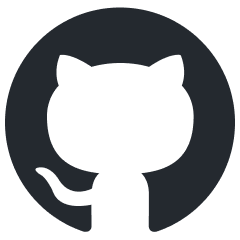
runhouse
The Runhouse Python client. Distribute and run AI workloads magically in Python, like PyTorch for ML infra.
Stars: 1008
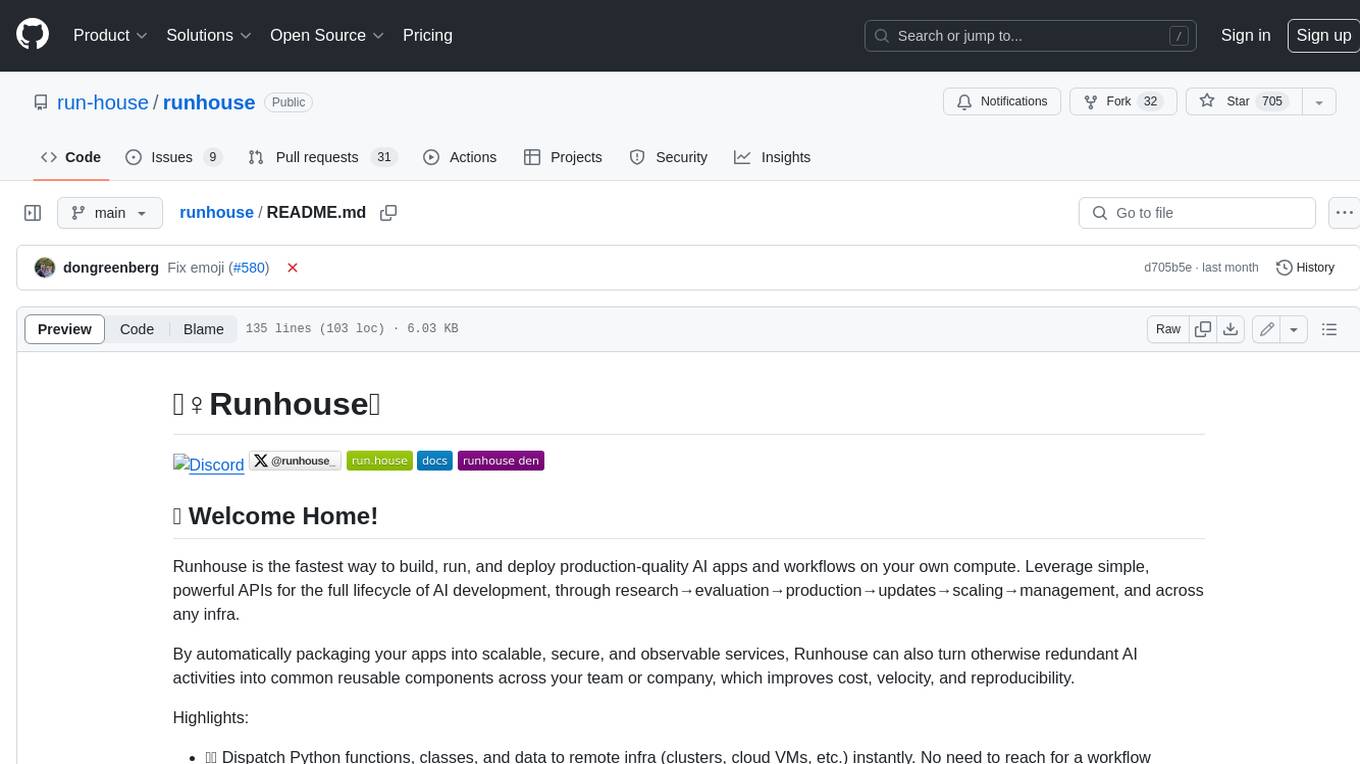
Runhouse is a tool that allows you to build, run, and deploy production-quality AI apps and workflows on your own compute. It provides simple, powerful APIs for the full lifecycle of AI development, from research to evaluation to production to updates to scaling to management, and across any infra. By automatically packaging your apps into scalable, secure, and observable services, Runhouse can also turn otherwise redundant AI activities into common reusable components across your team or company, which improves cost, velocity, and reproducibility.
README:
The Runhouse Python client provides a convenient way to access and effortlessly dispatch distributed training workloads to a pool of compute inside your own cloud.
This library requires Python 3.8 – 3.12.
Install the package with pip
:
pip install runhouse
For a detailed walkthrough and usage guide, see our docs and examples.
For any question or support request you may have, please email us ([email protected]) or message us on Discord.
For Tasks:
Click tags to check more tools for each tasksFor Jobs:
Alternative AI tools for runhouse
Similar Open Source Tools
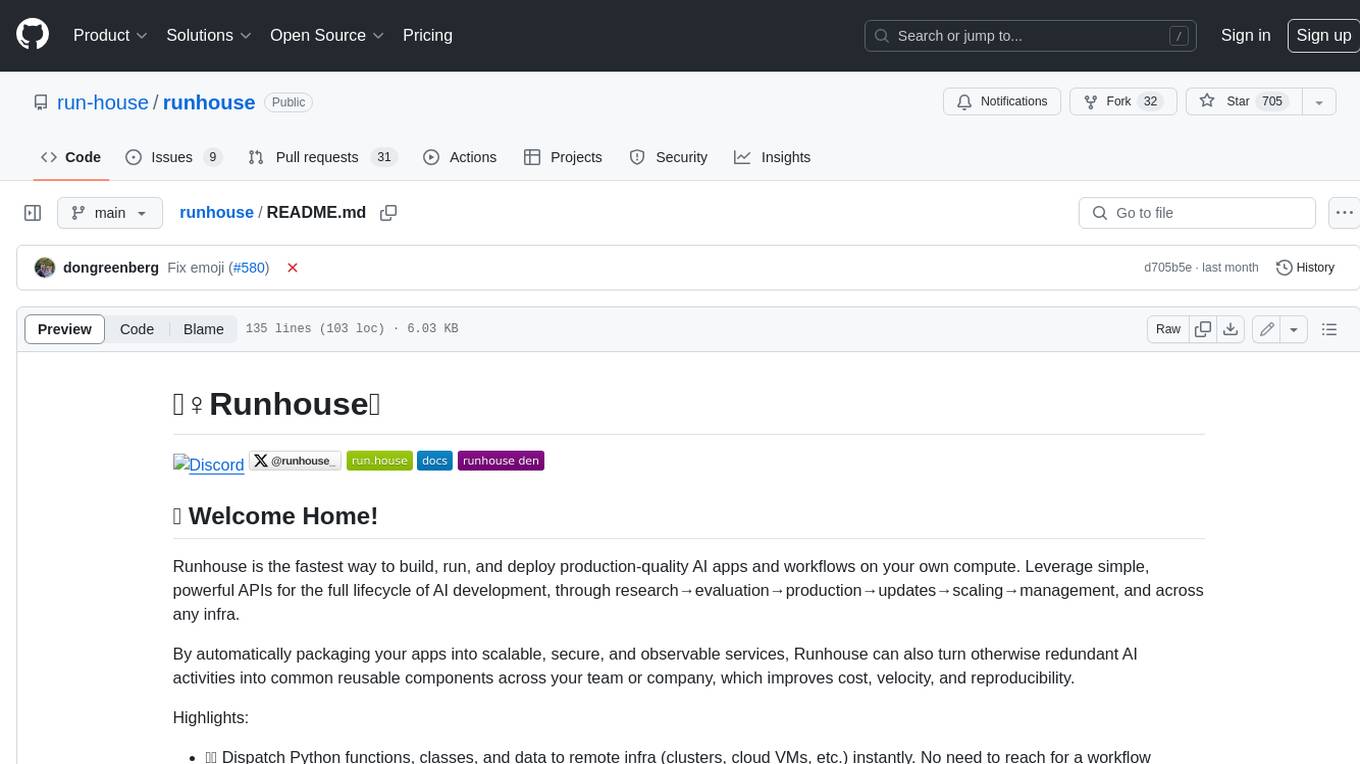
runhouse
Runhouse is a tool that allows you to build, run, and deploy production-quality AI apps and workflows on your own compute. It provides simple, powerful APIs for the full lifecycle of AI development, from research to evaluation to production to updates to scaling to management, and across any infra. By automatically packaging your apps into scalable, secure, and observable services, Runhouse can also turn otherwise redundant AI activities into common reusable components across your team or company, which improves cost, velocity, and reproducibility.
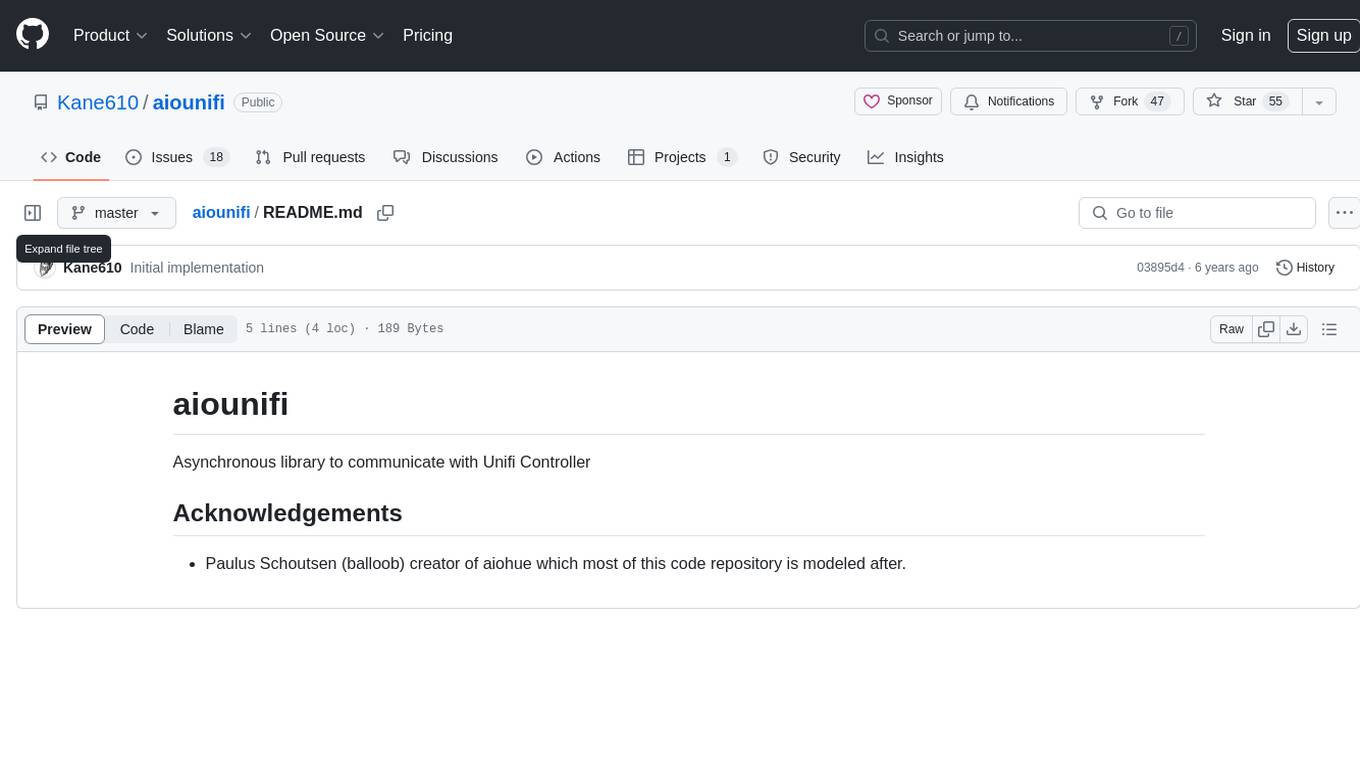
aiounifi
Aiounifi is a Python library that provides a simple interface for interacting with the Unifi Controller API. It allows users to easily manage their Unifi network devices, such as access points, switches, and gateways, through automated scripts or applications. With Aiounifi, users can retrieve device information, perform configuration changes, monitor network performance, and more, all through a convenient and efficient API wrapper. This library simplifies the process of integrating Unifi network management into custom solutions, making it ideal for network administrators, developers, and enthusiasts looking to automate and streamline their network operations.
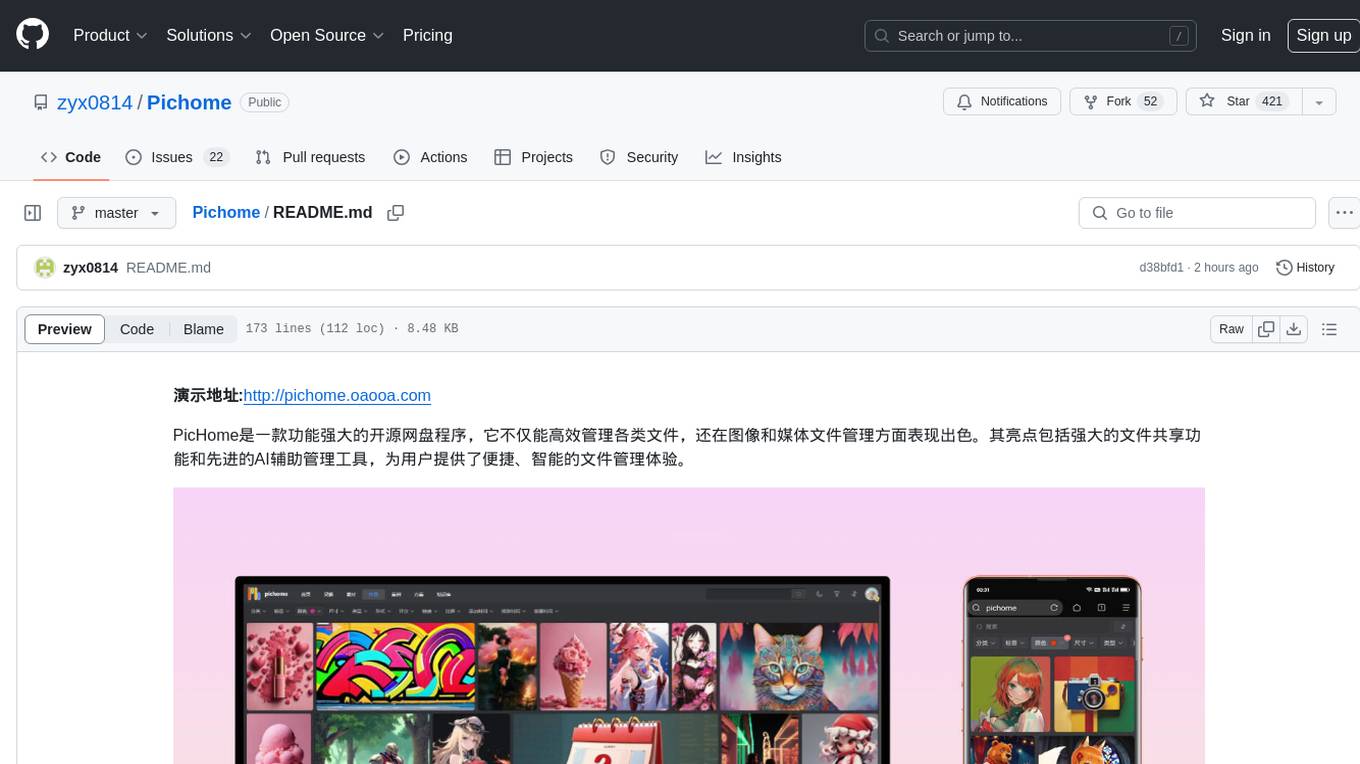
Pichome
PicHome is a powerful open-source cloud storage program that efficiently manages various types of files and excels in image and media file management. Its highlights include robust file sharing features and advanced AI-assisted management tools, providing users with a convenient and intelligent file management experience. The program offers diverse list modes, customizable file information display, enhanced quick file preview, advanced tagging, custom cover and preview images, multiple preview images, and multi-library management. Additionally, PicHome features strong file sharing capabilities, allowing users to share entire libraries, create personalized showcase web pages, and build complete data sharing websites. The AI-assisted management aspect includes AI file renaming, tagging, description writing, batch annotation, and file Q&A services, all aimed at improving file management efficiency. PicHome supports a wide range of file formats and can be applied in various scenarios such as e-commerce, gaming, design, development, enterprises, schools, labs, media, and entertainment institutions.
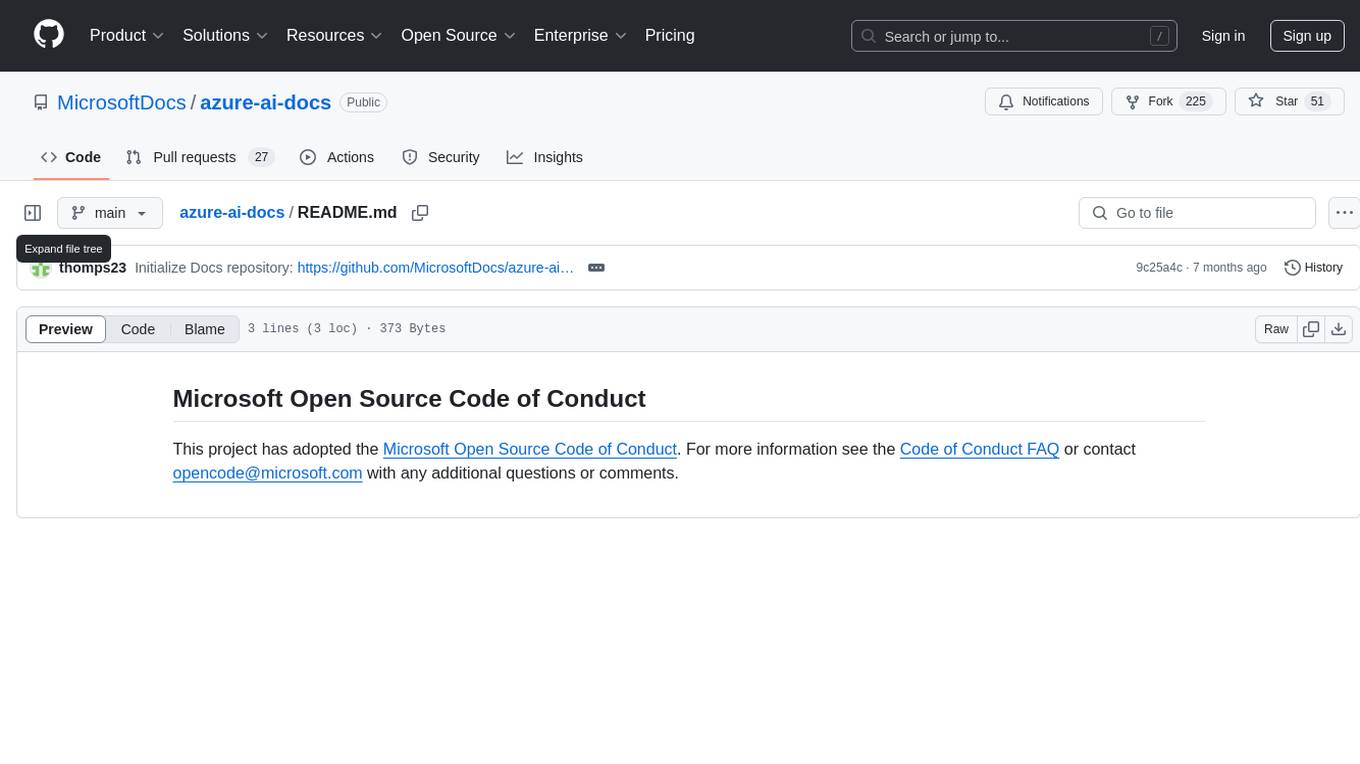
azure-ai-docs
Azure AI Docs is a repository that provides detailed documentation and resources for developers looking to leverage Microsoft's AI services on the Azure platform. The repository covers a wide range of topics including machine learning, natural language processing, computer vision, and more. Developers can find tutorials, code samples, best practices, and guidelines to help them integrate AI capabilities into their applications seamlessly.
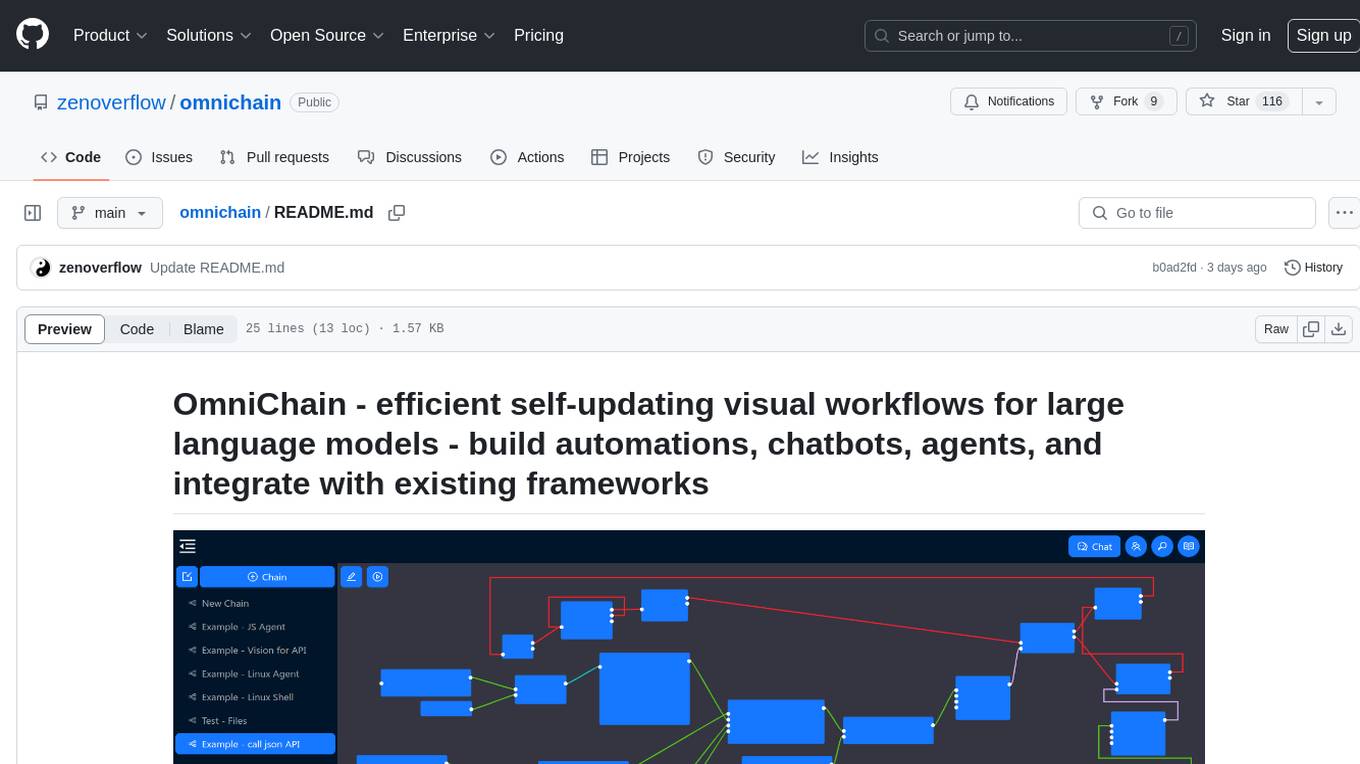
omnichain
OmniChain is a tool for building efficient self-updating visual workflows using AI language models, enabling users to automate tasks, create chatbots, agents, and integrate with existing frameworks. It allows users to create custom workflows guided by logic processes, store and recall information, and make decisions based on that information. The tool enables users to create tireless robot employees that operate 24/7, access the underlying operating system, generate and run NodeJS code snippets, and create custom agents and logic chains. OmniChain is self-hosted, open-source, and available for commercial use under the MIT license, with no coding skills required.
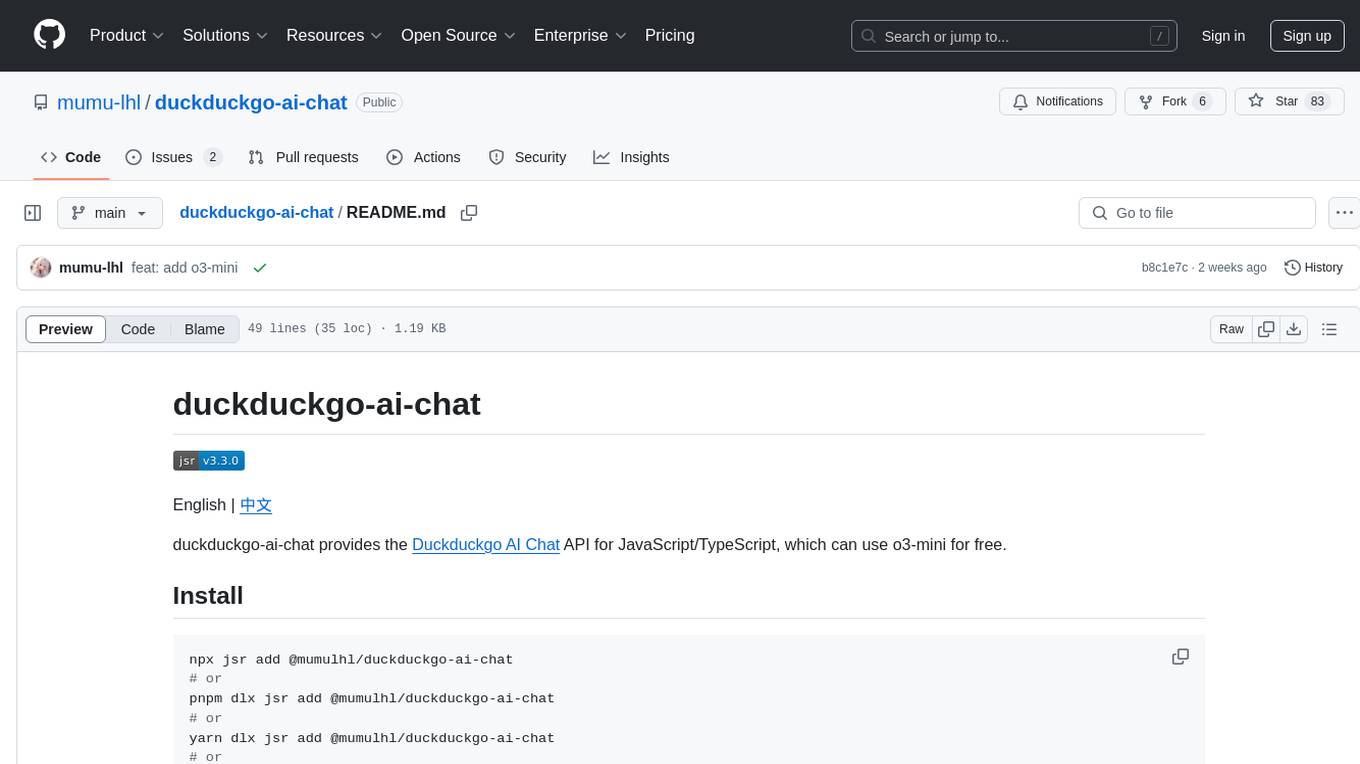
duckduckgo-ai-chat
This repository contains a chatbot tool powered by AI technology. The chatbot is designed to interact with users in a conversational manner, providing information and assistance on various topics. Users can engage with the chatbot to ask questions, seek recommendations, or simply have a casual conversation. The AI technology behind the chatbot enables it to understand natural language inputs and provide relevant responses, making the interaction more intuitive and engaging. The tool is versatile and can be customized for different use cases, such as customer support, information retrieval, or entertainment purposes. Overall, the chatbot offers a user-friendly and interactive experience, leveraging AI to enhance communication and engagement.
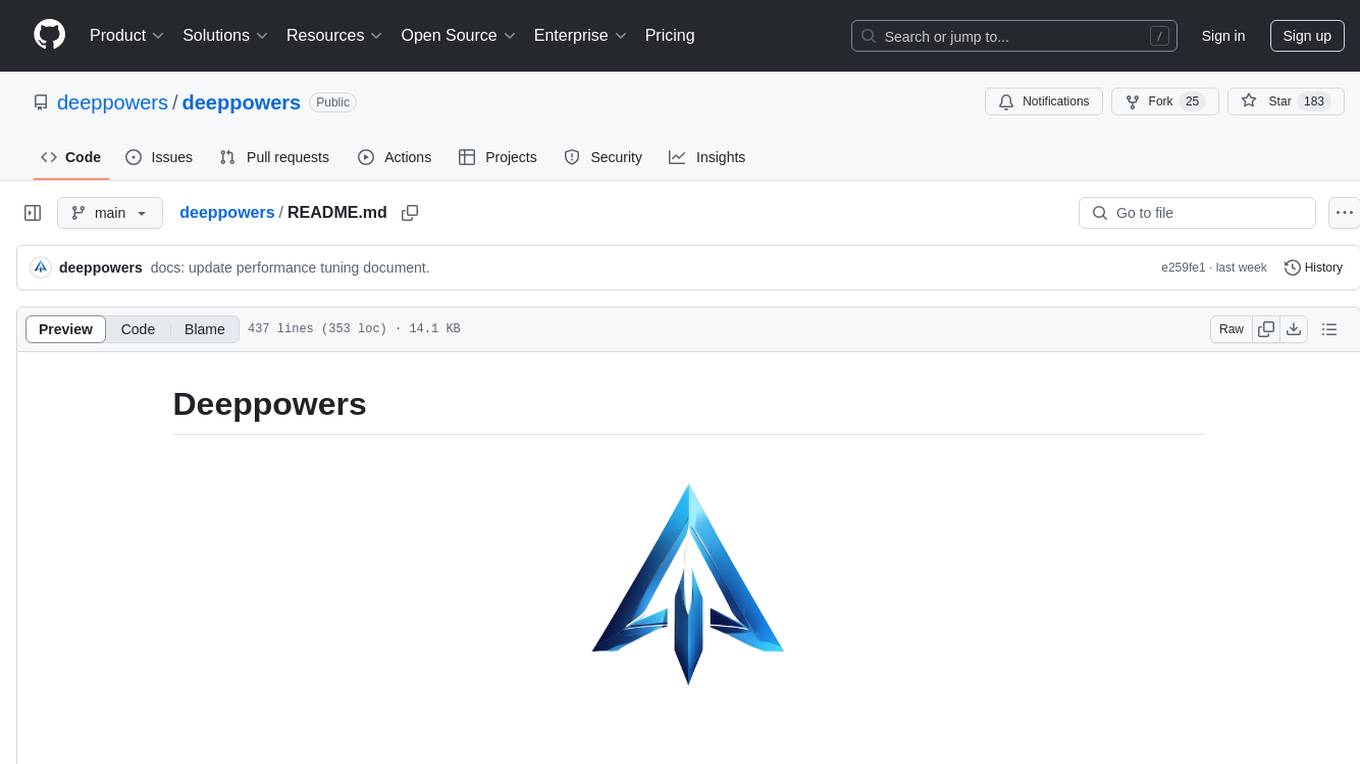
deeppowers
Deeppowers is a powerful Python library for deep learning applications. It provides a wide range of tools and utilities to simplify the process of building and training deep neural networks. With Deeppowers, users can easily create complex neural network architectures, perform efficient training and optimization, and deploy models for various tasks. The library is designed to be user-friendly and flexible, making it suitable for both beginners and experienced deep learning practitioners.
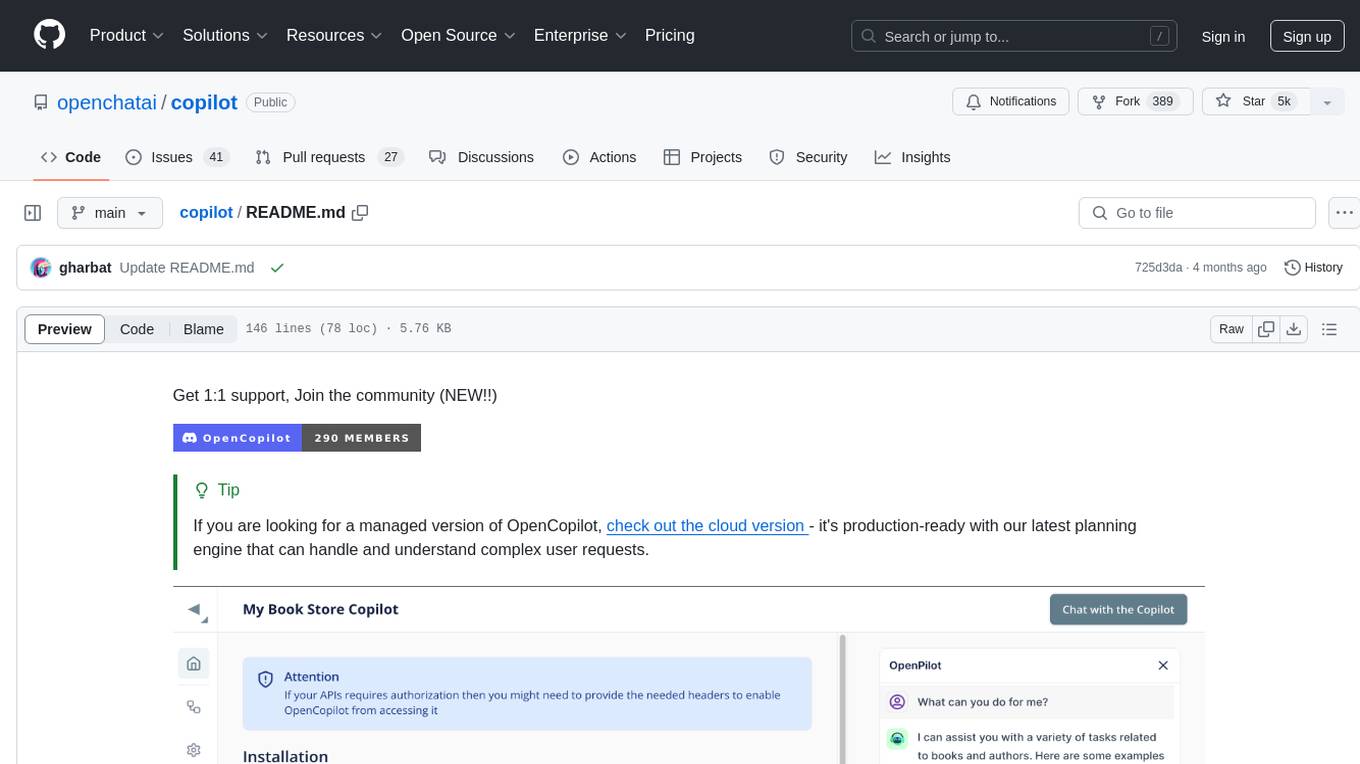
copilot
OpenCopilot is a tool that allows users to create their own AI copilot for their products. It integrates with APIs to execute calls as needed, using LLMs to determine the appropriate endpoint and payload. Users can define API actions, validate schemas, and integrate a user-friendly chat bubble into their SaaS app. The tool is capable of calling APIs, transforming responses, and populating request fields based on context. It is not suitable for handling large APIs without JSON transformers. Users can teach the copilot via flows and embed it in their app with minimal code.
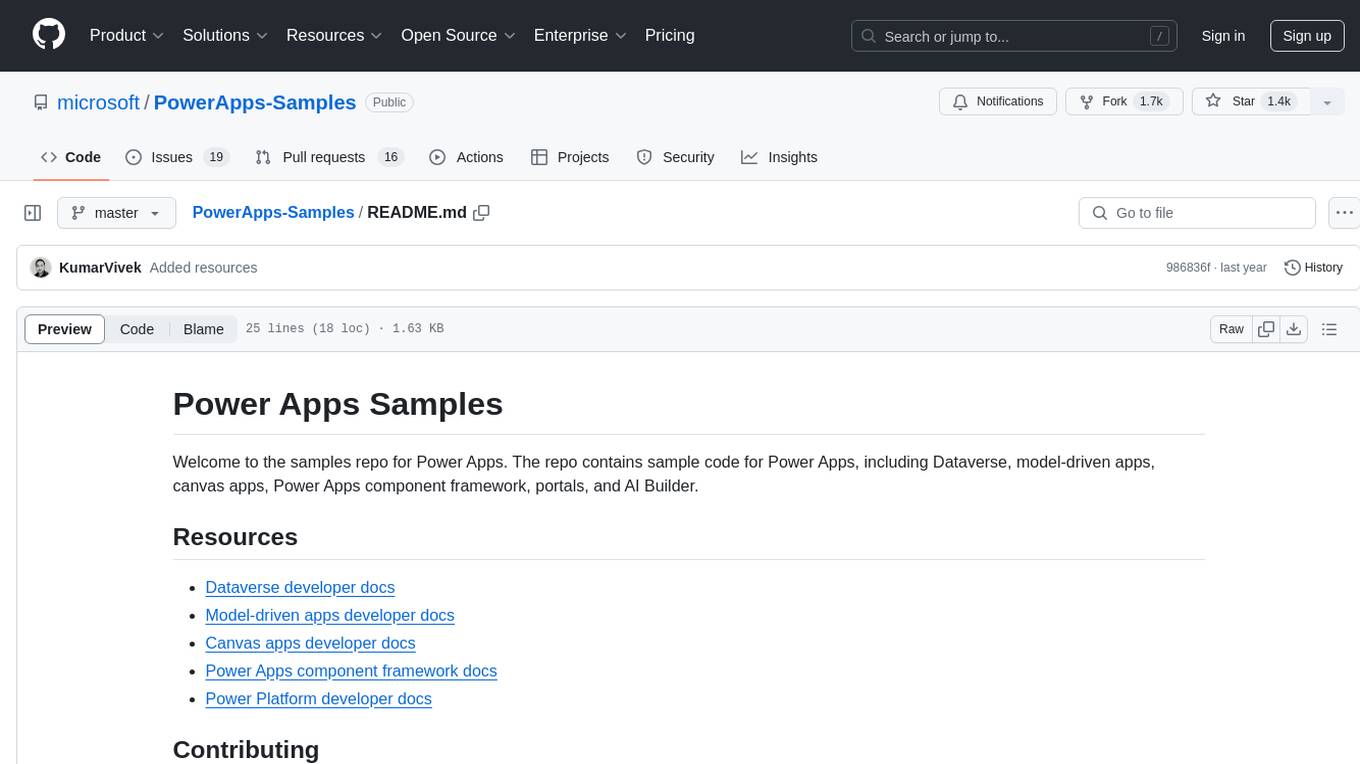
PowerApps-Samples
PowerApps-Samples is a repository containing sample code for Power Apps, covering various aspects such as Dataverse, model-driven apps, canvas apps, Power Apps component framework, portals, and AI Builder. It serves as a valuable resource for developers looking to explore and learn about different functionalities within Power Apps ecosystem.
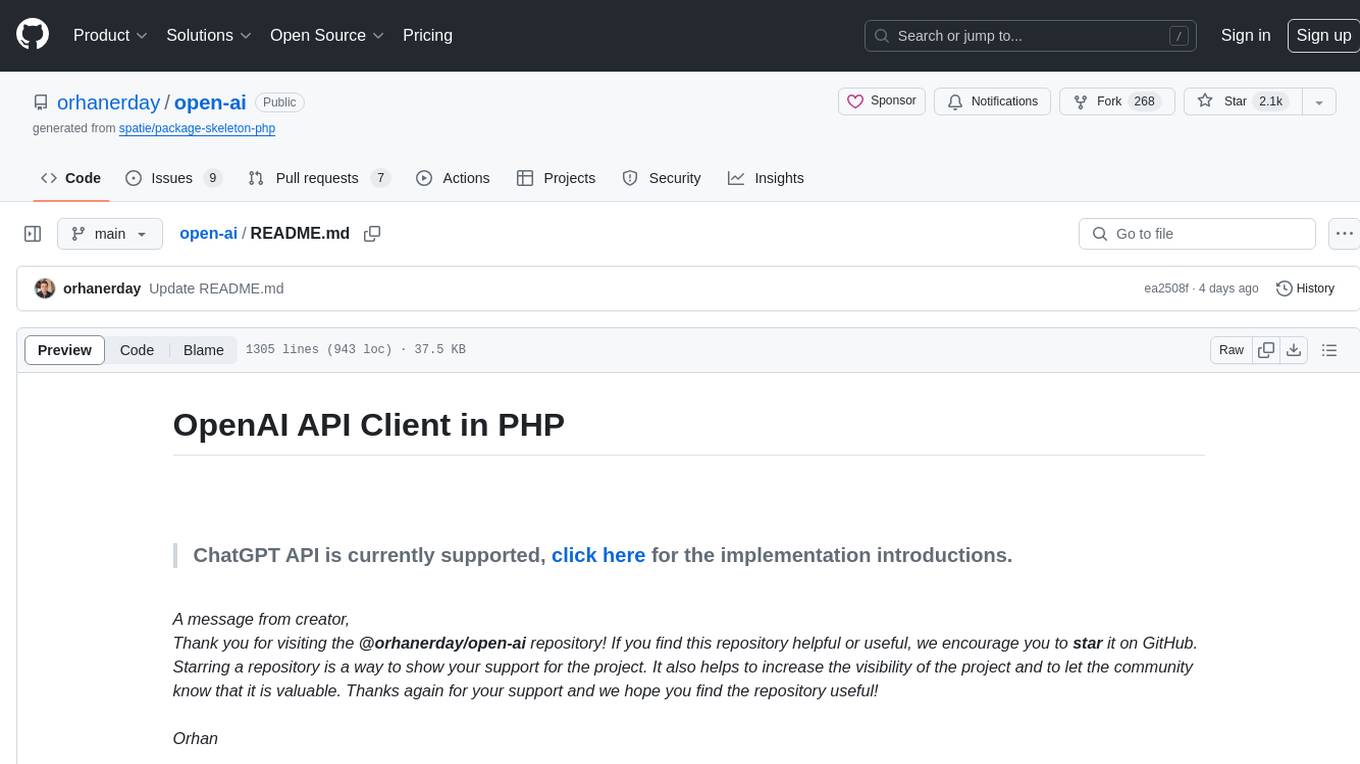
open-ai
Open AI is a powerful tool for artificial intelligence research and development. It provides a wide range of machine learning models and algorithms, making it easier for developers to create innovative AI applications. With Open AI, users can explore cutting-edge technologies such as natural language processing, computer vision, and reinforcement learning. The platform offers a user-friendly interface and comprehensive documentation to support users in building and deploying AI solutions. Whether you are a beginner or an experienced AI practitioner, Open AI offers the tools and resources you need to accelerate your AI projects and stay ahead in the rapidly evolving field of artificial intelligence.
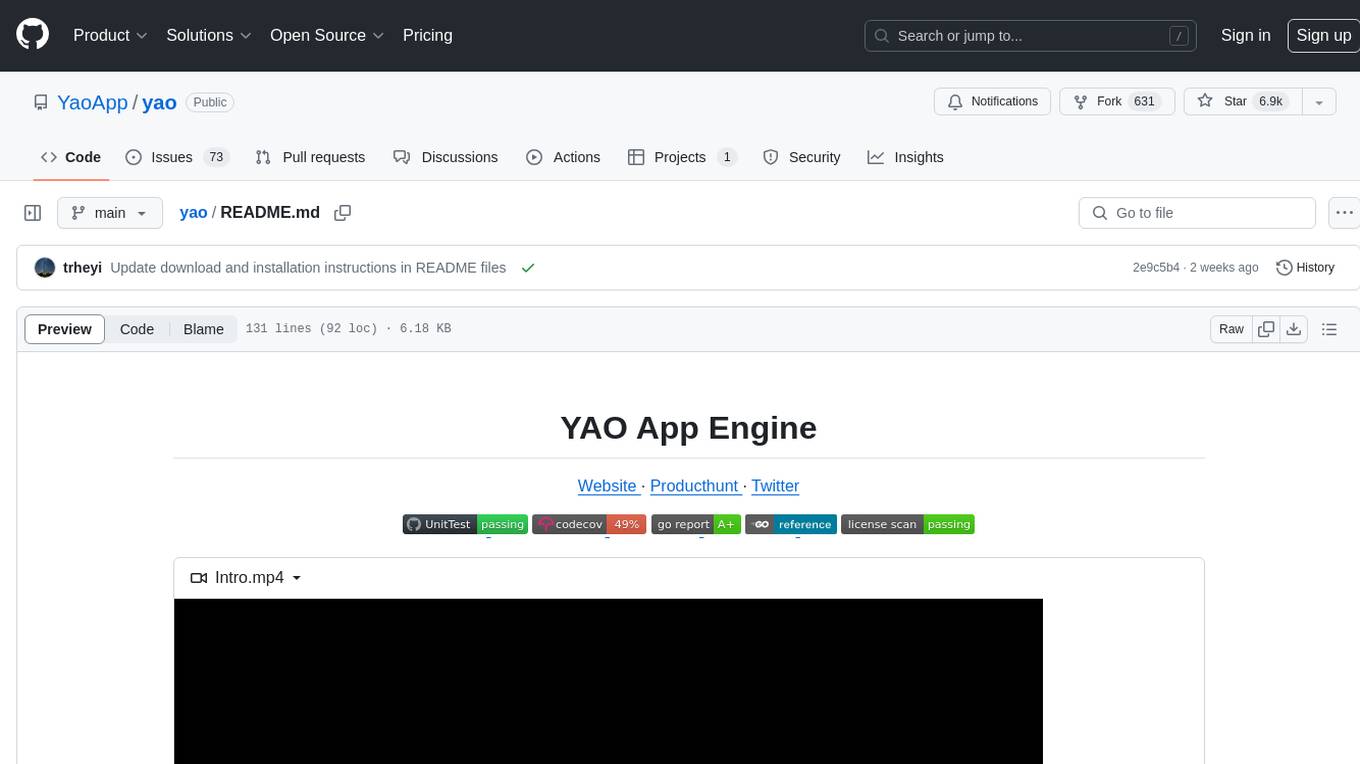
yao
YAO is an open-source application engine written in Golang, suitable for developing business systems, website/APP API, admin panel, and self-built low-code platforms. It adopts a flow-based programming model to implement functions by writing YAO DSL or using JavaScript. Yao allows developers to create web services by processes, creating a database model, writing API services, and describing dashboard interfaces just by JSON for web & hardware, and 10x productivity. It is based on the flow-based programming idea, developed in Go language, and supports multiple ways to expand the data stream processor. Yao has a built-in data management system, making it suitable for quickly making various management backgrounds, CRM, ERP, and other internal enterprise systems. It is highly versatile, efficient, and performs better than PHP, JAVA, and other languages.
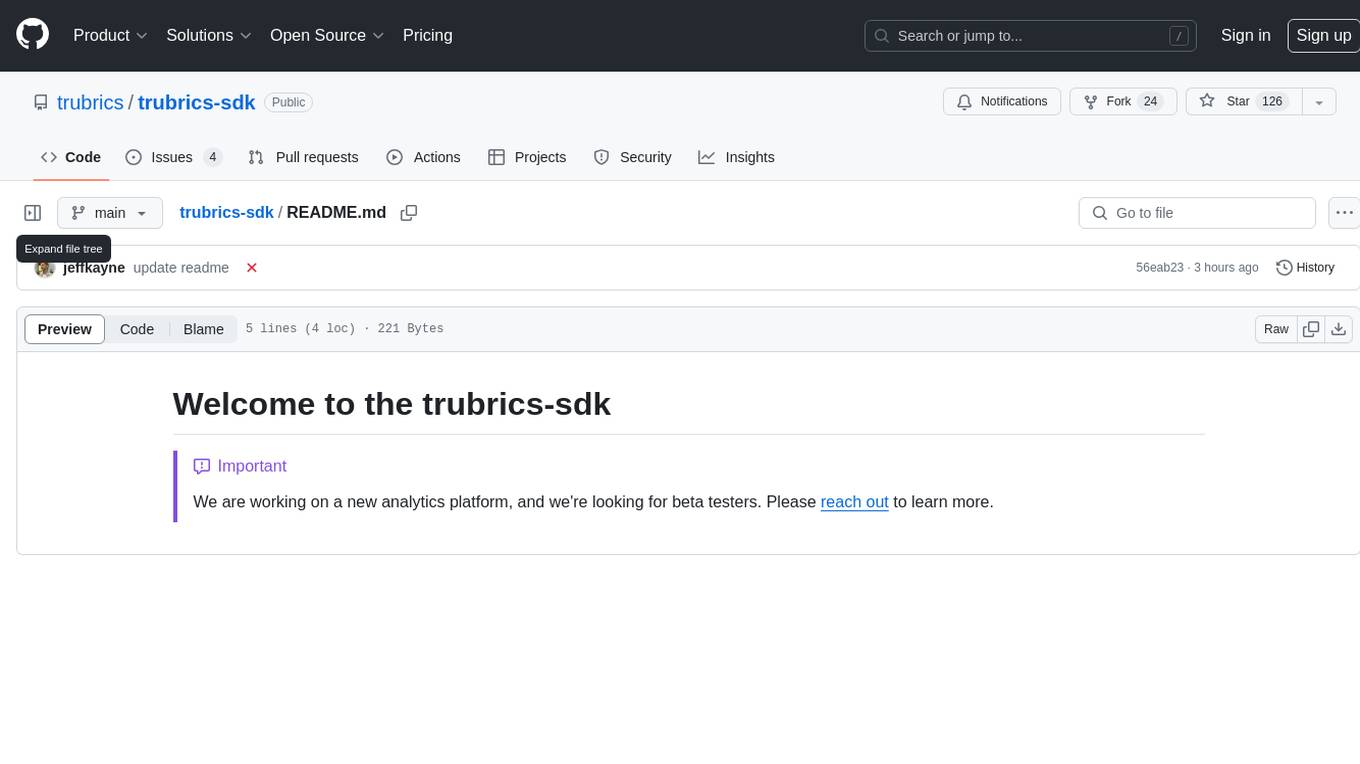
trubrics-sdk
Trubrics-sdk is a software development kit designed to facilitate the integration of analytics features into applications. It provides a set of tools and functionalities that enable developers to easily incorporate analytics capabilities, such as data collection, analysis, and reporting, into their software products. The SDK streamlines the process of implementing analytics solutions, allowing developers to focus on building and enhancing their applications' functionality and user experience. By leveraging trubrics-sdk, developers can quickly and efficiently integrate robust analytics features, gaining valuable insights into user behavior and application performance.
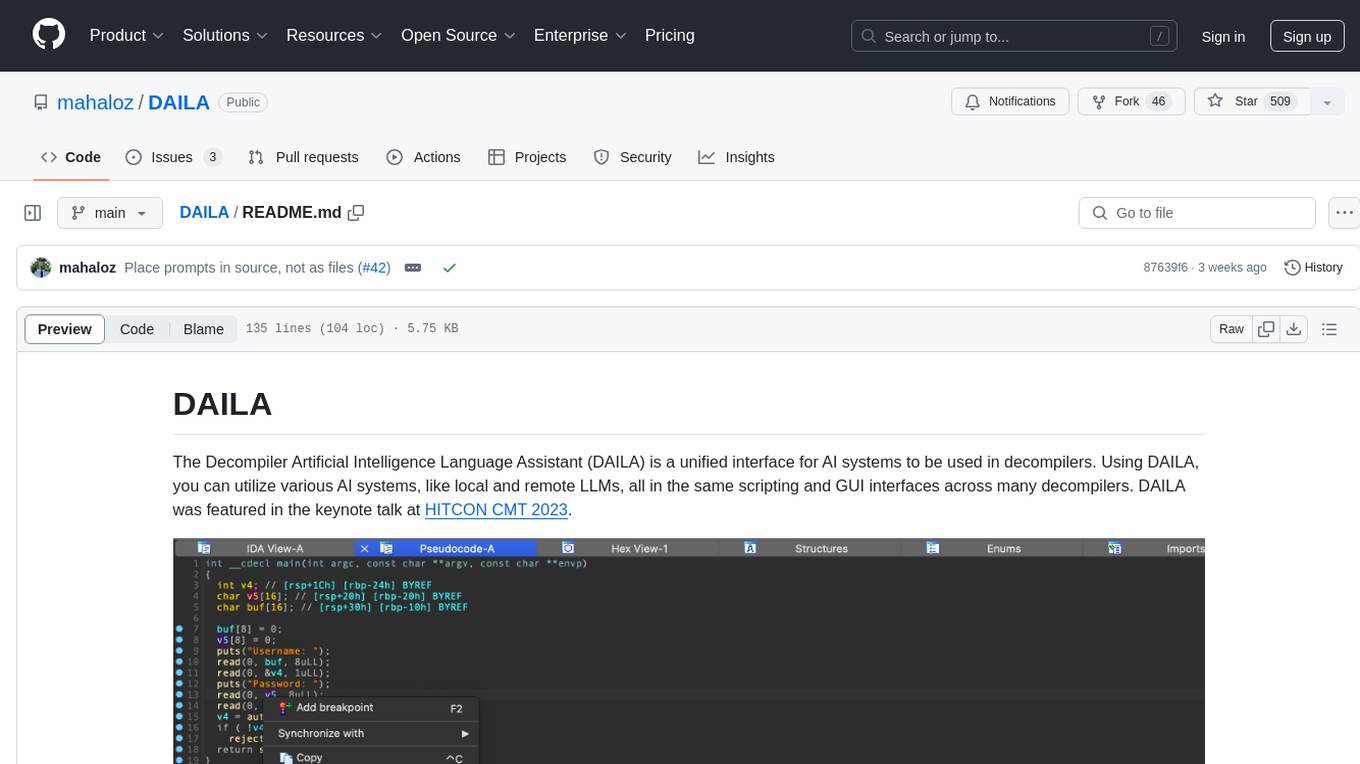
DAILA
DAILA is a unified interface for AI systems in decompilers, supporting various decompilers and AI systems. It allows users to utilize local and remote LLMs, like ChatGPT and Claude, and local models such as VarBERT. DAILA can be used as a decompiler plugin with GUI or as a scripting library. It also provides a Docker container for offline installations and supports tasks like summarizing functions and renaming variables in decompilation.
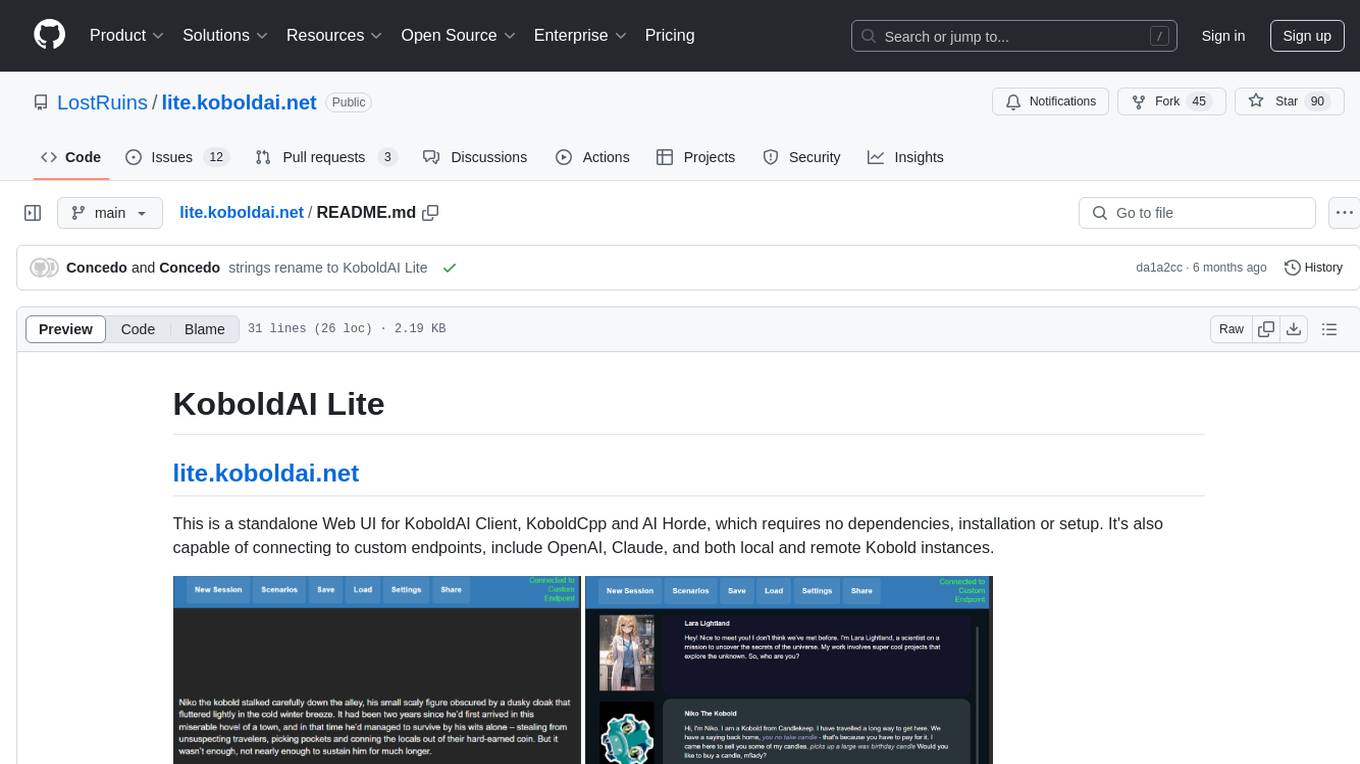
lite.koboldai.net
KoboldAI Lite is a standalone Web UI that serves as a text editor designed for use with generative LLMs. It is compatible with KoboldAI United and KoboldAI Client, bundled with KoboldCPP, and integrates with the AI Horde for text and image generation. The UI offers multiple modes for different writing styles, supports various file formats, includes premade scenarios, and allows easy sharing of stories. Users can enjoy features such as memory, undo/redo, text-to-speech, and a range of samplers and configurations. The tool is mobile-friendly and can be used directly from a browser without any setup or installation.
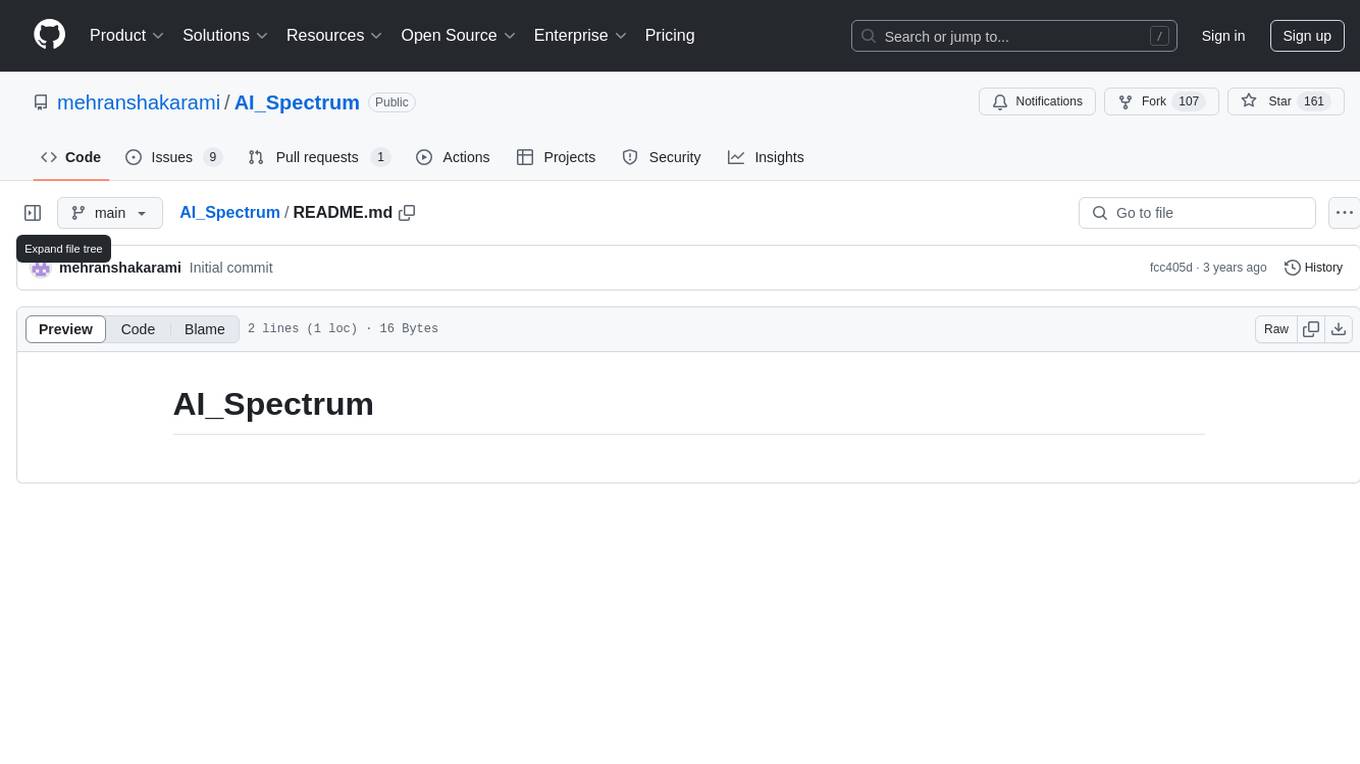
AI_Spectrum
AI_Spectrum is a versatile machine learning library that provides a wide range of tools and algorithms for building and deploying AI models. It offers a user-friendly interface for data preprocessing, model training, and evaluation. With AI_Spectrum, users can easily experiment with different machine learning techniques and optimize their models for various tasks. The library is designed to be flexible and scalable, making it suitable for both beginners and experienced data scientists.
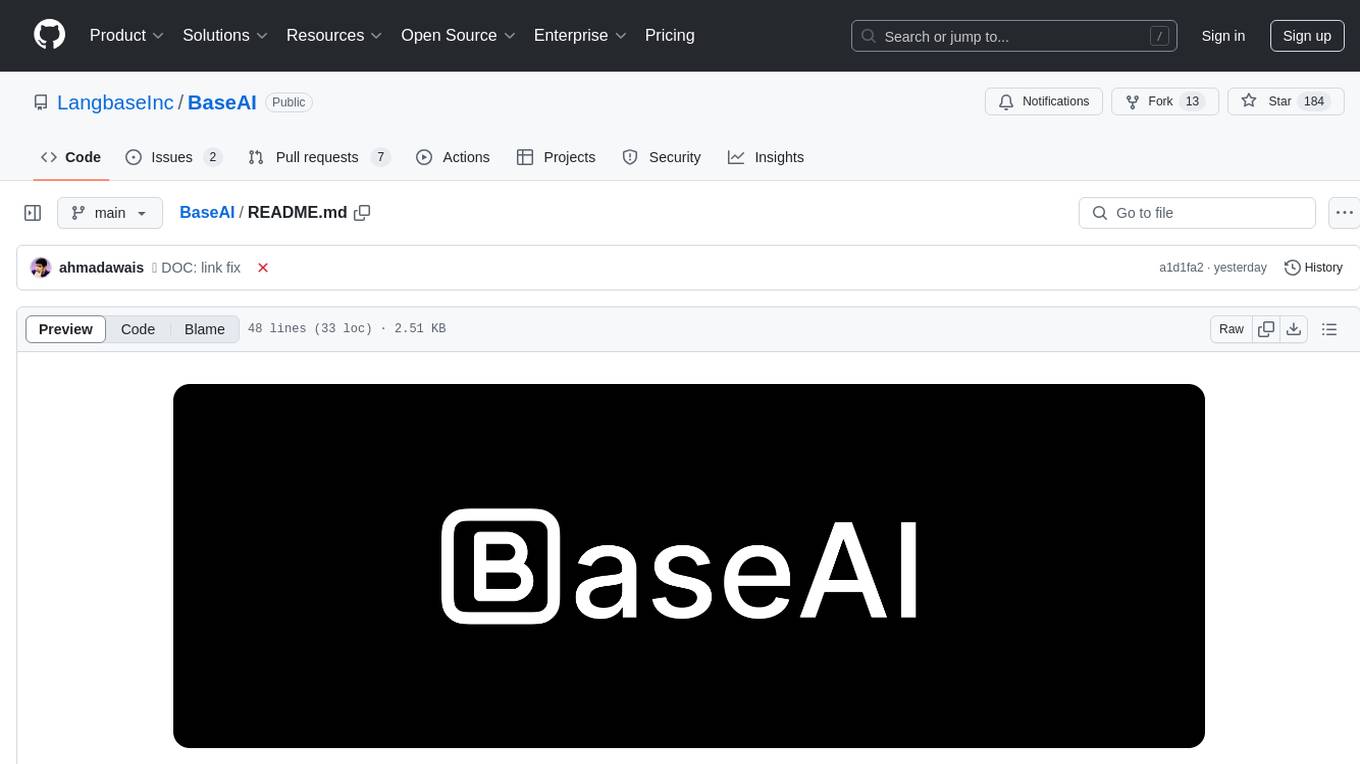
BaseAI
BaseAI is an AI framework designed for creating declarative and composable AI-powered LLM products. It enables the development of AI agent pipes locally, incorporating agentic tools and memory (RAG). The framework offers a learn guide for beginners to kickstart their journey with BaseAI. For detailed documentation, users can visit baseai.dev/docs. Contributions to BaseAI are encouraged, and interested individuals can refer to the Contributing Guide. The original authors of BaseAI include Ahmad Awais, Ashar Irfan, Saqib Ameen, Saad Irfan, and Ahmad Bilal. Security vulnerabilities can be reported privately via email to [email protected]. BaseAI aims to provide resources for learning AI agent development, utilizing agentic tools and memory.
For similar tasks
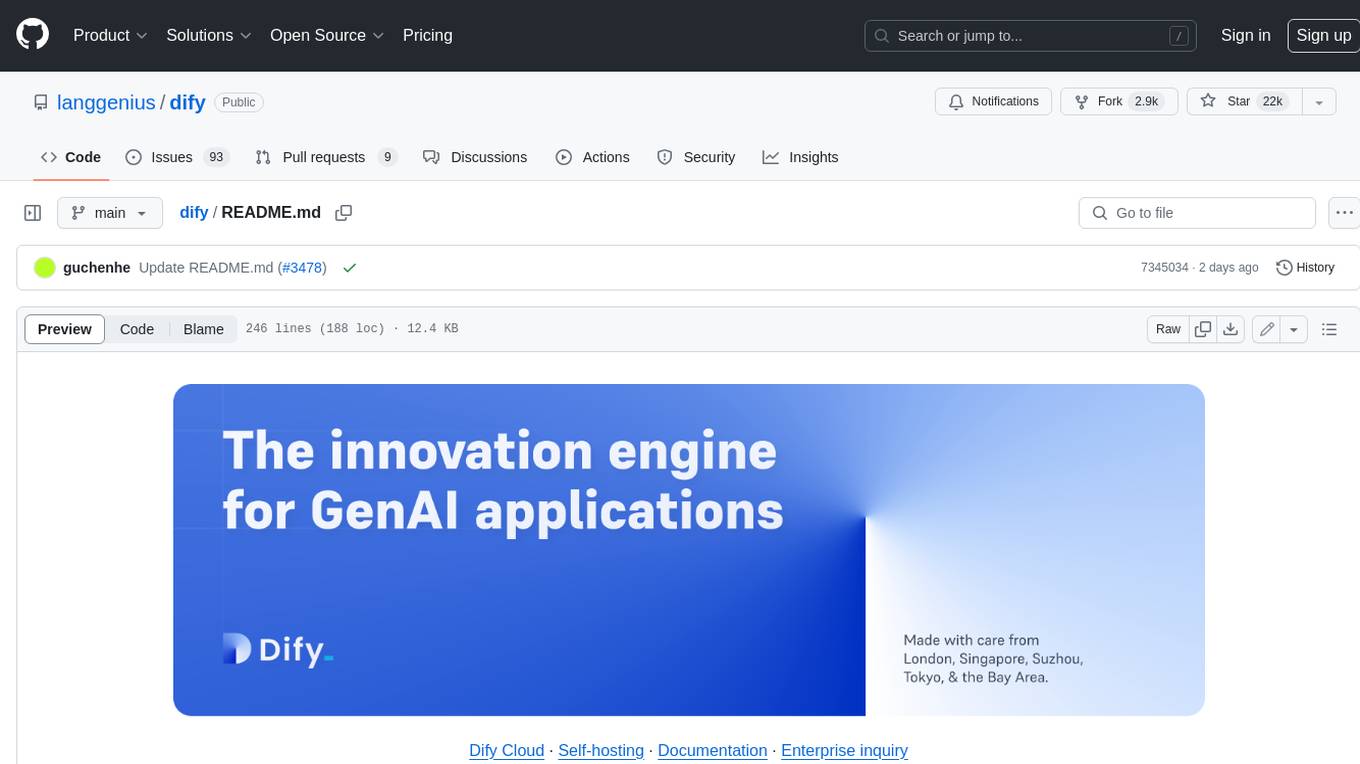
dify
Dify is an open-source LLM app development platform that combines AI workflow, RAG pipeline, agent capabilities, model management, observability features, and more. It allows users to quickly go from prototype to production. Key features include: 1. Workflow: Build and test powerful AI workflows on a visual canvas. 2. Comprehensive model support: Seamless integration with hundreds of proprietary / open-source LLMs from dozens of inference providers and self-hosted solutions. 3. Prompt IDE: Intuitive interface for crafting prompts, comparing model performance, and adding additional features. 4. RAG Pipeline: Extensive RAG capabilities that cover everything from document ingestion to retrieval. 5. Agent capabilities: Define agents based on LLM Function Calling or ReAct, and add pre-built or custom tools. 6. LLMOps: Monitor and analyze application logs and performance over time. 7. Backend-as-a-Service: All of Dify's offerings come with corresponding APIs for easy integration into your own business logic.
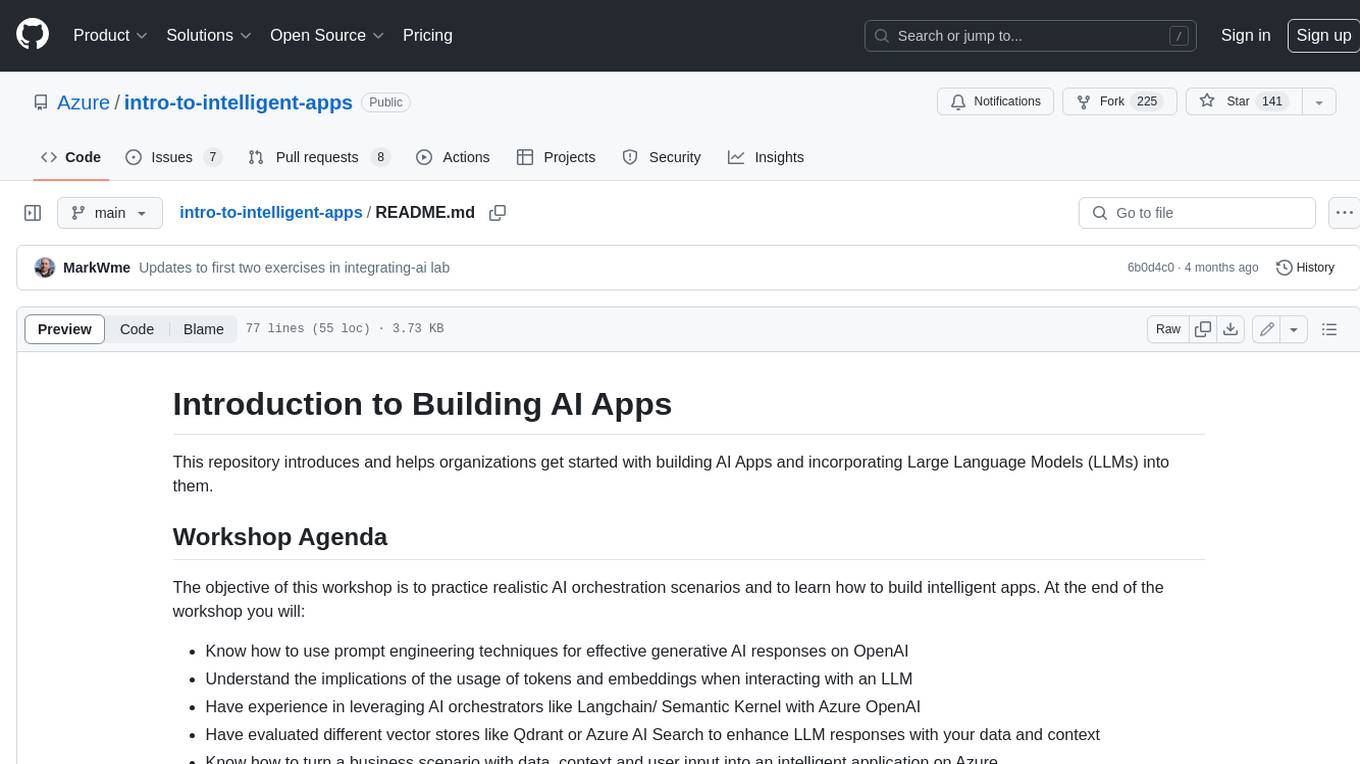
intro-to-intelligent-apps
This repository introduces and helps organizations get started with building AI Apps and incorporating Large Language Models (LLMs) into them. The workshop covers topics such as prompt engineering, AI orchestration, and deploying AI apps. Participants will learn how to use Azure OpenAI, Langchain/ Semantic Kernel, Qdrant, and Azure AI Search to build intelligent applications.
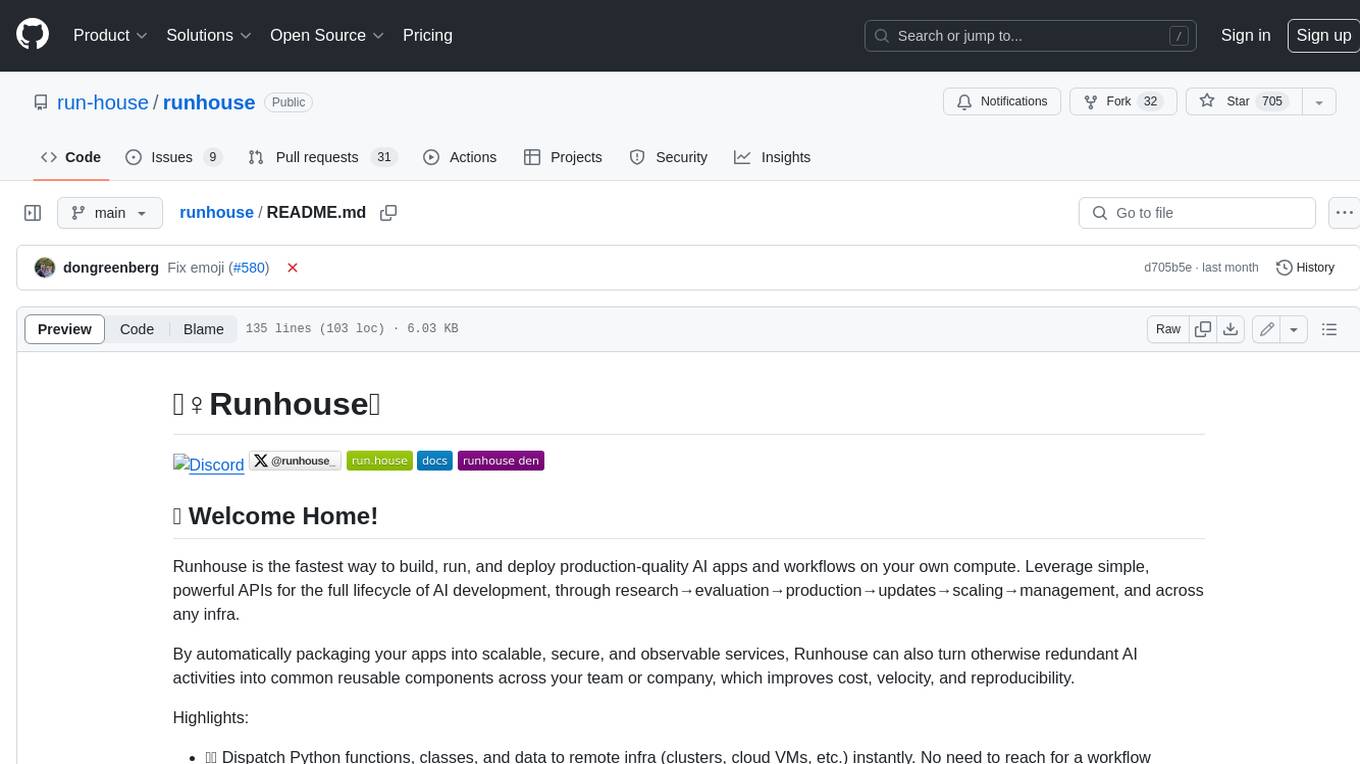
runhouse
Runhouse is a tool that allows you to build, run, and deploy production-quality AI apps and workflows on your own compute. It provides simple, powerful APIs for the full lifecycle of AI development, from research to evaluation to production to updates to scaling to management, and across any infra. By automatically packaging your apps into scalable, secure, and observable services, Runhouse can also turn otherwise redundant AI activities into common reusable components across your team or company, which improves cost, velocity, and reproducibility.
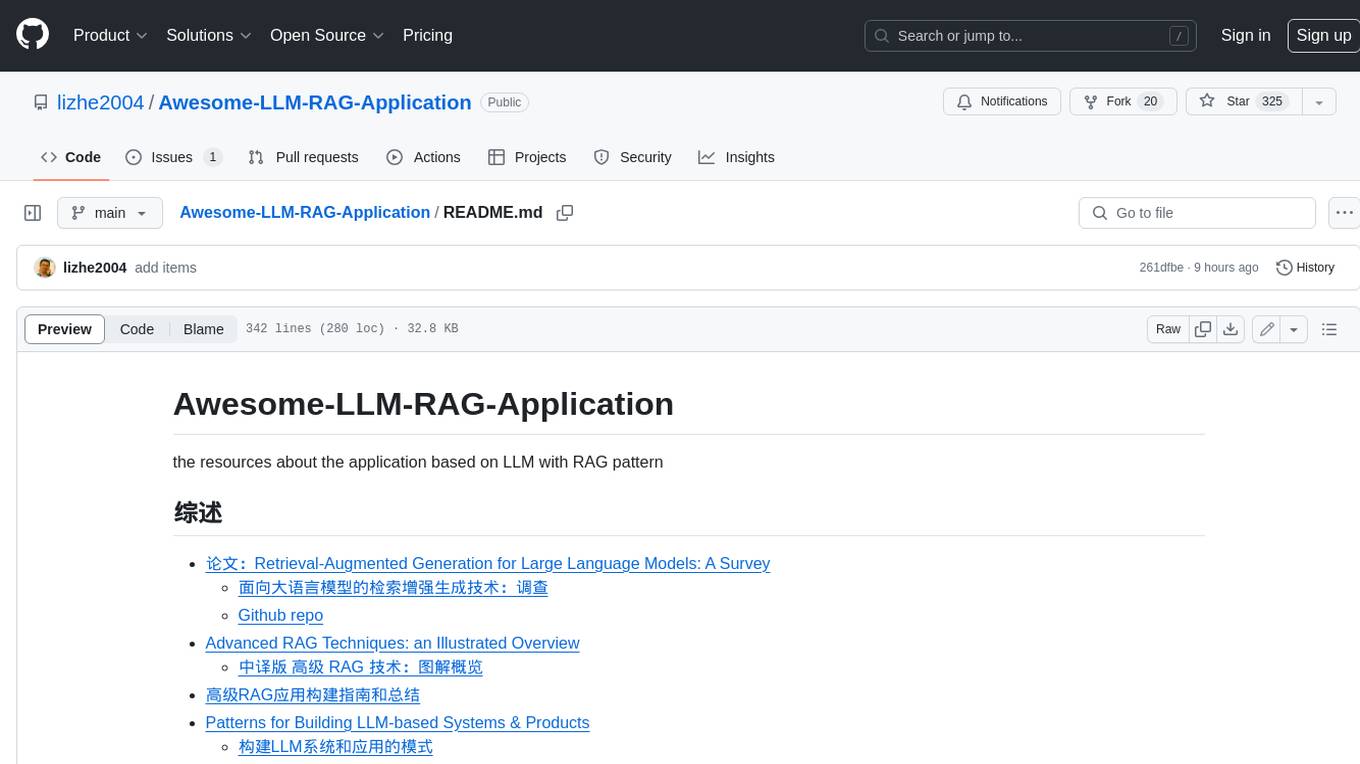
Awesome-LLM-RAG-Application
Awesome-LLM-RAG-Application is a repository that provides resources and information about applications based on Large Language Models (LLM) with Retrieval-Augmented Generation (RAG) pattern. It includes a survey paper, GitHub repo, and guides on advanced RAG techniques. The repository covers various aspects of RAG, including academic papers, evaluation benchmarks, downstream tasks, tools, and technologies. It also explores different frameworks, preprocessing tools, routing mechanisms, evaluation frameworks, embeddings, security guardrails, prompting tools, SQL enhancements, LLM deployment, observability tools, and more. The repository aims to offer comprehensive knowledge on RAG for readers interested in exploring and implementing LLM-based systems and products.
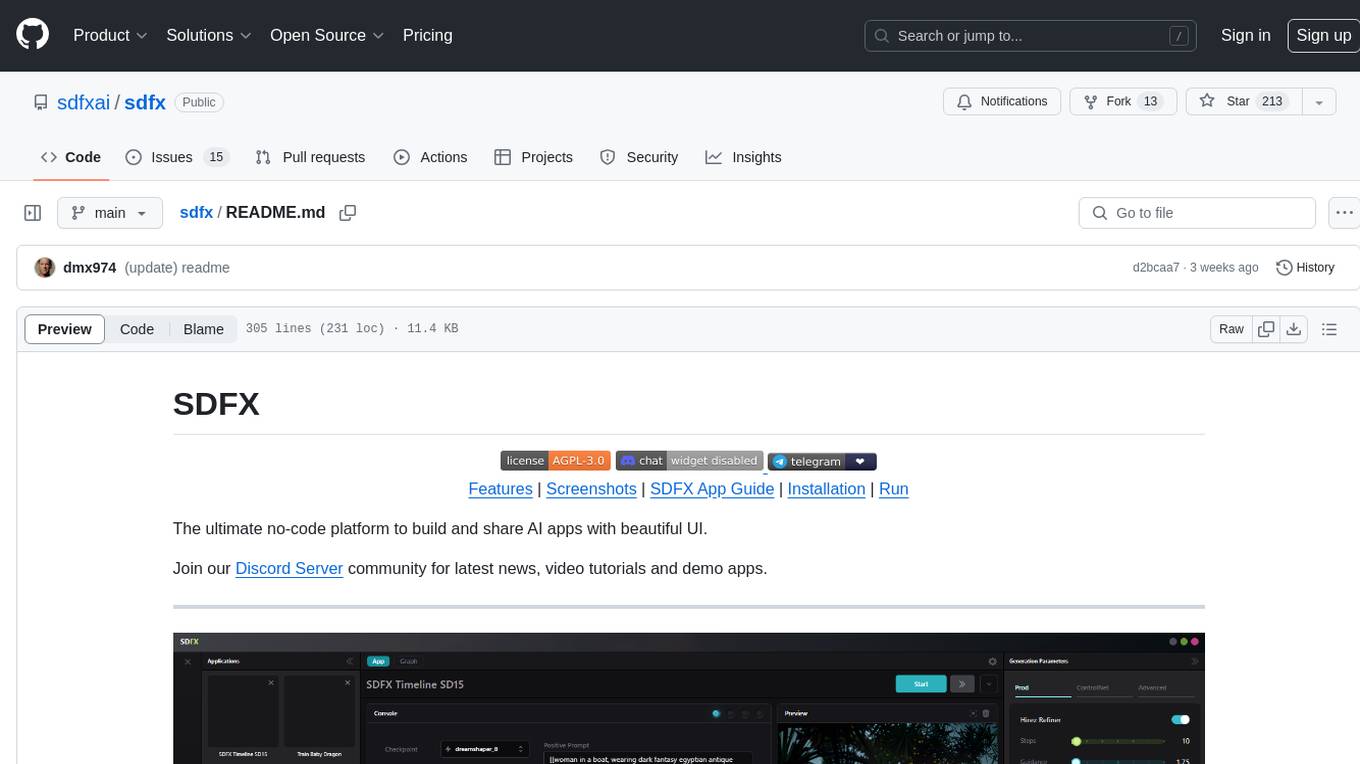
sdfx
SDFX is the ultimate no-code platform for building and sharing AI apps with beautiful UI. It enables the creation of user-friendly interfaces for complex workflows by combining Comfy workflow with a UI. The tool is designed to merge the benefits of form-based UI and graph-node based UI, allowing users to create intricate graphs with a high-level UI overlay. SDFX is fully compatible with ComfyUI, abstracting the need for installing ComfyUI. It offers features like animated graph navigation, node bookmarks, UI debugger, custom nodes manager, app and template export, image and mask editor, and more. The tool compiles as a native app or web app, making it easy to maintain and add new features.
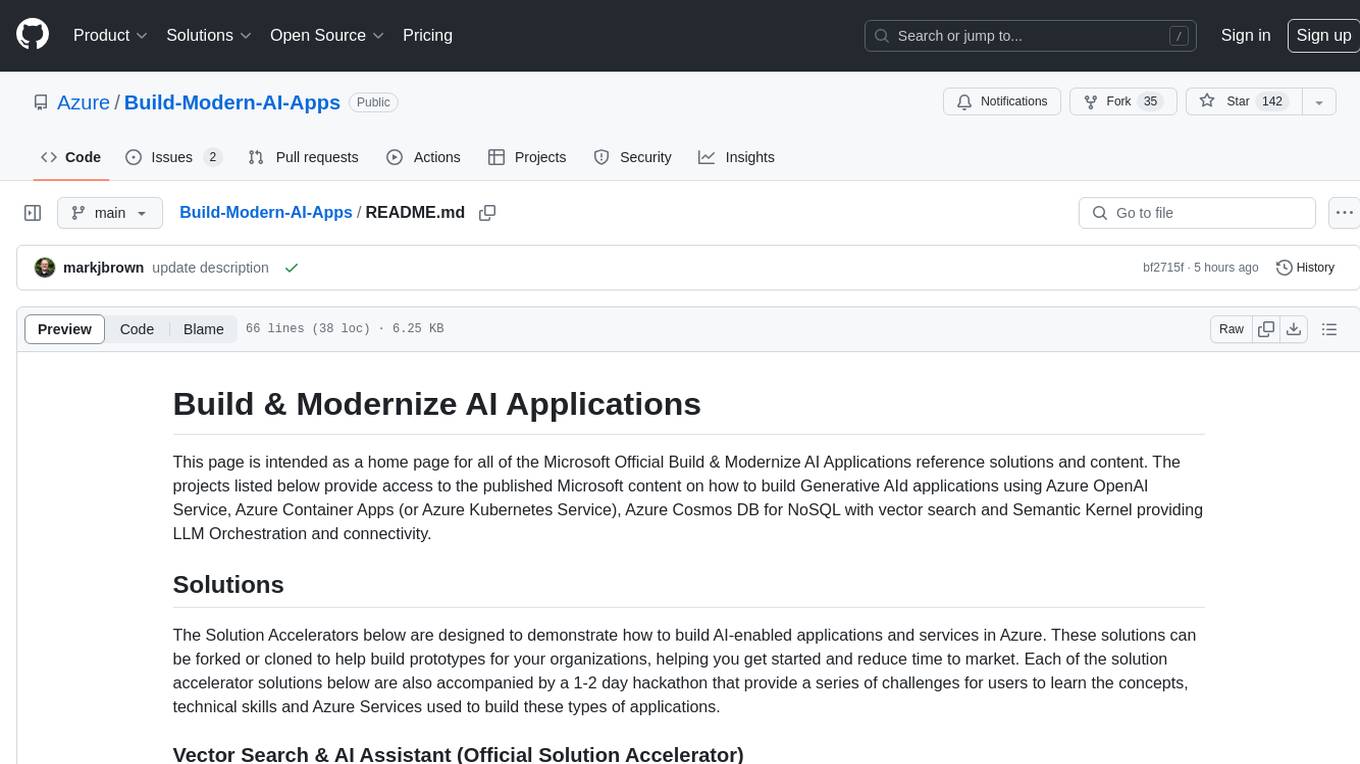
Build-Modern-AI-Apps
This repository serves as a hub for Microsoft Official Build & Modernize AI Applications reference solutions and content. It provides access to projects demonstrating how to build Generative AI applications using Azure services like Azure OpenAI, Azure Container Apps, Azure Kubernetes, and Azure Cosmos DB. The solutions include Vector Search & AI Assistant, Real-Time Payment and Transaction Processing, and Medical Claims Processing. Additionally, there are workshops like the Intelligent App Workshop for Microsoft Copilot Stack, focusing on infusing intelligence into traditional software systems using foundation models and design thinking.
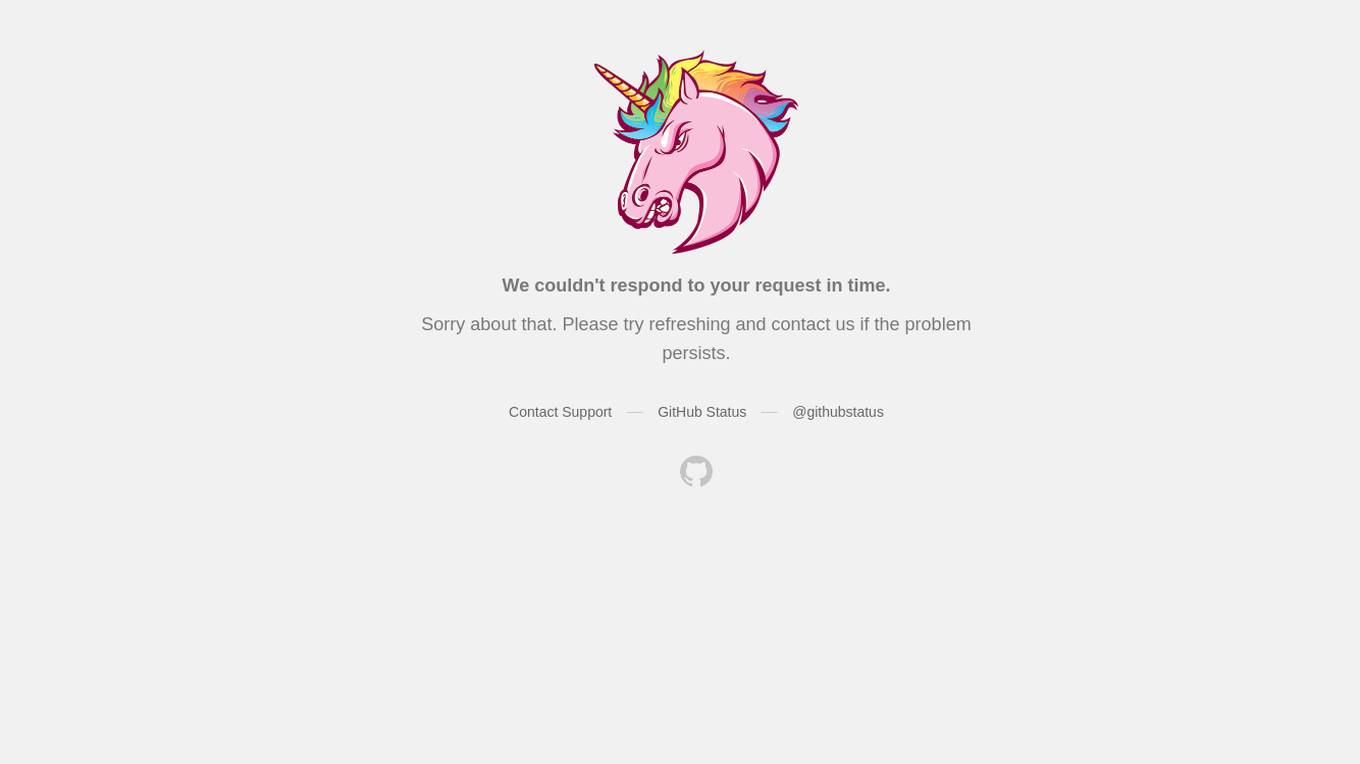
RAG_Hack
RAGHack is a hackathon focused on building AI applications using the power of RAG (Retrieval Augmented Generation). RAG combines large language models with search engine knowledge to provide contextually relevant answers. Participants can learn to build RAG apps on Azure AI using various languages and retrievers, explore frameworks like LangChain and Semantic Kernel, and leverage technologies such as agents and vision models. The hackathon features live streams, hack submissions, and prizes for innovative projects.
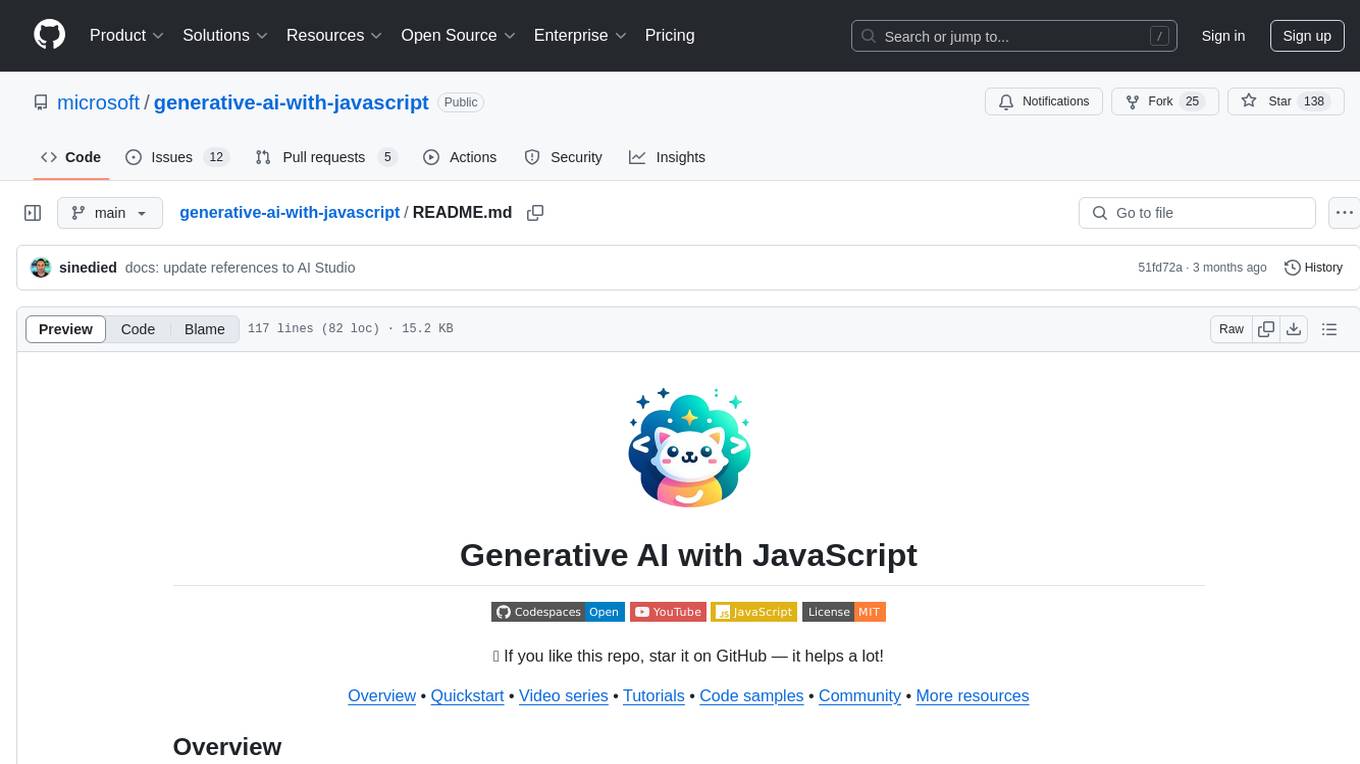
generative-ai-with-javascript
The 'Generative AI with JavaScript' repository is a comprehensive resource hub for JavaScript developers interested in delving into the world of Generative AI. It provides code samples, tutorials, and resources from a video series, offering best practices and tips to enhance AI skills. The repository covers the basics of generative AI, guides on building AI applications using JavaScript, from local development to deployment on Azure, and scaling AI models. It is a living repository with continuous updates, making it a valuable resource for both beginners and experienced developers looking to explore AI with JavaScript.
For similar jobs
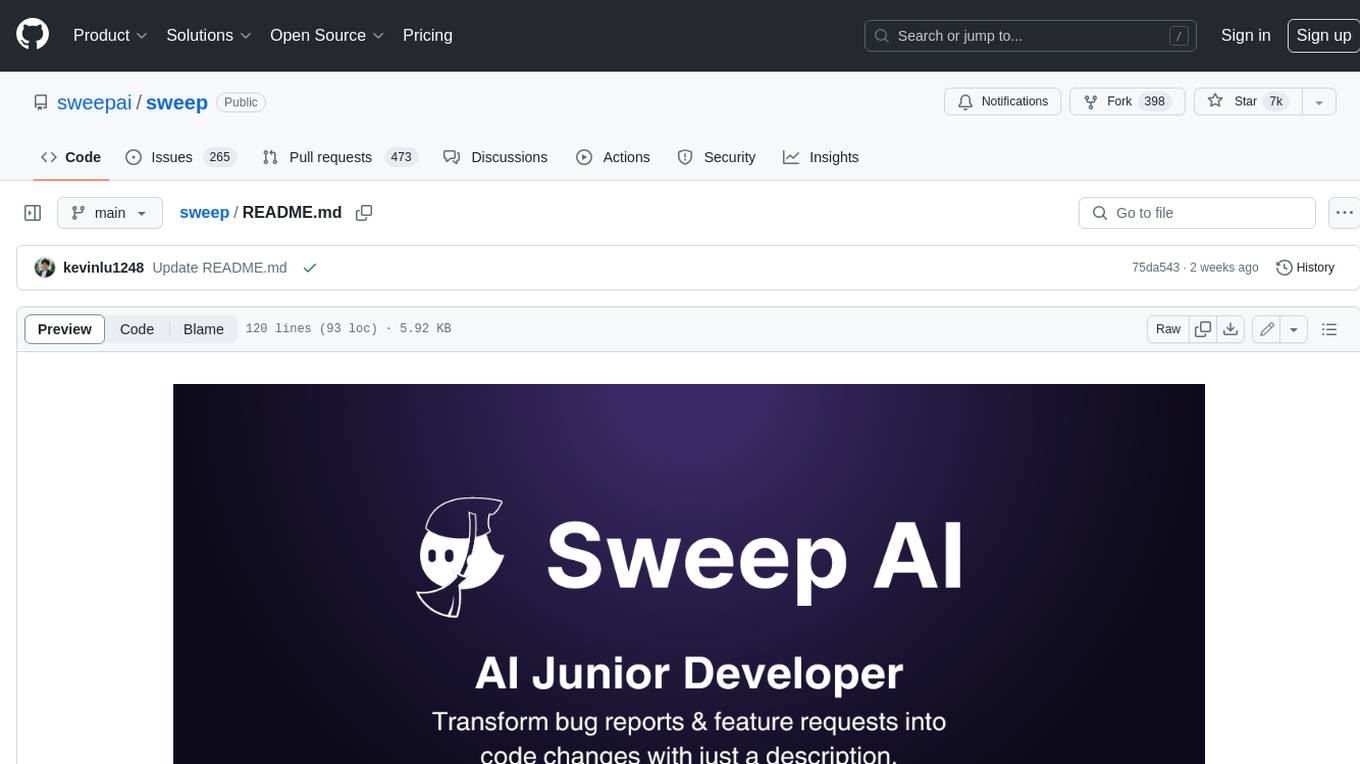
sweep
Sweep is an AI junior developer that turns bugs and feature requests into code changes. It automatically handles developer experience improvements like adding type hints and improving test coverage.
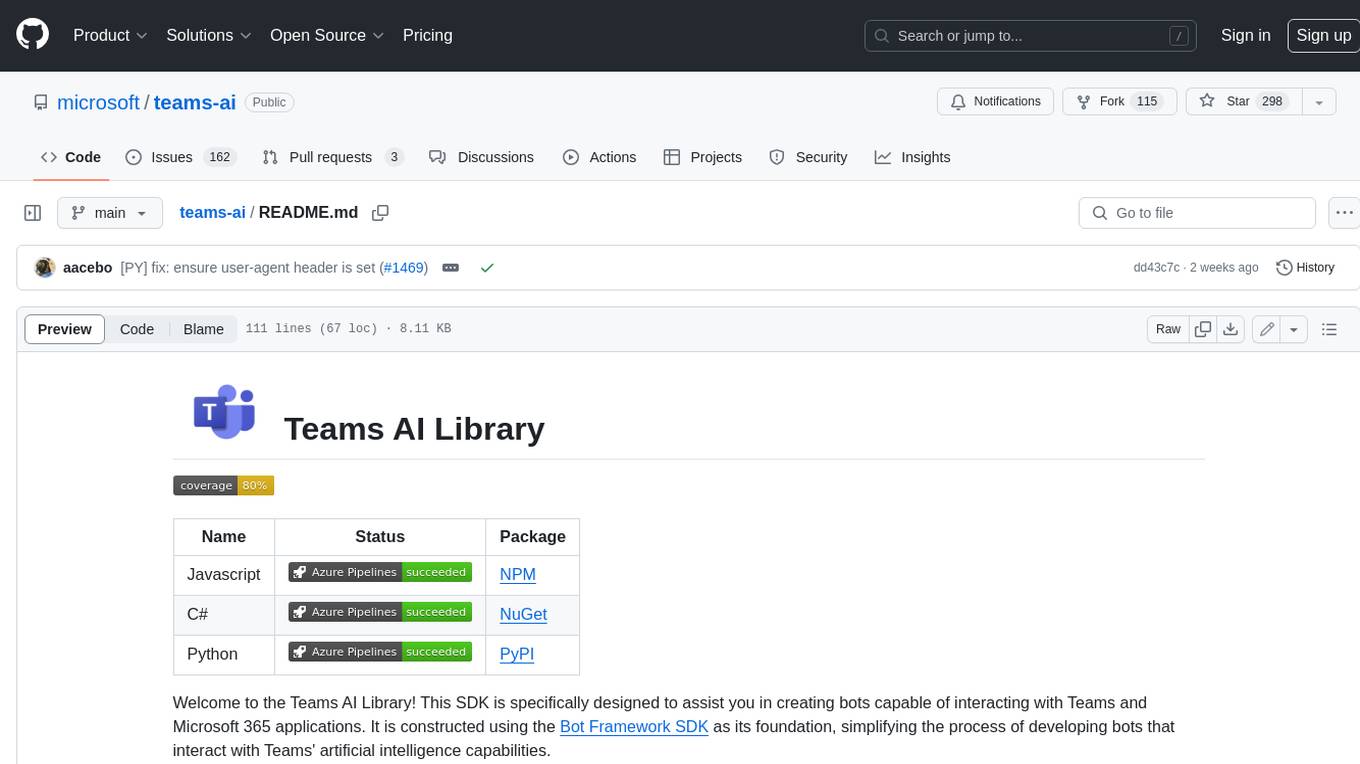
teams-ai
The Teams AI Library is a software development kit (SDK) that helps developers create bots that can interact with Teams and Microsoft 365 applications. It is built on top of the Bot Framework SDK and simplifies the process of developing bots that interact with Teams' artificial intelligence capabilities. The SDK is available for JavaScript/TypeScript, .NET, and Python.
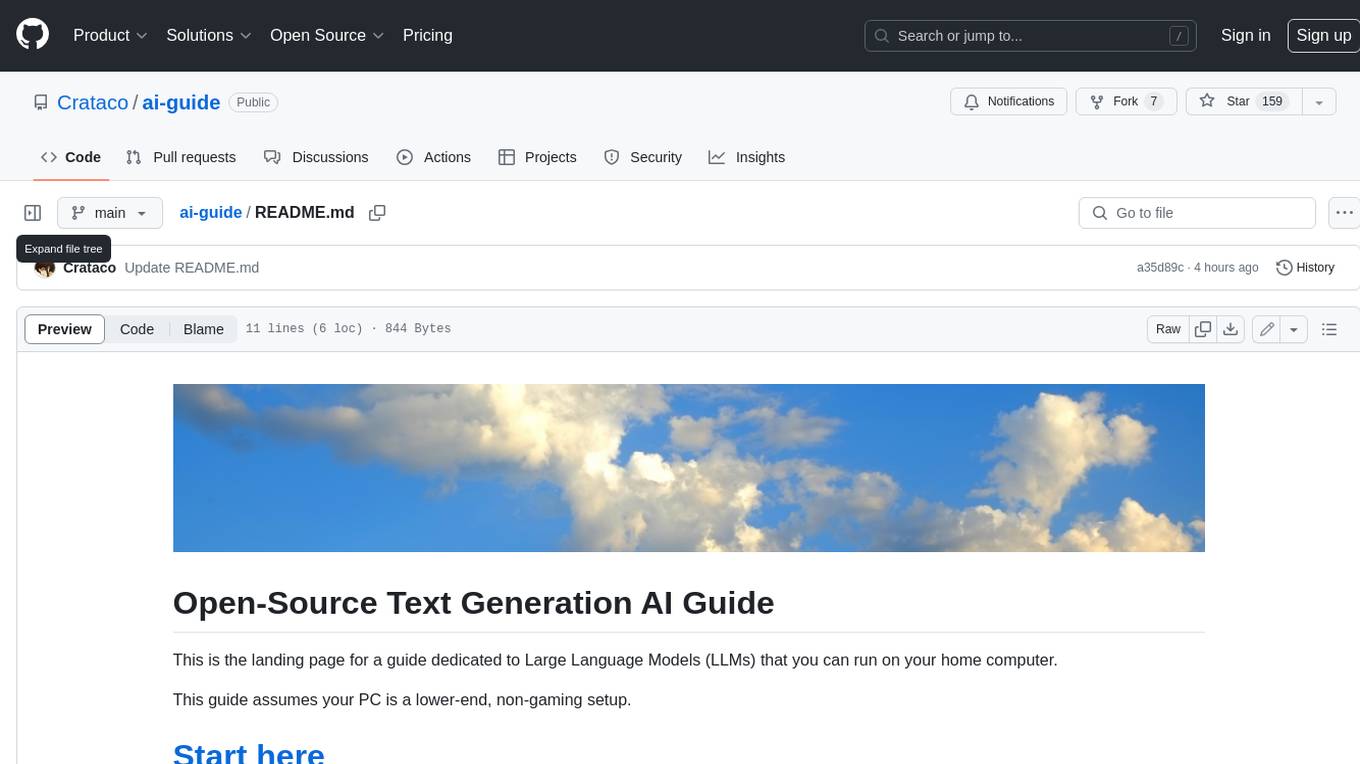
ai-guide
This guide is dedicated to Large Language Models (LLMs) that you can run on your home computer. It assumes your PC is a lower-end, non-gaming setup.
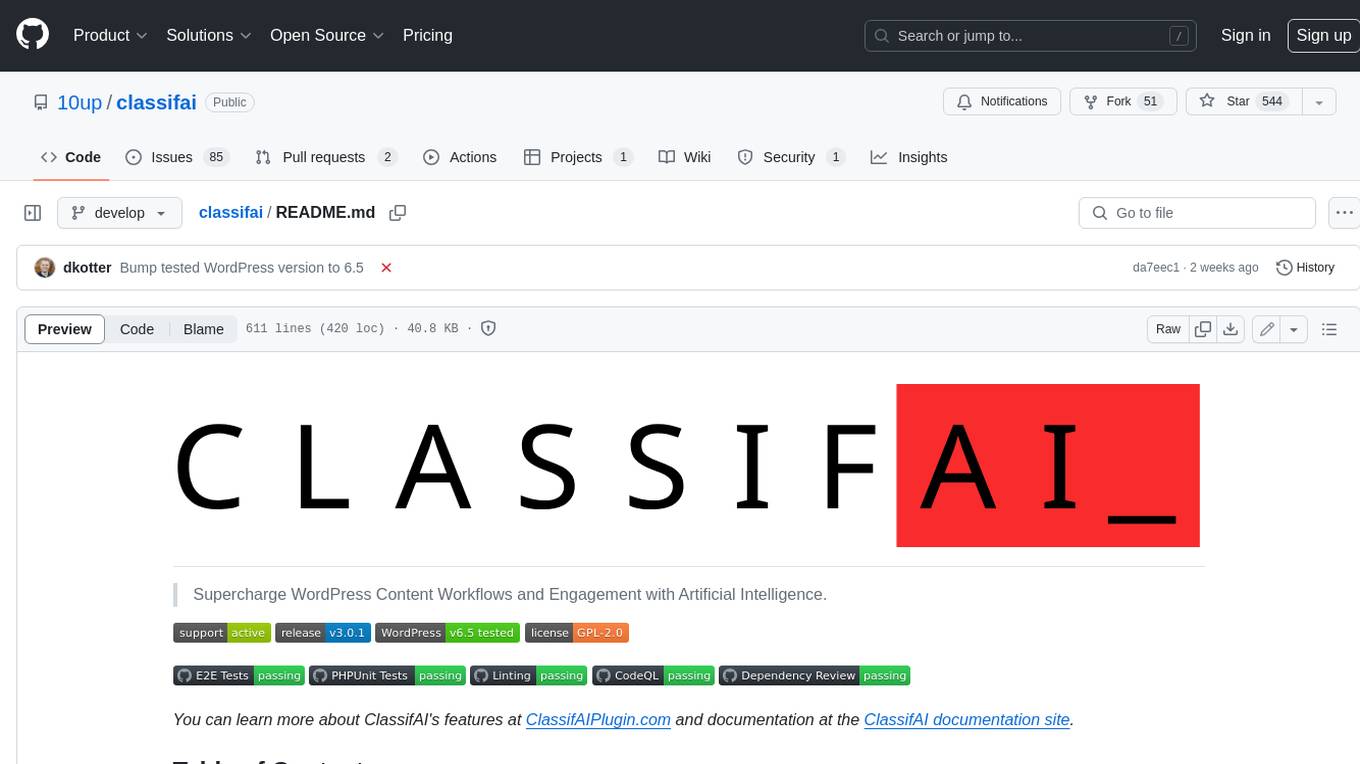
classifai
Supercharge WordPress Content Workflows and Engagement with Artificial Intelligence. Tap into leading cloud-based services like OpenAI, Microsoft Azure AI, Google Gemini and IBM Watson to augment your WordPress-powered websites. Publish content faster while improving SEO performance and increasing audience engagement. ClassifAI integrates Artificial Intelligence and Machine Learning technologies to lighten your workload and eliminate tedious tasks, giving you more time to create original content that matters.
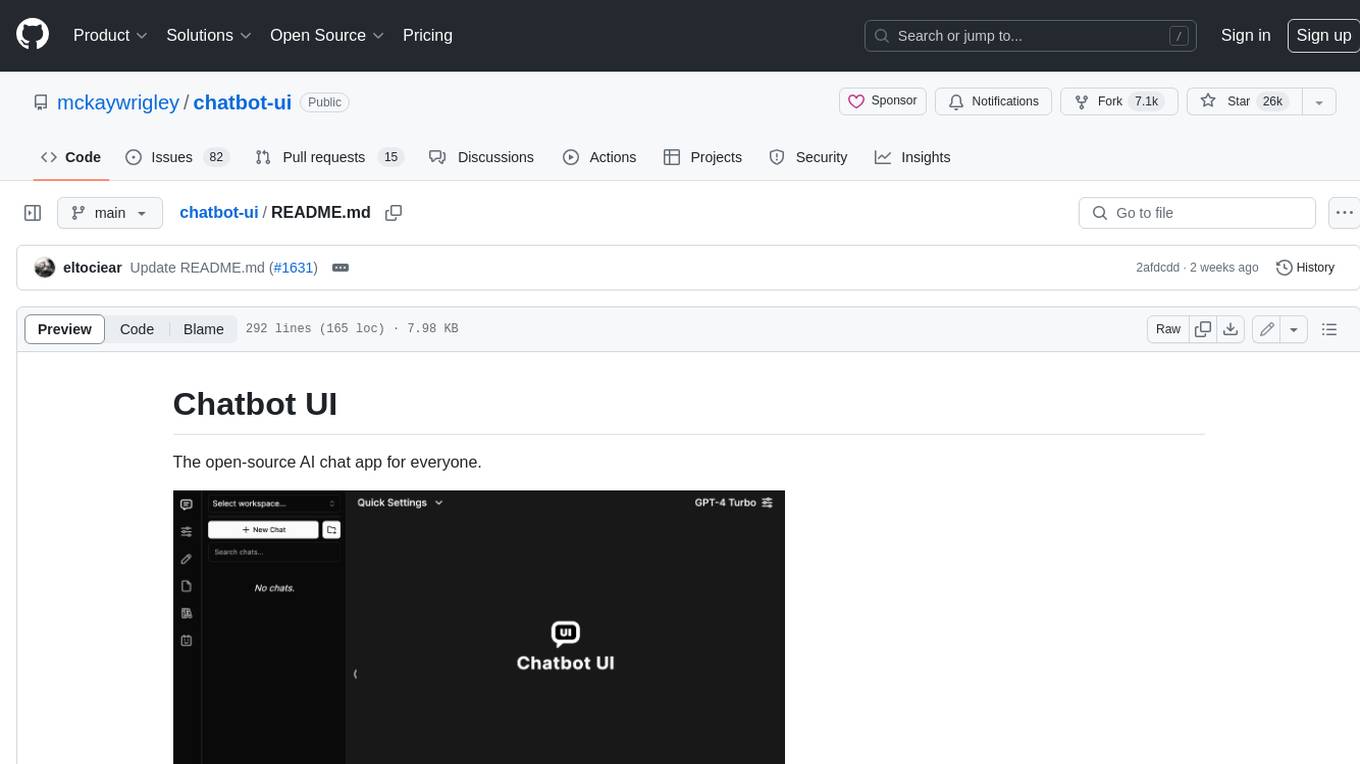
chatbot-ui
Chatbot UI is an open-source AI chat app that allows users to create and deploy their own AI chatbots. It is easy to use and can be customized to fit any need. Chatbot UI is perfect for businesses, developers, and anyone who wants to create a chatbot.
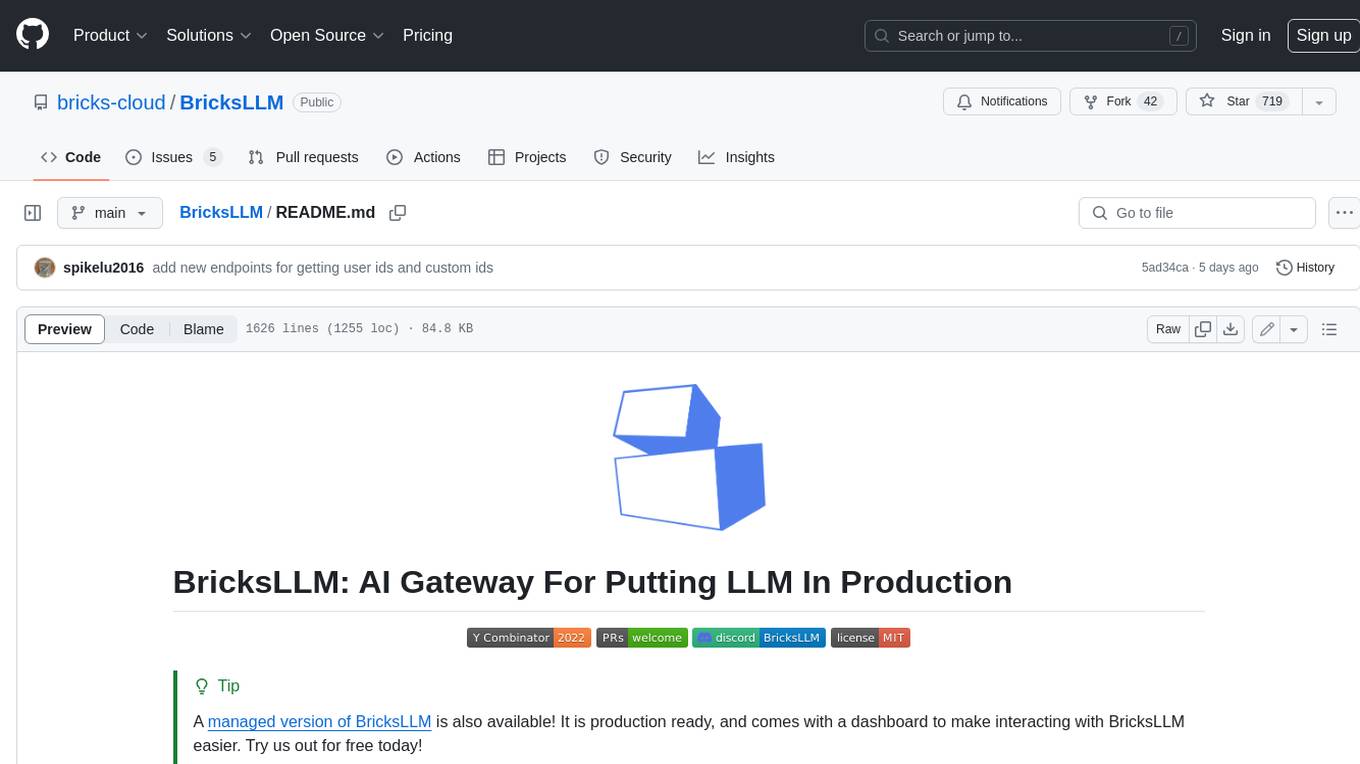
BricksLLM
BricksLLM is a cloud native AI gateway written in Go. Currently, it provides native support for OpenAI, Anthropic, Azure OpenAI and vLLM. BricksLLM aims to provide enterprise level infrastructure that can power any LLM production use cases. Here are some use cases for BricksLLM: * Set LLM usage limits for users on different pricing tiers * Track LLM usage on a per user and per organization basis * Block or redact requests containing PIIs * Improve LLM reliability with failovers, retries and caching * Distribute API keys with rate limits and cost limits for internal development/production use cases * Distribute API keys with rate limits and cost limits for students
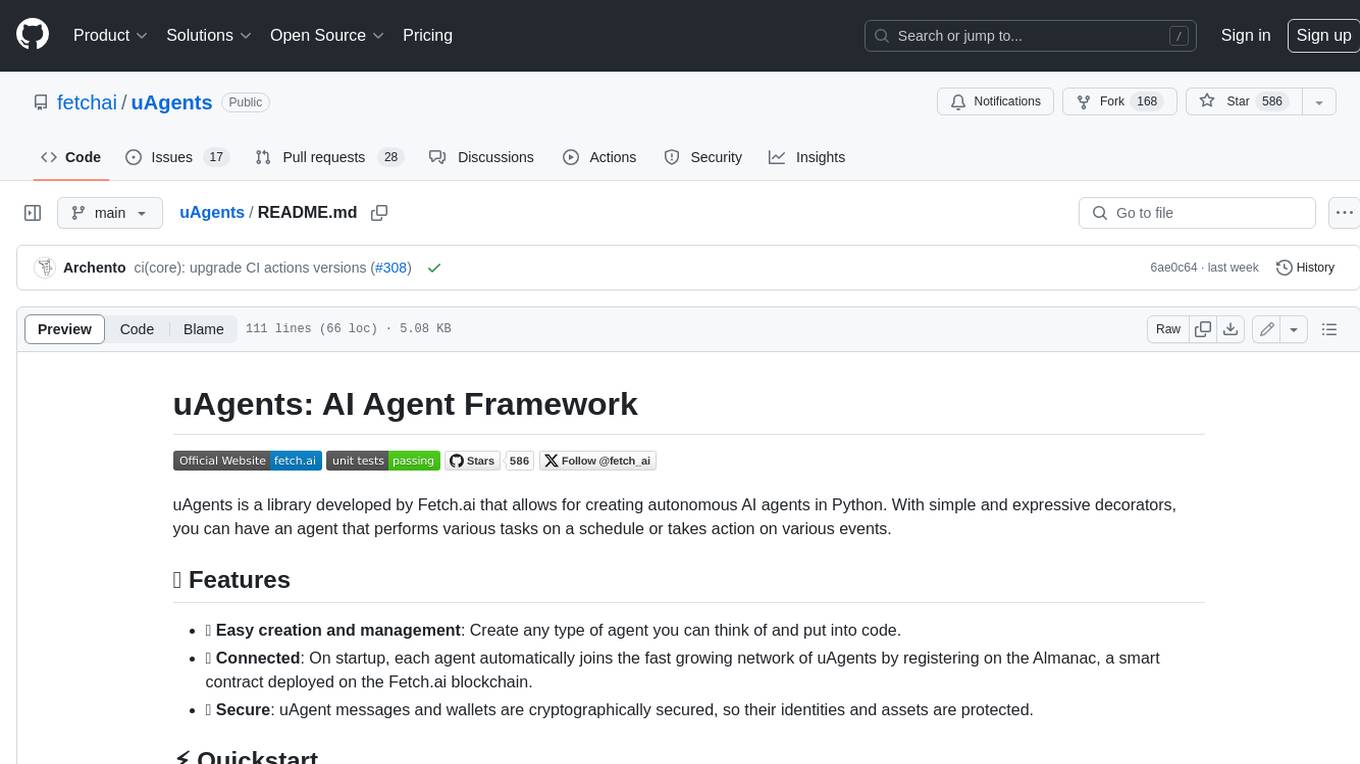
uAgents
uAgents is a Python library developed by Fetch.ai that allows for the creation of autonomous AI agents. These agents can perform various tasks on a schedule or take action on various events. uAgents are easy to create and manage, and they are connected to a fast-growing network of other uAgents. They are also secure, with cryptographically secured messages and wallets.
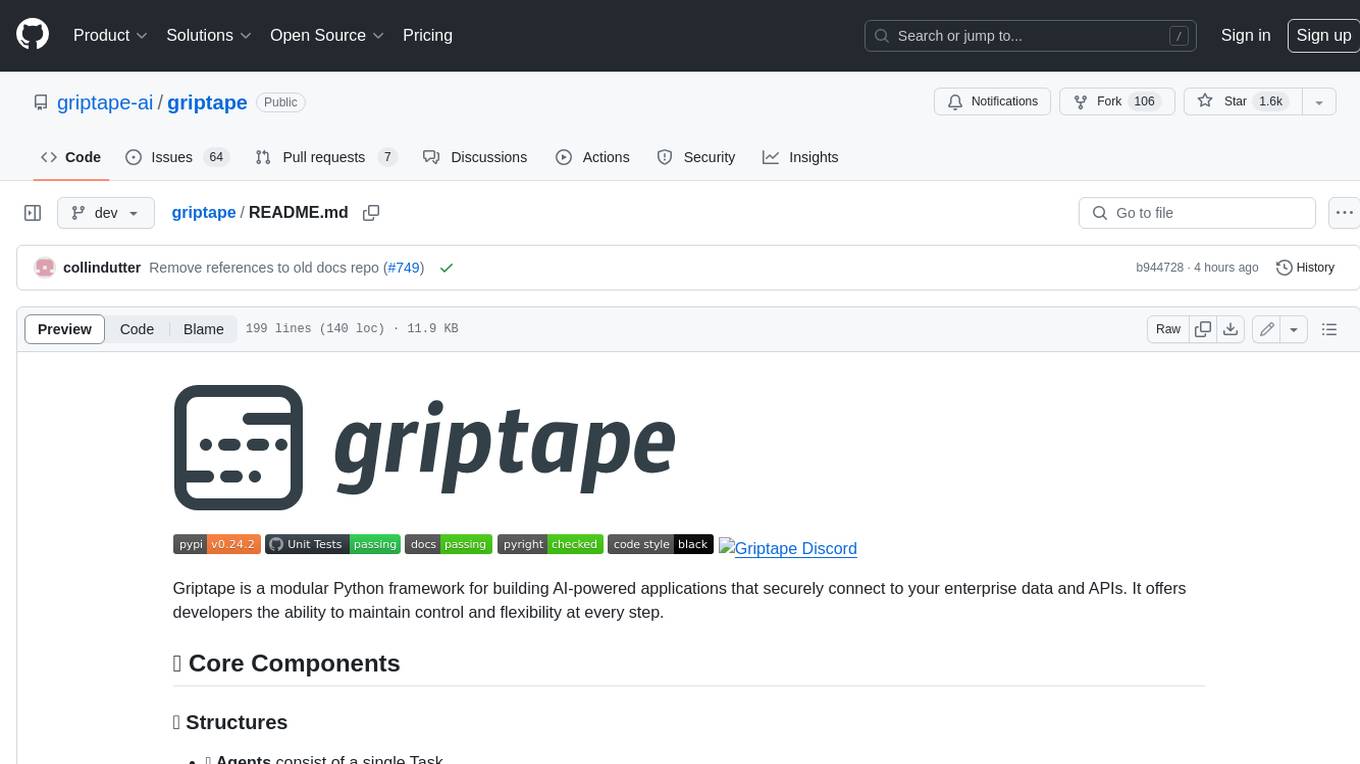
griptape
Griptape is a modular Python framework for building AI-powered applications that securely connect to your enterprise data and APIs. It offers developers the ability to maintain control and flexibility at every step. Griptape's core components include Structures (Agents, Pipelines, and Workflows), Tasks, Tools, Memory (Conversation Memory, Task Memory, and Meta Memory), Drivers (Prompt and Embedding Drivers, Vector Store Drivers, Image Generation Drivers, Image Query Drivers, SQL Drivers, Web Scraper Drivers, and Conversation Memory Drivers), Engines (Query Engines, Extraction Engines, Summary Engines, Image Generation Engines, and Image Query Engines), and additional components (Rulesets, Loaders, Artifacts, Chunkers, and Tokenizers). Griptape enables developers to create AI-powered applications with ease and efficiency.