Best AI tools for< Find Cafes >
20 - AI tool Sites
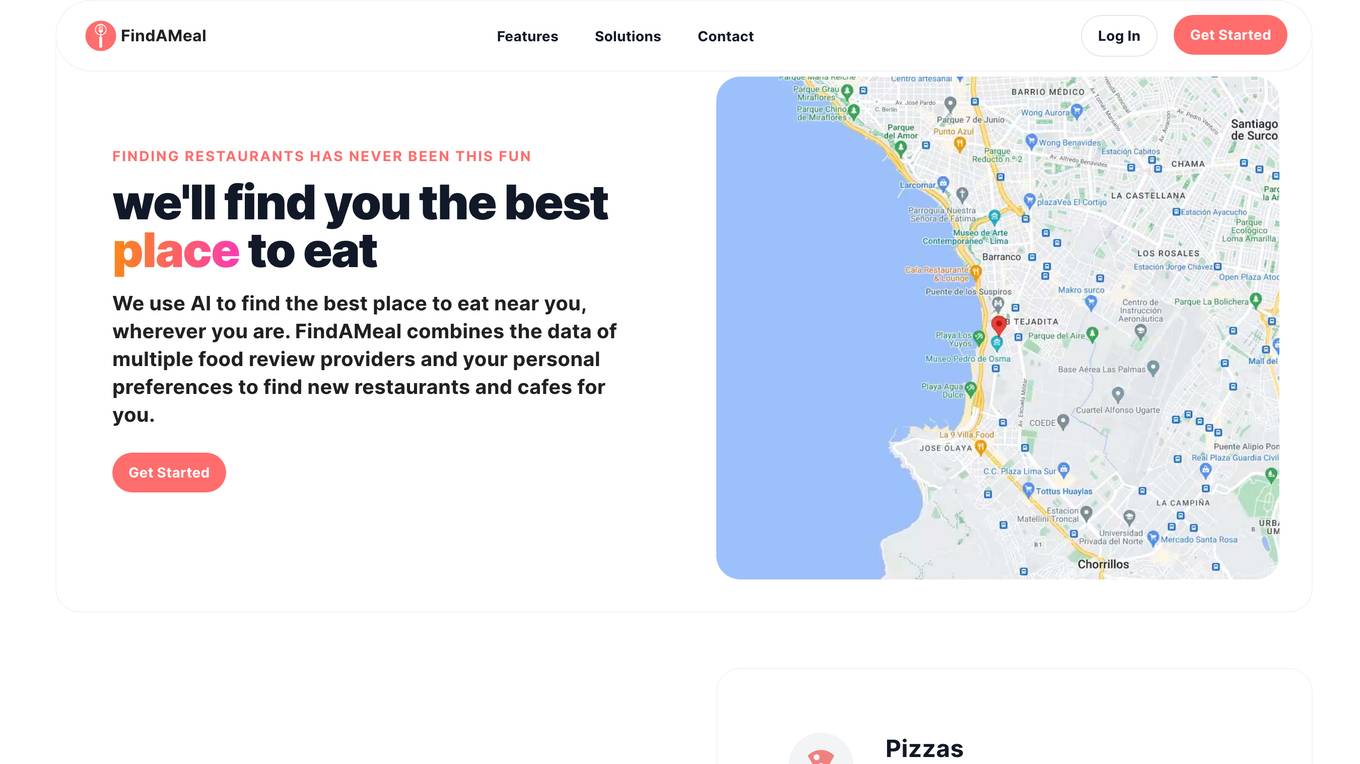
FindAMeal
FindAMeal is an AI-powered search engine that helps users discover nearby restaurants based on their personal preferences. By combining data from various food review providers and utilizing AI technology, FindAMeal simplifies the process of finding the perfect dining spot. Users can make any type of query, from finding a date spot to selecting a fancy restaurant for special occasions. The platform aggregates information from sources like Google, Yelp, and Foursquare to offer tailored recommendations, making it easy for users to explore new dining experiences.
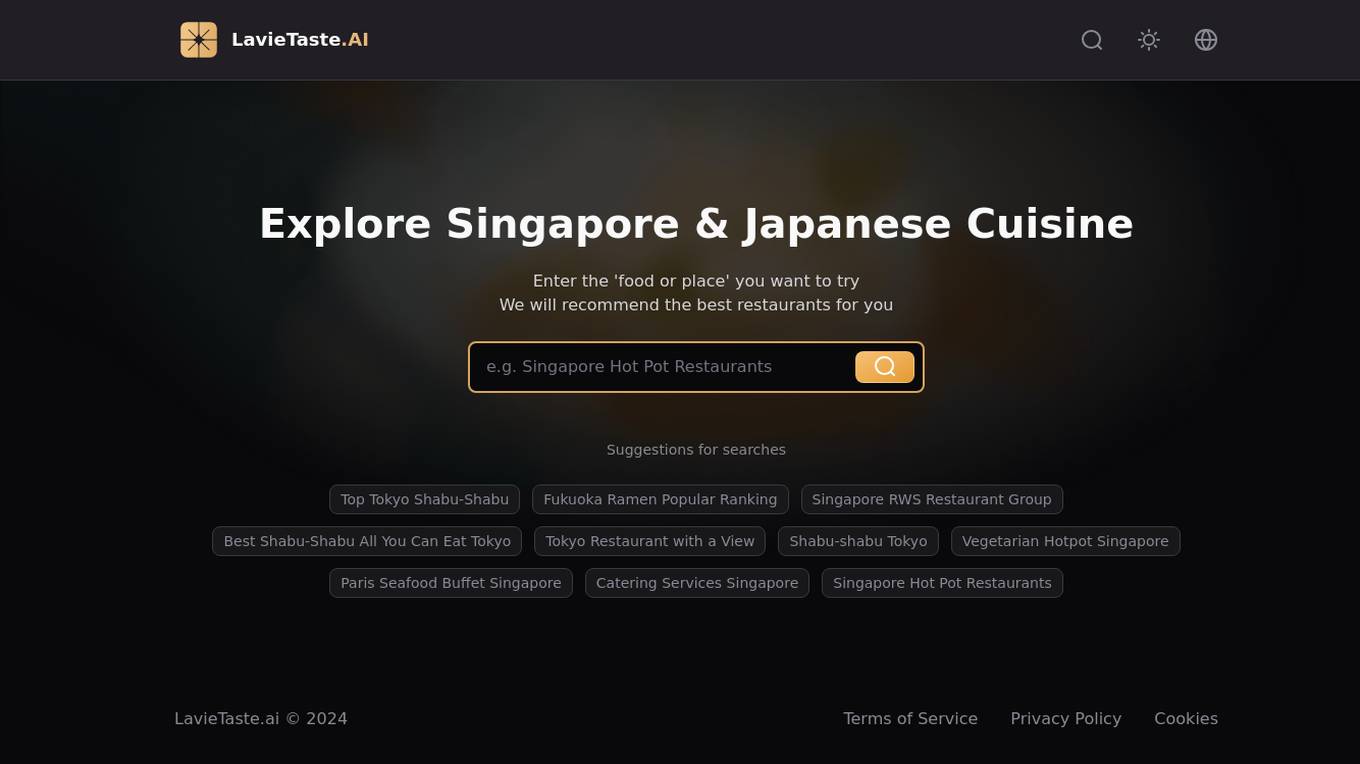
LavieTaste.AI
LavieTaste.AI is an AI-powered application that helps users explore and discover the best restaurants offering Singaporean and Japanese cuisine. By simply entering the desired food or place, the tool provides personalized recommendations for top dining experiences. Users can find a variety of options ranging from retro cafes to popular buffets and top steak houses. LavieTaste.AI aims to enhance the culinary journey of users by offering tailored suggestions based on their preferences and location.
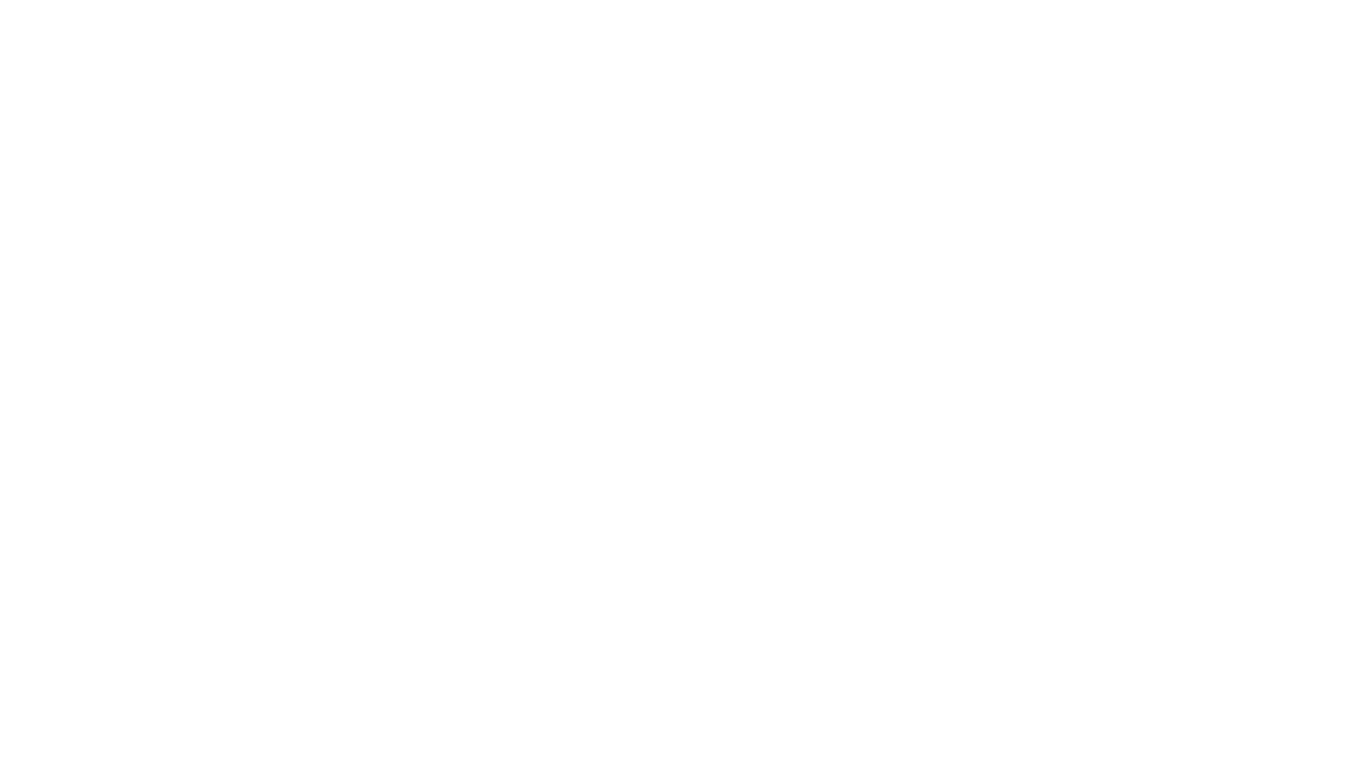
CodiumAI
CodiumAI is an AI-powered tool that helps developers write better code by generating meaningful tests, finding edge cases and suspicious behaviors, and suggesting improvements. It integrates with popular IDEs and Git platforms, and supports a wide range of programming languages. CodiumAI is designed to help developers save time, improve code quality, and stay confident in their code.
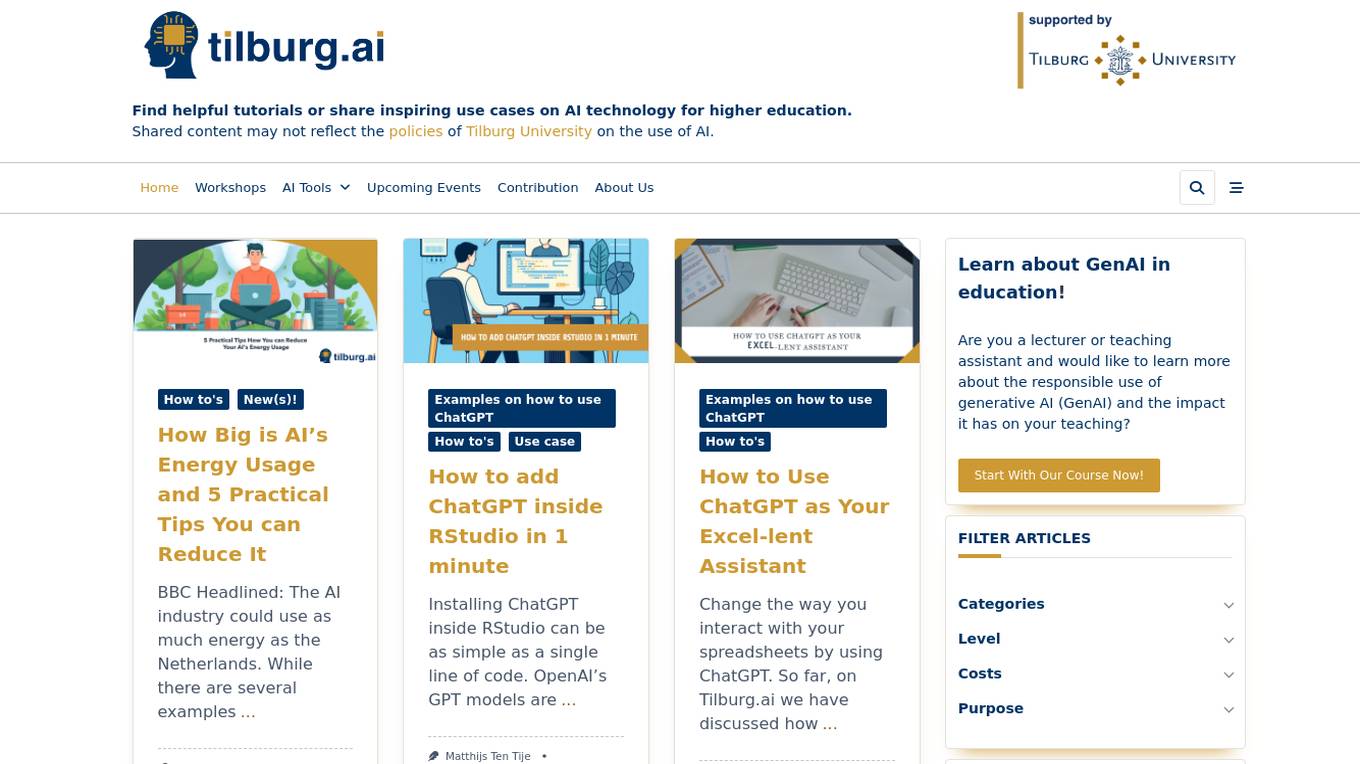
Tilburg.ai
Tilburg.ai is an AI tool that provides helpful tutorials and inspiring use cases on AI technology for higher education. Users can find tutorials on various AI applications, such as ChatGPT, OpenAI API, and AI for web scraping. The platform aims to educate and assist individuals in utilizing AI tools effectively in their educational endeavors.
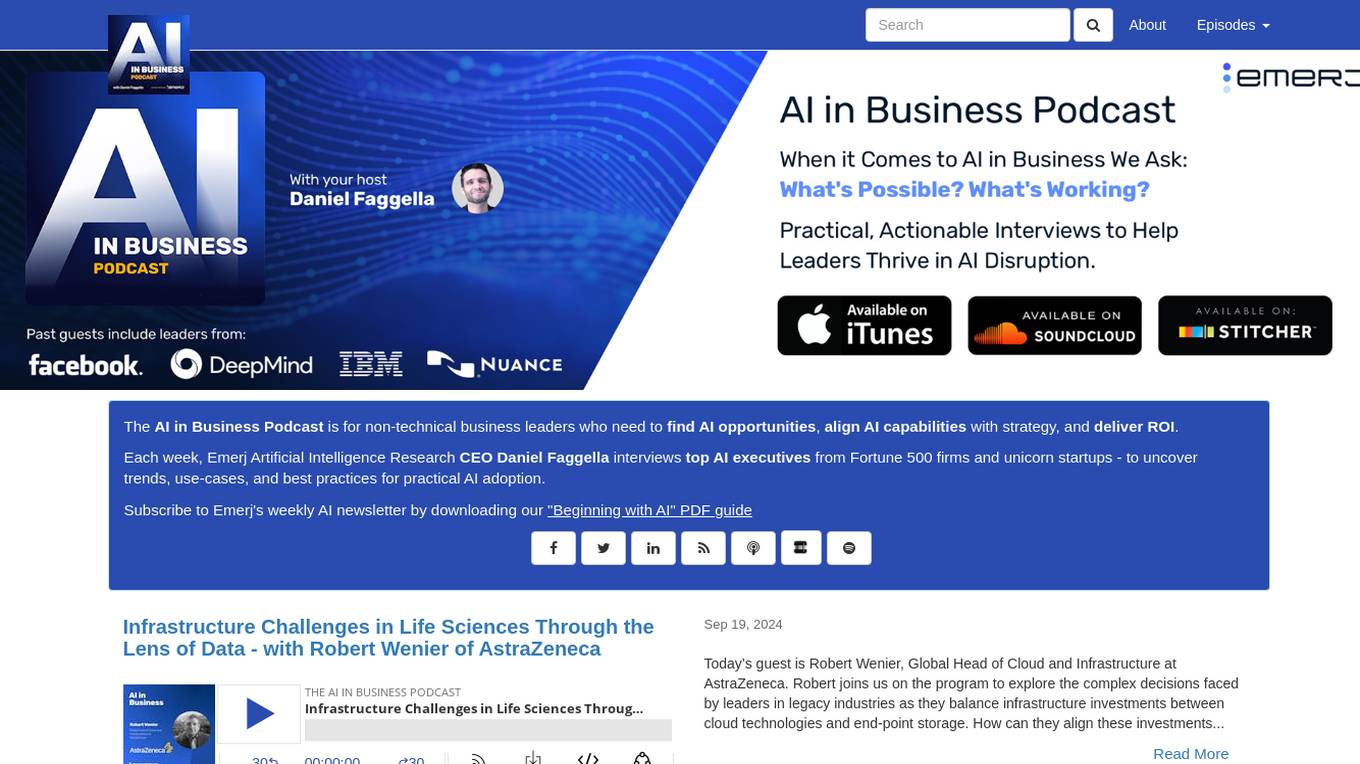
The AI in Business Podcast
The AI in Business Podcast is a platform designed for non-technical business leaders seeking AI opportunities, aligning AI capabilities with strategy, and achieving ROI. The podcast features interviews with top AI executives from Fortune 500 firms and unicorn startups, exploring trends, use-cases, and best practices for practical AI adoption.
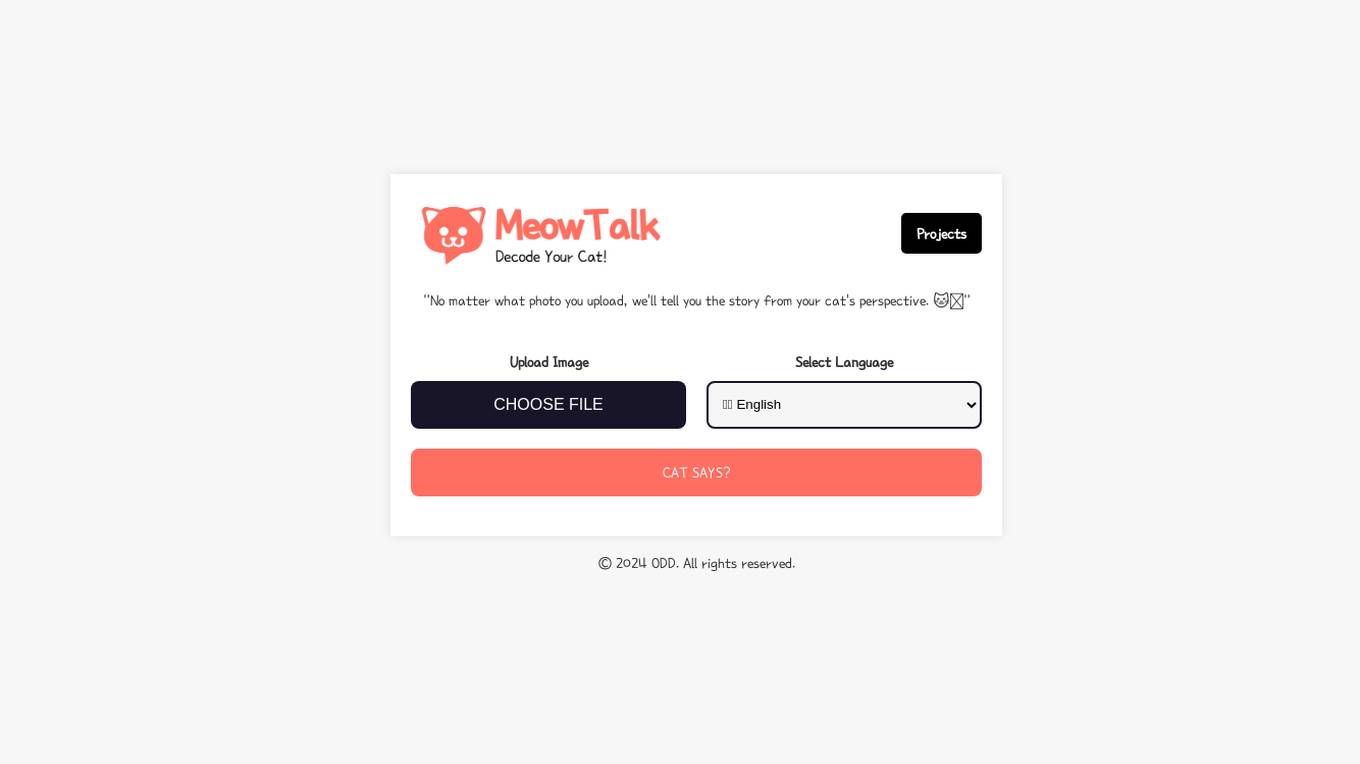
MeowTalk
MeowTalk is an AI tool that allows users to decode their cat's meows and understand what their feline friends are trying to communicate. By analyzing the sound patterns of your cat's meows, MeowTalk translates them into human language, providing insights into your cat's thoughts and feelings. With MeowTalk, you can bridge the communication gap between you and your cat, leading to a deeper understanding and stronger bond.
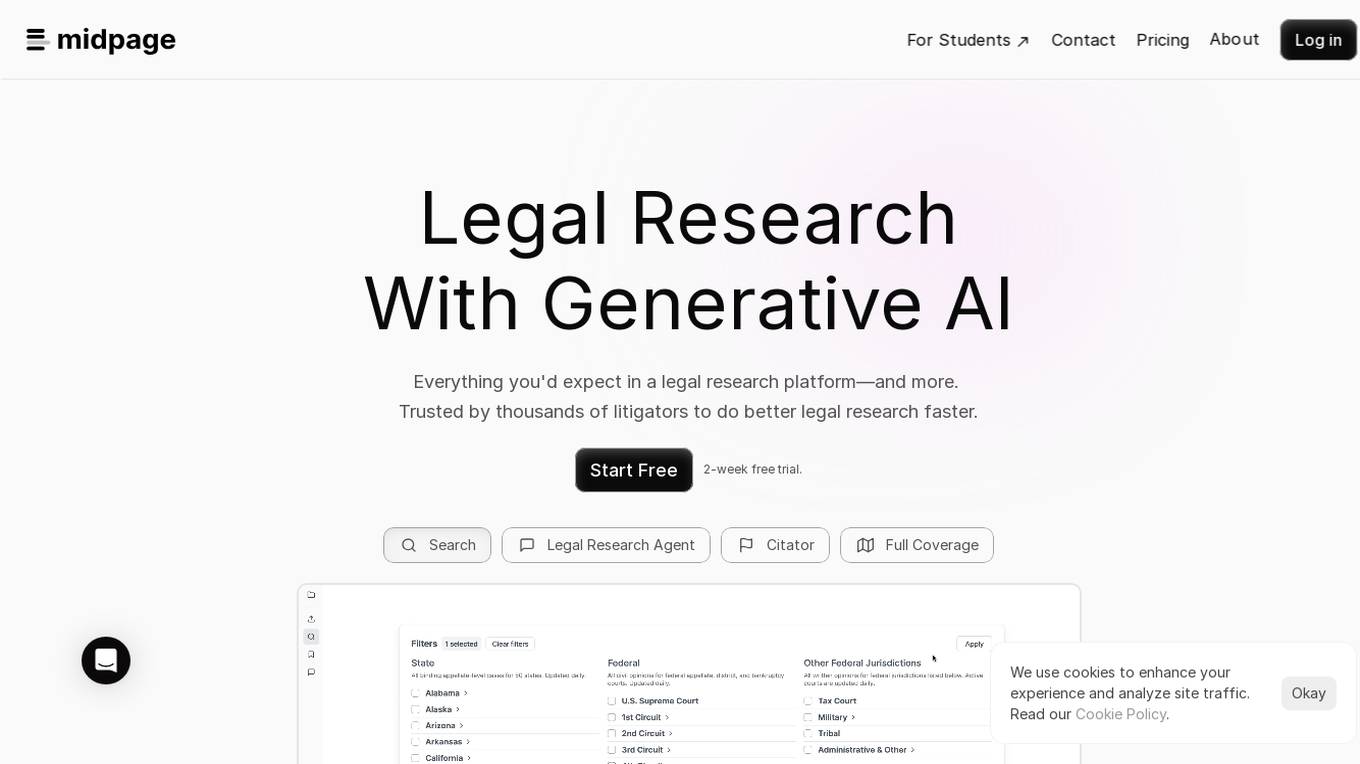
Midpage
Midpage is a legal research platform powered by Generative AI, designed to provide comprehensive legal research capabilities to students and professionals. The platform offers advanced features such as grid-based search, case filtering with AI, proactive annotations, and seamless integration of research into documents. Midpage aims to streamline the legal research process by leveraging AI technology to enhance efficiency and accuracy in analyzing legal cases and statutes.
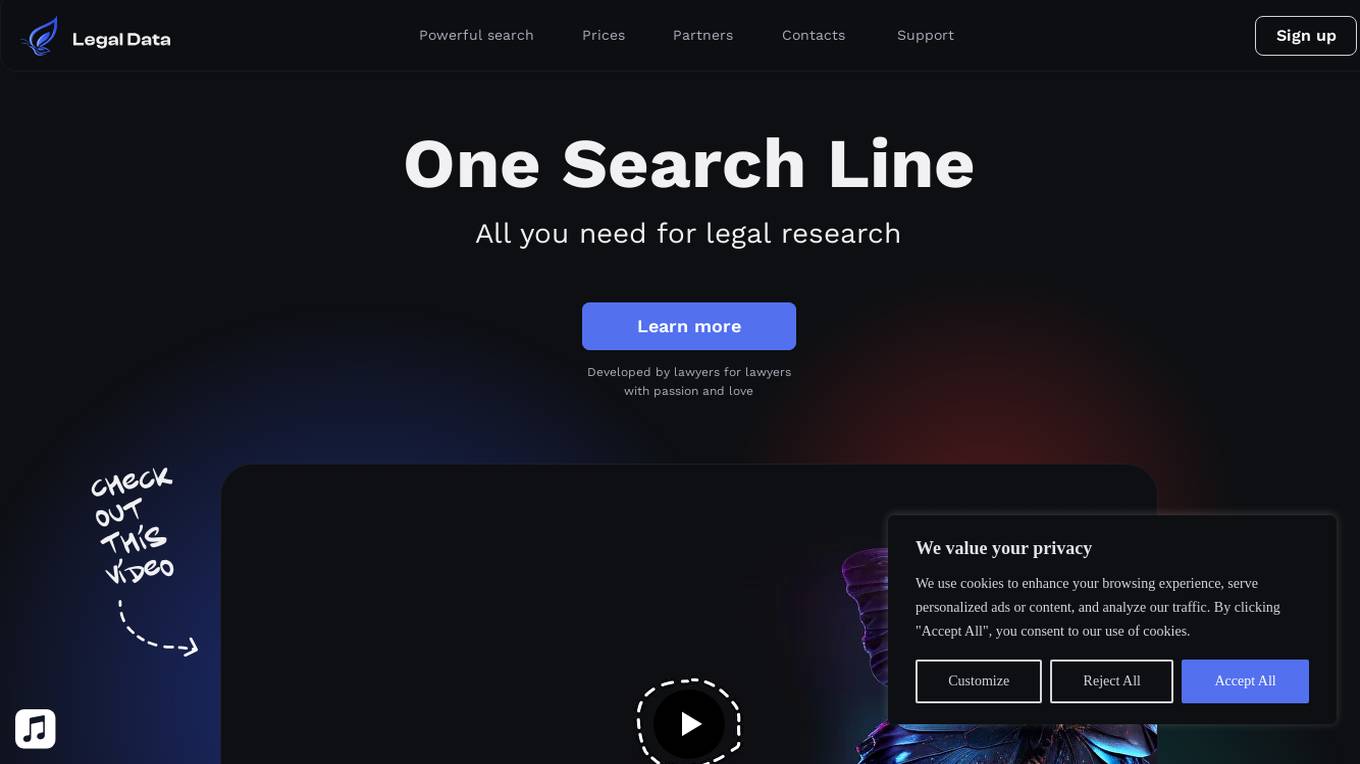
Legal Data
Legal Data is a comprehensive legal research platform developed by lawyers for lawyers. It offers a powerful search feature that covers various legal areas from commercial to criminal law. The platform recognizes synonyms, legalese, and abbreviations, corrects typos, and provides suggestions as you type. Additionally, Legal Data includes an AI-assistant called FlyBot, trained on carefully selected laws and cases, to provide accurate legal answers without fabricating information.
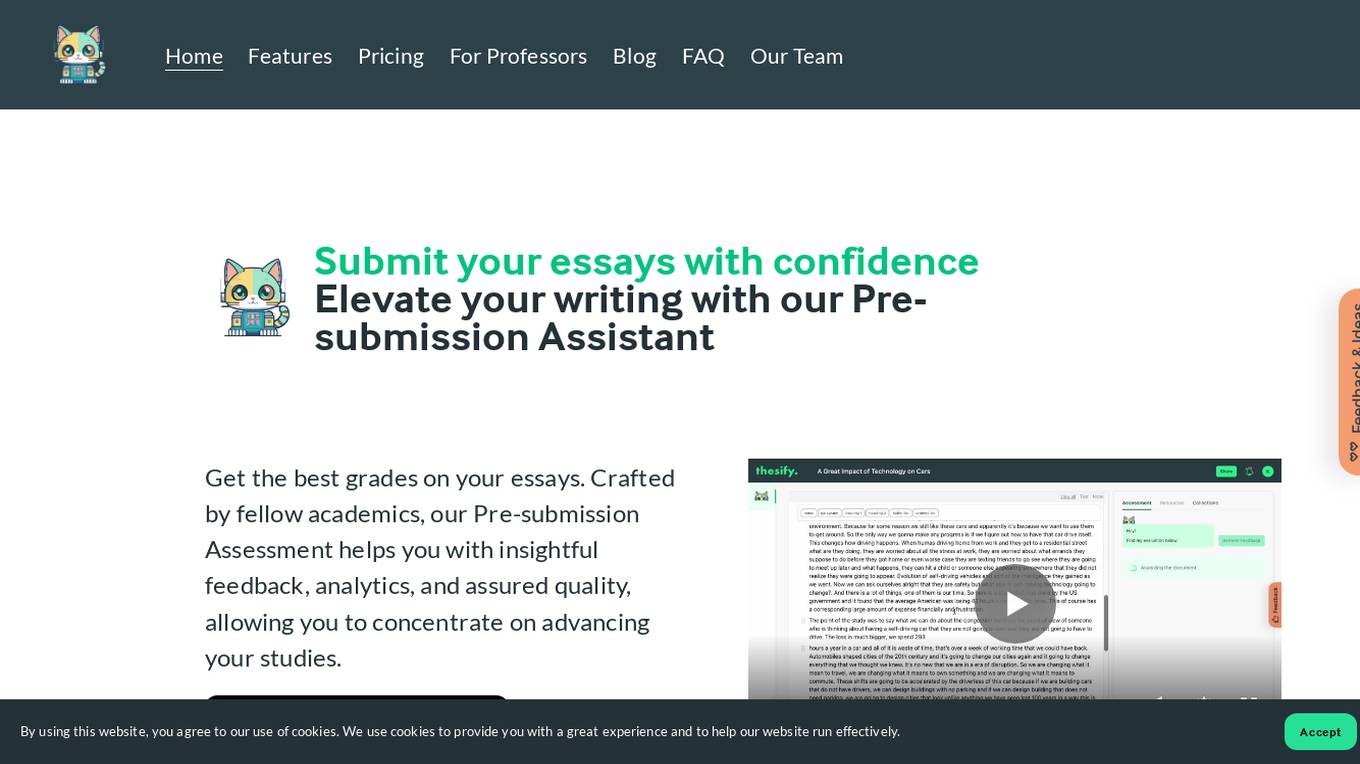
Thesify.ai
Thesify.ai is an AI essay writing tool designed to assist students in academic writing. It offers features such as writing-coach, semantic article search, pre-submission assessment, intelligent paper digest, and agile editor. The tool aims to provide AI-powered assistance, insights, and feedback to help students improve their writing skills while maintaining academic integrity.
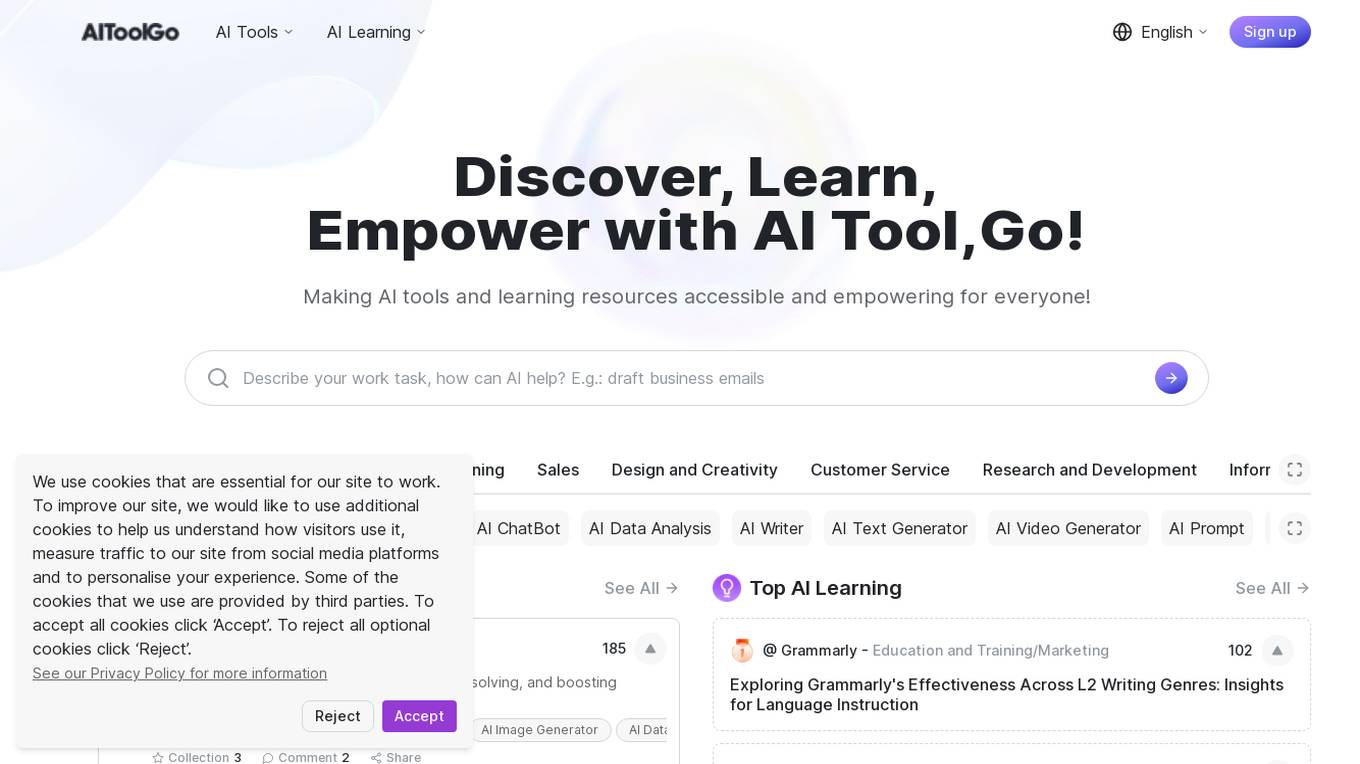
AiToolGo
AiToolGo is an AI learning platform that aims to make AI tools and learning resources accessible and empowering for everyone. The platform offers a curated collection of AI tools, tutorials, use cases, and expert insights across various industries. Whether you're a beginner or a pro, AiToolGo is designed to help you excel in AI skills. The platform provides top AI tools such as AI ChatBot, AI Image Generator, and AI Writer, along with top AI learning resources like AI Assistant, AI Text Generator, and AI Data Analysis. AiToolGo is a one-stop platform for discovering, learning, and empowering individuals with the potential of AI for both personal and professional growth.
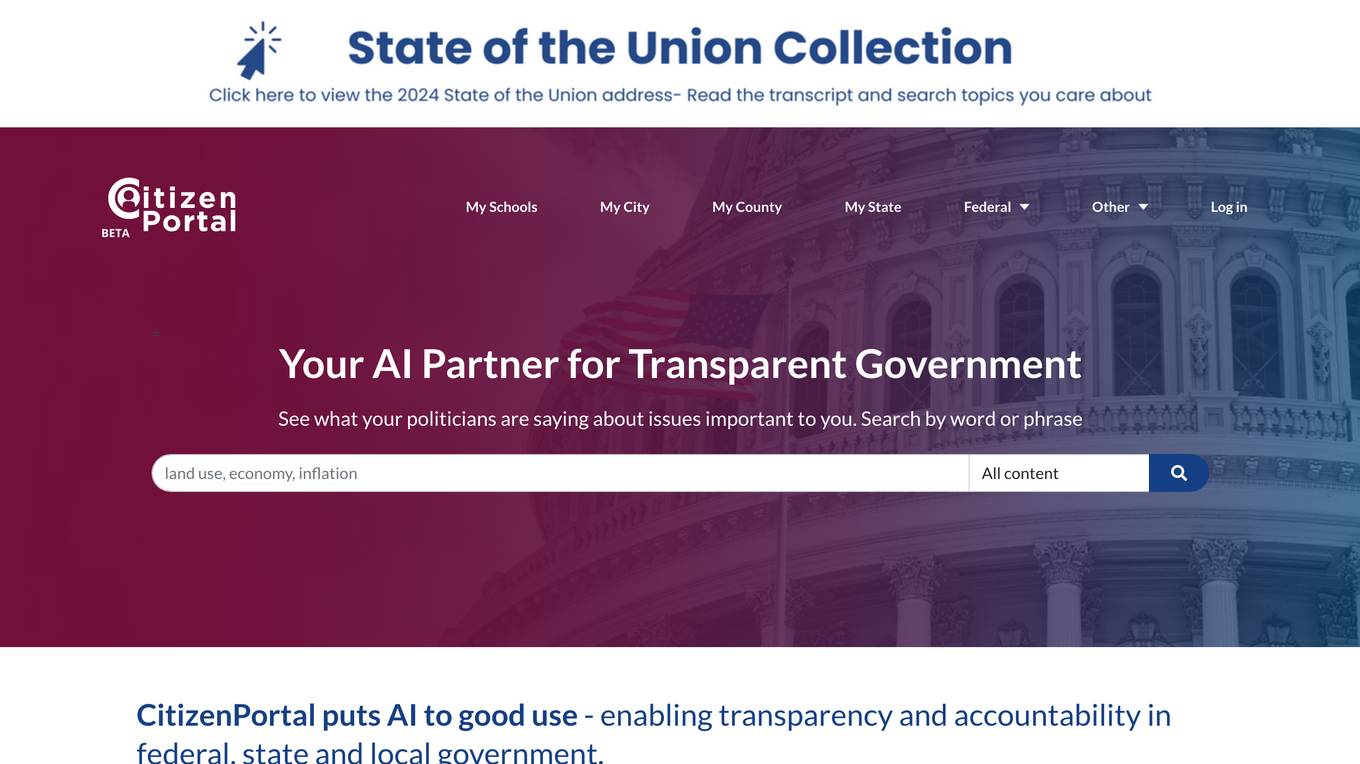
CitizenPortal.ai
CitizenPortal.ai is a comprehensive online platform designed for informed citizens to access a wide range of information related to local government, schools, city, county, state representatives, bills, media, podcasts, federal library, and more. Users can search and browse through various documents, including Congressional hearings, Executive Orders, Federalist Papers, Supreme Court cases, and State of the Union addresses. The platform offers a user-friendly interface with search options like Heat Map Search, Content Search, Article Search, and Congress GPT. CitizenPortal.ai aims to empower citizens by providing them with easy access to valuable information from official government sources.
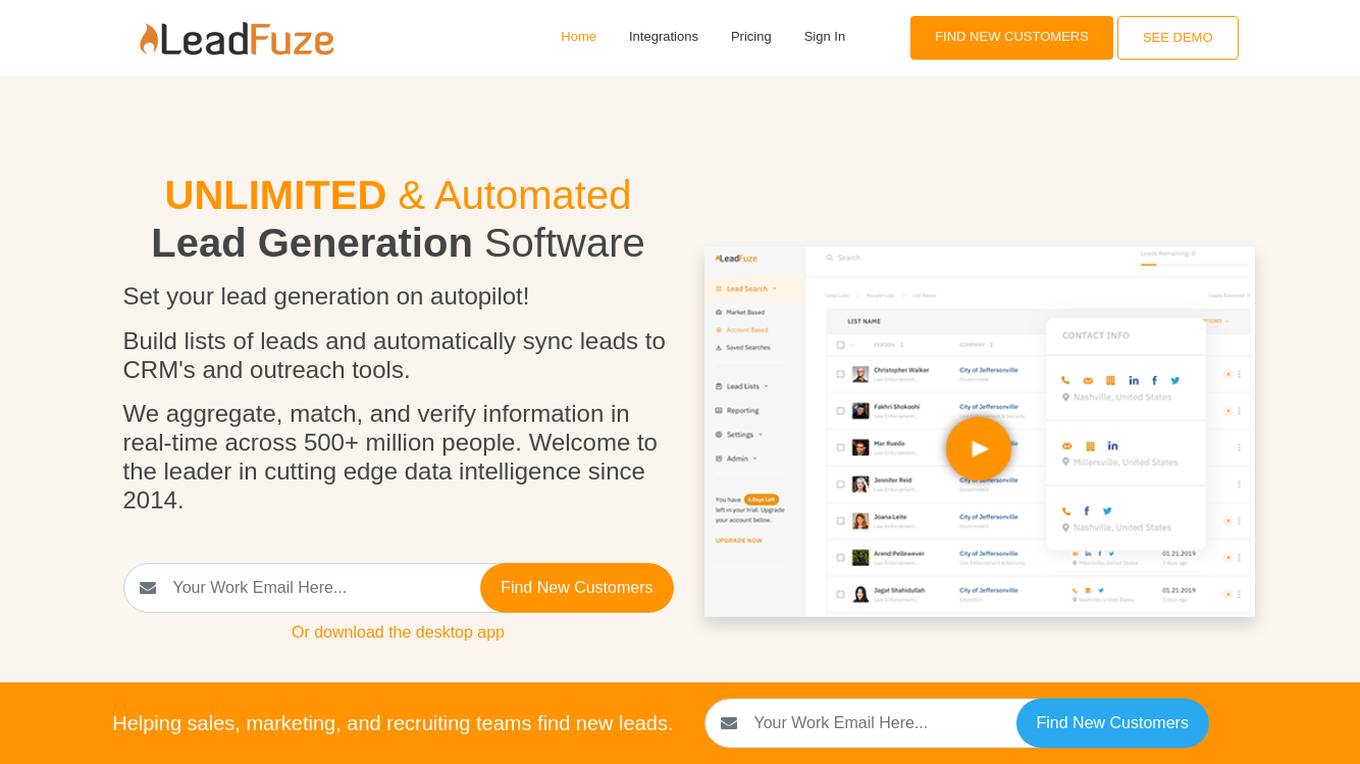
LeadFuze
LeadFuze is an AI-powered prospecting tool for business leads and candidate sourcing. It offers unlimited and automated lead generation software that sets lead generation on autopilot. LeadFuze aggregates, matches, and verifies information in real-time across 500+ million people. It provides accurate contact and data intelligence for revenue teams and recruiters, with top ratings for ease-of-use, quality of support, and ease-of-setup. The tool is built specifically for sales, marketing, and recruiting use cases, offering hyper-targeted lead searches, complete list building automation, and integration with CRM and workflow tools. LeadFuze's AI capabilities allow users to find new leads, search specific accounts, and automate lead generation workflows effortlessly.
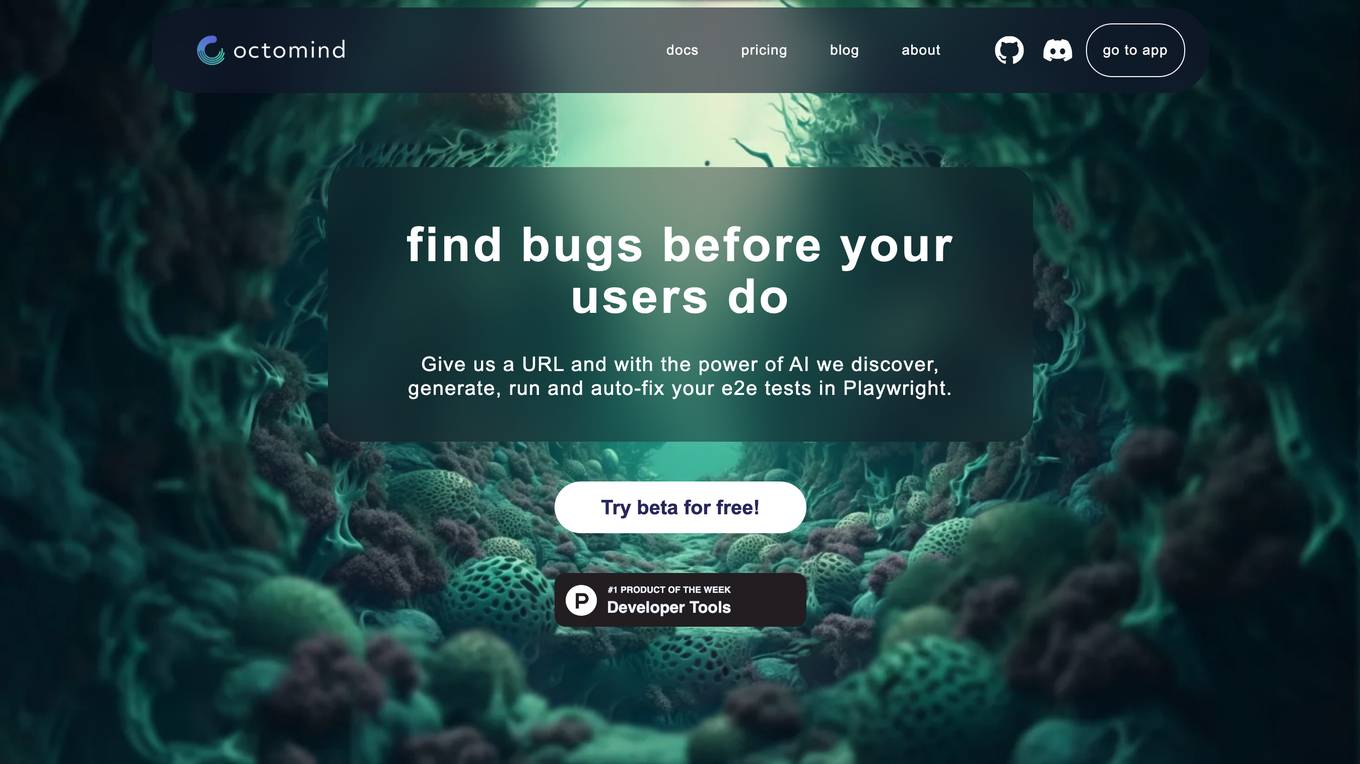
Octomind
Octomind is an AI-powered Playwright end-to-end testing tool for web applications. It automatically discovers, generates, and runs tests to find bugs before customers do. With features like auto-generating tests, running tests to find bugs, maintaining tests automatically, debugging apps, and not requiring code access, Octomind offers a seamless testing experience for developers. It provides real-world wins with testimonials from industry professionals and ensures stability, speed, and a better developer experience.
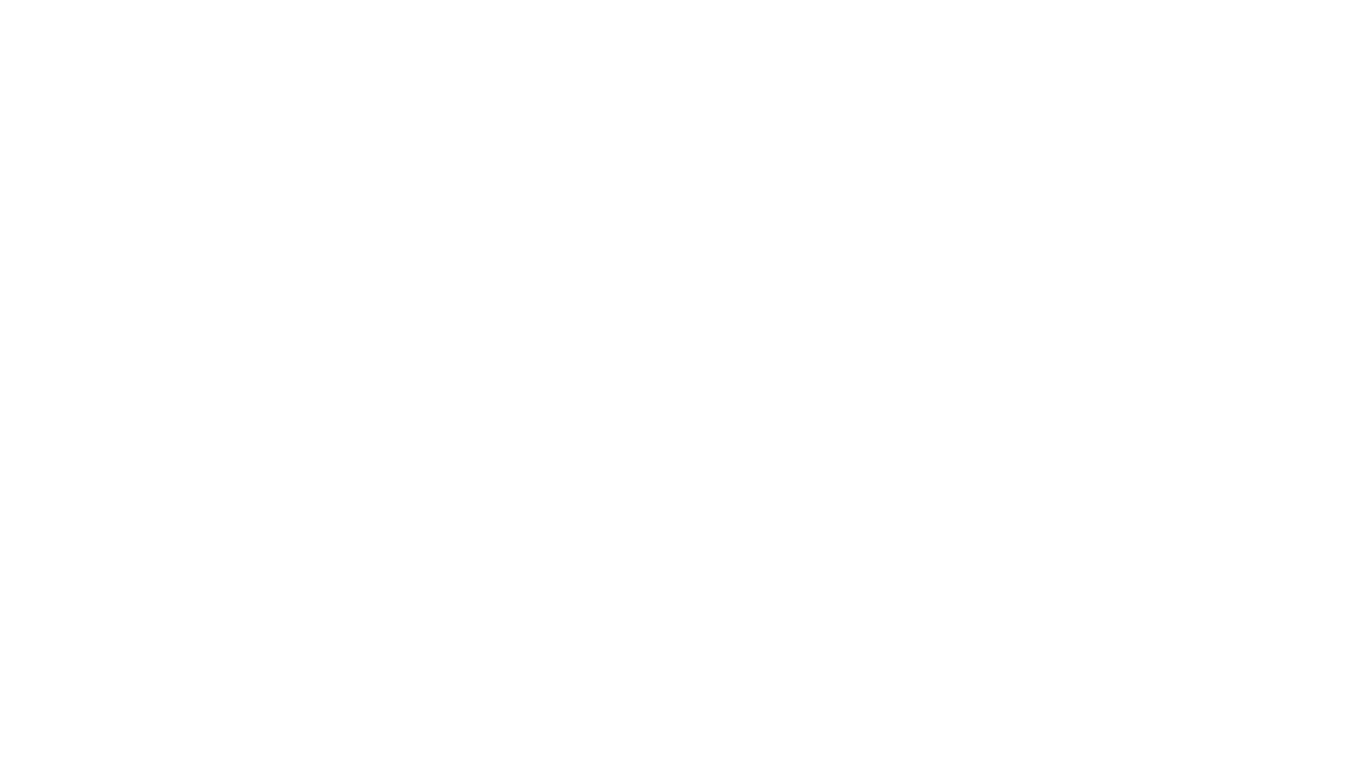
Dr. Amit Ray
Dr. Amit Ray is famous for his teachings on peace, compassion, meditation, non- violence, 114 chakras, compassionate AI, mindfulness, leadership and creativity. He is also famous for his contribution in the field of quantum computing and artificial intelligence. He has spent several years in high Himalaya in deep silent meditation. He is one of the rare meditation master, who has fully experienced the higher consciousness and fully conversant with the ancient meditation literature as well as modern high end researches. He often remains engrossed in deep meditation in the dense forests, caves, and snow-covered peaks of the Himalayas. At the same time he always keeps a watchful compassionate gaze for the well-being of the humanity. His compassion and meditation teachings transcends the limitations of worldly distinctions such as religion, country, culture, caste, class, color, history, and geography.
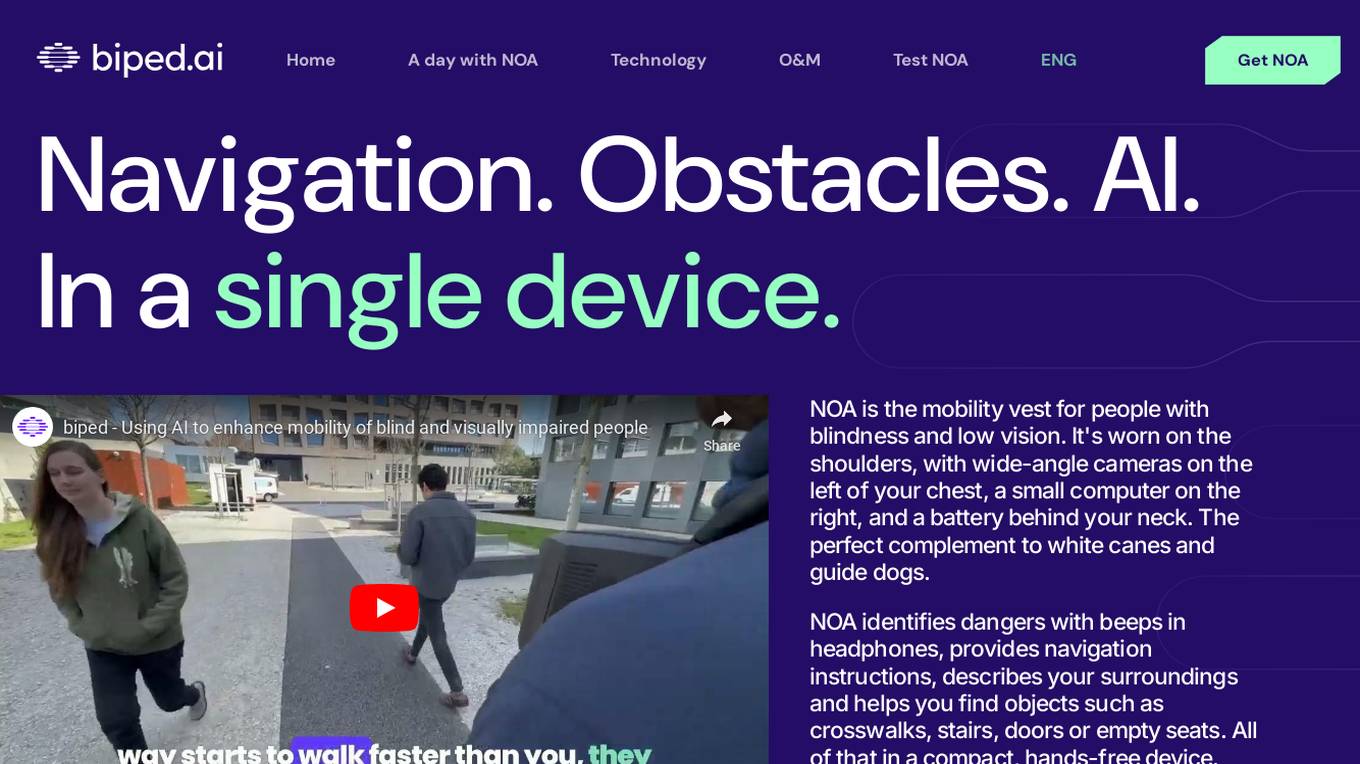
NOA
NOA by biped.ai is a revolutionary mobility vest designed to enhance the independence and safety of individuals with blindness and low vision. It combines cutting-edge AI technology with wearable devices to provide real-time navigation instructions, obstacle detection, and object finding capabilities. NOA is a hands-free solution that complements traditional mobility aids like white canes and guide dogs, offering a compact and lightweight design for seamless integration into daily life. Developed through extensive research and collaboration with experts in the field, NOA aims to empower users to navigate their surroundings with confidence and ease.
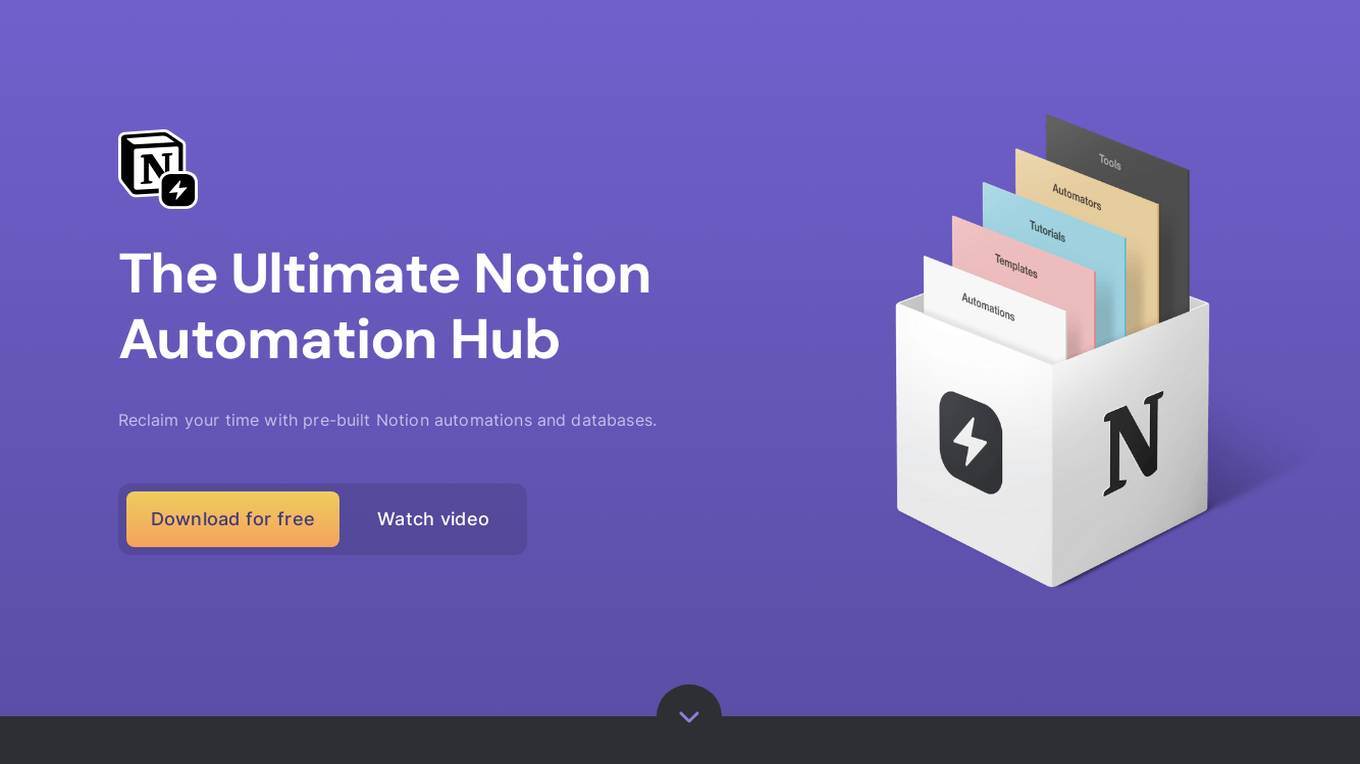
The Notion Automation Hub
The Notion Automation Hub is a website that provides pre-built Notion automations and databases to help users save time and improve their productivity. The website offers a variety of automations for different use cases, including job roles, workflows, and tasks. Users can also find pre-built database templates, Notion expert resources, and automation tools. The website is not affiliated with Notion Labs Inc.
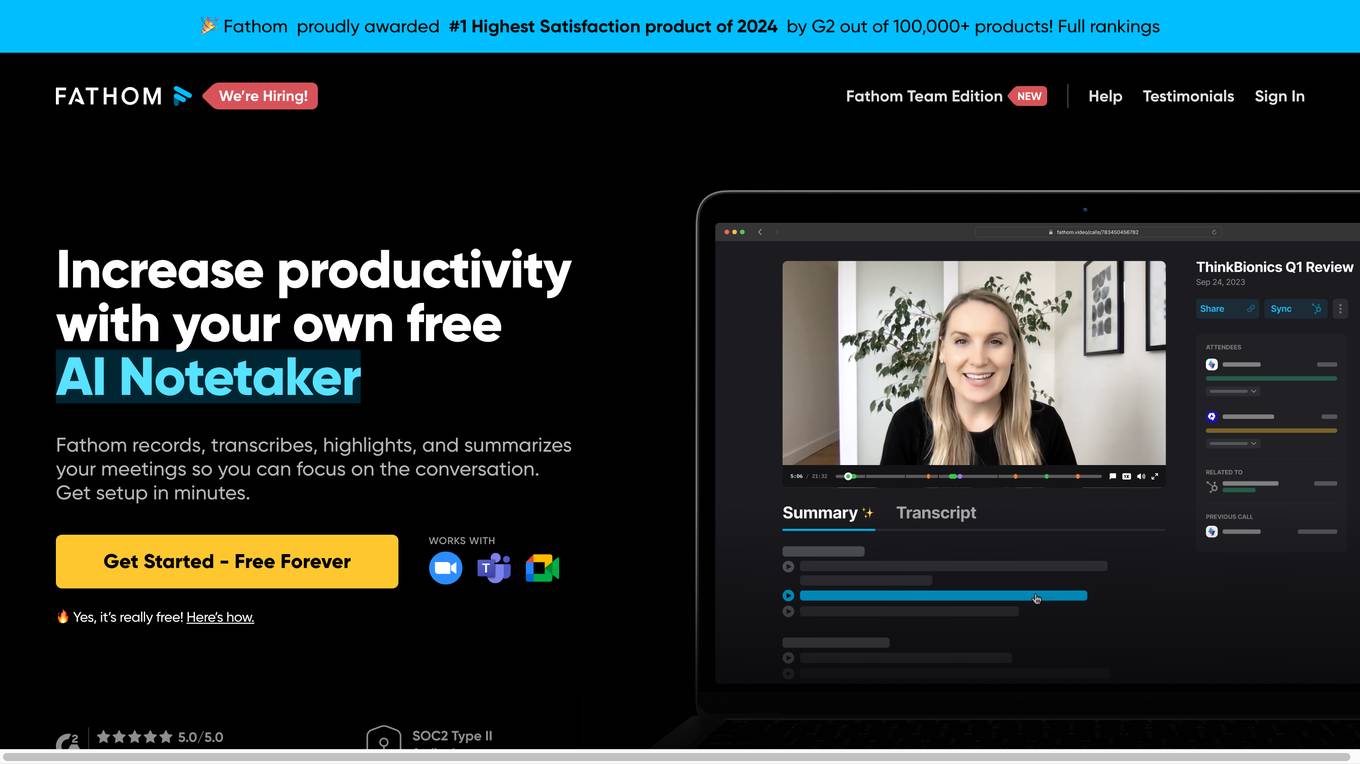
Fathom AI Notetaker
Fathom is an AI-powered note-taking tool that helps you record, transcribe, and summarize your meetings. It integrates with Zoom and Google Meet, and offers a range of features to help you stay organized and productive. **Key Features** * **Automatic recording and transcription:** Fathom automatically records and transcribes your meetings, so you can focus on the conversation instead of taking notes. * **AI-generated summaries:** Fathom uses AI to generate summaries of your meetings, which can save you time and help you identify key takeaways. * **Highlighting and bookmarking:** You can highlight and bookmark important moments in your meetings, so you can easily find them later. * **Sharing and collaboration:** You can share your meeting recordings and summaries with others, and collaborate on notes and action items. * **Integrations:** Fathom integrates with a range of other tools, including Zoom, Google Meet, Slack, and Asana. **Benefits** * **Save time:** Fathom can save you hours of time by automatically recording and transcribing your meetings. * **Stay organized:** Fathom helps you stay organized by providing a central place to store your meeting recordings and notes. * **Improve productivity:** Fathom can help you improve your productivity by providing you with easy access to the information you need from your meetings. * **Make better decisions:** Fathom can help you make better decisions by providing you with a clear understanding of what was discussed in your meetings. **Pricing** Fathom is free to use for individuals. There is also a paid Team Edition that offers additional features, such as: * **Unlimited storage:** The Team Edition gives you unlimited storage for your meeting recordings and notes. * **Team management:** The Team Edition allows you to manage your team's access to Fathom. * **Custom branding:** The Team Edition allows you to customize Fathom with your own branding. **Alternatives** * Otter.ai * Trint * Descript * Rev **Use Cases** * **Sales:** Fathom can help sales teams track their progress and identify opportunities. * **Customer success:** Fathom can help customer success teams build relationships with their customers and resolve issues quickly. * **Product development:** Fathom can help product development teams gather feedback from users and improve their products. * **Marketing:** Fathom can help marketing teams track the effectiveness of their campaigns and generate leads. * **Education:** Fathom can help educators record and share lectures and other materials with students. **FAQ** **Q: How much does Fathom cost?** A: Fathom is free to use for individuals. There is also a paid Team Edition that offers additional features. **Q: What are the benefits of using Fathom?** A: Fathom can save you time, help you stay organized, improve your productivity, and make better decisions. **Q: What are the alternatives to Fathom?** A: Some alternatives to Fathom include Otter.ai, Trint, Descript, and Rev. **Q: What are some use cases for Fathom?** A: Fathom can be used for a variety of purposes, including sales, customer success, product development, marketing, and education.
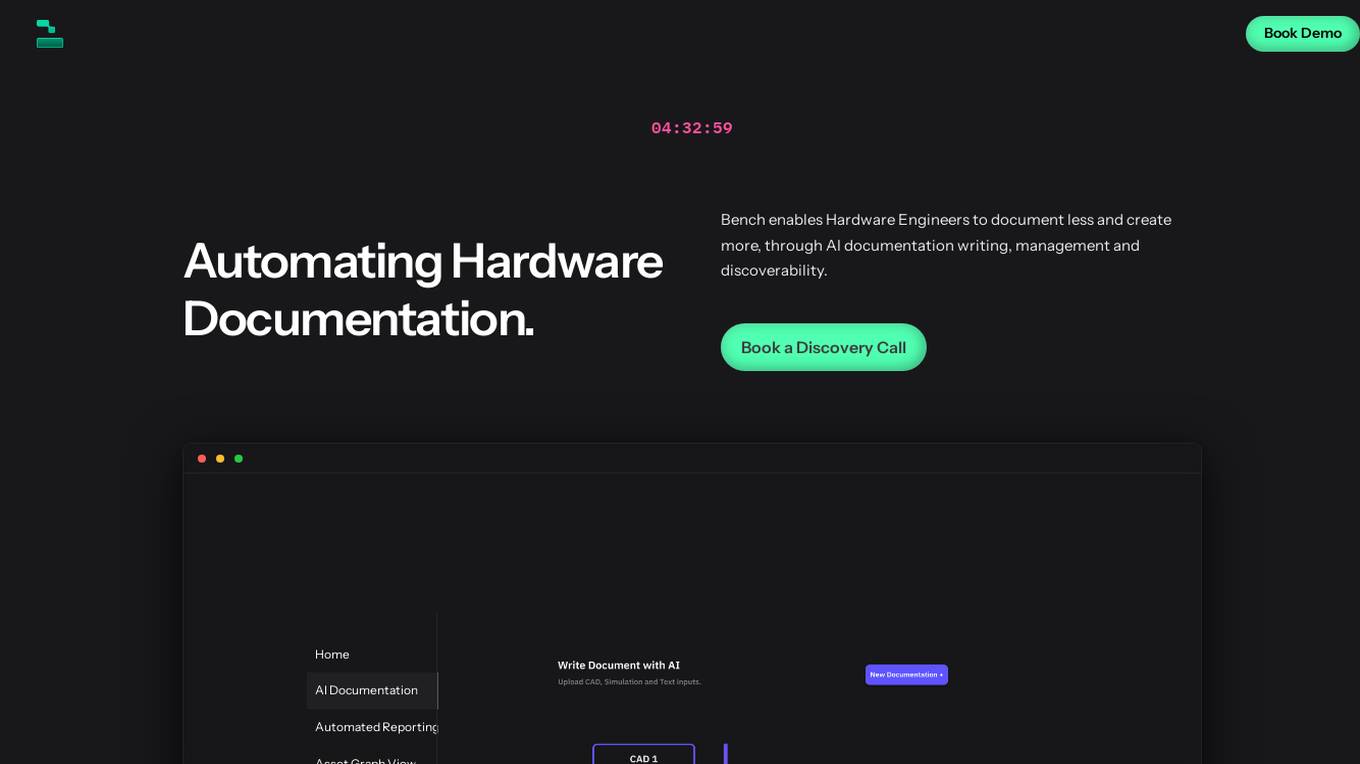
Bench
Bench is an AI tool designed to automate hardware documentation for Hardware Engineers. It helps users document less and create more by utilizing AI for documentation writing, management, and discoverability. The tool offers features such as adapting to specific use cases, AI documentation writing, single source of truth, data-rich asset pages, highlighting compliance gaps, automated reports, and physical asset logging. Bench is advantageous for increasing productivity, improving documentation accuracy, streamlining workflows, enhancing compliance, and enabling seamless integrations. However, it may have limitations in customization options, initial learning curve, and potential dependency on AI accuracy. The tool is suitable for Hardware Engineers, Technical Writers, Documentation Specialists, Compliance Officers, and Quality Assurance Engineers. Users can find Bench using keywords like AI documentation, hardware documentation automation, AI writing tool, documentation management tool, and asset logging AI. Tasks users can perform with Bench include automate documentation, manage assets, write AI documentation, generate reports, and log physical assets.
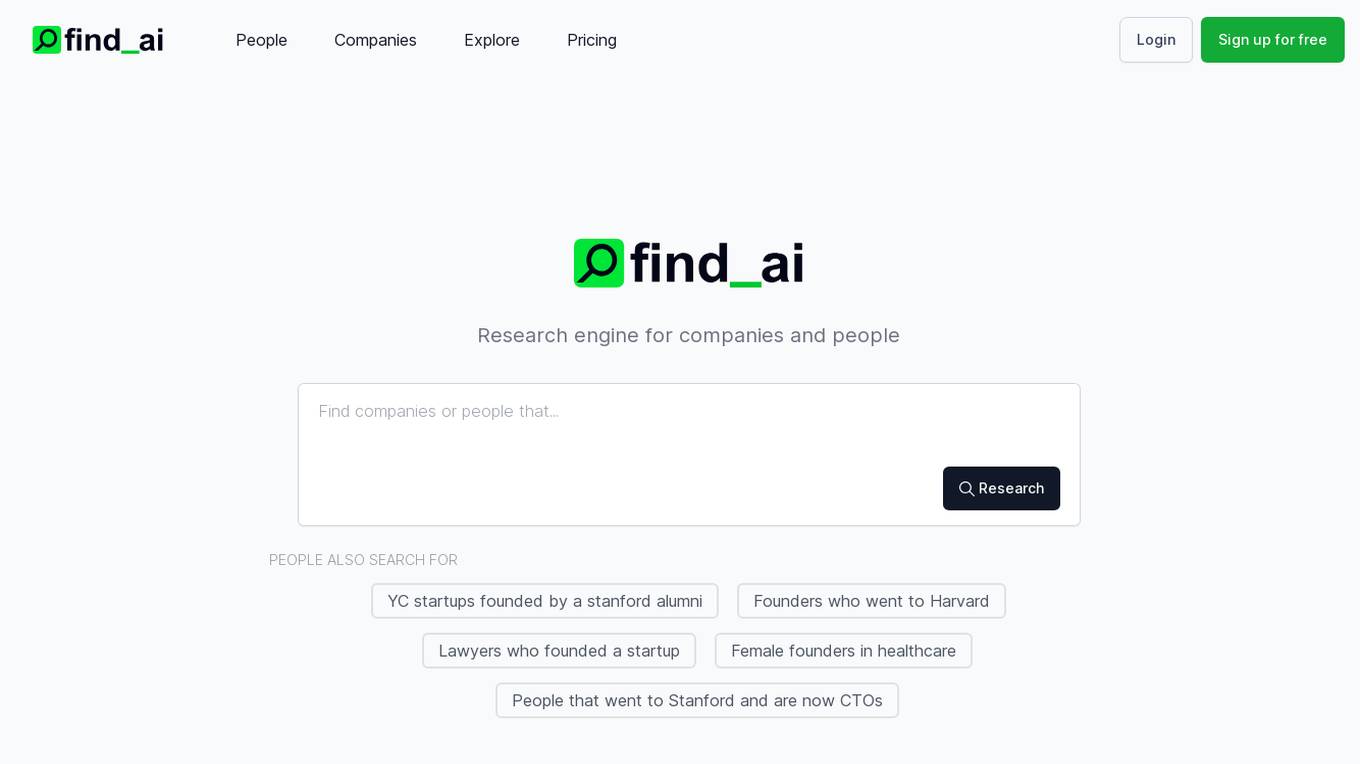
Find AI
Find AI is an AI-powered search engine that provides users with advanced search capabilities to unlock contact details and gain more accurate insights. The platform caters to individuals and companies looking to research people, companies, startups, founders, and more. Users can access email addresses and premium search features to explore a wide range of data related to various industries and sectors. Find AI offers a user-friendly interface and efficient search algorithms to deliver relevant results in a timely manner.
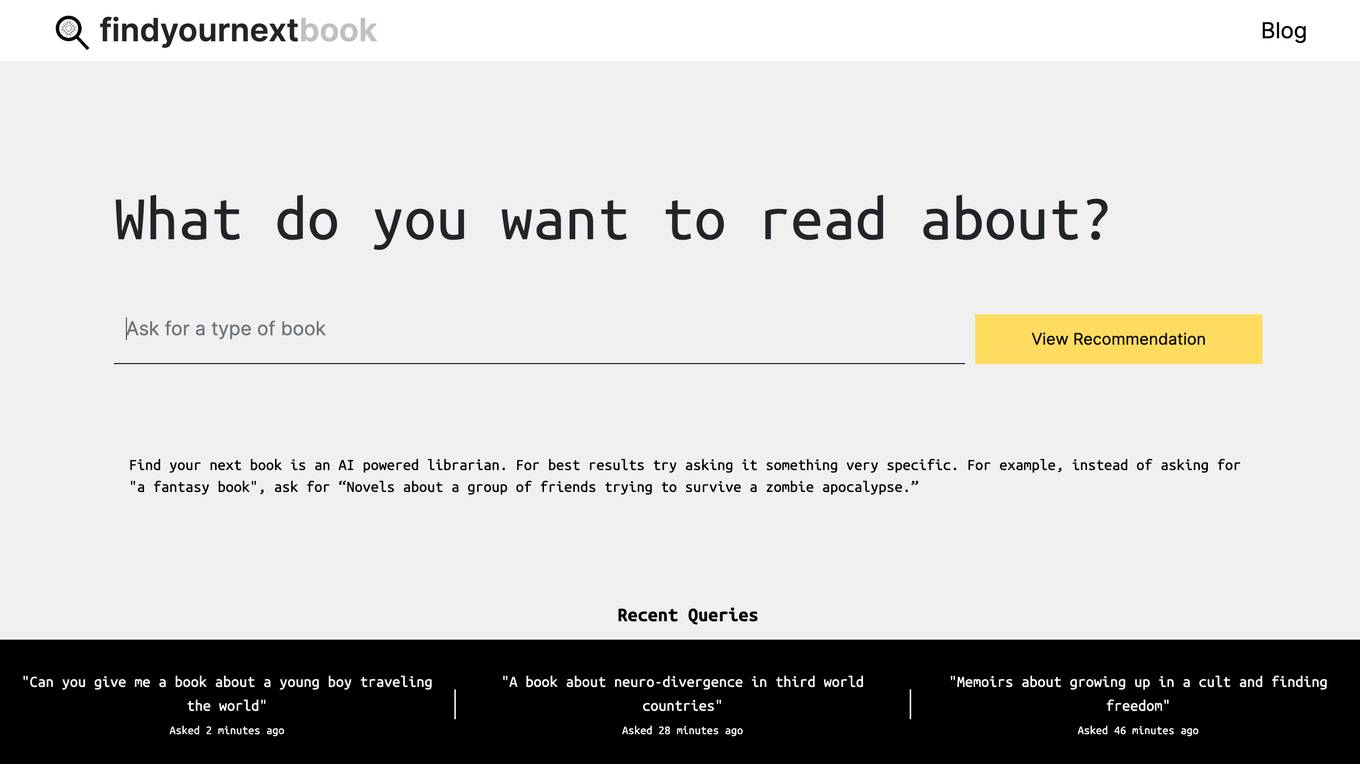
Find your next book
Find your next book is an AI-powered librarian that provides personalized book recommendations based on your preferences. It uses advanced algorithms to analyze your reading history, interests, and other factors to suggest books that you're likely to enjoy. The platform offers a wide range of genres and authors to choose from, making it easy to find your next favorite read.
20 - Open Source AI Tools
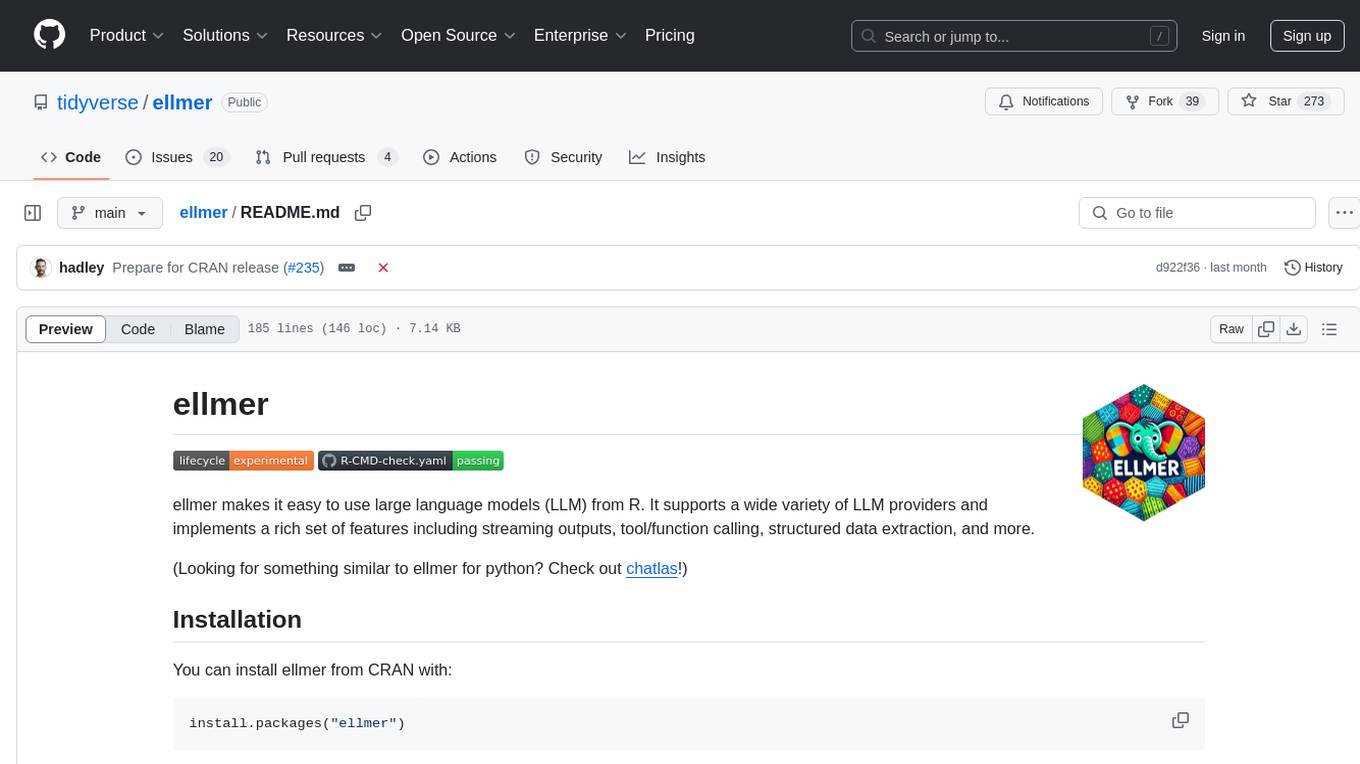
ellmer
ellmer is a tool that facilitates the use of large language models (LLM) from R. It supports various LLM providers and offers features such as streaming outputs, tool/function calling, and structured data extraction. Users can interact with ellmer in different ways, including interactive chat console, interactive method call, and programmatic chat. The tool provides support for multiple model providers and offers recommendations for different use cases, such as exploration or organizational use.
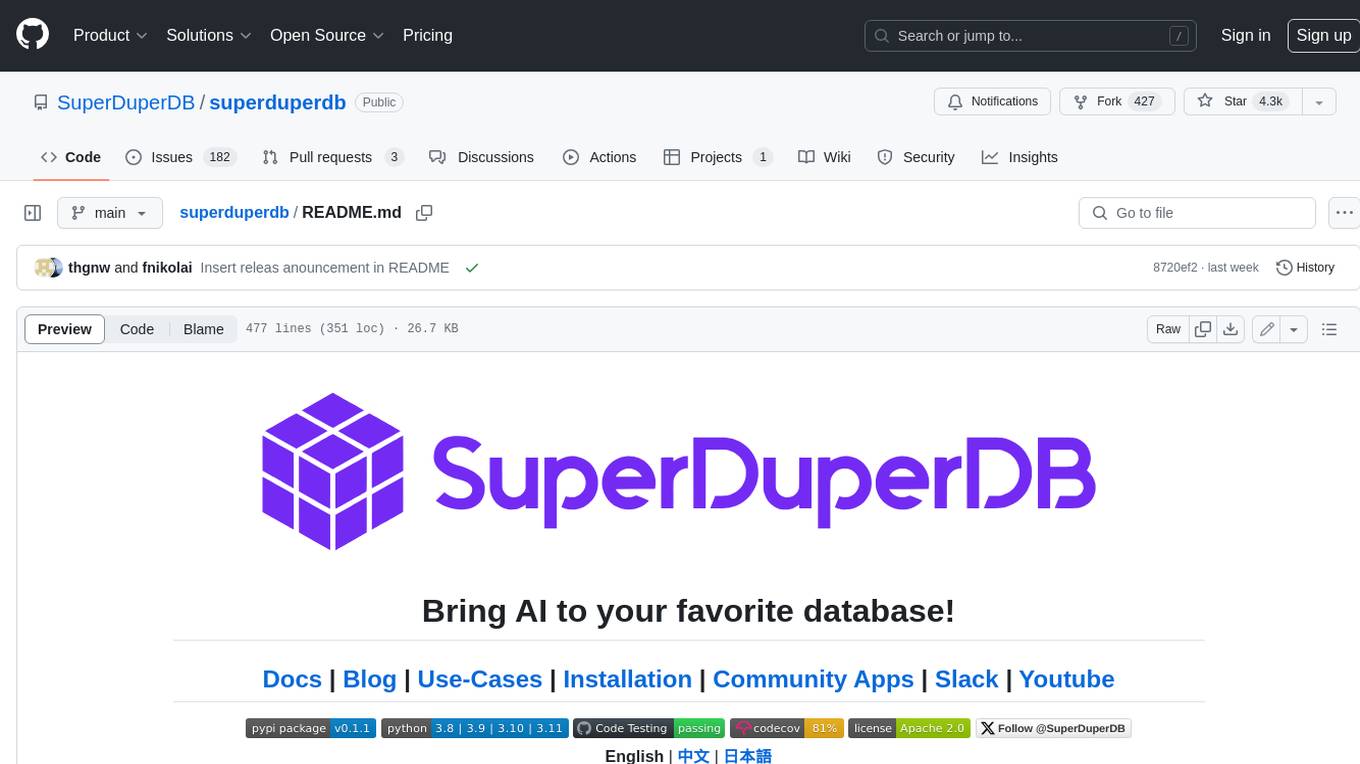
superduperdb
SuperDuperDB is a Python framework for integrating AI models, APIs, and vector search engines directly with your existing databases, including hosting of your own models, streaming inference and scalable model training/fine-tuning. Build, deploy and manage any AI application without the need for complex pipelines, infrastructure as well as specialized vector databases, and moving our data there, by integrating AI at your data's source: - Generative AI, LLMs, RAG, vector search - Standard machine learning use-cases (classification, segmentation, regression, forecasting recommendation etc.) - Custom AI use-cases involving specialized models - Even the most complex applications/workflows in which different models work together SuperDuperDB is **not** a database. Think `db = superduper(db)`: SuperDuperDB transforms your databases into an intelligent platform that allows you to leverage the full AI and Python ecosystem. A single development and deployment environment for all your AI applications in one place, fully scalable and easy to manage.
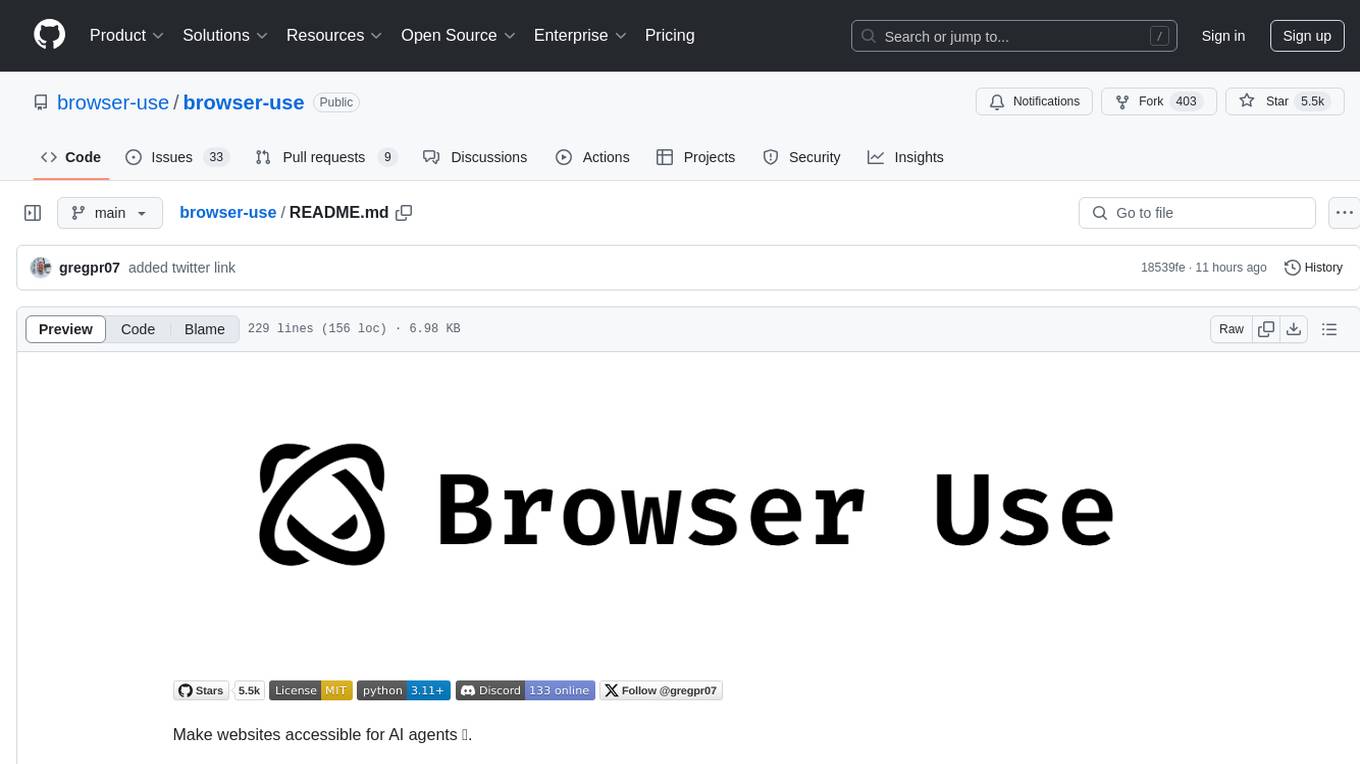
browser-use
Browser Use is a tool designed to make websites accessible for AI agents. It provides an easy way to connect AI agents with the browser, enabling users to perform tasks such as extracting vision and HTML elements, managing multiple tabs, and executing custom actions. The tool supports various language models and allows users to parallelize multiple agents for efficient processing. With features like self-correction and the ability to register custom actions, Browser Use offers a versatile solution for interacting with web content using AI technology.
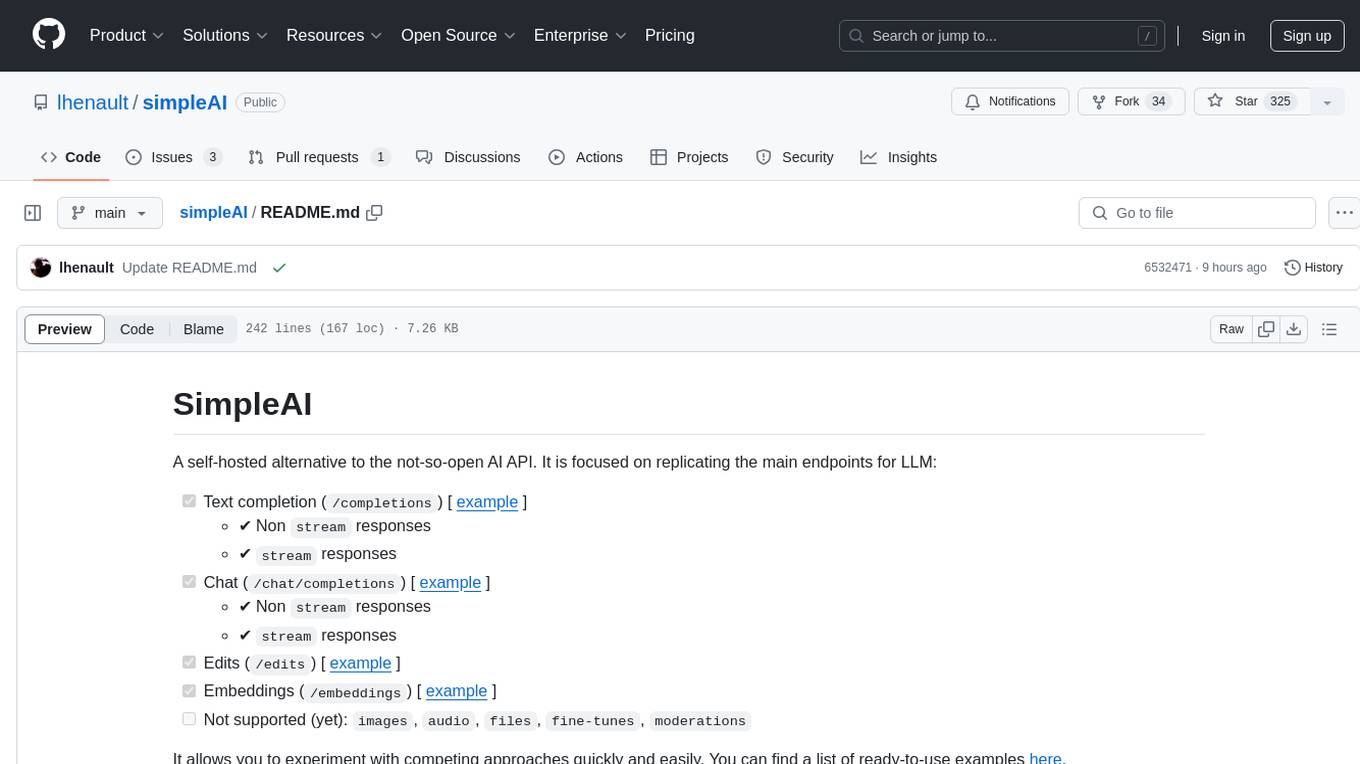
simpleAI
SimpleAI is a self-hosted alternative to the not-so-open AI API, focused on replicating main endpoints for LLM such as text completion, chat, edits, and embeddings. It allows quick experimentation with different models, creating benchmarks, and handling specific use cases without relying on external services. Users can integrate and declare models through gRPC, query endpoints using Swagger UI or API, and resolve common issues like CORS with FastAPI middleware. The project is open for contributions and welcomes PRs, issues, documentation, and more.
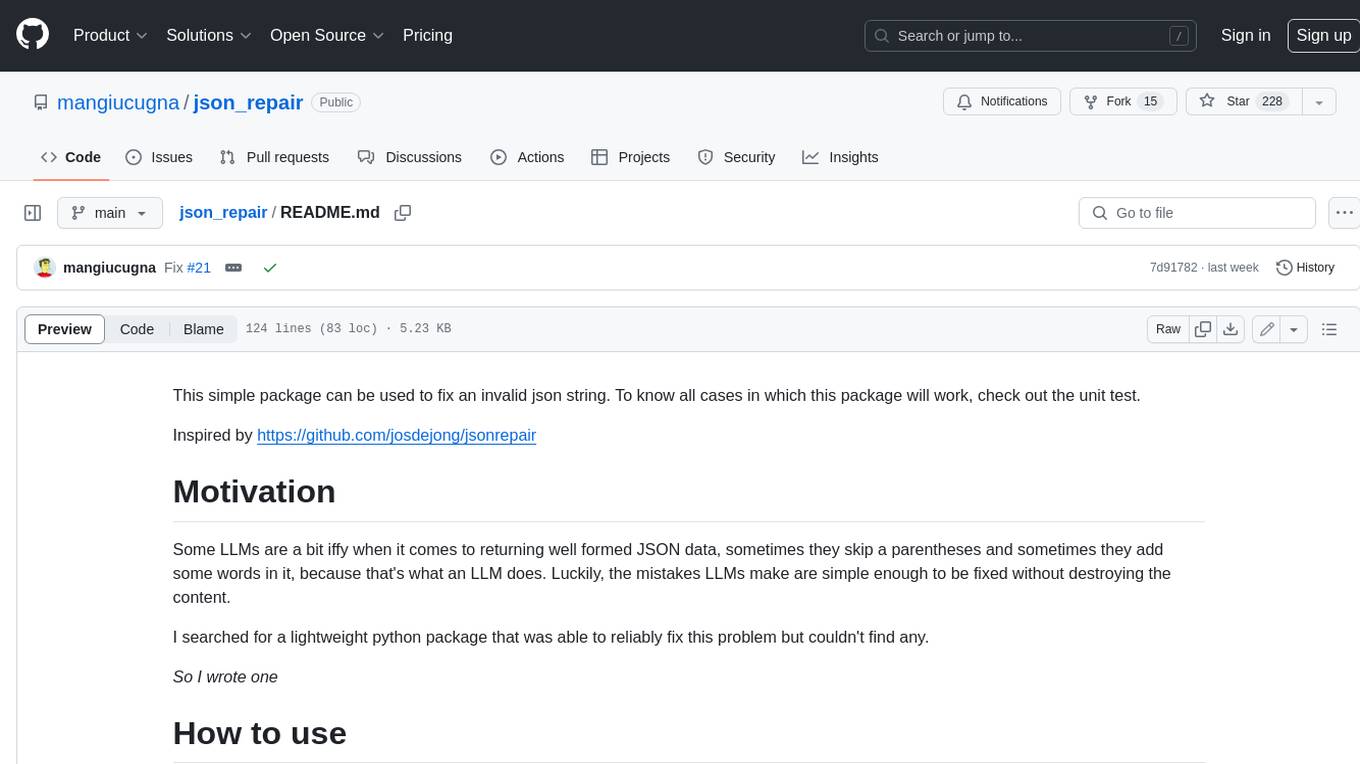
json_repair
This simple package can be used to fix an invalid json string. To know all cases in which this package will work, check out the unit test. Inspired by https://github.com/josdejong/jsonrepair Motivation Some LLMs are a bit iffy when it comes to returning well formed JSON data, sometimes they skip a parentheses and sometimes they add some words in it, because that's what an LLM does. Luckily, the mistakes LLMs make are simple enough to be fixed without destroying the content. I searched for a lightweight python package that was able to reliably fix this problem but couldn't find any. So I wrote one How to use from json_repair import repair_json good_json_string = repair_json(bad_json_string) # If the string was super broken this will return an empty string You can use this library to completely replace `json.loads()`: import json_repair decoded_object = json_repair.loads(json_string) or just import json_repair decoded_object = json_repair.repair_json(json_string, return_objects=True) Read json from a file or file descriptor JSON repair provides also a drop-in replacement for `json.load()`: import json_repair try: file_descriptor = open(fname, 'rb') except OSError: ... with file_descriptor: decoded_object = json_repair.load(file_descriptor) and another method to read from a file: import json_repair try: decoded_object = json_repair.from_file(json_file) except OSError: ... except IOError: ... Keep in mind that the library will not catch any IO-related exception and those will need to be managed by you Performance considerations If you find this library too slow because is using `json.loads()` you can skip that by passing `skip_json_loads=True` to `repair_json`. Like: from json_repair import repair_json good_json_string = repair_json(bad_json_string, skip_json_loads=True) I made a choice of not using any fast json library to avoid having any external dependency, so that anybody can use it regardless of their stack. Some rules of thumb to use: - Setting `return_objects=True` will always be faster because the parser returns an object already and it doesn't have serialize that object to JSON - `skip_json_loads` is faster only if you 100% know that the string is not a valid JSON - If you are having issues with escaping pass the string as **raw** string like: `r"string with escaping\"" Adding to requirements Please pin this library only on the major version! We use TDD and strict semantic versioning, there will be frequent updates and no breaking changes in minor and patch versions. To ensure that you only pin the major version of this library in your `requirements.txt`, specify the package name followed by the major version and a wildcard for minor and patch versions. For example: json_repair==0.* In this example, any version that starts with `0.` will be acceptable, allowing for updates on minor and patch versions. How it works This module will parse the JSON file following the BNF definition:
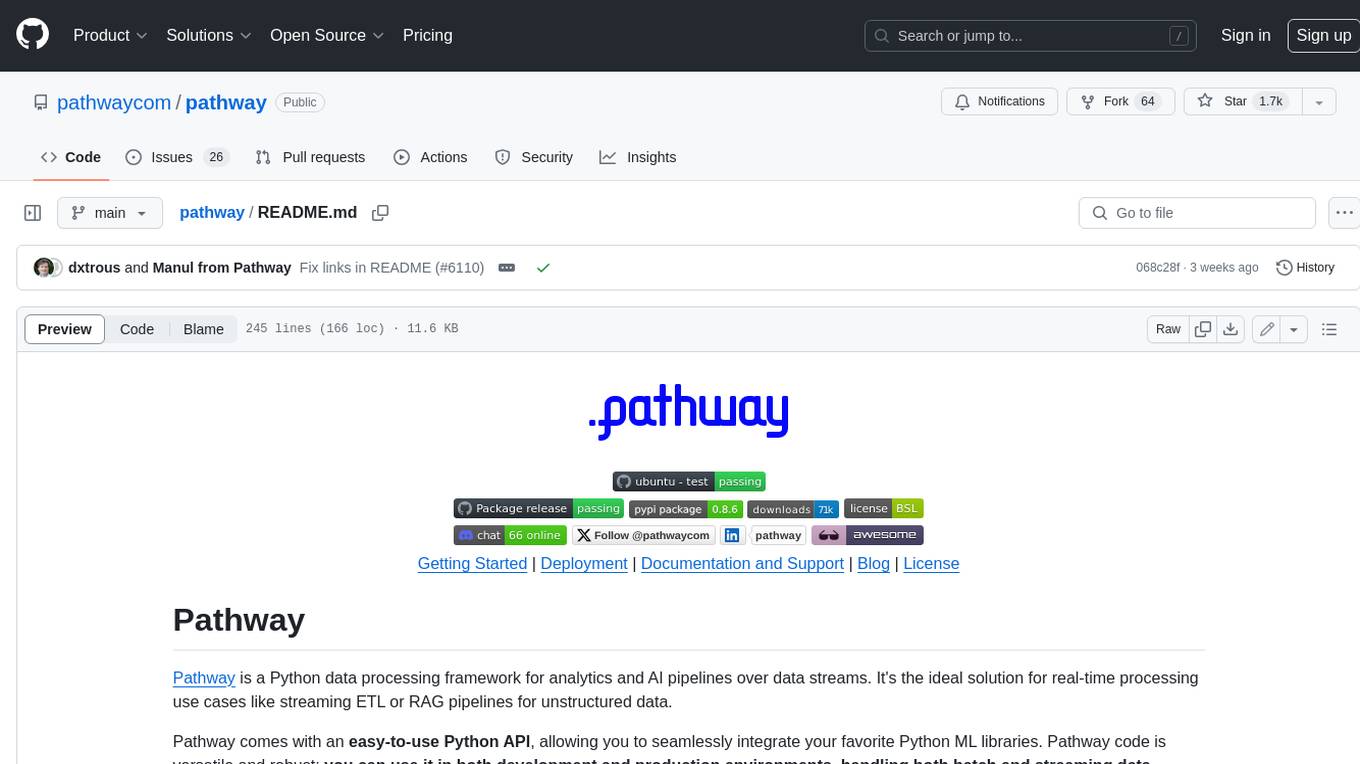
pathway
Pathway is a Python data processing framework for analytics and AI pipelines over data streams. It's the ideal solution for real-time processing use cases like streaming ETL or RAG pipelines for unstructured data. Pathway comes with an **easy-to-use Python API** , allowing you to seamlessly integrate your favorite Python ML libraries. Pathway code is versatile and robust: **you can use it in both development and production environments, handling both batch and streaming data effectively**. The same code can be used for local development, CI/CD tests, running batch jobs, handling stream replays, and processing data streams. Pathway is powered by a **scalable Rust engine** based on Differential Dataflow and performs incremental computation. Your Pathway code, despite being written in Python, is run by the Rust engine, enabling multithreading, multiprocessing, and distributed computations. All the pipeline is kept in memory and can be easily deployed with **Docker and Kubernetes**. You can install Pathway with pip: `pip install -U pathway` For any questions, you will find the community and team behind the project on Discord.
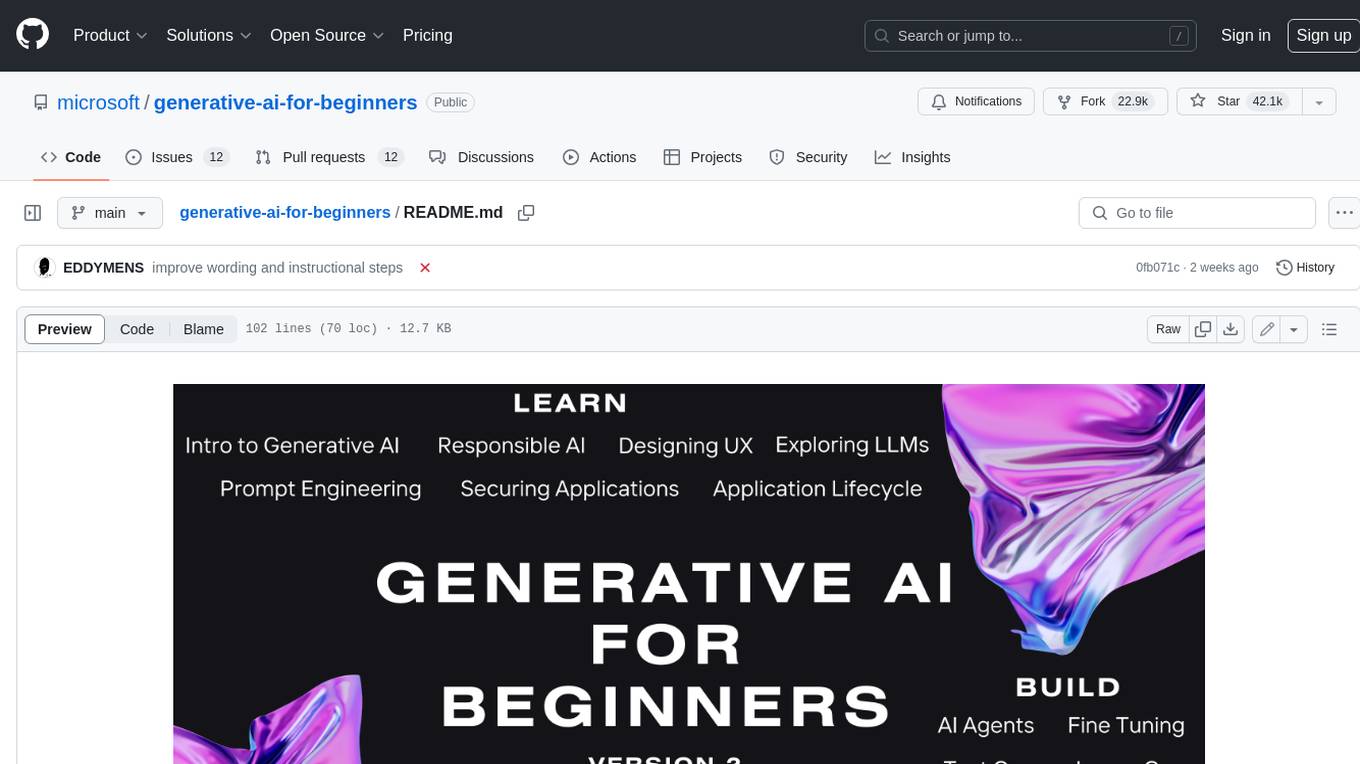
generative-ai-for-beginners
This course has 18 lessons. Each lesson covers its own topic so start wherever you like! Lessons are labeled either "Learn" lessons explaining a Generative AI concept or "Build" lessons that explain a concept and code examples in both **Python** and **TypeScript** when possible. Each lesson also includes a "Keep Learning" section with additional learning tools. **What You Need** * Access to the Azure OpenAI Service **OR** OpenAI API - _Only required to complete coding lessons_ * Basic knowledge of Python or Typescript is helpful - *For absolute beginners check out these Python and TypeScript courses. * A Github account to fork this entire repo to your own GitHub account We have created a **Course Setup** lesson to help you with setting up your development environment. Don't forget to star (🌟) this repo to find it easier later. ## 🧠 Ready to Deploy? If you are looking for more advanced code samples, check out our collection of Generative AI Code Samples in both **Python** and **TypeScript**. ## 🗣️ Meet Other Learners, Get Support Join our official AI Discord server to meet and network with other learners taking this course and get support. ## 🚀 Building a Startup? Sign up for Microsoft for Startups Founders Hub to receive **free OpenAI credits** and up to **$150k towards Azure credits to access OpenAI models through Azure OpenAI Services**. ## 🙏 Want to help? Do you have suggestions or found spelling or code errors? Raise an issue or Create a pull request ## 📂 Each lesson includes: * A short video introduction to the topic * A written lesson located in the README * Python and TypeScript code samples supporting Azure OpenAI and OpenAI API * Links to extra resources to continue your learning ## 🗃️ Lessons | | Lesson Link | Description | Additional Learning | | :-: | :------------------------------------------------------------------------------------------------------------------------------------------: | :---------------------------------------------------------------------------------------------: | ------------------------------------------------------------------------------ | | 00 | Course Setup | **Learn:** How to Setup Your Development Environment | Learn More | | 01 | Introduction to Generative AI and LLMs | **Learn:** Understanding what Generative AI is and how Large Language Models (LLMs) work. | Learn More | | 02 | Exploring and comparing different LLMs | **Learn:** How to select the right model for your use case | Learn More | | 03 | Using Generative AI Responsibly | **Learn:** How to build Generative AI Applications responsibly | Learn More | | 04 | Understanding Prompt Engineering Fundamentals | **Learn:** Hands-on Prompt Engineering Best Practices | Learn More | | 05 | Creating Advanced Prompts | **Learn:** How to apply prompt engineering techniques that improve the outcome of your prompts. | Learn More | | 06 | Building Text Generation Applications | **Build:** A text generation app using Azure OpenAI | Learn More | | 07 | Building Chat Applications | **Build:** Techniques for efficiently building and integrating chat applications. | Learn More | | 08 | Building Search Apps Vector Databases | **Build:** A search application that uses Embeddings to search for data. | Learn More | | 09 | Building Image Generation Applications | **Build:** A image generation application | Learn More | | 10 | Building Low Code AI Applications | **Build:** A Generative AI application using Low Code tools | Learn More | | 11 | Integrating External Applications with Function Calling | **Build:** What is function calling and its use cases for applications | Learn More | | 12 | Designing UX for AI Applications | **Learn:** How to apply UX design principles when developing Generative AI Applications | Learn More | | 13 | Securing Your Generative AI Applications | **Learn:** The threats and risks to AI systems and methods to secure these systems. | Learn More | | 14 | The Generative AI Application Lifecycle | **Learn:** The tools and metrics to manage the LLM Lifecycle and LLMOps | Learn More | | 15 | Retrieval Augmented Generation (RAG) and Vector Databases | **Build:** An application using a RAG Framework to retrieve embeddings from a Vector Databases | Learn More | | 16 | Open Source Models and Hugging Face | **Build:** An application using open source models available on Hugging Face | Learn More | | 17 | AI Agents | **Build:** An application using an AI Agent Framework | Learn More | | 18 | Fine-Tuning LLMs | **Learn:** The what, why and how of fine-tuning LLMs | Learn More |
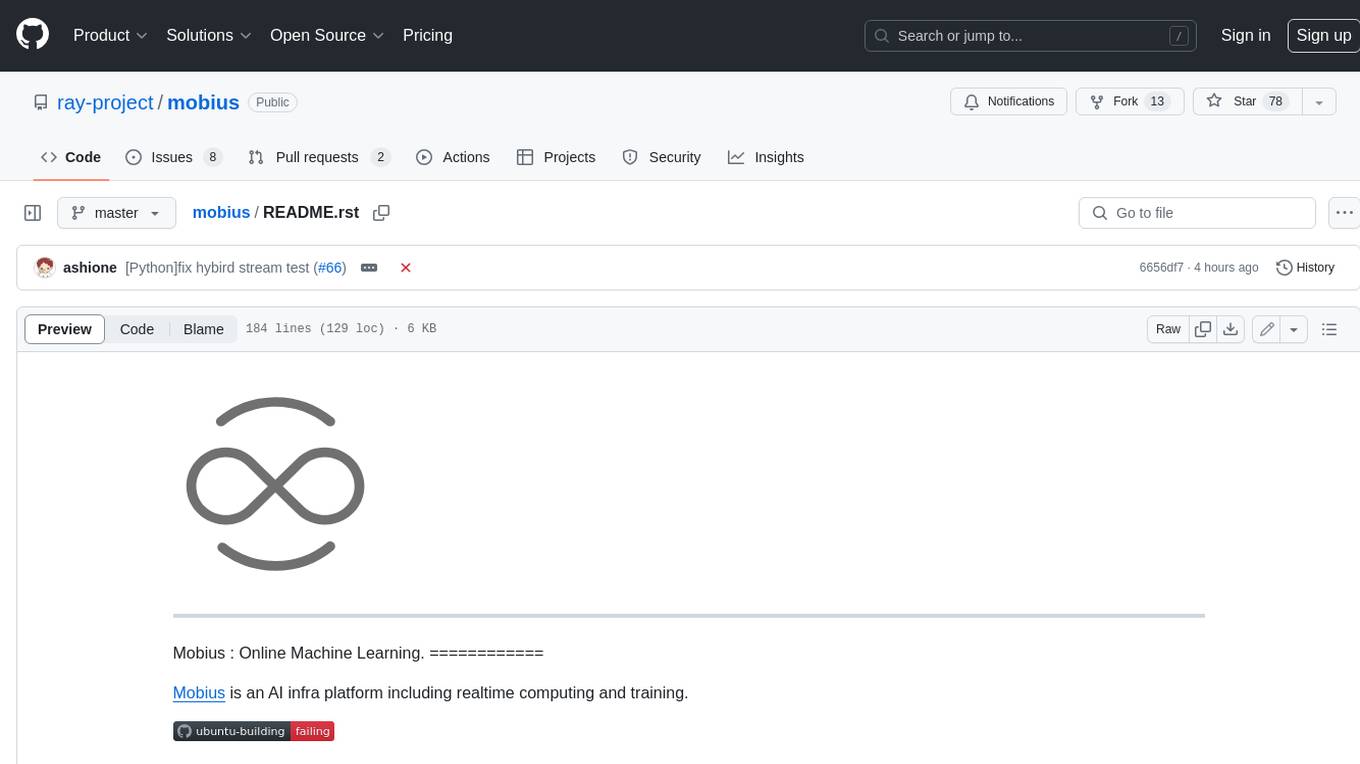
mobius
Mobius is an AI infra platform including realtime computing and training. It is built on Ray, a distributed computing framework, and provides a number of features that make it well-suited for online machine learning tasks. These features include: * **Cross Language**: Mobius can run in multiple languages (only Python and Java are supported currently) with high efficiency. You can implement your operator in different languages and run them in one job. * **Single Node Failover**: Mobius has a special failover mechanism that only needs to rollback the failed node itself, in most cases, to recover the job. This is a huge benefit if your job is sensitive about failure recovery time. * **AutoScaling**: Mobius can generate a new graph with different configurations in runtime without stopping the job. * **Fusion Training**: Mobius can combine TensorFlow/Pytorch and streaming, then building an e2e online machine learning pipeline. Mobius is still under development, but it has already been used to power a number of real-world applications, including: * A real-time recommendation system for a major e-commerce company * A fraud detection system for a large financial institution * A personalized news feed for a major news organization If you are interested in using Mobius for your own online machine learning projects, you can find more information in the documentation.
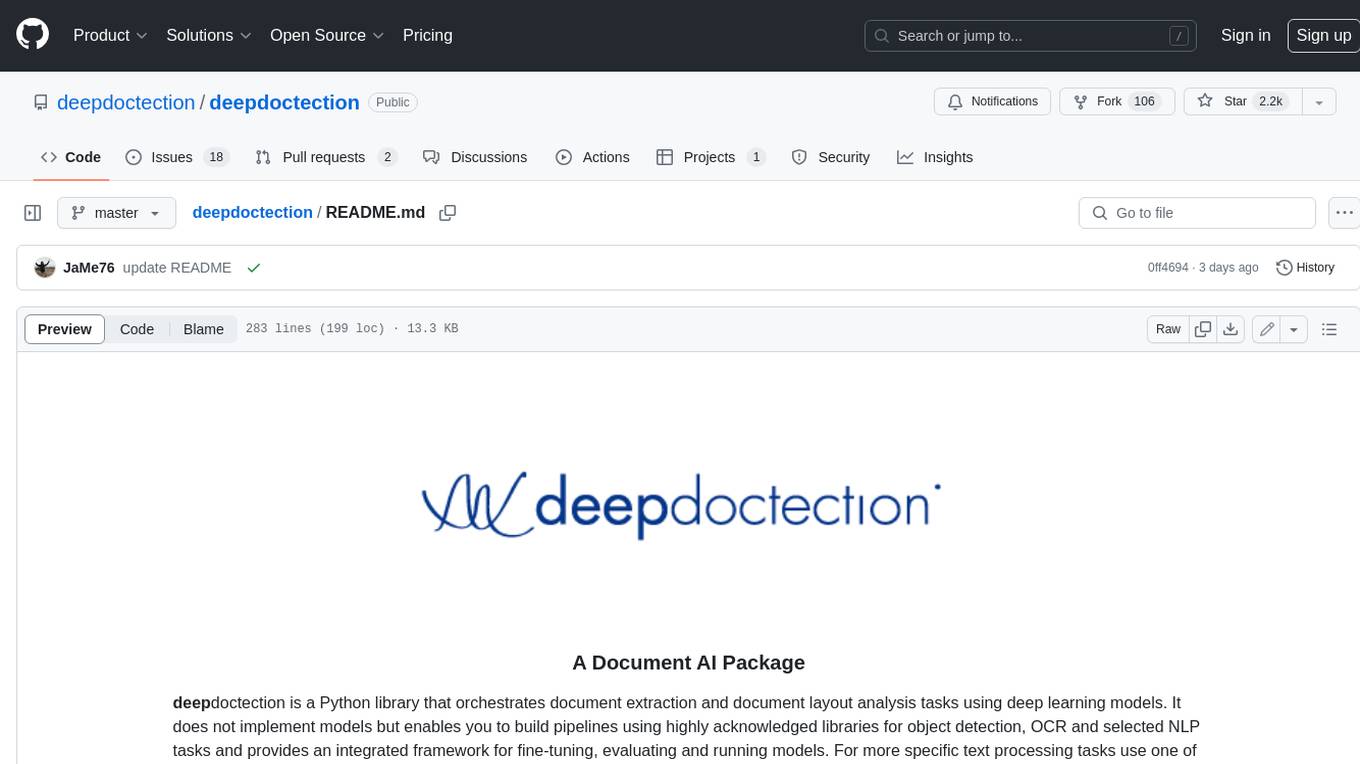
deepdoctection
**deep** doctection is a Python library that orchestrates document extraction and document layout analysis tasks using deep learning models. It does not implement models but enables you to build pipelines using highly acknowledged libraries for object detection, OCR and selected NLP tasks and provides an integrated framework for fine-tuning, evaluating and running models. For more specific text processing tasks use one of the many other great NLP libraries. **deep** doctection focuses on applications and is made for those who want to solve real world problems related to document extraction from PDFs or scans in various image formats. **deep** doctection provides model wrappers of supported libraries for various tasks to be integrated into pipelines. Its core function does not depend on any specific deep learning library. Selected models for the following tasks are currently supported: * Document layout analysis including table recognition in Tensorflow with **Tensorpack**, or PyTorch with **Detectron2**, * OCR with support of **Tesseract**, **DocTr** (Tensorflow and PyTorch implementations available) and a wrapper to an API for a commercial solution, * Text mining for native PDFs with **pdfplumber**, * Language detection with **fastText**, * Deskewing and rotating images with **jdeskew**. * Document and token classification with all LayoutLM models provided by the **Transformer library**. (Yes, you can use any LayoutLM-model with any of the provided OCR-or pdfplumber tools straight away!). * Table detection and table structure recognition with **table-transformer**. * There is a small dataset for token classification available and a lot of new tutorials to show, how to train and evaluate this dataset using LayoutLMv1, LayoutLMv2, LayoutXLM and LayoutLMv3. * Comprehensive configuration of **analyzer** like choosing different models, output parsing, OCR selection. Check this notebook or the docs for more infos. * Document layout analysis and table recognition now runs with **Torchscript** (CPU) as well and **Detectron2** is not required anymore for basic inference. * [**new**] More angle predictors for determining the rotation of a document based on **Tesseract** and **DocTr** (not contained in the built-in Analyzer). * [**new**] Token classification with **LiLT** via **transformers**. We have added a model wrapper for token classification with LiLT and added a some LiLT models to the model catalog that seem to look promising, especially if you want to train a model on non-english data. The training script for LayoutLM can be used for LiLT as well and we will be providing a notebook on how to train a model on a custom dataset soon. **deep** doctection provides on top of that methods for pre-processing inputs to models like cropping or resizing and to post-process results, like validating duplicate outputs, relating words to detected layout segments or ordering words into contiguous text. You will get an output in JSON format that you can customize even further by yourself. Have a look at the **introduction notebook** in the notebook repo for an easy start. Check the **release notes** for recent updates. **deep** doctection or its support libraries provide pre-trained models that are in most of the cases available at the **Hugging Face Model Hub** or that will be automatically downloaded once requested. For instance, you can find pre-trained object detection models from the Tensorpack or Detectron2 framework for coarse layout analysis, table cell detection and table recognition. Training is a substantial part to get pipelines ready on some specific domain, let it be document layout analysis, document classification or NER. **deep** doctection provides training scripts for models that are based on trainers developed from the library that hosts the model code. Moreover, **deep** doctection hosts code to some well established datasets like **Publaynet** that makes it easy to experiment. It also contains mappings from widely used data formats like COCO and it has a dataset framework (akin to **datasets** so that setting up training on a custom dataset becomes very easy. **This notebook** shows you how to do this. **deep** doctection comes equipped with a framework that allows you to evaluate predictions of a single or multiple models in a pipeline against some ground truth. Check again **here** how it is done. Having set up a pipeline it takes you a few lines of code to instantiate the pipeline and after a for loop all pages will be processed through the pipeline.
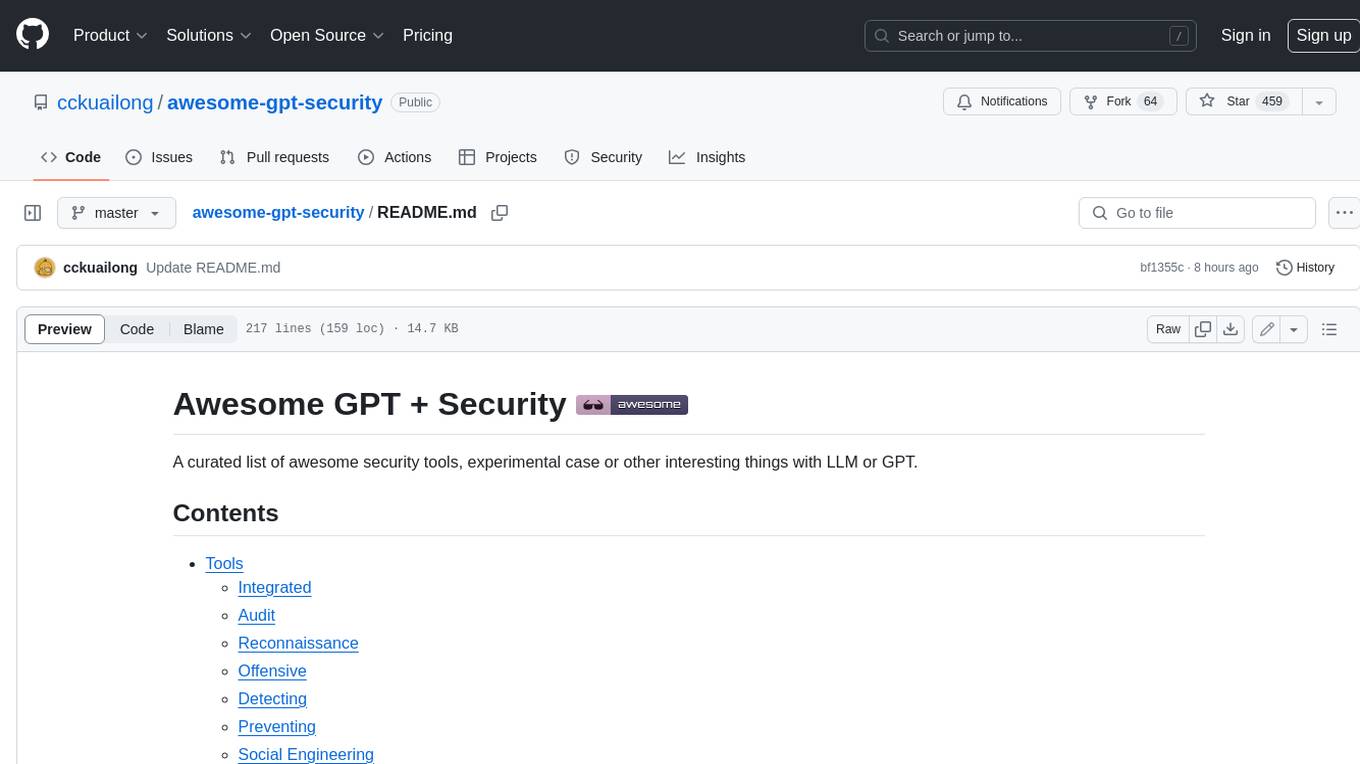
awesome-gpt-security
Awesome GPT + Security is a curated list of awesome security tools, experimental case or other interesting things with LLM or GPT. It includes tools for integrated security, auditing, reconnaissance, offensive security, detecting security issues, preventing security breaches, social engineering, reverse engineering, investigating security incidents, fixing security vulnerabilities, assessing security posture, and more. The list also includes experimental cases, academic research, blogs, and fun projects related to GPT security. Additionally, it provides resources on GPT security standards, bypassing security policies, bug bounty programs, cracking GPT APIs, and plugin security.
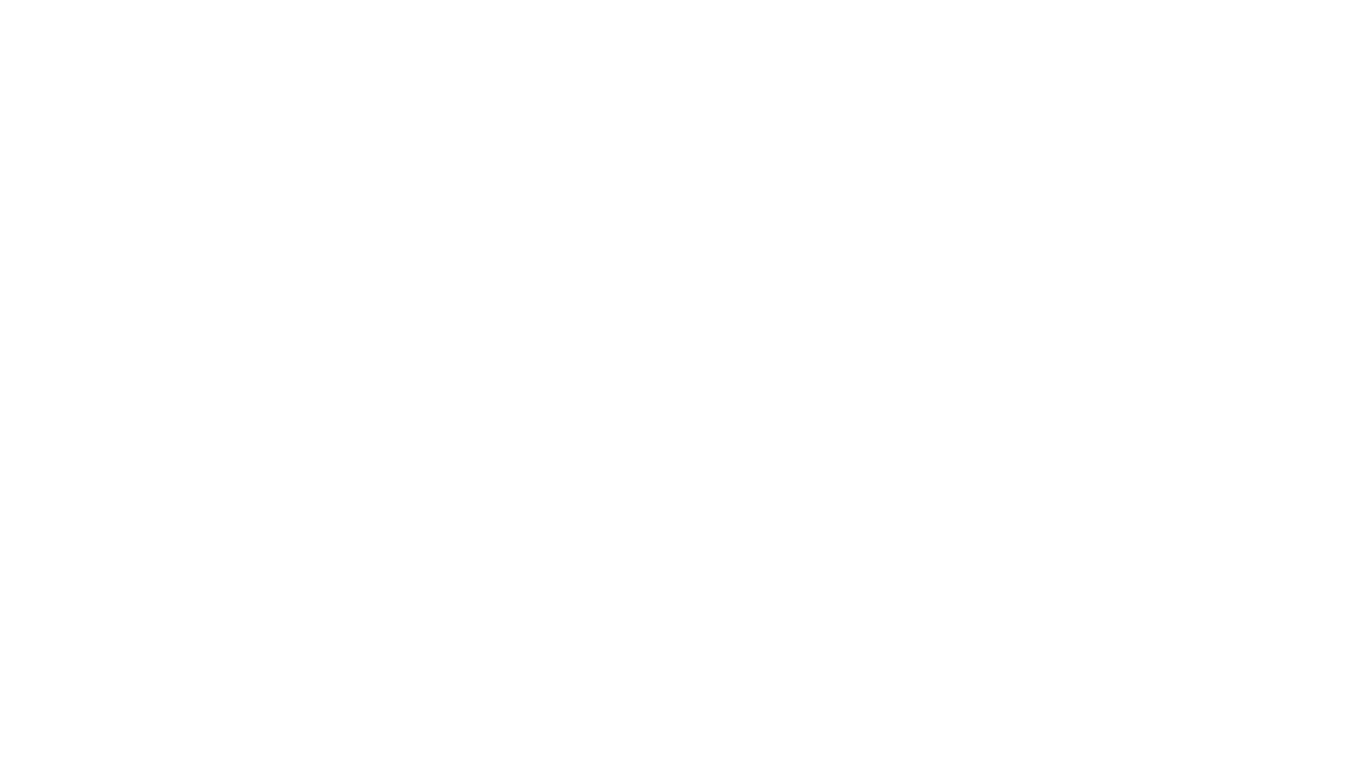
upgini
Upgini is an intelligent data search engine with a Python library that helps users find and add relevant features to their ML pipeline from various public, community, and premium external data sources. It automates the optimization of connected data sources by generating an optimal set of machine learning features using large language models, GraphNNs, and recurrent neural networks. The tool aims to simplify feature search and enrichment for external data to make it a standard approach in machine learning pipelines. It democratizes access to data sources for the data science community.
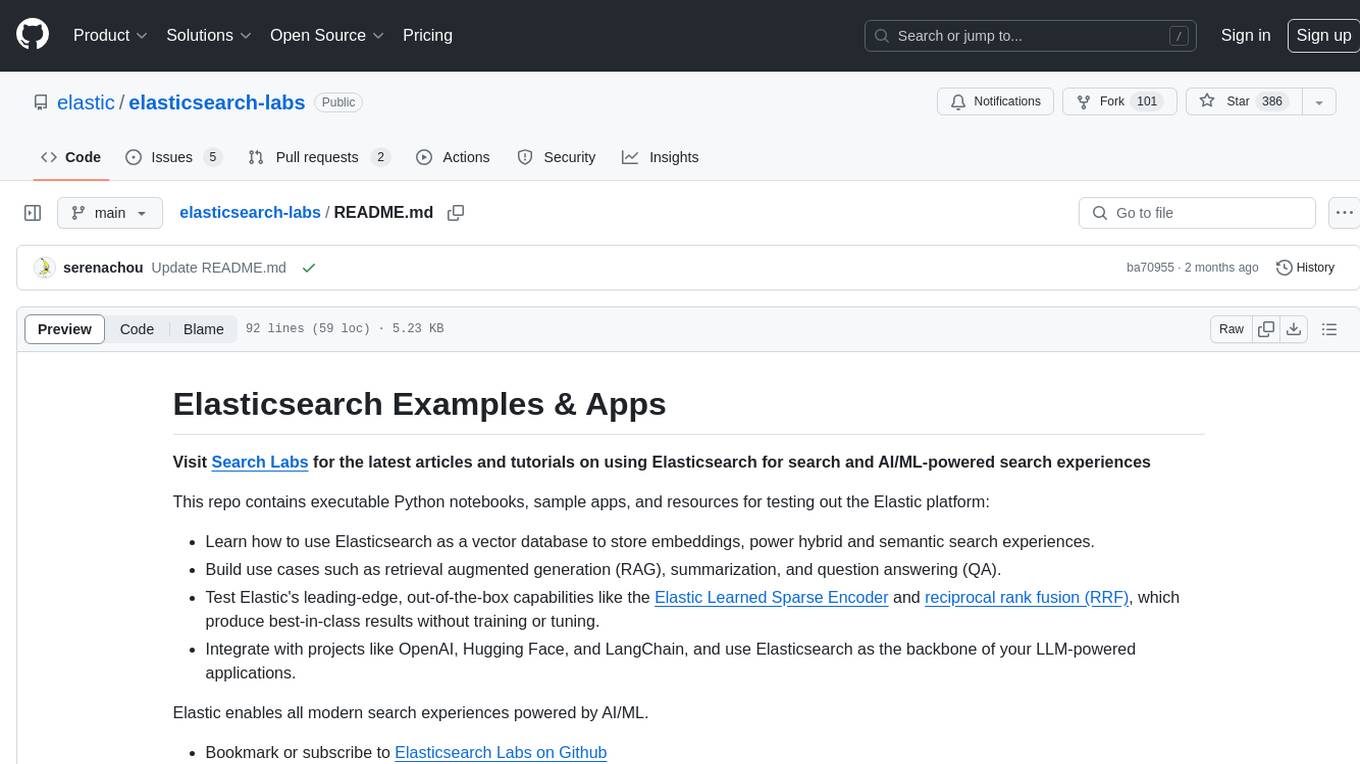
elasticsearch-labs
This repository contains executable Python notebooks, sample apps, and resources for testing out the Elastic platform. Users can learn how to use Elasticsearch as a vector database for storing embeddings, build use cases like retrieval augmented generation (RAG), summarization, and question answering (QA), and test Elastic's leading-edge capabilities like the Elastic Learned Sparse Encoder and reciprocal rank fusion (RRF). It also allows integration with projects like OpenAI, Hugging Face, and LangChain to power LLM-powered applications. The repository enables modern search experiences powered by AI/ML.
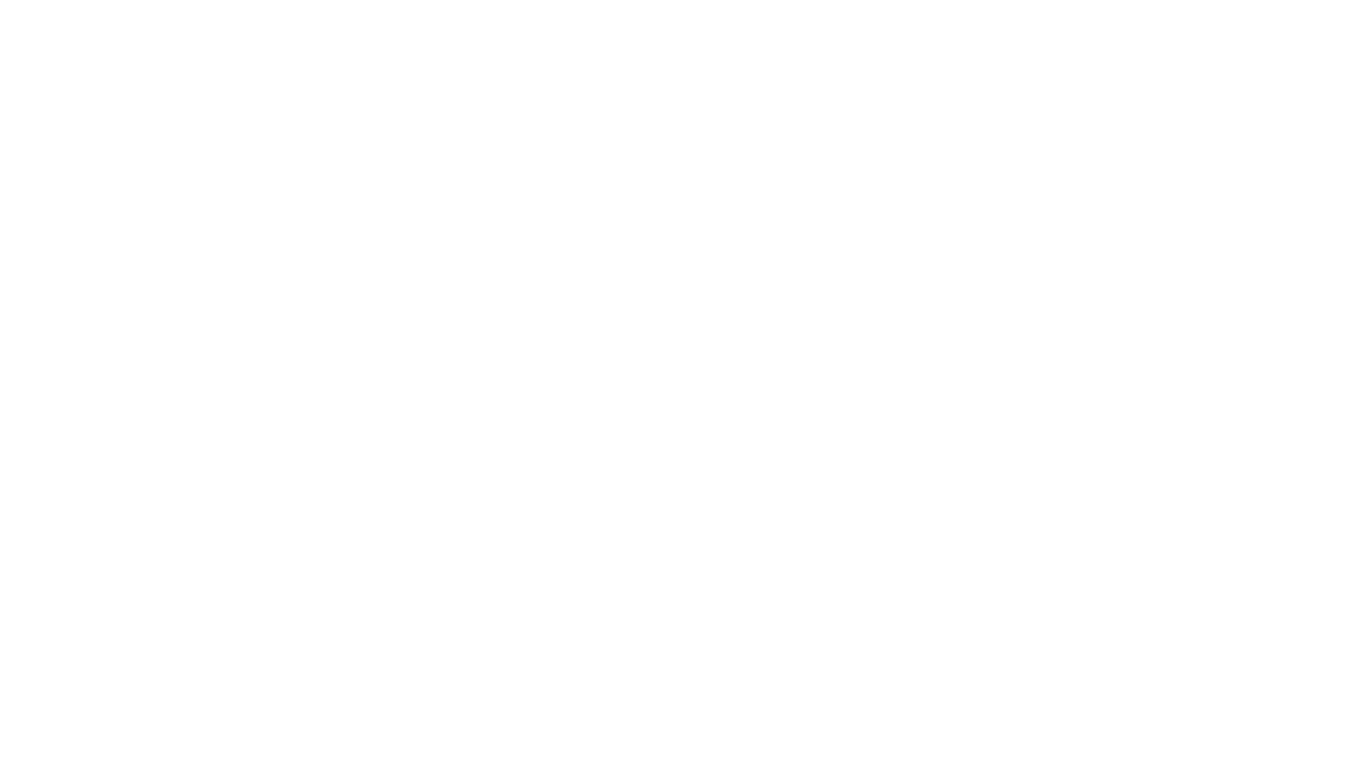
EvoMaster
EvoMaster is an open-source AI-driven tool that automatically generates system-level test cases for web/enterprise applications. It uses Evolutionary Algorithm and Dynamic Program Analysis to evolve test cases, maximizing code coverage and fault detection. It supports REST, GraphQL, and RPC APIs, with whitebox testing for JVM-compiled APIs. The tool generates JUnit tests in Java or Kotlin, focusing on fault detection, self-contained tests, SQL handling, and authentication. Known limitations include manual driver creation for whitebox testing and longer execution times for better results. EvoMaster has been funded by ERC and RCN grants.
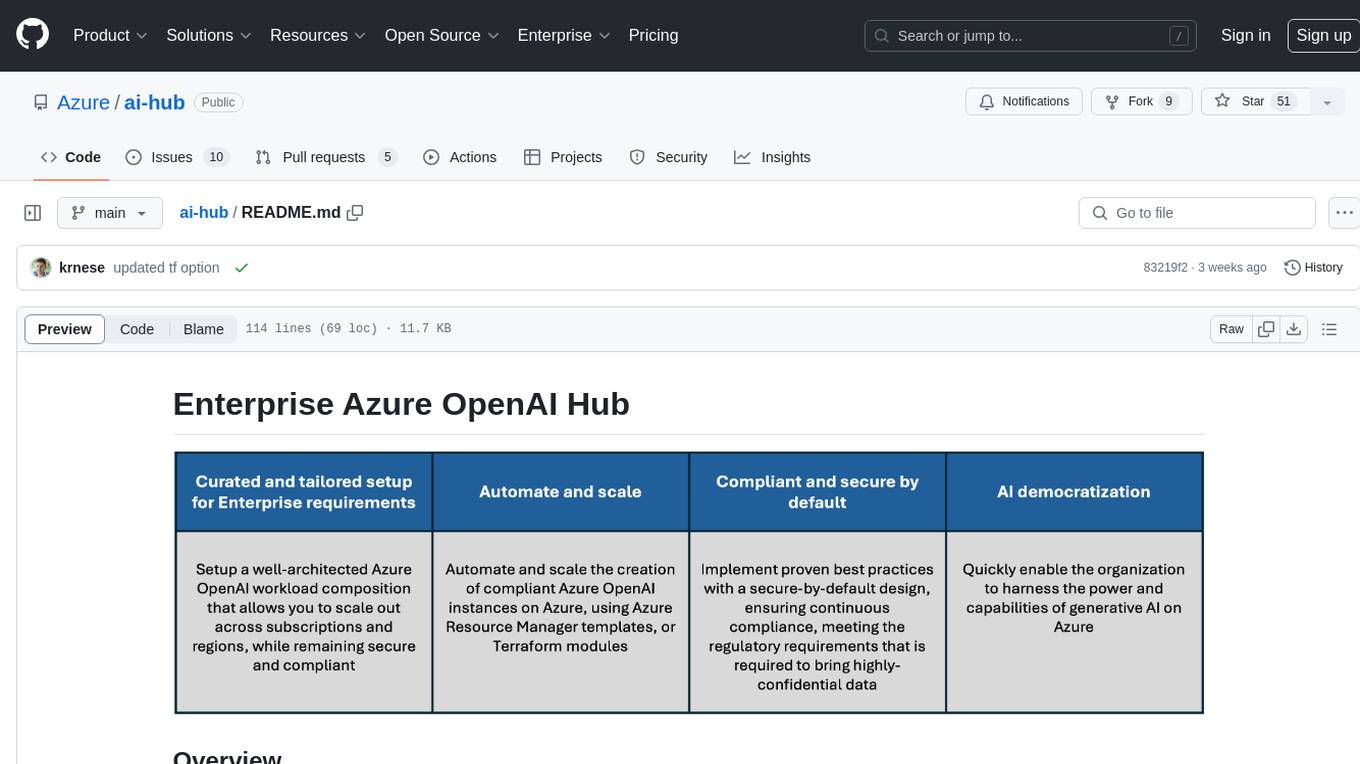
ai-hub
The Enterprise Azure OpenAI Hub is a comprehensive repository designed to guide users through the world of Generative AI on the Azure platform. It offers a structured learning experience to accelerate the transition from concept to production in an Enterprise context. The hub empowers users to explore various use cases with Azure services, ensuring security and compliance. It provides real-world examples and playbooks for practical insights into solving complex problems and developing cutting-edge AI solutions. The repository also serves as a library of proven patterns, aligning with industry standards and promoting best practices for secure and compliant AI development.
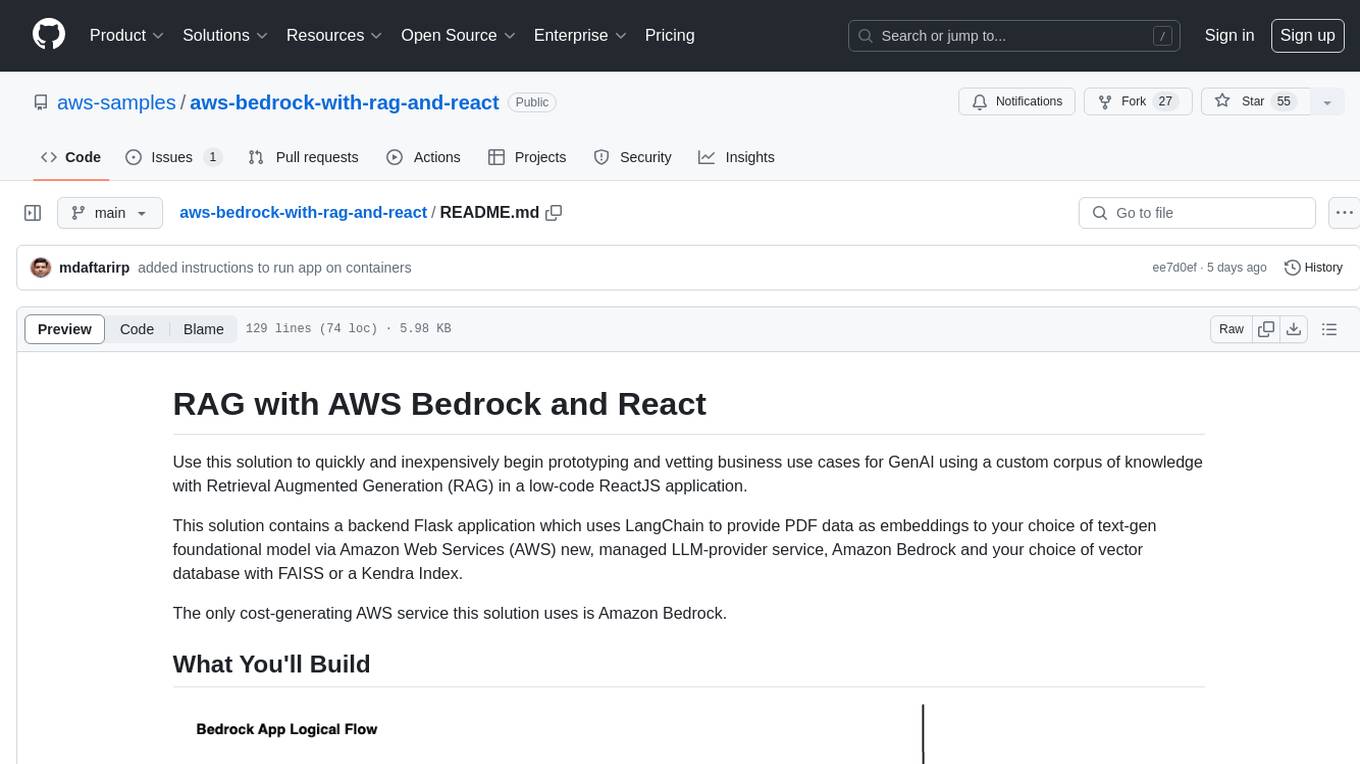
aws-bedrock-with-rag-and-react
This solution provides a low-code ReactJS application to prototype and vet business use cases for GenAI using Retrieval Augmented Generation (RAG). It includes a backend Flask application that uses LangChain to provide PDF data as embeddings to a text-gen model via Amazon Bedrock and a vector database with FAISS or Kendra Index. The solution utilizes Amazon Bedrock as the only cost-generating AWS service.
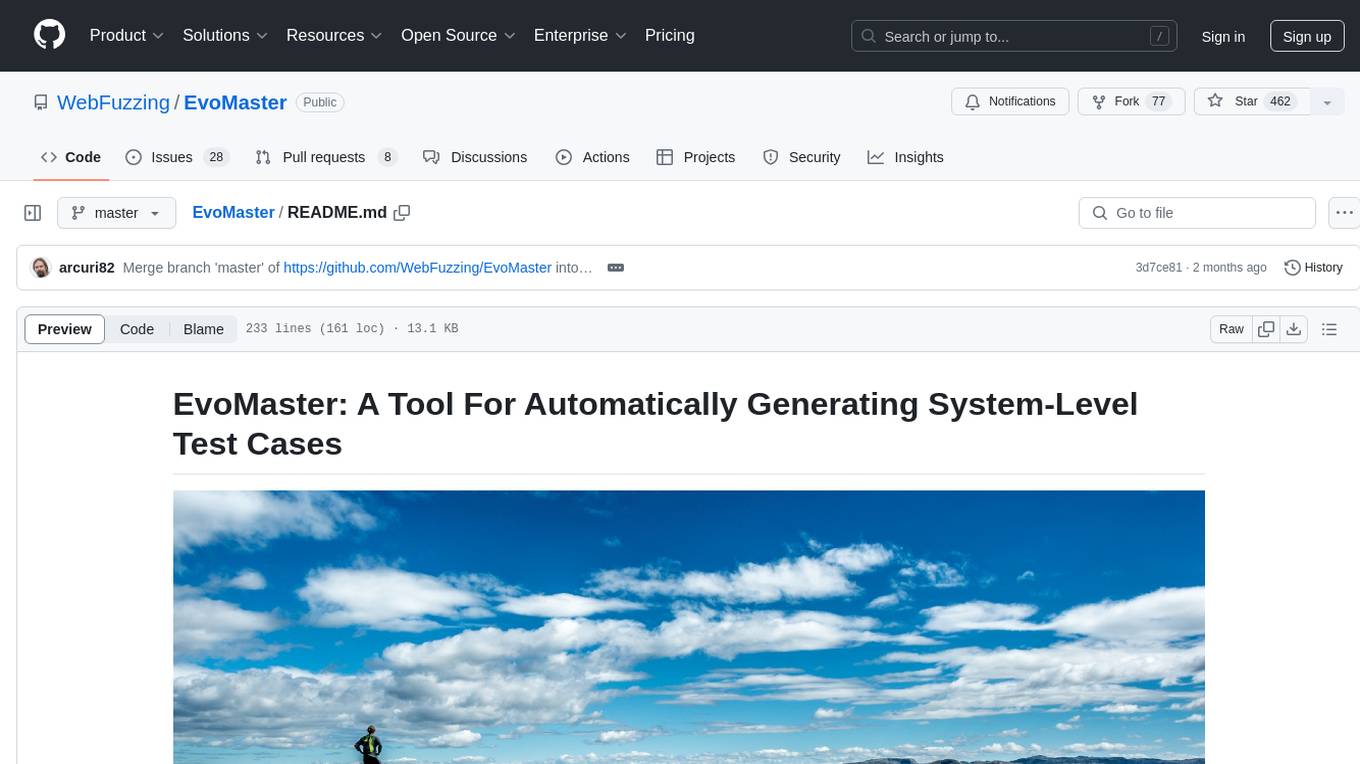
EvoMaster
EvoMaster is an open-source AI-driven tool that automatically generates system-level test cases for web/enterprise applications. It uses an Evolutionary Algorithm and Dynamic Program Analysis to evolve test cases, maximizing code coverage and fault detection. The tool supports REST, GraphQL, and RPC APIs, with whitebox testing for JVM-compiled languages. It generates JUnit tests, detects faults, handles SQL databases, and supports authentication. EvoMaster has been funded by the European Research Council and the Research Council of Norway.
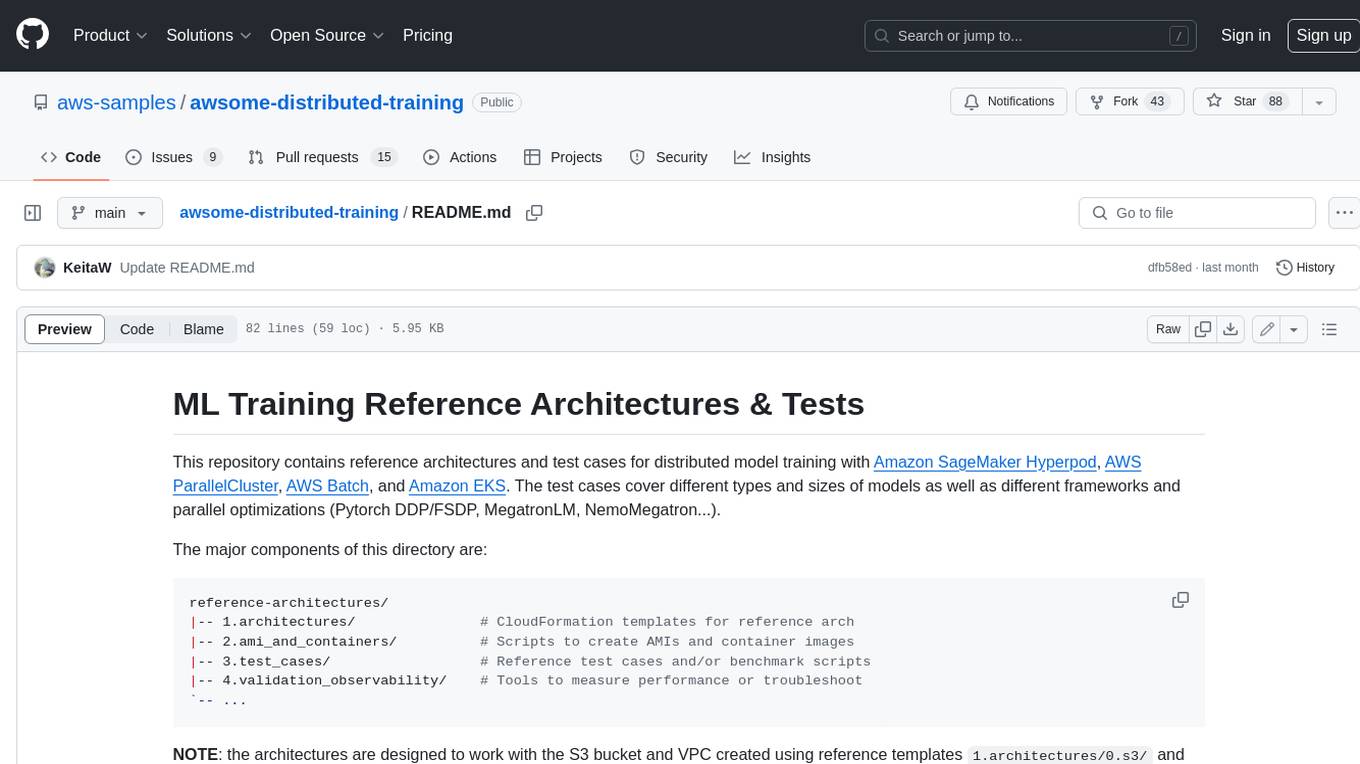
awsome-distributed-training
This repository contains reference architectures and test cases for distributed model training with Amazon SageMaker Hyperpod, AWS ParallelCluster, AWS Batch, and Amazon EKS. The test cases cover different types and sizes of models as well as different frameworks and parallel optimizations (Pytorch DDP/FSDP, MegatronLM, NemoMegatron...).
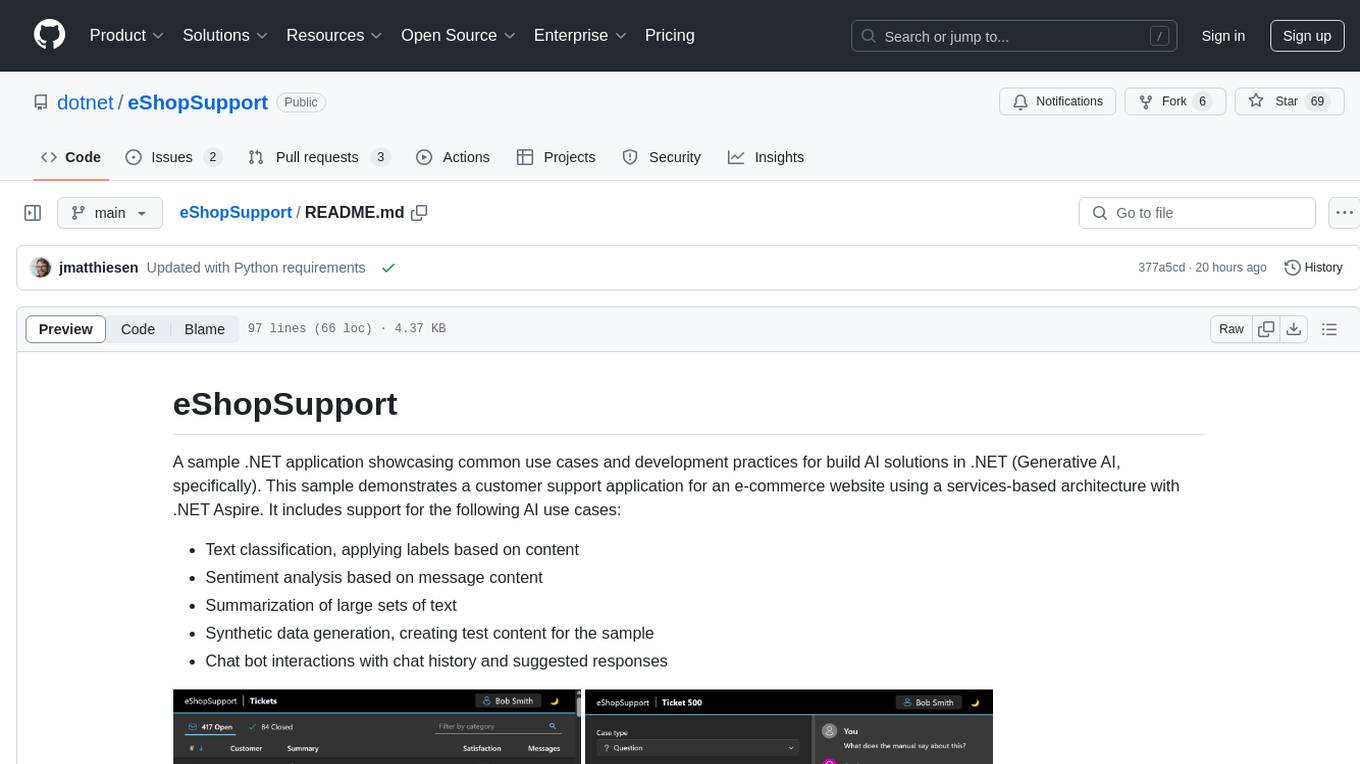
eShopSupport
eShopSupport is a sample .NET application showcasing common use cases and development practices for building AI solutions in .NET, specifically Generative AI. It demonstrates a customer support application for an e-commerce website using a services-based architecture with .NET Aspire. The application includes support for text classification, sentiment analysis, text summarization, synthetic data generation, and chat bot interactions. It also showcases development practices such as developing solutions locally, evaluating AI responses, leveraging Python projects, and deploying applications to the Cloud.
20 - OpenAI Gpts
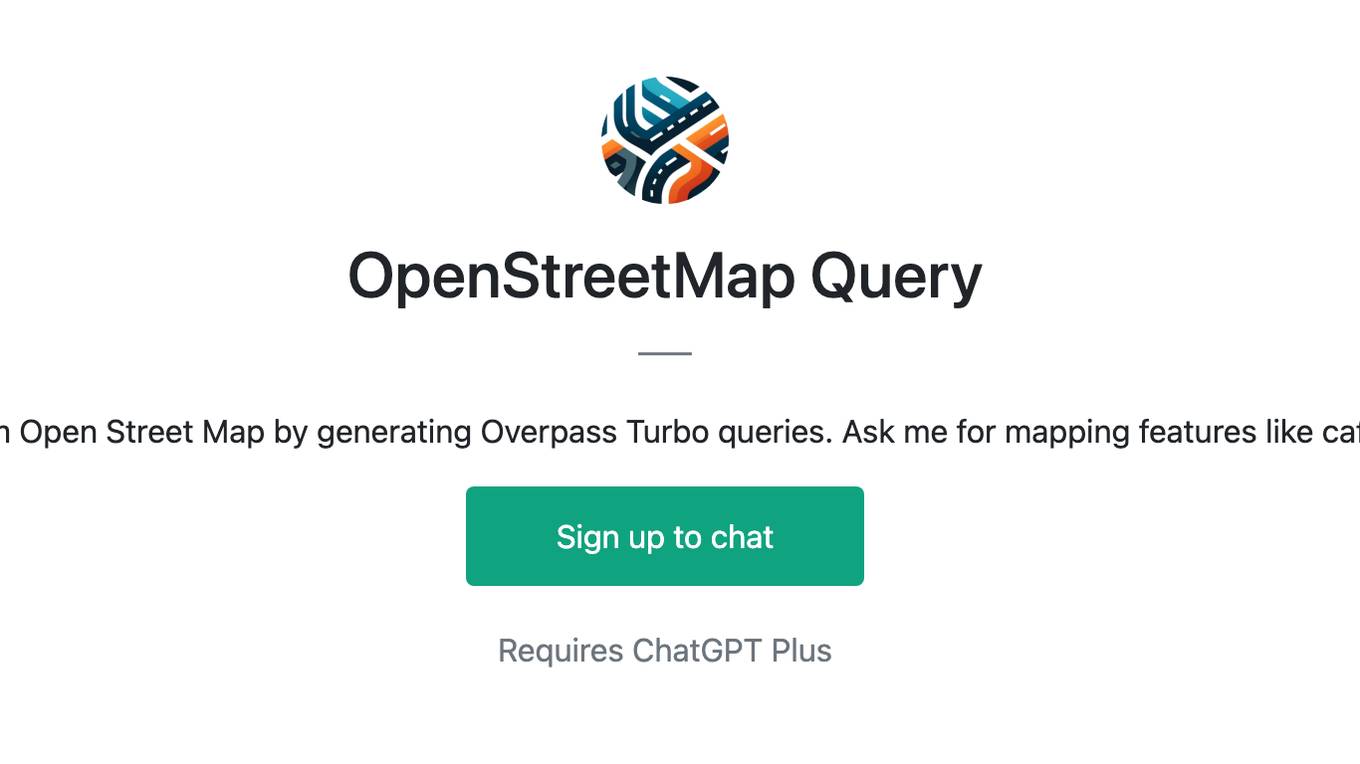
OpenStreetMap Query
Helps get map data from Open Street Map by generating Overpass Turbo queries. Ask me for mapping features like cafes, rivers or highways
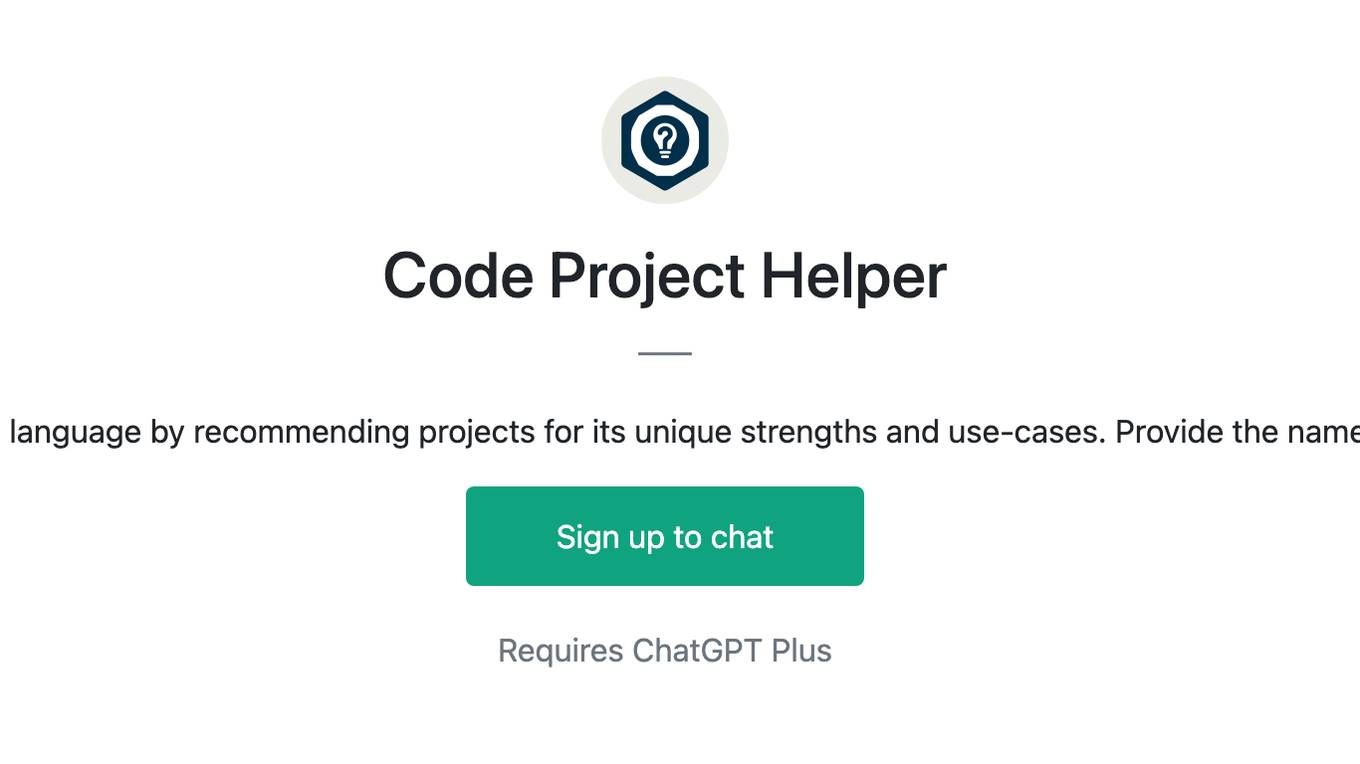
Code Project Helper
Helps with learning a programming language by recommending projects for its unique strengths and use-cases. Provide the name of language only as the prompt.
Pizza! Pizza! Lowdown
Expert on Little Caesar's Pizza, sharing accurate facts, stories, and figures.
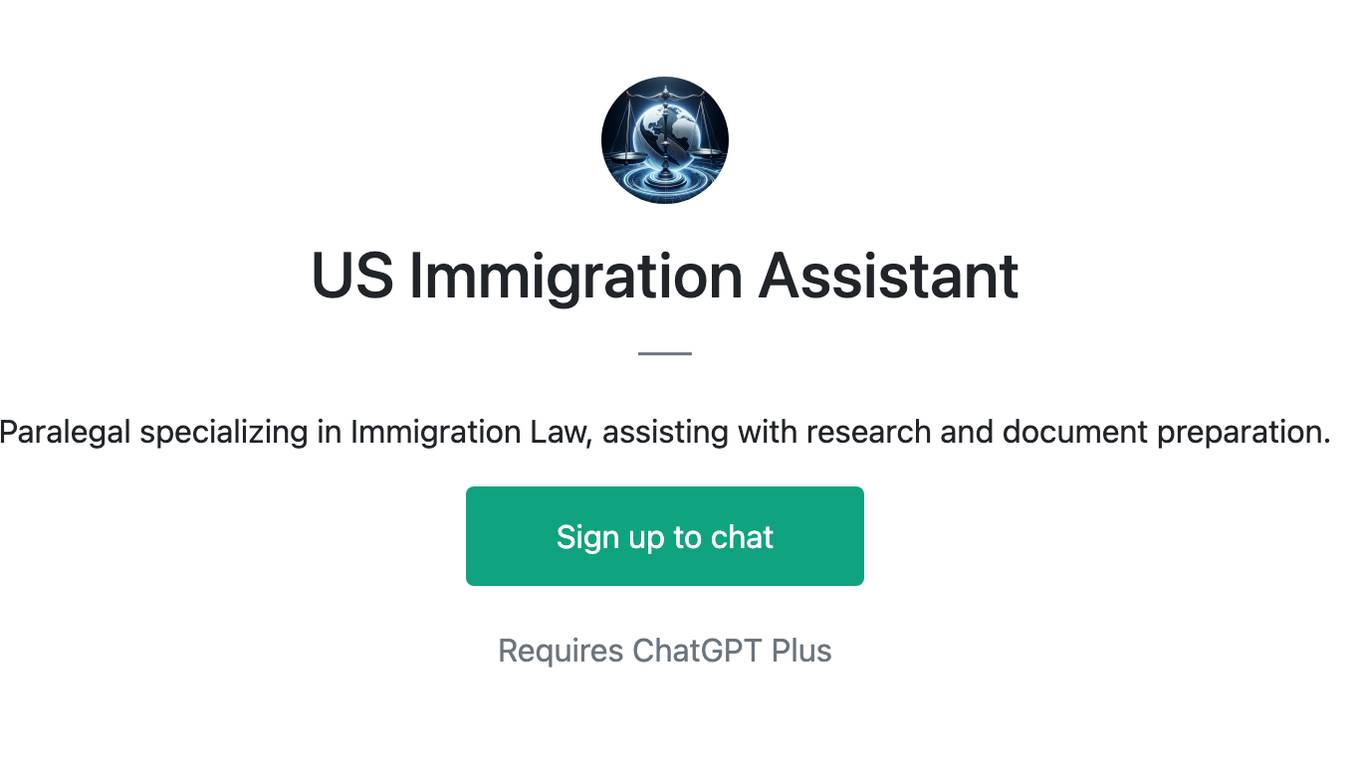
US Immigration Assistant
Paralegal specializing in Immigration Law, assisting with research and document preparation.
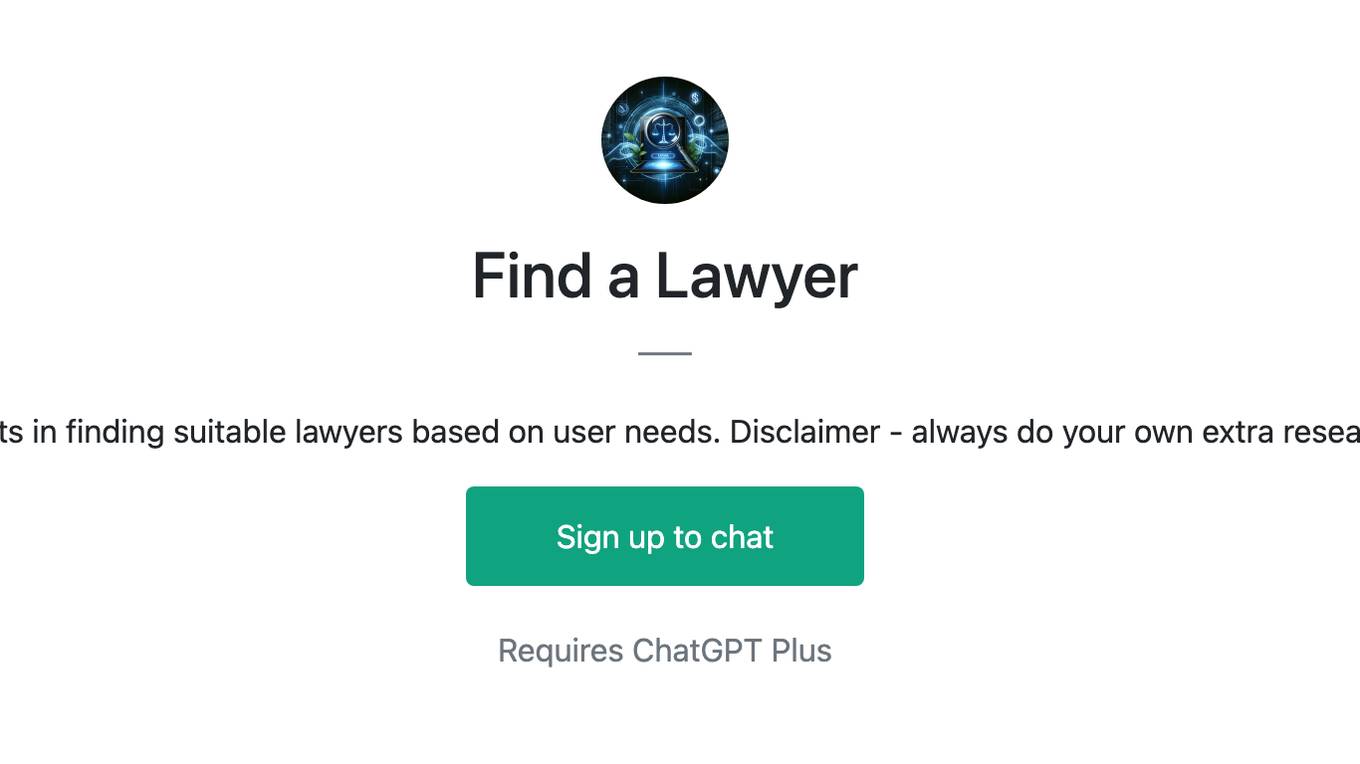
Find a Lawyer
Assists in finding suitable lawyers based on user needs. Disclaimer - always do your own extra research