Best AI tools for< Create Tests >
11 - AI tool Sites
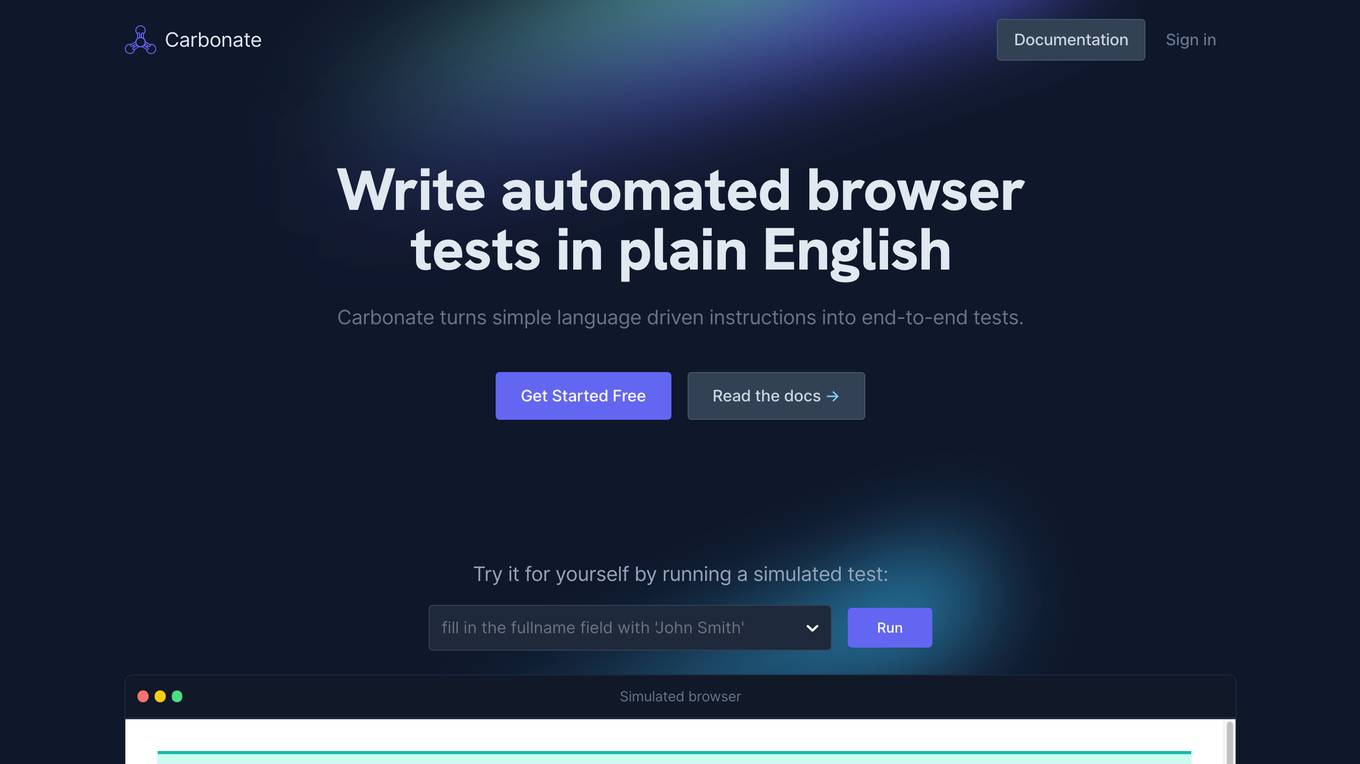
Carbonate
Carbonate is an AI-driven automated end-to-end testing tool that allows users to create auto-healing browser tests without any coding. By leveraging its unique AI engine, Carbonate generates test scripts from recorded tests, enabling users to run tests using a cloud test runner or within their own CI. With Carbonate, users can create tests in seconds by simply using their application, as the tool automatically detects interactions and records them as part of the test. Carbonate's intelligent AI recorder ensures that tests heal themselves and adapt to changes in the application, providing fast results without the hassle.
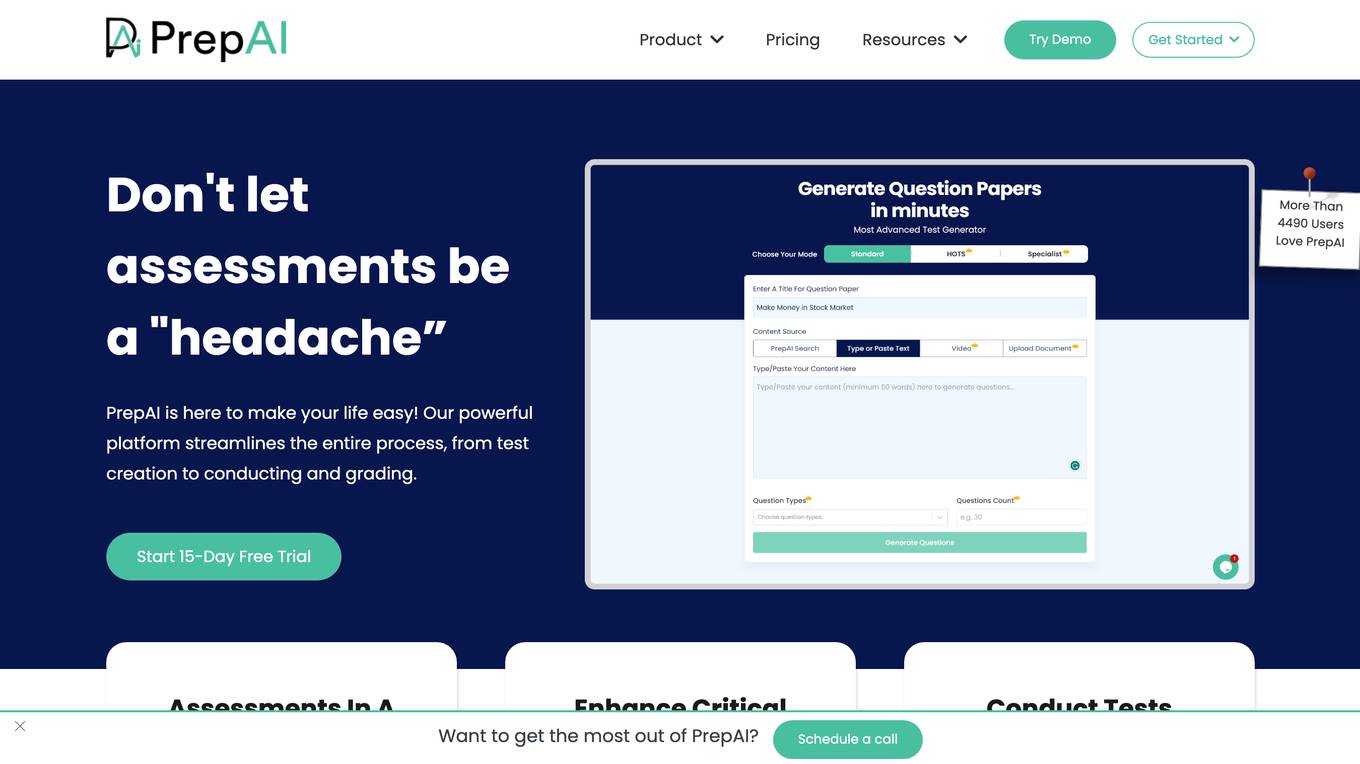
PrepAI
PrepAI is an advanced test generator that uses AI to help educators create high-quality assessments quickly and easily. With PrepAI, teachers can save time, engage students with unique questions, and prepare them for success. PrepAI offers a variety of features to make test creation easy, including multiple content input options, various question formats, and an easy-to-use dashboard. PrepAI also offers a variety of advantages for educators, including the ability to analyze higher-order thinking skills, conduct tests effortlessly, and access unlimited question sets.
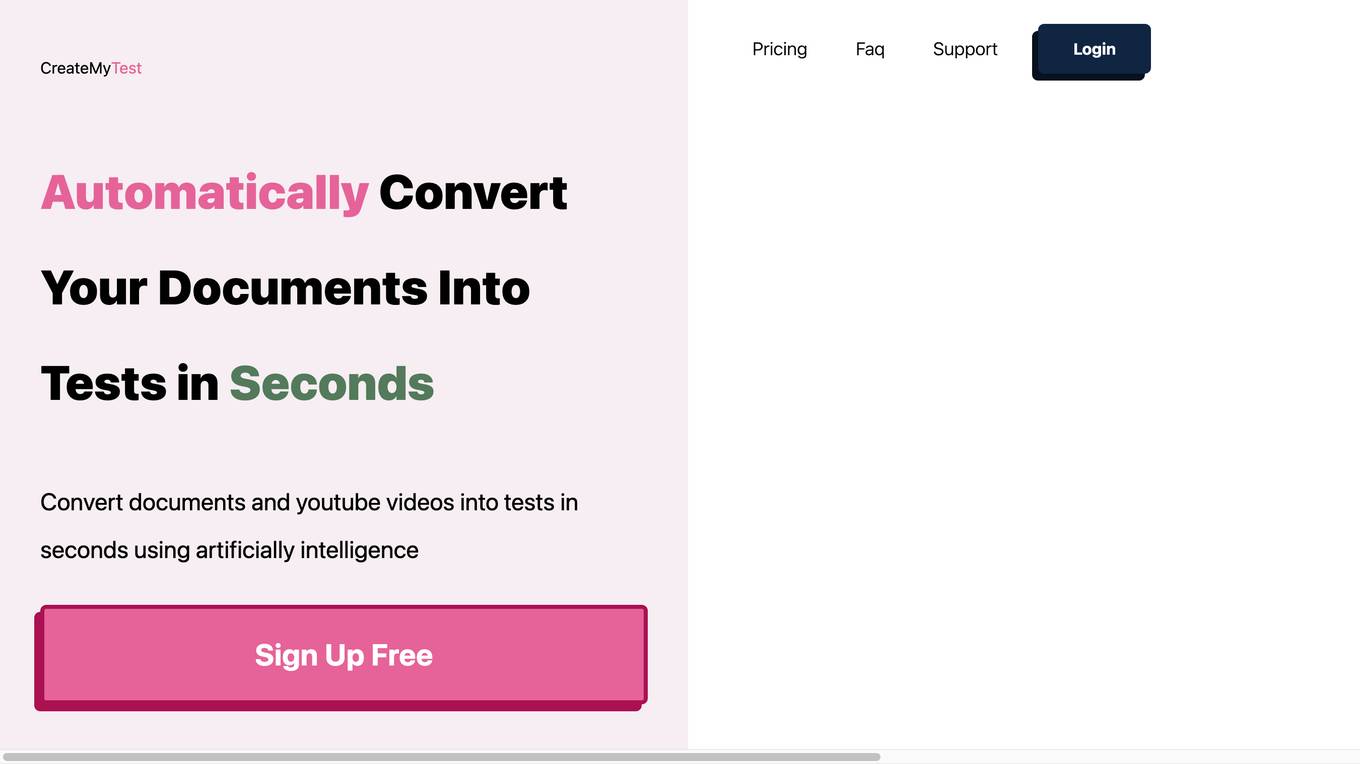
CreateMyTest
CreateMyTest is an online tool that uses artificial intelligence to automatically convert documents and YouTube videos into tests. It offers various question types, including multiple choice, true/false, matching, and fill in the blank. The platform aims to enhance studying by helping users retain knowledge through practice testing and reduce test anxiety.
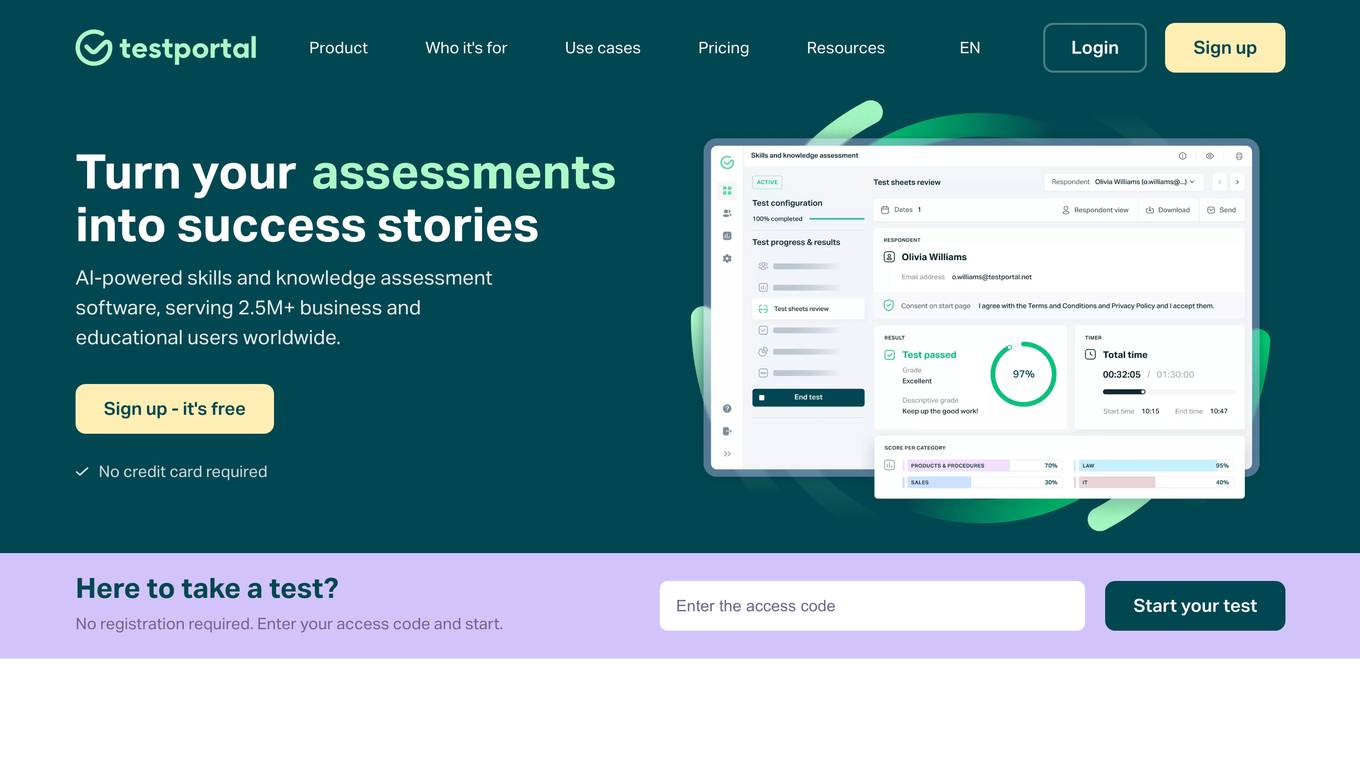
Testportal
Testportal is an online assessment platform that allows users to create their own tests, quizzes, and exams. It is used by businesses and educational institutions to assess the skills and knowledge of their employees and students. Testportal offers a variety of features, including AI-powered question generation, automatic grading, and comprehensive insights and analytics. It also integrates with Microsoft Teams and provides enterprise-grade security and data protection.
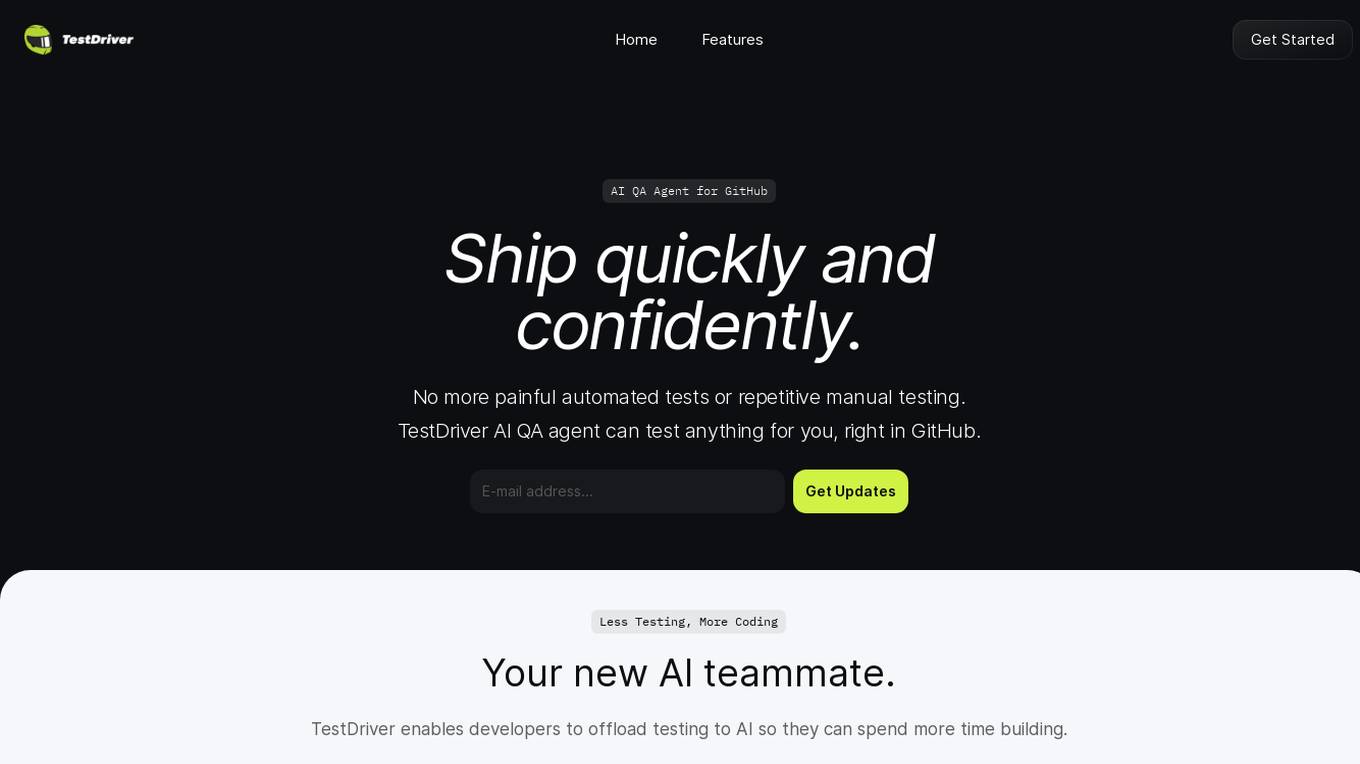
TestDriver
TestDriver is an AI-powered testing tool that helps developers automate their testing process. It can be integrated with GitHub and can test anything, right in the GitHub environment. TestDriver is easy to set up and use, and it can help developers save time and effort by offloading testing to AI. It uses Dashcam.io technology to provide end-to-end exploratory testing, allowing developers to see the screen, logs, and thought process as the AI completes its test.
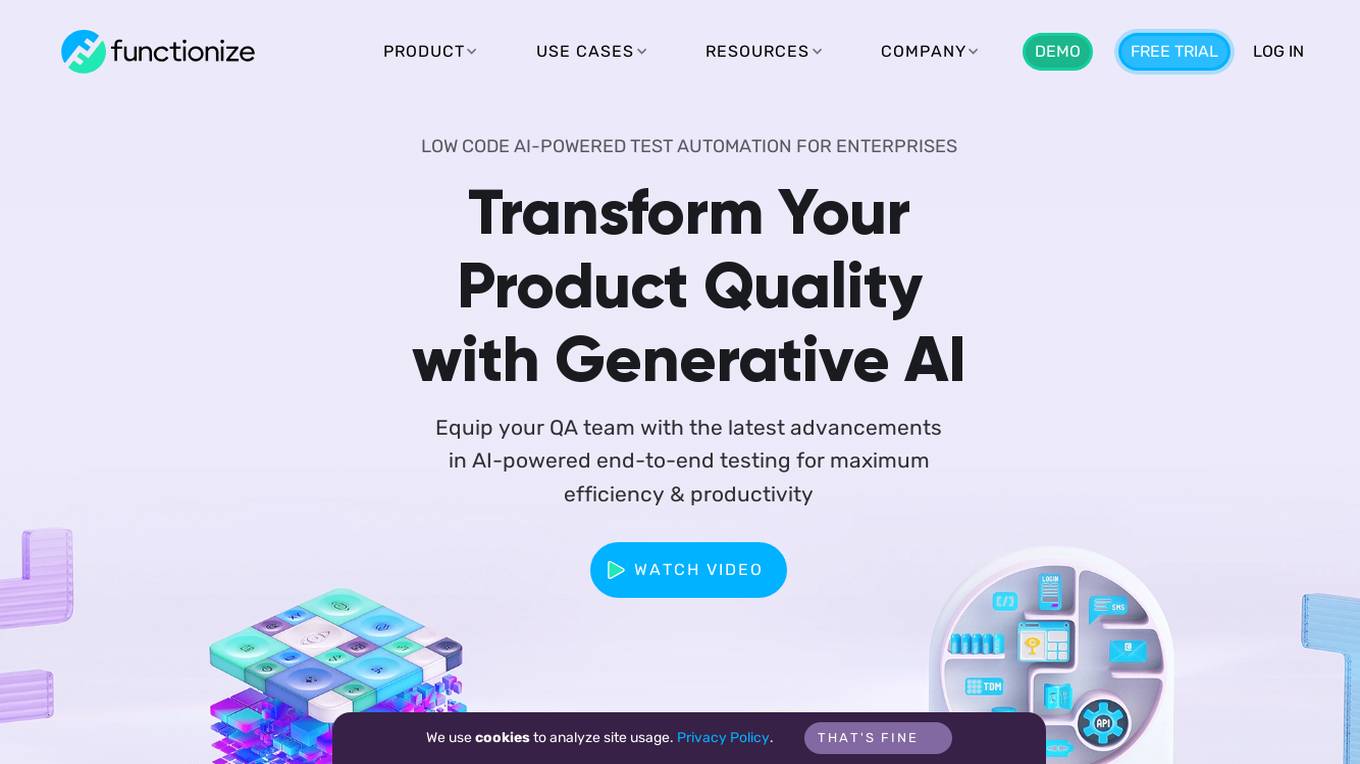
Functionize
Functionize is an AI-powered test automation platform that helps enterprises improve their product quality and release faster. It uses machine learning to automate test creation, maintenance, and execution, and provides a range of features to help teams collaborate and manage their testing process. Functionize integrates with popular CI/CD tools and DevOps pipelines, and offers a range of pricing options to suit different needs.
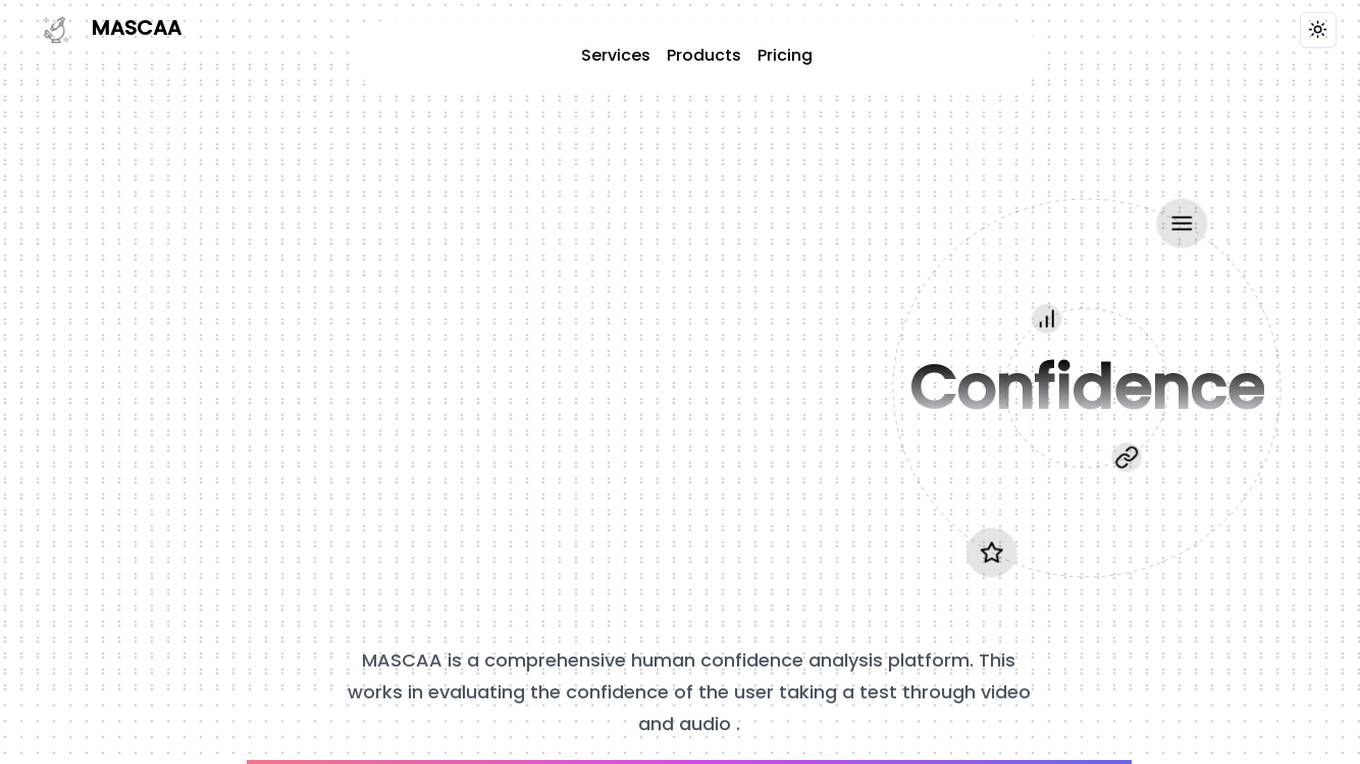
MASCAA
MASCAA is a comprehensive human confidence analysis platform that focuses on evaluating the confidence of users through video and audio during various tasks. It integrates advanced facial expression and voice analysis technologies to provide valuable feedback for students, instructors, individuals, businesses, and teams. MASCAA offers quick and easy test creation, evaluation, and confidence assessment for educational settings, personal use, startups, small organizations, universities, and large organizations. The platform aims to unlock long-term value and enhance customer experience by helping users assess and improve their confidence levels.
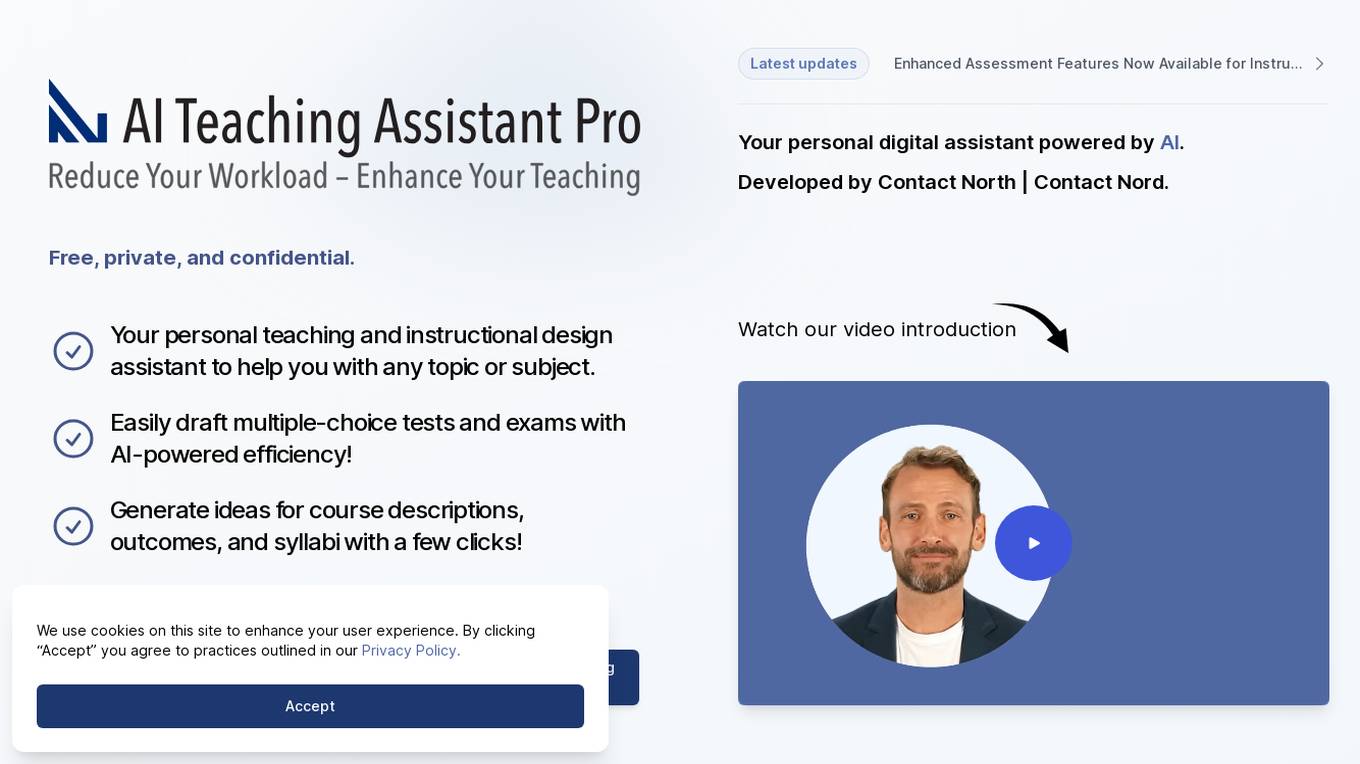
AI Teaching Assistant Pro
AI Teaching Assistant Pro is a free, private, and confidential AI-powered tool developed by Contact North | Contact Nord. It serves as a personal teaching and instructional design assistant to help instructors with various tasks related to course creation and assessment. The tool offers enhanced assessment features, including the ability to easily draft multiple-choice tests and exams with AI-powered efficiency. Instructors can also generate ideas for course descriptions, outcomes, and syllabi with just a few clicks. AI Teaching Assistant Pro aims to streamline the teaching process and provide valuable support to educators.
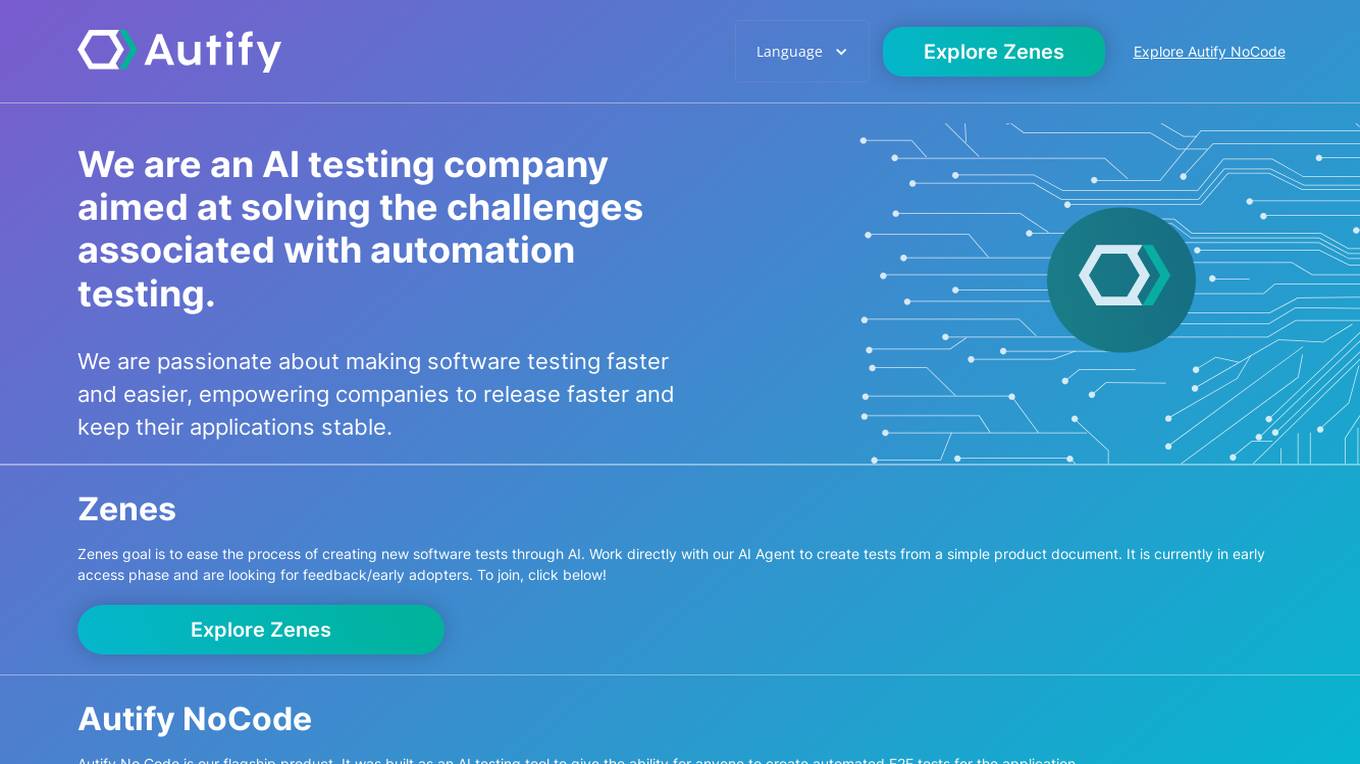
Autify
Autify is an AI testing company focused on solving challenges in automation testing. They aim to make software testing faster and easier, enabling companies to release faster and maintain application stability. Their flagship product, Autify No Code, allows anyone to create automated end-to-end tests for applications. Zenes, their new product, simplifies the process of creating new software tests through AI. Autify is dedicated to innovation in the automation testing space and is trusted by leading organizations.
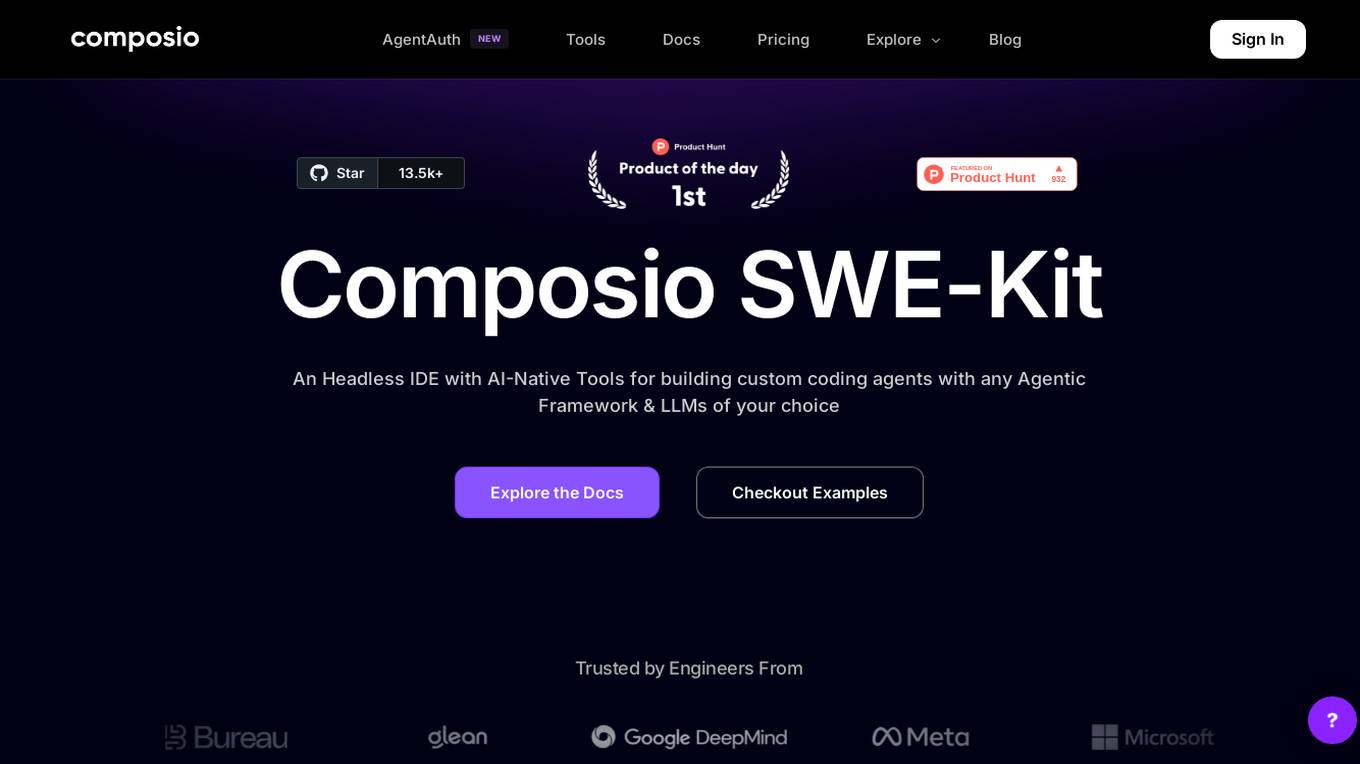
SWE Kit
SWE Kit is an open-source headless IDE designed for building custom coding agents with state-of-the-art performance. It offers AI-native tools to streamline the coding review process, enhance code quality, and optimize development efficiency. The application supports various agentic frameworks and LLM inference providers, providing a flexible runtime environment for seamless codebase interaction. With features like code analysis, code indexing, and third-party service integrations, SWE Kit empowers developers to create and run coding agents effortlessly.
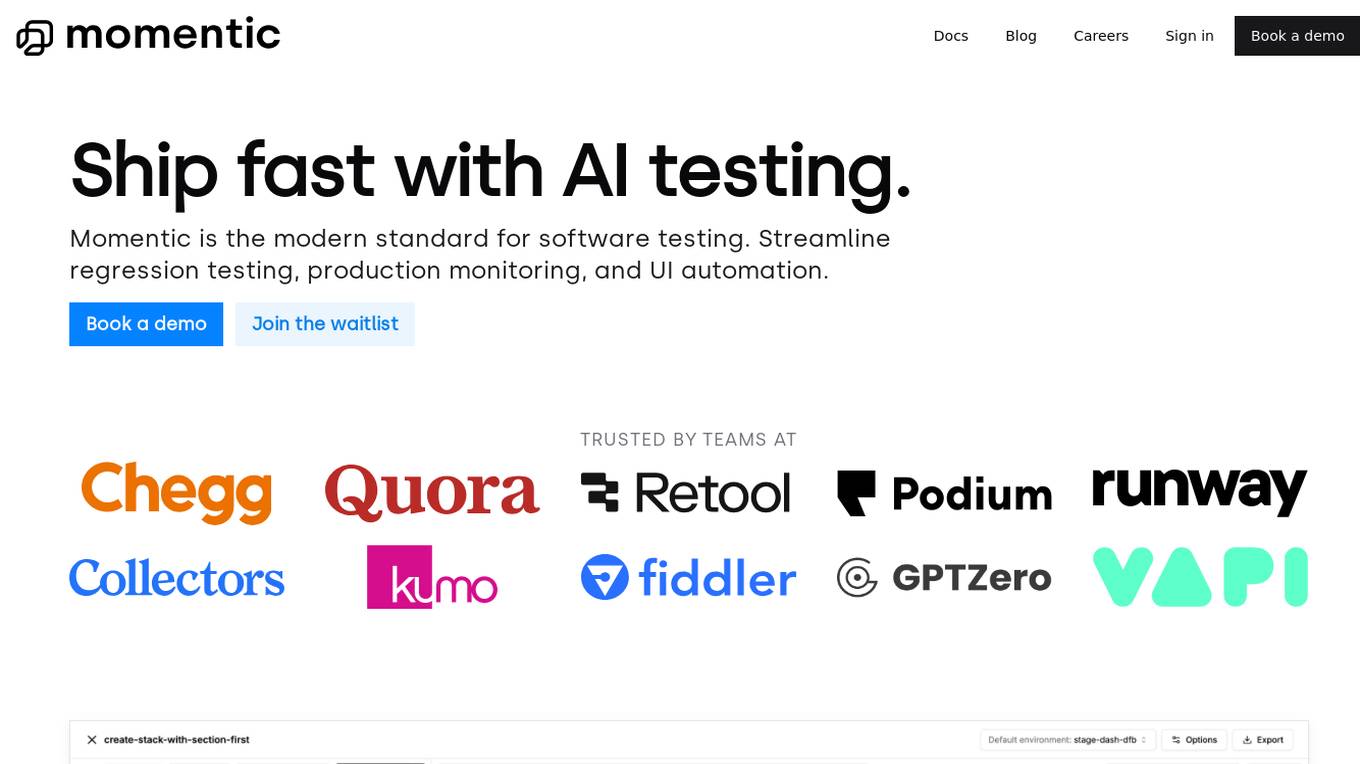
Momentic
Momentic is an AI testing tool that offers automated AI testing for software applications. It streamlines regression testing, production monitoring, and UI automation, making test automation easy with its AI capabilities. Momentic is designed to be simple to set up, easy to maintain, and accelerates team productivity by creating and deploying tests faster with its intuitive low-code editor. The tool adapts to applications, saves time with automated test maintenance, and allows testing anywhere, anytime using cloud, local, or CI/CD pipelines.
20 - Open Source AI Tools
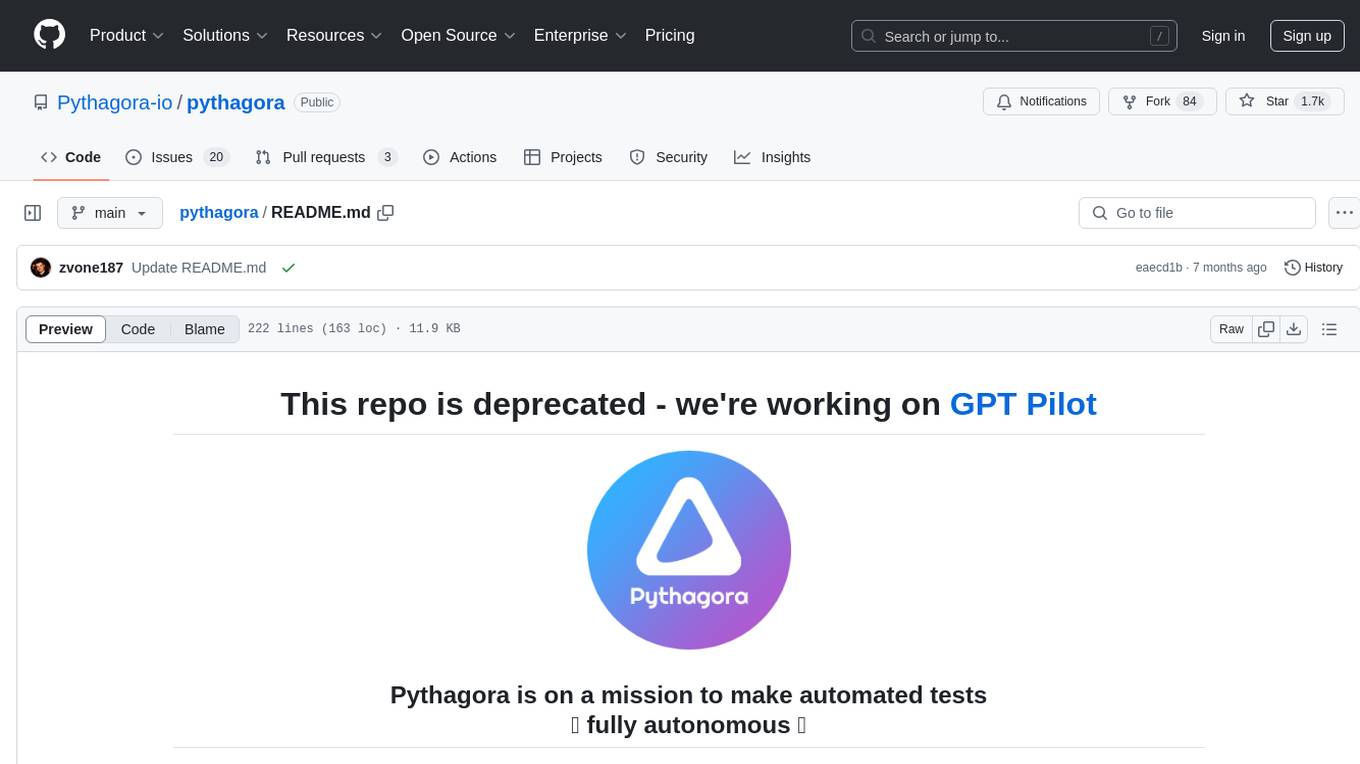
pythagora
Pythagora is an automated testing tool designed to generate unit tests using GPT-4. By running a single command, users can create tests for specific functions in their codebase. The tool leverages AST parsing to identify related functions and sends them to the Pythagora server for test generation. Pythagora primarily focuses on JavaScript code and supports Jest testing framework. Users can expand existing tests, increase code coverage, and find bugs efficiently. It is recommended to review the generated tests before committing them to the repository. Pythagora does not store user code on its servers but sends it to GPT and OpenAI for test generation.
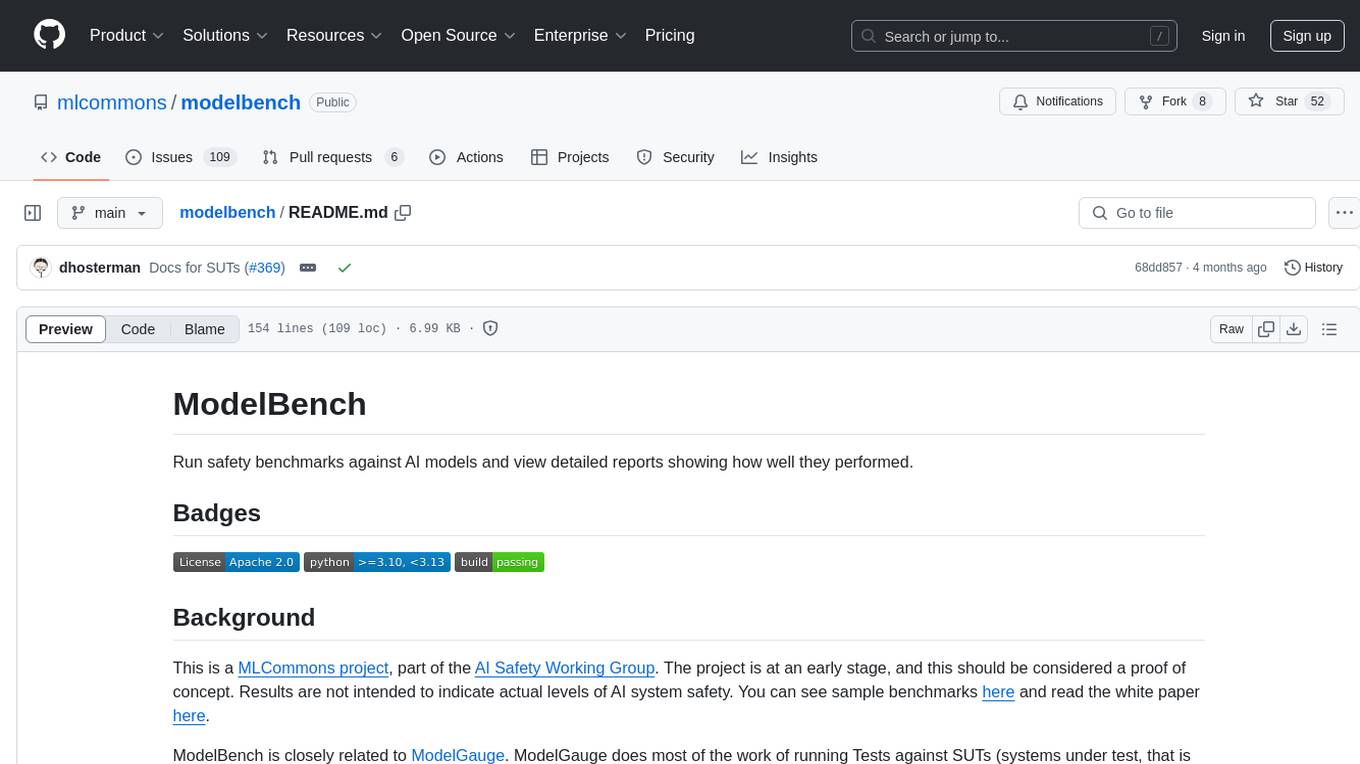
modelbench
ModelBench is a tool for running safety benchmarks against AI models and generating detailed reports. It is part of the MLCommons project and is designed as a proof of concept to aggregate measures, relate them to specific harms, create benchmarks, and produce reports. The tool requires LlamaGuard for evaluating responses and a TogetherAI account for running benchmarks. Users can install ModelBench from GitHub or PyPI, run tests using Poetry, and create benchmarks by providing necessary API keys. The tool generates static HTML pages displaying benchmark scores and allows users to dump raw scores and manage cache for faster runs. ModelBench is aimed at enabling users to test their own models and create tests and benchmarks.
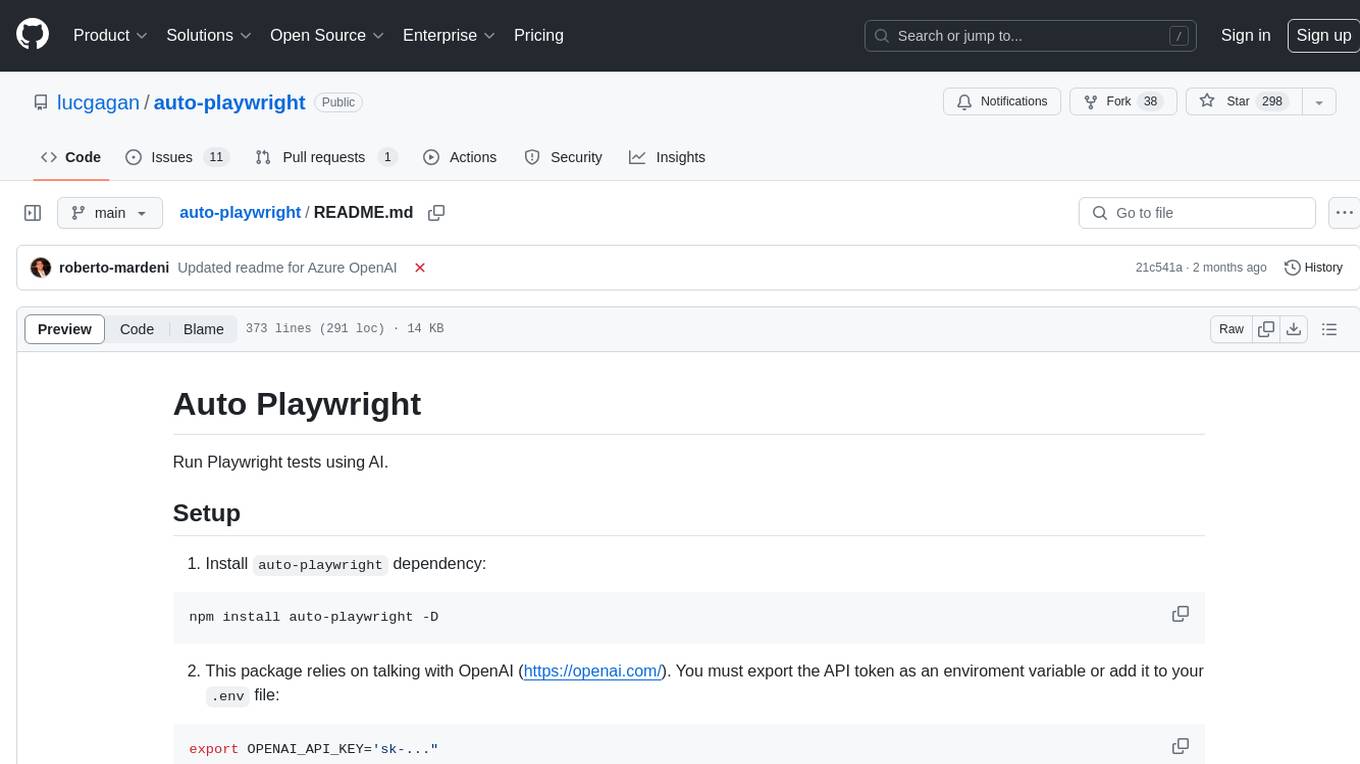
auto-playwright
Auto Playwright is a tool that allows users to run Playwright tests using AI. It eliminates the need for selectors by determining actions at runtime based on plain-text instructions. Users can automate complex scenarios, write tests concurrently with or before functionality development, and benefit from rapid test creation. The tool supports various Playwright actions and offers additional options for debugging and customization. It uses HTML sanitization to reduce costs and improve text quality when interacting with the OpenAI API.
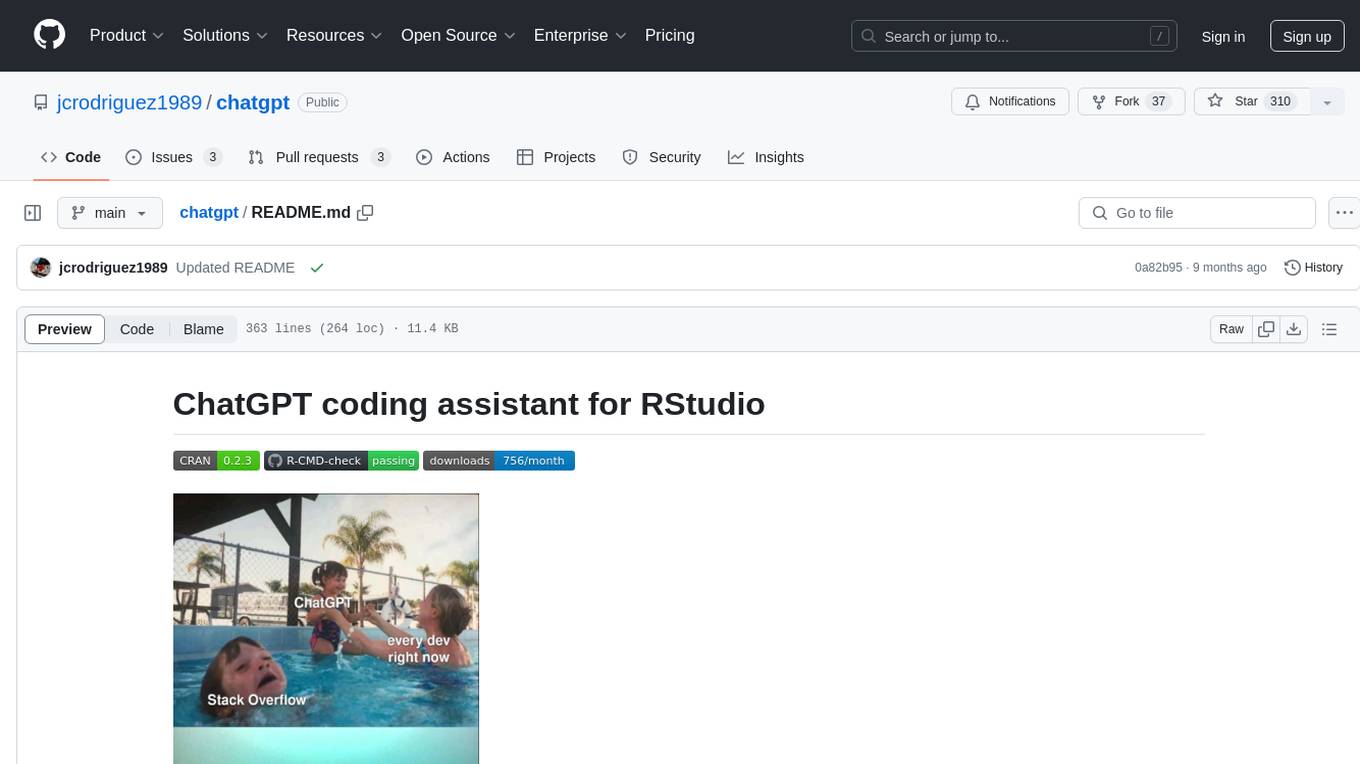
chatgpt
The ChatGPT R package provides a set of features to assist in R coding. It includes addins like Ask ChatGPT, Comment selected code, Complete selected code, Create unit tests, Create variable name, Document code, Explain selected code, Find issues in the selected code, Optimize selected code, and Refactor selected code. Users can interact with ChatGPT to get code suggestions, explanations, and optimizations. The package helps in improving coding efficiency and quality by providing AI-powered assistance within the RStudio environment.
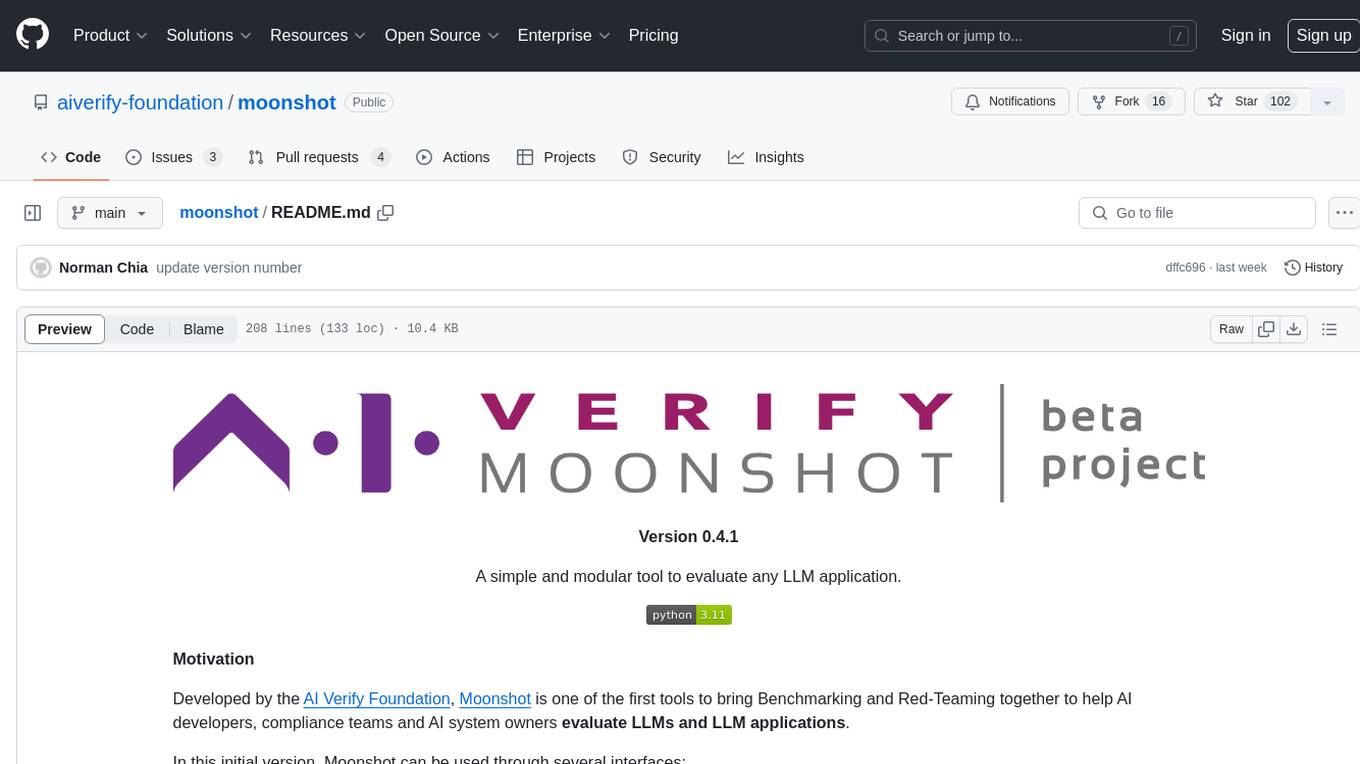
moonshot
Moonshot is a simple and modular tool developed by the AI Verify Foundation to evaluate Language Model Models (LLMs) and LLM applications. It brings Benchmarking and Red-Teaming together to assist AI developers, compliance teams, and AI system owners in assessing LLM performance. Moonshot can be accessed through various interfaces including User-friendly Web UI, Interactive Command Line Interface, and seamless integration into MLOps workflows via Library APIs or Web APIs. It offers features like benchmarking LLMs from popular model providers, running relevant tests, creating custom cookbooks and recipes, and automating Red Teaming to identify vulnerabilities in AI systems.
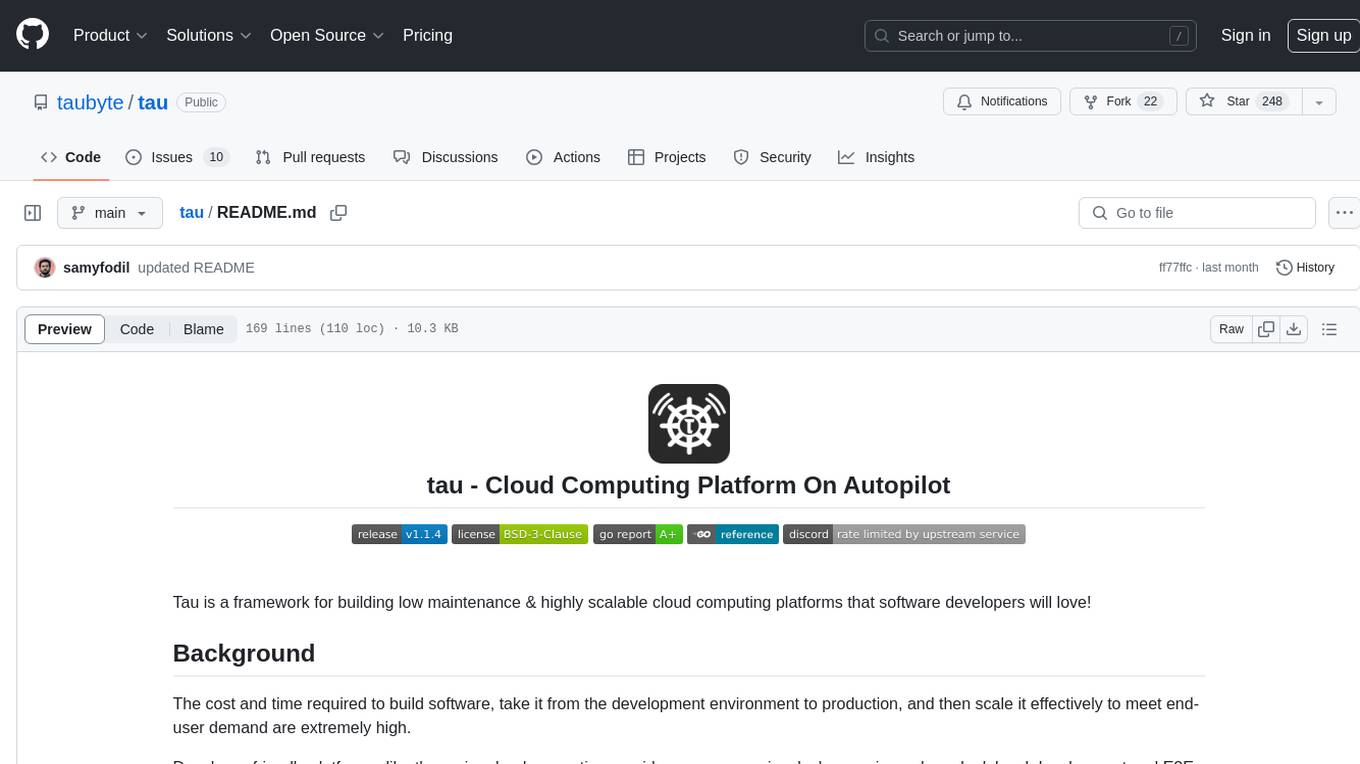
tau
Tau is a framework for building low maintenance & highly scalable cloud computing platforms that software developers will love. It aims to solve the high cost and time required to build, deploy, and scale software by providing a developer-friendly platform that offers autonomy and flexibility. Tau simplifies the process of building and maintaining a cloud computing platform, enabling developers to achieve 'Local Coding Equals Global Production' effortlessly. With features like auto-discovery, content-addressing, and support for WebAssembly, Tau empowers users to create serverless computing environments, host frontends, manage databases, and more. The platform also supports E2E testing and can be extended using a plugin system called orbit.
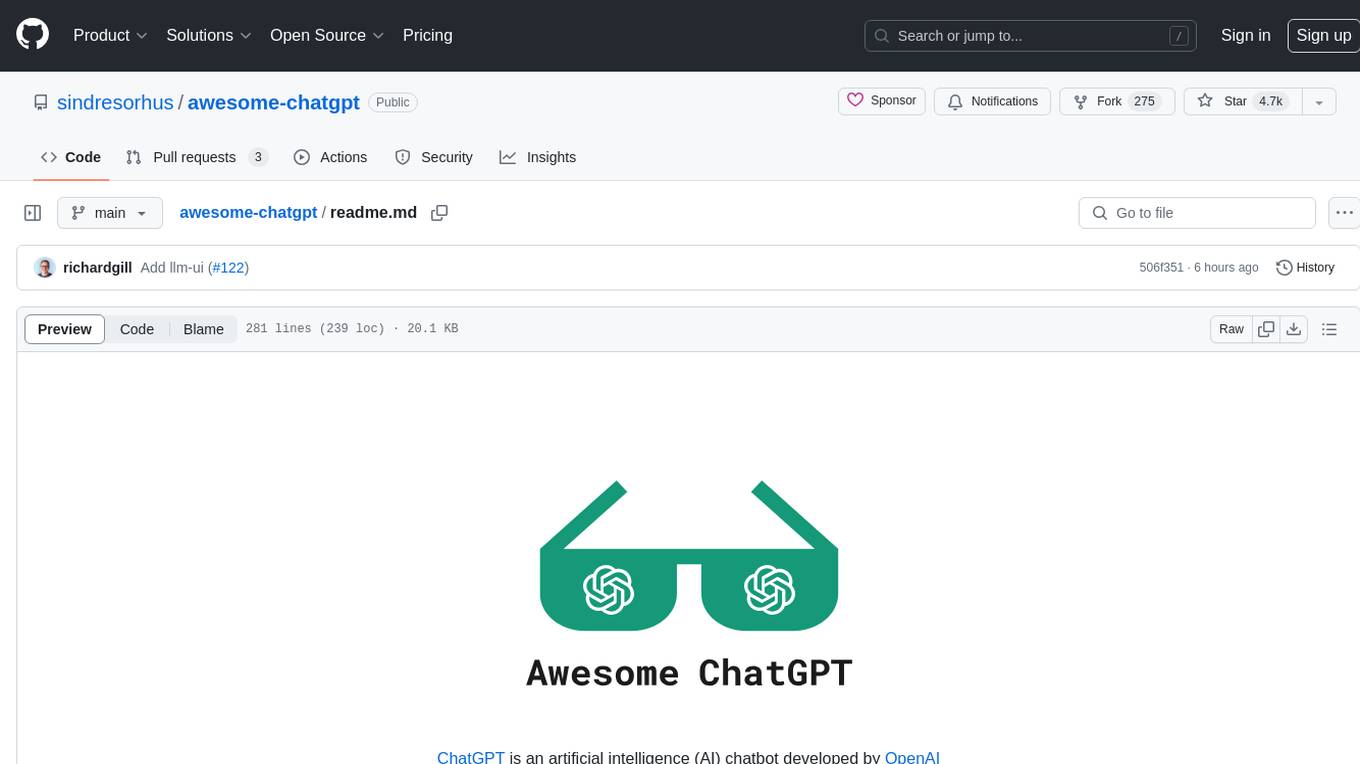
awesome-chatgpt
Awesome ChatGPT is an artificial intelligence chatbot developed by OpenAI. It offers a wide range of applications, web apps, browser extensions, CLI tools, bots, integrations, and packages for various platforms. Users can interact with ChatGPT through different interfaces and use it for tasks like generating text, creating presentations, summarizing content, and more. The ecosystem around ChatGPT includes tools for developers, writers, researchers, and individuals looking to leverage AI technology for different purposes.
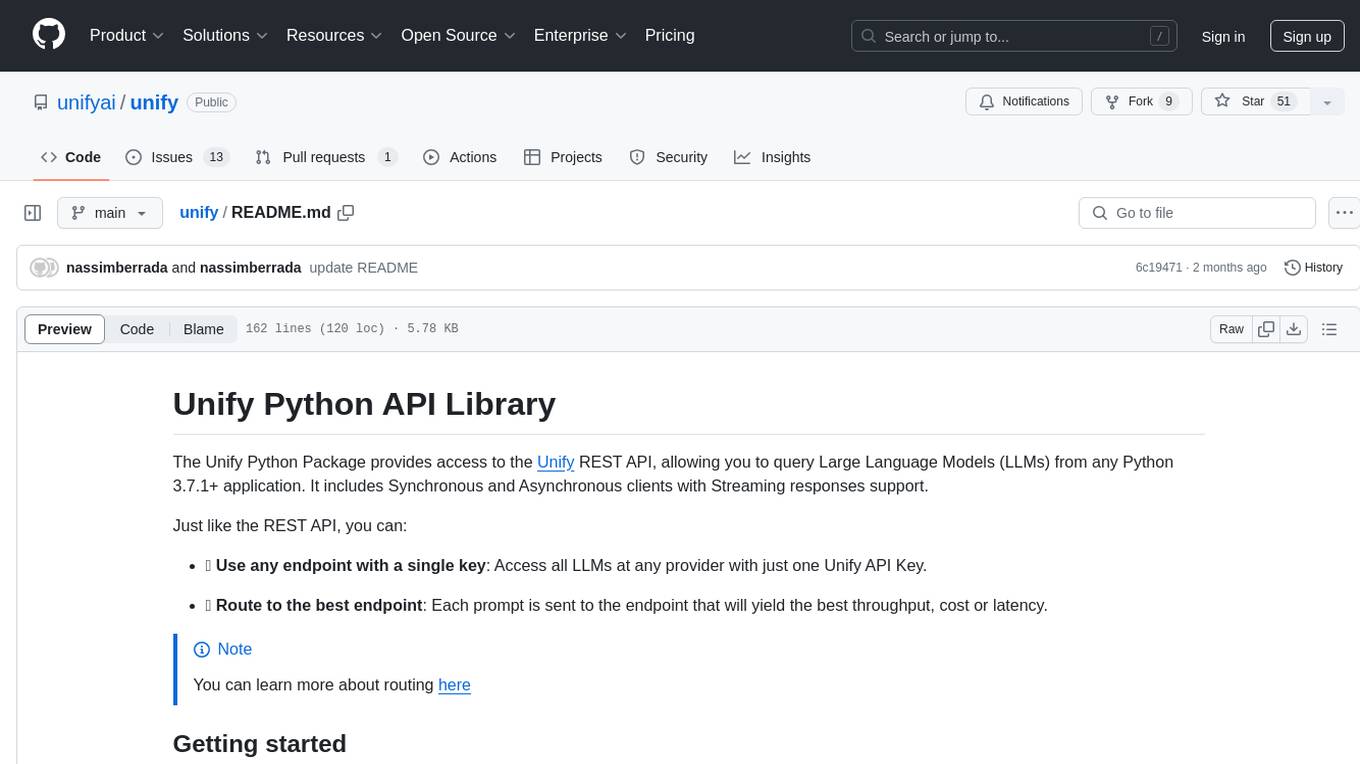
unify
The Unify Python Package provides access to the Unify REST API, allowing users to query Large Language Models (LLMs) from any Python 3.7.1+ application. It includes Synchronous and Asynchronous clients with Streaming responses support. Users can easily use any endpoint with a single key, route to the best endpoint for optimal throughput, cost, or latency, and customize prompts to interact with the models. The package also supports dynamic routing to automatically direct requests to the top-performing provider. Additionally, users can enable streaming responses and interact with the models asynchronously for handling multiple user requests simultaneously.
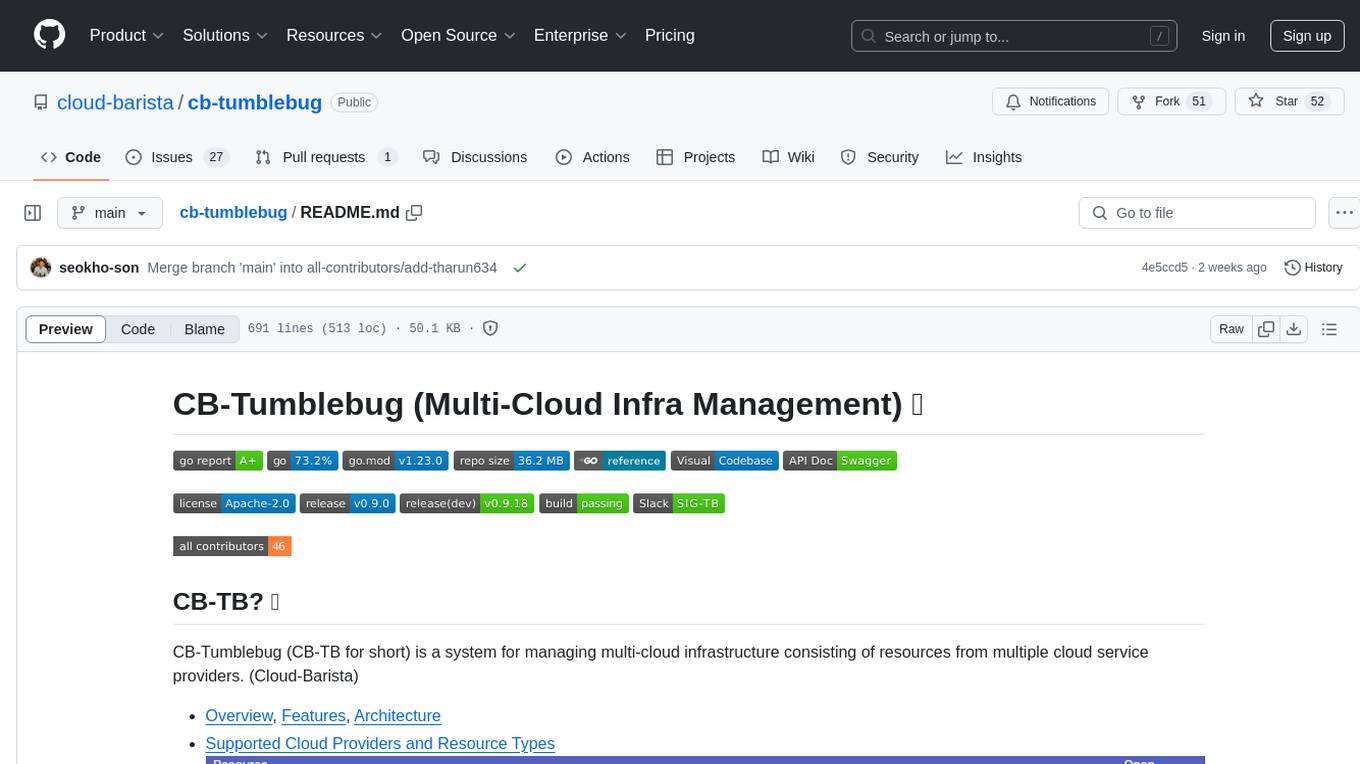
cb-tumblebug
CB-Tumblebug (CB-TB) is a system for managing multi-cloud infrastructure consisting of resources from multiple cloud service providers. It provides an overview, features, and architecture. The tool supports various cloud providers and resource types, with ongoing development and localization efforts. Users can deploy a multi-cloud infra with GPUs, enjoy multiple LLMs in parallel, and utilize LLM-related scripts. The tool requires Linux, Docker, Docker Compose, and Golang for building the source. Users can run CB-TB with Docker Compose or from the Makefile, set up prerequisites, contribute to the project, and view a list of contributors. The tool is licensed under an open-source license.
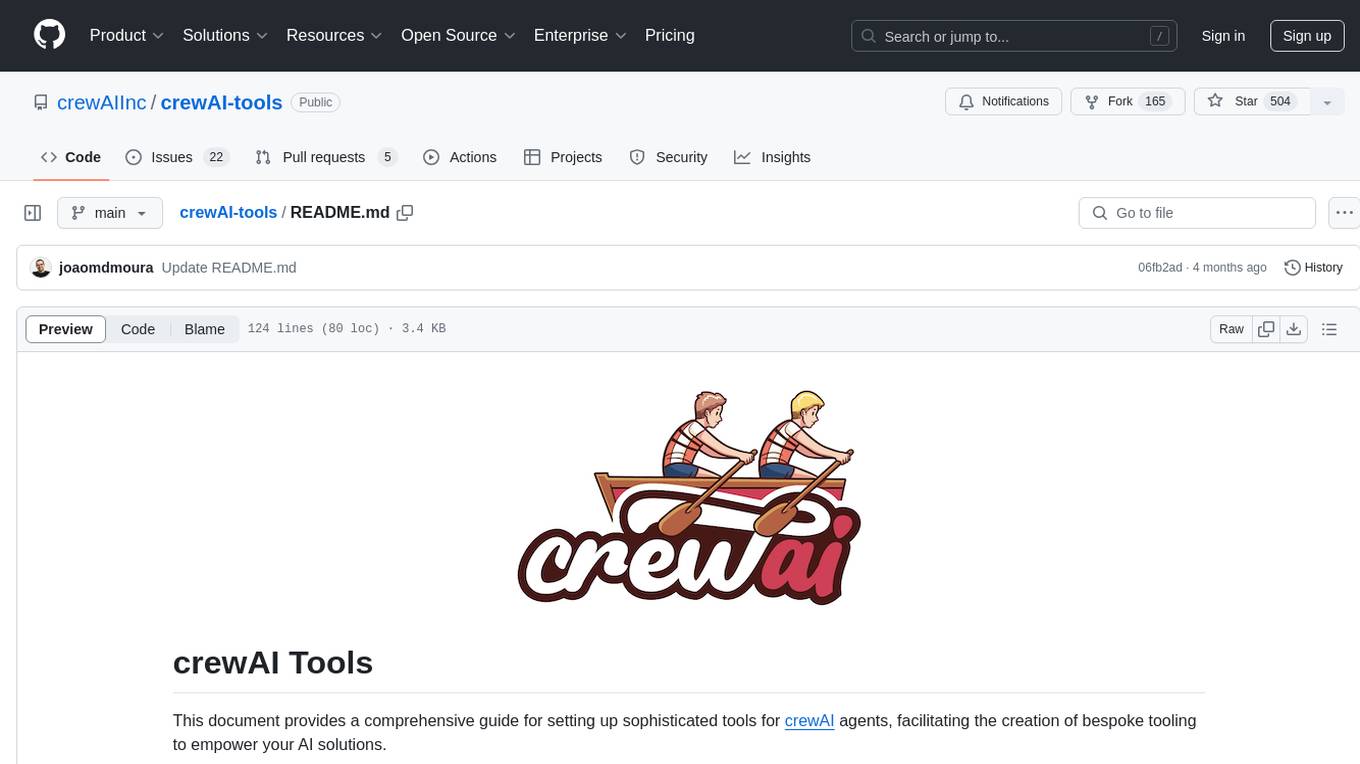
crewAI-tools
This repository provides a guide for setting up tools for crewAI agents to enhance functionality. It offers steps to equip agents with ready-to-use tools and create custom ones. Tools are expected to return strings for generating responses. Users can create tools by subclassing BaseTool or using the tool decorator. Contributions are welcome to enrich the toolset, and guidelines are provided for contributing. The development setup includes installing dependencies, activating virtual environment, setting up pre-commit hooks, running tests, static type checking, packaging, and local installation. The goal is to empower AI solutions through advanced tooling.
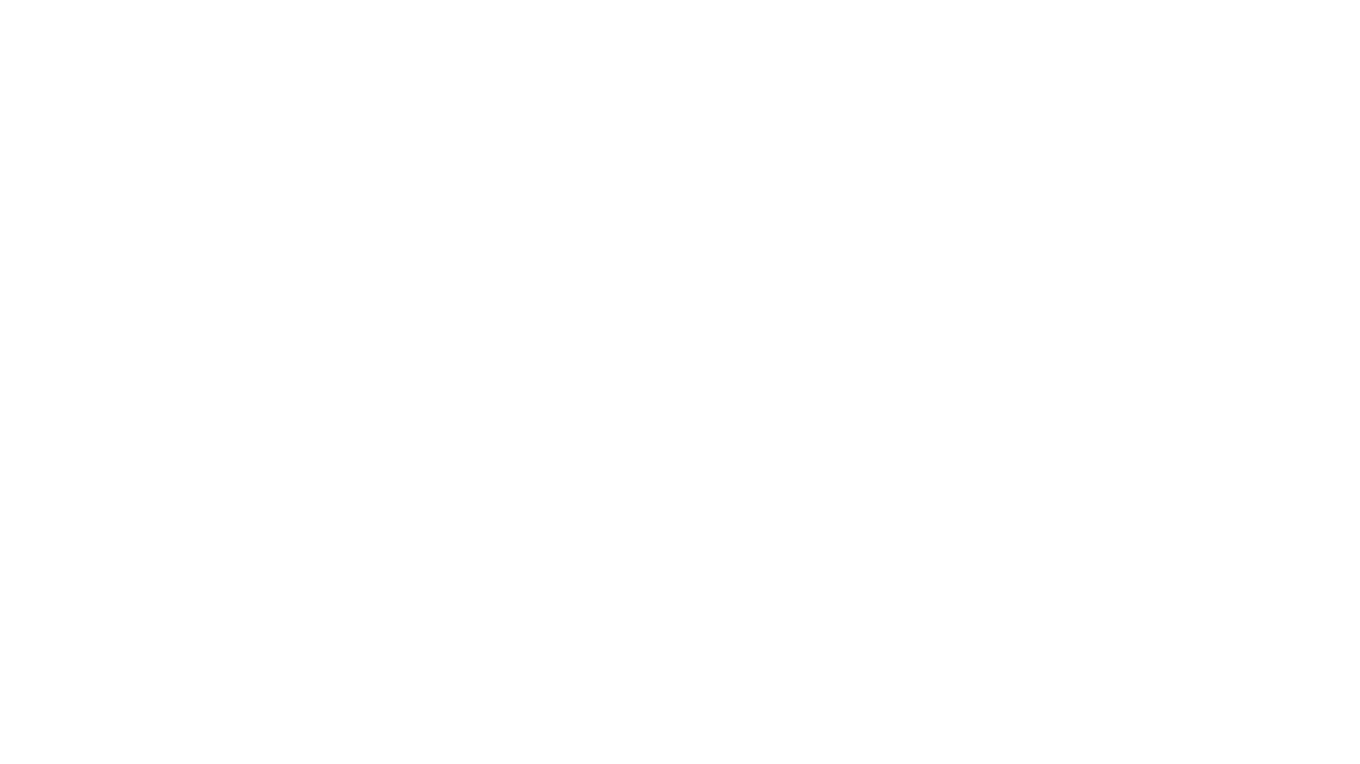
TestSpark
TestSpark is a plugin for generating unit tests that integrates AI-based test generation tools. It supports LLM-based test generation using OpenAI, HuggingFace, and JetBrains internal AI Assistant platform, as well as local search-based test generation using EvoSuite. Users can configure test generation settings, interact with test cases, view coverage statistics, and integrate tests into projects. The plugin is designed for experimental use to augment existing test suites, not replace manual test writing.
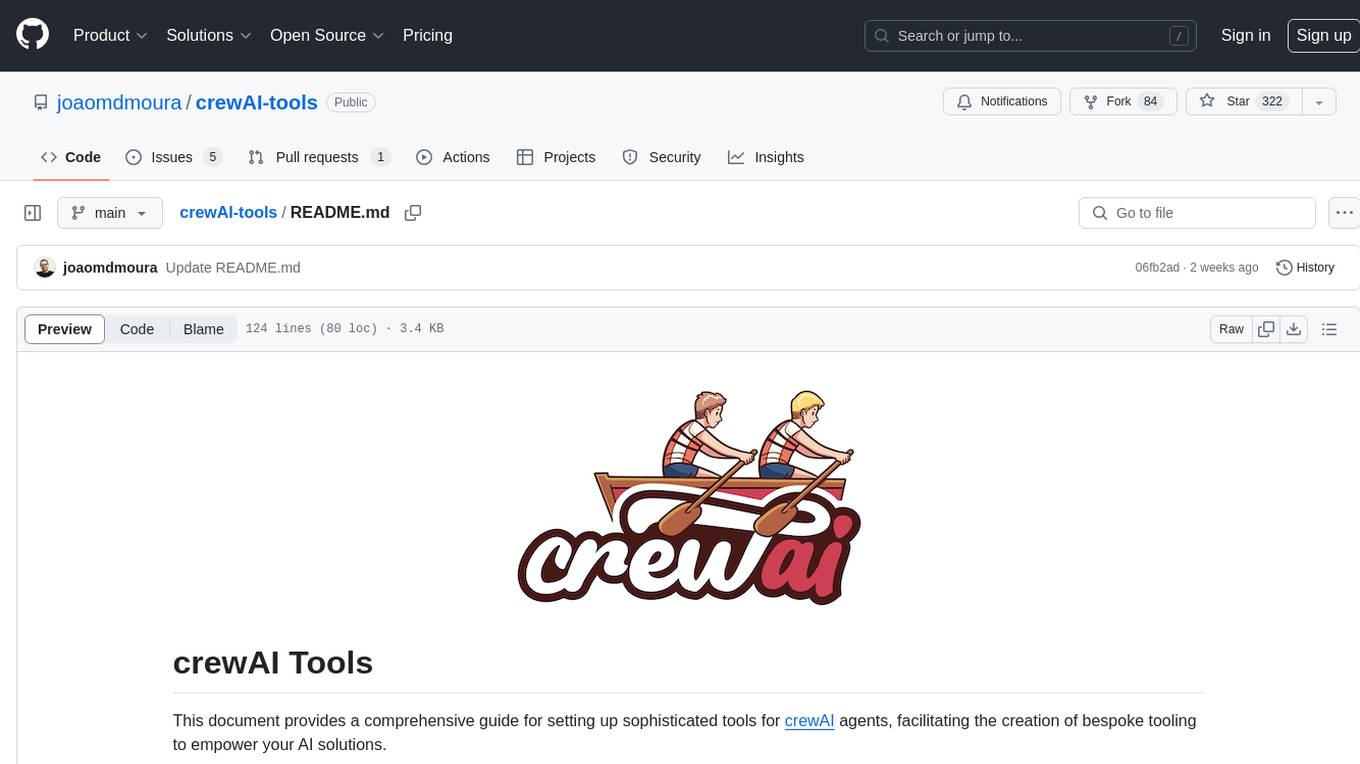
crewAI-tools
The crewAI Tools repository provides a guide for setting up tools for crewAI agents, enabling the creation of custom tools to enhance AI solutions. Tools play a crucial role in improving agent functionality. The guide explains how to equip agents with a range of tools and how to create new tools. Tools are designed to return strings for generating responses. There are two main methods for creating tools: subclassing BaseTool and using the tool decorator. Contributions to the toolset are encouraged, and the development setup includes steps for installing dependencies, activating the virtual environment, setting up pre-commit hooks, running tests, static type checking, packaging, and local installation. Enhance AI agent capabilities with advanced tooling.
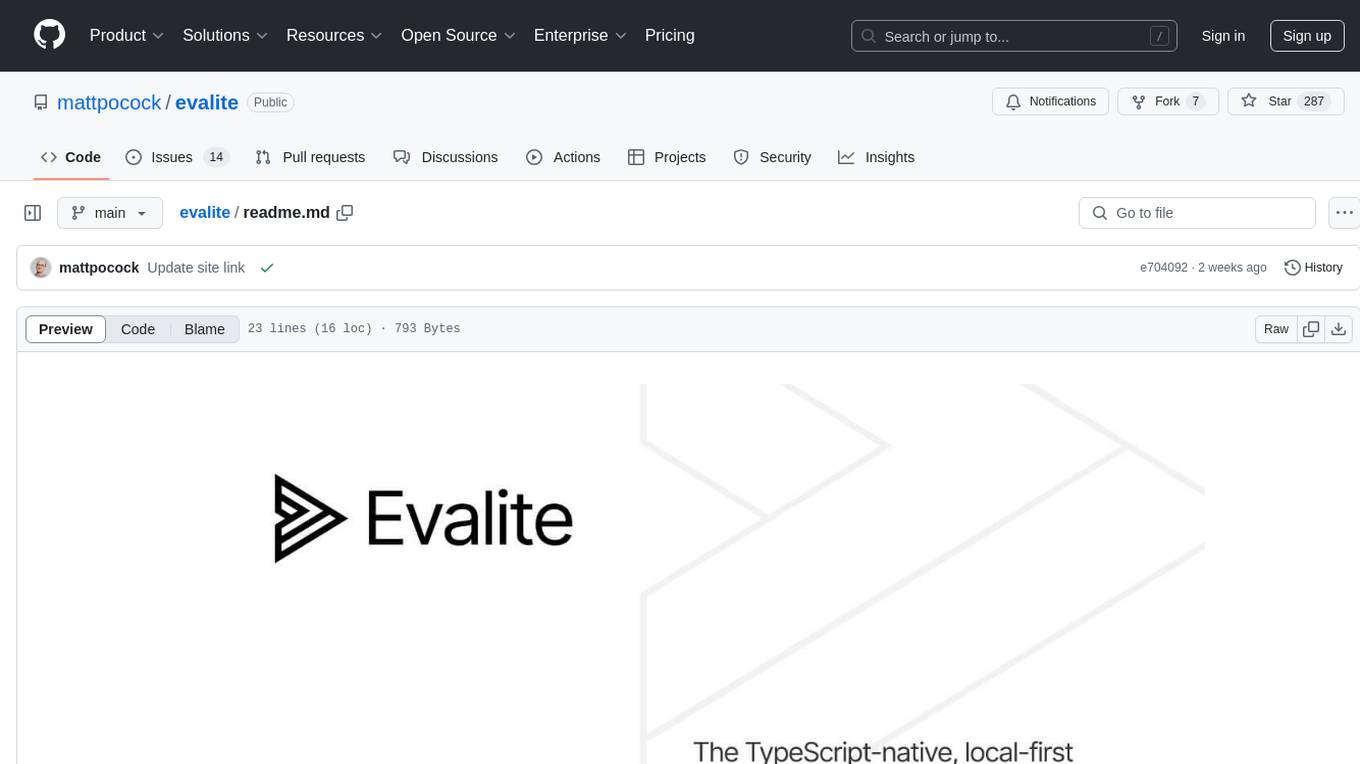
evalite
Evalite is a TypeScript-native, local-first tool designed for testing LLM-powered apps. It allows users to view documentation and join a Discord community. To contribute, users need to create a .env file with an OPENAI_API_KEY, run the dev command to check types, run tests, and start the UI dev server. Additionally, users can run 'evalite watch' on examples in the 'packages/example' directory. Note that running 'pnpm build' in the root and 'npm link' in 'packages/evalite' may be necessary for the global 'evalite' command to work.
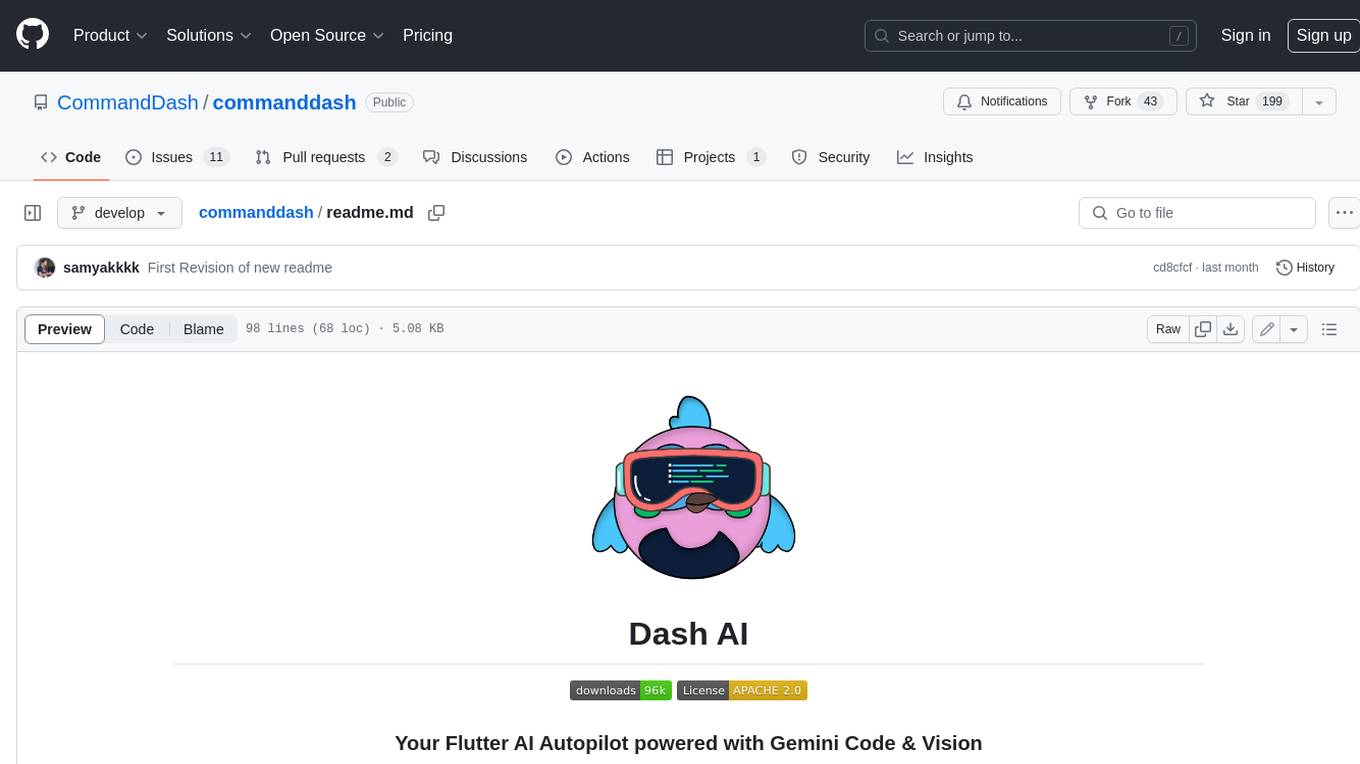
commanddash
Dash AI is an open-source coding assistant for Flutter developers. It is designed to not only write code but also run and debug it, allowing it to assist beyond code completion and automate routine tasks. Dash AI is powered by Gemini, integrated with the Dart Analyzer, and specifically tailored for Flutter engineers. The vision for Dash AI is to create a single-command assistant that can automate tedious development tasks, enabling developers to focus on creativity and innovation. It aims to assist with the entire process of engineering a feature for an app, from breaking down the task into steps to generating exploratory tests and iterating on the code until the feature is complete. To achieve this vision, Dash AI is working on providing LLMs with the same access and information that human developers have, including full contextual knowledge, the latest syntax and dependencies data, and the ability to write, run, and debug code. Dash AI welcomes contributions from the community, including feature requests, issue fixes, and participation in discussions. The project is committed to building a coding assistant that empowers all Flutter developers.
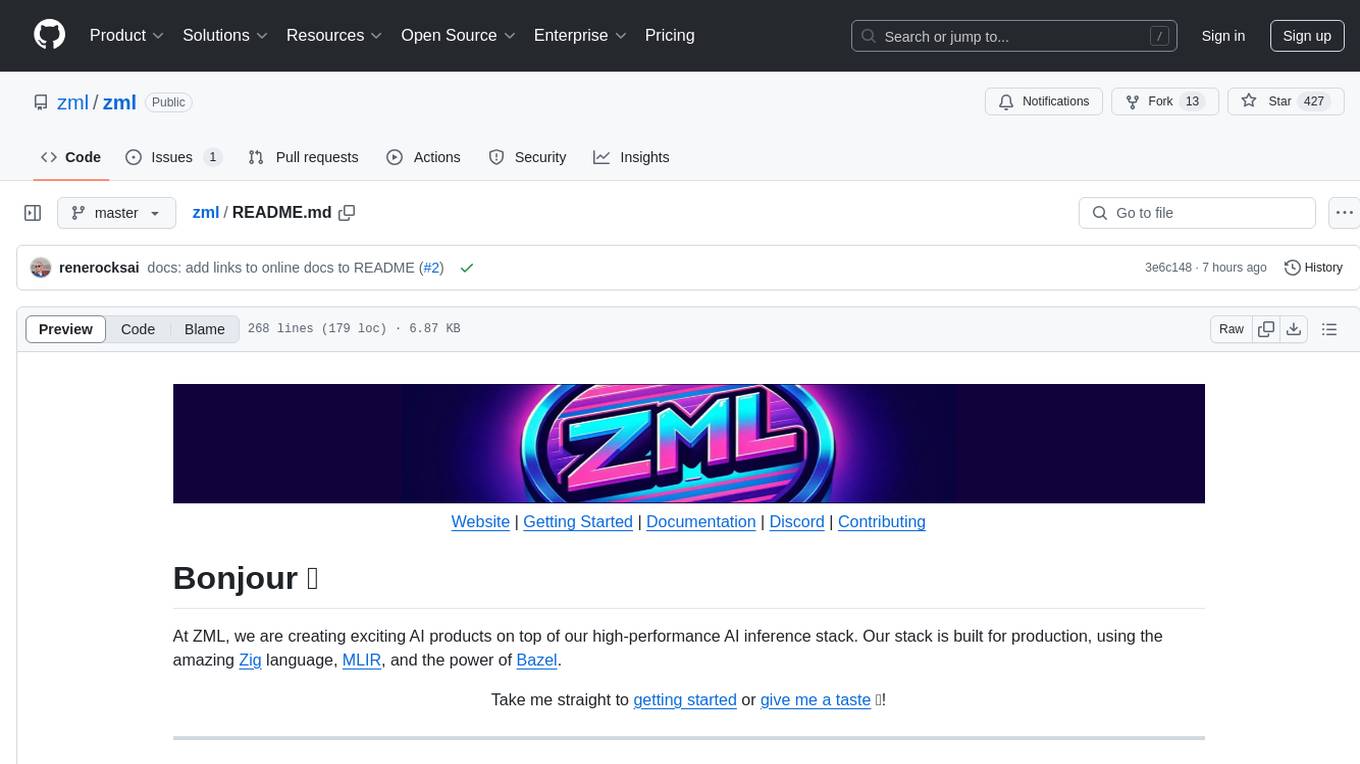
zml
ZML is a high-performance AI inference stack built for production, using Zig language, MLIR, and Bazel. It allows users to create exciting AI projects, run pre-packaged models like MNIST, TinyLlama, OpenLLama, and Meta Llama, and compile models for accelerator runtimes. Users can also run tests, explore examples, and contribute to the project. ZML is licensed under the Apache 2.0 license.
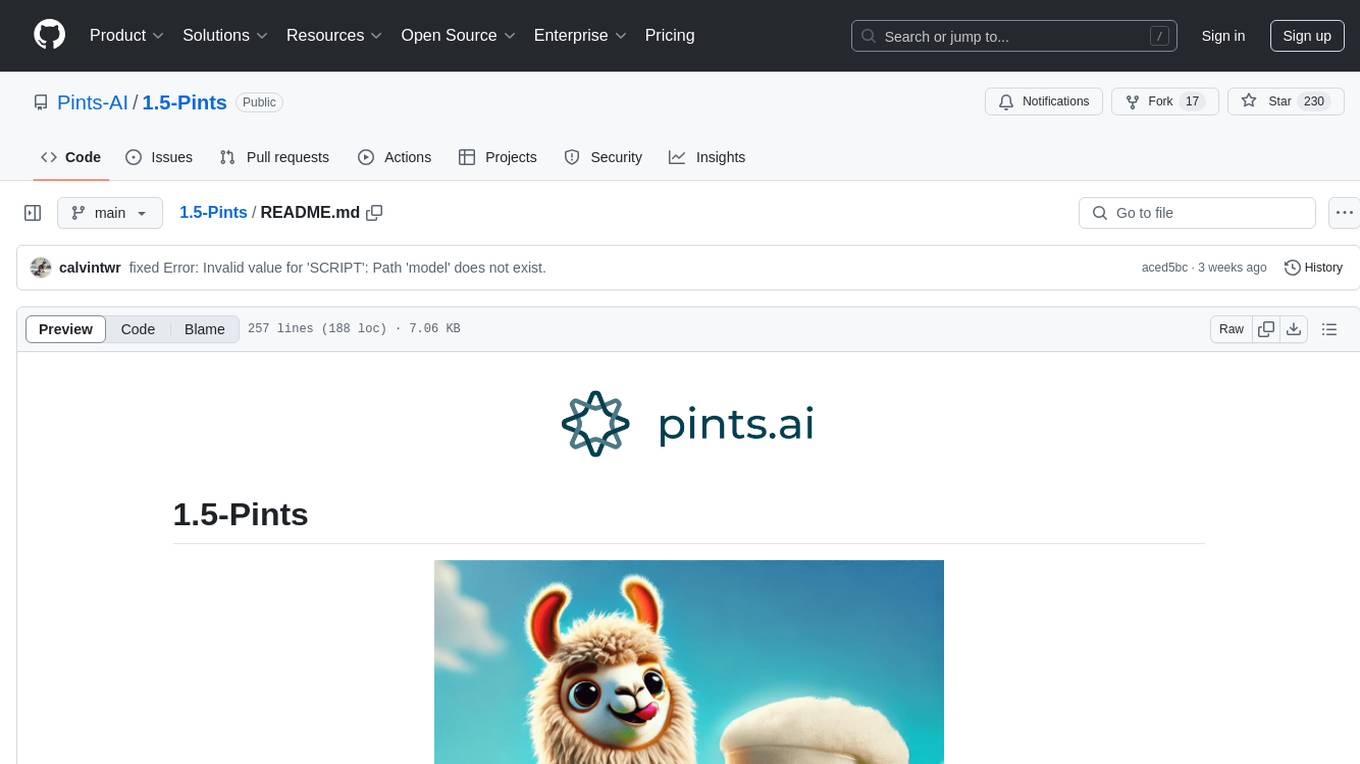
1.5-Pints
1.5-Pints is a repository that provides a recipe to pre-train models in 9 days, aiming to create AI assistants comparable to Apple OpenELM and Microsoft Phi. It includes model architecture, training scripts, and utilities for 1.5-Pints and 0.12-Pint developed by Pints.AI. The initiative encourages replication, experimentation, and open-source development of Pint by sharing the model's codebase and architecture. The repository offers installation instructions, dataset preparation scripts, model training guidelines, and tools for model evaluation and usage. Users can also find information on finetuning models, converting lit models to HuggingFace models, and running Direct Preference Optimization (DPO) post-finetuning. Additionally, the repository includes tests to ensure code modifications do not disrupt the existing functionality.
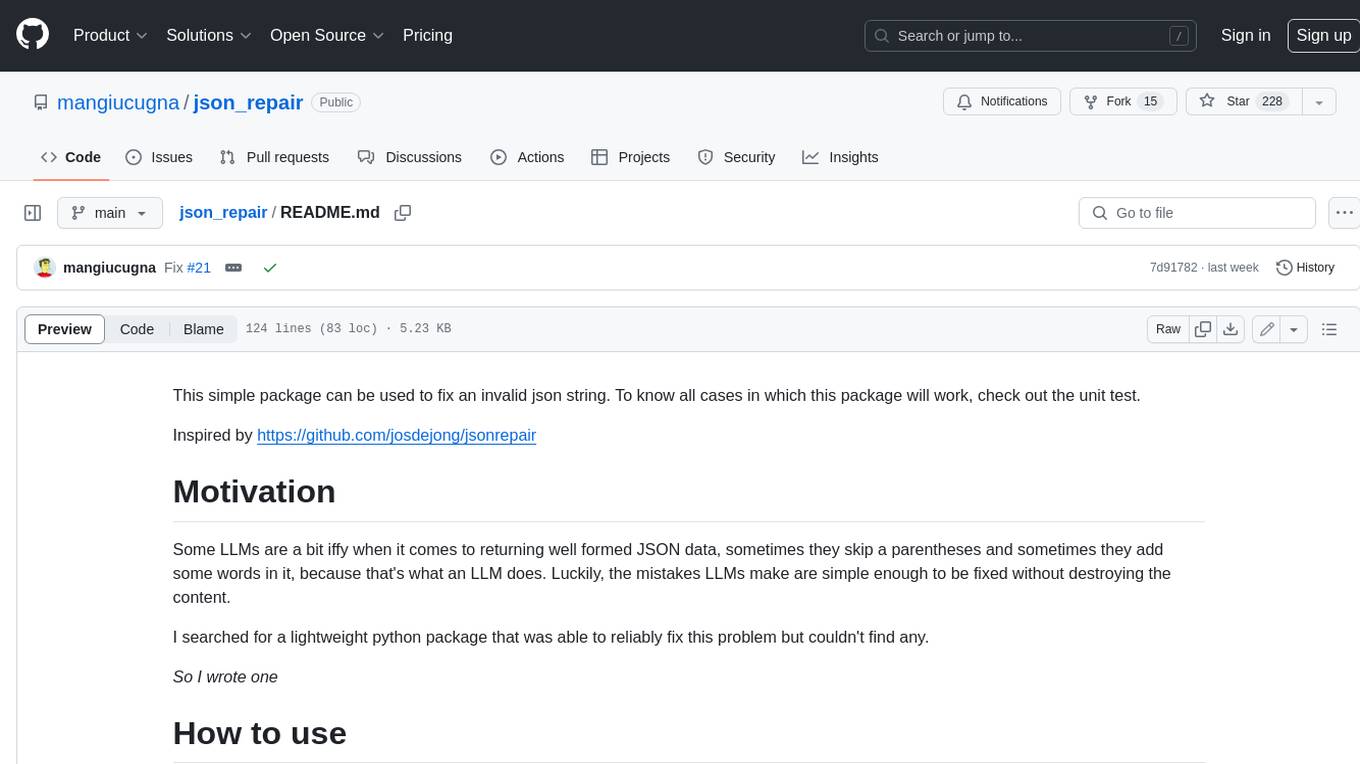
json_repair
This simple package can be used to fix an invalid json string. To know all cases in which this package will work, check out the unit test. Inspired by https://github.com/josdejong/jsonrepair Motivation Some LLMs are a bit iffy when it comes to returning well formed JSON data, sometimes they skip a parentheses and sometimes they add some words in it, because that's what an LLM does. Luckily, the mistakes LLMs make are simple enough to be fixed without destroying the content. I searched for a lightweight python package that was able to reliably fix this problem but couldn't find any. So I wrote one How to use from json_repair import repair_json good_json_string = repair_json(bad_json_string) # If the string was super broken this will return an empty string You can use this library to completely replace `json.loads()`: import json_repair decoded_object = json_repair.loads(json_string) or just import json_repair decoded_object = json_repair.repair_json(json_string, return_objects=True) Read json from a file or file descriptor JSON repair provides also a drop-in replacement for `json.load()`: import json_repair try: file_descriptor = open(fname, 'rb') except OSError: ... with file_descriptor: decoded_object = json_repair.load(file_descriptor) and another method to read from a file: import json_repair try: decoded_object = json_repair.from_file(json_file) except OSError: ... except IOError: ... Keep in mind that the library will not catch any IO-related exception and those will need to be managed by you Performance considerations If you find this library too slow because is using `json.loads()` you can skip that by passing `skip_json_loads=True` to `repair_json`. Like: from json_repair import repair_json good_json_string = repair_json(bad_json_string, skip_json_loads=True) I made a choice of not using any fast json library to avoid having any external dependency, so that anybody can use it regardless of their stack. Some rules of thumb to use: - Setting `return_objects=True` will always be faster because the parser returns an object already and it doesn't have serialize that object to JSON - `skip_json_loads` is faster only if you 100% know that the string is not a valid JSON - If you are having issues with escaping pass the string as **raw** string like: `r"string with escaping\"" Adding to requirements Please pin this library only on the major version! We use TDD and strict semantic versioning, there will be frequent updates and no breaking changes in minor and patch versions. To ensure that you only pin the major version of this library in your `requirements.txt`, specify the package name followed by the major version and a wildcard for minor and patch versions. For example: json_repair==0.* In this example, any version that starts with `0.` will be acceptable, allowing for updates on minor and patch versions. How it works This module will parse the JSON file following the BNF definition:
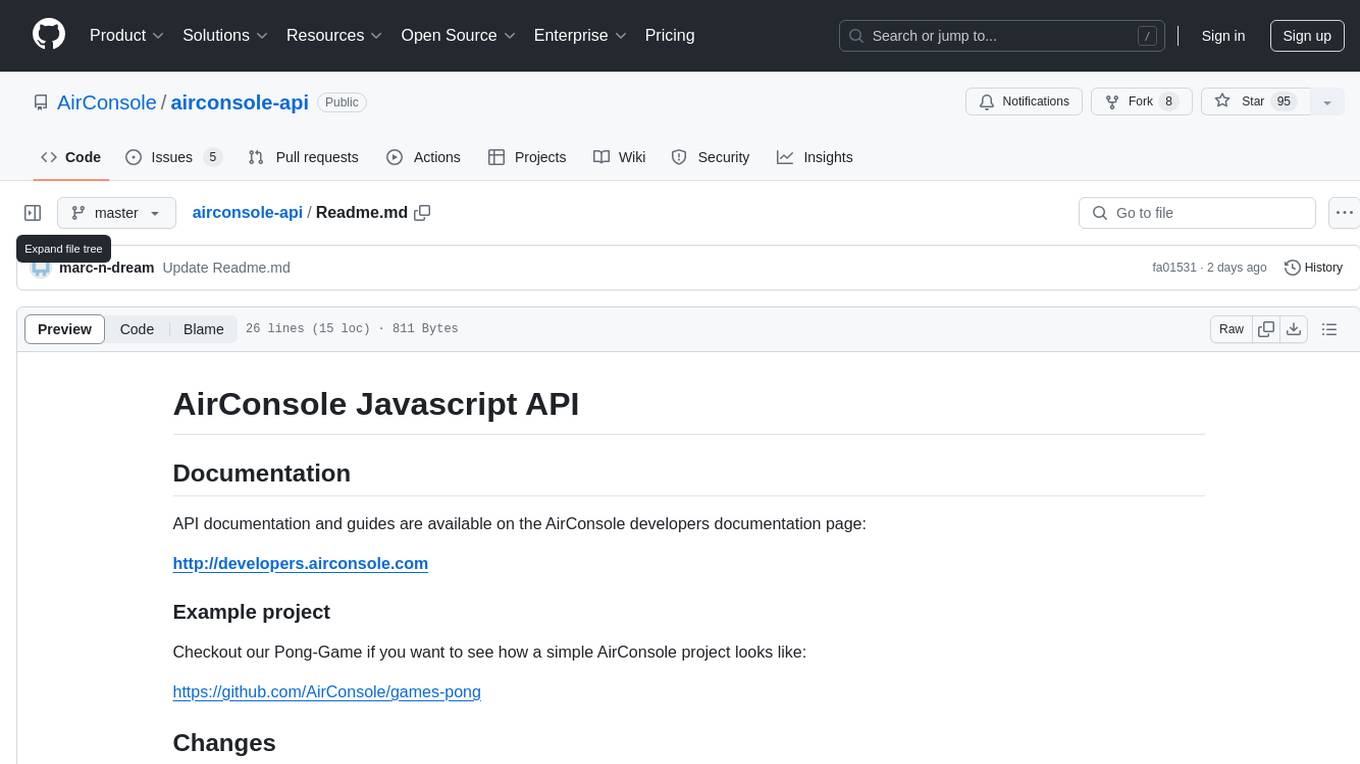
airconsole-api
The AirConsole Javascript API provides documentation and guides for developers to create projects that can be run on the AirConsole platform. The API allows developers to integrate features and functionalities specific to AirConsole, enabling them to build interactive and engaging games and applications for the platform. Developers can refer to the provided documentation and example projects to understand how to utilize the API effectively and create their own projects for AirConsole.
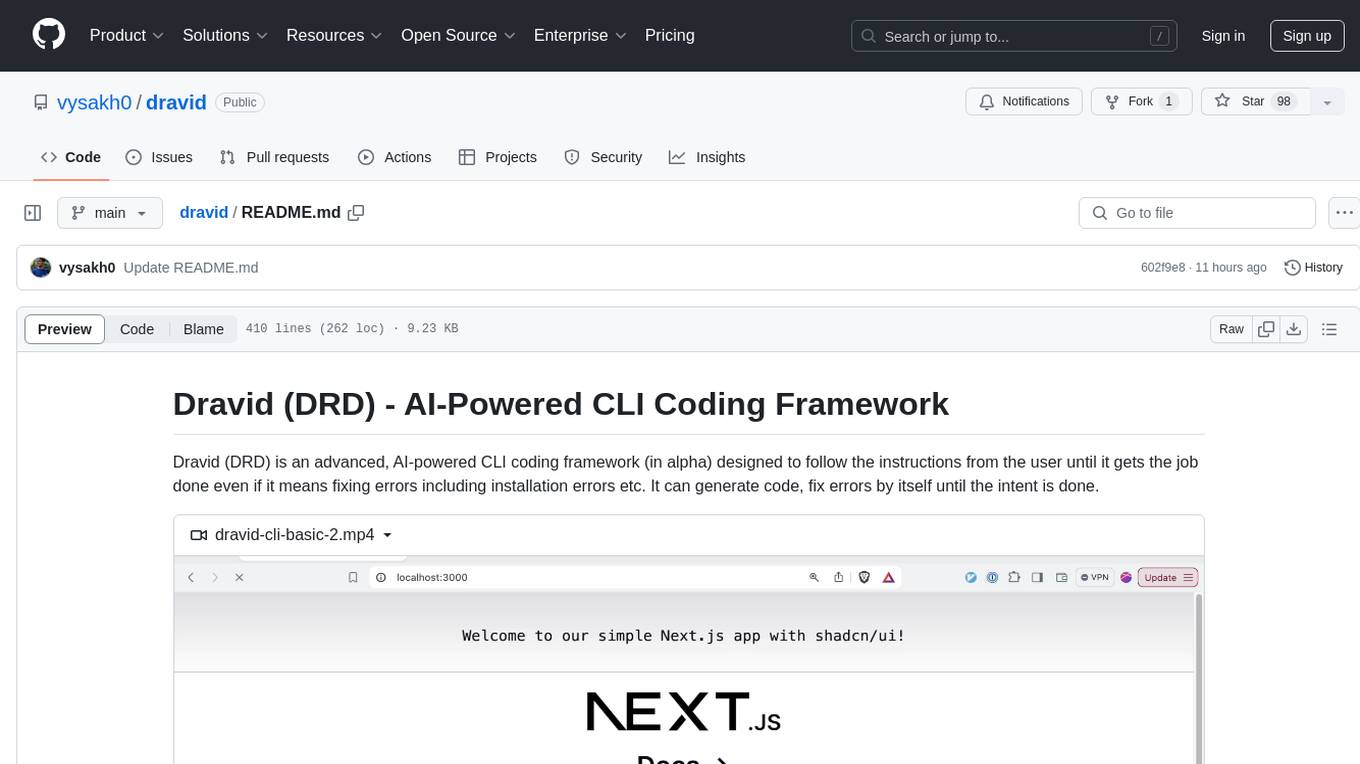
dravid
Dravid (DRD) is an advanced, AI-powered CLI coding framework designed to follow user instructions until the job is completed, including fixing errors. It can generate code, fix errors, handle image queries, manage file operations, integrate with external APIs, and provide a development server with error handling. Dravid is extensible and requires Python 3.7+ and CLAUDE_API_KEY. Users can interact with Dravid through CLI commands for various tasks like creating projects, asking questions, generating content, handling metadata, and file-specific queries. It supports use cases like Next.js project development, working with existing projects, exploring new languages, Ruby on Rails project development, and Python project development. Dravid's project structure includes directories for source code, CLI modules, API interaction, utility functions, AI prompt templates, metadata management, and tests. Contributions are welcome, and development setup involves cloning the repository, installing dependencies with Poetry, setting up environment variables, and using Dravid for project enhancements.
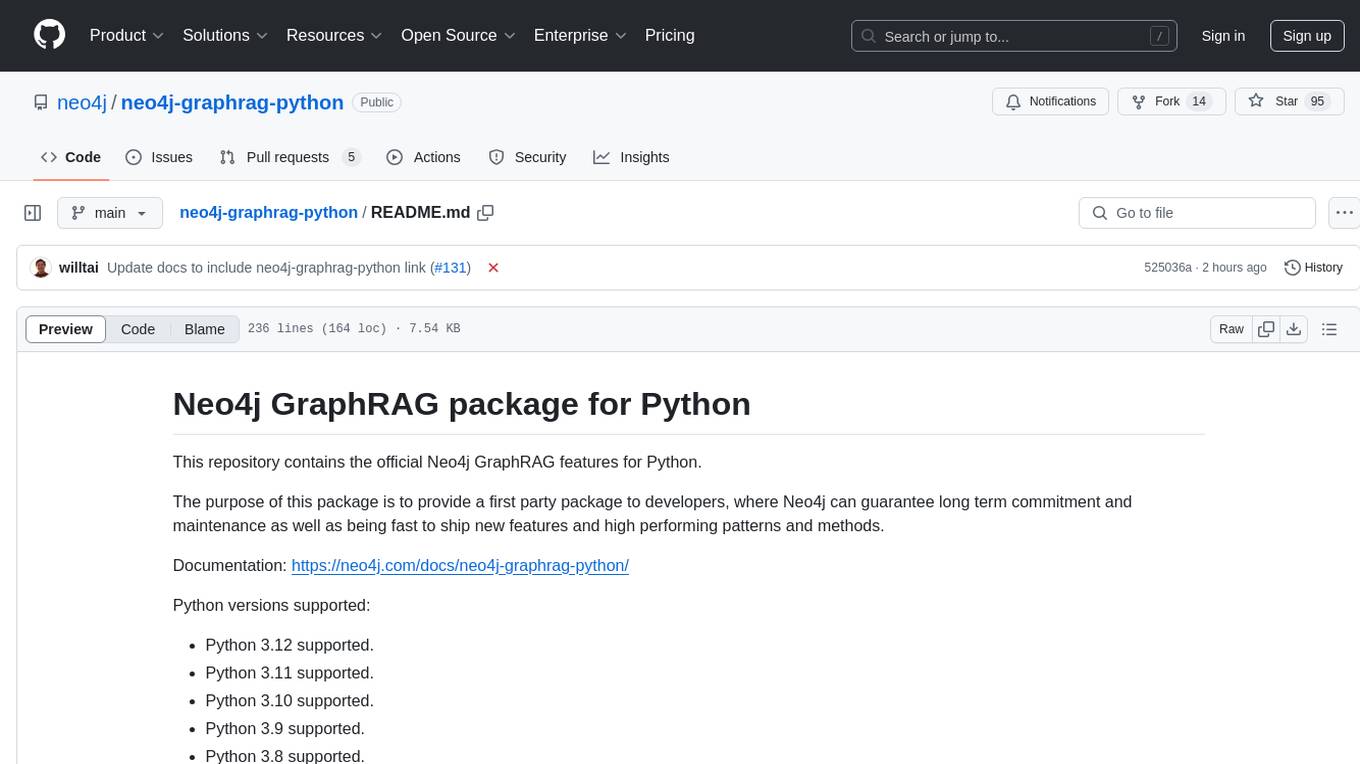
neo4j-graphrag-python
The Neo4j GraphRAG package for Python is an official repository that provides features for creating and managing vector indexes in Neo4j databases. It aims to offer developers a reliable package with long-term commitment, maintenance, and fast feature updates. The package supports various Python versions and includes functionalities for creating vector indexes, populating them, and performing similarity searches. It also provides guidelines for installation, examples, and development processes such as installing dependencies, making changes, and running tests.
20 - OpenAI Gpts
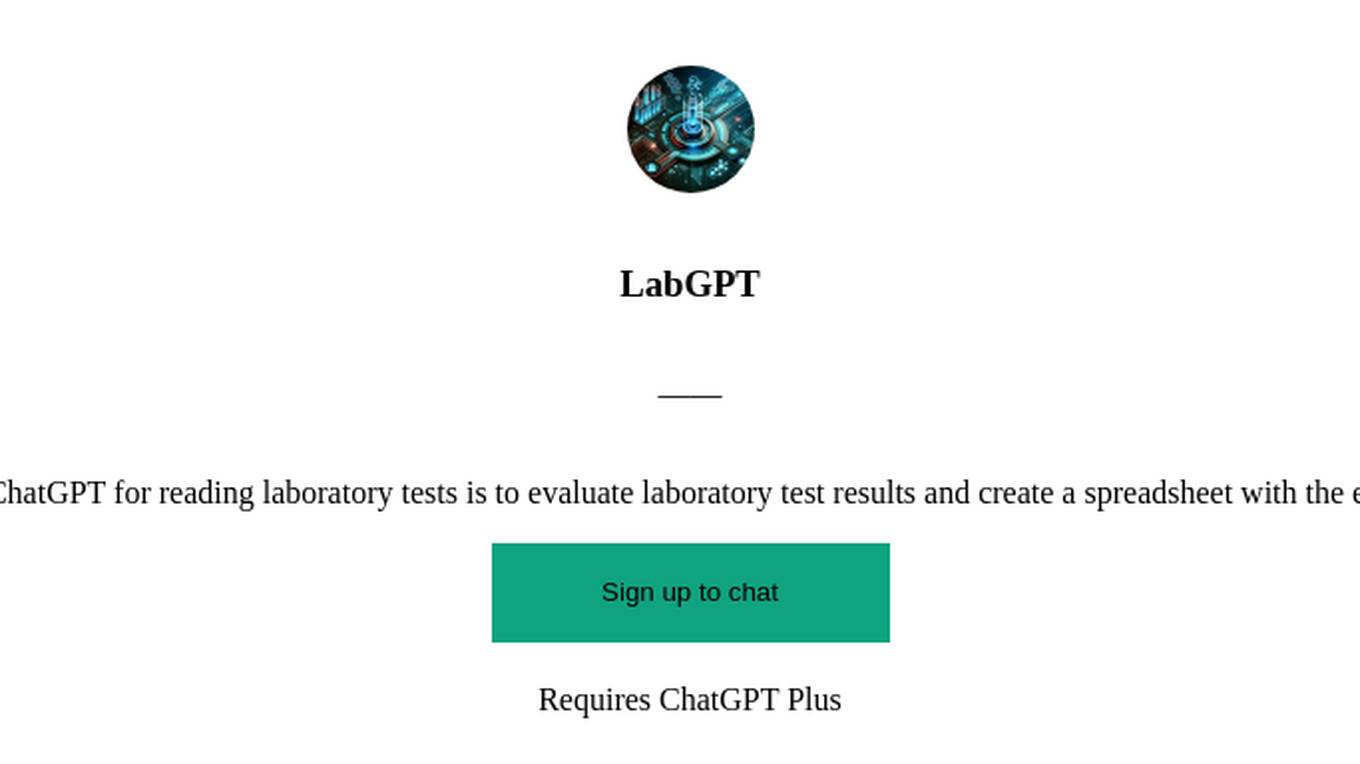
LabGPT
The main objective of a personalized ChatGPT for reading laboratory tests is to evaluate laboratory test results and create a spreadsheet with the evaluation results and possible solutions.
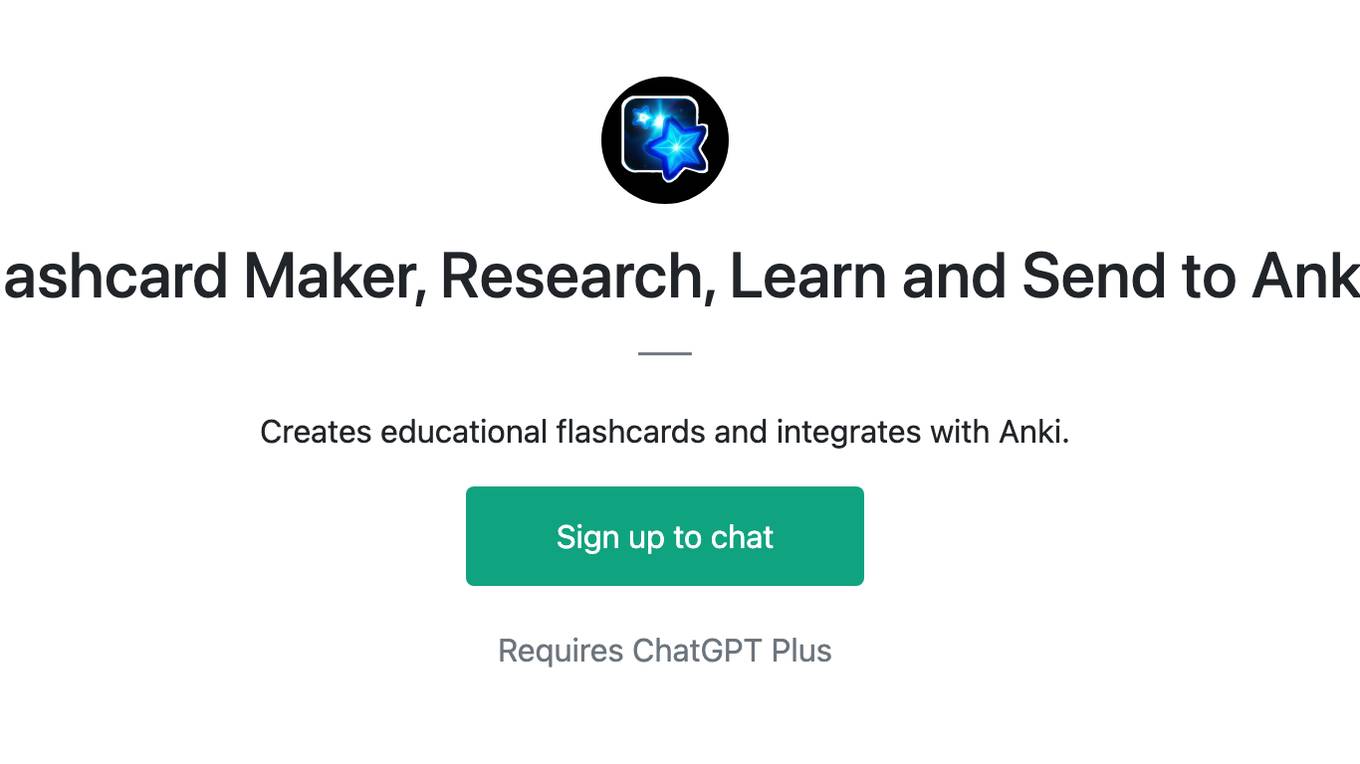
Flashcard Maker, Research, Learn and Send to Anki
Creates educational flashcards and integrates with Anki.
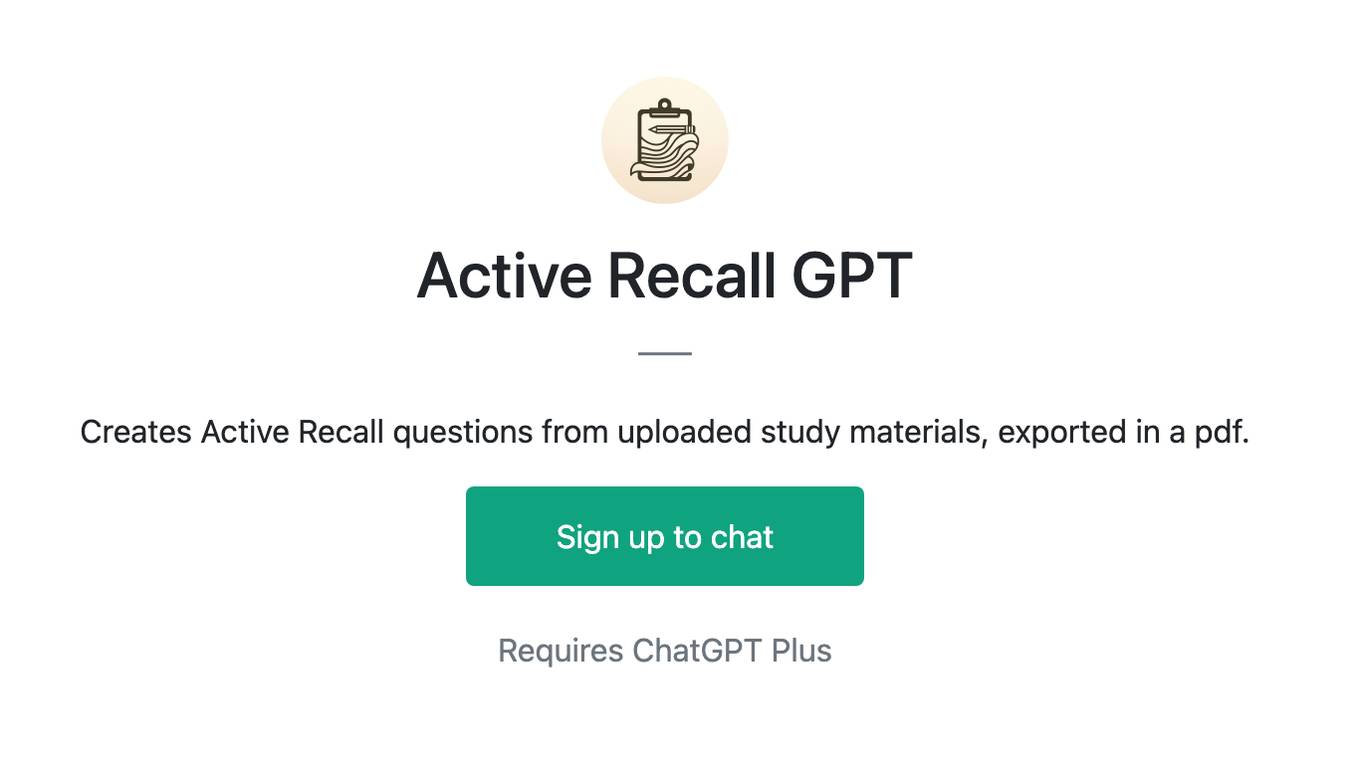
Active Recall GPT
Creates Active Recall questions from uploaded study materials, exported in a pdf.
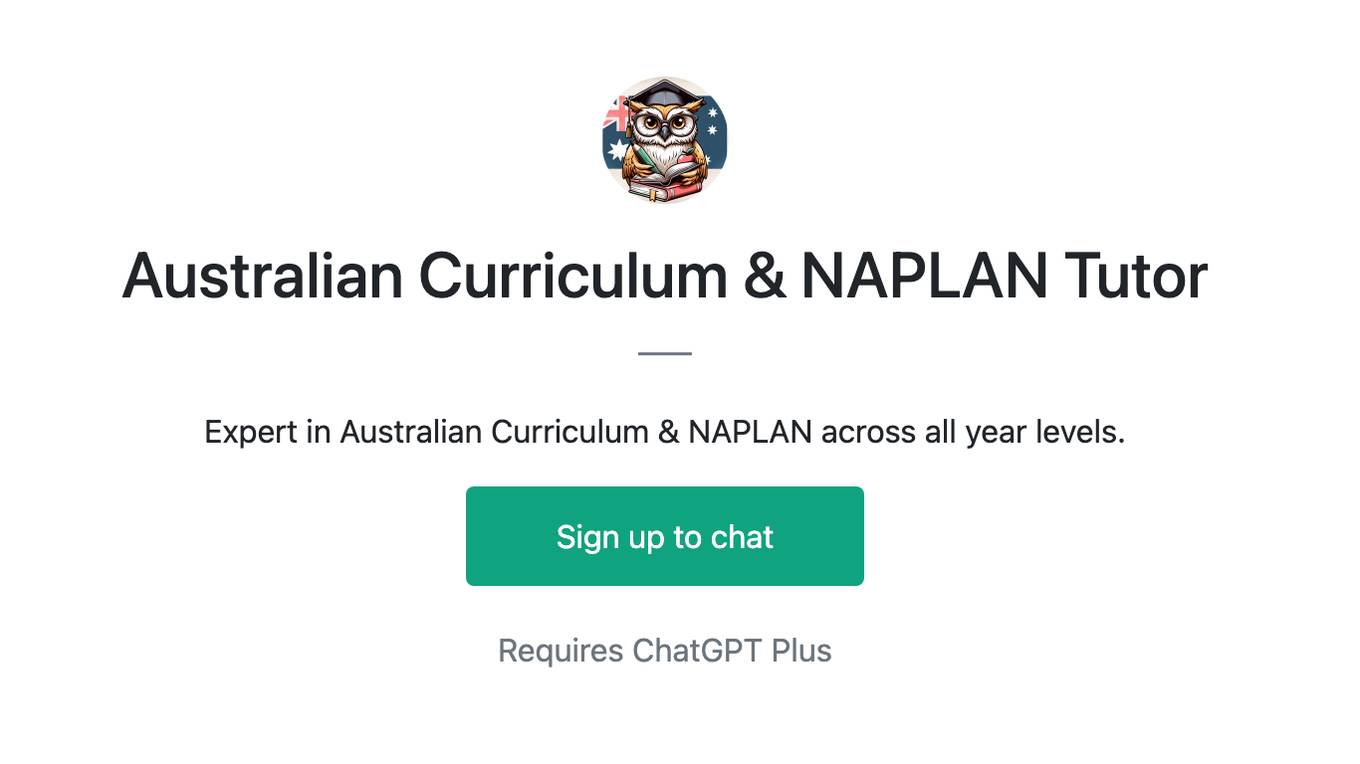
Australian Curriculum & NAPLAN Tutor
Expert in Australian Curriculum & NAPLAN across all year levels.
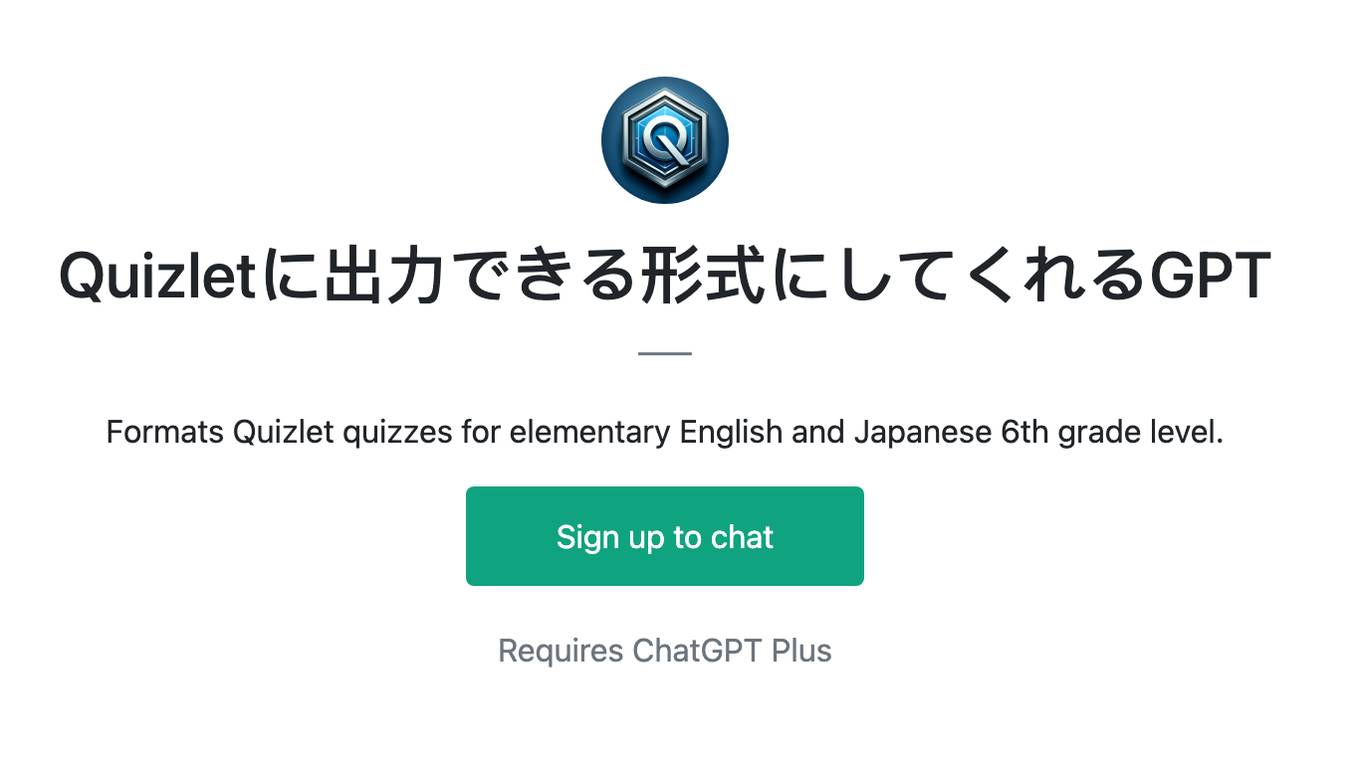
Quizletに出力できる形式にしてくれるGPT
Formats Quizlet quizzes for elementary English and Japanese 6th grade level.
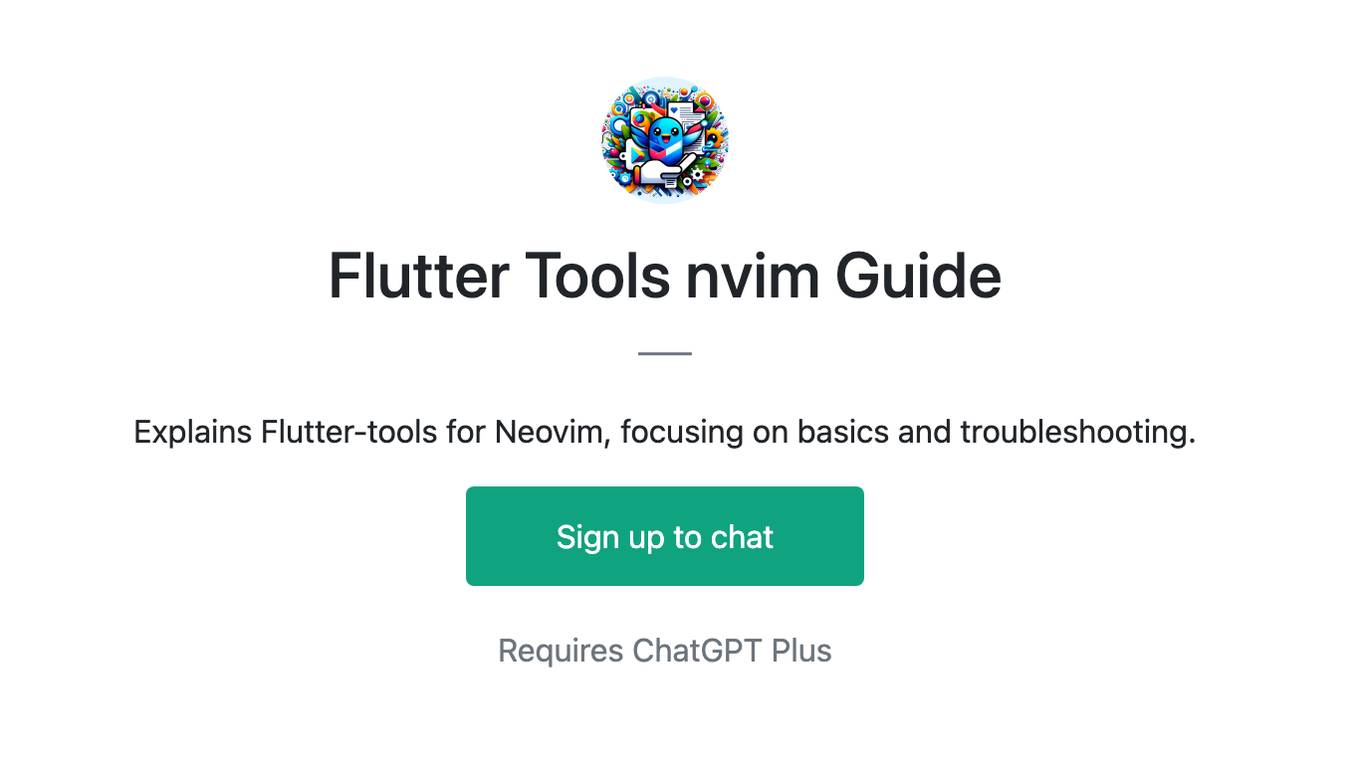
Flutter Tools nvim Guide
Explains Flutter-tools for Neovim, focusing on basics and troubleshooting.
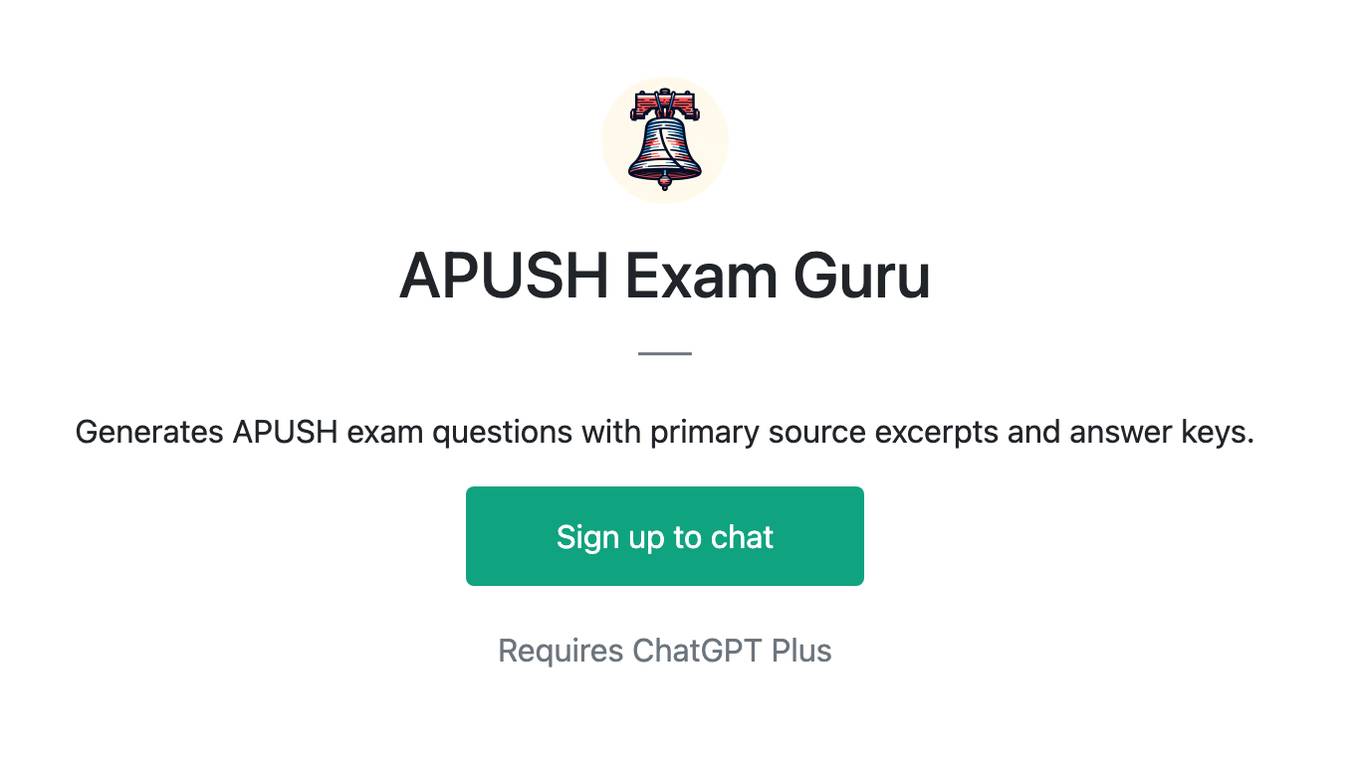
APUSH Exam Guru
Generates APUSH exam questions with primary source excerpts and answer keys.
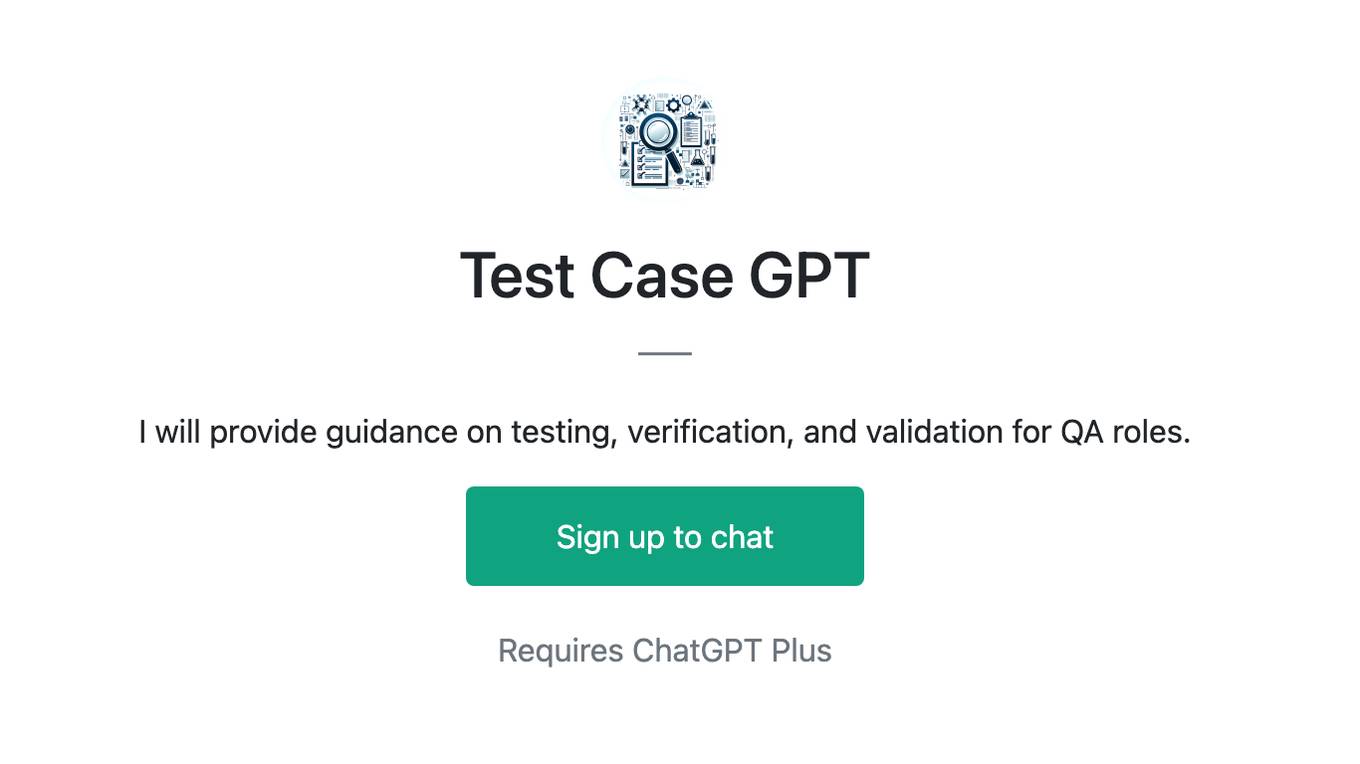
Test Case GPT
I will provide guidance on testing, verification, and validation for QA roles.