Best AI tools for< Mlops Engineer >
Infographic
20 - AI tool Sites
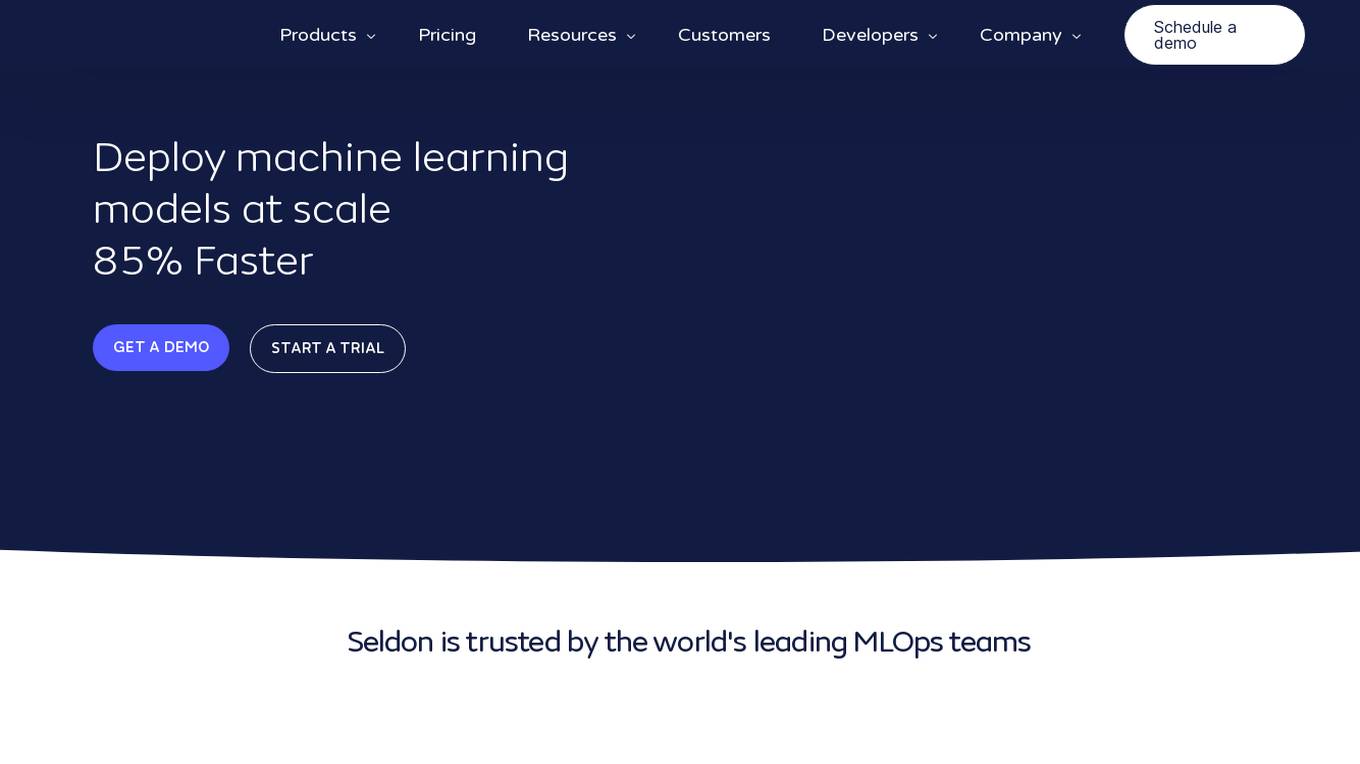
Seldon
Seldon is an MLOps platform that helps enterprises deploy, monitor, and manage machine learning models at scale. It provides a range of features to help organizations accelerate model deployment, optimize infrastructure resource allocation, and manage models and risk. Seldon is trusted by the world's leading MLOps teams and has been used to install and manage over 10 million ML models. With Seldon, organizations can reduce deployment time from months to minutes, increase efficiency, and reduce infrastructure and cloud costs.
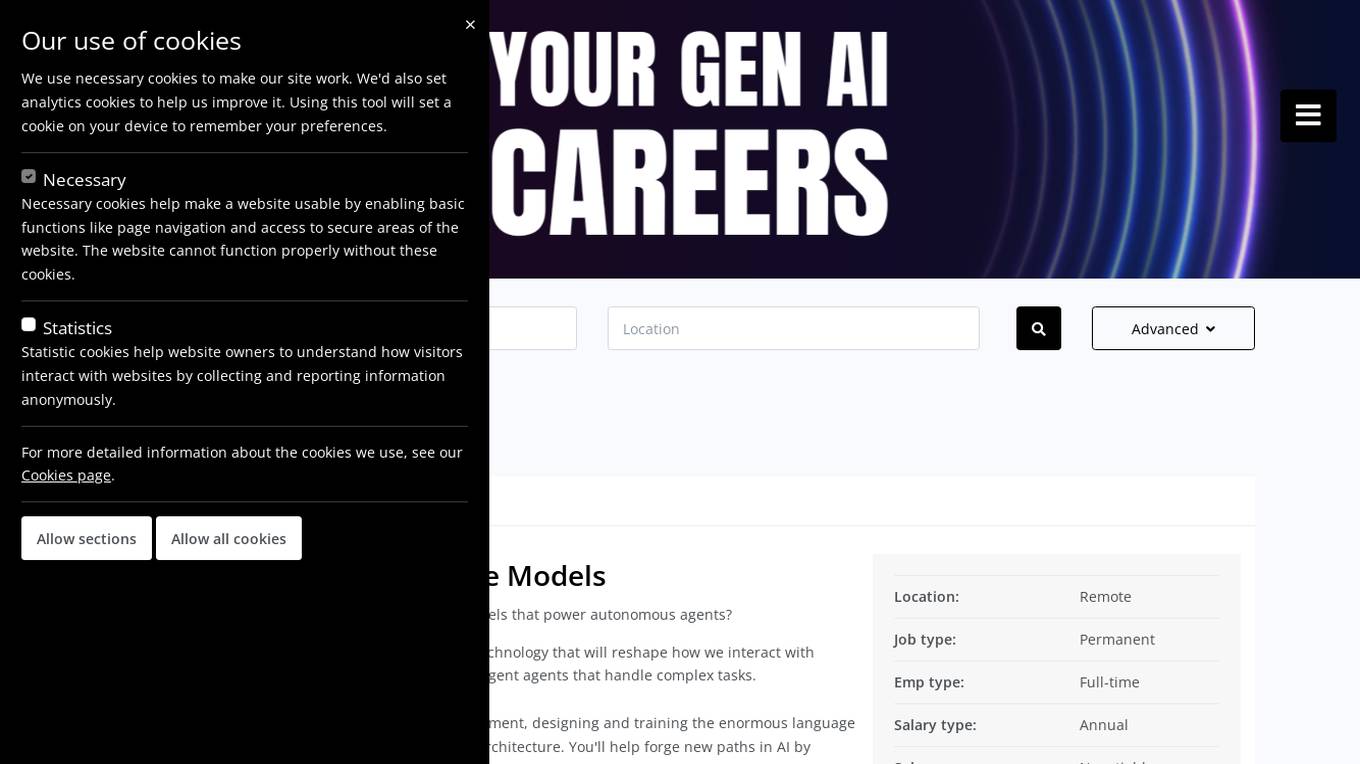
Techire AI Engagement Hub
Techire AI Engagement Hub is an AI application that offers a platform for job seekers and clients to connect in the field of Artificial Intelligence and related industries. The platform focuses on AI technologies such as AGI, AI Agents, Computer Vision, Conversational AI, ML tools, Natural Language Processing, Robotics, and more. It provides job listings, candidate submissions, client vacancies, and advanced search features tailored to the AI industry. Techire AI aims to revolutionize the way individuals interact with AI technologies by offering a diverse range of job opportunities and services.
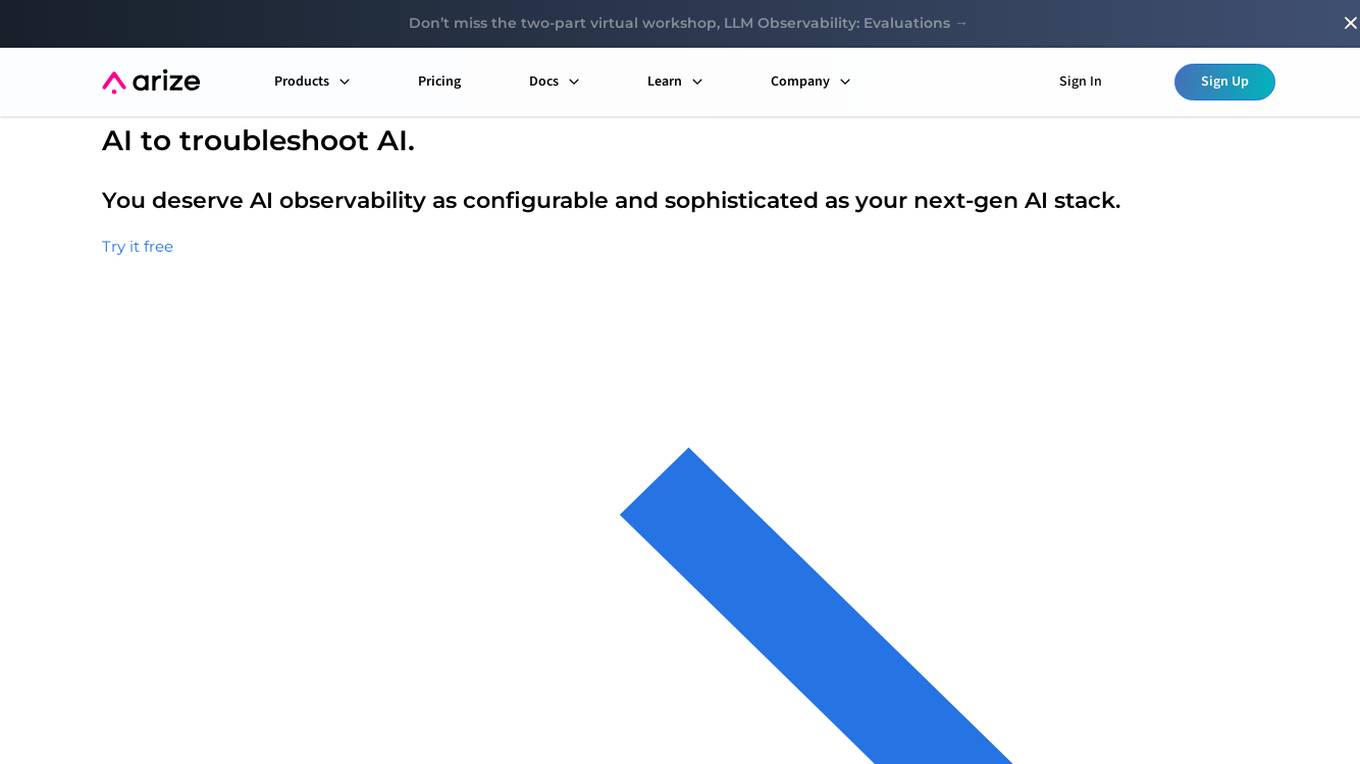
Arize AI
Arize AI is an AI observability tool designed to monitor and troubleshoot AI models in production. It provides configurable and sophisticated observability features to ensure the performance and reliability of next-gen AI stacks. With a focus on ML observability, Arize offers automated setup, a simple API, and a lightweight package for tracking model performance over time. The tool is trusted by top companies for its ability to surface insights, simplify issue root causing, and provide a dedicated customer success manager. Arize is battle-hardened for real-world scenarios, offering unparalleled performance, scalability, security, and compliance with industry standards like SOC 2 Type II and HIPAA.
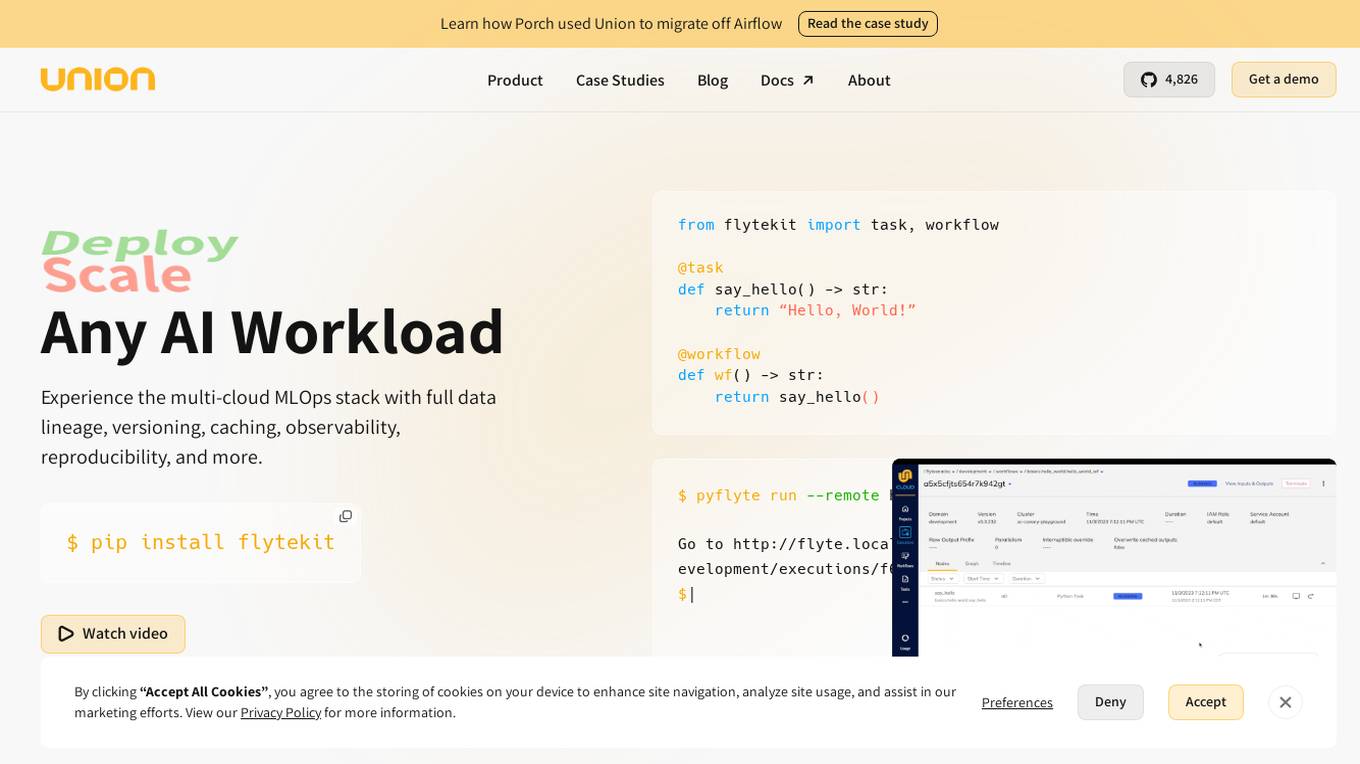
Union.ai
Union.ai is an infrastructure platform designed for AI, ML, and data workloads. It offers a scalable MLOps platform that optimizes resources, reduces costs, and fosters collaboration among team members. Union.ai provides features such as declarative infrastructure, data lineage tracking, accelerated datasets, and more to streamline AI orchestration on Kubernetes. It aims to simplify the management of AI, ML, and data workflows in production environments by addressing complexities and offering cost-effective strategies.
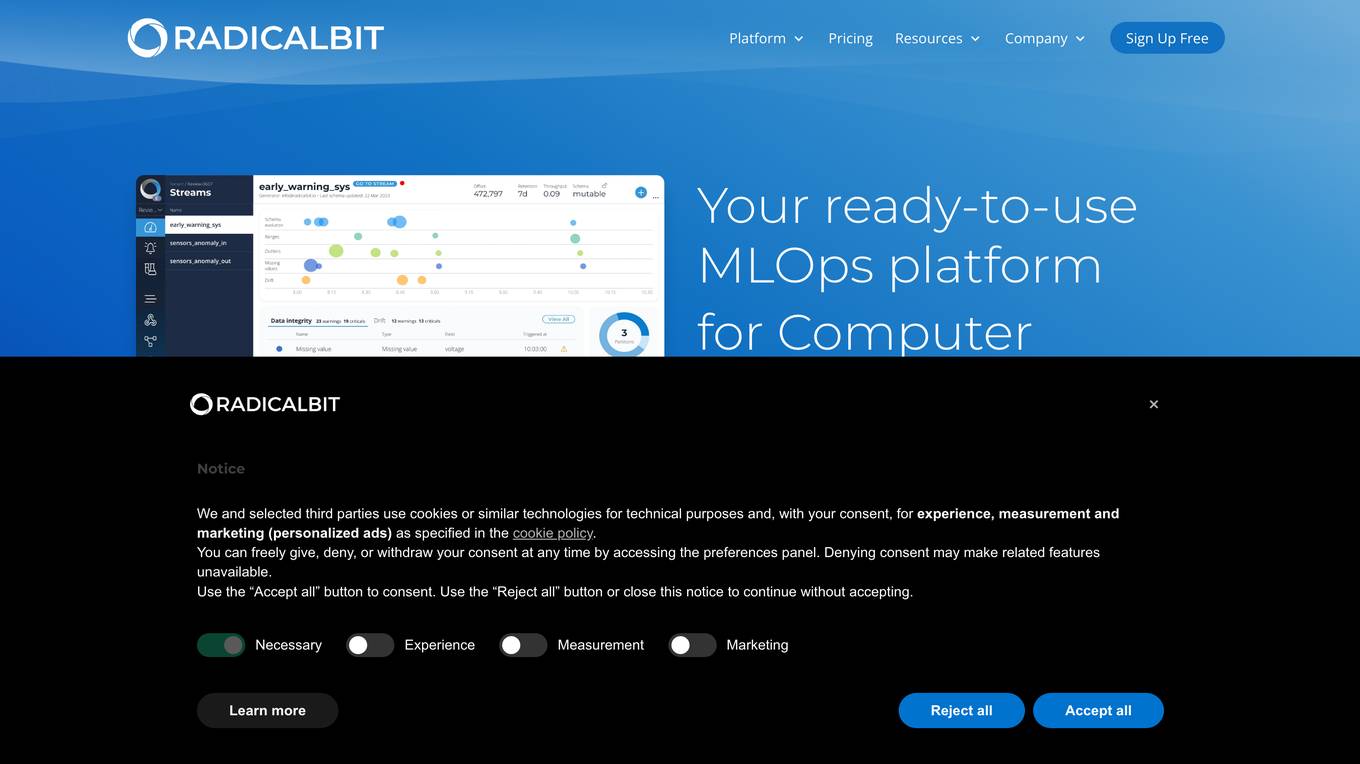
Radicalbit
Radicalbit is an MLOps and AI Observability platform that helps businesses deploy, serve, observe, and explain their AI models. It provides a range of features to help data teams maintain full control over the entire data lifecycle, including real-time data exploration, outlier and drift detection, and model monitoring in production. Radicalbit can be seamlessly integrated into any ML stack, whether SaaS or on-prem, and can be used to run AI applications in minutes.
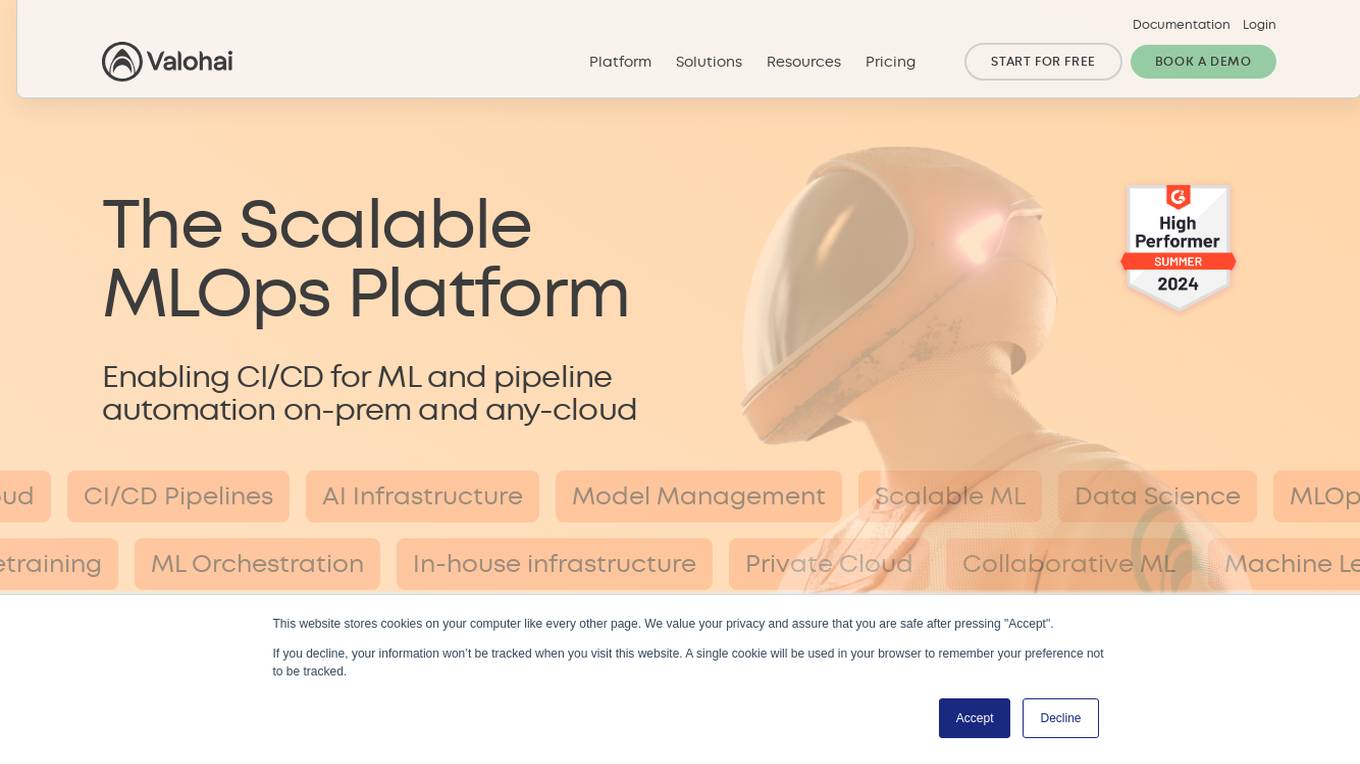
Valohai
Valohai is a scalable MLOps platform that enables Continuous Integration/Continuous Deployment (CI/CD) for machine learning and pipeline automation on-premises and across various cloud environments. It helps streamline complex machine learning workflows by offering framework-agnostic ML capabilities, automatic versioning with complete lineage of ML experiments, hybrid and multi-cloud support, scalability and performance optimization, streamlined collaboration among data scientists, IT, and business units, and smart orchestration of ML workloads on any infrastructure. Valohai also provides a knowledge repository for storing and sharing the entire model lifecycle, facilitating cross-functional collaboration, and allowing developers to build with total freedom using any libraries or frameworks.
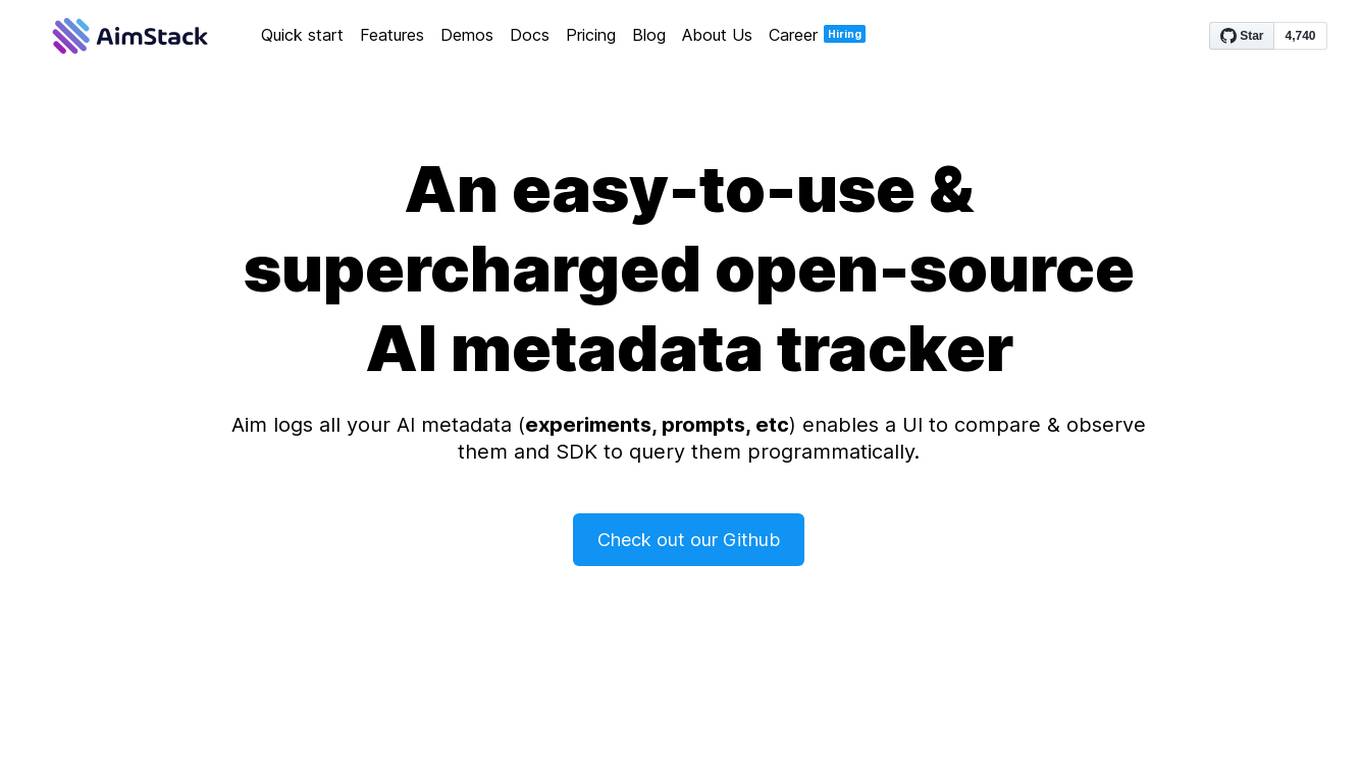
Aim
Aim is an open-source, self-hosted AI Metadata tracking tool designed to handle 100,000s of tracked metadata sequences. Two most famous AI metadata applications are: experiment tracking and prompt engineering. Aim provides a performant and beautiful UI for exploring and comparing training runs, prompt sessions.
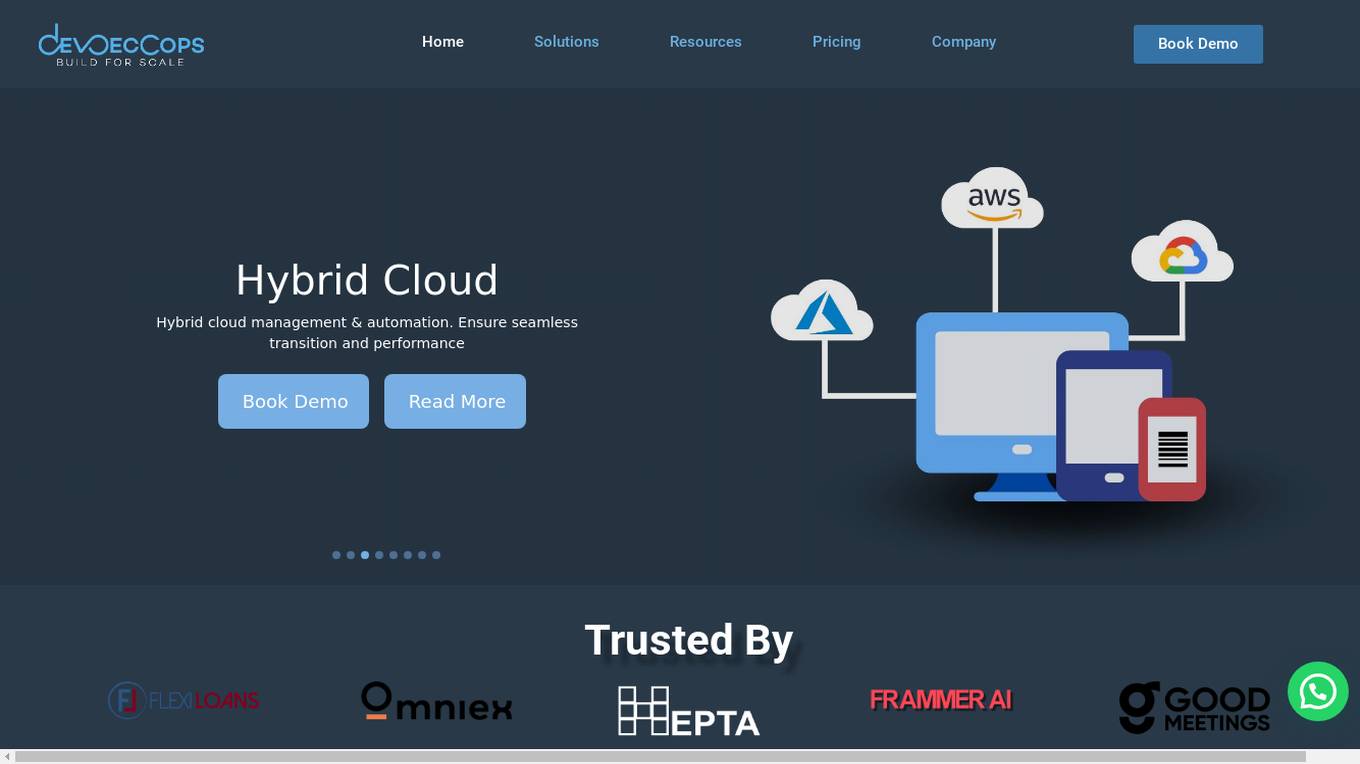
DevSecCops
DevSecCops is an AI-driven automation platform designed to revolutionize DevSecOps processes. The platform offers solutions for cloud optimization, machine learning operations, data engineering, application modernization, infrastructure monitoring, security, compliance, and more. With features like one-click infrastructure security scan, AI engine security fixes, compliance readiness using AI engine, and observability, DevSecCops aims to enhance developer productivity, reduce cloud costs, and ensure secure and compliant infrastructure management. The platform leverages AI technology to identify and resolve security issues swiftly, optimize AI workflows, and provide cost-saving techniques for cloud architecture.
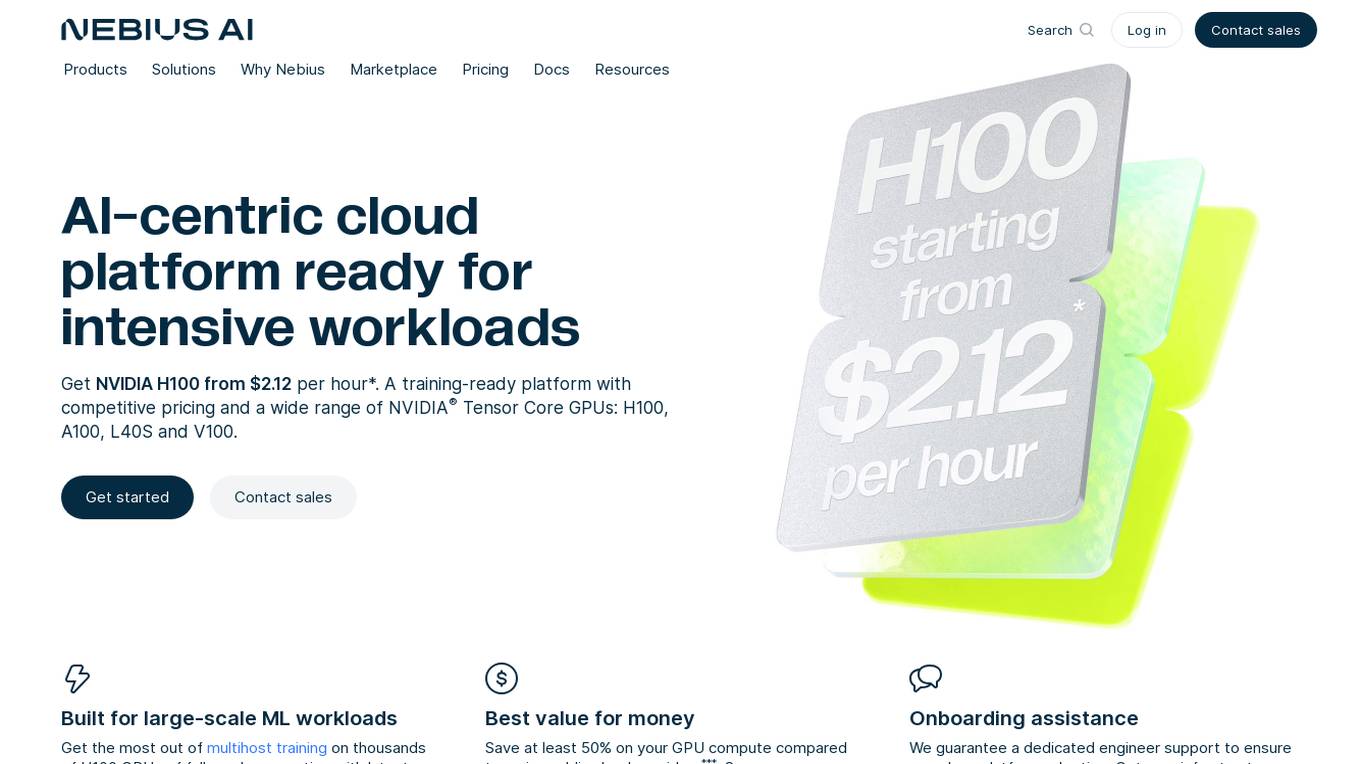
Nebius AI
Nebius AI is an AI-centric cloud platform designed to handle intensive workloads efficiently. It offers a range of advanced features to support various AI applications and projects. The platform ensures high performance and security for users, enabling them to leverage AI technology effectively in their work. With Nebius AI, users can access cutting-edge AI tools and resources to enhance their projects and streamline their workflows.
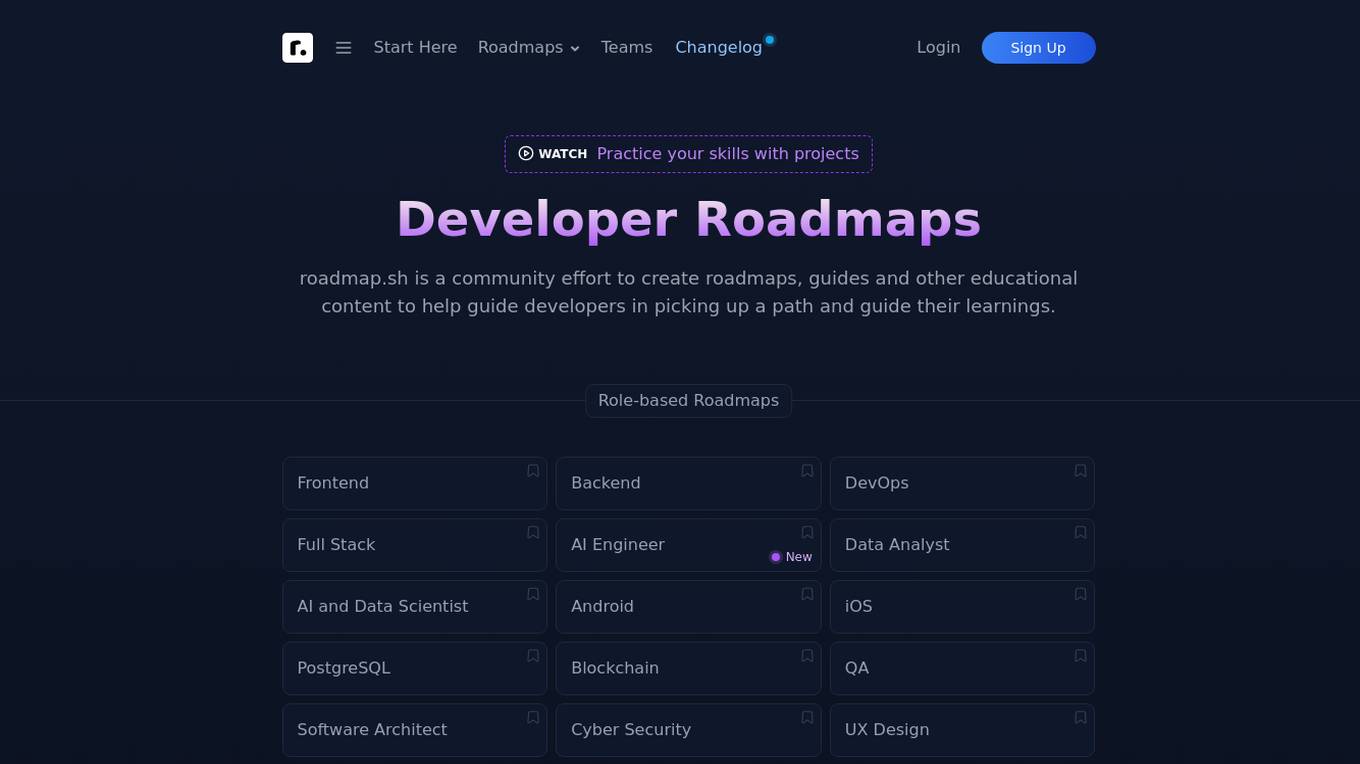
Developer Roadmaps
Developer Roadmaps (roadmap.sh) is a community-driven platform offering official roadmaps, guides, projects, best practices, questions, and videos to assist developers in skill development and career growth. It provides role-based and skill-based roadmaps covering various technologies and domains. The platform is actively maintained and continuously updated to enhance the learning experience for developers worldwide.
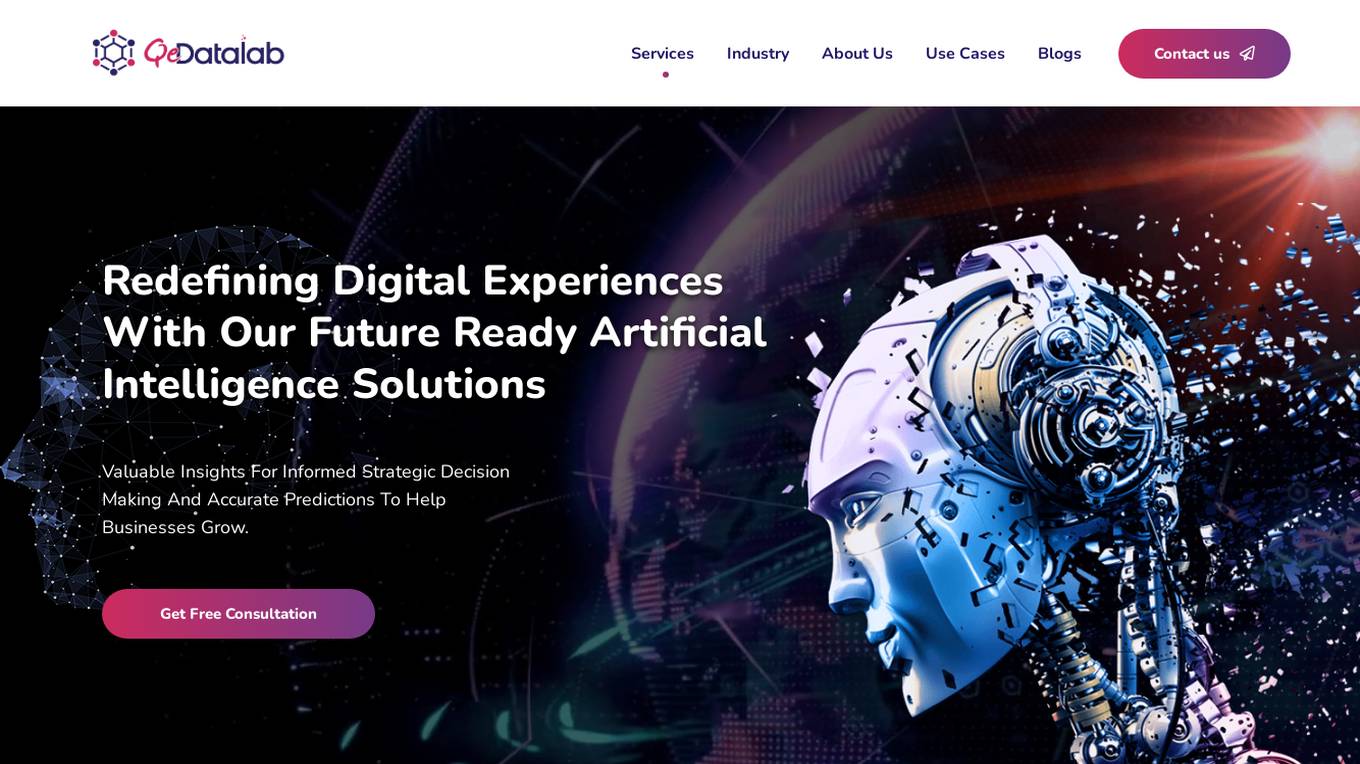
QeDatalab
QeDatalab is a leading data science consulting and AI company offering a wide range of services such as software consulting, generative AI consulting, artificial intelligence services, cloud enablement & automation, AI-driven mobile app development, IoT & IIoT data consulting, digital services, AI product development, MLOps consulting, and more. The company specializes in providing AI-powered solutions for industries like healthcare, manufacturing, retail, and education, helping businesses leverage data for informed strategic decision-making and accurate predictions. QeDatalab's team of experts offers end-to-end services, customized solutions, and a trusted partnership to ensure client success.
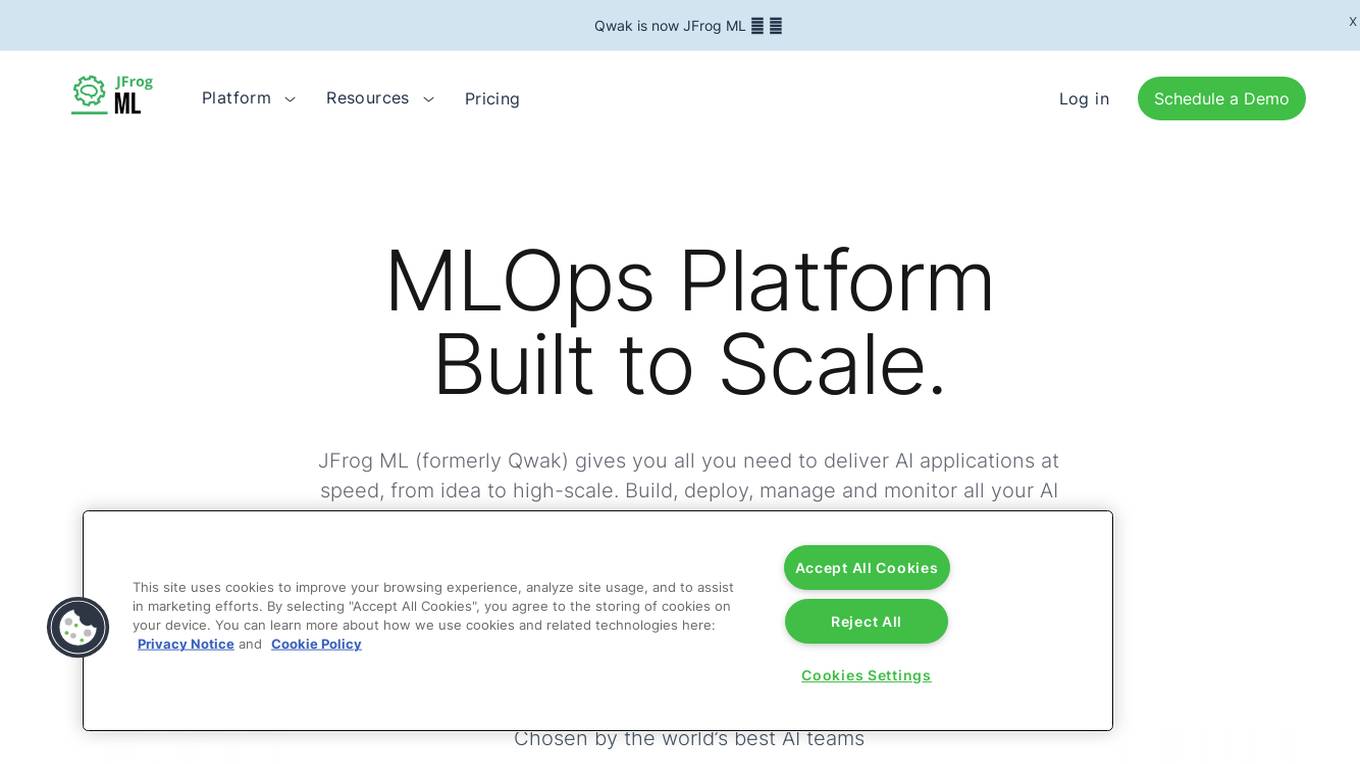
JFrog ML
JFrog ML is an AI platform designed to streamline AI development from prototype to production. It offers a unified MLOps platform to build, train, deploy, and manage AI workflows at scale. With features like Feature Store, LLMOps, and model monitoring, JFrog ML empowers AI teams to collaborate efficiently and optimize AI & ML models in production.
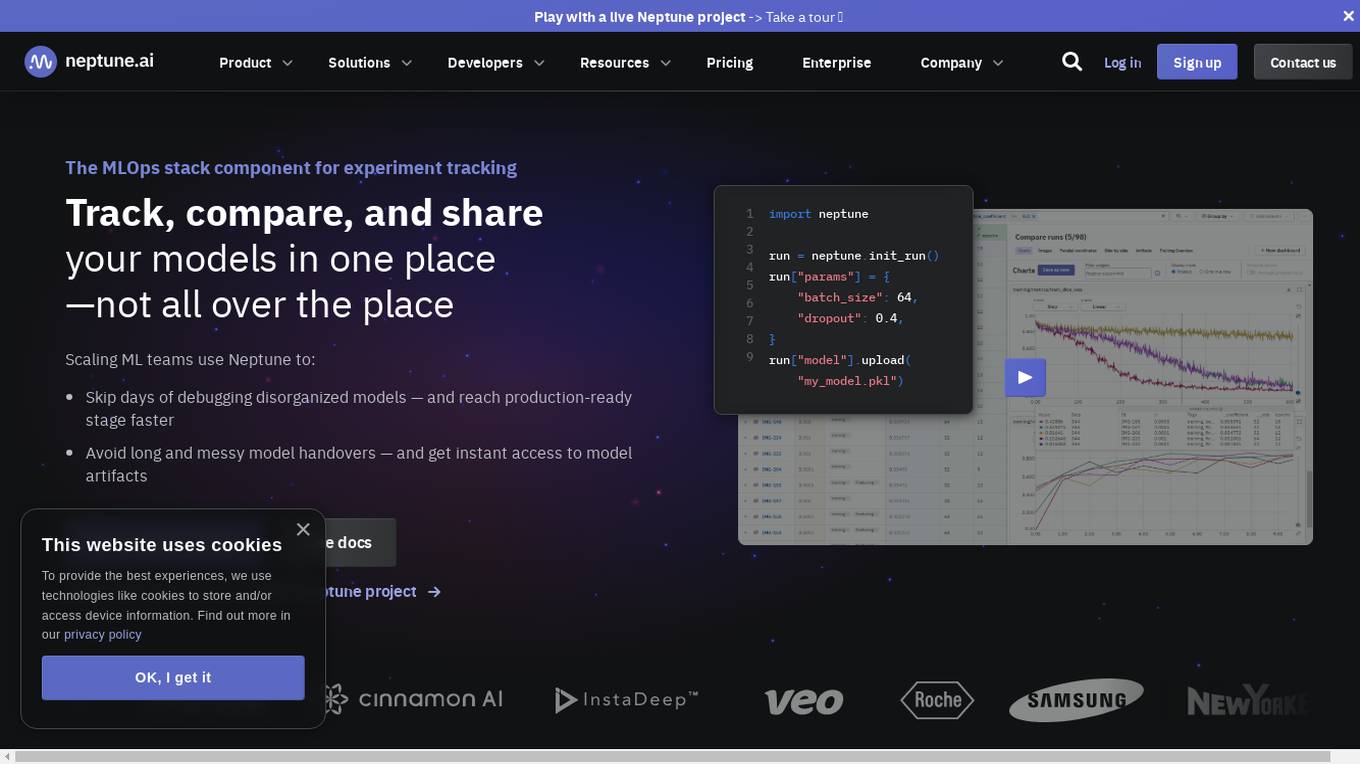
Neptune
Neptune is an MLOps stack component for experiment tracking. It allows users to track, compare, and share their models in one place. Neptune is used by scaling ML teams to skip days of debugging disorganized models, avoid long and messy model handovers, and start logging for free.
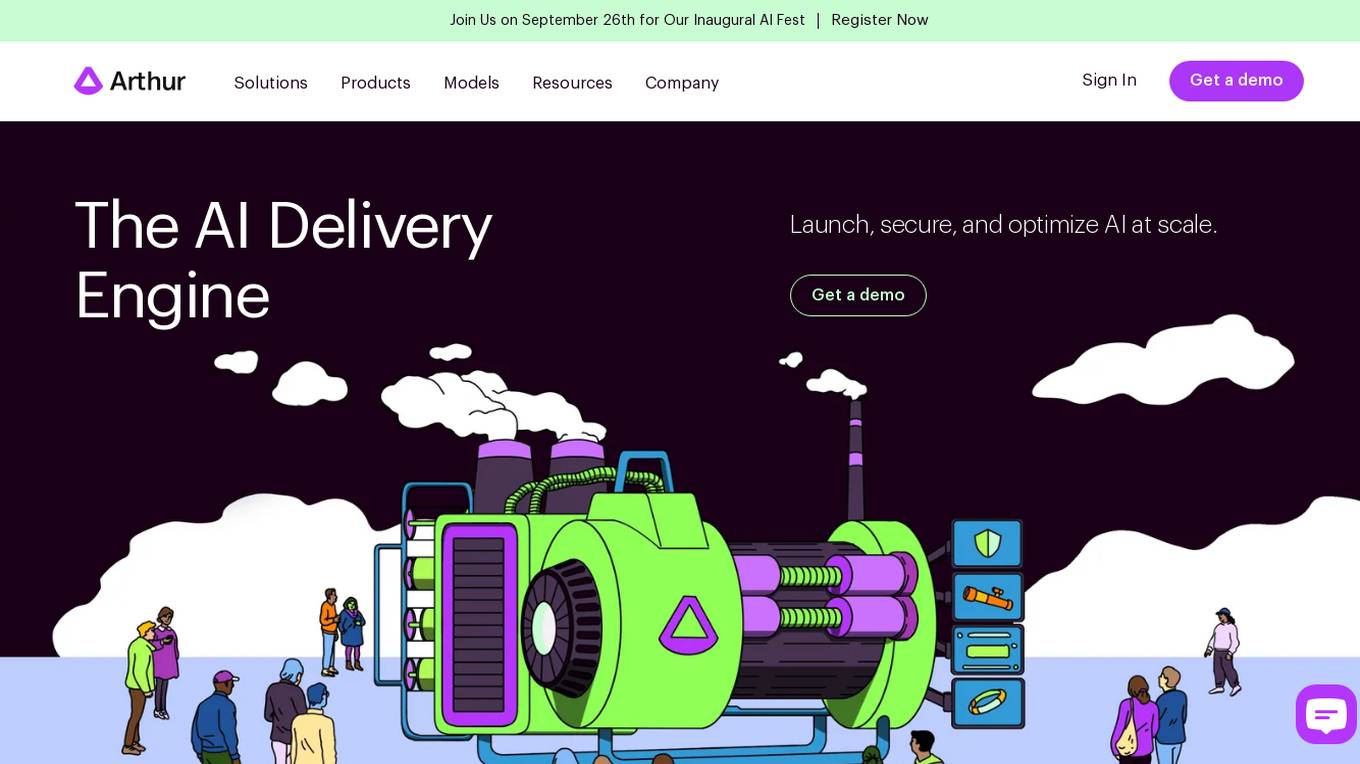
Arthur
Arthur is an industry-leading MLOps platform that simplifies deployment, monitoring, and management of traditional and generative AI models. It ensures scalability, security, compliance, and efficient enterprise use. Arthur's turnkey solutions enable companies to integrate the latest generative AI technologies into their operations, making informed, data-driven decisions. The platform offers open-source evaluation products, model-agnostic monitoring, deployment with leading data science tools, and model risk management capabilities. It emphasizes collaboration, security, and compliance with industry standards.
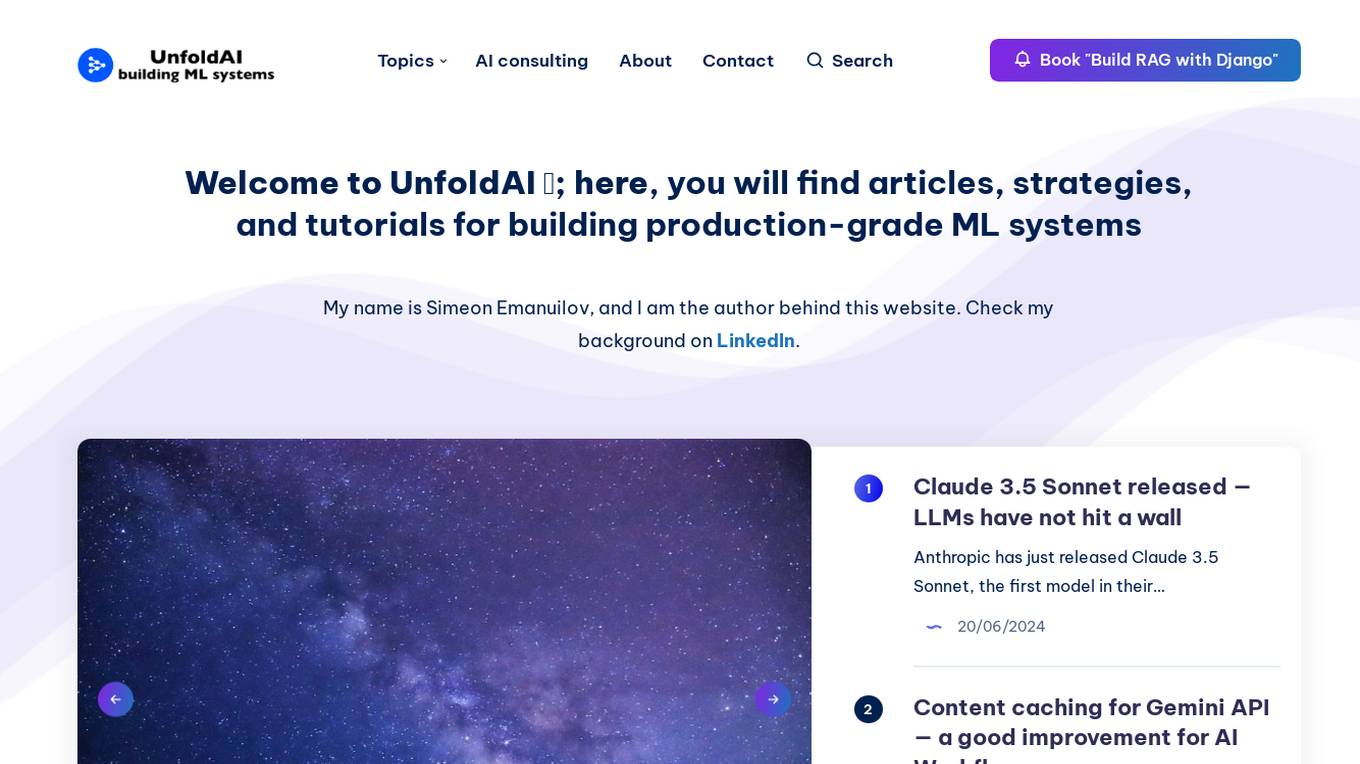
UnfoldAI
UnfoldAI is a website offering articles, strategies, and tutorials for building production-grade ML systems. Authored by Simeon Emanuilov, the site covers topics such as deep learning, computer vision, LLMs, programming, MLOps, performance, scalability, and AI consulting. It aims to provide insights and best practices for professionals in the field of machine learning to create robust, efficient, and scalable systems.
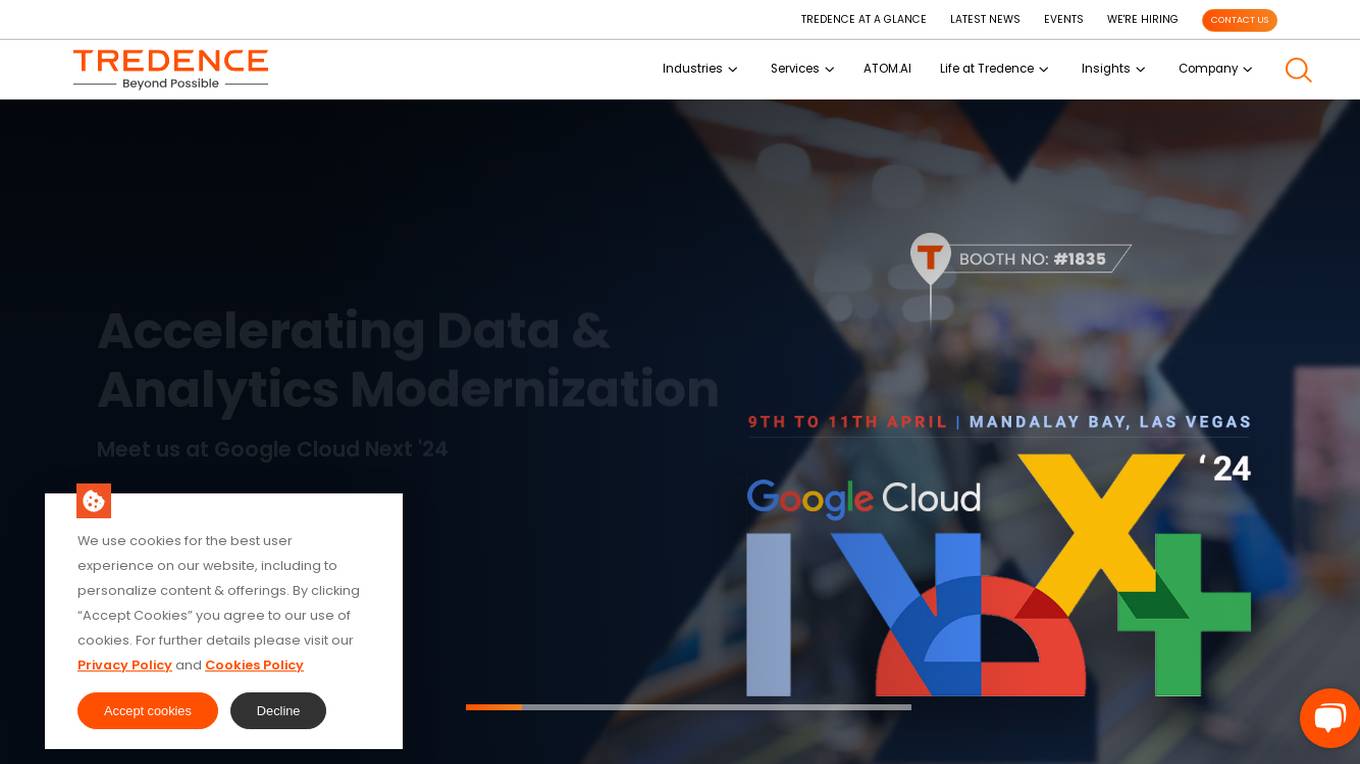
Tredence
Tredence is a data science and AI services company that provides end-to-end solutions for businesses across various industries. The company's services include data engineering, data analytics, AI consulting, and machine learning operations (MLOps). Tredence has a team of experienced data scientists and engineers who use their expertise to help businesses solve complex data challenges and achieve their business goals.
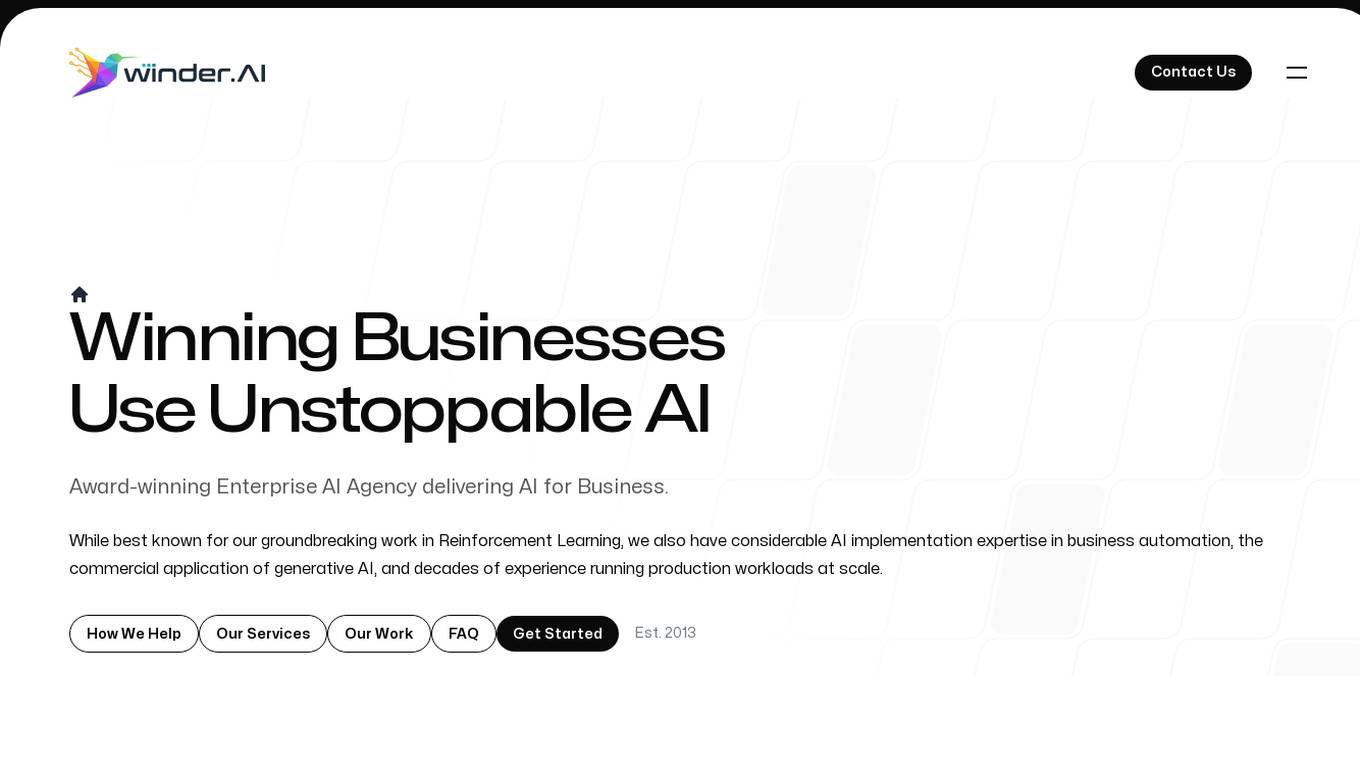
Winder.ai
Winder.ai is an award-winning Enterprise AI Agency that specializes in AI development, consulting, and product development. They have expertise in Reinforcement Learning, MLOps, and Data Science, offering services to help businesses automate processes, scale products, and unlock new markets. With a focus on delivering AI solutions at scale, Winder.ai collaborates with clients globally to enhance operational efficiency and drive innovation through AI technologies.
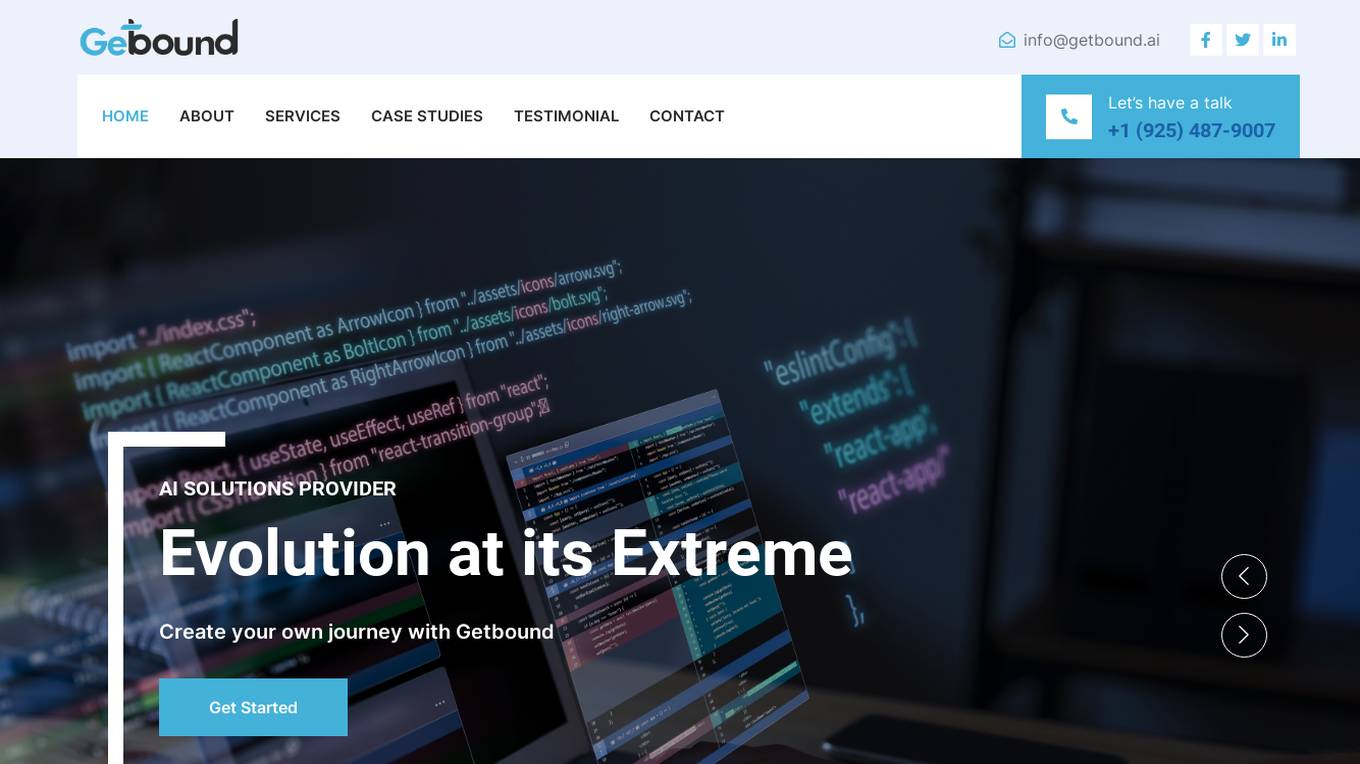
Getbound
Getbound is an AI solutions provider that enables companies to evaluate, customize, and scale technology solutions with artificial intelligence easily and quickly. They offer services such as AI consulting, NLP solutions, MLOps, generative AI development, data engineering services, and computer vision solutions. Getbound empowers businesses to turn data into savings, automate processes, and improve overall performance through AI technologies.
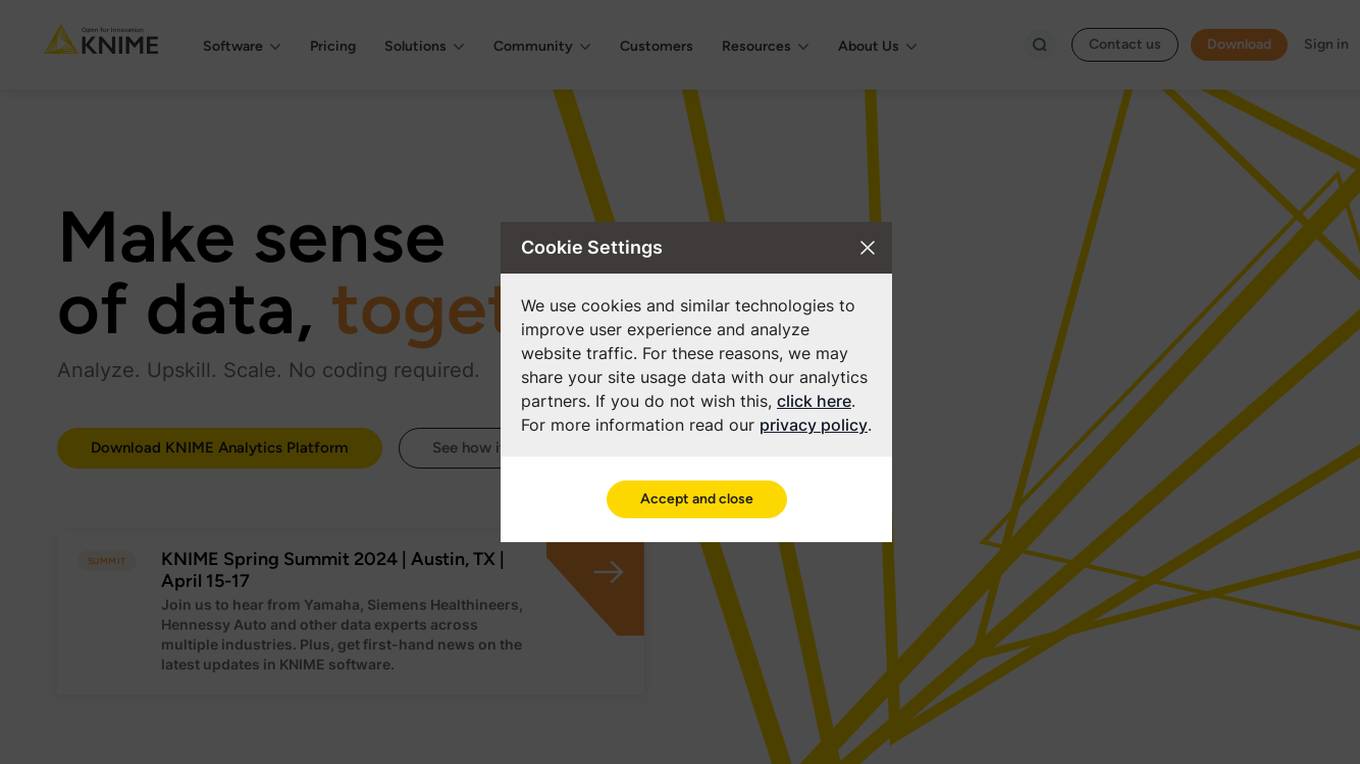
KNIME
KNIME is a data science platform that enables users to analyze, blend, transform, model, visualize, and deploy data science solutions without coding. It provides a range of features and advantages for business and domain experts, data experts, end users, and MLOps & IT professionals across various industries and departments.
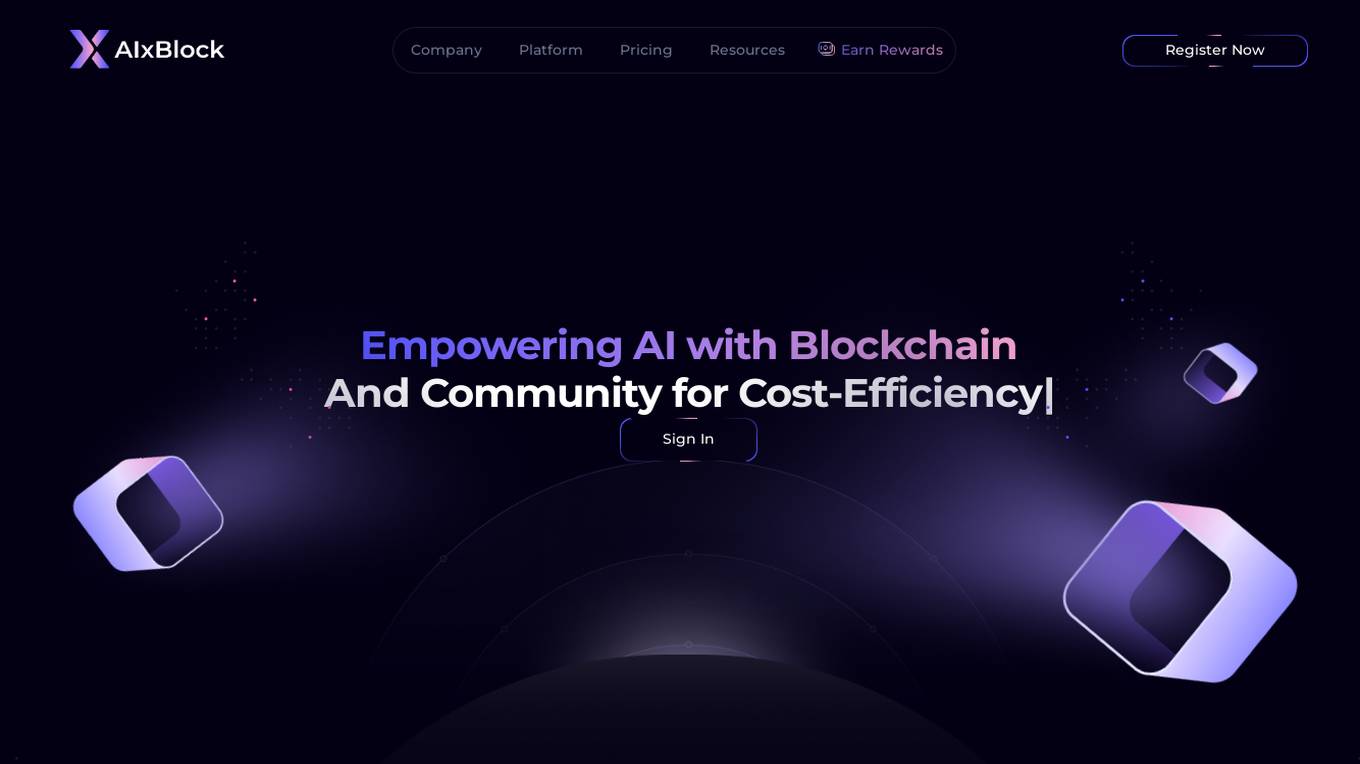
AIxBlock
AIxBlock is an AI tool that empowers users to unleash their AI initiatives on the Blockchain. The platform offers a comprehensive suite of features for building, deploying, and monitoring AI models, including AI data engine, multimodal-powered data crawler, auto annotation, consensus-driven labeling, MLOps platform, decentralized marketplaces, and more. By harnessing the power of blockchain technology, AIxBlock provides cost-efficient solutions for AI builders, compute suppliers, and freelancers to collaborate and benefit from decentralized supercomputing, P2P transactions, and consensus mechanisms.
20 - Open Source Tools
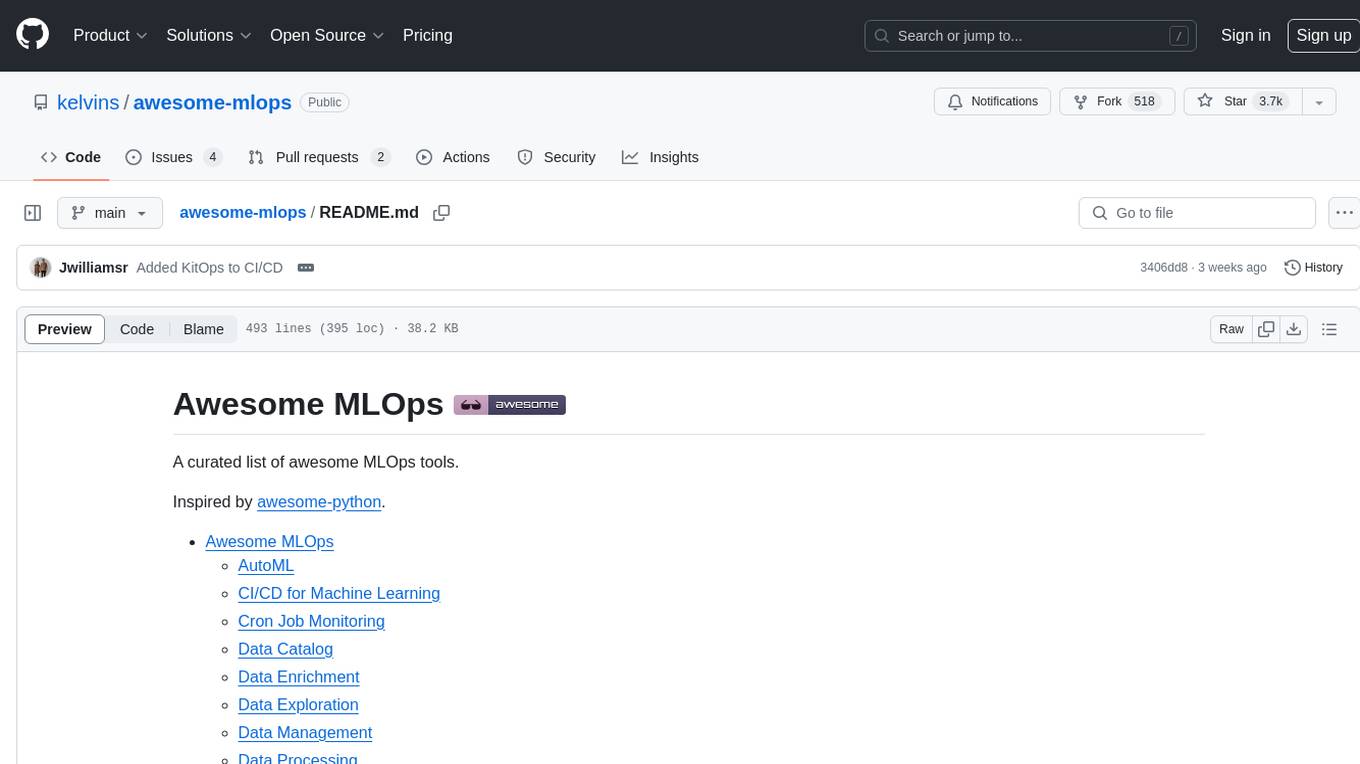
awesome-mlops
Awesome MLOps is a curated list of tools related to Machine Learning Operations, covering areas such as AutoML, CI/CD for Machine Learning, Data Cataloging, Data Enrichment, Data Exploration, Data Management, Data Processing, Data Validation, Data Visualization, Drift Detection, Feature Engineering, Feature Store, Hyperparameter Tuning, Knowledge Sharing, Machine Learning Platforms, Model Fairness and Privacy, Model Interpretability, Model Lifecycle, Model Serving, Model Testing & Validation, Optimization Tools, Simplification Tools, Visual Analysis and Debugging, and Workflow Tools. The repository provides a comprehensive collection of tools and resources for individuals and teams working in the field of MLOps.
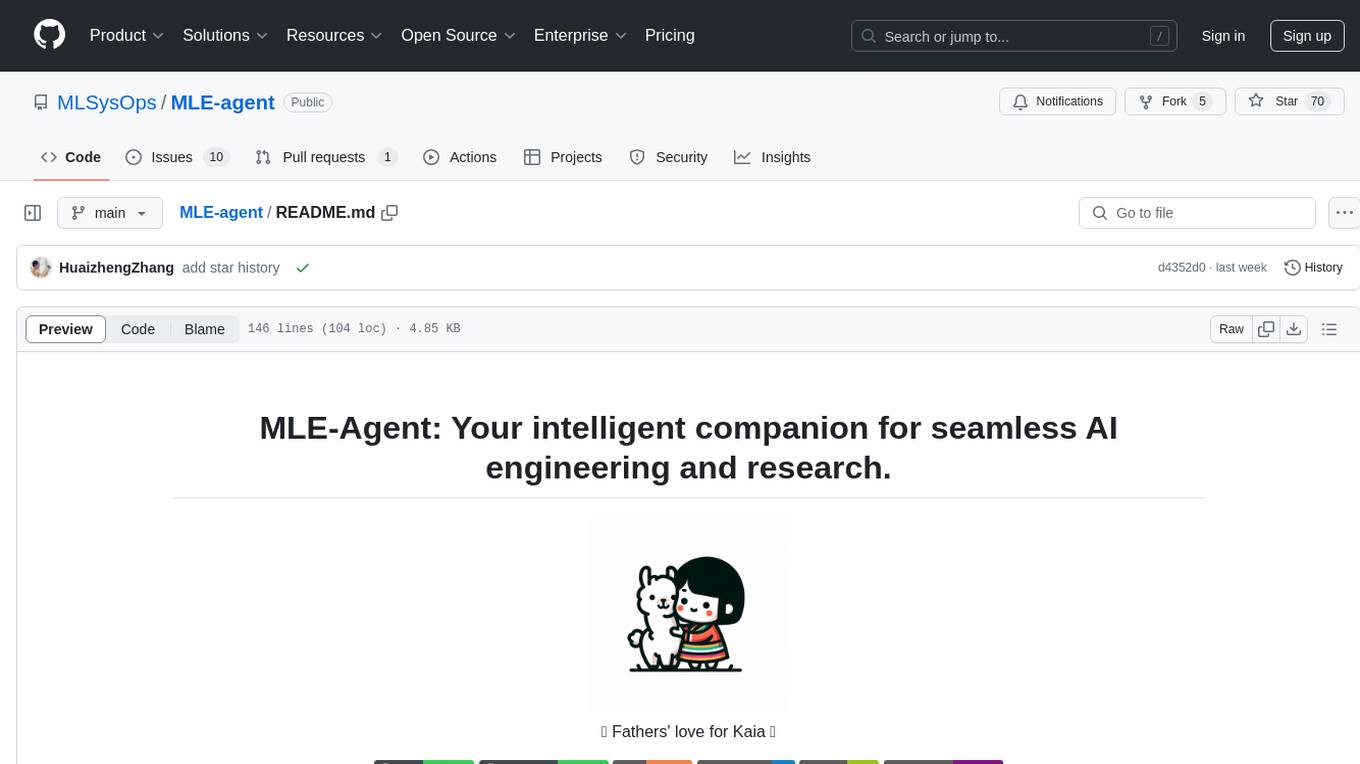
MLE-agent
MLE-Agent is an intelligent companion designed for machine learning engineers and researchers. It features autonomous baseline creation, integration with Arxiv and Papers with Code, smart debugging, file system organization, comprehensive tools integration, and an interactive CLI chat interface for seamless AI engineering and research workflows.
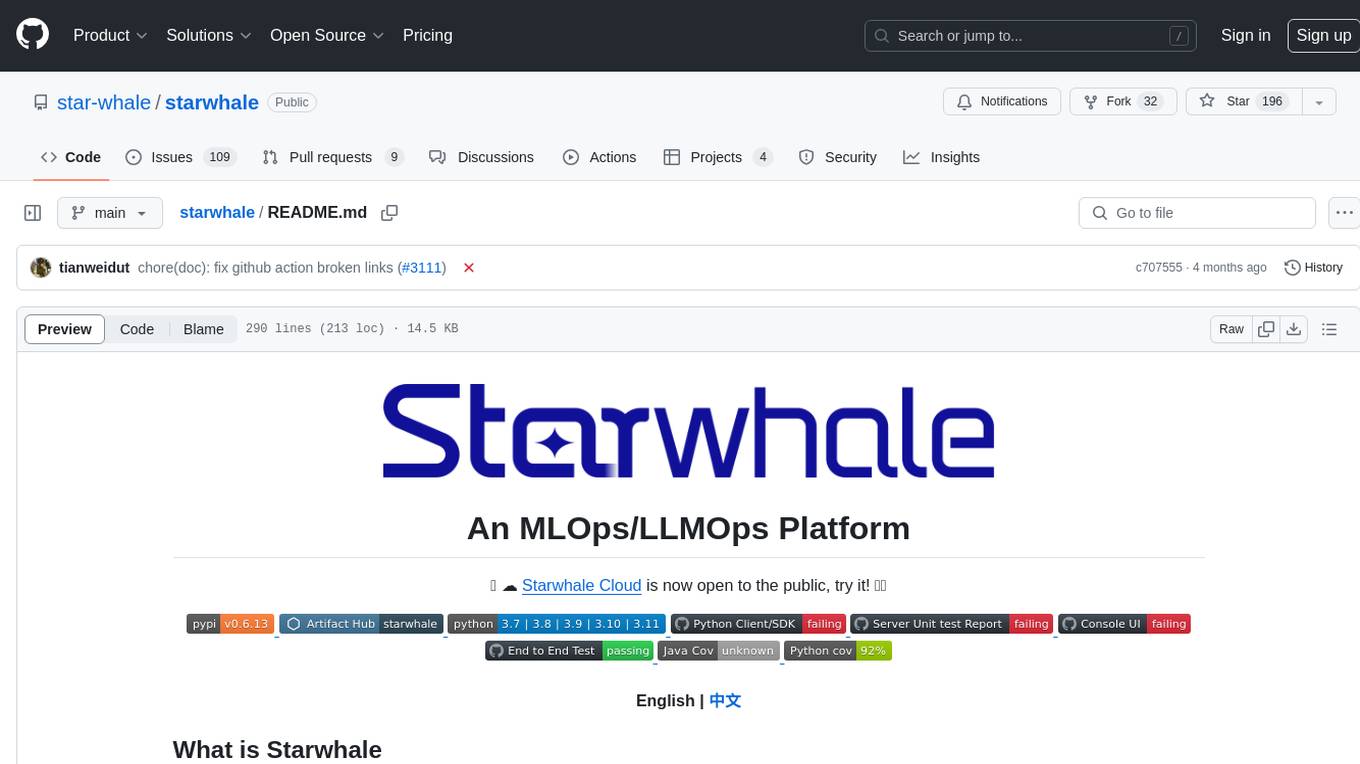
starwhale
Starwhale is an MLOps/LLMOps platform that brings efficiency and standardization to machine learning operations. It streamlines the model development lifecycle, enabling teams to optimize workflows around key areas like model building, evaluation, release, and fine-tuning. Starwhale abstracts Model, Runtime, and Dataset as first-class citizens, providing tailored capabilities for common workflow scenarios including Models Evaluation, Live Demo, and LLM Fine-tuning. It is an open-source platform designed for clarity and ease of use, empowering developers to build customized MLOps features tailored to their needs.
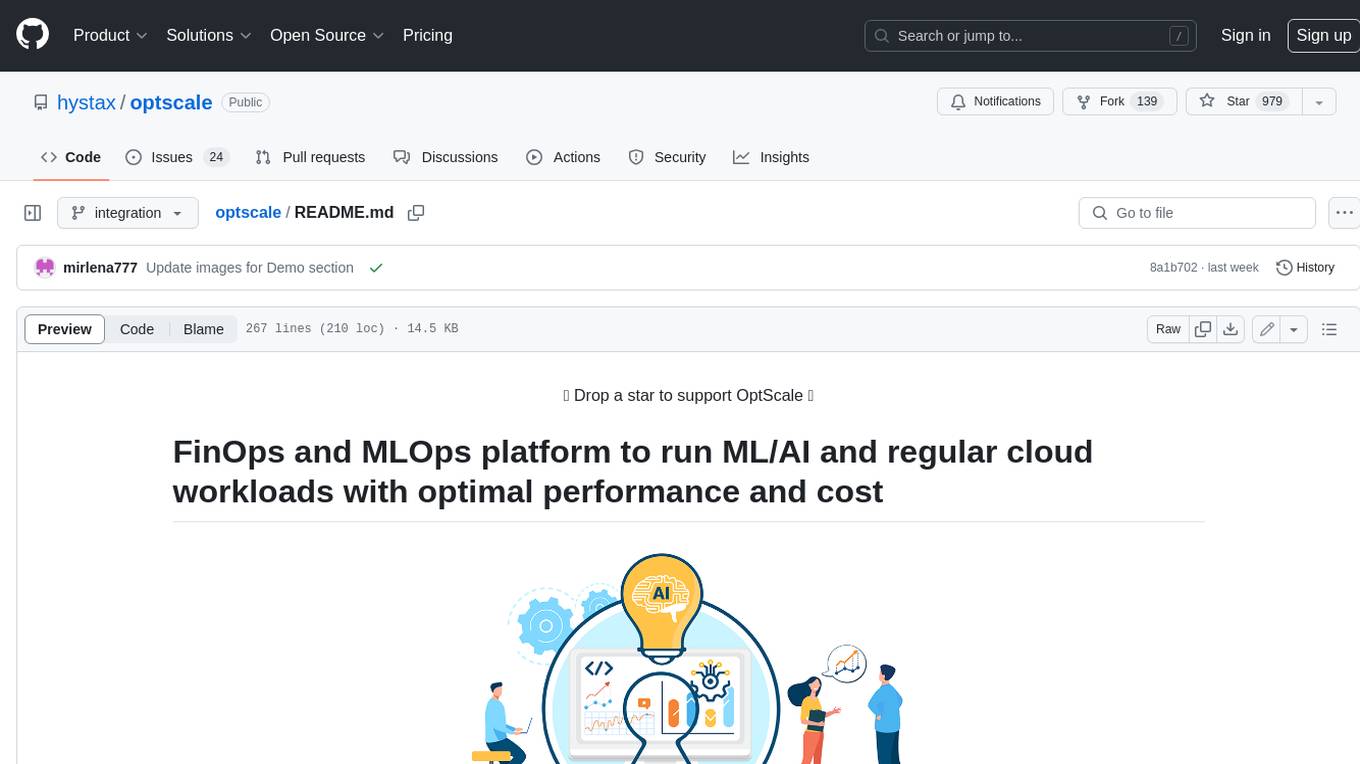
optscale
OptScale is an open-source FinOps and MLOps platform that provides cloud cost optimization for all types of organizations and MLOps capabilities like experiment tracking, model versioning, ML leaderboards.
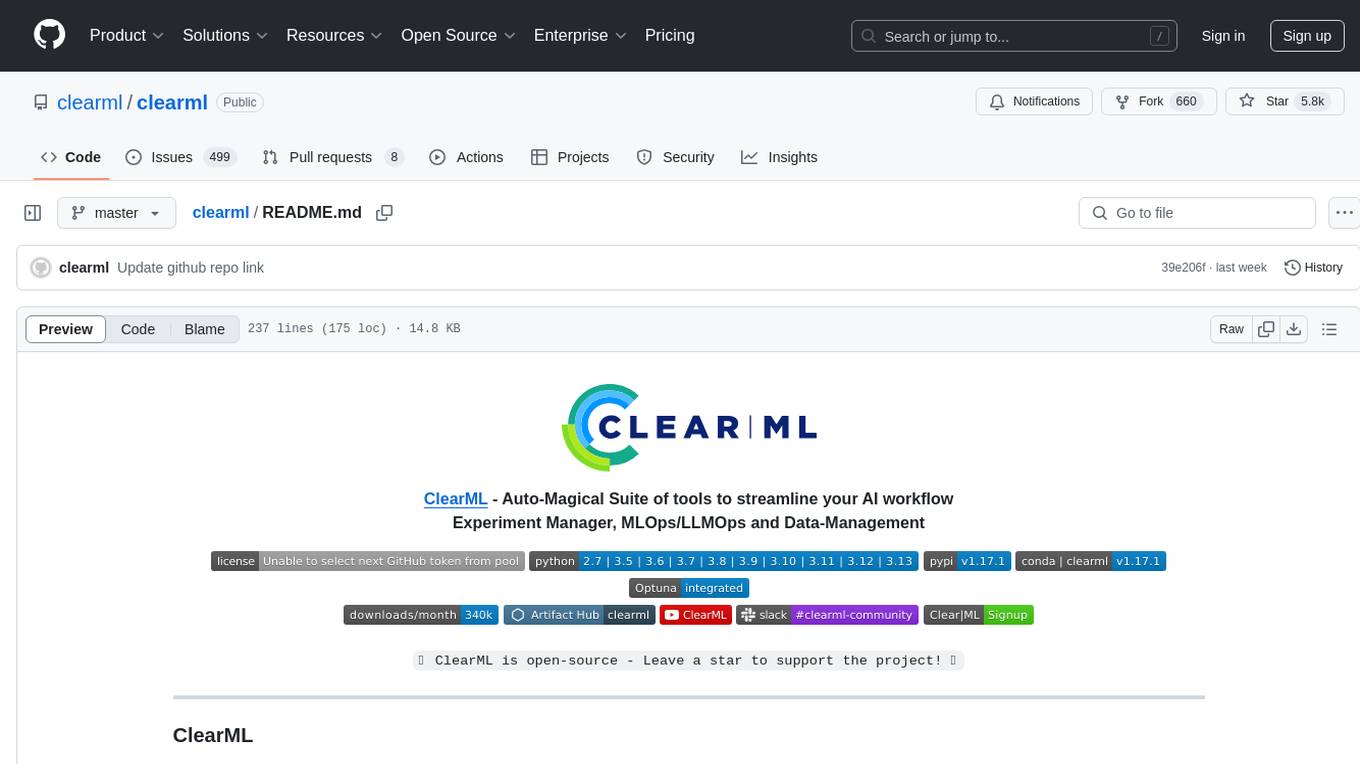
clearml
ClearML is an auto-magical suite of tools designed to streamline AI workflows. It includes modules for experiment management, MLOps/LLMOps, data management, model serving, and more. ClearML offers features like experiment tracking, model serving, orchestration, and automation. It supports various ML/DL frameworks and integrates with Jupyter Notebook and PyCharm for remote debugging. ClearML aims to simplify collaboration, automate processes, and enhance visibility in AI projects.
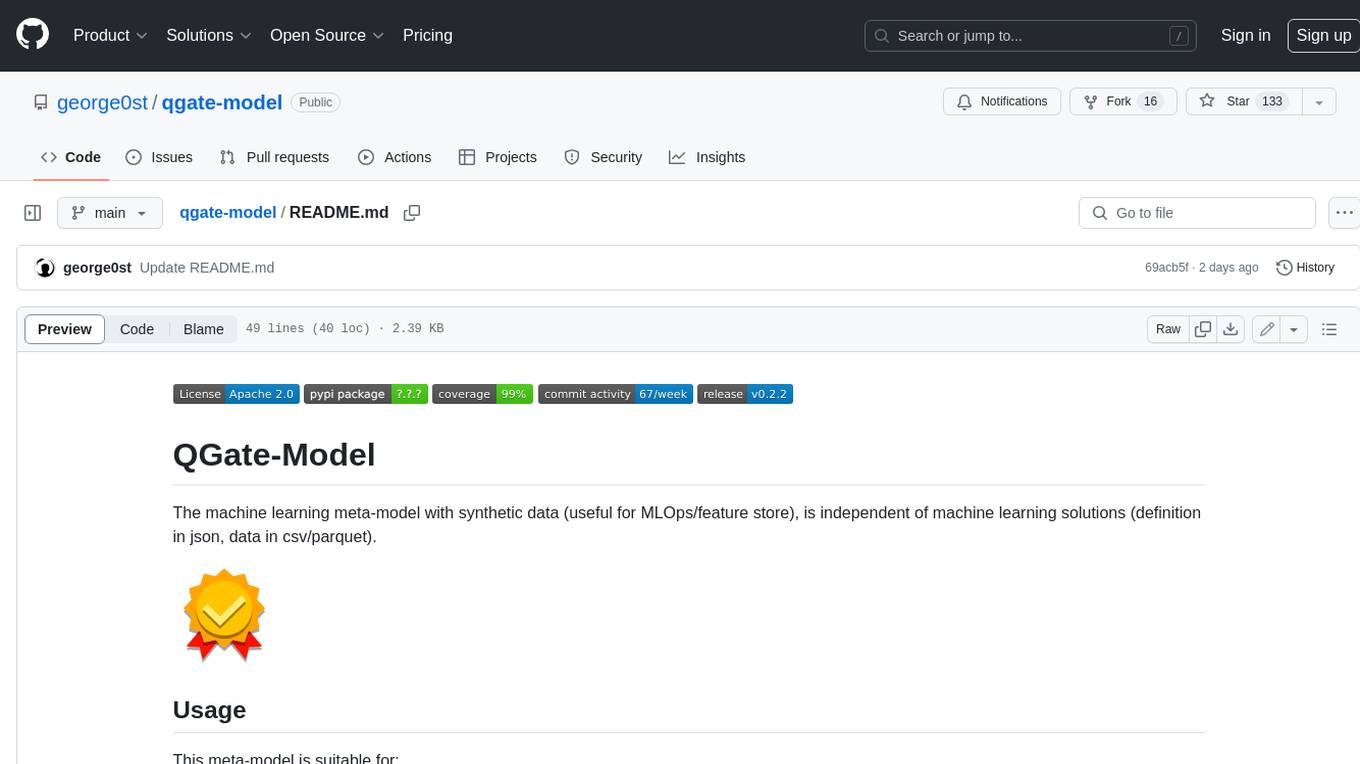
qgate-model
QGate-Model is a machine learning meta-model with synthetic data, designed for MLOps and feature store. It is independent of machine learning solutions, with definitions in JSON and data in CSV/parquet formats. This meta-model is useful for comparing capabilities and functions of machine learning solutions, independently testing new versions of machine learning solutions, and conducting various types of tests (unit, sanity, smoke, system, regression, function, acceptance, performance, shadow, etc.). It can also be used for external test coverage when internal test coverage is not available or weak.
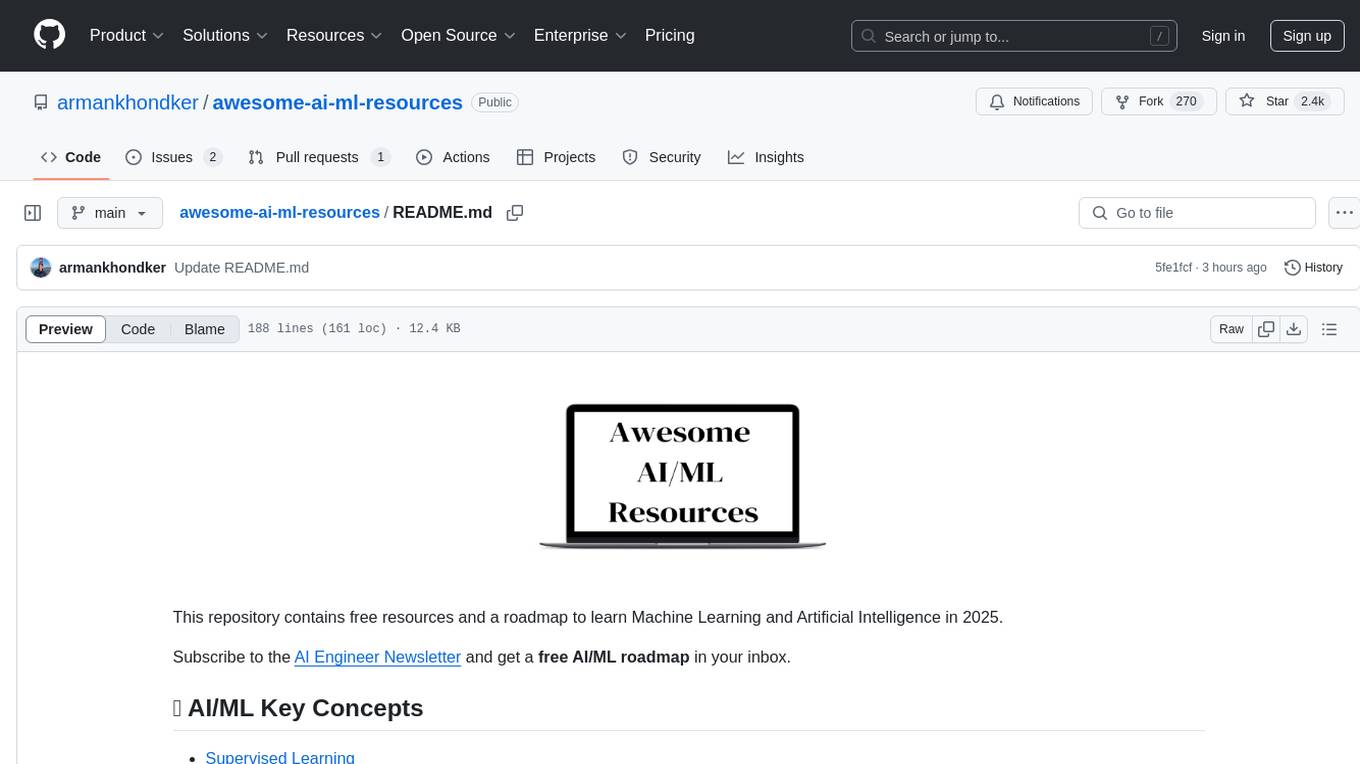
awesome-ai-ml-resources
This repository is a collection of free resources and a roadmap designed to help individuals learn Machine Learning and Artificial Intelligence concepts by providing key concepts, building blocks, roles, a learning roadmap, courses, certifications, books, tools & frameworks, research blogs, applied blogs, practice problems, communities, YouTube channels, newsletters, and must-read papers. It covers a wide range of topics from supervised learning to MLOps, offering guidance on learning paths, practical experience, and job interview preparation.
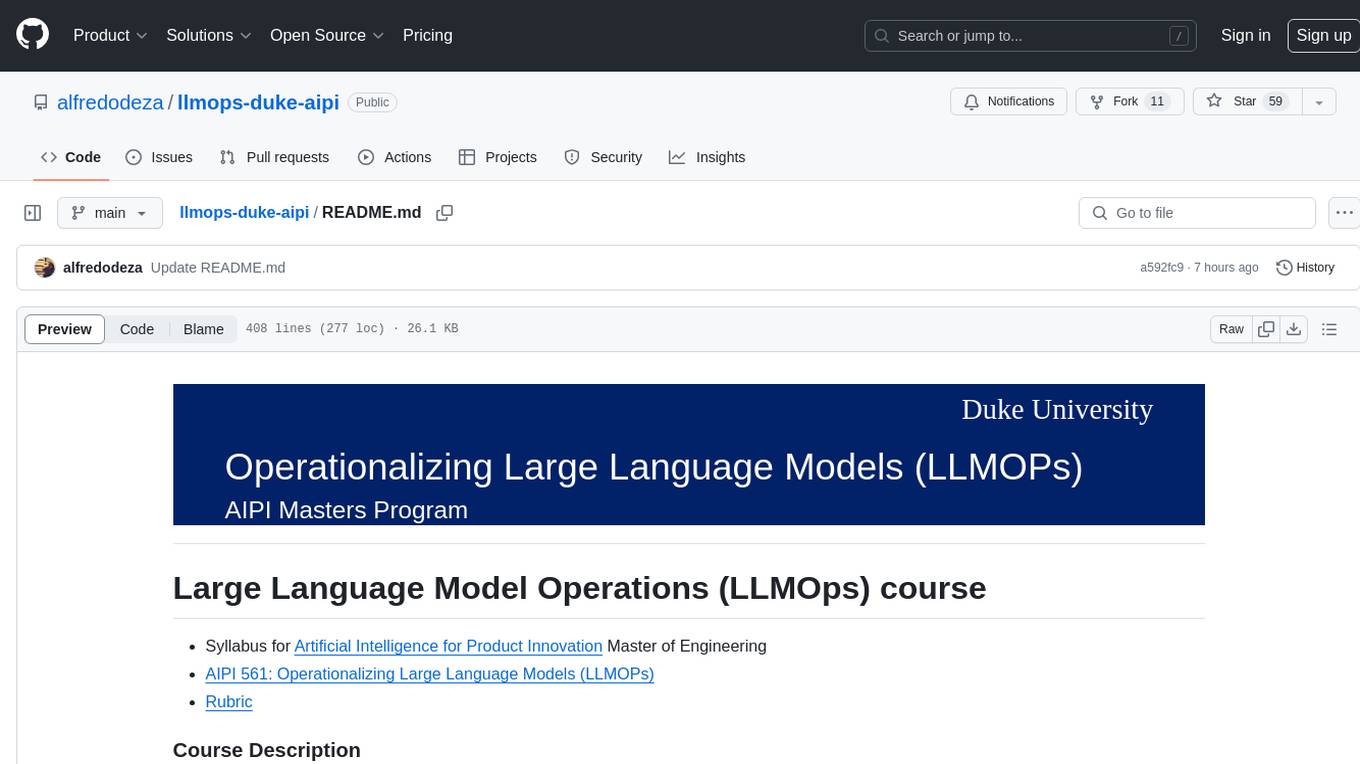
llmops-duke-aipi
LLMOps Duke AIPI is a course focused on operationalizing Large Language Models, teaching methodologies for developing applications using software development best practices with large language models. The course covers various topics such as generative AI concepts, setting up development environments, interacting with large language models, using local large language models, applied solutions with LLMs, extensibility using plugins and functions, retrieval augmented generation, introduction to Python web frameworks for APIs, DevOps principles, deploying machine learning APIs, LLM platforms, and final presentations. Students will learn to build, share, and present portfolios using Github, YouTube, and Linkedin, as well as develop non-linear life-long learning skills. Prerequisites include basic Linux and programming skills, with coursework available in Python or Rust. Additional resources and references are provided for further learning and exploration.
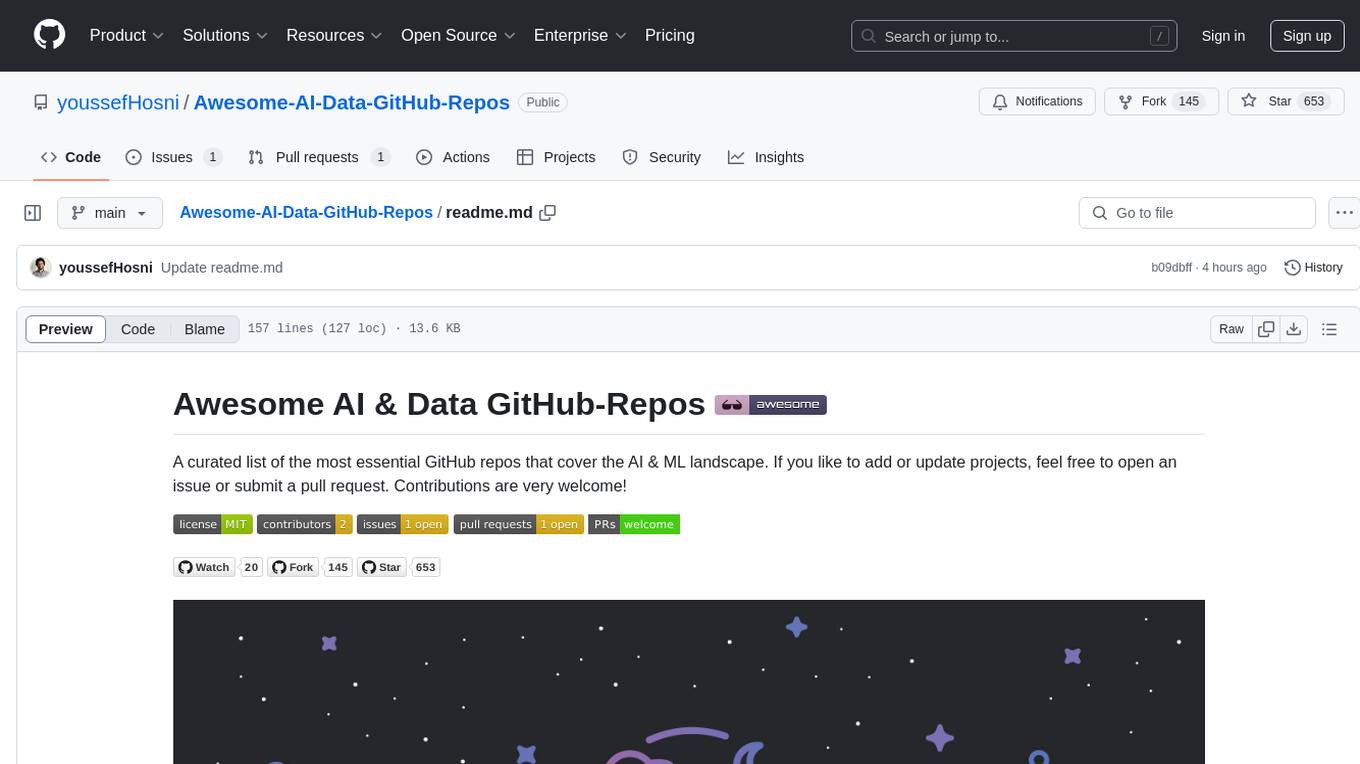
Awesome-AI-Data-GitHub-Repos
Awesome AI & Data GitHub-Repos is a curated list of essential GitHub repositories covering the AI & ML landscape. It includes resources for Natural Language Processing, Large Language Models, Computer Vision, Data Science, Machine Learning, MLOps, Data Engineering, SQL & Database, and Statistics. The repository aims to provide a comprehensive collection of projects and resources for individuals studying or working in the field of AI and data science.
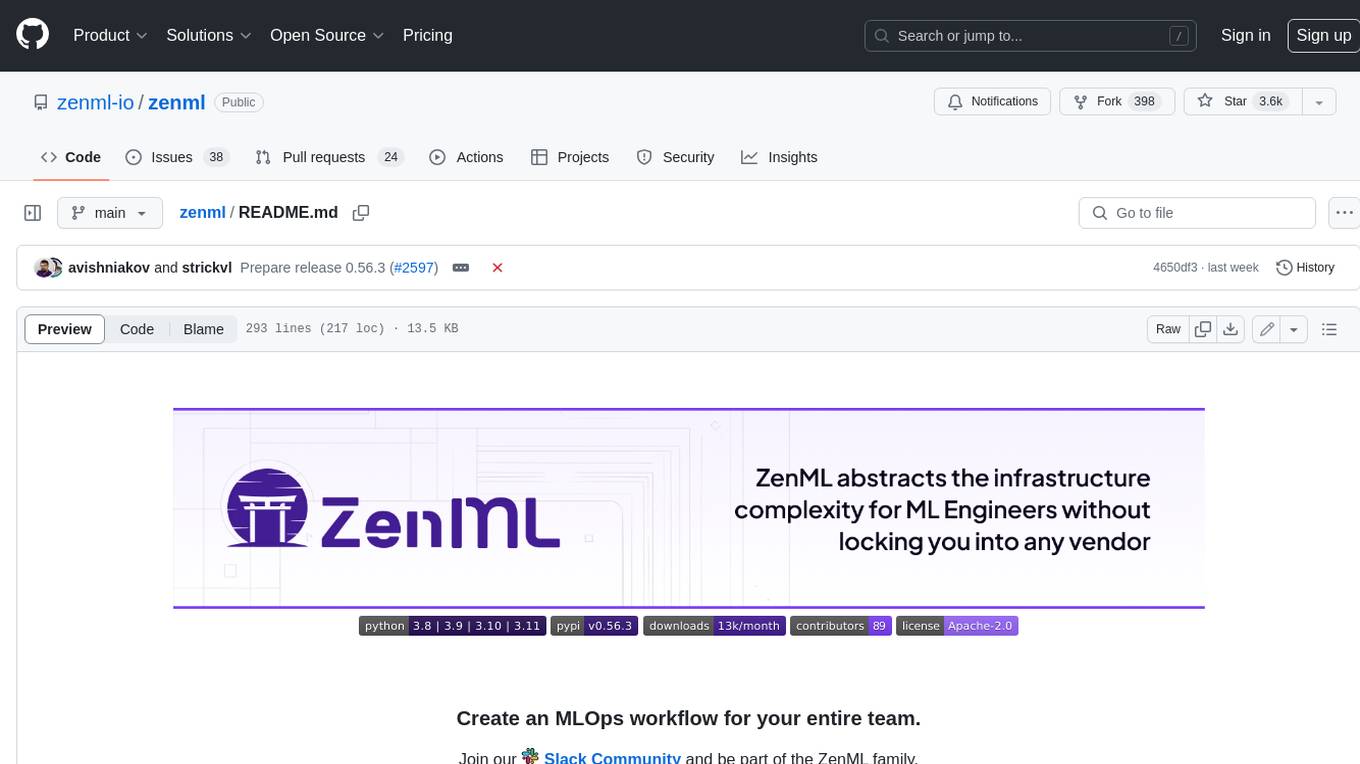
zenml
ZenML is an extensible, open-source MLOps framework for creating portable, production-ready machine learning pipelines. By decoupling infrastructure from code, ZenML enables developers across your organization to collaborate more effectively as they develop to production.
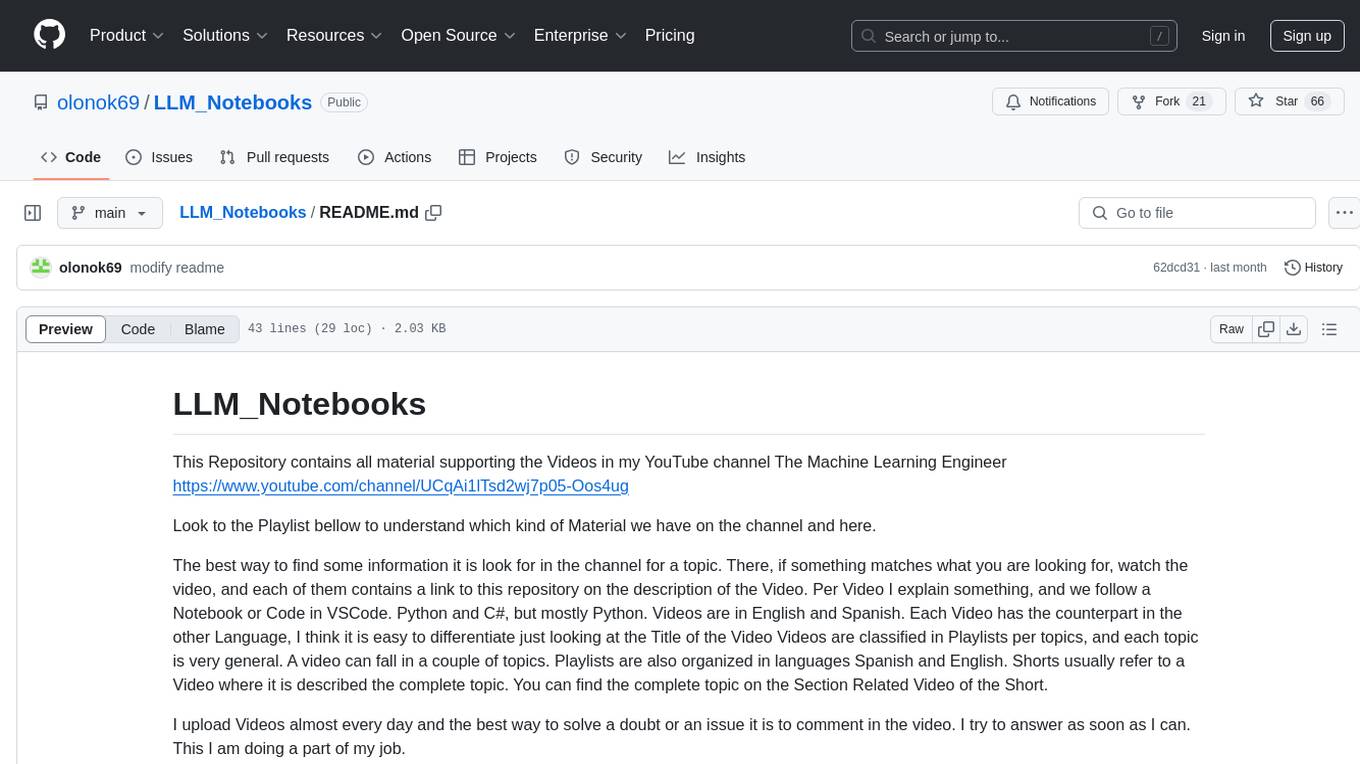
LLM_Notebooks
LLM_Notebooks is a repository supporting The Machine Learning Engineer YouTube channel. It contains materials related to various topics such as Generative AI, MLOps, ML projects, Azure Projects, Google VertexAi, ML Tricks, and more. The repository includes notebooks and code in Python and C#, with a focus on Python. The videos on the channel cover a wide range of topics in English and Spanish, organized into playlists based on general themes. The repository links are provided in the video descriptions for easy access. The creator uploads videos regularly and encourages viewers to subscribe, like, and leave constructive comments. The repository serves as a valuable resource for learning and exploring machine learning concepts and tools.
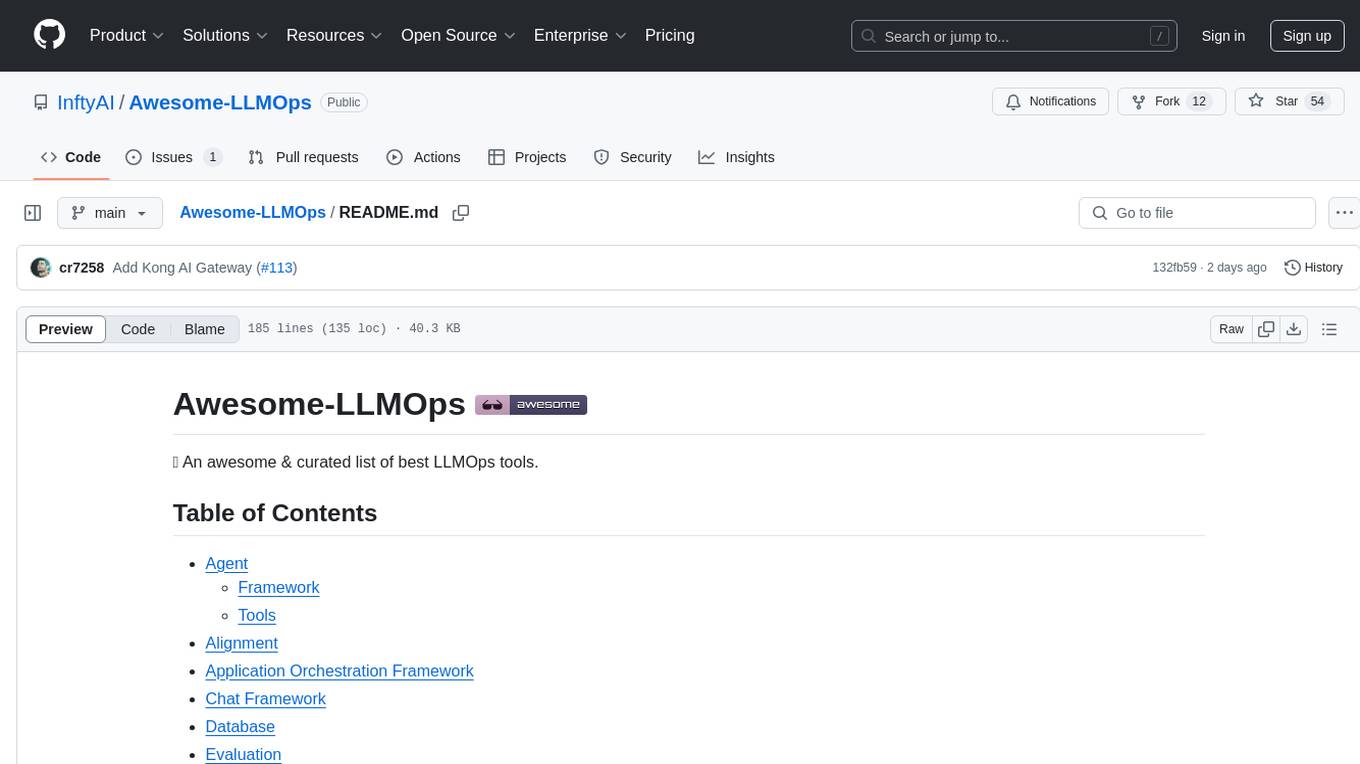
Awesome-LLMOps
Awesome-LLMOps is a curated list of the best LLMOps tools, providing a comprehensive collection of frameworks and tools for building, deploying, and managing large language models (LLMs) and AI agents. The repository includes a wide range of tools for tasks such as building multimodal AI agents, fine-tuning models, orchestrating applications, evaluating models, and serving models for inference. It covers various aspects of the machine learning operations (MLOps) lifecycle, from training to deployment and observability. The tools listed in this repository cater to the needs of developers, data scientists, and machine learning engineers working with large language models and AI applications.
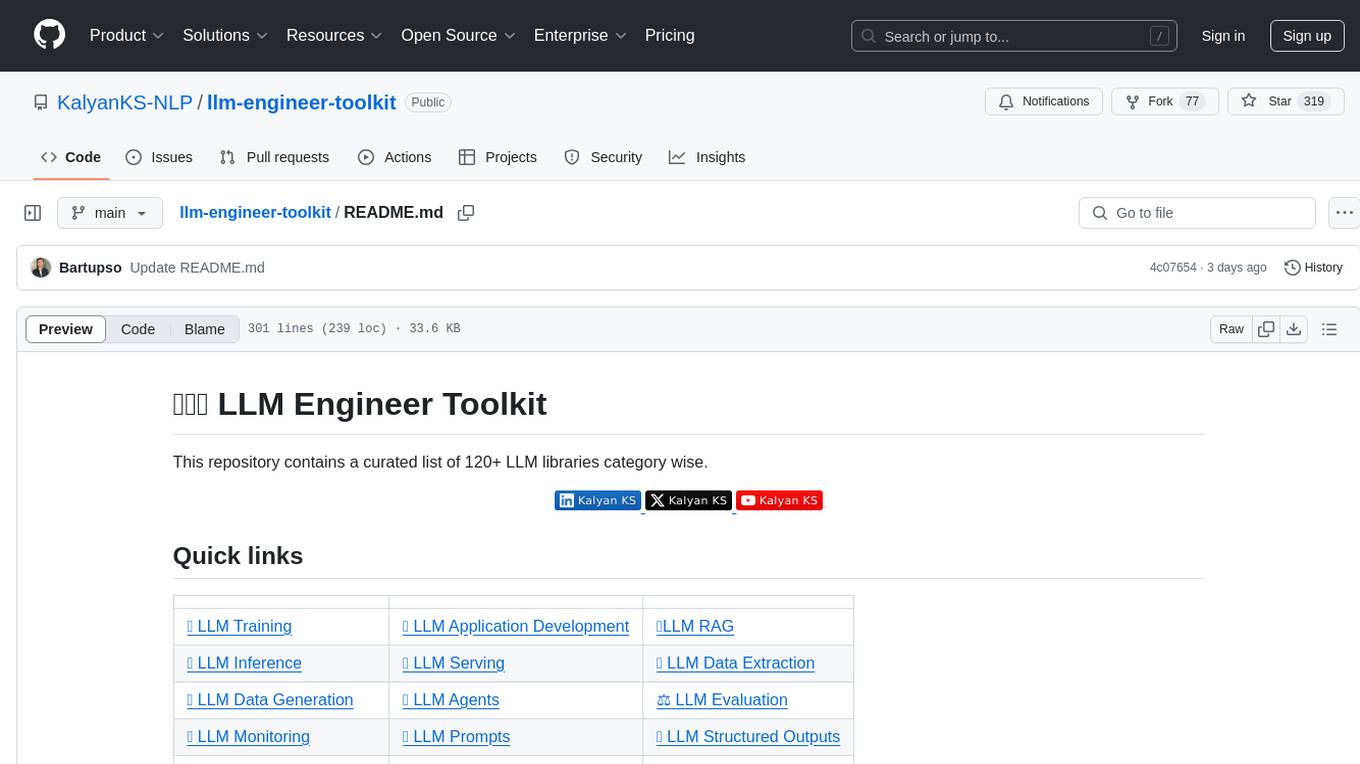
llm-engineer-toolkit
The LLM Engineer Toolkit is a curated repository containing over 120 LLM libraries categorized for various tasks such as training, application development, inference, serving, data extraction, data generation, agents, evaluation, monitoring, prompts, structured outputs, safety, security, embedding models, and other miscellaneous tools. It includes libraries for fine-tuning LLMs, building applications powered by LLMs, serving LLM models, extracting data, generating synthetic data, creating AI agents, evaluating LLM applications, monitoring LLM performance, optimizing prompts, handling structured outputs, ensuring safety and security, embedding models, and more. The toolkit covers a wide range of tools and frameworks to streamline the development, deployment, and optimization of large language models.
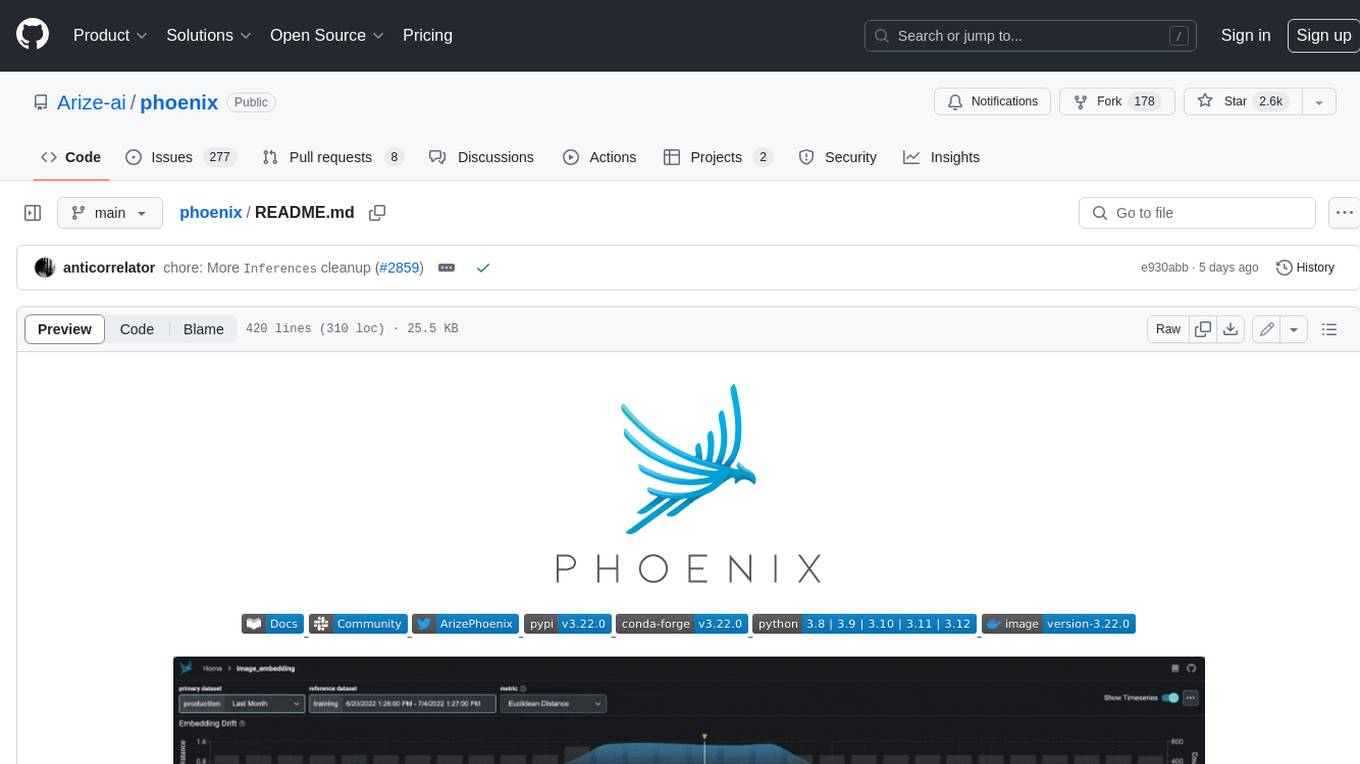
phoenix
Phoenix is a tool that provides MLOps and LLMOps insights at lightning speed with zero-config observability. It offers a notebook-first experience for monitoring models and LLM Applications by providing LLM Traces, LLM Evals, Embedding Analysis, RAG Analysis, and Structured Data Analysis. Users can trace through the execution of LLM Applications, evaluate generative models, explore embedding point-clouds, visualize generative application's search and retrieval process, and statistically analyze structured data. Phoenix is designed to help users troubleshoot problems related to retrieval, tool execution, relevance, toxicity, drift, and performance degradation.
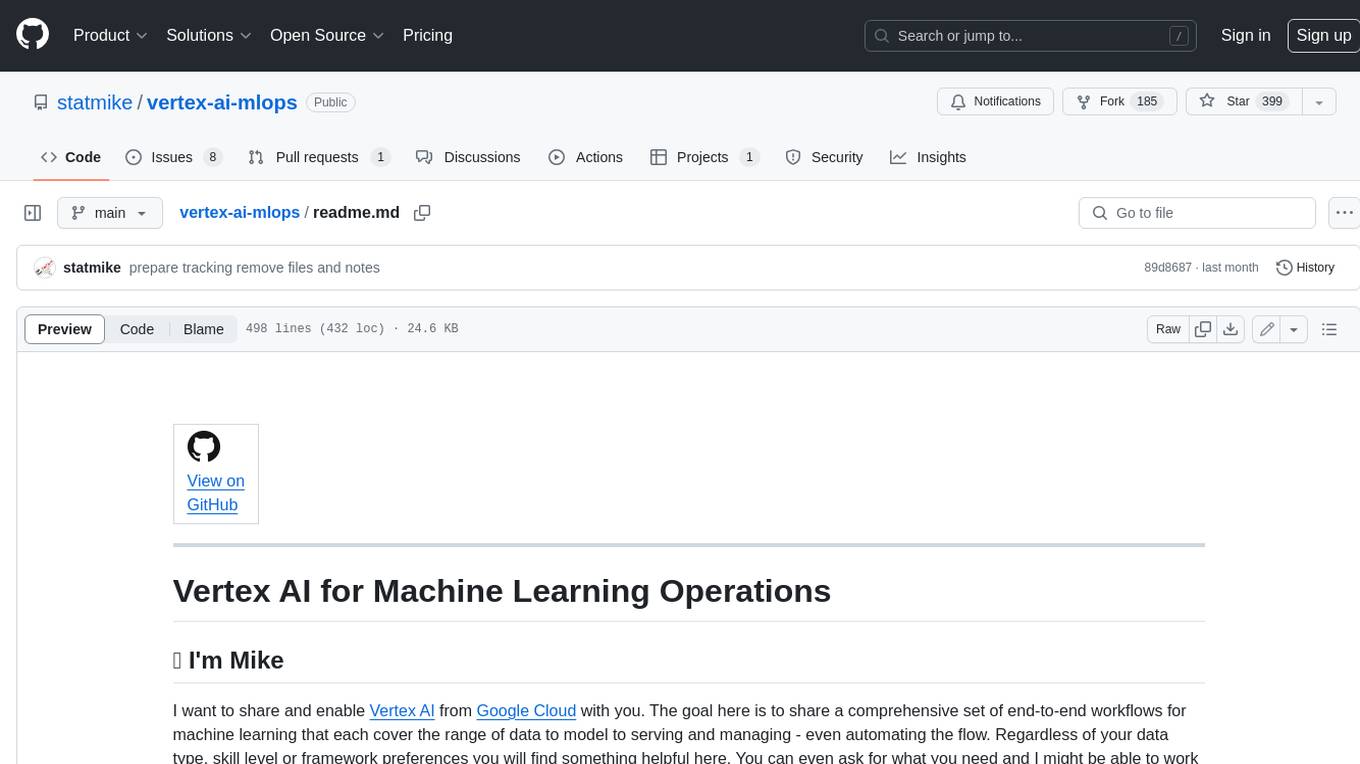
vertex-ai-mlops
Vertex AI is a platform for end-to-end model development. It consist of core components that make the processes of MLOps possible for design patterns of all types.
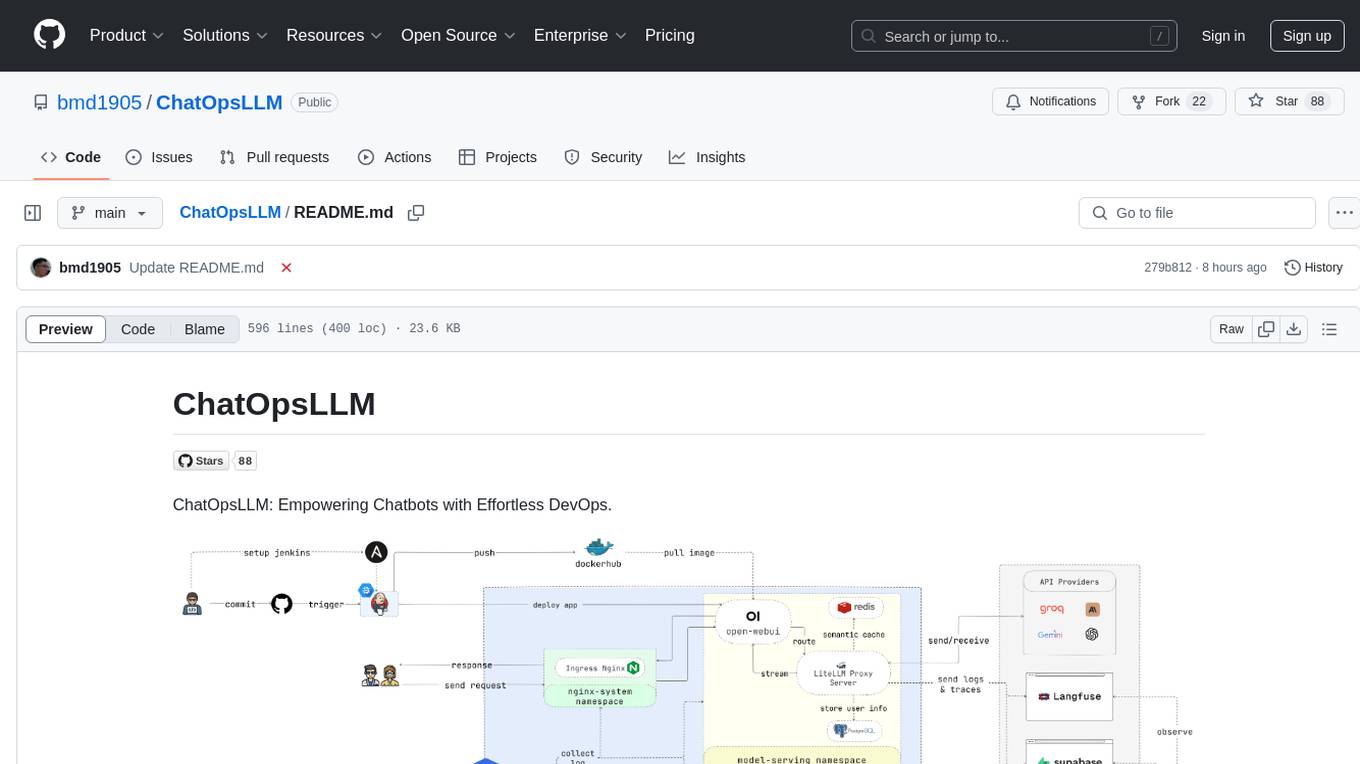
ChatOpsLLM
ChatOpsLLM is a project designed to empower chatbots with effortless DevOps capabilities. It provides an intuitive interface and streamlined workflows for managing and scaling language models. The project incorporates robust MLOps practices, including CI/CD pipelines with Jenkins and Ansible, monitoring with Prometheus and Grafana, and centralized logging with the ELK stack. Developers can find detailed documentation and instructions on the project's website.
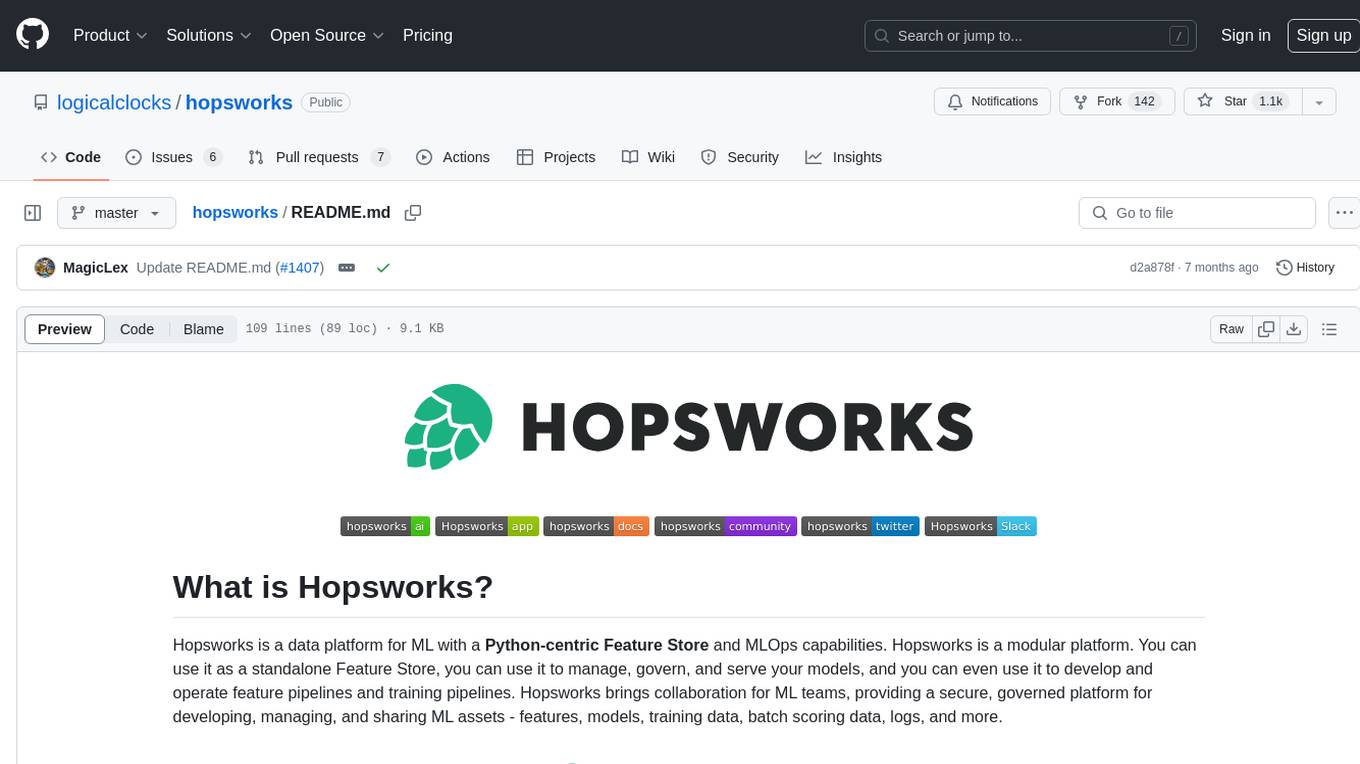
hopsworks
Hopsworks is a data platform for ML with a Python-centric Feature Store and MLOps capabilities. It provides collaboration for ML teams, offering a secure, governed platform for developing, managing, and sharing ML assets. Hopsworks supports project-based multi-tenancy, team collaboration, development tools for Data Science, and is available on any platform including managed cloud services and on-premise installations. The platform enables end-to-end responsibility from raw data to managed features and models, supports versioning, lineage, and provenance, and facilitates the complete MLOps life cycle.
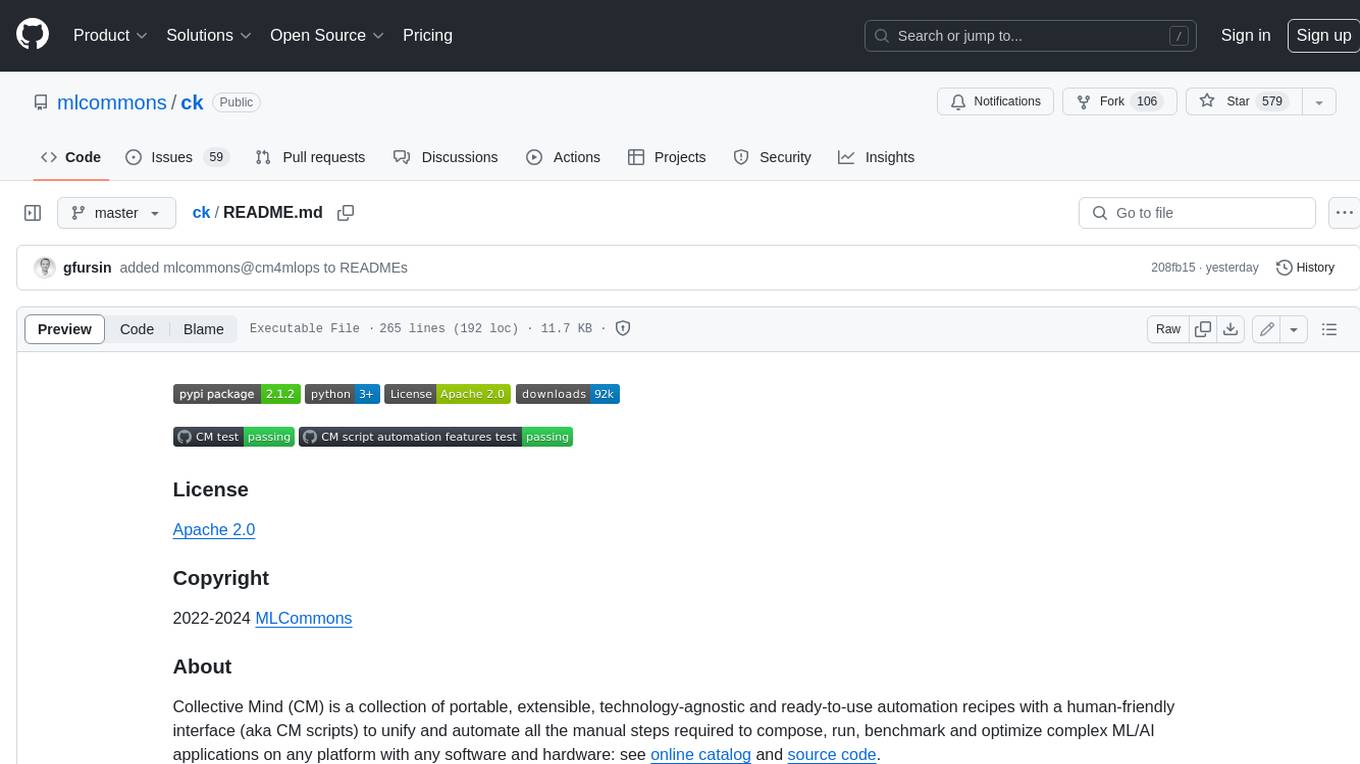
ck
Collective Mind (CM) is a collection of portable, extensible, technology-agnostic and ready-to-use automation recipes with a human-friendly interface (aka CM scripts) to unify and automate all the manual steps required to compose, run, benchmark and optimize complex ML/AI applications on any platform with any software and hardware: see online catalog and source code. CM scripts require Python 3.7+ with minimal dependencies and are continuously extended by the community and MLCommons members to run natively on Ubuntu, MacOS, Windows, RHEL, Debian, Amazon Linux and any other operating system, in a cloud or inside automatically generated containers while keeping backward compatibility - please don't hesitate to report encountered issues here and contact us via public Discord Server to help this collaborative engineering effort! CM scripts were originally developed based on the following requirements from the MLCommons members to help them automatically compose and optimize complex MLPerf benchmarks, applications and systems across diverse and continuously changing models, data sets, software and hardware from Nvidia, Intel, AMD, Google, Qualcomm, Amazon and other vendors: * must work out of the box with the default options and without the need to edit some paths, environment variables and configuration files; * must be non-intrusive, easy to debug and must reuse existing user scripts and automation tools (such as cmake, make, ML workflows, python poetry and containers) rather than substituting them; * must have a very simple and human-friendly command line with a Python API and minimal dependencies; * must require minimal or zero learning curve by using plain Python, native scripts, environment variables and simple JSON/YAML descriptions instead of inventing new workflow languages; * must have the same interface to run all automations natively, in a cloud or inside containers. CM scripts were successfully validated by MLCommons to modularize MLPerf inference benchmarks and help the community automate more than 95% of all performance and power submissions in the v3.1 round across more than 120 system configurations (models, frameworks, hardware) while reducing development and maintenance costs.
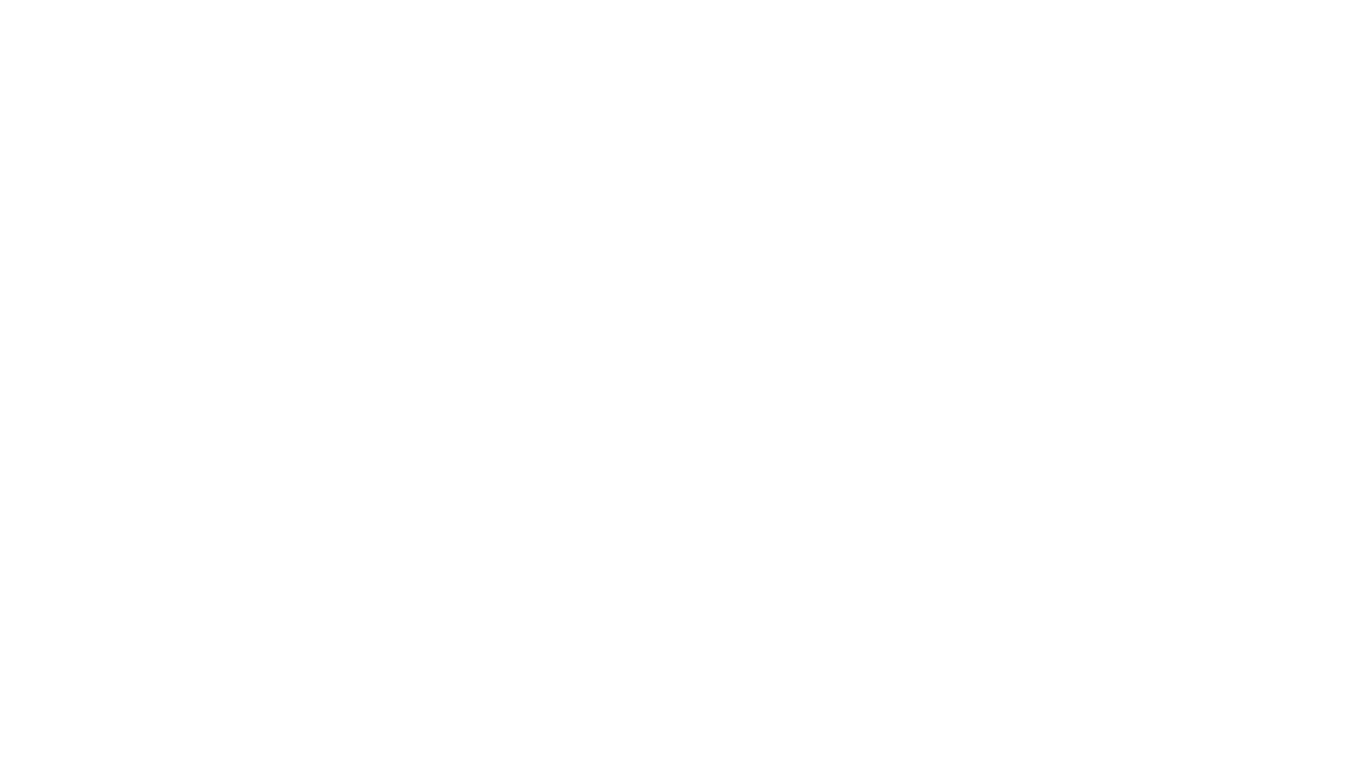
second-brain-ai-assistant-course
This open-source course teaches how to build an advanced RAG and LLM system using LLMOps and ML systems best practices. It helps you create an AI assistant that leverages your personal knowledge base to answer questions, summarize documents, and provide insights. The course covers topics such as LLM system architecture, pipeline orchestration, large-scale web crawling, model fine-tuning, and advanced RAG features. It is suitable for ML/AI engineers and data/software engineers & data scientists looking to level up to production AI systems. The course is free, with minimal costs for tools like OpenAI's API and Hugging Face's Dedicated Endpoints. Participants will build two separate Python applications for offline ML pipelines and online inference pipeline.