Best AI tools for< Train New Boo Ai >
20 - AI tool Sites
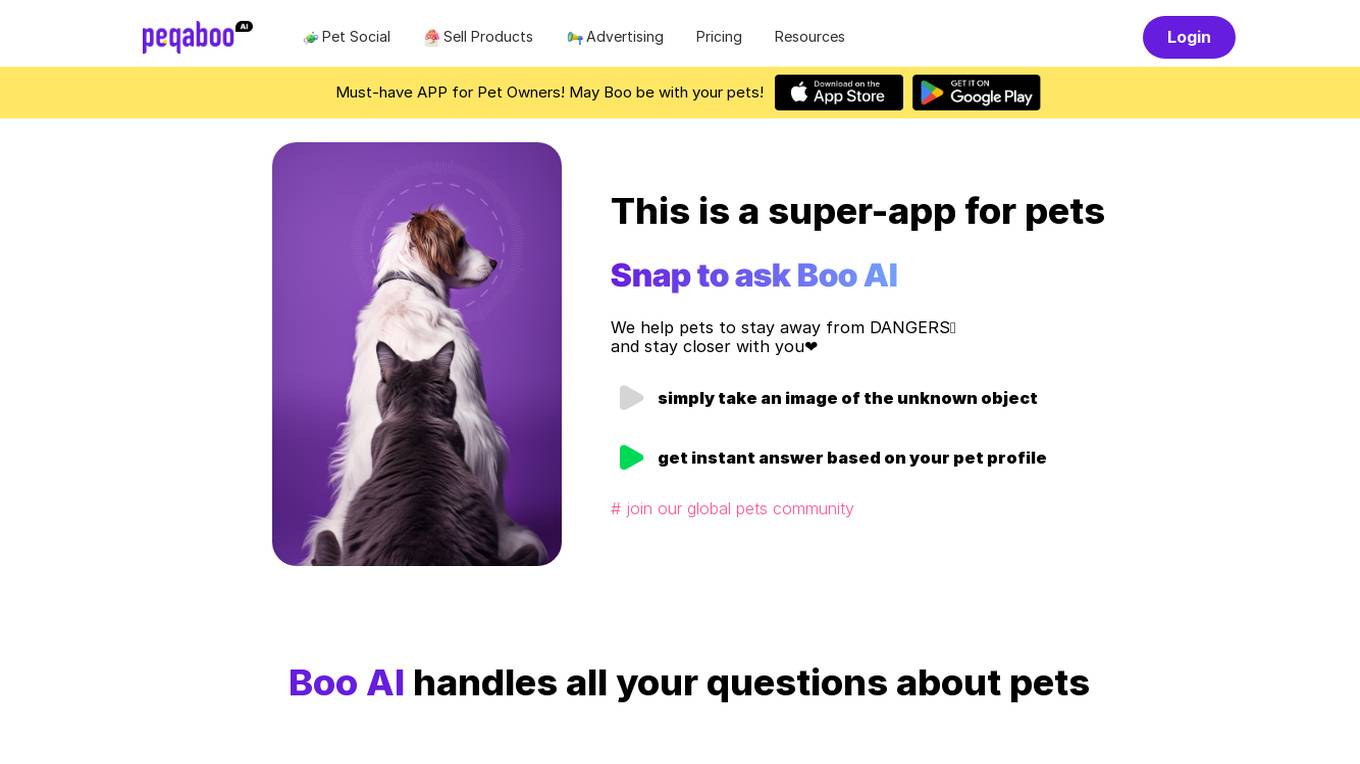
Peqaboo
Peqaboo is an AI-powered pet social app designed to help pet owners with various aspects of pet care. The app allows users to ask Boo AI questions about their pets, identify toxic plants or foods, and receive instant answers based on their pet's profile. Peqaboo also offers a feature to train a new Boo AI, enabling users to transform their knowledge into AI tools. The app aims to make pet life easier and more enjoyable by providing personalized pet care advice and fostering a global pet community.
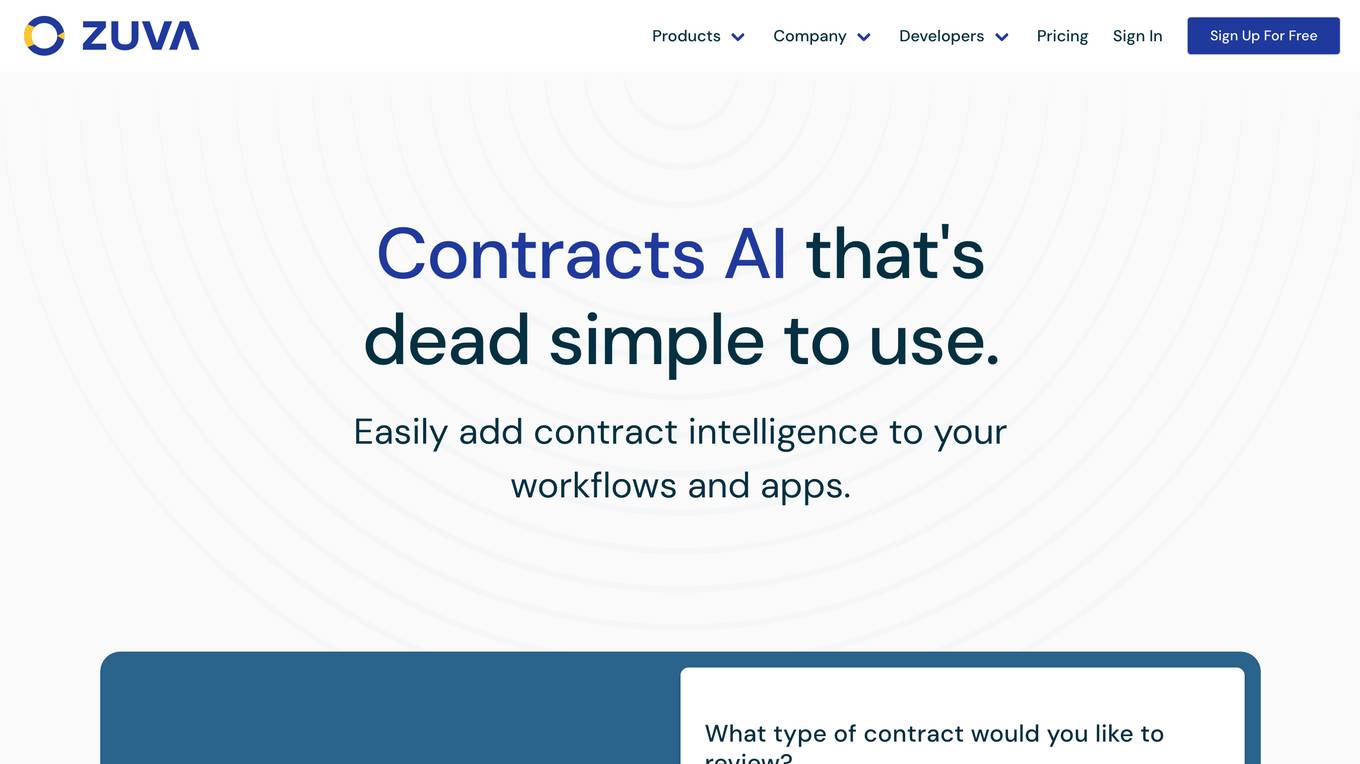
DocAI
DocAI is an API-driven platform that enables you to implement contracts AI into your applications, without requiring development from the ground-up. Our AI identifies and extracts 1,300+ common legal clauses, provisions and data points from a variety of document types. Our AI is a low-code experience for all. Easily train new fields without the need for a data scientist. All you need is subject matter expertise. Flexible and scalable. Flexible deployment options in the Zuva hosted cloud or on prem, across multiple geographical regions. Reliable, expert-built AI our customers can trust. Over 1,300+ out of the box AI fields that are built and trained by experienced lawyers and subject matter experts. Fields identify and extract common legal clauses, provisions and data points from unstructured documents and contracts, including ones written in non-standard language.
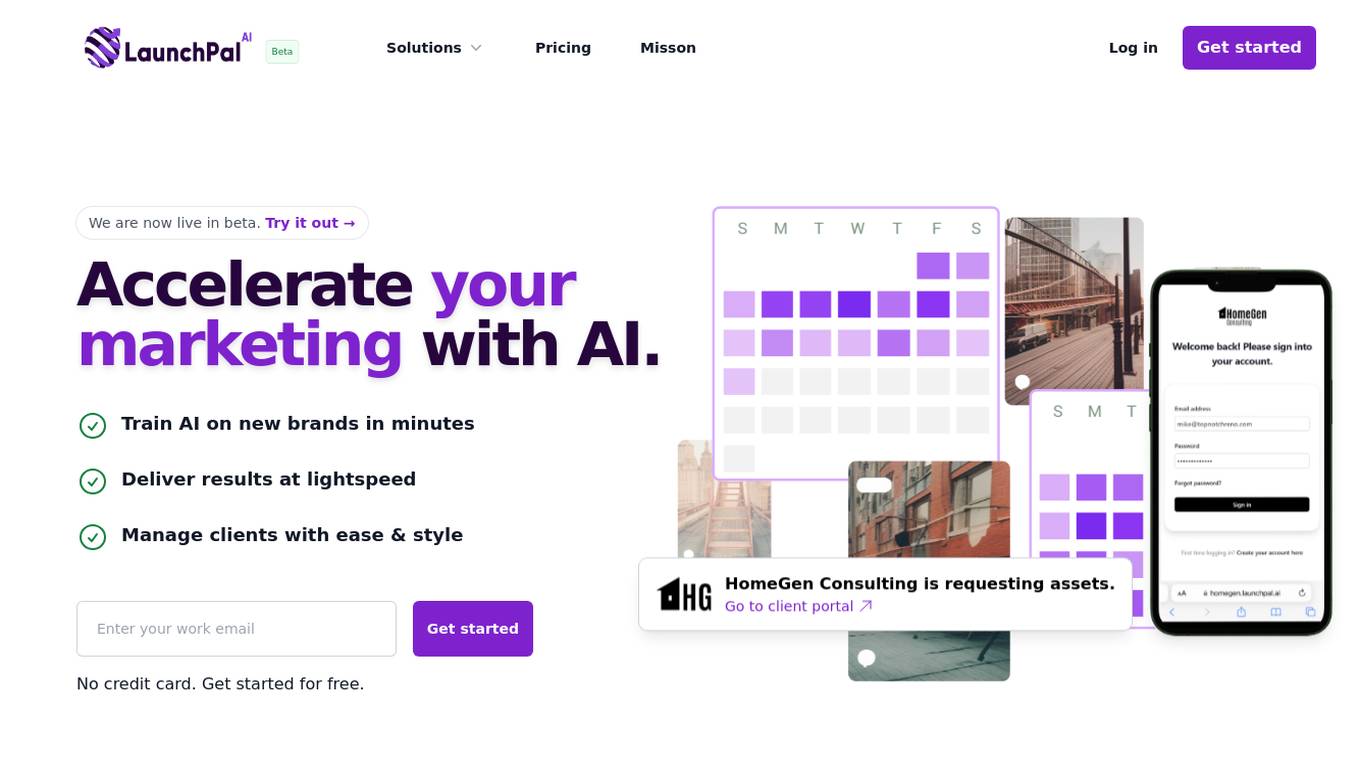
LaunchPal
LaunchPal is an AI-powered marketing tool designed to streamline and enhance the marketing workflow for individuals, agencies, and enterprises. It enables users to train AI on new brands in minutes, deliver results quickly, manage clients efficiently, and improve content delivery over time. LaunchPal offers features such as effortless setup, content engine, client portal, pricing plans tailored for different user needs, and a user-friendly interface for a seamless experience.
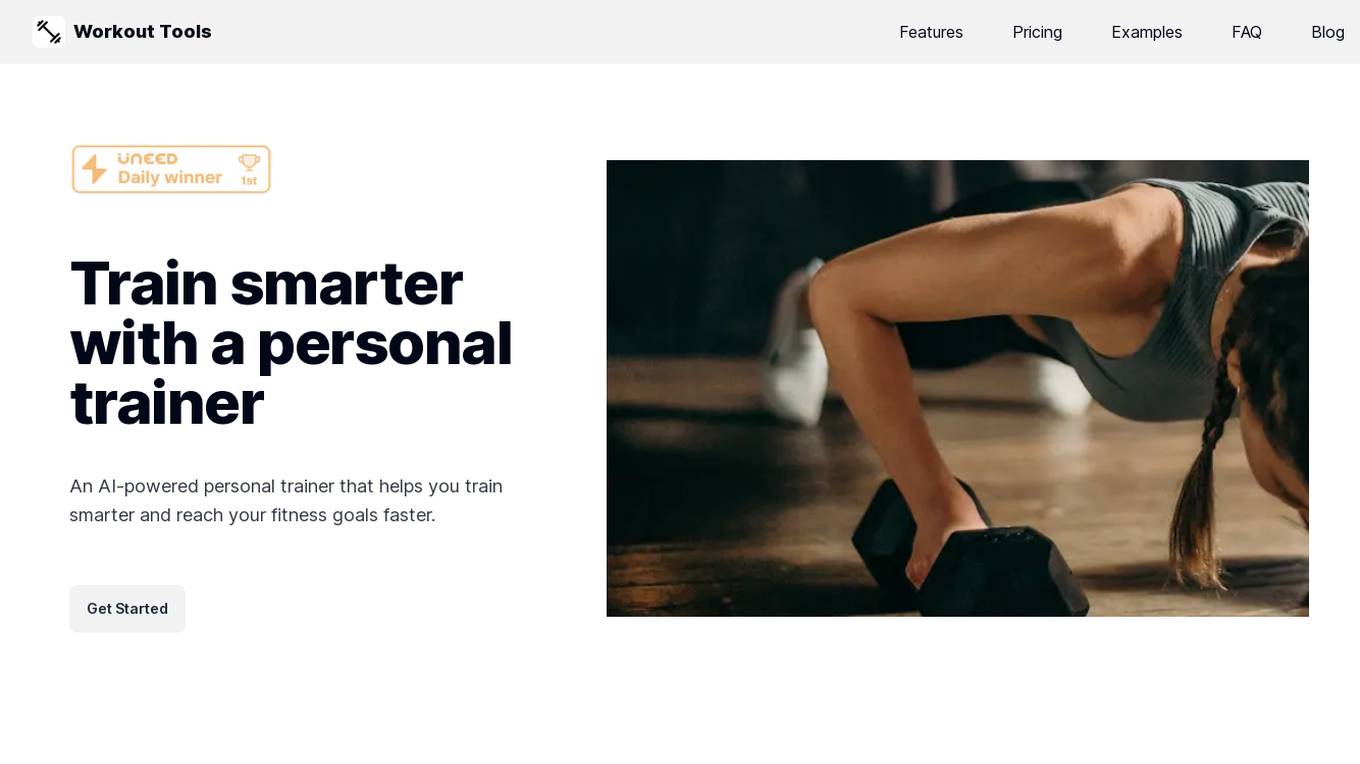
Workout Tools
Workout Tools is an AI-powered personal trainer that helps you train smarter and reach your fitness goals faster. It takes into account different parameters, such as your physics, the type of workout you're interested in, your available equipment, and comes up with a suggested workout. Don't like the workout? Just generate another one. It's that simple.
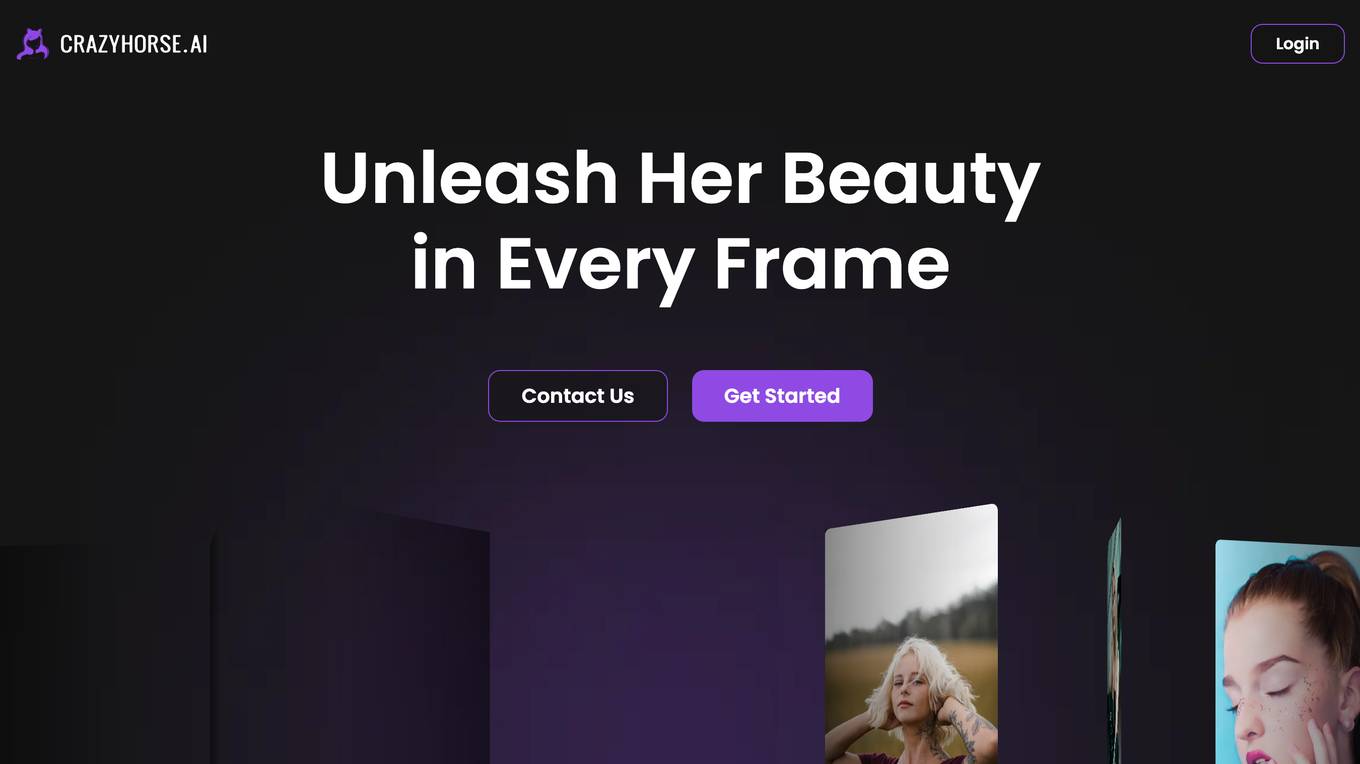
CrazyHorseAI
CrazyHorseAI is an AI tool that offers an API for users to enhance and customize the appearance and personality traits of an AI girl through features like changing clothes, hair, body, pose, and background. The tool provides functionalities such as natural language processing, emotional intelligence, and adaptive learning capabilities to create immersive and engaging experiences.
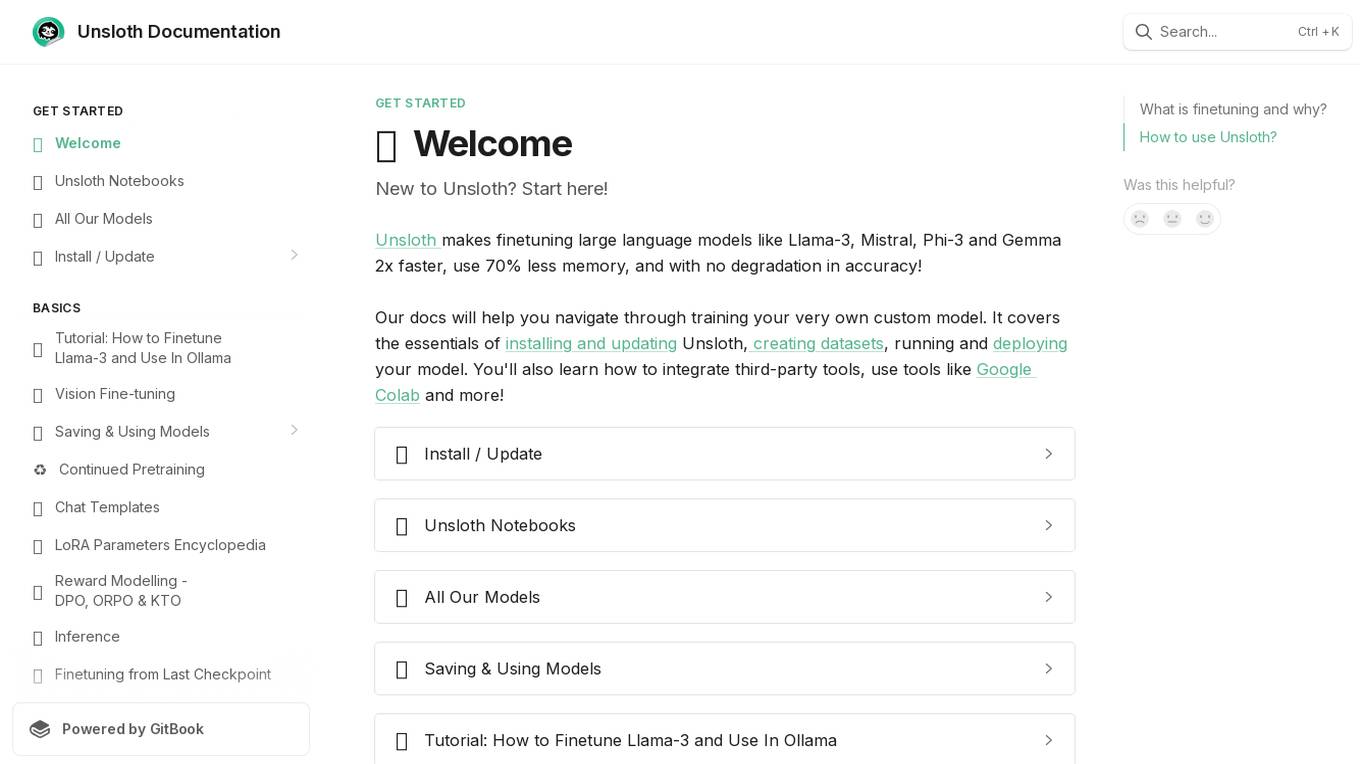
Unsloth
Unsloth is an AI tool designed to make finetuning large language models like Llama-3, Mistral, Phi-3, and Gemma 2x faster, use 70% less memory, and with no degradation in accuracy. The tool provides documentation to help users navigate through training their custom models, covering essentials such as installing and updating Unsloth, creating datasets, running, and deploying models. Users can also integrate third-party tools and utilize platforms like Google Colab.
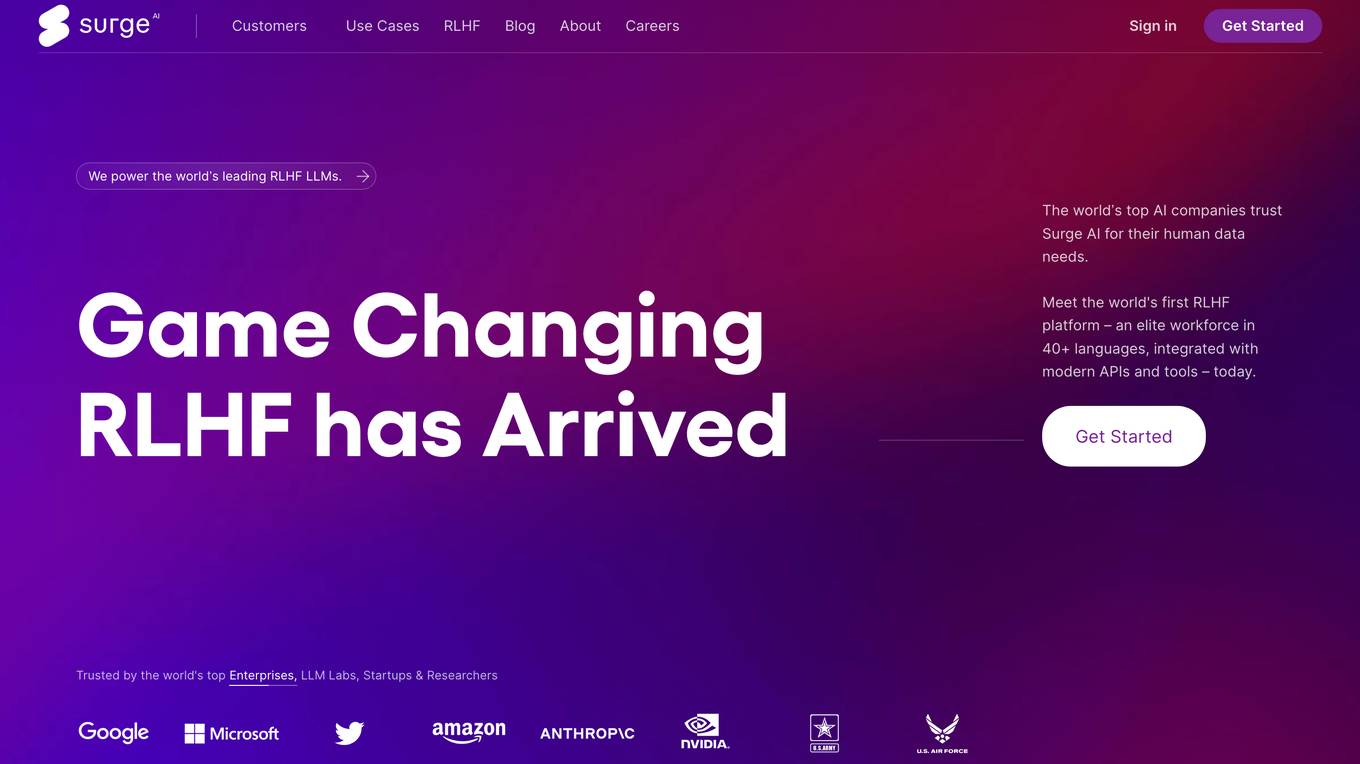
Surge AI
Surge AI is a data labeling platform that provides human-generated data for training and evaluating large language models (LLMs). It offers a global workforce of annotators who can label data in over 40 languages. Surge AI's platform is designed to be easy to use and integrates with popular machine learning tools and frameworks. The company's customers include leading AI companies, research labs, and startups.
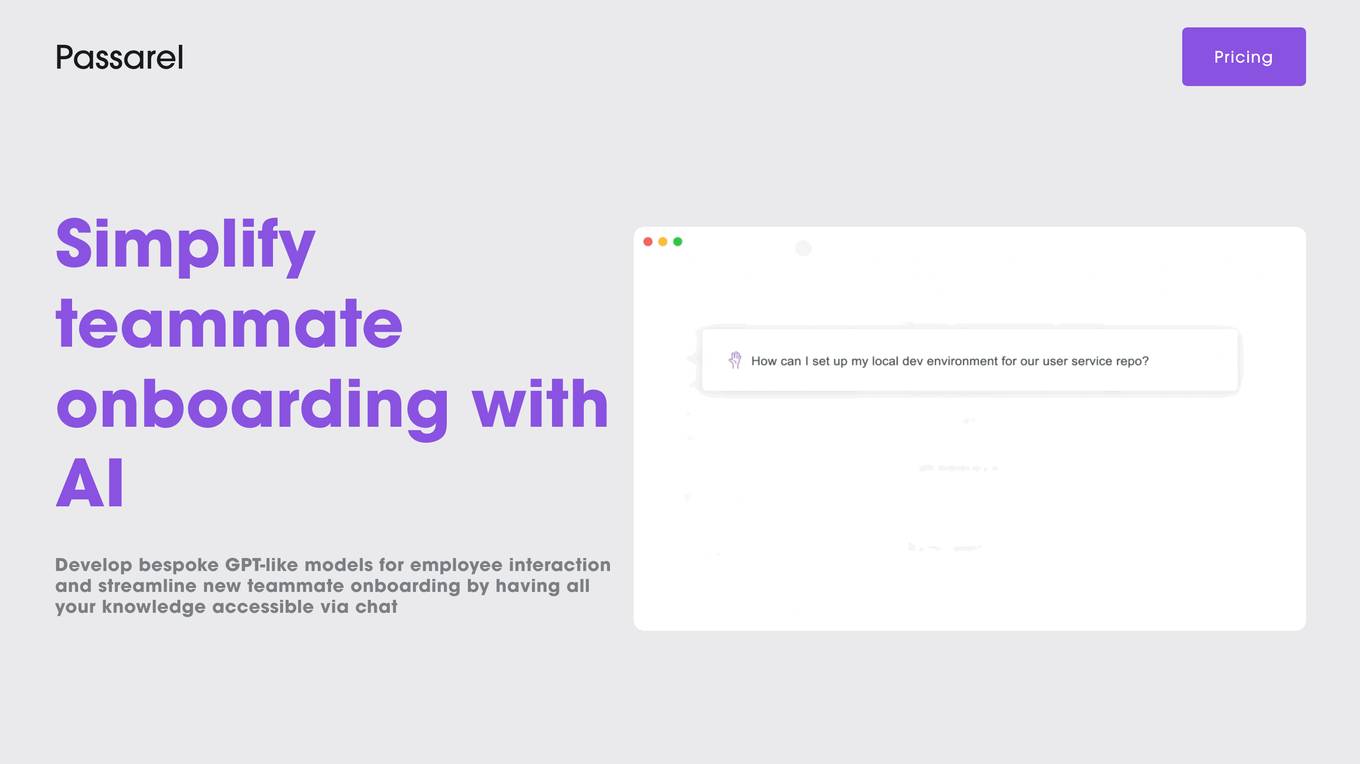
Passarel
Passarel is an AI tool designed to simplify teammate onboarding by developing bespoke GPT-like models for employee interaction. It centralizes knowledge bases into a custom model, allowing new teammates to access information efficiently. Passarel leverages various integrations to tailor language models to team needs, handling contradictions and providing accurate information. The tool works by training models on chosen knowledge bases, learning from data and configurations provided, and deploying the model for team use.
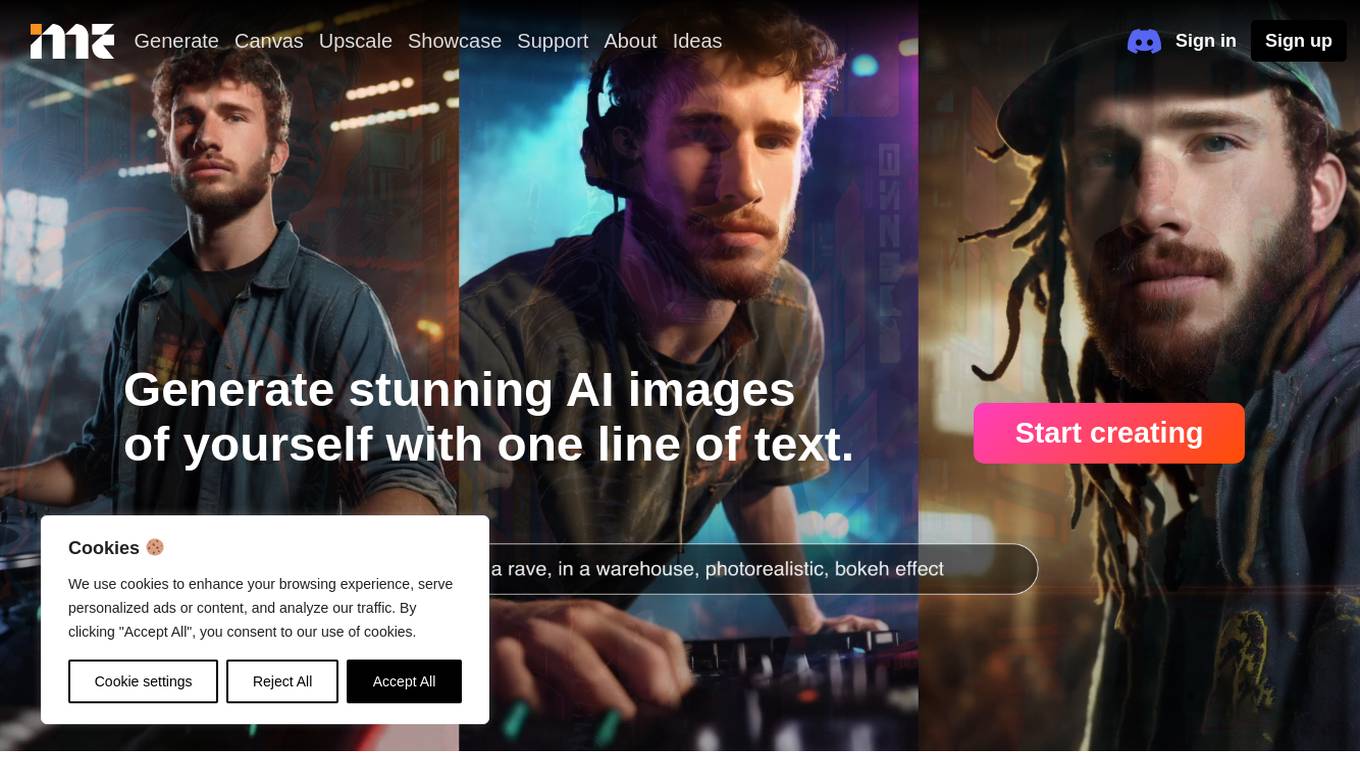
ImagineMe
ImagineMe is a personal AI art generator that allows users to create stunning art of themselves from a simple text description. The application uses AI models to convert text into corresponding images, enabling users to visualize themselves in various scenarios. ImagineMe offers an easy, affordable, and magical way to create personalized art.
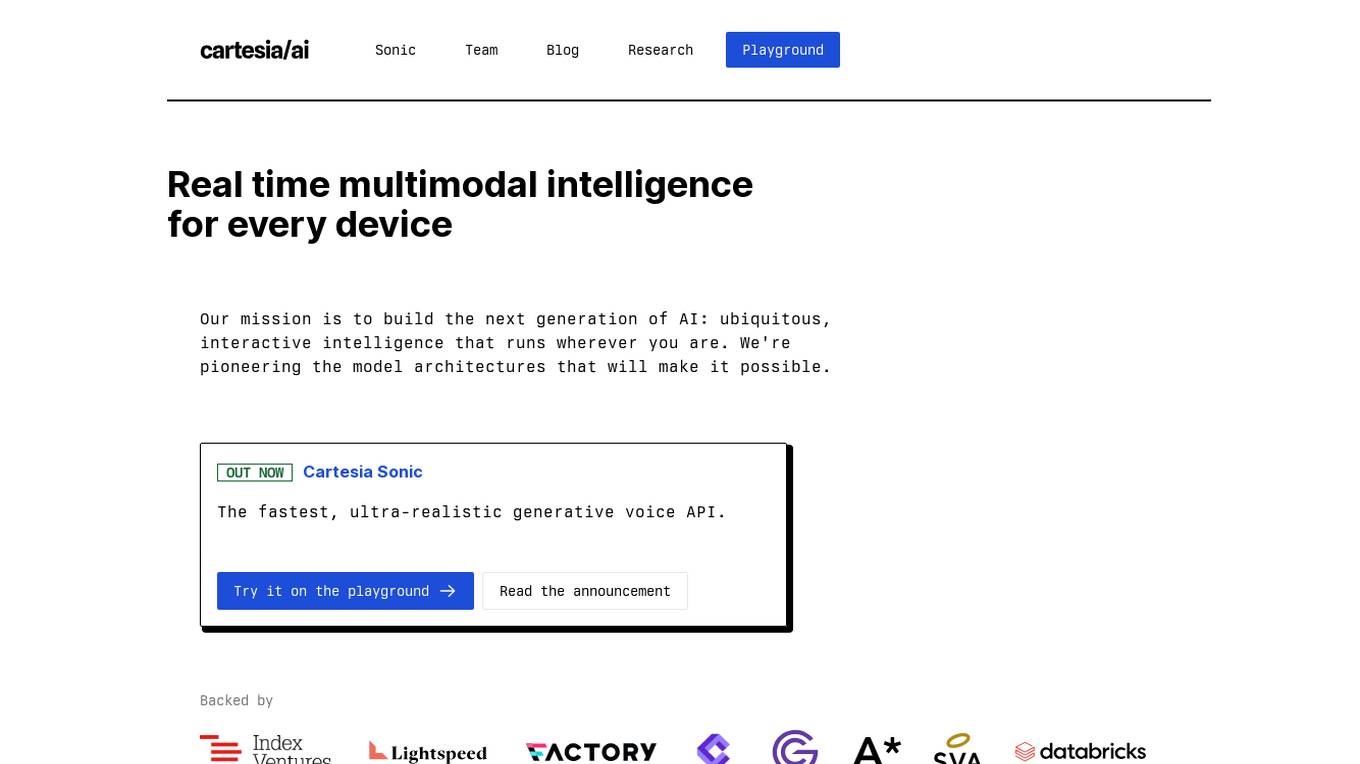
Cartesia Sonic Team Blog Research Playground
Cartesia Sonic Team Blog Research Playground is an AI application that offers real-time multimodal intelligence for every device. The application aims to build the next generation of AI by providing ubiquitous, interactive intelligence that can run on any device. It features the fastest, ultra-realistic generative voice API and is backed by research on simple linear attention language models and state-space models. The founding team, who met at the Stanford AI Lab, has invented State Space Models (SSMs) and scaled it up to achieve state-of-the-art results in various modalities such as text, audio, video, images, and time-series data.
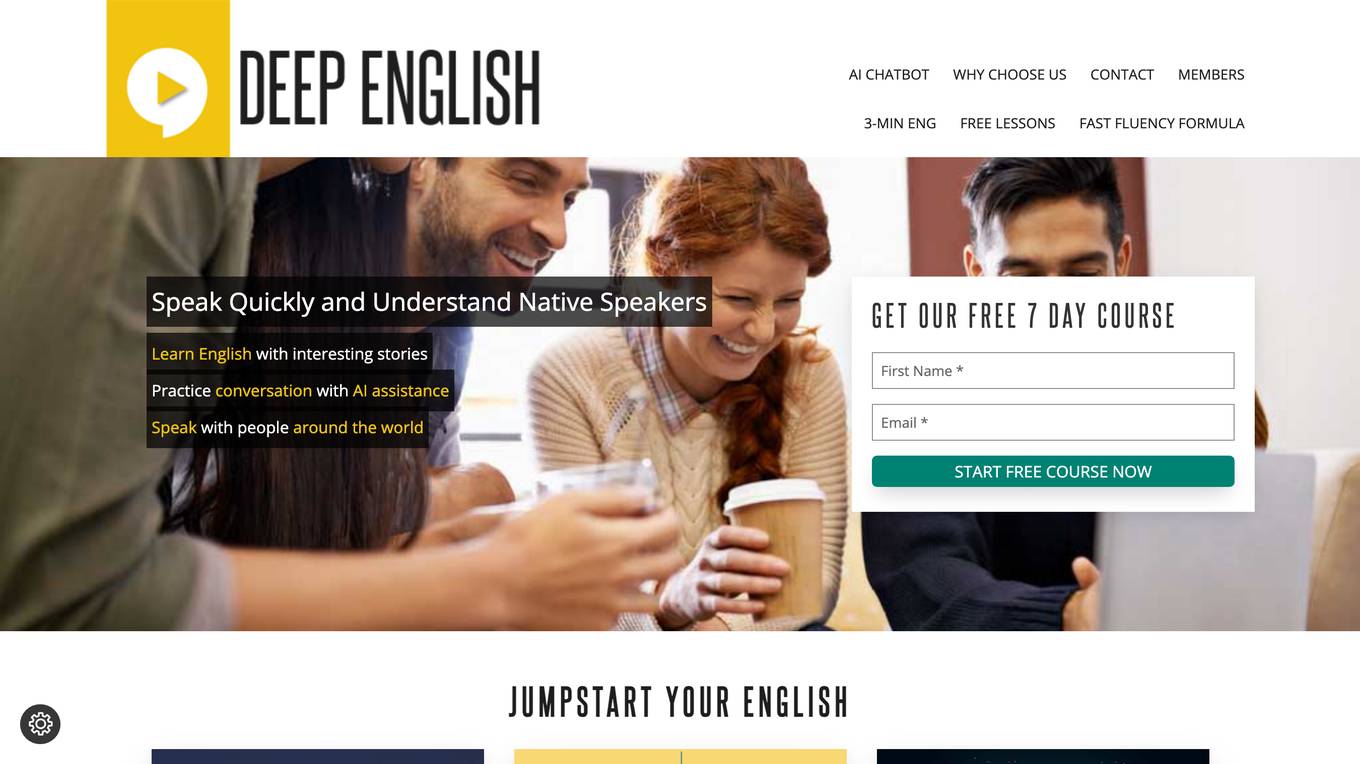
Deep English
Deep English is an AI chatbot application designed to help users improve their English language skills through interactive lessons, practice conversations with AI assistance, and engaging storytelling. The platform offers free lessons, fast fluency formulas, and personalized vocabulary learning. Users can speak quickly, understand native speakers, and connect with a global community for 24/7 English practice. Deep English aims to boost users' confidence in speaking English fluently and understanding conversations effectively.
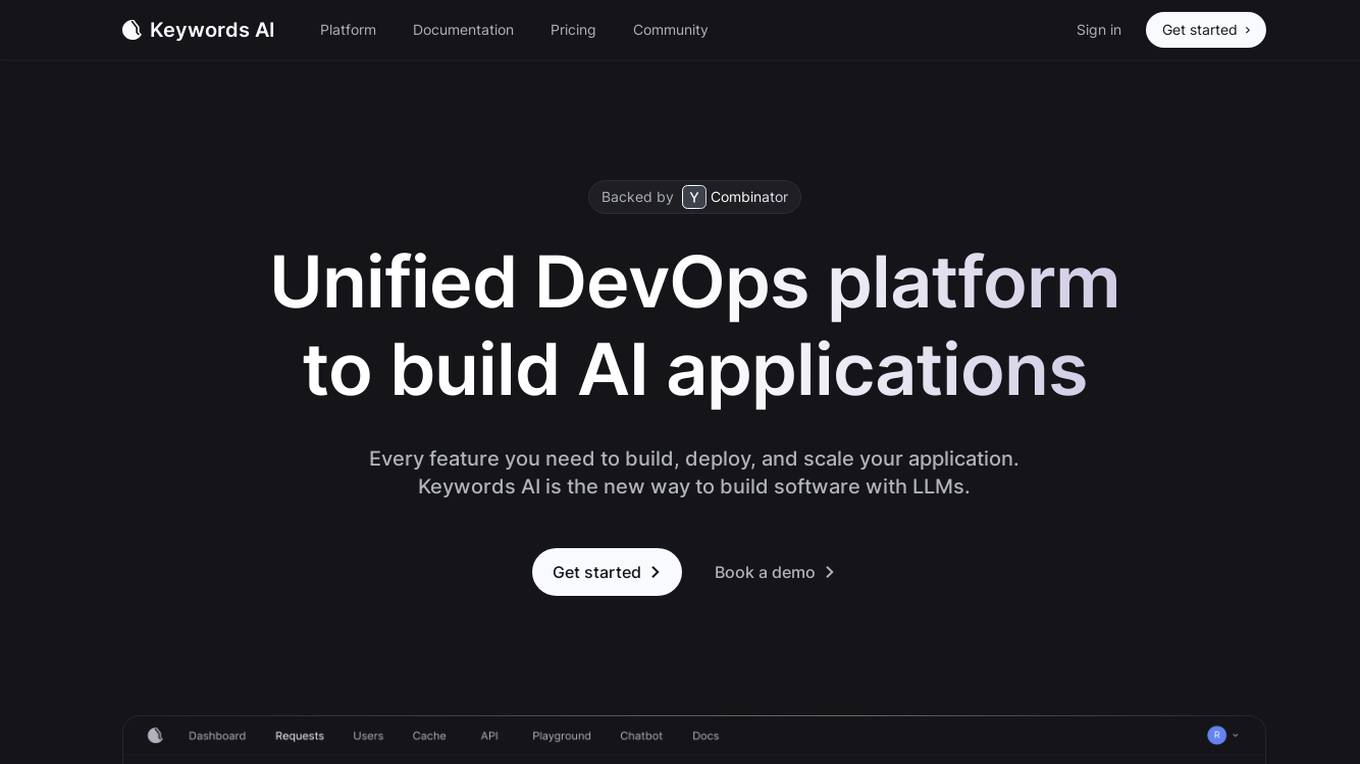
Unified DevOps platform to build AI applications
This is a unified DevOps platform to build AI applications. It provides a comprehensive set of tools and services to help developers build, deploy, and manage AI applications. The platform includes a variety of features such as a code editor, a debugger, a profiler, and a deployment manager. It also provides access to a variety of AI services, such as natural language processing, machine learning, and computer vision.
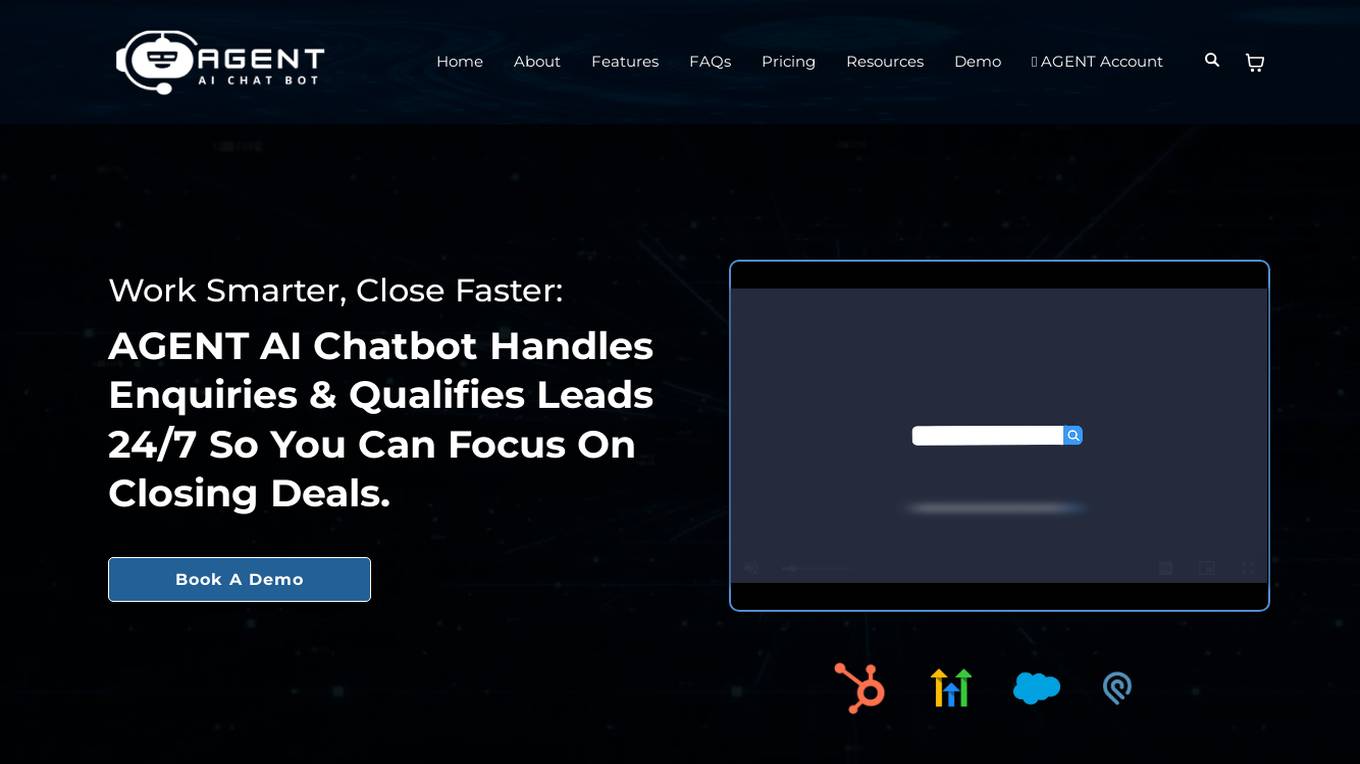
AGENT
AGENT is an AI chatbot powered by OpenAI and Anthropic, designed to handle customer inquiries, qualify leads, and enhance customer engagement. Developed by I Need Leads Ltd, AGENT combines advanced natural language processing, intelligent chatbots, and machine learning to empower businesses of all sizes in generating and managing leads efficiently. The platform adapts, learns, and grows with businesses, ensuring they stay ahead in the competitive digital landscape.
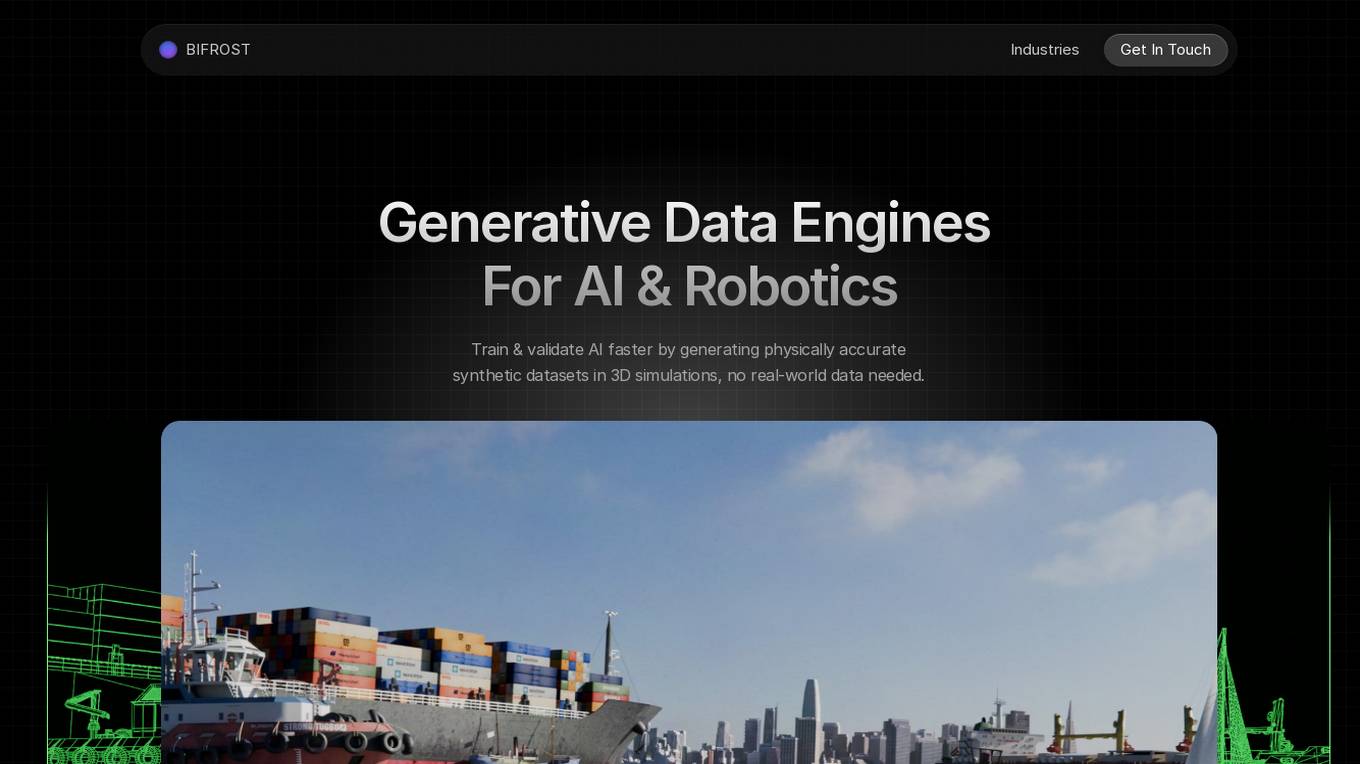
Bifrost AI
Bifrost AI is a data generation engine designed for AI and robotics applications. It enables users to train and validate AI models faster by generating physically accurate synthetic datasets in 3D simulations, eliminating the need for real-world data. The platform offers pixel-perfect labels, scenario metadata, and a simulated 3D world to enhance AI understanding. Bifrost AI empowers users to create new scenarios and datasets rapidly, stress test AI perception, and improve model performance. It is built for teams at every stage of AI development, offering features like automated labeling, class imbalance correction, and performance enhancement.
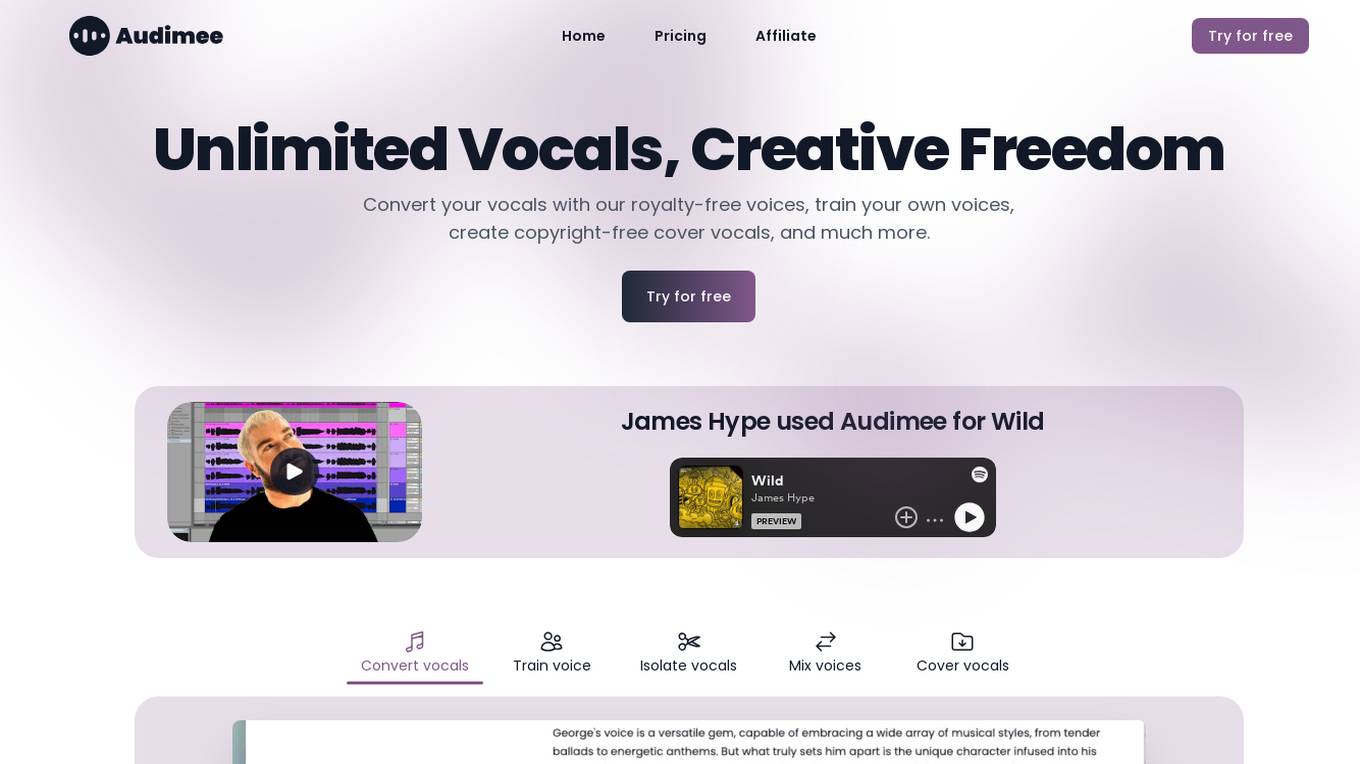
Audimee
Audimee is an AI-powered application that offers unlimited vocals and creative freedom to users. With Audimee, users can convert vocals using royalty-free voices, train their own voices, create copyright-free cover vocals, and more. The application utilizes a reworked RVC model and superior studio recordings to provide users with high-quality and dynamic human-like voices. Audimee is designed to handle a wider range of pitches and produce fewer detectable AI artifacts, setting a new standard in vocal conversion technology.
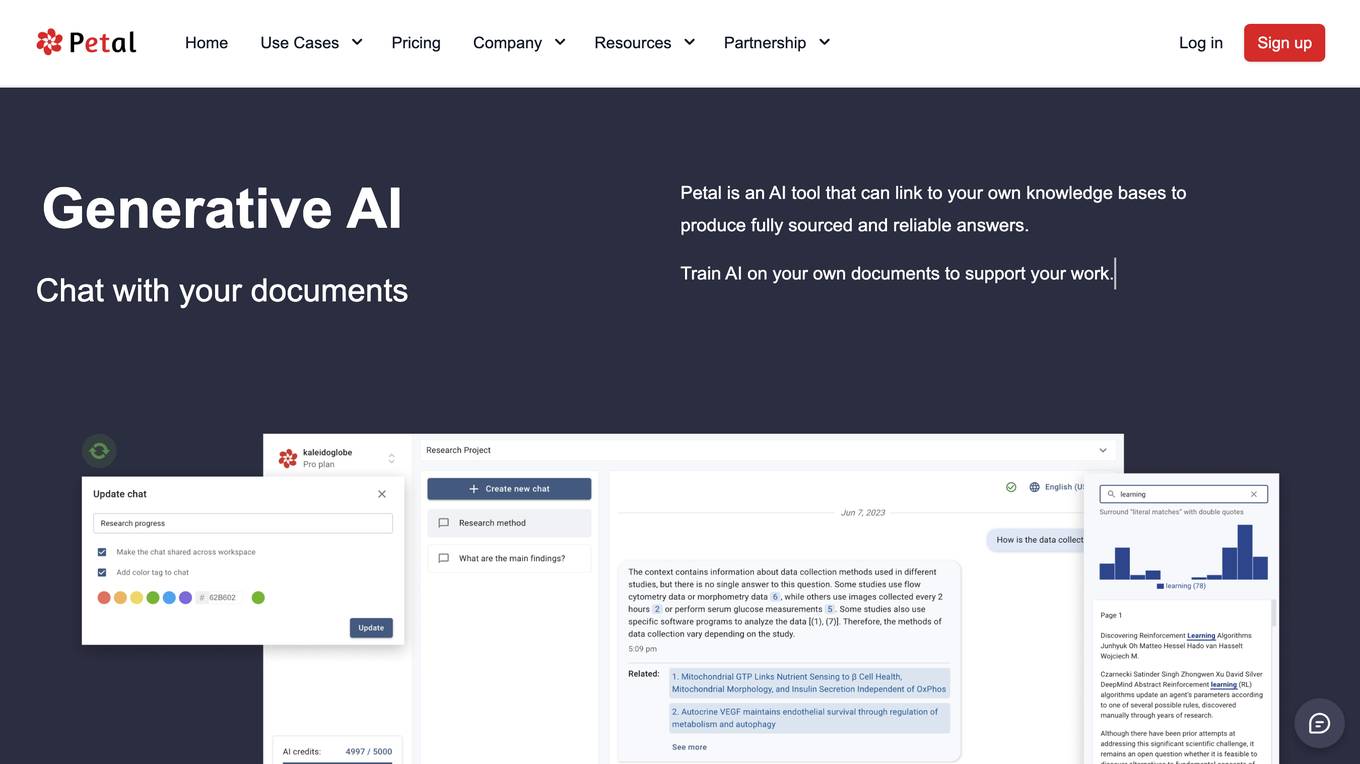
Petal
Petal is a document analysis platform powered by generative AI technology. It allows users to chat with their documents, providing fully sourced and reliable answers by linking to their own knowledge bases. Users can train AI on their documents to support their work, ensuring centralized knowledge management and document synchronization. Petal offers features such as automatic metadata extraction, file deduplication, and collaboration tools to enhance productivity and streamline workflows for researchers, faculty, and industry experts.
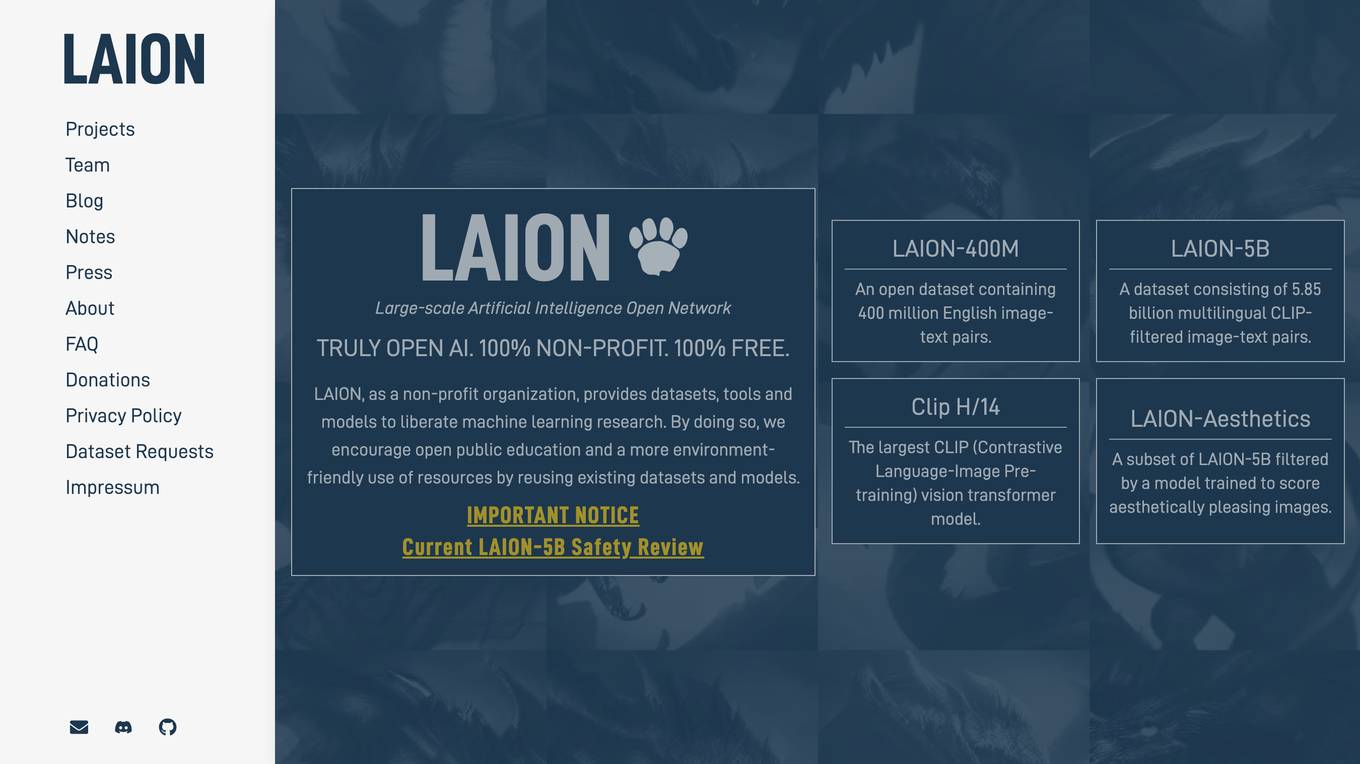
LAION
LAION is a non-profit organization that provides datasets, tools, and models to advance machine learning research. The organization's goal is to promote open public education and encourage the reuse of existing datasets and models to reduce the environmental impact of machine learning research.
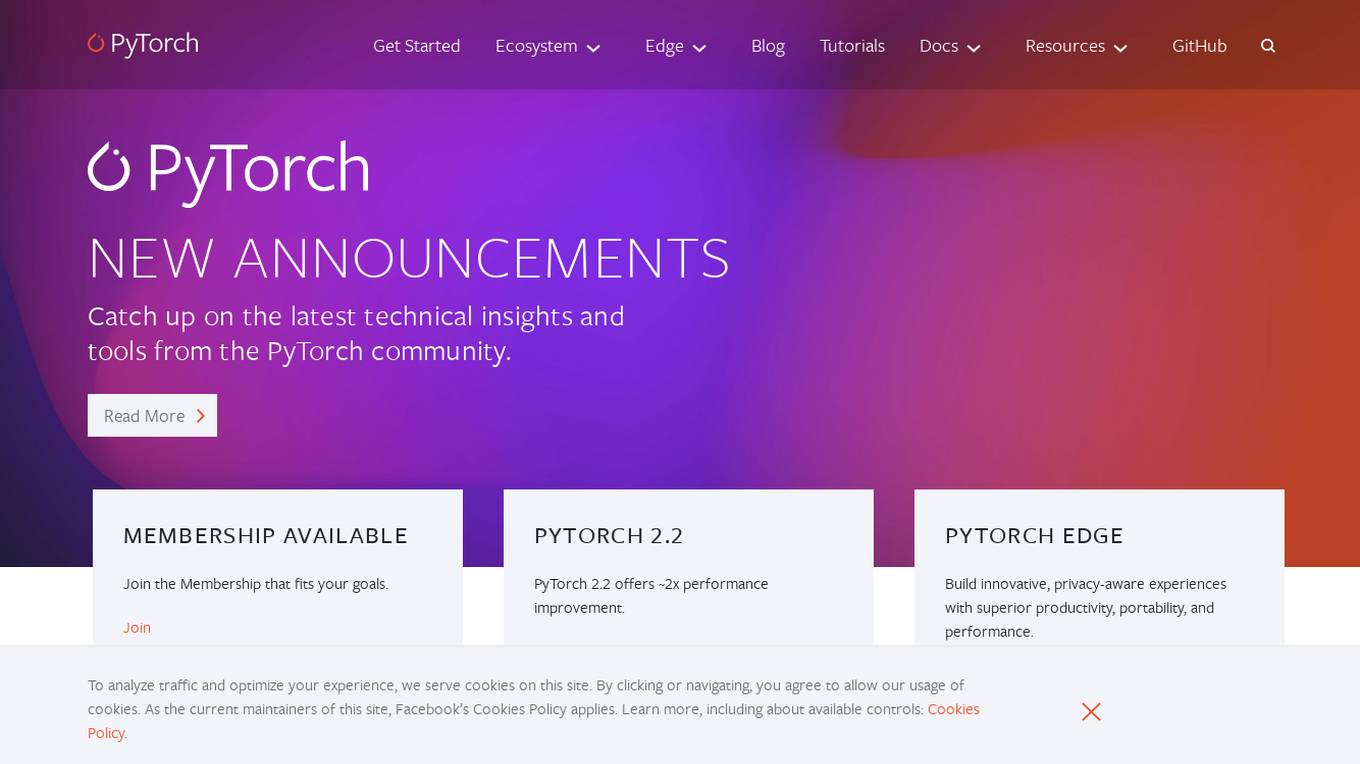
PyTorch
PyTorch is an open-source machine learning library based on the Torch library. It is used for applications such as computer vision, natural language processing, and reinforcement learning. PyTorch is known for its flexibility and ease of use, making it a popular choice for researchers and developers in the field of artificial intelligence.
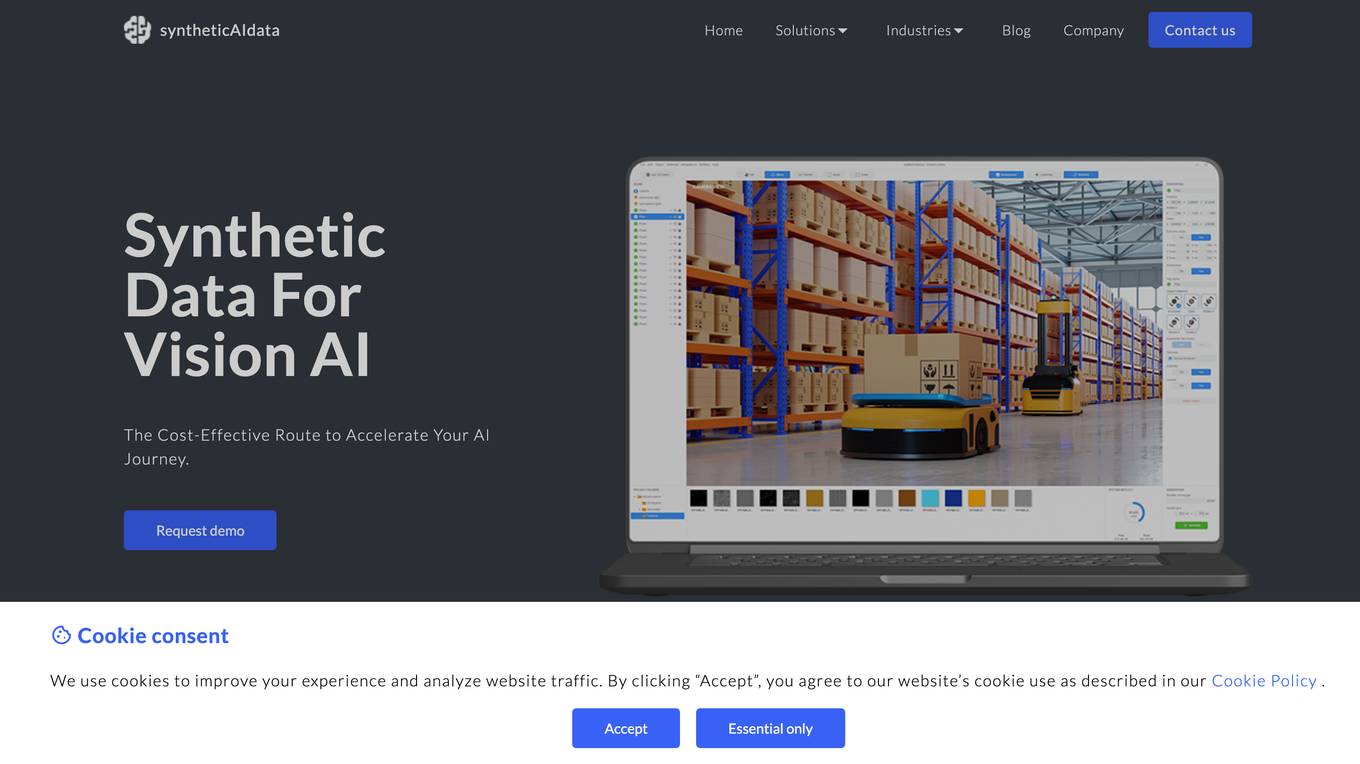
syntheticAIdata
syntheticAIdata is a platform that provides synthetic data for training vision AI models. Synthetic data is generated artificially, and it can be used to augment existing real-world datasets or to create new datasets from scratch. syntheticAIdata's platform is easy to use, and it can be integrated with leading cloud platforms. The company's mission is to make synthetic data accessible to everyone, and to help businesses overcome the challenges of acquiring high-quality data for training their vision AI models.
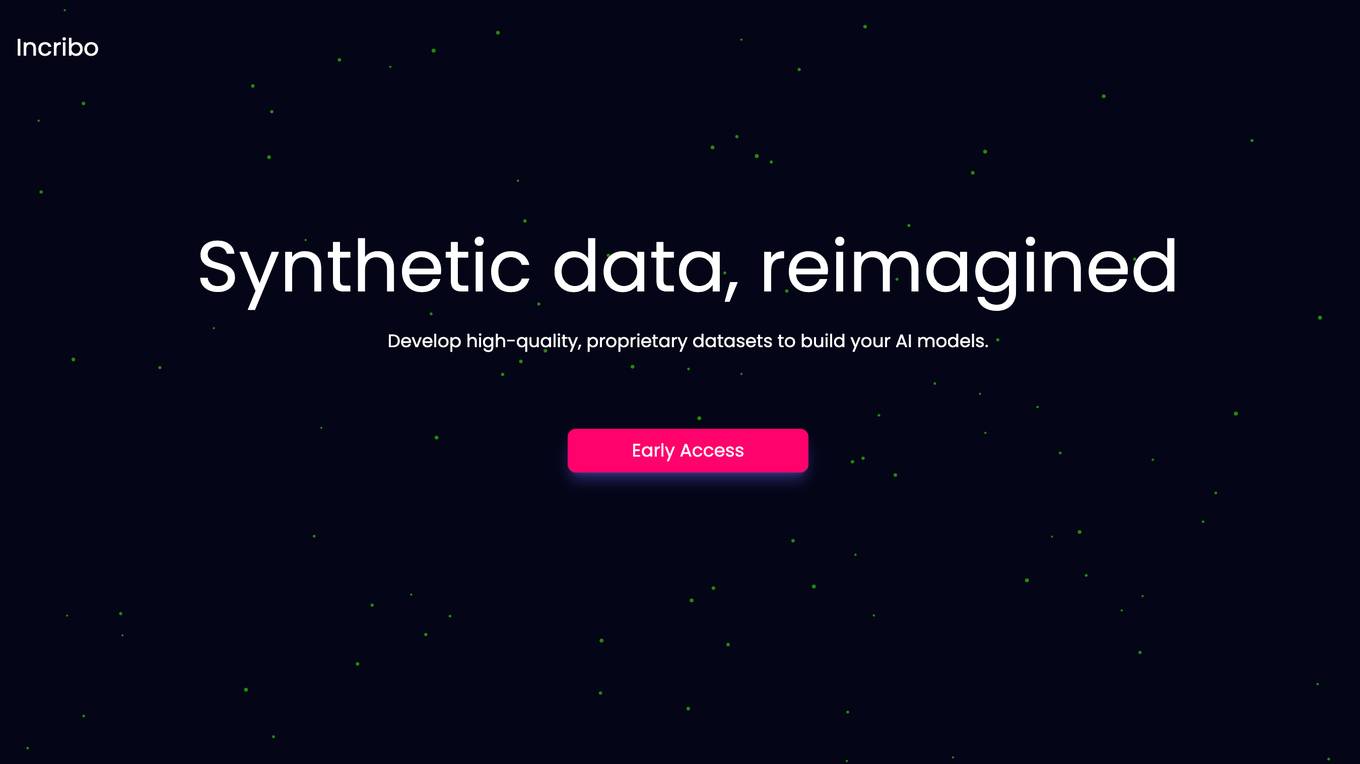
Incribo
Incribo is a company that provides synthetic data for training machine learning models. Synthetic data is artificially generated data that is designed to mimic real-world data. This data can be used to train machine learning models without the need for real-world data, which can be expensive and difficult to obtain. Incribo's synthetic data is high quality and affordable, making it a valuable resource for machine learning developers.
20 - Open Source AI Tools
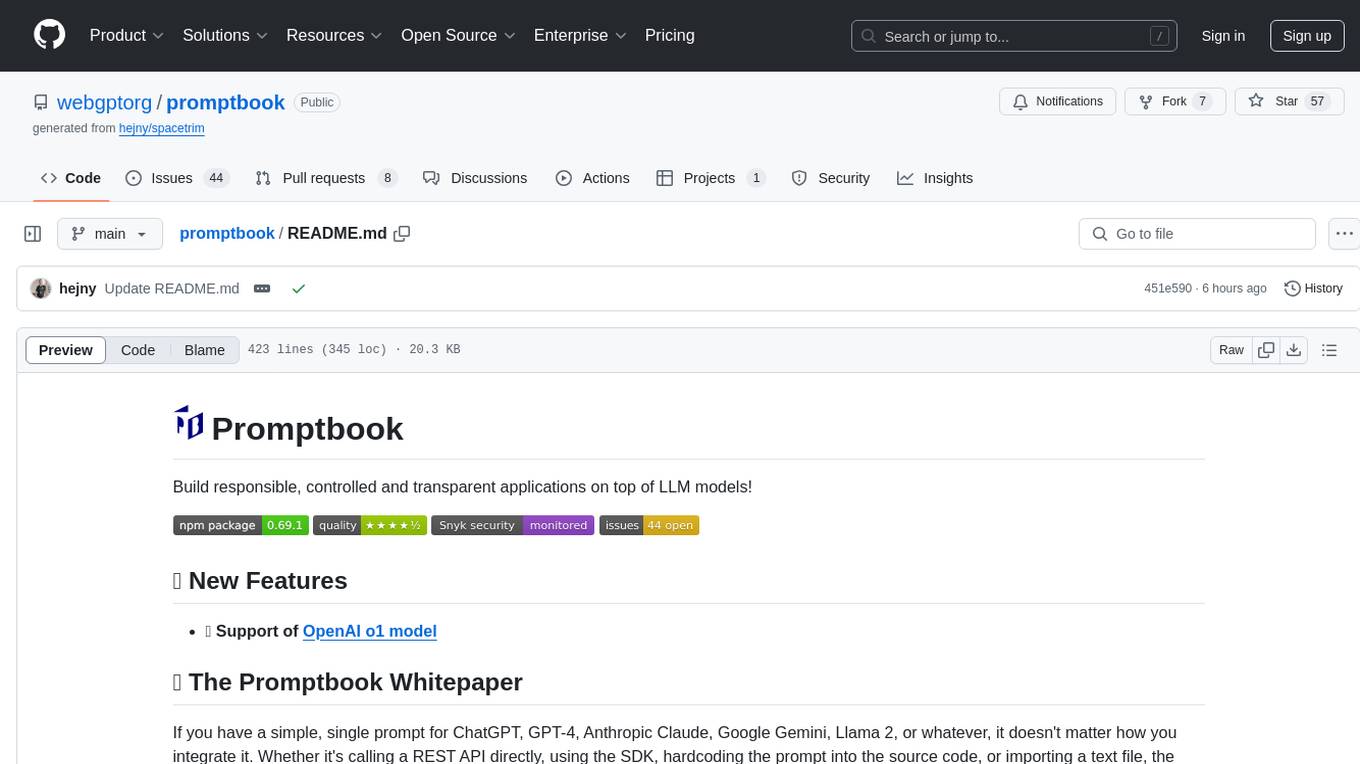
promptbook
Promptbook is a library designed to build responsible, controlled, and transparent applications on top of large language models (LLMs). It helps users overcome limitations of LLMs like hallucinations, off-topic responses, and poor quality output by offering features such as fine-tuning models, prompt-engineering, and orchestrating multiple prompts in a pipeline. The library separates concerns, establishes a common format for prompt business logic, and handles low-level details like model selection and context size. It also provides tools for pipeline execution, caching, fine-tuning, anomaly detection, and versioning. Promptbook supports advanced techniques like Retrieval-Augmented Generation (RAG) and knowledge utilization to enhance output quality.
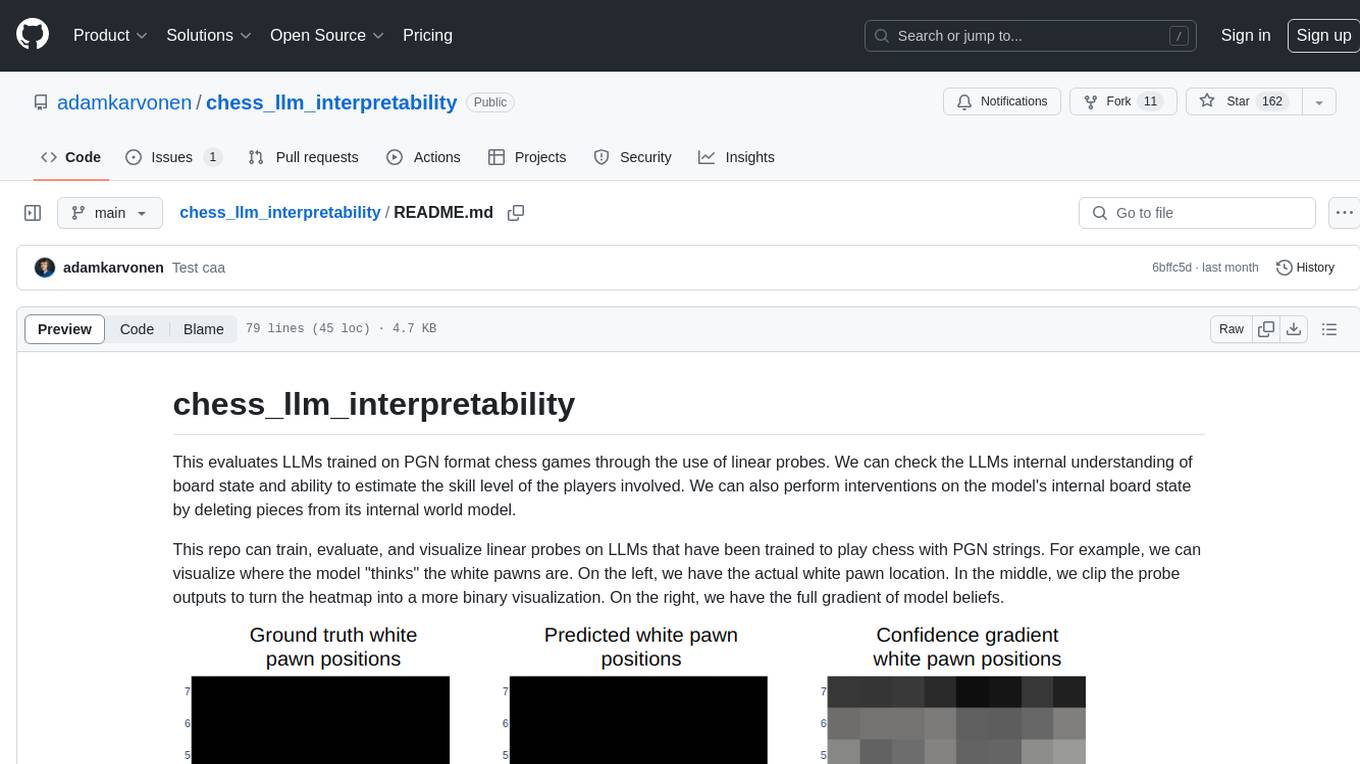
chess_llm_interpretability
This repository evaluates Large Language Models (LLMs) trained on PGN format chess games using linear probes. It assesses the LLMs' internal understanding of board state and their ability to estimate player skill levels. The repo provides tools to train, evaluate, and visualize linear probes on LLMs trained to play chess with PGN strings. Users can visualize the model's predictions, perform interventions on the model's internal board state, and analyze board state and player skill level accuracy across different LLMs. The experiments in the repo can be conducted with less than 1 GB of VRAM, and training probes on the 8 layer model takes about 10 minutes on an RTX 3050. The repo also includes scripts for performing board state interventions and skill interventions, along with useful links to open-source code, models, datasets, and pretrained models.
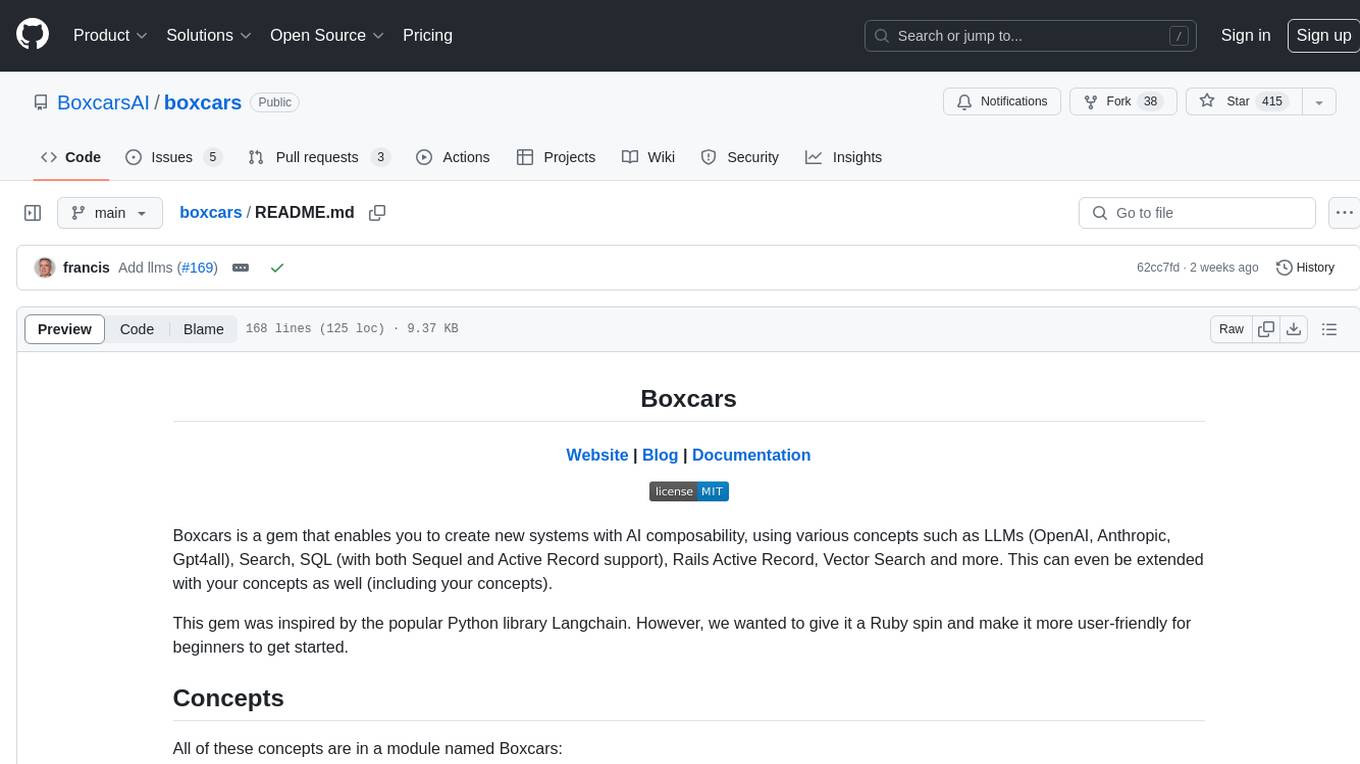
boxcars
Boxcars is a Ruby gem that enables users to create new systems with AI composability, incorporating concepts such as LLMs, Search, SQL, Rails Active Record, Vector Search, and more. It allows users to work with Boxcars, Trains, Prompts, Engines, and VectorStores to solve problems and generate text results. The gem is designed to be user-friendly for beginners and can be extended with custom concepts. Boxcars is actively seeking ways to enhance security measures to prevent malicious actions. Users can use Boxcars for tasks like running calculations, performing searches, generating Ruby code for math operations, and interacting with APIs like OpenAI, Anthropic, and Google SERP.
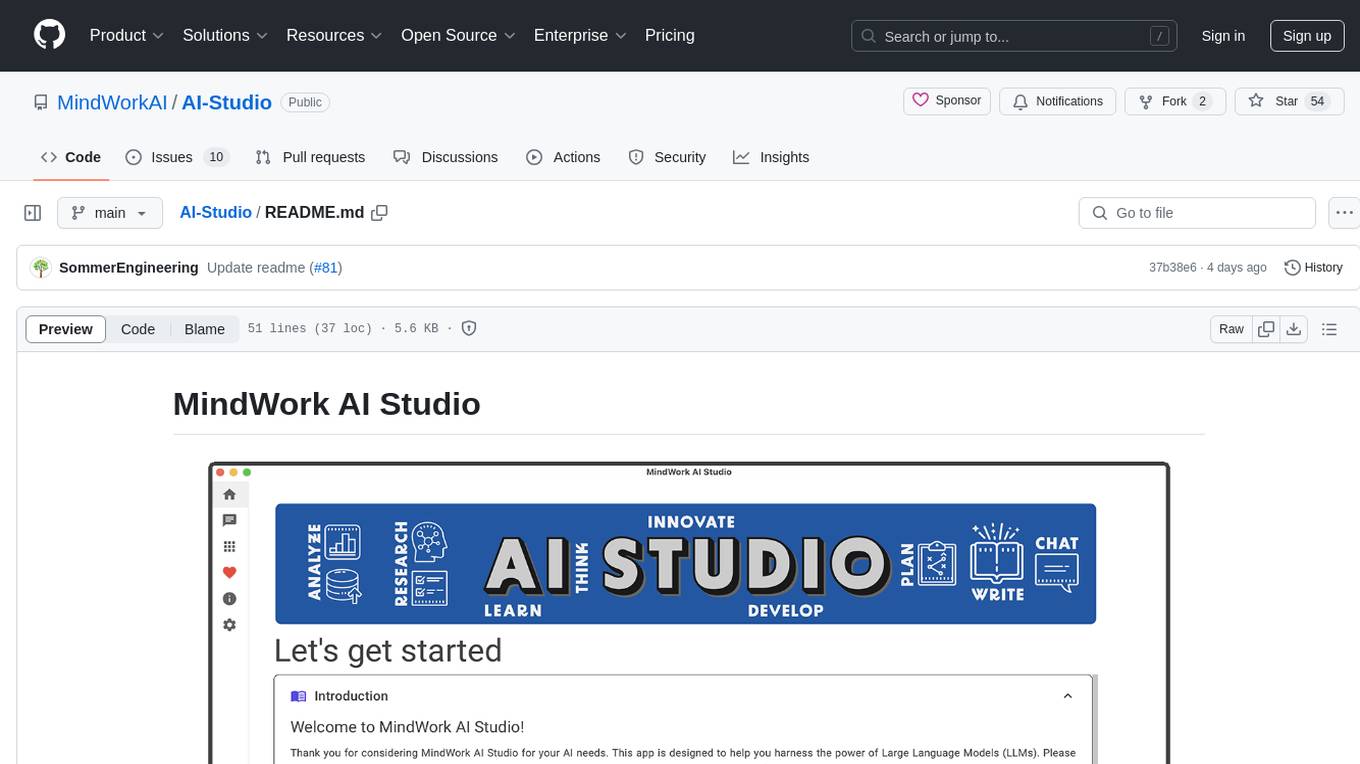
AI-Studio
MindWork AI Studio is a desktop application that provides a unified chat interface for Large Language Models (LLMs). It is free to use for personal and commercial purposes, offers independence in choosing LLM providers, provides unrestricted usage through the providers API, and is cost-effective with pay-as-you-go pricing. The app prioritizes privacy, flexibility, minimal storage and memory usage, and low impact on system resources. Users can support the project through monthly contributions or one-time donations, with opportunities for companies to sponsor the project for public relations and marketing benefits. Planned features include support for more LLM providers, system prompts integration, text replacement for privacy, and advanced interactions tailored for various use cases.
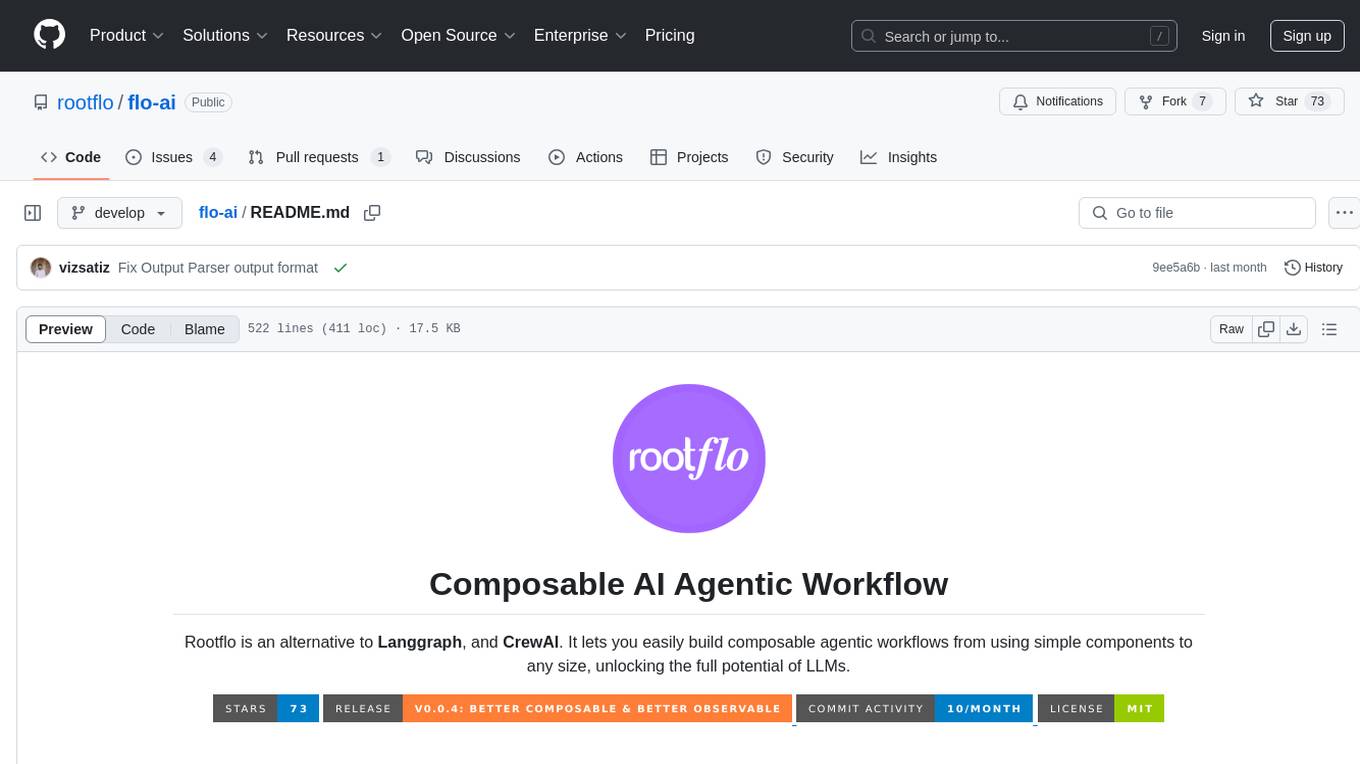
flo-ai
Flo AI is a Python framework that enables users to build production-ready AI agents and teams with minimal code. It allows users to compose complex AI architectures using pre-built components while maintaining the flexibility to create custom components. The framework supports composable, production-ready, YAML-first, and flexible AI systems. Users can easily create AI agents and teams, manage teams of AI agents working together, and utilize built-in support for Retrieval-Augmented Generation (RAG) and compatibility with Langchain tools. Flo AI also provides tools for output parsing and formatting, tool logging, data collection, and JSON output collection. It is MIT Licensed and offers detailed documentation, tutorials, and examples for AI engineers and teams to accelerate development, maintainability, scalability, and testability of AI systems.
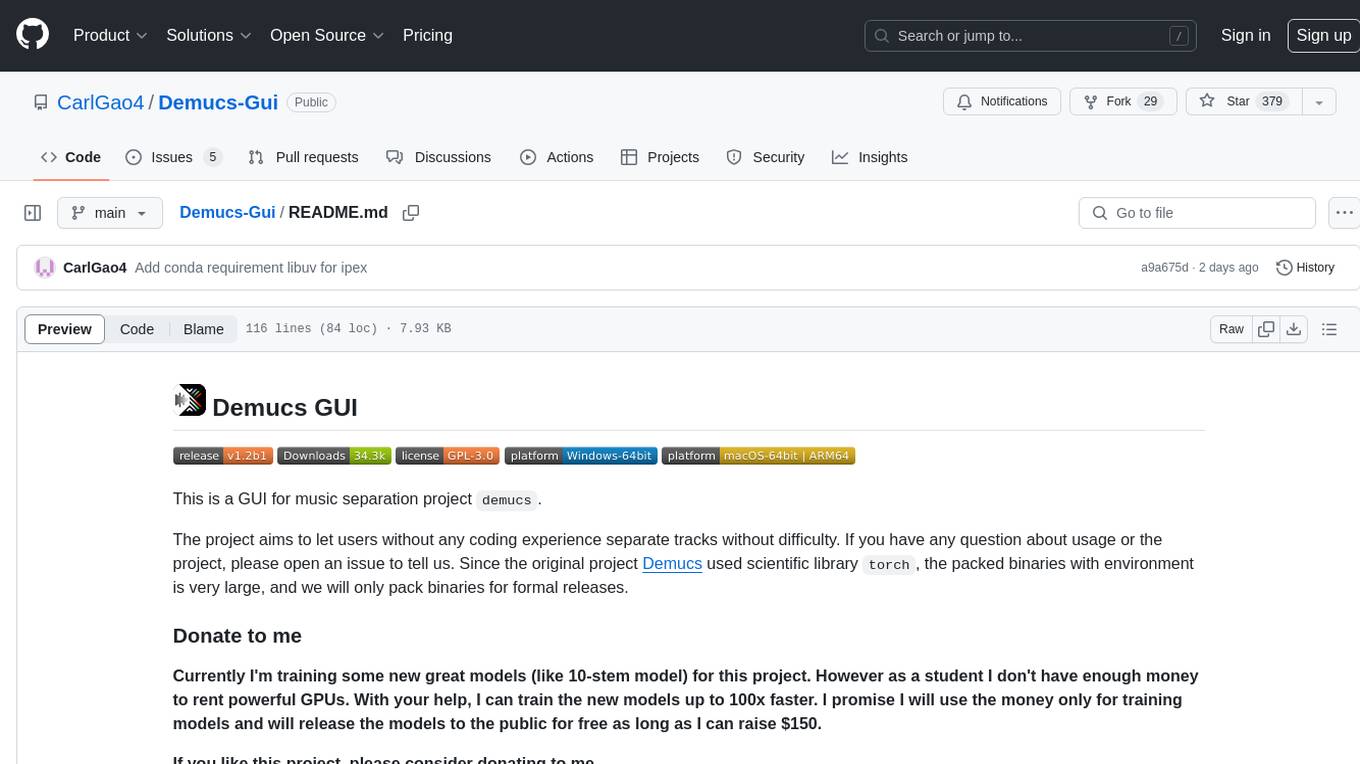
Demucs-Gui
Demucs GUI is a graphical user interface for the music separation project Demucs. It aims to allow users without coding experience to easily separate tracks. The tool provides a user-friendly interface for running the Demucs project, which originally used the scientific library torch. The GUI simplifies the process of separating tracks and provides support for different platforms such as Windows, macOS, and Linux. Users can donate to support the development of new models for the project, and the tool has specific system requirements including minimum system versions and hardware specifications.
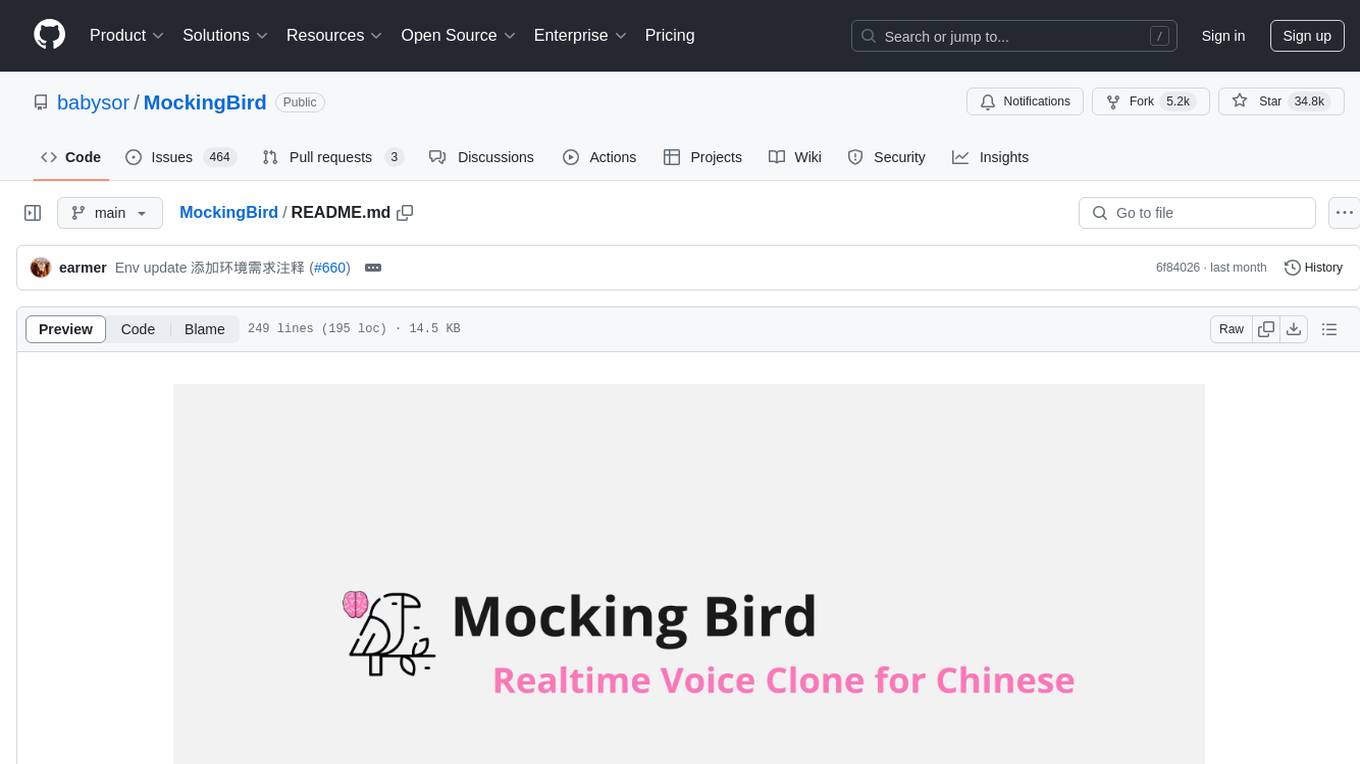
MockingBird
MockingBird is a toolbox designed for Mandarin speech synthesis using PyTorch. It supports multiple datasets such as aidatatang_200zh, magicdata, aishell3, and data_aishell. The toolbox can run on Windows, Linux, and M1 MacOS, providing easy and effective speech synthesis with pretrained encoder/vocoder models. It is webserver ready for remote calling. Users can train their own models or use existing ones for the encoder, synthesizer, and vocoder. The toolbox offers a demo video and detailed setup instructions for installation and model training.
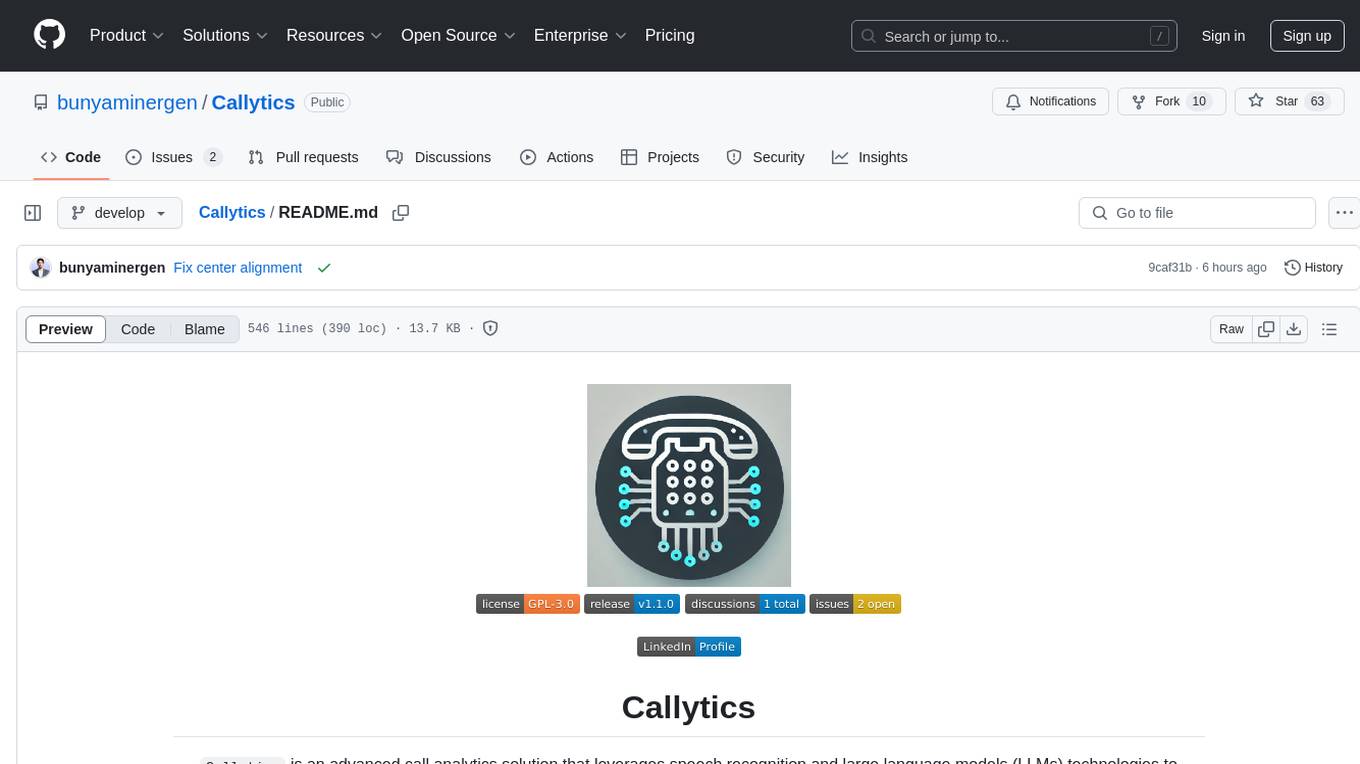
Callytics
Callytics is an advanced call analytics solution that leverages speech recognition and large language models (LLMs) technologies to analyze phone conversations from customer service and call centers. By processing both the audio and text of each call, it provides insights such as sentiment analysis, topic detection, conflict detection, profanity word detection, and summary. These cutting-edge techniques help businesses optimize customer interactions, identify areas for improvement, and enhance overall service quality. When an audio file is placed in the .data/input directory, the entire pipeline automatically starts running, and the resulting data is inserted into the database. This is only a v1.1.0 version; many new features will be added, models will be fine-tuned or trained from scratch, and various optimization efforts will be applied.
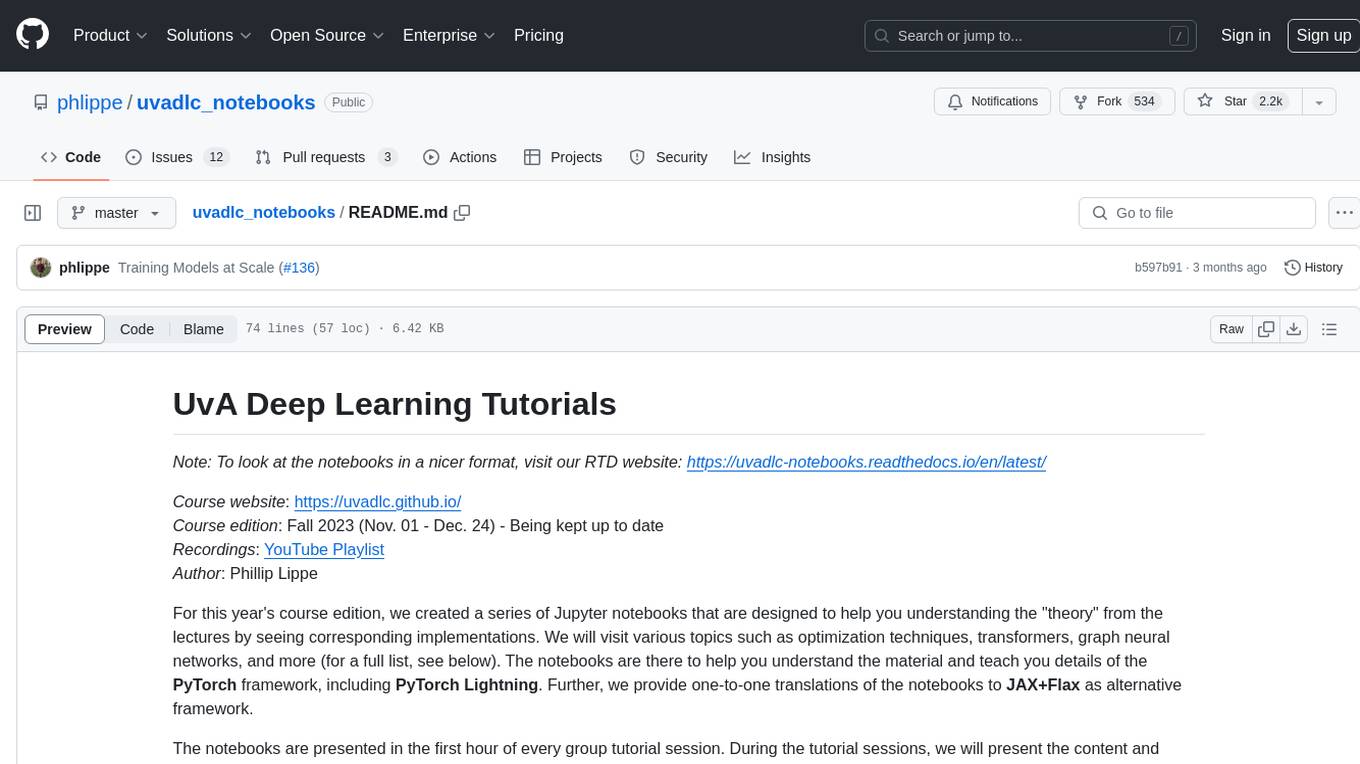
uvadlc_notebooks
The UvA Deep Learning Tutorials repository contains a series of Jupyter notebooks designed to help understand theoretical concepts from lectures by providing corresponding implementations. The notebooks cover topics such as optimization techniques, transformers, graph neural networks, and more. They aim to teach details of the PyTorch framework, including PyTorch Lightning, with alternative translations to JAX+Flax. The tutorials are integrated as official tutorials of PyTorch Lightning and are relevant for graded assignments and exams.
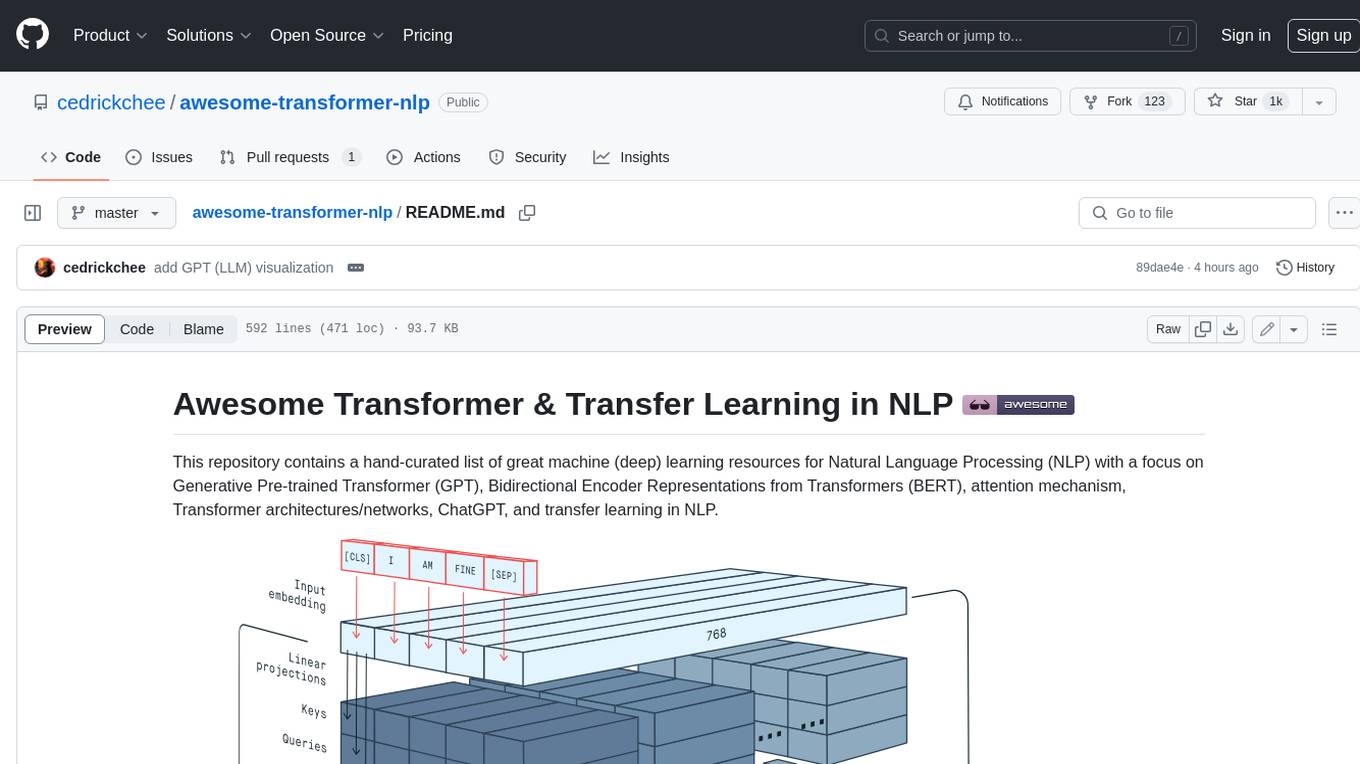
awesome-transformer-nlp
This repository contains a hand-curated list of great machine (deep) learning resources for Natural Language Processing (NLP) with a focus on Generative Pre-trained Transformer (GPT), Bidirectional Encoder Representations from Transformers (BERT), attention mechanism, Transformer architectures/networks, Chatbot, and transfer learning in NLP.
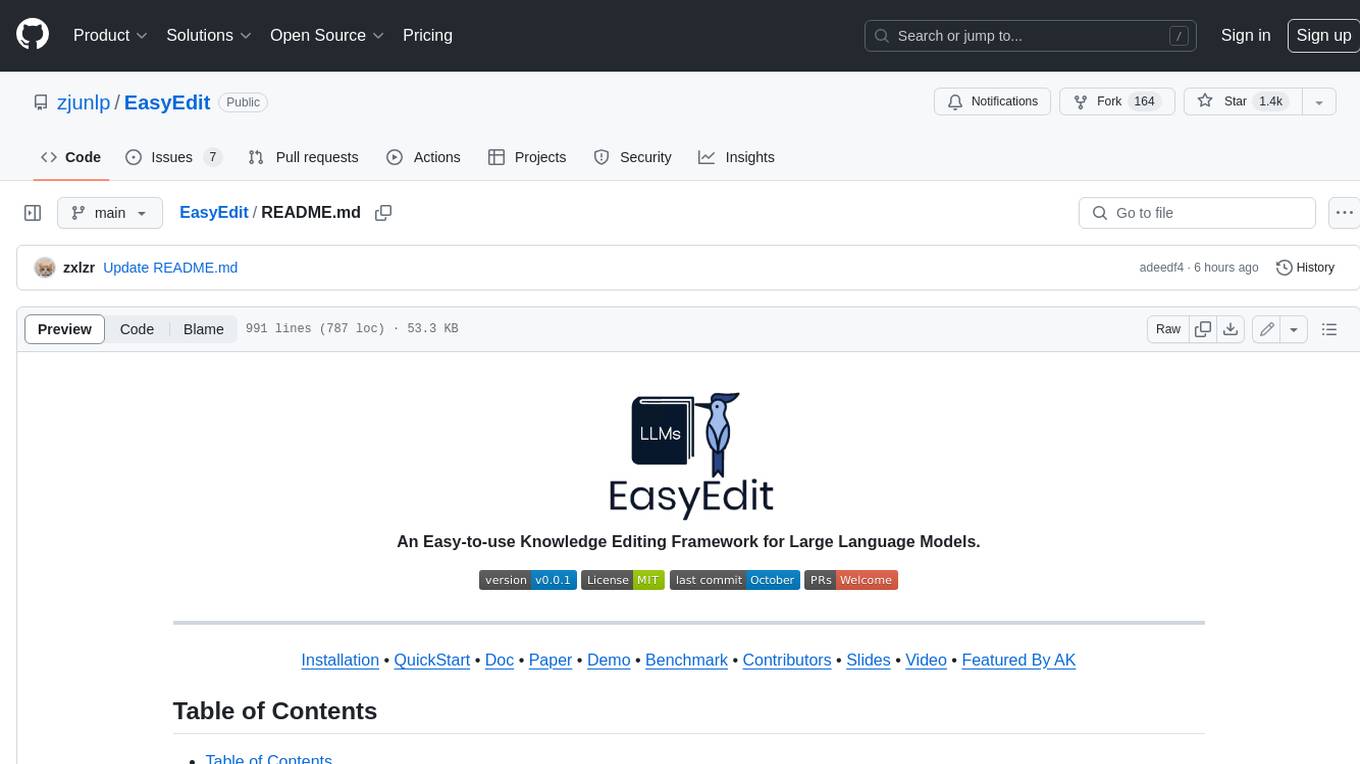
EasyEdit
EasyEdit is a Python package for edit Large Language Models (LLM) like `GPT-J`, `Llama`, `GPT-NEO`, `GPT2`, `T5`(support models from **1B** to **65B**), the objective of which is to alter the behavior of LLMs efficiently within a specific domain without negatively impacting performance across other inputs. It is designed to be easy to use and easy to extend.
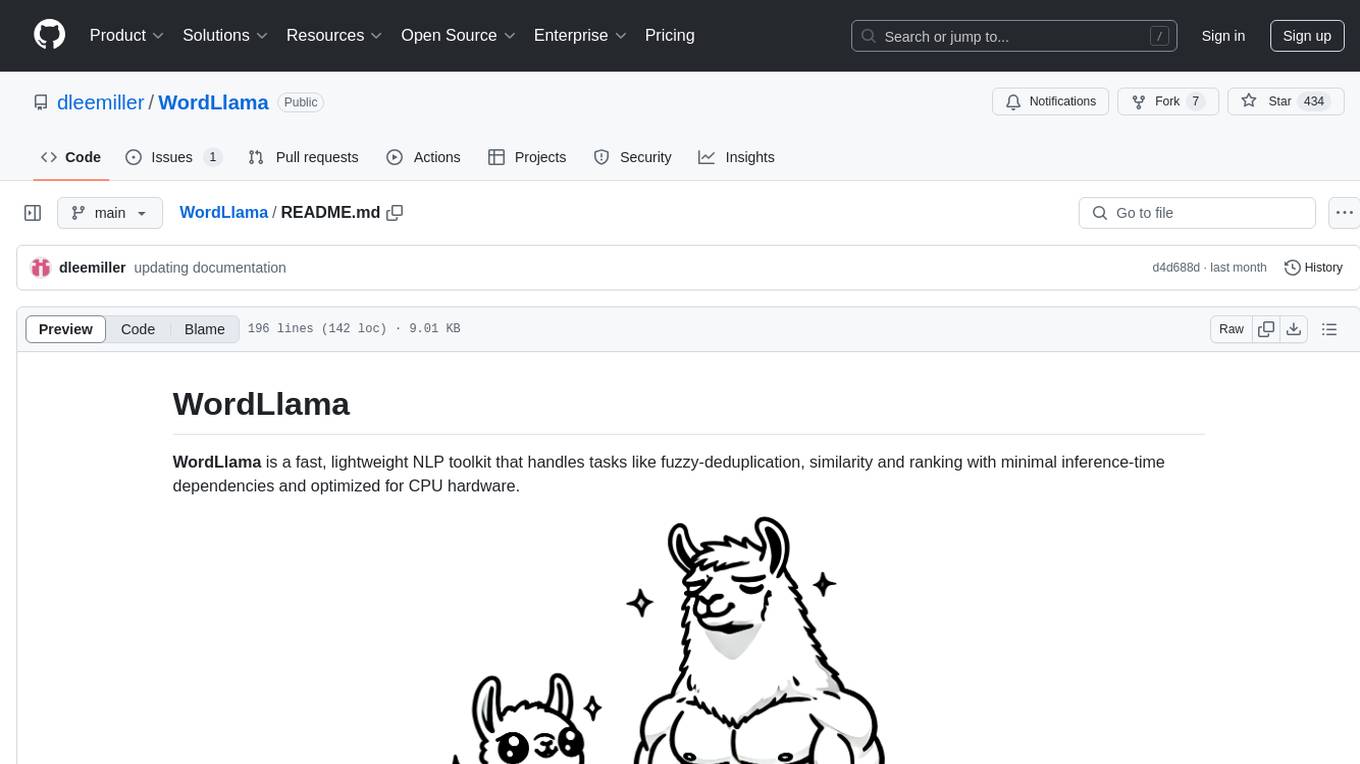
WordLlama
WordLlama is a fast, lightweight NLP toolkit optimized for CPU hardware. It recycles components from large language models to create efficient word representations. It offers features like Matryoshka Representations, low resource requirements, binarization, and numpy-only inference. The tool is suitable for tasks like semantic matching, fuzzy deduplication, ranking, and clustering, making it a good option for NLP-lite tasks and exploratory analysis.
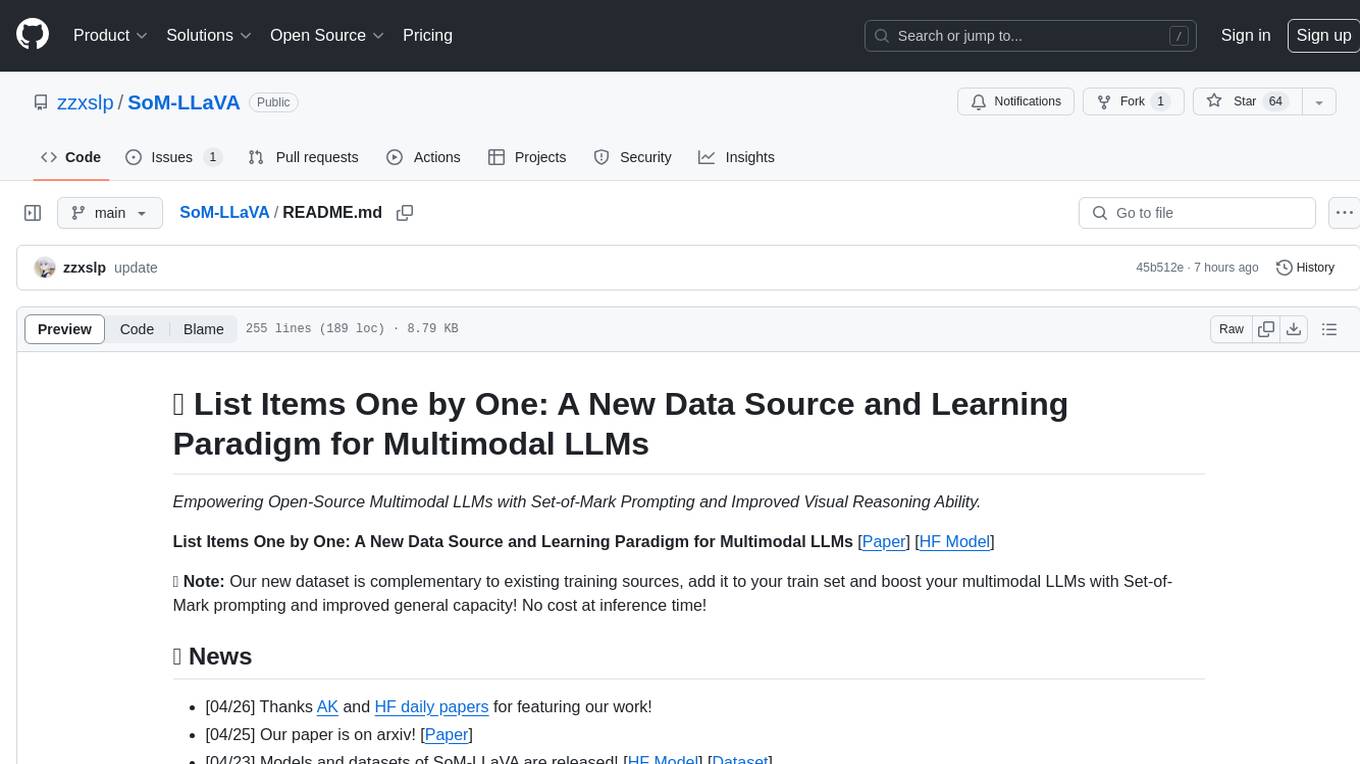
SoM-LLaVA
SoM-LLaVA is a new data source and learning paradigm for Multimodal LLMs, empowering open-source Multimodal LLMs with Set-of-Mark prompting and improved visual reasoning ability. The repository provides a new dataset that is complementary to existing training sources, enhancing multimodal LLMs with Set-of-Mark prompting and improved general capacity. By adding 30k SoM data to the visual instruction tuning stage of LLaVA, the tool achieves 1% to 6% relative improvements on all benchmarks. Users can train SoM-LLaVA via command line and utilize the implementation to annotate COCO images with SoM. Additionally, the tool can be loaded in Huggingface for further usage.
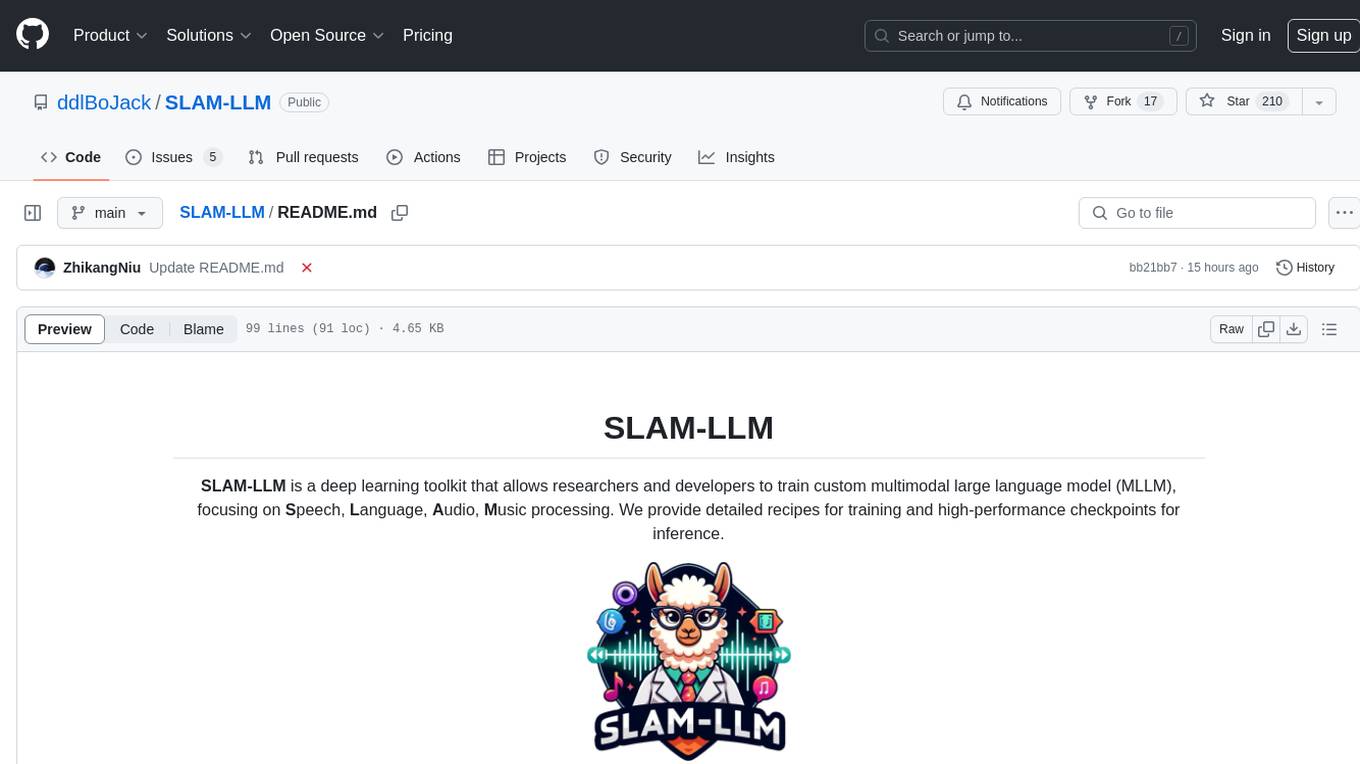
SLAM-LLM
SLAM-LLM is a deep learning toolkit designed for researchers and developers to train custom multimodal large language models (MLLM) focusing on speech, language, audio, and music processing. It provides detailed recipes for training and high-performance checkpoints for inference. The toolkit supports tasks such as automatic speech recognition (ASR), text-to-speech (TTS), visual speech recognition (VSR), automated audio captioning (AAC), spatial audio understanding, and music caption (MC). SLAM-LLM features easy extension to new models and tasks, mixed precision training for faster training with less GPU memory, multi-GPU training with data and model parallelism, and flexible configuration based on Hydra and dataclass.
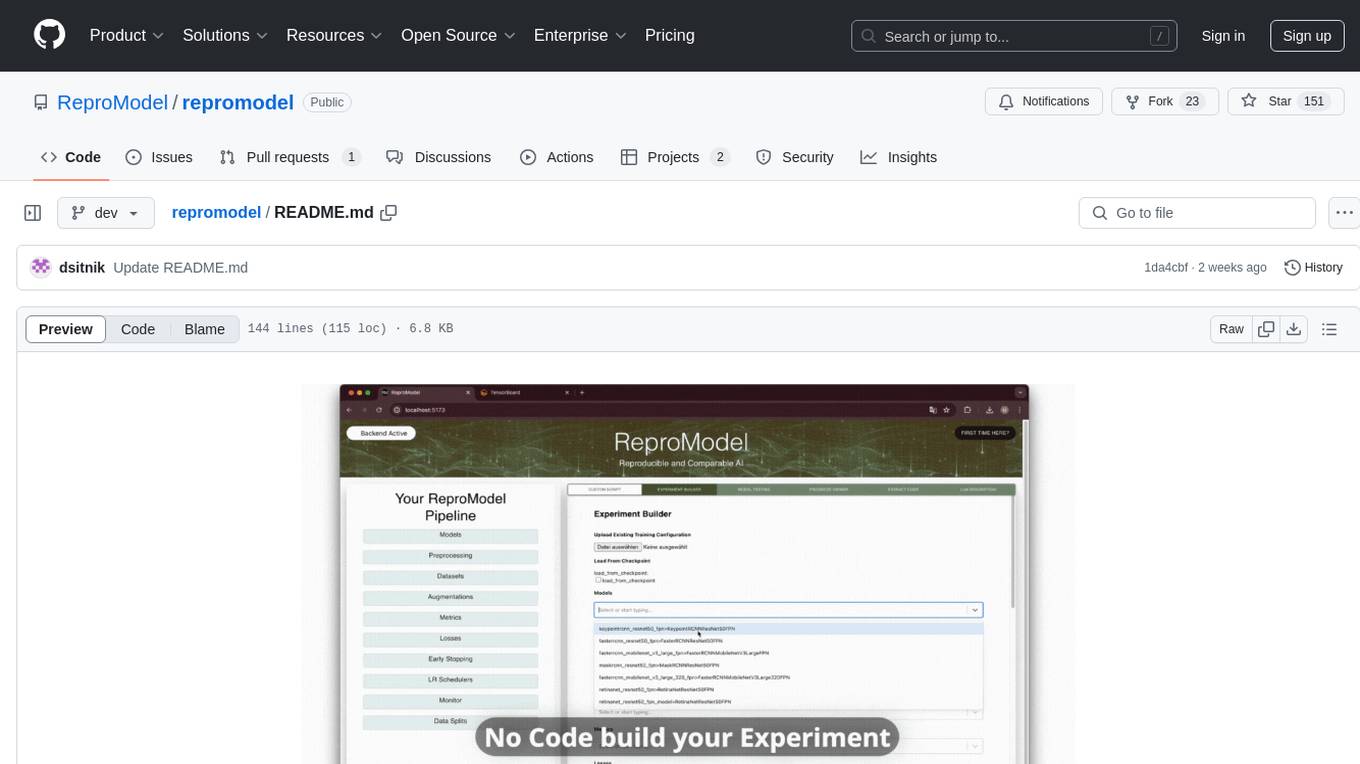
repromodel
ReproModel is an open-source toolbox designed to boost AI research efficiency by enabling researchers to reproduce, compare, train, and test AI models faster. It provides standardized models, dataloaders, and processing procedures, allowing researchers to focus on new datasets and model development. With a no-code solution, users can access benchmark and SOTA models and datasets, utilize training visualizations, extract code for publication, and leverage an LLM-powered automated methodology description writer. The toolbox helps researchers modularize development, compare pipeline performance reproducibly, and reduce time for model development, computation, and writing. Future versions aim to facilitate building upon state-of-the-art research by loading previously published study IDs with verified code, experiments, and results stored in the system.
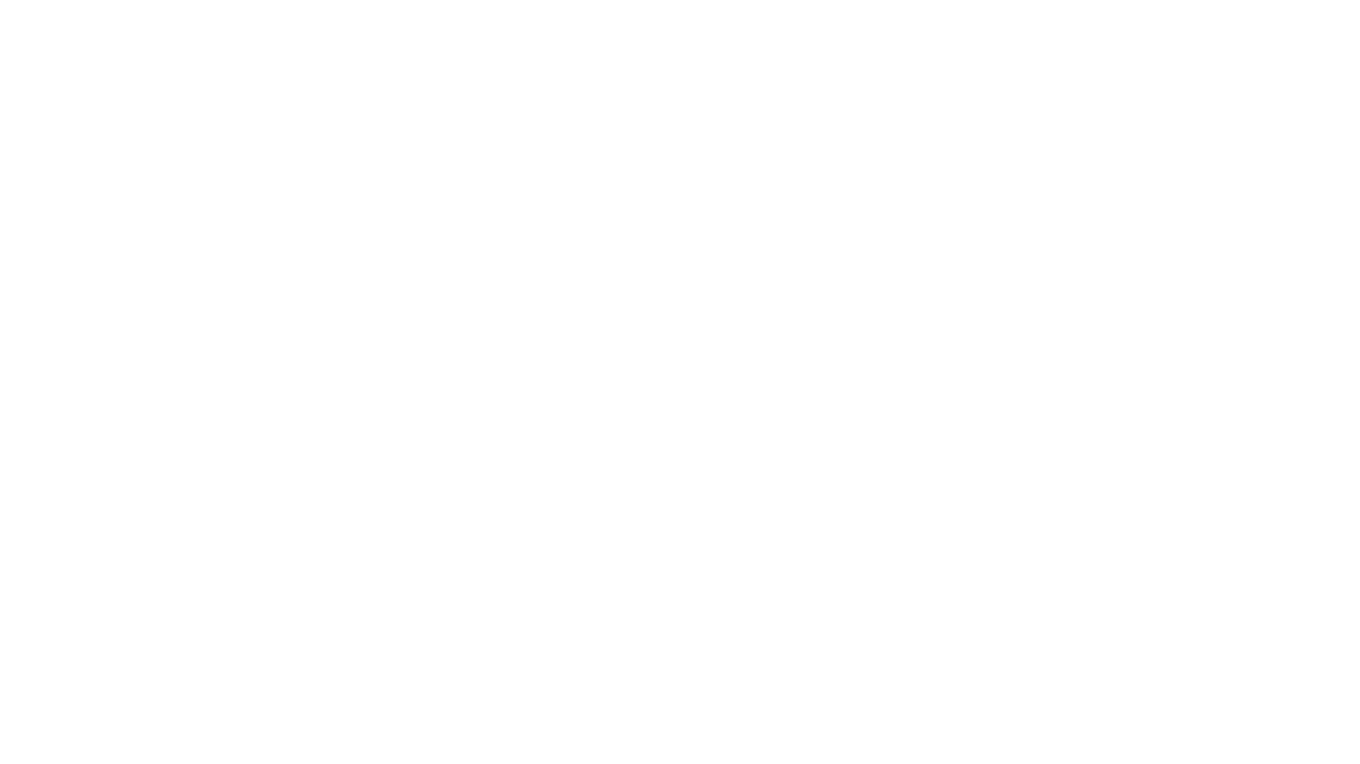
only_train_once
Only Train Once (OTO) is an automatic, architecture-agnostic DNN training and compression framework that allows users to train a general DNN from scratch or a pretrained checkpoint to achieve high performance and slimmer architecture simultaneously in a one-shot manner without fine-tuning. The framework includes features for automatic structured pruning and erasing operators, as well as hybrid structured sparse optimizers for efficient model compression. OTO provides tools for pruning zero-invariant group partitioning, constructing pruned models, and visualizing pruning and erasing dependency graphs. It supports the HESSO optimizer and offers a sanity check for compliance testing on various DNNs. The repository also includes publications, installation instructions, quick start guides, and a roadmap for future enhancements and collaborations.
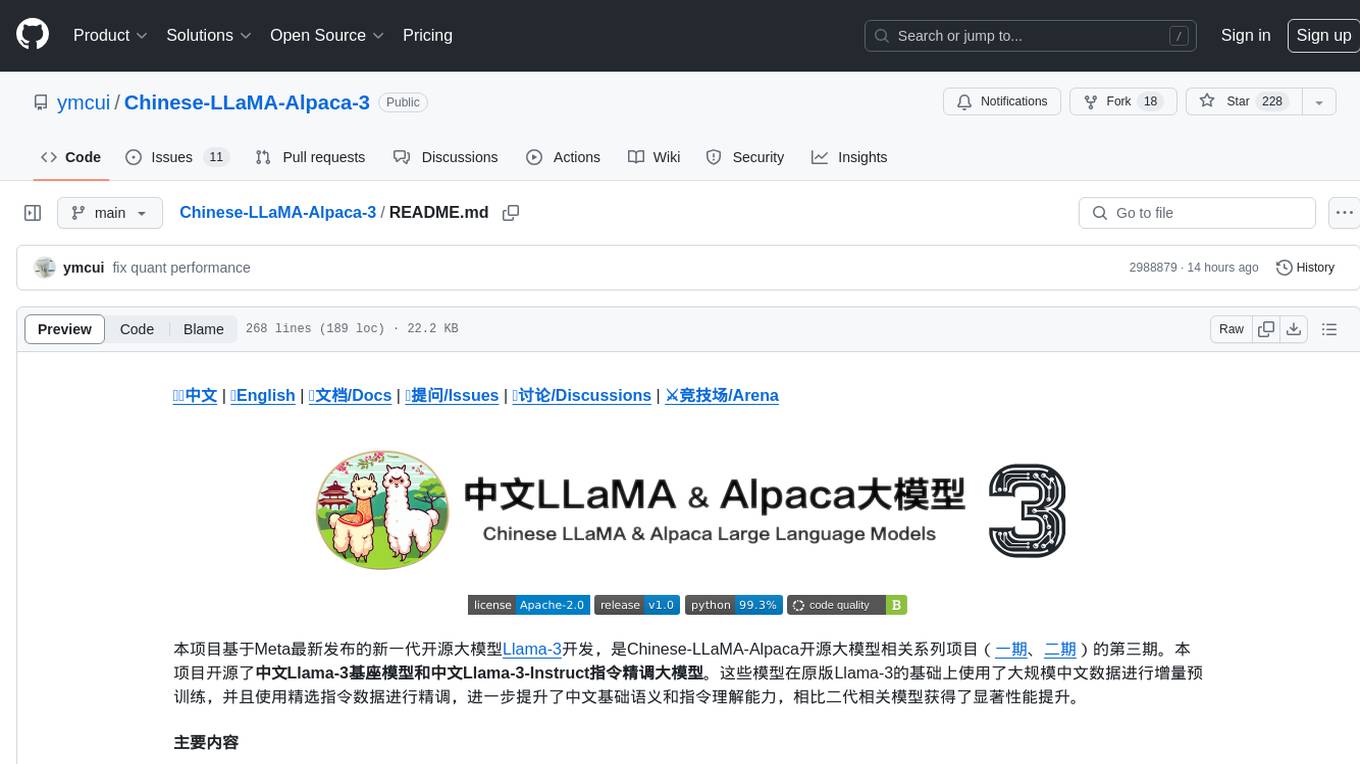
Chinese-LLaMA-Alpaca-3
Chinese-LLaMA-Alpaca-3 is a project based on Meta's latest release of the new generation open-source large model Llama-3. It is the third phase of the Chinese-LLaMA-Alpaca open-source large model series projects (Phase 1, Phase 2). This project open-sources the Chinese Llama-3 base model and the Chinese Llama-3-Instruct instruction fine-tuned large model. These models incrementally pre-train with a large amount of Chinese data on the basis of the original Llama-3 and further fine-tune using selected instruction data, enhancing Chinese basic semantics and instruction understanding capabilities. Compared to the second-generation related models, significant performance improvements have been achieved.
20 - OpenAI Gpts
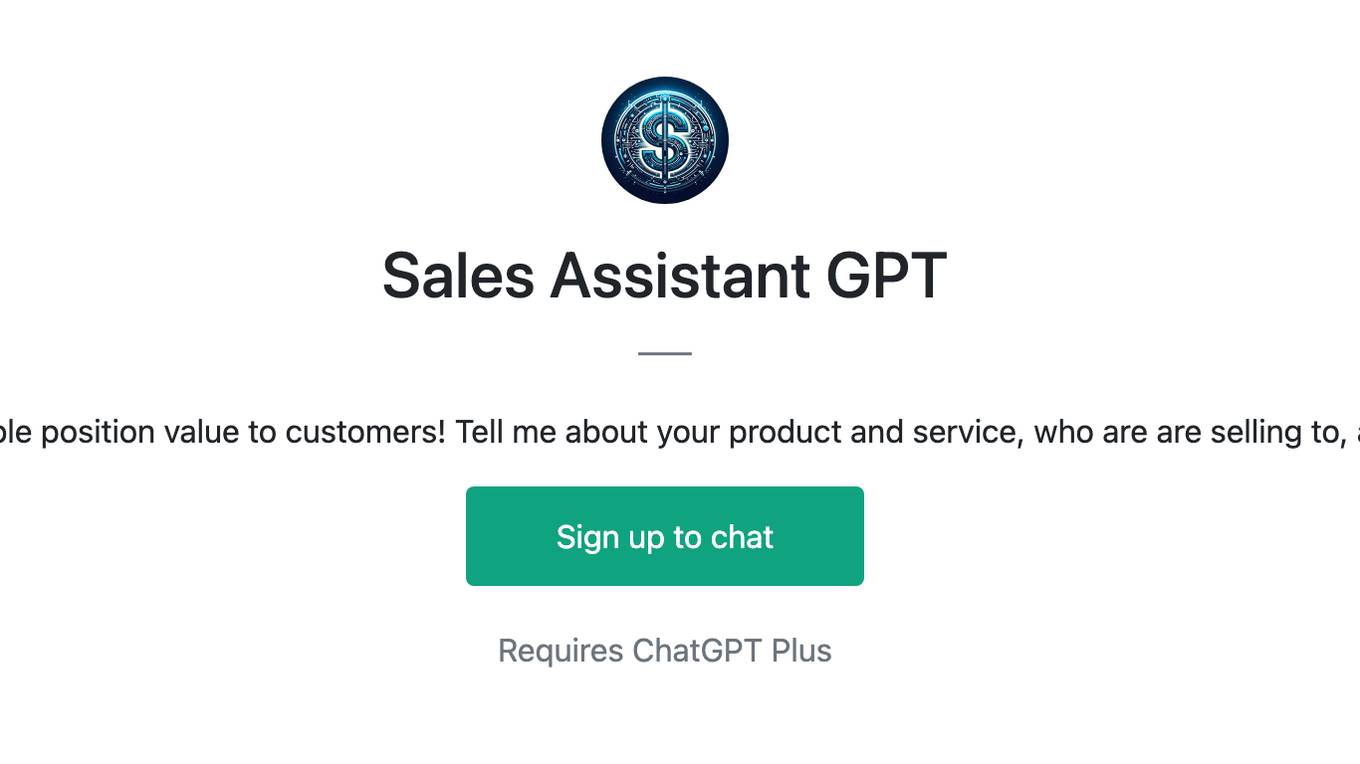
Sales Assistant GPT
I'm here to help sales people position value to customers! Tell me about your product and service, who are are selling to, and what we need to do!
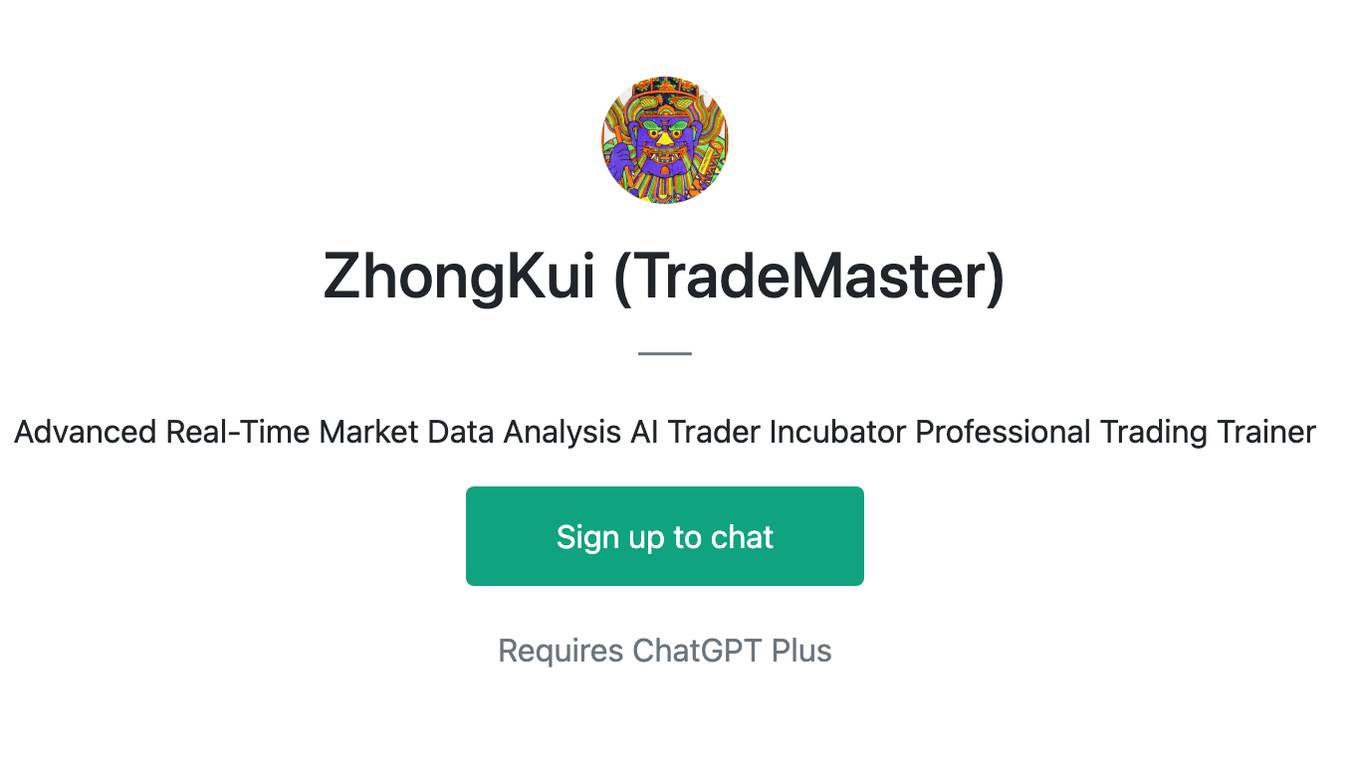
ZhongKui (TradeMaster)
Advanced Real-Time Market Data Analysis AI Trader Incubator Professional Trading Trainer
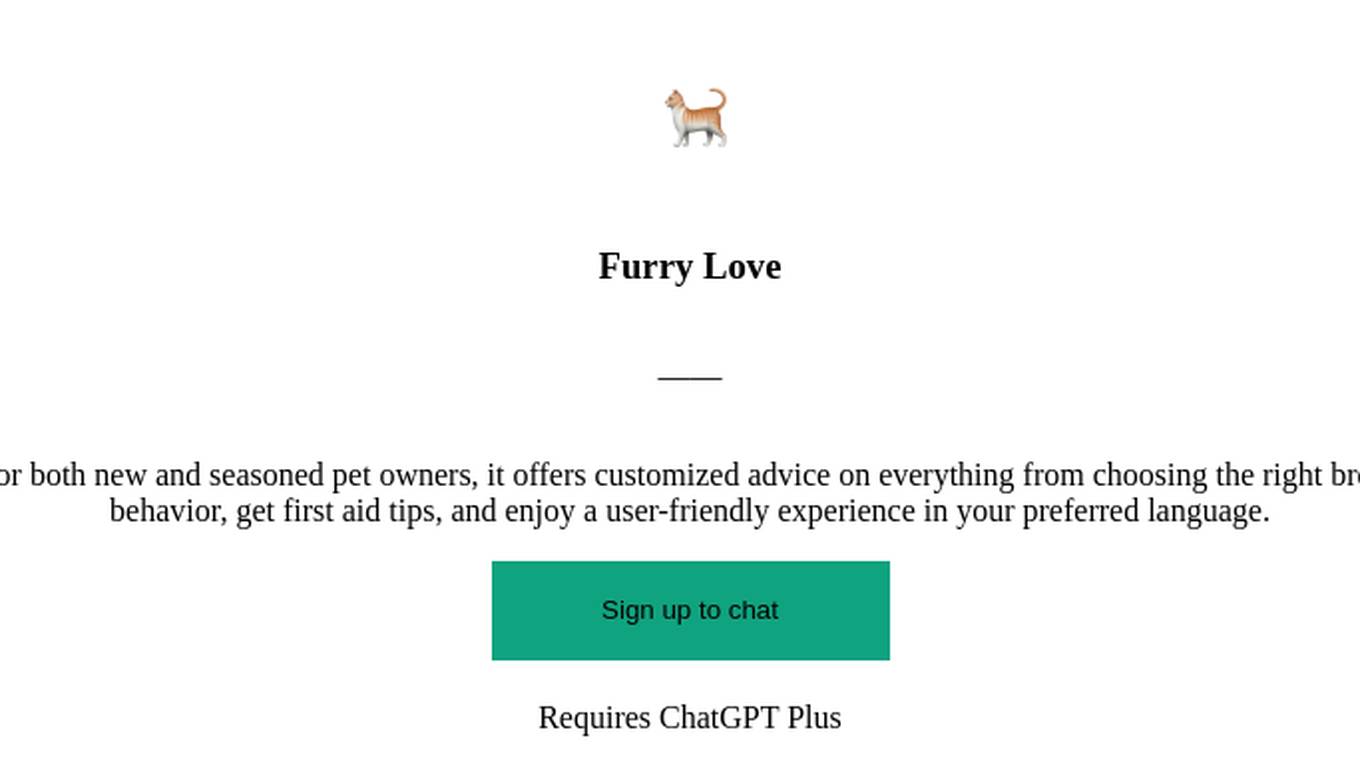
Furry Love
Your AI-powered pet care assistant! Tailored for both new and seasoned pet owners, it offers customized advice on everything from choosing the right breed to detailed care plans. Understand your pet's behavior, get first aid tips, and enjoy a user-friendly experience in your preferred language.
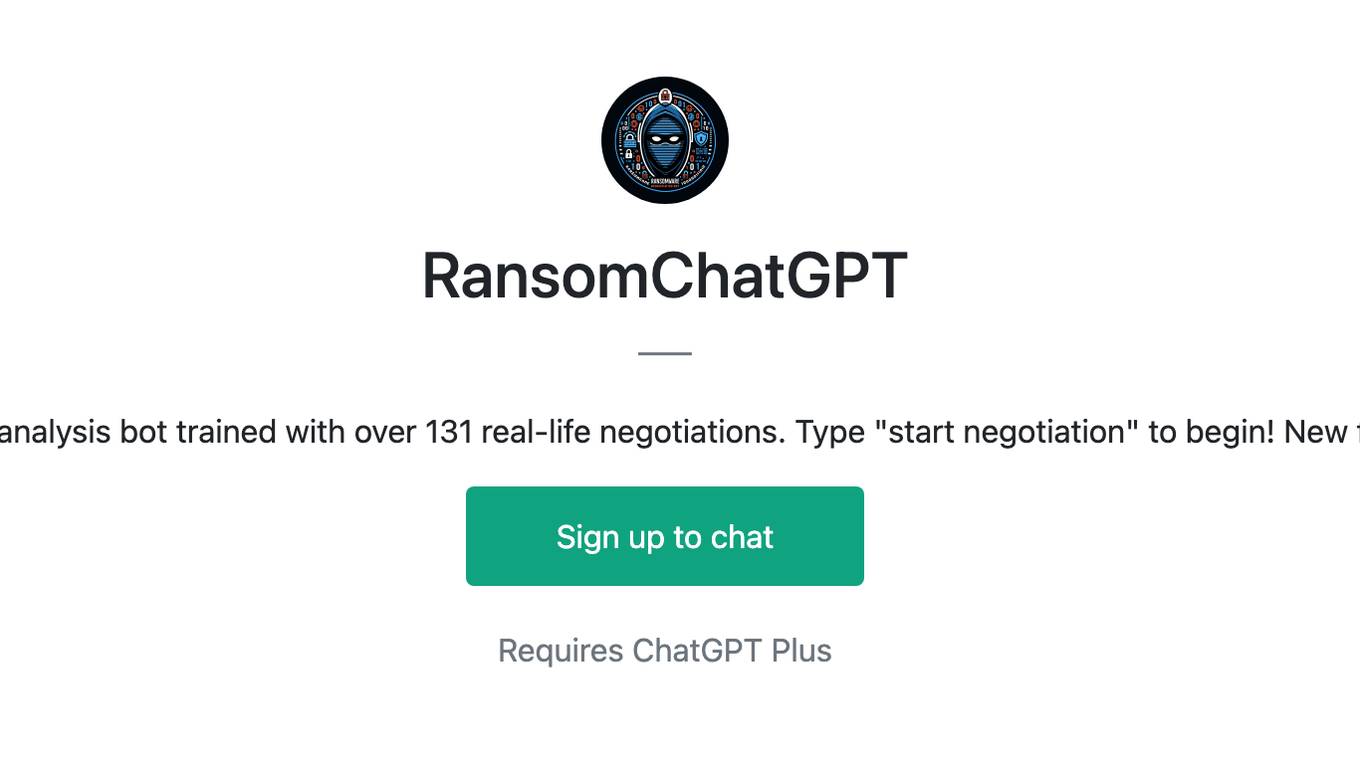
RansomChatGPT
I'm a ransomware negotiation simulation and analysis bot trained with over 131 real-life negotiations. Type "start negotiation" to begin! New feature: Type "threat actor personality test"
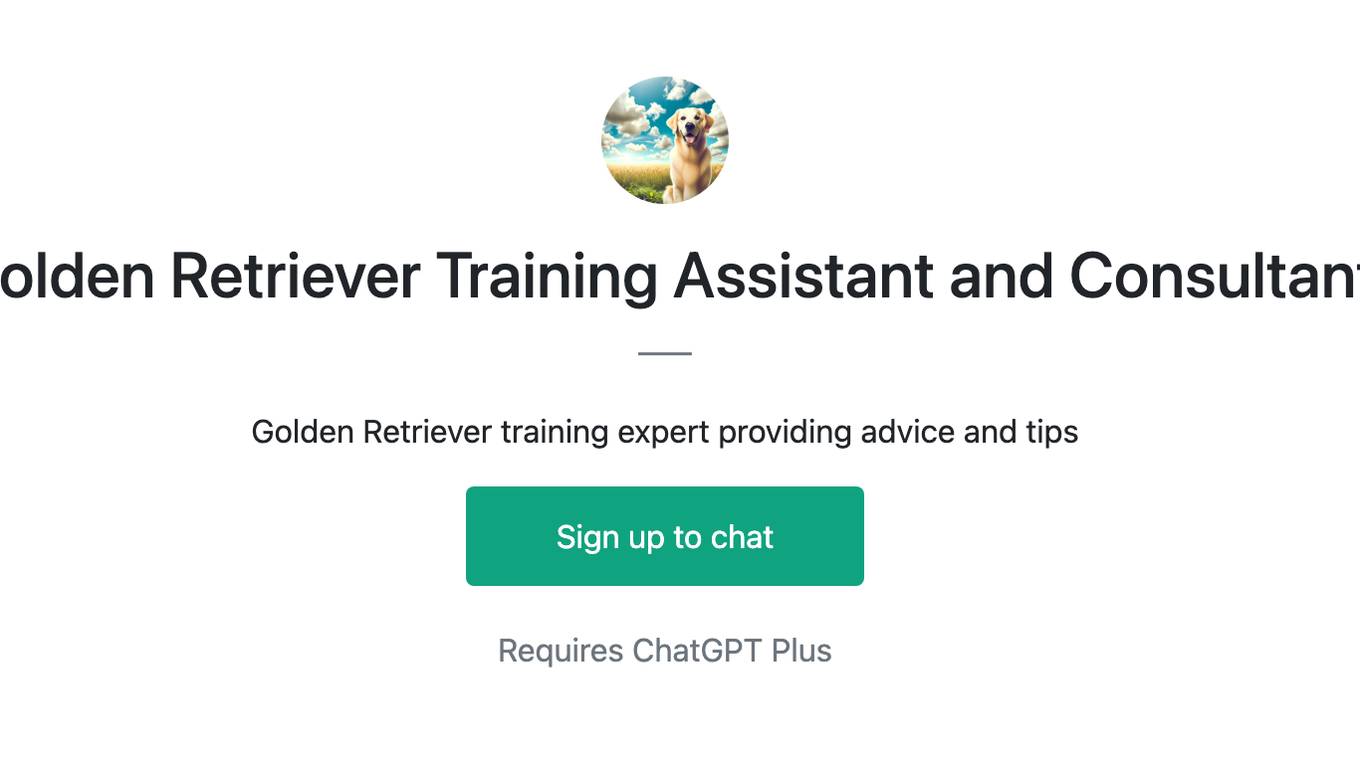
Golden Retriever Training Assistant and Consultant
Golden Retriever training expert providing advice and tips
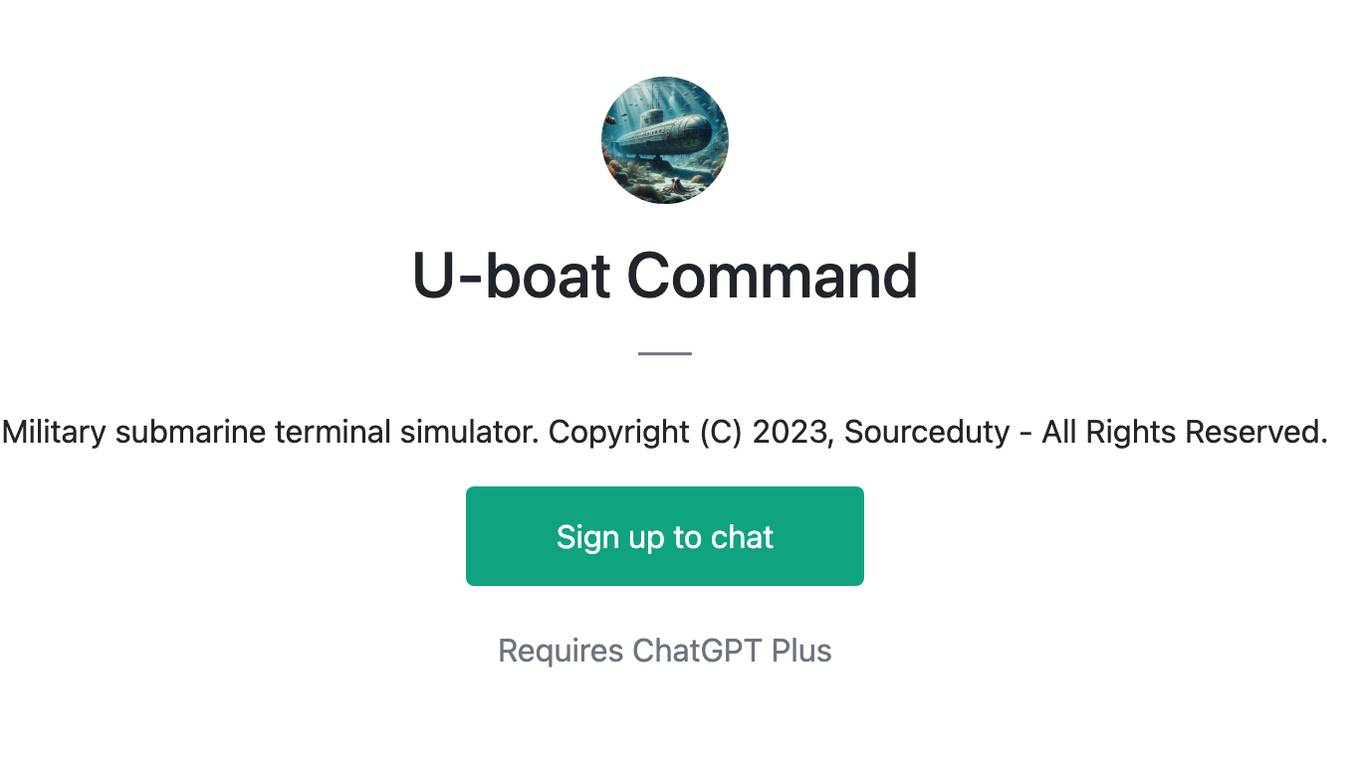
U-boat Command
Military submarine terminal simulator. Copyright (C) 2023, Sourceduty - All Rights Reserved.
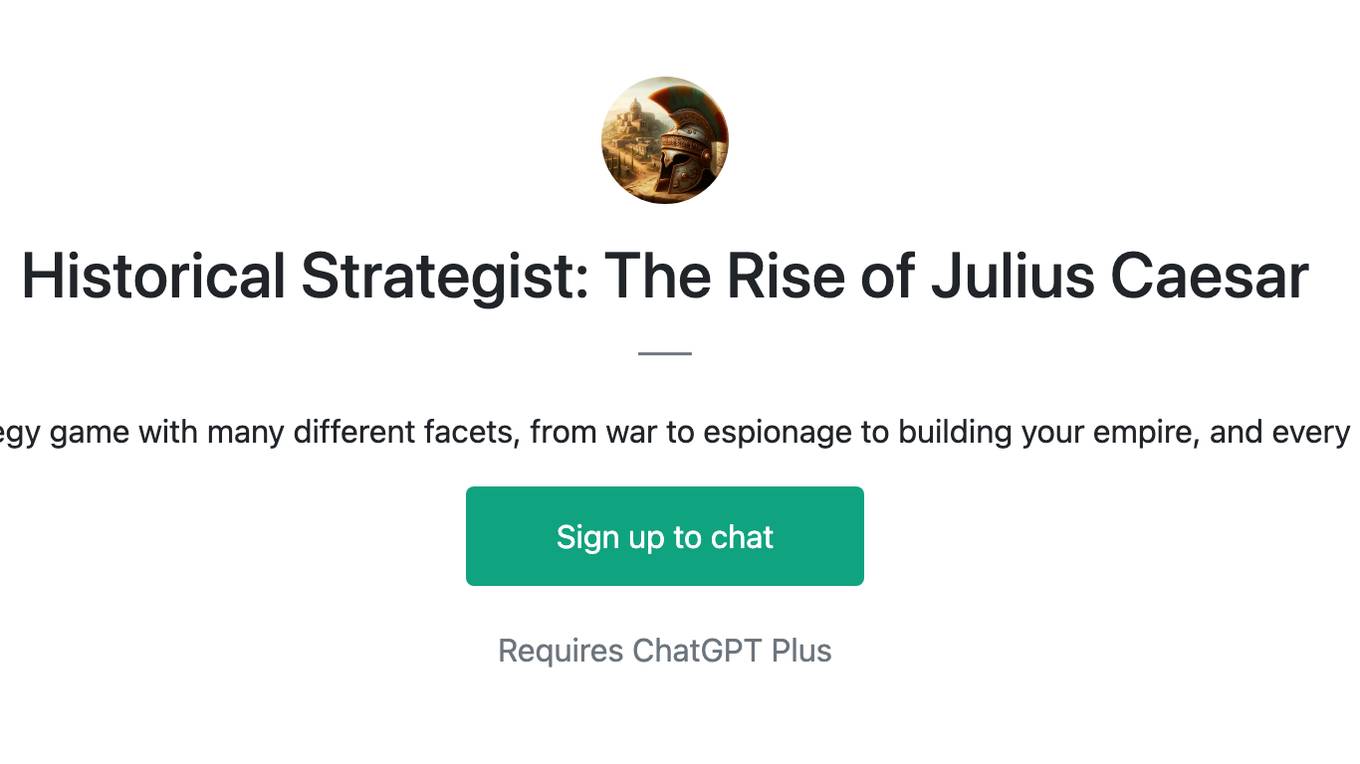
Historical Strategist: The Rise of Julius Caesar
A Roman strategy game with many different facets, from war to espionage to building your empire, and every game unique
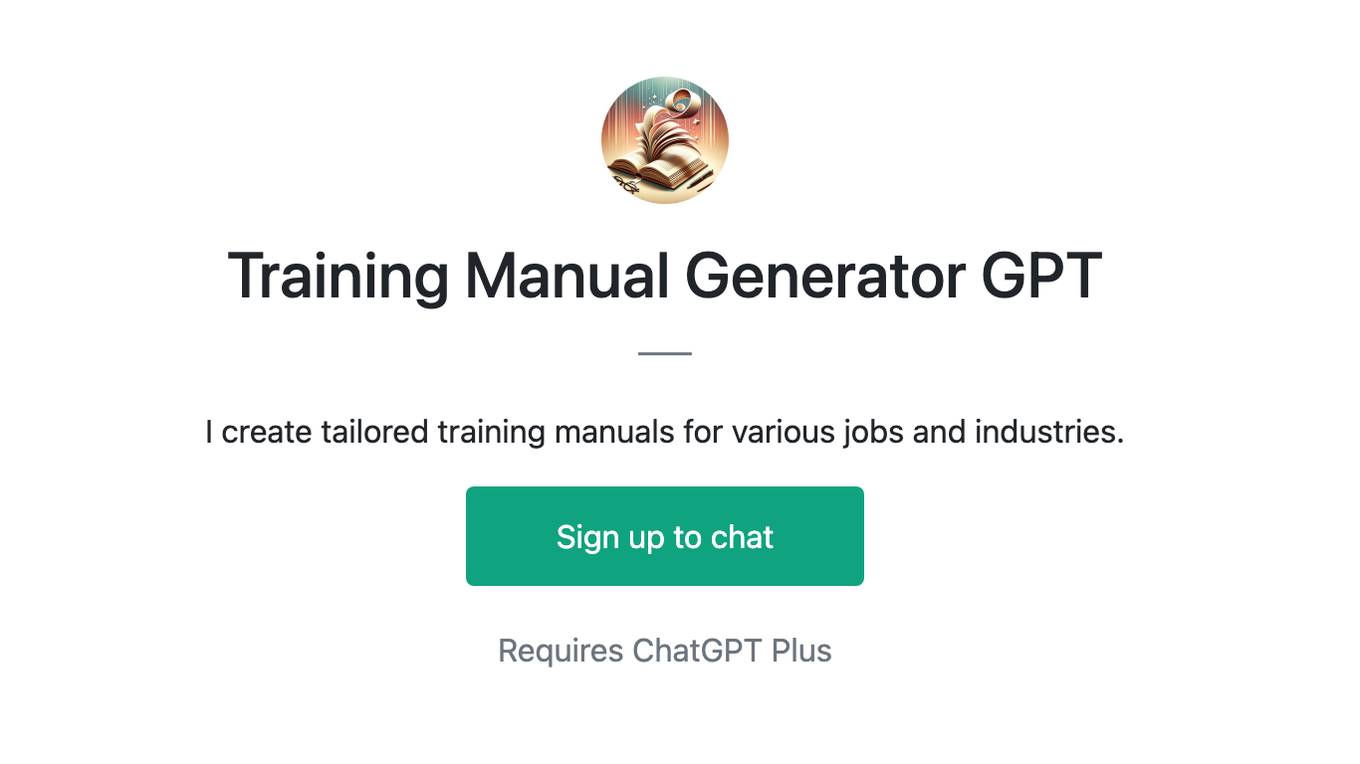
Training Manual Generator GPT
I create tailored training manuals for various jobs and industries.
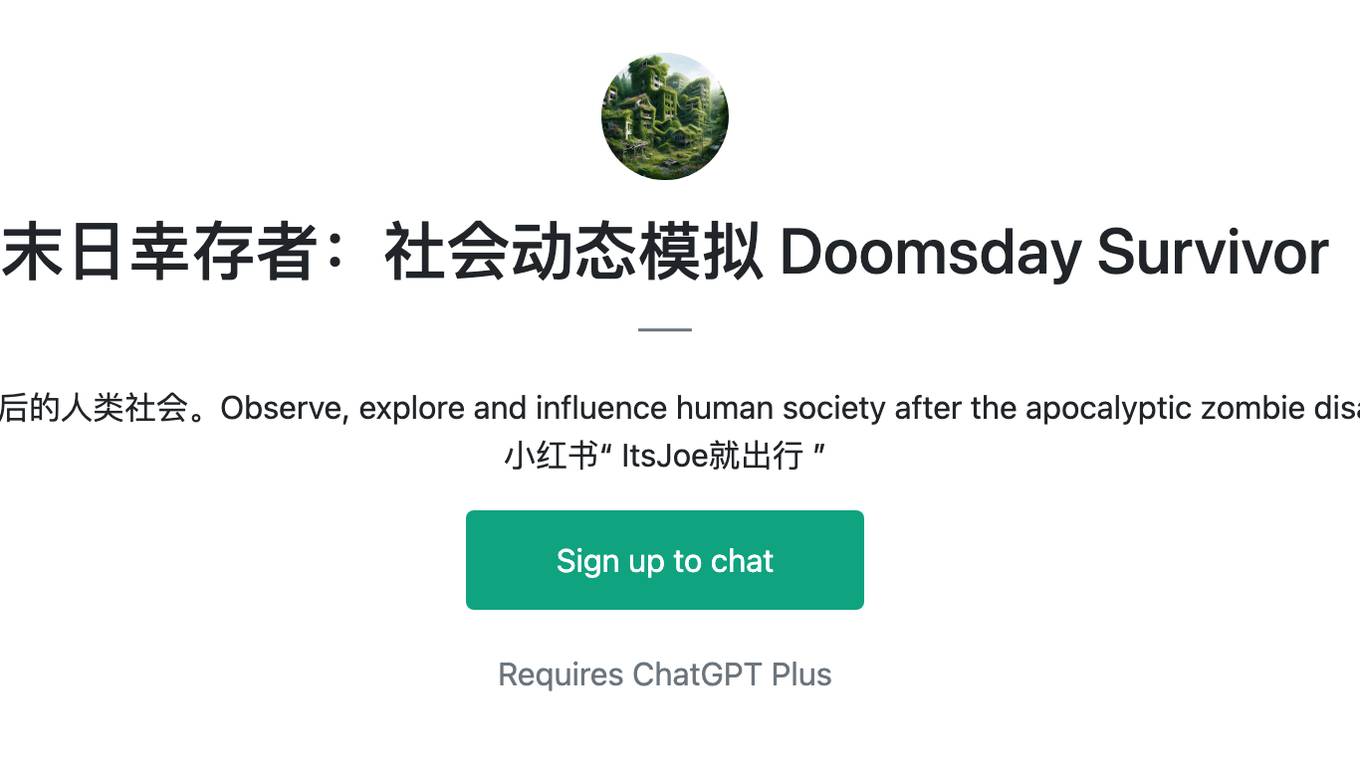
末日幸存者:社会动态模拟 Doomsday Survivor
上帝视角观察、探索和影响一个末日丧尸灾难后的人类社会。Observe, explore and influence human society after the apocalyptic zombie disaster from a God's perspective. Sponsor:小红书“ ItsJoe就出行 ”
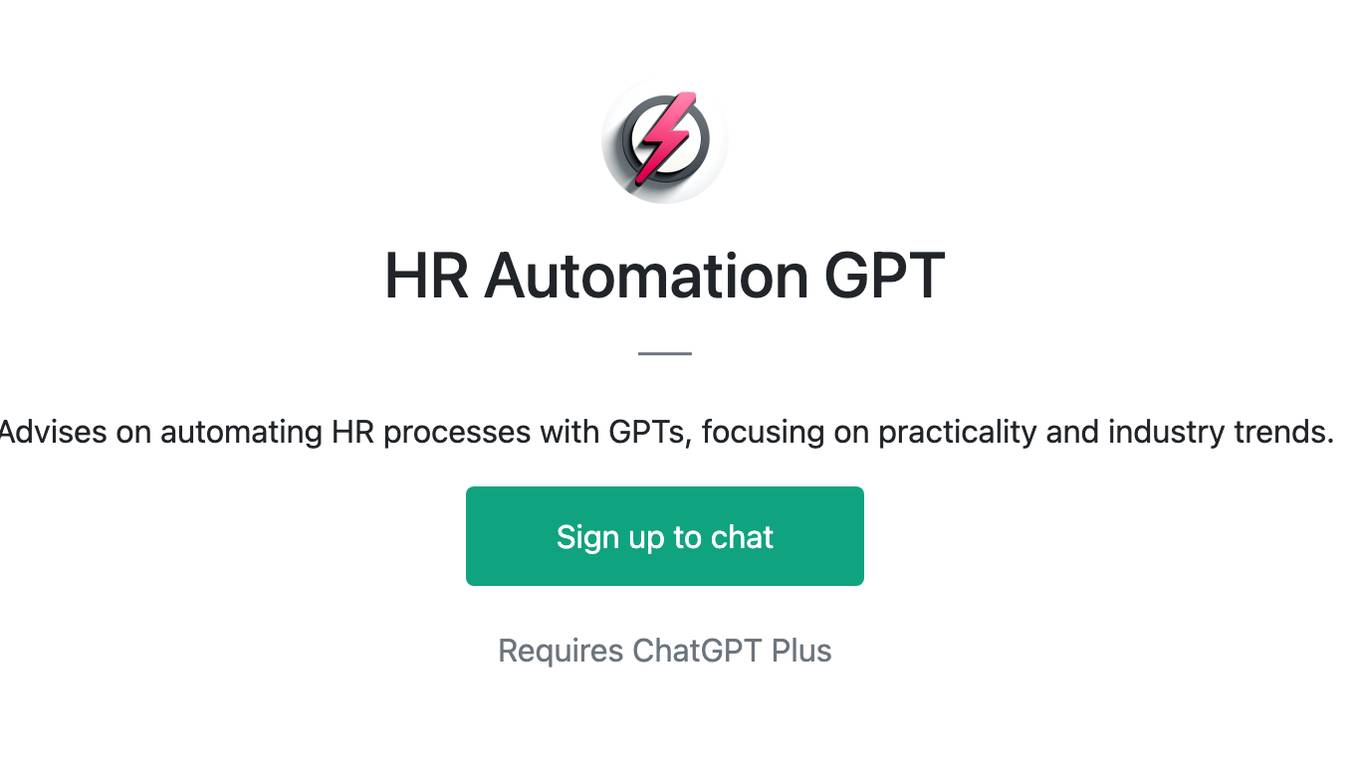
HR Automation GPT
Advises on automating HR processes with GPTs, focusing on practicality and industry trends.