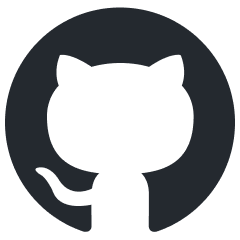
bytechef
Open-source, low-code, extendable API integration & workflow automation platform. Integrate your organizations or your SaaS product with any third party API
Stars: 292
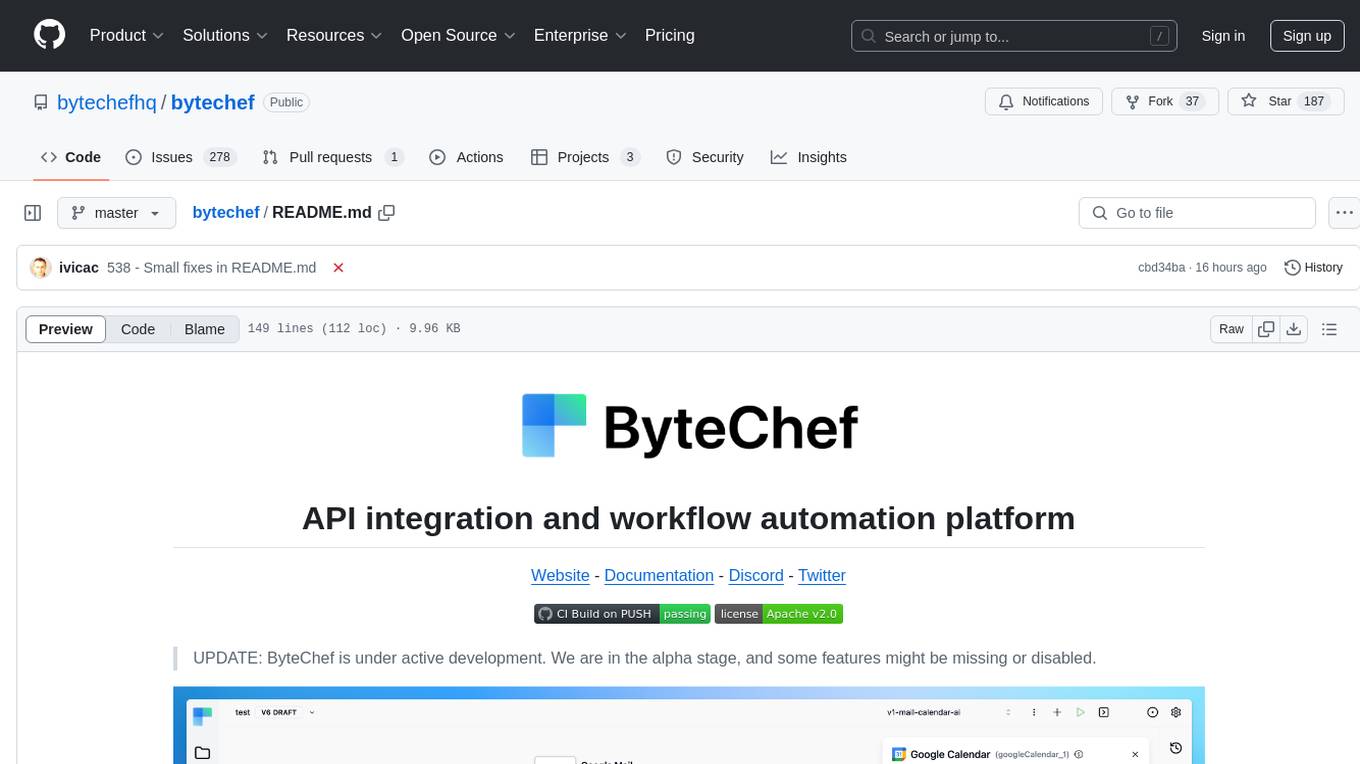
ByteChef is an open-source, low-code, extendable API integration and workflow automation platform. It provides an intuitive UI Workflow Editor, event-driven & scheduled workflows, multiple flow controls, built-in code editor supporting Java, JavaScript, Python, and Ruby, rich component ecosystem, extendable with custom connectors, AI-ready with built-in AI components, developer-ready to expose workflows as APIs, version control friendly, self-hosted, scalable, and resilient. It allows users to build and visualize workflows, automate tasks across SaaS apps, internal APIs, and databases, and handle millions of workflows with high availability and fault tolerance.
README:
Website - Documentation - Discord - Twitter
UPDATE: ByteChef is under active development. We are in the alpha stage, and some features might be missing or disabled.
ByteChef is an open-source, low-code, extendable API integration and workflow automation platform. ByteChef can help you as:
- An automation solution that allows you to integrate and build automation workflows across your SaaS apps, internal APIs, and databases.
- An embedded solution targeted explicitly for SaaS products, allowing your customers to integrate applications they use with your product.
- Intuitive UI Workflow Editor: build and visualize workflows via the UI editor by dragging and dropping components and defining their relations.
- Event-Driven & Scheduled Workflows: automate scheduled and real-time event-driven workflows via a simple trigger definition.
- Multiple flow controls: use the range of various flow controls such as condition, switch, loop, each, parallel, etc.
- Built-In Code Editor: if you need to go beyond no-code workflow definition, leverage our low-code capabilities and write workflow definitions in JSON format and blocks of the code executed during the workflow execution in one of the languages: Java, JavaScript, Python, and Ruby with syntax highlighting, auto-completion and real-time syntax validation.
- Rich Component Ecosystem: hundreds of components built in to extract data from any database, SaaS applications, internal APIs, or cloud storage.
- Extendable: develop custom connectors when no built-in connectors exist in the above-mentioned languages.
- AI ready: built-in AI components that can run multiple AI models and other AI algorithms.
- Developer ready: expose your workflows as APIs to be consumed by other applications or call directly APIs of targeted services. The platform handles authentication.
- Version Control Friendly: write your workflows from the UI editor and push them to your preferred Git branch directly from ByteChef, enabling best practices with CI/CD pipelines and version control systems.
- Self-hosted: install ByteChef on the premise to have complete control over execution and data, in addition to being able to use a hosted version.
- Scalable: it is designed to handle millions of workflows with high availability and fault tolerance. Start with one instance only, and scale as required.
- Structure & Resilience: bring resilience to your workflows with labels, sub-flows, retries, timeout, error handling, inputs, outputs that generate artifacts in the UI, variables, conditional branching, advanced scheduling, event triggers, dynamic tasks, sequential and parallel tasks, and skip tasks or triggers when needed by disabling them.
There are couple ways to give ByteChef a quick spin on your local machine. You can use this to test, learn or contribute.
Requirement: Docker Desktop - Docker compose allows you to configure and run several dependent docker containers. Some OS environments may not support it. In that case follow Method 2 described later.
This is the fastest possible way to start Bytechef. There is docker-compose.yml in the repository root. Either checkout repository locally to your machine or download file. Make sure you execute this command taking care of correct path to docker-compose.yml
file:
docker compose -f docker-compose.yml up
Both postgres database and bytechef docker container would start.
This option demands pinch of focus as it allows user to profile containers. Run the following commands from your terminal to have ByteChef up and running right away.
docker network create -d bridge bytechef_network
docker run --name postgres -d -p 5432:5432 \
--env POSTGRES_USER=postgres \
--env POSTGRES_PASSWORD=postgres \
--hostname postgres \
--network bytechef_network \
-v /opt/postgre/data:/var/lib/postgresql/data \
postgres:15-alpine
NOTE: -v
mount option is not mandatory. It mounts local DB storage to make easier access to DB infrastructure files.
docker run --name bytechef -it -p 8080:8080 \
--env BYTECHEF_DATASOURCE_URL=jdbc:postgresql://postgres:5432/bytechef \
--env BYTECHEF_DATASOURCE_USERNAME=postgres \
--env BYTECHEF_DATASOURCE_PASSWORD=postgres \
--env BYTECHEF_SECURITY_REMEMBER_ME_KEY=e48612ba1fd46fa7089fe9f5085d8d164b53ffb2 \
--network bytechef_network \
docker.bytechef.io/bytechef/bytechef:latest
NOTE: -it
(interactive) flag may be replaced with -d
(detached). Keep it interactive if you want to track logs which can be handy for troubleshooting. Use -p 8080:8080
to customize port.
Use browser and open http://localhost:8080/login (please take care about port - if port setting is modified in docker compose file or docker run command, this URL should be updated). Chose Create Account link to setup user and than use same user and password to sign in.
Documentation is available at docs.bytechef.io. It covers all the necessary information to get started with ByteChef, including installation, configuration, and usage.
For help, you can use one of these channels to ask a question:
- Discord - Discussions with the community and the team.
- GitHub - For bug reports and feature requests.
- Twitter - Get the product updates easily.
Check out our roadmap to get informed of the latest features released and the upcoming ones.
If you'd like to contribute, kindly read our Contributing Guide to learn and understand about our development process, how to propose bug fixes and improvements, and how to build and test your changes to ByteChef.
ByteChef is released under Apache License v2.0. See LICENSE for more information.
Made by community 💛
This project has started as a fork of Piper, an open-source, distributed workflow engine.
For Tasks:
Click tags to check more tools for each tasksFor Jobs:
Alternative AI tools for bytechef
Similar Open Source Tools
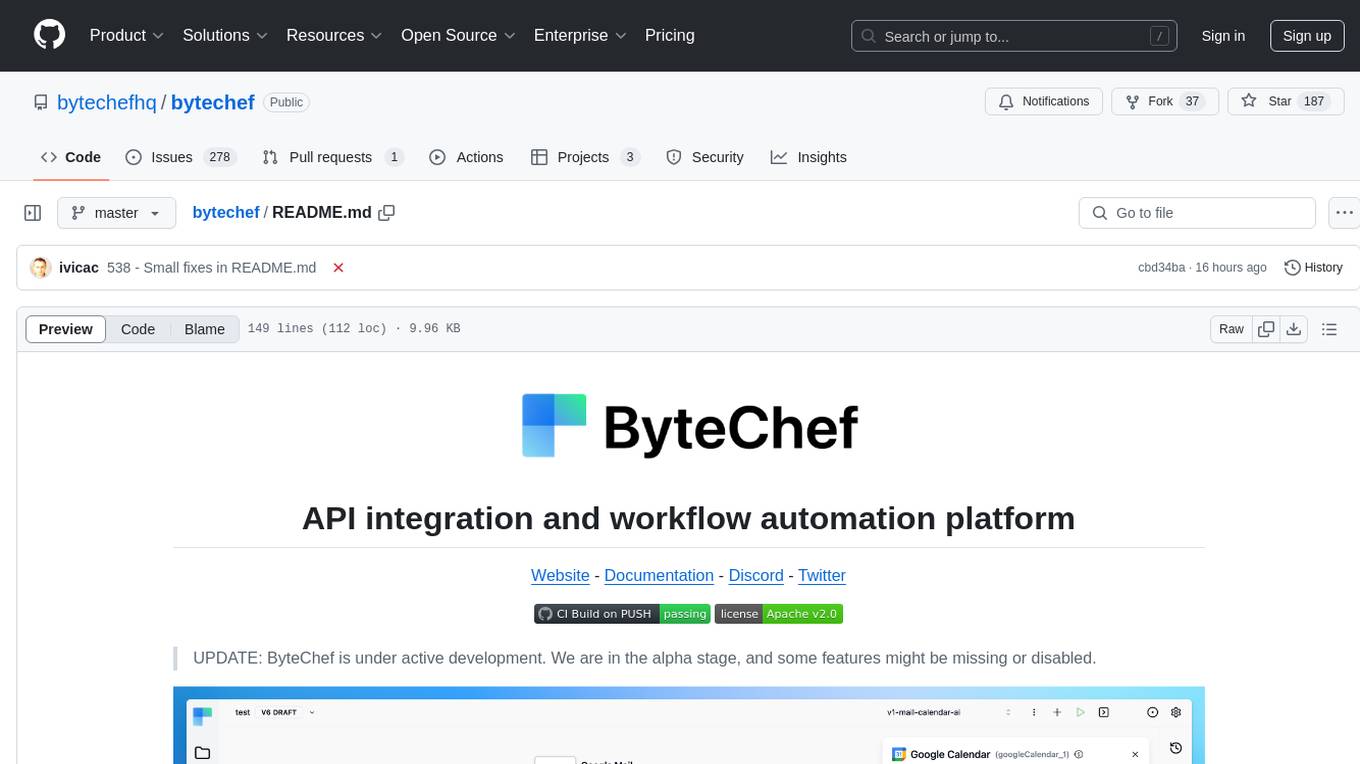
bytechef
ByteChef is an open-source, low-code, extendable API integration and workflow automation platform. It provides an intuitive UI Workflow Editor, event-driven & scheduled workflows, multiple flow controls, built-in code editor supporting Java, JavaScript, Python, and Ruby, rich component ecosystem, extendable with custom connectors, AI-ready with built-in AI components, developer-ready to expose workflows as APIs, version control friendly, self-hosted, scalable, and resilient. It allows users to build and visualize workflows, automate tasks across SaaS apps, internal APIs, and databases, and handle millions of workflows with high availability and fault tolerance.
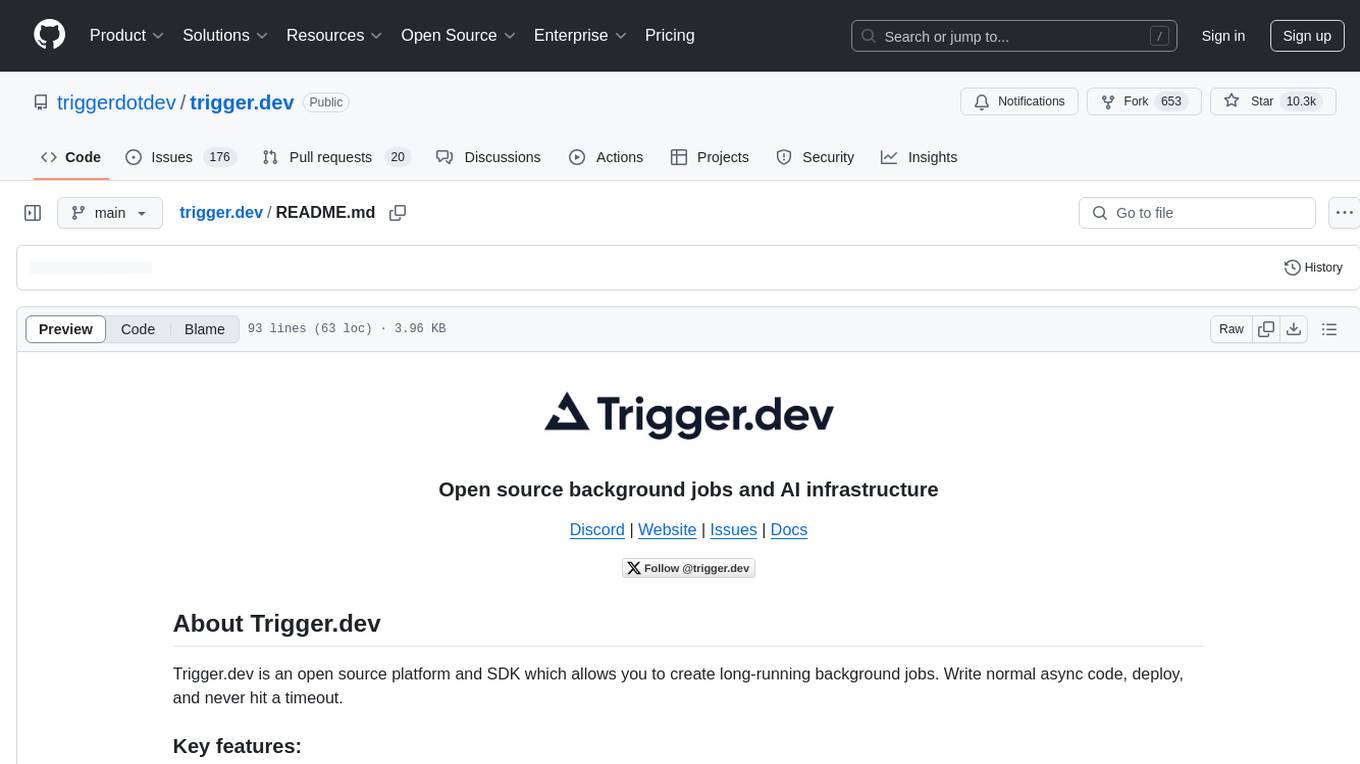
trigger.dev
Trigger.dev is an open source platform and SDK for creating long-running background jobs. It provides features like JavaScript and TypeScript SDK, no timeouts, retries, queues, schedules, observability, React hooks, Realtime API, custom alerts, elastic scaling, and works with existing tech stack. Users can create tasks in their codebase, deploy tasks using the SDK, manage tasks in different environments, and have full visibility of job runs. The platform offers a trace view of every task run for detailed monitoring. Getting started is easy with account creation, project setup, and onboarding instructions. Self-hosting and development guides are available for users interested in contributing or hosting Trigger.dev.
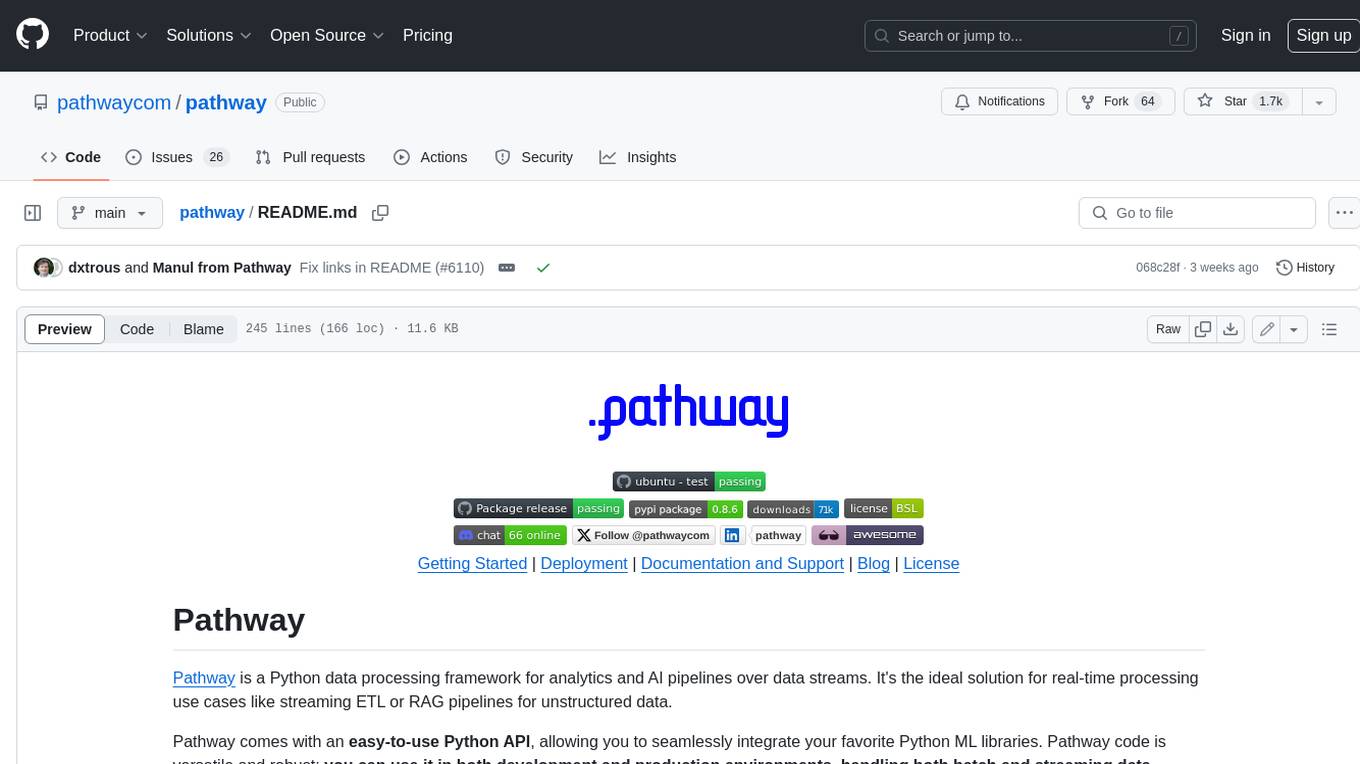
pathway
Pathway is a Python data processing framework for analytics and AI pipelines over data streams. It's the ideal solution for real-time processing use cases like streaming ETL or RAG pipelines for unstructured data. Pathway comes with an **easy-to-use Python API** , allowing you to seamlessly integrate your favorite Python ML libraries. Pathway code is versatile and robust: **you can use it in both development and production environments, handling both batch and streaming data effectively**. The same code can be used for local development, CI/CD tests, running batch jobs, handling stream replays, and processing data streams. Pathway is powered by a **scalable Rust engine** based on Differential Dataflow and performs incremental computation. Your Pathway code, despite being written in Python, is run by the Rust engine, enabling multithreading, multiprocessing, and distributed computations. All the pipeline is kept in memory and can be easily deployed with **Docker and Kubernetes**. You can install Pathway with pip: `pip install -U pathway` For any questions, you will find the community and team behind the project on Discord.
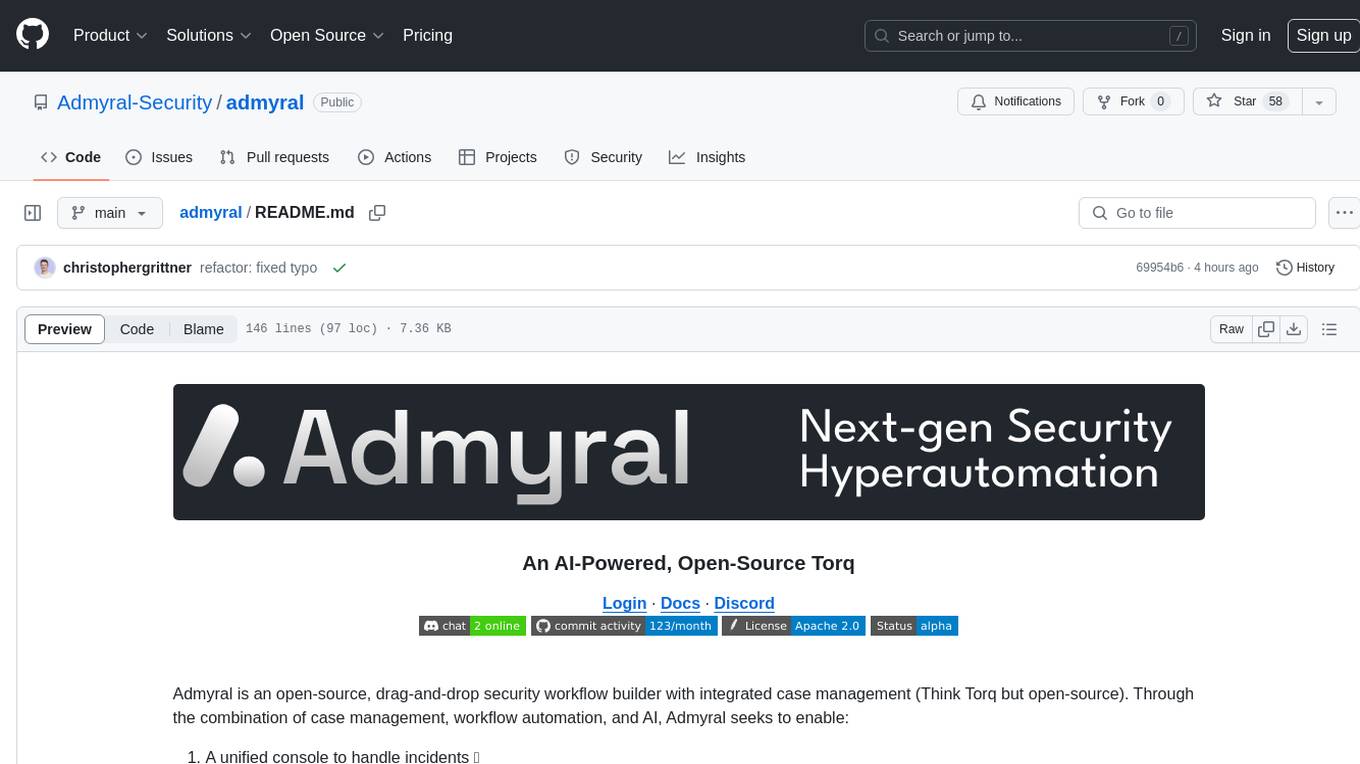
admyral
Admyral is an open-source Cybersecurity Automation & Investigation Assistant that provides a unified console for investigations and incident handling, workflow automation creation, automatic alert investigation, and next step suggestions for analysts. It aims to tackle alert fatigue and automate security workflows effectively by offering features like workflow actions, AI actions, case management, alert handling, and more. Admyral combines security automation and case management to streamline incident response processes and improve overall security posture. The tool is open-source, transparent, and community-driven, allowing users to self-host, contribute, and collaborate on integrations and features.
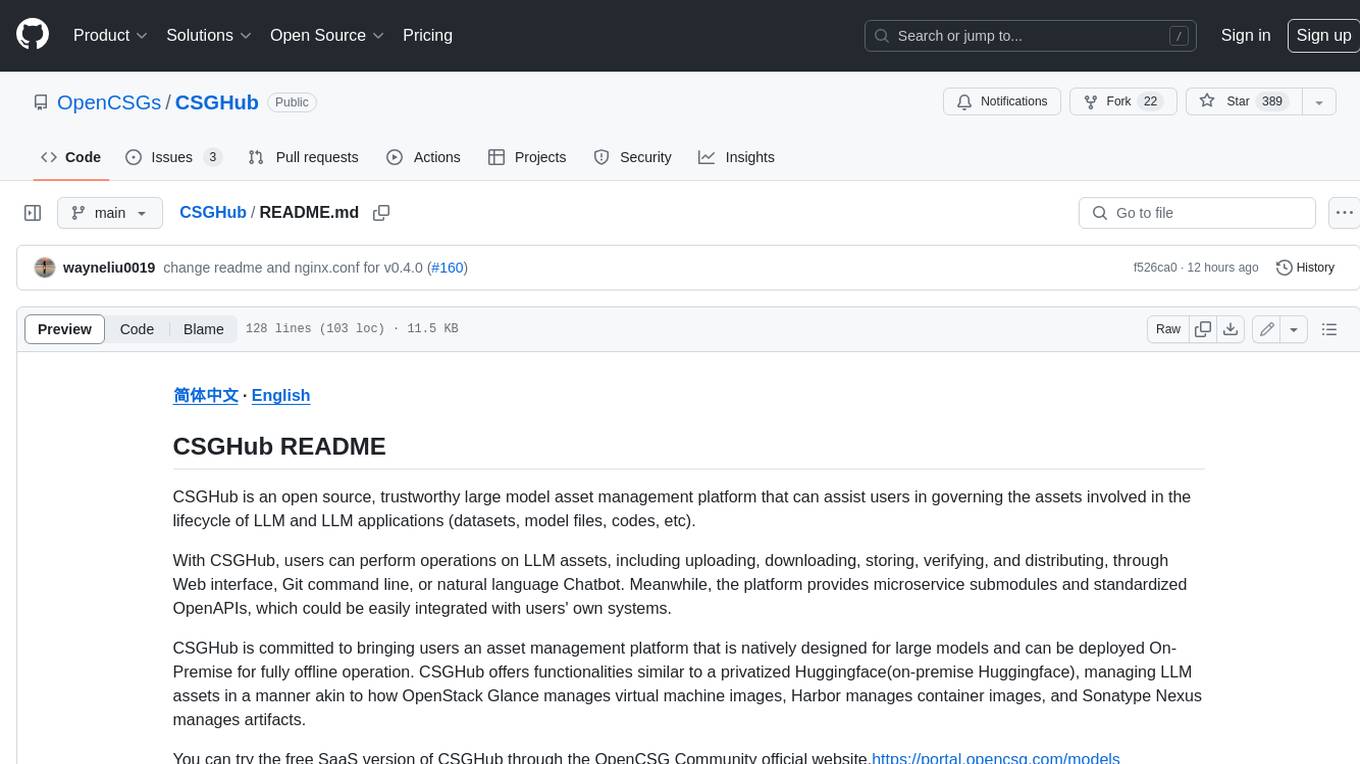
CSGHub
CSGHub is an open source, trustworthy large model asset management platform that can assist users in governing the assets involved in the lifecycle of LLM and LLM applications (datasets, model files, codes, etc). With CSGHub, users can perform operations on LLM assets, including uploading, downloading, storing, verifying, and distributing, through Web interface, Git command line, or natural language Chatbot. Meanwhile, the platform provides microservice submodules and standardized OpenAPIs, which could be easily integrated with users' own systems. CSGHub is committed to bringing users an asset management platform that is natively designed for large models and can be deployed On-Premise for fully offline operation. CSGHub offers functionalities similar to a privatized Huggingface(on-premise Huggingface), managing LLM assets in a manner akin to how OpenStack Glance manages virtual machine images, Harbor manages container images, and Sonatype Nexus manages artifacts.
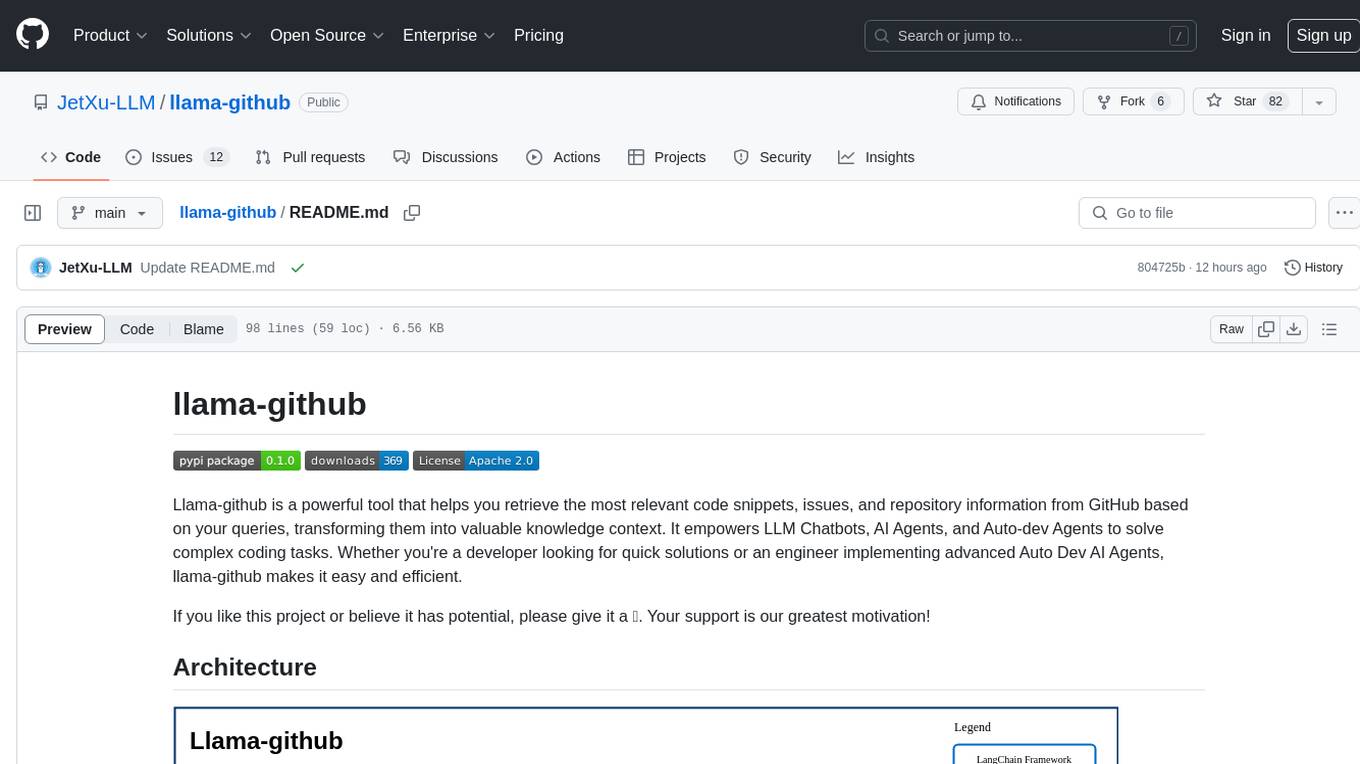
llama-github
Llama-github is a powerful tool that helps retrieve relevant code snippets, issues, and repository information from GitHub based on queries. It empowers AI agents and developers to solve coding tasks efficiently. With features like intelligent GitHub retrieval, repository pool caching, LLM-powered question analysis, and comprehensive context generation, llama-github excels at providing valuable knowledge context for development needs. It supports asynchronous processing, flexible LLM integration, robust authentication options, and logging/error handling for smooth operations and troubleshooting. The vision is to seamlessly integrate with GitHub for AI-driven development solutions, while the roadmap focuses on empowering LLMs to automatically resolve complex coding tasks.
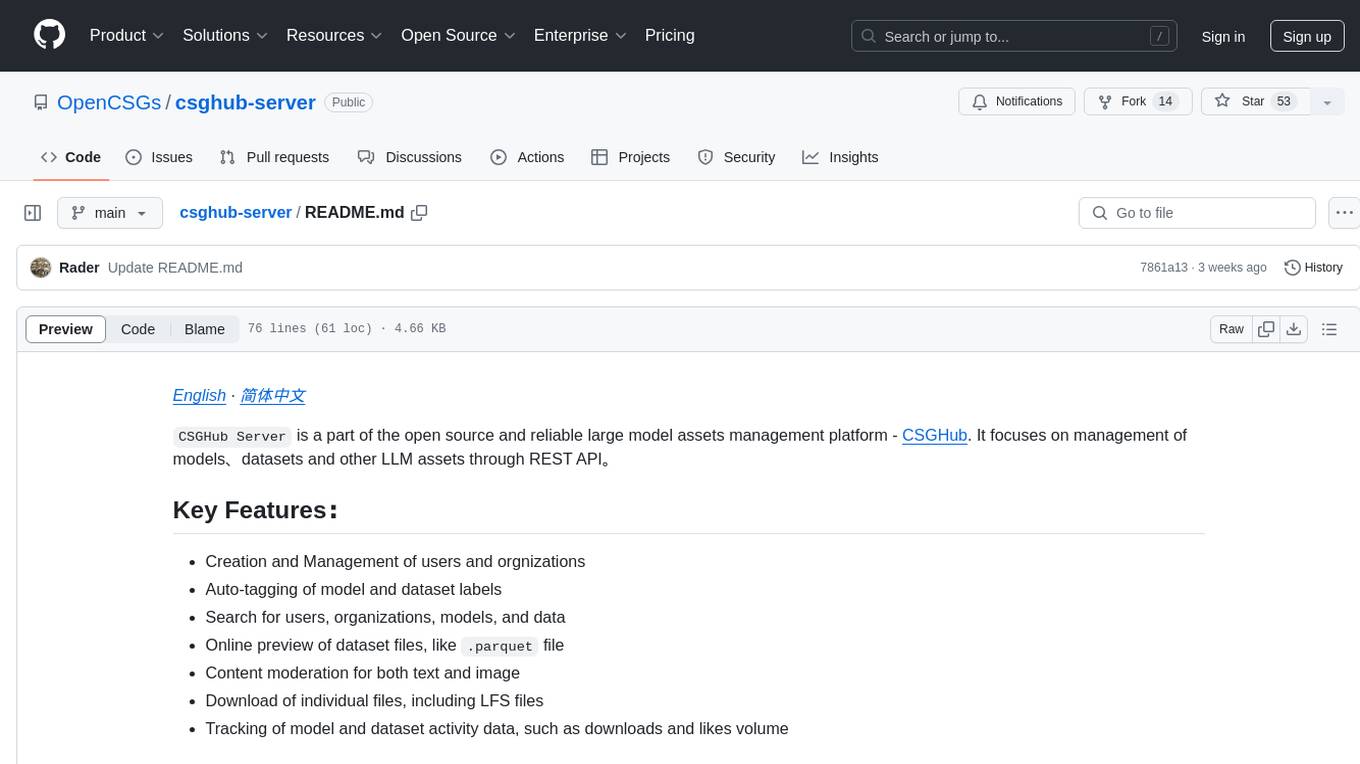
csghub-server
CSGHub Server is a part of the open source and reliable large model assets management platform - CSGHub. It focuses on management of models, datasets, and other LLM assets through REST API. Key features include creation and management of users and organizations, auto-tagging of model and dataset labels, search functionality, online preview of dataset files, content moderation for text and image, download of individual files, tracking of model and dataset activity data. The tool is extensible and customizable, supporting different git servers, flexible LFS storage system configuration, and content moderation options. The roadmap includes support for more Git servers, Git LFS, dataset online viewer, model/dataset auto-tag, S3 protocol support, model format conversion, and model one-click deploy. The project is licensed under Apache 2.0 and welcomes contributions.
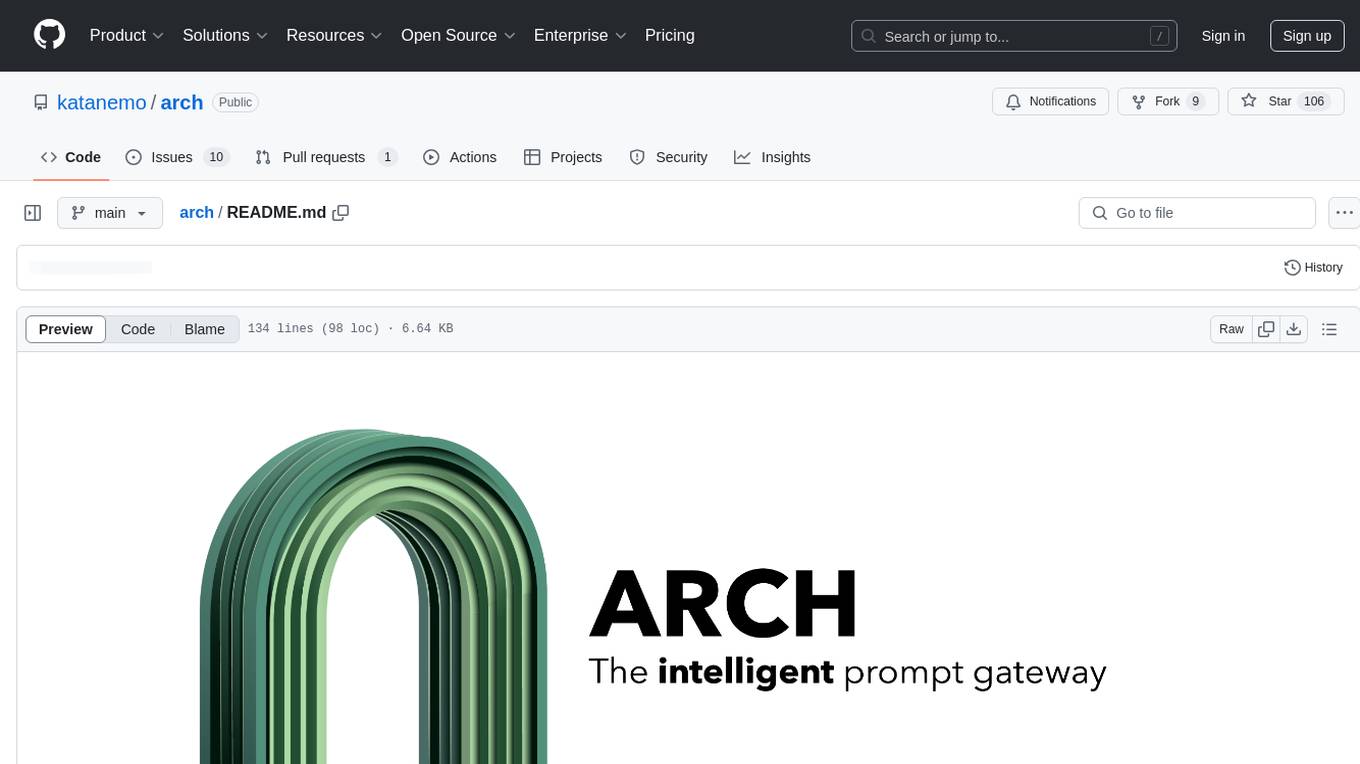
arch
Arch is an intelligent Layer 7 gateway designed to protect, observe, and personalize LLM applications with APIs. It handles tasks like detecting and rejecting jailbreak attempts, calling backend APIs, disaster recovery, and observability. Built on Envoy Proxy, it offers features like function calling, prompt guardrails, traffic management, and standards-based observability. Arch aims to improve the speed, security, and personalization of generative AI applications.
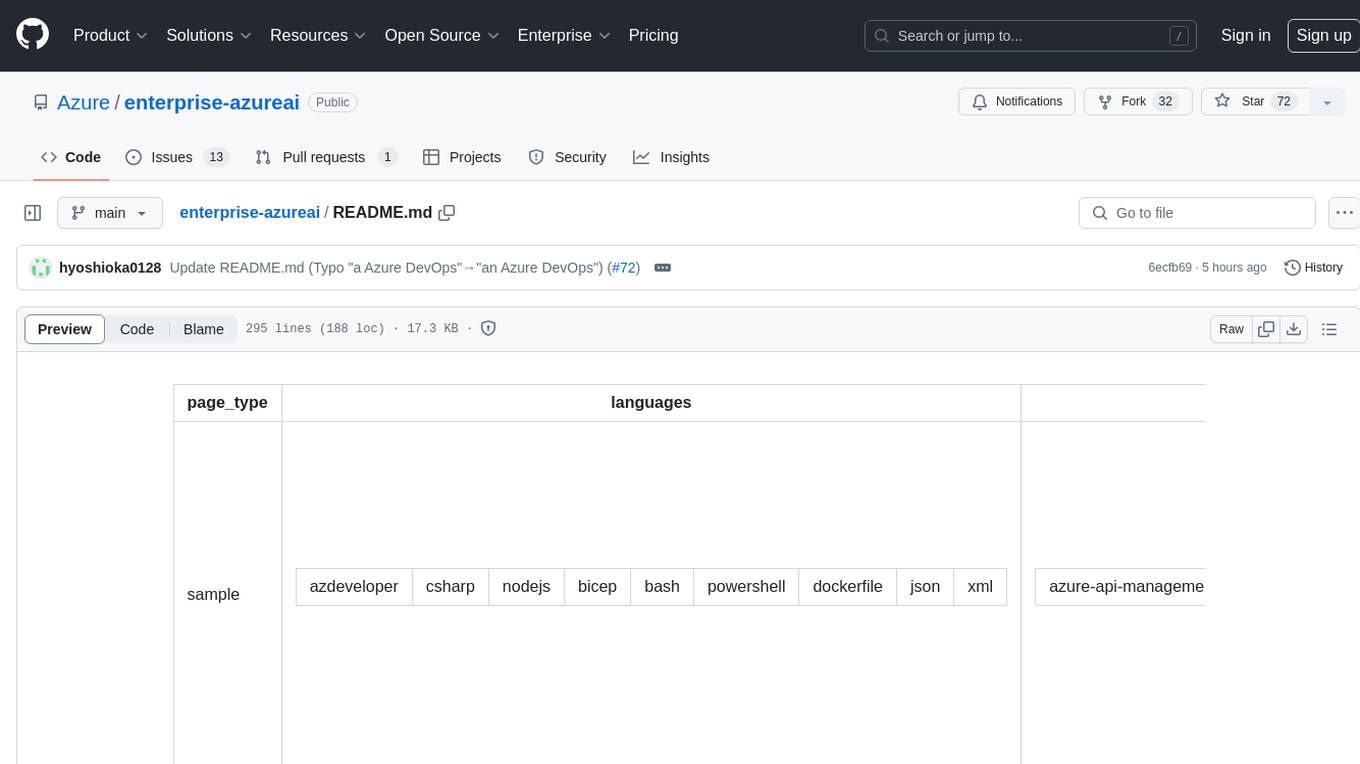
enterprise-azureai
Azure OpenAI Service is a central capability with Azure API Management, providing guidance and tools for organizations to implement Azure OpenAI in a production environment with an emphasis on cost control, secure access, and usage monitoring. It includes infrastructure-as-code templates, CI/CD pipelines, secure access management, usage monitoring, load balancing, streaming requests, and end-to-end samples like ChatApp and Azure Dashboards.
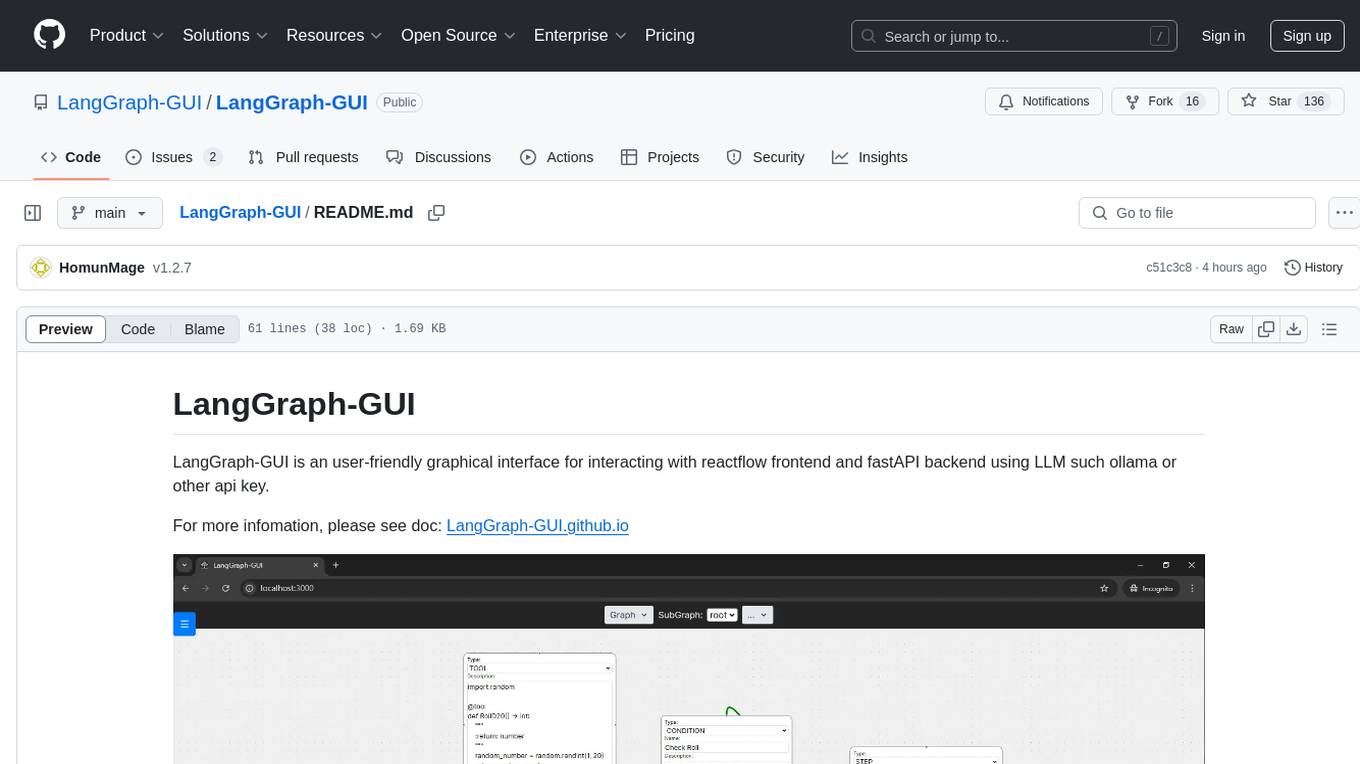
LangGraph-GUI
LangGraph-GUI is a user-friendly graphical interface for interacting with reactflow frontend and fastAPI backend using LLM such as ollama or other API key. It provides a convenient way to work with language models and APIs, offering a seamless experience for users to visualize and interact with the data flow. The tool simplifies the process of setting up the environment and accessing the application, making it easier for users to leverage the power of language models in their projects.
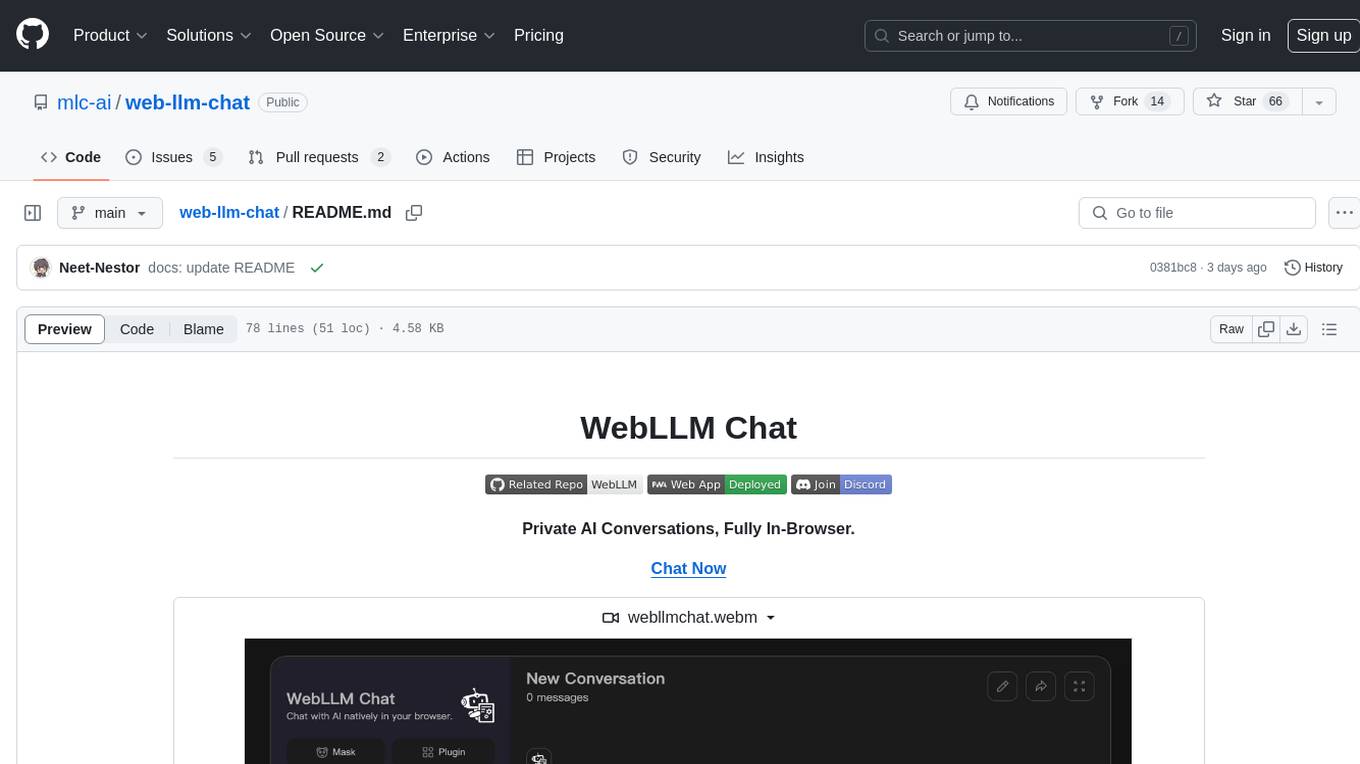
web-llm-chat
WebLLM Chat is a private AI chat interface that combines WebLLM with a user-friendly design, leveraging WebGPU to run large language models natively in your browser. It offers browser-native AI experience with WebGPU acceleration, guaranteed privacy as all data processing happens locally, offline accessibility, user-friendly interface with markdown support, and open-source customization. The project aims to democratize AI technology by making powerful tools accessible directly to end-users, enhancing the chatting experience and broadening the scope for deployment of self-hosted and customizable language models.
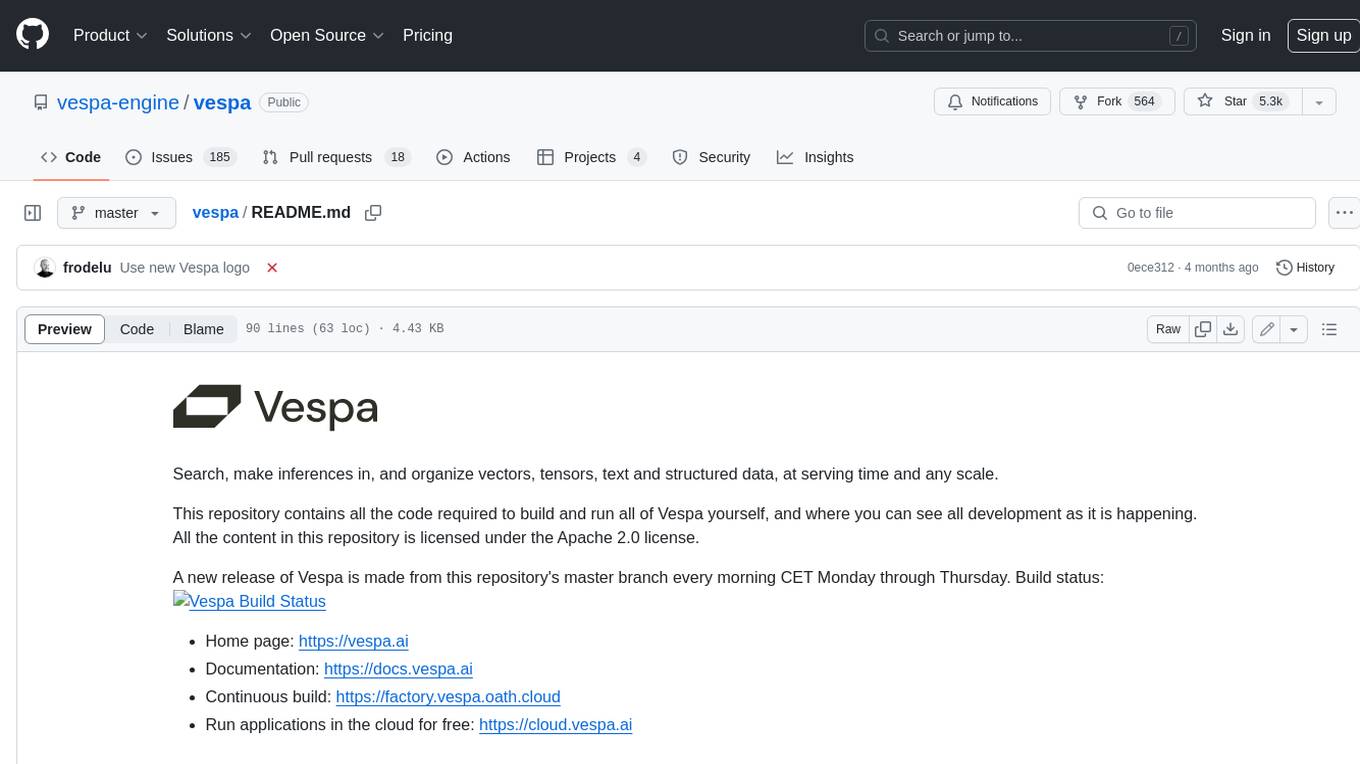
vespa
Vespa is a platform that performs operations such as selecting a subset of data in a large corpus, evaluating machine-learned models over the selected data, organizing and aggregating it, and returning it, typically in less than 100 milliseconds, all while the data corpus is continuously changing. It has been in development for many years and is used on a number of large internet services and apps which serve hundreds of thousands of queries from Vespa per second.
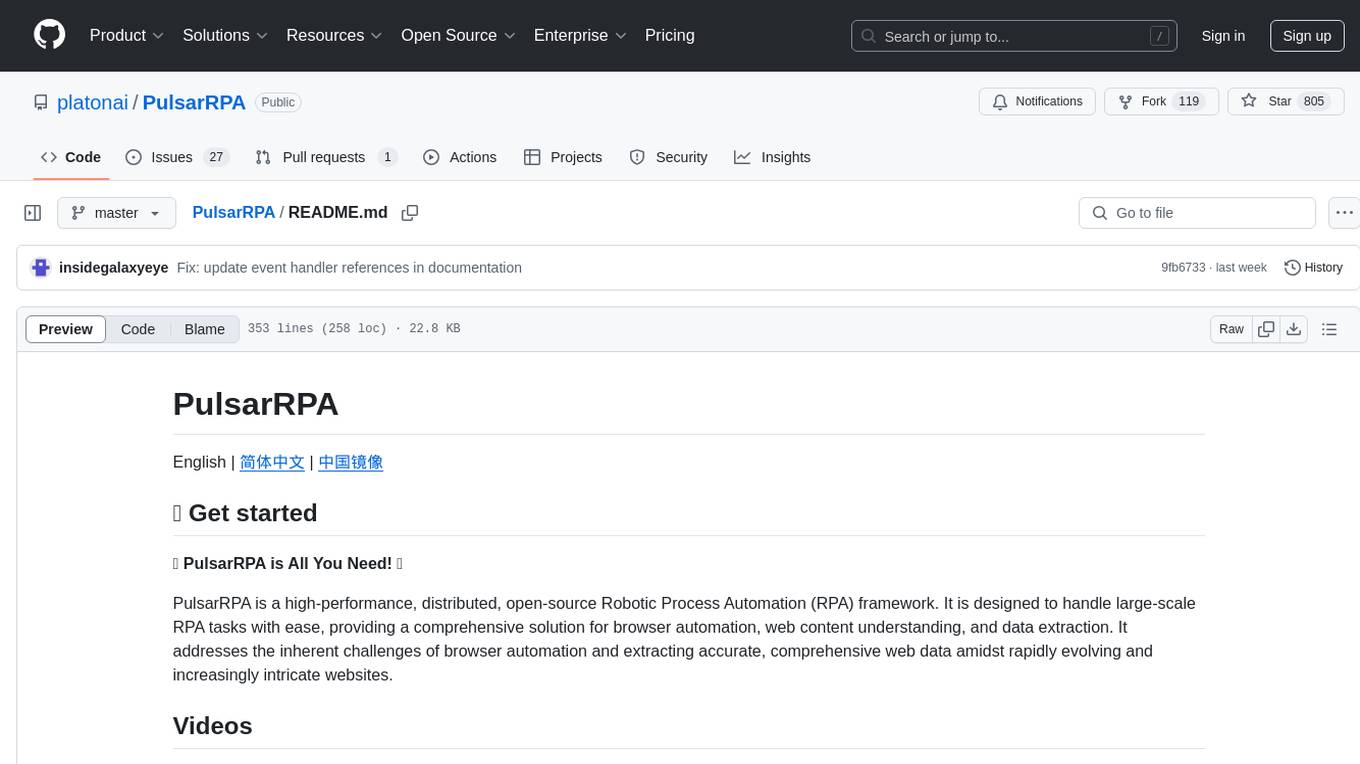
PulsarRPA
PulsarRPA is a high-performance, distributed, open-source Robotic Process Automation (RPA) framework designed to handle large-scale RPA tasks with ease. It provides a comprehensive solution for browser automation, web content understanding, and data extraction. PulsarRPA addresses challenges of browser automation and accurate web data extraction from complex and evolving websites. It incorporates innovative technologies like browser rendering, RPA, intelligent scraping, advanced DOM parsing, and distributed architecture to ensure efficient, accurate, and scalable web data extraction. The tool is open-source, customizable, and supports cutting-edge information extraction technology, making it a preferred solution for large-scale web data extraction.
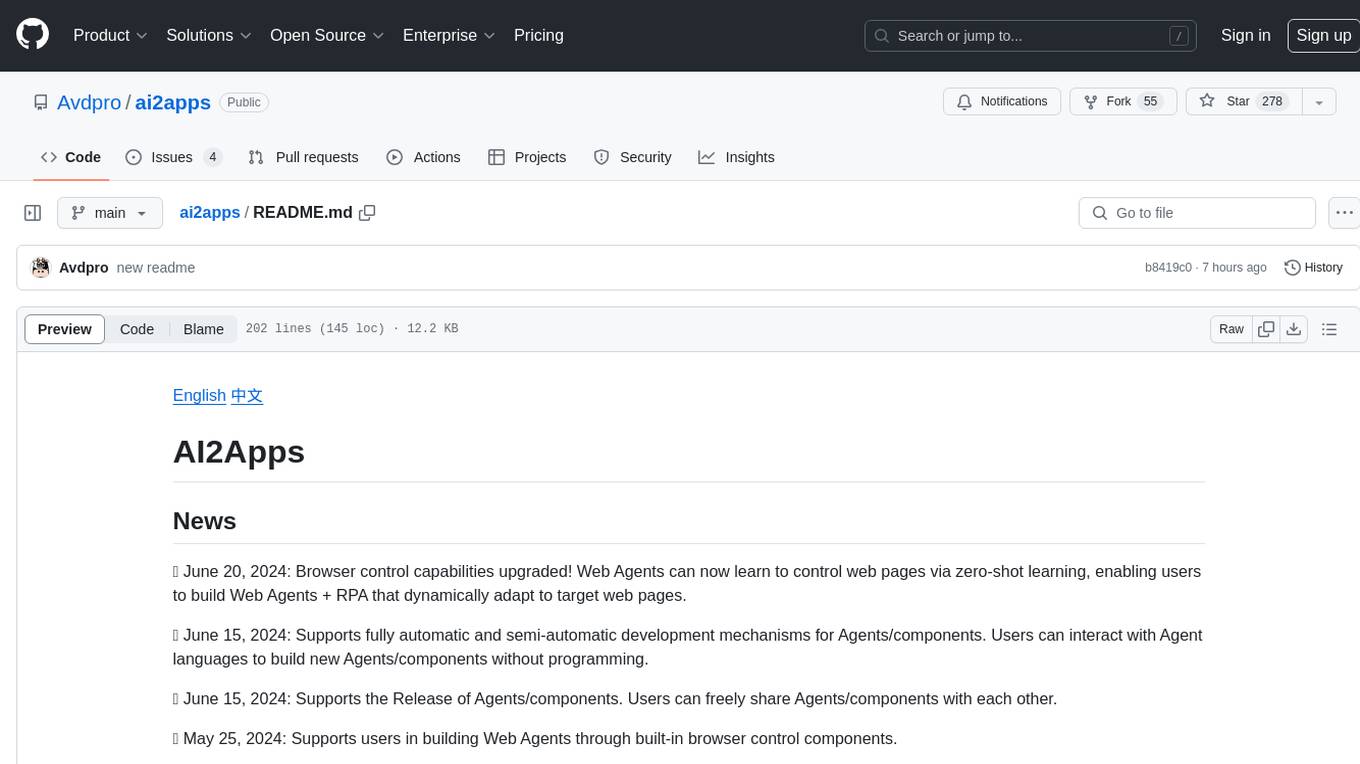
ai2apps
AI2Apps is a visual IDE for building LLM-based AI agent applications, enabling developers to efficiently create AI agents through drag-and-drop, with features like design-to-development for rapid prototyping, direct packaging of agents into apps, powerful debugging capabilities, enhanced user interaction, efficient team collaboration, flexible deployment, multilingual support, simplified product maintenance, and extensibility through plugins.
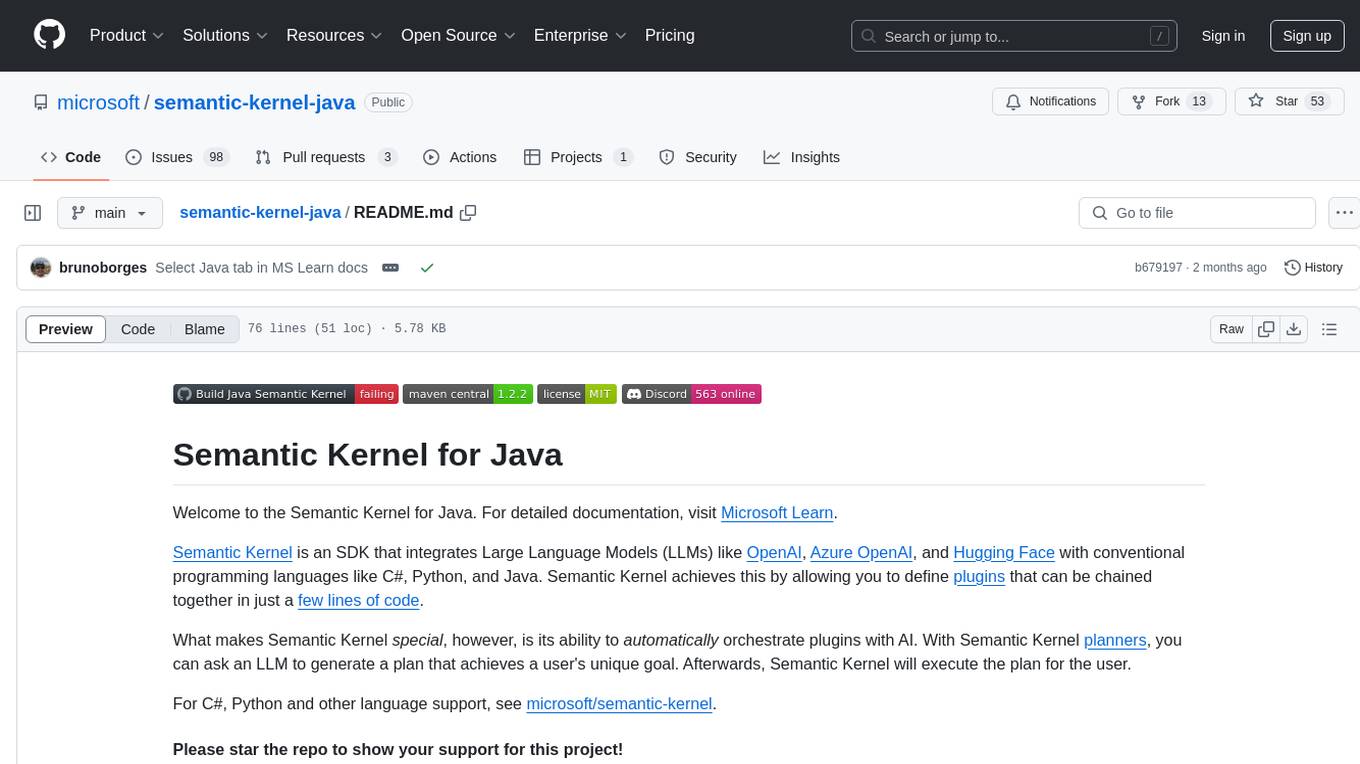
semantic-kernel-java
Semantic Kernel for Java is an SDK that integrates Large Language Models (LLMs) like OpenAI, Azure OpenAI, and Hugging Face with conventional programming languages like C#, Python, and Java. It allows defining plugins that can be chained together in just a few lines of code. The tool automatically orchestrates plugins with AI, enabling users to generate plans to achieve unique goals and execute them. The project welcomes contributions, bug reports, and suggestions from the community.
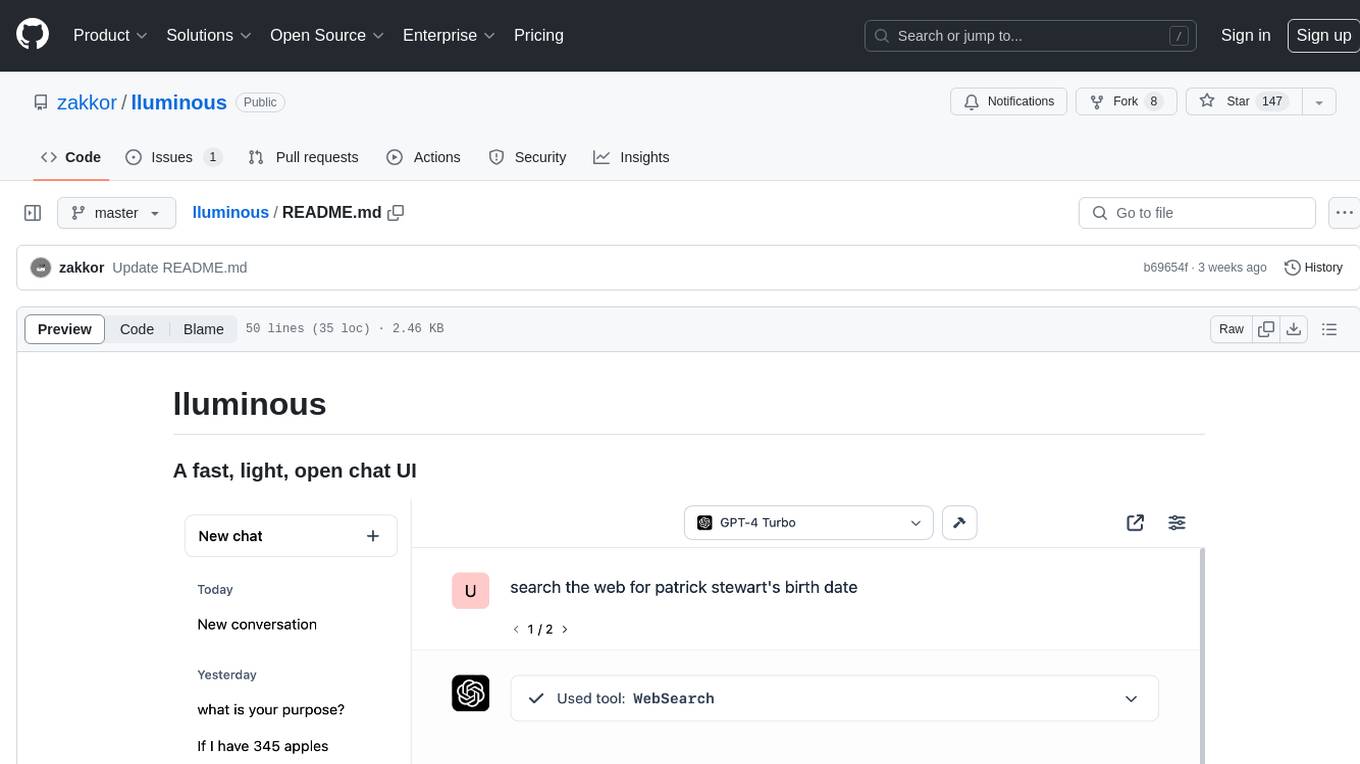
lluminous
lluminous is a fast and light open chat UI that supports multiple providers such as OpenAI, Anthropic, and Groq models. Users can easily plug in their API keys locally to access various models for tasks like multimodal input, image generation, multi-shot prompting, pre-filled responses, and more. The tool ensures privacy by storing all conversation history and keys locally on the user's device. Coming soon features include memory tool, file ingestion/embedding, embeddings-based web search, and prompt templates.
For similar tasks
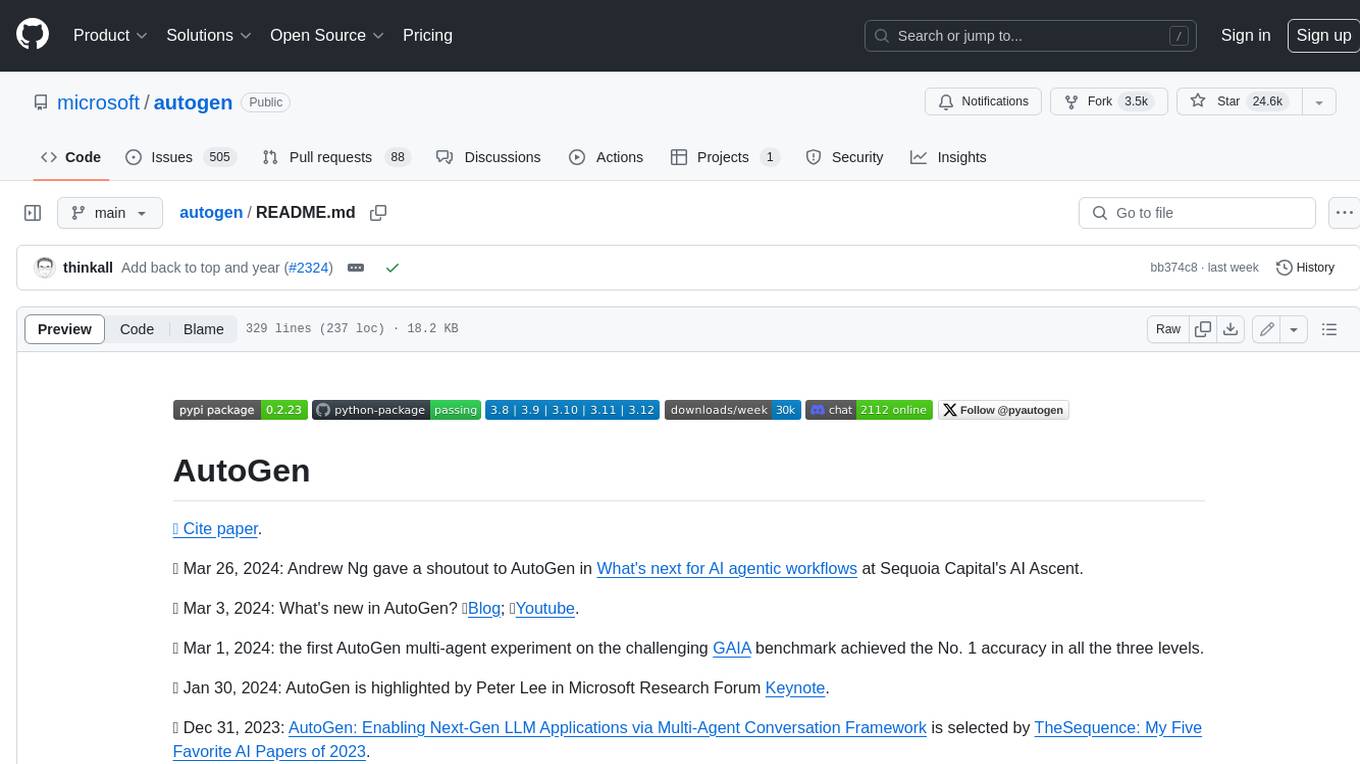
autogen
AutoGen is a framework that enables the development of LLM applications using multiple agents that can converse with each other to solve tasks. AutoGen agents are customizable, conversable, and seamlessly allow human participation. They can operate in various modes that employ combinations of LLMs, human inputs, and tools.
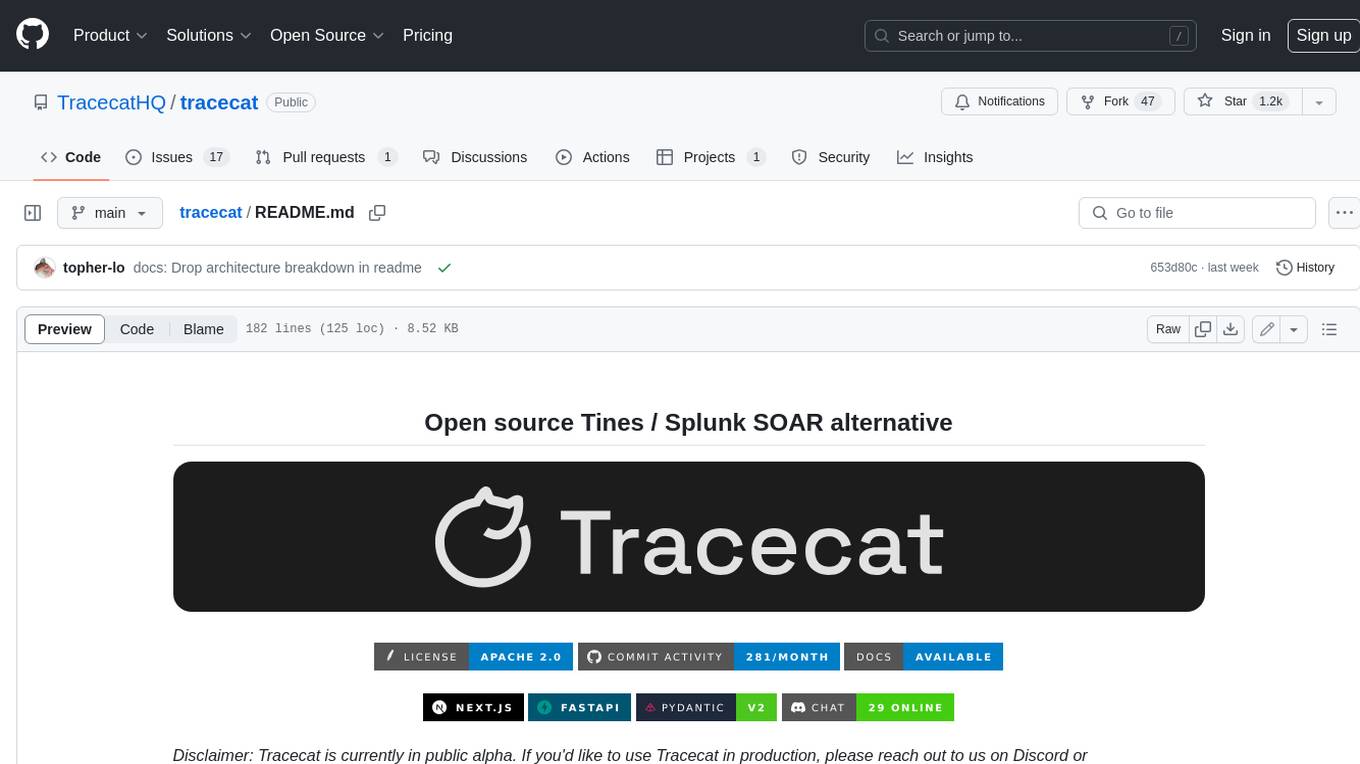
tracecat
Tracecat is an open-source automation platform for security teams. It's designed to be simple but powerful, with a focus on AI features and a practitioner-obsessed UI/UX. Tracecat can be used to automate a variety of tasks, including phishing email investigation, evidence collection, and remediation plan generation.
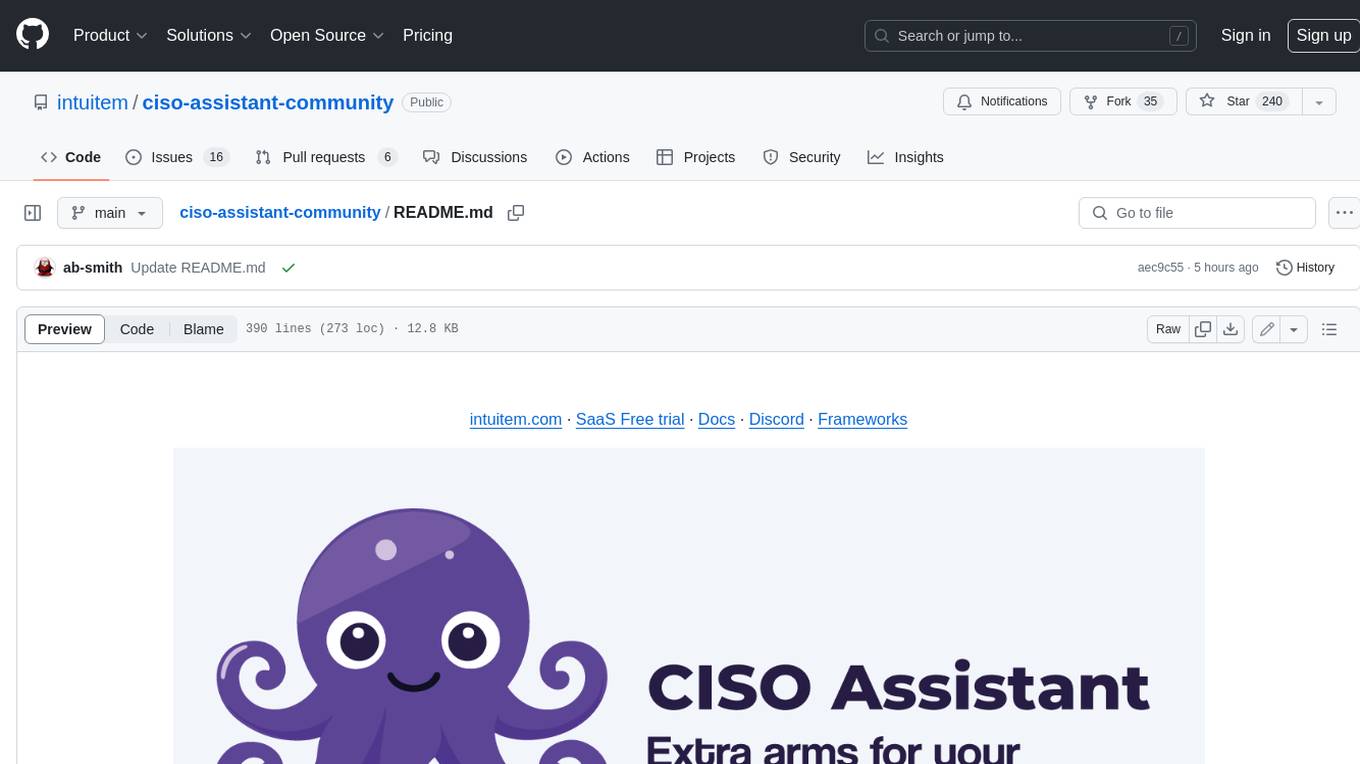
ciso-assistant-community
CISO Assistant is a tool that helps organizations manage their cybersecurity posture and compliance. It provides a centralized platform for managing security controls, threats, and risks. CISO Assistant also includes a library of pre-built frameworks and tools to help organizations quickly and easily implement best practices.
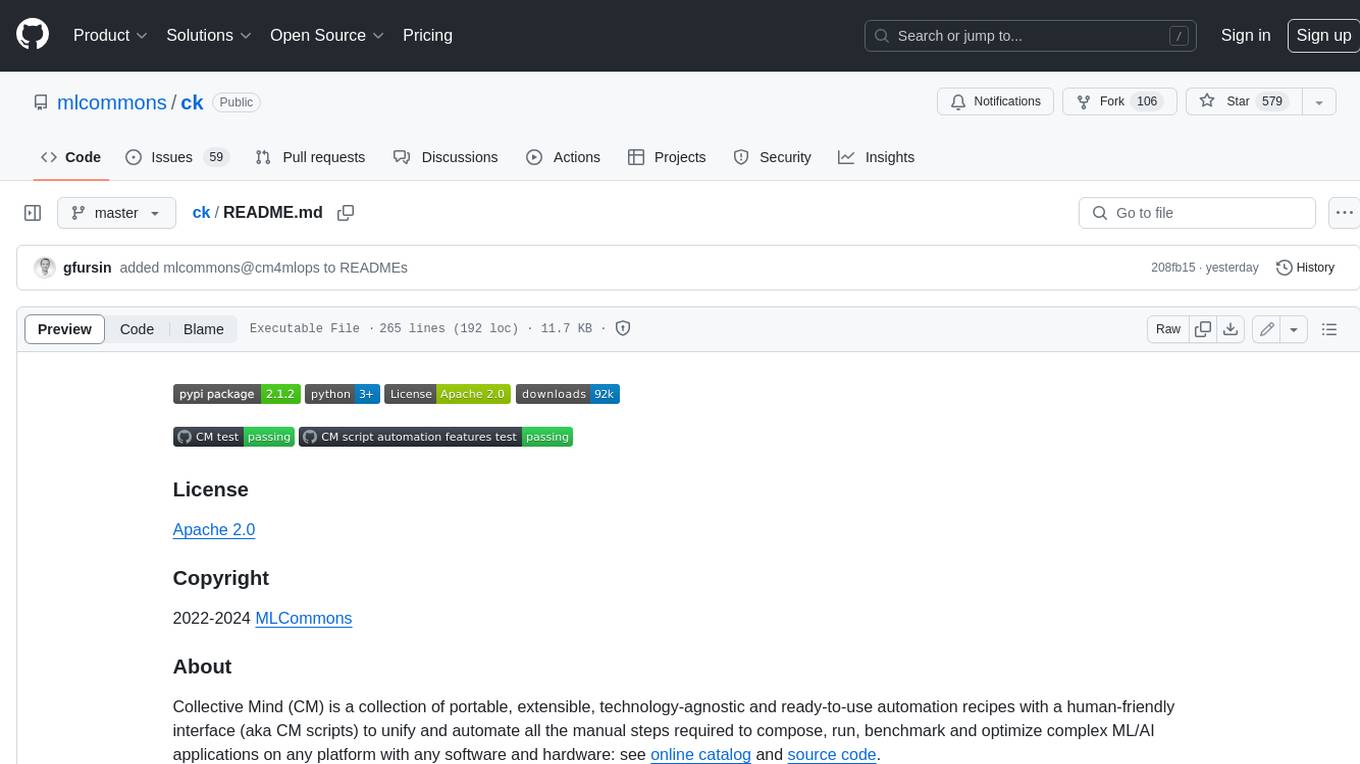
ck
Collective Mind (CM) is a collection of portable, extensible, technology-agnostic and ready-to-use automation recipes with a human-friendly interface (aka CM scripts) to unify and automate all the manual steps required to compose, run, benchmark and optimize complex ML/AI applications on any platform with any software and hardware: see online catalog and source code. CM scripts require Python 3.7+ with minimal dependencies and are continuously extended by the community and MLCommons members to run natively on Ubuntu, MacOS, Windows, RHEL, Debian, Amazon Linux and any other operating system, in a cloud or inside automatically generated containers while keeping backward compatibility - please don't hesitate to report encountered issues here and contact us via public Discord Server to help this collaborative engineering effort! CM scripts were originally developed based on the following requirements from the MLCommons members to help them automatically compose and optimize complex MLPerf benchmarks, applications and systems across diverse and continuously changing models, data sets, software and hardware from Nvidia, Intel, AMD, Google, Qualcomm, Amazon and other vendors: * must work out of the box with the default options and without the need to edit some paths, environment variables and configuration files; * must be non-intrusive, easy to debug and must reuse existing user scripts and automation tools (such as cmake, make, ML workflows, python poetry and containers) rather than substituting them; * must have a very simple and human-friendly command line with a Python API and minimal dependencies; * must require minimal or zero learning curve by using plain Python, native scripts, environment variables and simple JSON/YAML descriptions instead of inventing new workflow languages; * must have the same interface to run all automations natively, in a cloud or inside containers. CM scripts were successfully validated by MLCommons to modularize MLPerf inference benchmarks and help the community automate more than 95% of all performance and power submissions in the v3.1 round across more than 120 system configurations (models, frameworks, hardware) while reducing development and maintenance costs.
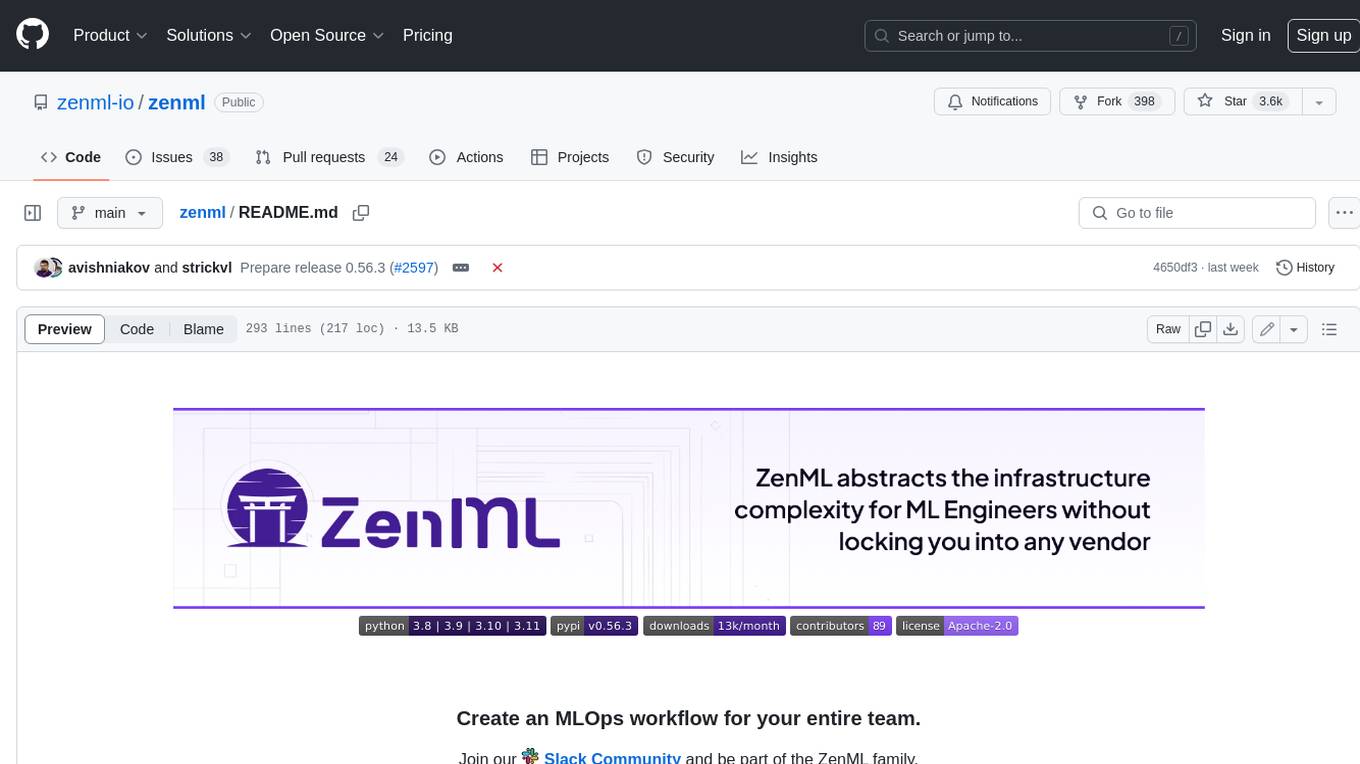
zenml
ZenML is an extensible, open-source MLOps framework for creating portable, production-ready machine learning pipelines. By decoupling infrastructure from code, ZenML enables developers across your organization to collaborate more effectively as they develop to production.
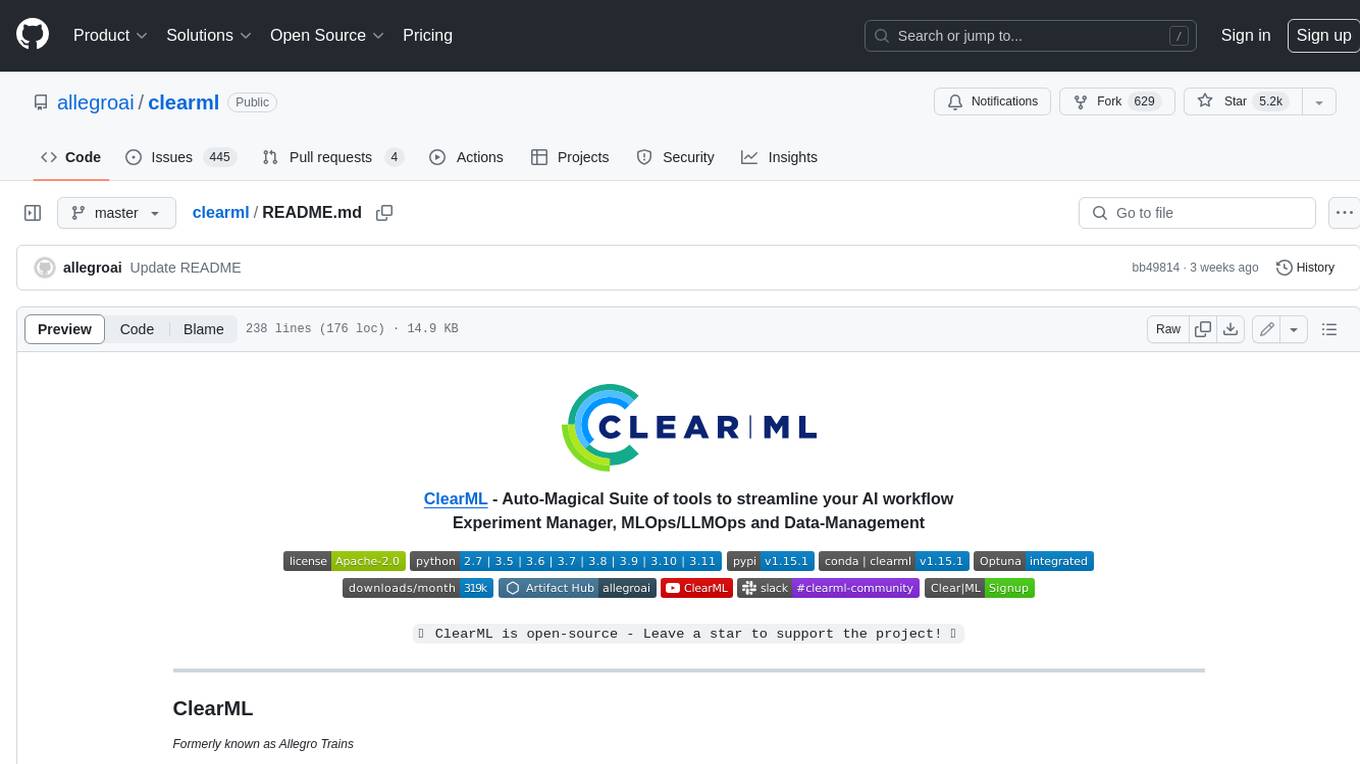
clearml
ClearML is a suite of tools designed to streamline the machine learning workflow. It includes an experiment manager, MLOps/LLMOps, data management, and model serving capabilities. ClearML is open-source and offers a free tier hosting option. It supports various ML/DL frameworks and integrates with Jupyter Notebook and PyCharm. ClearML provides extensive logging capabilities, including source control info, execution environment, hyper-parameters, and experiment outputs. It also offers automation features, such as remote job execution and pipeline creation. ClearML is designed to be easy to integrate, requiring only two lines of code to add to existing scripts. It aims to improve collaboration, visibility, and data transparency within ML teams.
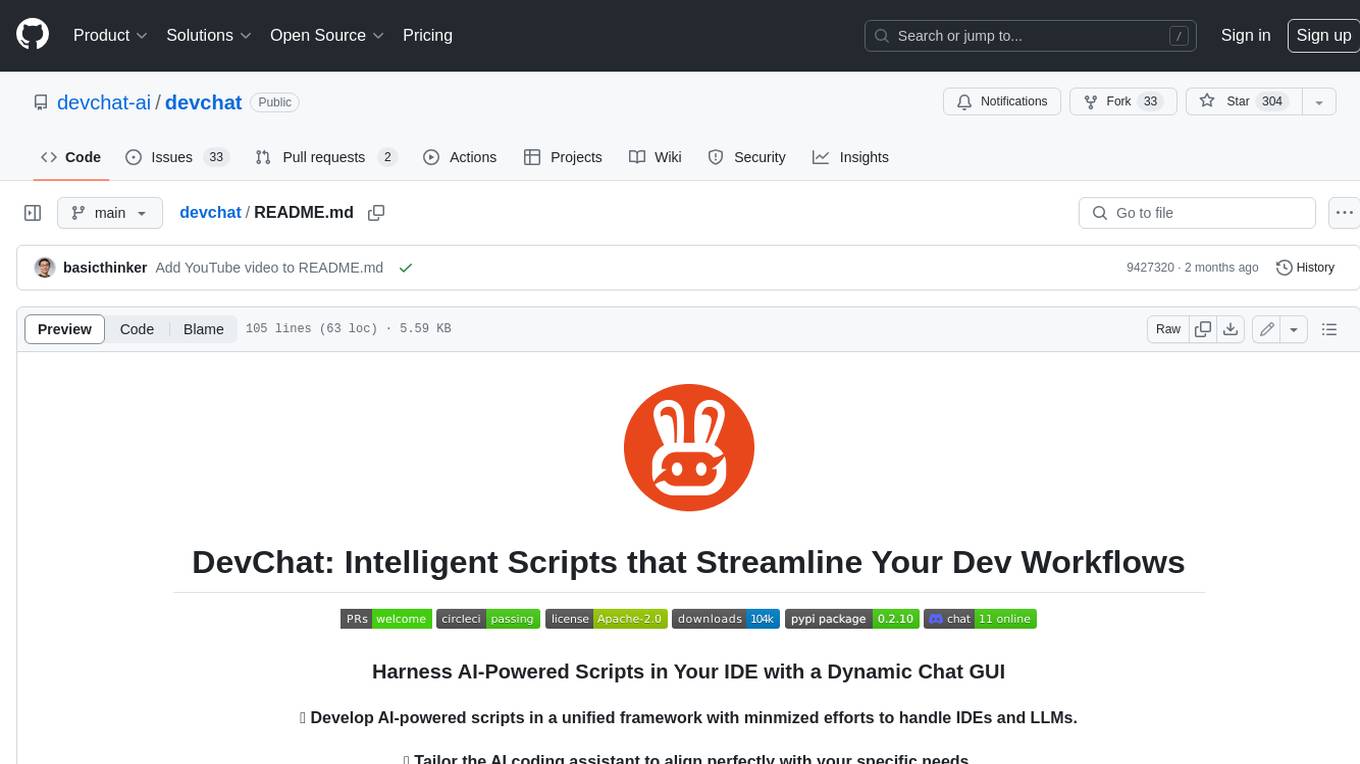
devchat
DevChat is an open-source workflow engine that enables developers to create intelligent, automated workflows for engaging with users through a chat panel within their IDEs. It combines script writing flexibility, latest AI models, and an intuitive chat GUI to enhance user experience and productivity. DevChat simplifies the integration of AI in software development, unlocking new possibilities for developers.
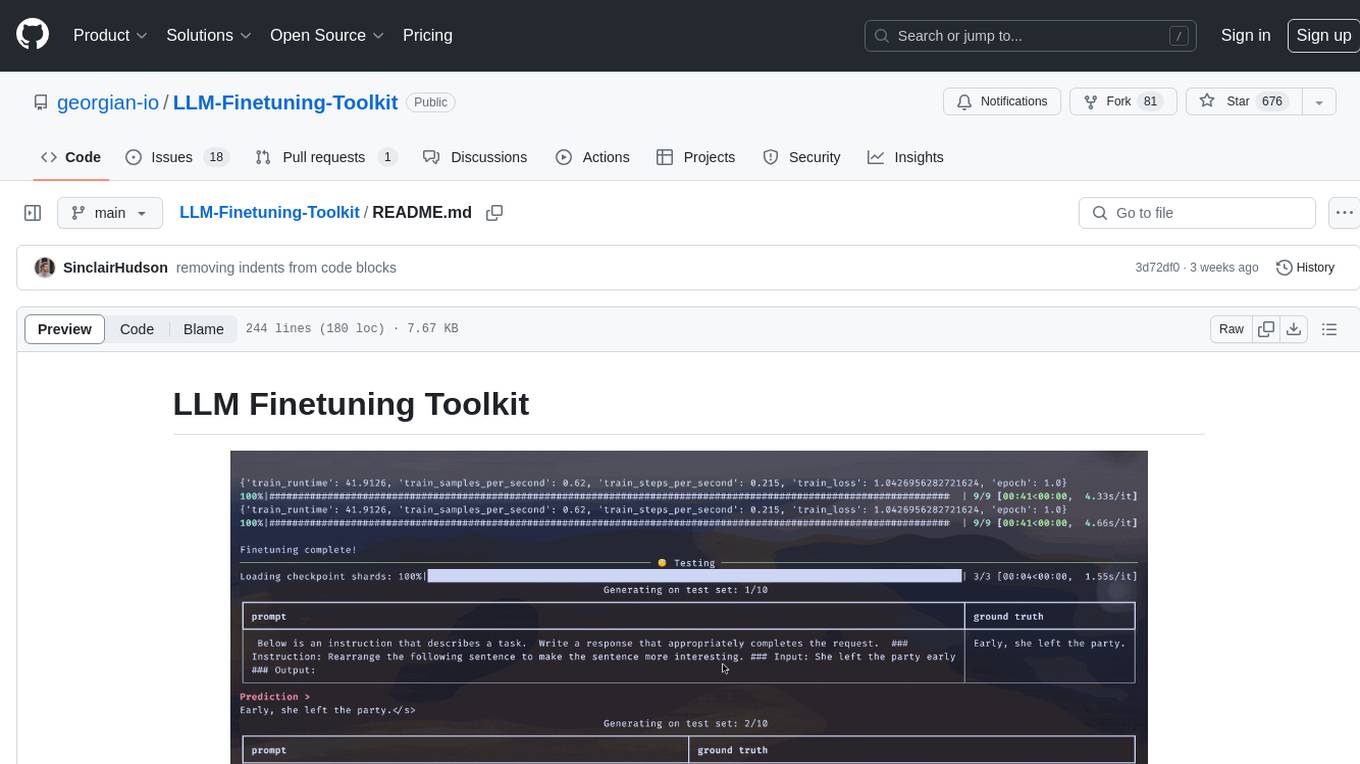
LLM-Finetuning-Toolkit
LLM Finetuning toolkit is a config-based CLI tool for launching a series of LLM fine-tuning experiments on your data and gathering their results. It allows users to control all elements of a typical experimentation pipeline - prompts, open-source LLMs, optimization strategy, and LLM testing - through a single YAML configuration file. The toolkit supports basic, intermediate, and advanced usage scenarios, enabling users to run custom experiments, conduct ablation studies, and automate fine-tuning workflows. It provides features for data ingestion, model definition, training, inference, quality assurance, and artifact outputs, making it a comprehensive tool for fine-tuning large language models.
For similar jobs
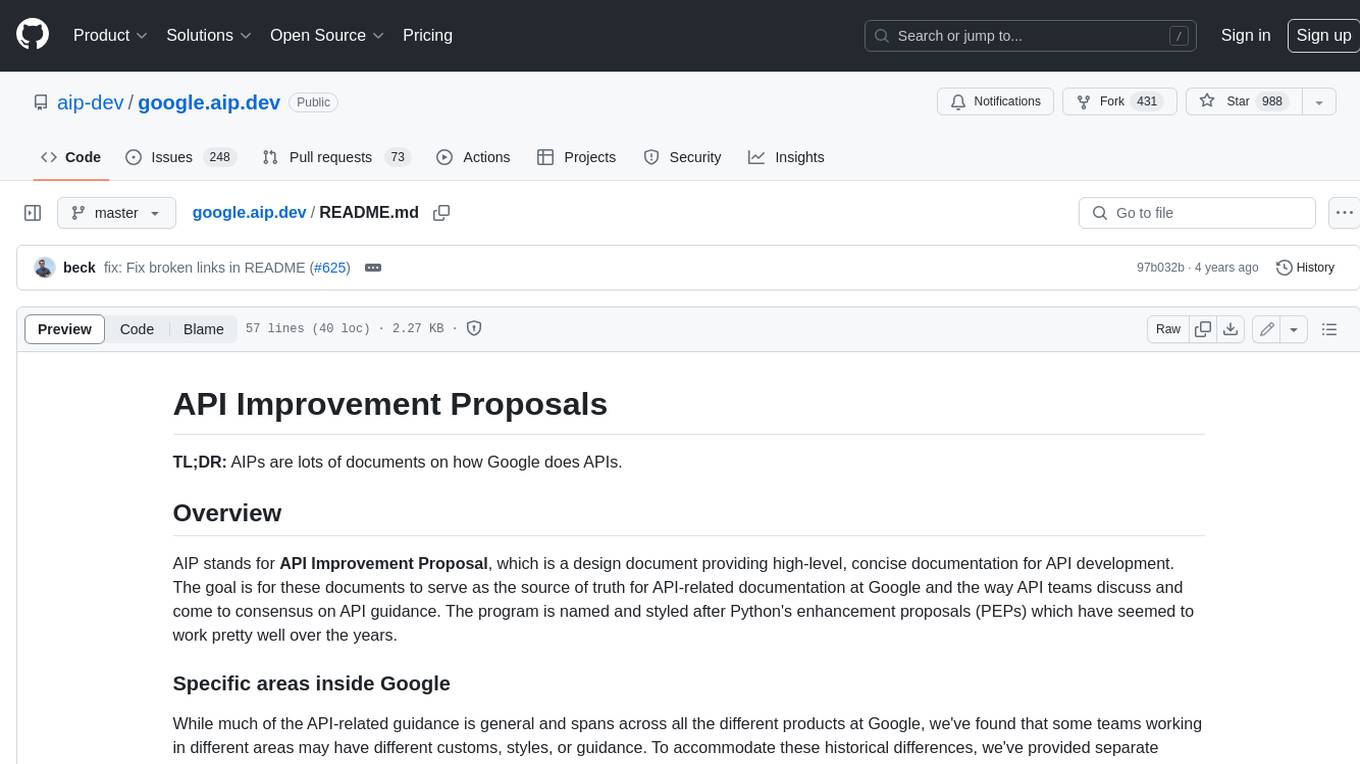
google.aip.dev
API Improvement Proposals (AIPs) are design documents that provide high-level, concise documentation for API development at Google. The goal of AIPs is to serve as the source of truth for API-related documentation and to facilitate discussion and consensus among API teams. AIPs are similar to Python's enhancement proposals (PEPs) and are organized into different areas within Google to accommodate historical differences in customs, styles, and guidance.
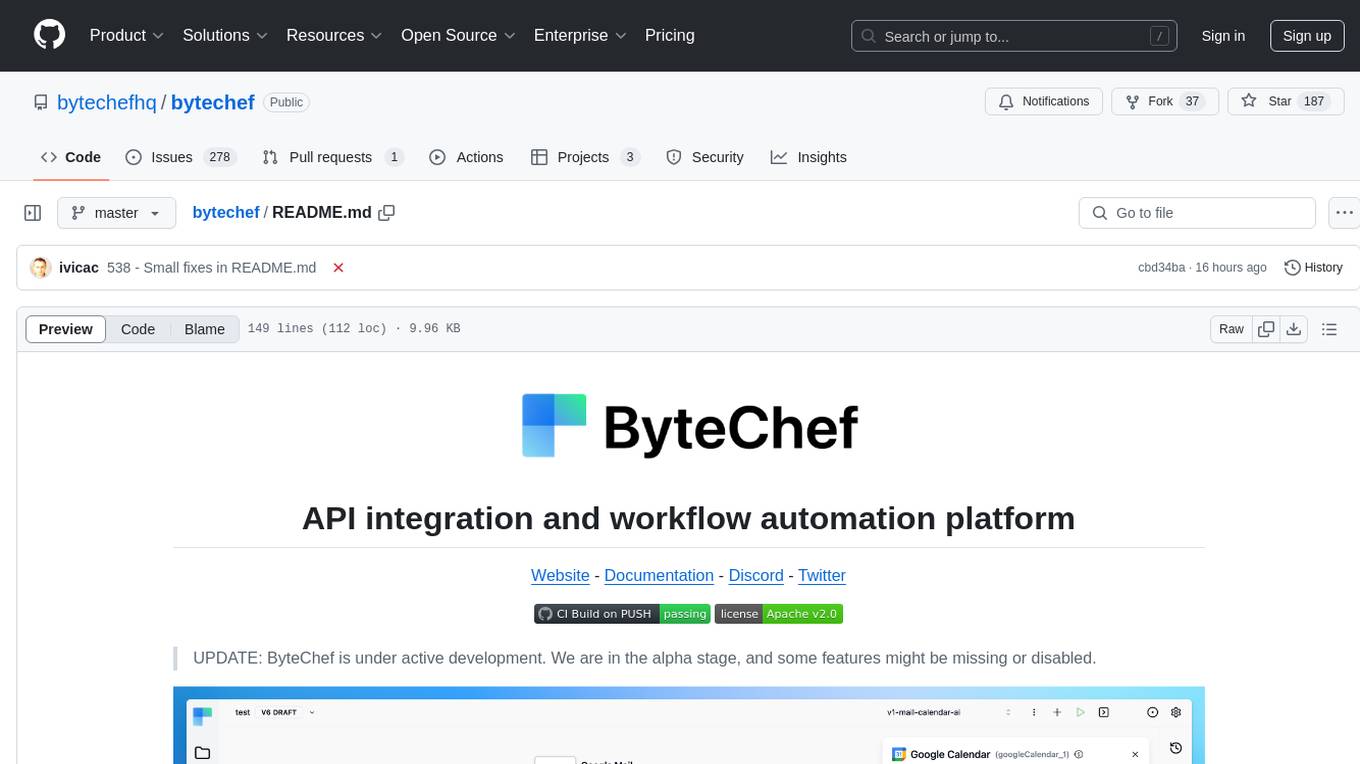
bytechef
ByteChef is an open-source, low-code, extendable API integration and workflow automation platform. It provides an intuitive UI Workflow Editor, event-driven & scheduled workflows, multiple flow controls, built-in code editor supporting Java, JavaScript, Python, and Ruby, rich component ecosystem, extendable with custom connectors, AI-ready with built-in AI components, developer-ready to expose workflows as APIs, version control friendly, self-hosted, scalable, and resilient. It allows users to build and visualize workflows, automate tasks across SaaS apps, internal APIs, and databases, and handle millions of workflows with high availability and fault tolerance.
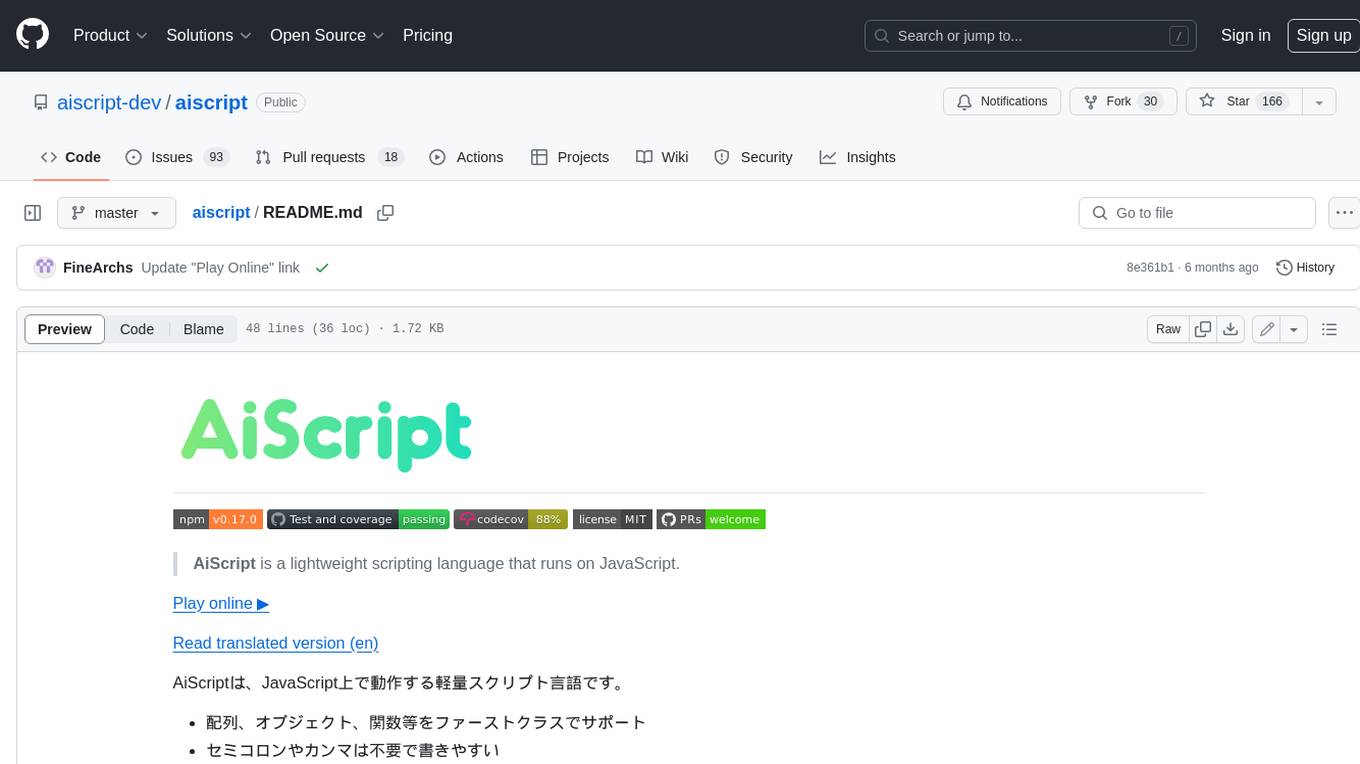
aiscript
AiScript is a lightweight scripting language that runs on JavaScript. It supports arrays, objects, and functions as first-class citizens, and is easy to write without the need for semicolons or commas. AiScript runs in a secure sandbox environment, preventing infinite loops from freezing the host. It also allows for easy provision of variables and functions from the host.
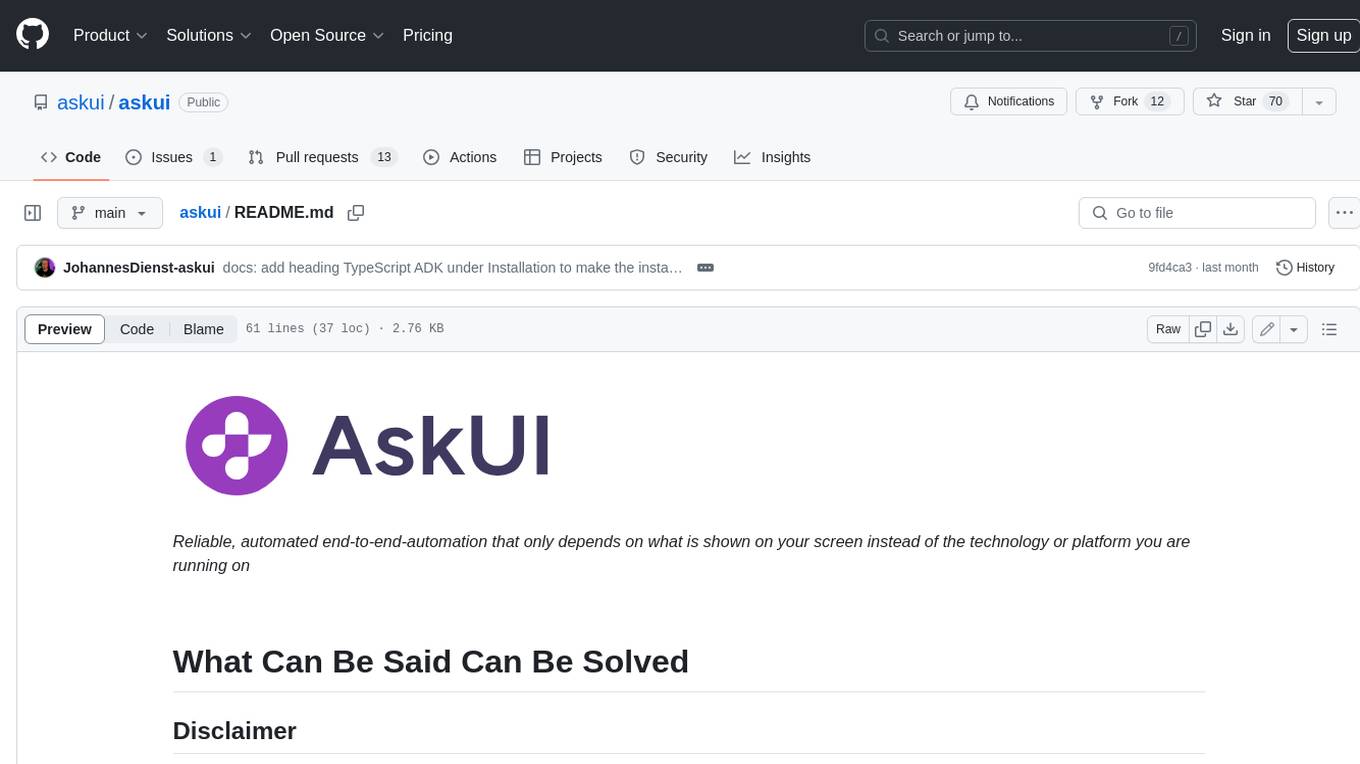
askui
AskUI is a reliable, automated end-to-end automation tool that only depends on what is shown on your screen instead of the technology or platform you are running on.
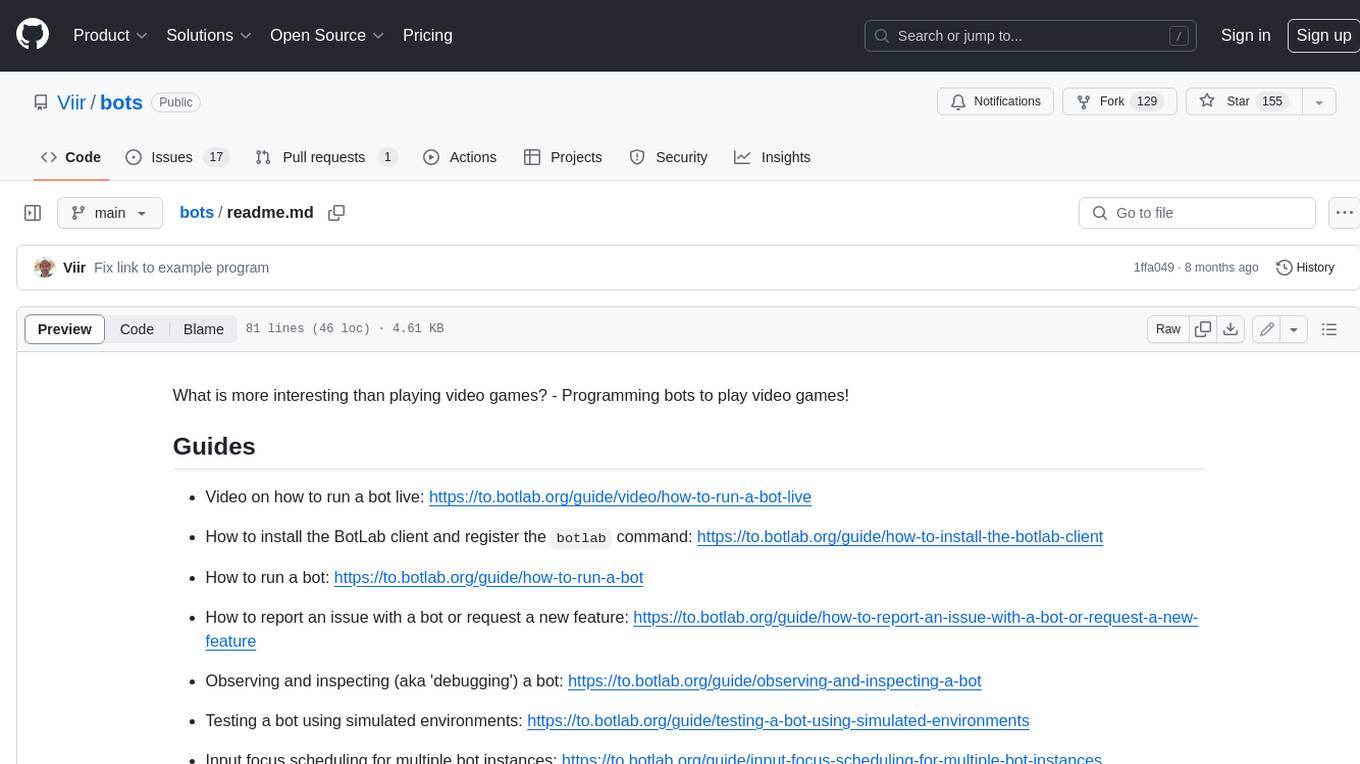
bots
The 'bots' repository is a collection of guides, tools, and example bots for programming bots to play video games. It provides resources on running bots live, installing the BotLab client, debugging bots, testing bots in simulated environments, and more. The repository also includes example bots for games like EVE Online, Tribal Wars 2, and Elvenar. Users can learn about developing bots for specific games, syntax of the Elm programming language, and tools for memory reading development. Additionally, there are guides on bot programming, contributing to BotLab, and exploring Elm syntax and core library.
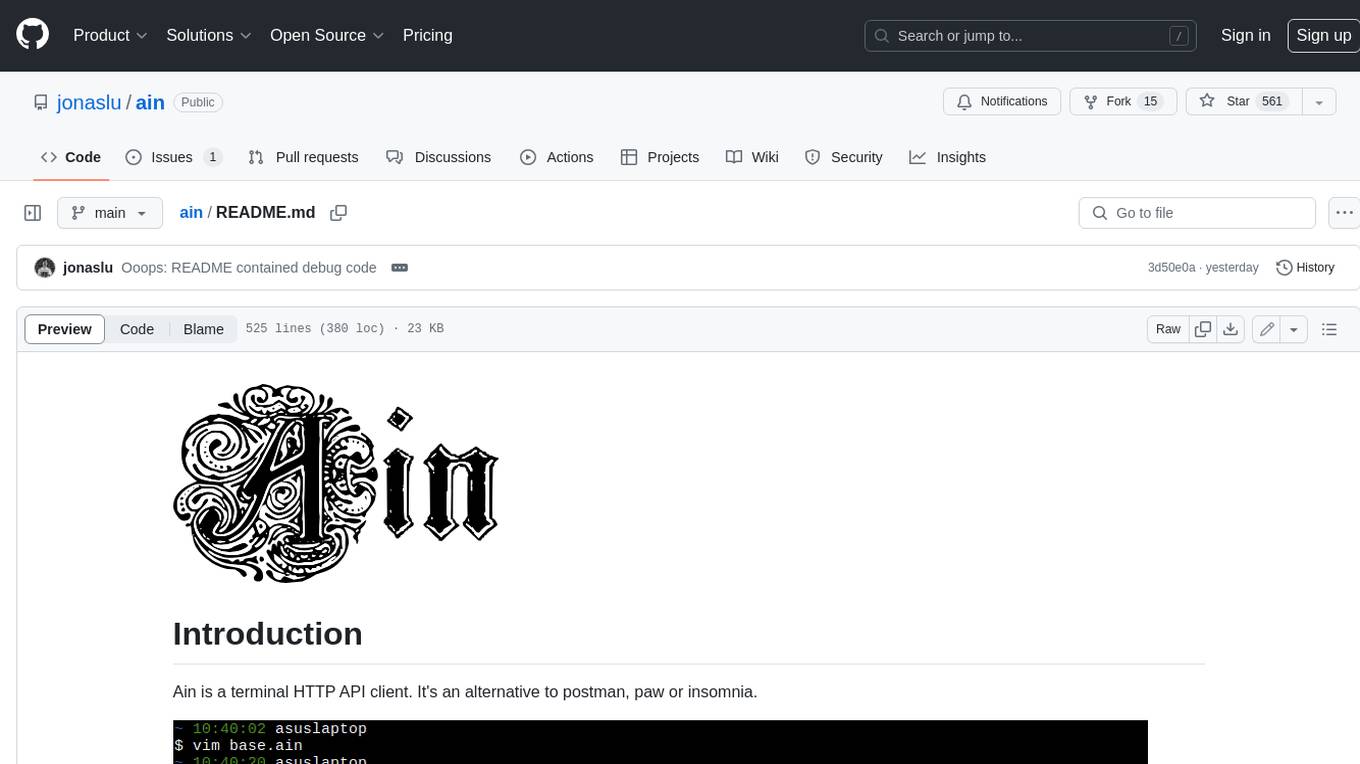
ain
Ain is a terminal HTTP API client designed for scripting input and processing output via pipes. It allows flexible organization of APIs using files and folders, supports shell-scripts and executables for common tasks, handles url-encoding, and enables sharing the resulting curl, wget, or httpie command-line. Users can put things that change in environment variables or .env-files, and pipe the API output for further processing. Ain targets users who work with many APIs using a simple file format and uses curl, wget, or httpie to make the actual calls.
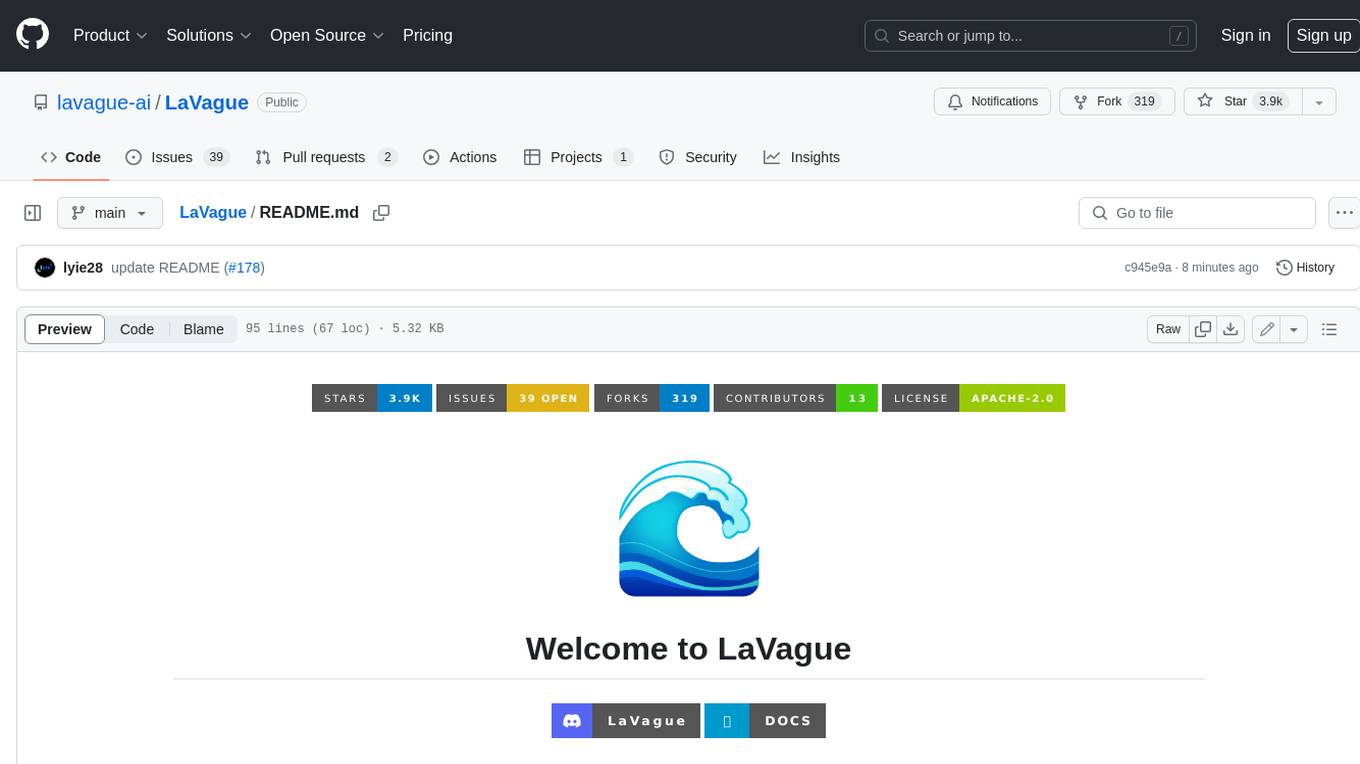
LaVague
LaVague is an open-source Large Action Model framework that uses advanced AI techniques to compile natural language instructions into browser automation code. It leverages Selenium or Playwright for browser actions. Users can interact with LaVague through an interactive Gradio interface to automate web interactions. The tool requires an OpenAI API key for default examples and offers a Playwright integration guide. Contributors can help by working on outlined tasks, submitting PRs, and engaging with the community on Discord. The project roadmap is available to track progress, but users should exercise caution when executing LLM-generated code using 'exec'.
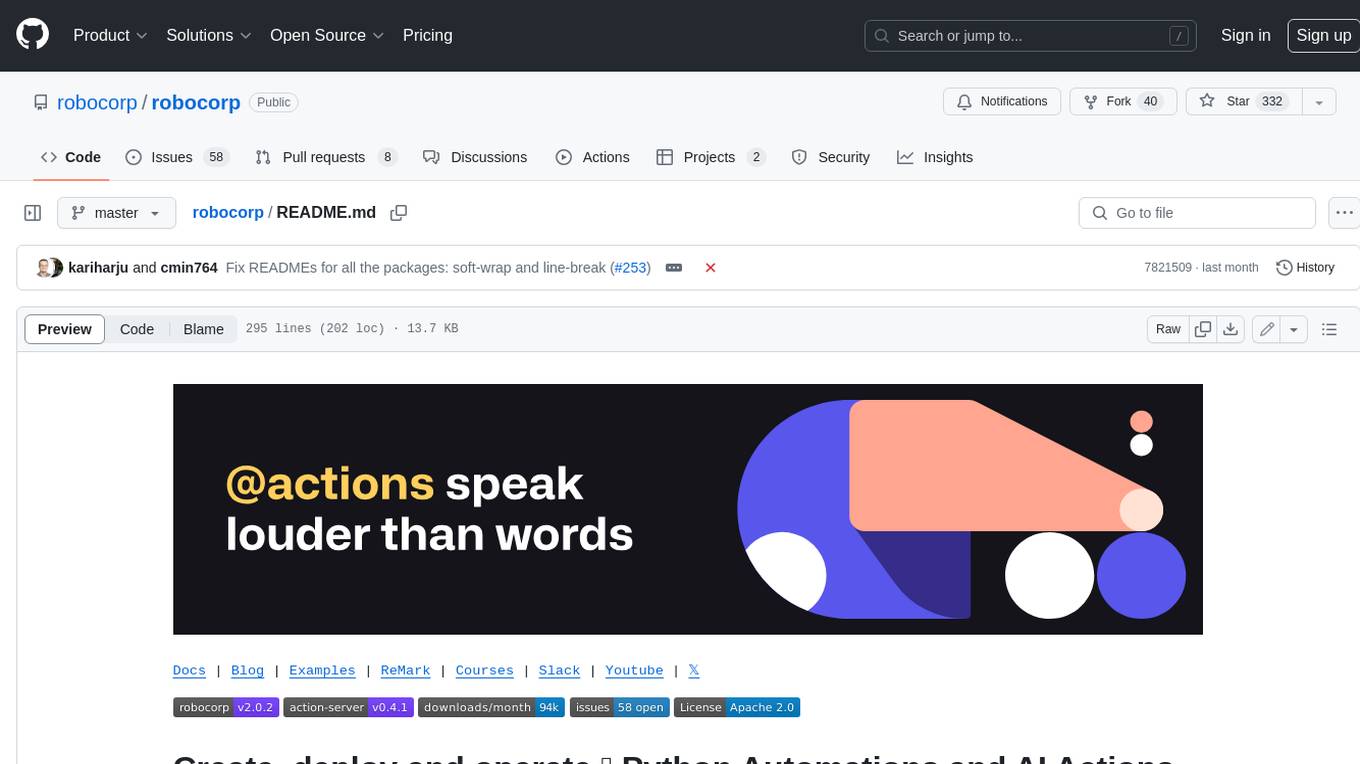
robocorp
Robocorp is a platform that allows users to create, deploy, and operate Python automations and AI actions. It provides an easy way to extend the capabilities of AI agents, assistants, and copilots with custom actions written in Python. Users can create and deploy tools, skills, loaders, and plugins that securely connect any AI Assistant platform to their data and applications. The Robocorp Action Server makes Python scripts compatible with ChatGPT and LangChain by automatically creating and exposing an API based on function declaration, type hints, and docstrings. It simplifies the process of developing and deploying AI actions, enabling users to interact with AI frameworks effortlessly.