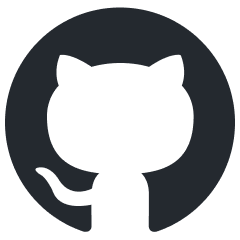
guardrails
Adding guardrails to large language models.
Stars: 4392
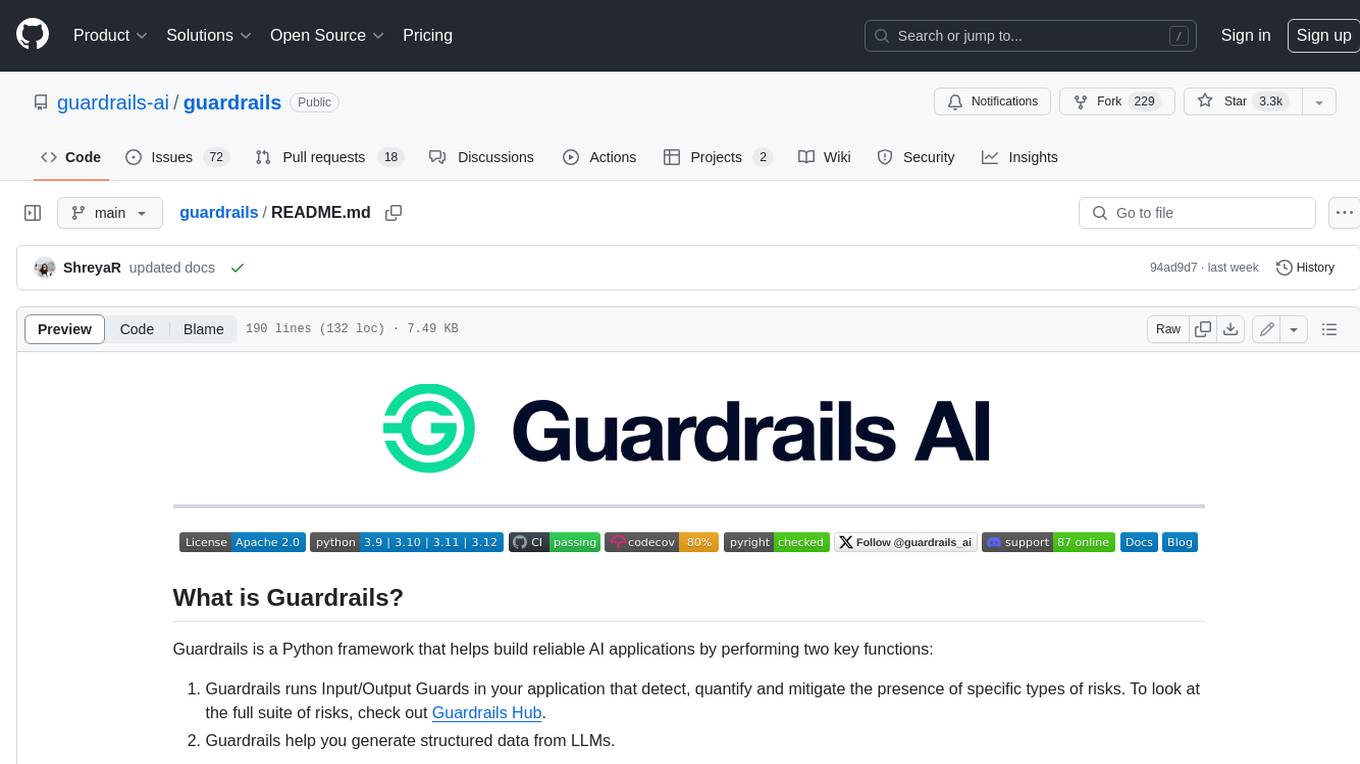
Guardrails is a Python framework that helps build reliable AI applications by performing two key functions: 1. Guardrails runs Input/Output Guards in your application that detect, quantify and mitigate the presence of specific types of risks. To look at the full suite of risks, check out Guardrails Hub. 2. Guardrails help you generate structured data from LLMs.
README:
Guardrails is a Python framework that helps build reliable AI applications by performing two key functions:
- Guardrails runs Input/Output Guards in your application that detect, quantify and mitigate the presence of specific types of risks. To look at the full suite of risks, check out Guardrails Hub.
- Guardrails help you generate structured data from LLMs.
Guardrails Hub is a collection of pre-built measures of specific types of risks (called 'validators'). Multiple validators can be combined together into Input and Output Guards that intercept the inputs and outputs of LLMs. Visit Guardrails Hub to see the full list of validators and their documentation.
pip install guardrails-ai
-
Download and configure the Guardrails Hub CLI.
pip install guardrails-ai guardrails configure
-
Install a guardrail from Guardrails Hub.
guardrails hub install hub://guardrails/regex_match
-
Create a Guard from the installed guardrail.
from guardrails import Guard, OnFailAction from guardrails.hub import RegexMatch guard = Guard().use( RegexMatch, regex="\(?\d{3}\)?-? *\d{3}-? *-?\d{4}", on_fail=OnFailAction.EXCEPTION ) guard.validate("123-456-7890") # Guardrail passes try: guard.validate("1234-789-0000") # Guardrail fails except Exception as e: print(e)
Output:
Validation failed for field with errors: Result must match \(?\d{3}\)?-? *\d{3}-? *-?\d{4}
-
Run multiple guardrails within a Guard. First, install the necessary guardrails from Guardrails Hub.
guardrails hub install hub://guardrails/competitor_check guardrails hub install hub://guardrails/toxic_language
Then, create a Guard from the installed guardrails.
from guardrails import Guard, OnFailAction from guardrails.hub import CompetitorCheck, ToxicLanguage guard = Guard().use_many( CompetitorCheck(["Apple", "Microsoft", "Google"], on_fail=OnFailAction.EXCEPTION), ToxicLanguage(threshold=0.5, validation_method="sentence", on_fail=OnFailAction.EXCEPTION) ) guard.validate( """An apple a day keeps a doctor away. This is good advice for keeping your health.""" ) # Both the guardrails pass try: guard.validate( """Shut the hell up! Apple just released a new iPhone.""" ) # Both the guardrails fail except Exception as e: print(e)
Output:
Validation failed for field with errors: Found the following competitors: [['Apple']]. Please avoid naming those competitors next time, The following sentences in your response were found to be toxic: - Shut the hell up!
Let's go through an example where we ask an LLM to generate fake pet names. To do this, we'll create a Pydantic BaseModel that represents the structure of the output we want.
from pydantic import BaseModel, Field
class Pet(BaseModel):
pet_type: str = Field(description="Species of pet")
name: str = Field(description="a unique pet name")
Now, create a Guard from the Pet
class. The Guard can be used to call the LLM in a manner so that the output is formatted to the Pet
class. Under the hood, this is done by either of two methods:
- Function calling: For LLMs that support function calling, we generate structured data using the function call syntax.
- Prompt optimization: For LLMs that don't support function calling, we add the schema of the expected output to the prompt so that the LLM can generate structured data.
from guardrails import Guard
import openai
prompt = """
What kind of pet should I get and what should I name it?
${gr.complete_json_suffix_v2}
"""
guard = Guard.for_pydantic(output_class=Pet, prompt=prompt)
raw_output, validated_output, *rest = guard(
llm_api=openai.completions.create,
engine="gpt-3.5-turbo-instruct"
)
print(validated_output)
This prints:
{
"pet_type": "dog",
"name": "Buddy
}
Guardrails can be set up as a standalone service served by Flask with guardrails start
, allowing you to interact with it via a REST API. This approach simplifies development and deployment of Guardrails-powered applications.
- Install:
pip install "guardrails-ai"
- Configure:
guardrails configure
- Create a config:
guardrails create --validators=hub://guardrails/two_words --name=two-word-guard
- Start the dev server:
guardrails start --config=./config.py
- Interact with the dev server via the snippets below
# with the guardrails client
import guardrails as gr
gr.settings.use_server = True
guard = gr.Guard(name='two-word-guard')
guard.validate('this is more than two words')
# or with the openai sdk
import openai
openai.base_url = "http://localhost:8000/guards/two-word-guard/openai/v1/"
os.environ["OPENAI_API_KEY"] = "youropenaikey"
messages = [
{
"role": "user",
"content": "tell me about an apple with 3 words exactly",
},
]
completion = openai.chat.completions.create(
model="gpt-4o-mini",
messages=messages,
)
For production deployments, we recommend using Docker with Gunicorn as the WSGI server for improved performance and scalability.
You can reach out to us on Discord or Twitter.
Yes, Guardrails can be used with proprietary and open-source LLMs. Check out this guide on how to use Guardrails with any LLM.
Yes, you can create your own validators and contribute them to Guardrails Hub. Check out this guide on how to create your own validators.
Guardrails can be used with Python and JavaScript. Check out the docs on how to use Guardrails from JavaScript. We are working on adding support for other languages. If you would like to contribute to Guardrails, please reach out to us on Discord or Twitter.
We welcome contributions to Guardrails!
Get started by checking out Github issues and check out the Contributing Guide. Feel free to open an issue, or reach out if you would like to add to the project!
For Tasks:
Click tags to check more tools for each tasksFor Jobs:
Alternative AI tools for guardrails
Similar Open Source Tools
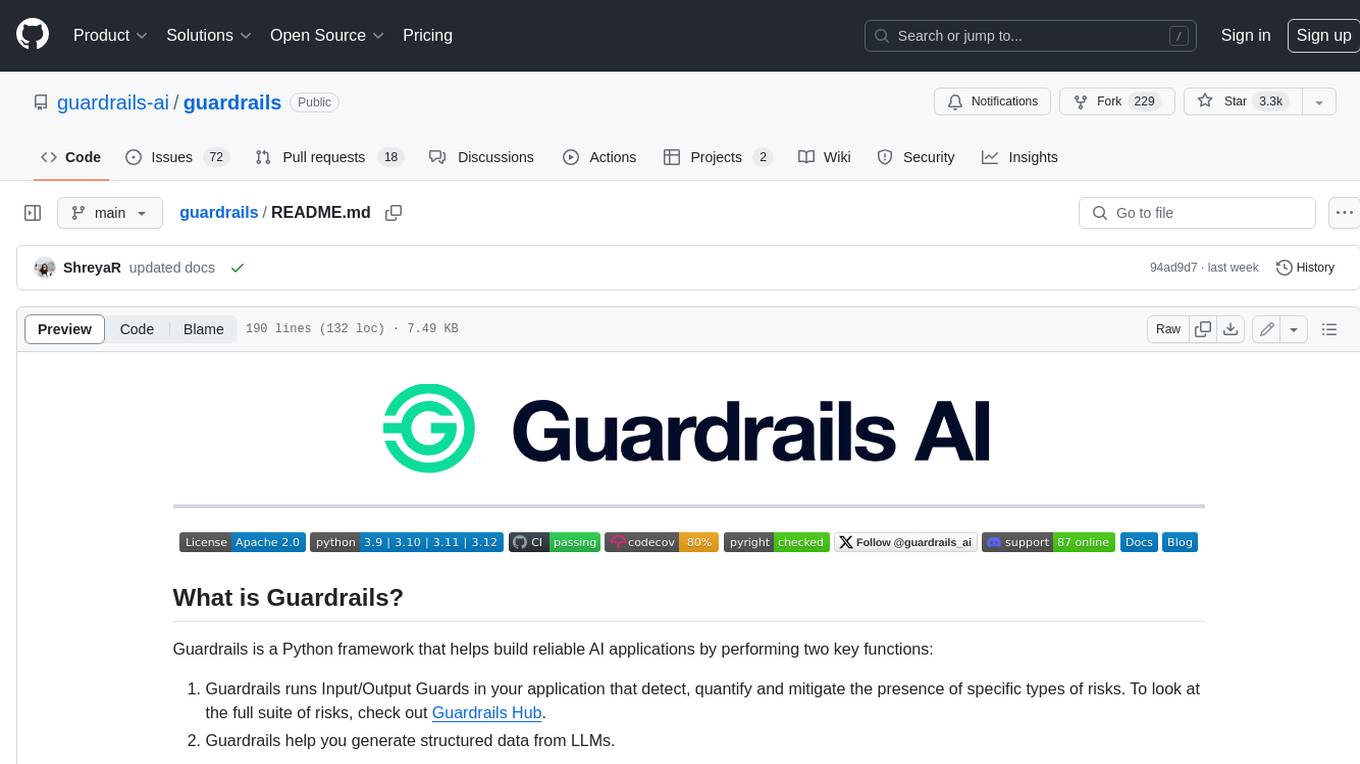
guardrails
Guardrails is a Python framework that helps build reliable AI applications by performing two key functions: 1. Guardrails runs Input/Output Guards in your application that detect, quantify and mitigate the presence of specific types of risks. To look at the full suite of risks, check out Guardrails Hub. 2. Guardrails help you generate structured data from LLMs.
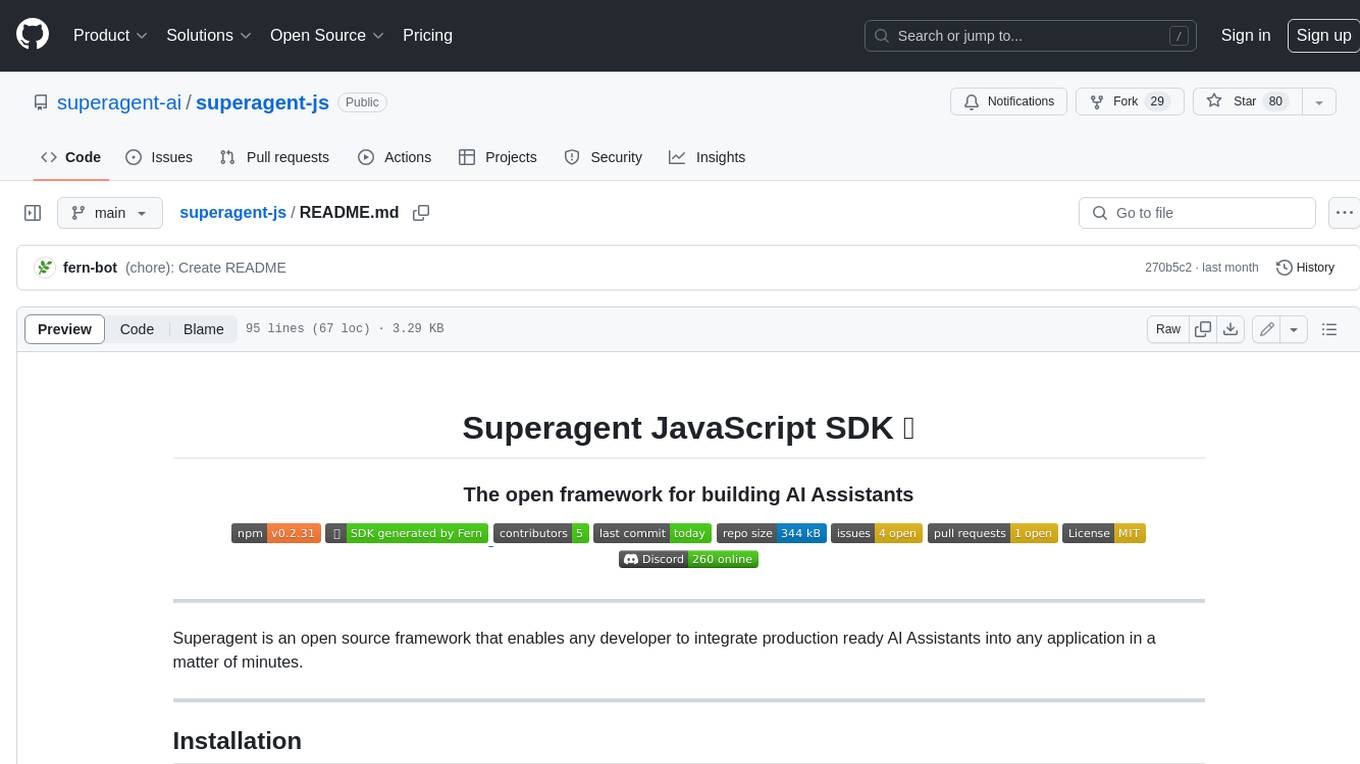
superagent-js
Superagent is an open source framework that enables any developer to integrate production ready AI Assistants into any application in a matter of minutes.
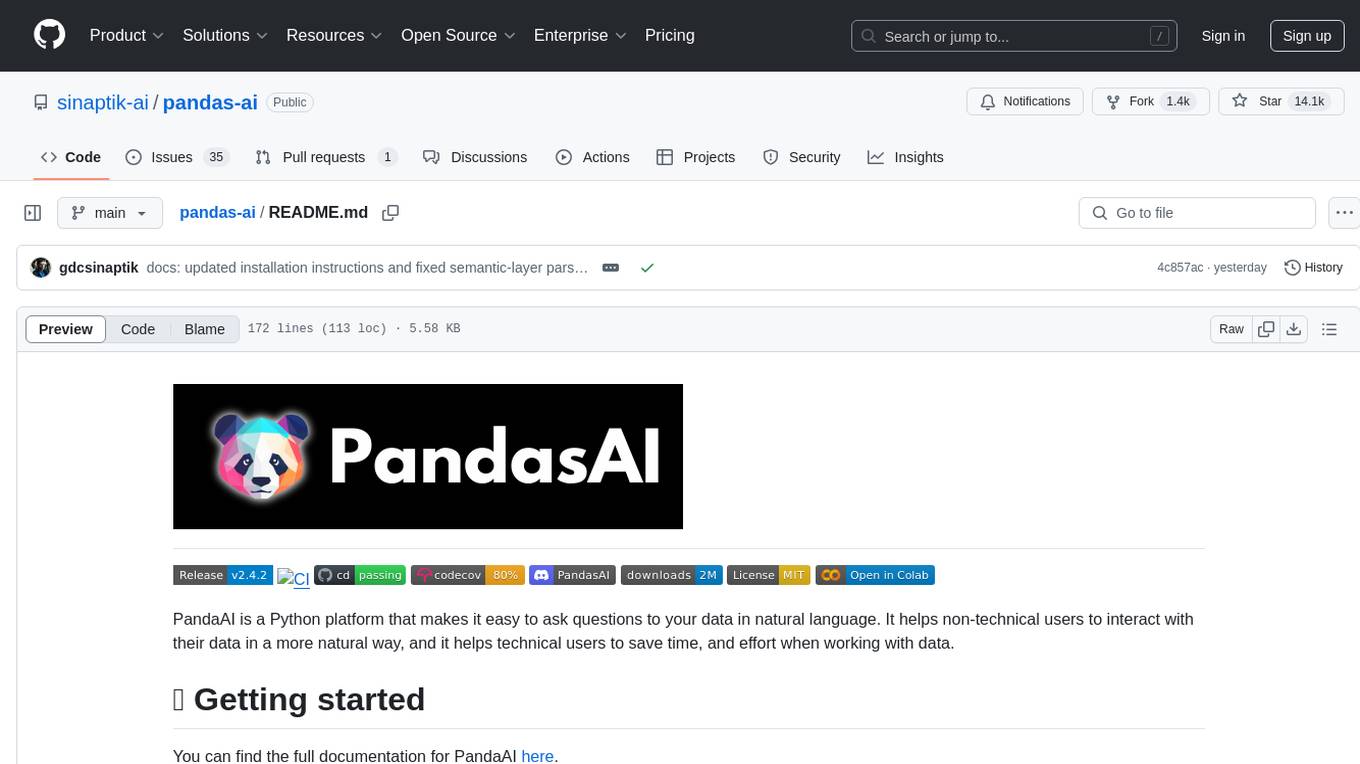
pandas-ai
PandaAI is a Python platform that enables users to interact with their data in natural language, catering to both non-technical and technical users. It simplifies data querying and analysis, offering conversational data analytics capabilities with minimal code. Users can ask questions, visualize charts, and compare dataframes effortlessly. The tool aims to streamline data exploration and decision-making processes by providing a user-friendly interface for data manipulation and analysis.
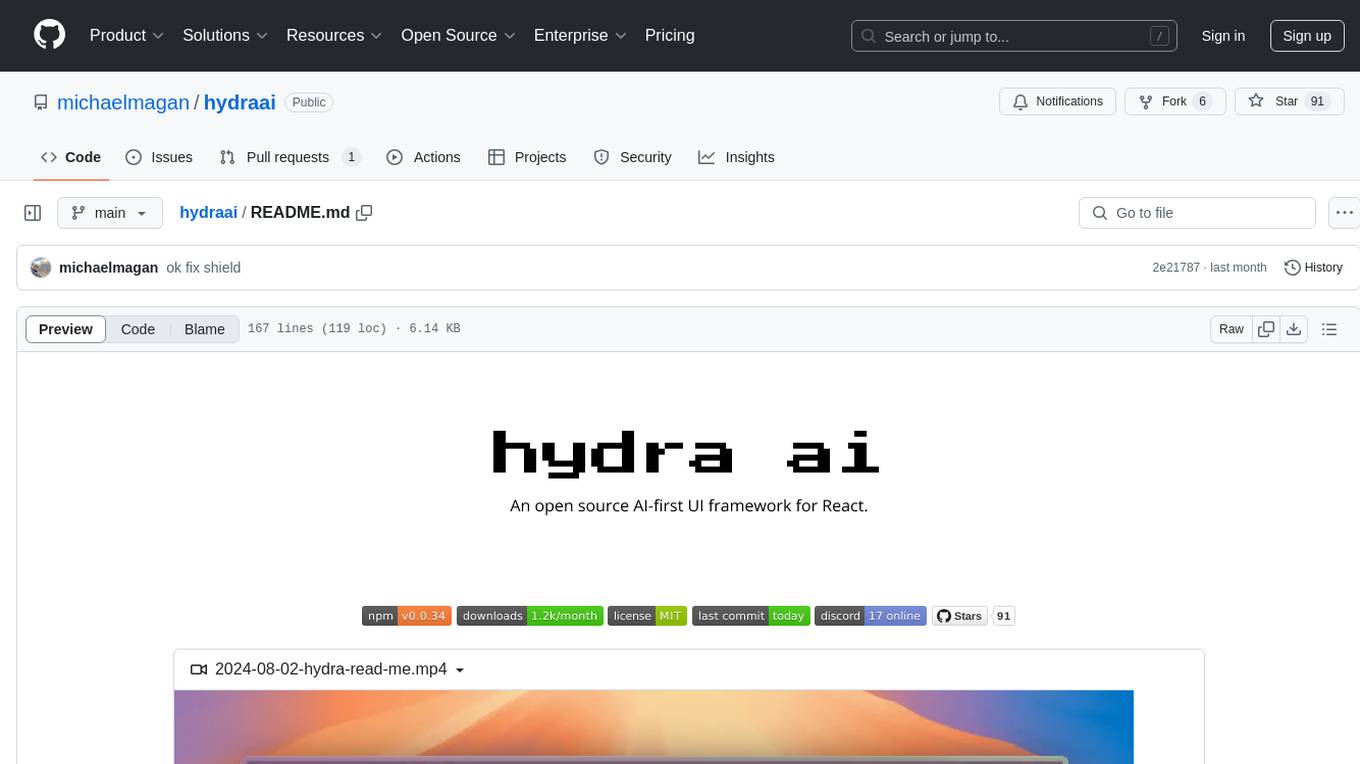
hydraai
Generate React components on-the-fly at runtime using AI. Register your components, and let Hydra choose when to show them in your App. Hydra development is still early, and patterns for different types of components and apps are still being developed. Join the discord to chat with the developers. Expects to be used in a NextJS project. Components that have function props do not work.
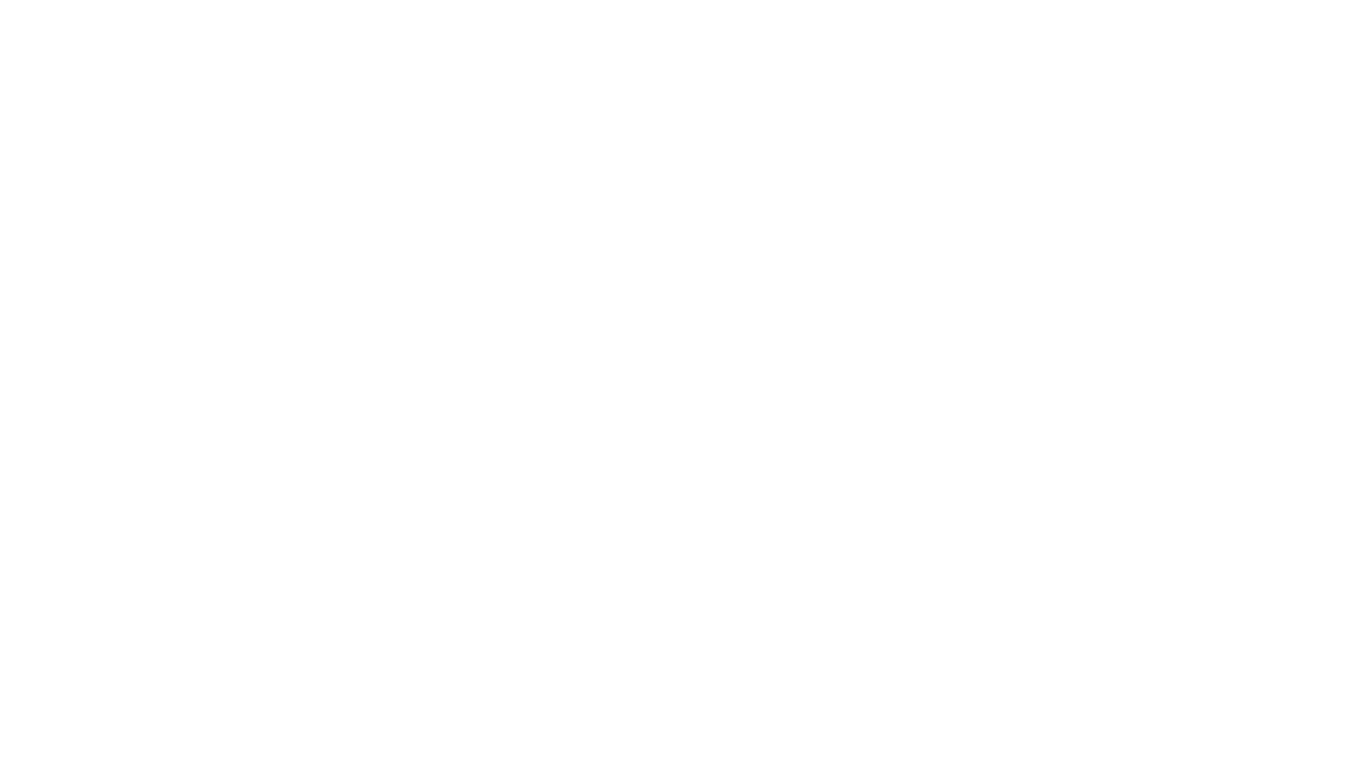
raglite
RAGLite is a Python toolkit for Retrieval-Augmented Generation (RAG) with PostgreSQL or SQLite. It offers configurable options for choosing LLM providers, database types, and rerankers. The toolkit is fast and permissive, utilizing lightweight dependencies and hardware acceleration. RAGLite provides features like PDF to Markdown conversion, multi-vector chunk embedding, optimal semantic chunking, hybrid search capabilities, adaptive retrieval, and improved output quality. It is extensible with a built-in Model Context Protocol server, customizable ChatGPT-like frontend, document conversion to Markdown, and evaluation tools. Users can configure RAGLite for various tasks like configuring, inserting documents, running RAG pipelines, computing query adapters, evaluating performance, running MCP servers, and serving frontends.
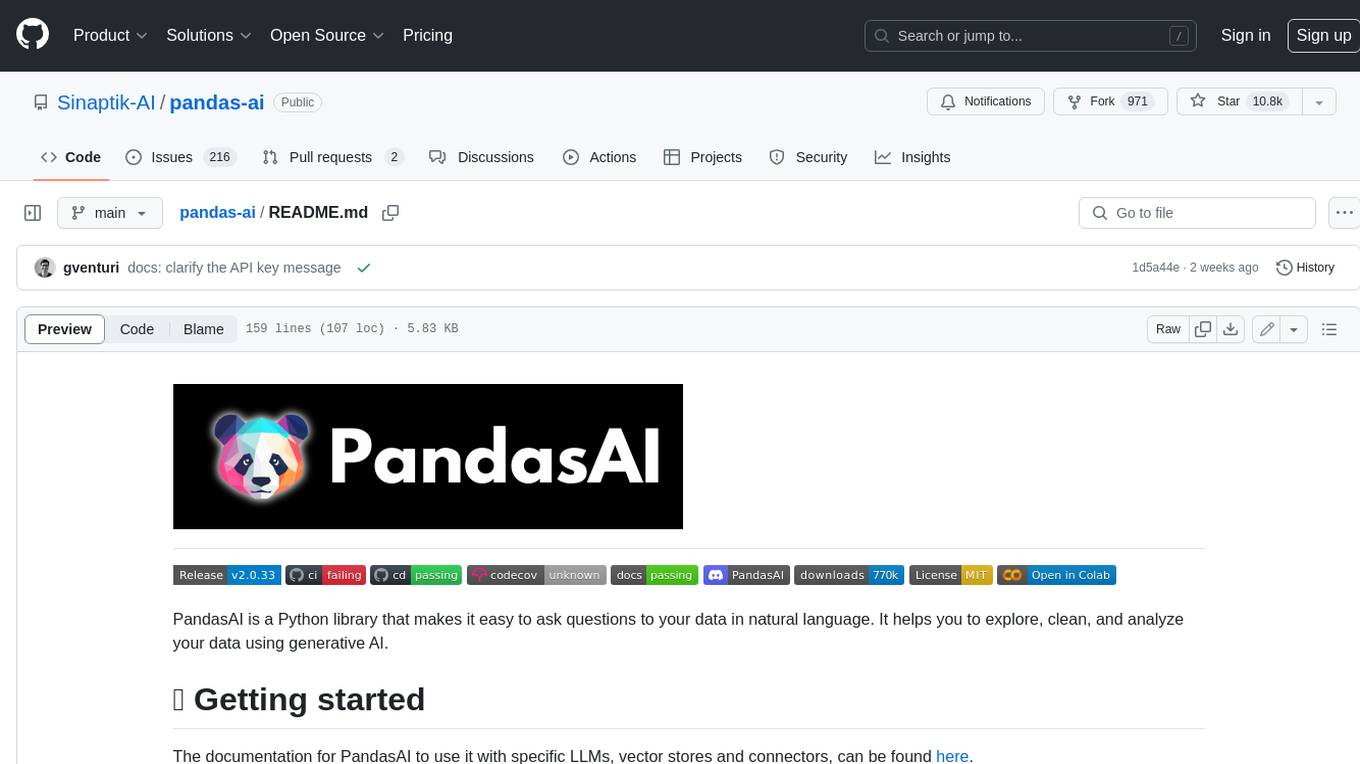
pandas-ai
PandasAI is a Python library that makes it easy to ask questions to your data in natural language. It helps you to explore, clean, and analyze your data using generative AI.
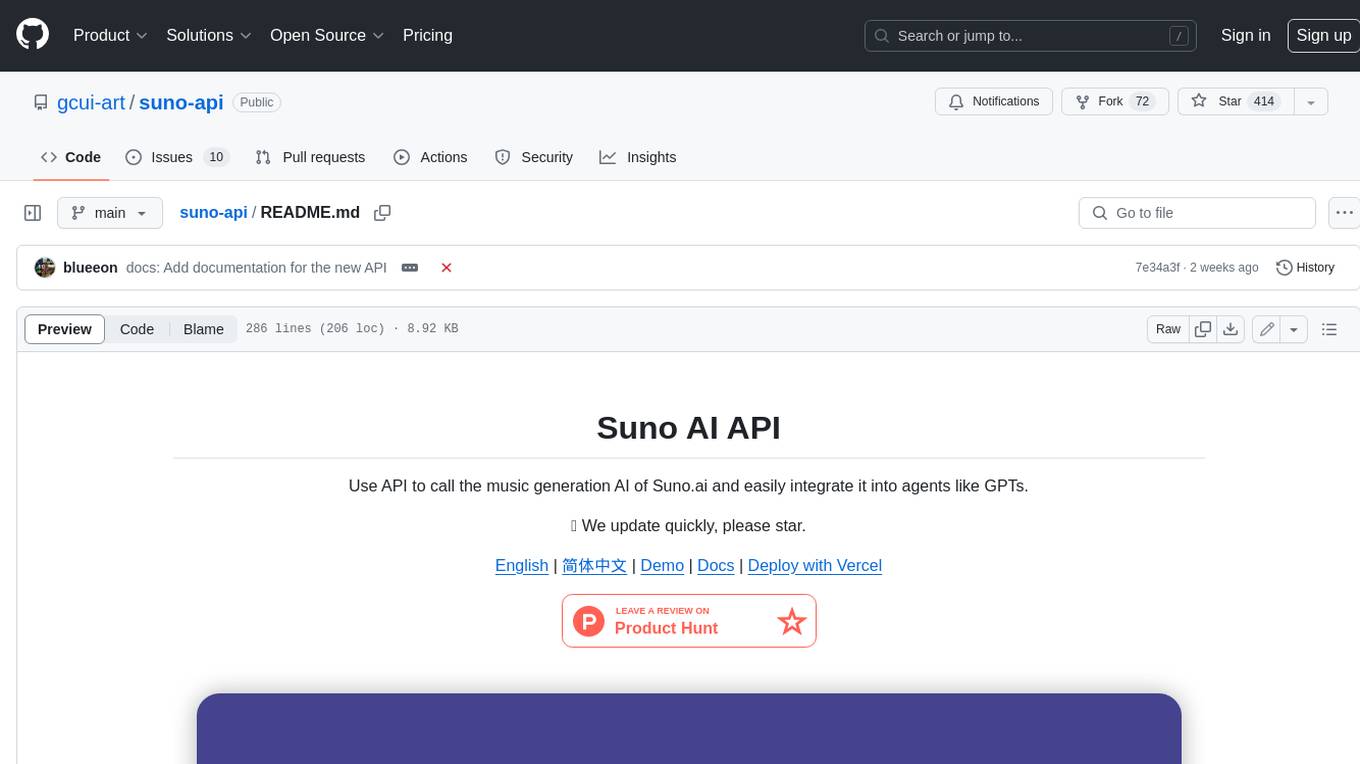
suno-api
Suno AI API is an open-source project that allows developers to integrate the music generation capabilities of Suno.ai into their own applications. The API provides a simple and convenient way to generate music, lyrics, and other audio content using Suno.ai's powerful AI models. With Suno AI API, developers can easily add music generation functionality to their apps, websites, and other projects.
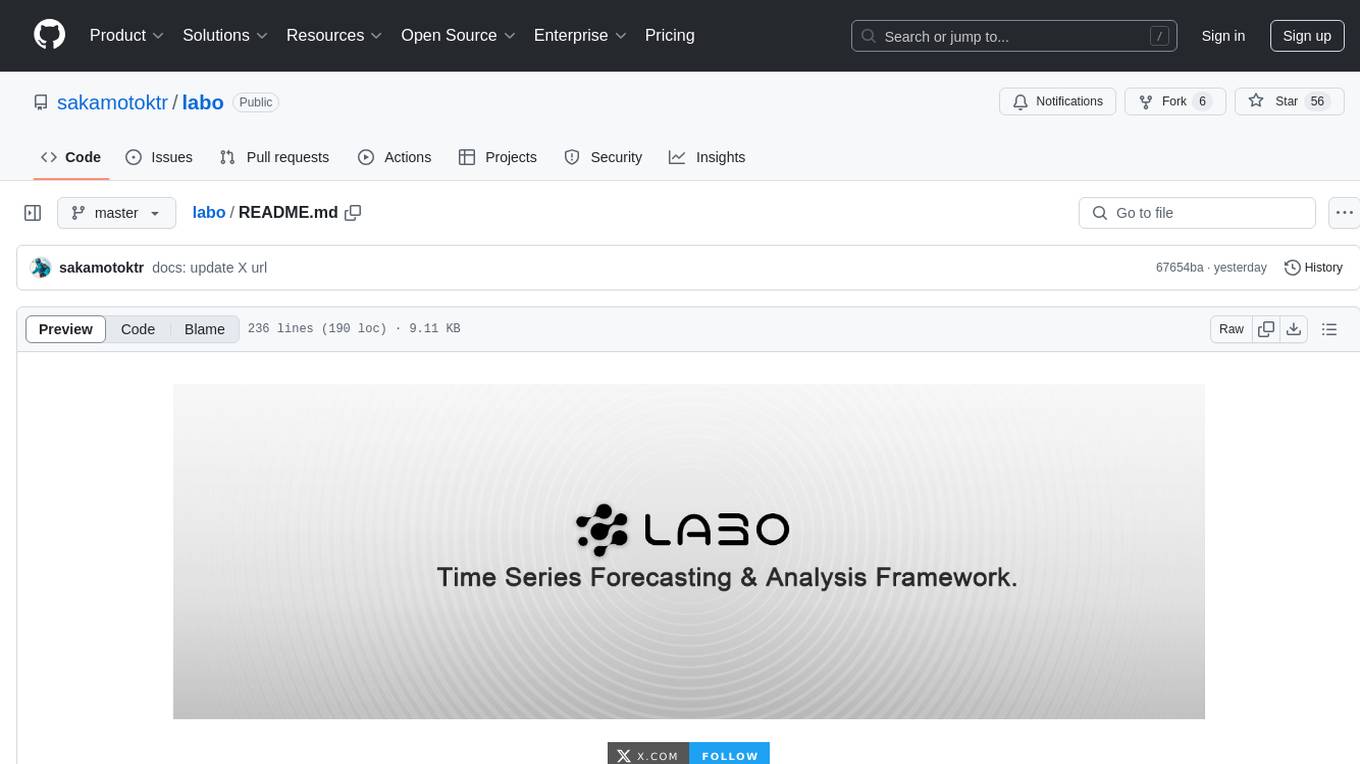
labo
LABO is a time series forecasting and analysis framework that integrates pre-trained and fine-tuned LLMs with multi-domain agent-based systems. It allows users to create and tune agents easily for various scenarios, such as stock market trend prediction and web public opinion analysis. LABO requires a specific runtime environment setup, including system requirements, Python environment, dependency installations, and configurations. Users can fine-tune their own models using LABO's Low-Rank Adaptation (LoRA) for computational efficiency and continuous model updates. Additionally, LABO provides a Python library for building model training pipelines and customizing agents for specific tasks.
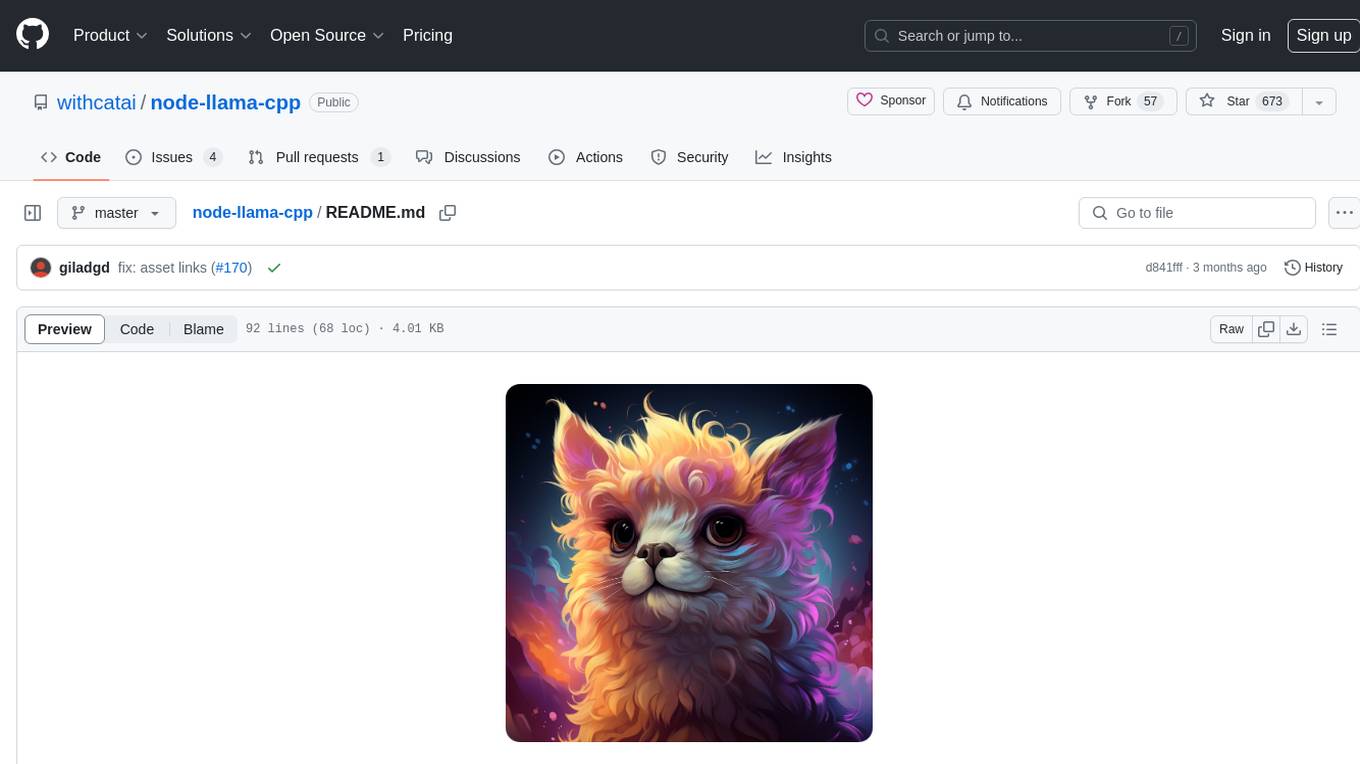
node-llama-cpp
node-llama-cpp is a tool that allows users to run AI models locally on their machines. It provides pre-built bindings with the option to build from source using cmake. Users can interact with text generation models, chat with models using a chat wrapper, and force models to generate output in a parseable format like JSON. The tool supports Metal and CUDA, offers CLI functionality for chatting with models without coding, and ensures up-to-date compatibility with the latest version of llama.cpp. Installation includes pre-built binaries for macOS, Linux, and Windows, with the option to build from source if binaries are not available for the platform.
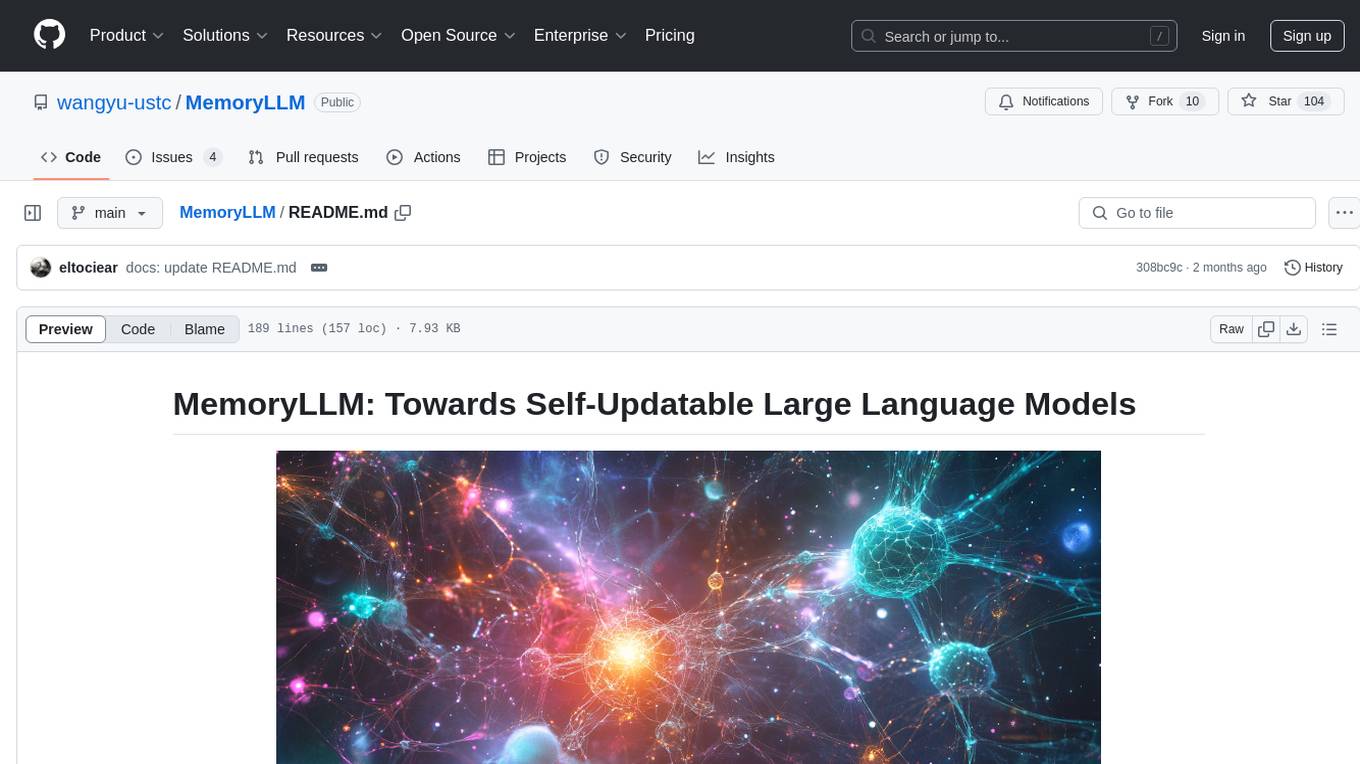
MemoryLLM
MemoryLLM is a large language model designed for self-updating capabilities. It offers pretrained models with different memory capacities and features, such as chat models. The repository provides training code, evaluation scripts, and datasets for custom experiments. MemoryLLM aims to enhance knowledge retention and performance on various natural language processing tasks.
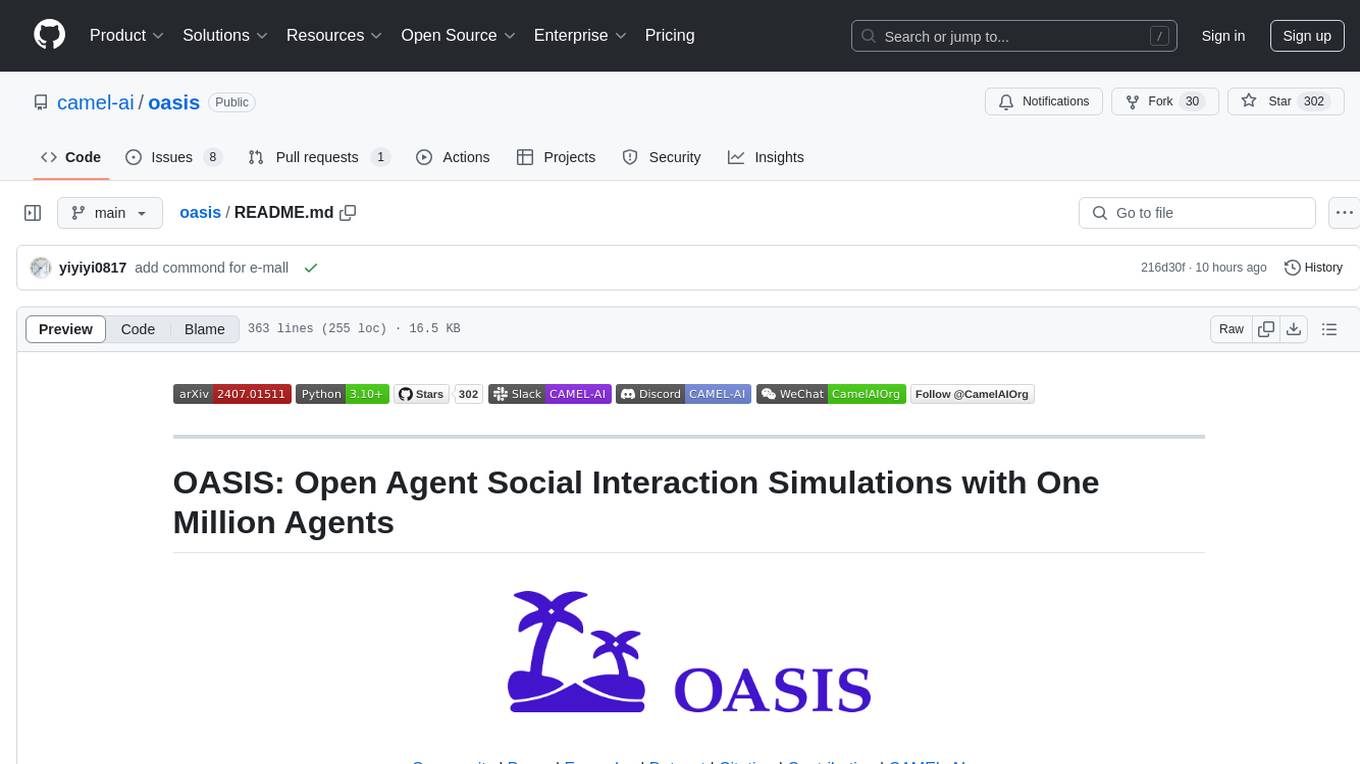
oasis
OASIS is a scalable, open-source social media simulator that integrates large language models with rule-based agents to realistically mimic the behavior of up to one million users on platforms like Twitter and Reddit. It facilitates the study of complex social phenomena such as information spread, group polarization, and herd behavior, offering a versatile tool for exploring diverse social dynamics and user interactions in digital environments. With features like scalability, dynamic environments, diverse action spaces, and integrated recommendation systems, OASIS provides a comprehensive platform for simulating social media interactions at a large scale.
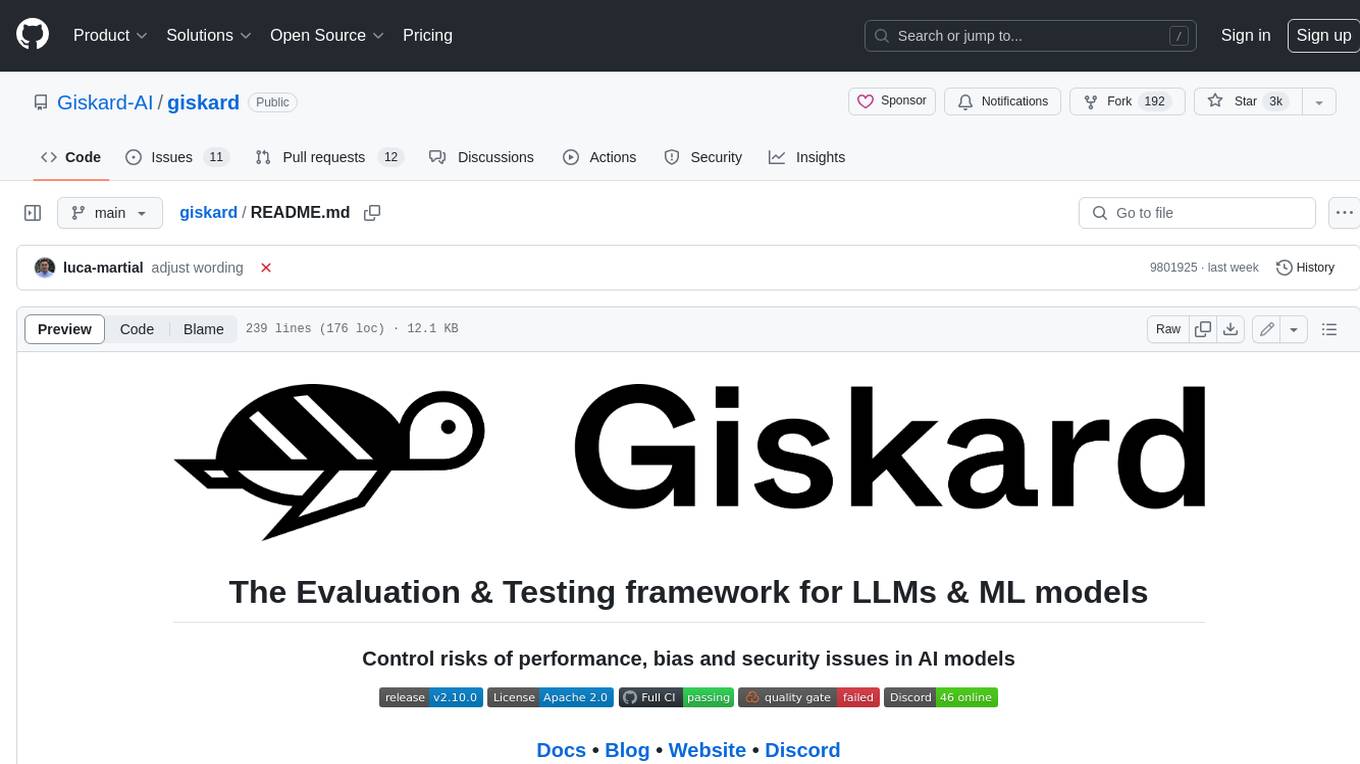
giskard
Giskard is an open-source Python library that automatically detects performance, bias & security issues in AI applications. The library covers LLM-based applications such as RAG agents, all the way to traditional ML models for tabular data.
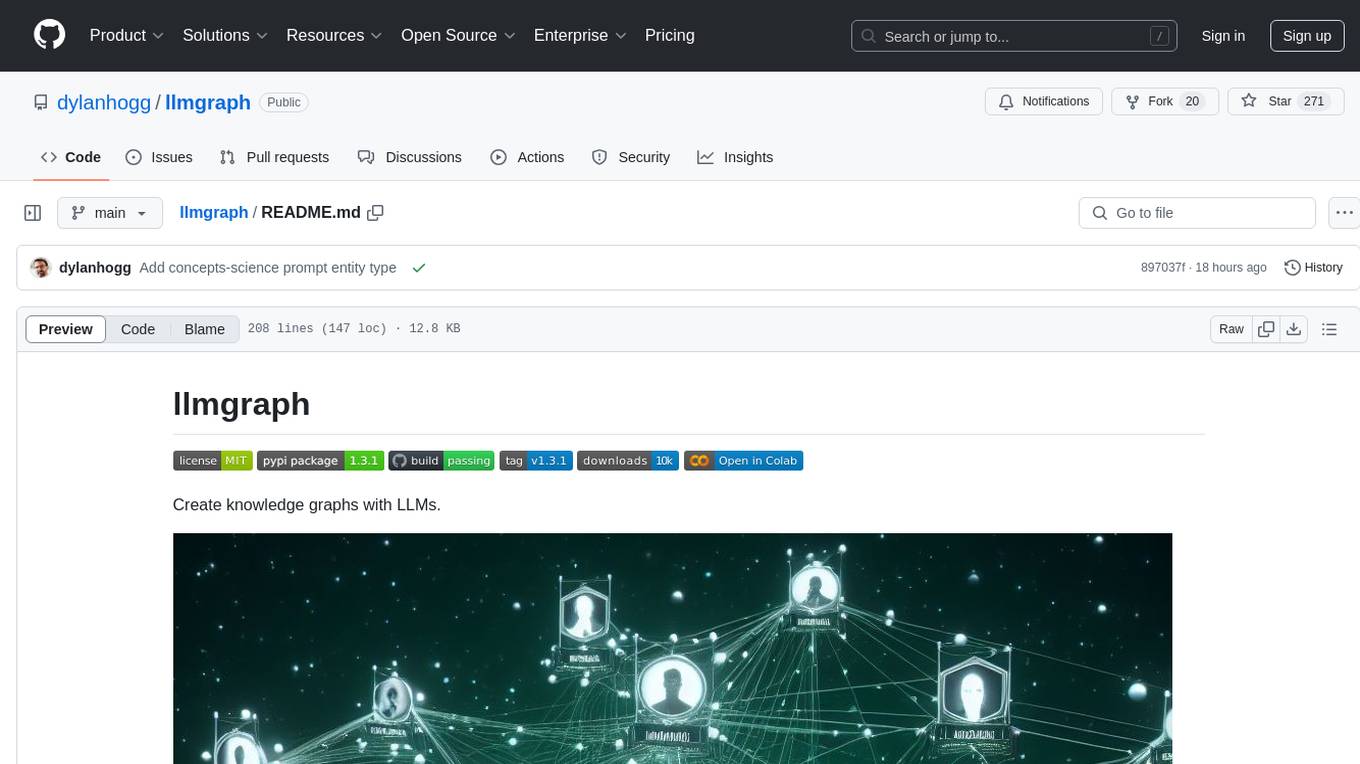
llmgraph
llmgraph is a tool that enables users to create knowledge graphs in GraphML, GEXF, and HTML formats by extracting world knowledge from large language models (LLMs) like ChatGPT. It supports various entity types and relationships, offers cache support for efficient graph growth, and provides insights into LLM costs. Users can customize the model used and interact with different LLM providers. The tool allows users to generate interactive graphs based on a specified entity type and Wikipedia link, making it a valuable resource for knowledge graph creation and exploration.
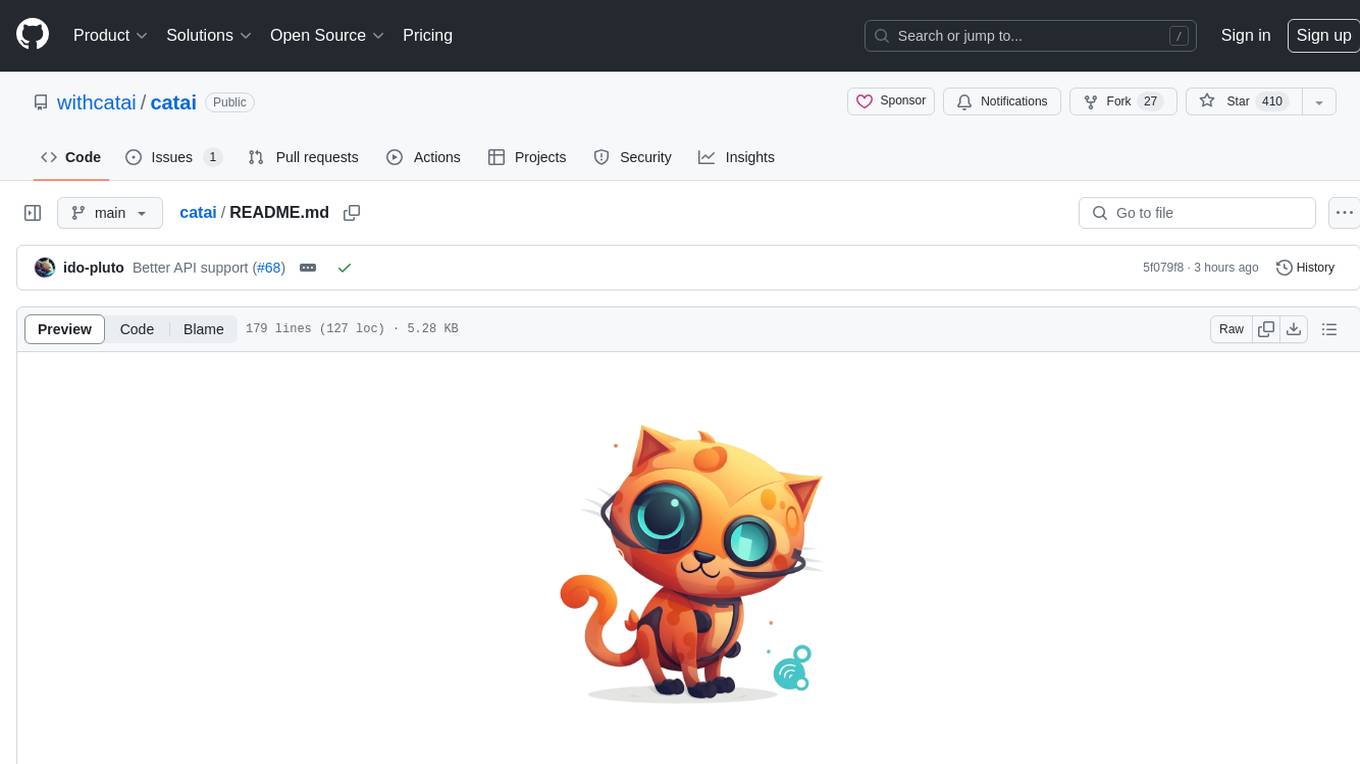
catai
CatAI is a tool that allows users to run GGUF models on their computer with a chat UI. It serves as a local AI assistant inspired by Node-Llama-Cpp and Llama.cpp. The tool provides features such as auto-detecting programming language, showing original messages by clicking on user icons, real-time text streaming, and fast model downloads. Users can interact with the tool through a CLI that supports commands for installing, listing, setting, serving, updating, and removing models. CatAI is cross-platform and supports Windows, Linux, and Mac. It utilizes node-llama-cpp and offers a simple API for asking model questions. Additionally, developers can integrate the tool with node-llama-cpp@beta for model management and chatting. The configuration can be edited via the web UI, and contributions to the project are welcome. The tool is licensed under Llama.cpp's license.
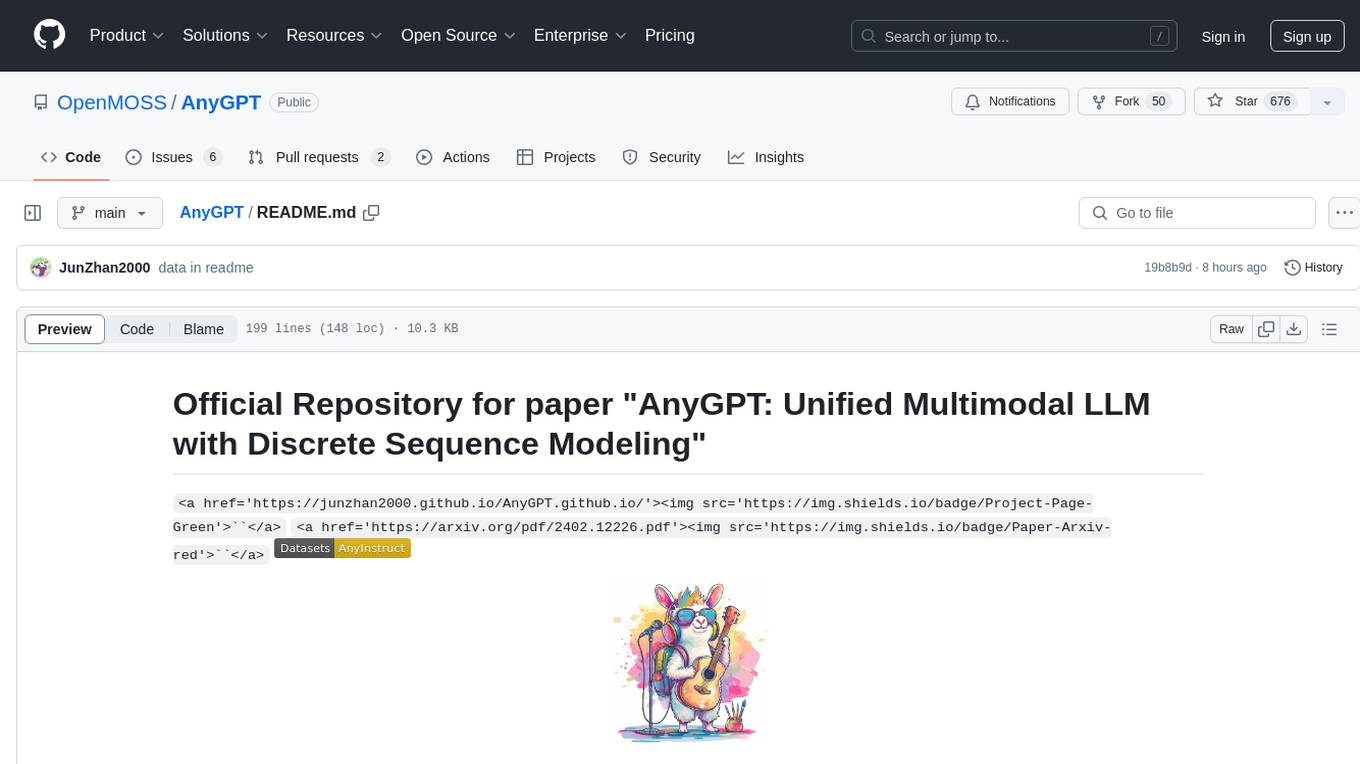
AnyGPT
AnyGPT is a unified multimodal language model that utilizes discrete representations for processing various modalities like speech, text, images, and music. It aligns the modalities for intermodal conversions and text processing. AnyInstruct dataset is constructed for generative models. The model proposes a generative training scheme using Next Token Prediction task for training on a Large Language Model (LLM). It aims to compress vast multimodal data on the internet into a single model for emerging capabilities. The tool supports tasks like text-to-image, image captioning, ASR, TTS, text-to-music, and music captioning.
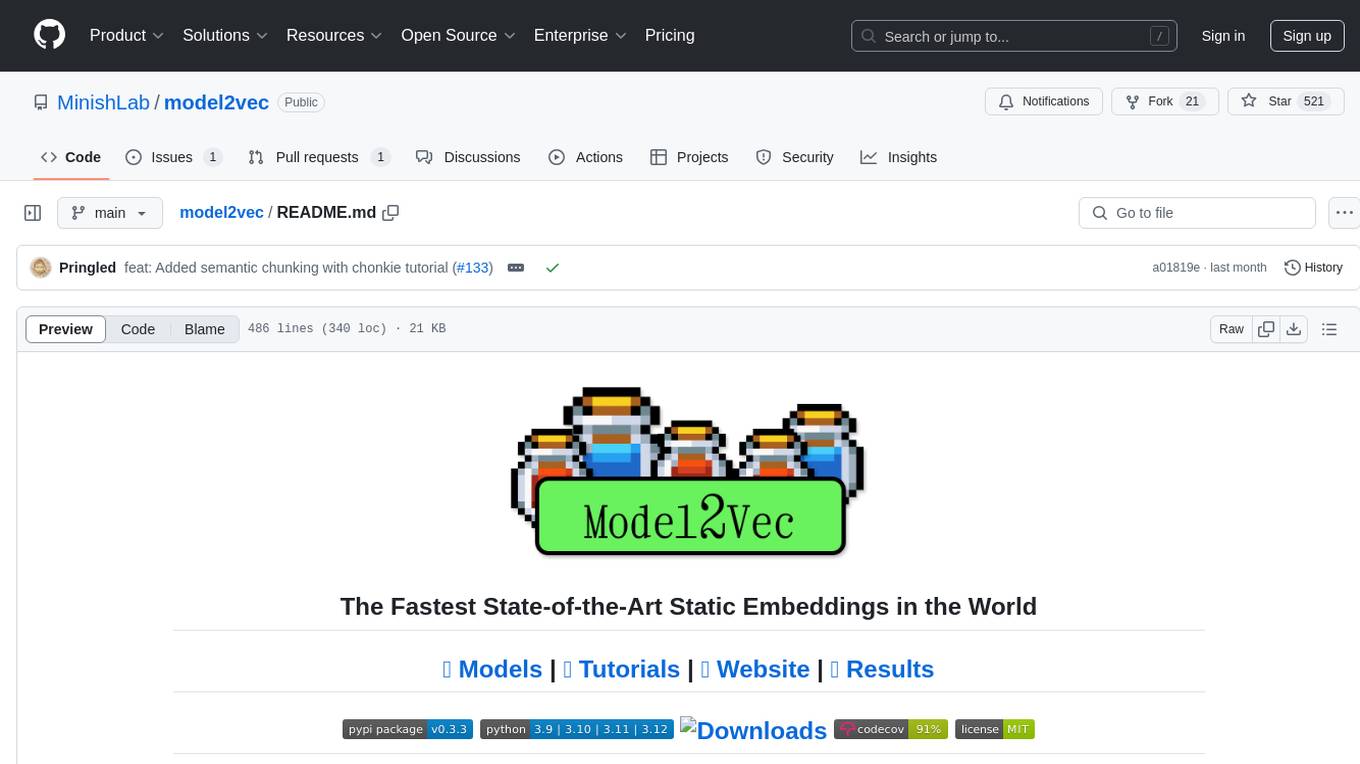
model2vec
Model2Vec is a technique to turn any sentence transformer into a really small static model, reducing model size by 15x and making the models up to 500x faster, with a small drop in performance. It outperforms other static embedding models like GLoVe and BPEmb, is lightweight with only `numpy` as a major dependency, offers fast inference, dataset-free distillation, and is integrated into Sentence Transformers, txtai, and Chonkie. Model2Vec creates powerful models by passing a vocabulary through a sentence transformer model, reducing dimensionality using PCA, and weighting embeddings using zipf weighting. Users can distill their own models or use pre-trained models from the HuggingFace hub. Evaluation can be done using the provided evaluation package. Model2Vec is licensed under MIT.
For similar tasks
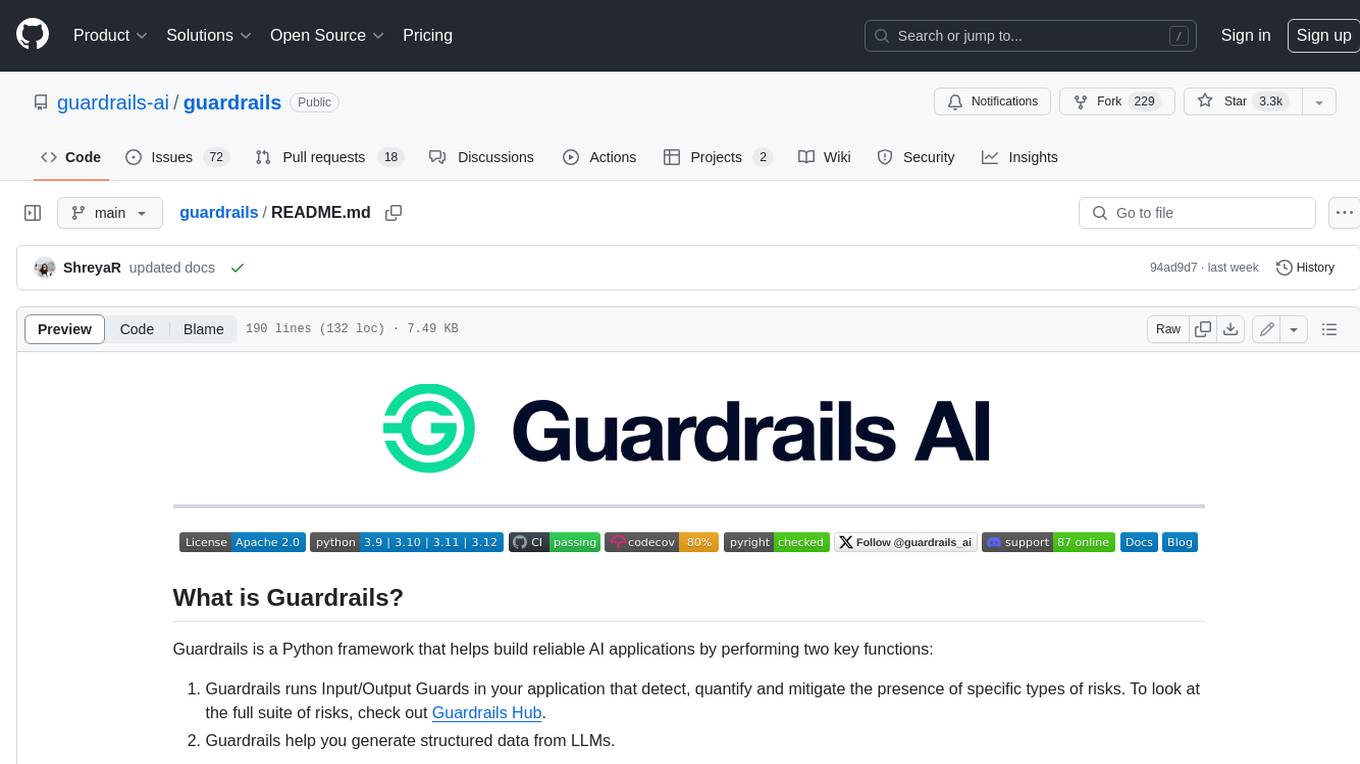
guardrails
Guardrails is a Python framework that helps build reliable AI applications by performing two key functions: 1. Guardrails runs Input/Output Guards in your application that detect, quantify and mitigate the presence of specific types of risks. To look at the full suite of risks, check out Guardrails Hub. 2. Guardrails help you generate structured data from LLMs.
For similar jobs
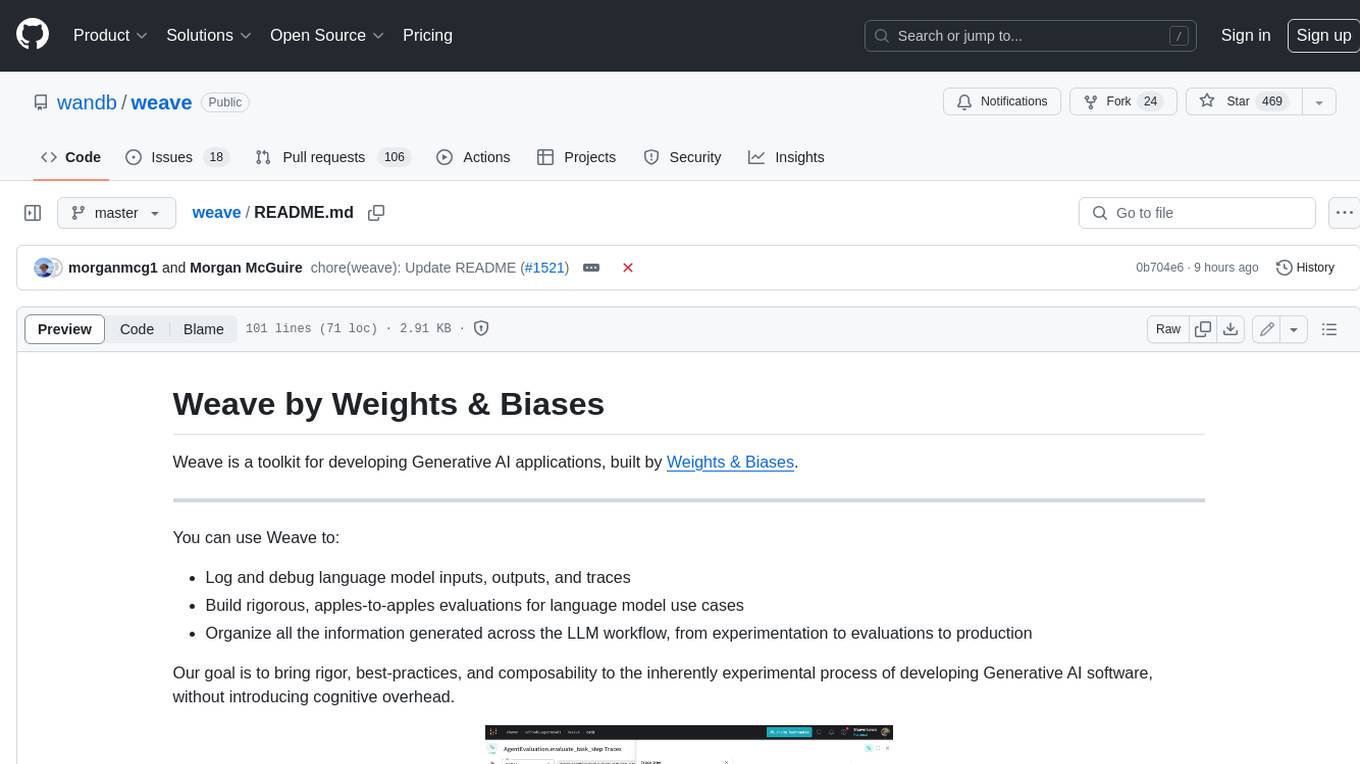
weave
Weave is a toolkit for developing Generative AI applications, built by Weights & Biases. With Weave, you can log and debug language model inputs, outputs, and traces; build rigorous, apples-to-apples evaluations for language model use cases; and organize all the information generated across the LLM workflow, from experimentation to evaluations to production. Weave aims to bring rigor, best-practices, and composability to the inherently experimental process of developing Generative AI software, without introducing cognitive overhead.
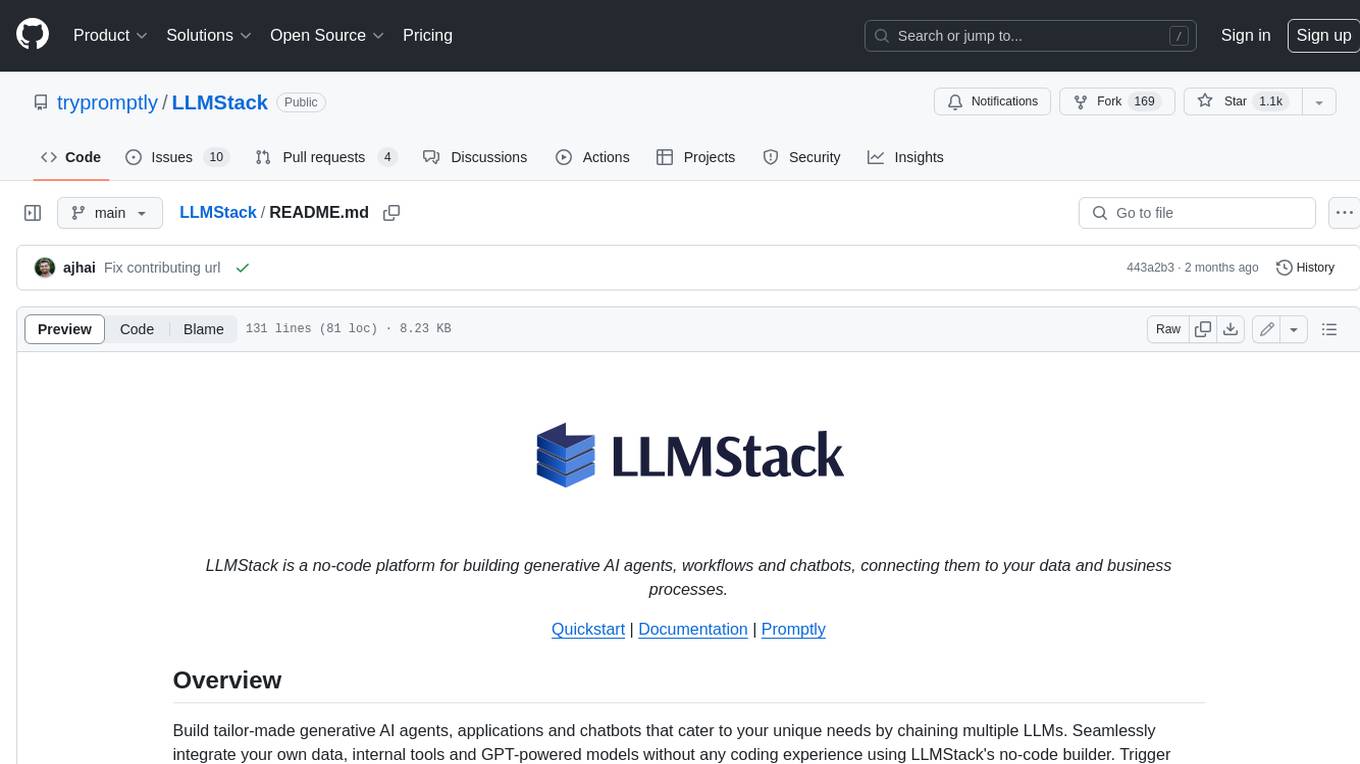
LLMStack
LLMStack is a no-code platform for building generative AI agents, workflows, and chatbots. It allows users to connect their own data, internal tools, and GPT-powered models without any coding experience. LLMStack can be deployed to the cloud or on-premise and can be accessed via HTTP API or triggered from Slack or Discord.
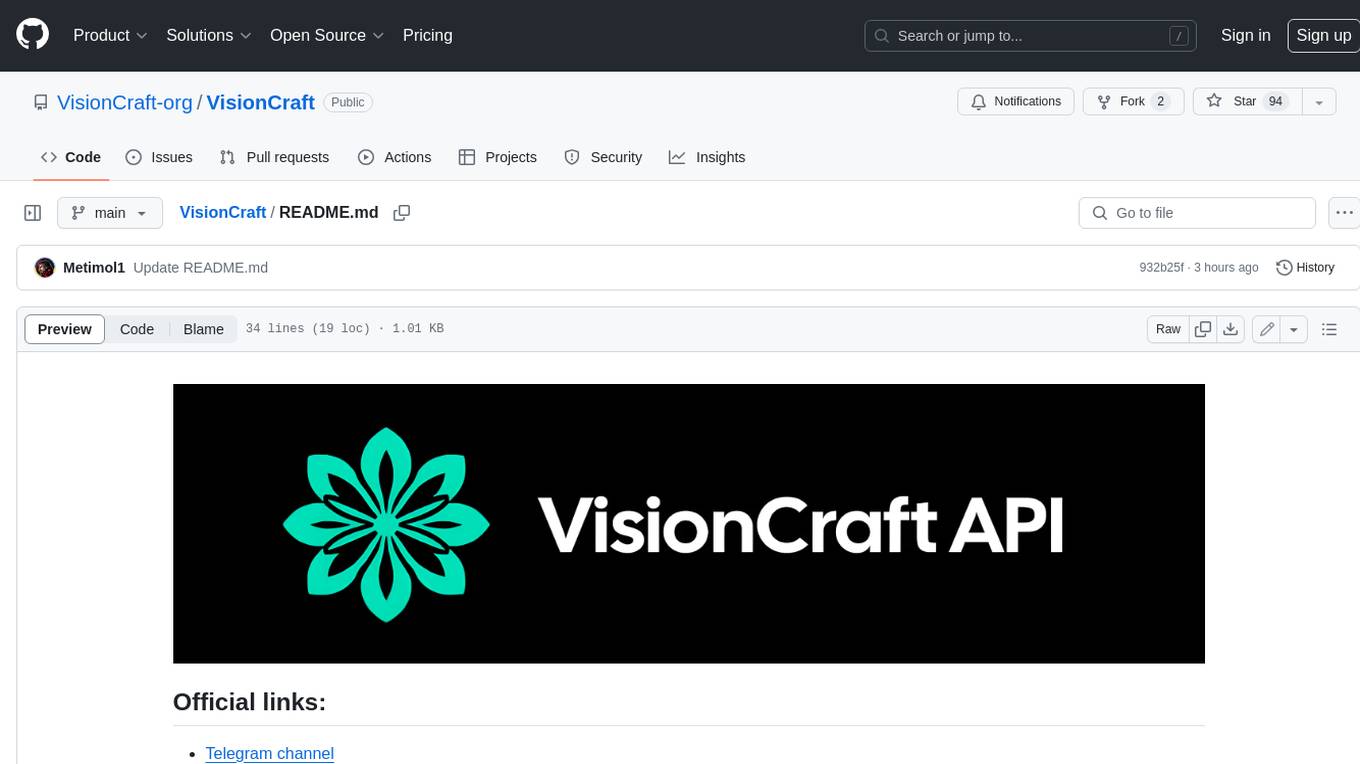
VisionCraft
The VisionCraft API is a free API for using over 100 different AI models. From images to sound.
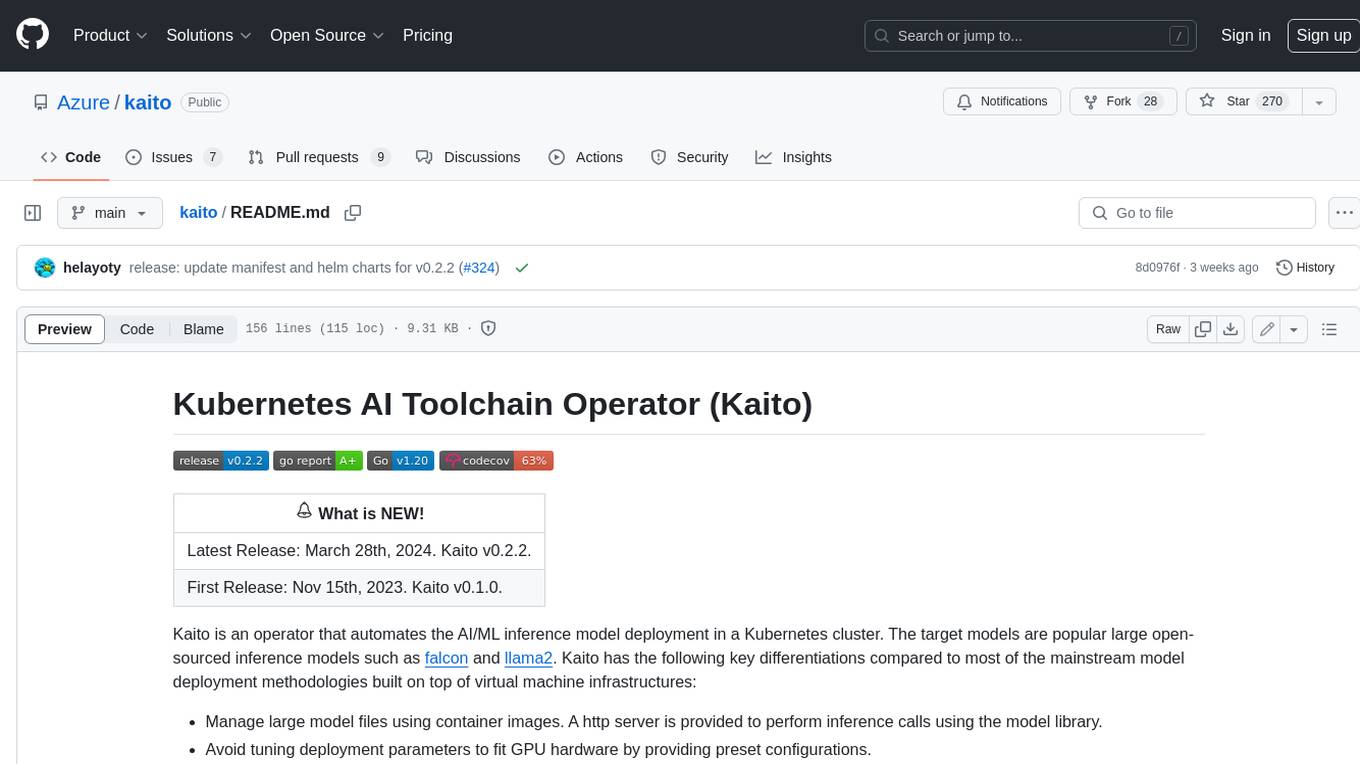
kaito
Kaito is an operator that automates the AI/ML inference model deployment in a Kubernetes cluster. It manages large model files using container images, avoids tuning deployment parameters to fit GPU hardware by providing preset configurations, auto-provisions GPU nodes based on model requirements, and hosts large model images in the public Microsoft Container Registry (MCR) if the license allows. Using Kaito, the workflow of onboarding large AI inference models in Kubernetes is largely simplified.
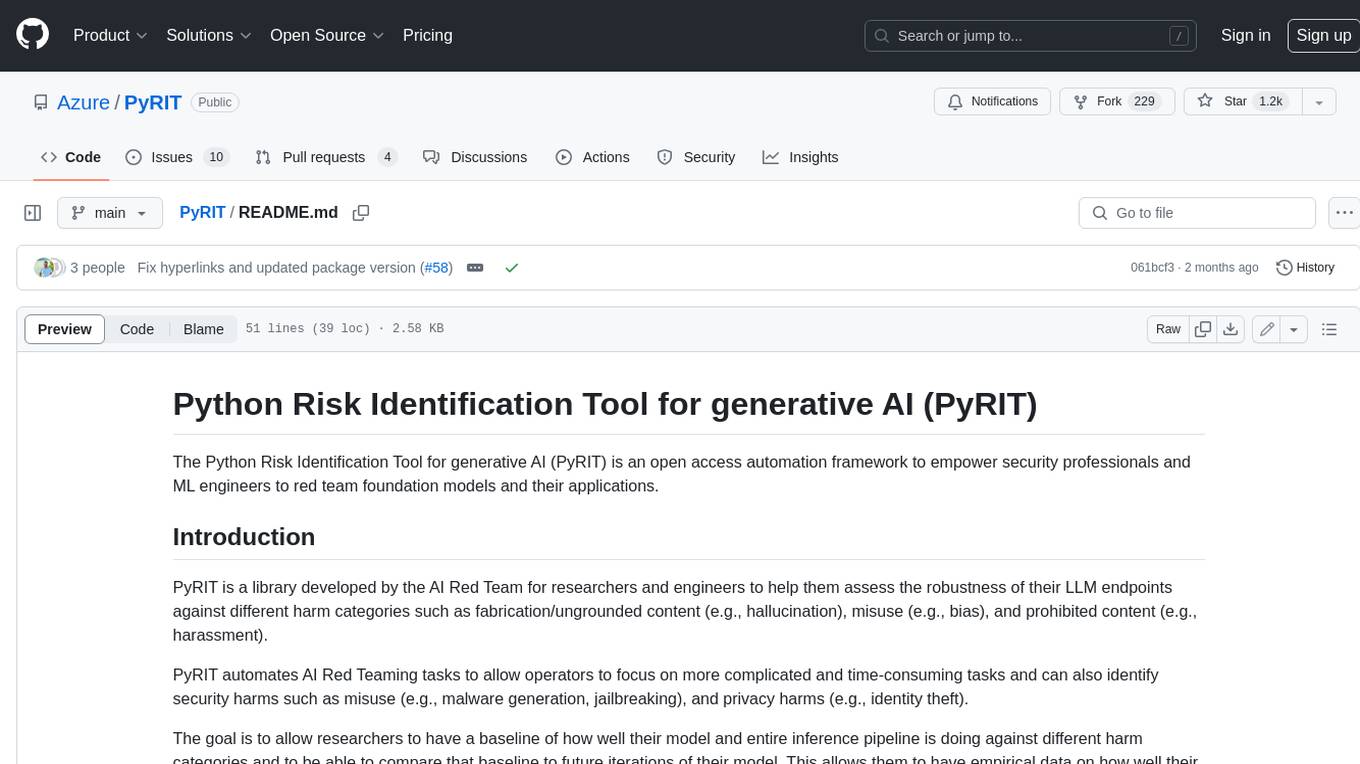
PyRIT
PyRIT is an open access automation framework designed to empower security professionals and ML engineers to red team foundation models and their applications. It automates AI Red Teaming tasks to allow operators to focus on more complicated and time-consuming tasks and can also identify security harms such as misuse (e.g., malware generation, jailbreaking), and privacy harms (e.g., identity theft). The goal is to allow researchers to have a baseline of how well their model and entire inference pipeline is doing against different harm categories and to be able to compare that baseline to future iterations of their model. This allows them to have empirical data on how well their model is doing today, and detect any degradation of performance based on future improvements.
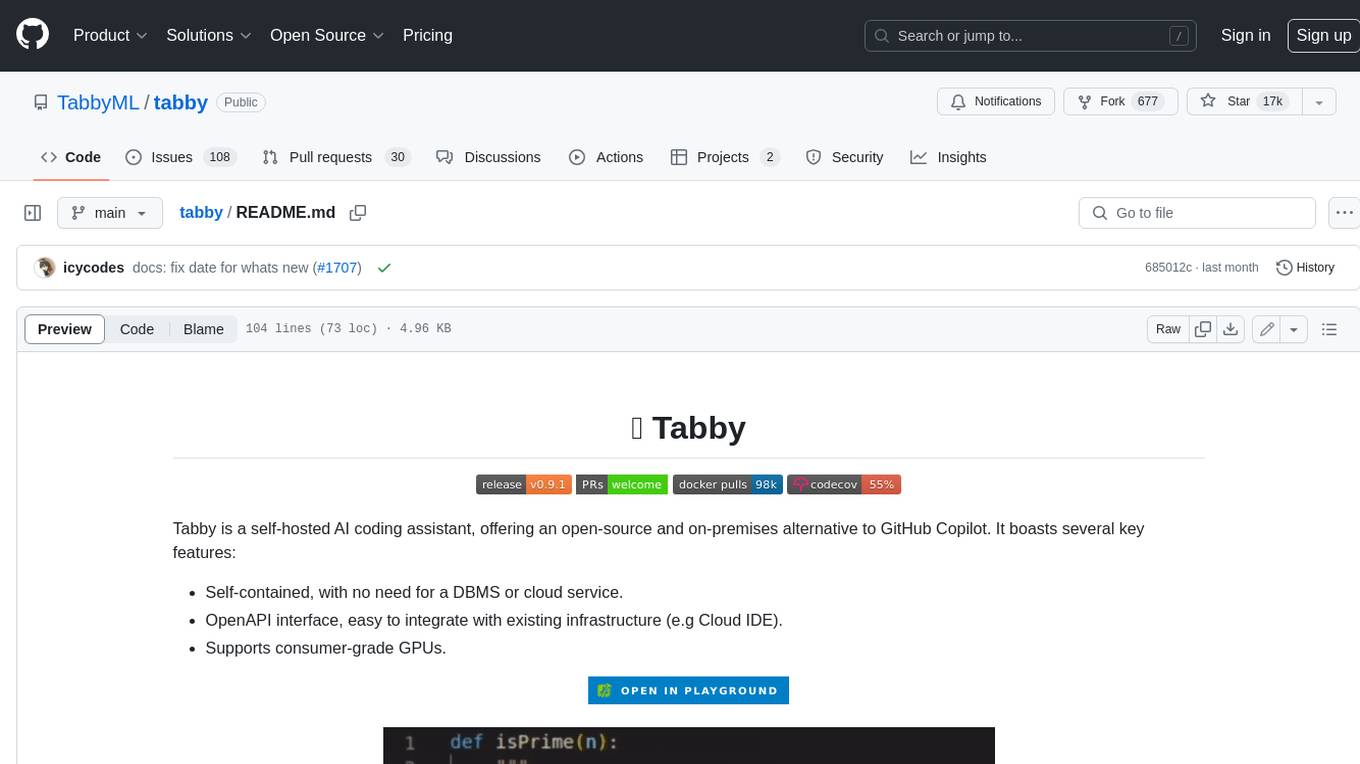
tabby
Tabby is a self-hosted AI coding assistant, offering an open-source and on-premises alternative to GitHub Copilot. It boasts several key features: * Self-contained, with no need for a DBMS or cloud service. * OpenAPI interface, easy to integrate with existing infrastructure (e.g Cloud IDE). * Supports consumer-grade GPUs.
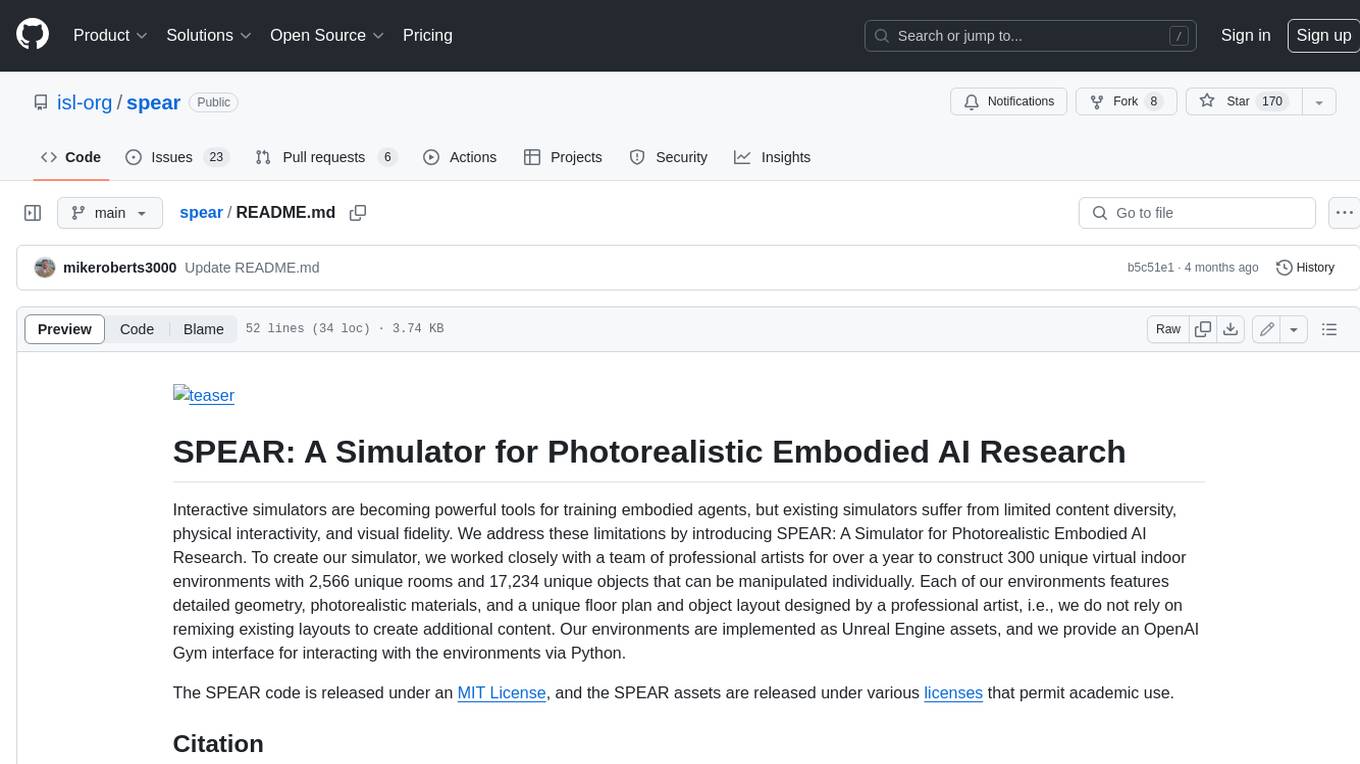
spear
SPEAR (Simulator for Photorealistic Embodied AI Research) is a powerful tool for training embodied agents. It features 300 unique virtual indoor environments with 2,566 unique rooms and 17,234 unique objects that can be manipulated individually. Each environment is designed by a professional artist and features detailed geometry, photorealistic materials, and a unique floor plan and object layout. SPEAR is implemented as Unreal Engine assets and provides an OpenAI Gym interface for interacting with the environments via Python.
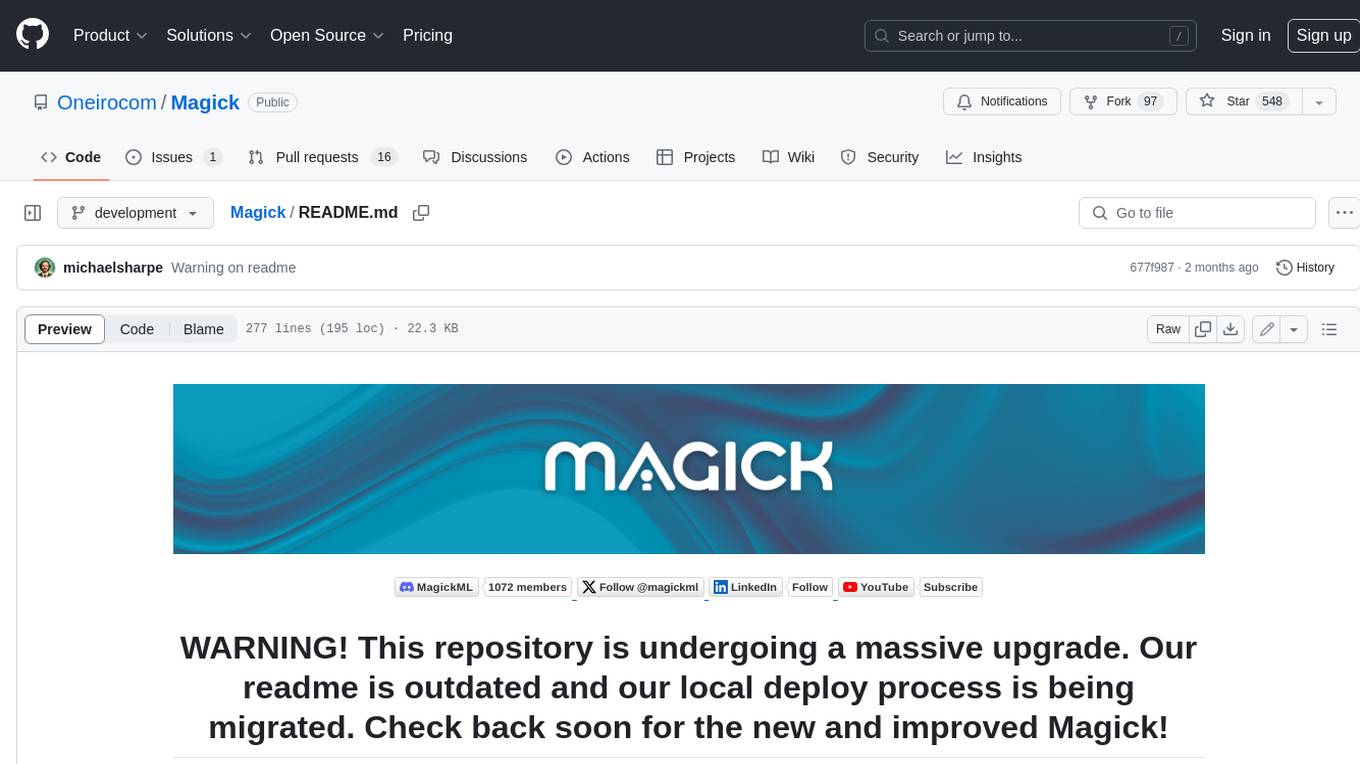
Magick
Magick is a groundbreaking visual AIDE (Artificial Intelligence Development Environment) for no-code data pipelines and multimodal agents. Magick can connect to other services and comes with nodes and templates well-suited for intelligent agents, chatbots, complex reasoning systems and realistic characters.