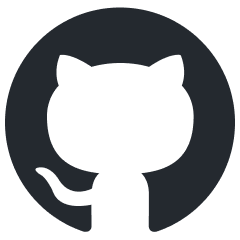
CustomSuggestionServiceForCopilotForXcode
Use locally run models (OpenAI compatible APIs, Tabby, Ollama) to generate code suggestions for Xcode.
Stars: 71
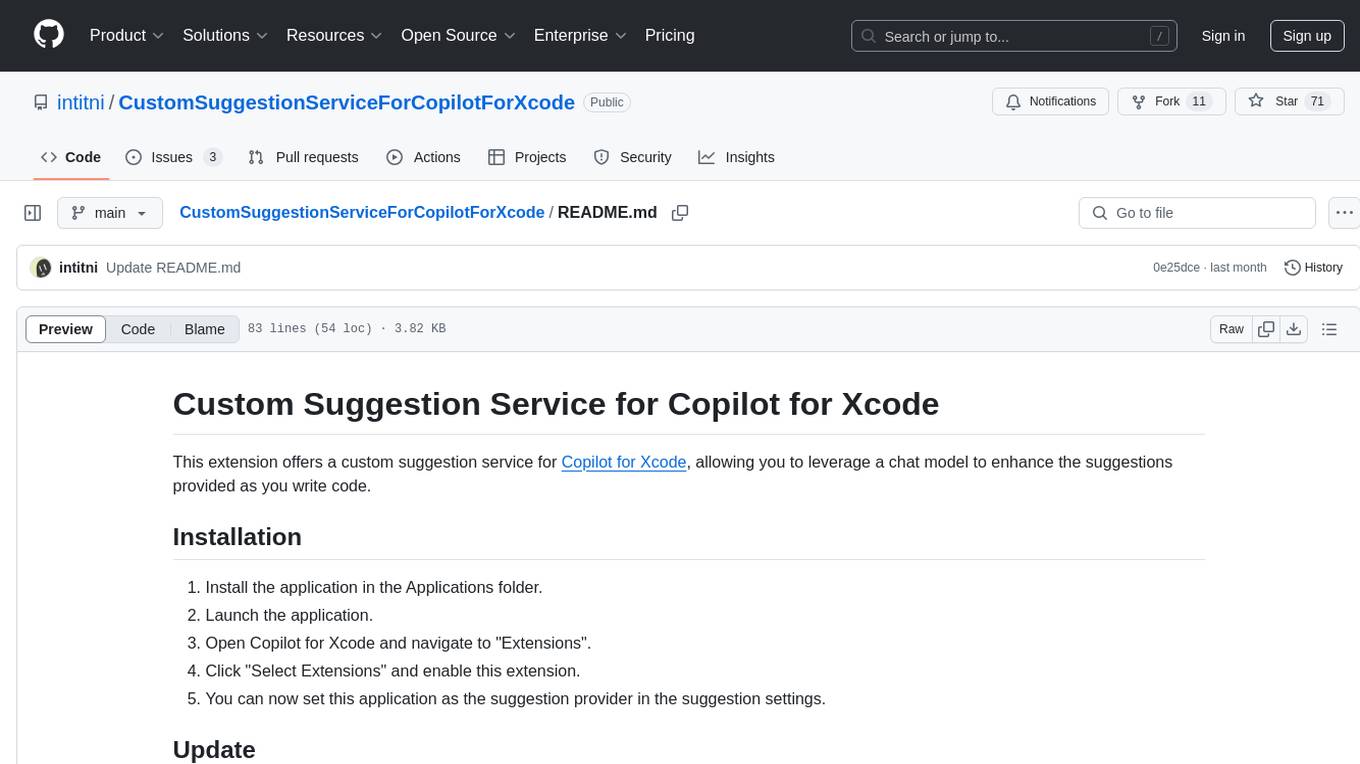
README:
This extension offers a custom suggestion service for Copilot for Xcode, allowing you to leverage a chat model to enhance the suggestions provided as you write code.
- Install the application in the Applications folder.
- Launch the application.
- Open Copilot for Xcode and navigate to "Extensions".
- Click "Select Extensions" and enable this extension.
- You can now set this application as the suggestion provider in the suggestion settings.
To update the app, you can do so directly within the app itself. Once updated, you should perform one of the following steps to ensure Copilot for Xcode recognizes the new version:
- Restart the
CopilotForXcodeExtensionService
. - Alternatively, terminate the "Custom Suggestion Service (CopilotForXcodeExtensionService)" process, open the extension manager in Copilot for Xcode, and click "Restart Extensions".
We are exploring better methods to tweak the update process.
The app supports three types of suggestion services:
- Models with chat completions API
- Models with completions API
- Models with FIM API
- Tabby
If you are new to running a model locally, you can try Ollama and LM Studio.
-
Use Tabby since they have extensive experience in code completion.
-
Use models with completions API with Fill-in-the-Middle support (for example, codellama:7b-code), and use the "Fill-in-the-Middle" strategy.
You can find some examples here.
-
Use models with FIM API.
When using custom models to generate suggestions, it is recommended to setup a lower suggestion limit for faster generation.
In other situations, it is advisable to use a custom model with the completions API over a chat completions API, and employ the default request strategy.
Ensure that the prompt format remains as simple as the following:
{System}
{User}
{Assistant}
The template format differs in different tools.
- Fill-in-the-Middle: It uses special tokens to guide the models to generate suggestions. The models need to support FIM to use it (codellama:xb-code, startcoder, etc.). You need to setup a prompt format to allow it to work properly. The default prompt format is for codellama.
- Fill-in-the-Middle with System Prompt: The previous one doesn't have a system prompt telling it what to do. You can try to use it in models that don't support FIM.
- Anthropic Optimized: This strategy is optimized for Anthropic chat models. You can try it on other chat models, too.
- Default: This strategy meticulously explains the context to the model, prompting it to generate a suggestion.
- Naive: This strategy rearranges the code in a naive way to trick the model into believing it's appending code at the end of a file.
- Continue: This strategy employs the "Please Continue" technique to persuade the model that it has started a suggestion and must continue to complete it. (Only effective with the chat completion API).
- Load
qwen2.5-coder:32b
with Ollama. - Setup the model with the completions API.
- Set the request strategy to "Fill-in-the-Middle".
- Set prompt format to
<|fim_prefix|>{prefix}<|fim_suffix|>{suffix}<|fim_middle|>
and turn on Raw Prompt.
Prompt engineering is a challenging task, and your assistance is invaluable.
The most complex things are located within the Core
package.
- To add a new service, please refer to the
CodeCompletionService
folder. - To add new request strategies, check out the
SuggestionService
folder. - To add a new instructions of using a local model, update the
Example Use of Local Models
in the README.md.
For Tasks:
Click tags to check more tools for each tasksFor Jobs:
Alternative AI tools for CustomSuggestionServiceForCopilotForXcode
Similar Open Source Tools
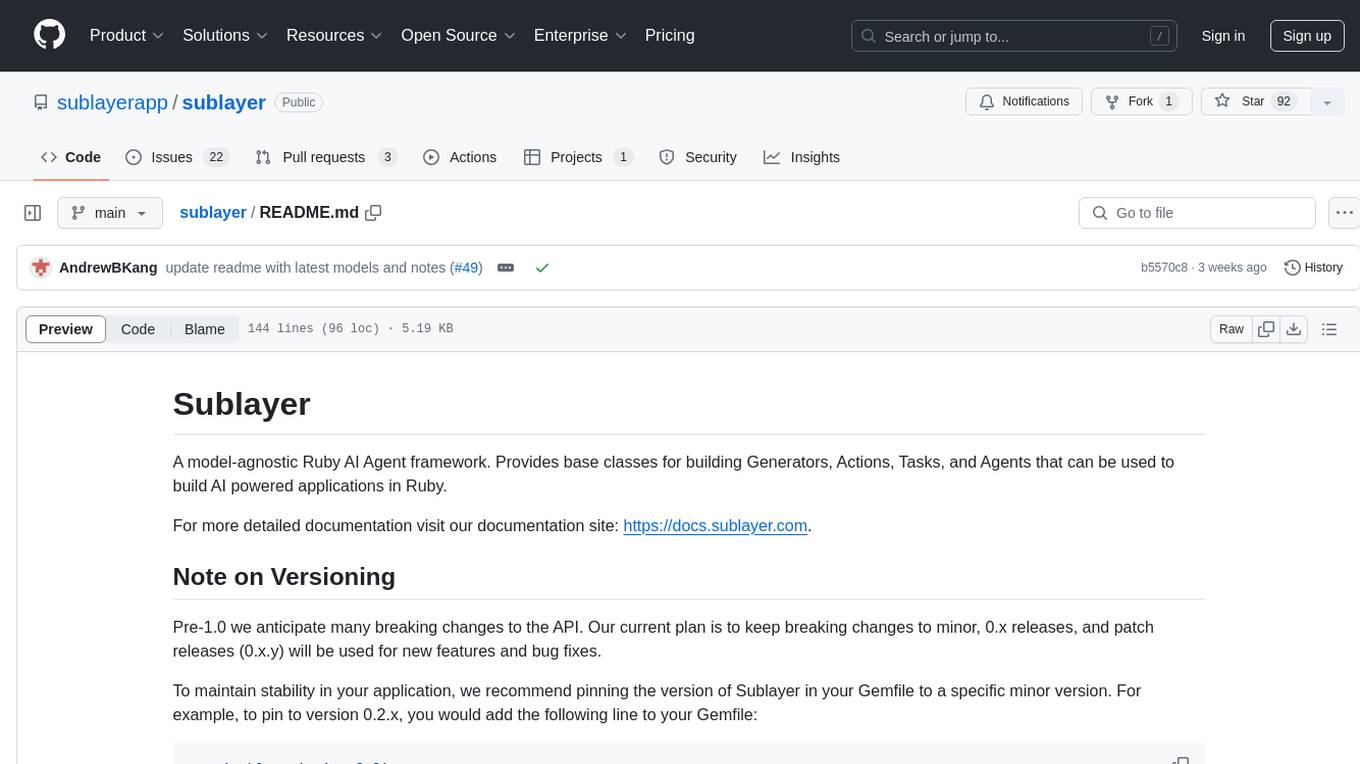
sublayer
Sublayer is a model-agnostic Ruby AI Agent framework that provides base classes for building Generators, Actions, Tasks, and Agents to create AI-powered applications in Ruby. It supports various AI models and providers, such as OpenAI, Gemini, and Claude. Generators generate specific outputs, Actions perform operations, Agents are autonomous entities for tasks or monitoring, and Triggers decide when Agents are activated. The framework offers sample Generators and usage examples for building AI applications.
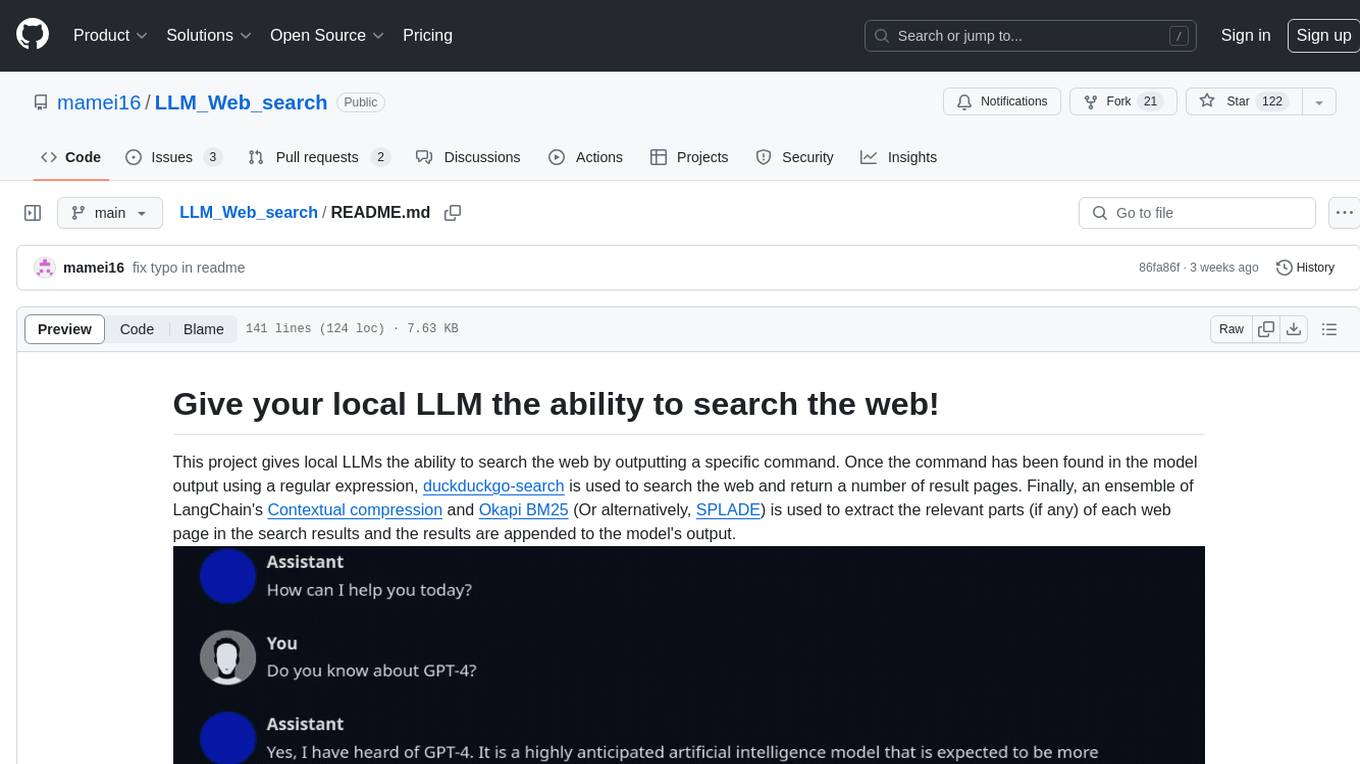
LLM_Web_search
LLM_Web_search project gives local LLMs the ability to search the web by outputting a specific command. It uses regular expressions to extract search queries from model output and then utilizes duckduckgo-search to search the web. LangChain's Contextual compression and Okapi BM25 or SPLADE are used to extract relevant parts of web pages in search results. The extracted results are appended to the model's output.
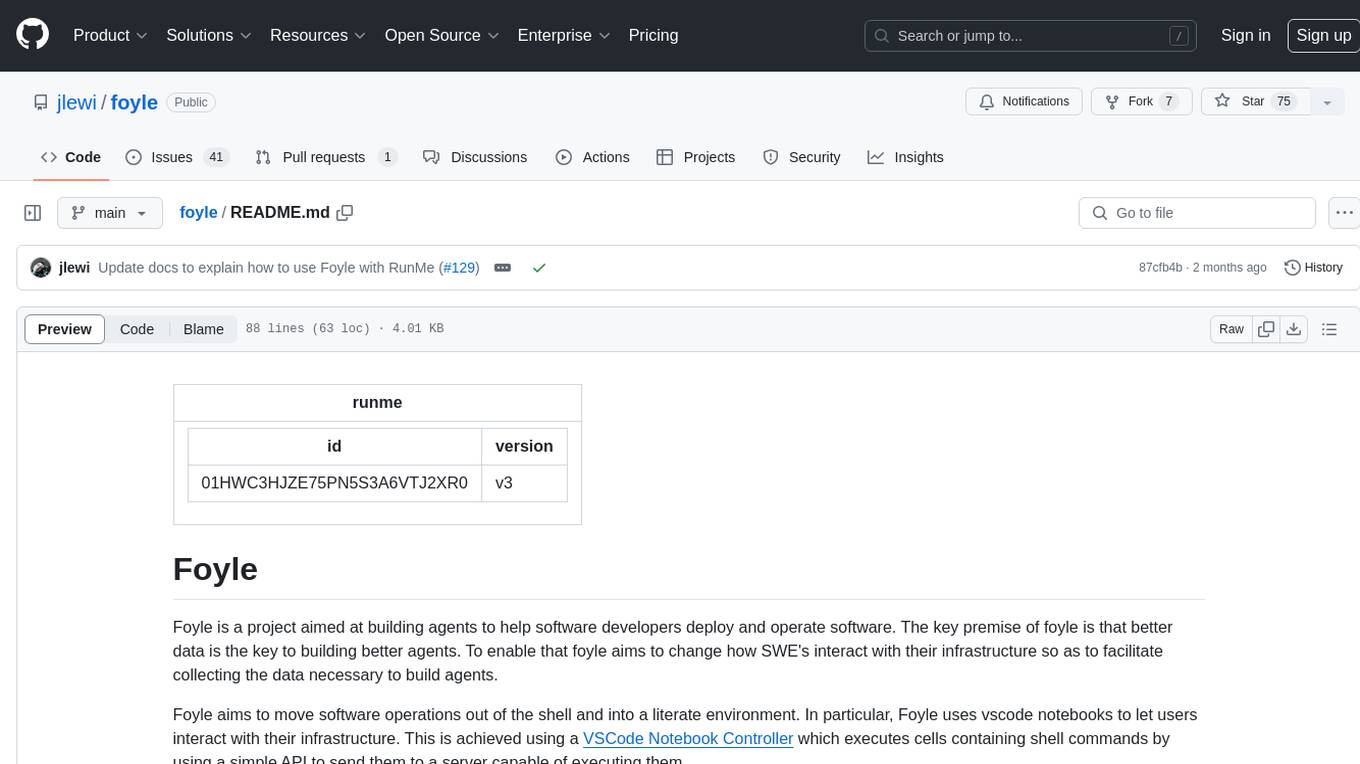
foyle
Foyle is a project focused on building agents to assist software developers in deploying and operating software. It aims to improve agent performance by collecting human feedback on agent suggestions and human examples of reasoning traces. Foyle utilizes a literate environment using vscode notebooks to interact with infrastructure, capturing prompts, AI-provided answers, and user corrections. The goal is to continuously retrain AI to enhance performance. Additionally, Foyle emphasizes the importance of reasoning traces for training agents to work with internal systems, providing a self-documenting process for operations and troubleshooting.
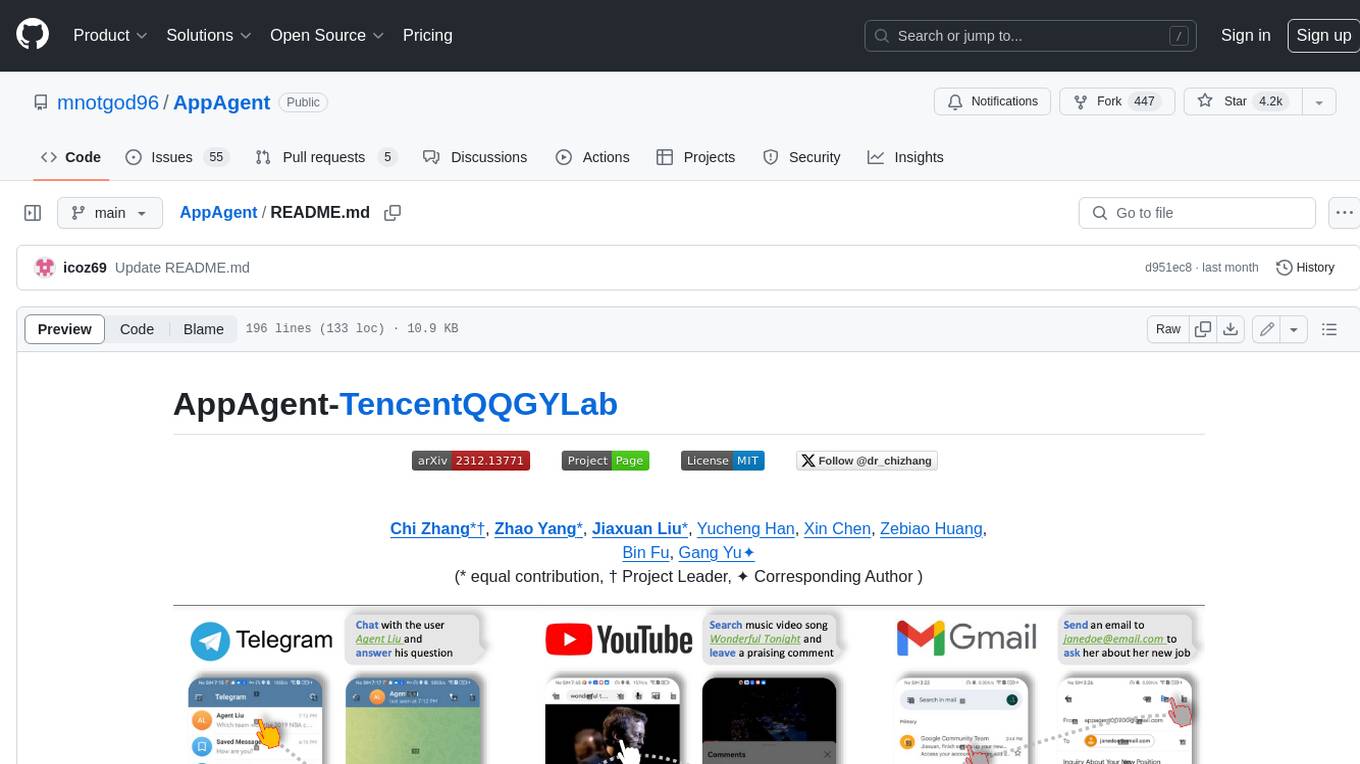
AppAgent
AppAgent is a novel LLM-based multimodal agent framework designed to operate smartphone applications. Our framework enables the agent to operate smartphone applications through a simplified action space, mimicking human-like interactions such as tapping and swiping. This novel approach bypasses the need for system back-end access, thereby broadening its applicability across diverse apps. Central to our agent's functionality is its innovative learning method. The agent learns to navigate and use new apps either through autonomous exploration or by observing human demonstrations. This process generates a knowledge base that the agent refers to for executing complex tasks across different applications.
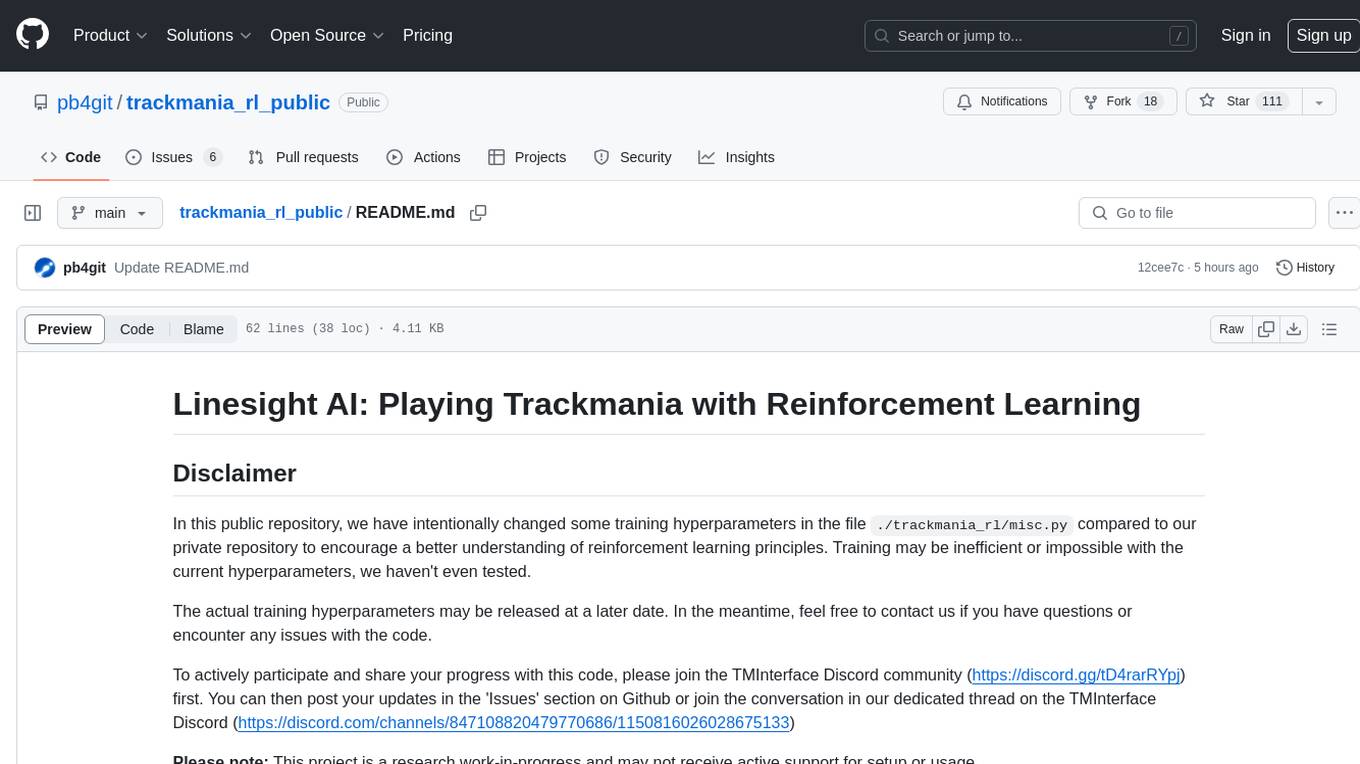
trackmania_rl_public
This repository contains the reinforcement learning training code for Trackmania AI with Reinforcement Learning. It is a research work-in-progress project that aims to apply reinforcement learning principles to play Trackmania. The code is constantly evolving and may not be clean or easily usable. The training hyperparameters are intentionally changed in the public repository to encourage understanding of reinforcement learning principles. The project may not receive active support for setup or usage at the moment.
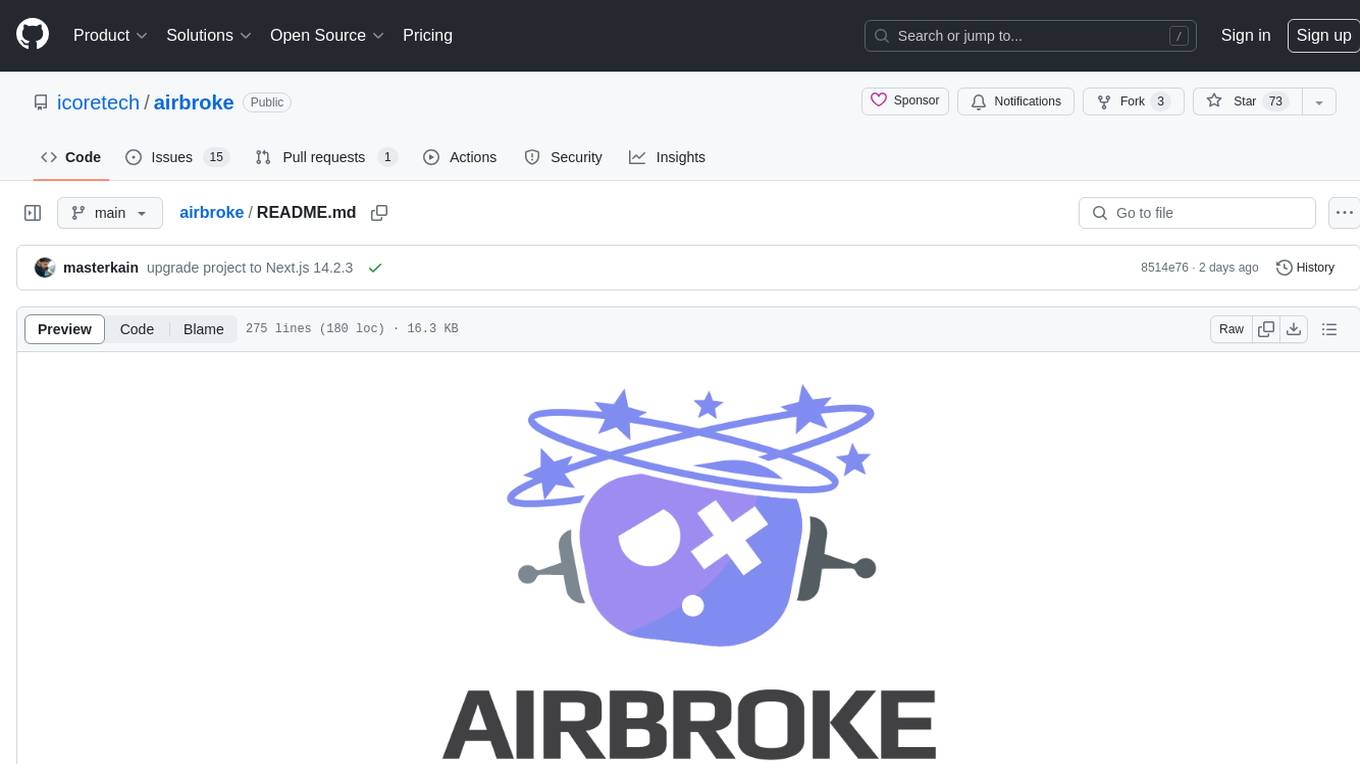
airbroke
Airbroke is an open-source error catcher tool designed for modern web applications. It provides a PostgreSQL-based backend with an Airbrake-compatible HTTP collector endpoint and a React-based frontend for error management. The tool focuses on simplicity, maintaining a small database footprint even under heavy data ingestion. Users can ask AI about issues, replay HTTP exceptions, and save/manage bookmarks for important occurrences. Airbroke supports multiple OAuth providers for secure user authentication and offers occurrence charts for better insights into error occurrences. The tool can be deployed in various ways, including building from source, using Docker images, deploying on Vercel, Render.com, Kubernetes with Helm, or Docker Compose. It requires Node.js, PostgreSQL, and specific system resources for deployment.
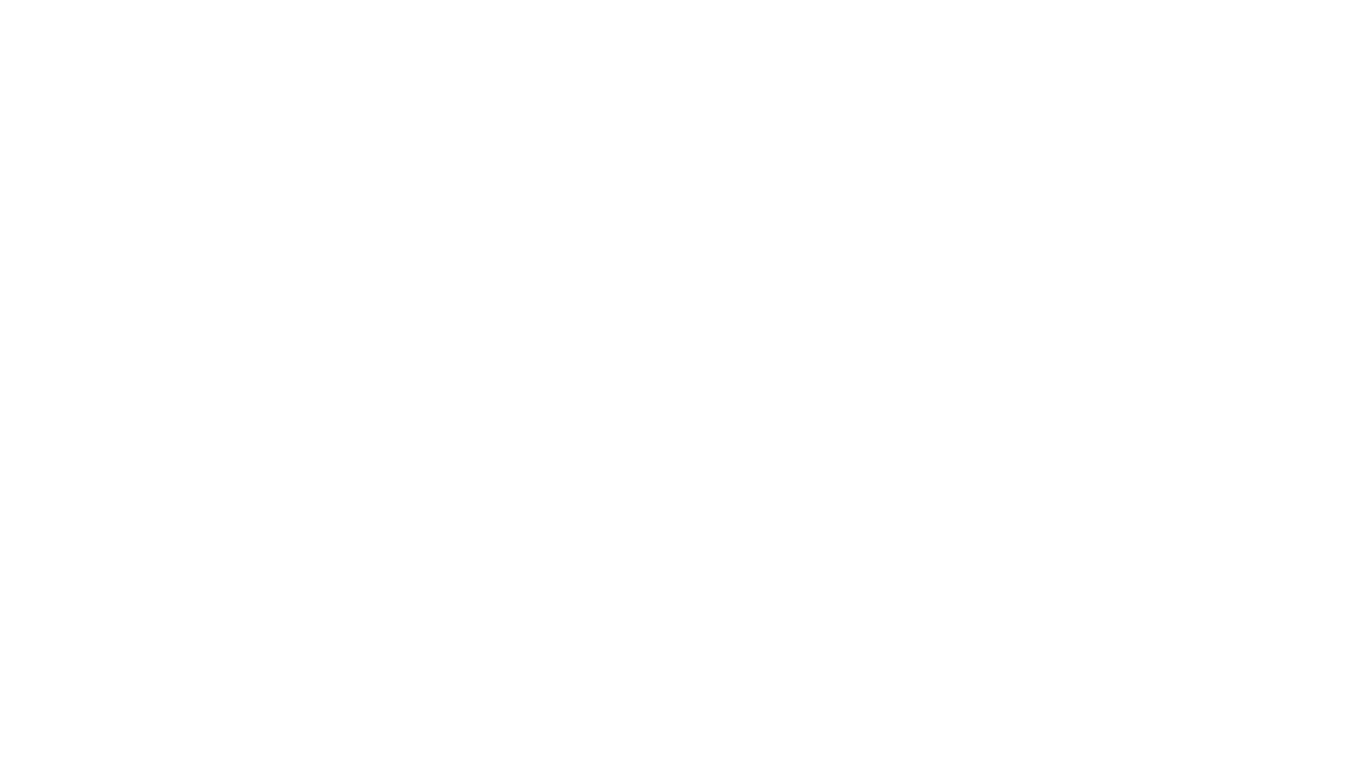
brokk
Brokk is a code assistant designed to understand code semantically, allowing LLMs to work effectively on large codebases. It offers features like agentic search, summarizing related classes, parsing stack traces, adding source for usages, and autonomously fixing errors. Users can interact with Brokk through different panels and commands, enabling them to manipulate context, ask questions, search codebase, run shell commands, and more. Brokk helps with tasks like debugging regressions, exploring codebase, AI-powered refactoring, and working with dependencies. It is particularly useful for making complex, multi-file edits with o1pro.
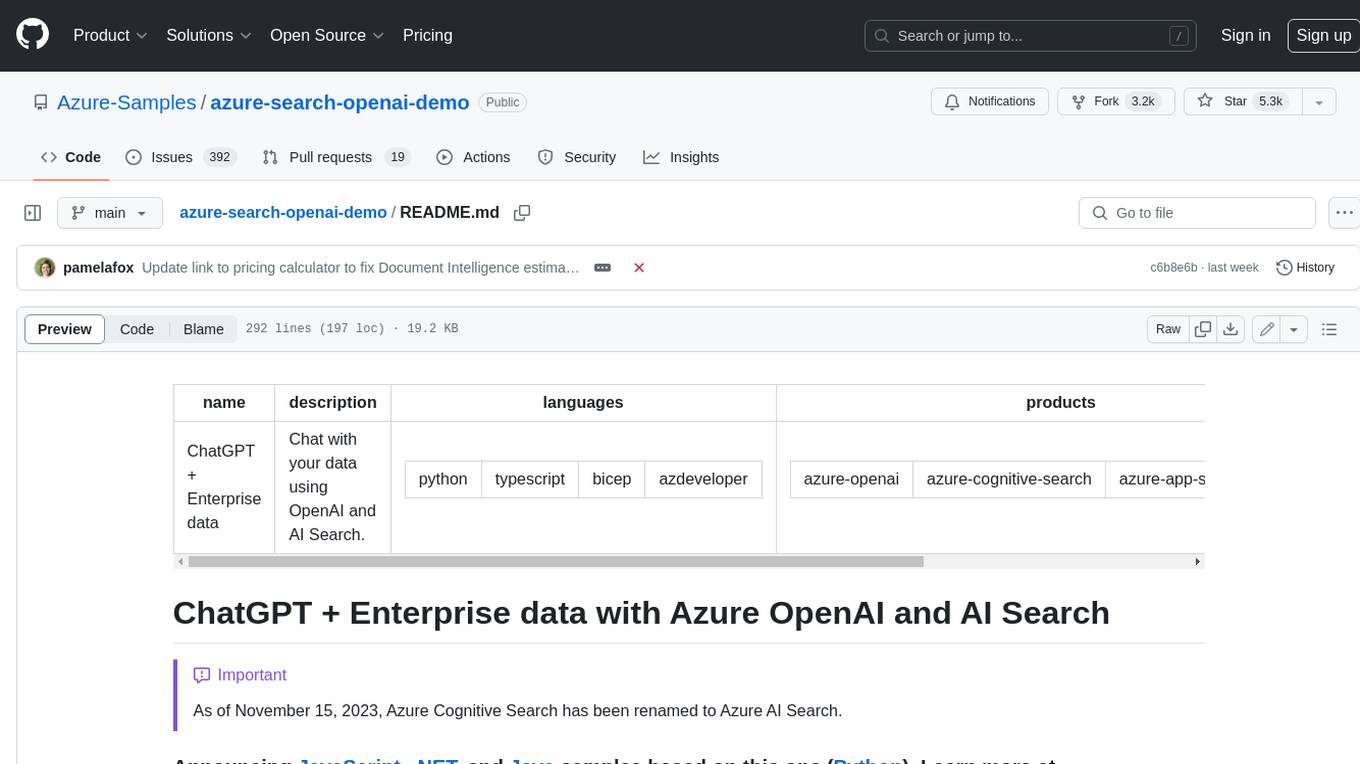
azure-search-openai-demo
This sample demonstrates a few approaches for creating ChatGPT-like experiences over your own data using the Retrieval Augmented Generation pattern. It uses Azure OpenAI Service to access a GPT model (gpt-35-turbo), and Azure AI Search for data indexing and retrieval. The repo includes sample data so it's ready to try end to end. In this sample application we use a fictitious company called Contoso Electronics, and the experience allows its employees to ask questions about the benefits, internal policies, as well as job descriptions and roles.
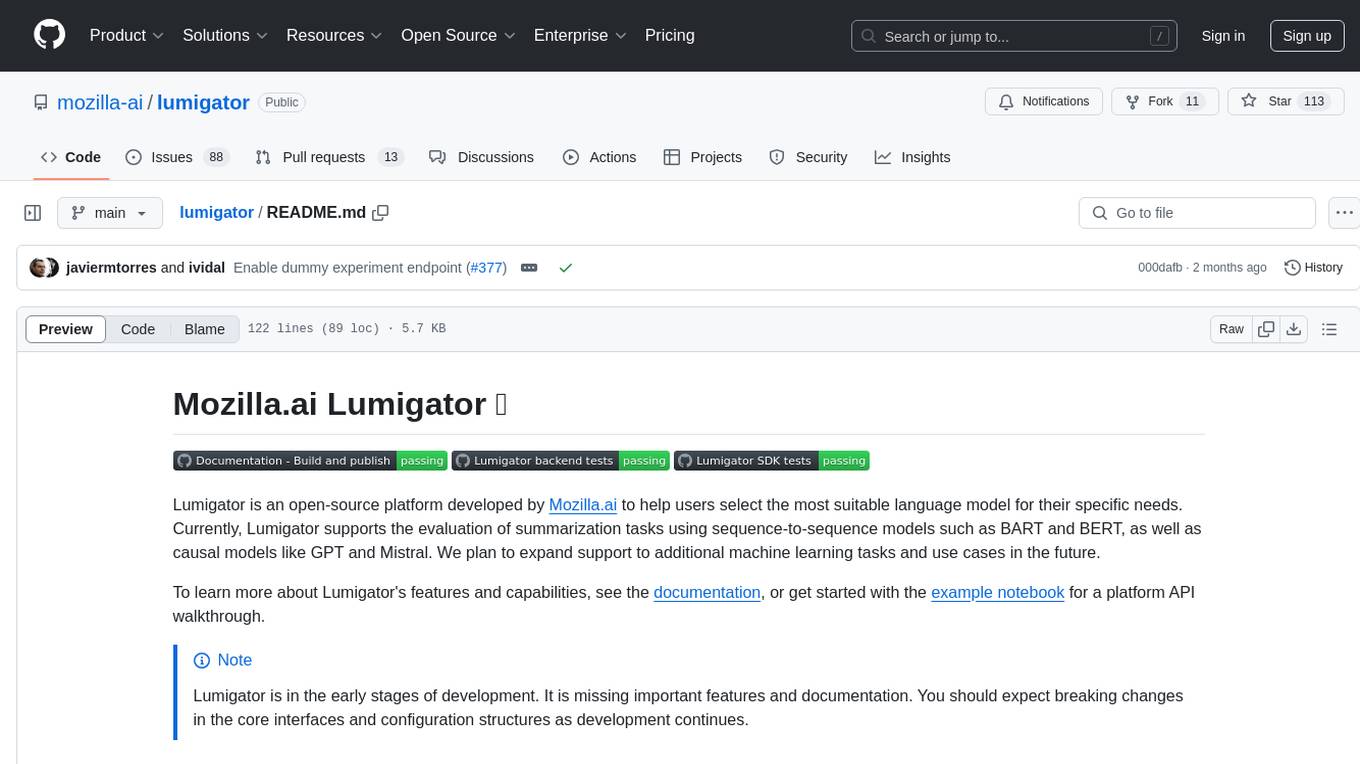
lumigator
Lumigator is an open-source platform developed by Mozilla.ai to help users select the most suitable language model for their specific needs. It supports the evaluation of summarization tasks using sequence-to-sequence models such as BART and BERT, as well as causal models like GPT and Mistral. The platform aims to make model selection transparent, efficient, and empowering by providing a framework for comparing LLMs using task-specific metrics to evaluate how well a model fits a project's needs. Lumigator is in the early stages of development and plans to expand support to additional machine learning tasks and use cases in the future.
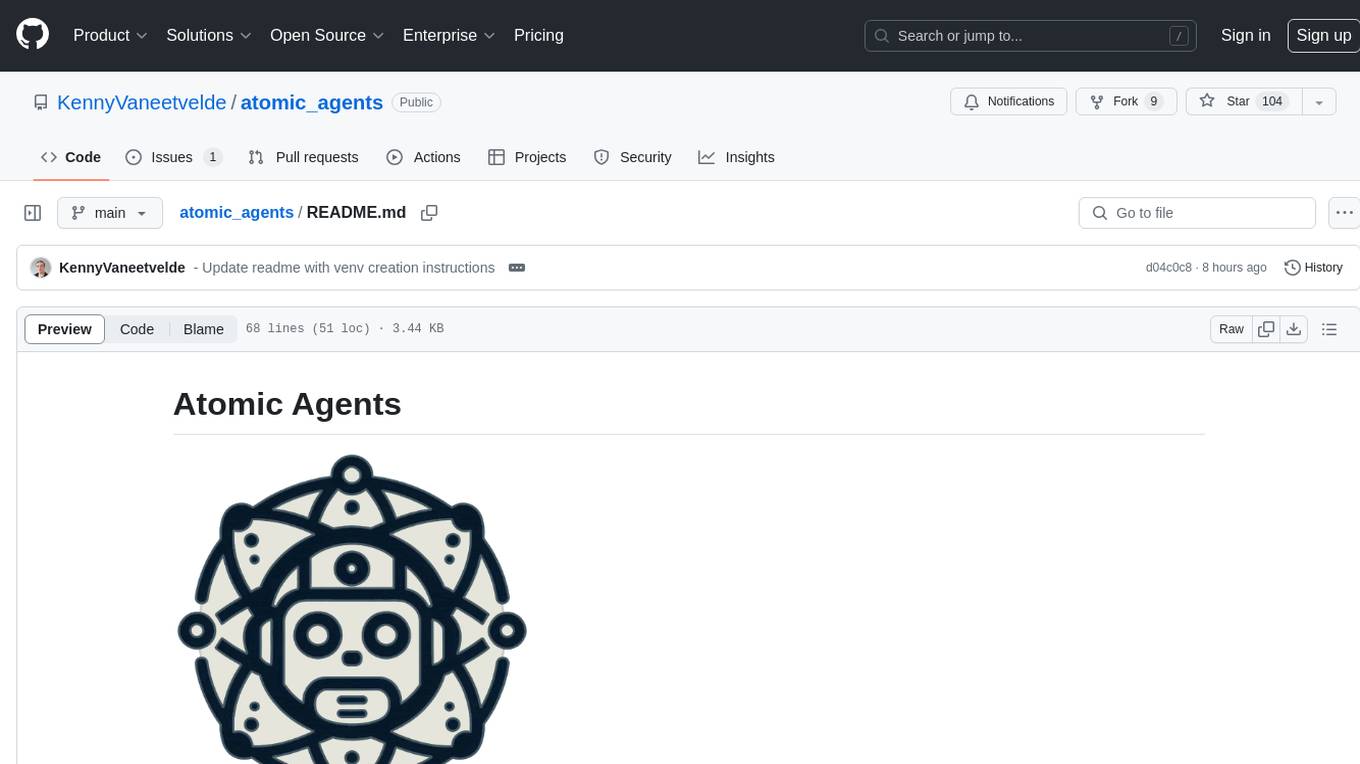
atomic_agents
Atomic Agents is a modular and extensible framework designed for creating powerful applications. It follows the principles of Atomic Design, emphasizing small and single-purpose components. Leveraging Pydantic for data validation and serialization, the framework offers a set of tools and agents that can be combined to build AI applications. It depends on the Instructor package and supports various APIs like OpenAI, Cohere, Anthropic, and Gemini. Atomic Agents is suitable for developers looking to create AI agents with a focus on modularity and flexibility.
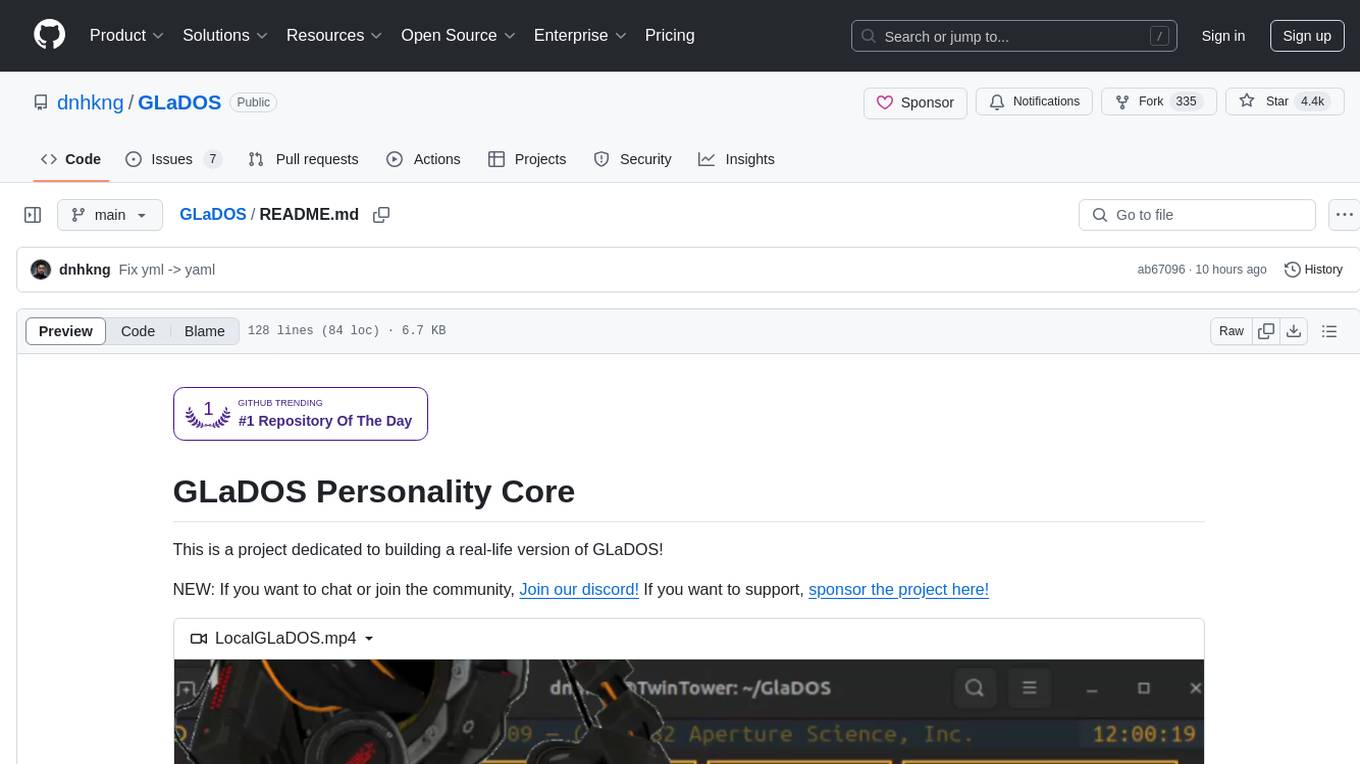
GLaDOS
GLaDOS Personality Core is a project dedicated to building a real-life version of GLaDOS, an aware, interactive, and embodied AI system. The project aims to train GLaDOS voice generator, create a 'Personality Core,' develop medium- and long-term memory, provide vision capabilities, design 3D-printable parts, and build an animatronics system. The software architecture focuses on low-latency voice interactions and minimal dependencies. The hardware system includes servo- and stepper-motors, 3D printable parts for GLaDOS's body, animations for expression, and a vision system for tracking and interaction. Installation instructions involve setting up a local LLM server, installing drivers, and running GLaDOS on different operating systems.
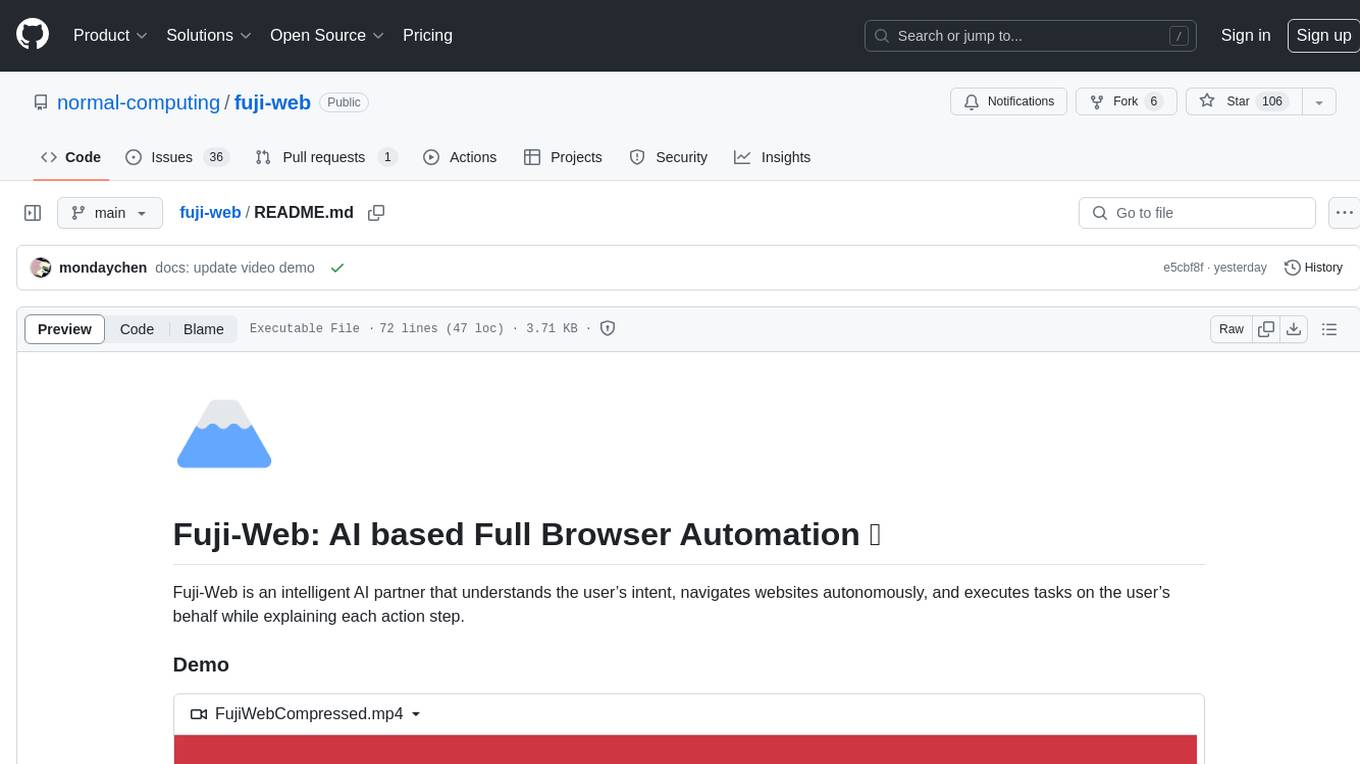
fuji-web
Fuji-Web is an intelligent AI partner designed for full browser automation. It autonomously navigates websites and performs tasks on behalf of the user while providing explanations for each action step. Users can easily install the extension in their browser, access the Fuji icon to input tasks, and interact with the tool to streamline web browsing tasks. The tool aims to enhance user productivity by automating repetitive web actions and providing a seamless browsing experience.
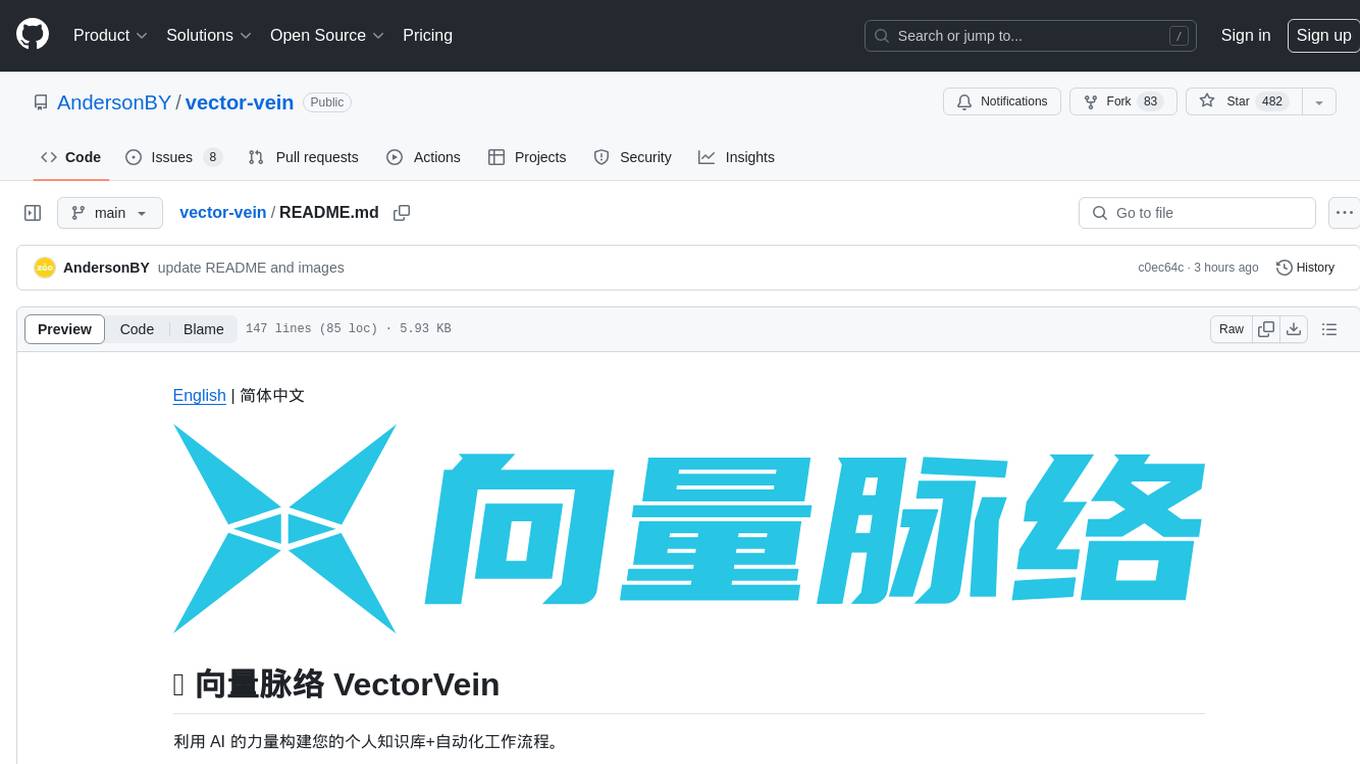
vector-vein
VectorVein is a no-code AI workflow software inspired by LangChain and langflow, aiming to combine the powerful capabilities of large language models and enable users to achieve intelligent and automated daily workflows through simple drag-and-drop actions. Users can create powerful workflows without the need for programming, automating all tasks with ease. The software allows users to define inputs, outputs, and processing methods to create customized workflow processes for various tasks such as translation, mind mapping, summarizing web articles, and automatic categorization of customer reviews.
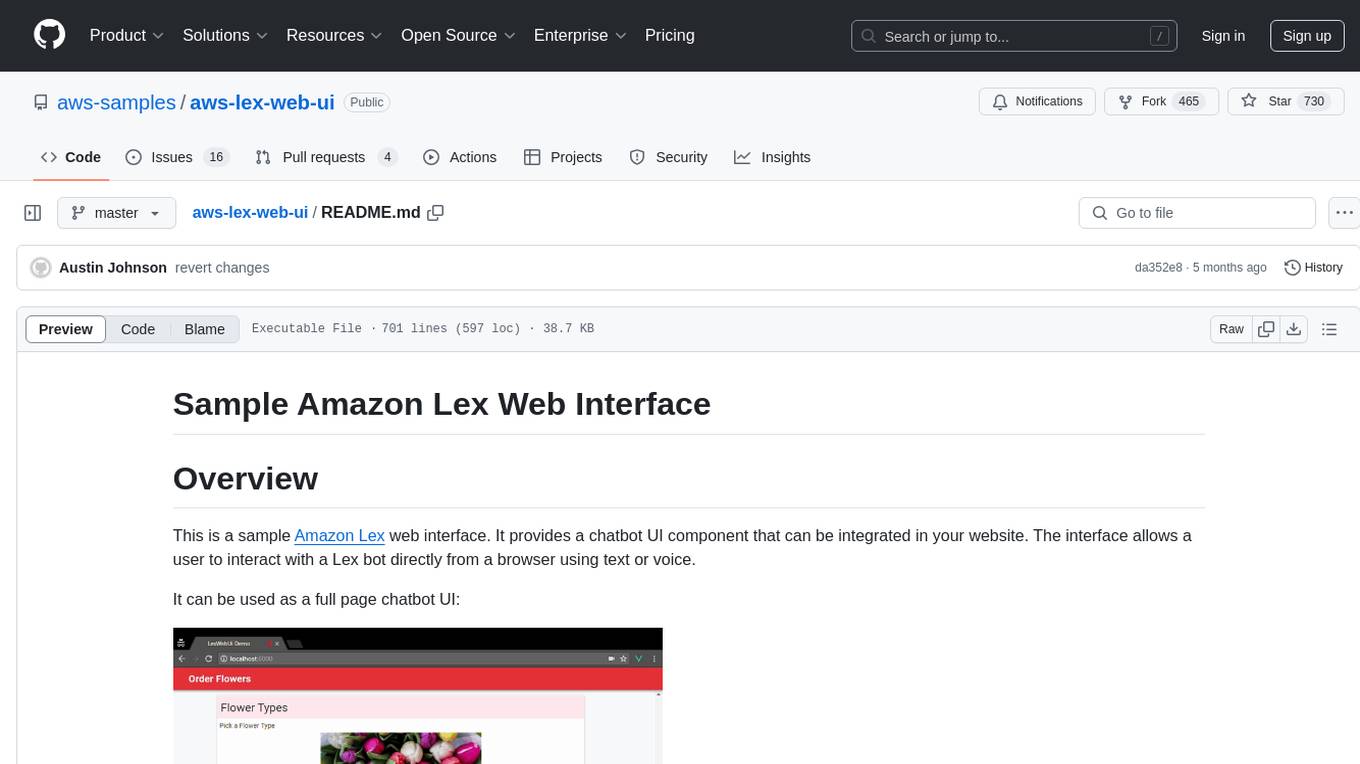
aws-lex-web-ui
The AWS Lex Web UI is a sample Amazon Lex web interface that provides a chatbot UI component for integration into websites. It supports voice and text interactions, Lex response cards, and programmable configuration using JavaScript. The interface can be used as a full-page chatbot UI or embedded as a widget. It offers mobile-ready responsive UI, seamless voice-text switching, and interactive messaging support. The project includes CloudFormation templates for easy deployment and customization. Users can modify configurations, integrate the UI into existing sites, and deploy using various methods like CloudFormation, pre-built libraries, or npm installation.
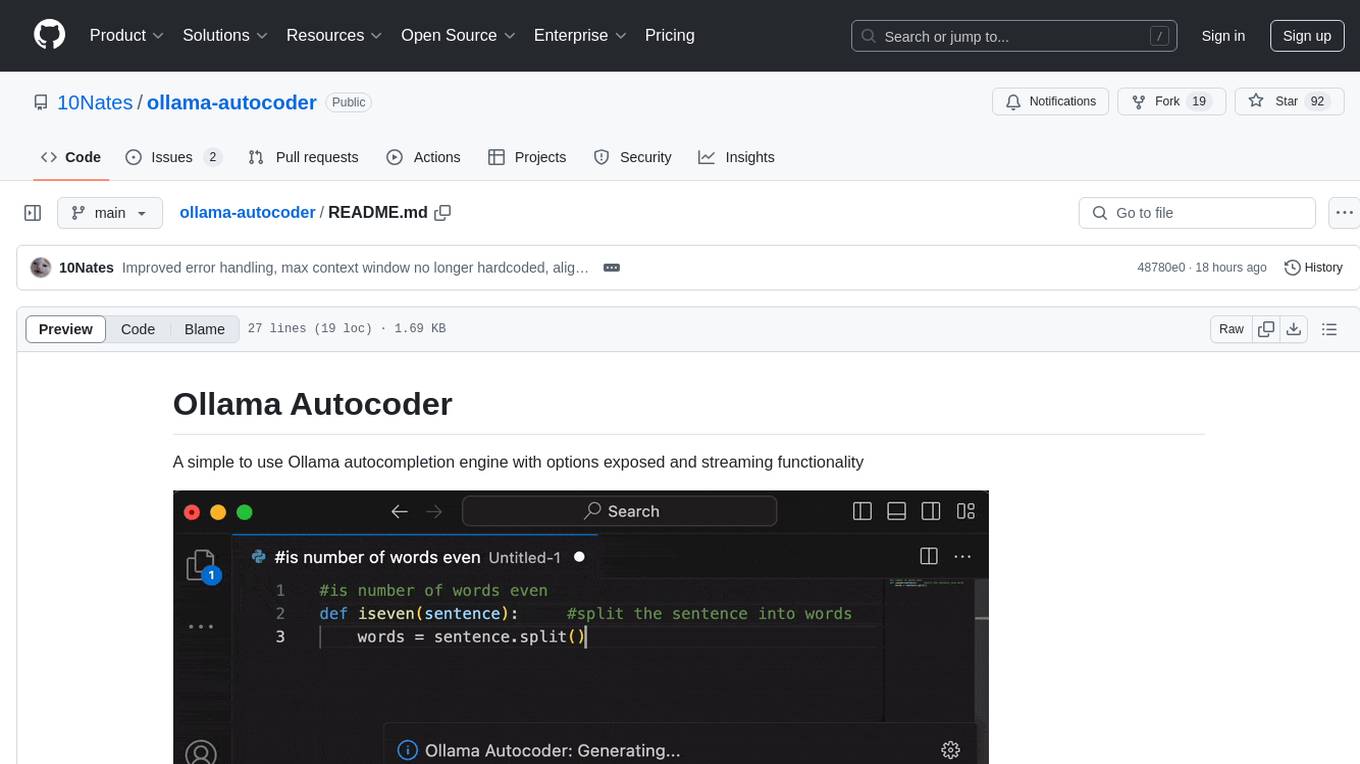
ollama-autocoder
Ollama Autocoder is a simple to use autocompletion engine that integrates with Ollama AI. It provides options for streaming functionality and requires specific settings for optimal performance. Users can easily generate text completions by pressing a key or using a command pallete. The tool is designed to work with Ollama API and a specified model, offering real-time generation of text suggestions.