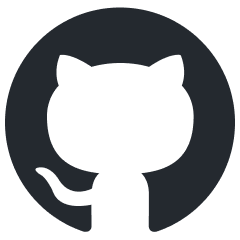
semantic-kernel
Integrate cutting-edge LLM technology quickly and easily into your apps
Stars: 23313
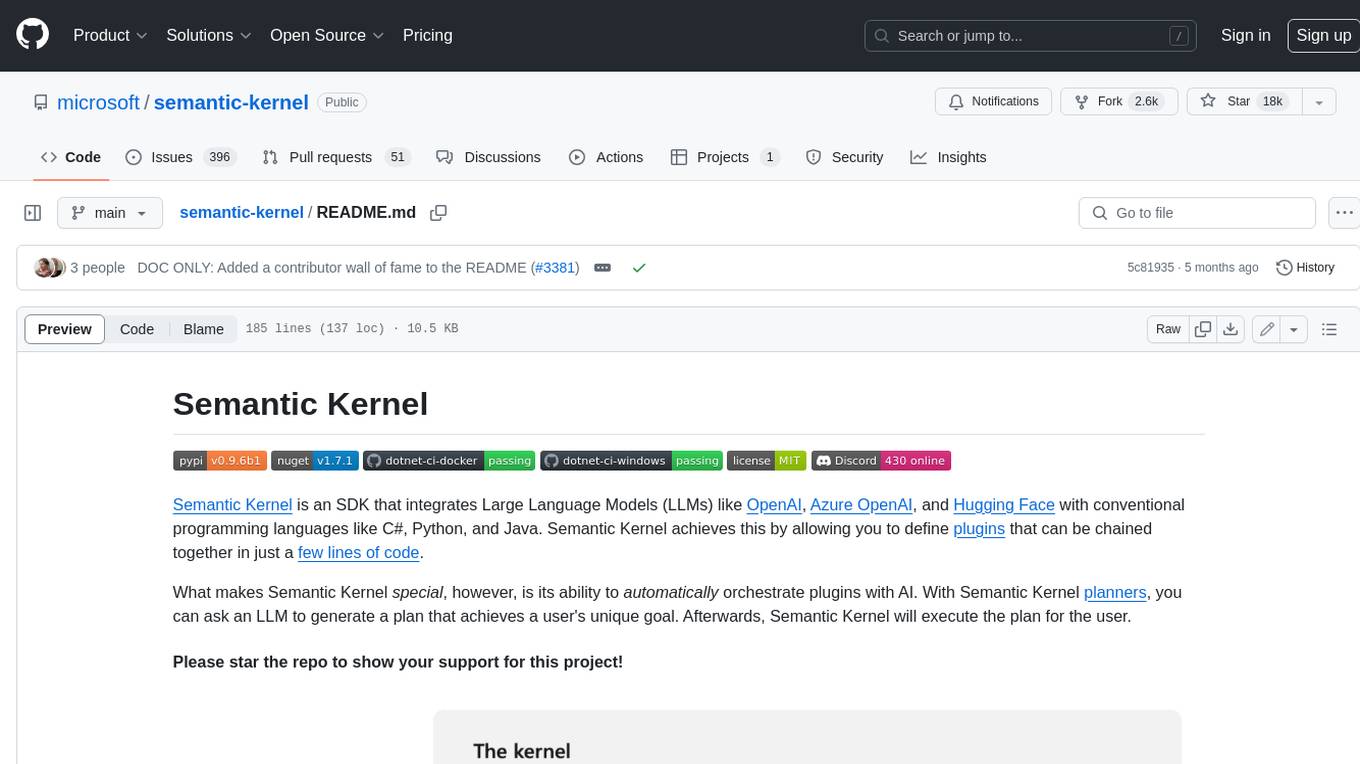
Semantic Kernel is an SDK that integrates Large Language Models (LLMs) like OpenAI, Azure OpenAI, and Hugging Face with conventional programming languages like C#, Python, and Java. Semantic Kernel achieves this by allowing you to define plugins that can be chained together in just a few lines of code. What makes Semantic Kernel _special_ , however, is its ability to _automatically_ orchestrate plugins with AI. With Semantic Kernel planners, you can ask an LLM to generate a plan that achieves a user's unique goal. Afterwards, Semantic Kernel will execute the plan for the user.
README:
Semantic Kernel is an SDK that integrates Large Language Models (LLMs) like OpenAI, Azure OpenAI, and Hugging Face with conventional programming languages like C#, Python, and Java. Semantic Kernel achieves this by allowing you to define plugins that can be chained together in just a few lines of code.
What makes Semantic Kernel special, however, is its ability to automatically orchestrate plugins with AI. With Semantic Kernel planners, you can ask an LLM to generate a plan that achieves a user's unique goal. Afterwards, Semantic Kernel will execute the plan for the user.
It provides:
- abstractions for AI services (such as chat, text to images, audio to text, etc.) and memory stores
- implementations of those abstractions for services from OpenAI, Azure OpenAI, Hugging Face, local models, and more, and for a multitude of vector databases, such as those from Chroma, Qdrant, Milvus, and Azure
- a common representation for plugins, which can then be orchestrated automatically by AI
- the ability to create such plugins from a multitude of sources, including from OpenAPI specifications, prompts, and arbitrary code written in the target language
- extensible support for prompt management and rendering, including built-in handling of common formats like Handlebars and Liquid
- and a wealth of functionality layered on top of these abstractions, such as filters for responsible AI, dependency injection integration, and more.
Semantic Kernel is utilized by enterprises due to its flexibility, modularity and observability. Backed with security enhancing capabilities like telemetry support, and hooks and filters so you’ll feel confident you’re delivering responsible AI solutions at scale. Semantic Kernel was designed to be future proof, easily connecting your code to the latest AI models evolving with the technology as it advances. When new models are released, you’ll simply swap them out without needing to rewrite your entire codebase.
The Semantic Kernel SDK is available in C#, Python, and Java. To get started, choose your preferred language below. See the Feature Matrix for a breakdown of feature parity between our currently supported languages.
![]() |
|
|
The quickest way to get started with the basics is to get an API key from either OpenAI or Azure OpenAI and to run one of the C#, Python, and Java console applications/scripts below.
- Go to the Quick start page here and follow the steps to dive in.
- After Installing the SDK, we advise you follow the steps and code detailed to write your first console app.
- Go to the Quick start page here and follow the steps to dive in.
- You'll need to ensure that you toggle to Python in the Choose a programming language table at the top of the page.
The Java code is in the semantic-kernel-java repository. See semantic-kernel-java build for instructions on how to build and run the Java code.
Please file Java Semantic Kernel specific issues in the semantic-kernel-java repository.
The fastest way to learn how to use Semantic Kernel is with our C# and Python Jupyter notebooks. These notebooks demonstrate how to use Semantic Kernel with code snippets that you can run with a push of a button.
Once you've finished the getting started notebooks, you can then check out the main walkthroughs on our Learn site. Each sample comes with a completed C# and Python project that you can run locally.
Finally, refer to our API references for more details on the C# and Python APIs:
- C# API reference
- Python API reference
- Java API reference (coming soon)
The Semantic Kernel extension for Visual Studio Code makes it easy to design and test semantic functions. The extension provides an interface for designing semantic functions and allows you to test them with the push of a button with your existing models and data.
We welcome your contributions and suggestions to SK community! One of the easiest ways to participate is to engage in discussions in the GitHub repository. Bug reports and fixes are welcome!
For new features, components, or extensions, please open an issue and discuss with us before sending a PR. This is to avoid rejection as we might be taking the core in a different direction, but also to consider the impact on the larger ecosystem.
To learn more and get started:
-
Read the documentation
-
Learn how to contribute to the project
-
Ask questions in the GitHub discussions
-
Ask questions in the Discord community
-
Follow the team on our blog
This project has adopted the Microsoft Open Source Code of Conduct. For more information see the Code of Conduct FAQ or contact [email protected] with any additional questions or comments.
Copyright (c) Microsoft Corporation. All rights reserved.
Licensed under the MIT license.
For Tasks:
Click tags to check more tools for each tasksFor Jobs:
Alternative AI tools for semantic-kernel
Similar Open Source Tools
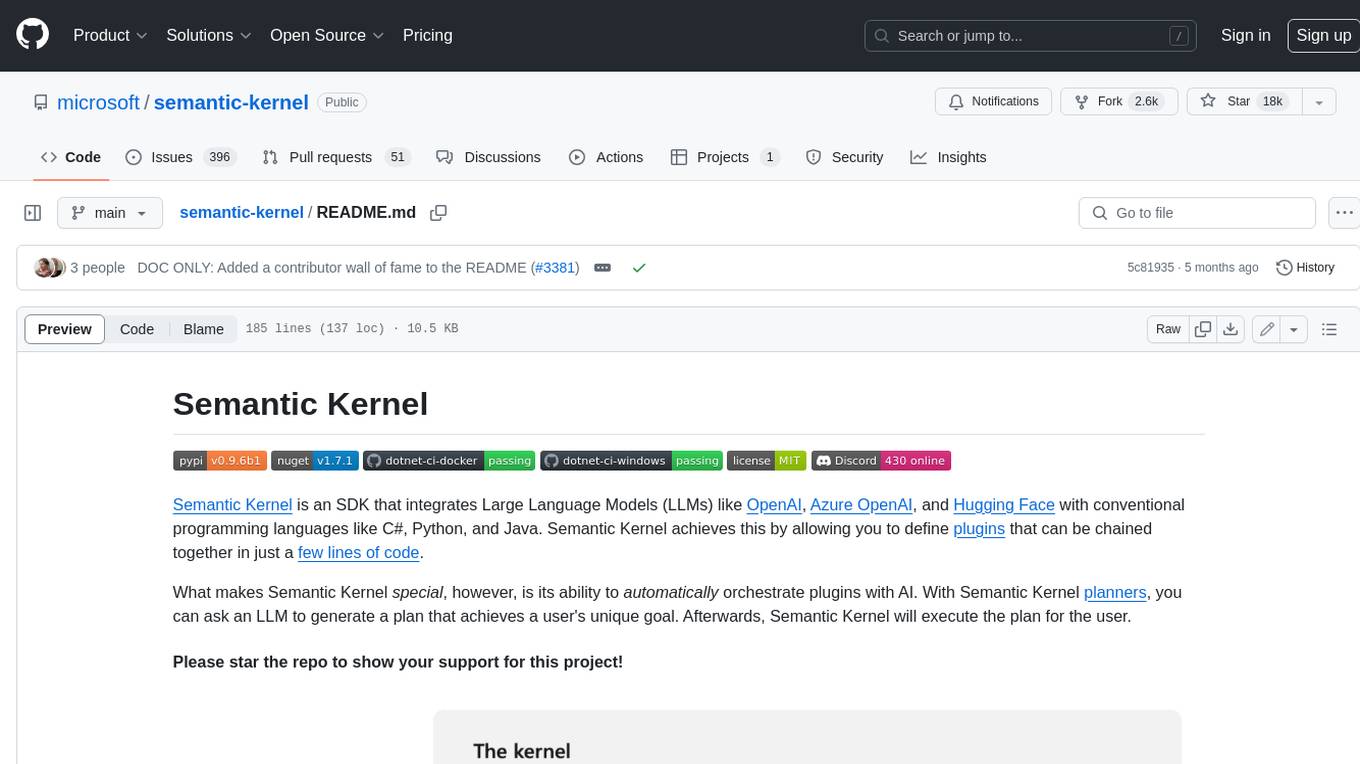
semantic-kernel
Semantic Kernel is an SDK that integrates Large Language Models (LLMs) like OpenAI, Azure OpenAI, and Hugging Face with conventional programming languages like C#, Python, and Java. Semantic Kernel achieves this by allowing you to define plugins that can be chained together in just a few lines of code. What makes Semantic Kernel _special_ , however, is its ability to _automatically_ orchestrate plugins with AI. With Semantic Kernel planners, you can ask an LLM to generate a plan that achieves a user's unique goal. Afterwards, Semantic Kernel will execute the plan for the user.
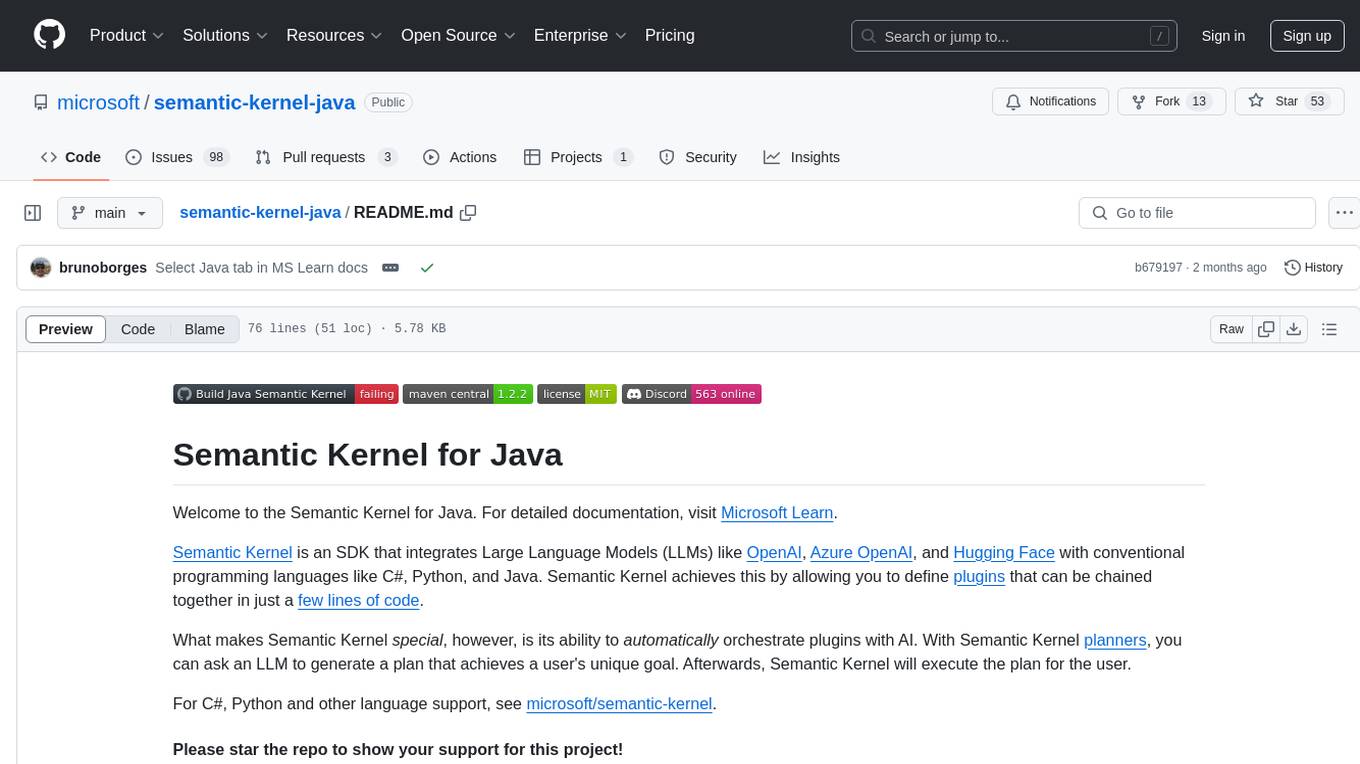
semantic-kernel-java
Semantic Kernel for Java is an SDK that integrates Large Language Models (LLMs) like OpenAI, Azure OpenAI, and Hugging Face with conventional programming languages like C#, Python, and Java. It allows defining plugins that can be chained together in just a few lines of code. The tool automatically orchestrates plugins with AI, enabling users to generate plans to achieve unique goals and execute them. The project welcomes contributions, bug reports, and suggestions from the community.
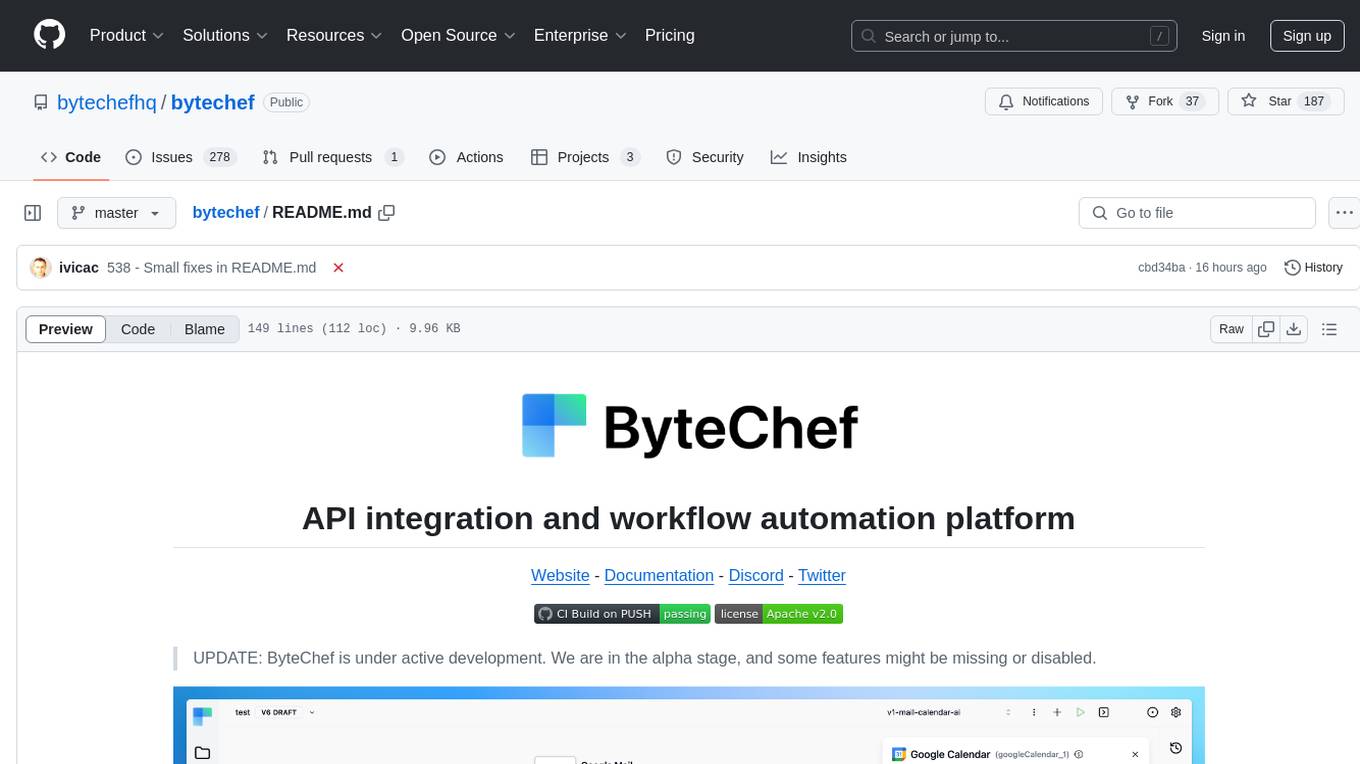
bytechef
ByteChef is an open-source, low-code, extendable API integration and workflow automation platform. It provides an intuitive UI Workflow Editor, event-driven & scheduled workflows, multiple flow controls, built-in code editor supporting Java, JavaScript, Python, and Ruby, rich component ecosystem, extendable with custom connectors, AI-ready with built-in AI components, developer-ready to expose workflows as APIs, version control friendly, self-hosted, scalable, and resilient. It allows users to build and visualize workflows, automate tasks across SaaS apps, internal APIs, and databases, and handle millions of workflows with high availability and fault tolerance.
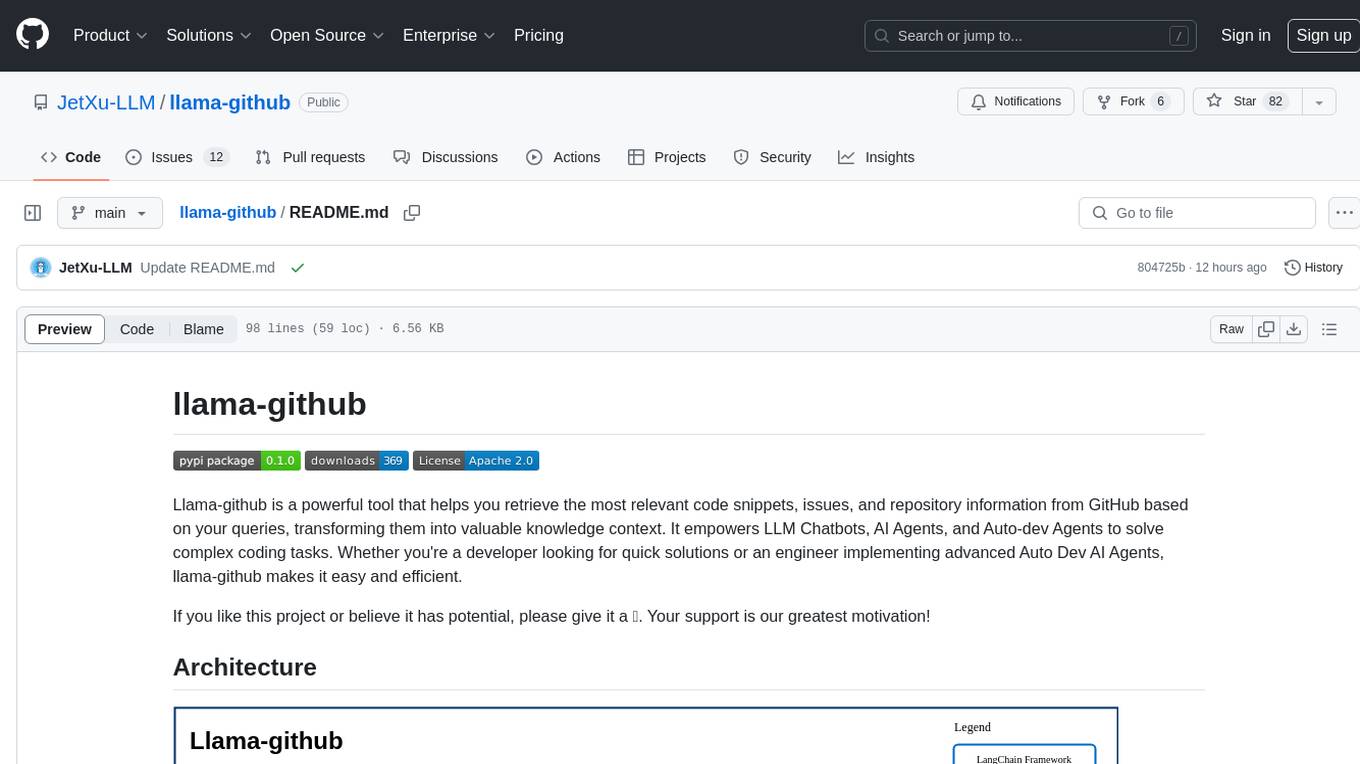
llama-github
Llama-github is a powerful tool that helps retrieve relevant code snippets, issues, and repository information from GitHub based on queries. It empowers AI agents and developers to solve coding tasks efficiently. With features like intelligent GitHub retrieval, repository pool caching, LLM-powered question analysis, and comprehensive context generation, llama-github excels at providing valuable knowledge context for development needs. It supports asynchronous processing, flexible LLM integration, robust authentication options, and logging/error handling for smooth operations and troubleshooting. The vision is to seamlessly integrate with GitHub for AI-driven development solutions, while the roadmap focuses on empowering LLMs to automatically resolve complex coding tasks.
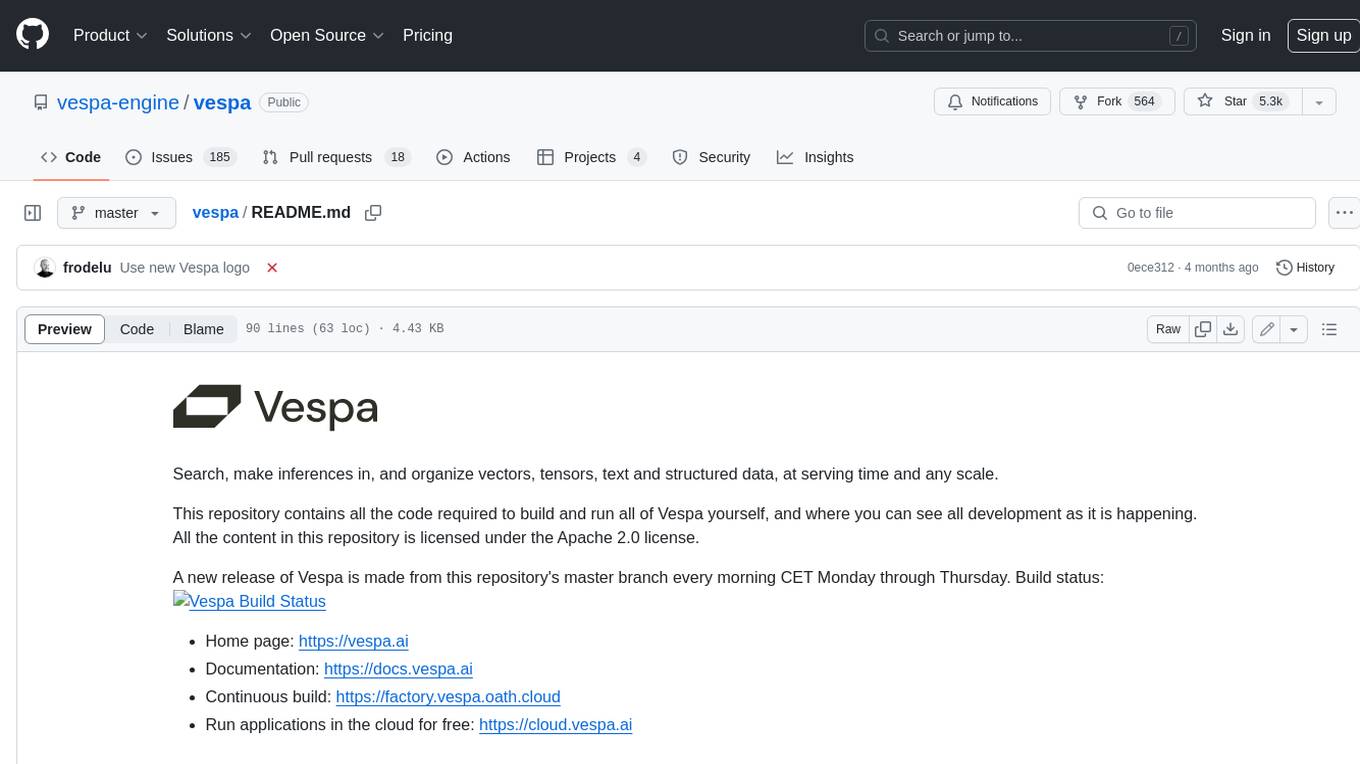
vespa
Vespa is a platform that performs operations such as selecting a subset of data in a large corpus, evaluating machine-learned models over the selected data, organizing and aggregating it, and returning it, typically in less than 100 milliseconds, all while the data corpus is continuously changing. It has been in development for many years and is used on a number of large internet services and apps which serve hundreds of thousands of queries from Vespa per second.
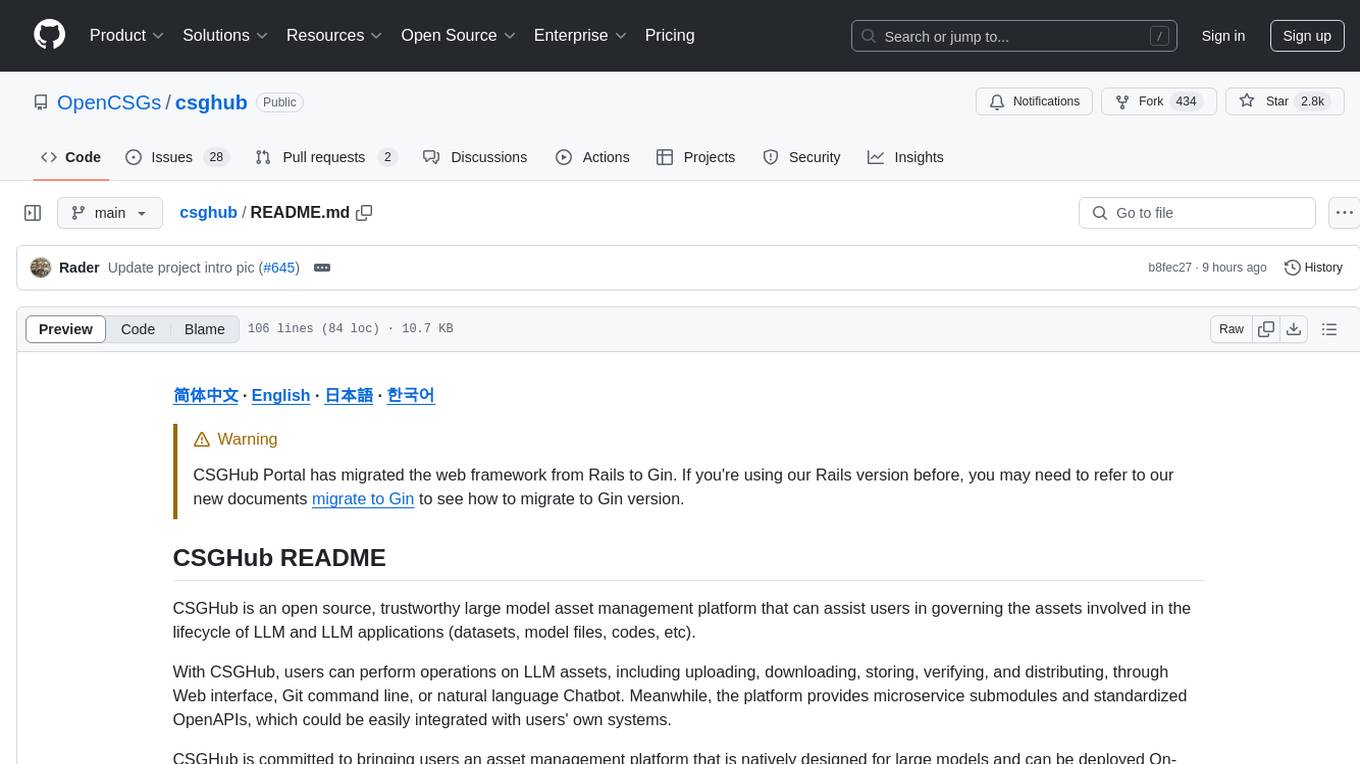
csghub
CSGHub is an open source platform for managing large model assets, including datasets, model files, and codes. It offers functionalities similar to a privatized Huggingface, managing assets in a manner akin to how OpenStack Glance manages virtual machine images. Users can perform operations such as uploading, downloading, storing, verifying, and distributing assets through various interfaces. The platform provides microservice submodules and standardized OpenAPIs for easy integration with users' systems. CSGHub is designed for large models and can be deployed On-Premise for offline operation.
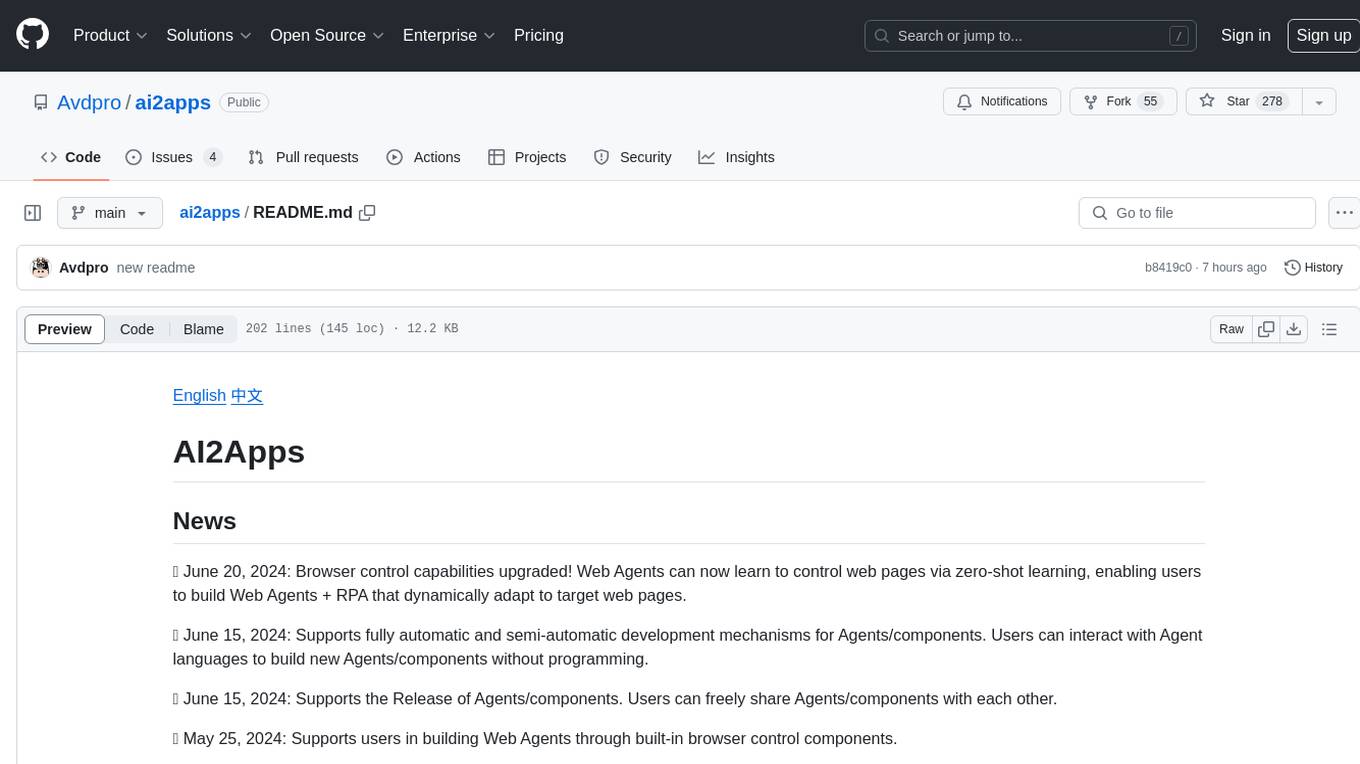
ai2apps
AI2Apps is a visual IDE for building LLM-based AI agent applications, enabling developers to efficiently create AI agents through drag-and-drop, with features like design-to-development for rapid prototyping, direct packaging of agents into apps, powerful debugging capabilities, enhanced user interaction, efficient team collaboration, flexible deployment, multilingual support, simplified product maintenance, and extensibility through plugins.
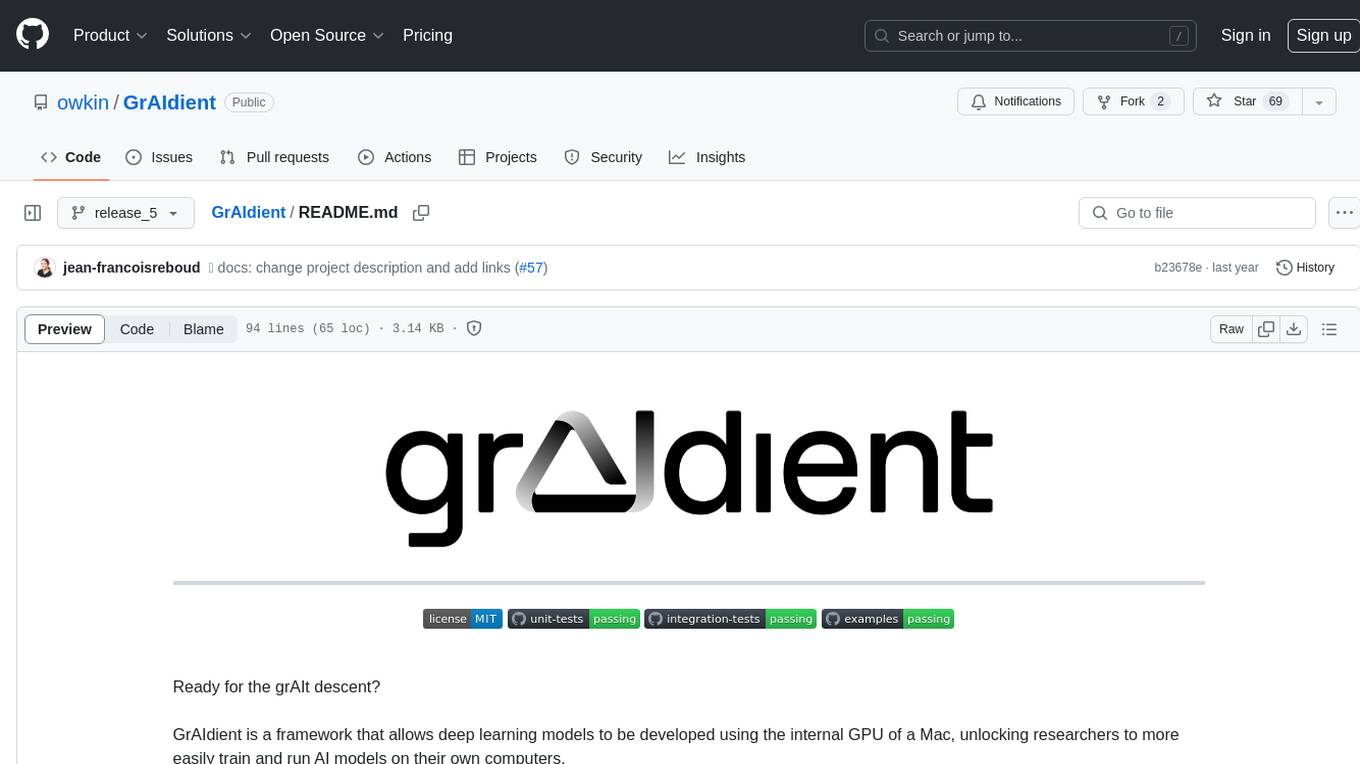
GrAIdient
GrAIdient is a framework designed to enable the development of deep learning models using the internal GPU of a Mac. It provides access to the graph of layers, allowing for unique model design with greater understanding, control, and reproducibility. The goal is to challenge the understanding of deep learning models, transitioning from black box to white box models. Key features include direct access to layers, native Mac GPU support, Swift language implementation, gradient checking, PyTorch interoperability, and more. The documentation covers main concepts, architecture, and examples. GrAIdient is MIT licensed.
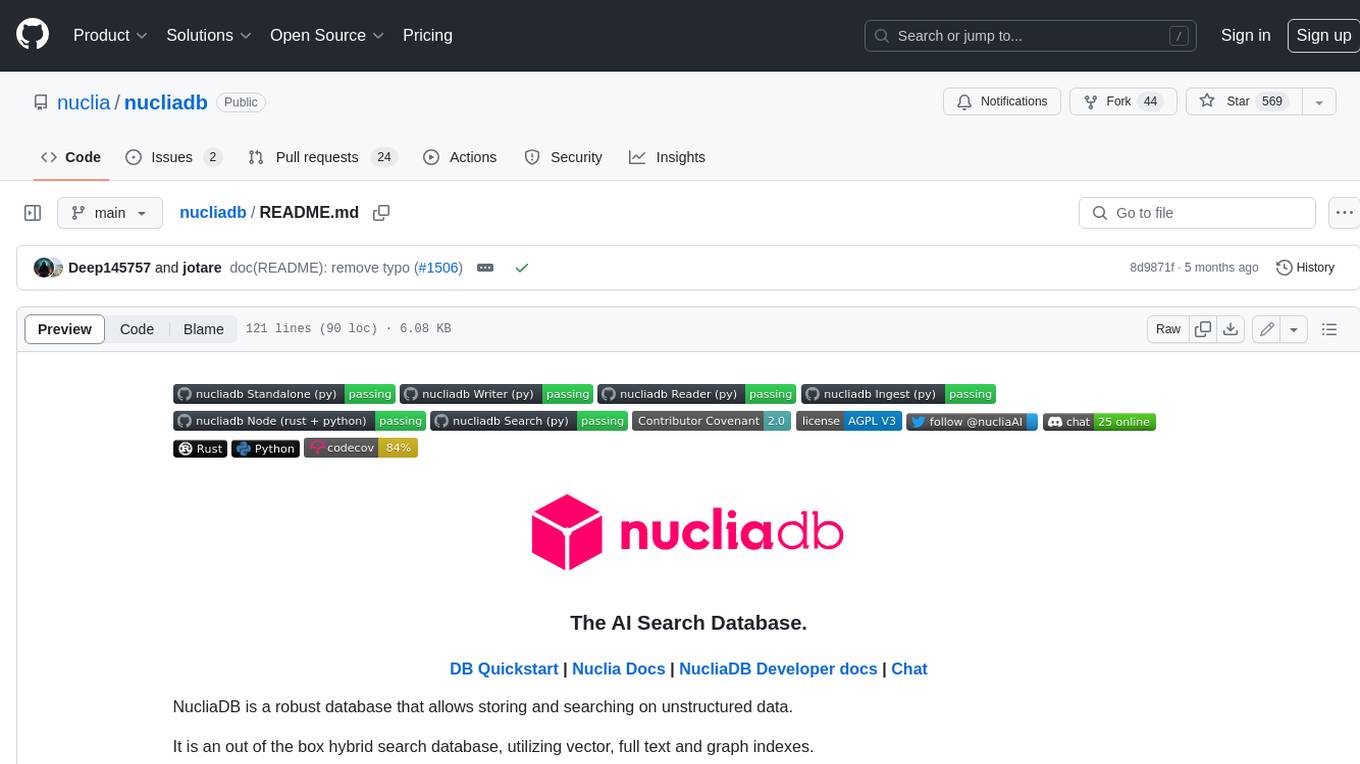
nucliadb
NucliaDB is a robust database that allows storing and searching on unstructured data. It is an out of the box hybrid search database, utilizing vector, full text and graph indexes. NucliaDB is written in Rust and Python. We designed it to index large datasets and provide multi-teanant support. When utilizing NucliaDB with Nuclia cloud, you are able to the power of an NLP database without the hassle of data extraction, enrichment and inference. We do all the hard work for you.
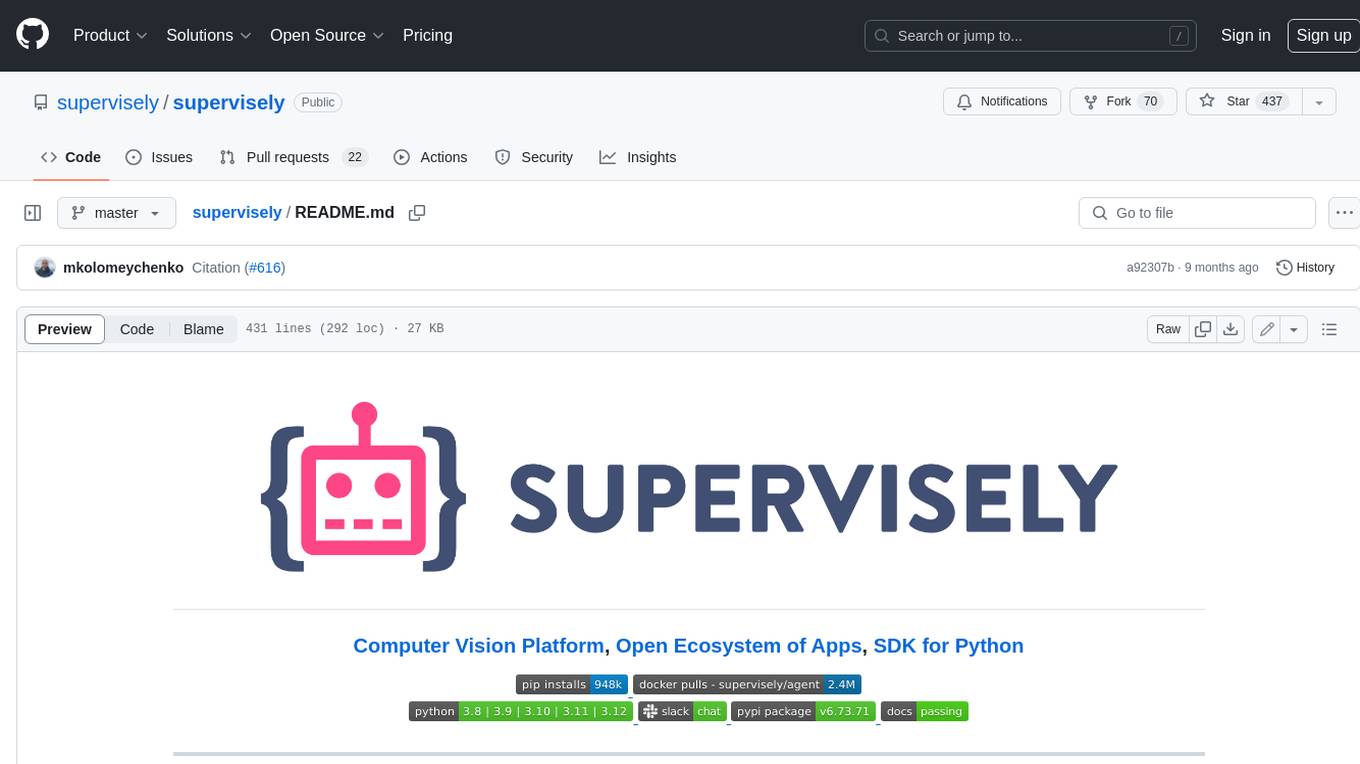
supervisely
Supervisely is a computer vision platform that provides a range of tools and services for developing and deploying computer vision solutions. It includes a data labeling platform, a model training platform, and a marketplace for computer vision apps. Supervisely is used by a variety of organizations, including Fortune 500 companies, research institutions, and government agencies.
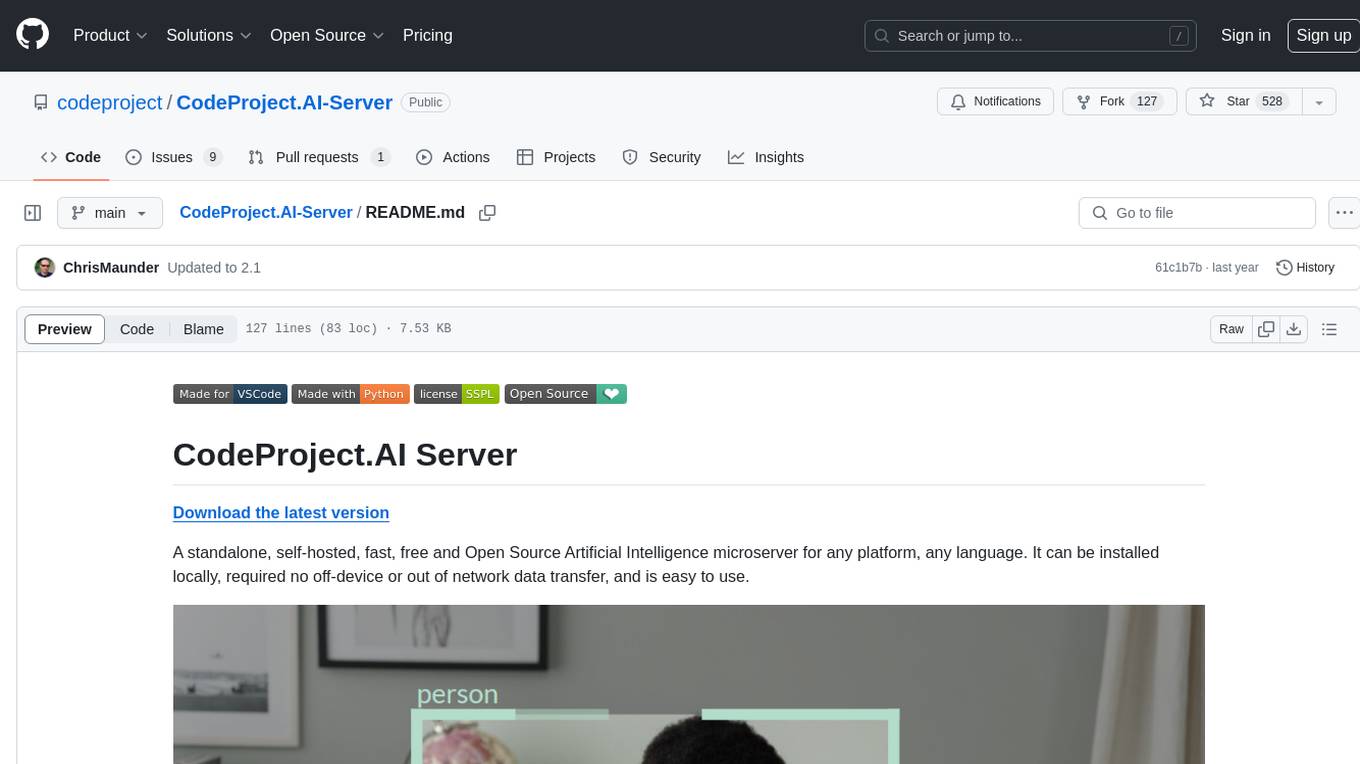
CodeProject.AI-Server
CodeProject.AI Server is a standalone, self-hosted, fast, free, and open-source Artificial Intelligence microserver designed for any platform and language. It can be installed locally without the need for off-device or out-of-network data transfer, providing an easy-to-use solution for developers interested in AI programming. The server includes a HTTP REST API server, backend analysis services, and the source code, enabling users to perform various AI tasks locally without relying on external services or cloud computing. Current capabilities include object detection, face detection, scene recognition, sentiment analysis, and more, with ongoing feature expansions planned. The project aims to promote AI development, simplify AI implementation, focus on core use-cases, and leverage the expertise of the developer community.
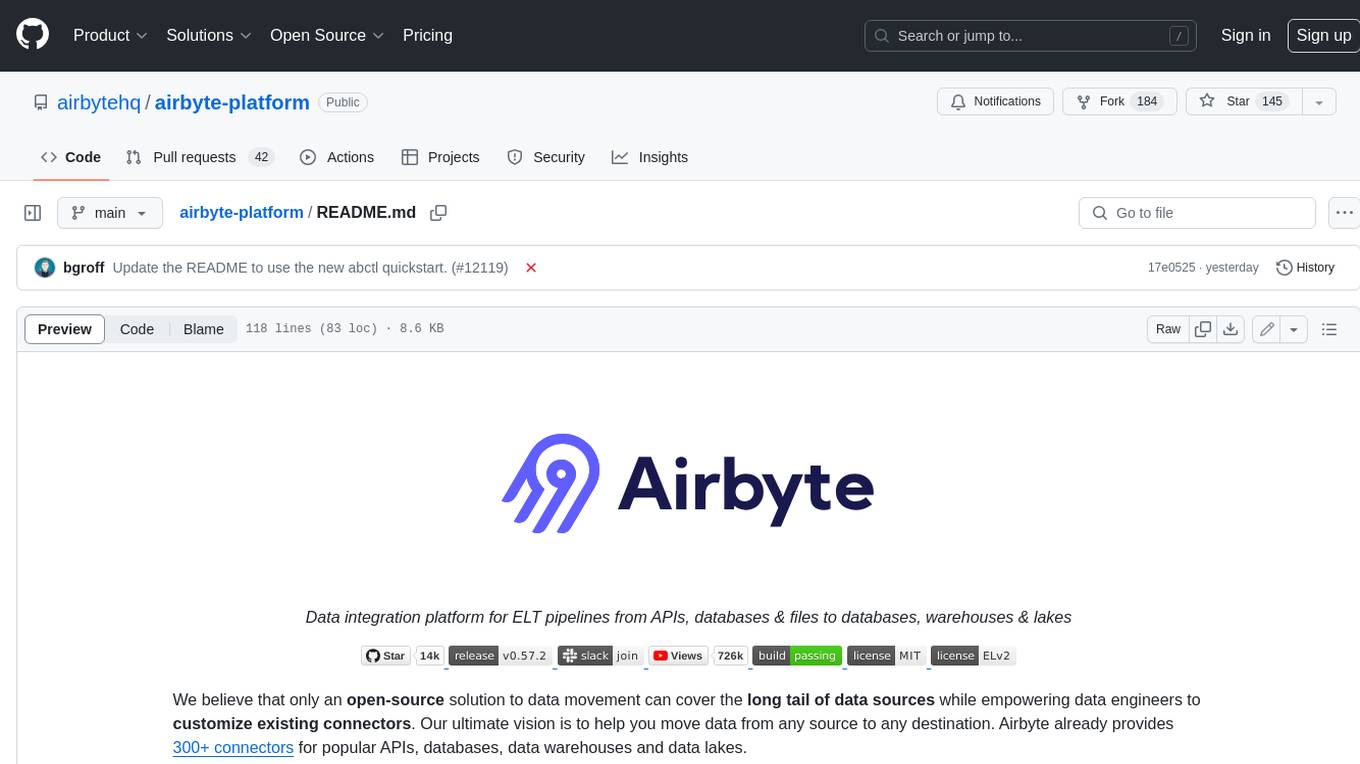
airbyte-platform
Airbyte is an open-source data integration platform that makes it easy to move data from any source to any destination. With Airbyte, you can build and manage data pipelines without writing any code. Airbyte provides a library of pre-built connectors that make it easy to connect to popular data sources and destinations. You can also create your own connectors using Airbyte's low-code Connector Development Kit (CDK). Airbyte is used by data engineers and analysts at companies of all sizes to move data for a variety of purposes, including data warehousing, data analysis, and machine learning.
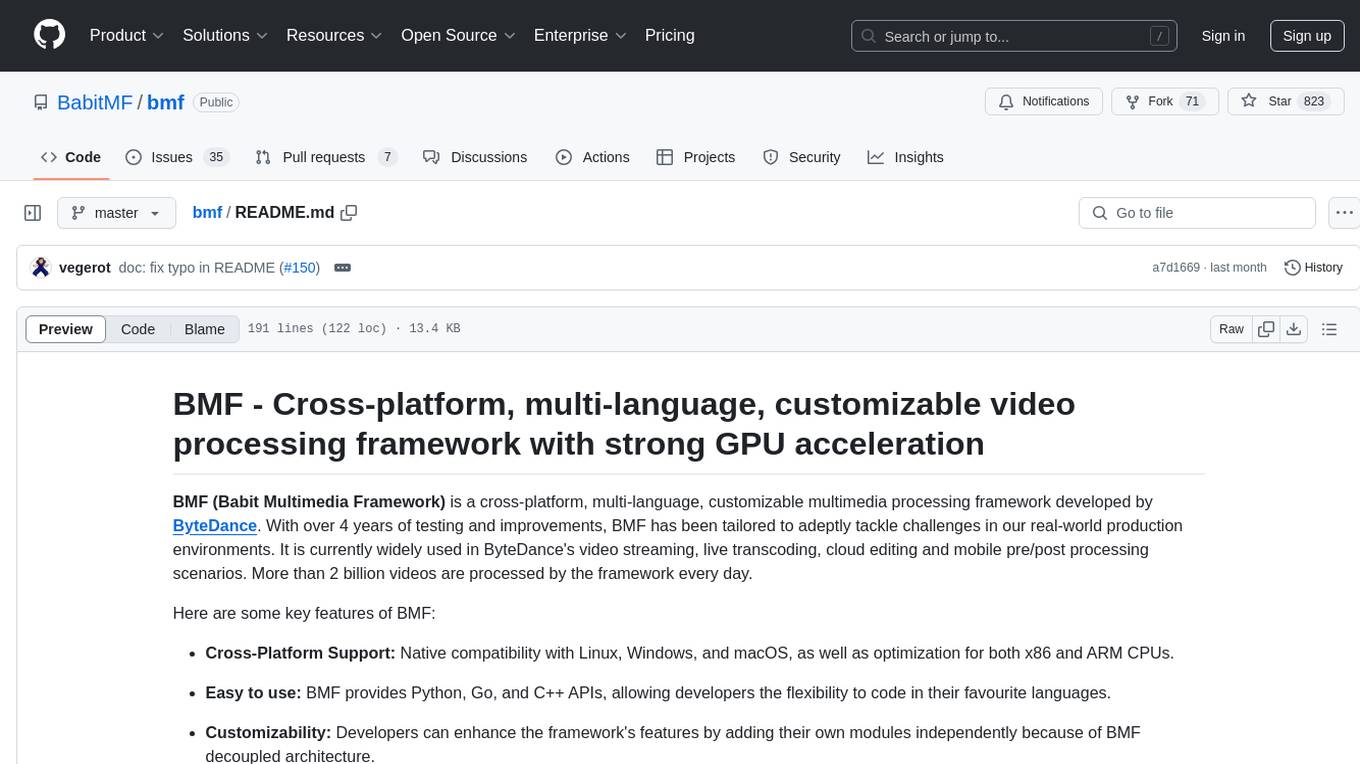
bmf
BMF (Babit Multimedia Framework) is a cross-platform, multi-language, customizable multimedia processing framework developed by ByteDance. It offers native compatibility with Linux, Windows, and macOS, Python, Go, and C++ APIs, and high performance with strong GPU acceleration. BMF allows developers to enhance its features independently and provides efficient data conversion across popular frameworks and hardware devices. BMFLite is a client-side lightweight framework used in apps like Douyin/Xigua, serving over one billion users daily. BMF is widely used in video streaming, live transcoding, cloud editing, and mobile pre/post processing scenarios.
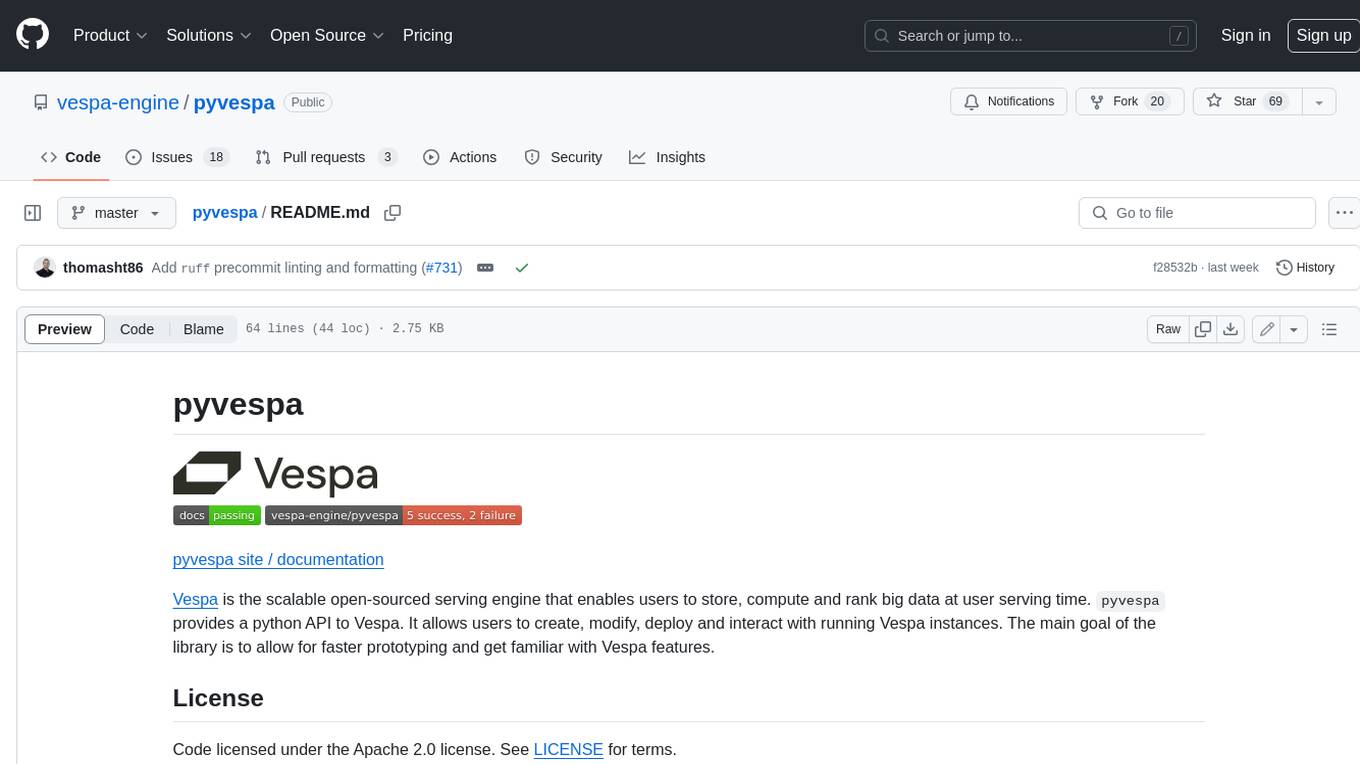
pyvespa
Vespa is a scalable open-source serving engine that enables users to store, compute, and rank big data at user serving time. Pyvespa provides a Python API to Vespa, allowing users to create, modify, deploy, and interact with running Vespa instances. The library's primary purpose is to facilitate faster prototyping and familiarization with Vespa features.
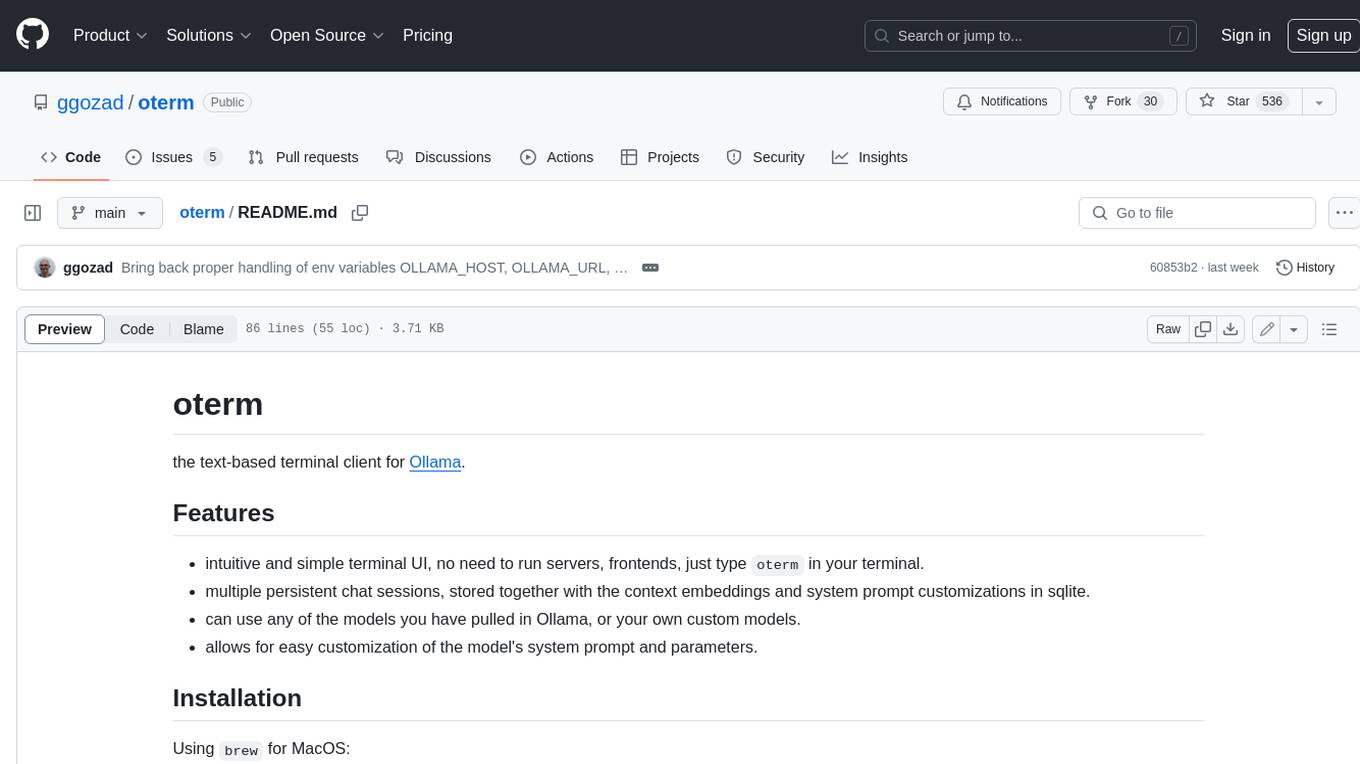
oterm
Oterm is a text-based terminal client for Ollama, a large language model. It provides an intuitive and simple terminal UI, allowing users to interact with Ollama without running servers or frontends. Oterm supports multiple persistent chat sessions, which are stored along with context embeddings and system prompt customizations in a SQLite database. Users can easily customize the model's system prompt and parameters, and select from any of the models they have pulled in Ollama or their own custom models. Oterm also supports keyboard shortcuts for creating new chat sessions, editing existing sessions, renaming sessions, exporting sessions as markdown, deleting sessions, toggling between dark and light themes, quitting the application, switching to multiline input mode, selecting images to include with messages, and navigating through the history of previous prompts. Oterm is licensed under the MIT License.
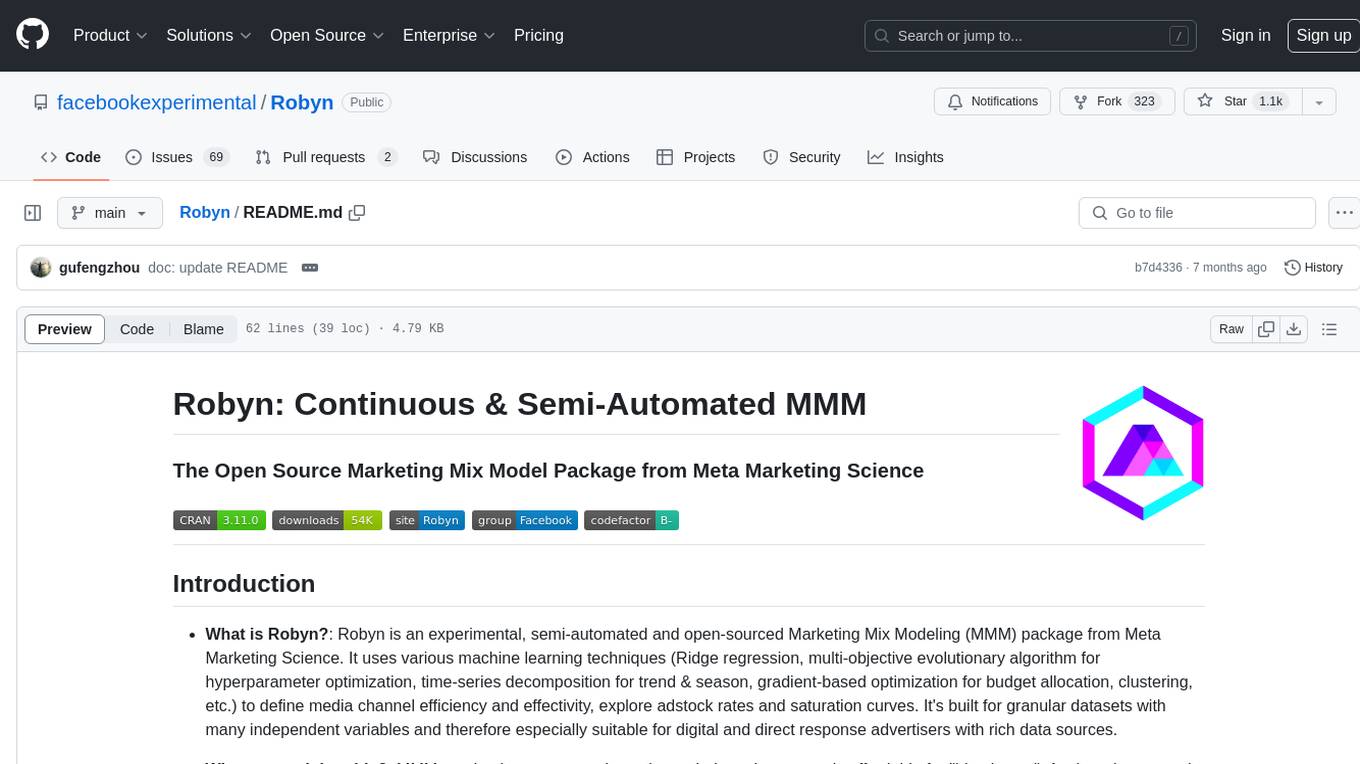
Robyn
Robyn is an experimental, semi-automated and open-sourced Marketing Mix Modeling (MMM) package from Meta Marketing Science. It uses various machine learning techniques to define media channel efficiency and effectivity, explore adstock rates and saturation curves. Built for granular datasets with many independent variables, especially suitable for digital and direct response advertisers with rich data sources. Aiming to democratize MMM, make it accessible for advertisers of all sizes, and contribute to the measurement landscape.
For similar tasks
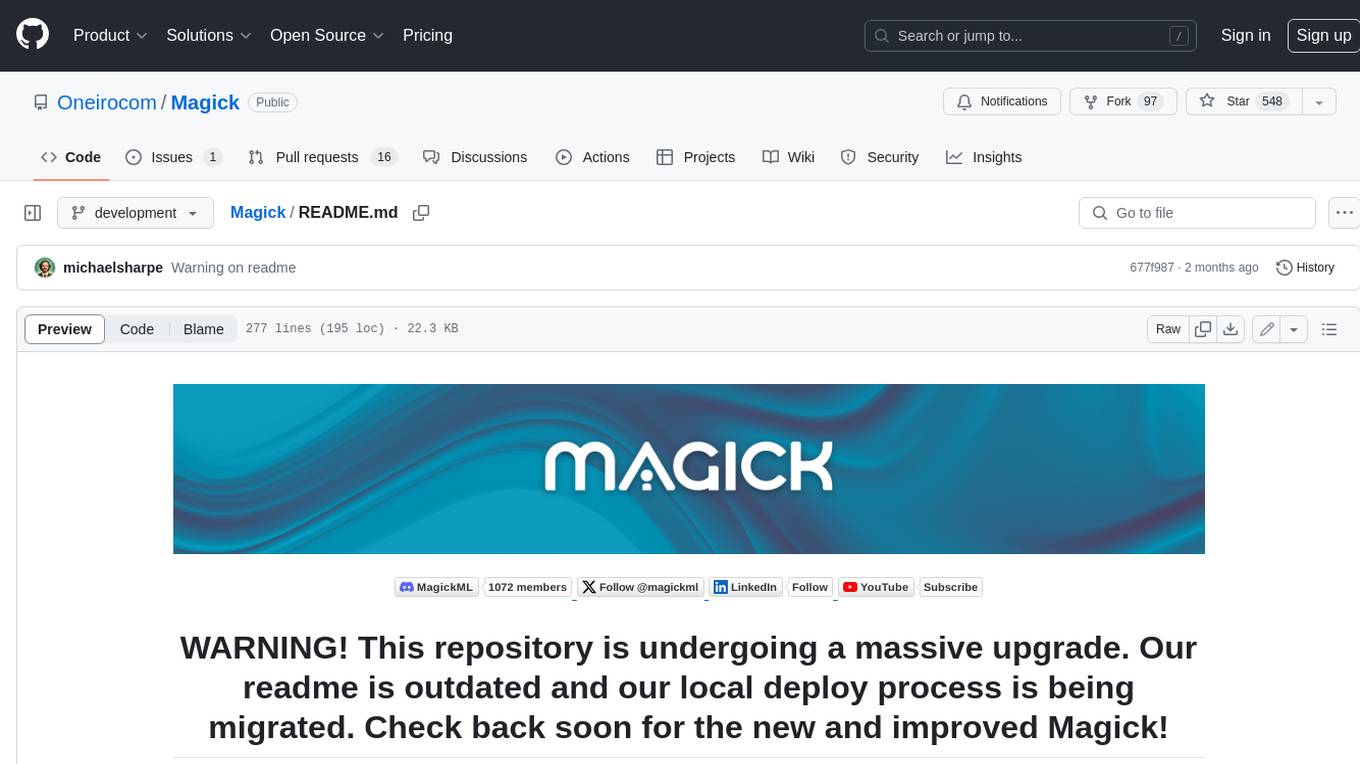
Magick
Magick is a groundbreaking visual AIDE (Artificial Intelligence Development Environment) for no-code data pipelines and multimodal agents. Magick can connect to other services and comes with nodes and templates well-suited for intelligent agents, chatbots, complex reasoning systems and realistic characters.
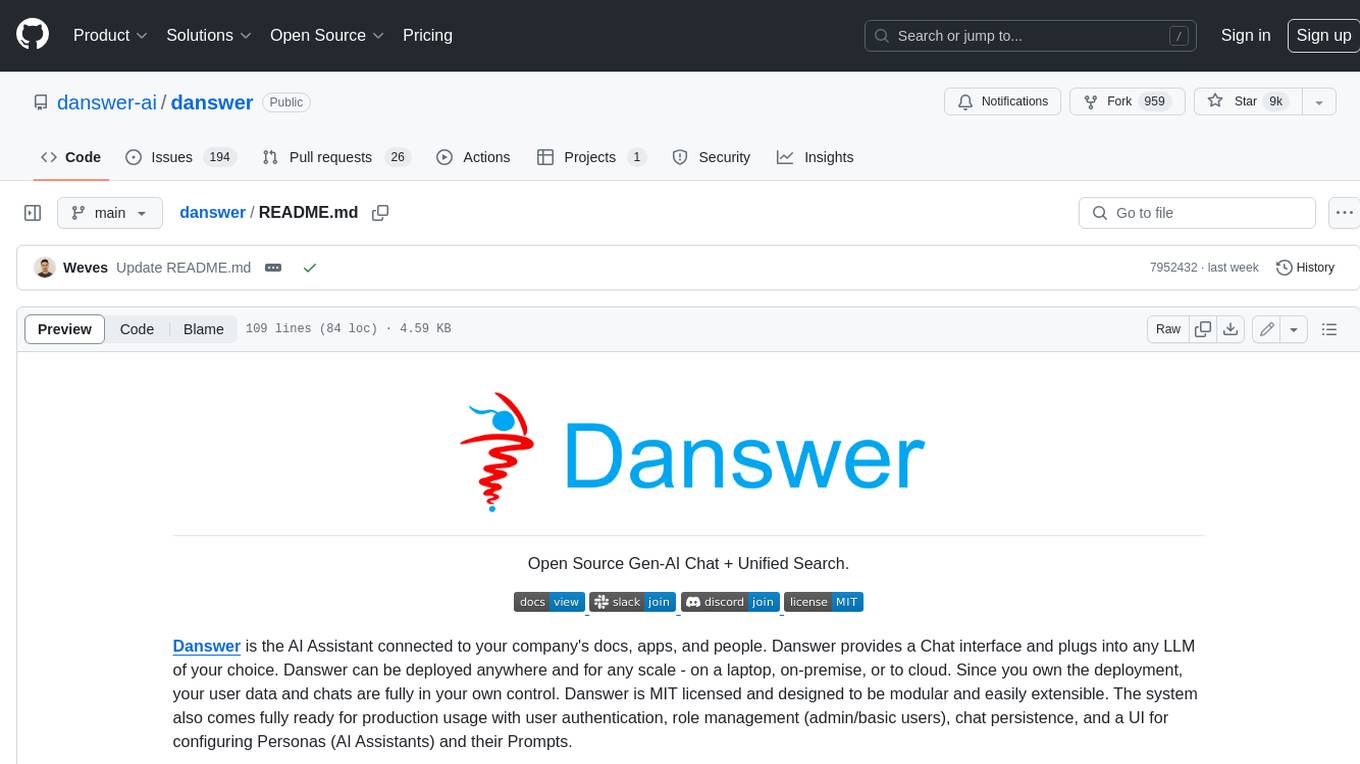
danswer
Danswer is an open-source Gen-AI Chat and Unified Search tool that connects to your company's docs, apps, and people. It provides a Chat interface and plugs into any LLM of your choice. Danswer can be deployed anywhere and for any scale - on a laptop, on-premise, or to cloud. Since you own the deployment, your user data and chats are fully in your own control. Danswer is MIT licensed and designed to be modular and easily extensible. The system also comes fully ready for production usage with user authentication, role management (admin/basic users), chat persistence, and a UI for configuring Personas (AI Assistants) and their Prompts. Danswer also serves as a Unified Search across all common workplace tools such as Slack, Google Drive, Confluence, etc. By combining LLMs and team specific knowledge, Danswer becomes a subject matter expert for the team. Imagine ChatGPT if it had access to your team's unique knowledge! It enables questions such as "A customer wants feature X, is this already supported?" or "Where's the pull request for feature Y?"
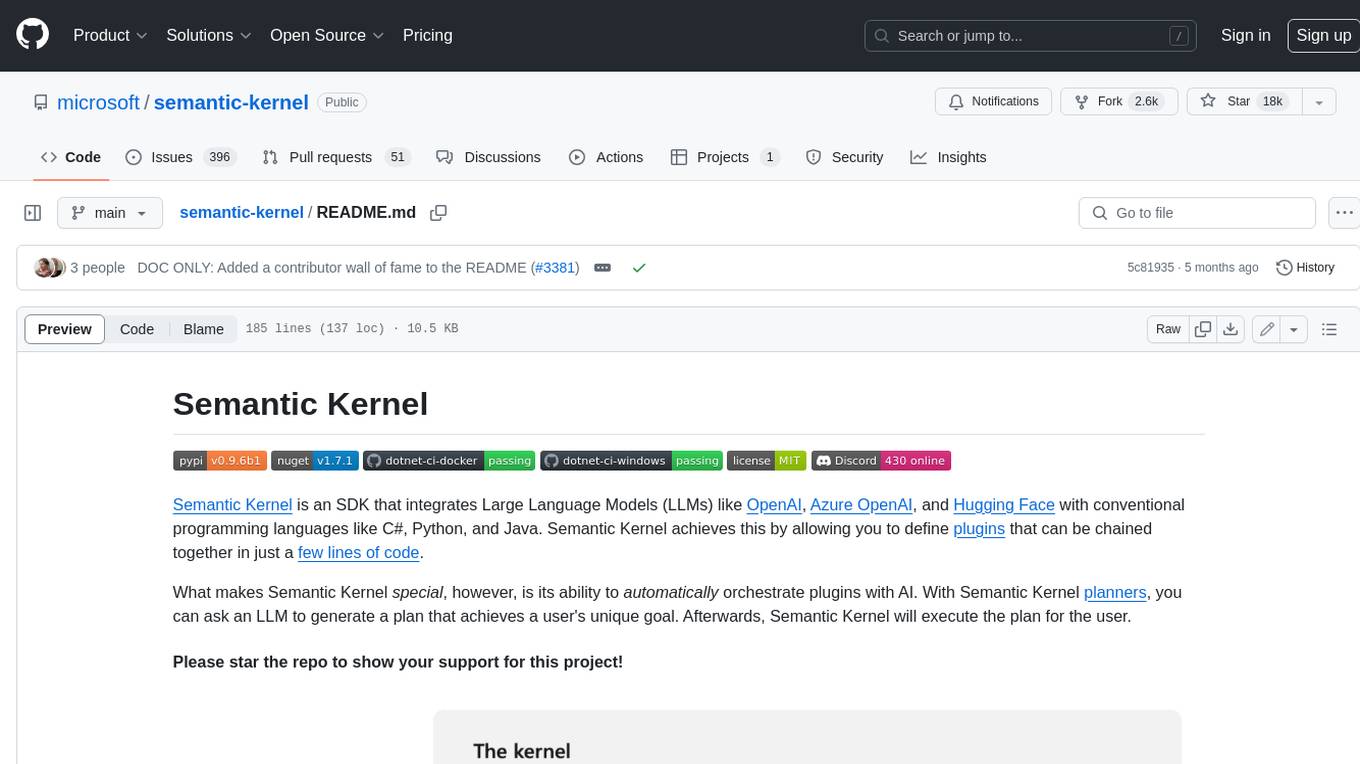
semantic-kernel
Semantic Kernel is an SDK that integrates Large Language Models (LLMs) like OpenAI, Azure OpenAI, and Hugging Face with conventional programming languages like C#, Python, and Java. Semantic Kernel achieves this by allowing you to define plugins that can be chained together in just a few lines of code. What makes Semantic Kernel _special_ , however, is its ability to _automatically_ orchestrate plugins with AI. With Semantic Kernel planners, you can ask an LLM to generate a plan that achieves a user's unique goal. Afterwards, Semantic Kernel will execute the plan for the user.
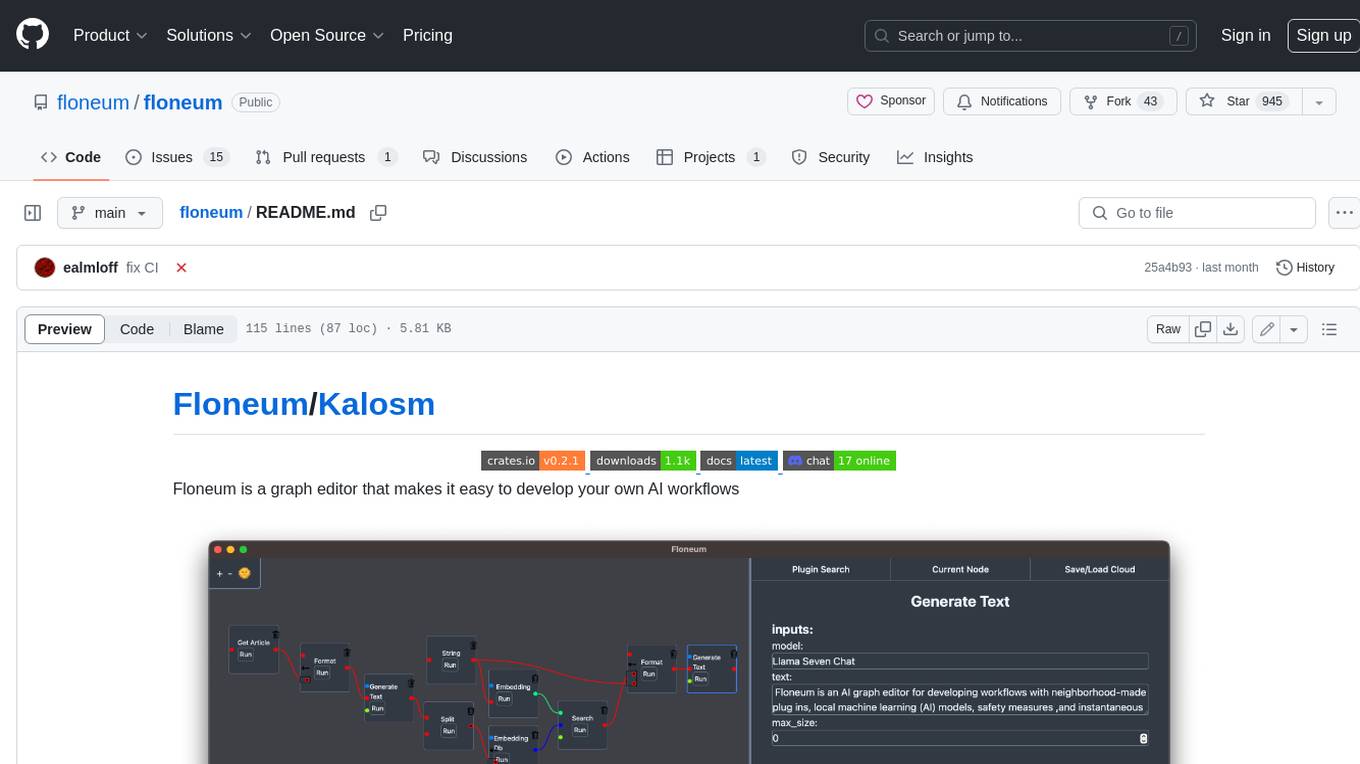
floneum
Floneum is a graph editor that makes it easy to develop your own AI workflows. It uses large language models (LLMs) to run AI models locally, without any external dependencies or even a GPU. This makes it easy to use LLMs with your own data, without worrying about privacy. Floneum also has a plugin system that allows you to improve the performance of LLMs and make them work better for your specific use case. Plugins can be used in any language that supports web assembly, and they can control the output of LLMs with a process similar to JSONformer or guidance.
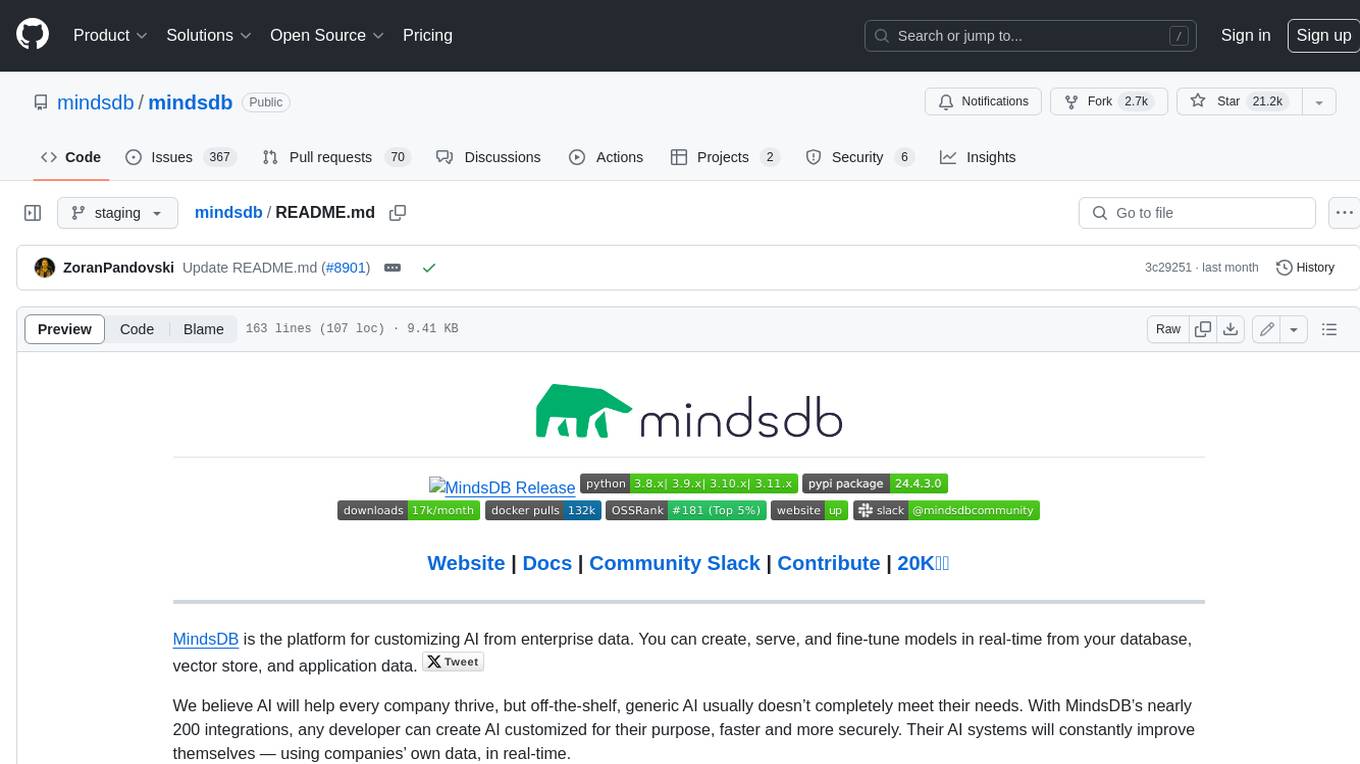
mindsdb
MindsDB is a platform for customizing AI from enterprise data. You can create, serve, and fine-tune models in real-time from your database, vector store, and application data. MindsDB "enhances" SQL syntax with AI capabilities to make it accessible for developers worldwide. With MindsDB’s nearly 200 integrations, any developer can create AI customized for their purpose, faster and more securely. Their AI systems will constantly improve themselves — using companies’ own data, in real-time.
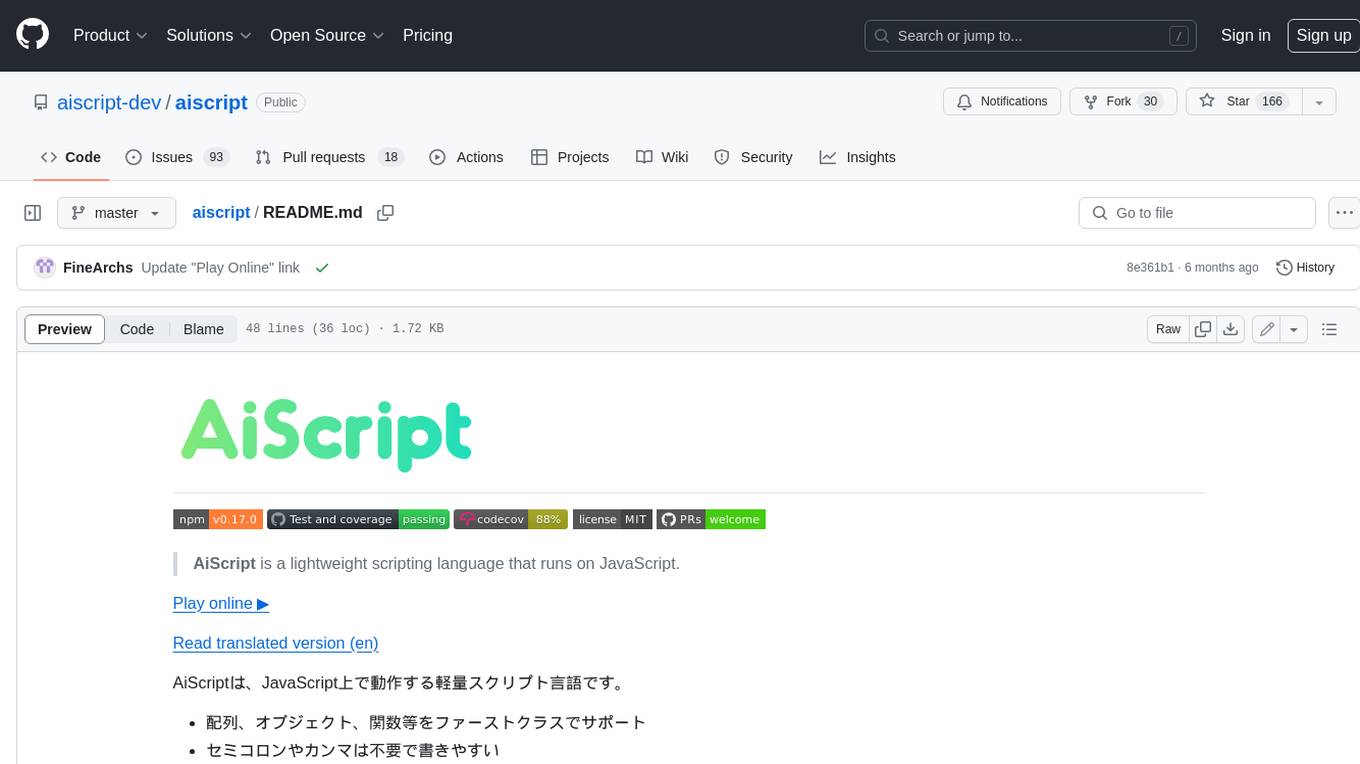
aiscript
AiScript is a lightweight scripting language that runs on JavaScript. It supports arrays, objects, and functions as first-class citizens, and is easy to write without the need for semicolons or commas. AiScript runs in a secure sandbox environment, preventing infinite loops from freezing the host. It also allows for easy provision of variables and functions from the host.
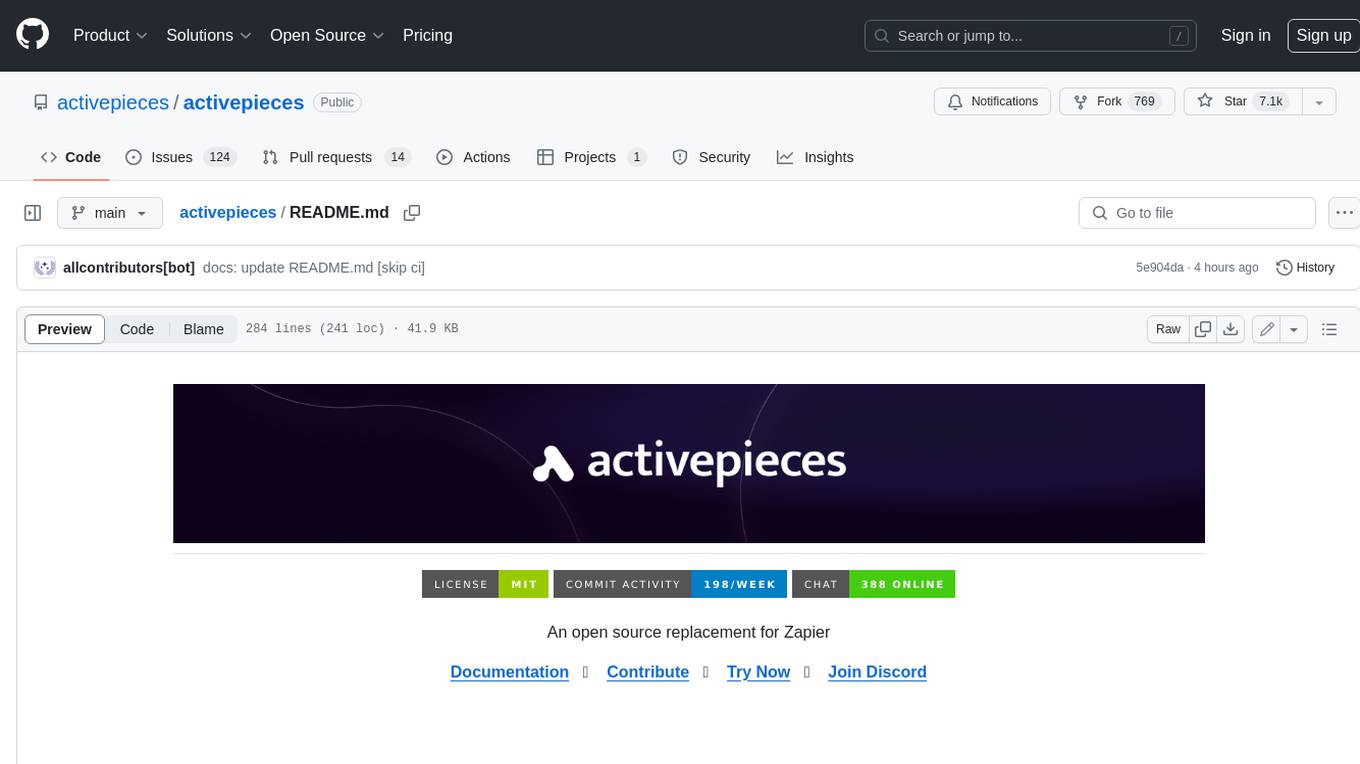
activepieces
Activepieces is an open source replacement for Zapier, designed to be extensible through a type-safe pieces framework written in Typescript. It features a user-friendly Workflow Builder with support for Branches, Loops, and Drag and Drop. Activepieces integrates with Google Sheets, OpenAI, Discord, and RSS, along with 80+ other integrations. The list of supported integrations continues to grow rapidly, thanks to valuable contributions from the community. Activepieces is an open ecosystem; all piece source code is available in the repository, and they are versioned and published directly to npmjs.com upon contributions. If you cannot find a specific piece on the pieces roadmap, please submit a request by visiting the following link: Request Piece Alternatively, if you are a developer, you can quickly build your own piece using our TypeScript framework. For guidance, please refer to the following guide: Contributor's Guide
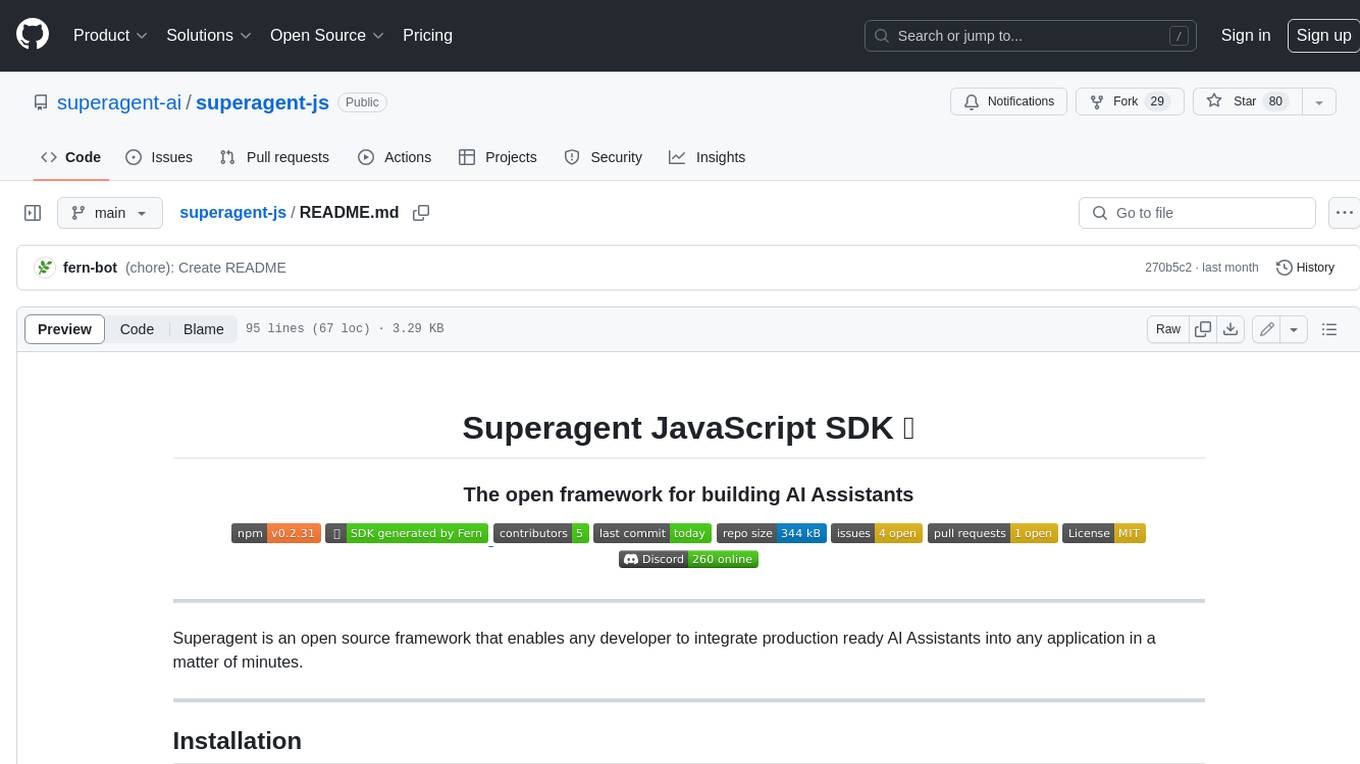
superagent-js
Superagent is an open source framework that enables any developer to integrate production ready AI Assistants into any application in a matter of minutes.
For similar jobs
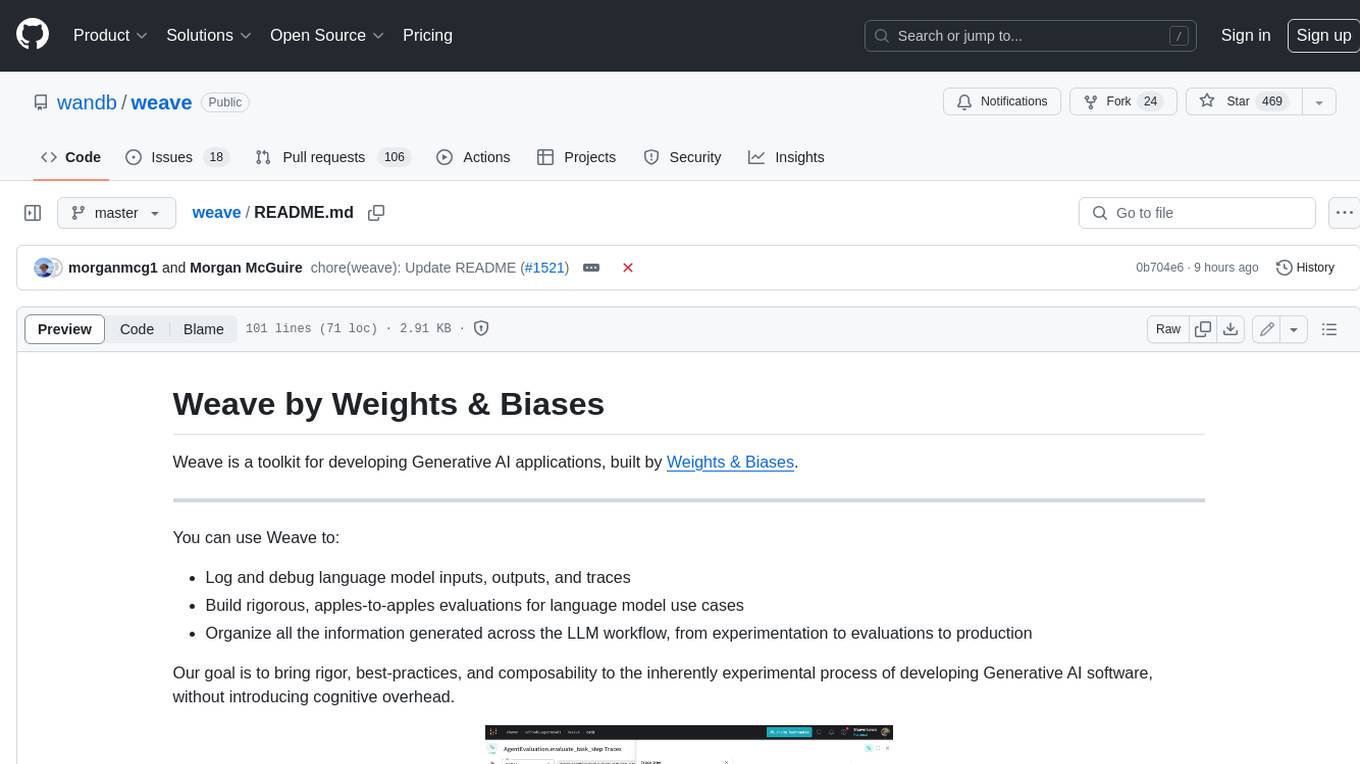
weave
Weave is a toolkit for developing Generative AI applications, built by Weights & Biases. With Weave, you can log and debug language model inputs, outputs, and traces; build rigorous, apples-to-apples evaluations for language model use cases; and organize all the information generated across the LLM workflow, from experimentation to evaluations to production. Weave aims to bring rigor, best-practices, and composability to the inherently experimental process of developing Generative AI software, without introducing cognitive overhead.
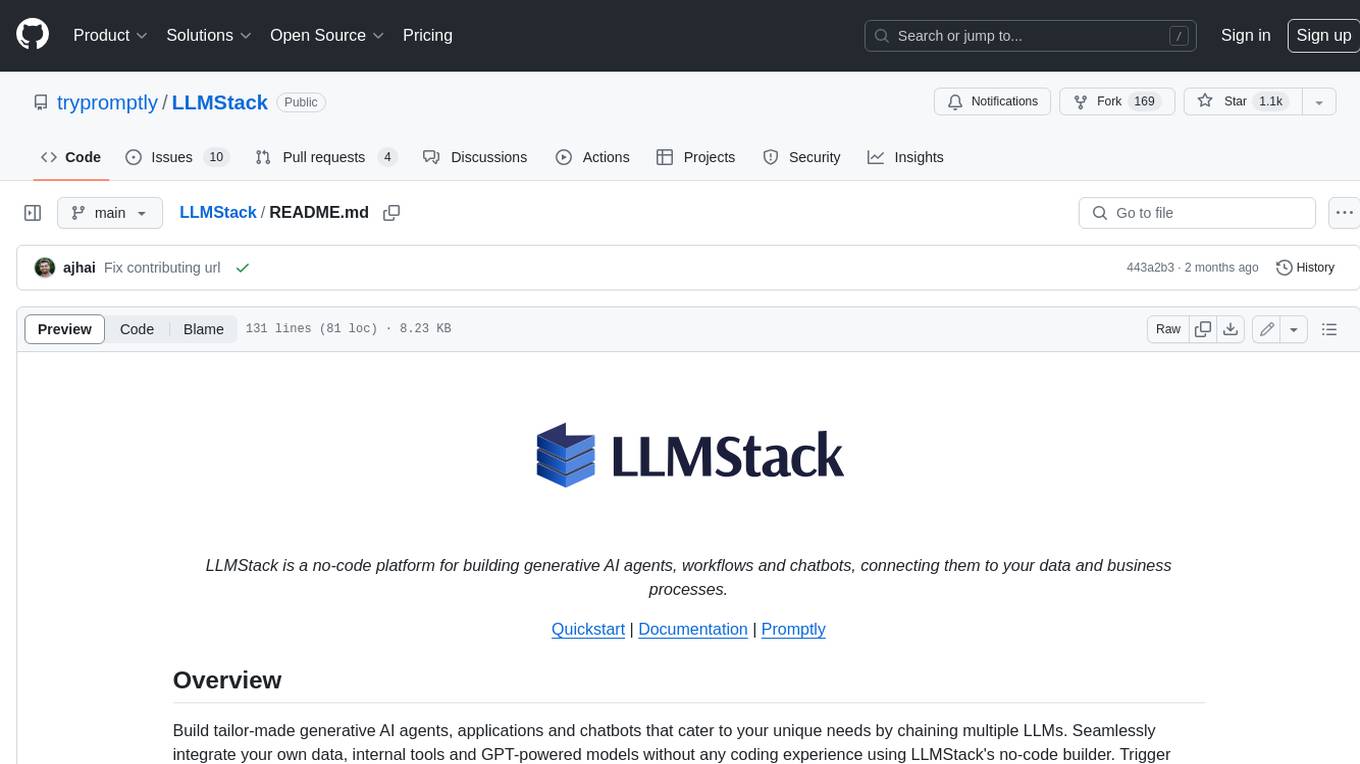
LLMStack
LLMStack is a no-code platform for building generative AI agents, workflows, and chatbots. It allows users to connect their own data, internal tools, and GPT-powered models without any coding experience. LLMStack can be deployed to the cloud or on-premise and can be accessed via HTTP API or triggered from Slack or Discord.
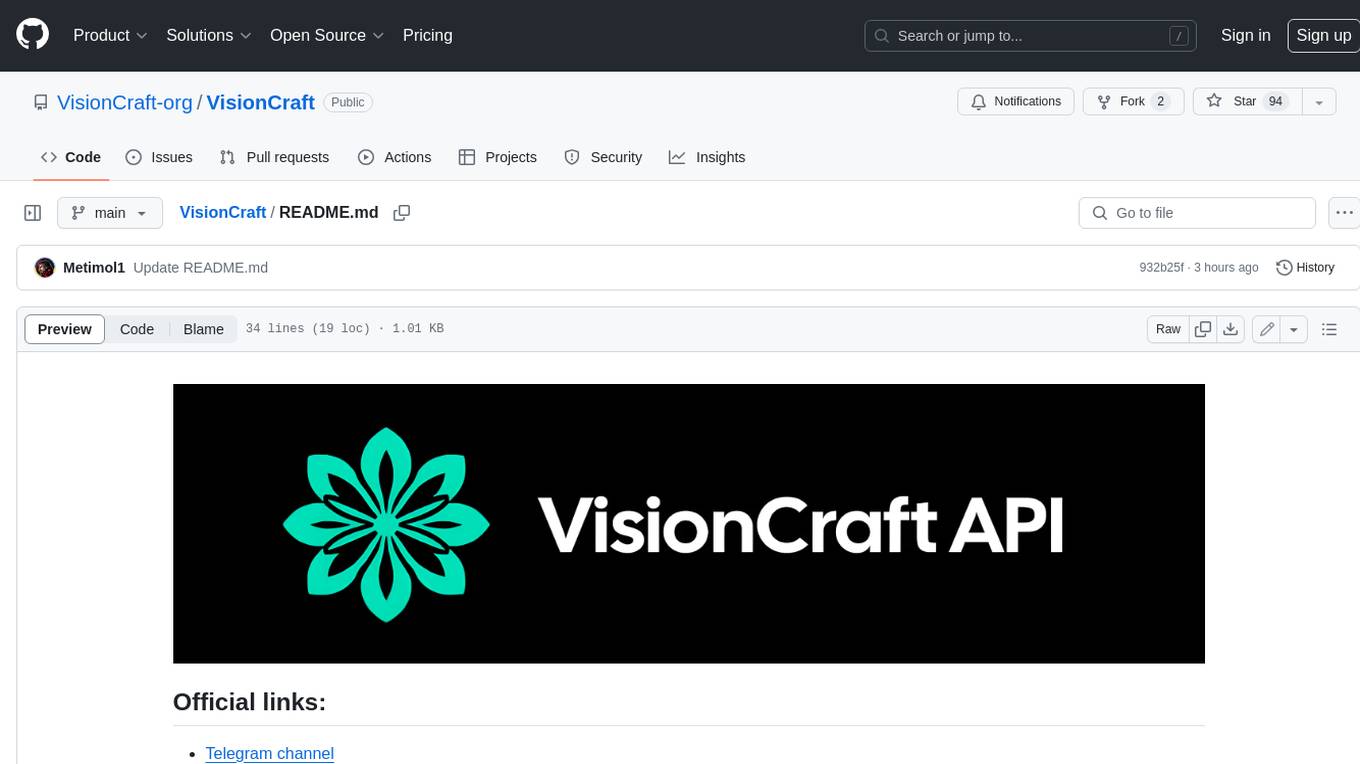
VisionCraft
The VisionCraft API is a free API for using over 100 different AI models. From images to sound.
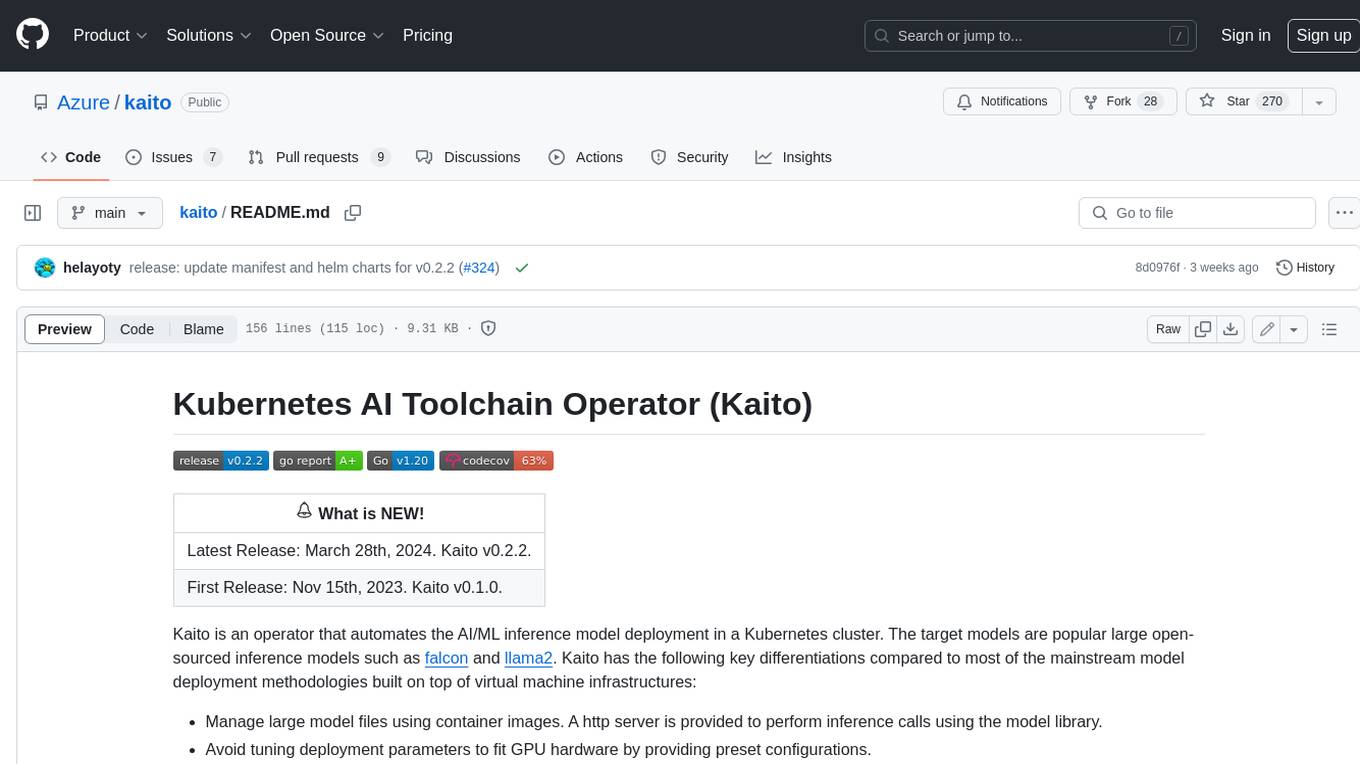
kaito
Kaito is an operator that automates the AI/ML inference model deployment in a Kubernetes cluster. It manages large model files using container images, avoids tuning deployment parameters to fit GPU hardware by providing preset configurations, auto-provisions GPU nodes based on model requirements, and hosts large model images in the public Microsoft Container Registry (MCR) if the license allows. Using Kaito, the workflow of onboarding large AI inference models in Kubernetes is largely simplified.
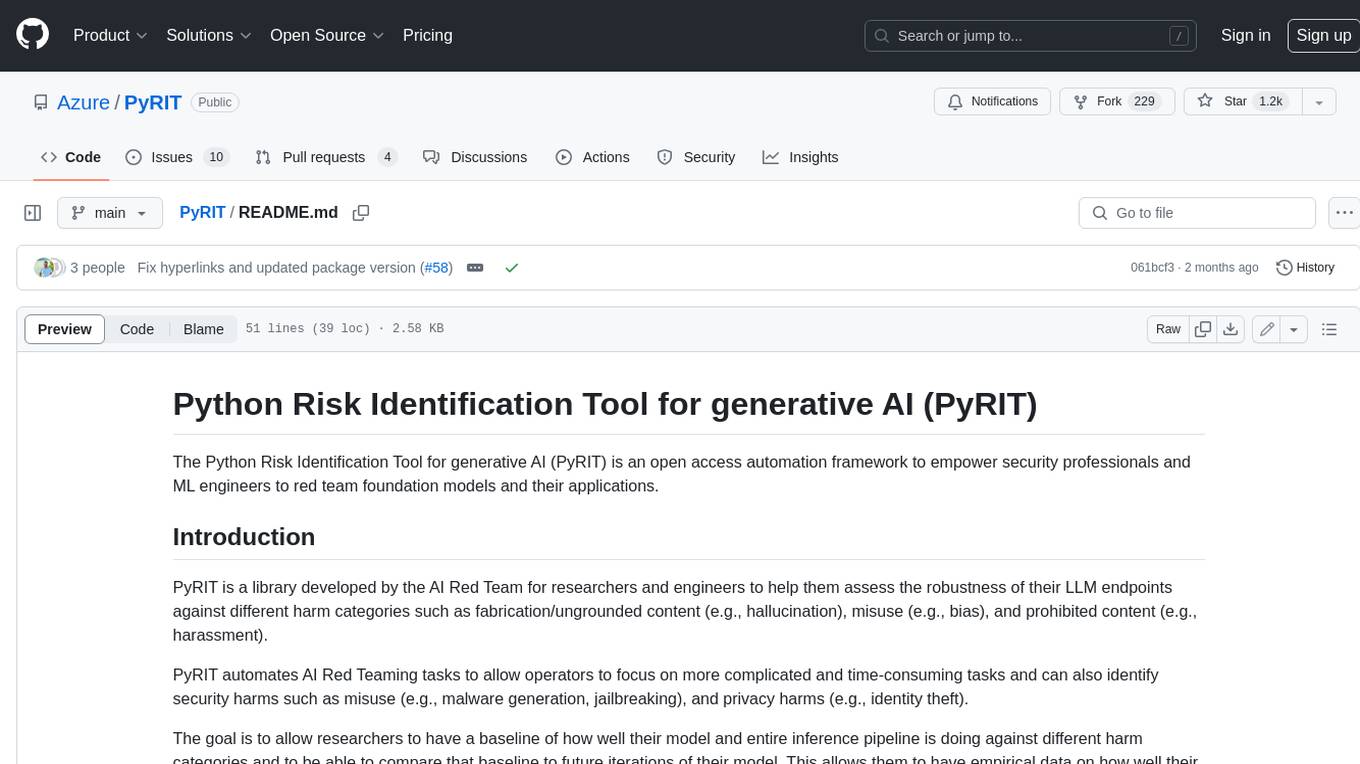
PyRIT
PyRIT is an open access automation framework designed to empower security professionals and ML engineers to red team foundation models and their applications. It automates AI Red Teaming tasks to allow operators to focus on more complicated and time-consuming tasks and can also identify security harms such as misuse (e.g., malware generation, jailbreaking), and privacy harms (e.g., identity theft). The goal is to allow researchers to have a baseline of how well their model and entire inference pipeline is doing against different harm categories and to be able to compare that baseline to future iterations of their model. This allows them to have empirical data on how well their model is doing today, and detect any degradation of performance based on future improvements.
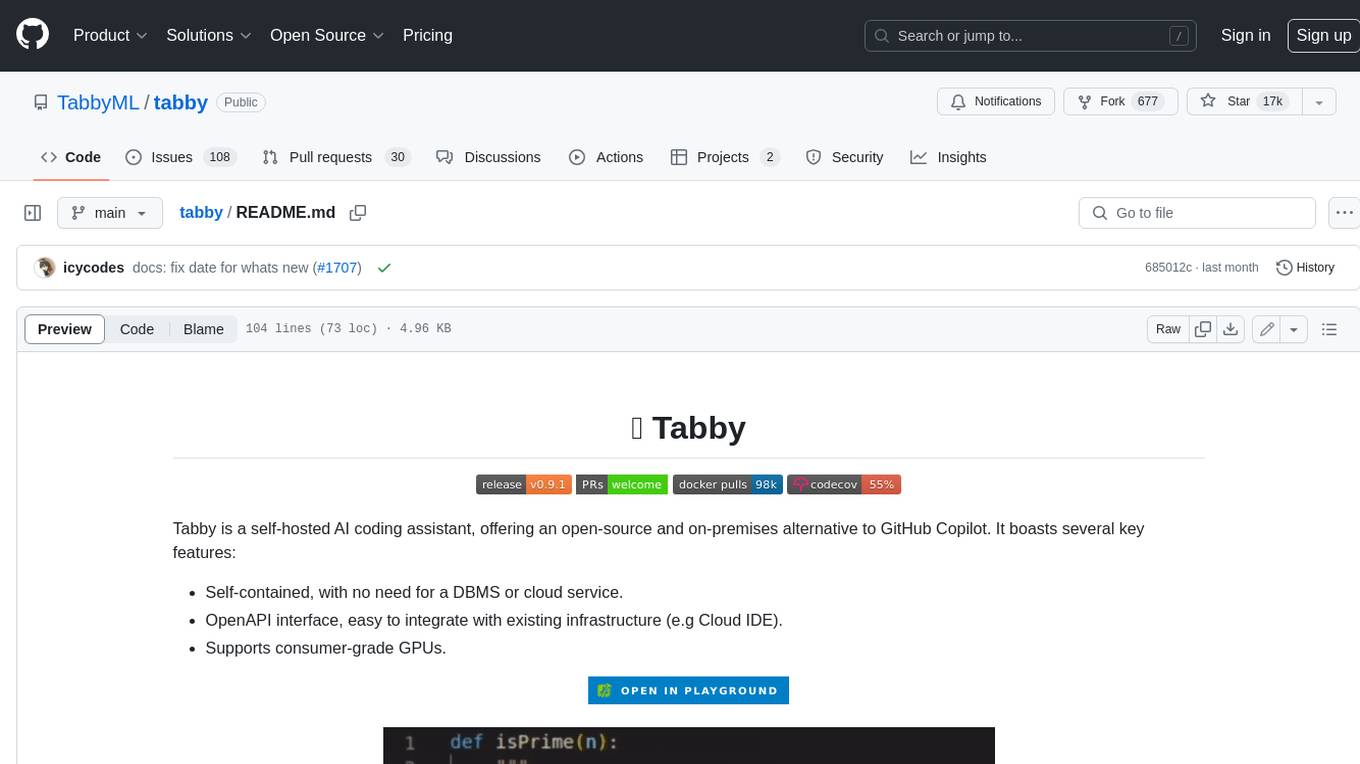
tabby
Tabby is a self-hosted AI coding assistant, offering an open-source and on-premises alternative to GitHub Copilot. It boasts several key features: * Self-contained, with no need for a DBMS or cloud service. * OpenAPI interface, easy to integrate with existing infrastructure (e.g Cloud IDE). * Supports consumer-grade GPUs.
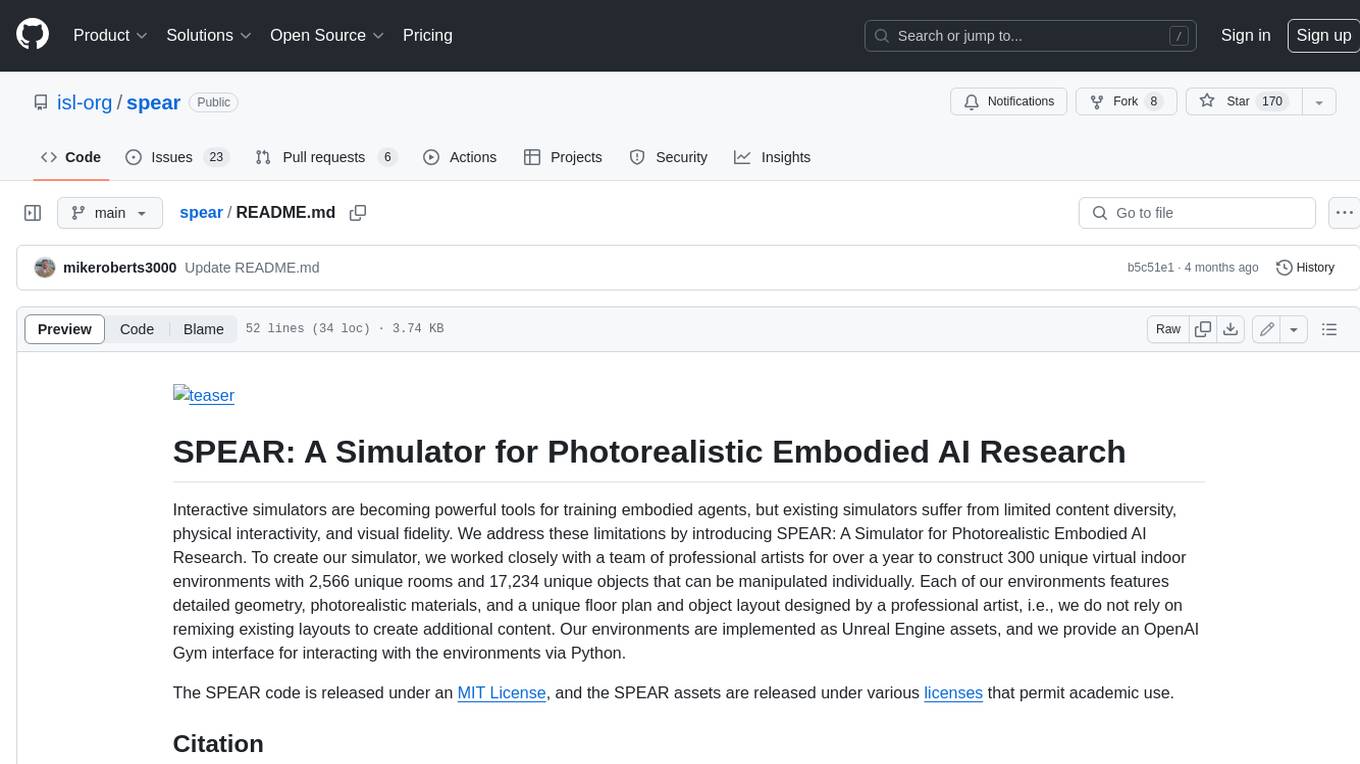
spear
SPEAR (Simulator for Photorealistic Embodied AI Research) is a powerful tool for training embodied agents. It features 300 unique virtual indoor environments with 2,566 unique rooms and 17,234 unique objects that can be manipulated individually. Each environment is designed by a professional artist and features detailed geometry, photorealistic materials, and a unique floor plan and object layout. SPEAR is implemented as Unreal Engine assets and provides an OpenAI Gym interface for interacting with the environments via Python.
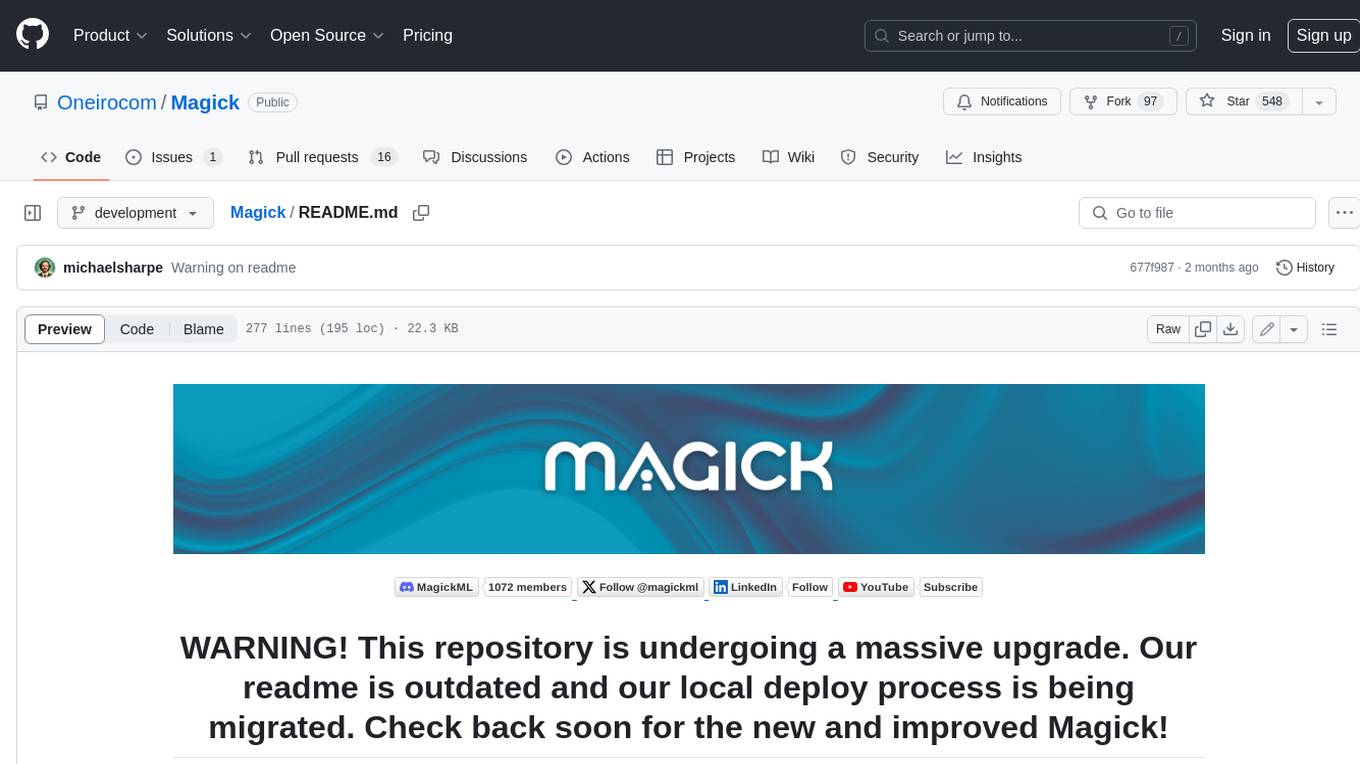
Magick
Magick is a groundbreaking visual AIDE (Artificial Intelligence Development Environment) for no-code data pipelines and multimodal agents. Magick can connect to other services and comes with nodes and templates well-suited for intelligent agents, chatbots, complex reasoning systems and realistic characters.