing compared to competitors like OpenAI o1, making it an attractive choice for developers and enterprises.
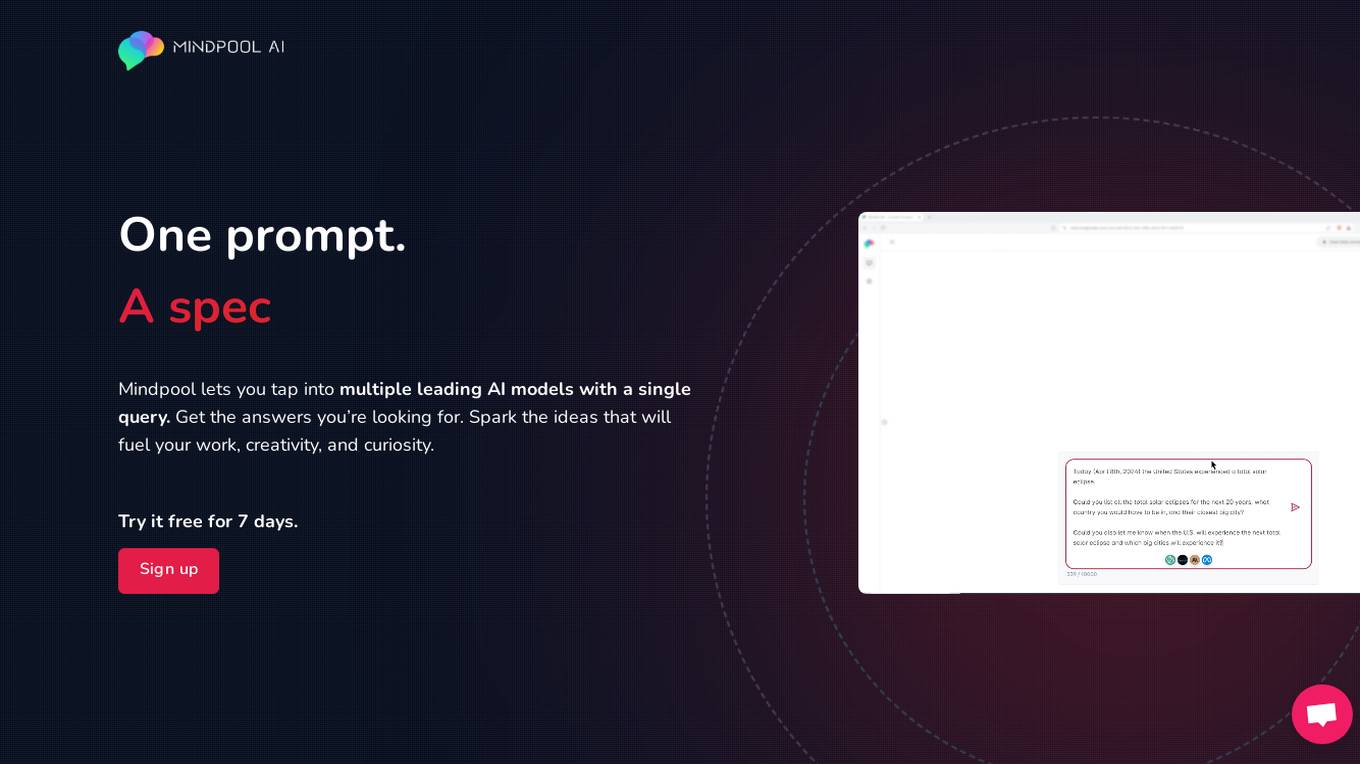
MindpoolAI
MindpoolAI is a tool that allows users to access multiple leading AI models with a single query. This means that users can get the answers they are looking for, spark ideas, and fuel their work, creativity, and curiosity. MindpoolAI is easy to use and does not require any technical expertise. Users simply need to enter their prompt and select the AI models they want to compare. MindpoolAI will then send the query to the selected models and present the results in an easy-to-understand format.
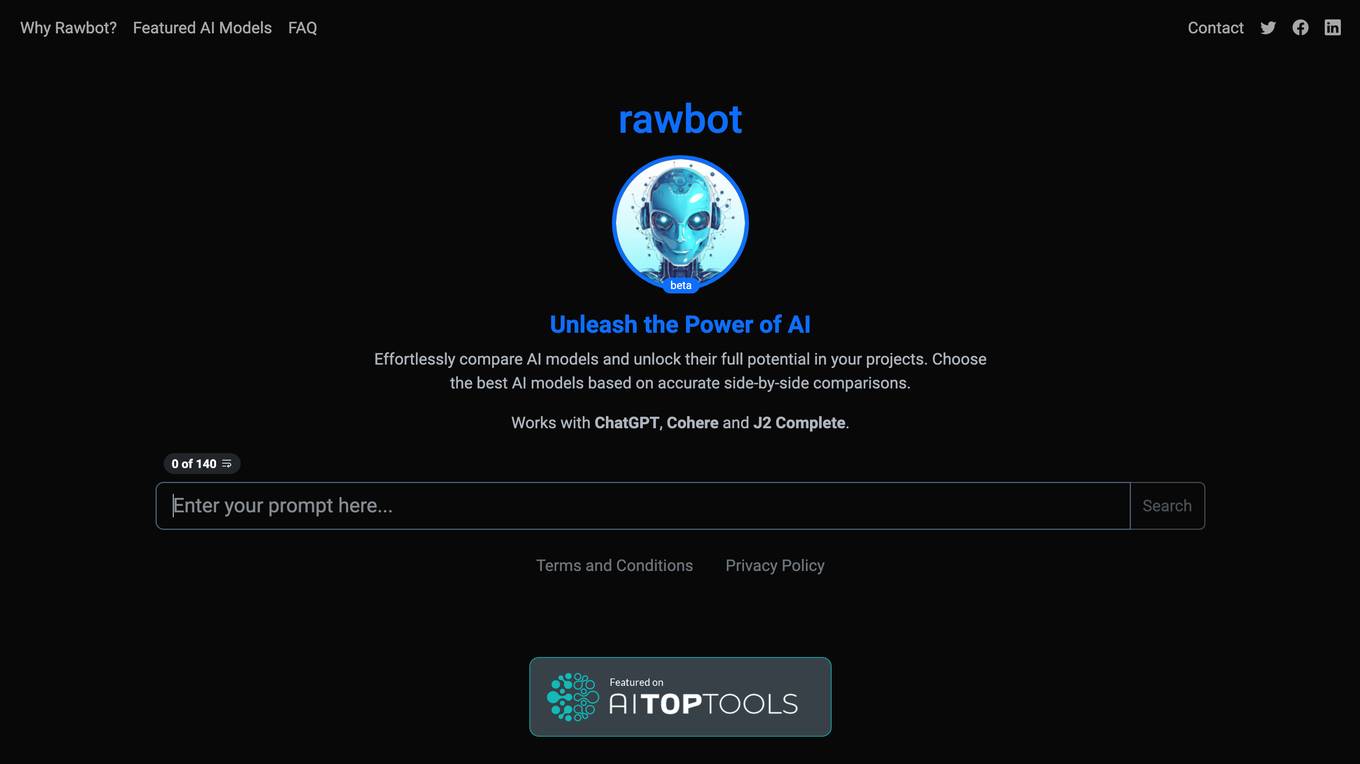
Rawbot
Rawbot is an AI model comparison tool designed to simplify the selection process by enabling users to identify and understand the strengths and weaknesses of various AI models. It allows users to compare AI models based on performance optimization, strengths and weaknesses identification, customization and tuning, cost and efficiency analysis, and informed decision-making. Rawbot is a user-friendly platform that supports a wide range of popular and emerging AI models, making it a premier destination for researchers, developers, and business leaders to make informed decisions about AI models that best fit their needs.
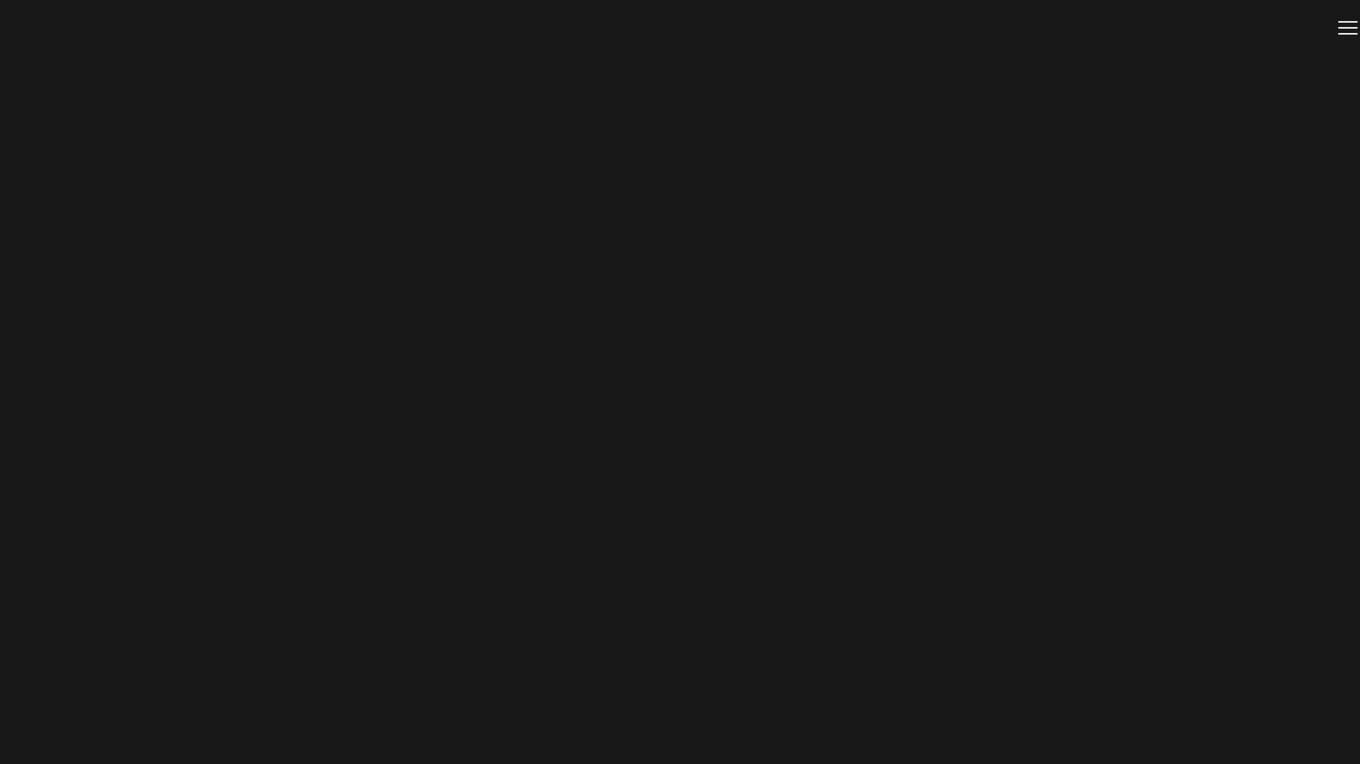
ChatGPT
ChatGPT is a large language model developed by OpenAI. It is designed to understand and generate human-like text, and can be used for a variety of tasks such as answering questions, writing stories, and translating languages. ChatGPT is free to use, and can be accessed through a web interface or via an API.
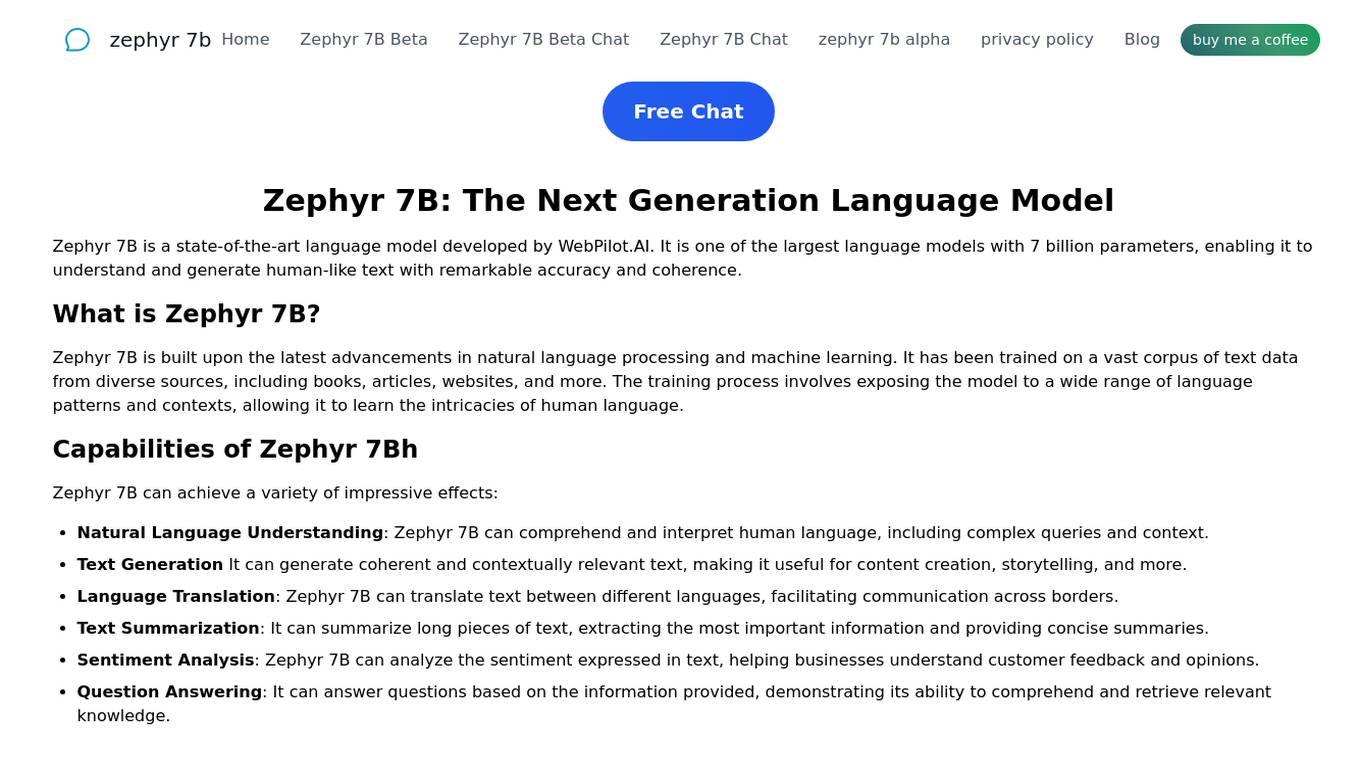
Zephyr 7B
Zephyr 7B is a state-of-the-art language model developed by WebPilot.AI with 7 billion parameters. It can understand and generate human-like text with remarkable accuracy and coherence. The model is built upon the latest advancements in natural language processing and machine learning, trained on a vast corpus of text data from diverse sources. Zephyr 7B offers capabilities such as natural language understanding, text generation, language translation, text summarization, sentiment analysis, and question answering. It represents a significant advancement in natural language processing, making it a powerful tool for content creation, customer support, research, and more.
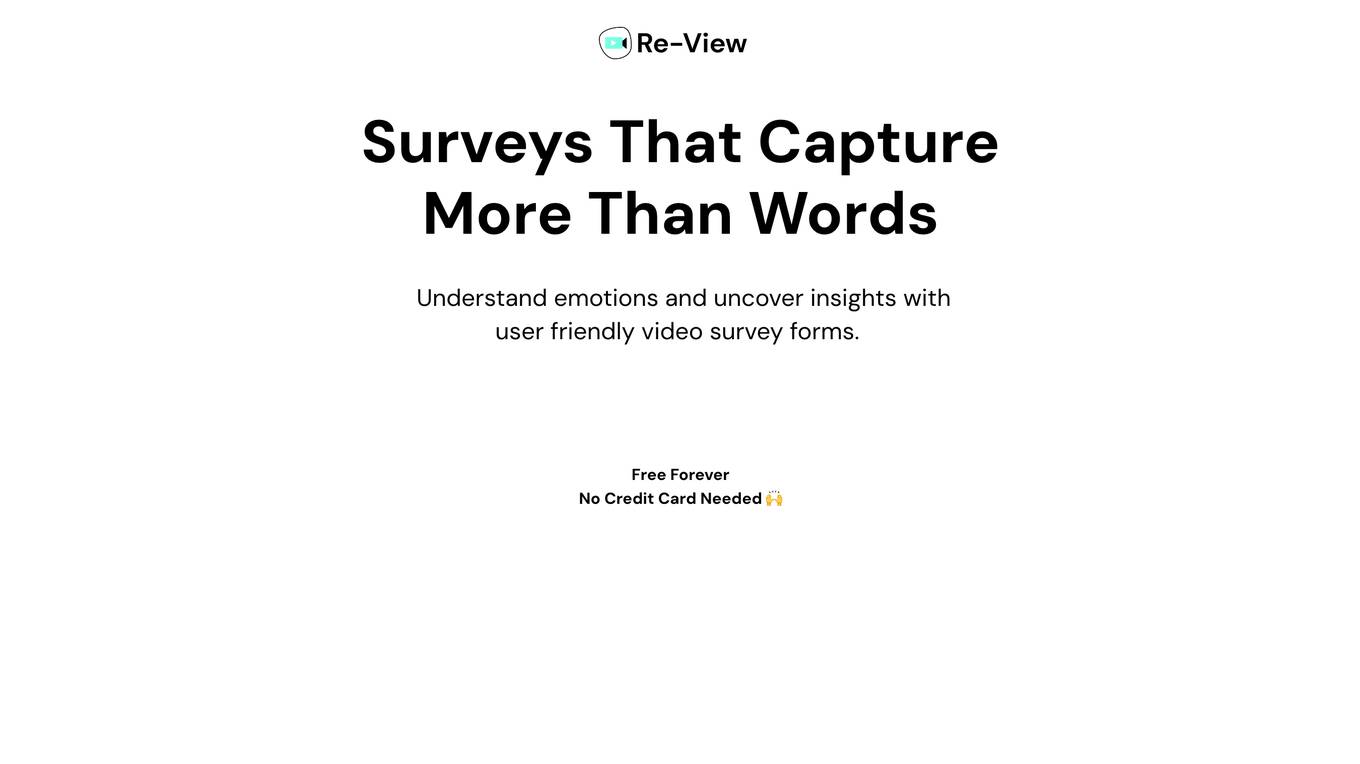
Re-View
Re-View is an AI-powered platform that enables users to conduct surveys that capture more than words by utilizing user-friendly video survey forms. The platform allows users to understand emotions, uncover insights, and collect more and better data through authentic emotional connections. With features like automatic insights, efficient research at scale, stunning simplicity, and powerful research capabilities, Re-View offers a practical pricing model that makes research accessible to all. Users can easily create surveys, analyze responses with AI assistance, and gain valuable research reports to support decision-making.
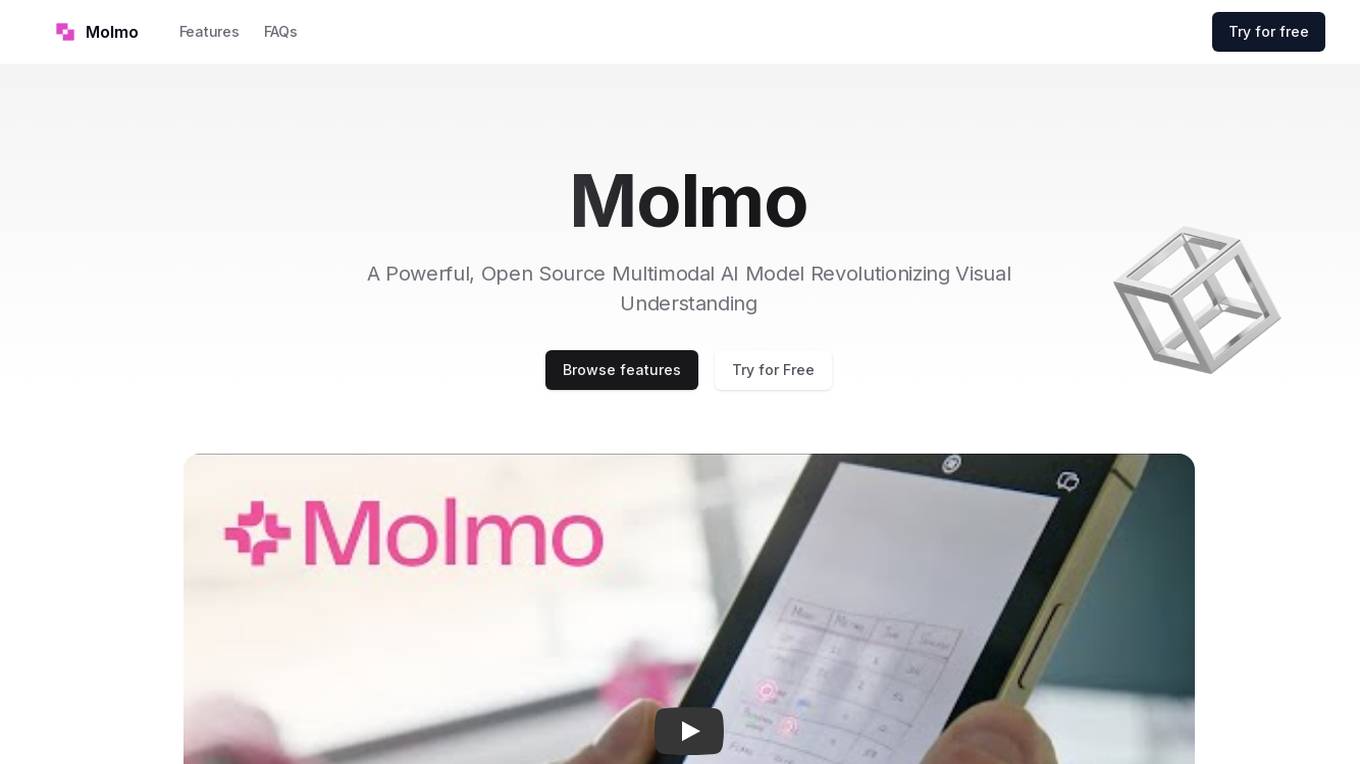
Molmo AI
Molmo AI is a powerful, open-source multimodal AI model revolutionizing visual understanding. It helps developers easily build tools that can understand images and interact with the world in useful ways. Molmo AI offers exceptional image understanding, efficient data usage, open and accessible features, on-device compatibility, and a new era in multimodal AI development. It closes the gap between open and closed AI models, empowers the AI community with open access, and efficiently utilizes data for superior performance.
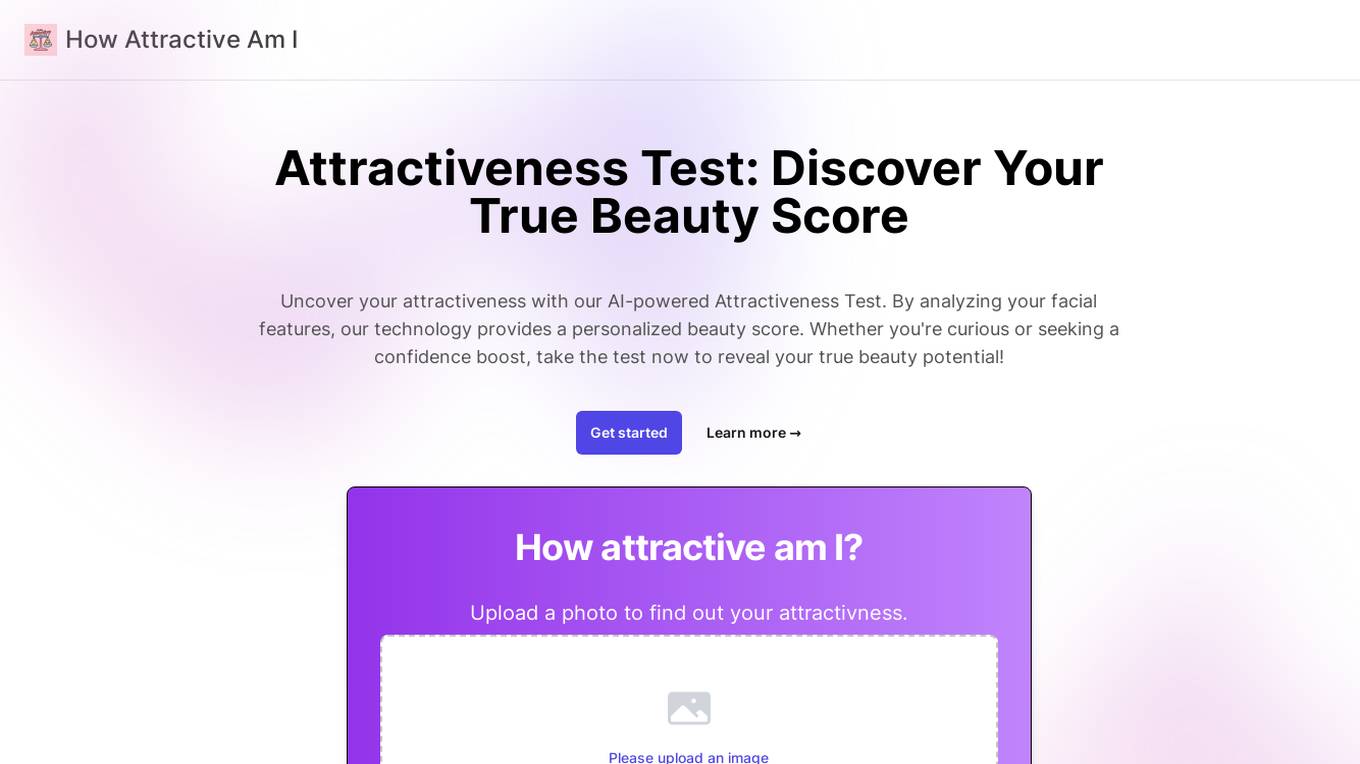
How Attractive Am I
How Attractive Am I is an AI-powered tool that analyzes facial features to calculate an attractiveness score. By evaluating symmetry and proportions, the tool provides personalized beauty scores. Users can upload a photo to discover their true beauty potential. The tool ensures accuracy by providing guidelines for taking photos and offers a fun and insightful way to understand facial appeal.
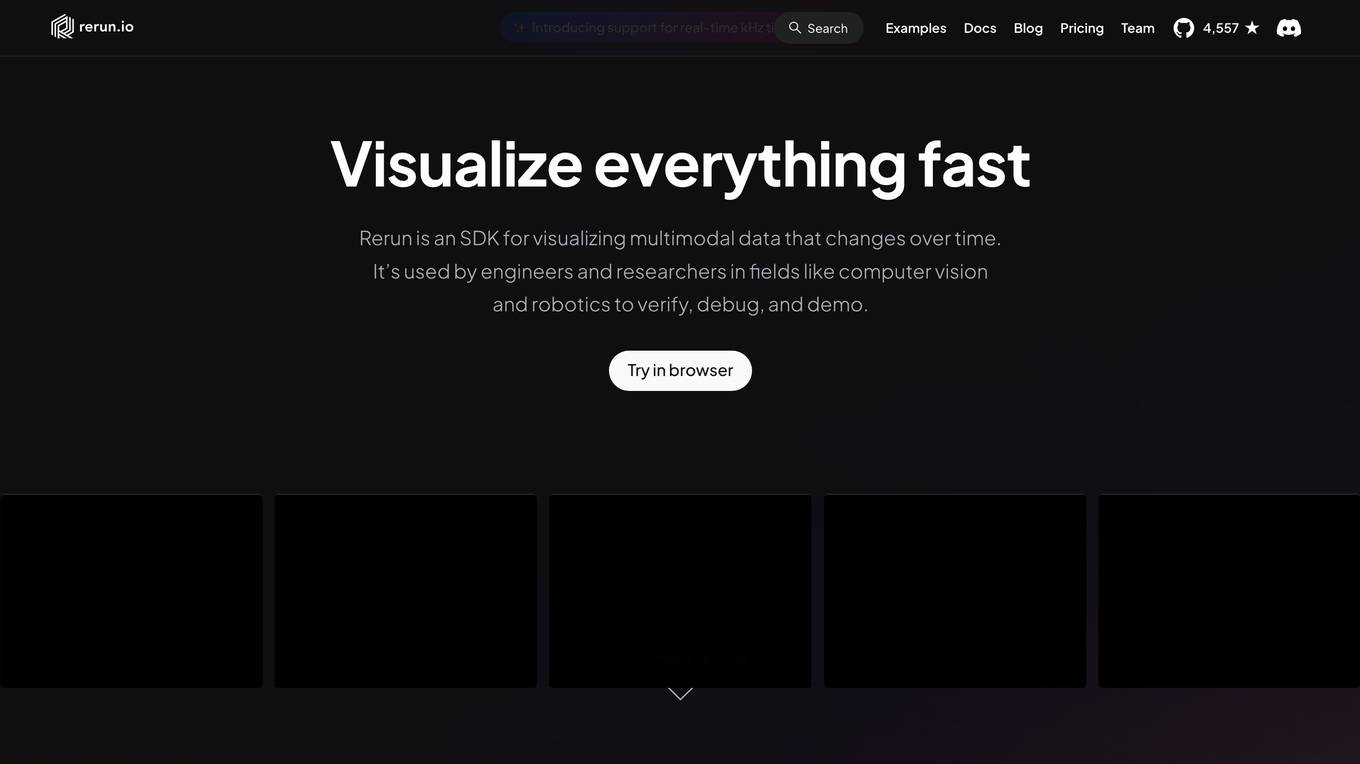
Rerun
Rerun is an SDK, time-series database, and visualizer for temporal and multimodal data. It is used in fields like robotics, spatial computing, 2D/3D simulation, and finance to verify, debug, and explain data. Rerun allows users to log data like tensors, point clouds, and text to create streams, visualize and interact with live and recorded streams, build layouts, customize visualizations, and extend data and UI functionalities. The application provides a composable data model, dynamic schemas, and custom views for enhanced data visualization and analysis.
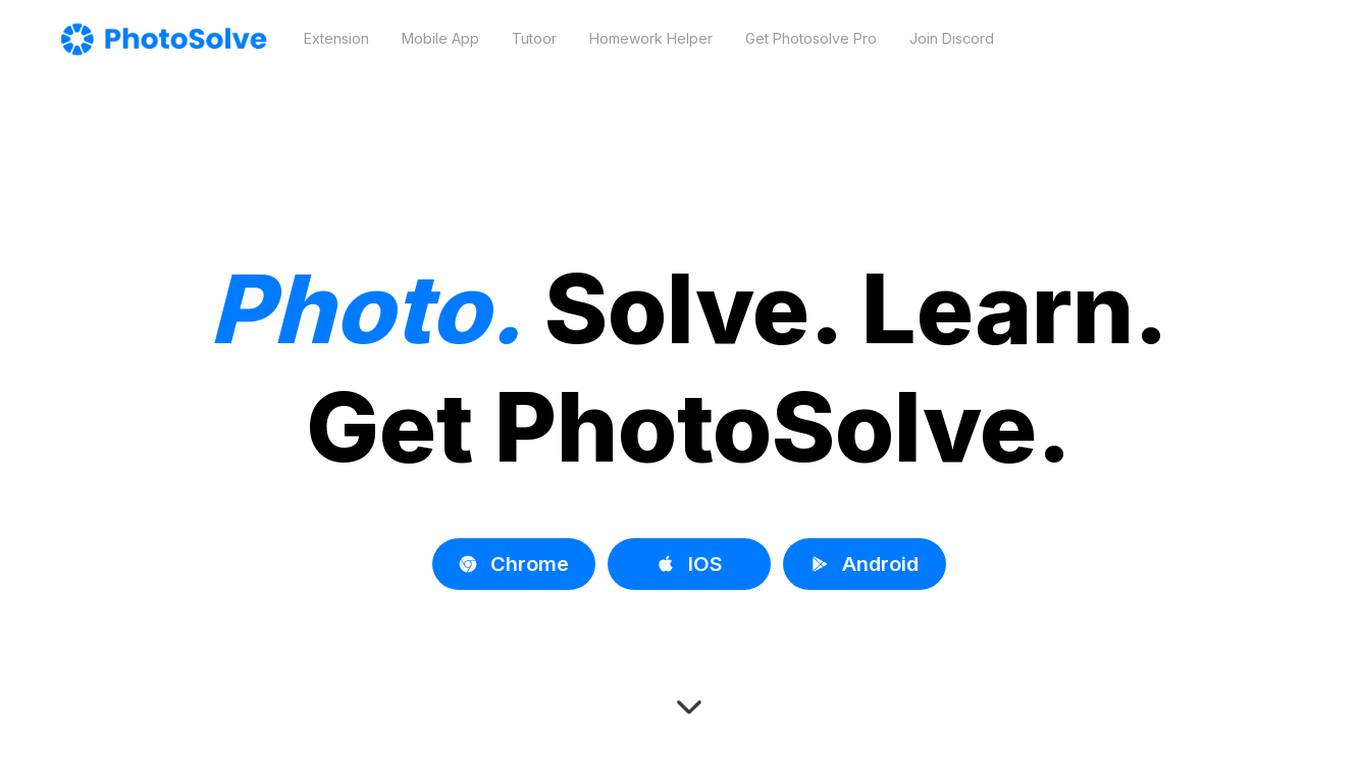
Photosolve
Photosolve is an AI-powered educational tool that helps students, teachers, researchers, and writers to quickly find accurate answers to their questions. It offers a Chrome extension and mobile app for easy access to its features. With over 10 million questions answered and growing, Photosolve revolutionizes learning by providing detailed explanations along with answers. Users can upload materials for analysis, have conversations with AI, generate flashcards, and enhance their knowledge with customizable quizzes. The application uses a custom-built AI model for higher accuracy compared to general AI models, ensuring reliable results for academic success.
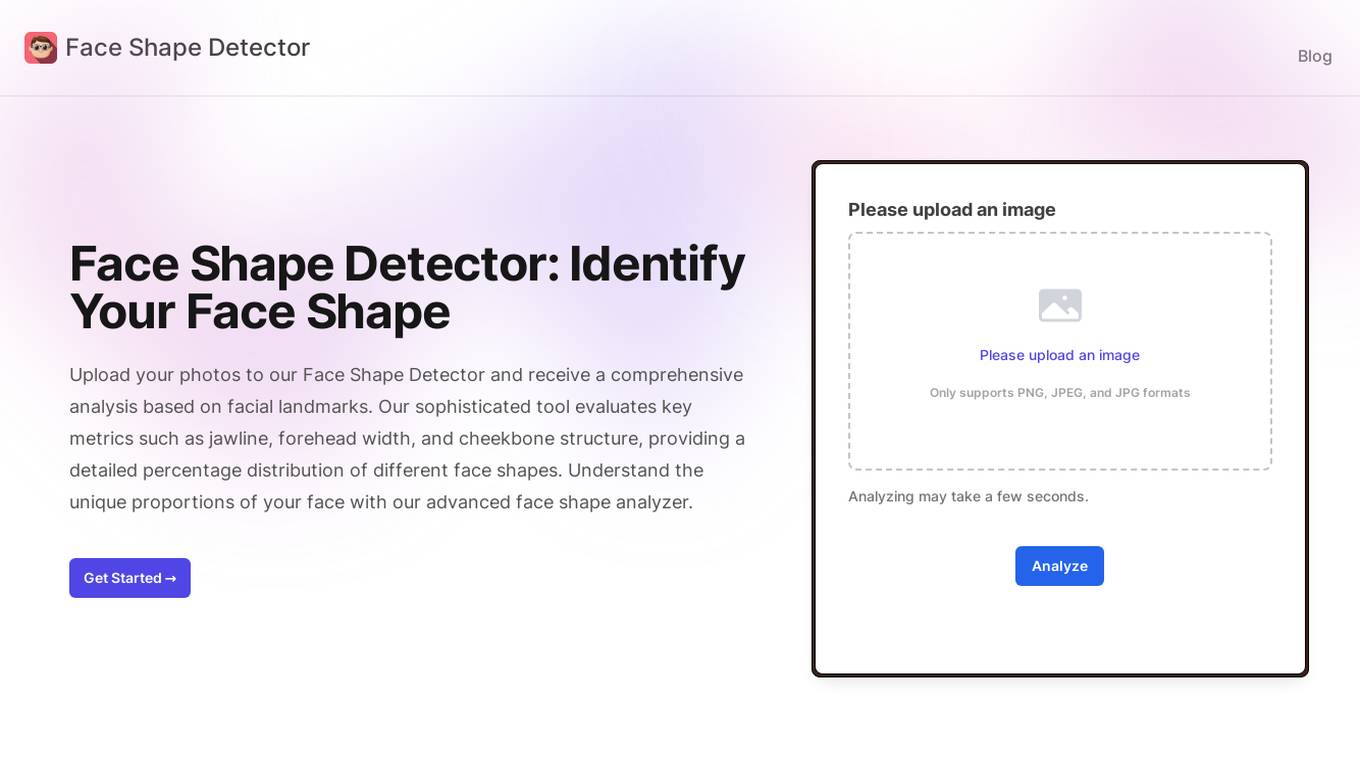
Face Shape Detector
Face Shape Detector is an advanced AI tool that analyzes facial landmarks in uploaded photos to identify the user's face shape and provide percentage distributions for different face shapes. It utilizes sophisticated algorithms to assess key metrics such as jawline, forehead width, and cheekbone structure, delivering detailed insights into facial proportions. Users can explore the power of facial analysis, understand their unique face shape, and receive quick and accurate results through this intuitive tool.
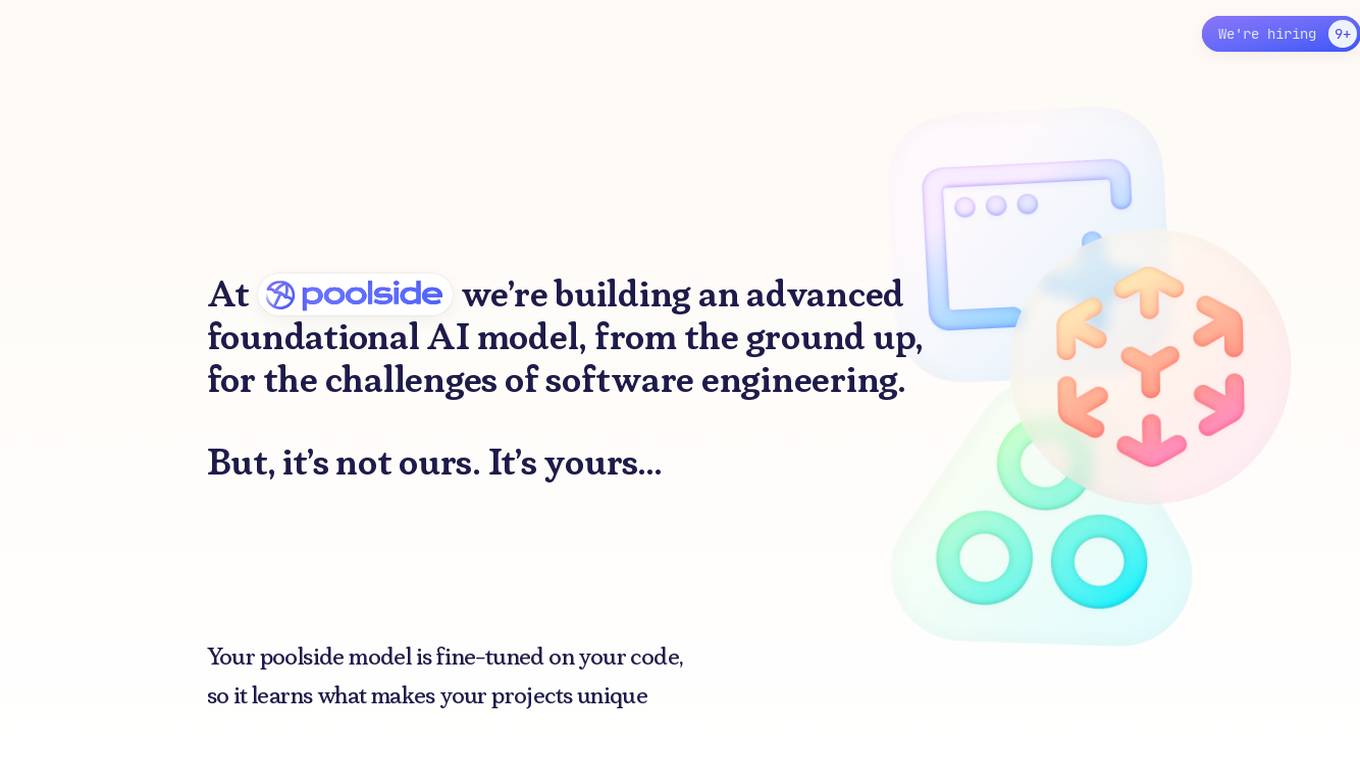
poolside
poolside is an advanced foundational AI model designed specifically for software engineering challenges. It allows users to fine-tune the model on their own code, enabling it to understand project uniqueness and complexities that generic models can't grasp. The platform aims to empower teams to build better, faster, and happier by providing a personalized AI model that continuously improves. In addition to the AI model for writing code, poolside offers an intuitive editor assistant and an API for developers to leverage.
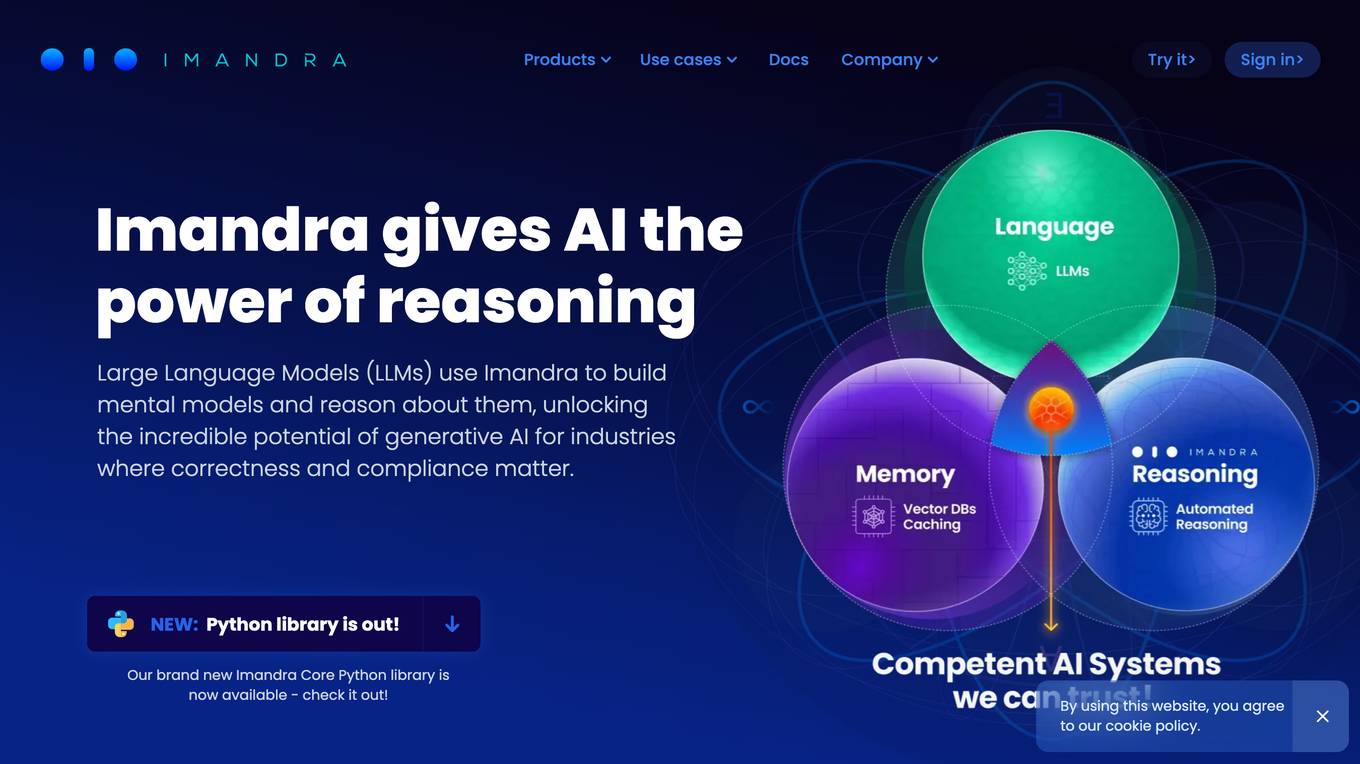
Imandra
Imandra is a company that provides automated logical reasoning for Large Language Models (LLMs). Imandra's technology allows LLMs to build mental models and reason about them, unlocking the potential of generative AI for industries where correctness and compliance matter. Imandra's platform is used by leading financial firms, the US Air Force, and DARPA.
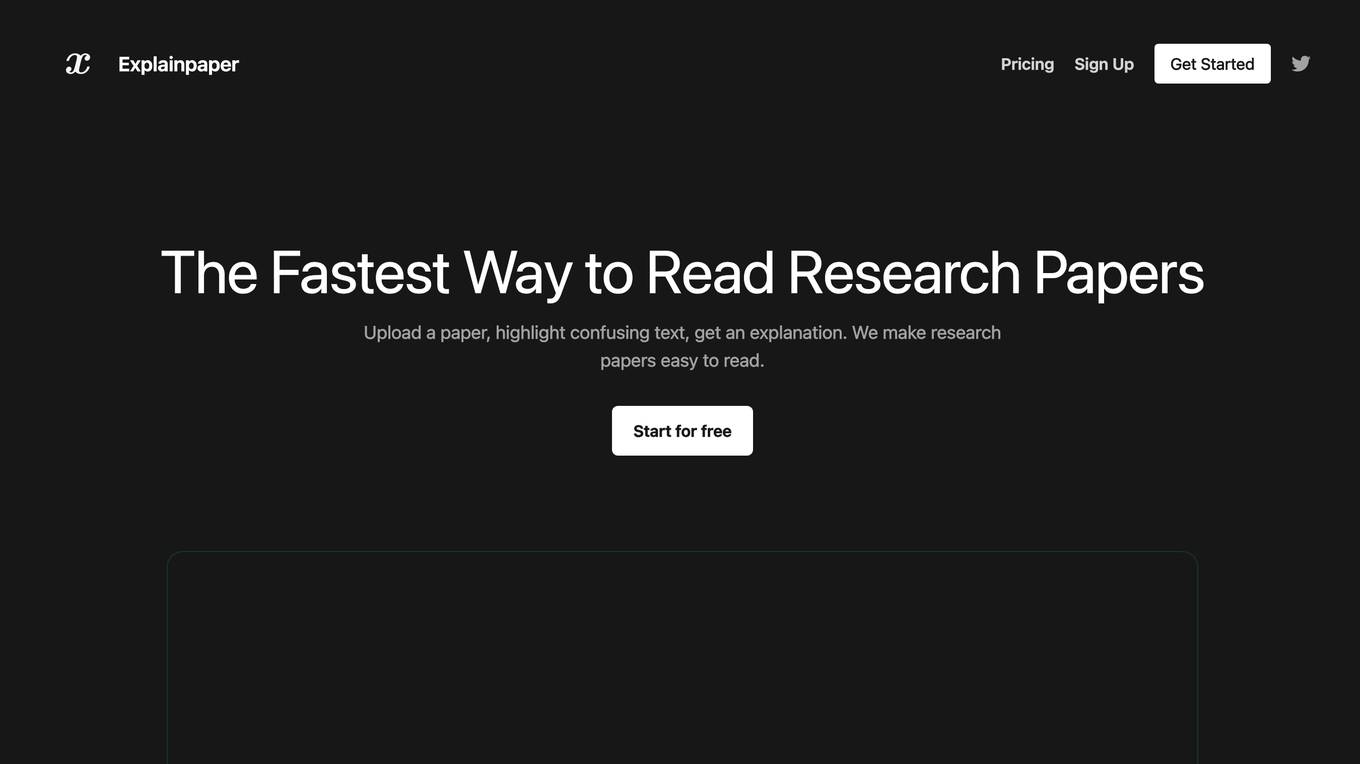
Explainpaper
Explainpaper is an AI-powered tool designed to simplify the process of reading and understanding research papers. Users can upload a paper, highlight confusing text, and receive explanations using an AI model. The tool aims to make complex concepts more accessible and help researchers, students, and enthusiasts comprehend academic content more efficiently.
20 - Open Source AI Tools
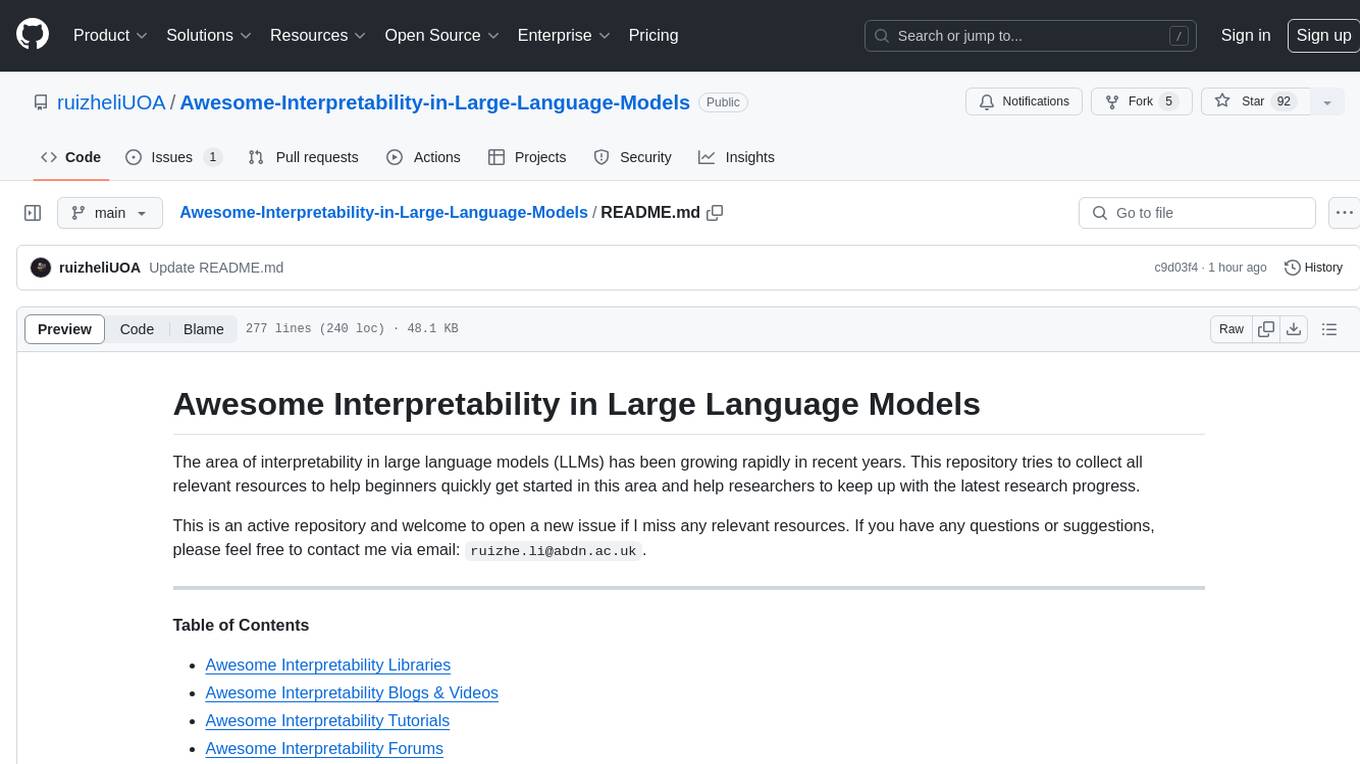
Awesome-Interpretability-in-Large-Language-Models
This repository is a collection of resources focused on interpretability in large language models (LLMs). It aims to help beginners get started in the area and keep researchers updated on the latest progress. It includes libraries, blogs, tutorials, forums, tools, programs, papers, and more related to interpretability in LLMs.
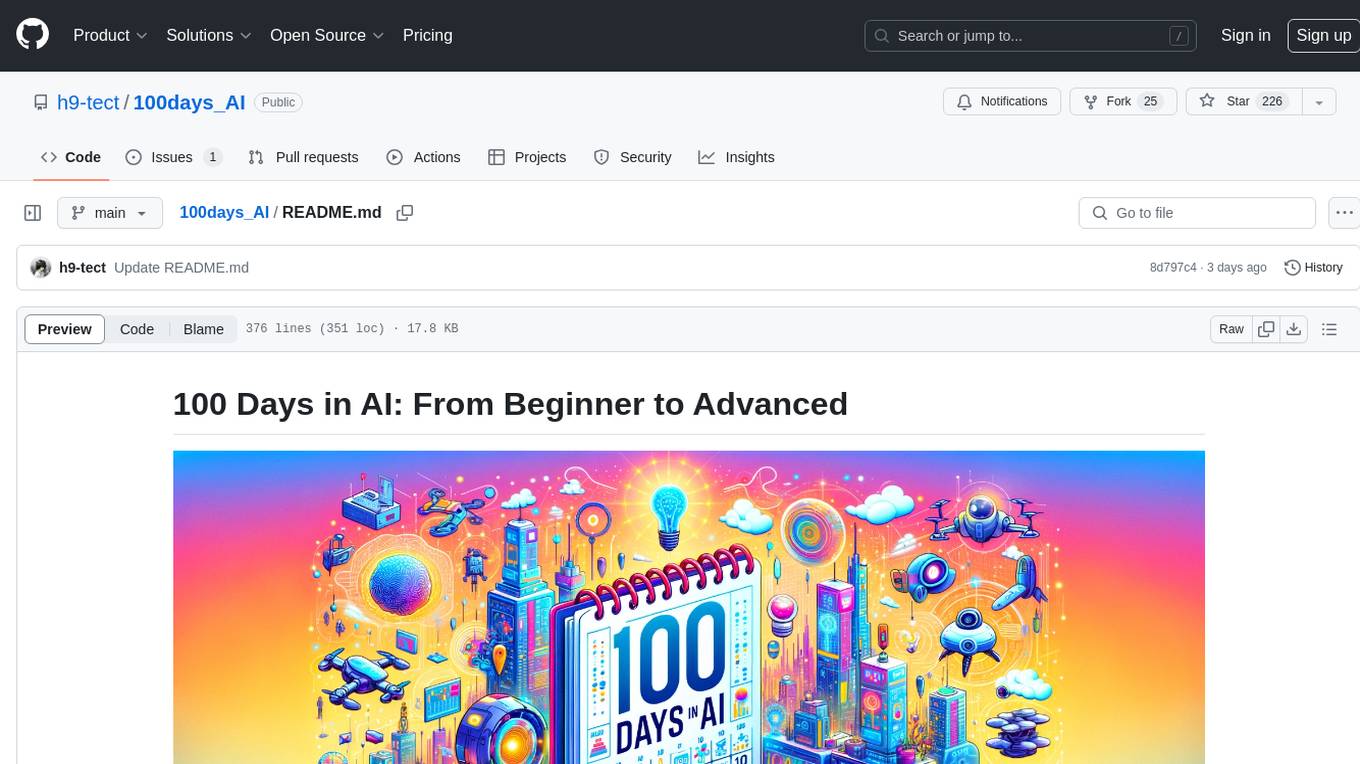
100days_AI
The 100 Days in AI repository provides a comprehensive roadmap for individuals to learn Artificial Intelligence over a period of 100 days. It covers topics ranging from basic programming in Python to advanced concepts in AI, including machine learning, deep learning, and specialized AI topics. The repository includes daily tasks, resources, and exercises to ensure a structured learning experience. By following this roadmap, users can gain a solid understanding of AI and be prepared to work on real-world AI projects.
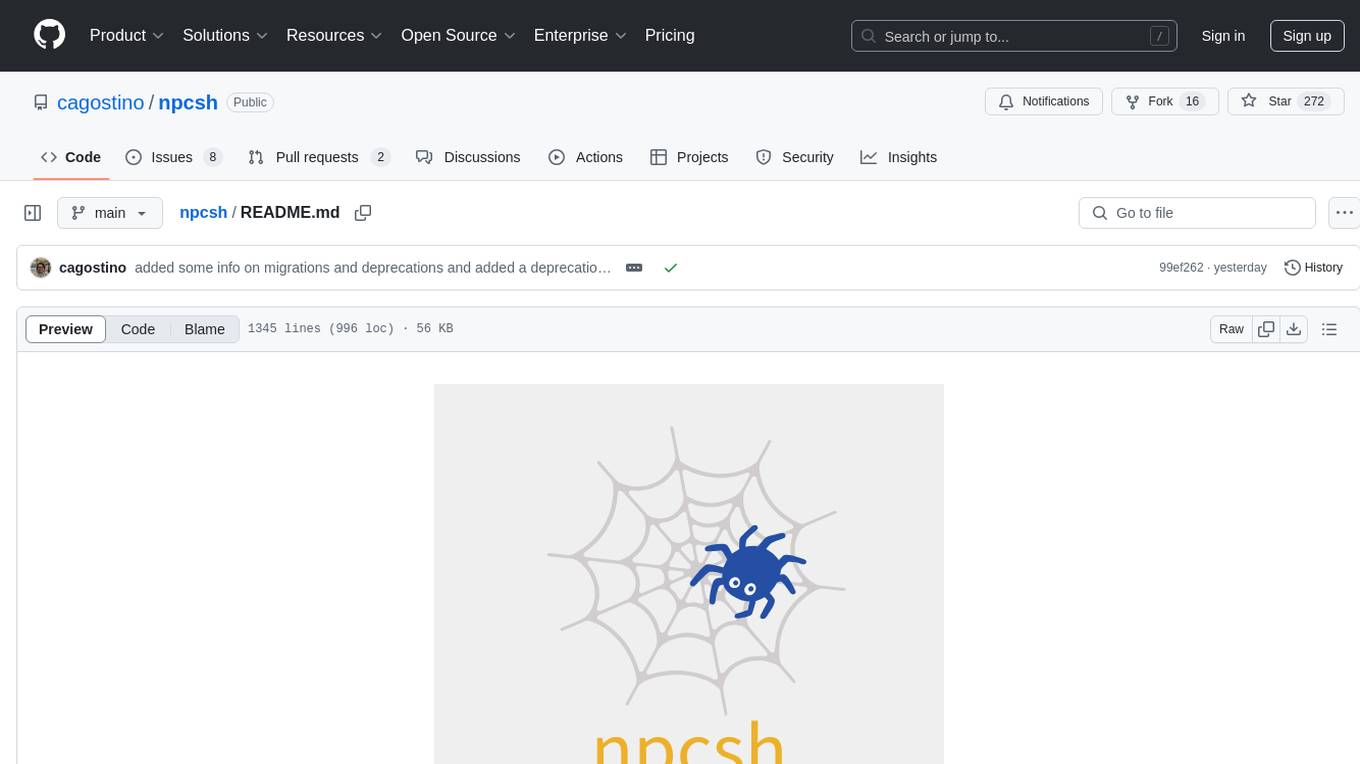
npcsh
`npcsh` is a python-based command-line tool designed to integrate Large Language Models (LLMs) and Agents into one's daily workflow by making them available and easily configurable through the command line shell. It leverages the power of LLMs to understand natural language commands and questions, execute tasks, answer queries, and provide relevant information from local files and the web. Users can also build their own tools and call them like macros from the shell. `npcsh` allows users to take advantage of agents (i.e. NPCs) through a managed system, tailoring NPCs to specific tasks and workflows. The tool is extensible with Python, providing useful functions for interacting with LLMs, including explicit coverage for popular providers like ollama, anthropic, openai, gemini, deepseek, and openai-like providers. Users can set up a flask server to expose their NPC team for use as a backend service, run SQL models defined in their project, execute assembly lines, and verify the integrity of their NPC team's interrelations. Users can execute bash commands directly, use favorite command-line tools like VIM, Emacs, ipython, sqlite3, git, pipe the output of these commands to LLMs, or pass LLM results to bash commands.
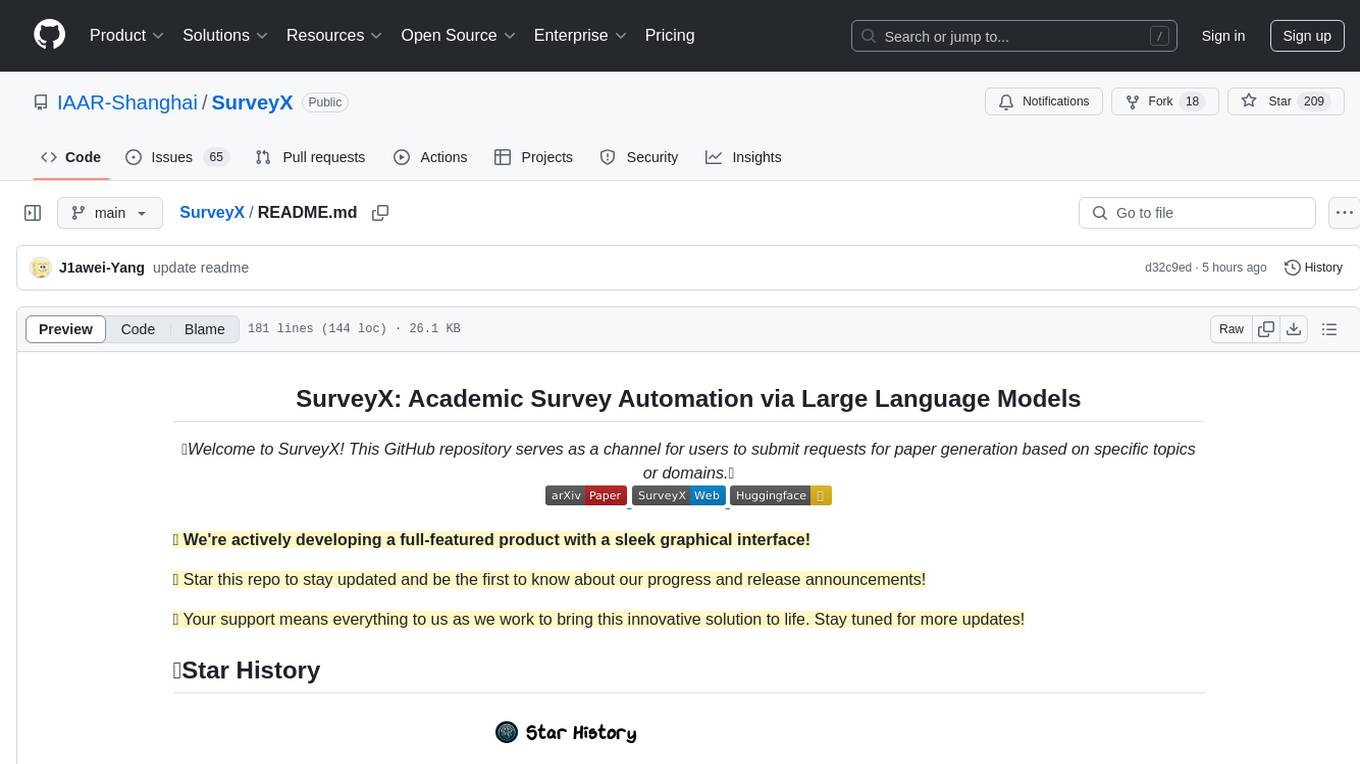
SurveyX
SurveyX is an advanced academic survey automation system that leverages Large Language Models (LLMs) to generate high-quality, domain-specific academic papers and surveys. Users can request comprehensive academic papers or surveys tailored to specific topics by providing a paper title and keywords for literature retrieval. The system streamlines academic research by automating paper creation, saving users time and effort in compiling research content.
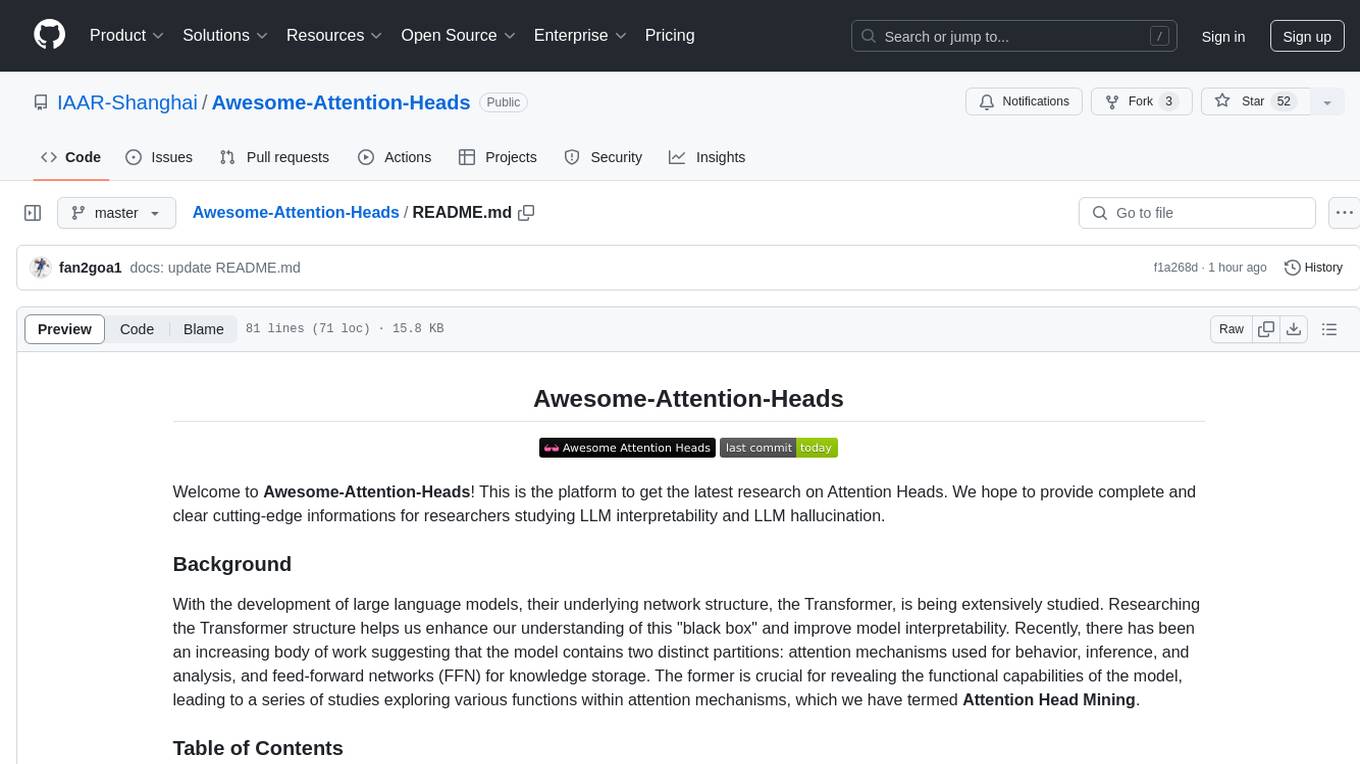
Awesome-Attention-Heads
Awesome-Attention-Heads is a platform providing the latest research on Attention Heads, focusing on enhancing understanding of Transformer structure for model interpretability. It explores attention mechanisms for behavior, inference, and analysis, alongside feed-forward networks for knowledge storage. The repository aims to support researchers studying LLM interpretability and hallucination by offering cutting-edge information on Attention Head Mining.
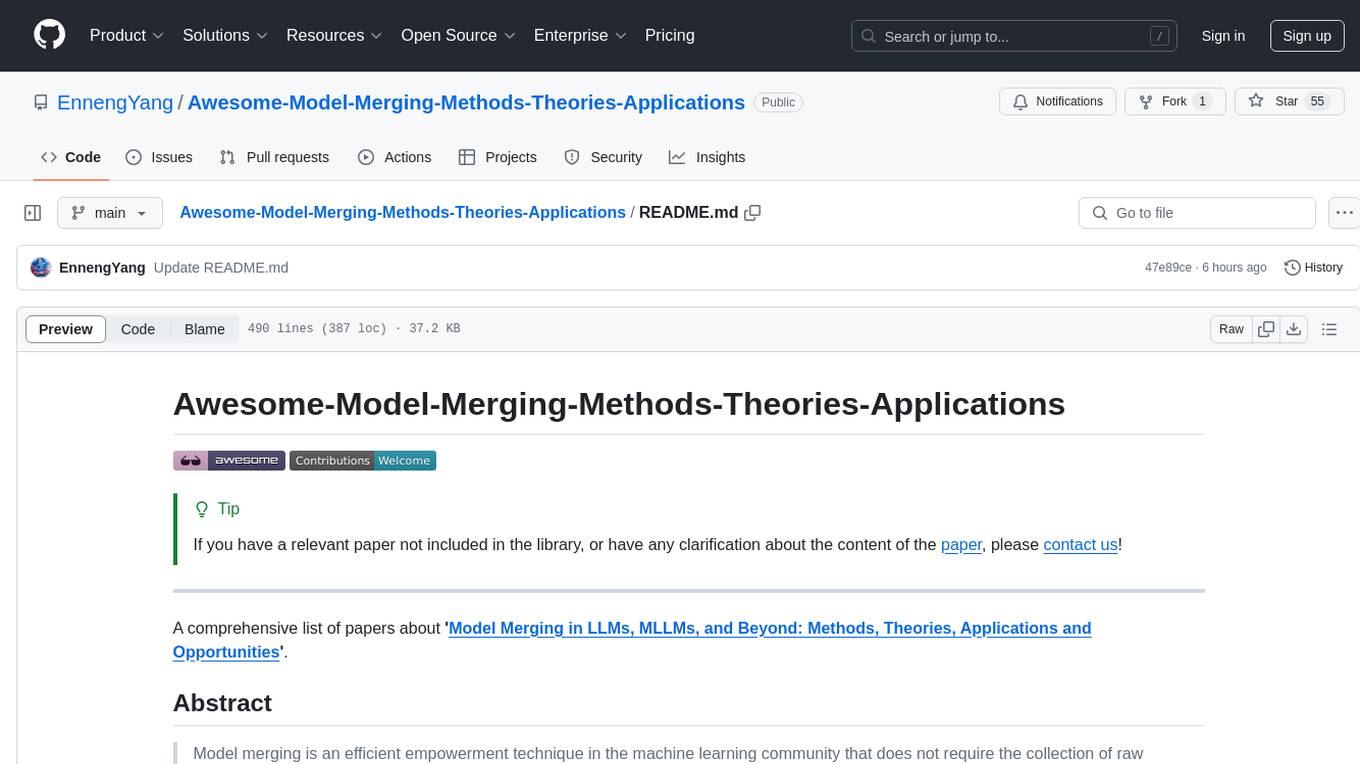
Awesome-Model-Merging-Methods-Theories-Applications
A comprehensive repository focusing on 'Model Merging in LLMs, MLLMs, and Beyond', providing an exhaustive overview of model merging methods, theories, applications, and future research directions. The repository covers various advanced methods, applications in foundation models, different machine learning subfields, and tasks like pre-merging methods, architecture transformation, weight alignment, basic merging methods, and more.
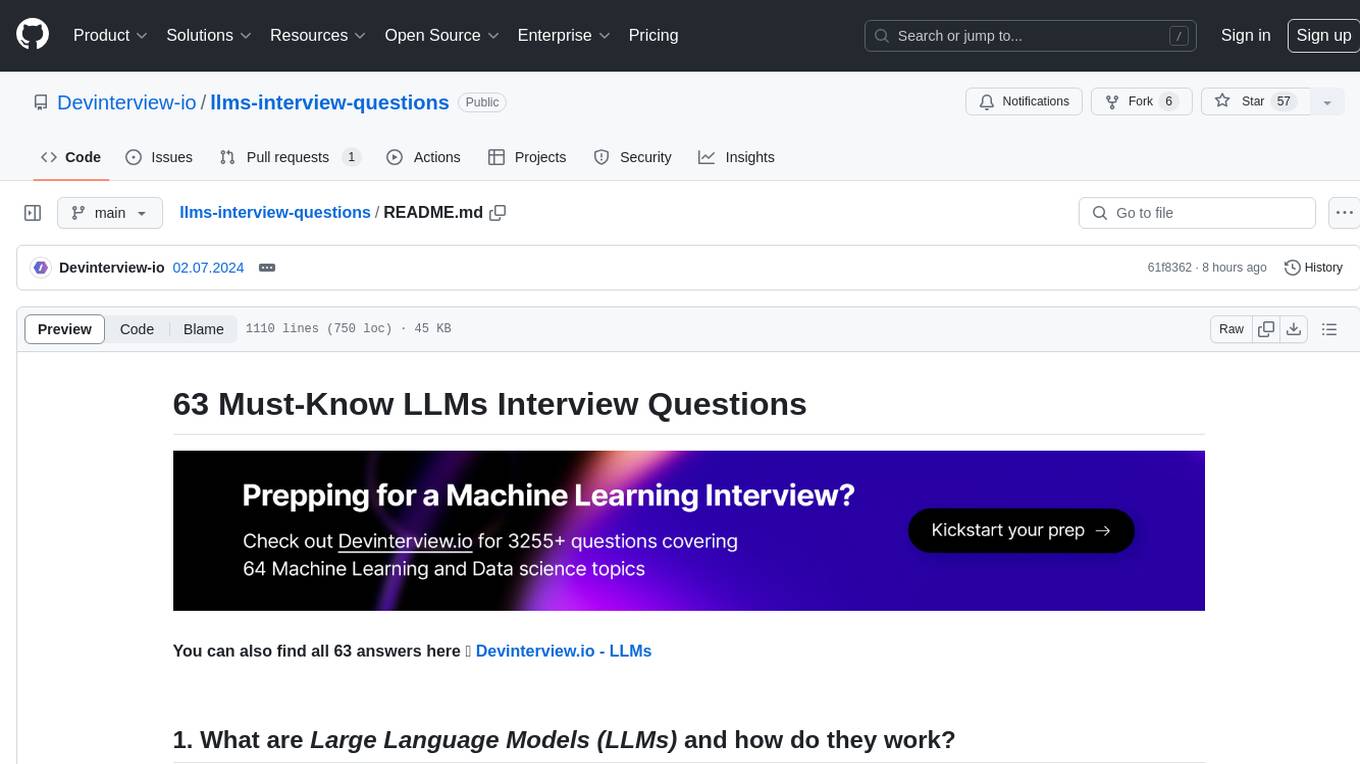
llms-interview-questions
This repository contains a comprehensive collection of 63 must-know Large Language Models (LLMs) interview questions. It covers topics such as the architecture of LLMs, transformer models, attention mechanisms, training processes, encoder-decoder frameworks, differences between LLMs and traditional statistical language models, handling context and long-term dependencies, transformers for parallelization, applications of LLMs, sentiment analysis, language translation, conversation AI, chatbots, and more. The readme provides detailed explanations, code examples, and insights into utilizing LLMs for various tasks.
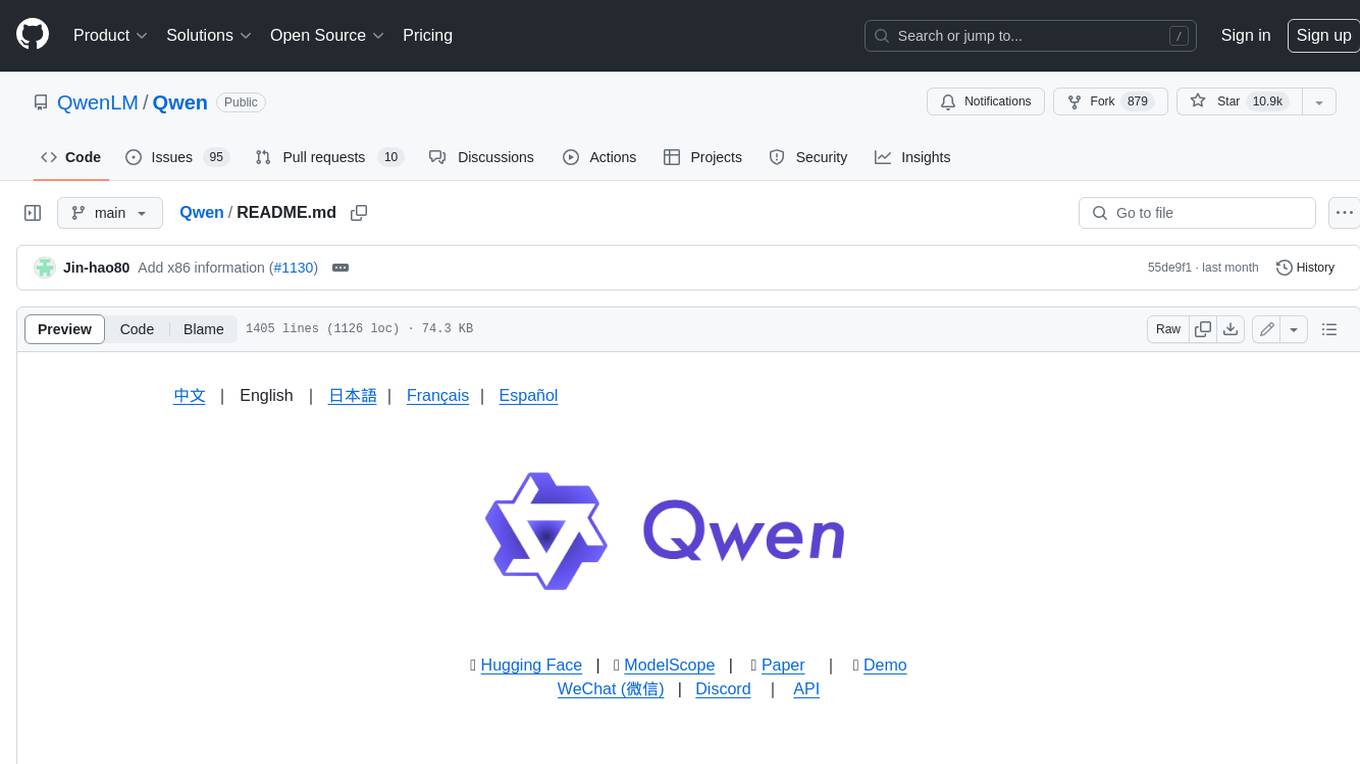
Qwen
Qwen is a series of large language models developed by Alibaba DAMO Academy. It outperforms the baseline models of similar model sizes on a series of benchmark datasets, e.g., MMLU, C-Eval, GSM8K, MATH, HumanEval, MBPP, BBH, etc., which evaluate the models’ capabilities on natural language understanding, mathematic problem solving, coding, etc. Qwen models outperform the baseline models of similar model sizes on a series of benchmark datasets, e.g., MMLU, C-Eval, GSM8K, MATH, HumanEval, MBPP, BBH, etc., which evaluate the models’ capabilities on natural language understanding, mathematic problem solving, coding, etc. Qwen-72B achieves better performance than LLaMA2-70B on all tasks and outperforms GPT-3.5 on 7 out of 10 tasks.
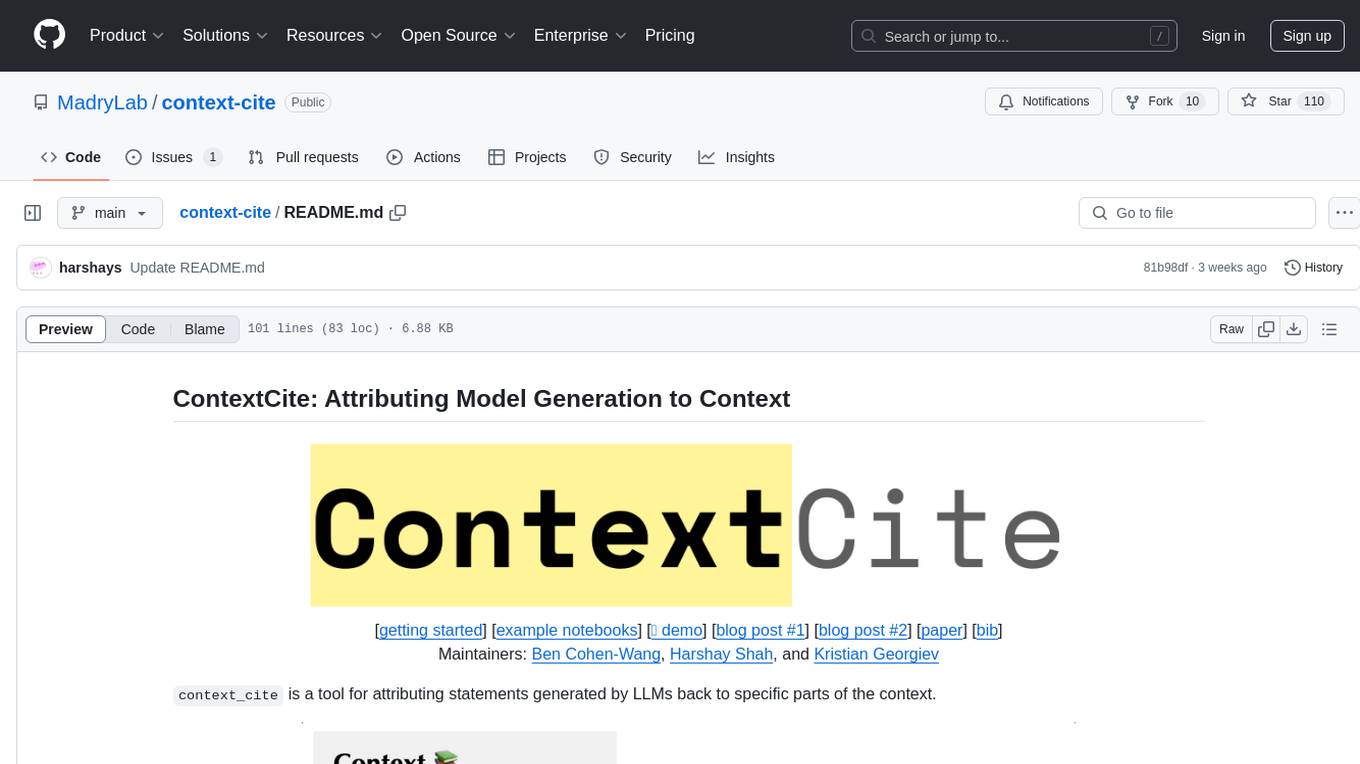
context-cite
ContextCite is a tool for attributing statements generated by LLMs back to specific parts of the context. It allows users to analyze and understand the sources of information used by language models in generating responses. By providing attributions, users can gain insights into how the model makes decisions and where the information comes from.
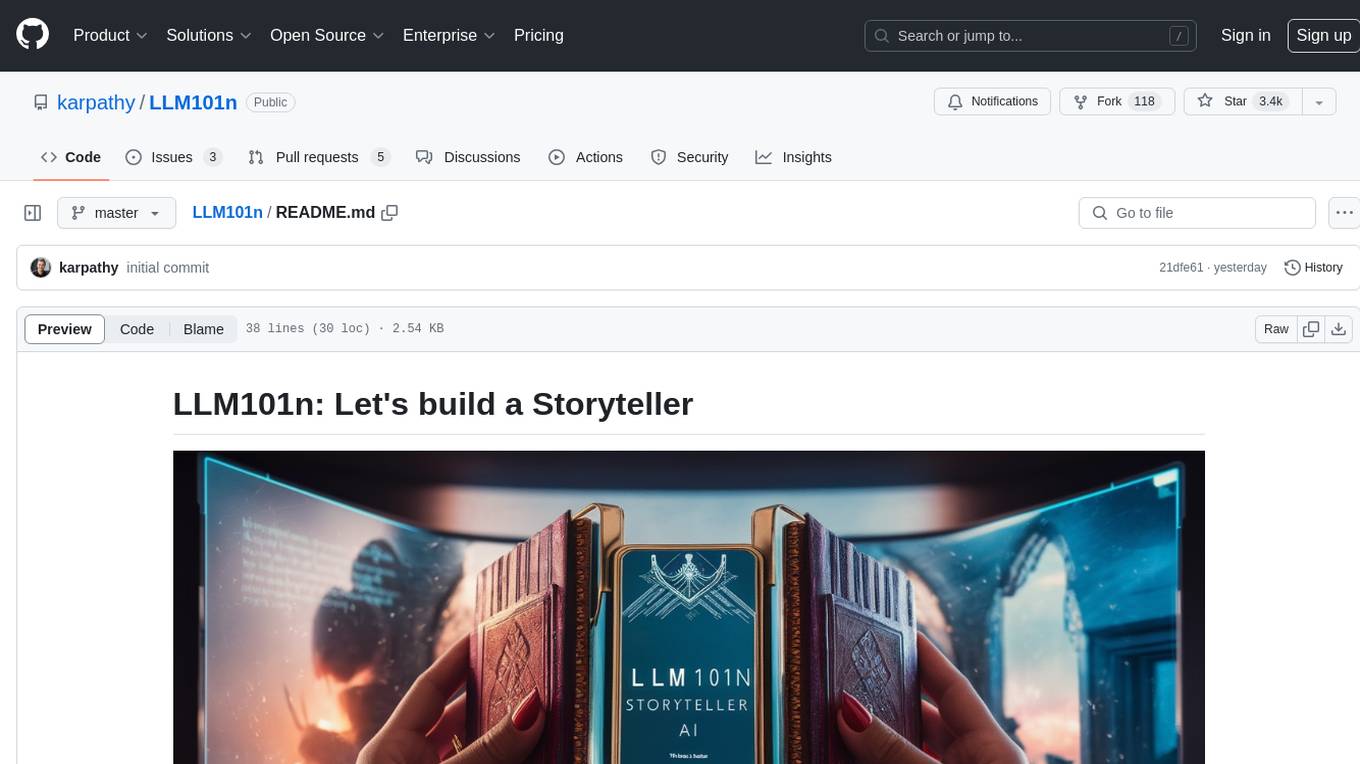
LLM101n
LLM101n is a course focused on building a Storyteller AI Large Language Model (LLM) from scratch in Python, C, and CUDA. The course covers various topics such as language modeling, machine learning, attention mechanisms, tokenization, optimization, device usage, precision training, distributed optimization, datasets, inference, finetuning, deployment, and multimodal applications. Participants will gain a deep understanding of AI, LLMs, and deep learning through hands-on projects and practical examples.
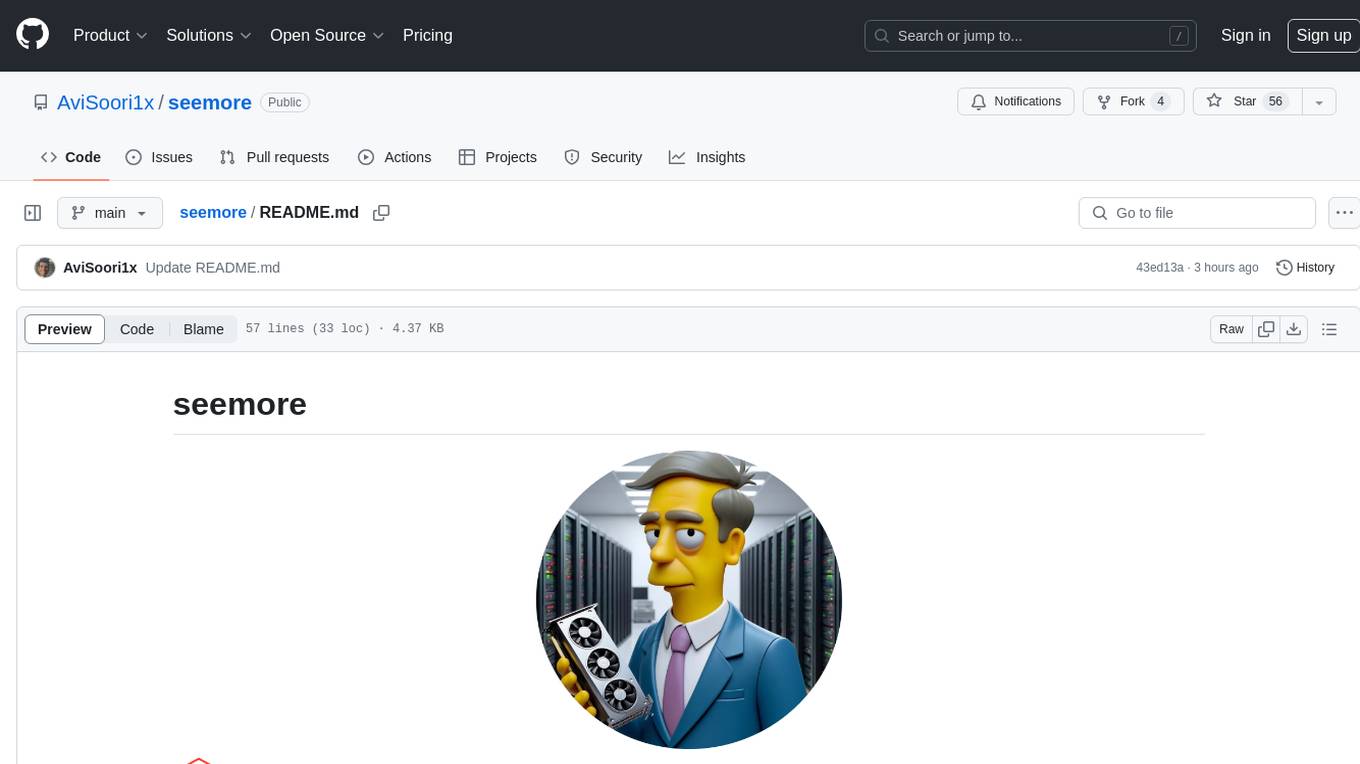
seemore
seemore is a vision language model developed in Pytorch, implementing components like image encoder, vision-language projector, and decoder language model. The model is built from scratch, including attention mechanisms and patch creation. It is designed for readability and hackability, with the intention to be improved upon. The implementation is based on public publications and borrows attention mechanism from makemore by Andrej Kapathy. The code was developed on Databricks using a single A100 for compute, and MLFlow is used for tracking metrics. The tool aims to provide a simplistic version of vision language models like Grok 1.5/GPT-4 Vision, suitable for experimentation and learning.
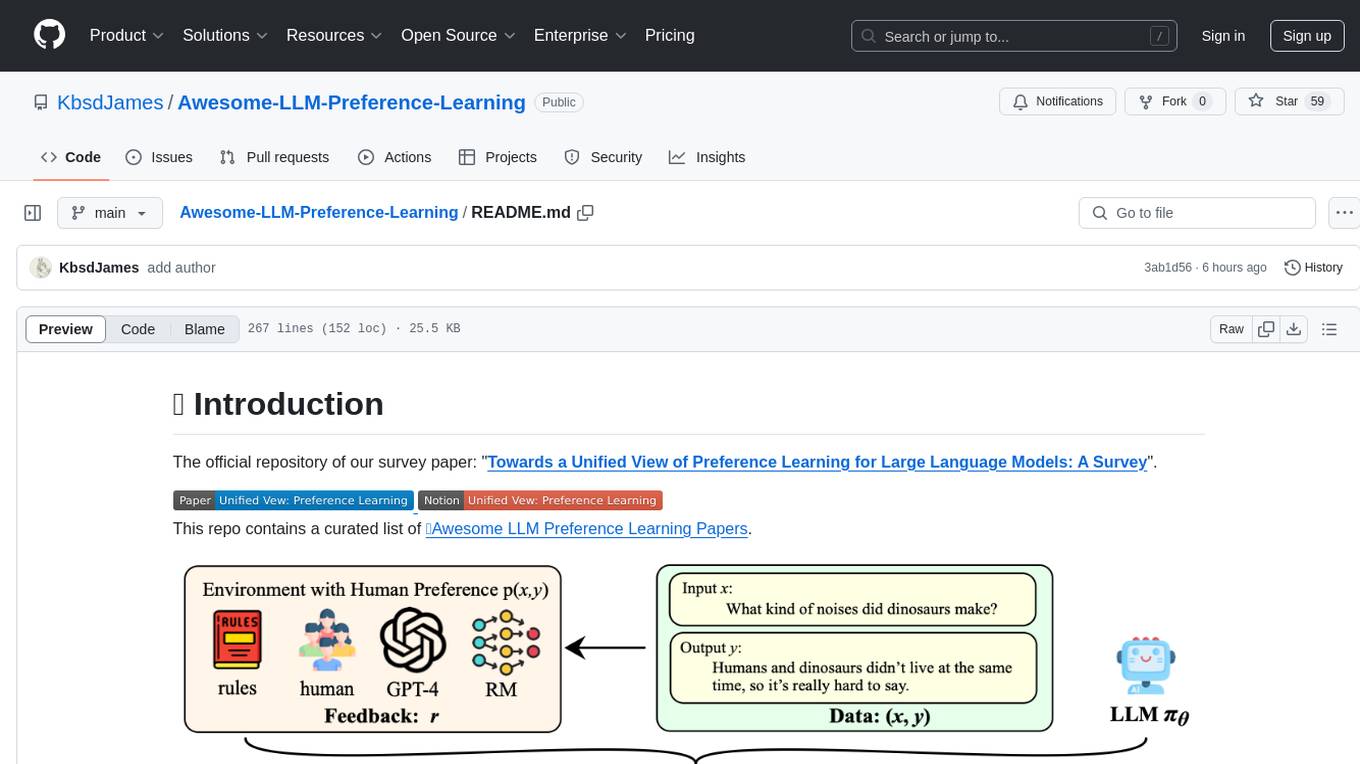
Awesome-LLM-Preference-Learning
The repository 'Awesome-LLM-Preference-Learning' is the official repository of a survey paper titled 'Towards a Unified View of Preference Learning for Large Language Models: A Survey'. It contains a curated list of papers related to preference learning for Large Language Models (LLMs). The repository covers various aspects of preference learning, including on-policy and off-policy methods, feedback mechanisms, reward models, algorithms, evaluation techniques, and more. The papers included in the repository explore different approaches to aligning LLMs with human preferences, improving mathematical reasoning in LLMs, enhancing code generation, and optimizing language model performance.
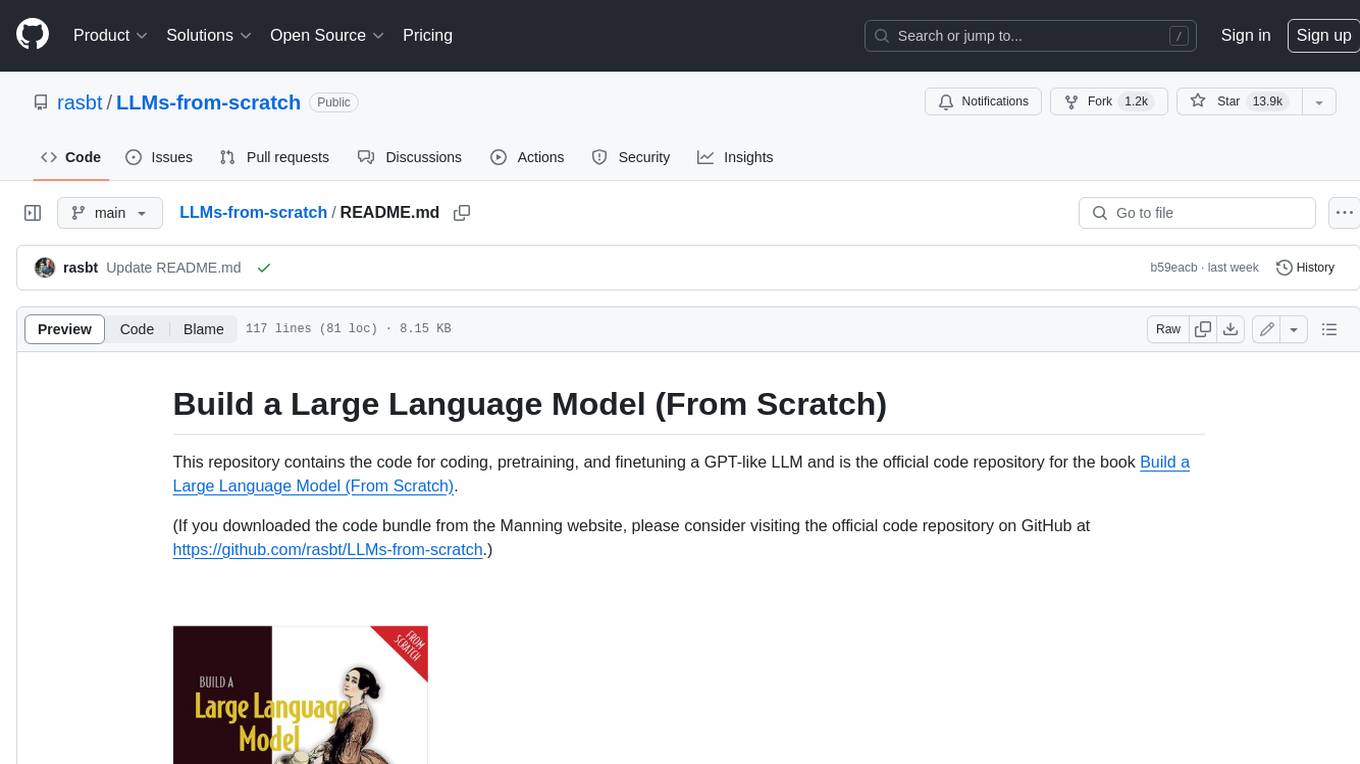
LLMs-from-scratch
This repository contains the code for coding, pretraining, and finetuning a GPT-like LLM and is the official code repository for the book Build a Large Language Model (From Scratch). In _Build a Large Language Model (From Scratch)_, you'll discover how LLMs work from the inside out. In this book, I'll guide you step by step through creating your own LLM, explaining each stage with clear text, diagrams, and examples. The method described in this book for training and developing your own small-but-functional model for educational purposes mirrors the approach used in creating large-scale foundational models such as those behind ChatGPT.
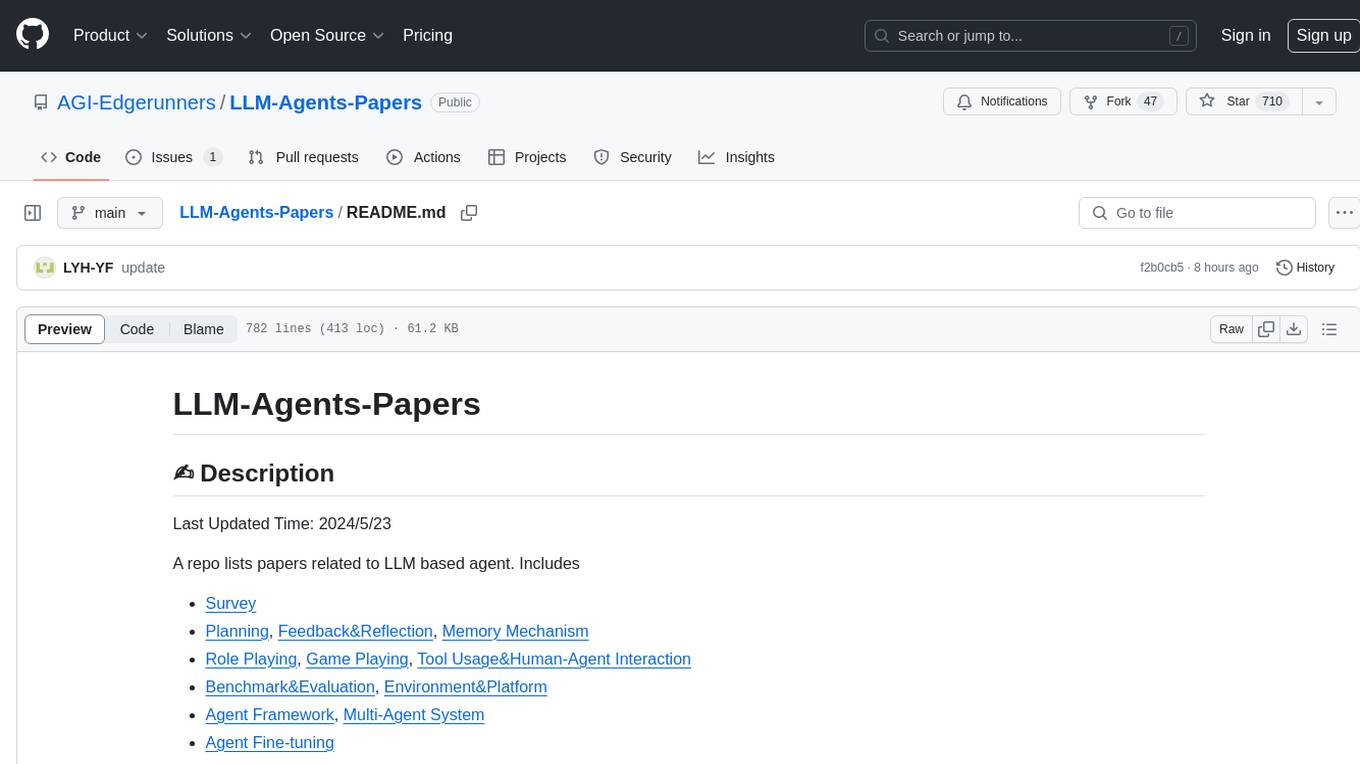
LLM-Agents-Papers
A repository that lists papers related to Large Language Model (LLM) based agents. The repository covers various topics including survey, planning, feedback & reflection, memory mechanism, role playing, game playing, tool usage & human-agent interaction, benchmark & evaluation, environment & platform, agent framework, multi-agent system, and agent fine-tuning. It provides a comprehensive collection of research papers on LLM-based agents, exploring different aspects of AI agent architectures and applications.
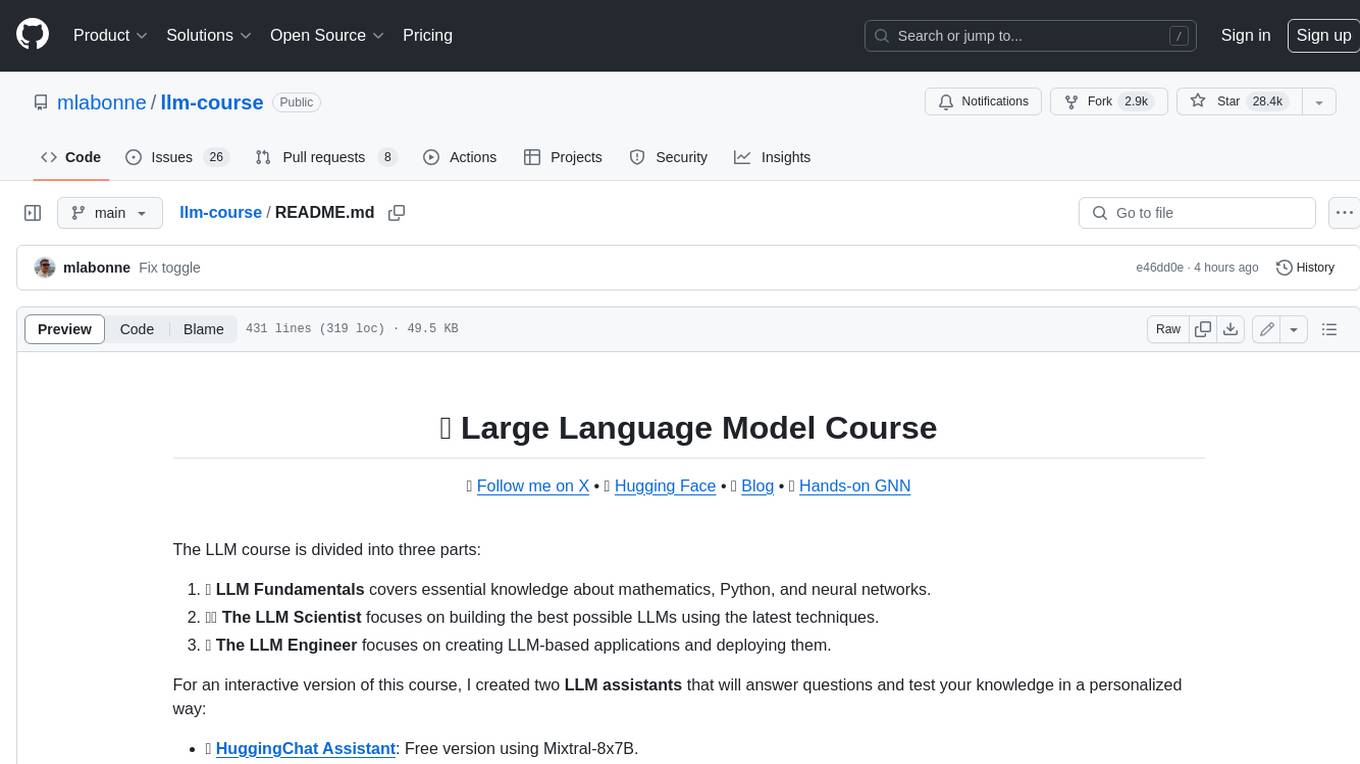
llm-course
The LLM course is divided into three parts: 1. 🧩 **LLM Fundamentals** covers essential knowledge about mathematics, Python, and neural networks. 2. 🧑🔬 **The LLM Scientist** focuses on building the best possible LLMs using the latest techniques. 3. 👷 **The LLM Engineer** focuses on creating LLM-based applications and deploying them. For an interactive version of this course, I created two **LLM assistants** that will answer questions and test your knowledge in a personalized way: * 🤗 **HuggingChat Assistant**: Free version using Mixtral-8x7B. * 🤖 **ChatGPT Assistant**: Requires a premium account. ## 📝 Notebooks A list of notebooks and articles related to large language models. ### Tools | Notebook | Description | Notebook | |----------|-------------|----------| | 🧐 LLM AutoEval | Automatically evaluate your LLMs using RunPod |  | | 🥱 LazyMergekit | Easily merge models using MergeKit in one click. |  | | 🦎 LazyAxolotl | Fine-tune models in the cloud using Axolotl in one click. |  | | ⚡ AutoQuant | Quantize LLMs in GGUF, GPTQ, EXL2, AWQ, and HQQ formats in one click. |  | | 🌳 Model Family Tree | Visualize the family tree of merged models. |  | | 🚀 ZeroSpace | Automatically create a Gradio chat interface using a free ZeroGPU. |  |
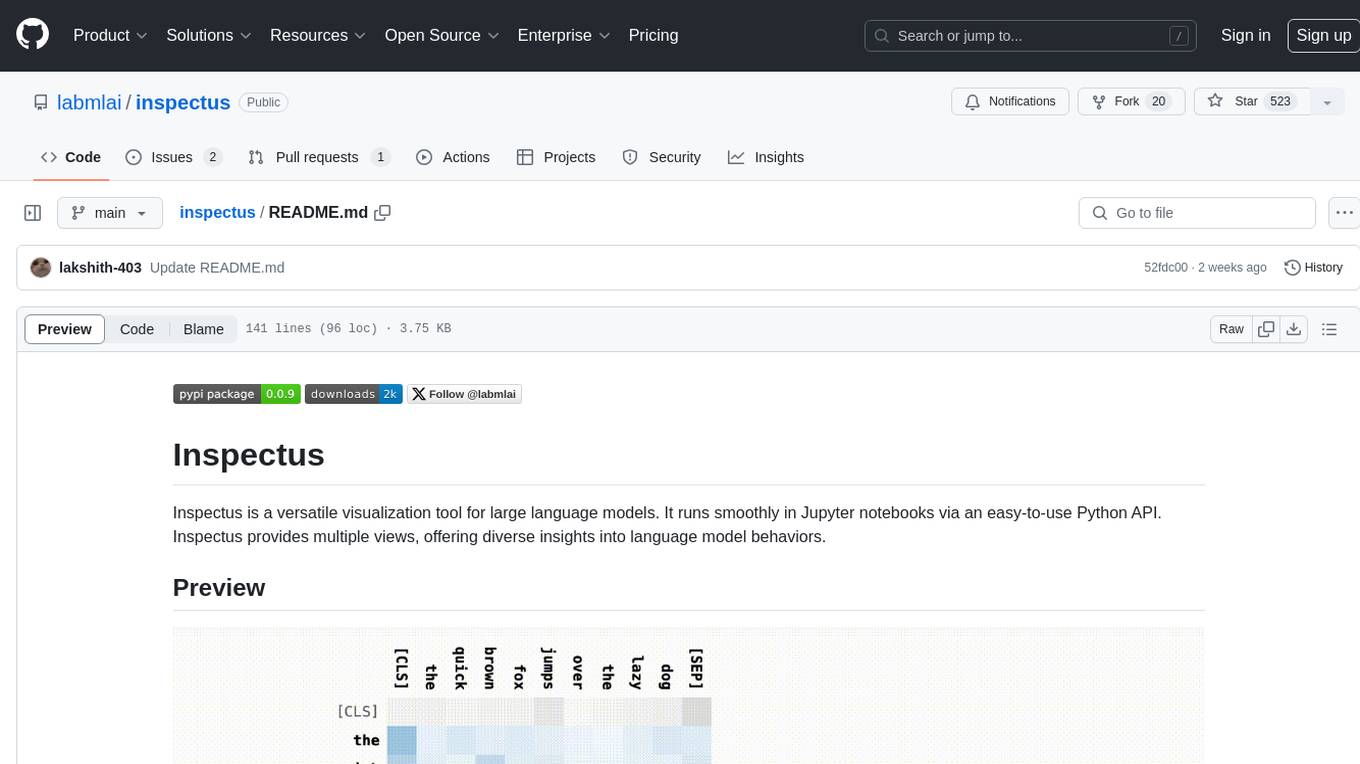
inspectus
Inspectus is a versatile visualization tool for large language models. It provides multiple views, including Attention Matrix, Query Token Heatmap, Key Token Heatmap, and Dimension Heatmap, to offer insights into language model behaviors. Users can interact with the tool in Jupyter notebooks through an easy-to-use Python API. Inspectus allows users to visualize attention scores between tokens, analyze how tokens focus on each other during processing, and explore the relationships between query and key tokens. The tool supports the visualization of attention maps from Huggingface transformers and custom attention maps, making it a valuable resource for researchers and developers working with language models.
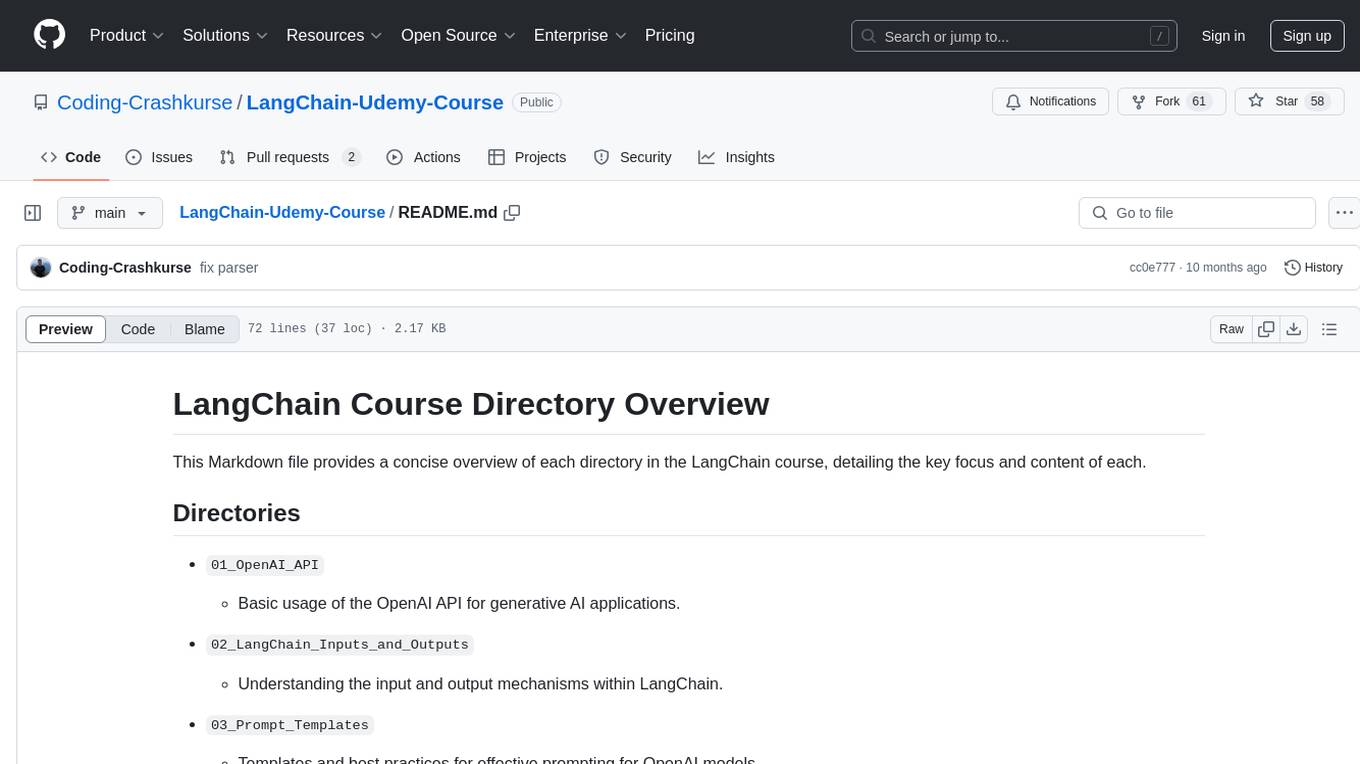
LangChain-Udemy-Course
LangChain-Udemy-Course is a comprehensive course directory focusing on LangChain, a framework for generative AI applications. The course covers various aspects such as OpenAI API usage, prompt templates, Chains exploration, callback functions, memory techniques, RAG implementation, autonomous agents, hybrid search, LangSmith utilization, microservice architecture, and LangChain Expression Language. Learners gain theoretical knowledge and practical insights to understand and apply LangChain effectively in generative AI scenarios.
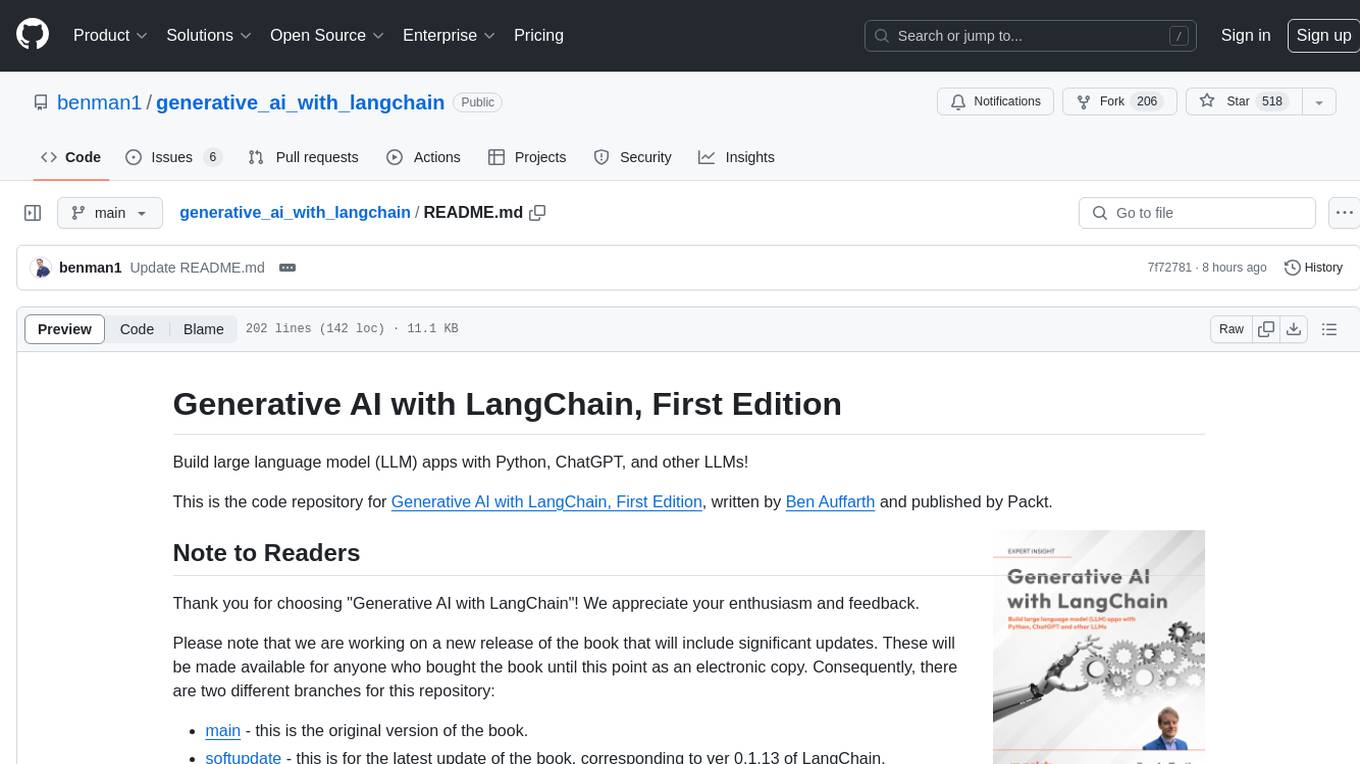
generative_ai_with_langchain
Generative AI with LangChain is a code repository for building large language model (LLM) apps with Python, ChatGPT, and other LLMs. The repository provides code examples, instructions, and configurations for creating generative AI applications using the LangChain framework. It covers topics such as setting up the development environment, installing dependencies with Conda or Pip, using Docker for environment setup, and setting API keys securely. The repository also emphasizes stability, code updates, and user engagement through issue reporting and feedback. It aims to empower users to leverage generative AI technologies for tasks like building chatbots, question-answering systems, software development aids, and data analysis applications.
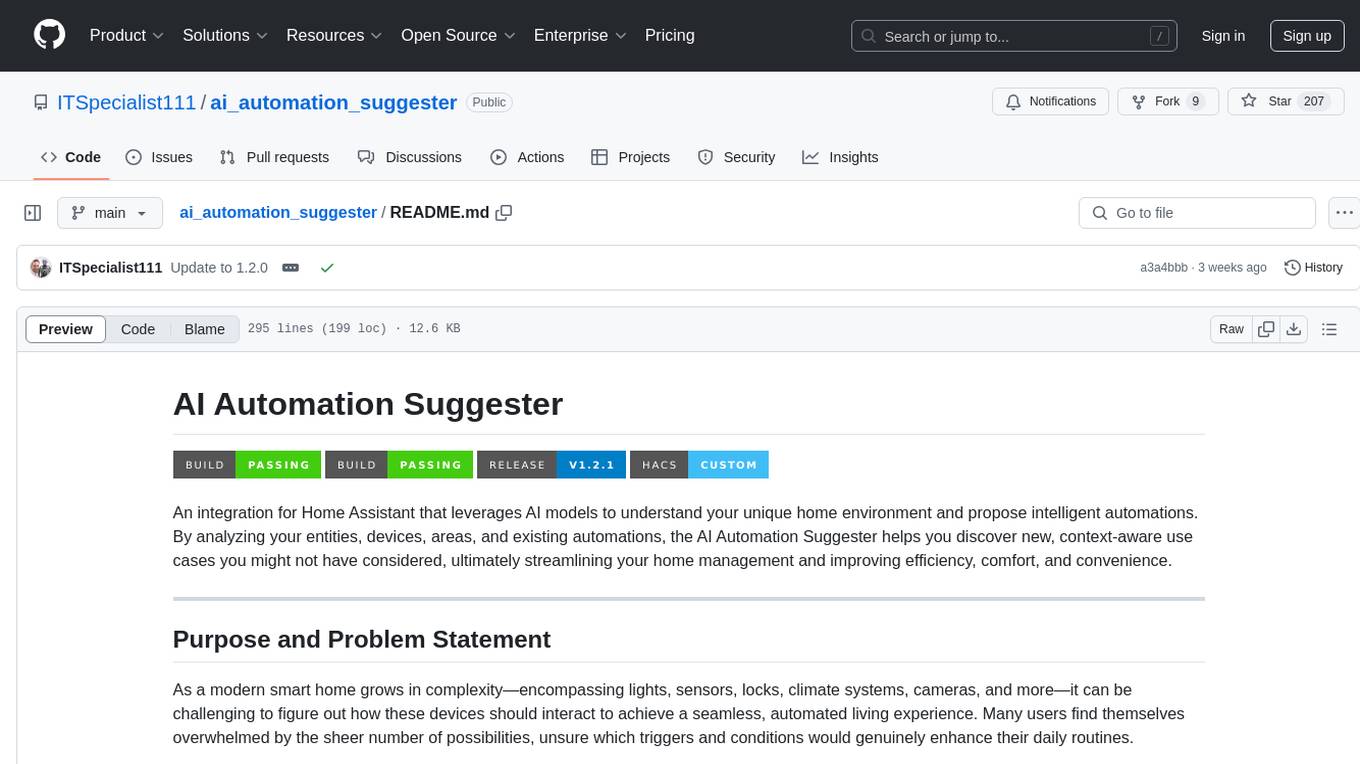
ai_automation_suggester
An integration for Home Assistant that leverages AI models to understand your unique home environment and propose intelligent automations. By analyzing your entities, devices, areas, and existing automations, the AI Automation Suggester helps you discover new, context-aware use cases you might not have considered, ultimately streamlining your home management and improving efficiency, comfort, and convenience. The tool acts as a personal automation consultant, providing actionable YAML-based automations that can save energy, improve security, enhance comfort, and reduce manual intervention. It turns the complexity of a large Home Assistant environment into actionable insights and tangible benefits.
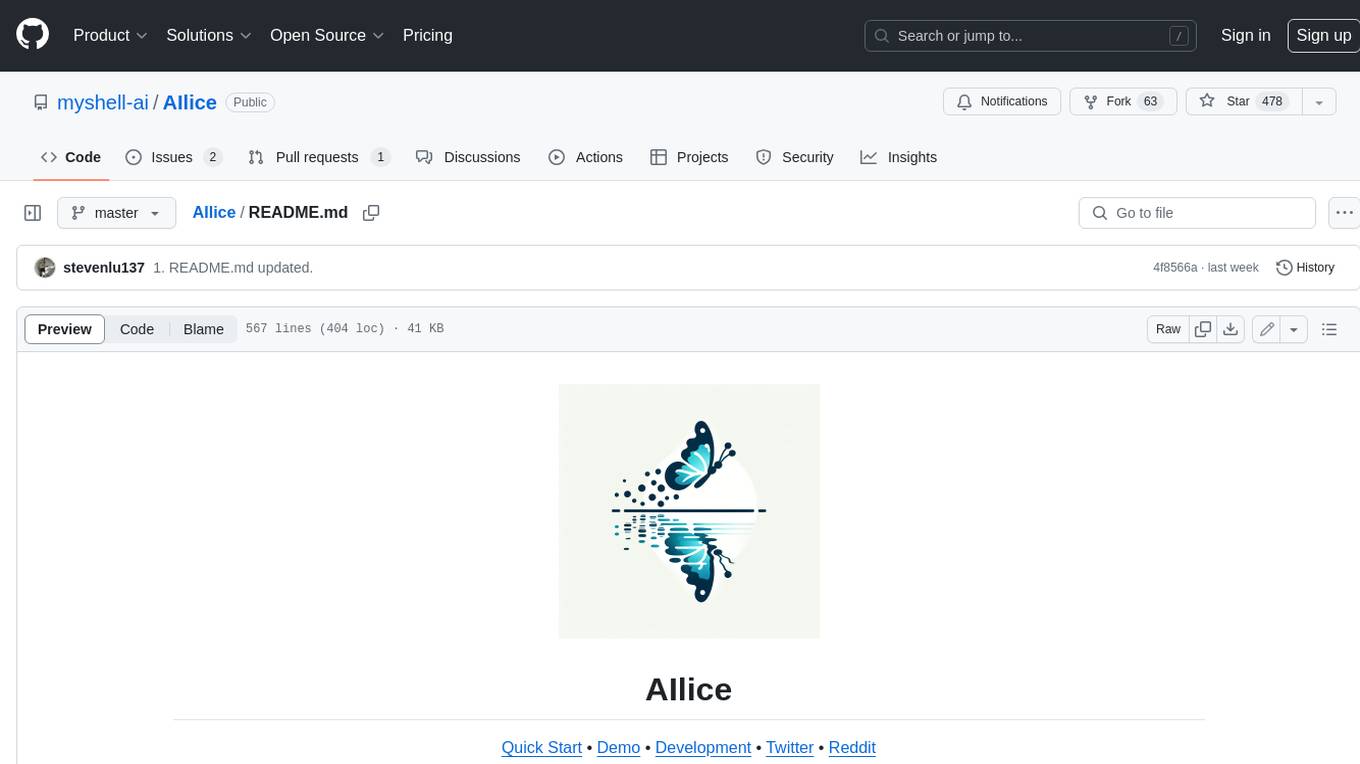
AIlice
AIlice is a fully autonomous, general-purpose AI agent that aims to create a standalone artificial intelligence assistant, similar to JARVIS, based on the open-source LLM. AIlice achieves this goal by building a "text computer" that uses a Large Language Model (LLM) as its core processor. Currently, AIlice demonstrates proficiency in a range of tasks, including thematic research, coding, system management, literature reviews, and complex hybrid tasks that go beyond these basic capabilities. AIlice has reached near-perfect performance in everyday tasks using GPT-4 and is making strides towards practical application with the latest open-source models. We will ultimately achieve self-evolution of AI agents. That is, AI agents will autonomously build their own feature expansions and new types of agents, unleashing LLM's knowledge and reasoning capabilities into the real world seamlessly.
20 - OpenAI Gpts
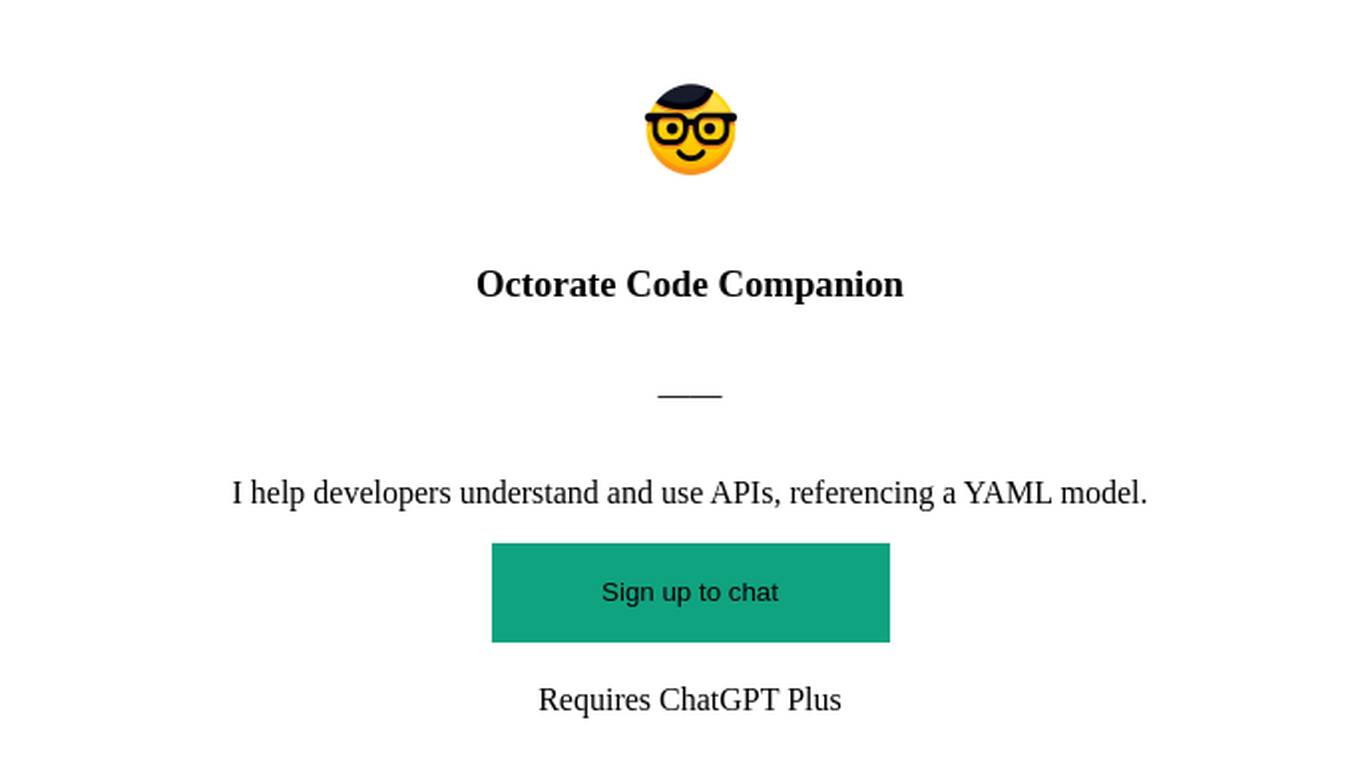
Octorate Code Companion
I help developers understand and use APIs, referencing a YAML model.
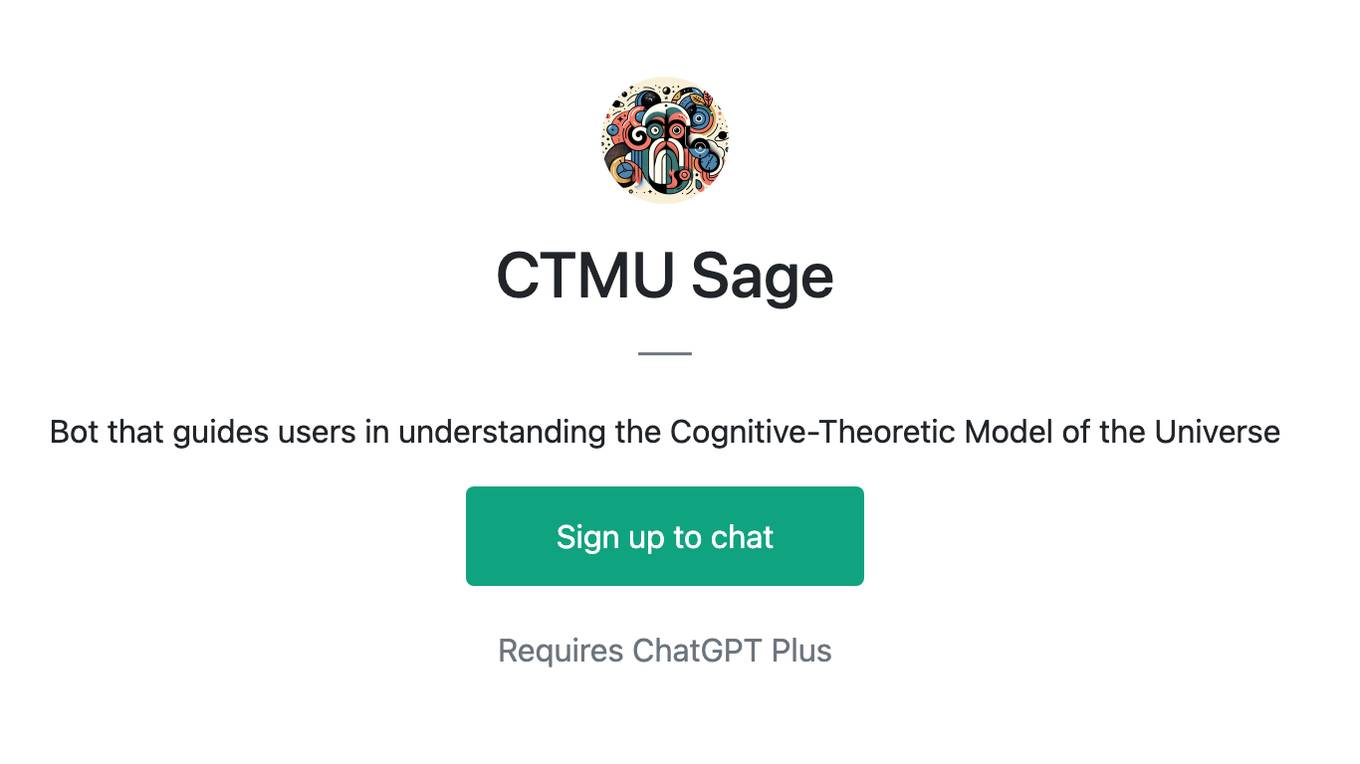
CTMU Sage
Bot that guides users in understanding the Cognitive-Theoretic Model of the Universe
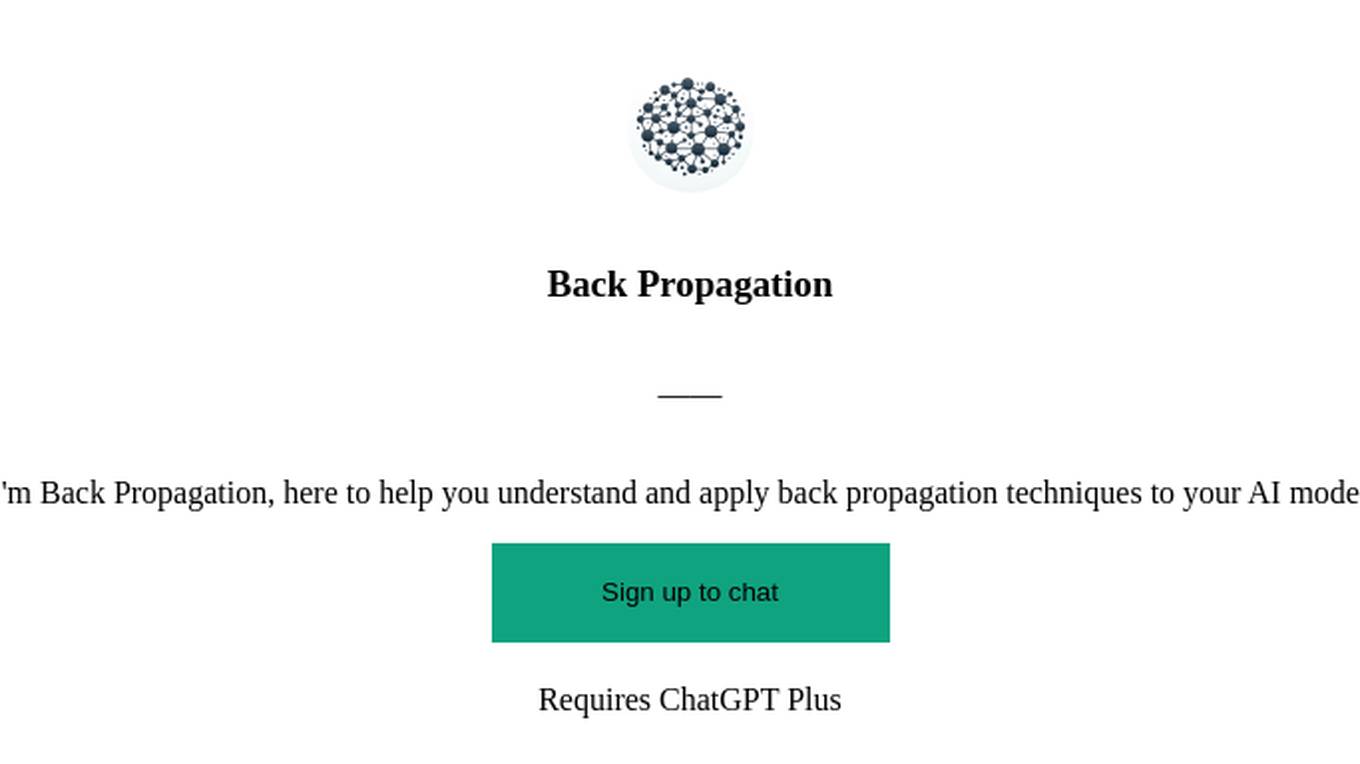
Back Propagation
I'm Back Propagation, here to help you understand and apply back propagation techniques to your AI models.
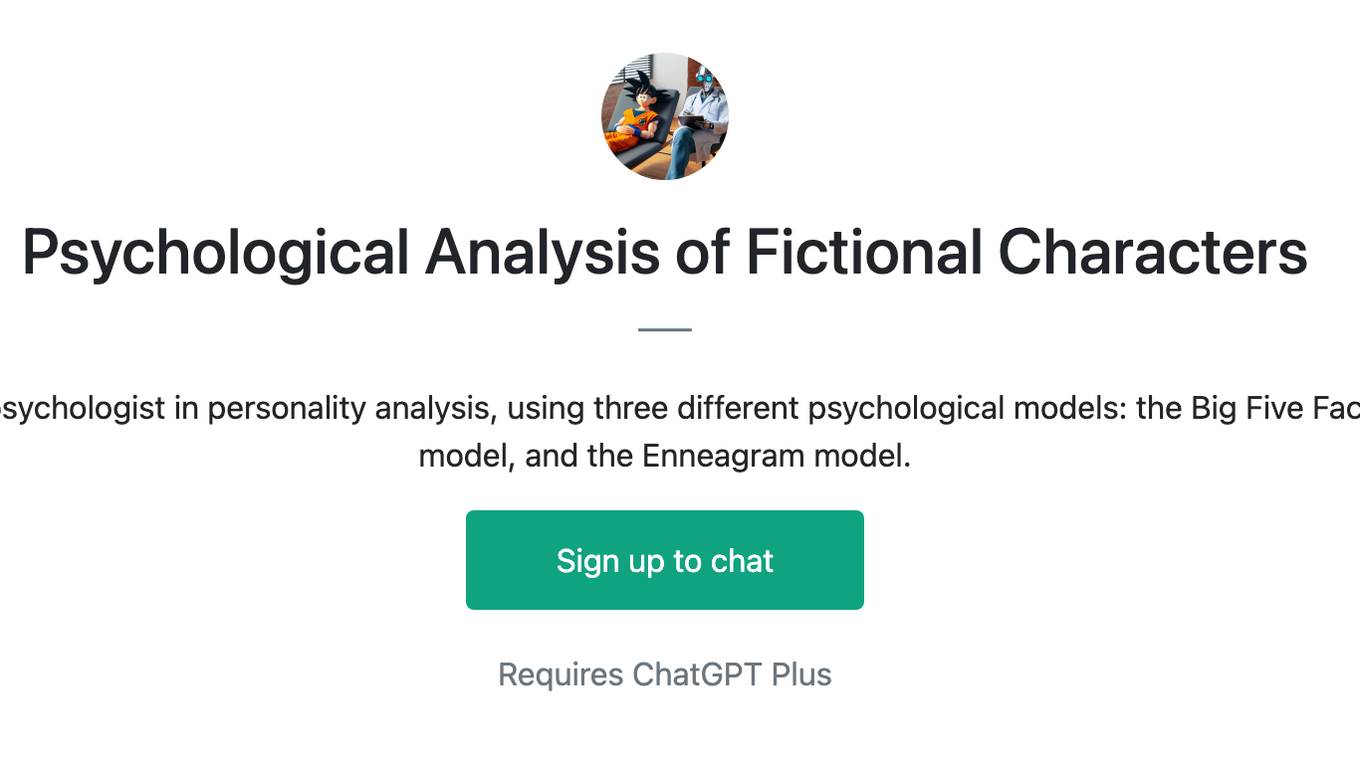
Psychological Analysis of Fictional Characters
This prompt is designed to act as an expert psychologist in personality analysis, using three different psychological models: the Big Five Factor model, the Myers-Briggs Sixteen Types model, and the Enneagram model.
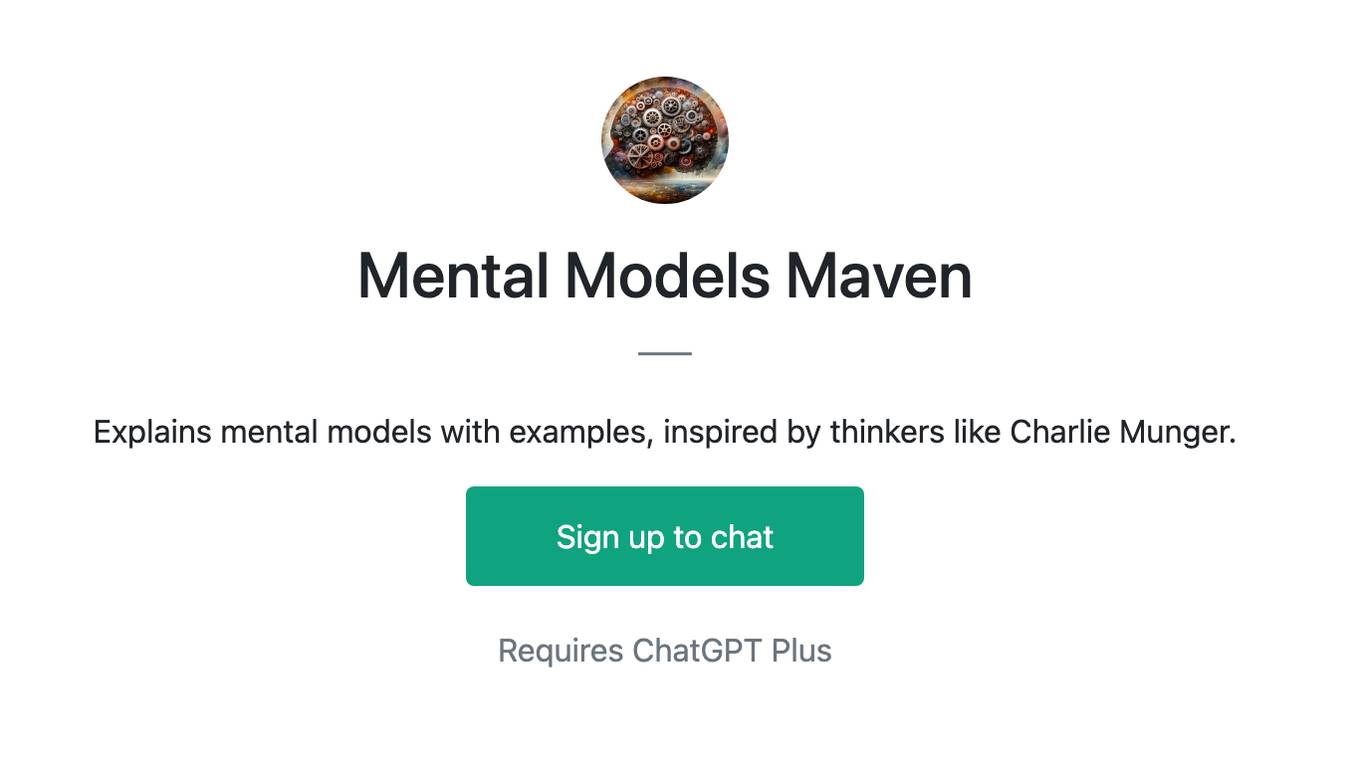
Mental Models Maven
Explains mental models with examples, inspired by thinkers like Charlie Munger.
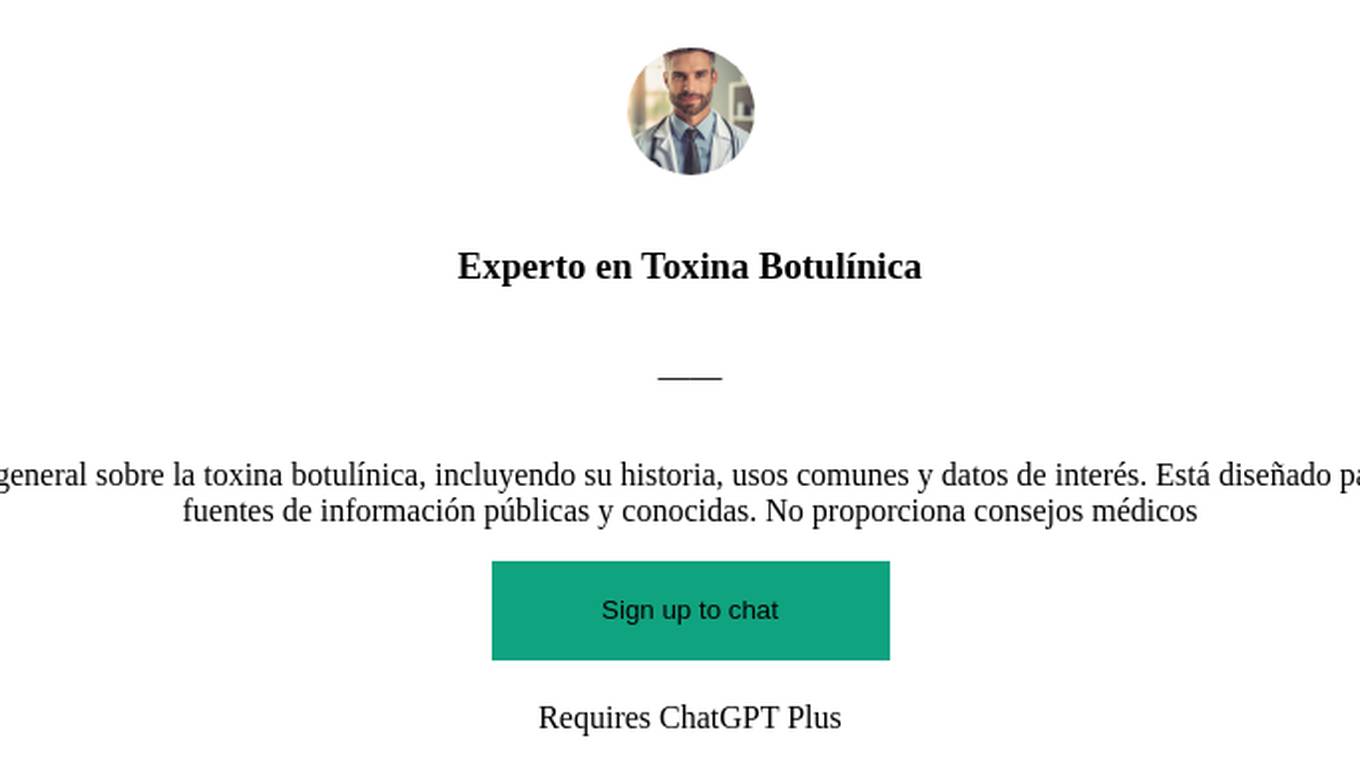
Experto en Toxina Botulínica
Este modelo de GPT proporciona información general sobre la toxina botulínica, incluyendo su historia, usos comunes y datos de interés. Está diseñado para educar y ofrecer una visión general basada en fuentes de información públicas y conocidas. No proporciona consejos médicos
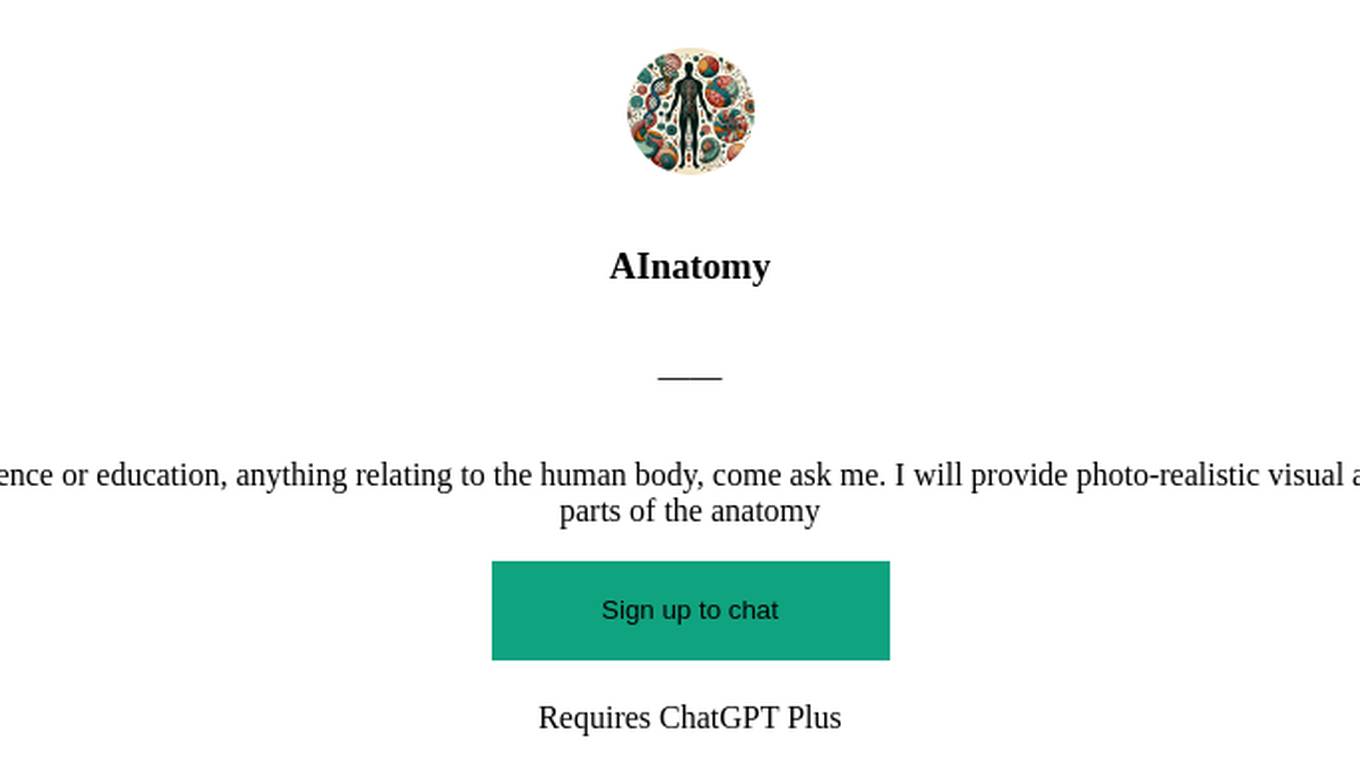
AInatomy
An expert in Human Anatomy, be it for art or science or education, anything relating to the human body, come ask me. I will provide photo-realistic visual aids and AI created models to expound on different parts of the anatomy
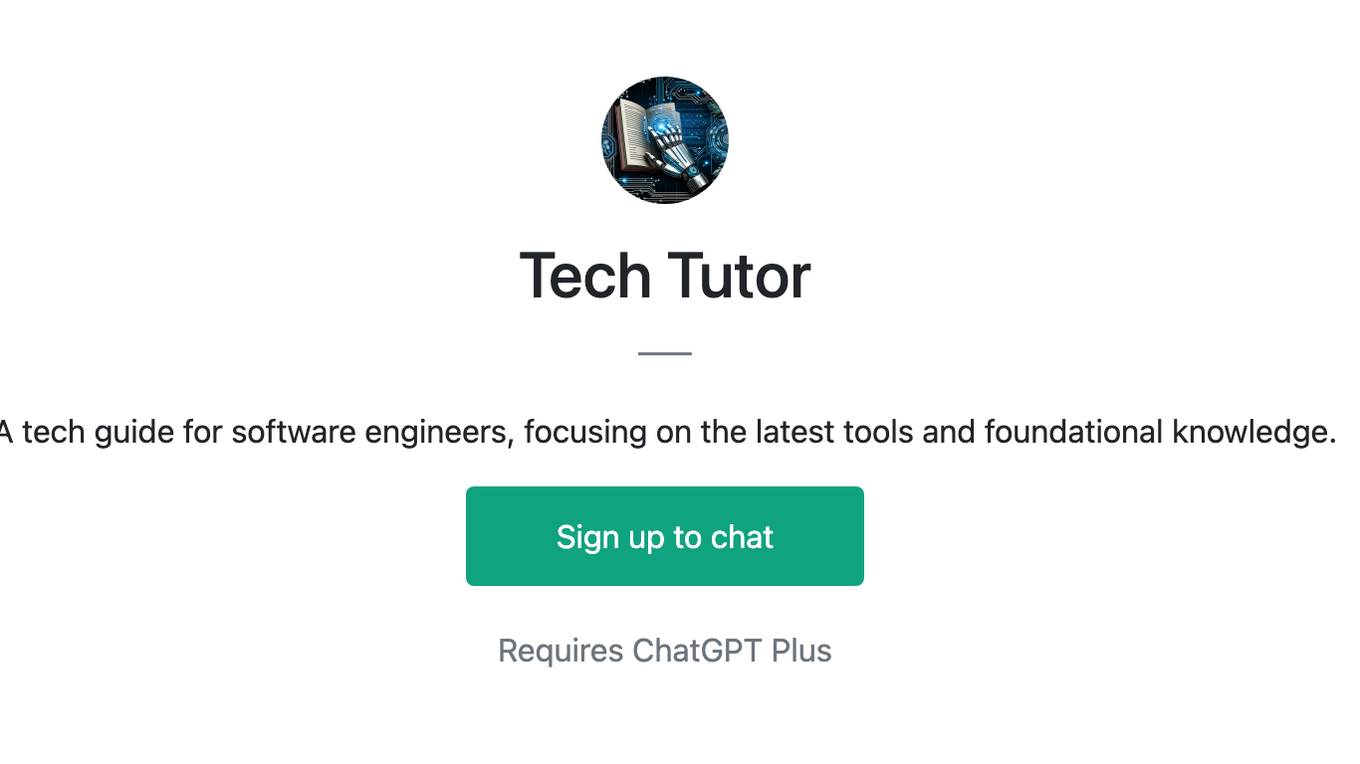
Tech Tutor
A tech guide for software engineers, focusing on the latest tools and foundational knowledge.
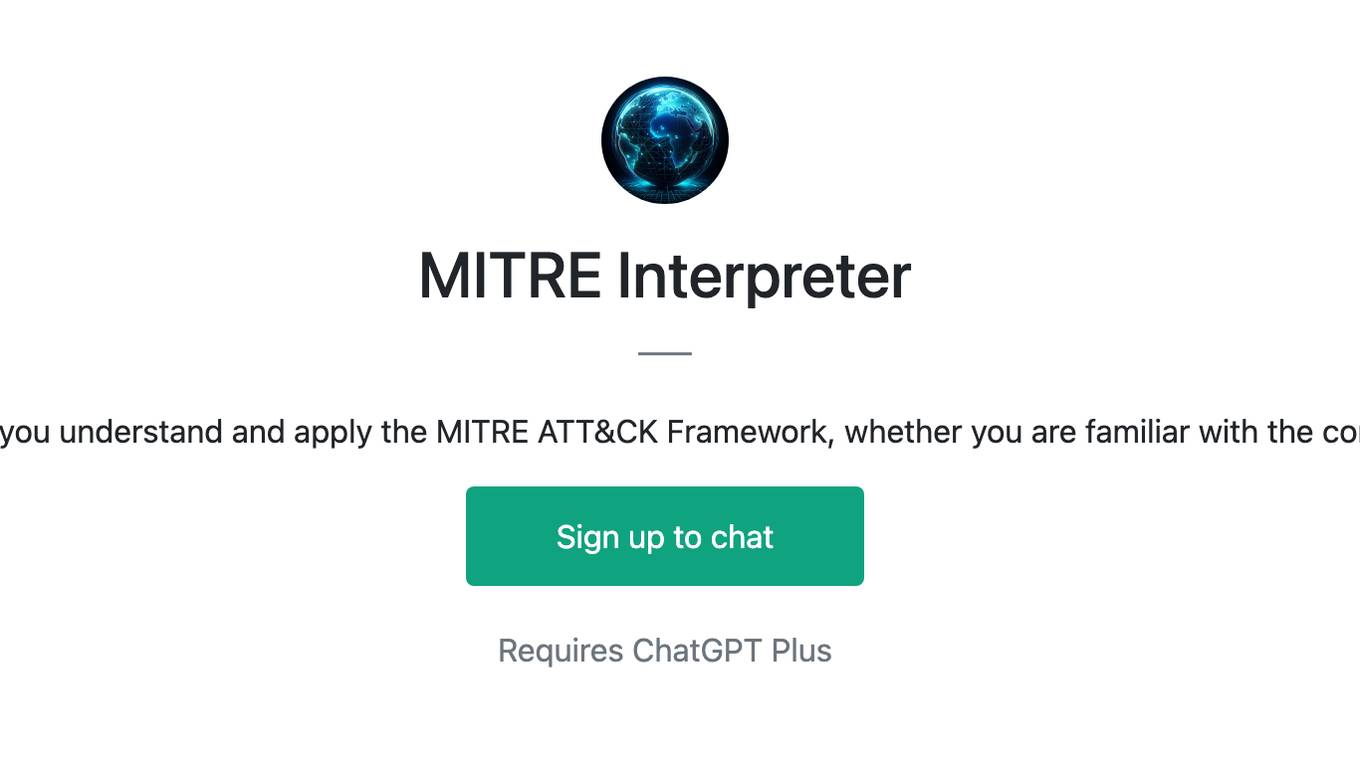
MITRE Interpreter
This GPT helps you understand and apply the MITRE ATT&CK Framework, whether you are familiar with the concepts or not.