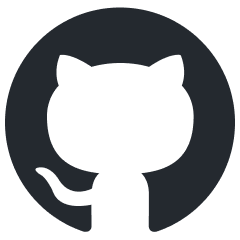
spark-ai-python
星火大模型 python sdk库
Stars: 197
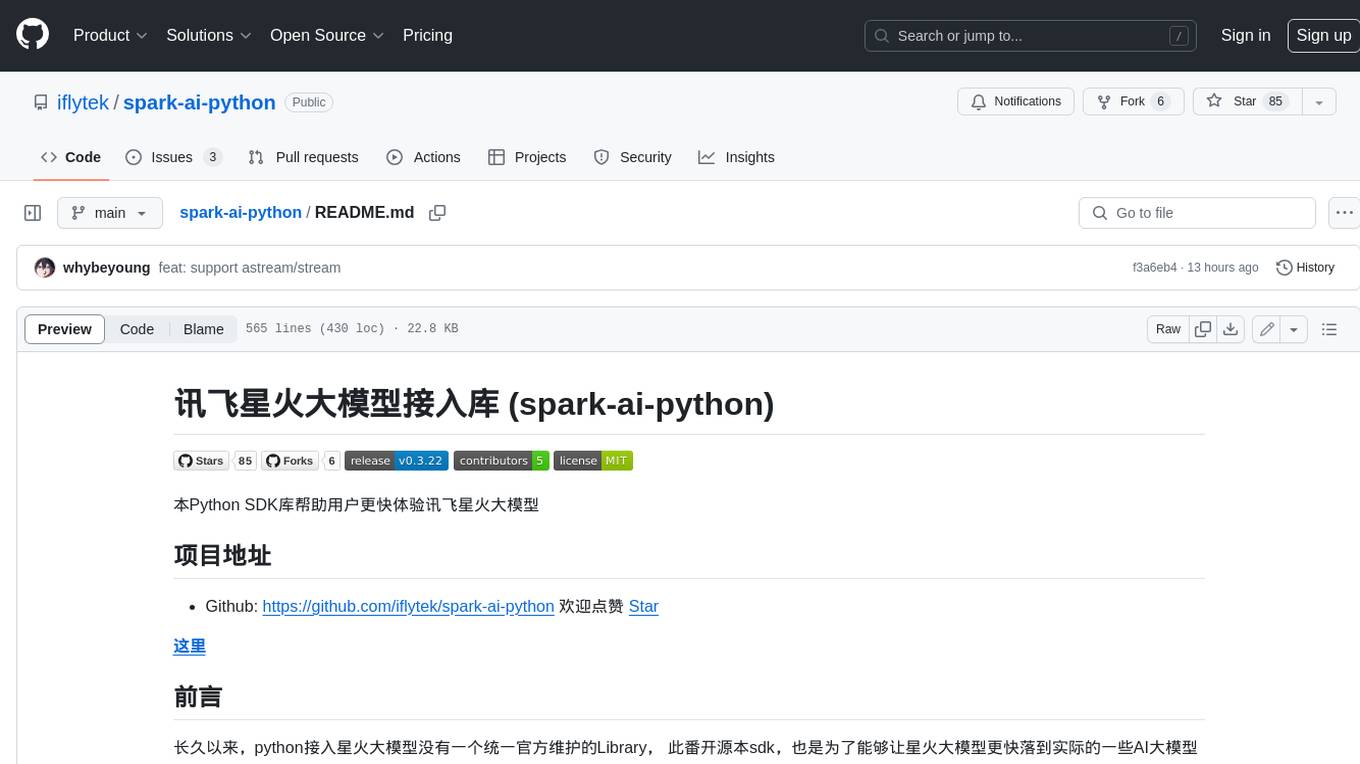
本Python SDK库帮助用户更快体验讯飞星火大模型
README:
本Python SDK库帮助用户更快体验讯飞星火大模型,更简单,更值得依赖
- Github: https://github.com/iflytek/spark-ai-python 欢迎点赞 Star
长久以来,python接入星火大模型没有一个统一官方维护的Library, 此番开源本sdk,也是为了能够让星火大模型更快落到实际的一些AI大模型应用相关的开发任务中去,简化python用户调用大模型成本。
目前基于Langchain的一些基础数据类型移植开发得到本项目,部分核心实现如有雷同,纯属"学习"! 感谢开源的力量,希望讯飞开源越做越好,星火大模型效果越来越好!。
本logo出自星火大模型
感谢社区(Langchain项目以及SparkLLM部分committer)[项目正在开发中]
- [x] 支持LLamaIndex,详细用法请参考 LLamIndex Support
- [x] 支持AutoGen,详细用法请参考 AutoGen Support
- [x] 支持星火图片理解大模型,详细用法请参考 ImageUnderstanding Support
- [x] 开源框架AutoGPT/AutoGen/MetaGpt/Langchain/PromptFlow/.... 快速集成星火示例
- [x] 极简的接入,快速调用讯飞星火大模型
- [x] 已发布pypi [国内源均可安装]
- [x] 本地代理方式星火SparkAPI转OpenAI接口(让你快速在开源agent框架集成星火大模型)
- [x] 无缝对接讯飞Maas平台微调训练托管的大模型API
- [ ] SDK方式适配OpenAI接口 ChatCompletion接口
- [x] SDK方式适配OpenAI Embedding接口 实验性支持
- [ ] 支持 HTTP SPARK API
- [x] 支持大模型多模态等能力 (目前已支持图片理解大模型)
- [ ] Golang版本SDK进行中
- [ ] 对接 liteLLM
- [x] 新增 stream接口支持
项目仅支持 Python3.8+
如果你不需要源码,只需要通过 pip
快速安装
pip install --upgrade spark_ai_python
国内使用:
pip install -i https://pypi.tuna.tsinghua.edu.cn/simple spark_ai_python
如果清华源版本不可用,请使用一下命令升级到最新版本:
pip install -i https://repo.model.xfyun.cn/api/packages/administrator/pypi/simple spark_ai_python --upgrade
Install from source with:
pip install -e .
-
前置条件 需要在 xfyun.cn 申请有权限的
- app_id
- api_key
- api_secret
-
URL/Domain配置请查看doc
-
运行测试脚本需要提前将 .env.example 拷贝为
.env
并配置其中变量
tests/examples/llm_test.py
import os
from sparkai.llm.llm import ChatSparkLLM, ChunkPrintHandler
from sparkai.core.messages import ChatMessage
try:
from dotenv import load_dotenv
except ImportError:
raise RuntimeError('Python environment for SPARK AI is not completely set up: required package "python-dotenv" is missing.') from None
load_dotenv()
if __name__ == '__main__':
from sparkai.core.callbacks import StdOutCallbackHandler
spark = ChatSparkLLM(
spark_api_url=os.environ["SPARKAI_URL"],
spark_app_id=os.environ["SPARKAI_APP_ID"],
spark_api_key=os.environ["SPARKAI_API_KEY"],
spark_api_secret=os.environ["SPARKAI_API_SECRET"],
spark_llm_domain=os.environ["SPARKAI_DOMAIN"],
streaming=False,
)
messages = [ChatMessage(
role="user",
content='你好呀'
)]
handler = ChunkPrintHandler()
a = spark.generate([messages], callbacks=[handler])
print(a)
print(a.generations[0][0].text)
print(a.llm_output)
注意当streaming
设置为 False
的时候, callbacks 并不起作用。
tests/examples/llm_test.py
import os
from sparkai.llm.llm import ChatSparkLLM, ChunkPrintHandler
from sparkai.core.messages import ChatMessage
try:
from dotenv import load_dotenv
except ImportError:
raise RuntimeError('Python environment for SPARK AI is not completely set up: required package "python-dotenv" is missing.') from None
load_dotenv()
def test_stream():
from sparkai.core.callbacks import StdOutCallbackHandler
spark = ChatSparkLLM(
spark_api_url=os.environ["SPARKAI_URL"],
spark_app_id=os.environ["SPARKAI_APP_ID"],
spark_api_key=os.environ["SPARKAI_API_KEY"],
spark_api_secret=os.environ["SPARKAI_API_SECRET"],
spark_llm_domain=os.environ["SPARKAI_DOMAIN"],
request_timeout=30, #
streaming=True,
)
messages = [ChatMessage(
role="user",
content='作为AutoSpark的创建者角色,当前需要你帮我分析并生成任务,你当前主要目标是:\n1. 帮我写个贪吃蛇python游戏\n\n\n\n\n当前执行任务节点是: `编写贪吃蛇游戏的界面设计`\n\n任务执行历史是:\n`\nTask: 使用ThinkingTool分析贪吃蛇游戏的需求\nResult: Error2: {\'error\': \'Could not parse invalid format: 根据任务节点,我将使用ThinkingTool来分析贪吃蛇游戏的需求。首先,我们需要理解游戏的基本功能和规则。贪吃蛇游戏的主要目标是控制一条蛇在屏幕上移动,吃到食物后蛇会变长,碰到自己的身体或者屏幕边缘则游戏结束。\\n\\n接下来,我们可以使用CodingTool来编写贪吃蛇游戏的代码。首先,我们需要定义以下核心类和方法:\\n\\n1. Snake类:用于表示贪吃蛇的状态,包括蛇的身体、移动方向等。\\n2. Food类:用于表示食物的位置。\\n3. Game类:用于控制游戏的进行,包括初始化游戏、更新蛇的位置、检查碰撞等。\\n4. main函数:用于启动游戏。\\n\\n接下来,我们将这些类和方法的代码写入文件中。\\n\\n最后,我们可以使用WriteTestTool来编写测试用例,确保我们的代码能够正确地运行。测试用例应该包括以下内容:\\n\\n1. 测试游戏是否能正确初始化。\\n2. 测试蛇是否能正确移动。\\n3. 测试蛇是否能正确吃到食物并变长。\\n4. 测试蛇是否能正确碰到自己的身体或屏幕边缘导致游戏结束。 exceptionNot get command from llm response...\'}. \nTask: 编写贪吃蛇游戏的spec文件\nResult: Error2: {\'error\': \'Could not parse invalid format: 根据任务节点,我将使用`WriteSpecTool`来编写贪吃蛇游戏的spec文件。\\n\\n首先,我们需要定义以下核心类和方法:\\n1. Snake类:用于表示贪吃蛇的状态,包括蛇的身体、移动方向等。\\n2. Food类:用于表示食物的位置。\\n3. Game类:用于控制游戏的进行,包括初始化游戏、更新蛇的位置、检查碰撞等。\\n4. main函数:用于启动游戏。\\n\\n接下来,我们将这些类和方法的代码写入文件中。\\n\\n最后,我们可以使用`WriteTestTool`来编写测试用例,确保我们的代码能够正确地运行。测试用例应该包括以下内容:\\n1. 测试游戏是否能正确初始化。\\n2. 测试蛇是否能正确移动。\\n3. 测试蛇是否能正确吃到食物并变长。\\n4. 测试蛇是否能正确碰到自己的身体或屏幕边缘导致游戏结束。 exceptionNot get command from llm response...\'}. \n\n`\n\n根据上述背景信息,你的任务是需要理解当前的任务节点关键信息,创建一个规划,解释为什么要这么做,并且提及一些需要注意的事项,必须从下述TOOLS中挑选一个命令用于下一步执行。\n\nTOOLS:\n1. "ThinkingTool": Intelligent problem-solving assistant that comprehends tasks, identifies key variables, and makes efficient decisions, all while providing detailed, self-driven reasoning for its choices. Do not assume anything, take the details from given data only., args : task_description: "<task_description>",\n2. "WriteSpecTool": A tool to write the spec of a program., args : task_description: "<task_description>",spec_file_name: "<spec_file_name>",\n3. "CodingTool": You will get instructions for code to write. You will write a very long answer. Make sure that every detail of the architecture is, in the end, implemented as code. Think step by step and reason yourself to the right decisions to make sure we get it right. You will first lay out the names of the core classes, functions, methods that will be necessary, as well as a quick comment on their purpose. Then you will output the content of each file including ALL code., args : code_description: "<code_description>",\n4. "WriteTestTool": 您是一位超级聪明的开发人员,使用测试驱动开发根据规范编写测试。\n请根据上述规范生成测试。测试应该尽可能简单, 但仍然涵盖了所有功能。\n将它们写入文件中, args : test_description: "<test_description>",test_file_name: "<test_file_name>",\n\n\n\n约束条件:\n1. 请注意返回的命令名称和参数不要被引号包裹\n2. 命令名称必须是TOOLS中的已知的\n3. 你只能生成一个待执行命令名称及其对应参数\n4. 你生成的命令必须是用来解决 `编写贪吃蛇游戏的界面设计`\n\n在之后的每次回答中,你必须严格遵从上述约束条件并按照如下JsonSchema约束返回响应:\n\n{\n "$schema": "http://json-schema.org/draft-07/schema#",\n "type": "object",\n "properties": {\n "thoughts": {\n "type": "object",\n "properties": {\n "reasoning": {\n "type": "string",\n "description": "short reasoning",\n }\n },\n "required": ["reasoning"]\n },\n "tool": {\n "type": "object",\n "properties": {\n "name": {\n "type": "string",\n "description": "tool name",\n },\n "args": {\n "type": "object",\n "description": "tool arguments",\n }\n },\n "required": ["name", "args"]\n }\n }\n}'
)]
handler = ChunkPrintHandler()
a = spark.generate([messages], callbacks=[handler])
print(a)
其中 ChunkPrintHandler
为回调类,可以在回调类处理流式响应的chunk,
该类简单实现如下:
class ChunkPrintHandler(BaseCallbackHandler):
"""Callback Handler that prints to std out."""
def __init__(self, color: Optional[str] = None) -> None:
"""Initialize callback handler."""
self.color = color
def on_llm_new_token(self, token: str,
*,
chunk: None,
**kwargs: Any,):
print(token)
print(kwargs)
# token 为模型生成的token
# 可以check kwargs内容,kwargs中 llm_output中有usage相关信息, final表示是否是最后一帧
上述在 on_llm_new_token 实现您的流式处理逻辑,如需定制流式处理逻辑,请参考上述实现,继承: BaseCallbackHandler
直接遍历生成器(同步stream接口):
def test_starcoder2():
from sparkai.log.logger import logger
#logger.setLevel("debug")
from sparkai.core.callbacks import StdOutCallbackHandler
messages = [{'role': 'user',
'content': "帮我生成一段代码,爬取baidu.com"}]
spark = ChatSparkLLM(
spark_api_url="wss://xingchen-api.cn-huabei-1.xf-yun.com/v1.1/chat",
spark_app_id=os.environ["SPARKAI_APP_ID"],
spark_api_key=os.environ["SPARKAI_API_KEY"],
spark_api_secret=os.environ["SPARKAI_API_SECRET"],
spark_llm_domain="xsstarcoder27binst",
streaming=True,
max_tokens= 1024,
)
messages = [
ChatMessage(
role="user",
content=messages[0]['content']
)]
a = spark.stream(messages)
for message in a:
print(message)
或者是异步astream接口:
async def test_astream():
from sparkai.log.logger import logger
#logger.setLevel("debug")
from sparkai.core.callbacks import StdOutCallbackHandler
messages = [{'role': 'user',
'content': "帮我生成一段代码,爬取baidu.com"}]
spark = ChatSparkLLM(
spark_api_url="wss://xingchen-api.cn-huabei-1.xf-yun.com/v1.1/chat",
spark_app_id=os.environ["SPARKAI_APP_ID"],
spark_api_key=os.environ["SPARKAI_API_KEY"],
spark_api_secret=os.environ["SPARKAI_API_SECRET"],
spark_llm_domain="xsstarcoder27binst",
streaming=True,
max_tokens= 1024,
)
messages = [
ChatMessage(
role="user",
content=messages[0]['content']
)]
handler = AsyncChunkPrintHandler()
a = spark.astream(messages, config={"callbacks": [handler]})
async for message in a:
print(message)
if __name__ == '__main__':
import asyncio
asyncio.run(test_astream())
比如将 mulitply 乘法函数定义传入 ChatSparkLLM
from sparkai.core.utils.function_calling import convert_to_openai_tool, convert_to_openai_function
def multiply(a,b :int) -> int:
"""你是一个乘法计算器,可以帮我计算两个数的乘积,例如:计算1乘1等于几或计算1*1等于几
Args:
a: 输入a
b: 输入b
Return:
返回 a*b 结果
"""
print("hello success")
return a*b
def test_function_call():
from sparkai.core.callbacks import StdOutCallbackHandler
messages = [{'role': 'user',
'content': "帮我算下 12乘以12"}]
spark = ChatSparkLLM(
spark_api_url=os.environ["SPARKAI_URL"],
spark_app_id=os.environ["SPARKAI_APP_ID"],
spark_api_key=os.environ["SPARKAI_API_KEY"],
spark_api_secret=os.environ["SPARKAI_API_SECRET"],
spark_llm_domain=os.environ["SPARKAI_DOMAIN"],
streaming=False,
)
function_definition =[convert_to_openai_function(multiply)]
print(json.dumps(convert_to_openai_tool(multiply),ensure_ascii=False))
messages = [ChatMessage(
role="user",
content=messages[0]['content']
)]
handler = ChunkPrintHandler()
a = spark.generate([messages], callbacks=[handler],function_definition=function_definition)
print(a)
print(a.generations[0][0].text)
print(a.llm_output)
得到输出
PASSED [100%]{"type": "function", "function": {"name": "multiply", "description": "乘法函数,\nArgs:\n a: 输入a\n b: 输入b\nReturn:\n 返回 a*b 结果", "parameters": {"type": "object", "properties": {"b": {"type": "integer"}}, "required": ["a", "b"]}}}
generations=[[ChatGeneration(message=FunctionCallMessage(content='', function_call={'arguments': '{"a":12,"b":12}', 'name': 'multiply'}))]] llm_output={'token_usage': {'question_tokens': 9, 'prompt_tokens': 9, 'completion_tokens': 0, 'total_tokens': 9}} run=[RunInfo(run_id=UUID('95bf4e2e-6c90-41aa-9ddf-51b707d4d3c7'))]
generations=[[ChatGeneration(message=FunctionCallMessage(content='', function_call={'arguments': '{"a":12,"b":12}', 'name': 'operator_multiply'}))]] llm_output={'token_usage': {'question_tokens': 9, 'prompt_tokens': 9, 'completion_tokens': 0, 'total_tokens': 9}} run=[RunInfo(run_id=UUID('64bb65bd-948b-4354-bb72-e6847cc0a21b'))]
{'token_usage': {'question_tokens': 9, 'prompt_tokens': 9, 'completion_tokens': 0, 'total_tokens': 9}}
仅供用于调试或者应用于三方框架快速集成星火
python -m sparkai.spark_proxy.main
运行后如下:
INFO: Started server process [57295]
INFO: Waiting for application startup.
INFO: Application startup complete.
INFO: Uvicorn running on http://0.0.0.0:8008 (Press CTRL+C to quit)
之后再需要配置OPENAI
配置上述 本地url 和 星火 key&secret&appid"
组成的key即可以openai接口形式调用星火大模型
- open_api_key: 配置格式key为:
key&secret&appid"
格式的key - openai_base_url: 你本地 ip:8008端口
具体操作流程参见本地代理方式星火SparkAPI转OpenAI接口
Before using chroma vector store, please install it by running pip install llama-index-vector-stores-chroma.### 省略其他代码
from sparkai.embedding.sparkai_base import SparkAiEmbeddingModel
from llama_index.core import VectorStoreIndex, SimpleDirectoryReader
from llama_index.vector_stores.chroma import ChromaVectorStore
from llama_index.core import StorageContext
import chromadb
import os
from sparkai.embedding.spark_embedding import SparkEmbeddingFunction
from llama_index.core import VectorStoreIndex, SimpleDirectoryReader, Settings
from sparkai.frameworks.llama_index import SparkAI
try:
from dotenv import load_dotenv
except ImportError:
raise RuntimeError(
'Python environment for SPARK AI is not completely set up: required package "python-dotenv" is missing.') from None
load_dotenv()
def llama_query():
chroma_client = chromadb.Client()
chroma_collection = chroma_client.get_or_create_collection(name="spark")
# define embedding function
embed_model = SparkAiEmbeddingModel(spark_embedding_app_id=os.environ['SPARK_Embedding_APP_ID'],
spark_embedding_api_key=os.environ['SPARK_Embedding_API_KEY'],
spark_embedding_api_secret=os.environ['SPARK_Embedding_API_SECRET'],
spark_embedding_domain=os.environ['SPARKAI_Embedding_DOMAIN'],
QPS=2)
# define LLM Model
sparkai = SparkAI(
spark_api_url=os.environ["SPARKAI_URL"],
spark_app_id=os.environ["SPARKAI_APP_ID"],
spark_api_key=os.environ["SPARKAI_API_KEY"],
spark_api_secret=os.environ["SPARKAI_API_SECRET"],
spark_llm_domain=os.environ["SPARKAI_DOMAIN"],
streaming=False,
)
# load documents
# Invoke-WebRequest -Uri 'https://raw.githubusercontent.com/run-llama/llama_index/main/docs/docs/examples/data/paul_graham/paul_graham_essay.txt' -OutFile 'data\paul_graham\paul_graham_essay.txt'
documents = SimpleDirectoryReader("D:\data\paul_graham").load_data()
# set up ChromaVectorStore and load in data
vector_store = ChromaVectorStore(chroma_collection=chroma_collection)
storage_context = StorageContext.from_defaults(vector_store=vector_store)
index = VectorStoreIndex.from_documents(documents, storage_context=storage_context, embed_model=embed_model)
# query
query_engine = index.as_query_engine(llm=sparkai, similarity_top_k=2)
response = query_engine.query("What did the author do growing up?")
print(response)
if __name__ == "__main__":
llama_query()
- 需配置embedding model和LLM model两部分,SPARKAI_Embedding_DOMAIN为query或para。具体详见https://www.xfyun.cn/doc/spark/Embedding_api.html#_3-%E8%AF%B7%E6%B1%82
- embedding功能申请授权请参考:https://www.xfyun.cn/services/embedding
示例结果如下:
微软出品的AutoGen是业界出名的多Agent智能体框架。 通过几行Import即可让autogen原生支持【星火大模型】
from sparkai.frameworks.autogen import SparkAI
import autogen
from autogen.agentchat.contrib.retrieve_assistant_agent import RetrieveAssistantAgent
spark_config = autogen.config_list_from_json(
"sparkai_autogen.json",
filter_dict={"model_client_cls": ["SparkAI"]},
)
llm_config = {
"timeout": 600,
"cache_seed": None, # change the seed for different trials
"config_list": spark_config,
"temperature": 0,
}
# 1. create an RetrieveAssistantAgent instance named "assistant"
assistant = RetrieveAssistantAgent(
name="assistant",
system_message="You are a helpful assistant.",
llm_config=llm_config
)
# 注册SparkAI类进入 agent
assistant.register_model_client(model_client_cls=SparkAI)
其中sparkai_autogen.json
内容如下:
其中星火的domain对应 下面配置model
[
{
"api_key": "<spark_api_key>&<spark_api_secret>&<spark_app_id>",
"base_url": "wss://spark-api.xf-yun.com/v3.5/chat",
"model_client_cls": "SparkAI",
"model": "generalv3.5",
"stream": true,
"params": {
"request_timeout": 61
}
}
]
支持讯飞星火 图片理解
大模型:
import base64
image_content = base64.b64encode(open("spark_llama_index.png",'rb').read())
spark = ChatSparkLLM(
spark_app_id=os.environ["SPARKAI_APP_ID"],
spark_api_key=os.environ["SPARKAI_API_KEY"],
spark_api_secret=os.environ["SPARKAI_API_SECRET"],
spark_llm_domain="image",
streaming=False,
user_agent="test"
)
messages = [ImageChatMessage(
role="user",
content=image_content,
content_type="image"
),ImageChatMessage(
role="user",
content="这是什么图",
content_type="text"
)]
handler = ChunkPrintHandler()
a = spark.generate([messages], callbacks=[])
参考如下 test方法:
from sparkai.embedding.spark_embedding import Embeddingmodel, SparkEmbeddingFunction
import chromadb
def test_embedding():
model = Embeddingmodel(
spark_app_id="id",
spark_api_key="key",
spark_api_secret="secret",
spark_domain="query",
)
# desc = {"messages":[{"content":"cc","role":"user"}]}
desc = {"content": "cc", "role": "user"}
# 调用embedding方法
a = model.embedding(text=desc, kind='text')
# print(len(a))
print(a)
def test_chroma_embedding():
chroma_client = chromadb.Client()
sparkmodel = SparkEmbeddingFunction(
spark_app_id="id",
spark_api_key="key",
spark_api_secret="secret",
spark_domain="query",
)
a = sparkmodel(["This is a document", "This is another document"])
# print(type(a))
# print(a[0])
# print(a[0][1])
# 可以正确的生成embedding结果
collection = chroma_client.get_or_create_collection(name="my_collection", embedding_function=sparkmodel)
# 为什么是None
collection.add(
documents=["This is a document", "cc", "1122"],
metadatas=[{"source": "my_source"}, {"source": "my_source"}, {"source": "my_source"}],
ids=["id1", "id2", "id3"]
)
# print(collection.peek()) #显示前五条数据
print(collection.count()) # 数据库中数据量
results = collection.query(
query_texts=["ac", 'documents'],
n_results=2
)
print(results) # 查询结果
if __name__ == "__main__":
test_embedding()
test_chroma_embedding()
可通过构造 model_kwargs
字典传入Client配置中即可
spark = ChatSparkLLM(
spark_api_url="wss://xingchen-api.cn-huabei-1.xf-yun.com/v1.1/chat",
spark_app_id=os.environ["SPARKAI_APP_ID"],
spark_api_key=os.environ["SPARKAI_API_KEY"],
spark_api_secret=os.environ["SPARKAI_API_SECRET"],
spark_llm_domain="xspark13b6k",
streaming=True,
max_tokens=1024,
model_kwargs={"search_disable": False},
)
可通过构造 model_kwargs
字典传入patch_id
即可,适用于lora或小包类型类型推理
async def test_13b_lora():
from sparkai.log.logger import logger
logger.setLevel("debug")
from sparkai.core.callbacks import StdOutCallbackHandler
messages = [{'role': 'user',
'content': "卧槽"}]
spark = ChatSparkLLM(
spark_api_url="wss://xingchen-api.cn-huabei-1.xf-yun.com/v1.1/chat",
spark_app_id=os.environ["SPARKAI_APP_ID"],
spark_api_key=os.environ["SPARKAI_API_KEY"],
spark_api_secret=os.environ["SPARKAI_API_SECRET"],
spark_llm_domain="xspark13b6k",
streaming=True,
max_tokens=1024,
model_kwargs={"patch_id": "210267877777408"},
)
messages = [
ChatMessage(
role="user",
content=messages[0]['content']
)]
handler = AsyncChunkPrintHandler()
a = spark.astream(messages, config={"callbacks": [handler]})
async for message in a:
print(message)
设置日志级别:
from sparkai.log.logger import logger
logger.setLevel("debug")
扫码加入交流群
- 项目目前开发阶段,有一些冗余代码,人力有限,部分思想借鉴开源实现
- Client当前不支持多路复用,多线程使用时, 每个线程需要单独实例化Client
- 当前流式接口不支持每帧统计token数量,需要sparkapi正式支持该特性后, sdk会同步支持。
- wss://spark-api.xf-yun.com/v3.5/chat
For Tasks:
Click tags to check more tools for each tasksFor Jobs:
Alternative AI tools for spark-ai-python
Similar Open Source Tools
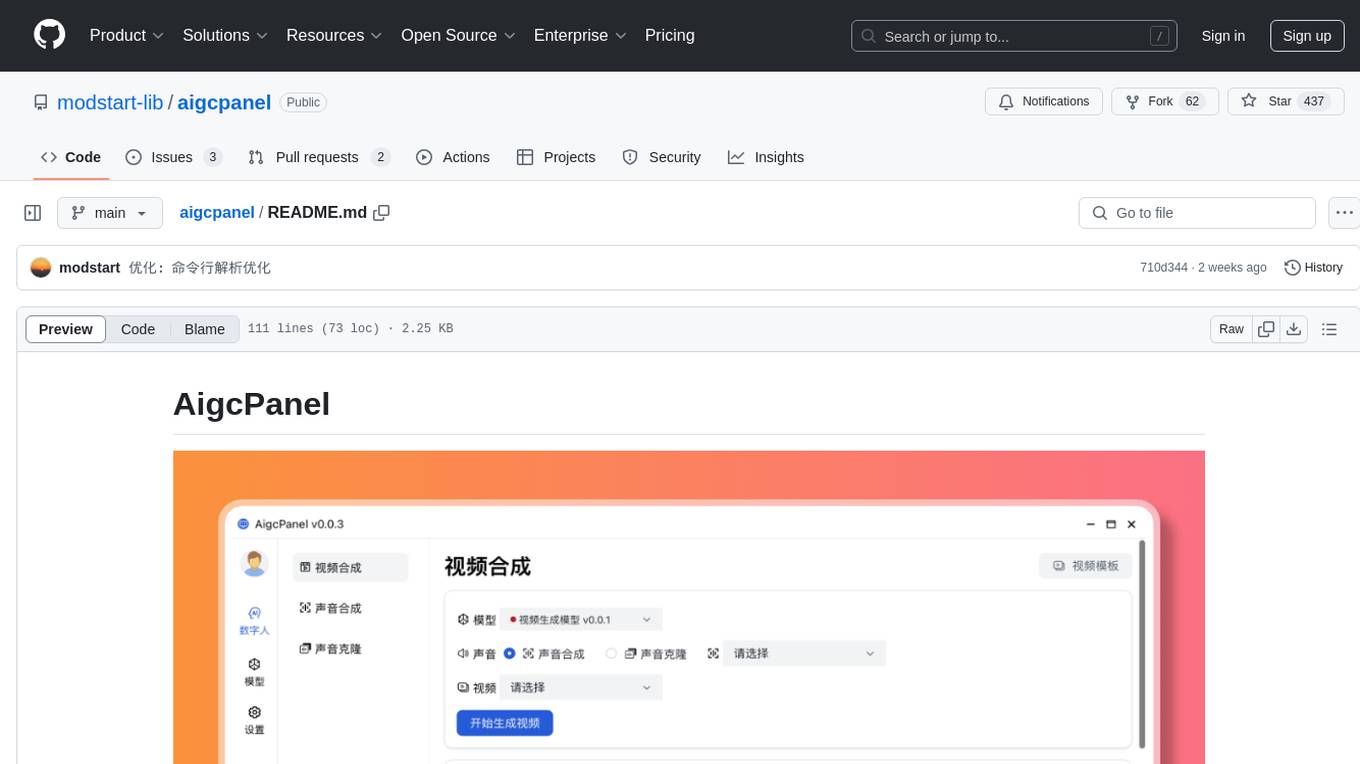
aigcpanel
AigcPanel is a simple and easy-to-use all-in-one AI digital human system that even beginners can use. It supports video synthesis, voice synthesis, voice cloning, simplifies local model management, and allows one-click import and use of AI models. It prohibits the use of this product for illegal activities and users must comply with the laws and regulations of the People's Republic of China.
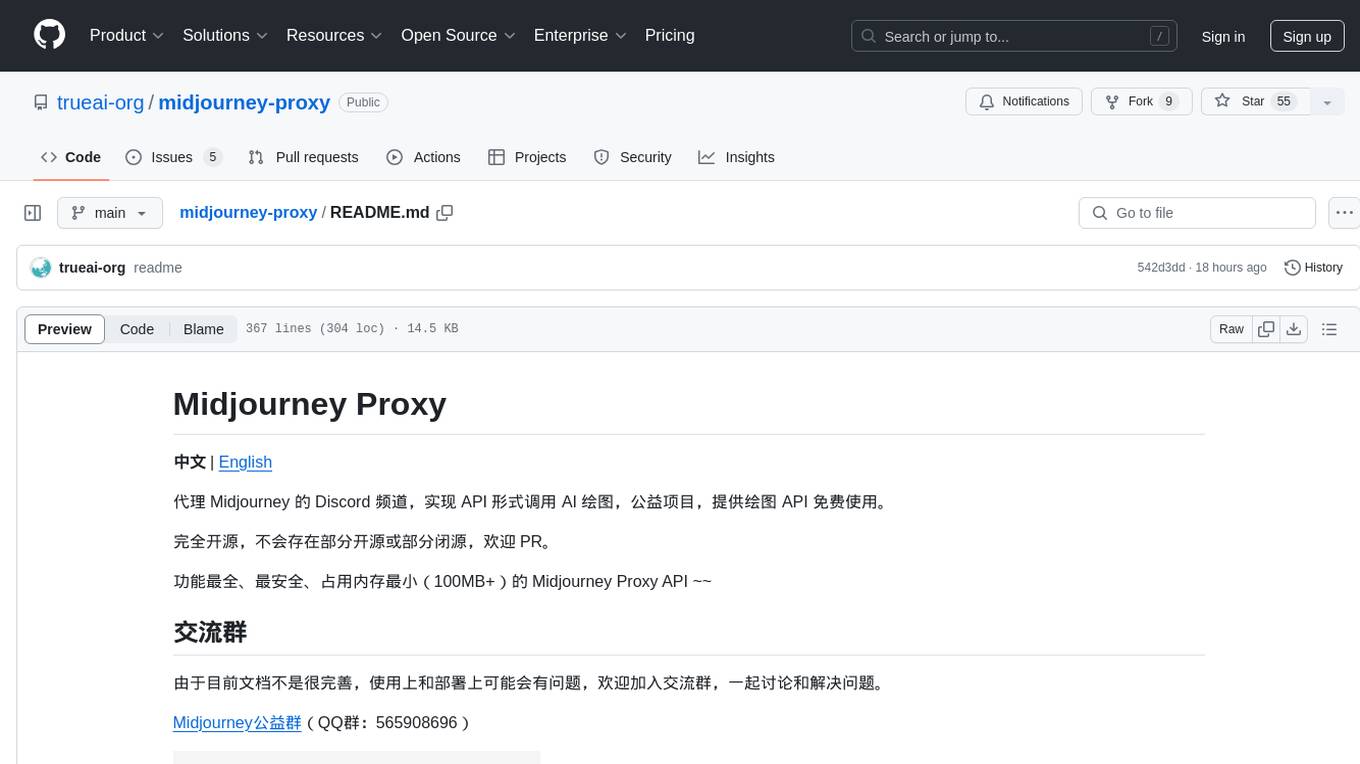
midjourney-proxy
Midjourney Proxy is an open-source project that acts as a proxy for the Midjourney Discord channel, allowing API-based AI drawing calls for charitable purposes. It provides drawing API for free use, ensuring full functionality, security, and minimal memory usage. The project supports various commands and actions related to Imagine, Blend, Describe, and more. It also offers real-time progress tracking, Chinese prompt translation, sensitive word pre-detection, user-token connection via wss for error information retrieval, and various account configuration options. Additionally, it includes features like image zooming, seed value retrieval, account-specific speed mode settings, multiple account configurations, and more. The project aims to support mainstream drawing clients and API calls, with features like task hierarchy, Remix mode, image saving, and CDN acceleration, among others.
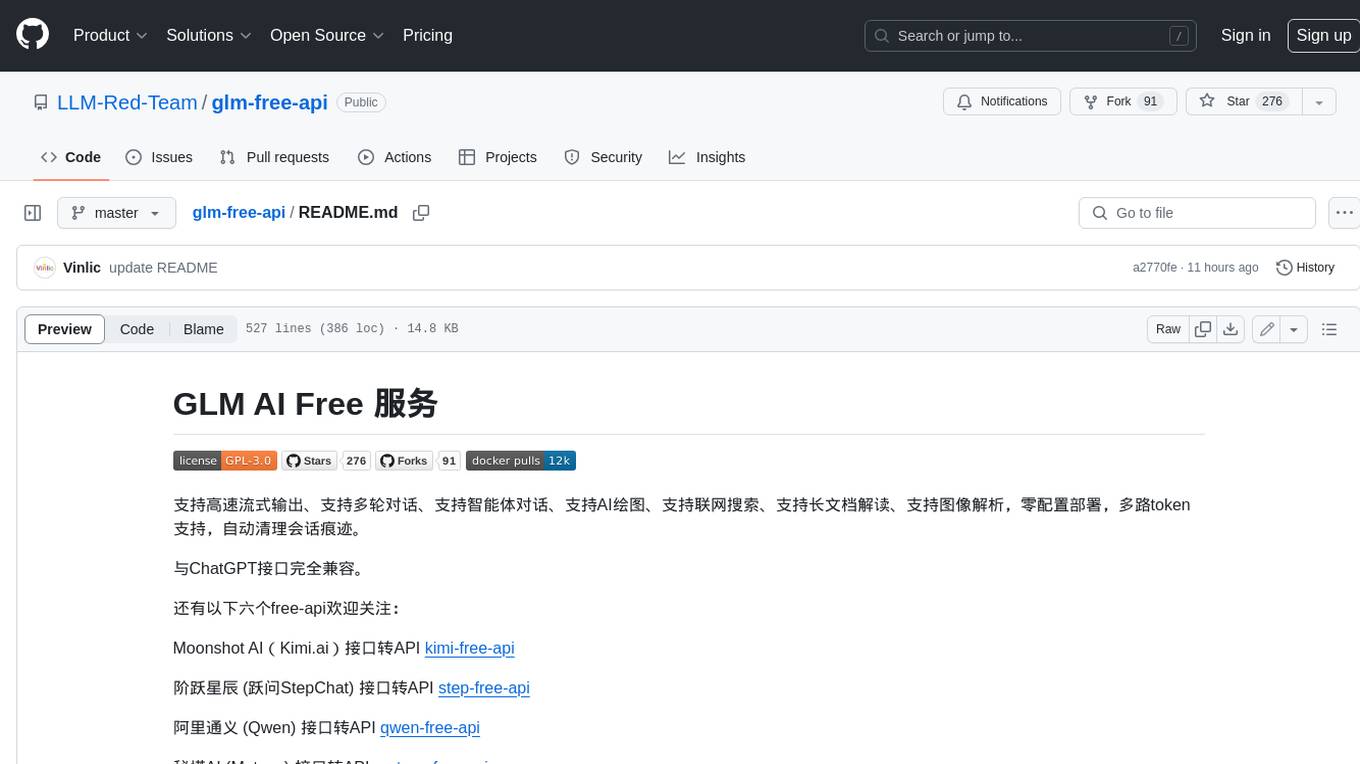
glm-free-api
GLM AI Free 服务 provides high-speed streaming output, multi-turn dialogue support, intelligent agent dialogue support, AI drawing support, online search support, long document interpretation support, image parsing support. It offers zero-configuration deployment, multi-token support, and automatic session trace cleaning. It is fully compatible with the ChatGPT interface. The repository also includes six other free APIs for various services like Moonshot AI, StepChat, Qwen, Metaso, Spark, and Emohaa. The tool supports tasks such as chat completions, AI drawing, document interpretation, image parsing, and refresh token survival check.
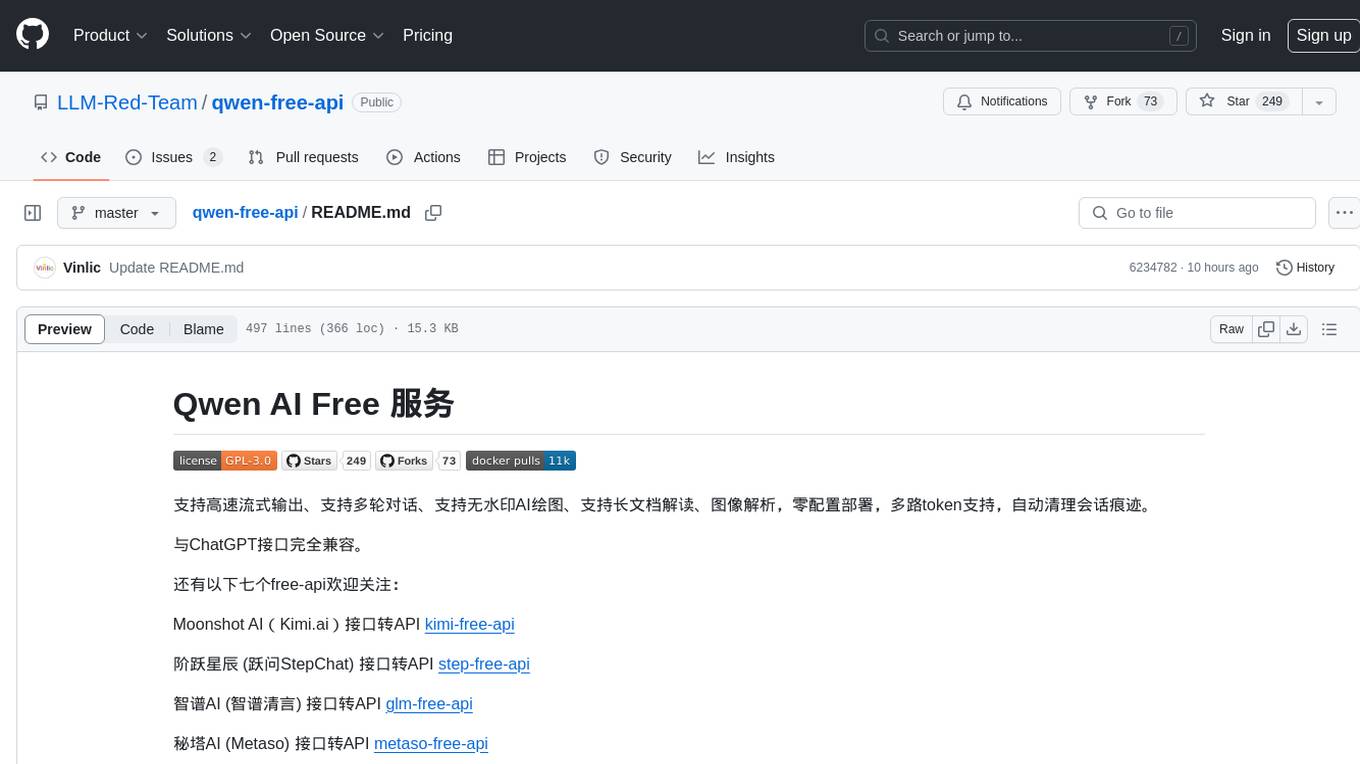
qwen-free-api
Qwen AI Free service supports high-speed streaming output, multi-turn dialogue, watermark-free AI drawing, long document interpretation, image parsing, zero-configuration deployment, multi-token support, automatic session trace cleaning. It is fully compatible with the ChatGPT interface. The repository provides various free APIs for different AI services. Users can access the service through different deployment methods like Docker, Docker-compose, Render, Vercel, and native deployment. It offers interfaces for chat completions, AI drawing, document interpretation, image parsing, and token checking. Users need to provide 'login_tongyi_ticket' for authorization. The project emphasizes research, learning, and personal use only, discouraging commercial use to avoid service pressure on the official platform.
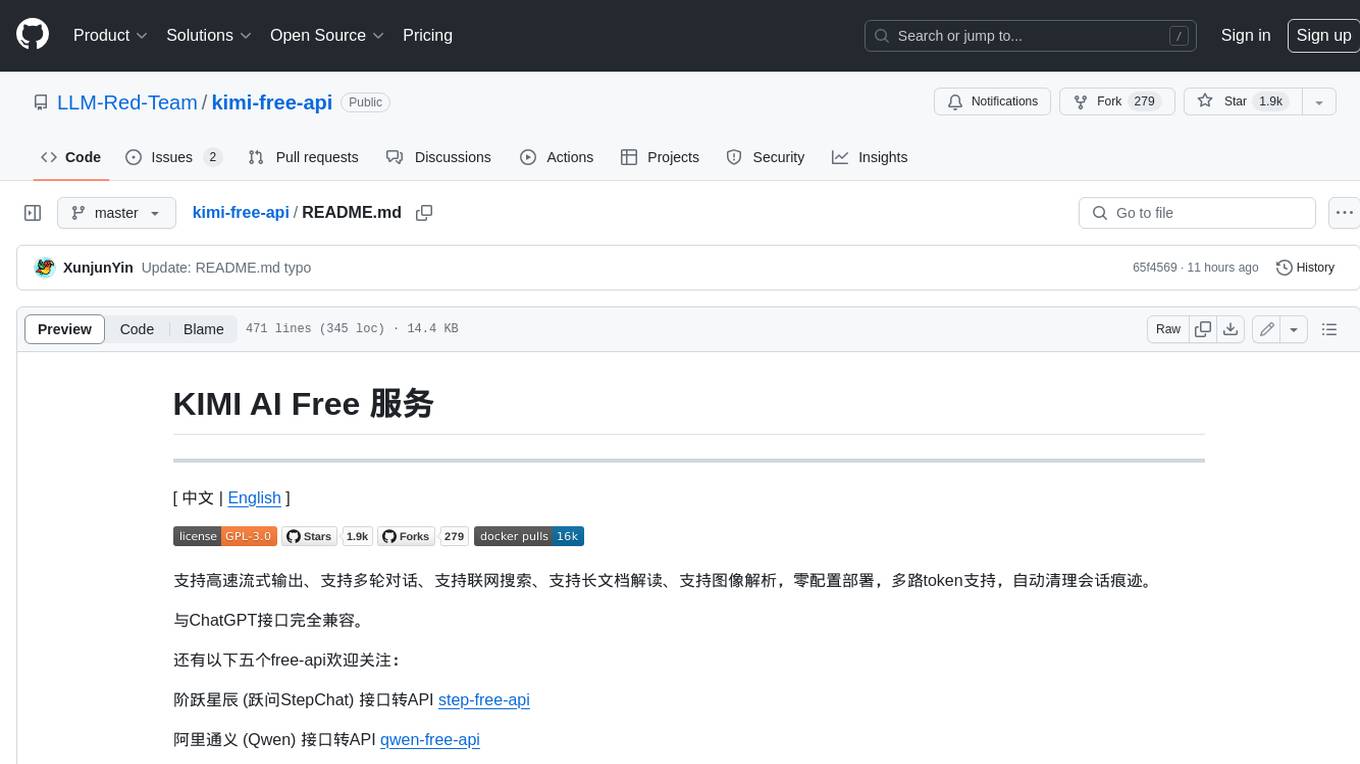
kimi-free-api
KIMI AI Free 服务 支持高速流式输出、支持多轮对话、支持联网搜索、支持长文档解读、支持图像解析,零配置部署,多路token支持,自动清理会话痕迹。 与ChatGPT接口完全兼容。 还有以下五个free-api欢迎关注: 阶跃星辰 (跃问StepChat) 接口转API step-free-api 阿里通义 (Qwen) 接口转API qwen-free-api ZhipuAI (智谱清言) 接口转API glm-free-api 秘塔AI (metaso) 接口转API metaso-free-api 聆心智能 (Emohaa) 接口转API emohaa-free-api
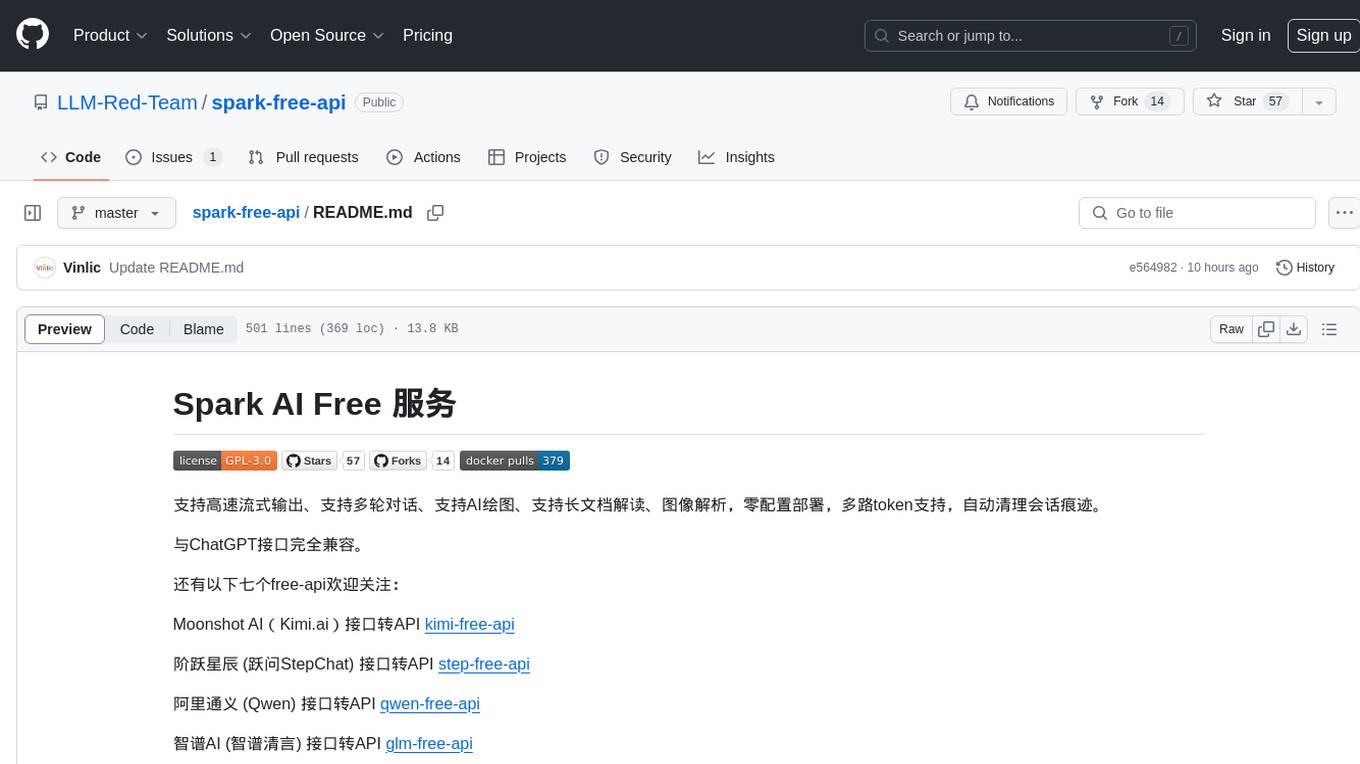
spark-free-api
Spark AI Free 服务 provides high-speed streaming output, multi-turn dialogue support, AI drawing support, long document interpretation, and image parsing. It offers zero-configuration deployment, multi-token support, and automatic session trace cleaning. It is fully compatible with the ChatGPT interface. The repository includes multiple free-api projects for various AI services. Users can access the API for tasks such as chat completions, AI drawing, document interpretation, image analysis, and ssoSessionId live checking. The project also provides guidelines for deployment using Docker, Docker-compose, Render, Vercel, and native deployment methods. It recommends using custom clients for faster and simpler access to the free-api series projects.
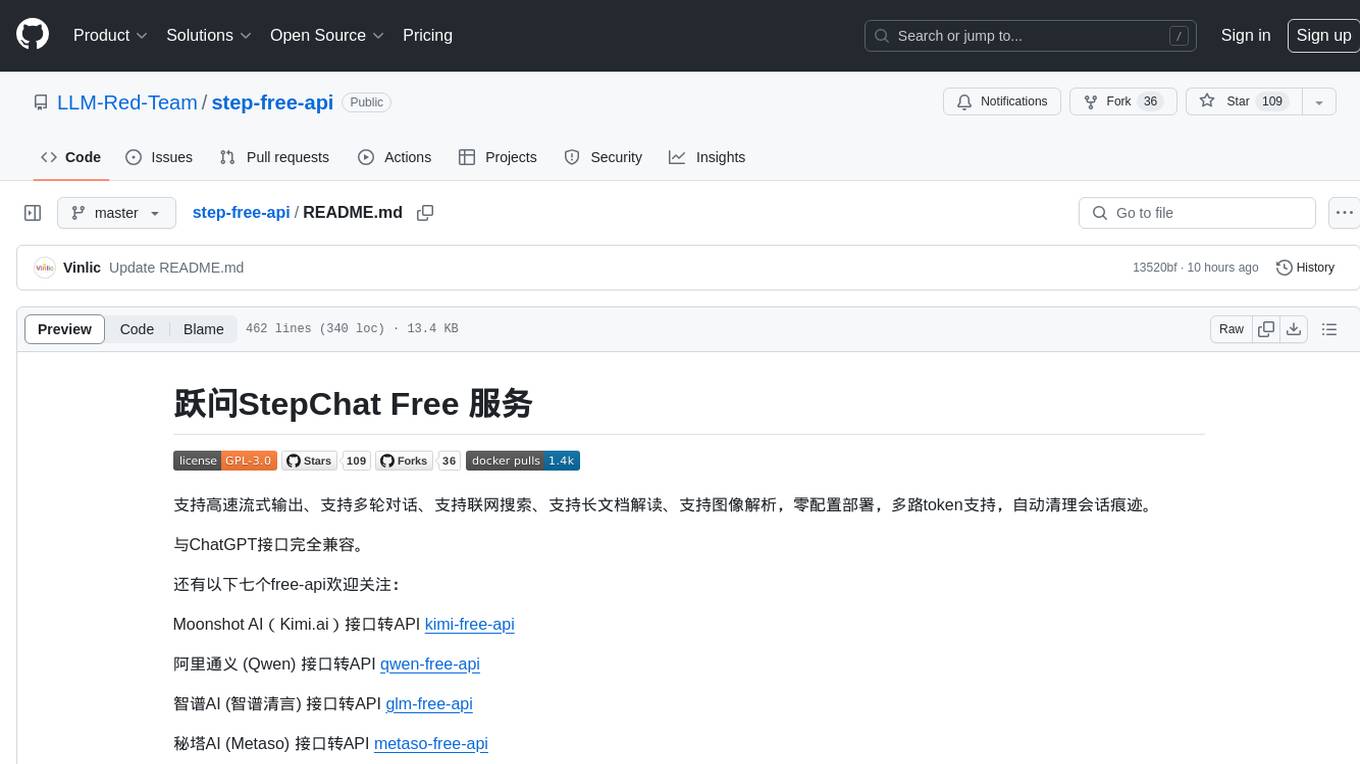
step-free-api
The StepChat Free service provides high-speed streaming output, multi-turn dialogue support, online search support, long document interpretation, and image parsing. It offers zero-configuration deployment, multi-token support, and automatic session trace cleaning. It is fully compatible with the ChatGPT interface. Additionally, it provides seven other free APIs for various services. The repository includes a disclaimer about using reverse APIs and encourages users to avoid commercial use to prevent service pressure on the official platform. It offers online testing links, showcases different demos, and provides deployment guides for Docker, Docker-compose, Render, Vercel, and native deployments. The repository also includes information on using multiple accounts, optimizing Nginx reverse proxy, and checking the liveliness of refresh tokens.
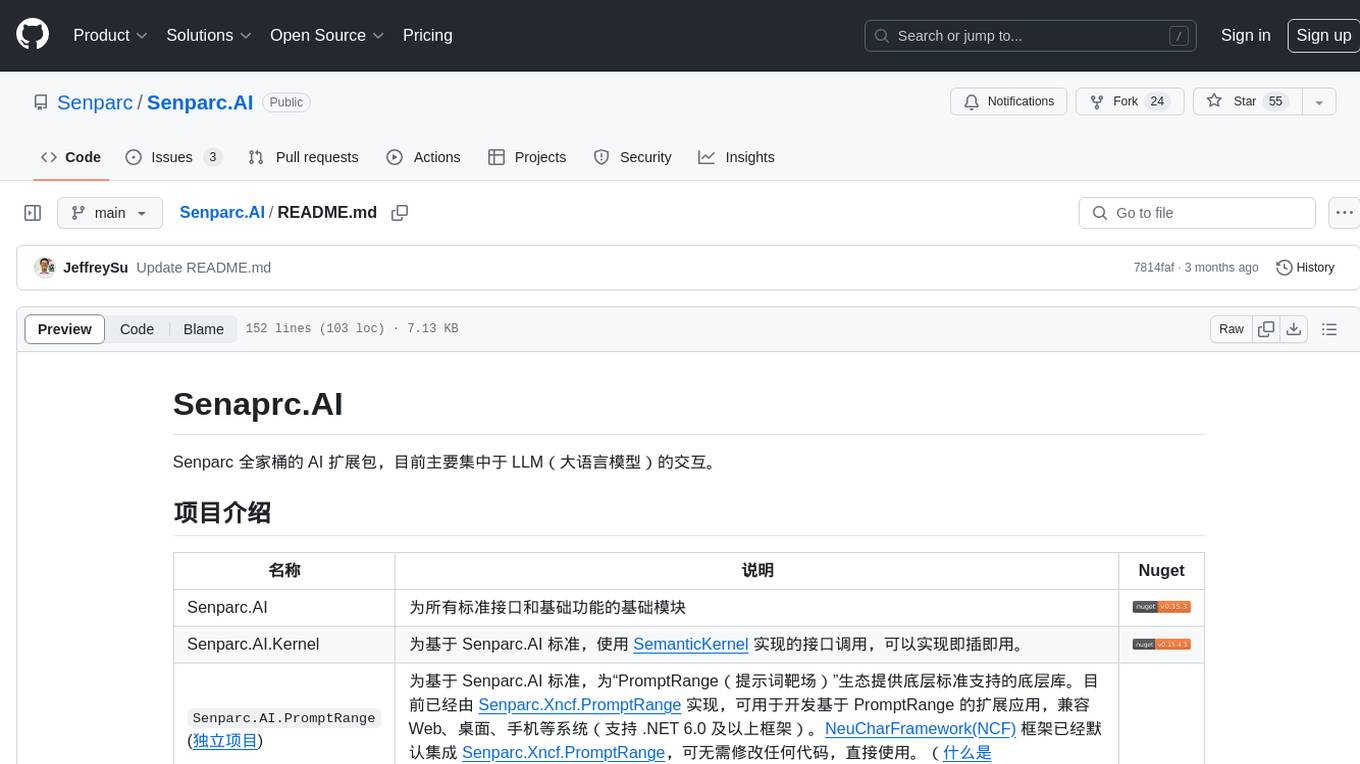
Senparc.AI
Senparc.AI is an AI extension package for the Senparc ecosystem, focusing on LLM (Large Language Models) interaction. It provides modules for standard interfaces and basic functionalities, as well as interfaces using SemanticKernel for plug-and-play capabilities. The package also includes a library for supporting the 'PromptRange' ecosystem, compatible with various systems and frameworks. Users can configure different AI platforms and models, define AI interface parameters, and run AI functions easily. The package offers examples and commands for dialogue, embedding, and DallE drawing operations.
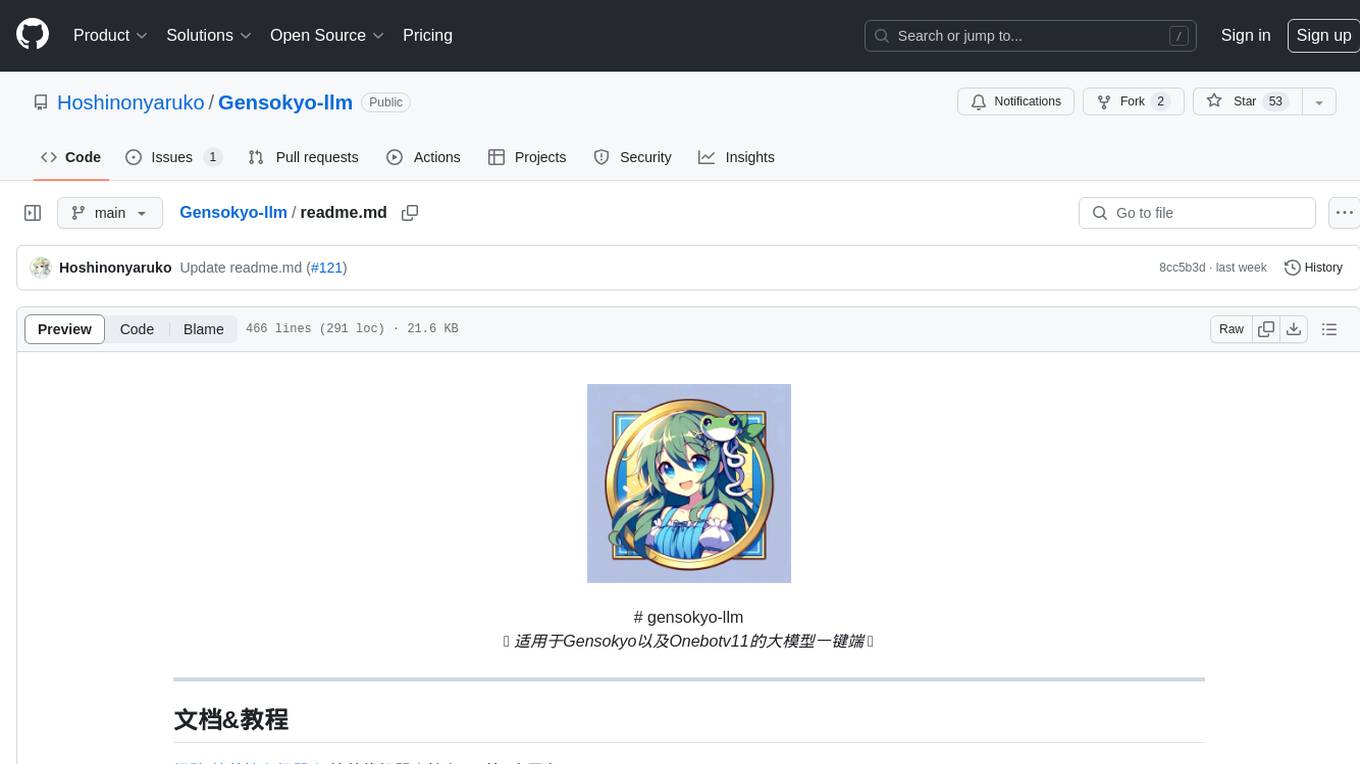
Gensokyo-llm
Gensokyo-llm is a tool designed for Gensokyo and Onebotv11, providing a one-click solution for large models. It supports various Onebotv11 standard frameworks, HTTP-API, and reverse WS. The tool is lightweight, with built-in SQLite for context maintenance and proxy support. It allows easy integration with the Gensokyo framework by configuring reverse HTTP and forward HTTP addresses. Users can set system settings, role cards, and context length. Additionally, it offers an openai original flavor API with automatic context. The tool can be used as an API or integrated with QQ channel robots. It supports converting GPT's SSE type and ensures memory safety in concurrent SSE environments. The tool also supports multiple users simultaneously transmitting SSE bidirectionally.
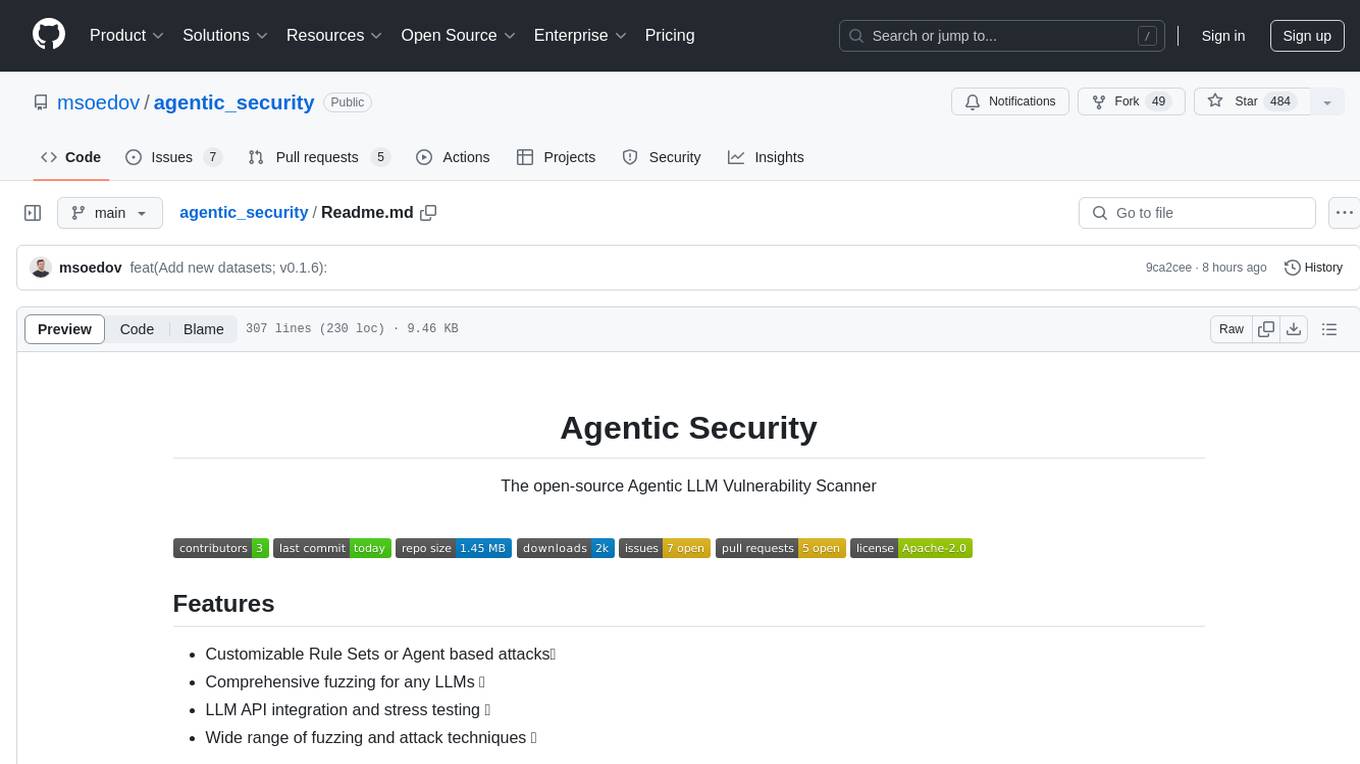
agentic_security
Agentic Security is an open-source vulnerability scanner designed for safety scanning, offering customizable rule sets and agent-based attacks. It provides comprehensive fuzzing for any LLMs, LLM API integration, and stress testing with a wide range of fuzzing and attack techniques. The tool is not a foolproof solution but aims to enhance security measures against potential threats. It offers installation via pip and supports quick start commands for easy setup. Users can utilize the tool for LLM integration, adding custom datasets, running CI checks, extending dataset collections, and dynamic datasets with mutations. The tool also includes a probe endpoint for integration testing. The roadmap includes expanding dataset variety, introducing new attack vectors, developing an attacker LLM, and integrating OWASP Top 10 classification.
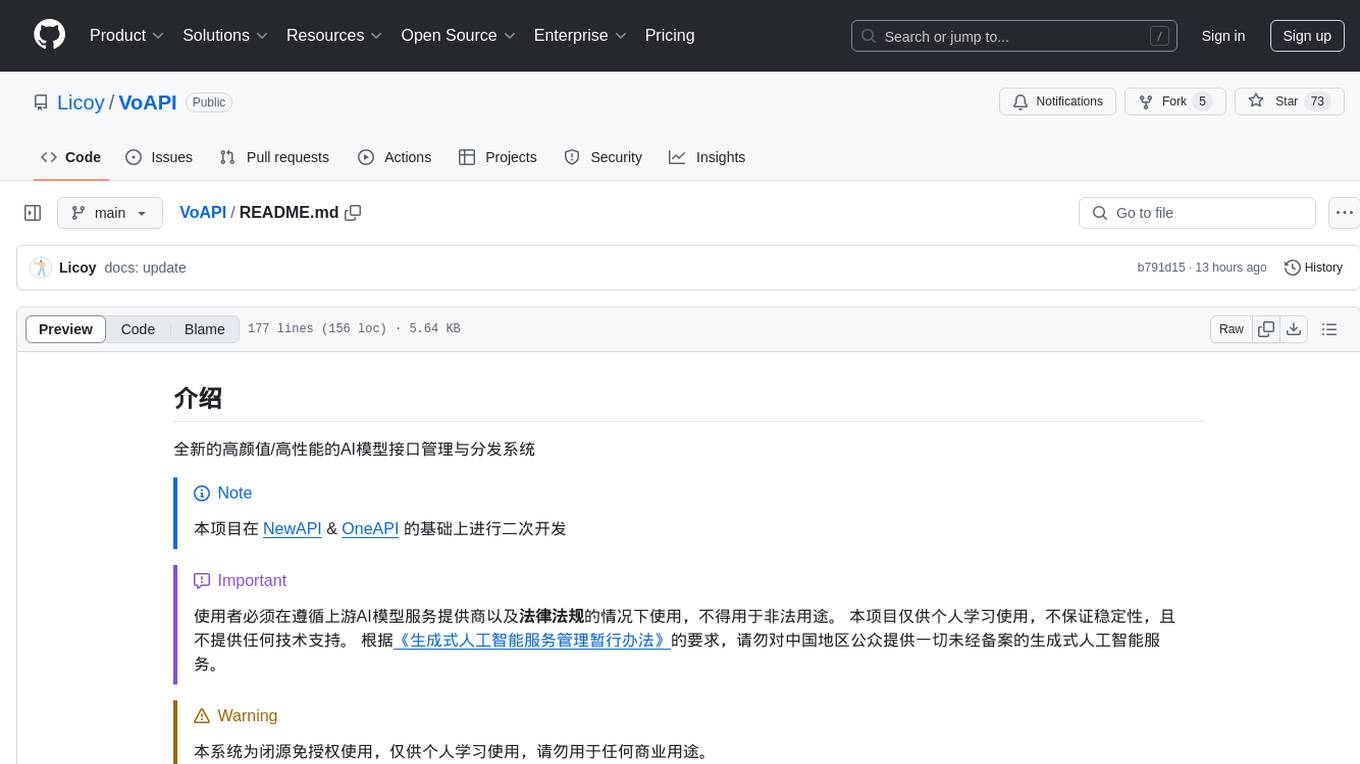
VoAPI
VoAPI is a new high-value/high-performance AI model interface management and distribution system. It is a closed-source tool for personal learning use only, not for commercial purposes. Users must comply with upstream AI model service providers and legal regulations. The system offers a visually appealing interface, independent development documentation page support, service monitoring page configuration support, and third-party login support. It also optimizes interface elements, user registration time support, data operation button positioning, and more.
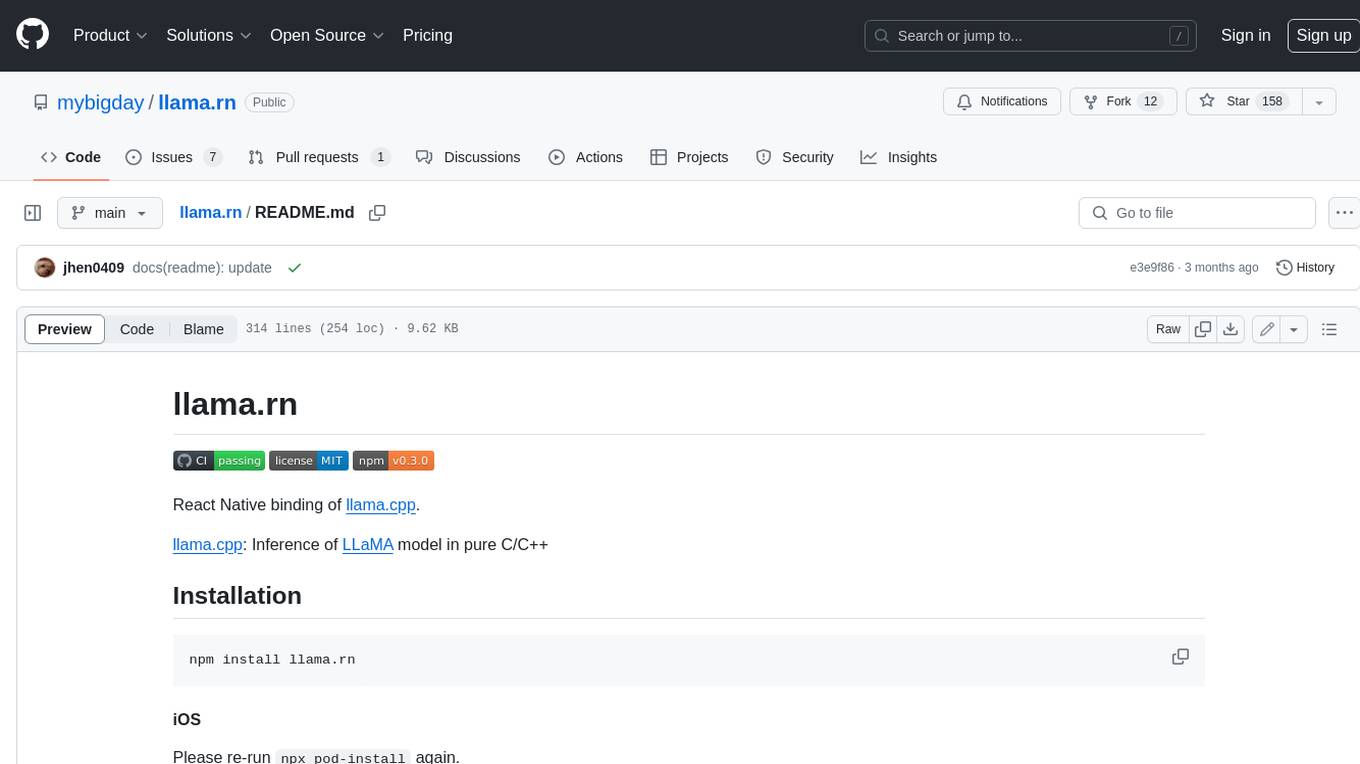
llama.rn
React Native binding of llama.cpp, which is an inference of LLaMA model in pure C/C++. This tool allows you to use the LLaMA model in your React Native applications for various tasks such as text completion, tokenization, detokenization, and embedding. It provides a convenient interface to interact with the LLaMA model and supports features like grammar sampling and mocking for testing purposes.
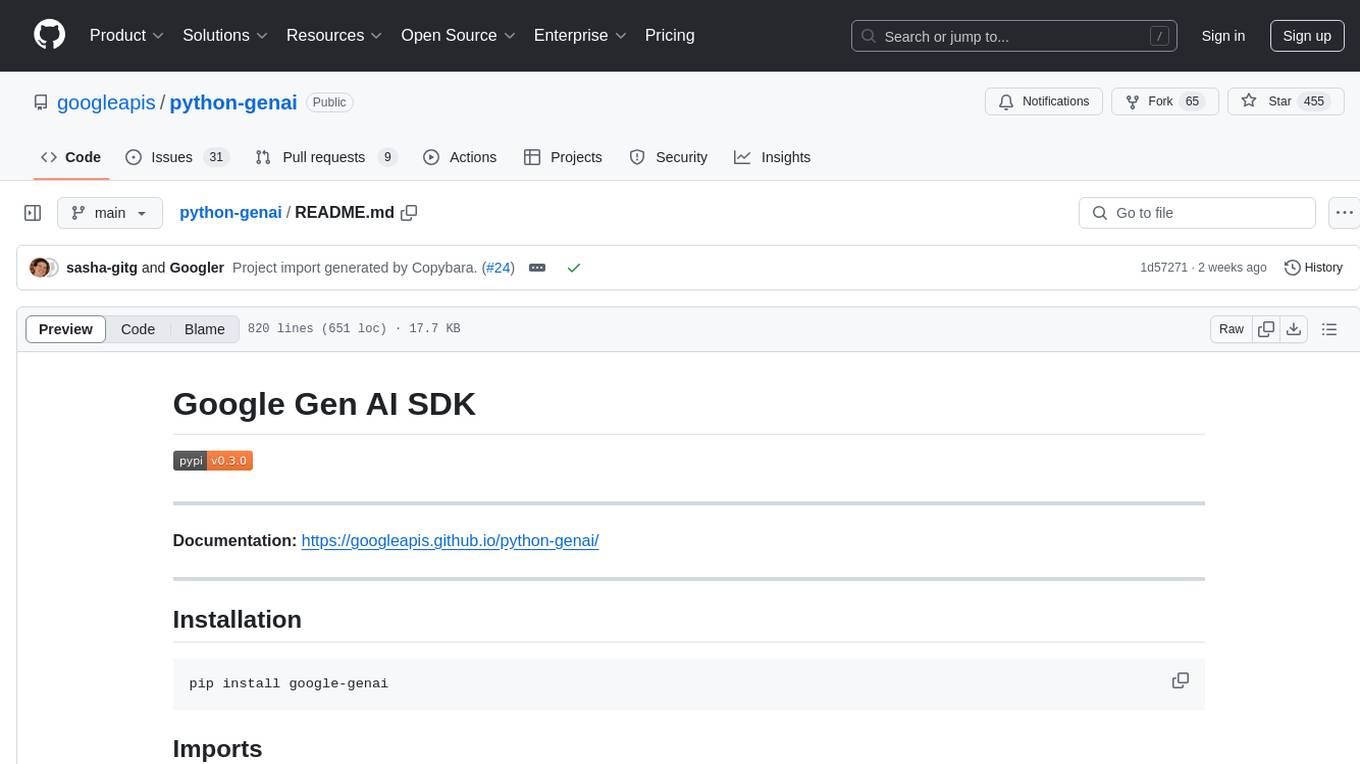
python-genai
The Google Gen AI SDK is a Python library that provides access to Google AI and Vertex AI services. It allows users to create clients for different services, work with parameter types, models, generate content, call functions, handle JSON response schemas, stream text and image content, perform async operations, count and compute tokens, embed content, generate and upscale images, edit images, work with files, create and get cached content, tune models, distill models, perform batch predictions, and more. The SDK supports various features like automatic function support, manual function declaration, JSON response schema support, streaming for text and image content, async methods, tuning job APIs, distillation, batch prediction, and more.
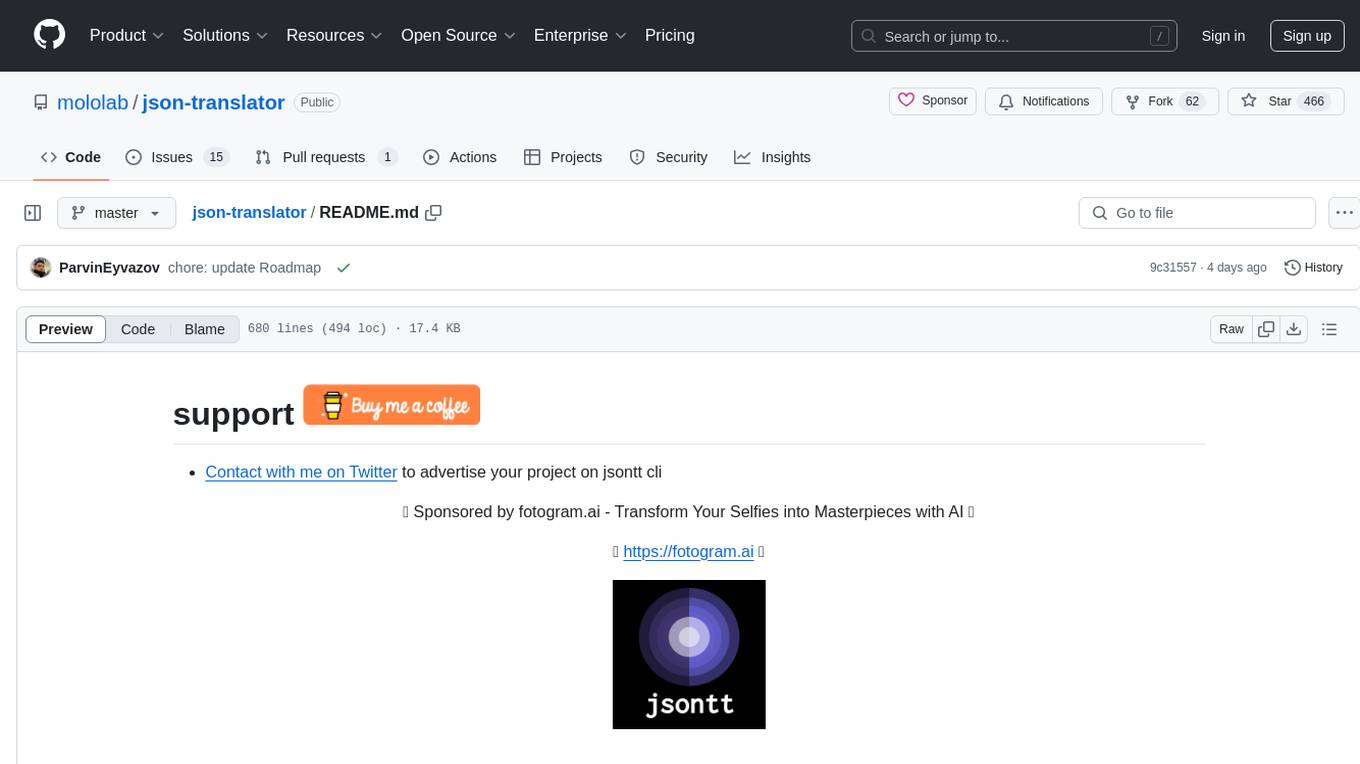
json-translator
The json-translator repository provides a free tool to translate JSON/YAML files or JSON objects into different languages using various translation modules. It supports CLI usage and package support, allowing users to translate words, sentences, JSON objects, and JSON files. The tool also offers multi-language translation, ignoring specific words, and safe translation practices. Users can contribute to the project by updating CLI, translation functions, JSON operations, and more. The roadmap includes features like Libre Translate option, Argos Translate option, Bing Translate option, and support for additional translation modules.
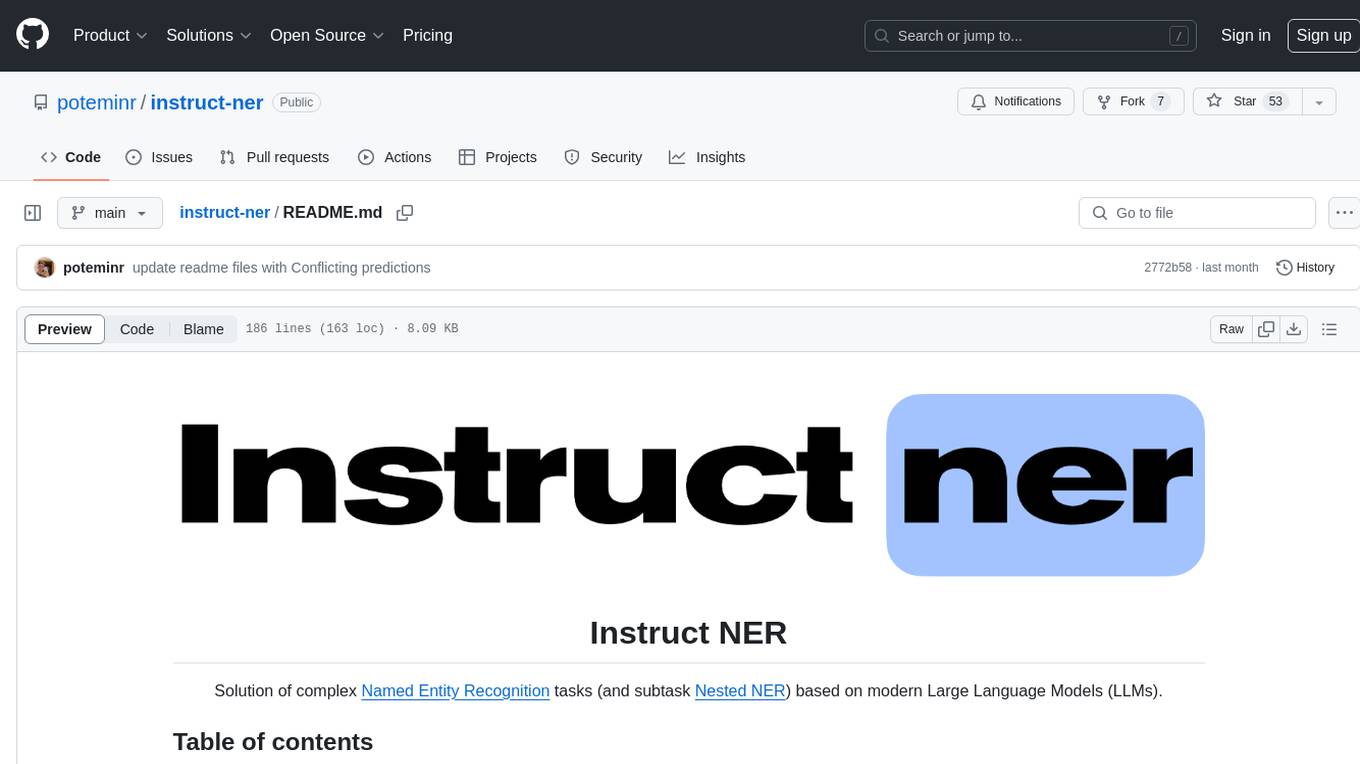
instruct-ner
Instruct NER is a solution for complex Named Entity Recognition tasks, including Nested NER, based on modern Large Language Models (LLMs). It provides tools for dataset creation, training, automatic metric calculation, inference, error analysis, and model implementation. Users can create instructions for LLM, build dictionaries with labels, and generate model input templates. The tool supports various entity types and datasets, such as RuDReC, NEREL-BIO, CoNLL-2003, and MultiCoNER II. It offers training scripts for LLMs and metric calculation functions. Instruct NER models like Llama, Mistral, T5, and RWKV are implemented, with HuggingFace models available for adaptation and merging.
For similar tasks
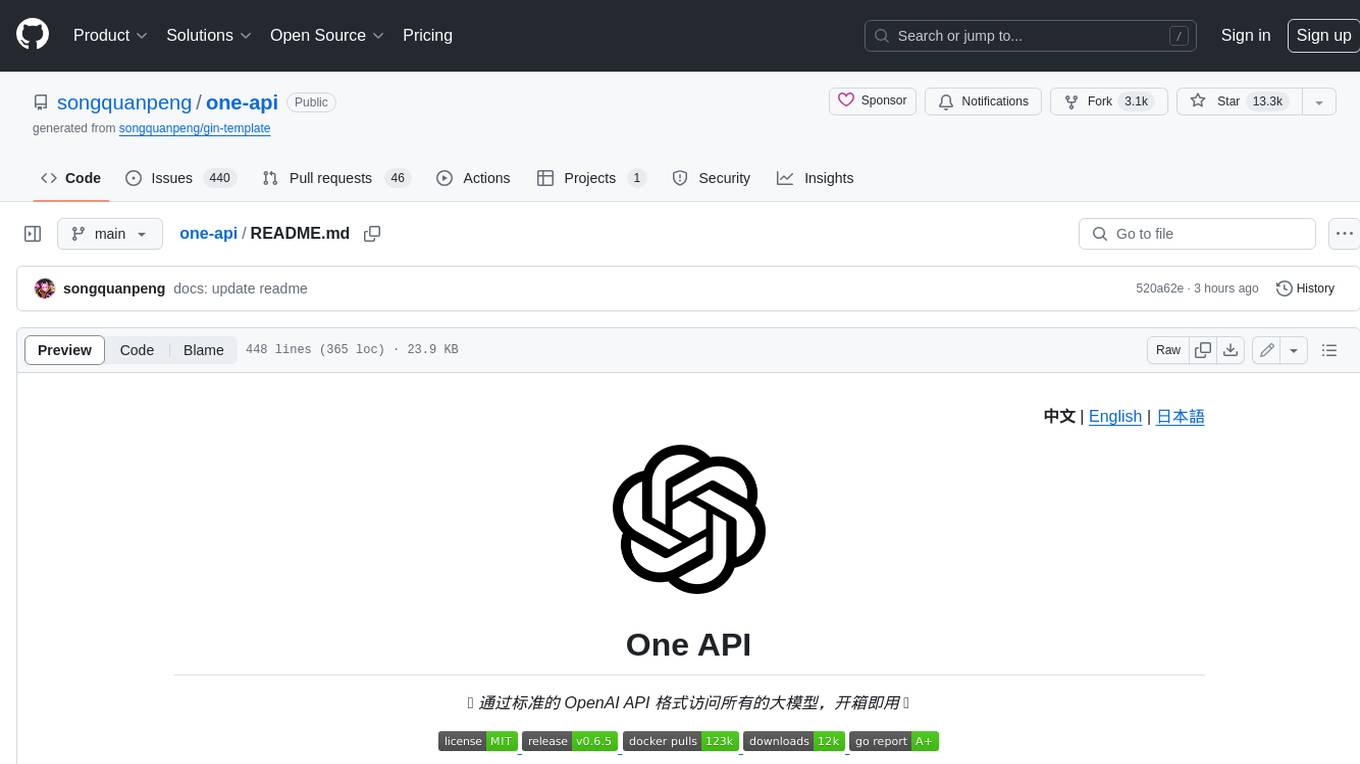
one-api
One API 是一个开源项目,它通过标准的 OpenAI API 格式访问所有的大模型,开箱即用。它支持多种大模型,包括 OpenAI ChatGPT 系列模型、Anthropic Claude 系列模型、Google PaLM2/Gemini 系列模型、Mistral 系列模型、百度文心一言系列模型、阿里通义千问系列模型、讯飞星火认知大模型、智谱 ChatGLM 系列模型、360 智脑、腾讯混元大模型、Moonshot AI、百川大模型、MINIMAX、Groq、Ollama、零一万物、阶跃星辰。One API 还支持配置镜像以及众多第三方代理服务,支持通过负载均衡的方式访问多个渠道,支持 stream 模式,支持多机部署,支持令牌管理,支持兑换码管理,支持渠道管理,支持用户分组以及渠道分组,支持渠道设置模型列表,支持查看额度明细,支持用户邀请奖励,支持以美元为单位显示额度,支持发布公告,设置充值链接,设置新用户初始额度,支持模型映射,支持失败自动重试,支持绘图接口,支持 Cloudflare AI Gateway,支持丰富的自定义设置,支持通过系统访问令牌调用管理 API,进而**在无需二开的情况下扩展和自定义** One API 的功能,支持 Cloudflare Turnstile 用户校验,支持用户管理,支持多种用户登录注册方式,支持主题切换,配合 Message Pusher 可将报警信息推送到多种 App 上。
For similar jobs
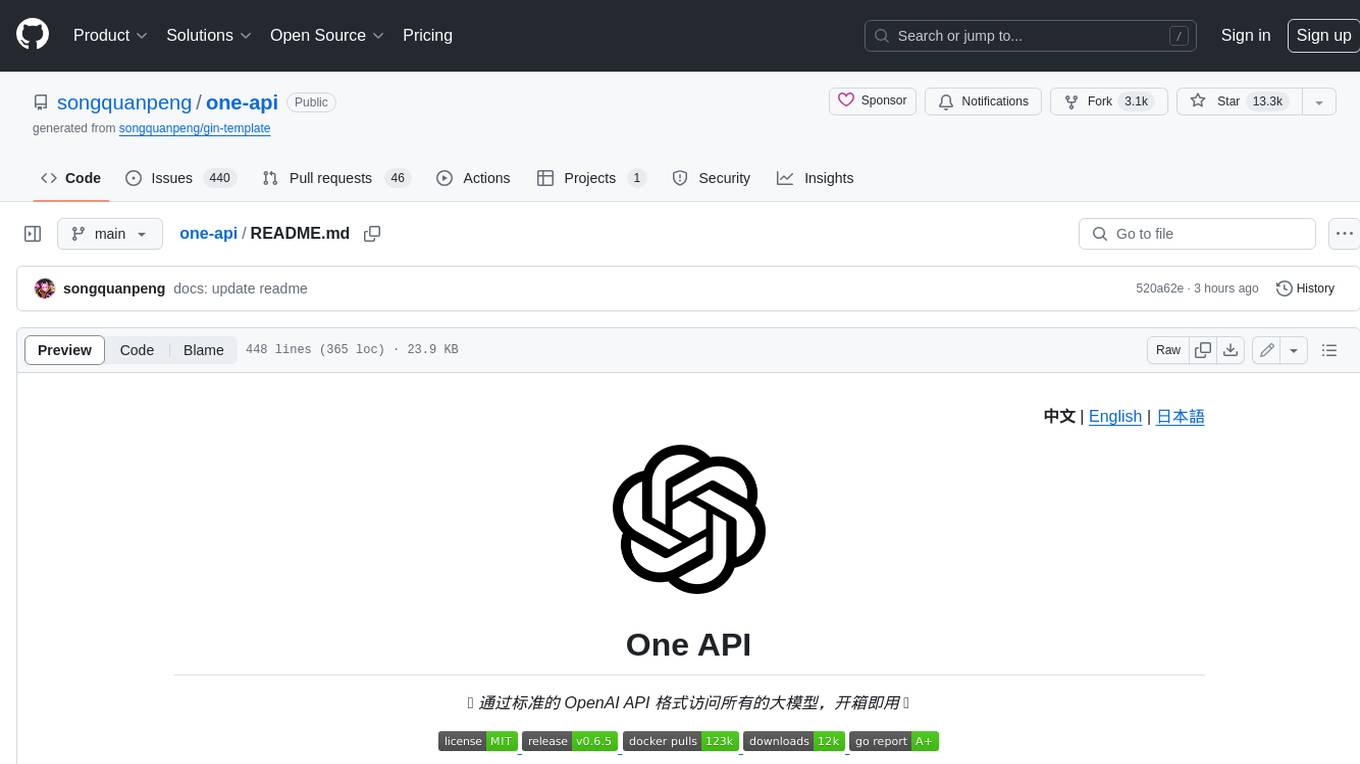
one-api
One API 是一个开源项目,它通过标准的 OpenAI API 格式访问所有的大模型,开箱即用。它支持多种大模型,包括 OpenAI ChatGPT 系列模型、Anthropic Claude 系列模型、Google PaLM2/Gemini 系列模型、Mistral 系列模型、百度文心一言系列模型、阿里通义千问系列模型、讯飞星火认知大模型、智谱 ChatGLM 系列模型、360 智脑、腾讯混元大模型、Moonshot AI、百川大模型、MINIMAX、Groq、Ollama、零一万物、阶跃星辰。One API 还支持配置镜像以及众多第三方代理服务,支持通过负载均衡的方式访问多个渠道,支持 stream 模式,支持多机部署,支持令牌管理,支持兑换码管理,支持渠道管理,支持用户分组以及渠道分组,支持渠道设置模型列表,支持查看额度明细,支持用户邀请奖励,支持以美元为单位显示额度,支持发布公告,设置充值链接,设置新用户初始额度,支持模型映射,支持失败自动重试,支持绘图接口,支持 Cloudflare AI Gateway,支持丰富的自定义设置,支持通过系统访问令牌调用管理 API,进而**在无需二开的情况下扩展和自定义** One API 的功能,支持 Cloudflare Turnstile 用户校验,支持用户管理,支持多种用户登录注册方式,支持主题切换,配合 Message Pusher 可将报警信息推送到多种 App 上。