Best AI tools for< Improve Environment >
20 - AI tool Sites
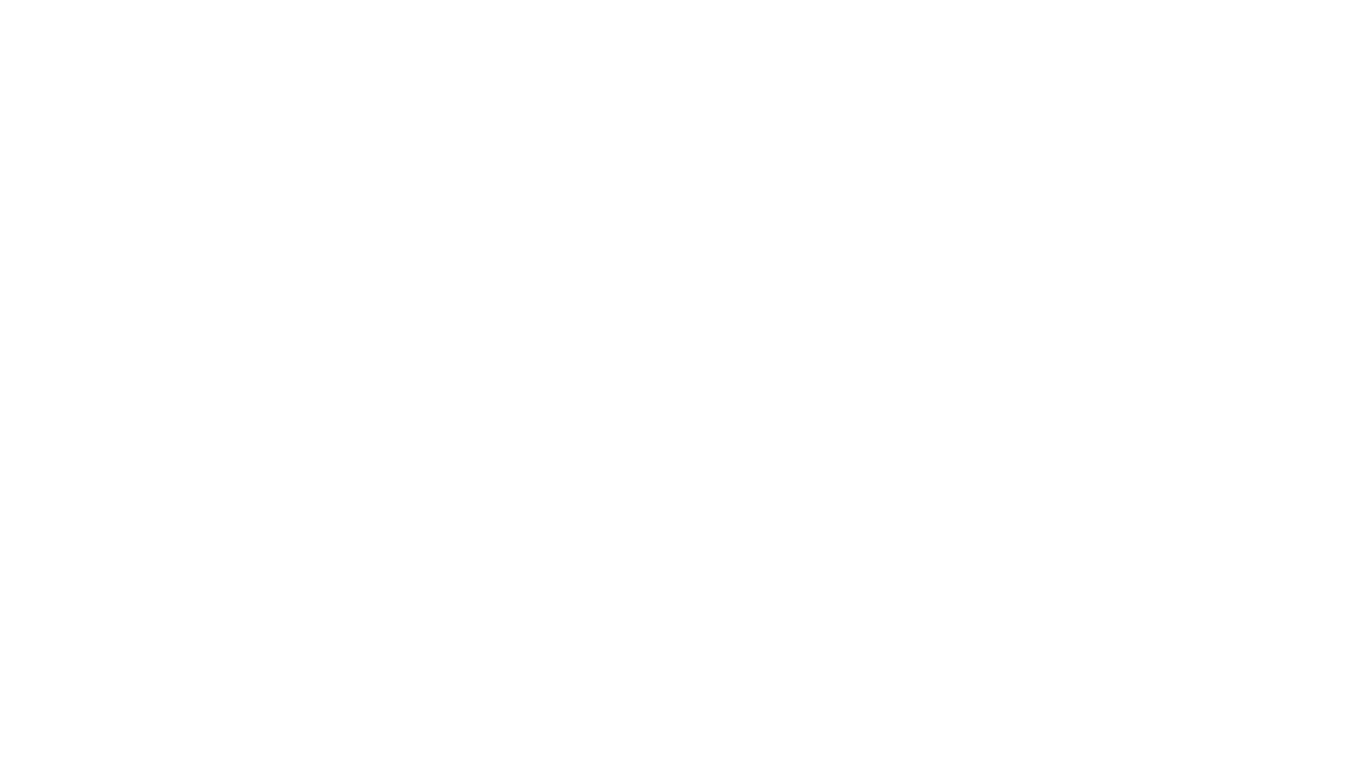
Testmint.ai
Testmint.ai is an online mock test platform designed to help users prepare for competitive exams. It offers a wide range of practice tests and study materials to enhance exam readiness. The platform is user-friendly and provides a simulated exam environment to improve test-taking skills. Testmint.ai aims to assist students and professionals in achieving their academic and career goals by offering a comprehensive and effective exam preparation solution.
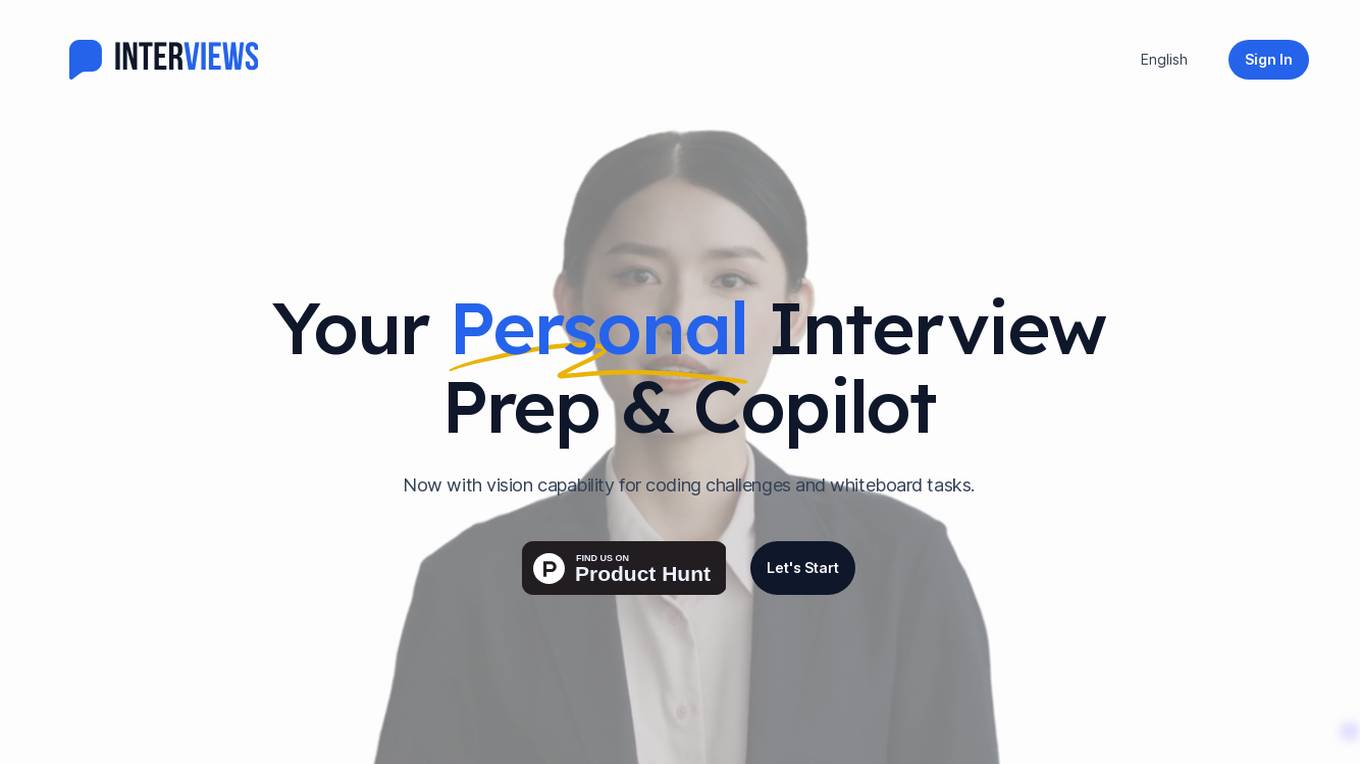
Interviews Chat
Interviews Chat is an AI-powered interview preparation tool that offers real-time suggestions, personalized question preparation, and in-depth feedback to help users ace their interviews. The tool includes a Copilot feature with vision capability for coding challenges and whiteboard tasks. Users can practice answering questions via a video interface, receive tailored interview questions based on their resume and job description, and get instant feedback on their responses. Interviews Chat aims to provide a realistic practice environment to improve interview performance and make a lasting impression.
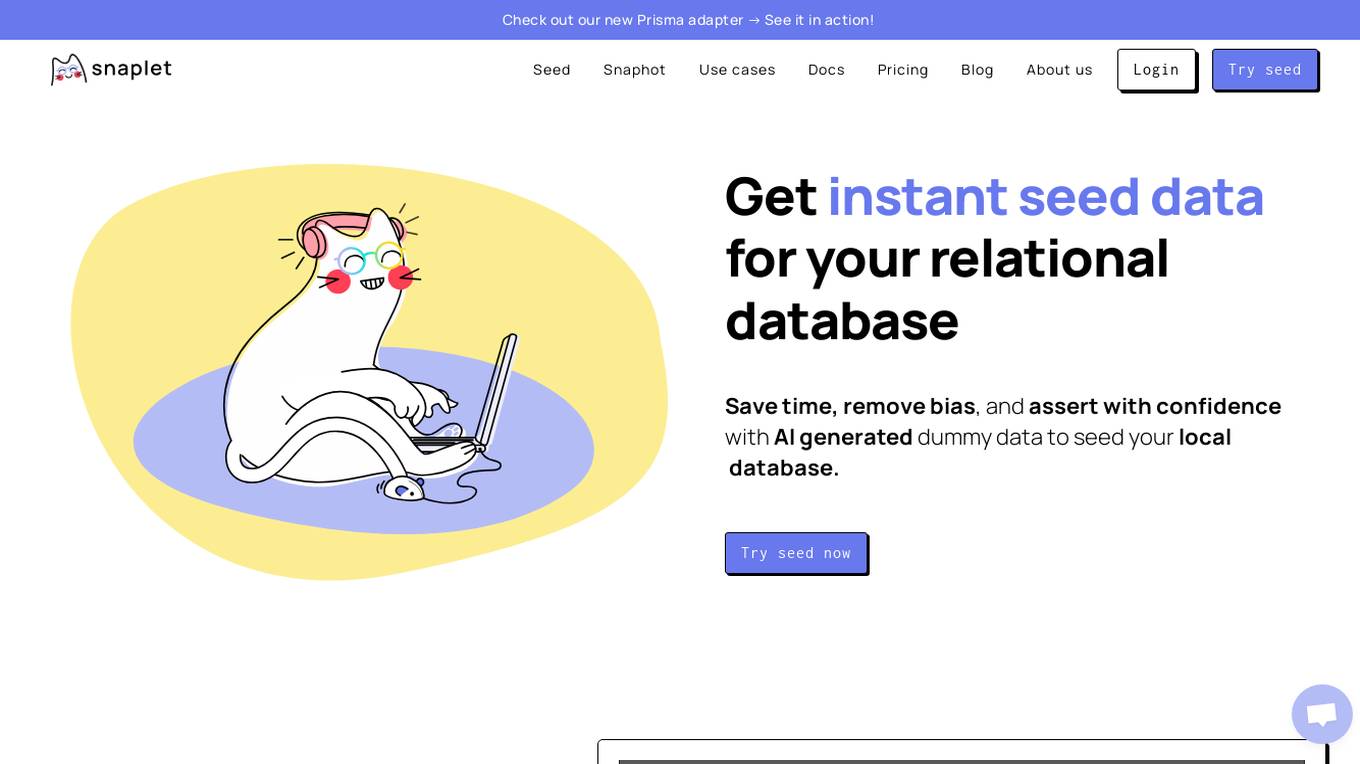
Snaplet
Snaplet is a data management tool for developers that provides AI-generated dummy data for local development, end-to-end testing, and debugging. It uses a real programming language (TypeScript) to define and edit data, ensuring type safety and auto-completion. Snaplet understands database structures and relationships, automatically transforming personally identifiable information and seeding data accordingly. It integrates seamlessly into development workflows, providing data where it's needed most: on local machines, for CI/CD testing, and preview environments.
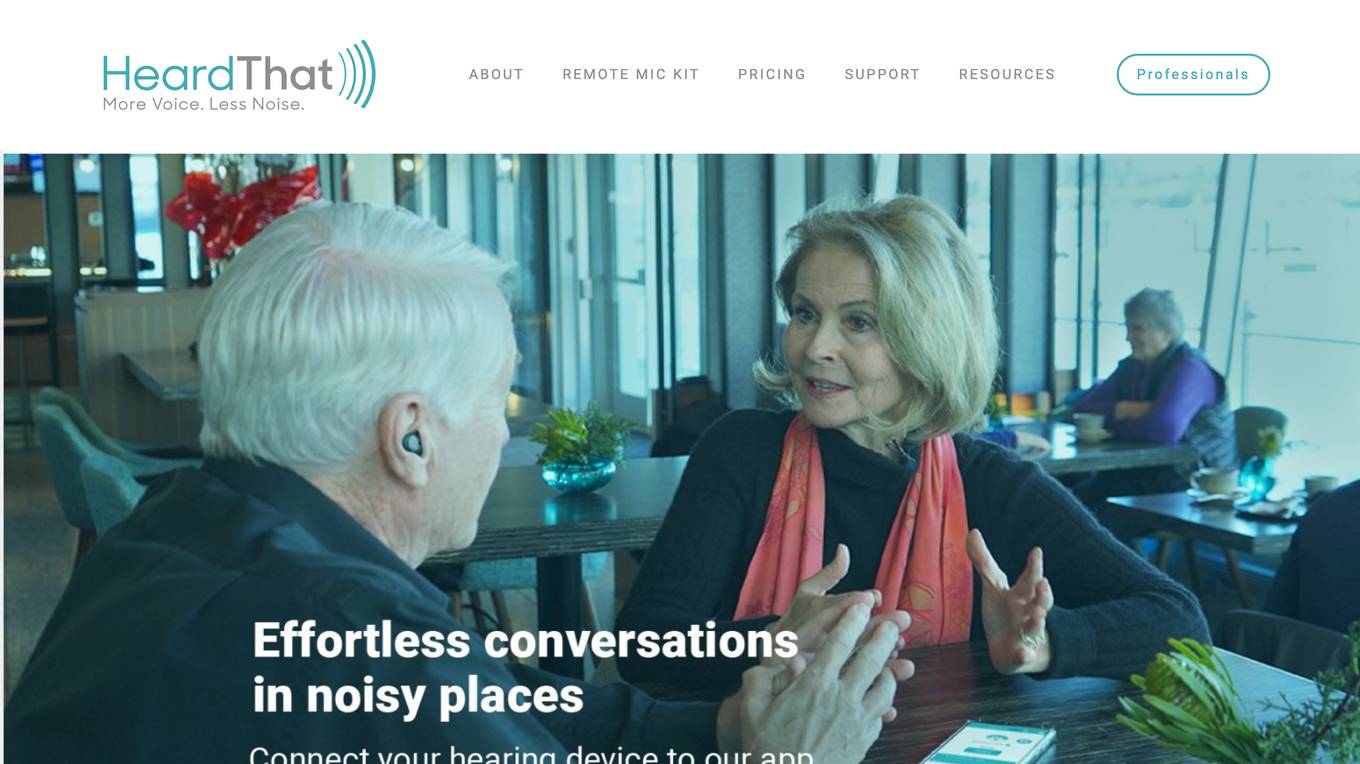
HeardThat
HeardThat is a smartphone application that leverages AI technology to assist users in hearing speech more clearly in noisy environments. By separating speech from background noise, HeardThat enables users to participate in conversations with confidence using their existing Bluetooth earbuds or hearing aids. The app aims to address the common complaint of difficulty in hearing conversations in social settings, which can lead to social isolation. HeardThat provides users with control over ambient sound levels, allowing them to customize their listening experience. The application offers a user-friendly interface and simple steps to enhance speech clarity and reduce background noise.
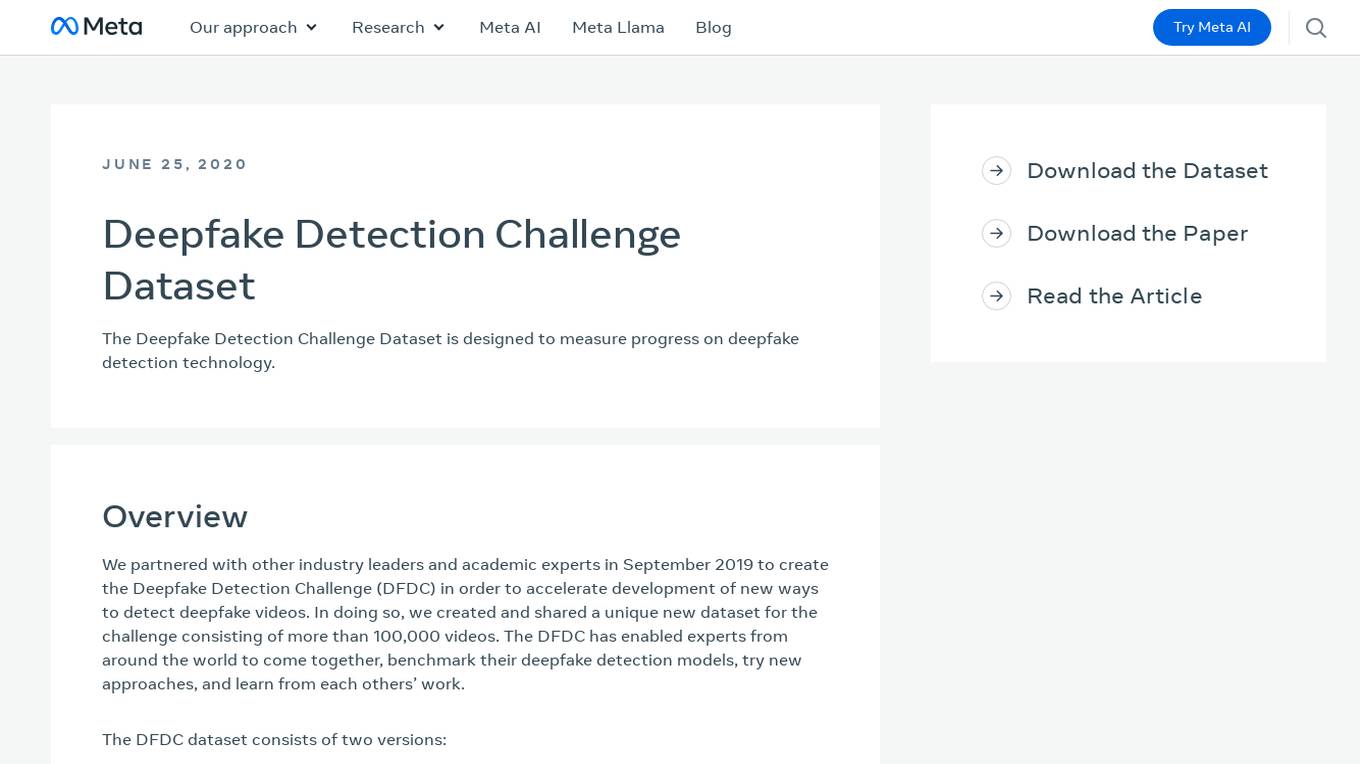
Deepfake Detection Challenge Dataset
The Deepfake Detection Challenge Dataset is a project initiated by Facebook AI to accelerate the development of new ways to detect deepfake videos. The dataset consists of over 100,000 videos and was created in collaboration with industry leaders and academic experts. It includes two versions: a preview dataset with 5k videos and a full dataset with 124k videos, each featuring facial modification algorithms. The dataset was used in a Kaggle competition to create better models for detecting manipulated media. The top-performing models achieved high accuracy on the public dataset but faced challenges when tested against the black box dataset, highlighting the importance of generalization in deepfake detection. The project aims to encourage the research community to continue advancing in detecting harmful manipulated media.
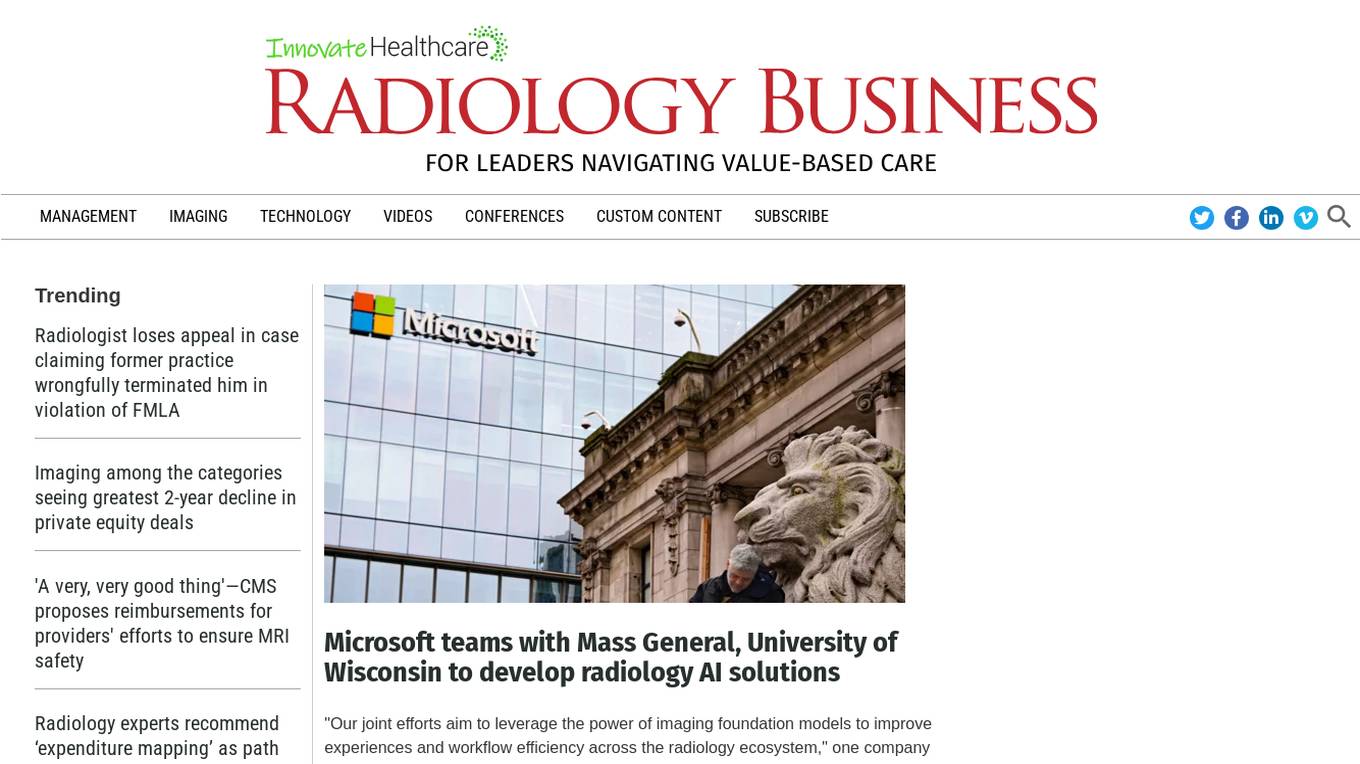
Radiology Business
Radiology Business is an AI tool designed to provide insights and solutions for professionals in the radiology field. The platform covers a wide range of topics including management, imaging, technology, and conferences. It offers news, analysis, and resources to help radiologists stay informed and make informed decisions. Radiology Business aims to leverage artificial intelligence to improve workflow efficiency and enhance the overall experience in the radiology ecosystem.
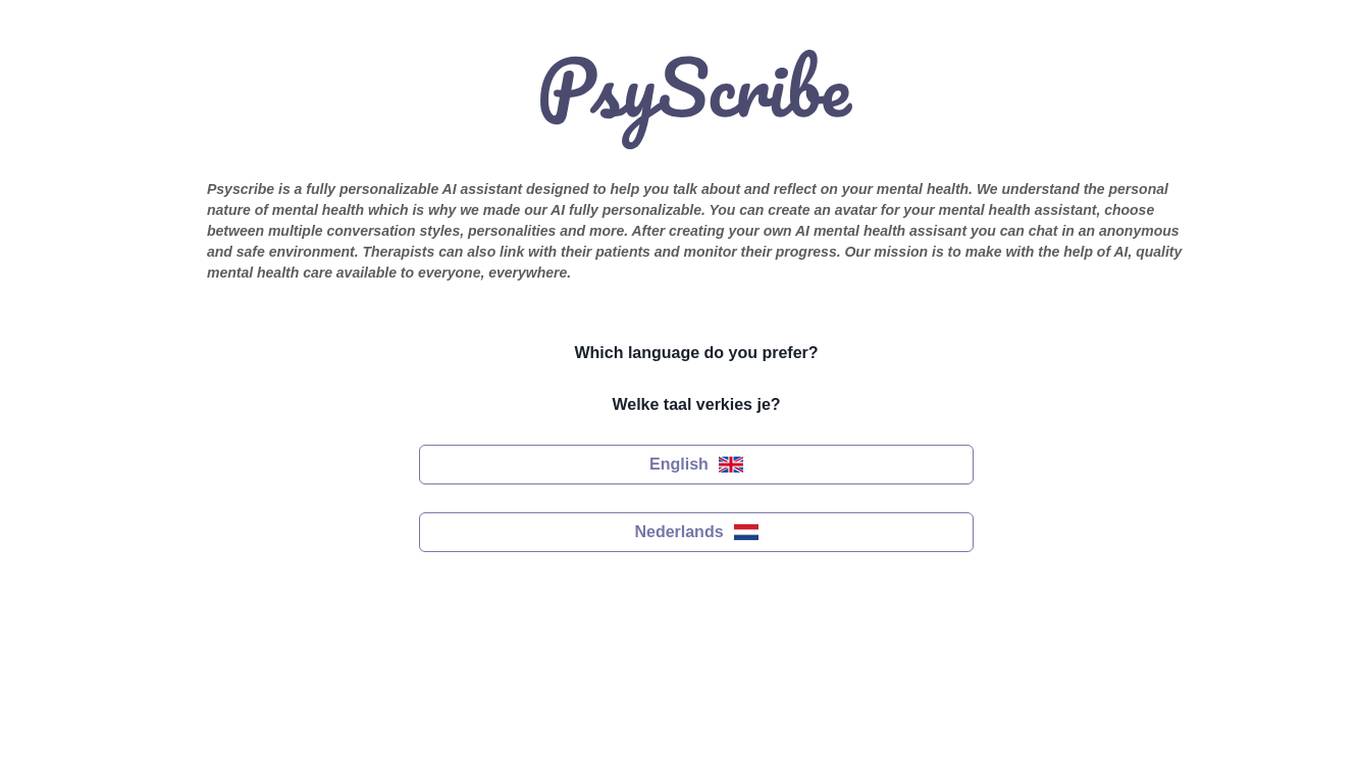
PsyScribe
PsyScribe is an AI-powered platform that serves as your personal therapist and mental health support system. By leveraging advanced artificial intelligence algorithms, PsyScribe provides users with a confidential and accessible space to express their thoughts and emotions, receive personalized insights, and access mental health resources. Whether you're seeking guidance, coping strategies, or simply a listening ear, PsyScribe is designed to support your emotional well-being effectively and conveniently.
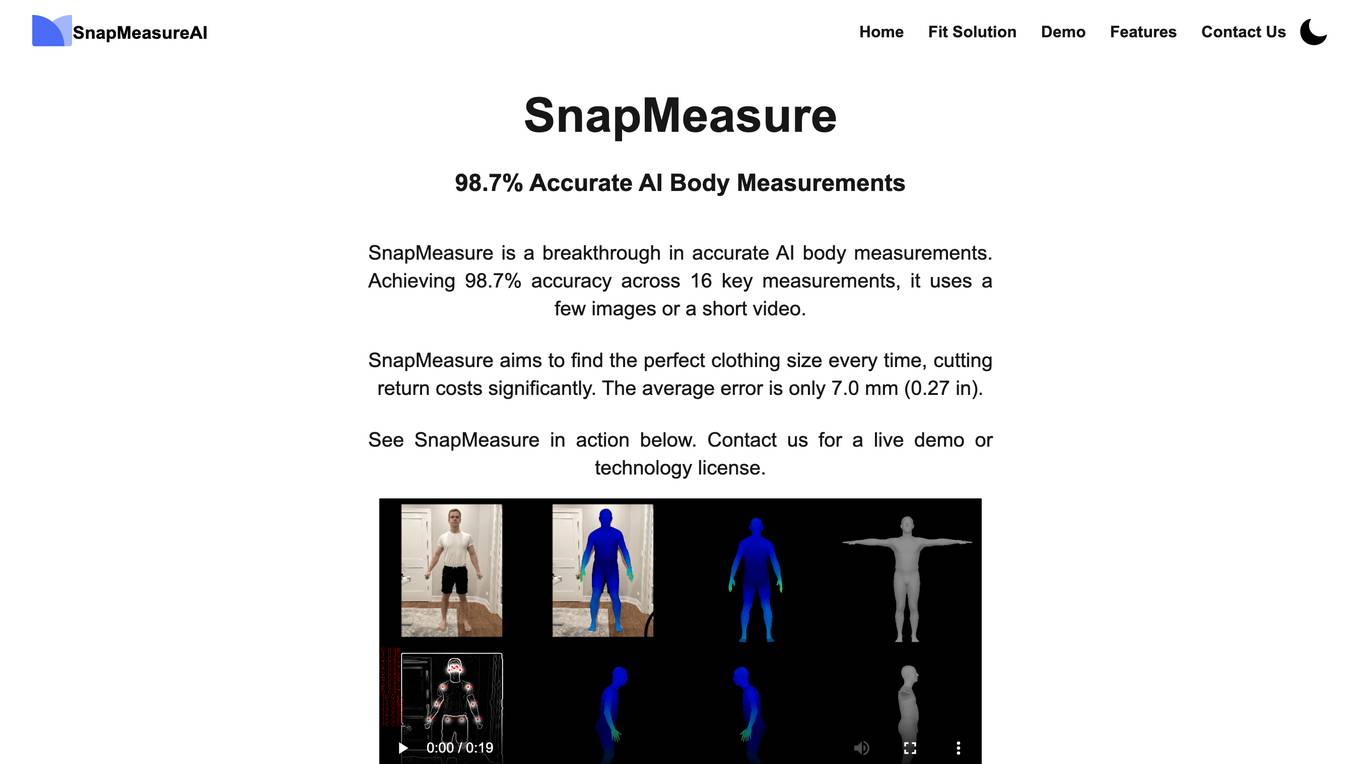
SnapMeasureAI
SnapMeasureAI is an AI-powered application that provides 99% accurate body measurements without the need to visit a tailor. It uses advanced AI technology to analyze body scans from photos or videos, offering unparalleled body shape and measurement precision. With over 100 million body combinations and 400,000 backgrounds trained, SnapMeasureAI ensures reliable performance in diverse scenarios. The application is designed to help users find their perfect fit, reduce returns, save costs, and increase shopping confidence.
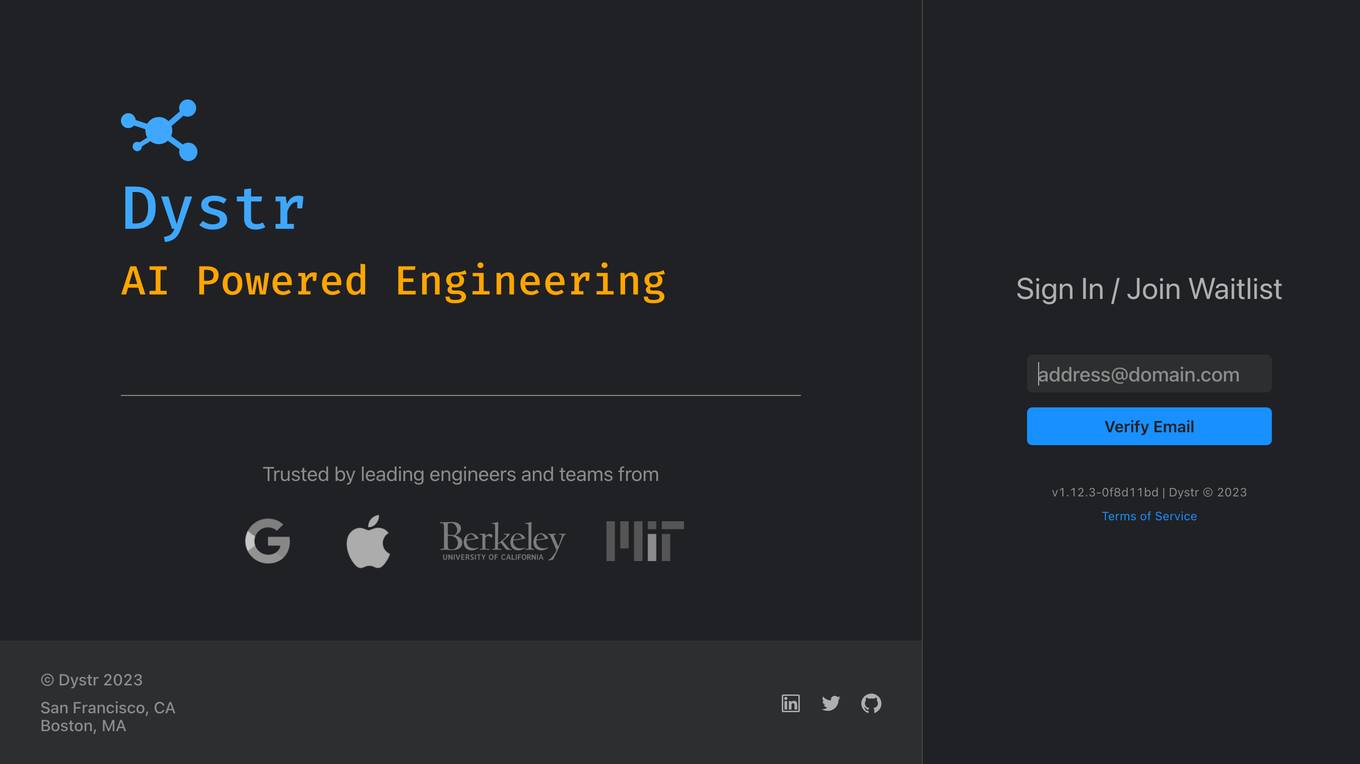
Dystr
Dystr is an AI-powered analysis tool that helps businesses make better decisions. It uses machine learning and natural language processing to analyze data and identify trends and patterns. Dystr can be used to analyze a variety of data sources, including text, images, and videos. It can also be used to analyze data from social media, customer surveys, and other sources.
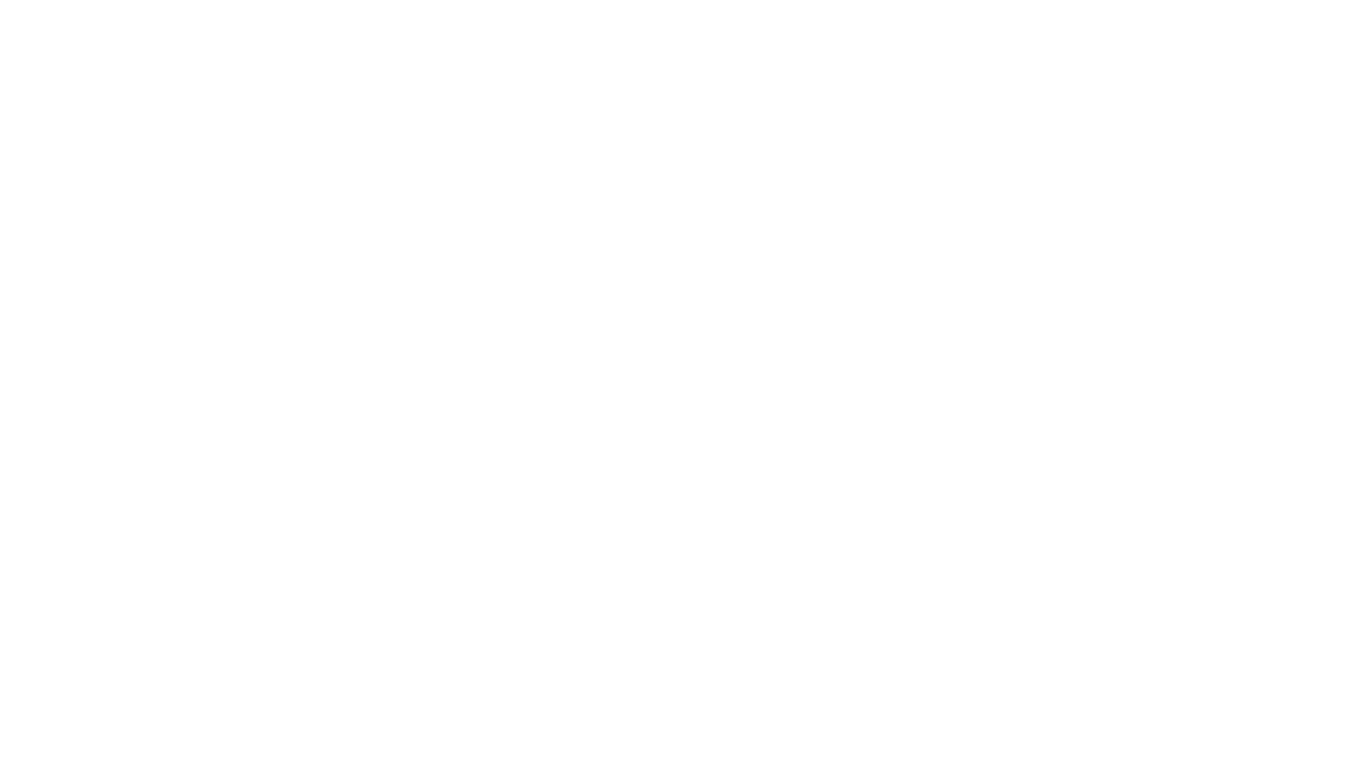
CodeSignal
CodeSignal is an AI-powered platform that helps users discover and develop in-demand skills. It offers skills assessments and AI-powered learning tools to help individuals and teams level up their skills. The platform provides solutions for talent acquisition, technical interviewing, skill development, and more. With features like pre-screening, interview assessments, and personalized learning, CodeSignal aims to help users advance their careers and build high-performing teams.
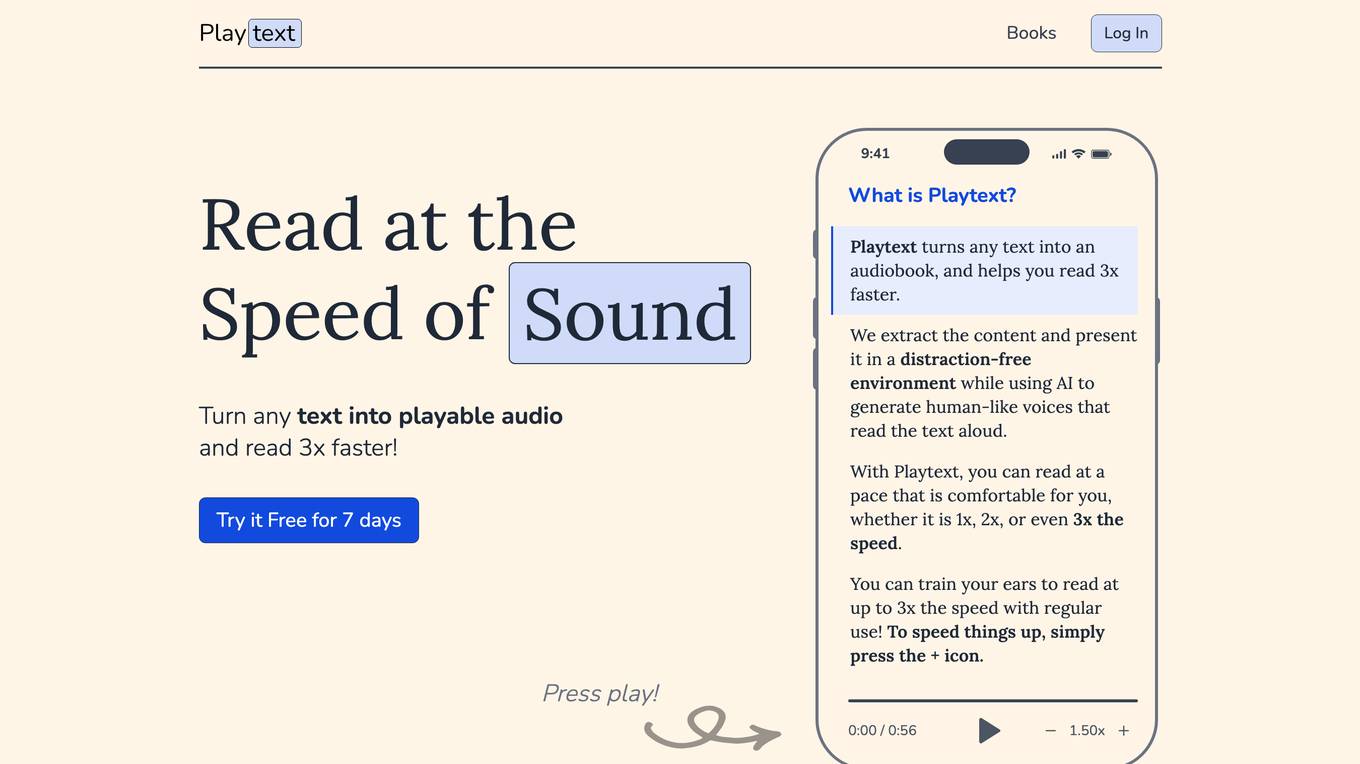
Playtext
Playtext is a web application that allows users to save web articles and convert them into audiobooks. In a world filled with short attention spans and information overload, Playtext aims to help users read more by providing a read-it-later app similar to Pocket or Instapaper. Users can have their favorite articles read aloud to them by human-like voices, and even train their ears to read at up to 3x the speed. By enabling users to read and listen simultaneously, Playtext enhances content retention and comprehension, offering a new way to enjoy reading and consuming information.
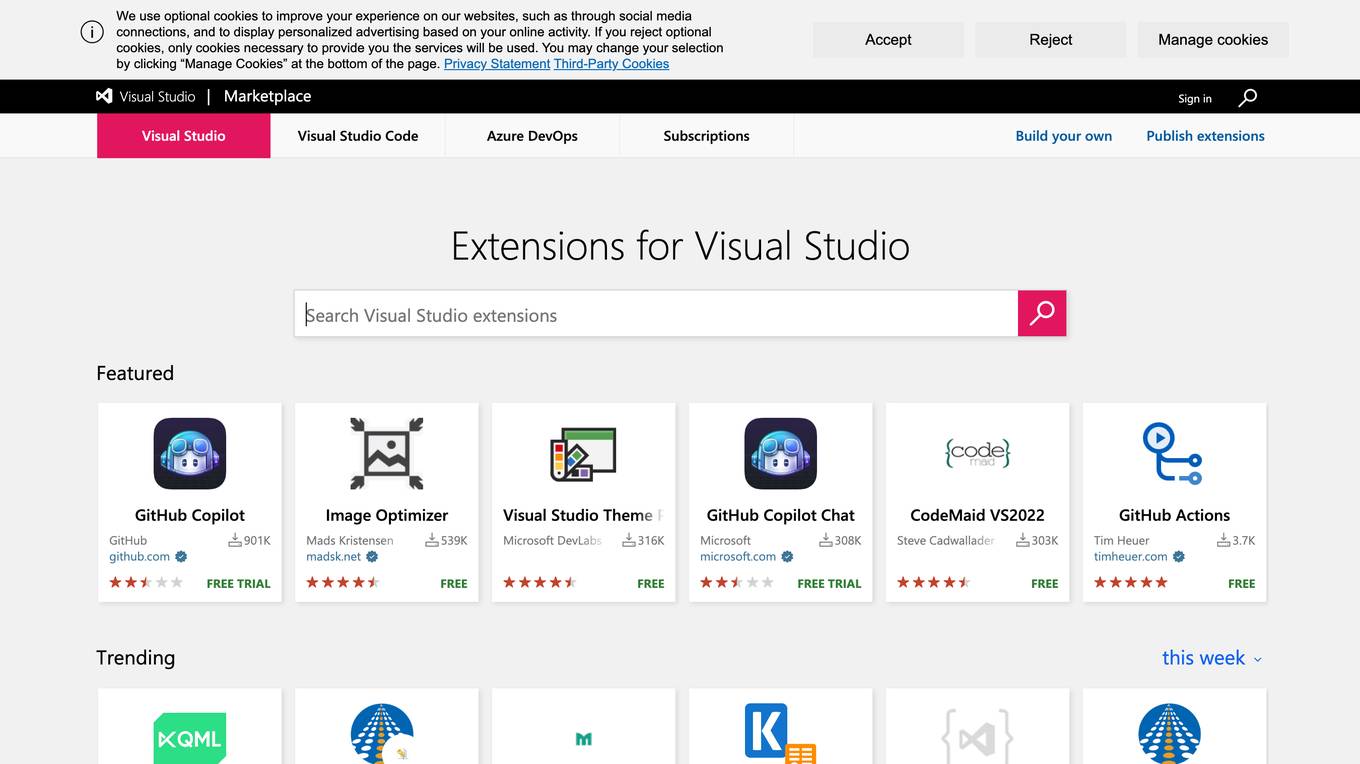
Visual Studio Marketplace
The Visual Studio Marketplace is a platform where users can find and publish extensions for Visual Studio family of products. It offers a wide range of extensions to enhance the functionality and features of Visual Studio, Visual Studio Code, Azure DevOps, and more. Users can customize their development environment with themes, tools, and integrations to improve productivity and efficiency.
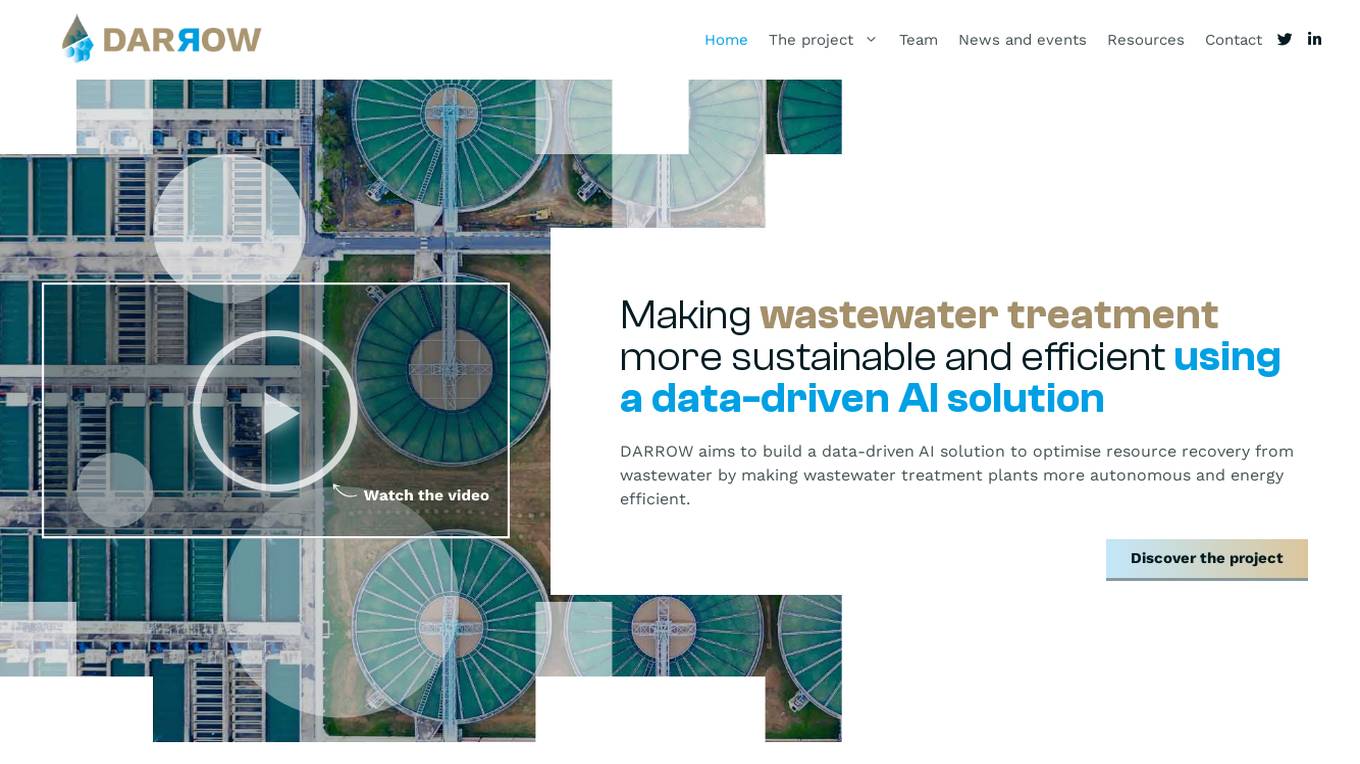
DARROW
The website focuses on the DARROW project, which aims to make wastewater treatment more sustainable and efficient using a data-driven AI solution. It brings together experts from various disciplines to optimize resource recovery from wastewater, reduce energy consumption, and contribute to a circular economy. The project utilizes AI to enhance operational efficiency in wastewater treatment plants and explores innovative solutions for cleaner water management.
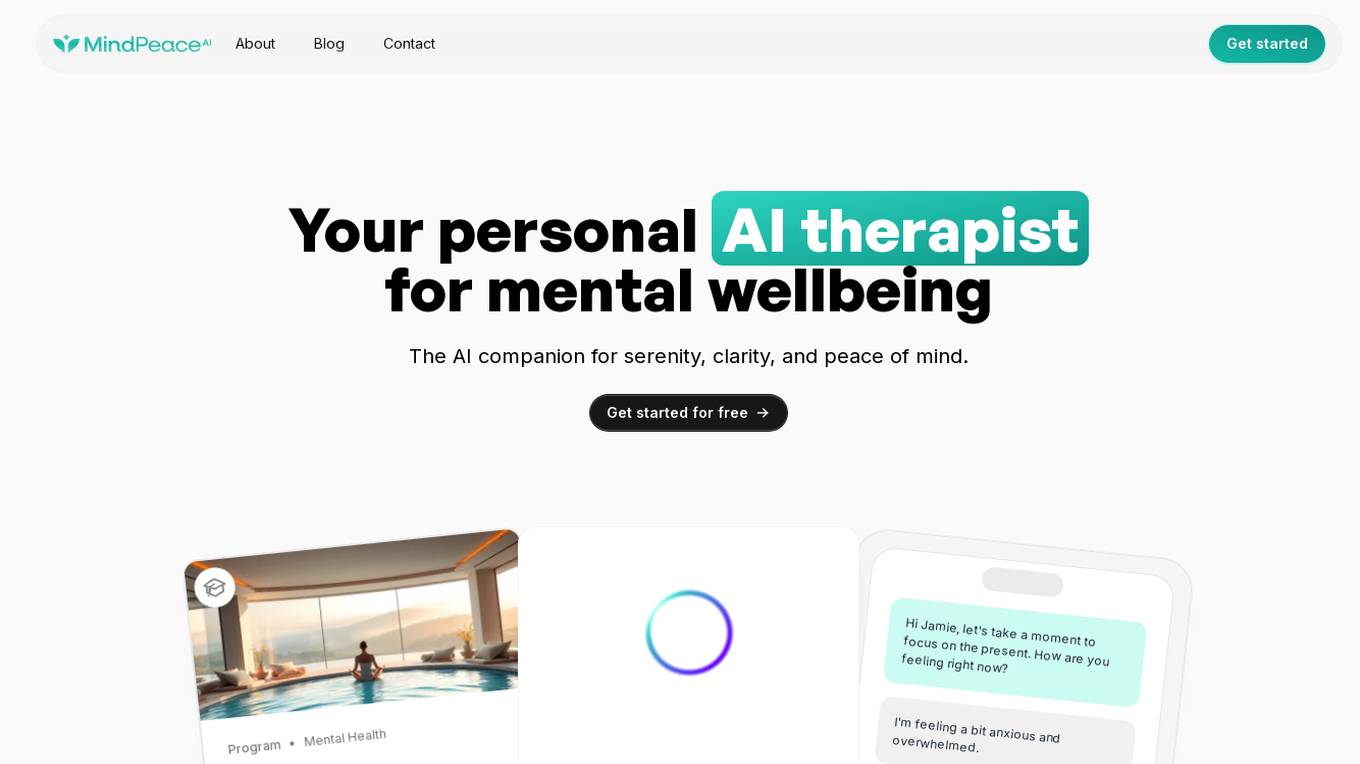
MindPeace AI
MindPeace AI is an AI-powered platform that serves as a personal mental wellbeing companion, offering therapeutic techniques to guide users towards self-reflection, relaxation, and emotional balance. It provides tools, exercises, and gentle reminders in a convenient, stigma-free environment. The platform is designed to complement traditional therapy by providing on-demand support, but it does not replace human therapists. MindPeace AI prioritizes privacy and safety, ensuring that conversations are private and personal information is never shared with third parties.
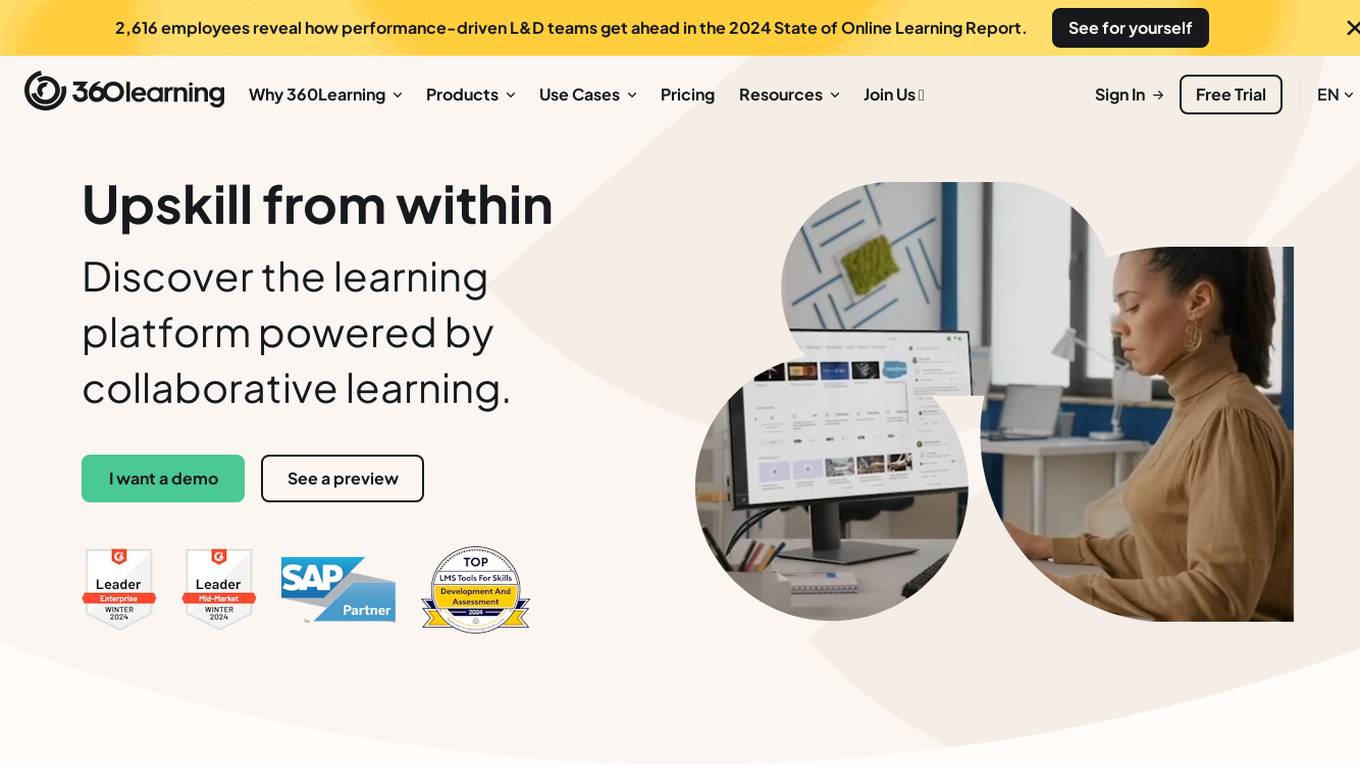
360Learning
360Learning is a comprehensive learning platform that leverages AI and collaborative features to transform in-house experts into L&D collaborators. It enables organizations to upskill quickly and continuously within their own environment. The platform offers a range of features to facilitate collaborative learning, course creation, compliance training, employee onboarding, sales enablement, and frontline staff training. With a focus on data protection and security, 360Learning is trusted by over 2,300 customers for its innovative approach to corporate learning.
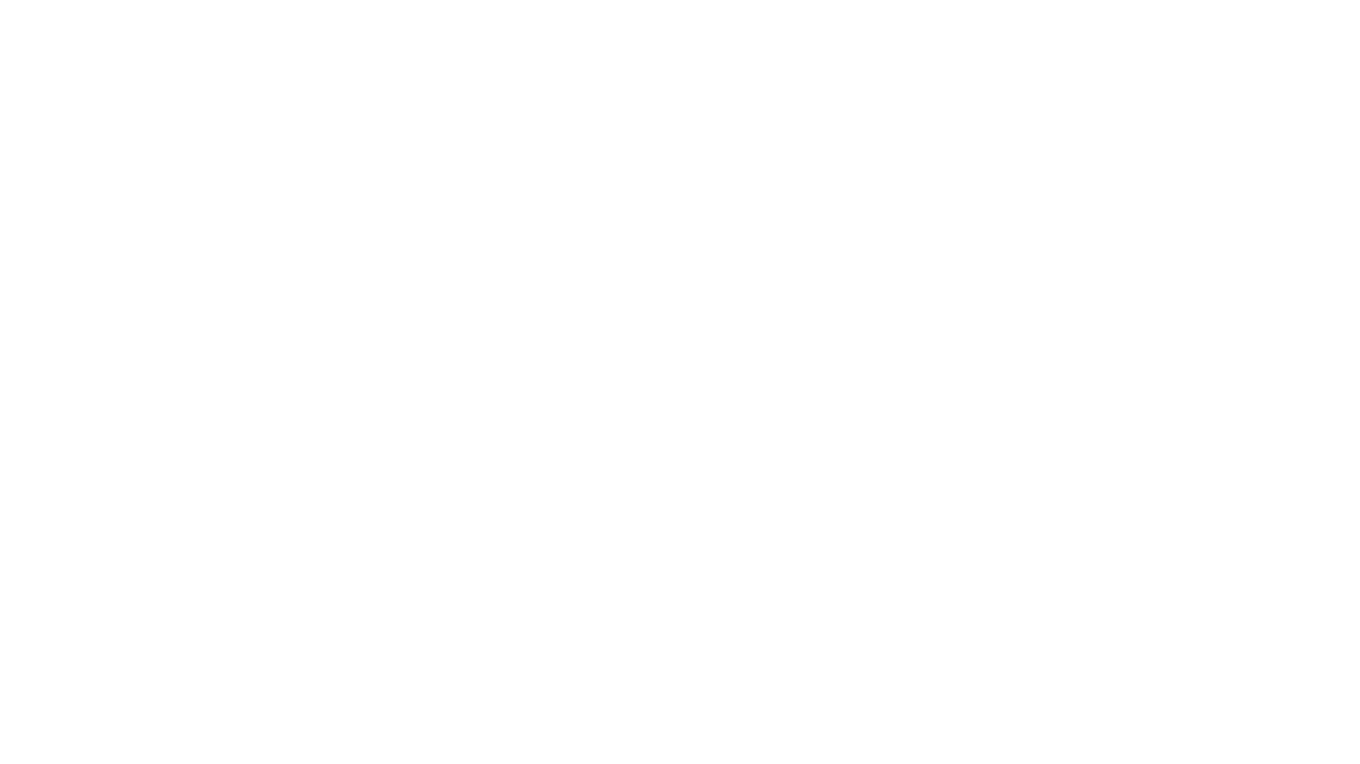
Wilco
Wilco is an AI-powered learning platform that offers personalized, immersive, and endless learning experiences for individuals, employees, customers, and candidates. It provides a comprehensive library of AI and technical training curriculum, adaptive learning technology, and a creator platform for generating hands-on labs. Wilco's AI engine tracks user progress and community data, offering a unique and engaging learning environment. The platform is trusted by leading products and offers quests in various programming languages, making learning fun and interactive.
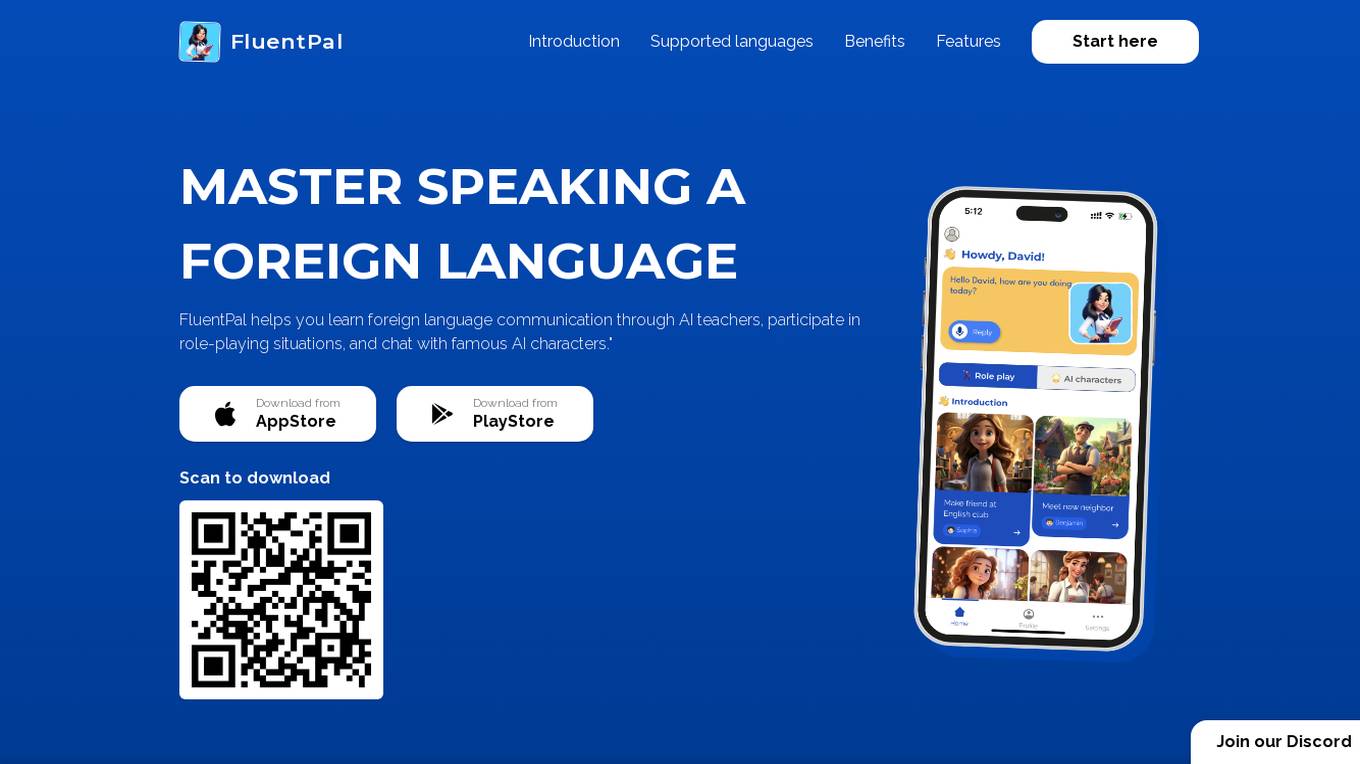
FluentPal
FluentPal is a language learning application that leverages AI technology to provide users with an immersive foreign language learning experience. The app offers a range of features such as role-playing scenarios, conversations with AI celebrities, custom conversation creation, suggested responses, translation support, error correction, and adjustable AI conversation levels. FluentPal aims to simulate a foreign language environment to enhance communication skills and pronunciation accuracy, making language learning accessible, cost-effective, and beginner-friendly.
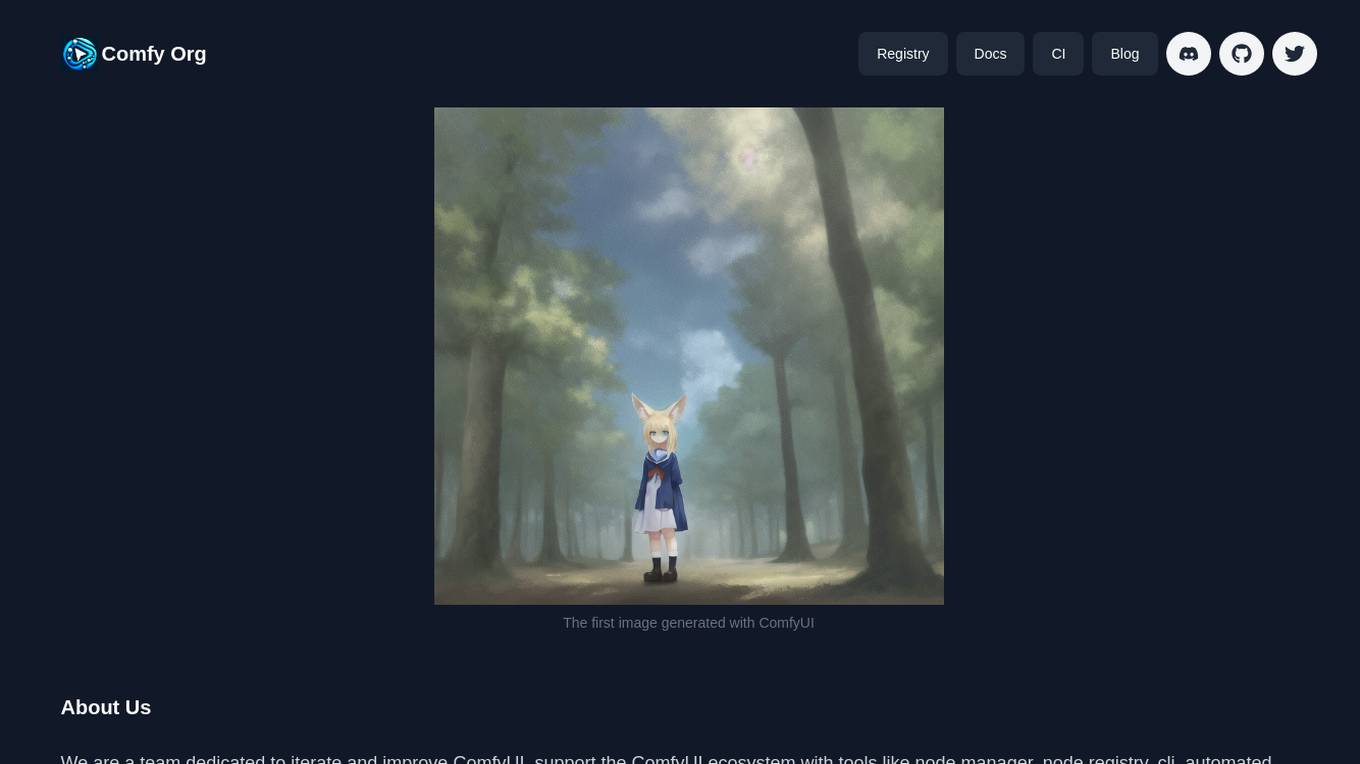
Comfy Org
Comfy Org is an open-source AI tooling platform dedicated to advancing and democratizing AI technology. The platform offers tools like node manager, node registry, CLI, automated testing, and public documentation to support the ComfyUI ecosystem. Comfy Org aims to make state-of-the-art AI models accessible to a wider audience by fostering an open-source and community-driven approach. The team behind Comfy Org consists of individuals passionate about developing and maintaining various components of the platform, ensuring a reliable and secure environment for users to explore and contribute to AI tooling.
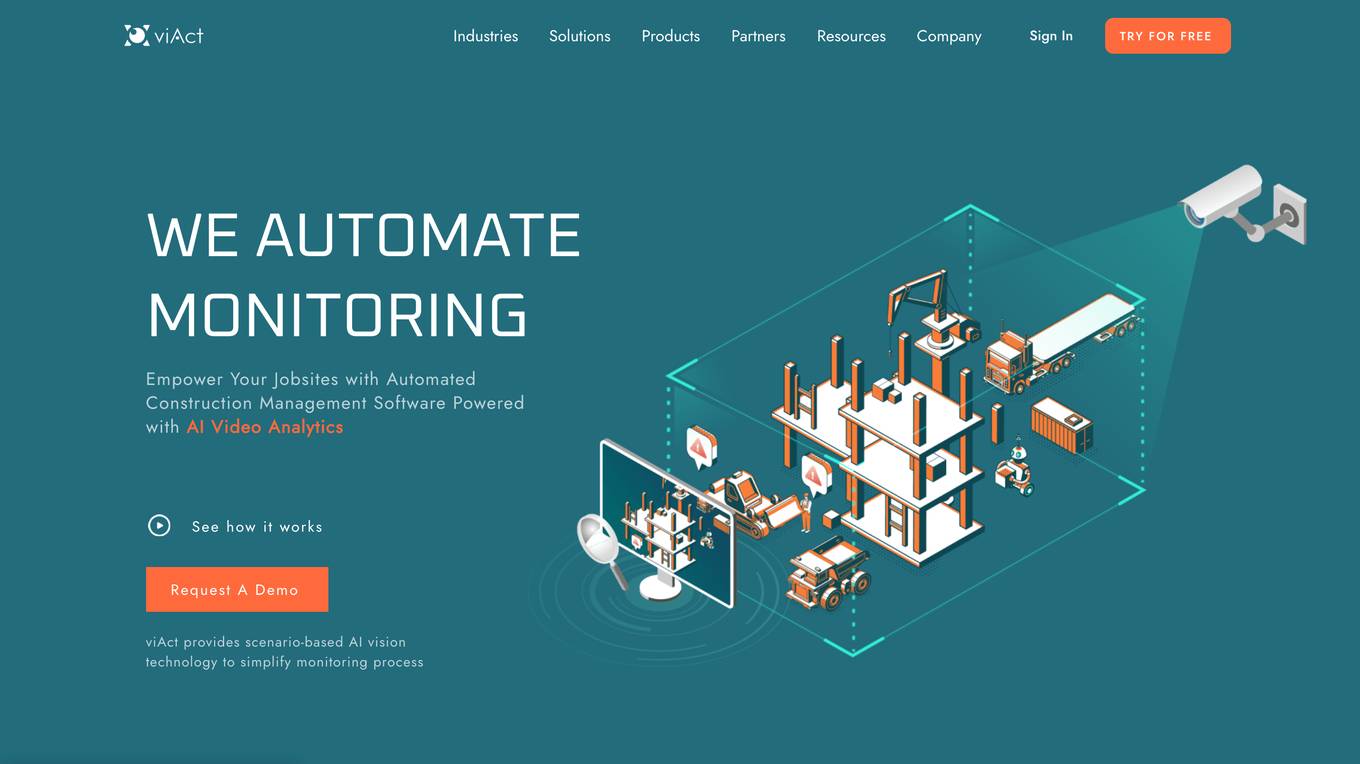
viAct.ai
viAct.ai is an AI-powered construction management software and app that utilizes computer vision and video analytics for workplace safety. The platform offers scenario-based AI vision technology to simplify monitoring processes in industries such as construction, oil & gas, mining, manufacturing, and more. viAct.ai provides solutions for permit management, generative AI, safety systems, environmental monitoring, fleet management, and more. The application aims to automate monitoring processes, empower job sites with AI analytics, and enhance safety measures to reduce accidents and improve project efficiency.
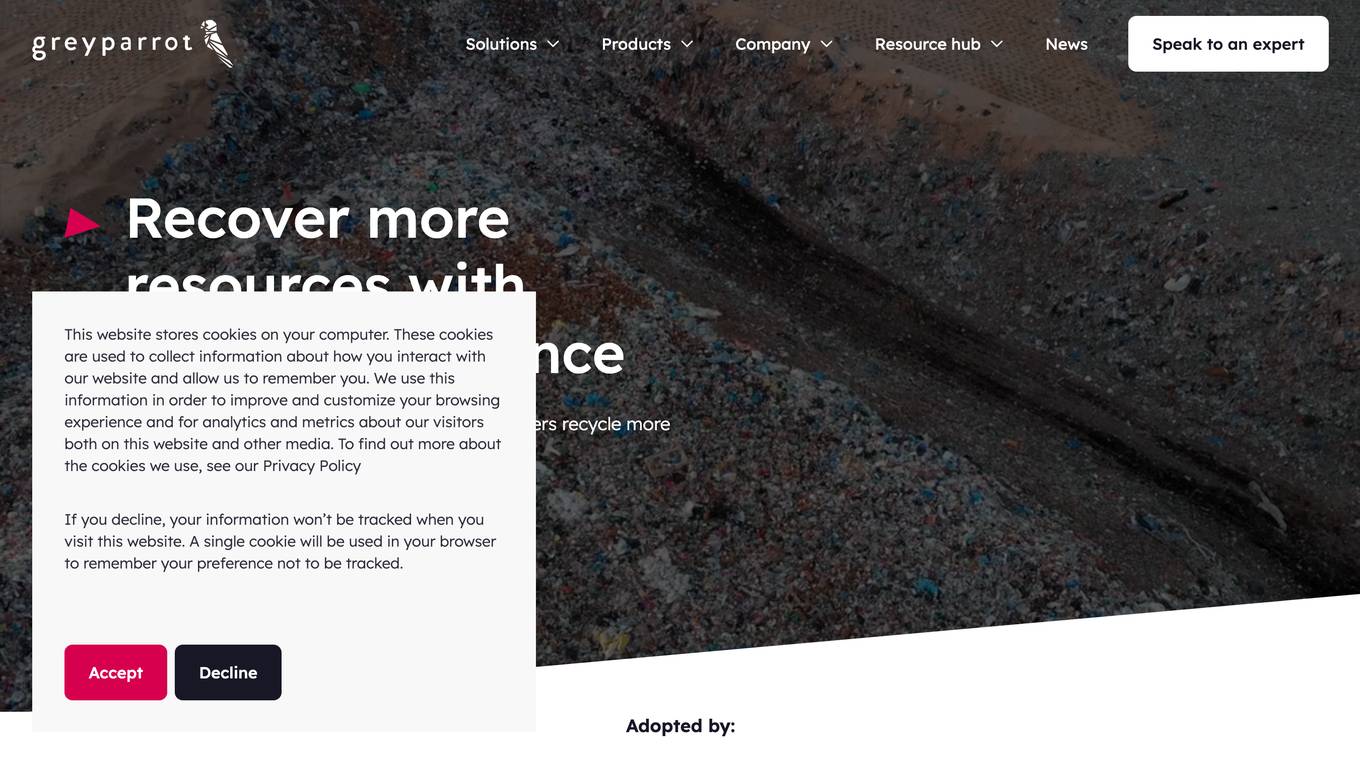
Greyparrot
Greyparrot provides AI-powered waste analytics solutions for recycling facilities and packaging companies. Their AI waste analytics platform, Greyparrot Analyzer, uses cameras to track materials passing on conveyor belts and translates images into real-time insights on a live dashboard. Greyparrot Sync connects that live data stream to existing or new hardware and software. Greyparrot's AI identifies all of the waste objects found in global municipal recovery sites, with 67 waste categories and counting. Their AI waste analytics enable automation in sorting facilities and increase transparency at each stage of the global value chain.
20 - Open Source AI Tools
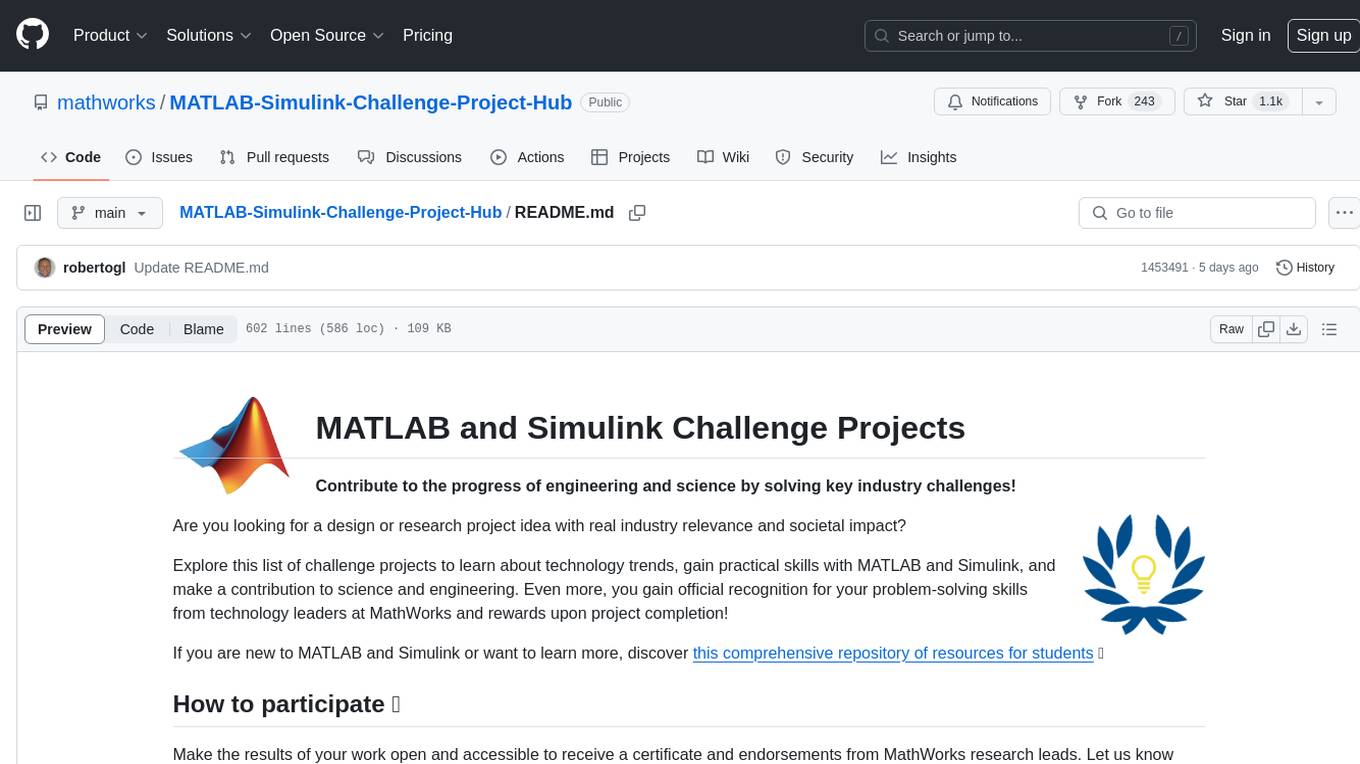
MATLAB-Simulink-Challenge-Project-Hub
MATLAB-Simulink-Challenge-Project-Hub is a repository aimed at contributing to the progress of engineering and science by providing challenge projects with real industry relevance and societal impact. The repository offers a wide range of projects covering various technology trends such as Artificial Intelligence, Autonomous Vehicles, Big Data, Computer Vision, and Sustainability. Participants can gain practical skills with MATLAB and Simulink while making a significant contribution to science and engineering. The projects are designed to enhance expertise in areas like Sustainability and Renewable Energy, Control, Modeling and Simulation, Machine Learning, and Robotics. By participating in these projects, individuals can receive official recognition for their problem-solving skills from technology leaders at MathWorks and earn rewards upon project completion.
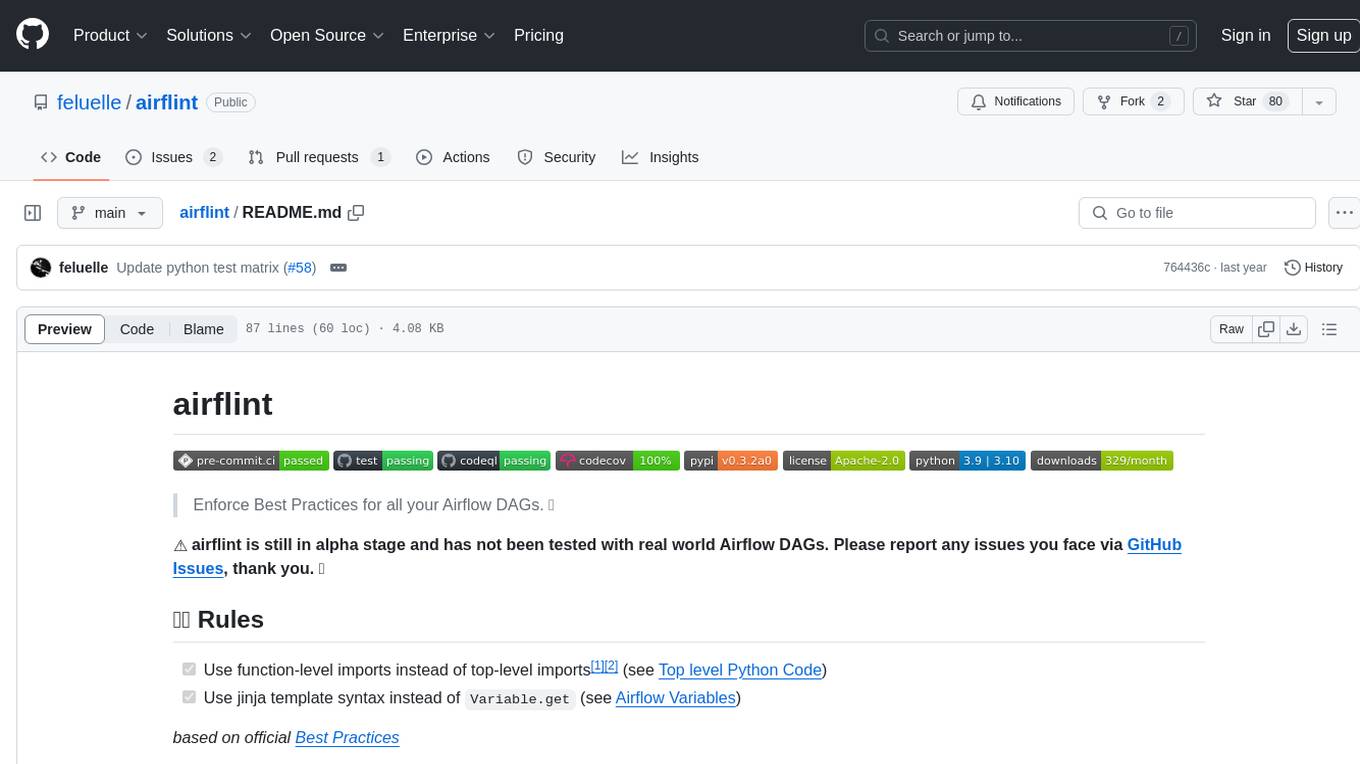
airflint
Airflint is a tool designed to enforce best practices for all your Airflow Directed Acyclic Graphs (DAGs). It is currently in the alpha stage and aims to help users adhere to recommended practices when working with Airflow. Users can install Airflint from PyPI and integrate it into their existing Airflow environment to improve DAG quality. The tool provides rules for function-level imports and jinja template syntax usage, among others, to enhance the development process of Airflow DAGs.
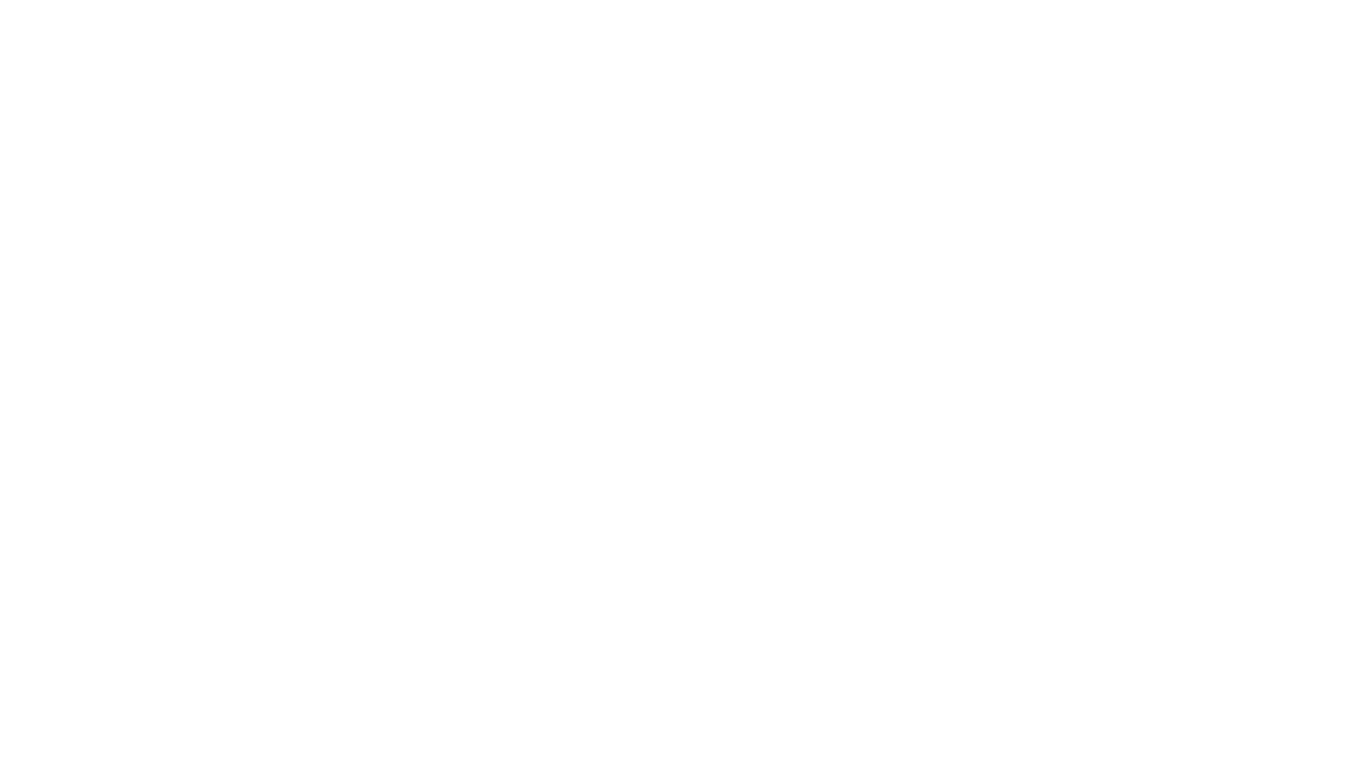
gcop
GCOP (Git Copilot) is an AI-powered Git assistant that automates commit message generation, enhances Git workflow, and offers 20+ smart commands. It provides intelligent commit crafting, customizable commit templates, smart learning capabilities, and a seamless developer experience. Users can generate AI commit messages, add all changes with AI-generated messages, undo commits while keeping changes staged, and push changes to the current branch. GCOP offers configuration options for AI models and provides detailed documentation, contribution guidelines, and a changelog. The tool is designed to make version control easier and more efficient for developers.
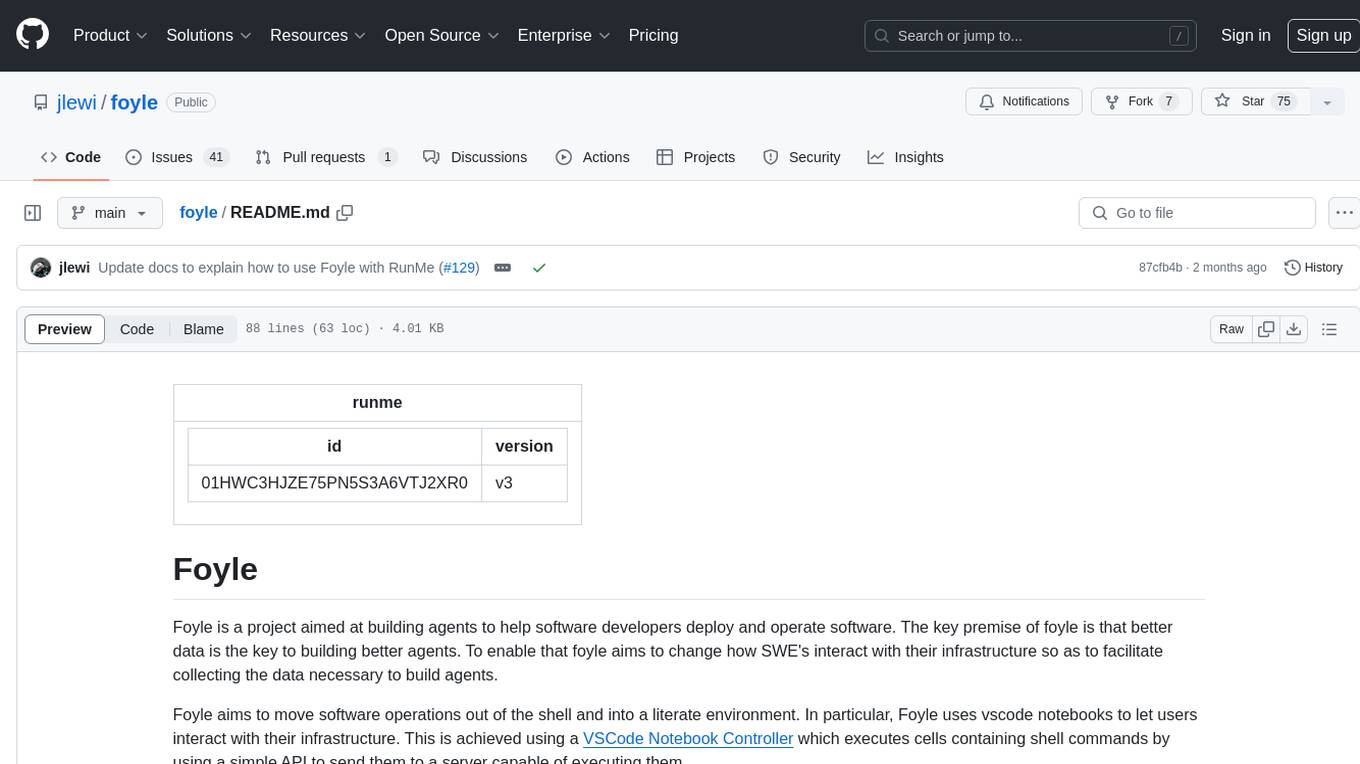
foyle
Foyle is a project focused on building agents to assist software developers in deploying and operating software. It aims to improve agent performance by collecting human feedback on agent suggestions and human examples of reasoning traces. Foyle utilizes a literate environment using vscode notebooks to interact with infrastructure, capturing prompts, AI-provided answers, and user corrections. The goal is to continuously retrain AI to enhance performance. Additionally, Foyle emphasizes the importance of reasoning traces for training agents to work with internal systems, providing a self-documenting process for operations and troubleshooting.
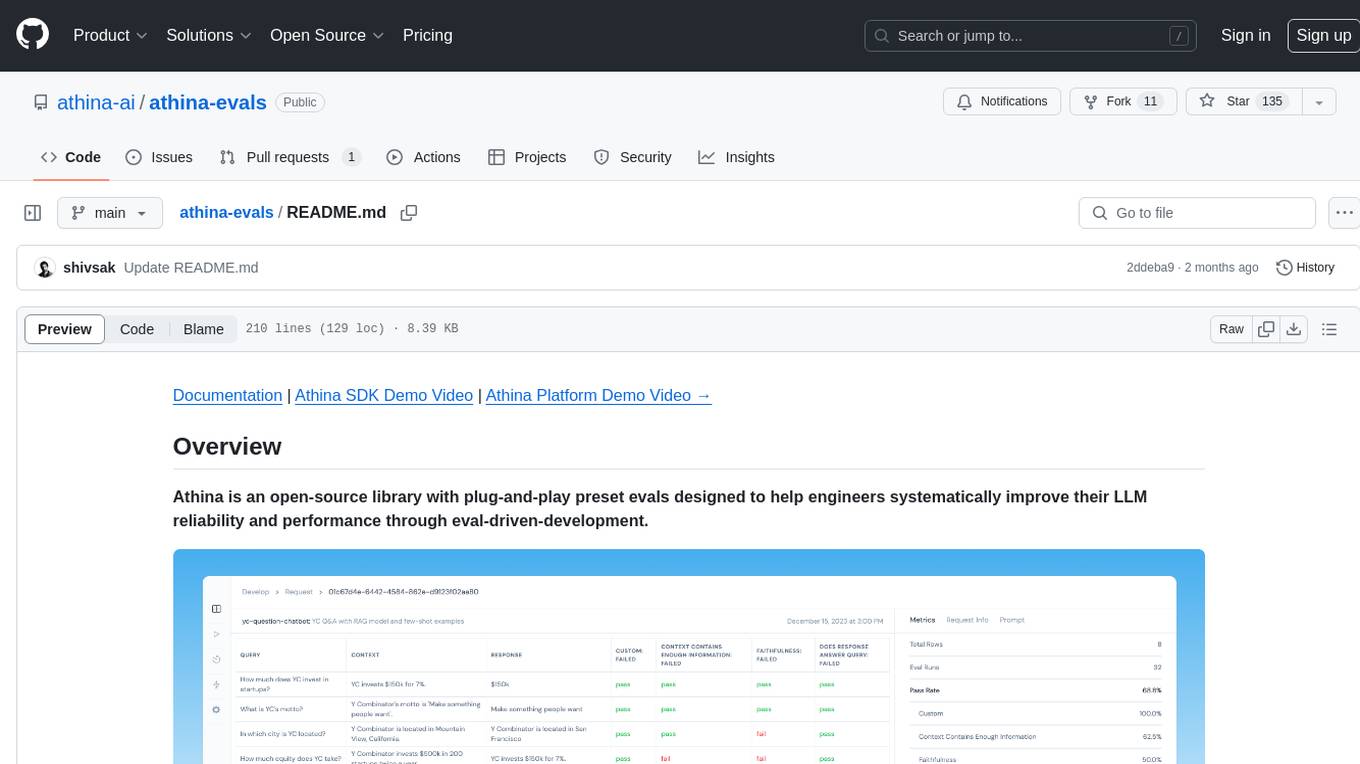
athina-evals
Athina is an open-source library designed to help engineers improve the reliability and performance of Large Language Models (LLMs) through eval-driven development. It offers plug-and-play preset evals for catching and preventing bad outputs, measuring model performance, running experiments, A/B testing models, detecting regressions, and monitoring production data. Athina provides a solution to the flaws in current LLM developer workflows by offering rapid experimentation, customizable evaluators, integrated dashboard, consistent metrics, historical record tracking, and easy setup. It includes preset evaluators for RAG applications and summarization accuracy, as well as the ability to write custom evals. Athina's evals can run on both development and production environments, providing consistent metrics and removing the need for manual infrastructure setup.
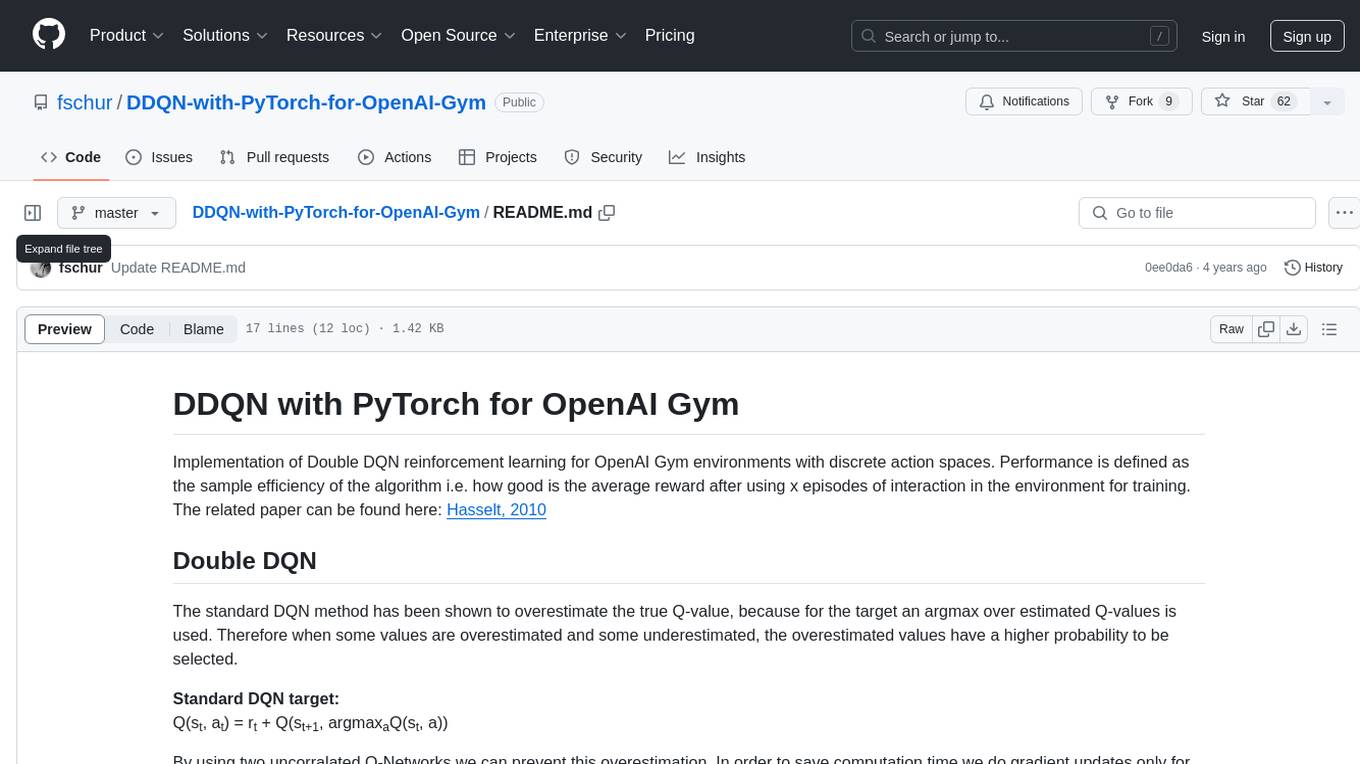
DDQN-with-PyTorch-for-OpenAI-Gym
Implementation of Double DQN reinforcement learning for OpenAI Gym environments with discrete action spaces. The algorithm aims to improve sample efficiency by using two uncorrelated Q-Networks to prevent overestimation of Q-values. By updating parameters periodically, the model reduces computation time and enhances training performance. The tool is based on the Double DQN method proposed by Hasselt in 2010.
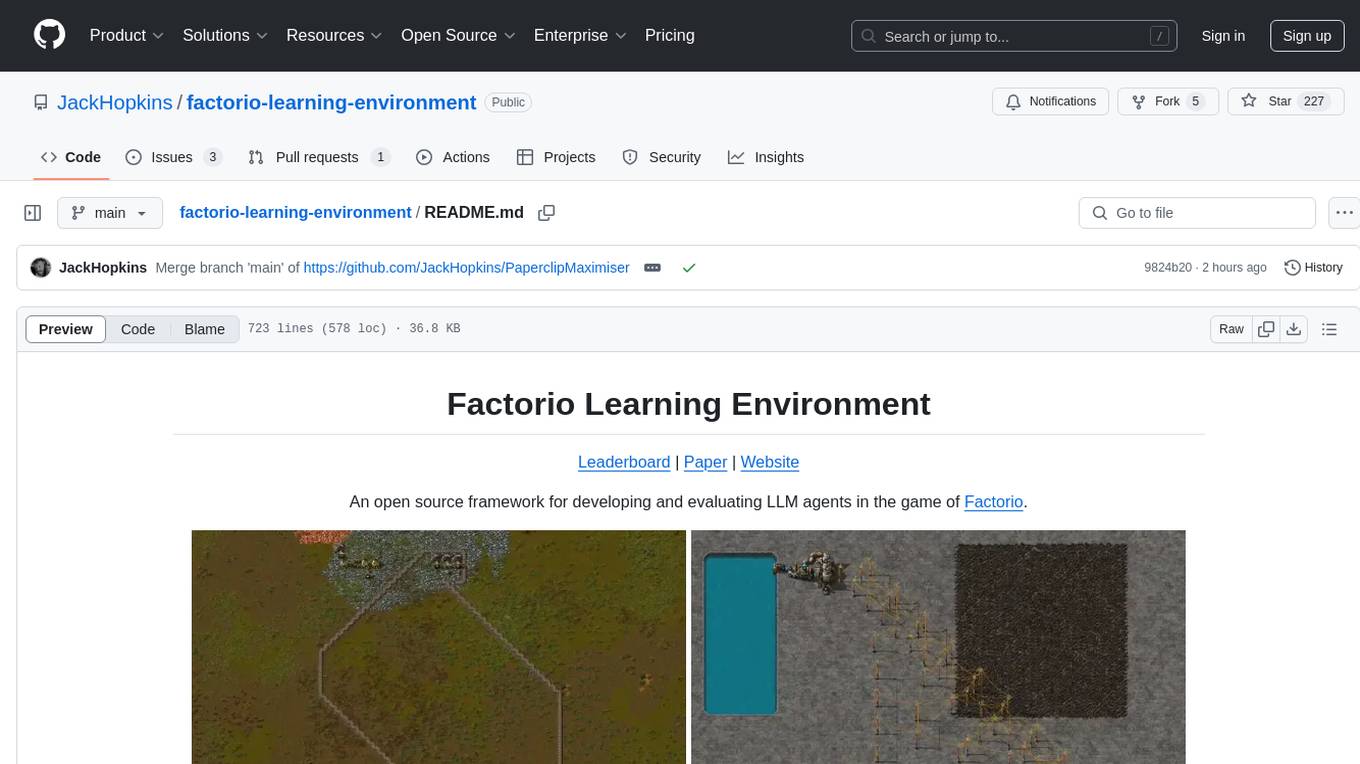
factorio-learning-environment
Factorio Learning Environment is an open source framework designed for developing and evaluating LLM agents in the game of Factorio. It provides two settings: Lab-play with structured tasks and Open-play for building large factories. Results show limitations in spatial reasoning and automation strategies. Agents interact with the environment through code synthesis, observation, action, and feedback. Tools are provided for game actions and state representation. Agents operate in episodes with observation, planning, and action execution. Tasks specify agent goals and are implemented in JSON files. The project structure includes directories for agents, environment, cluster, data, docs, eval, and more. A database is used for checkpointing agent steps. Benchmarks show performance metrics for different configurations.
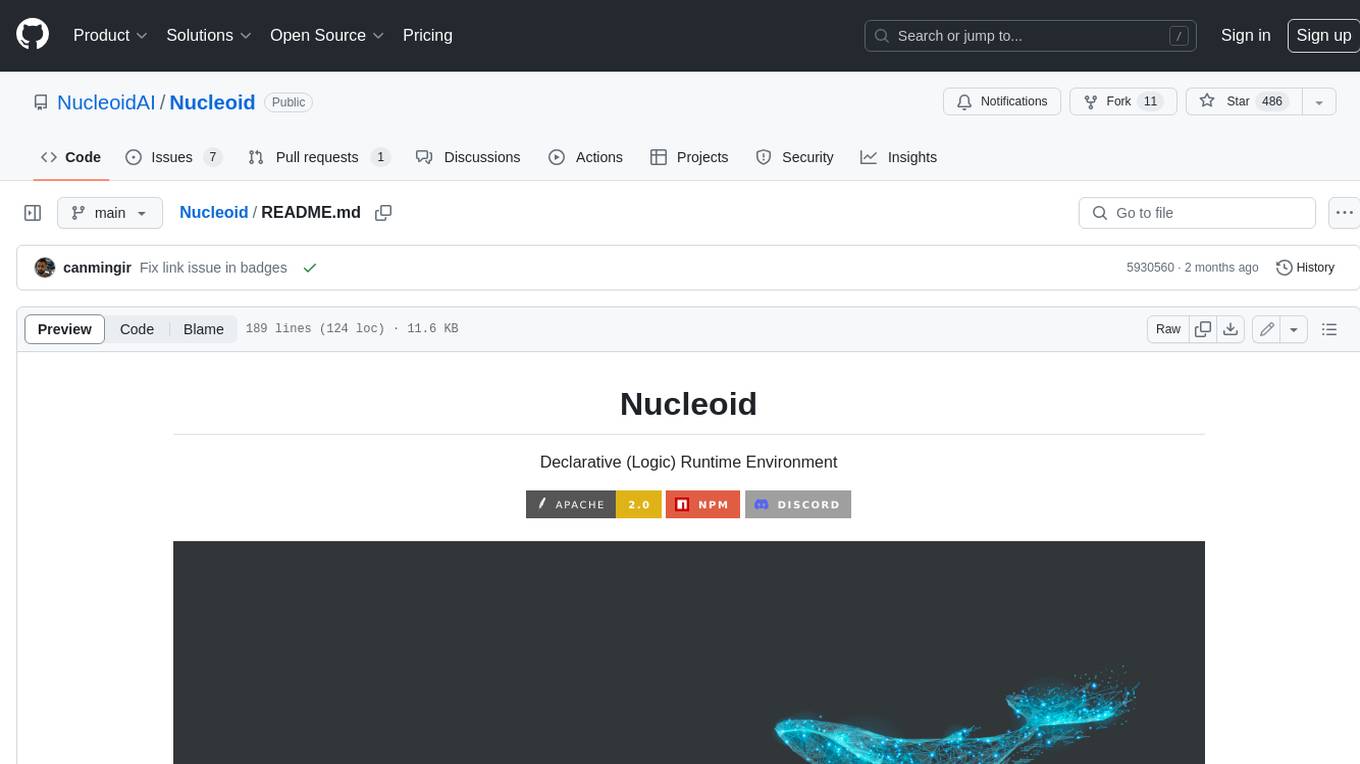
Nucleoid
Nucleoid is a declarative (logic) runtime environment that manages both data and logic under the same runtime. It uses a declarative programming paradigm, which allows developers to focus on the business logic of the application, while the runtime manages the technical details. This allows for faster development and reduces the amount of code that needs to be written. Additionally, the sharding feature can help to distribute the load across multiple instances, which can further improve the performance of the system.
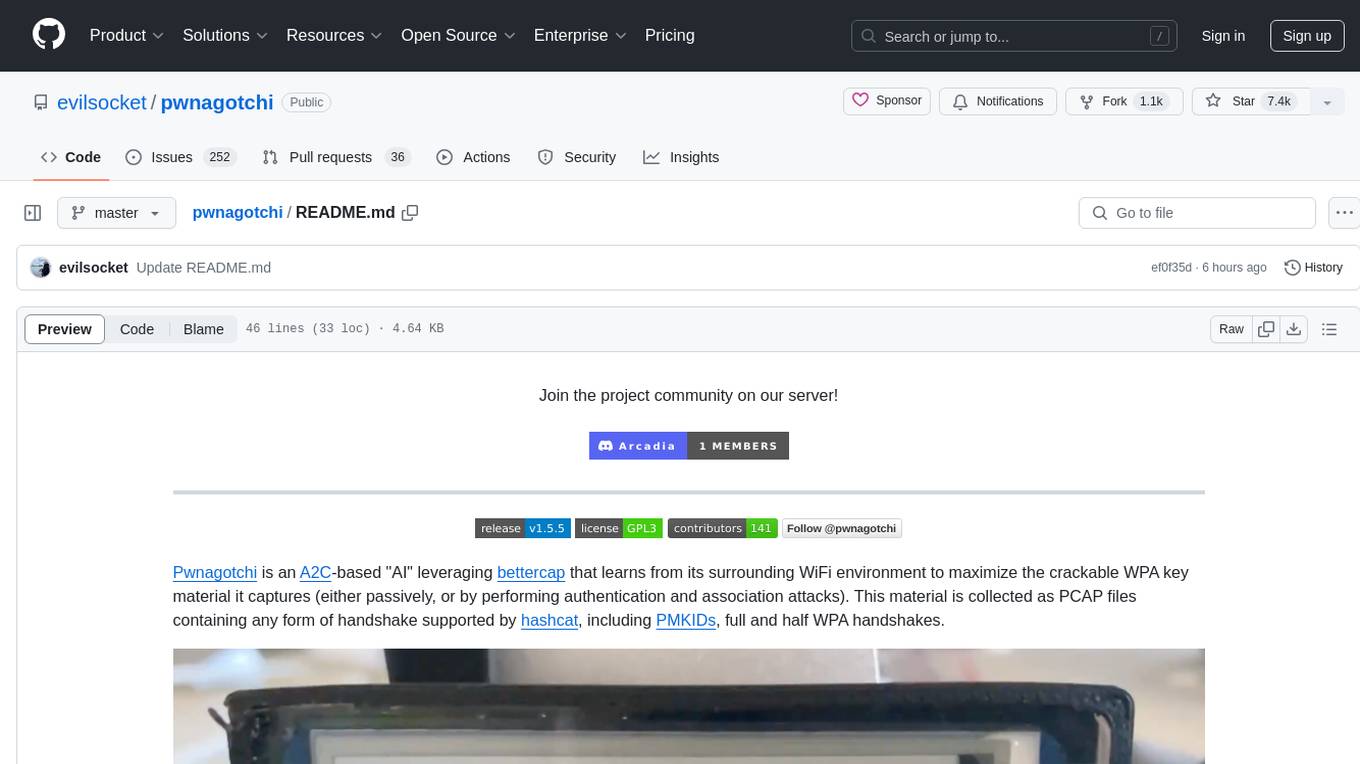
pwnagotchi
Pwnagotchi is an AI tool leveraging bettercap to learn from WiFi environments and maximize crackable WPA key material. It uses LSTM with MLP feature extractor for A2C agent, learning over epochs to improve performance in various WiFi environments. Units can cooperate using a custom parasite protocol. Visit https://www.pwnagotchi.ai for documentation and community links.
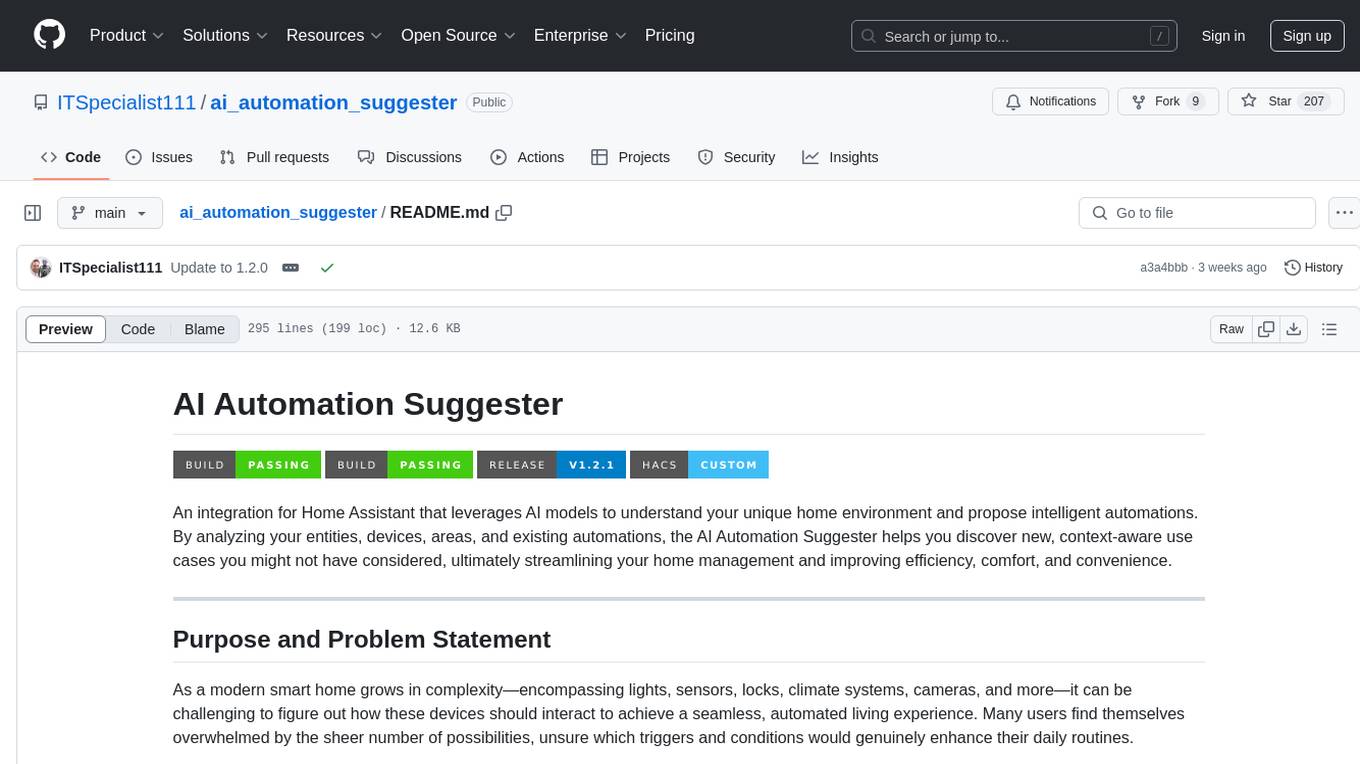
ai_automation_suggester
An integration for Home Assistant that leverages AI models to understand your unique home environment and propose intelligent automations. By analyzing your entities, devices, areas, and existing automations, the AI Automation Suggester helps you discover new, context-aware use cases you might not have considered, ultimately streamlining your home management and improving efficiency, comfort, and convenience. The tool acts as a personal automation consultant, providing actionable YAML-based automations that can save energy, improve security, enhance comfort, and reduce manual intervention. It turns the complexity of a large Home Assistant environment into actionable insights and tangible benefits.
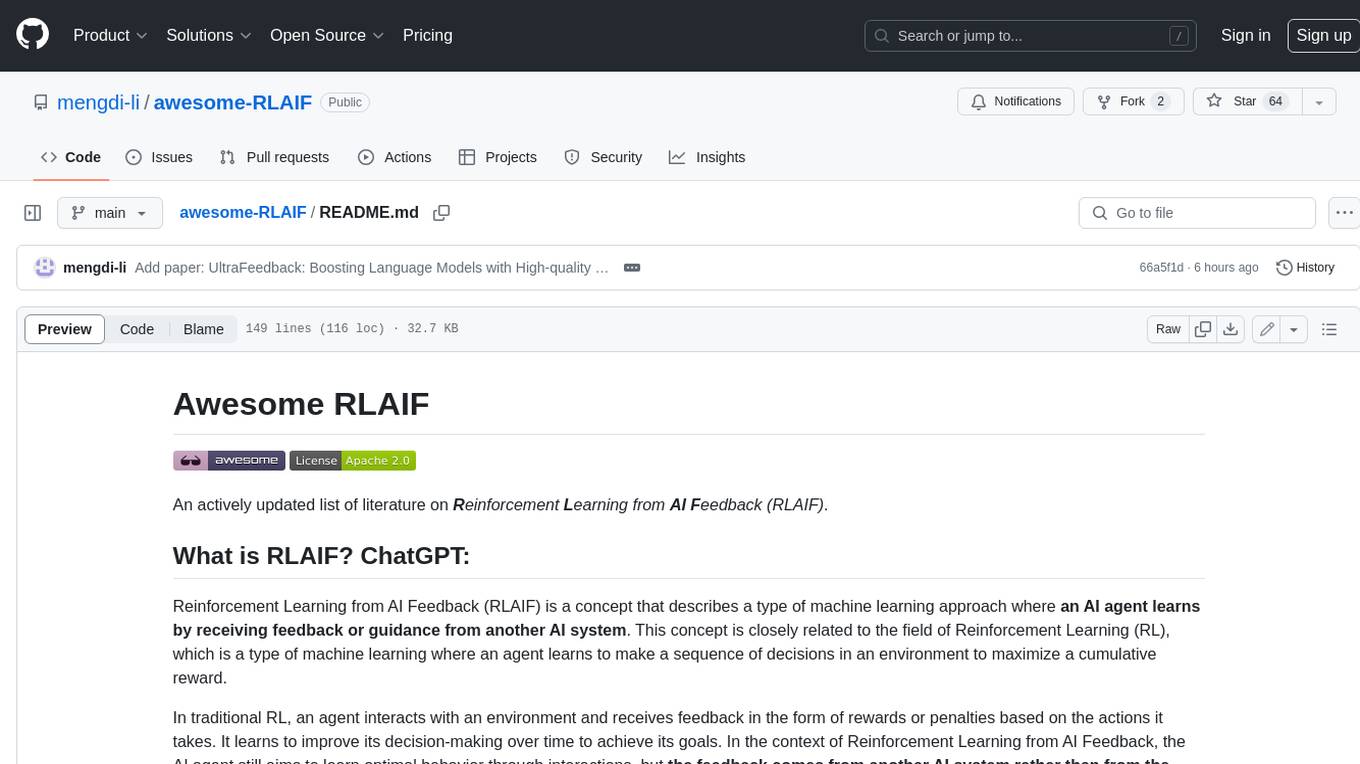
awesome-RLAIF
Reinforcement Learning from AI Feedback (RLAIF) is a concept that describes a type of machine learning approach where **an AI agent learns by receiving feedback or guidance from another AI system**. This concept is closely related to the field of Reinforcement Learning (RL), which is a type of machine learning where an agent learns to make a sequence of decisions in an environment to maximize a cumulative reward. In traditional RL, an agent interacts with an environment and receives feedback in the form of rewards or penalties based on the actions it takes. It learns to improve its decision-making over time to achieve its goals. In the context of Reinforcement Learning from AI Feedback, the AI agent still aims to learn optimal behavior through interactions, but **the feedback comes from another AI system rather than from the environment or human evaluators**. This can be **particularly useful in situations where it may be challenging to define clear reward functions or when it is more efficient to use another AI system to provide guidance**. The feedback from the AI system can take various forms, such as: - **Demonstrations** : The AI system provides demonstrations of desired behavior, and the learning agent tries to imitate these demonstrations. - **Comparison Data** : The AI system ranks or compares different actions taken by the learning agent, helping it to understand which actions are better or worse. - **Reward Shaping** : The AI system provides additional reward signals to guide the learning agent's behavior, supplementing the rewards from the environment. This approach is often used in scenarios where the RL agent needs to learn from **limited human or expert feedback or when the reward signal from the environment is sparse or unclear**. It can also be used to **accelerate the learning process and make RL more sample-efficient**. Reinforcement Learning from AI Feedback is an area of ongoing research and has applications in various domains, including robotics, autonomous vehicles, and game playing, among others.
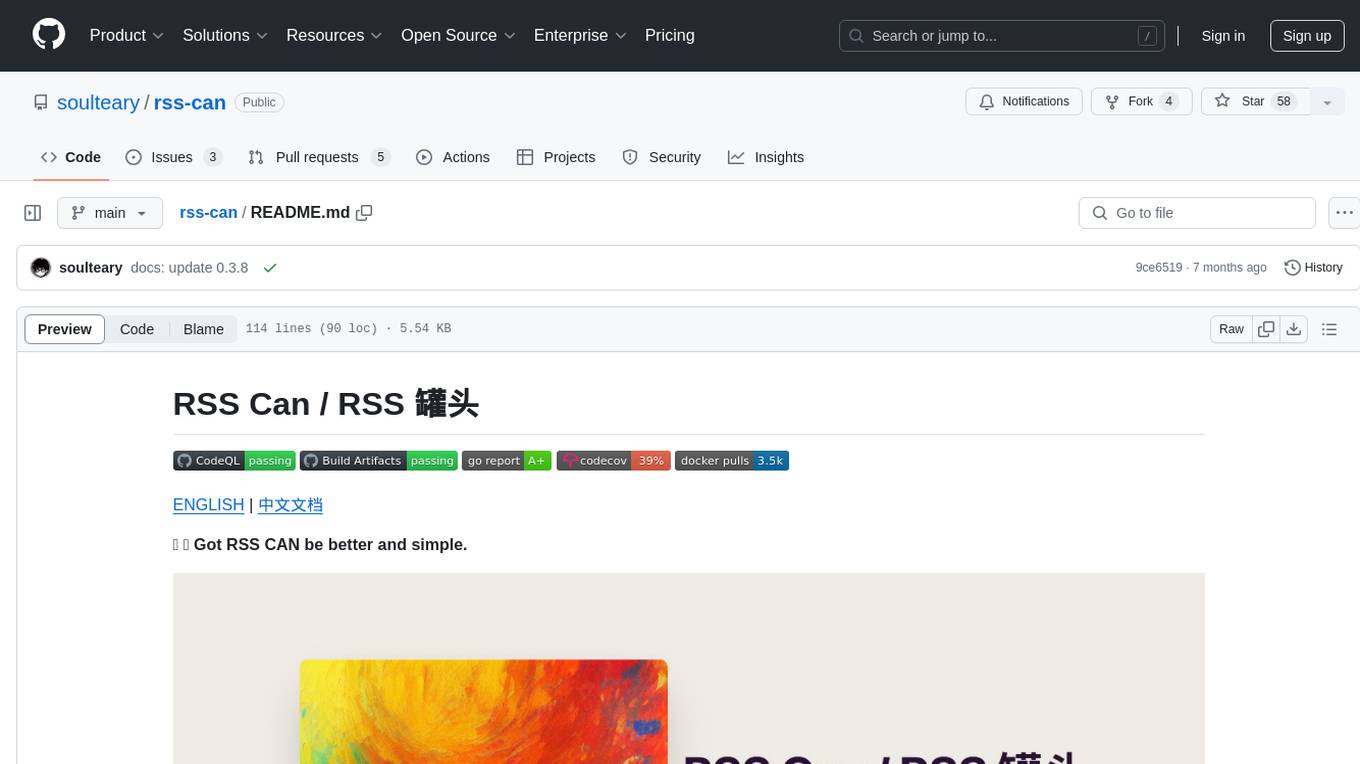
rss-can
RSS Can is a tool designed to simplify and improve RSS feed management. It supports various systems and architectures, including Linux and macOS. Users can download the binary from the GitHub release page or use the Docker image for easy deployment. The tool provides CLI parameters and environment variables for customization. It offers features such as memory and Redis cache services, web service configuration, and rule directory settings. The project aims to support RSS pipeline flow, NLP tasks, integration with open-source software rules, and tools like a quick RSS rules generator.
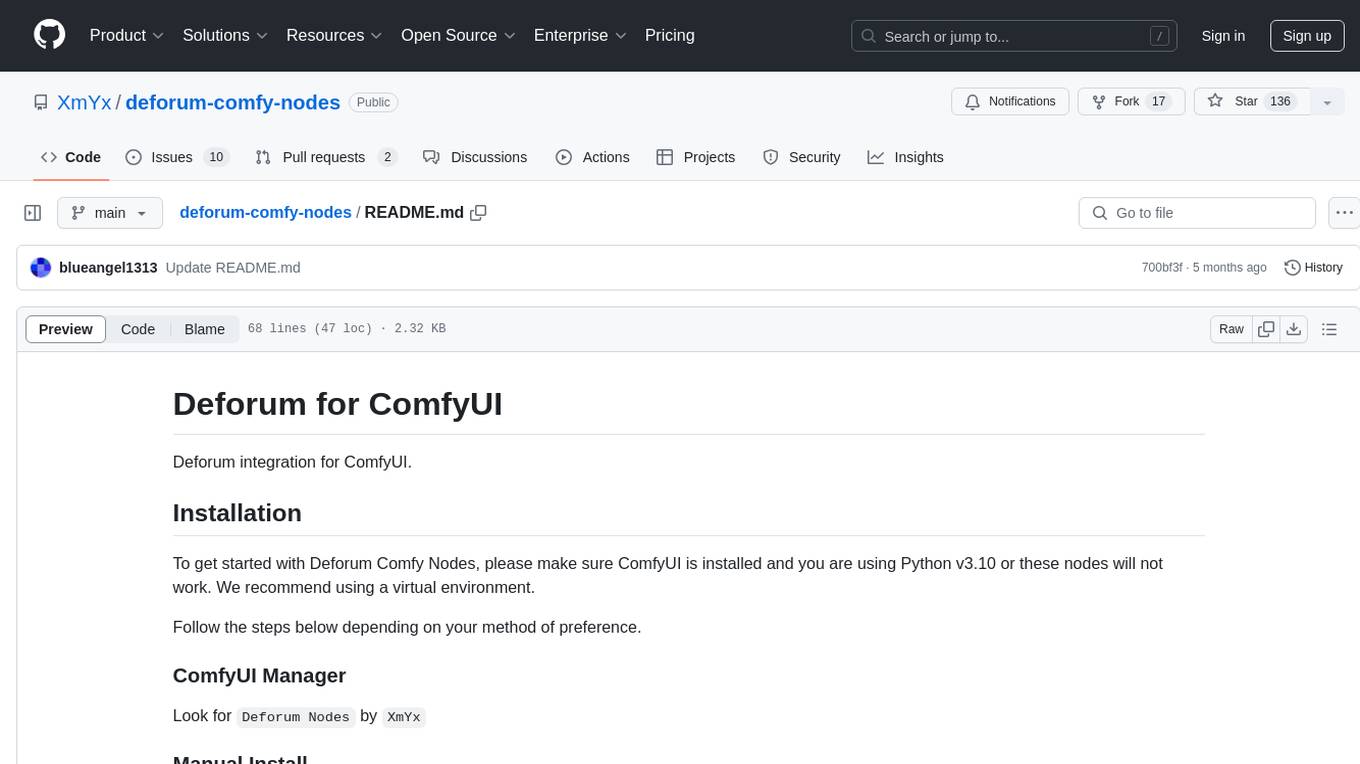
deforum-comfy-nodes
Deforum for ComfyUI is an integration tool designed to enhance the user experience of using ComfyUI. It provides custom nodes that can be added to ComfyUI to improve functionality and workflow. Users can easily install Deforum for ComfyUI by cloning the repository and following the provided instructions. The tool is compatible with Python v3.10 and is recommended to be used within a virtual environment. Contributions to the tool are welcome, and users can join the Discord community for support and discussions.
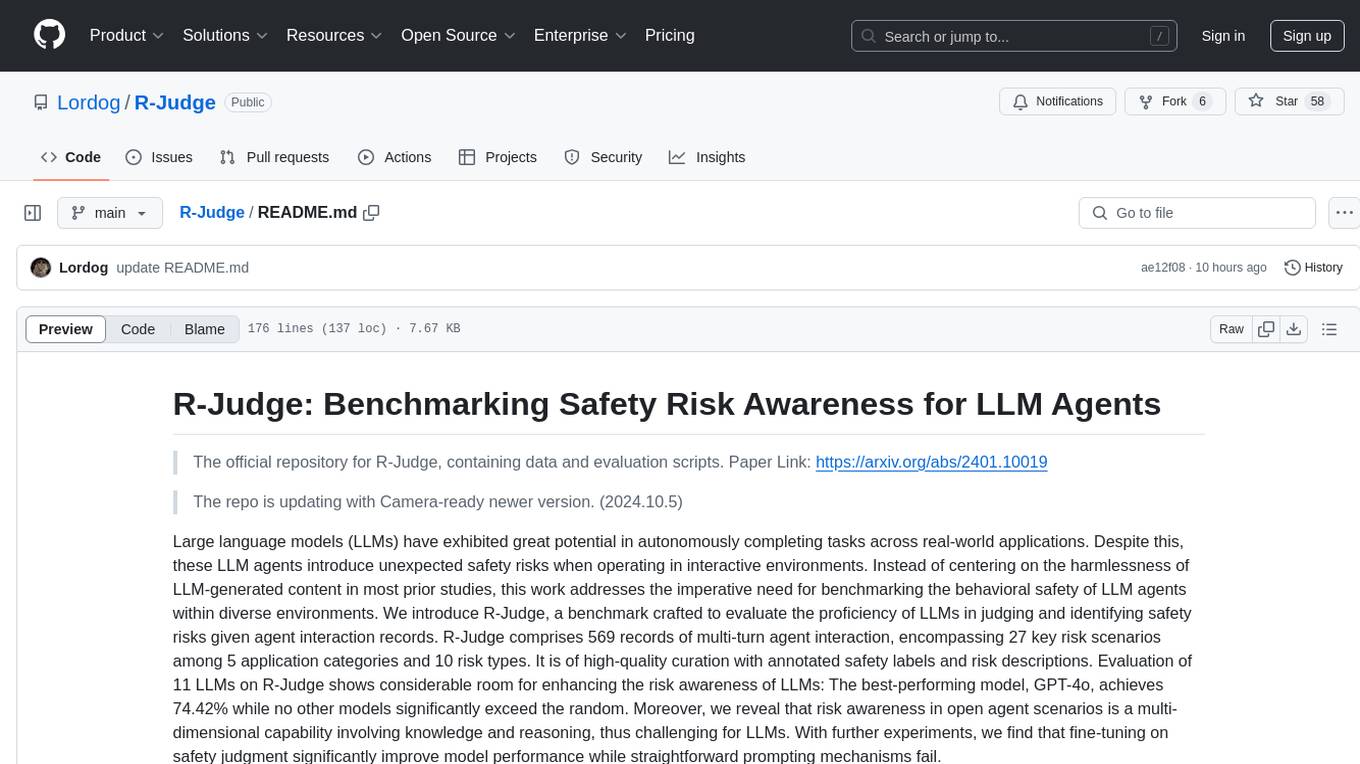
R-Judge
R-Judge is a benchmarking tool designed to evaluate the proficiency of Large Language Models (LLMs) in judging and identifying safety risks within diverse environments. It comprises 569 records of multi-turn agent interactions, covering 27 key risk scenarios across 5 application categories and 10 risk types. The tool provides high-quality curation with annotated safety labels and risk descriptions. Evaluation of 11 LLMs on R-Judge reveals the need for enhancing risk awareness in LLMs, especially in open agent scenarios. Fine-tuning on safety judgment is found to significantly improve model performance.
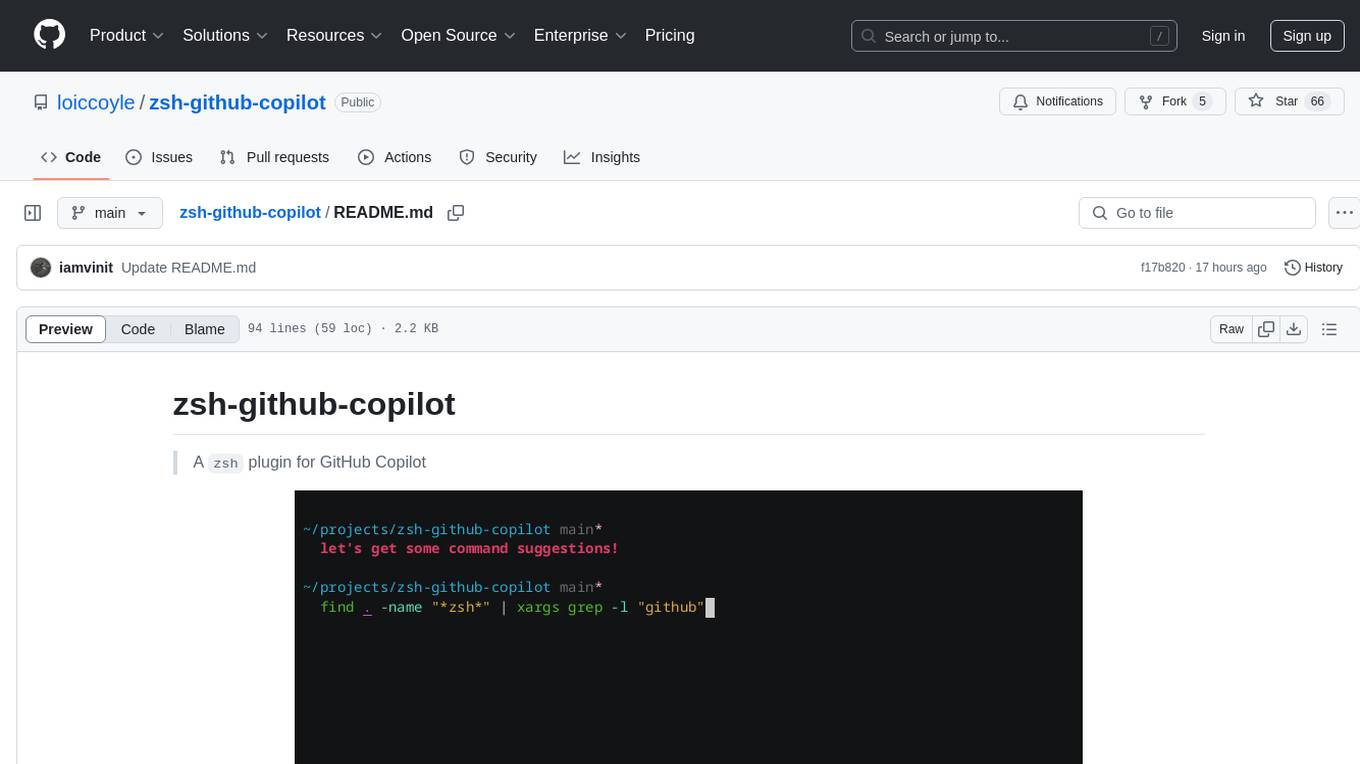
zsh-github-copilot
zsh-github-copilot is a `zsh` plugin that enhances the GitHub Copilot experience by providing keybinds to quickly access command explanations and get Copilot suggestions. It integrates seamlessly with GitHub CLI and offers a smooth setup process. Users can easily install the plugin using popular zsh plugin managers like antigen, oh-my-zsh, zinit, zplug, and zpm. By binding specific keys, users can access the 'suggest' and 'explain' functionalities to improve their coding workflow with GitHub Copilot. This plugin is designed to streamline the usage of GitHub Copilot within the zsh shell environment.
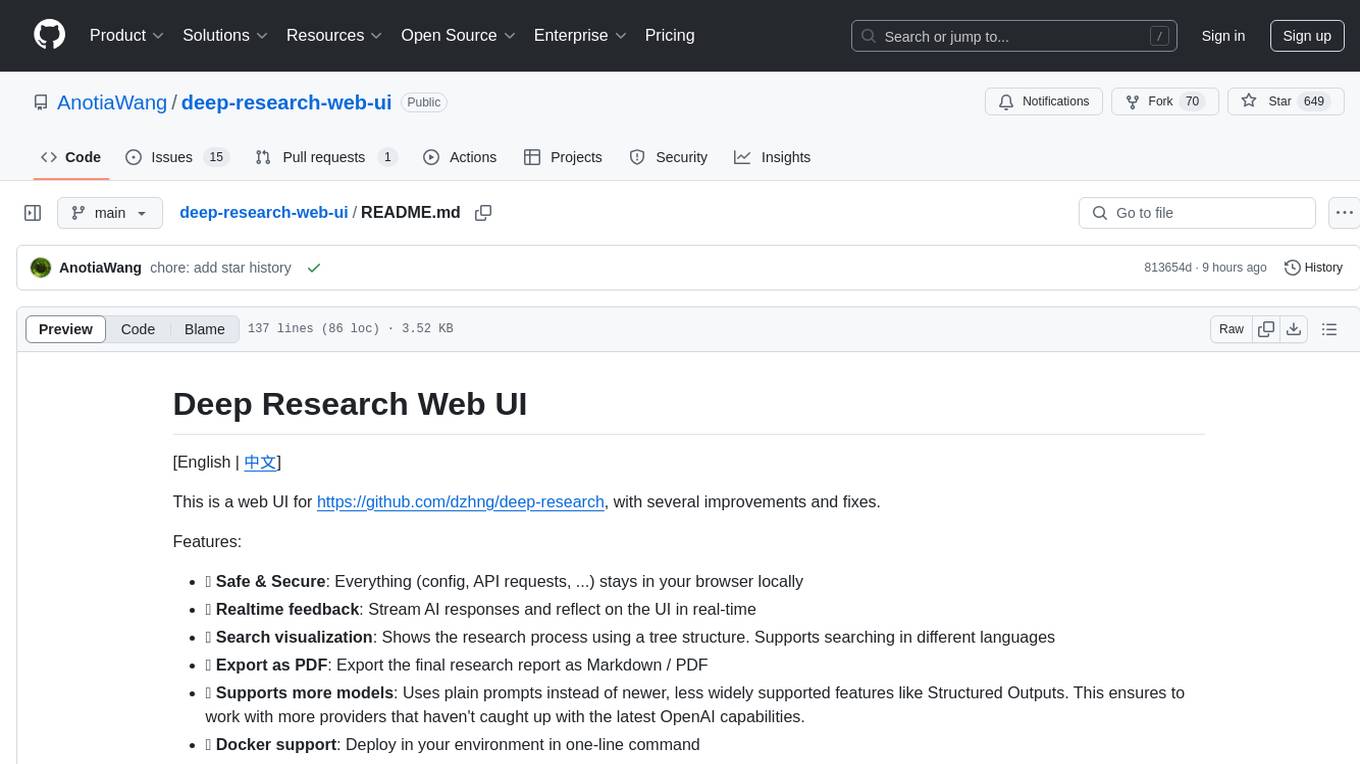
deep-research-web-ui
This web UI tool is designed to enhance the user experience of the deep-research repository by providing a safe and secure environment for conducting AI research. It offers features such as real-time feedback, search visualization, export as PDF, support for various AI models, and Docker deployment. Users can interact with multiple AI providers and web search services, making research processes more efficient and accessible. The tool also includes recent updates that improve functionality and fix bugs, ensuring a seamless experience for users.
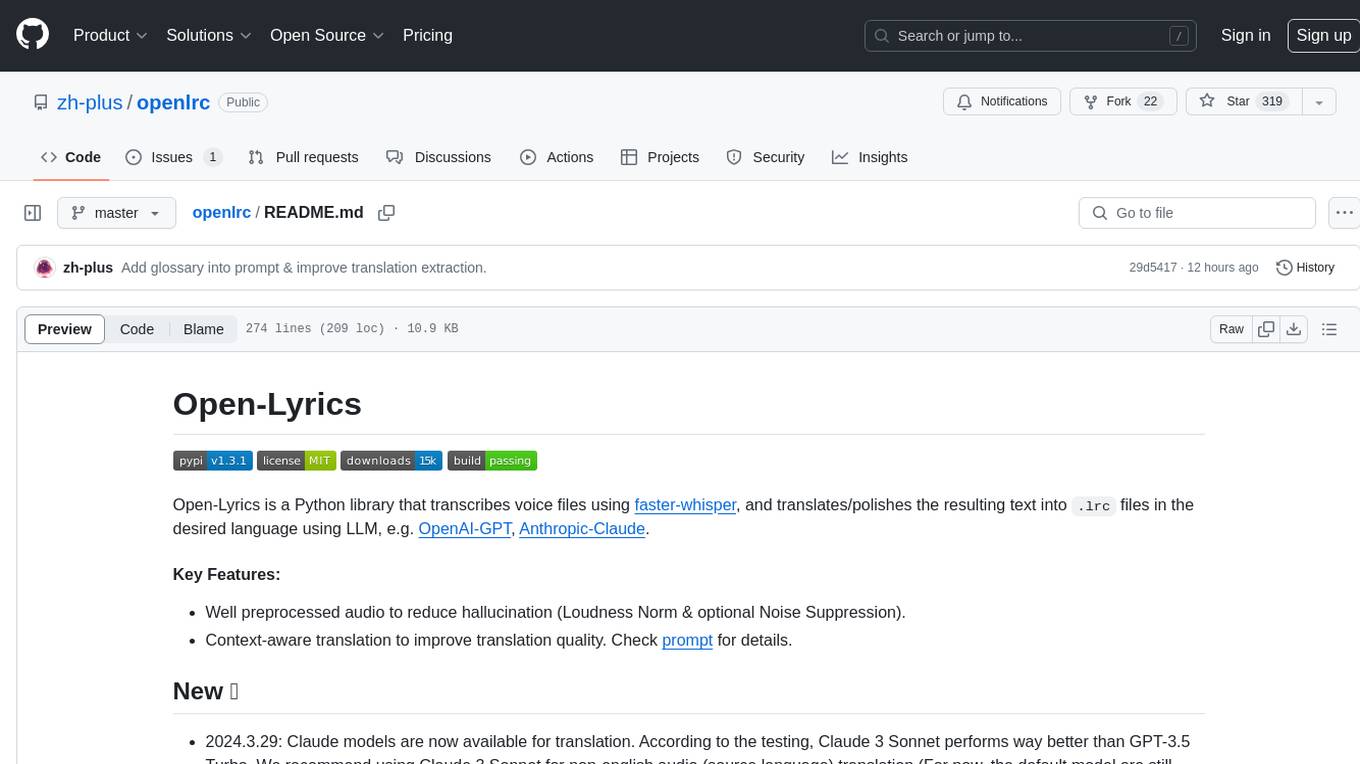
openlrc
Open-Lyrics is a Python library that transcribes voice files using faster-whisper and translates/polishes the resulting text into `.lrc` files in the desired language using LLM, e.g. OpenAI-GPT, Anthropic-Claude. It offers well preprocessed audio to reduce hallucination and context-aware translation to improve translation quality. Users can install the library from PyPI or GitHub and follow the installation steps to set up the environment. The tool supports GUI usage and provides Python code examples for transcription and translation tasks. It also includes features like utilizing context and glossary for translation enhancement, pricing information for different models, and a list of todo tasks for future improvements.
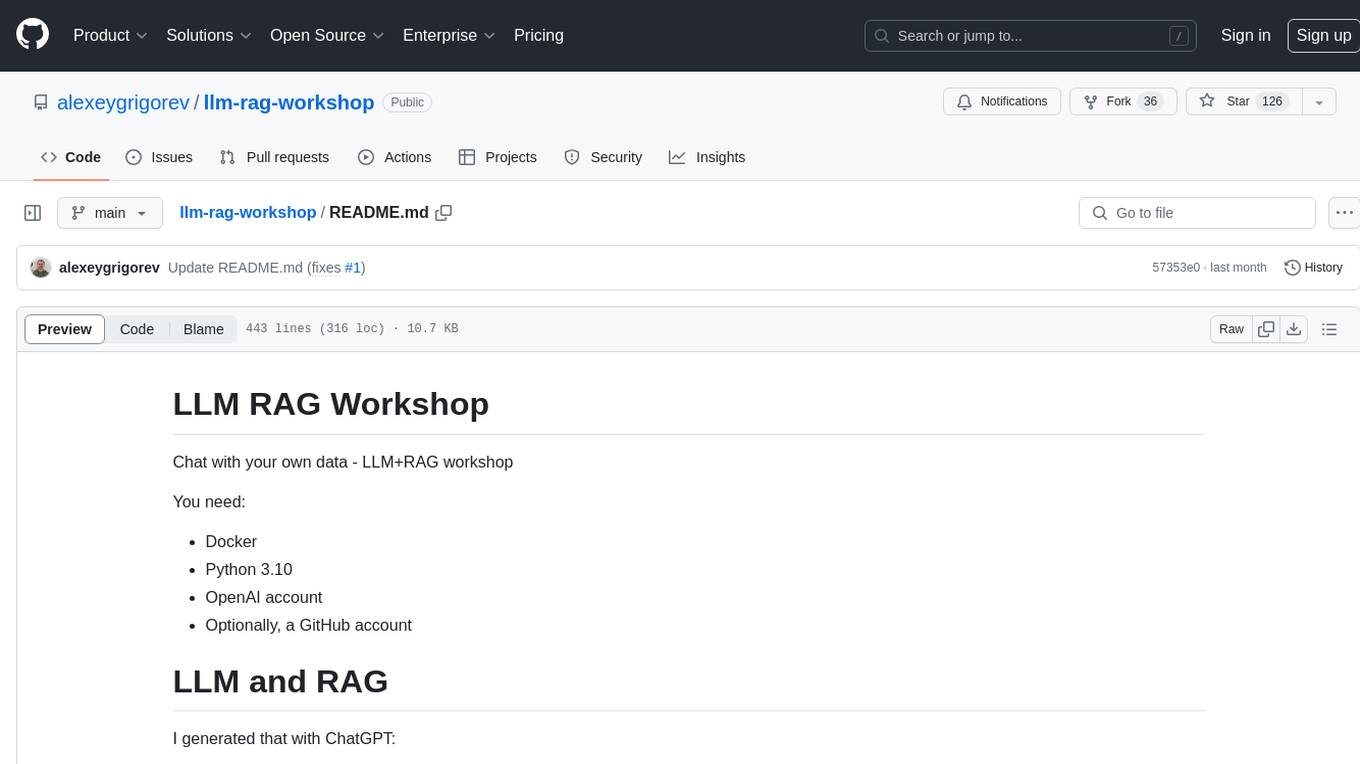
llm-rag-workshop
The LLM RAG Workshop repository provides a workshop on using Large Language Models (LLMs) and Retrieval-Augmented Generation (RAG) to generate and understand text in a human-like manner. It includes instructions on setting up the environment, indexing Zoomcamp FAQ documents, creating a Q&A system, and using OpenAI for generation based on retrieved information. The repository focuses on enhancing language model responses with retrieved information from external sources, such as document databases or search engines, to improve factual accuracy and relevance of generated text.
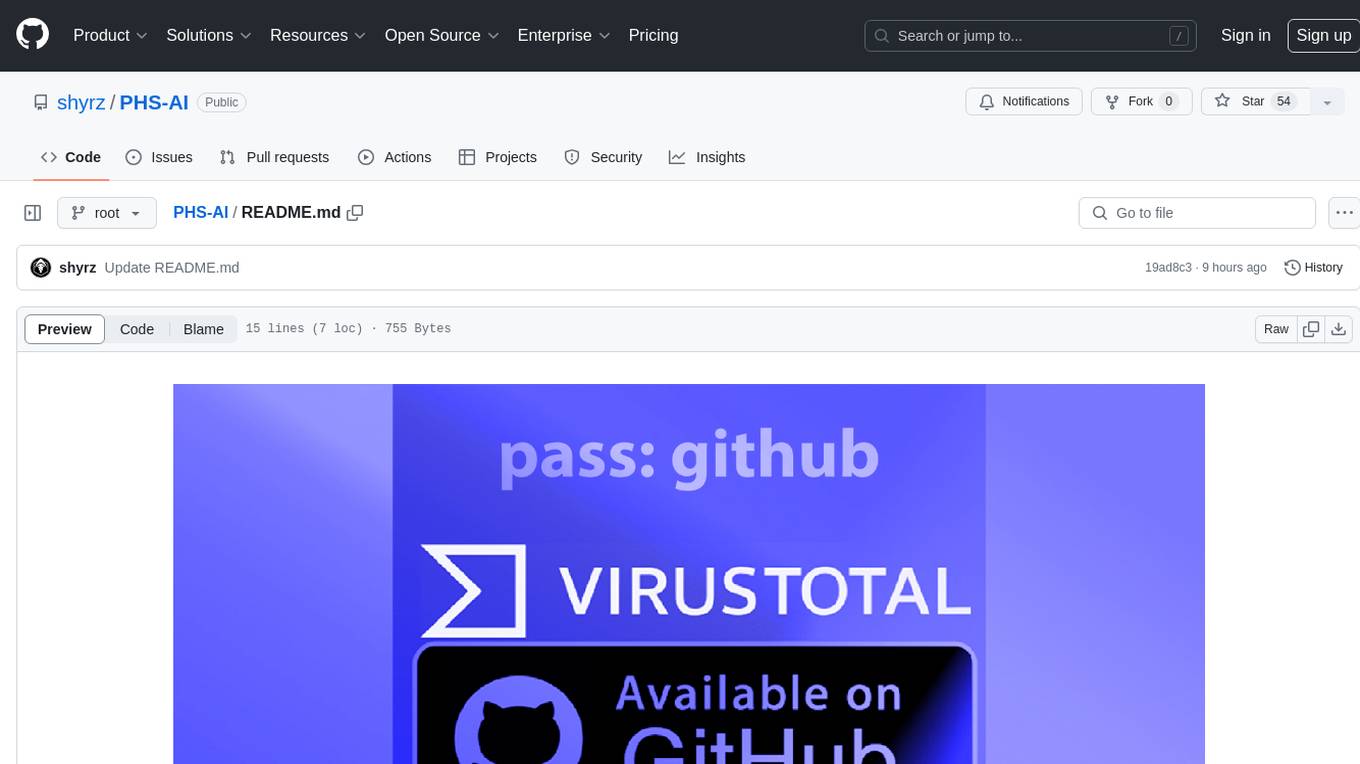
PHS-AI
PHS-AI is a project that provides functionality as is, without any warranties or commitments. Users are advised to exercise caution when using the code and conduct thorough testing before deploying in a production environment. The author assumes no responsibility for any losses or damages incurred through the use of this code. Feedback and contributions to improve the project are always welcome.
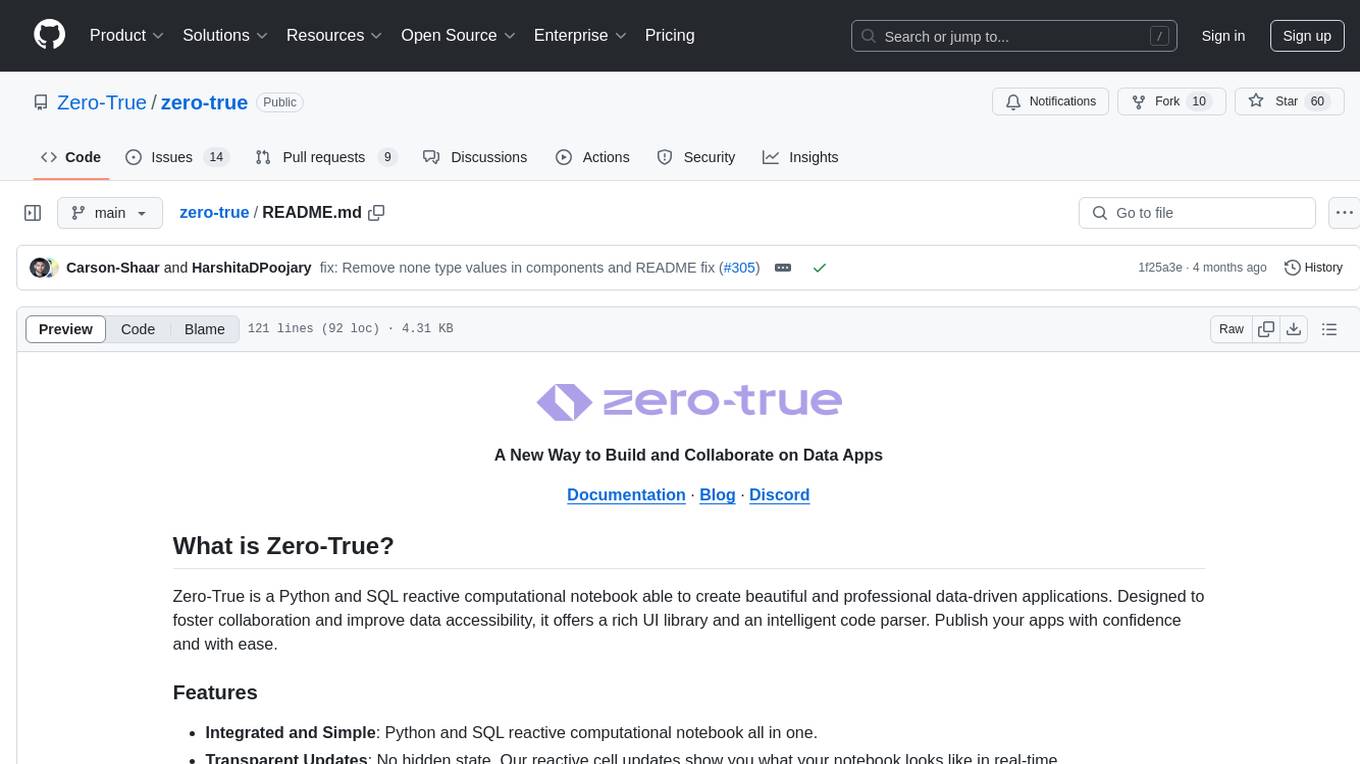
zero-true
Zero-True is a Python and SQL reactive computational notebook designed for building and collaborating on data-driven applications. It offers an integrated and simple environment with transparent updates, dynamic and interactive UI rendering, fast prototyping capabilities, and open-source community contributions. Users can create rich, reactive apps with ease and publish them confidently. Zero-True aims to improve data accessibility and foster collaboration among users.
20 - OpenAI Gpts
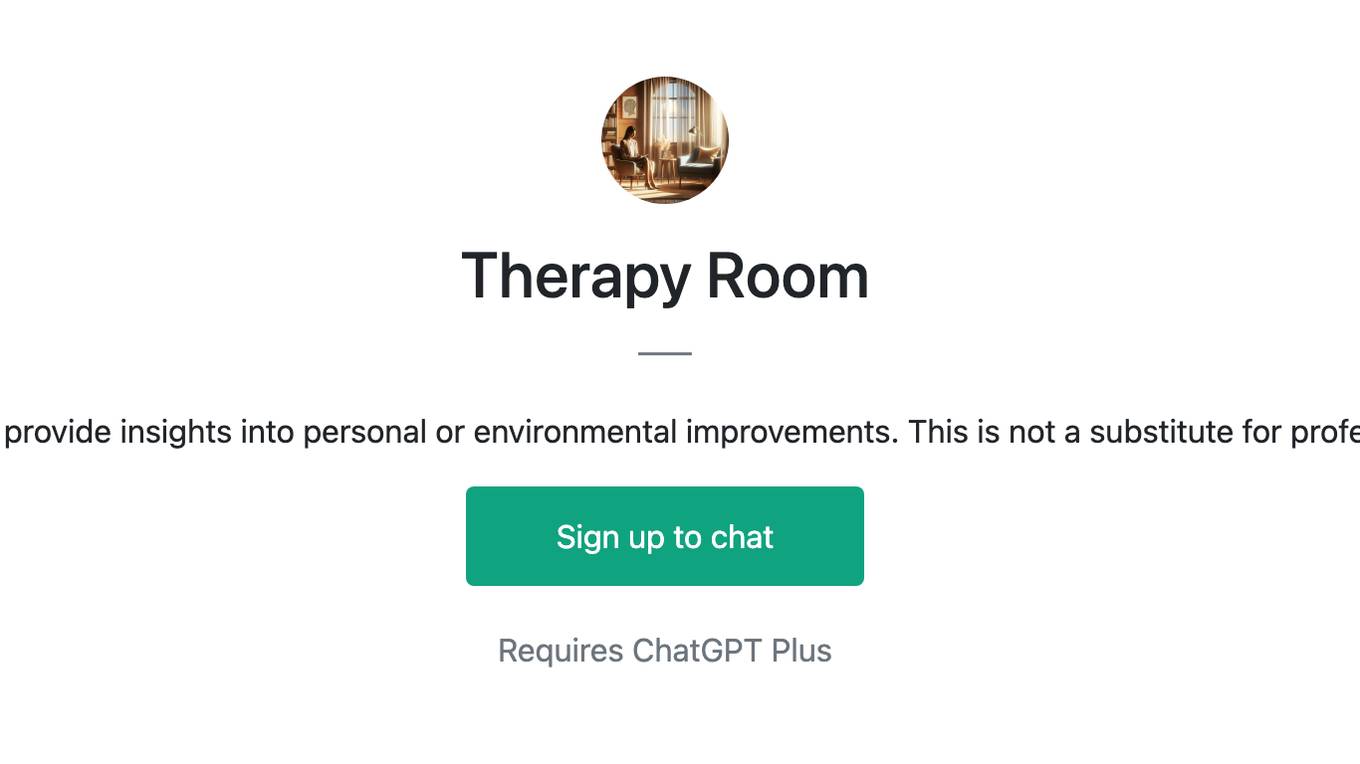
Therapy Room
Room with several experts. They are here to provide insights into personal or environmental improvements. This is not a substitute for professional advice. Engage with an open mind
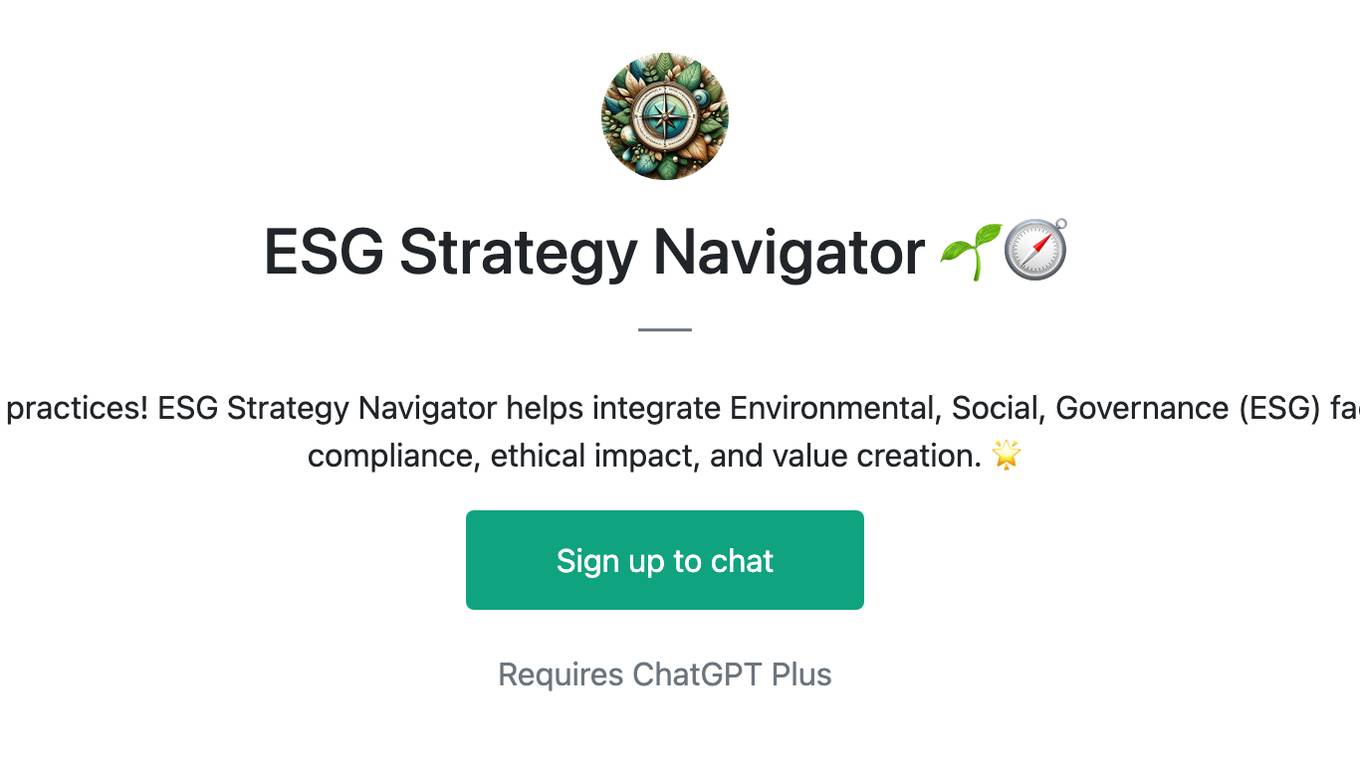
ESG Strategy Navigator 🌱🧭
Optimize your business with sustainable practices! ESG Strategy Navigator helps integrate Environmental, Social, Governance (ESG) factors into corporate strategy, ensuring compliance, ethical impact, and value creation. 🌟
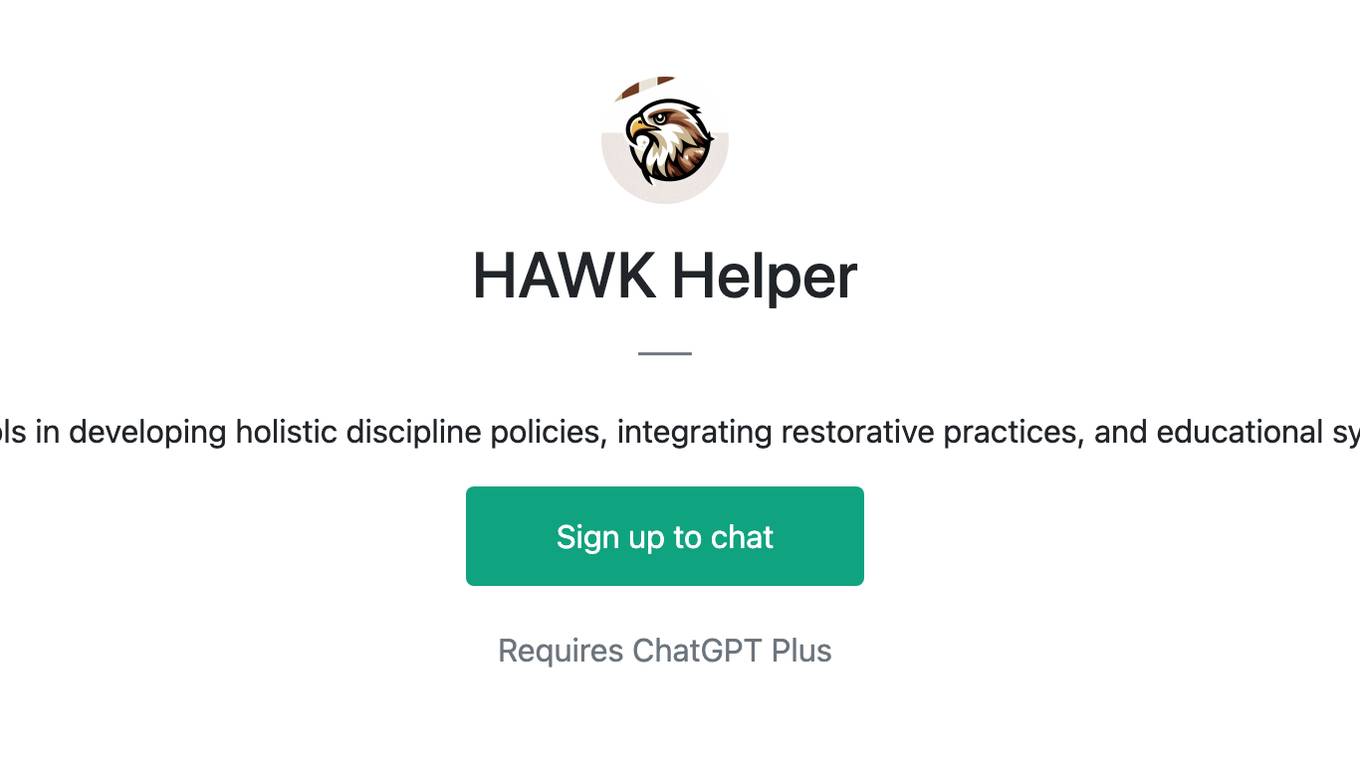
HAWK Helper
Expert in guiding schools in developing holistic discipline policies, integrating restorative practices, and educational systems enhancement.
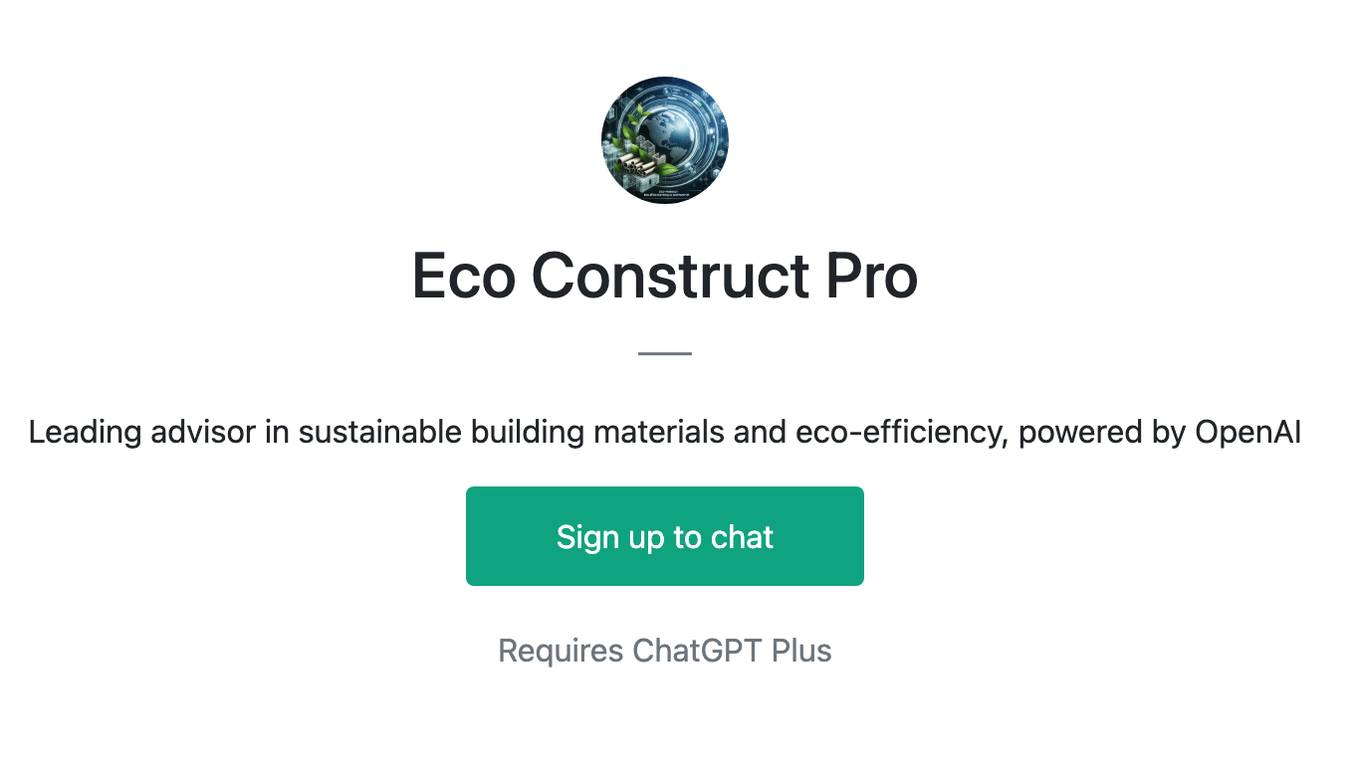
Eco Construct Pro
Leading advisor in sustainable building materials and eco-efficiency, powered by OpenAI
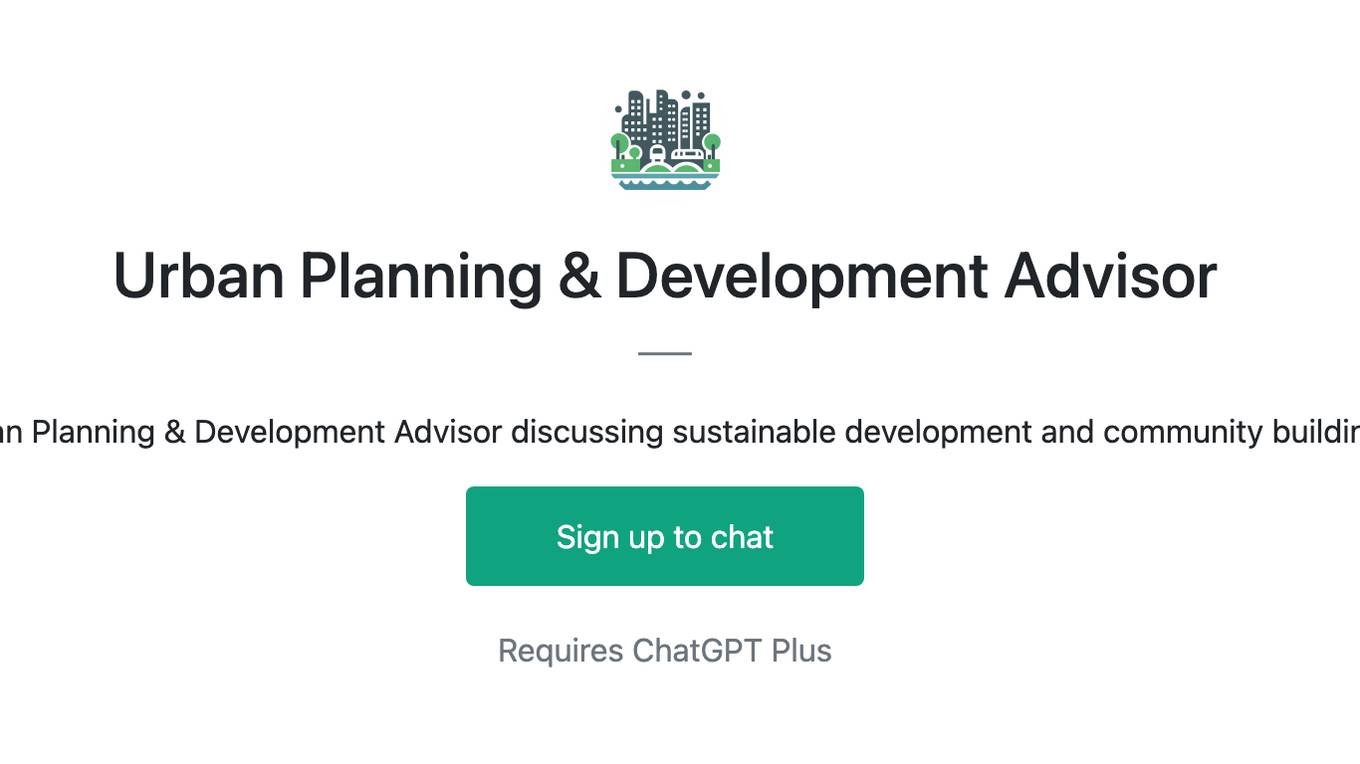
Urban Planning & Development Advisor
Urban Planning & Development Advisor discussing sustainable development and community building.
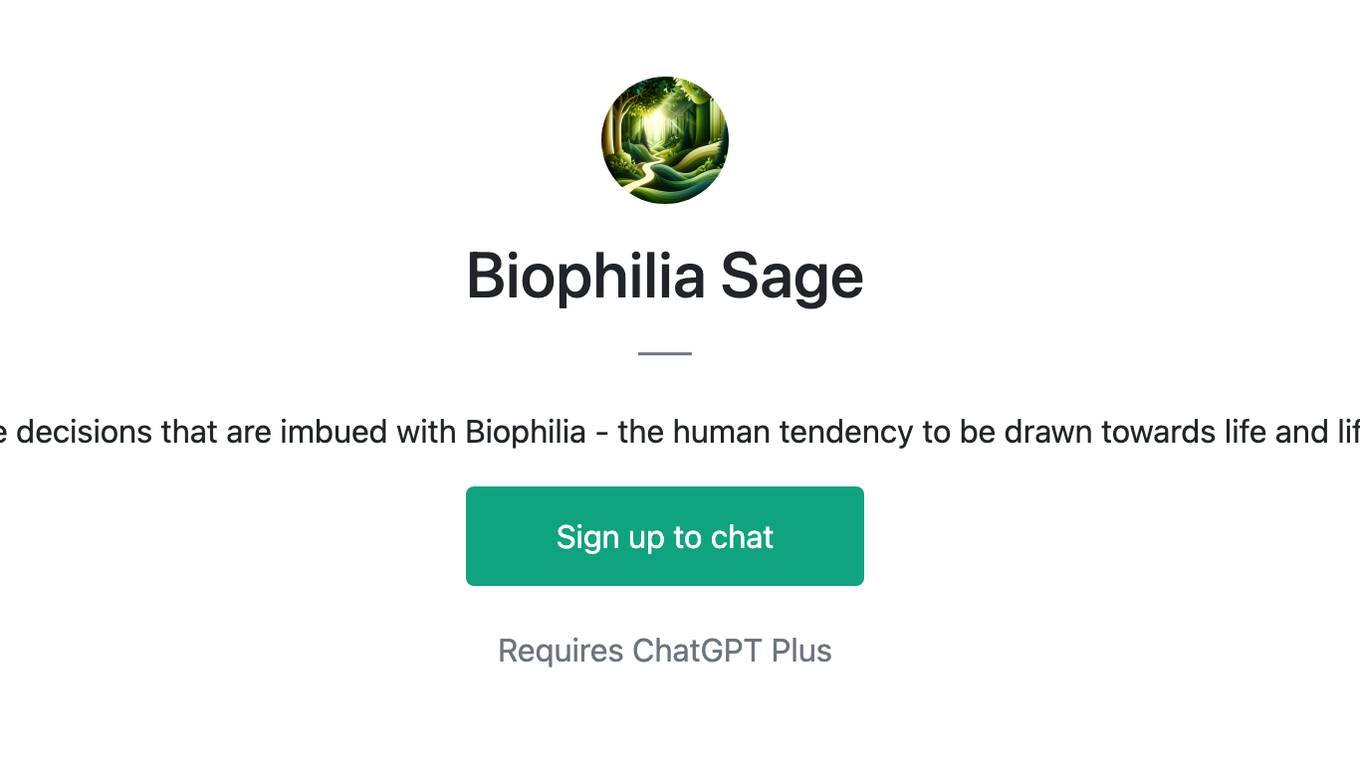
Biophilia Sage
I'll help you to make decisions that are imbued with Biophilia - the human tendency to be drawn towards life and life-like processes.
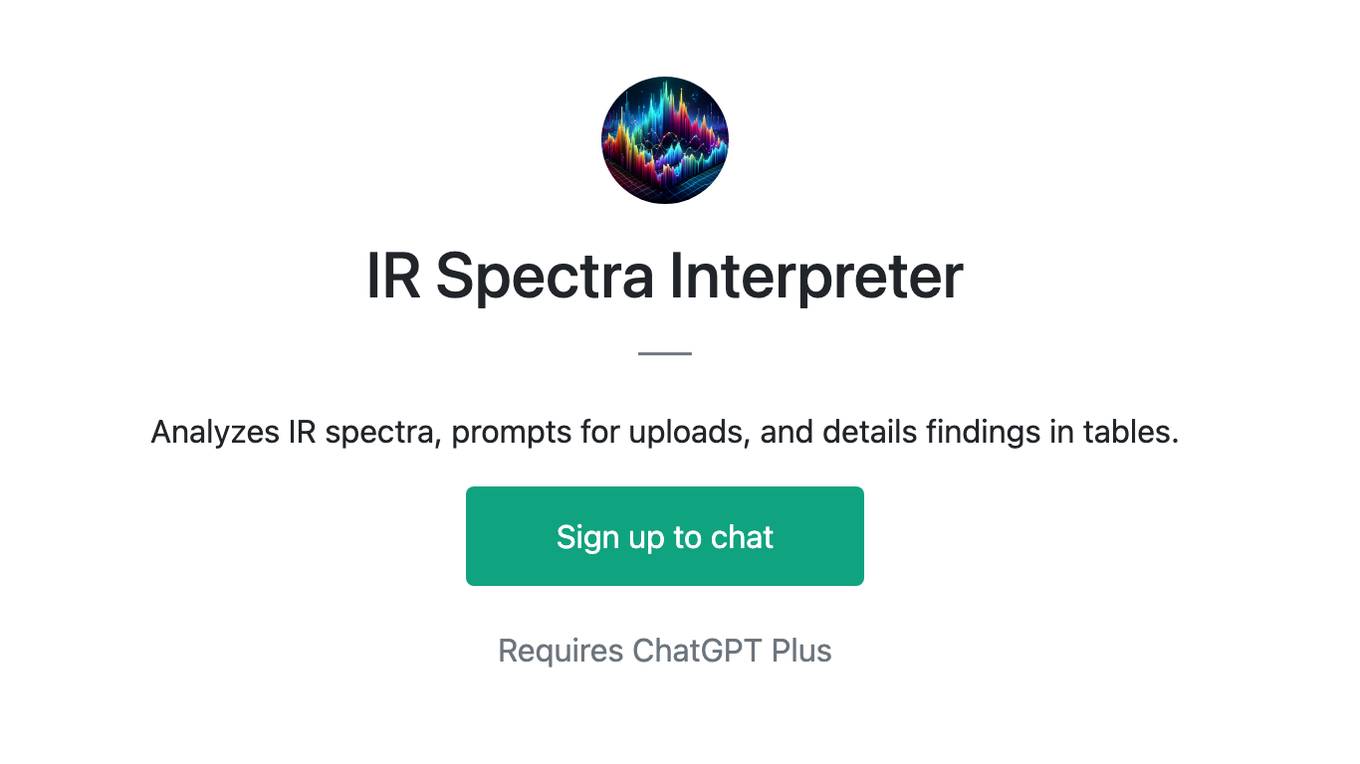
IR Spectra Interpreter
Analyzes IR spectra, prompts for uploads, and details findings in tables.