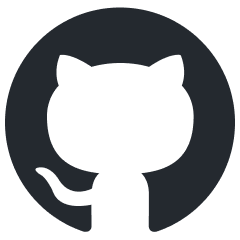
llm-python
Large Language Models (LLMs) tutorials & sample scripts, ft. langchain, openai, llamaindex, gpt, chromadb & pinecone
Stars: 673
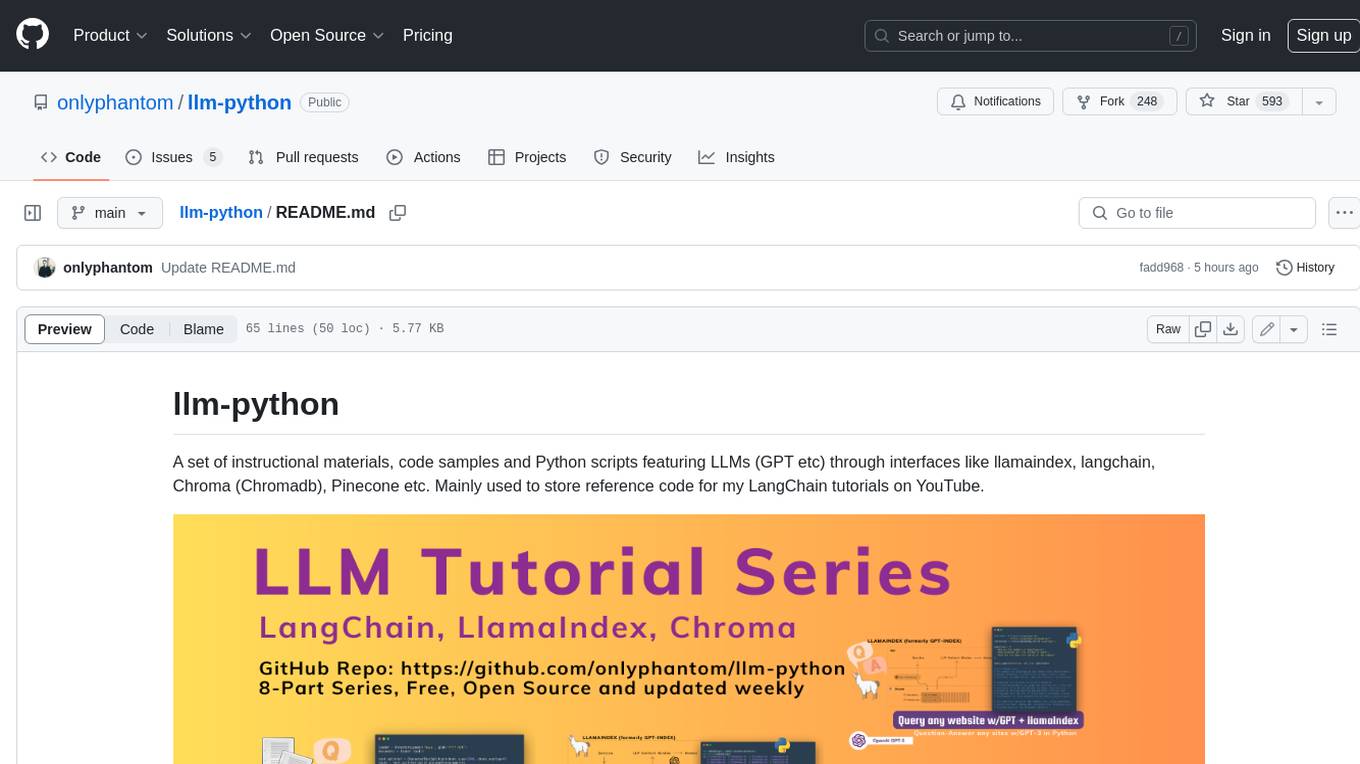
A set of instructional materials, code samples and Python scripts featuring LLMs (GPT etc) through interfaces like llamaindex, langchain, Chroma (Chromadb), Pinecone etc. Mainly used to store reference code for my LangChain tutorials on YouTube.
README:
A set of instructional materials, code samples and Python scripts featuring LLMs (GPT etc) through interfaces like llamaindex, langchain, Chroma (Chromadb), Pinecone etc. Mainly used to store reference code for my LangChain tutorials on YouTube.
Learn LangChain from my YouTube channel (~8 hours of LLM hands-on building tutorials); Each lesson is accompanied by the corresponding code in this repo and is designed to be self-contained -- while still focused on some key concepts in LLM (large language model) development and tooling.
Feel free to pick and choose your starting point based on your learning goals:
Part | LLM Tutorial | Link | Video Duration |
---|---|---|---|
1 | OpenAI tutorial and video walkthrough | Tutorial Video | 26:56 |
2 | LangChain + OpenAI tutorial: Building a Q&A system w/ own text data | Tutorial Video | 20:00 |
3 | LangChain + OpenAI to chat w/ (query) own Database / CSV | Tutorial Video | 19:30 |
4 | LangChain + HuggingFace's Inference API (no OpenAI credits required!) | Tutorial Video | 24:36 |
5 | Understanding Embeddings in LLMs | Tutorial Video | 29:22 |
6 | Query any website with LLamaIndex + GPT3 (ft. Chromadb, Trafilatura) | Tutorial Video | 11:11 |
7 | Locally-hosted, offline LLM w/LlamaIndex + OPT (open source, instruction-tuning LLM) | Tutorial Video | 32:27 |
8 | Building an AI Language Tutor: Pinecone + LlamaIndex + GPT-3 + BeautifulSoup | Tutorial Video | 51:08 |
9 | Building a queryable journal 💬 w/ OpenAI, markdown & LlamaIndex 🦙 | Tutorial Video | 40:29 |
10 | Making a Sci-Fi game w/ Cohere LLM + Stability.ai: Generative AI tutorial | Tutorial Video | 1:02:20 |
11 | GPT builds entire party invitation app from prompt (ft. SMOL Developer) | Tutorial Video | 41:33 |
12 | A language for LLM prompt design: Guidance | Tutorial Video | 43:15 |
13 | You should use LangChain's Caching! | Tutorial Video | 25:37 |
14 | Build Chat AI apps with Steamlit + LangChain | Tutorial Video | 32:11 |
The full lesson playlist can be found here.
- Clone this repo
- Install requirements:
pip install -r requirements.txt
- Some sample data are provided to you in the
news
foldeer, but you can use your own data by replacing the content (or adding to it) with your own text files. - Create a
.env
file which contains your OpenAI API key. You can get one from here.HUGGINGFACEHUB_API_TOKEN
andPINECONE_API_KEY
are optional, but they are used in some of the lessons.-
Lesson 10 uses Cohere and Stability AI, both of which offers a free tier (no credit card required). You can add the respective keys as
COHERE_API_KEY
andSTABILITY_API_KEY
in the.env
file.
-
Lesson 10 uses Cohere and Stability AI, both of which offers a free tier (no credit card required). You can add the respective keys as
The .env
file should look like this:
OPENAI_API_KEY=your_api_key_here
# optionals (not required for most of the series)
HUGGINGFACEHUB_API_TOKEN=your_api_token_here
PINECONE_API_KEY=your_api_key_here
HuggingFace and Pinecone are optional but is recommended if you want to use the Inference API and explore those models outside of the OpenAI ecosystem. This is demonstrated in Part 3 of the tutorial series.
5. Run the examples in any order you want. For example, python 6_team.py
will run the website Q&A example, which uses GPT-3 to answer questions about a company and the team of people working at Supertype.ai. Watch the corresponding video to follow along each of the examples.
💡 Thanks to the work of @VanillaMacchiato, this project is updated as of 2023-06-30 to use the latest version of LlamaIndex (0.6.31) and LangChain (0.0.209). Installing the dependencies should be as simple as
pip install -r requirements.txt
. If you encounter any issues, please let me know.
If you're watching the LLM video tutorials, they may have very minor differences (typically 1-2 lines of code that needs to be changed) from the code in this repo since these videos have been released with the respective versions at the time of recording (LlamaIndex 0.5.7 and LangChain 0.0.157). Please refer to the code in this repo for the latest version of the code.
I will try to keep this repo up to date with the latest version of the libraries, but if you encounter any issues, please: (1) raise a discussion through Issues or (2) volunteer a PR to update the code.
NOTE: triton
package is supported only for the x86_64 architecture. If you have problems with installing it, see the triton compatibility guide. Specifically, errors like ERROR: Could not find a version that satisfies the requirement triton (from versions: none) ERROR: No matching distribution found for triton
.
uname -p
should give you the processor's name.
I run a mentorship program under Supertype Fellowship. The program is self-paced and free, with a community of other learners and practitioners around the world (English-speaking). You can optionally book a 1-on-1 session with my team of mentors to help you through video tutoring and code reviews.
MIT © Supertype 2024
For Tasks:
Click tags to check more tools for each tasksFor Jobs:
Alternative AI tools for llm-python
Similar Open Source Tools
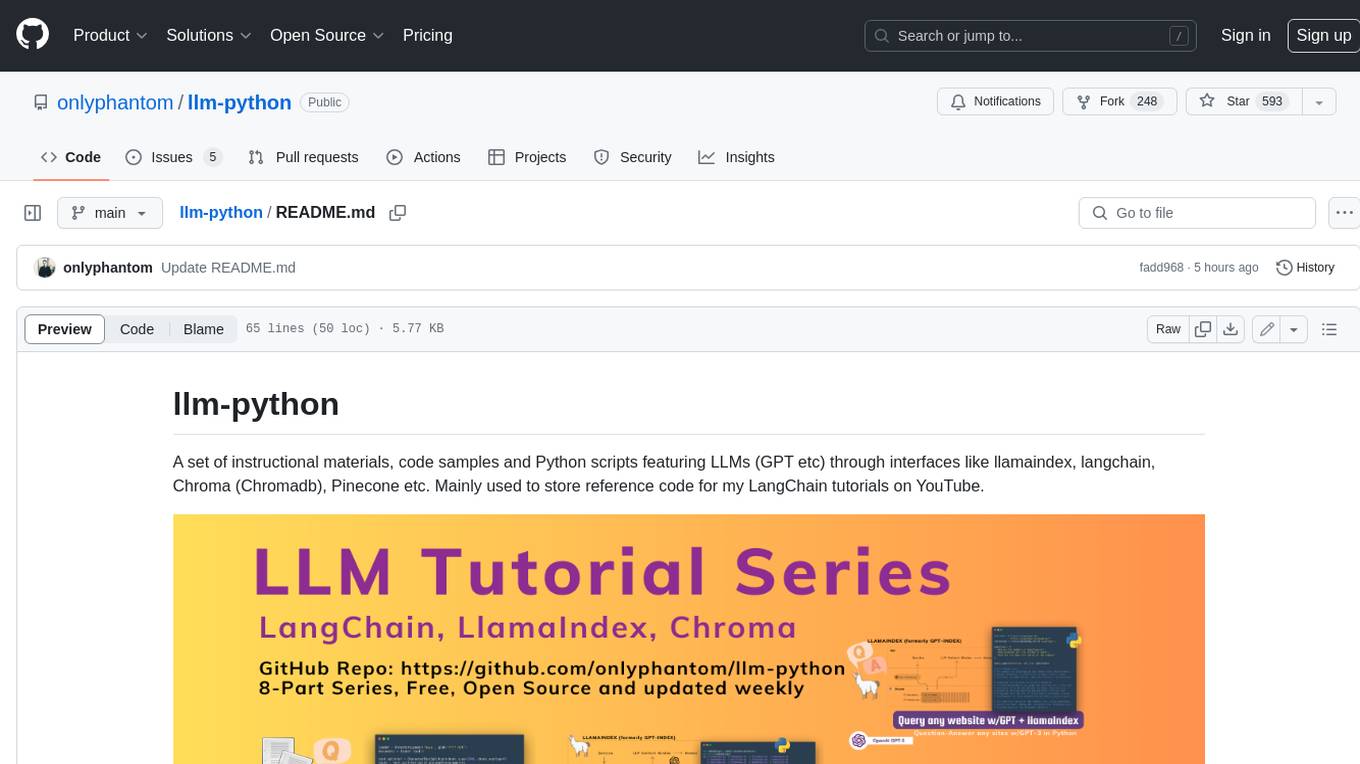
llm-python
A set of instructional materials, code samples and Python scripts featuring LLMs (GPT etc) through interfaces like llamaindex, langchain, Chroma (Chromadb), Pinecone etc. Mainly used to store reference code for my LangChain tutorials on YouTube.
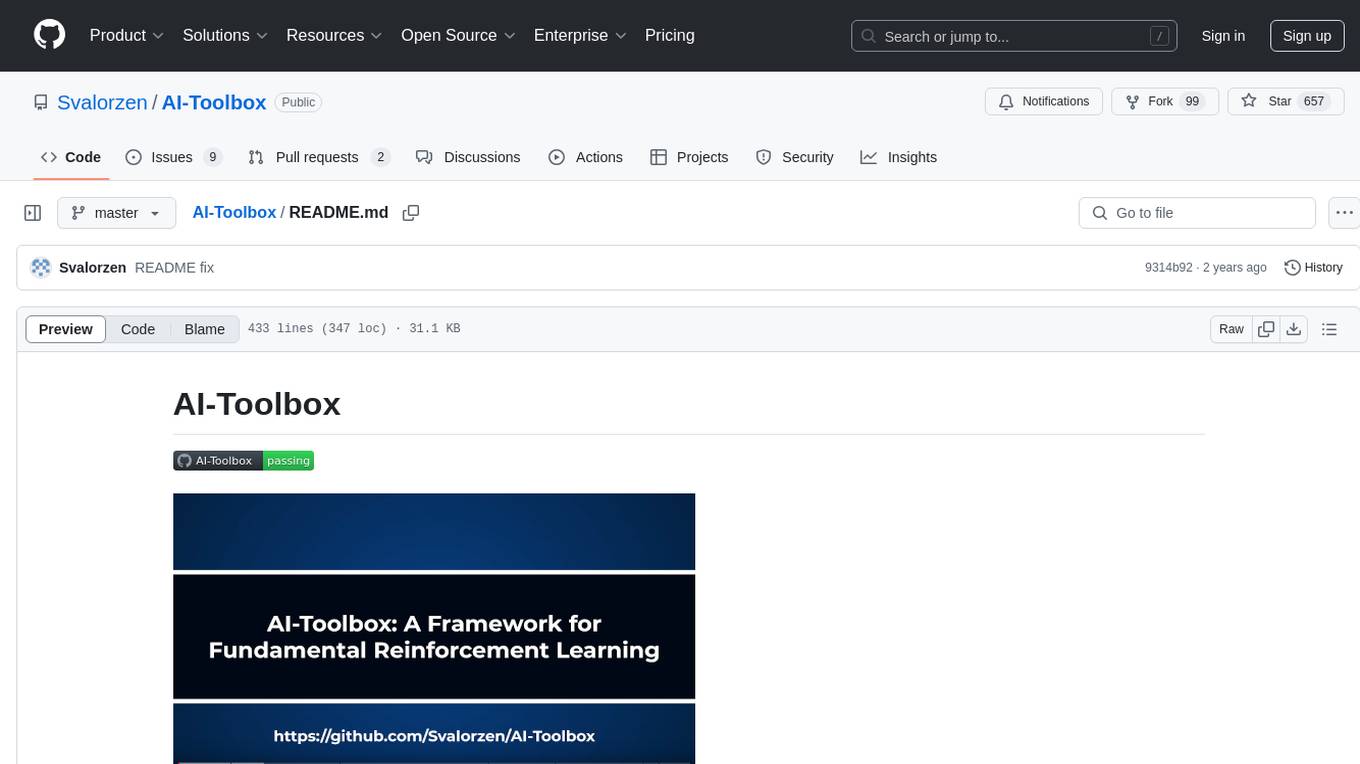
AI-Toolbox
AI-Toolbox is a C++ library aimed at representing and solving common AI problems, with a focus on MDPs, POMDPs, and related algorithms. It provides an easy-to-use interface that is extensible to many problems while maintaining readable code. The toolbox includes tutorials for beginners in reinforcement learning and offers Python bindings for seamless integration. It features utilities for combinatorics, polytopes, linear programming, sampling, distributions, statistics, belief updating, data structures, logging, seeding, and more. Additionally, it supports bandit/normal games, single agent MDP/stochastic games, single agent POMDP, and factored/joint multi-agent scenarios.
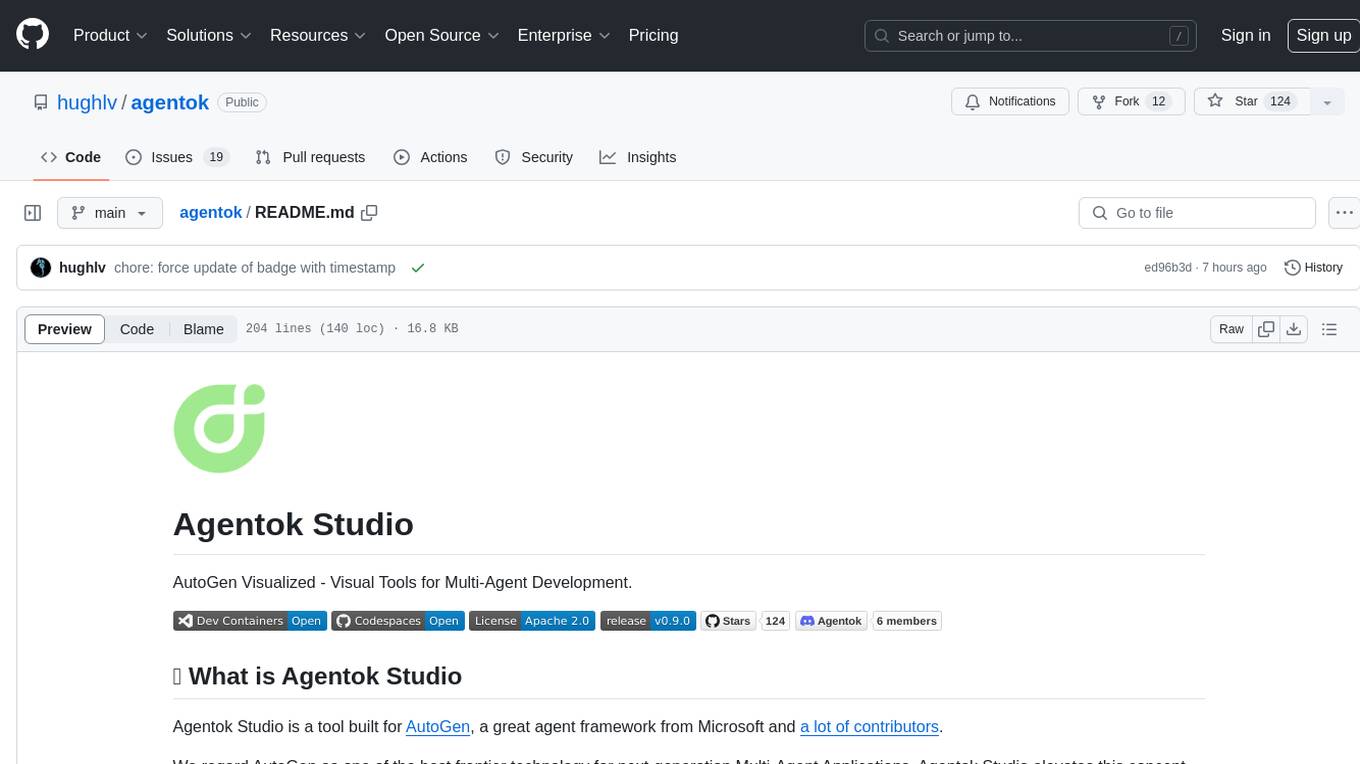
flowgen
FlowGen is a tool built for AutoGen, a great agent framework from Microsoft and a lot of contributors. It provides intuitive visual tools that streamline the construction and oversight of complex agent-based workflows, simplifying the process for creators and developers. Users can create Autoflows, chat with agents, and share flow templates. The tool is fully dockerized and supports deployment on Railway.app. Contributions to the project are welcome, and the platform uses semantic-release for versioning and releases.
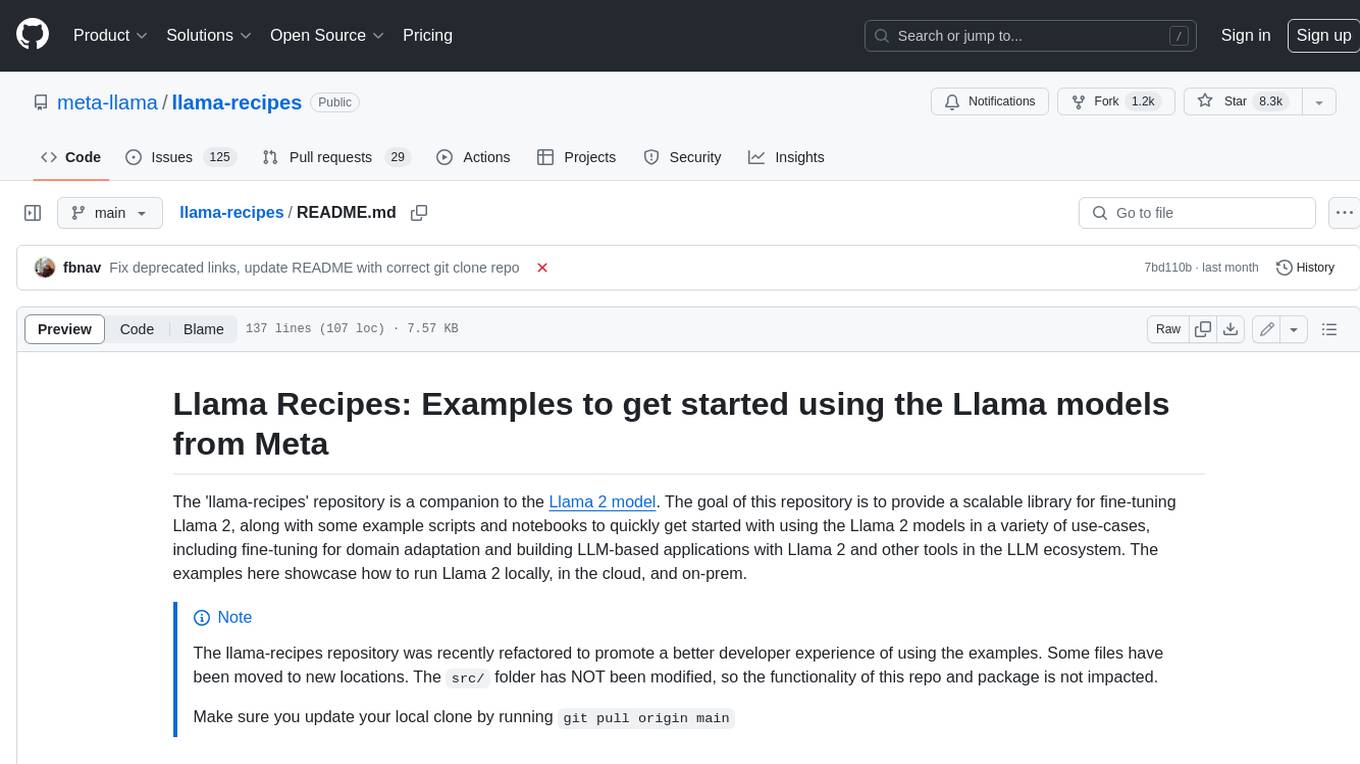
llama-recipes
The llama-recipes repository provides a scalable library for fine-tuning Llama 2, along with example scripts and notebooks to quickly get started with using the Llama 2 models in a variety of use-cases, including fine-tuning for domain adaptation and building LLM-based applications with Llama 2 and other tools in the LLM ecosystem. The examples here showcase how to run Llama 2 locally, in the cloud, and on-prem.
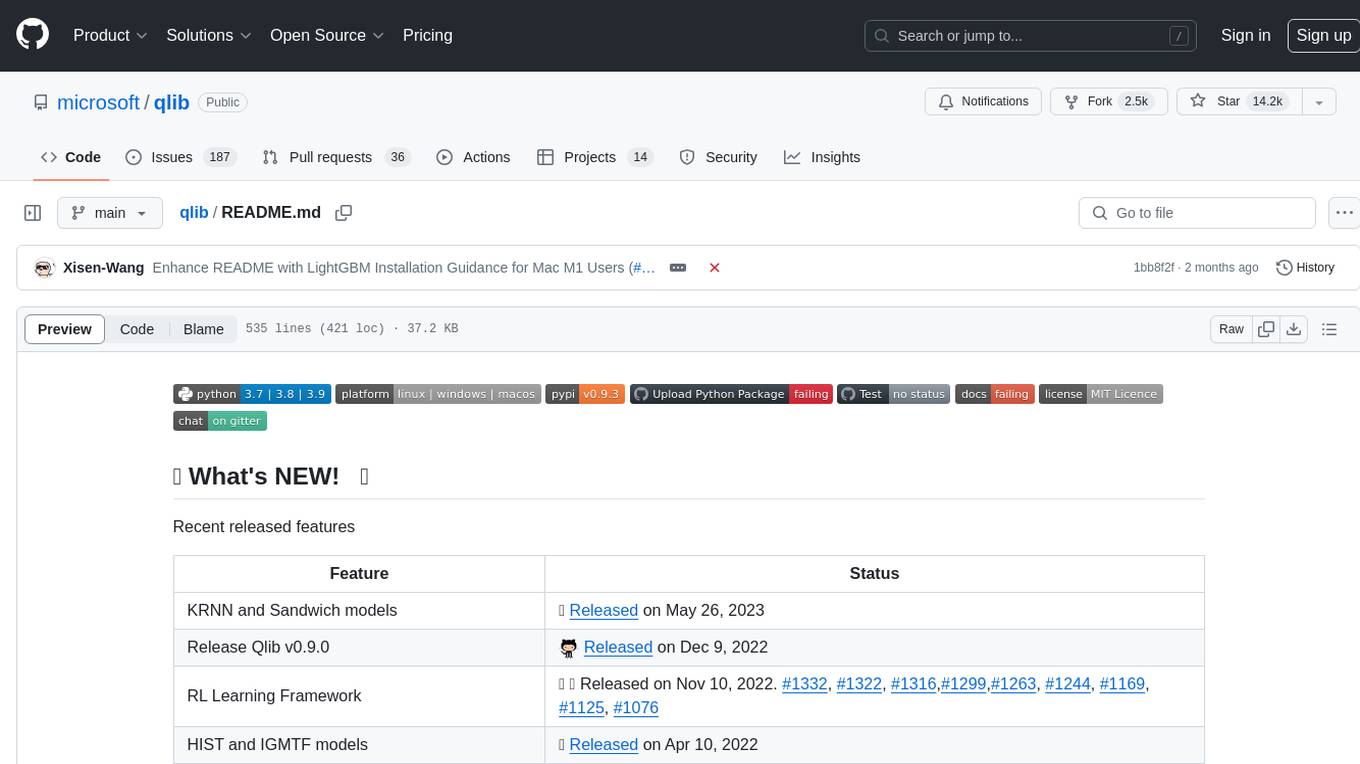
qlib
Qlib is an open-source, AI-oriented quantitative investment platform that supports diverse machine learning modeling paradigms, including supervised learning, market dynamics modeling, and reinforcement learning. It covers the entire chain of quantitative investment, from alpha seeking to order execution. The platform empowers researchers to explore ideas and implement productions using AI technologies in quantitative investment. Qlib collaboratively solves key challenges in quantitative investment by releasing state-of-the-art research works in various paradigms. It provides a full ML pipeline for data processing, model training, and back-testing, enabling users to perform tasks such as forecasting market patterns, adapting to market dynamics, and modeling continuous investment decisions.
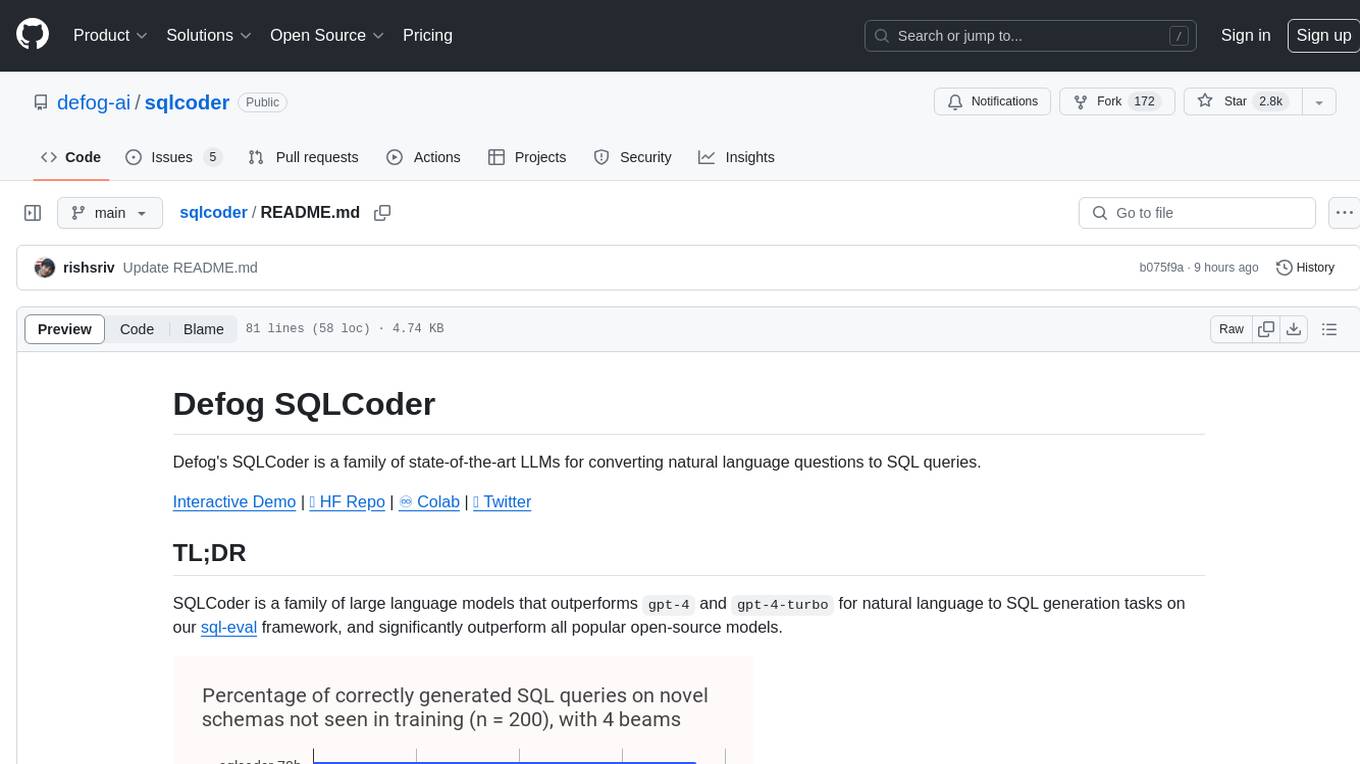
sqlcoder
Defog's SQLCoder is a family of state-of-the-art large language models (LLMs) designed for converting natural language questions into SQL queries. It outperforms popular open-source models like gpt-4 and gpt-4-turbo on SQL generation tasks. SQLCoder has been trained on more than 20,000 human-curated questions based on 10 different schemas, and the model weights are licensed under CC BY-SA 4.0. Users can interact with SQLCoder through the 'transformers' library and run queries using the 'sqlcoder launch' command in the terminal. The tool has been tested on NVIDIA GPUs with more than 16GB VRAM and Apple Silicon devices with some limitations. SQLCoder offers a demo on their website and supports quantized versions of the model for consumer GPUs with sufficient memory.
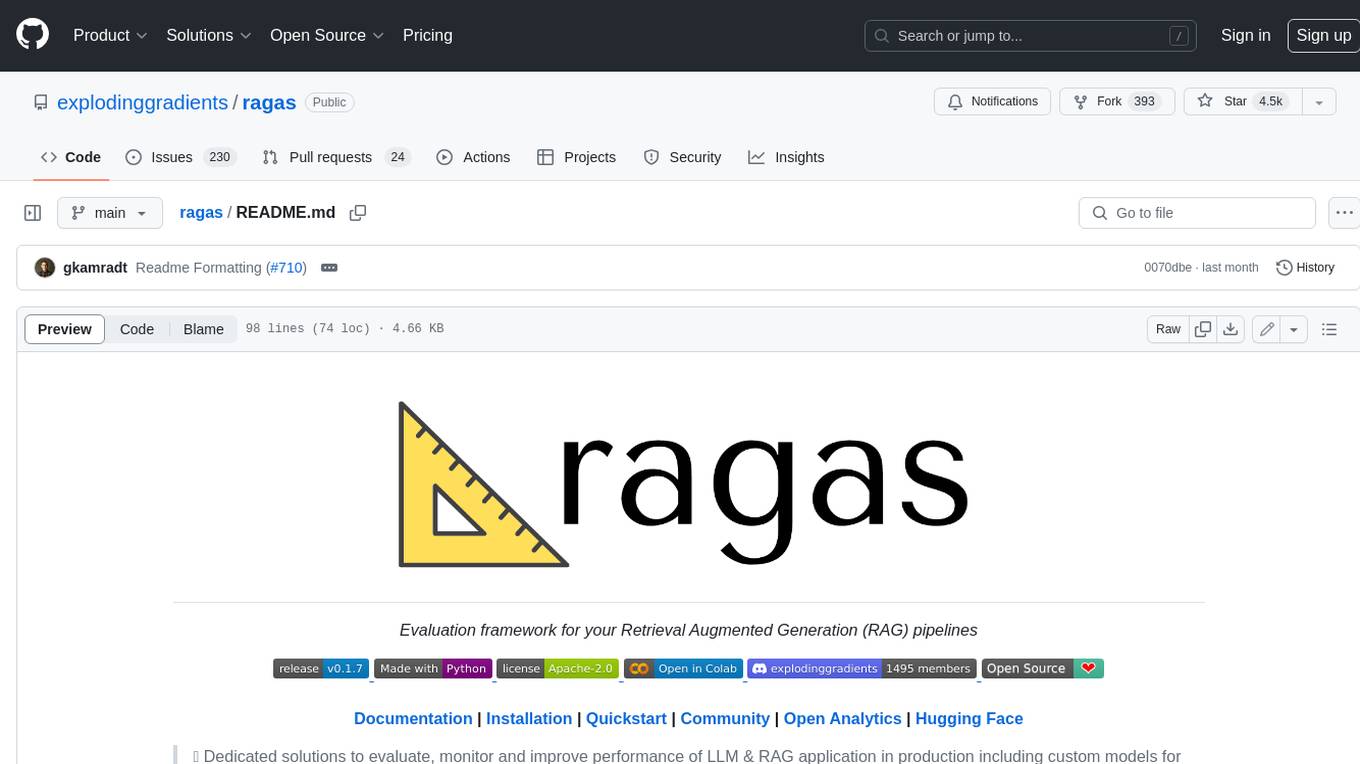
ragas
Ragas is a framework that helps you evaluate your Retrieval Augmented Generation (RAG) pipelines. RAG denotes a class of LLM applications that use external data to augment the LLM’s context. There are existing tools and frameworks that help you build these pipelines but evaluating it and quantifying your pipeline performance can be hard. This is where Ragas (RAG Assessment) comes in. Ragas provides you with the tools based on the latest research for evaluating LLM-generated text to give you insights about your RAG pipeline. Ragas can be integrated with your CI/CD to provide continuous checks to ensure performance.
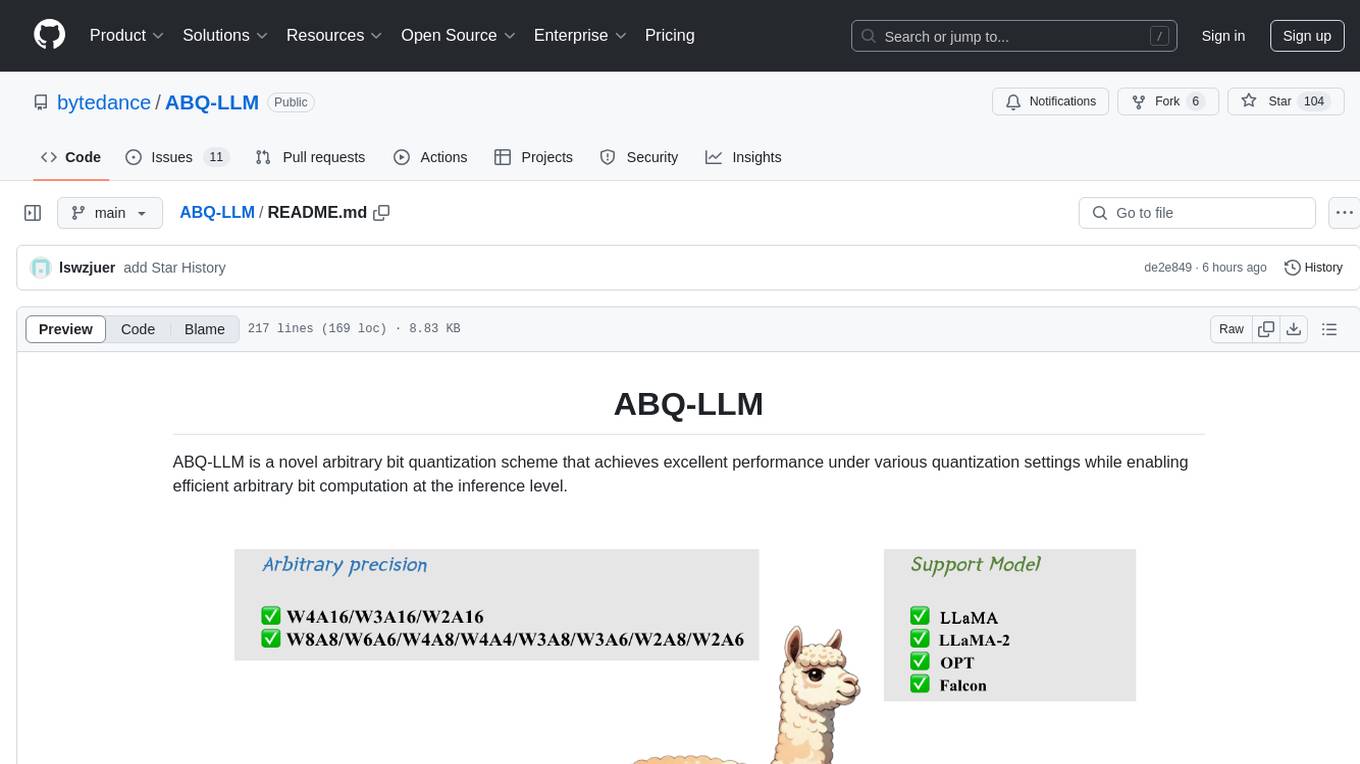
ABQ-LLM
ABQ-LLM is a novel arbitrary bit quantization scheme that achieves excellent performance under various quantization settings while enabling efficient arbitrary bit computation at the inference level. The algorithm supports precise weight-only quantization and weight-activation quantization. It provides pre-trained model weights and a set of out-of-the-box quantization operators for arbitrary bit model inference in modern architectures.
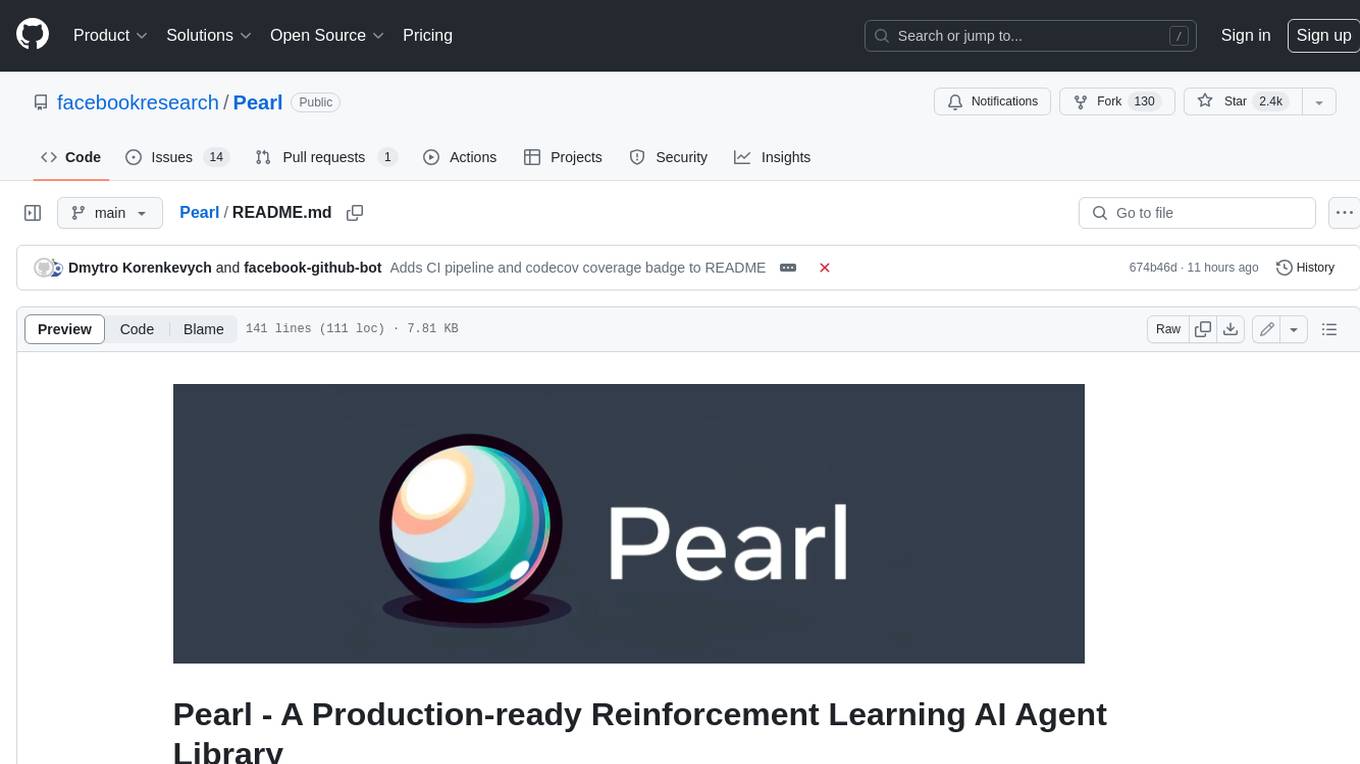
Pearl
Pearl is a production-ready Reinforcement Learning AI agent library open-sourced by the Applied Reinforcement Learning team at Meta. It enables researchers and practitioners to develop Reinforcement Learning AI agents that prioritize cumulative long-term feedback over immediate feedback and can adapt to environments with limited observability, sparse feedback, and high stochasticity. Pearl offers a diverse set of unique features for production environments, including dynamic action spaces, offline learning, intelligent neural exploration, safe decision making, history summarization, and data augmentation.
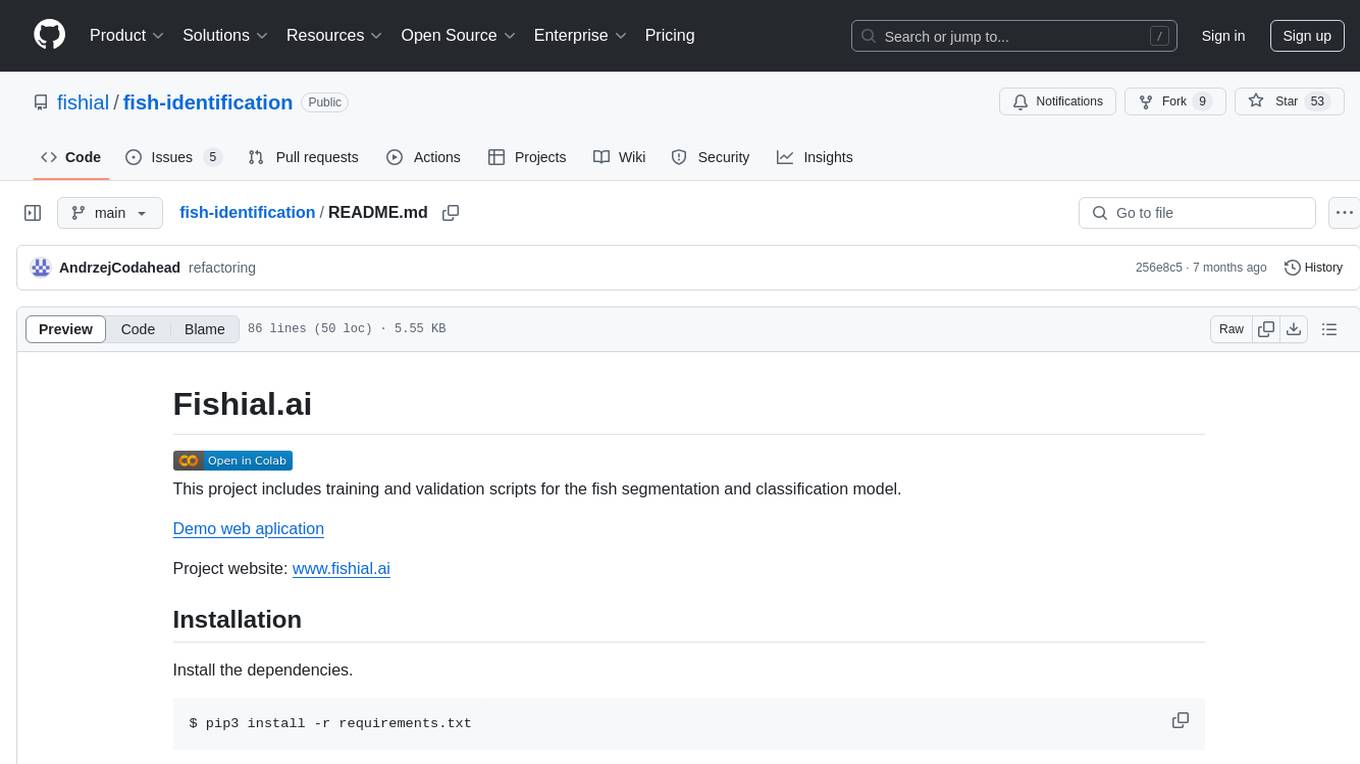
fish-identification
Fishial.ai is a project focused on training and validating scripts for fish segmentation and classification models. It includes various scripts for automatic training with different loss functions, dataset manipulation, and model setup using Detectron2 API. The project also provides tools for converting classification models to TorchScript format and creating training datasets. The models available include MaskRCNN for fish segmentation and various versions of ResNet18 for fish classification with different class counts and features. The project aims to facilitate fish identification and analysis through machine learning techniques.
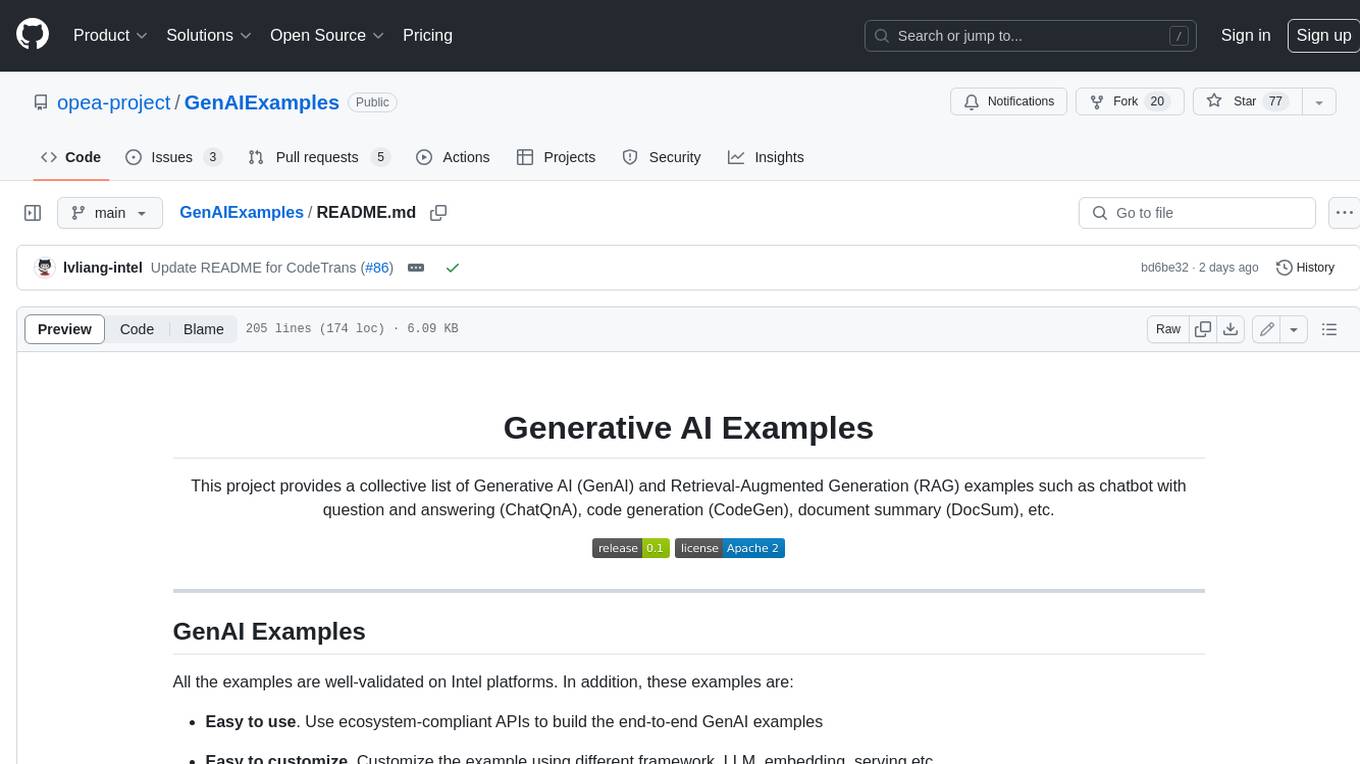
GenAIExamples
This project provides a collective list of Generative AI (GenAI) and Retrieval-Augmented Generation (RAG) examples such as chatbot with question and answering (ChatQnA), code generation (CodeGen), document summary (DocSum), etc.
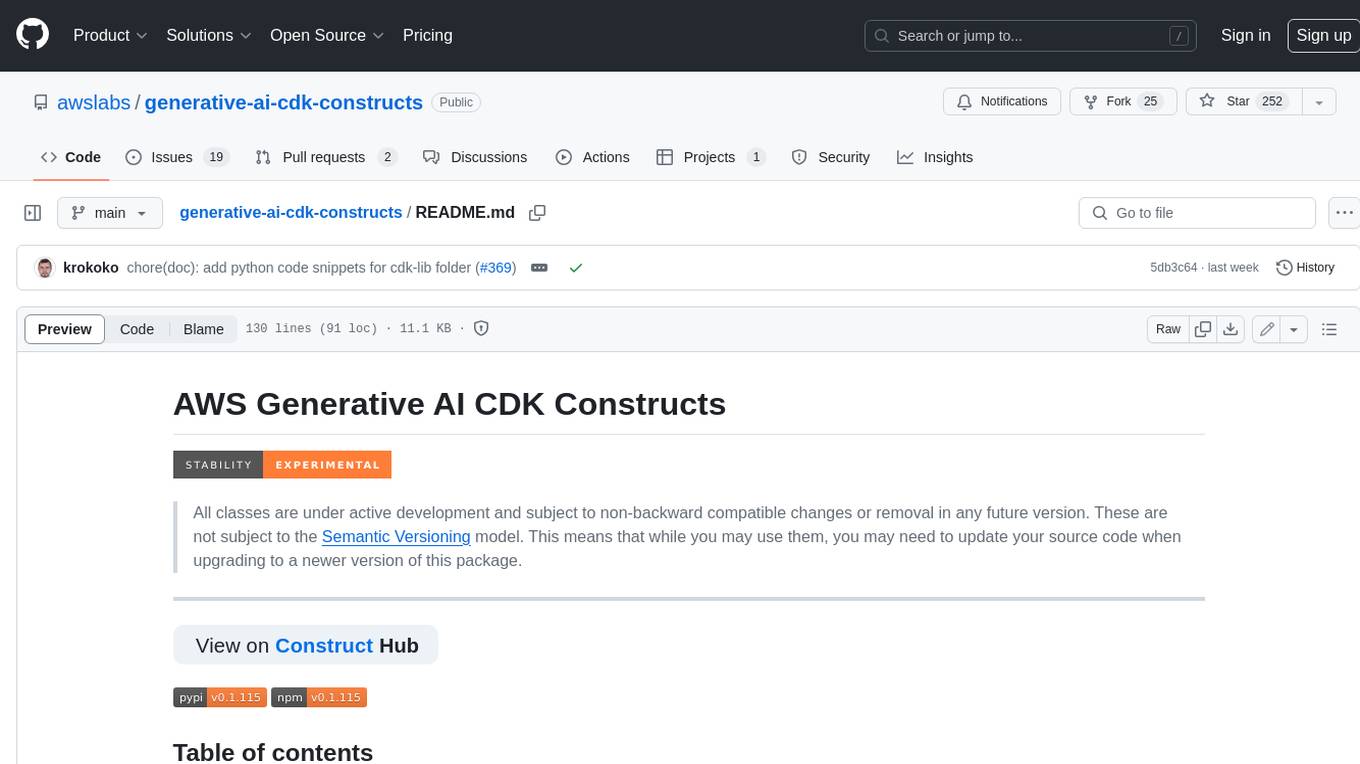
generative-ai-cdk-constructs
The AWS Generative AI Constructs Library is an open-source extension of the AWS Cloud Development Kit (AWS CDK) that provides multi-service, well-architected patterns for quickly defining solutions in code to create predictable and repeatable infrastructure, called constructs. The goal of AWS Generative AI CDK Constructs is to help developers build generative AI solutions using pattern-based definitions for their architecture. The patterns defined in AWS Generative AI CDK Constructs are high level, multi-service abstractions of AWS CDK constructs that have default configurations based on well-architected best practices. The library is organized into logical modules using object-oriented techniques to create each architectural pattern model.
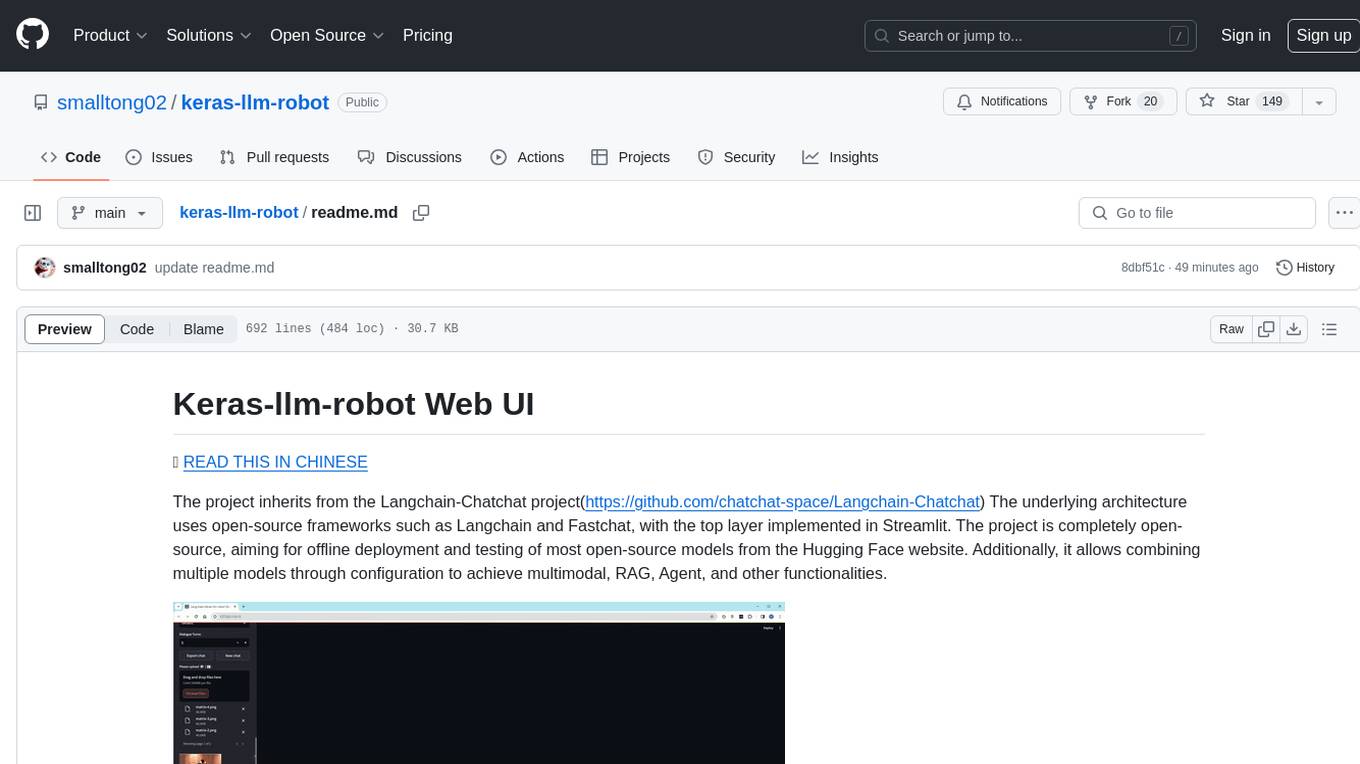
keras-llm-robot
The Keras-llm-robot Web UI project is an open-source tool designed for offline deployment and testing of various open-source models from the Hugging Face website. It allows users to combine multiple models through configuration to achieve functionalities like multimodal, RAG, Agent, and more. The project consists of three main interfaces: chat interface for language models, configuration interface for loading models, and tools & agent interface for auxiliary models. Users can interact with the language model through text, voice, and image inputs, and the tool supports features like model loading, quantization, fine-tuning, role-playing, code interpretation, speech recognition, image recognition, network search engine, and function calling.
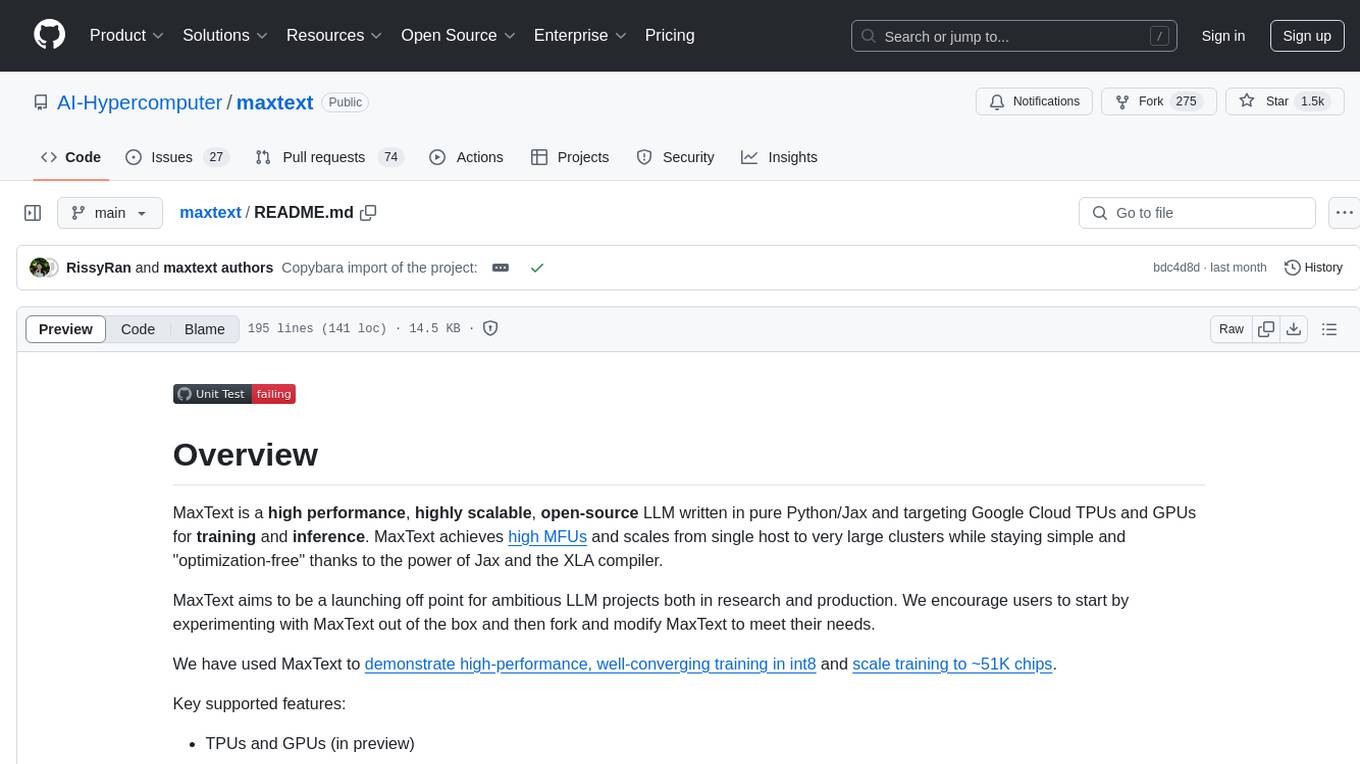
maxtext
MaxText is a high performance, highly scalable, open-source Large Language Model (LLM) written in pure Python/Jax targeting Google Cloud TPUs and GPUs for training and inference. It aims to be a launching off point for ambitious LLM projects in research and production, supporting TPUs and GPUs, models like Llama2, Mistral, and Gemma. MaxText provides specific instructions for getting started, runtime performance results, comparison to alternatives, and features like stack trace collection, ahead of time compilation for TPUs and GPUs, and automatic upload of logs to Vertex Tensorboard.
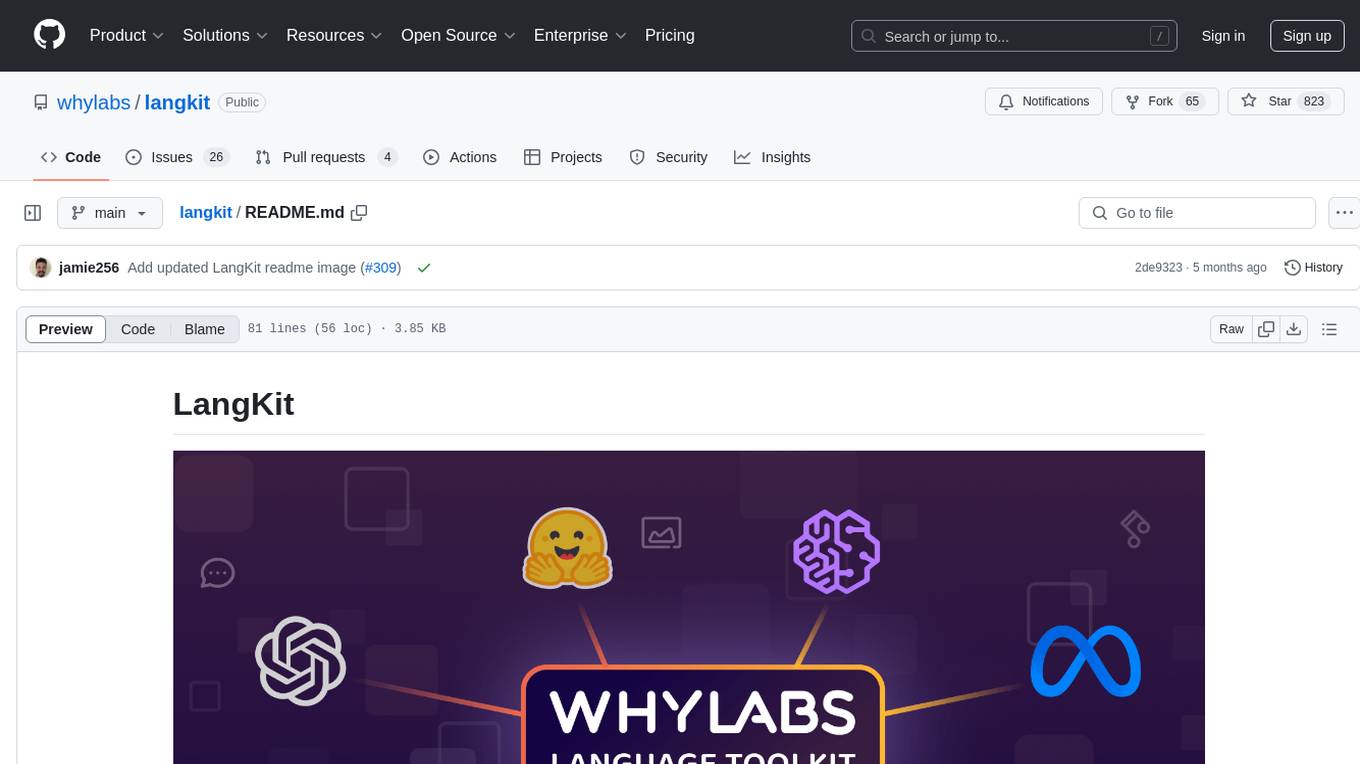
langkit
LangKit is an open-source text metrics toolkit for monitoring language models. It offers methods for extracting signals from input/output text, compatible with whylogs. Features include text quality, relevance, security, sentiment, toxicity analysis. Installation via PyPI. Modules contain UDFs for whylogs. Benchmarks show throughput on AWS instances. FAQs available.
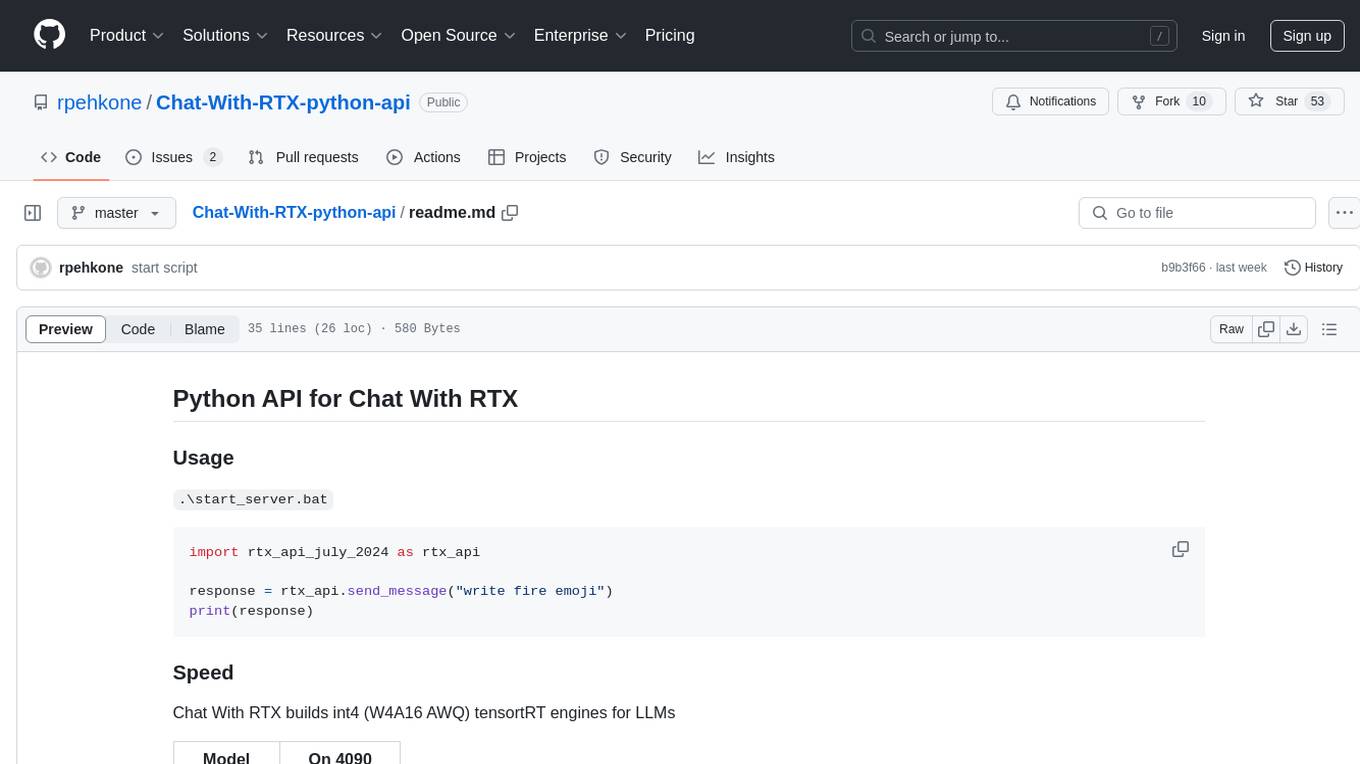
Chat-With-RTX-python-api
This repository contains a Python API for Chat With RTX, which allows users to interact with RTX models for natural language processing. The API provides functionality to send messages and receive responses from various LLM models. It also includes information on the speed of different models supported by Chat With RTX. The repository has a history of updates, including the removal of a feature and the addition of a new model for speech-to-text conversion. The repository is licensed under CC0.
For similar tasks
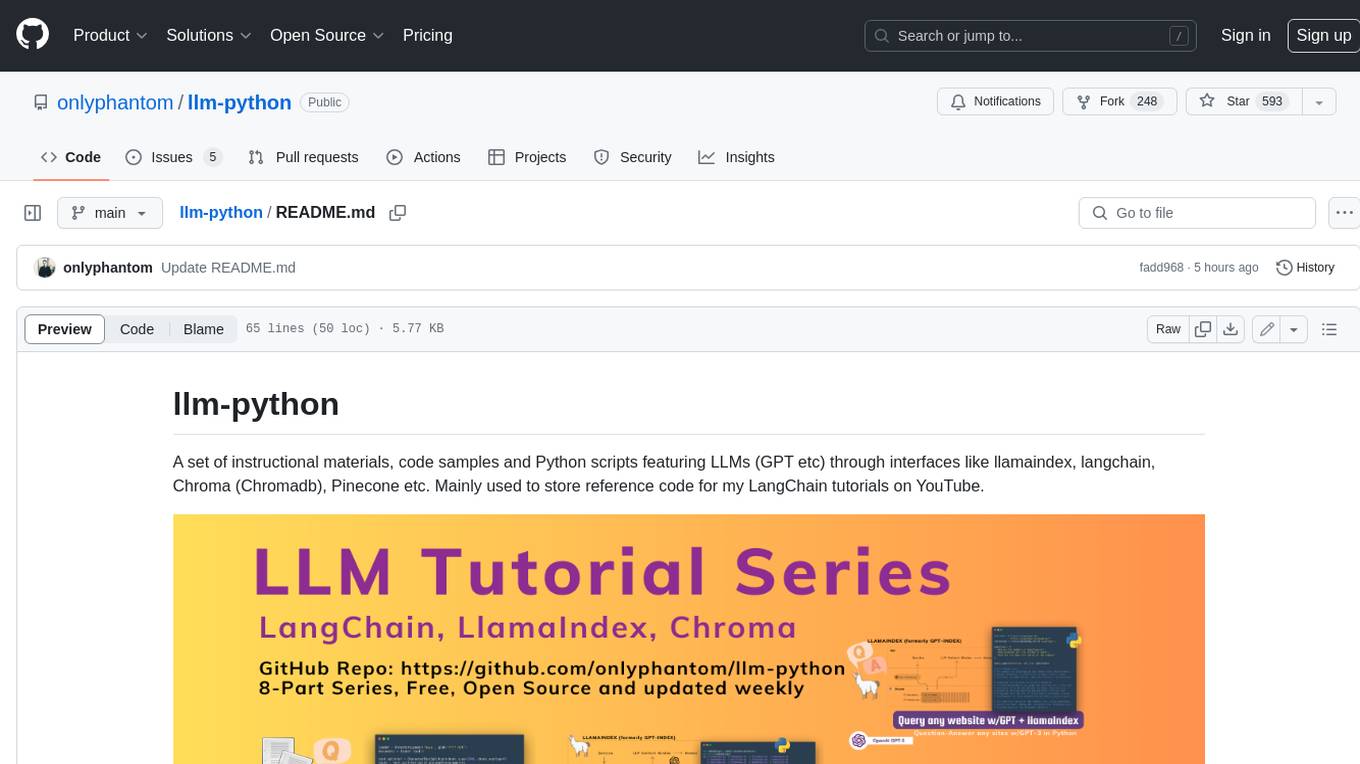
llm-python
A set of instructional materials, code samples and Python scripts featuring LLMs (GPT etc) through interfaces like llamaindex, langchain, Chroma (Chromadb), Pinecone etc. Mainly used to store reference code for my LangChain tutorials on YouTube.
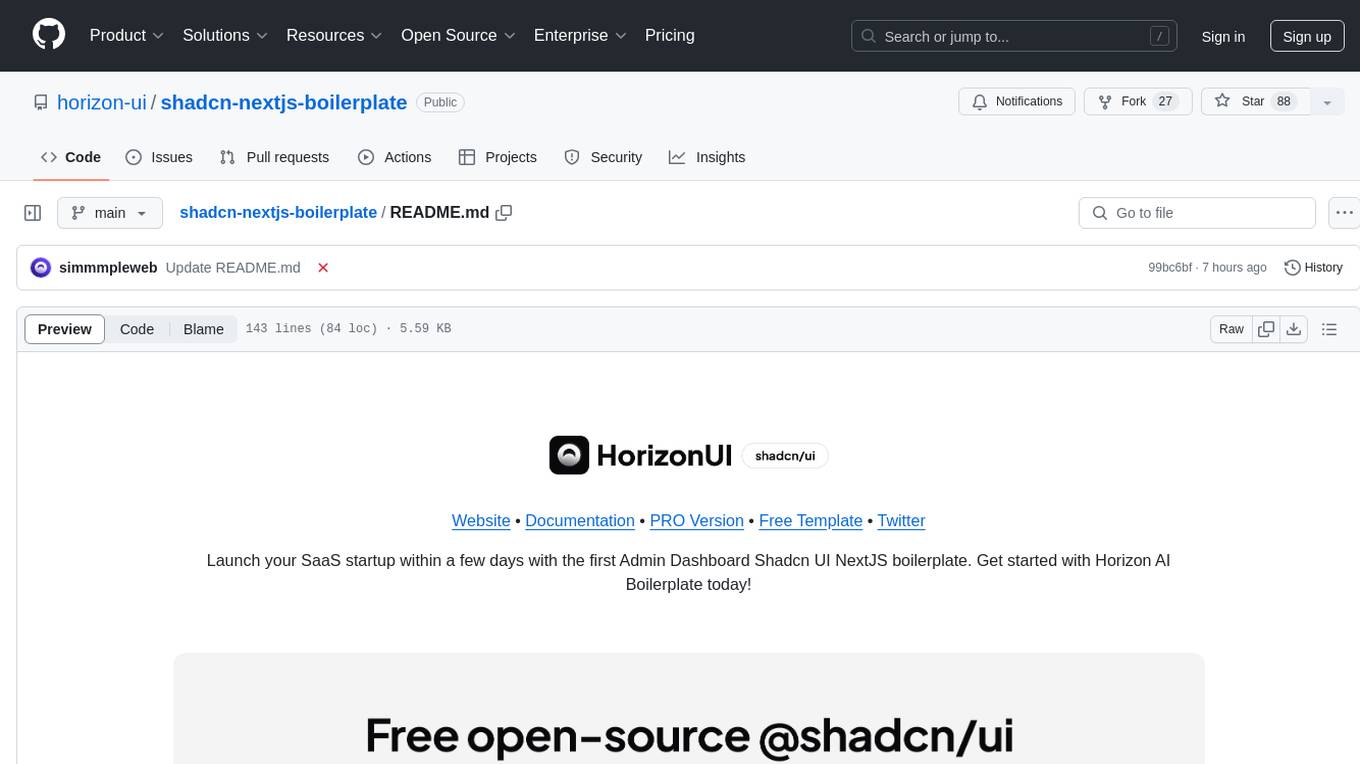
shadcn-nextjs-boilerplate
Horizon AI Boilerplate is an open-source Admin Dashboard template designed for Shadcn UI, NextJS, and Tailwind CSS. It provides over 30 dark/light frontend elements for creating Chat AI SaaS Apps quickly. The documentation is detailed and complex, guiding users through installation and usage. Users can start their local server with simple commands. The tool requires a valid OpenAI API key for ChatGPT functionality. Additionally, a Figma version is available for design purposes. The PRO version offers more components and pages. Users can report issues on GitHub and connect with the community via Discord. The tool credits open-source resources like Shadcn UI Library, NextJS Subscription Payments, and ChatBot UI by mckaywrigley.
For similar jobs
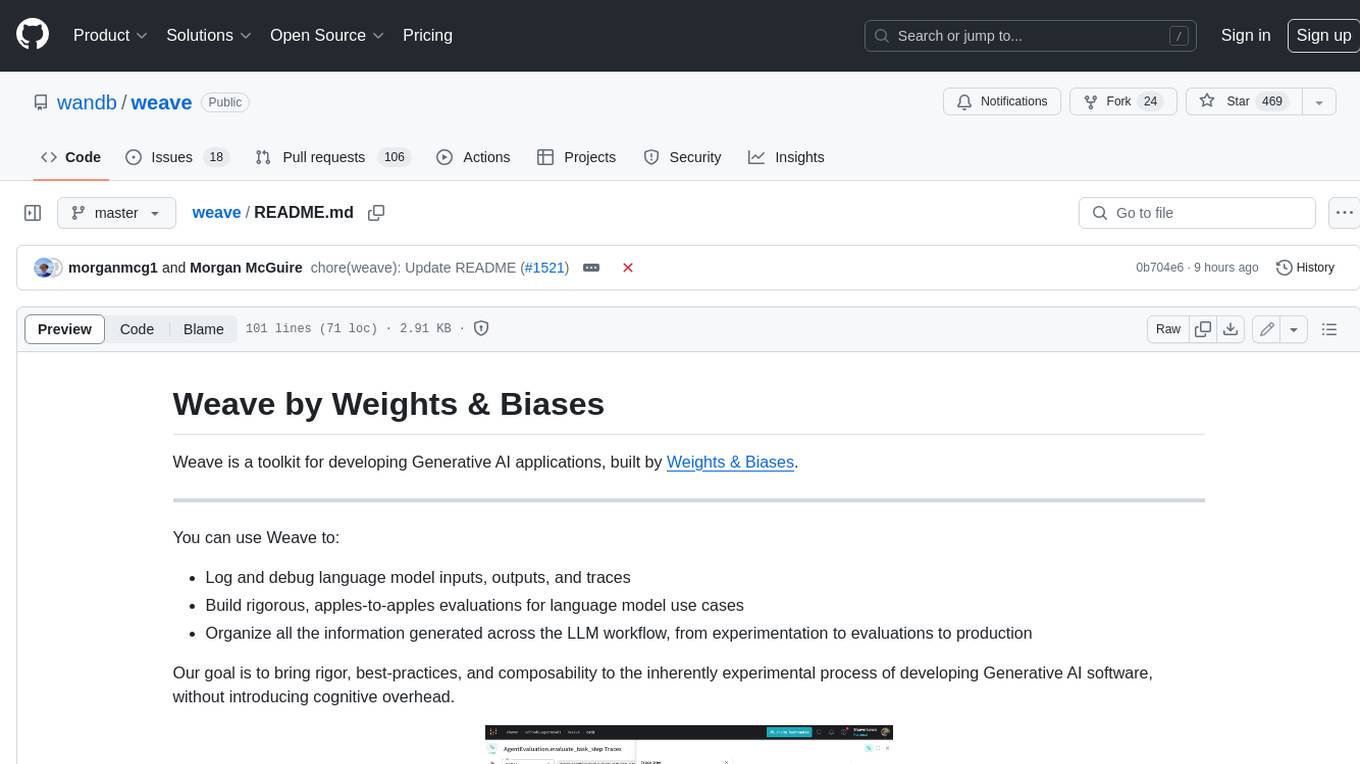
weave
Weave is a toolkit for developing Generative AI applications, built by Weights & Biases. With Weave, you can log and debug language model inputs, outputs, and traces; build rigorous, apples-to-apples evaluations for language model use cases; and organize all the information generated across the LLM workflow, from experimentation to evaluations to production. Weave aims to bring rigor, best-practices, and composability to the inherently experimental process of developing Generative AI software, without introducing cognitive overhead.
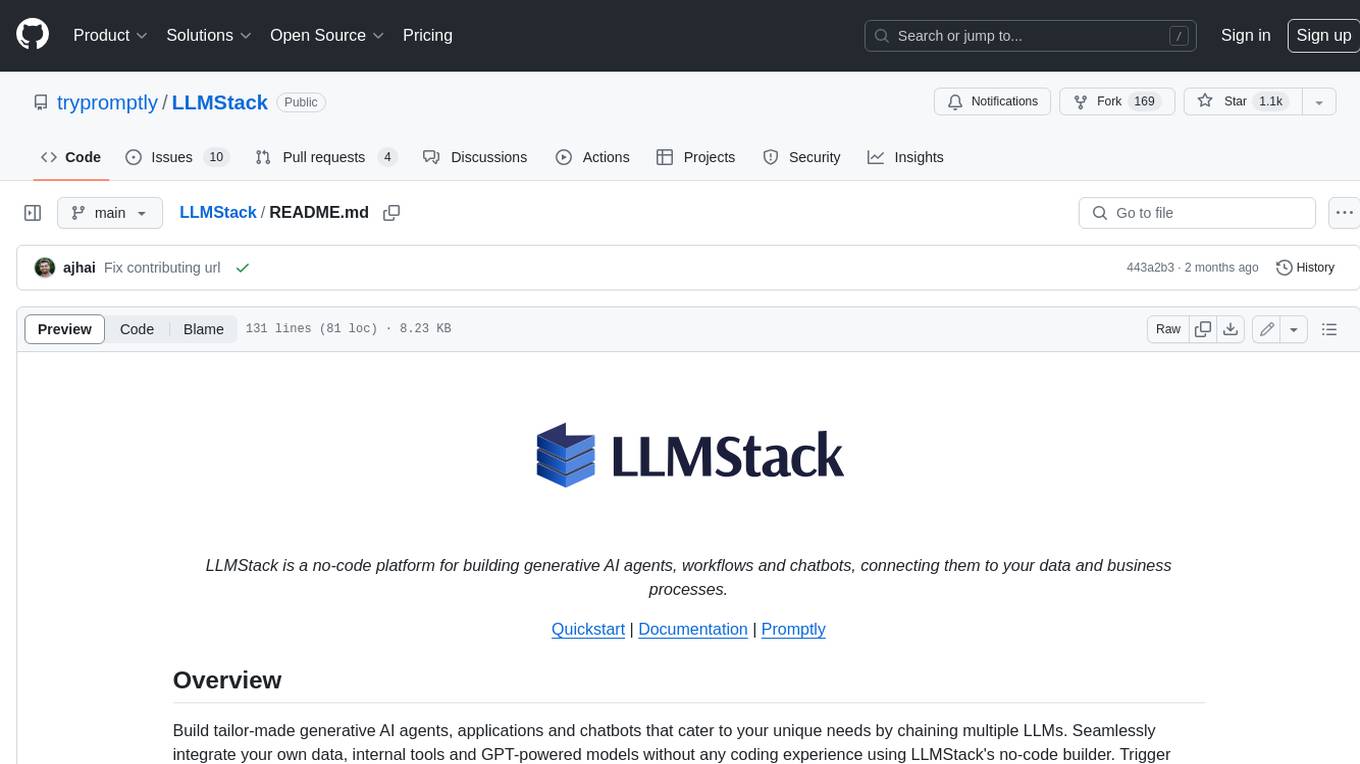
LLMStack
LLMStack is a no-code platform for building generative AI agents, workflows, and chatbots. It allows users to connect their own data, internal tools, and GPT-powered models without any coding experience. LLMStack can be deployed to the cloud or on-premise and can be accessed via HTTP API or triggered from Slack or Discord.
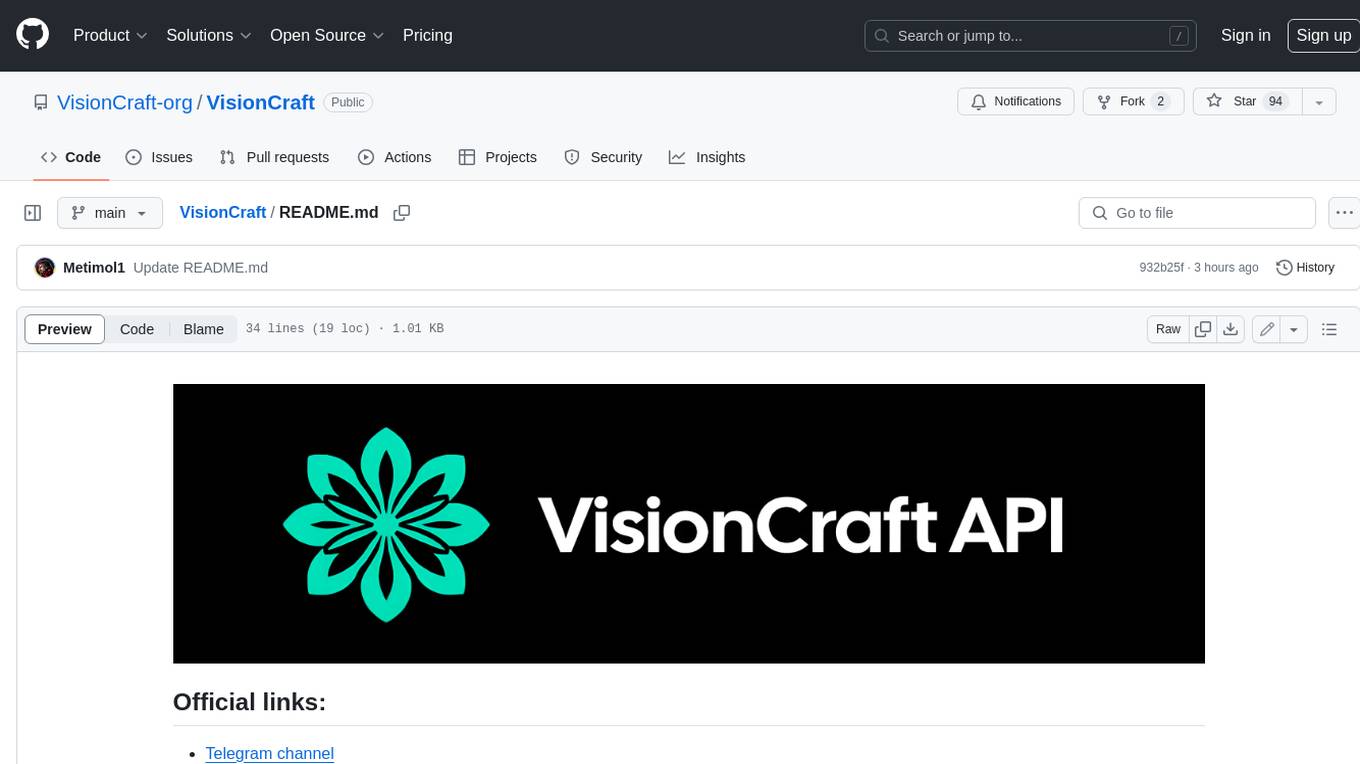
VisionCraft
The VisionCraft API is a free API for using over 100 different AI models. From images to sound.
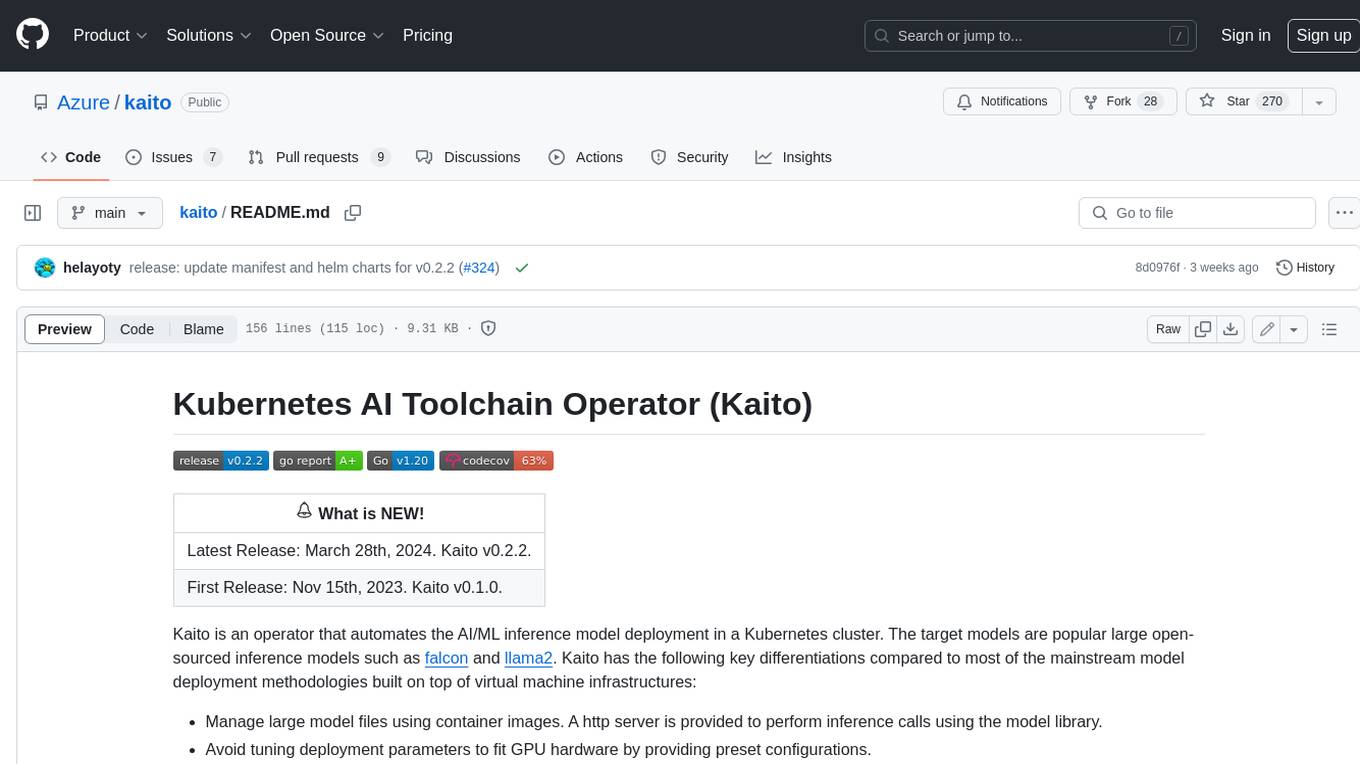
kaito
Kaito is an operator that automates the AI/ML inference model deployment in a Kubernetes cluster. It manages large model files using container images, avoids tuning deployment parameters to fit GPU hardware by providing preset configurations, auto-provisions GPU nodes based on model requirements, and hosts large model images in the public Microsoft Container Registry (MCR) if the license allows. Using Kaito, the workflow of onboarding large AI inference models in Kubernetes is largely simplified.
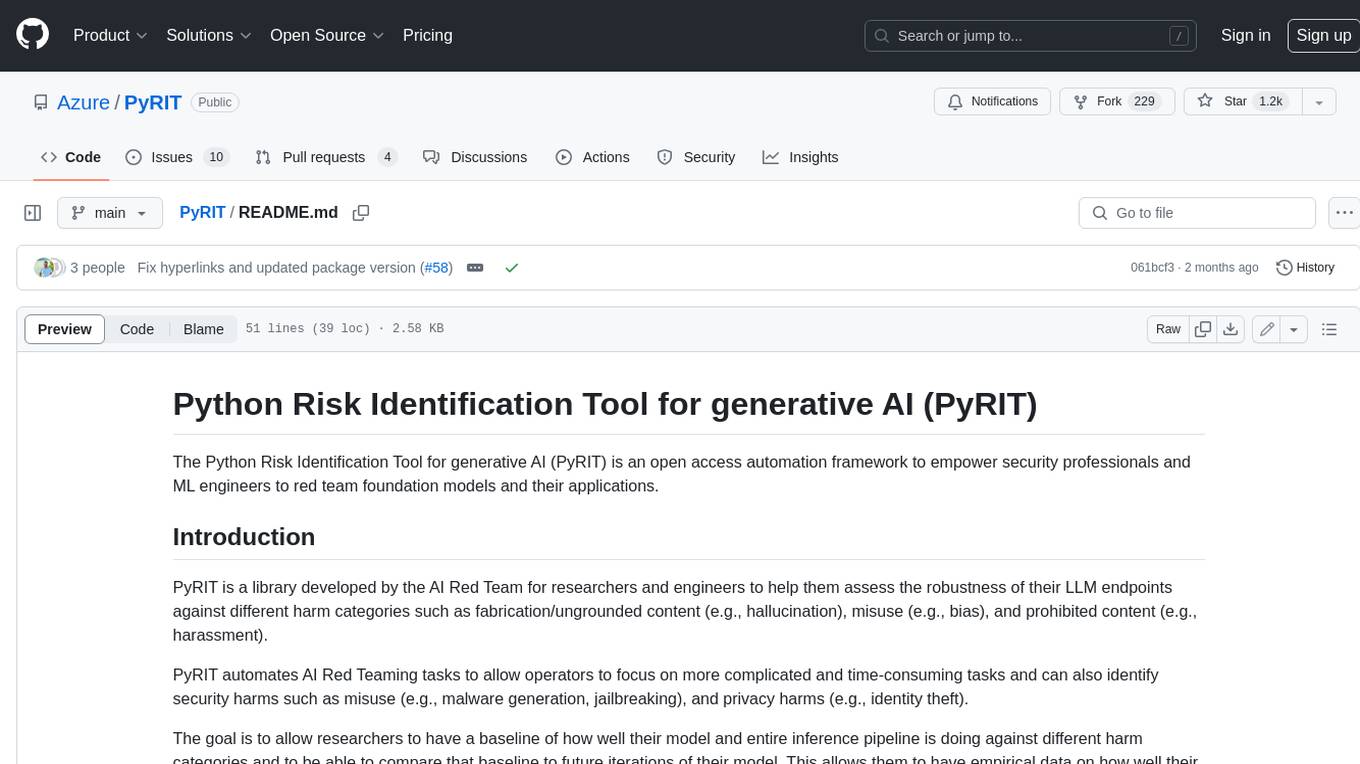
PyRIT
PyRIT is an open access automation framework designed to empower security professionals and ML engineers to red team foundation models and their applications. It automates AI Red Teaming tasks to allow operators to focus on more complicated and time-consuming tasks and can also identify security harms such as misuse (e.g., malware generation, jailbreaking), and privacy harms (e.g., identity theft). The goal is to allow researchers to have a baseline of how well their model and entire inference pipeline is doing against different harm categories and to be able to compare that baseline to future iterations of their model. This allows them to have empirical data on how well their model is doing today, and detect any degradation of performance based on future improvements.
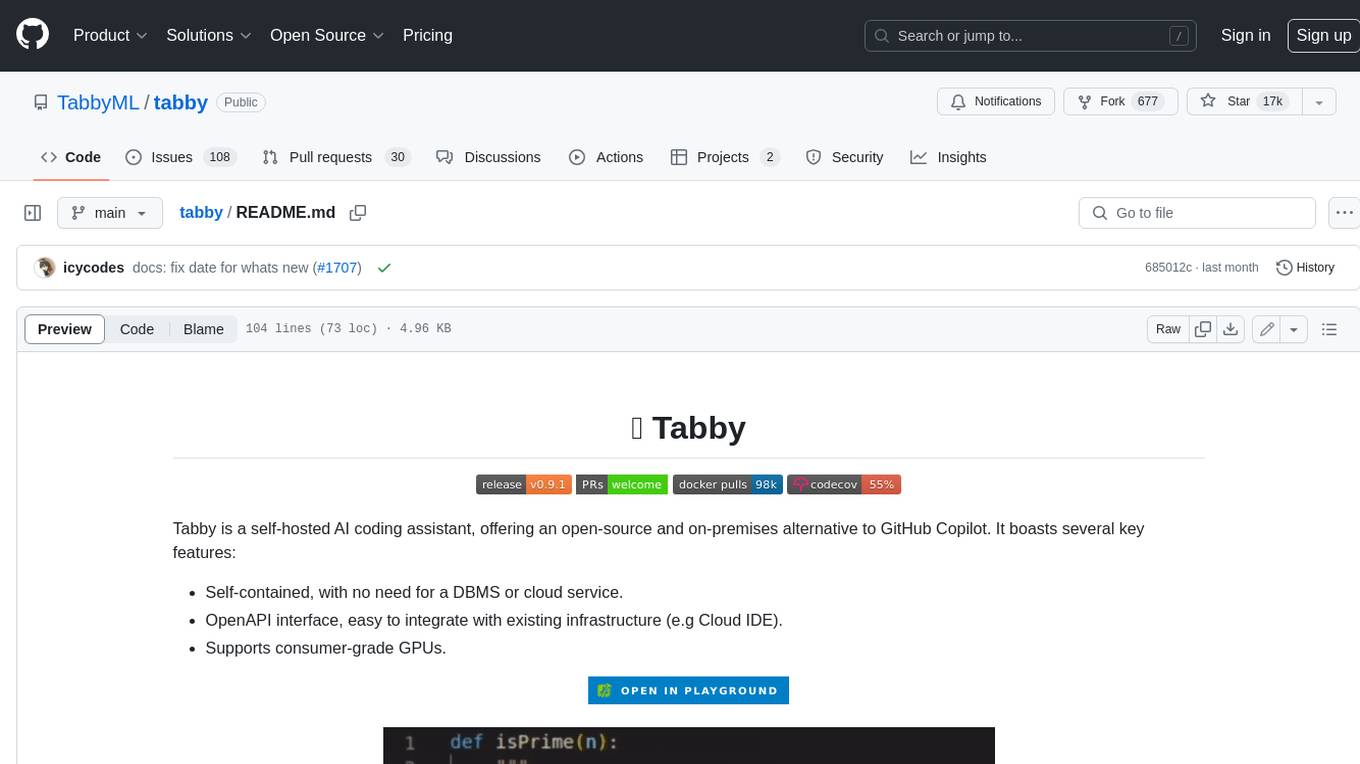
tabby
Tabby is a self-hosted AI coding assistant, offering an open-source and on-premises alternative to GitHub Copilot. It boasts several key features: * Self-contained, with no need for a DBMS or cloud service. * OpenAPI interface, easy to integrate with existing infrastructure (e.g Cloud IDE). * Supports consumer-grade GPUs.
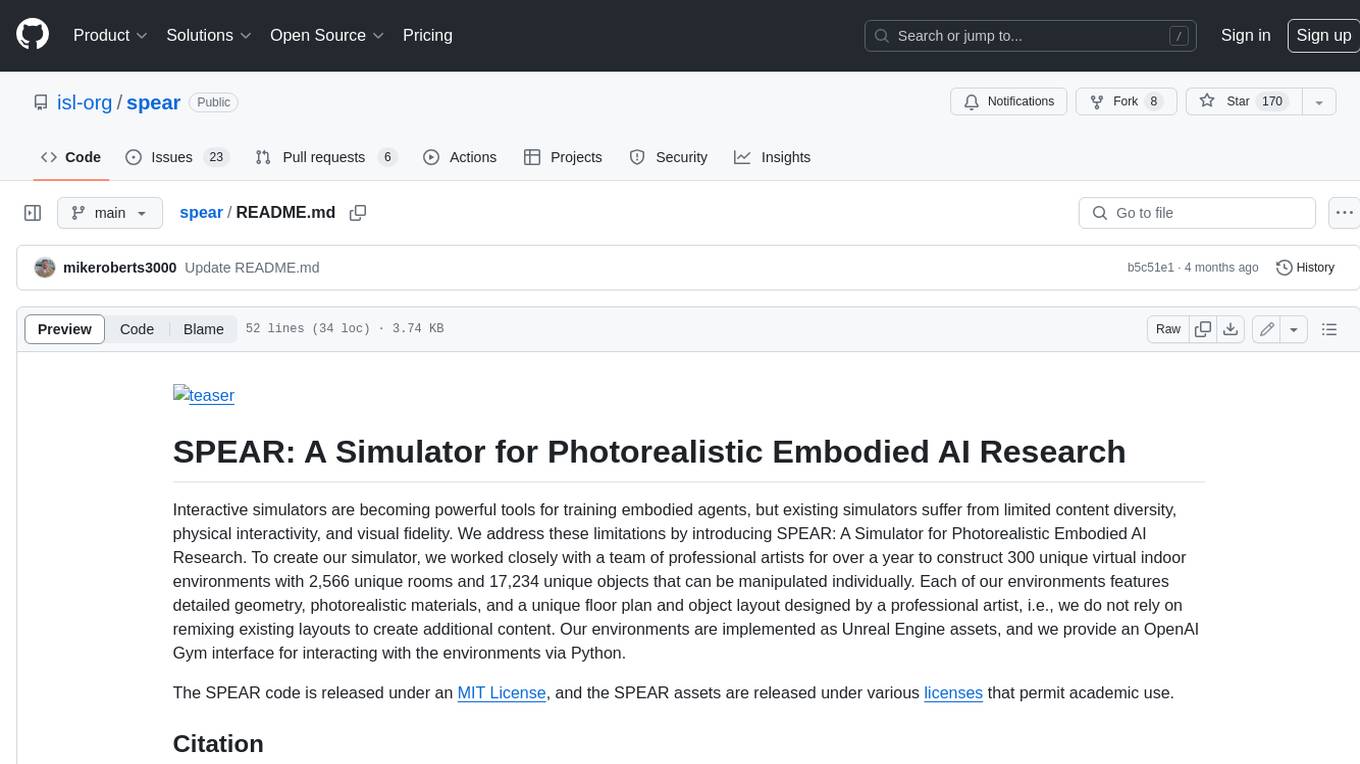
spear
SPEAR (Simulator for Photorealistic Embodied AI Research) is a powerful tool for training embodied agents. It features 300 unique virtual indoor environments with 2,566 unique rooms and 17,234 unique objects that can be manipulated individually. Each environment is designed by a professional artist and features detailed geometry, photorealistic materials, and a unique floor plan and object layout. SPEAR is implemented as Unreal Engine assets and provides an OpenAI Gym interface for interacting with the environments via Python.
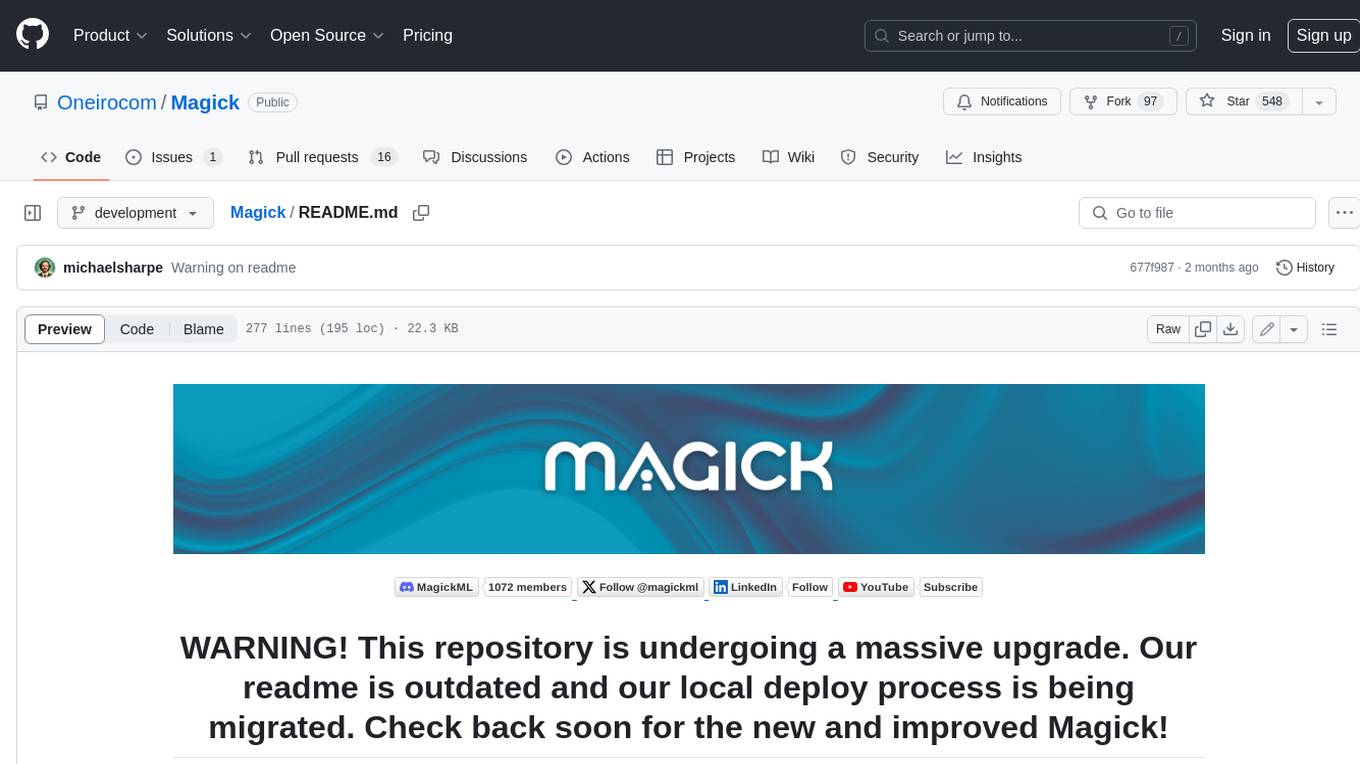
Magick
Magick is a groundbreaking visual AIDE (Artificial Intelligence Development Environment) for no-code data pipelines and multimodal agents. Magick can connect to other services and comes with nodes and templates well-suited for intelligent agents, chatbots, complex reasoning systems and realistic characters.