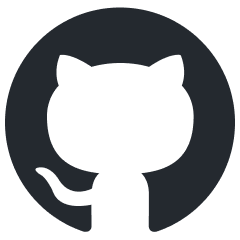
TheoremExplainAgent
Official Repo for "TheoremExplainAgent: Towards Multimodal Explanations for LLM Theorem Understanding"
Stars: 966
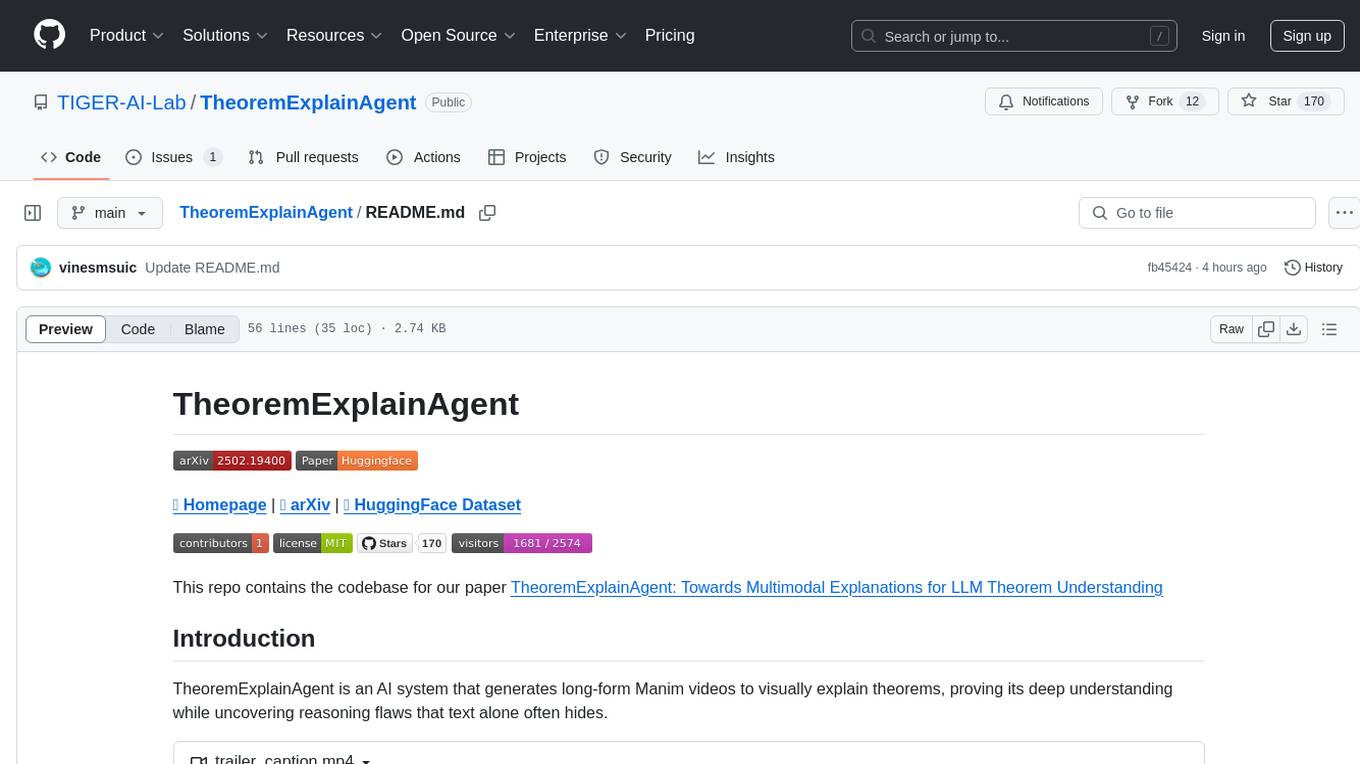
TheoremExplainAgent is an AI system that generates long-form Manim videos to visually explain theorems, proving its deep understanding while uncovering reasoning flaws that text alone often hides. The codebase for the paper 'TheoremExplainAgent: Towards Multimodal Explanations for LLM Theorem Understanding' is available in this repository. It provides a tool for creating multimodal explanations for theorem understanding using AI technology.
README:
π Homepage | π arXiv | π€ HuggingFace Dataset
This repo contains the codebase for our paper TheoremExplainAgent: Towards Multimodal Explanations for LLM Theorem Understanding
TheoremExplainAgent is an AI system that generates long-form Manim videos to visually explain theorems, proving its deep understanding while uncovering reasoning flaws that text alone often hides.
https://github.com/user-attachments/assets/17f2f4f2-8f2c-4abc-b377-ac92ebda69f3
- 2025 Mar 3: Generation code and Evaluation code released. Thanks for the wait!
- Setting up conda environment
conda create --name tea python=3.12.8
conda activate tea
pip install -r requirements.txt
-
You may also need to install latex and other dependencies for Manim Community. Look at Manim Installation Docs for more details.
-
Then Download the Kokoro model and voices using the commands to enable TTS service.
mkdir -p models && wget -P models https://github.com/thewh1teagle/kokoro-onnx/releases/download/model-files/kokoro-v0_19.onnx && wget -P models https://github.com/thewh1teagle/kokoro-onnx/releases/download/model-files/voices.bin
- Create
.env
based on.env.template
, filling in the environmental variables according to the models you choose to use. See LiteLLM for reference.
Your .env
file should look like the following:
# OpenAI
OPENAI_API_KEY=""
# Azure OpenAI
AZURE_API_KEY=""
AZURE_API_BASE=""
AZURE_API_VERSION=""
# Google Vertex AI
VERTEXAI_PROJECT=""
VERTEXAI_LOCATION=""
GOOGLE_APPLICATION_CREDENTIALS=""
# Google Gemini
GEMINI_API_KEY=""
...
# Kokoro TTS Settings
KOKORO_MODEL_PATH="models/kokoro-v0_19.onnx"
KOKORO_VOICES_PATH="models/voices.bin"
KOKORO_DEFAULT_VOICE="af"
KOKORO_DEFAULT_SPEED="1.0"
KOKORO_DEFAULT_LANG="en-us"
Fill in the API keys according to the model you wanted to use.
- Configure Python path. Note that you need to configure the python path to make it work. Otherwise you may encounter import issues (like not being able to import src etc.)
export PYTHONPATH=$(pwd):$PYTHONPATH
Look at the FAQ section in this README doc if you encountered any errors.
The model naming follows the LiteLLM convention. For details on how models should be named, please refer to the LiteLLM documentation.
python generate_video.py \
--model "openai/o3-mini" \
--helper_model "openai/o3-mini" \
--output_dir "output/your_exp_name" \
--topic "your_topic" \
--context "description of your topic, e.g. 'This is a topic about the properties of a triangle'" \
Example:
python generate_video.py \
--model "openai/o3-mini" \
--helper_model "openai/o3-mini" \
--output_dir "output/my_exp_name" \
--topic "Big O notation" \
--context "most common type of asymptotic notation in computer science used to measure worst case complexity" \
python generate_video.py \
--model "openai/o3-mini" \
--helper_model "openai/o3-mini" \
--output_dir "output/my_exp_name" \
--theorems_path data/thb_easy/math.json \
--max_scene_concurrency 7 \
--max_topic_concurrency 20 \
Before using RAG, download the RAG documentation from this Google Drive link. After downloading, unzip the file. For example, if you unzip it to data/rag/manim_docs
, then you should set --manim_docs_path
to data/rag/manim_docs
. The vector database will be created the first time you run with RAG.
python generate_video.py \
--model "openai/o3-mini" \
--helper_model "openai/o3-mini" \
--output_dir "output/with_rag/o3-mini/vtutorbench_easy/math" \
--topic "Big O notation" \
--context "most common type of asymptotic notation in computer science used to measure worst case complexity" \
--use_rag \
--chroma_db_path "data/rag/chroma_db" \
--manim_docs_path "data/rag/manim_docs" \
--embedding_model "vertex_ai/text-embedding-005"
We support more options for generation, see below for more details:
usage: generate_video.py [-h]
[--model]
[--topic TOPIC] [--context CONTEXT]
[--helper_model]
[--only_gen_vid] [--only_combine] [--peek_existing_videos] [--output_dir OUTPUT_DIR] [--theorems_path THEOREMS_PATH]
[--sample_size SAMPLE_SIZE] [--verbose] [--max_retries MAX_RETRIES] [--use_rag] [--use_visual_fix_code]
[--chroma_db_path CHROMA_DB_PATH] [--manim_docs_path MANIM_DOCS_PATH]
[--embedding_model {azure/text-embedding-3-large,vertex_ai/text-embedding-005}] [--use_context_learning]
[--context_learning_path CONTEXT_LEARNING_PATH] [--use_langfuse] [--max_scene_concurrency MAX_SCENE_CONCURRENCY]
[--max_topic_concurrency MAX_TOPIC_CONCURRENCY] [--debug_combine_topic DEBUG_COMBINE_TOPIC] [--only_plan] [--check_status]
[--only_render] [--scenes SCENES [SCENES ...]]
Generate Manim videos using AI
options:
-h, --help show this help message and exit
--model Select the AI model to use
--topic TOPIC Topic to generate videos for
--context CONTEXT Context of the topic
--helper_model Select the helper model to use
--only_gen_vid Only generate videos to existing plans
--only_combine Only combine videos
--peek_existing_videos, --peek
Peek at existing videos
--output_dir OUTPUT_DIR
Output directory
--theorems_path THEOREMS_PATH
Path to theorems json file
--sample_size SAMPLE_SIZE, --sample SAMPLE_SIZE
Number of theorems to sample
--verbose Print verbose output
--max_retries MAX_RETRIES
Maximum number of retries for code generation
--use_rag, --rag Use Retrieval Augmented Generation
--use_visual_fix_code, --visual_fix_code
Use VLM to fix code with rendered visuals
--chroma_db_path CHROMA_DB_PATH
Path to Chroma DB
--manim_docs_path MANIM_DOCS_PATH
Path to manim docs
--embedding_model {azure/text-embedding-3-large,vertex_ai/text-embedding-005}
Select the embedding model to use
--use_context_learning
Use context learning with example Manim code
--context_learning_path CONTEXT_LEARNING_PATH
Path to context learning examples
--use_langfuse Enable Langfuse logging
--max_scene_concurrency MAX_SCENE_CONCURRENCY
Maximum number of scenes to process concurrently
--max_topic_concurrency MAX_TOPIC_CONCURRENCY
Maximum number of topics to process concurrently
--debug_combine_topic DEBUG_COMBINE_TOPIC
Debug combine videos
--only_plan Only generate scene outline and implementation plans
--check_status Check planning and code status for all theorems
--only_render Only render scenes without combining videos
--scenes SCENES [SCENES ...]
Specific scenes to process (if theorems_path is provided)
Note that Gemini and GPT4o is required for evaluation.
Currently, evaluation requires a video file and a subtitle file (SRT format).
Video evaluation:
usage: evaluate.py [-h]
[--model_text {gemini/gemini-1.5-pro-002,gemini/gemini-1.5-flash-002,gemini/gemini-2.0-flash-001,vertex_ai/gemini-1.5-flash-002,vertex_ai/gemini-1.5-pro-002,vertex_ai/gemini-2.0-flash-001,openai/o3-mini,gpt-4o,azure/gpt-4o,azure/gpt-4o-mini,bedrock/anthropic.claude-3-5-sonnet-20240620-v1:0,bedrock/anthropic.claude-3-5-sonnet-20241022-v2:0,bedrock/anthropic.claude-3-5-haiku-20241022-v1:0,bedrock/us.anthropic.claude-3-7-sonnet-20250219-v1:0}]
[--model_video {gemini/gemini-1.5-pro-002,gemini/gemini-2.0-flash-exp,gemini/gemini-2.0-pro-exp-02-05}]
[--model_image {gemini/gemini-1.5-pro-002,gemini/gemini-1.5-flash-002,gemini/gemini-2.0-flash-001,vertex_ai/gemini-1.5-flash-002,vertex_ai/gemini-1.5-pro-002,vertex_ai/gemini-2.0-flash-001,openai/o3-mini,gpt-4o,azure/gpt-4o,azure/gpt-4o-mini,bedrock/anthropic.claude-3-5-sonnet-20240620-v1:0,bedrock/anthropic.claude-3-5-sonnet-20241022-v2:0,bedrock/anthropic.claude-3-5-haiku-20241022-v1:0,bedrock/us.anthropic.claude-3-7-sonnet-20250219-v1:0}]
[--eval_type {text,video,image,all}] --file_path FILE_PATH --output_folder OUTPUT_FOLDER [--retry_limit RETRY_LIMIT] [--combine] [--bulk_evaluate] [--target_fps TARGET_FPS]
[--use_parent_folder_as_topic] [--max_workers MAX_WORKERS]
Automatic evaluation of theorem explanation videos with LLMs
options:
-h, --help show this help message and exit
--model_text {gemini/gemini-1.5-pro-002,gemini/gemini-1.5-flash-002,gemini/gemini-2.0-flash-001,vertex_ai/gemini-1.5-flash-002,vertex_ai/gemini-1.5-pro-002,vertex_ai/gemini-2.0-flash-001,openai/o3-mini,gpt-4o,azure/gpt-4o,azure/gpt-4o-mini,bedrock/anthropic.claude-3-5-sonnet-20240620-v1:0,bedrock/anthropic.claude-3-5-sonnet-20241022-v2:0,bedrock/anthropic.claude-3-5-haiku-20241022-v1:0,bedrock/us.anthropic.claude-3-7-sonnet-20250219-v1:0}
Select the AI model to use for text evaluation
--model_video {gemini/gemini-1.5-pro-002,gemini/gemini-2.0-flash-exp,gemini/gemini-2.0-pro-exp-02-05}
Select the AI model to use for video evaluation
--model_image {gemini/gemini-1.5-pro-002,gemini/gemini-1.5-flash-002,gemini/gemini-2.0-flash-001,vertex_ai/gemini-1.5-flash-002,vertex_ai/gemini-1.5-pro-002,vertex_ai/gemini-2.0-flash-001,openai/o3-mini,gpt-4o,azure/gpt-4o,azure/gpt-4o-mini,bedrock/anthropic.claude-3-5-sonnet-20240620-v1:0,bedrock/anthropic.claude-3-5-sonnet-20241022-v2:0,bedrock/anthropic.claude-3-5-haiku-20241022-v1:0,bedrock/us.anthropic.claude-3-7-sonnet-20250219-v1:0}
Select the AI model to use for image evaluation
--eval_type {text,video,image,all}
Type of evaluation to perform
--file_path FILE_PATH
Path to a file or a theorem folder
--output_folder OUTPUT_FOLDER
Directory to store the evaluation files
--retry_limit RETRY_LIMIT
Number of retry attempts for each inference
--combine Combine all results into a single JSON file
--bulk_evaluate Evaluate a folder of theorems together
--target_fps TARGET_FPS
Target FPS for video processing. If not set, original video FPS will be used
--use_parent_folder_as_topic
Use parent folder name as topic name for single file evaluation
--max_workers MAX_WORKERS
Maximum number of concurrent workers for parallel processing
- For
file_path
, it is recommended to pass a folder containing both an MP4 file and an SRT file.
Q: Error src.utils.kokoro_voiceover import KokoroService # You MUST import like this as this is our custom voiceover service. ^^^^^^^^^^^^^^^^^^^^^^^^^^^^^^^^^^^^^^^^^^^^^^^^^^^^ ModuleNotFoundError: No module named 'src'
.
A: Please run export PYTHONPATH=$(pwd):$PYTHONPATH
when you start a new terminal.
Q: Error Files not found
A: Check your Manim installation.
Q: Error latex ...
A: Check your latex installation.
Q: The output is not showing response?
A: It could be API-related issues. Make sure your .env
file is properly configured (fill in your API keys), or you can enable litellm debug mode to figure out the issues.
Please kindly cite our paper if you use our code, data, models or results:
@misc{ku2025theoremexplainagentmultimodalexplanationsllm,
title={TheoremExplainAgent: Towards Multimodal Explanations for LLM Theorem Understanding},
author={Max Ku and Thomas Chong and Jonathan Leung and Krish Shah and Alvin Yu and Wenhu Chen},
year={2025},
eprint={2502.19400},
archivePrefix={arXiv},
primaryClass={cs.AI},
url={https://arxiv.org/abs/2502.19400},
}
This project is released under the the MIT License.
We want to thank Votee AI for sponsoring API keys to access the close-sourced models.
The code is built upon the below repositories, we thank all the contributors for open-sourcing.
- Manim Community
- kokoro-manim-voiceover
- manim-physics
- manim-Chemistry
- ManimML
- manim-dsa
- manim-circuit
This work is intended for research purposes only. The authors do not encourage or endorse the use of this codebase for commercial applications. The code is provided "as is" without any warranties, and users assume all responsibility for its use.
Tested Environment: MacOS, Linux
For Tasks:
Click tags to check more tools for each tasksFor Jobs:
Alternative AI tools for TheoremExplainAgent
Similar Open Source Tools
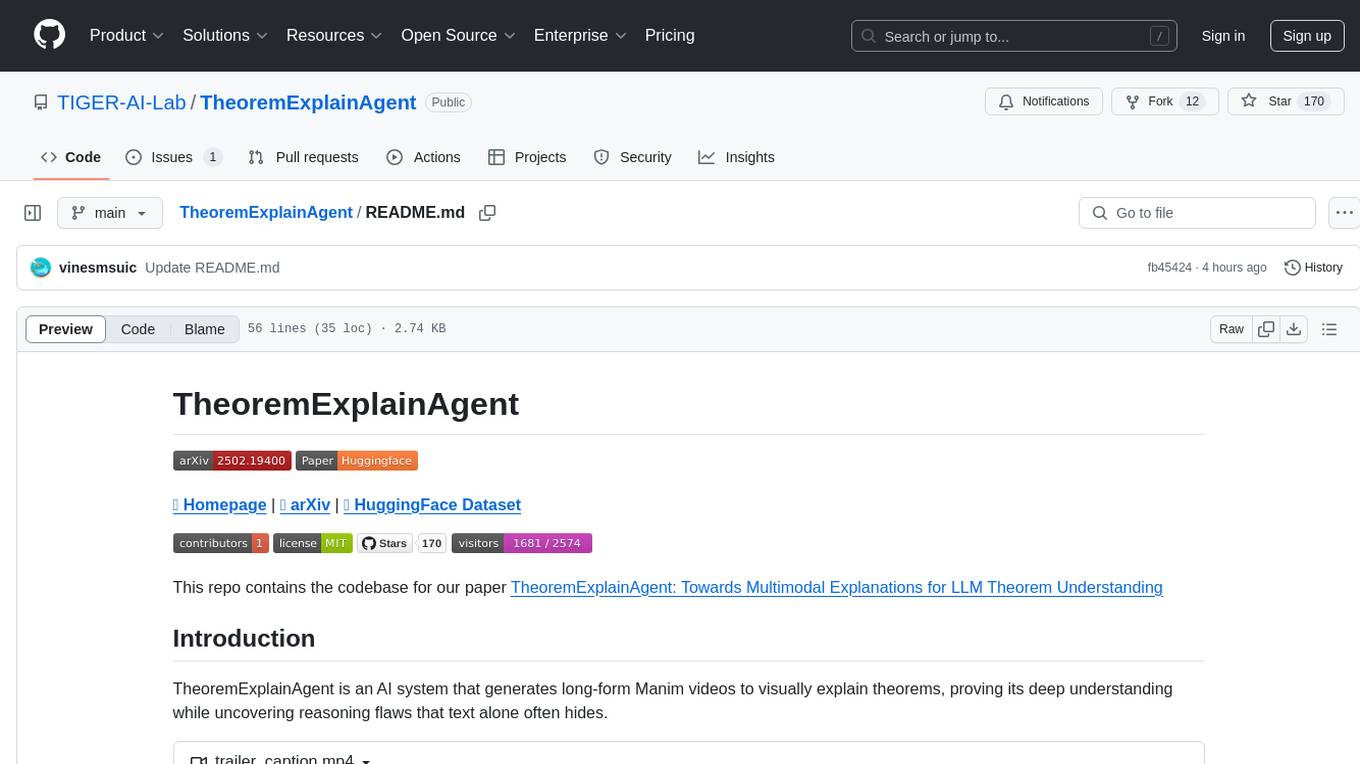
TheoremExplainAgent
TheoremExplainAgent is an AI system that generates long-form Manim videos to visually explain theorems, proving its deep understanding while uncovering reasoning flaws that text alone often hides. The codebase for the paper 'TheoremExplainAgent: Towards Multimodal Explanations for LLM Theorem Understanding' is available in this repository. It provides a tool for creating multimodal explanations for theorem understanding using AI technology.
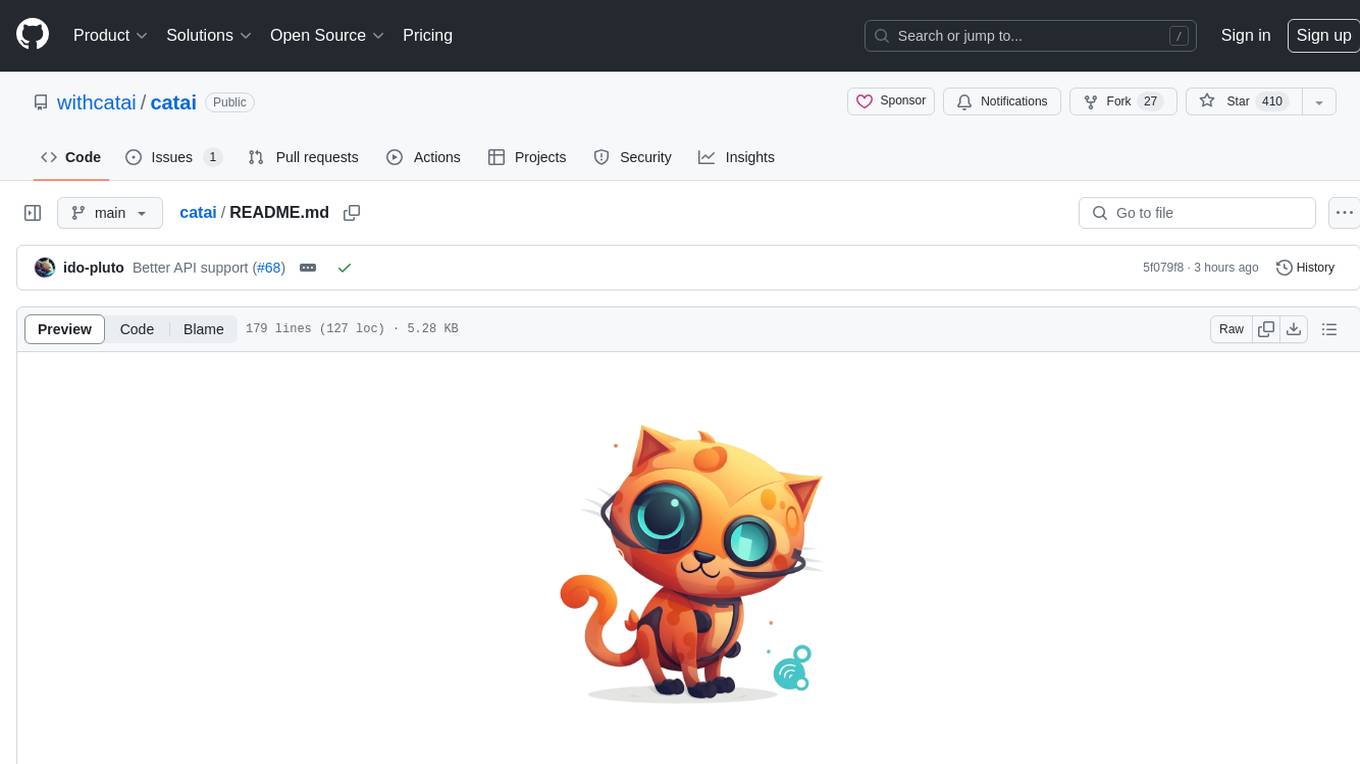
catai
CatAI is a tool that allows users to run GGUF models on their computer with a chat UI. It serves as a local AI assistant inspired by Node-Llama-Cpp and Llama.cpp. The tool provides features such as auto-detecting programming language, showing original messages by clicking on user icons, real-time text streaming, and fast model downloads. Users can interact with the tool through a CLI that supports commands for installing, listing, setting, serving, updating, and removing models. CatAI is cross-platform and supports Windows, Linux, and Mac. It utilizes node-llama-cpp and offers a simple API for asking model questions. Additionally, developers can integrate the tool with node-llama-cpp@beta for model management and chatting. The configuration can be edited via the web UI, and contributions to the project are welcome. The tool is licensed under Llama.cpp's license.
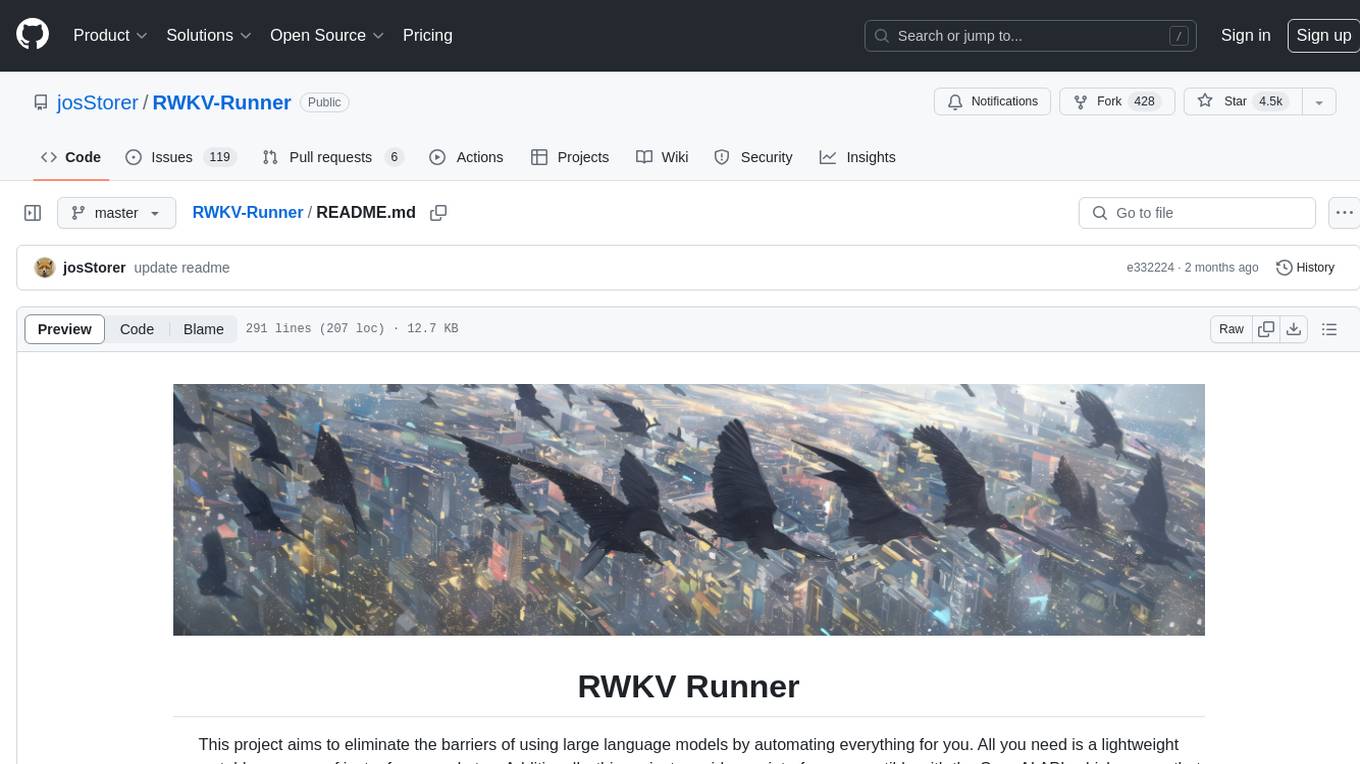
RWKV-Runner
RWKV Runner is a project designed to simplify the usage of large language models by automating various processes. It provides a lightweight executable program and is compatible with the OpenAI API. Users can deploy the backend on a server and use the program as a client. The project offers features like model management, VRAM configurations, user-friendly chat interface, WebUI option, parameter configuration, model conversion tool, download management, LoRA Finetune, and multilingual localization. It can be used for various tasks such as chat, completion, composition, and model inspection.
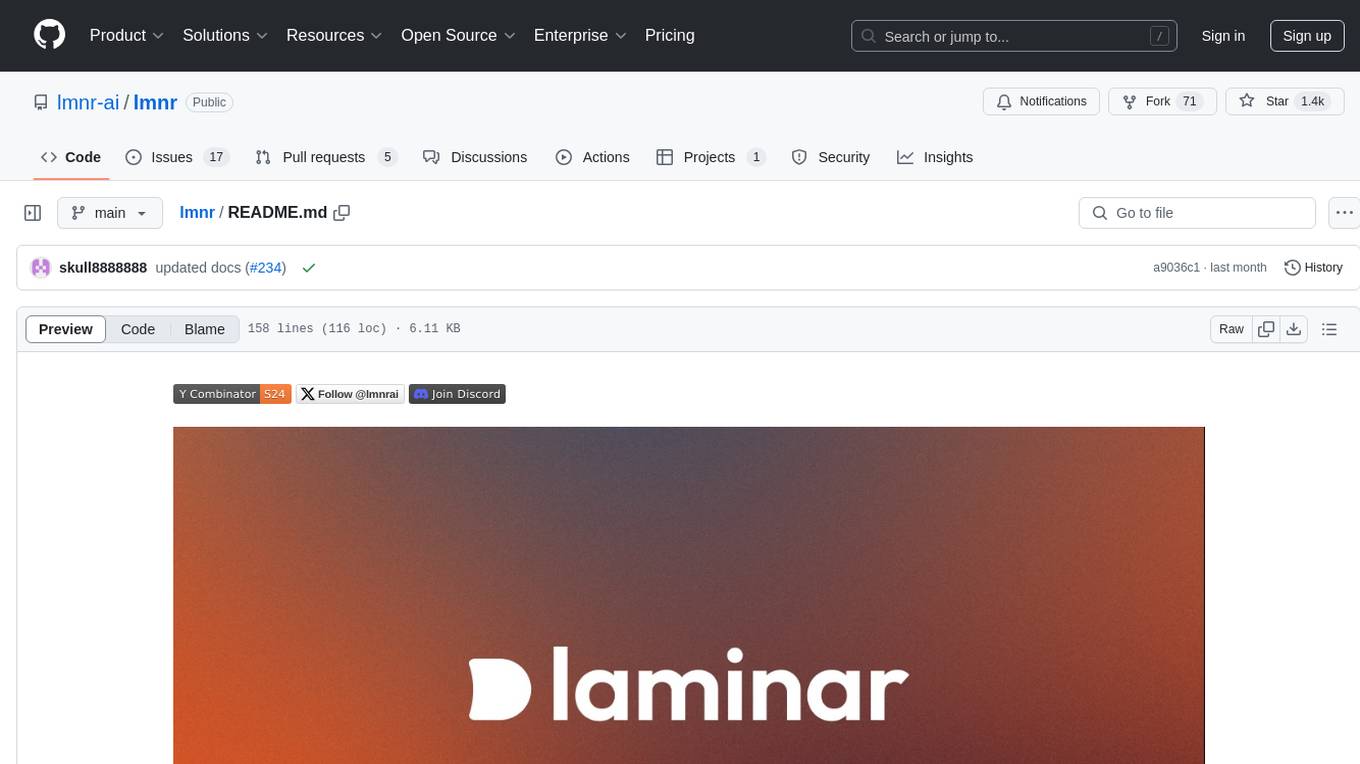
lmnr
Laminar is an all-in-one open-source platform designed for engineering AI products. It allows users to trace, evaluate, label, and analyze LLM data efficiently. The platform offers features such as automatic tracing of common AI frameworks and SDKs, local and online evaluations, simple UI for data labeling, dataset management, and scalability with gRPC communication. Laminar is built with a modern open-source stack including RabbitMQ, Postgres, Clickhouse, and Qdrant for semantic similarity search. It provides fast and beautiful dashboards for traces, evaluations, and labels, making it a comprehensive tool for AI product development.
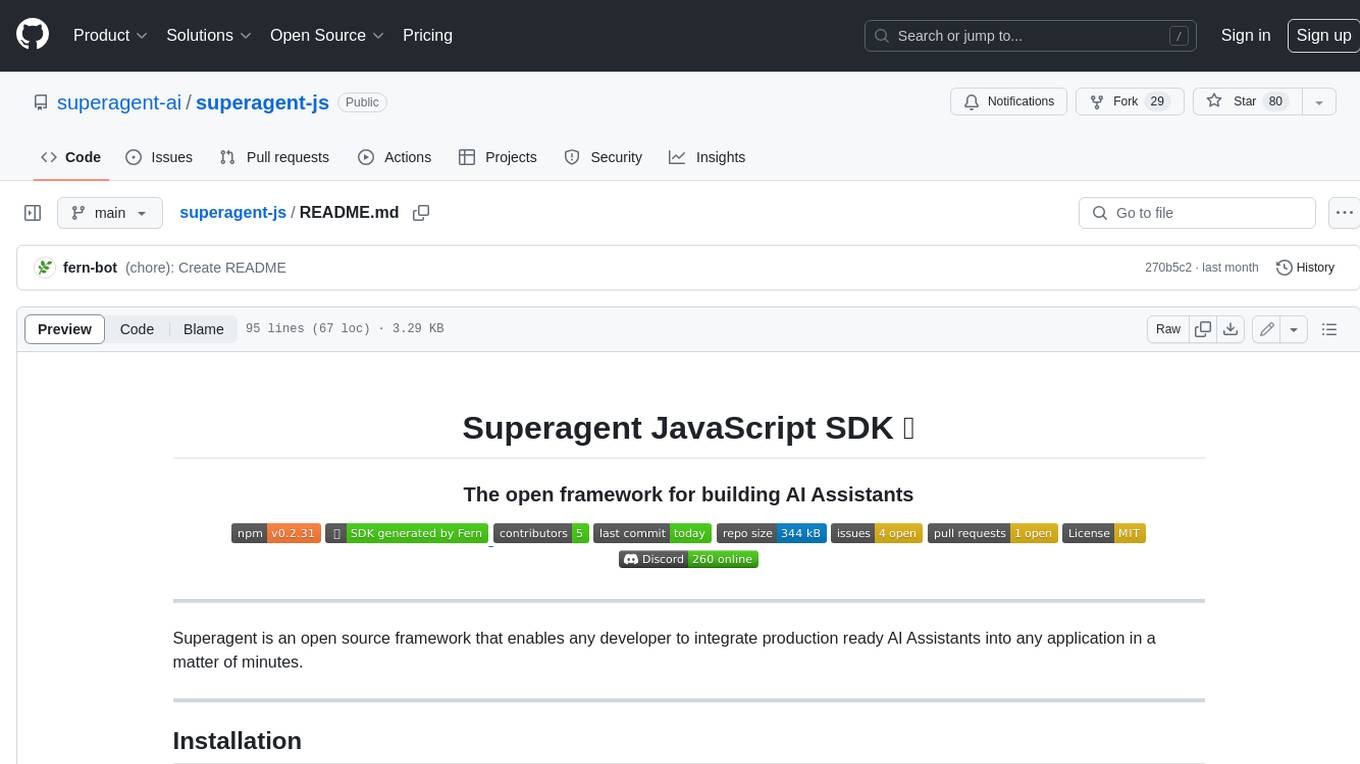
superagent-js
Superagent is an open source framework that enables any developer to integrate production ready AI Assistants into any application in a matter of minutes.
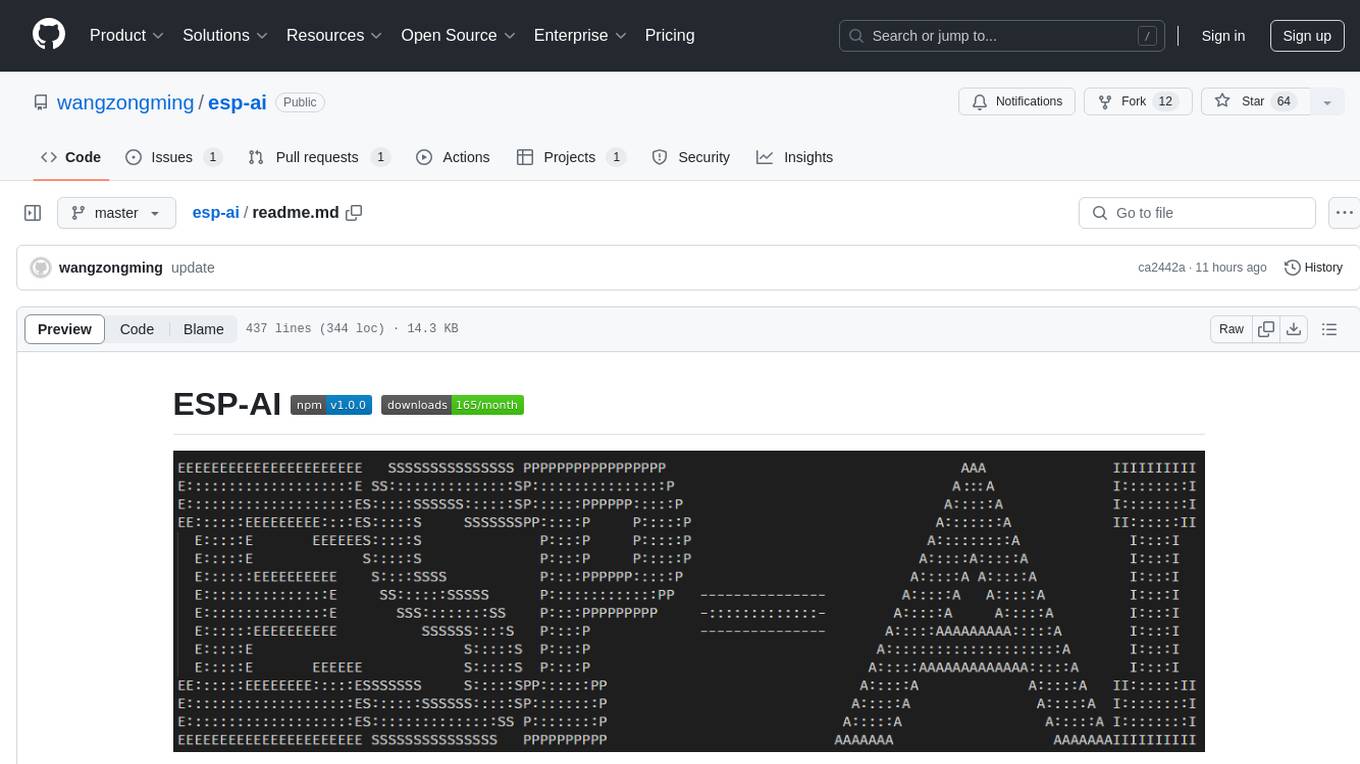
esp-ai
ESP-AI provides a complete AI conversation solution for your development board, including IAT+LLM+TTS integration solutions for ESP32 series development boards. It can be injected into projects without affecting existing ones. By providing keys from platforms like iFlytek, Jiling, and local services, you can run the services without worrying about interactions between services or between development boards and services. The project's server-side code is based on Node.js, and the hardware code is based on Arduino IDE.
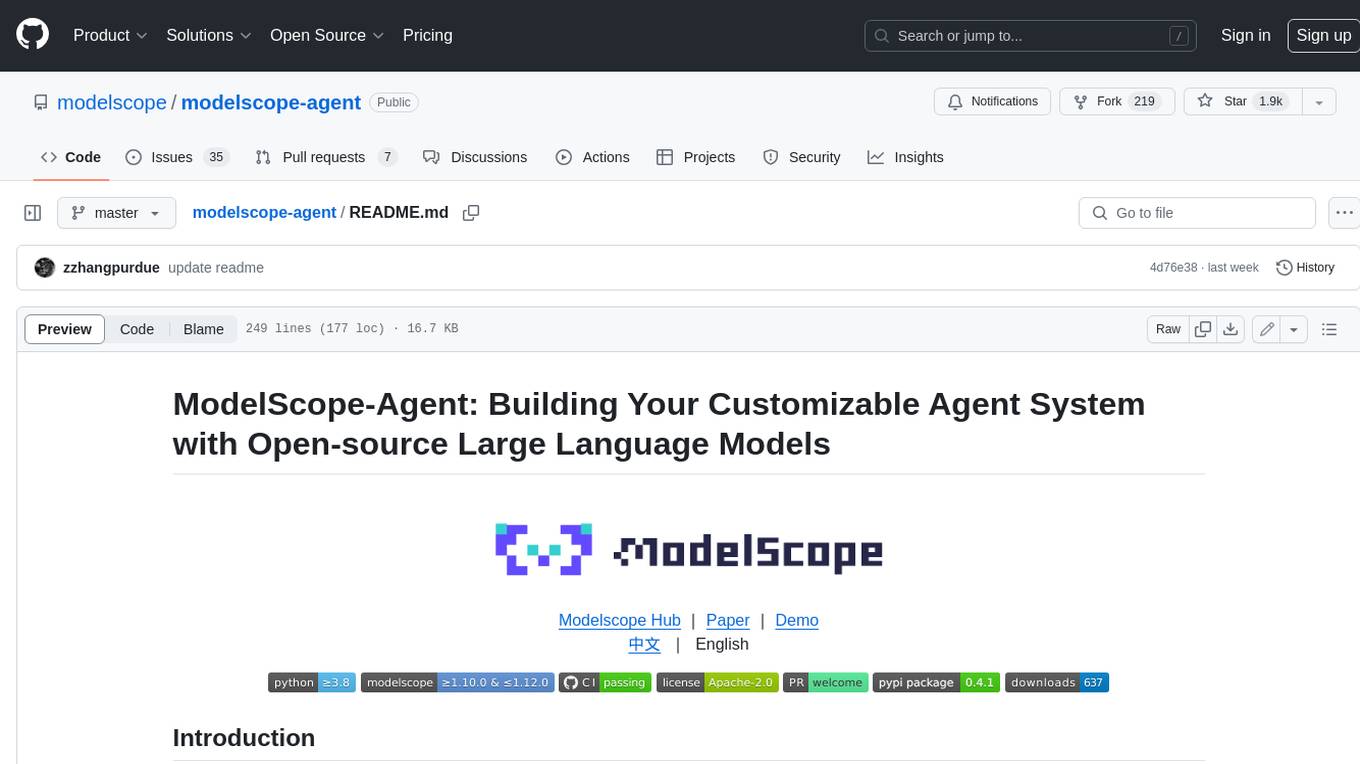
modelscope-agent
ModelScope-Agent is a customizable and scalable Agent framework. A single agent has abilities such as role-playing, LLM calling, tool usage, planning, and memory. It mainly has the following characteristics: - **Simple Agent Implementation Process**: Simply specify the role instruction, LLM name, and tool name list to implement an Agent application. The framework automatically arranges workflows for tool usage, planning, and memory. - **Rich models and tools**: The framework is equipped with rich LLM interfaces, such as Dashscope and Modelscope model interfaces, OpenAI model interfaces, etc. Built in rich tools, such as **code interpreter**, **weather query**, **text to image**, **web browsing**, etc., make it easy to customize exclusive agents. - **Unified interface and high scalability**: The framework has clear tools and LLM registration mechanism, making it convenient for users to expand more diverse Agent applications. - **Low coupling**: Developers can easily use built-in tools, LLM, memory, and other components without the need to bind higher-level agents.
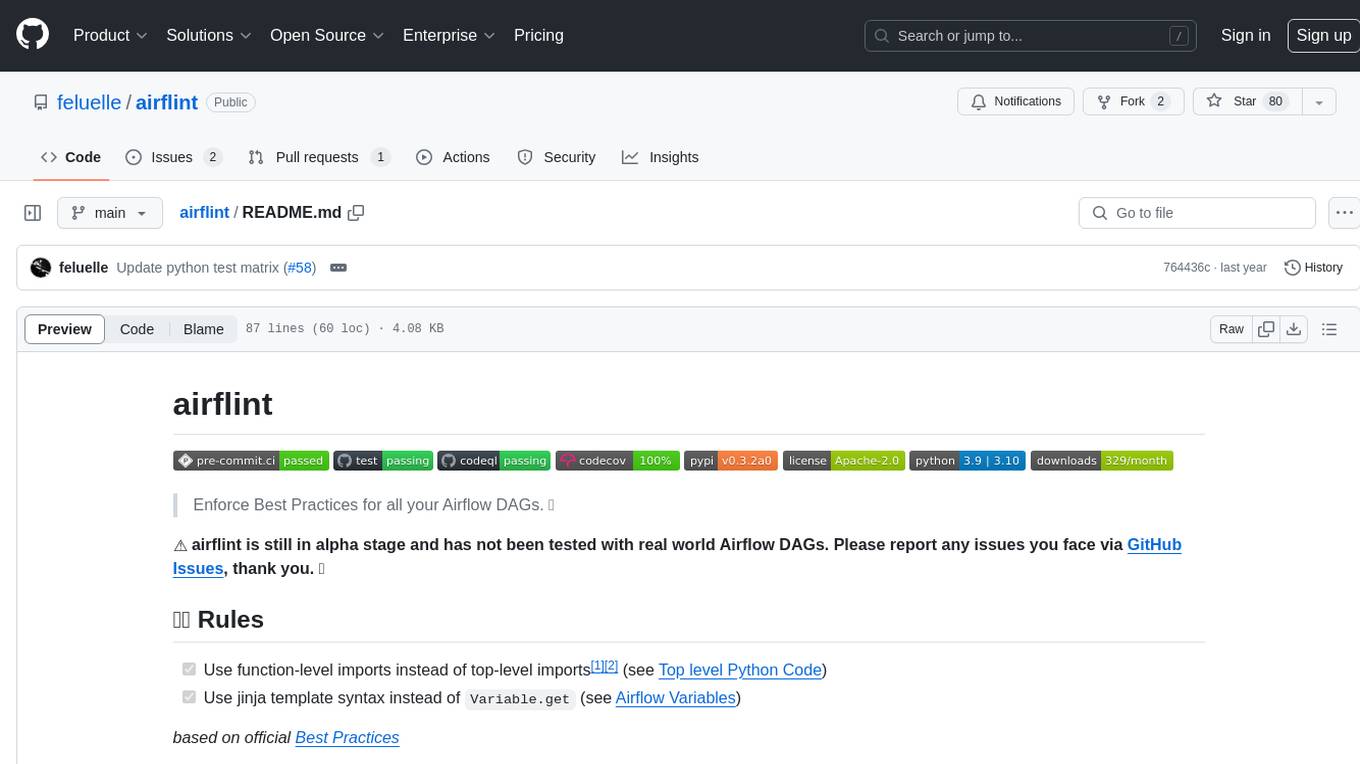
airflint
Airflint is a tool designed to enforce best practices for all your Airflow Directed Acyclic Graphs (DAGs). It is currently in the alpha stage and aims to help users adhere to recommended practices when working with Airflow. Users can install Airflint from PyPI and integrate it into their existing Airflow environment to improve DAG quality. The tool provides rules for function-level imports and jinja template syntax usage, among others, to enhance the development process of Airflow DAGs.
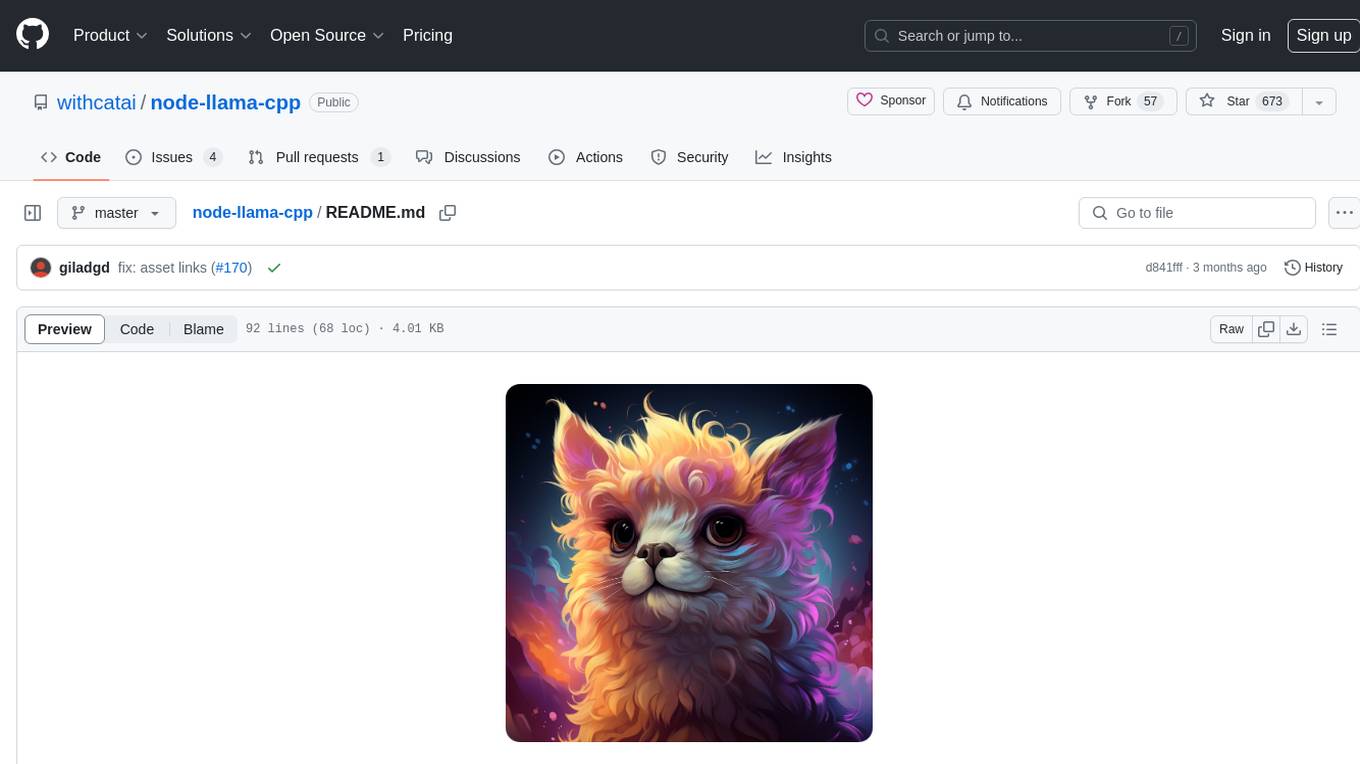
node-llama-cpp
node-llama-cpp is a tool that allows users to run AI models locally on their machines. It provides pre-built bindings with the option to build from source using cmake. Users can interact with text generation models, chat with models using a chat wrapper, and force models to generate output in a parseable format like JSON. The tool supports Metal and CUDA, offers CLI functionality for chatting with models without coding, and ensures up-to-date compatibility with the latest version of llama.cpp. Installation includes pre-built binaries for macOS, Linux, and Windows, with the option to build from source if binaries are not available for the platform.
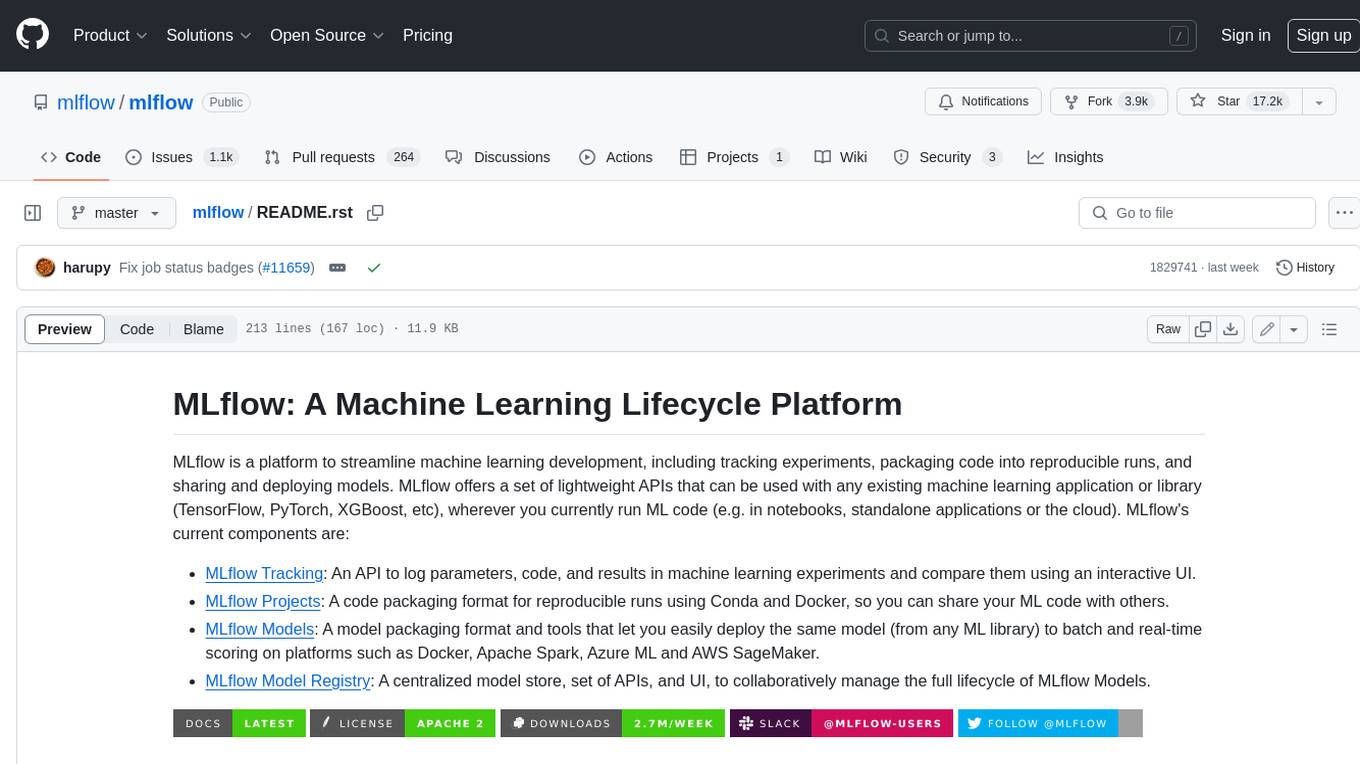
mlflow
MLflow is a platform to streamline machine learning development, including tracking experiments, packaging code into reproducible runs, and sharing and deploying models. MLflow offers a set of lightweight APIs that can be used with any existing machine learning application or library (TensorFlow, PyTorch, XGBoost, etc), wherever you currently run ML code (e.g. in notebooks, standalone applications or the cloud). MLflow's current components are:
* `MLflow Tracking
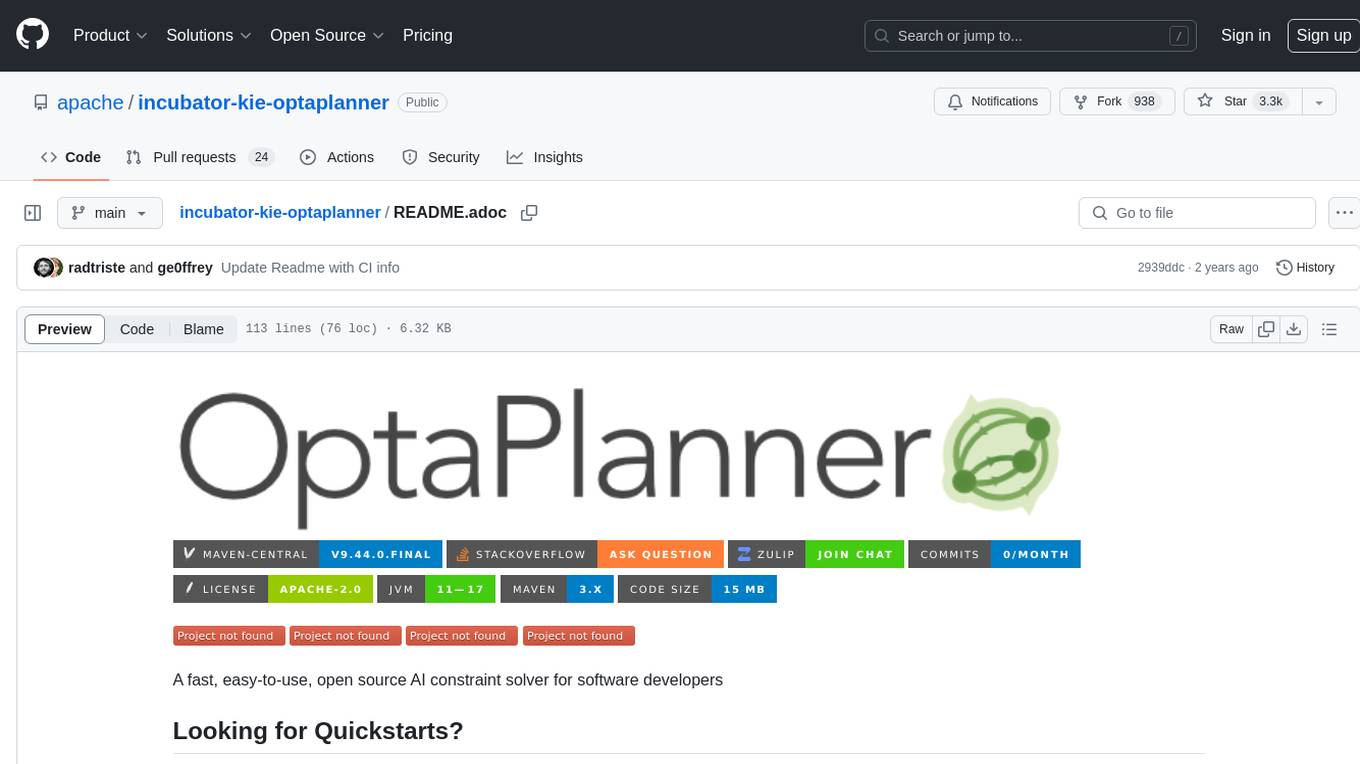
incubator-kie-optaplanner
A fast, easy-to-use, open source AI constraint solver for software developers. OptaPlanner is a powerful tool that helps developers solve complex optimization problems by providing a constraint satisfaction solver. It allows users to model and solve planning and scheduling problems efficiently, improving decision-making processes and resource allocation. With OptaPlanner, developers can easily integrate optimization capabilities into their applications, leading to better performance and cost-effectiveness.
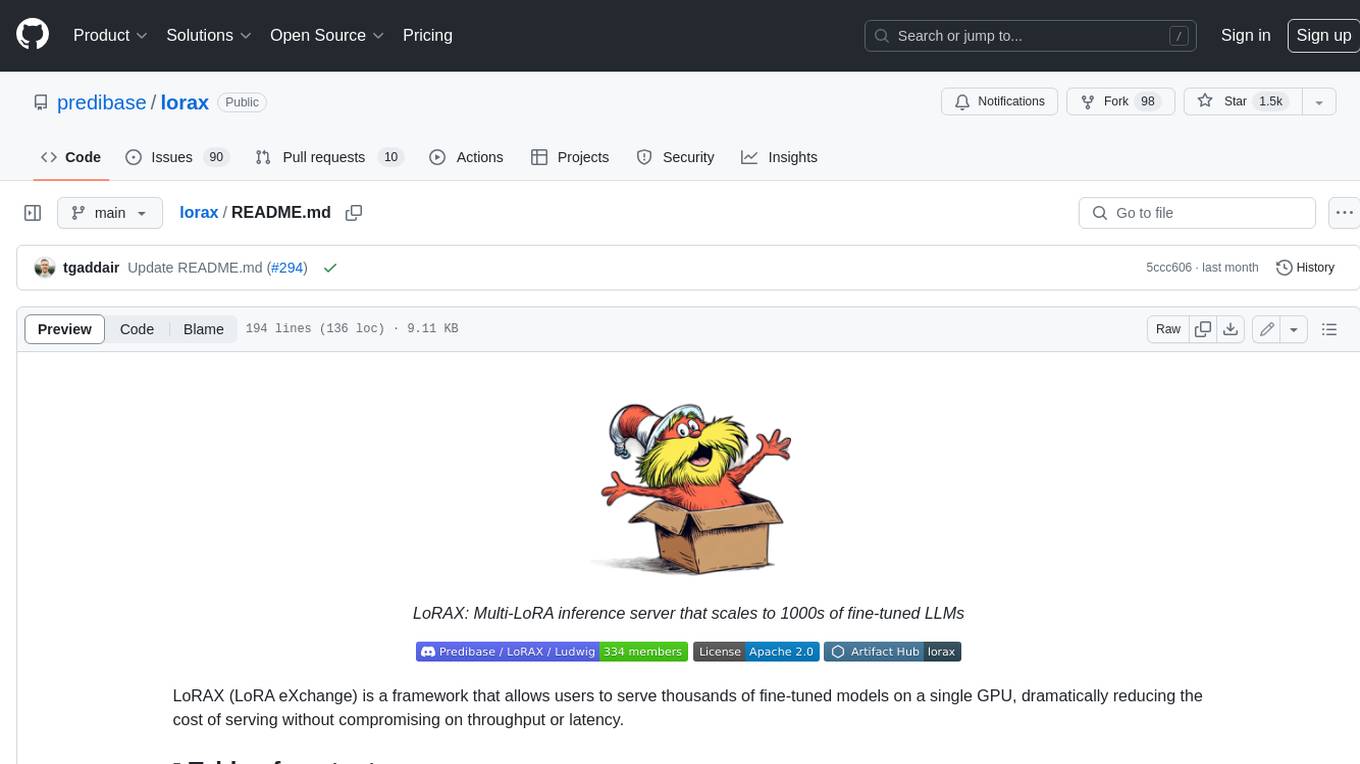
lorax
LoRAX is a framework that allows users to serve thousands of fine-tuned models on a single GPU, dramatically reducing the cost of serving without compromising on throughput or latency. It features dynamic adapter loading, heterogeneous continuous batching, adapter exchange scheduling, optimized inference, and is ready for production with prebuilt Docker images, Helm charts for Kubernetes, Prometheus metrics, and distributed tracing with Open Telemetry. LoRAX supports a number of Large Language Models as the base model including Llama, Mistral, and Qwen, and any of the linear layers in the model can be adapted via LoRA and loaded in LoRAX.
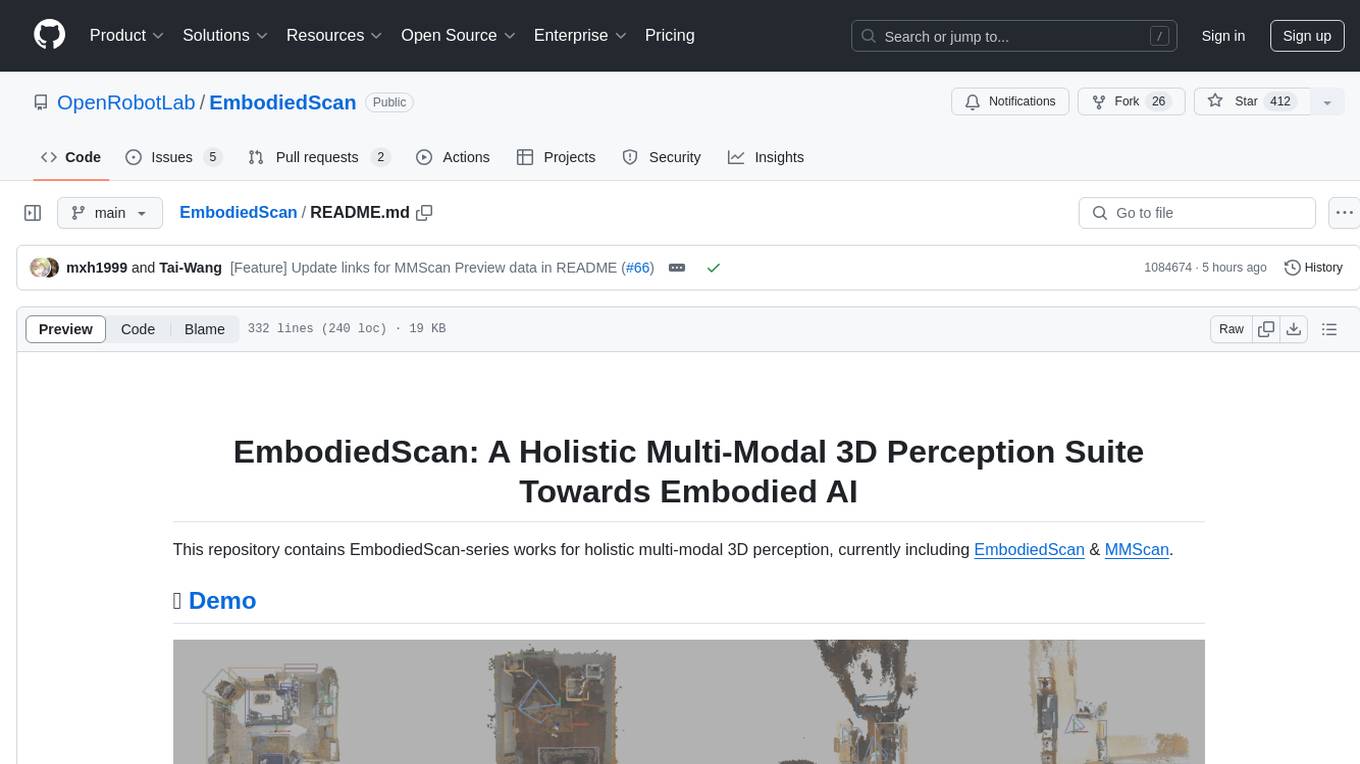
EmbodiedScan
EmbodiedScan is a holistic multi-modal 3D perception suite designed for embodied AI. It introduces a multi-modal, ego-centric 3D perception dataset and benchmark for holistic 3D scene understanding. The dataset includes over 5k scans with 1M ego-centric RGB-D views, 1M language prompts, 160k 3D-oriented boxes spanning 760 categories, and dense semantic occupancy with 80 common categories. The suite includes a baseline framework named Embodied Perceptron, capable of processing multi-modal inputs for 3D perception tasks and language-grounded tasks.
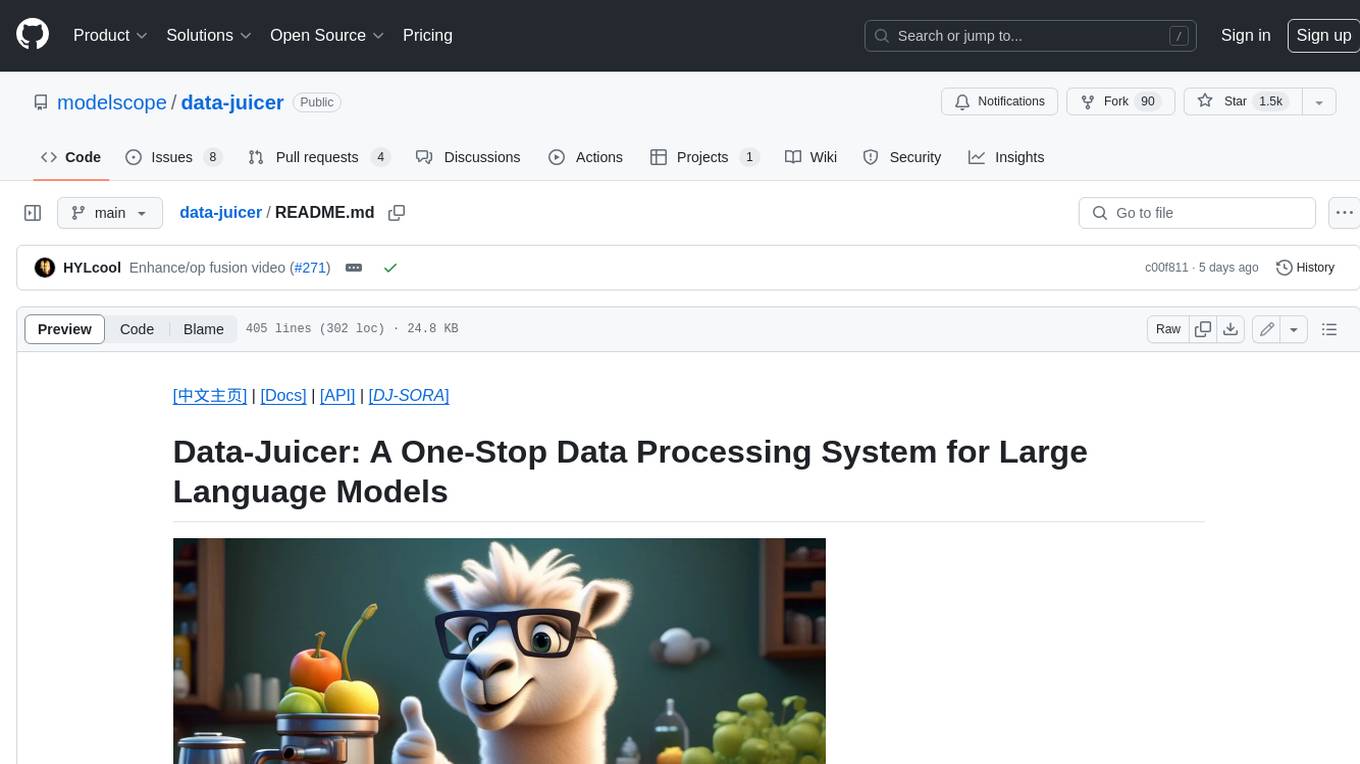
data-juicer
Data-Juicer is a one-stop data processing system to make data higher-quality, juicier, and more digestible for LLMs. It is a systematic & reusable library of 80+ core OPs, 20+ reusable config recipes, and 20+ feature-rich dedicated toolkits, designed to function independently of specific LLM datasets and processing pipelines. Data-Juicer allows detailed data analyses with an automated report generation feature for a deeper understanding of your dataset. Coupled with multi-dimension automatic evaluation capabilities, it supports a timely feedback loop at multiple stages in the LLM development process. Data-Juicer offers tens of pre-built data processing recipes for pre-training, fine-tuning, en, zh, and more scenarios. It provides a speedy data processing pipeline requiring less memory and CPU usage, optimized for maximum productivity. Data-Juicer is flexible & extensible, accommodating most types of data formats and allowing flexible combinations of OPs. It is designed for simplicity, with comprehensive documentation, easy start guides and demo configs, and intuitive configuration with simple adding/removing OPs from existing configs.
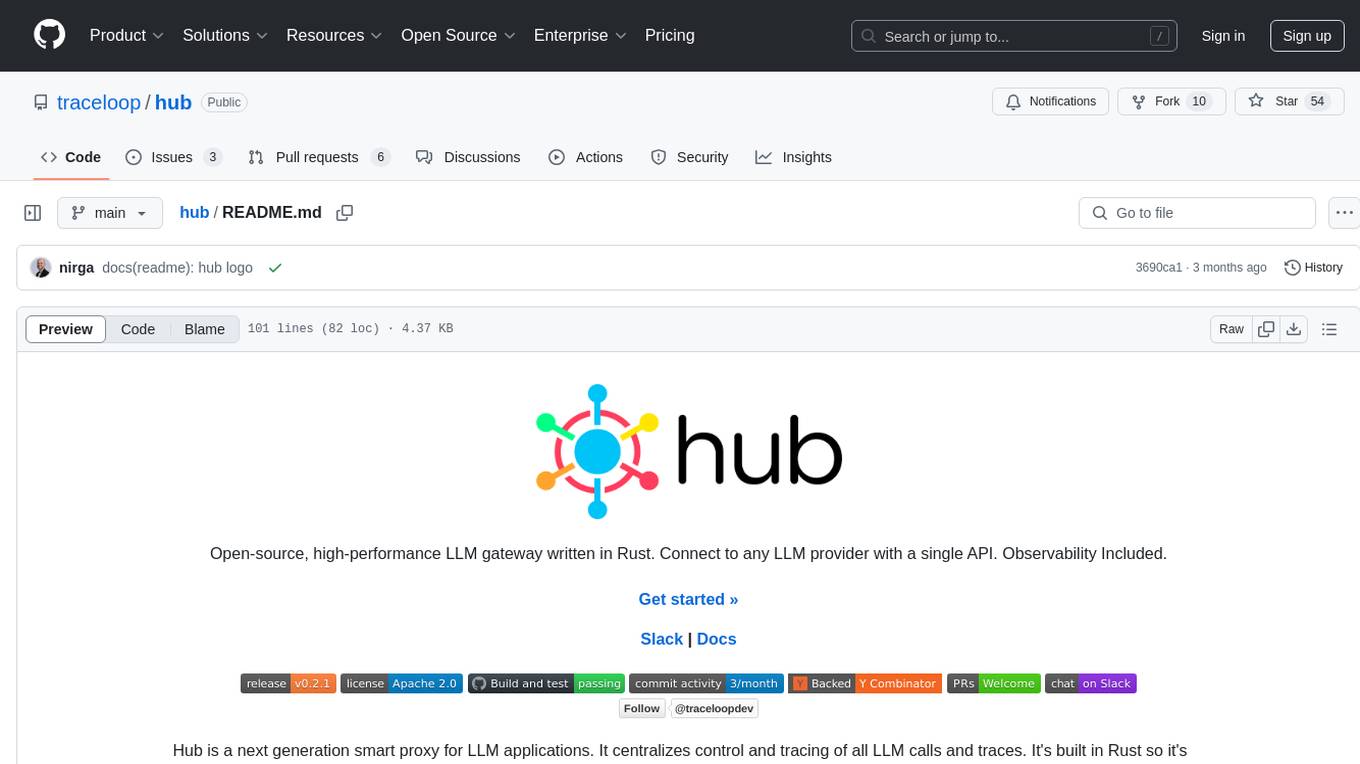
hub
Hub is an open-source, high-performance LLM gateway written in Rust. It serves as a smart proxy for LLM applications, centralizing control and tracing of all LLM calls and traces. Built for efficiency, it provides a single API to connect to any LLM provider. The tool is designed to be fast, efficient, and completely open-source under the Apache 2.0 license.
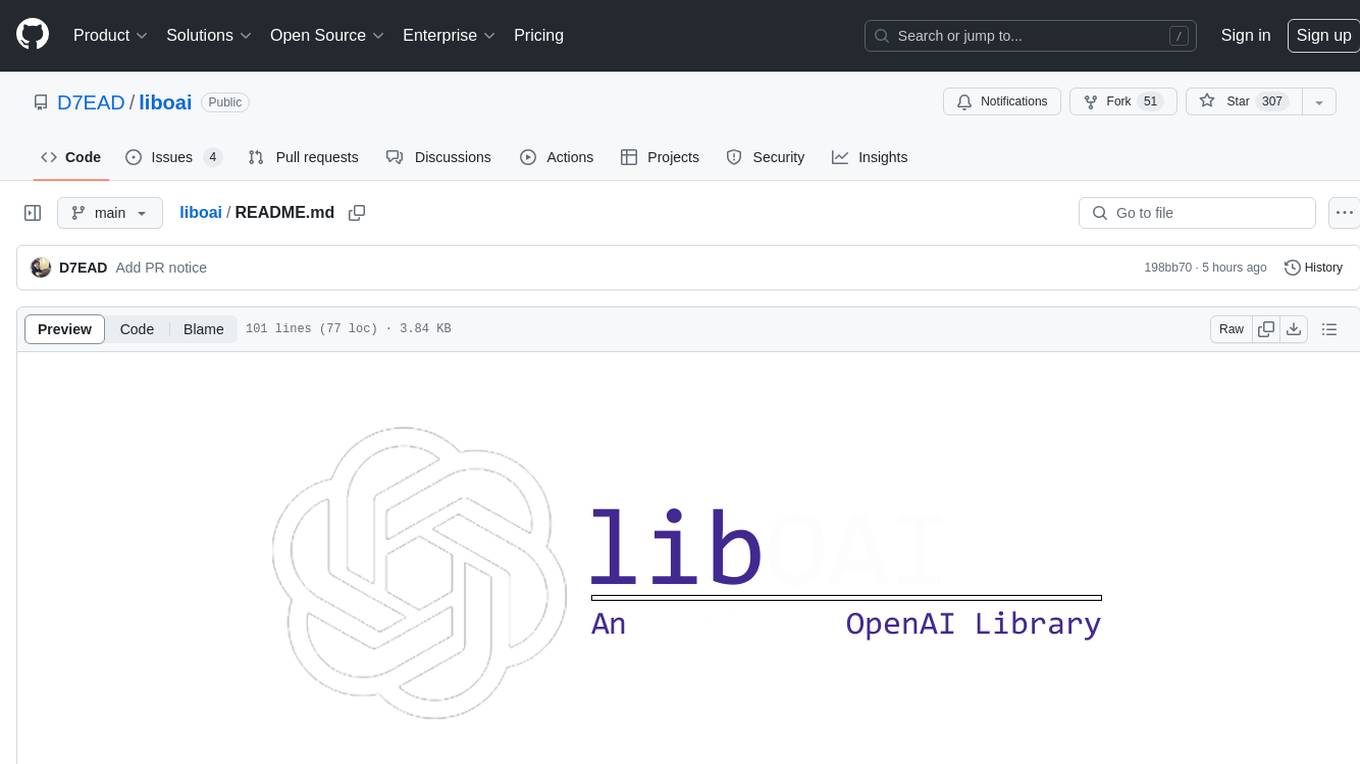
liboai
liboai is a simple C++17 library for the OpenAI API, providing developers with access to OpenAI endpoints through a collection of methods and classes. It serves as a spiritual port of OpenAI's Python library, 'openai', with similar structure and features. The library supports various functionalities such as ChatGPT, Audio, Azure, Functions, Image DALLΒ·E, Models, Completions, Edit, Embeddings, Files, Fine-tunes, Moderation, and Asynchronous Support. Users can easily integrate the library into their C++ projects to interact with OpenAI services.
For similar tasks
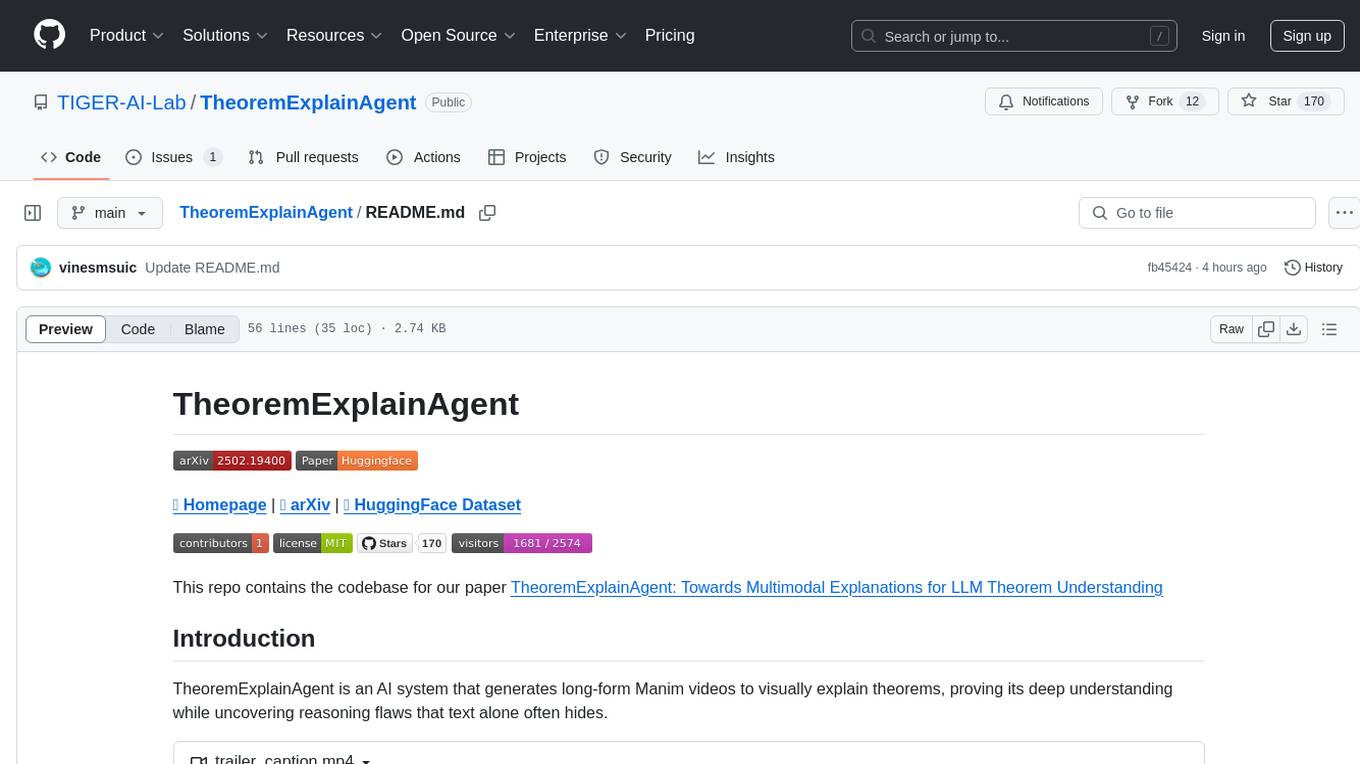
TheoremExplainAgent
TheoremExplainAgent is an AI system that generates long-form Manim videos to visually explain theorems, proving its deep understanding while uncovering reasoning flaws that text alone often hides. The codebase for the paper 'TheoremExplainAgent: Towards Multimodal Explanations for LLM Theorem Understanding' is available in this repository. It provides a tool for creating multimodal explanations for theorem understanding using AI technology.
For similar jobs
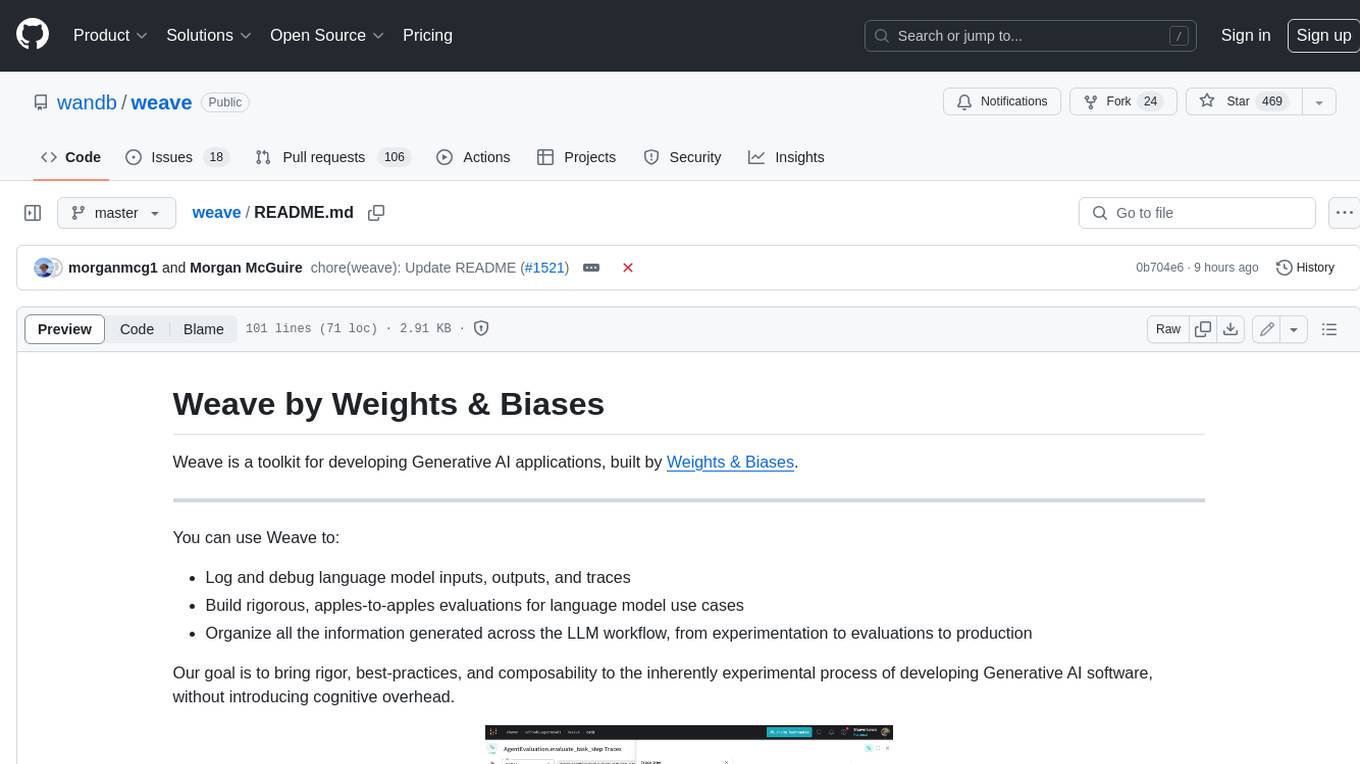
weave
Weave is a toolkit for developing Generative AI applications, built by Weights & Biases. With Weave, you can log and debug language model inputs, outputs, and traces; build rigorous, apples-to-apples evaluations for language model use cases; and organize all the information generated across the LLM workflow, from experimentation to evaluations to production. Weave aims to bring rigor, best-practices, and composability to the inherently experimental process of developing Generative AI software, without introducing cognitive overhead.
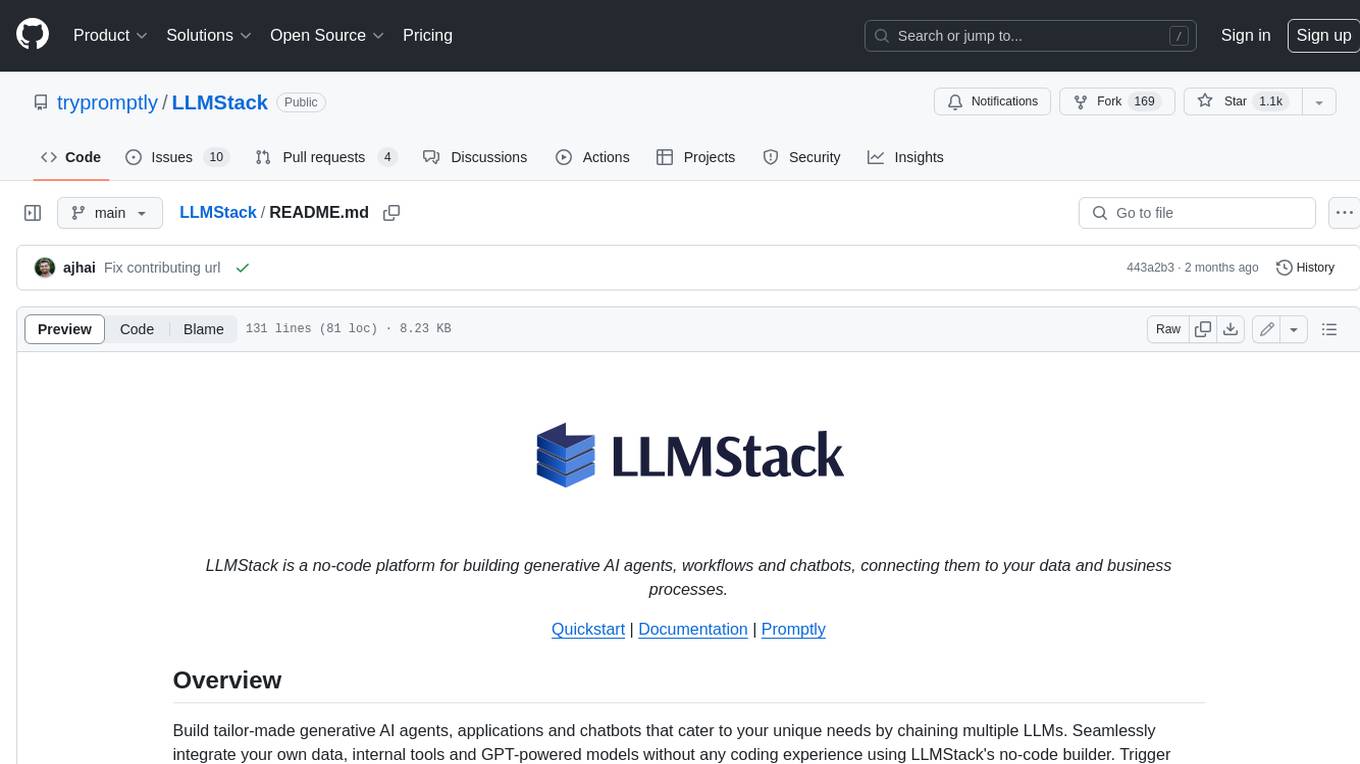
LLMStack
LLMStack is a no-code platform for building generative AI agents, workflows, and chatbots. It allows users to connect their own data, internal tools, and GPT-powered models without any coding experience. LLMStack can be deployed to the cloud or on-premise and can be accessed via HTTP API or triggered from Slack or Discord.
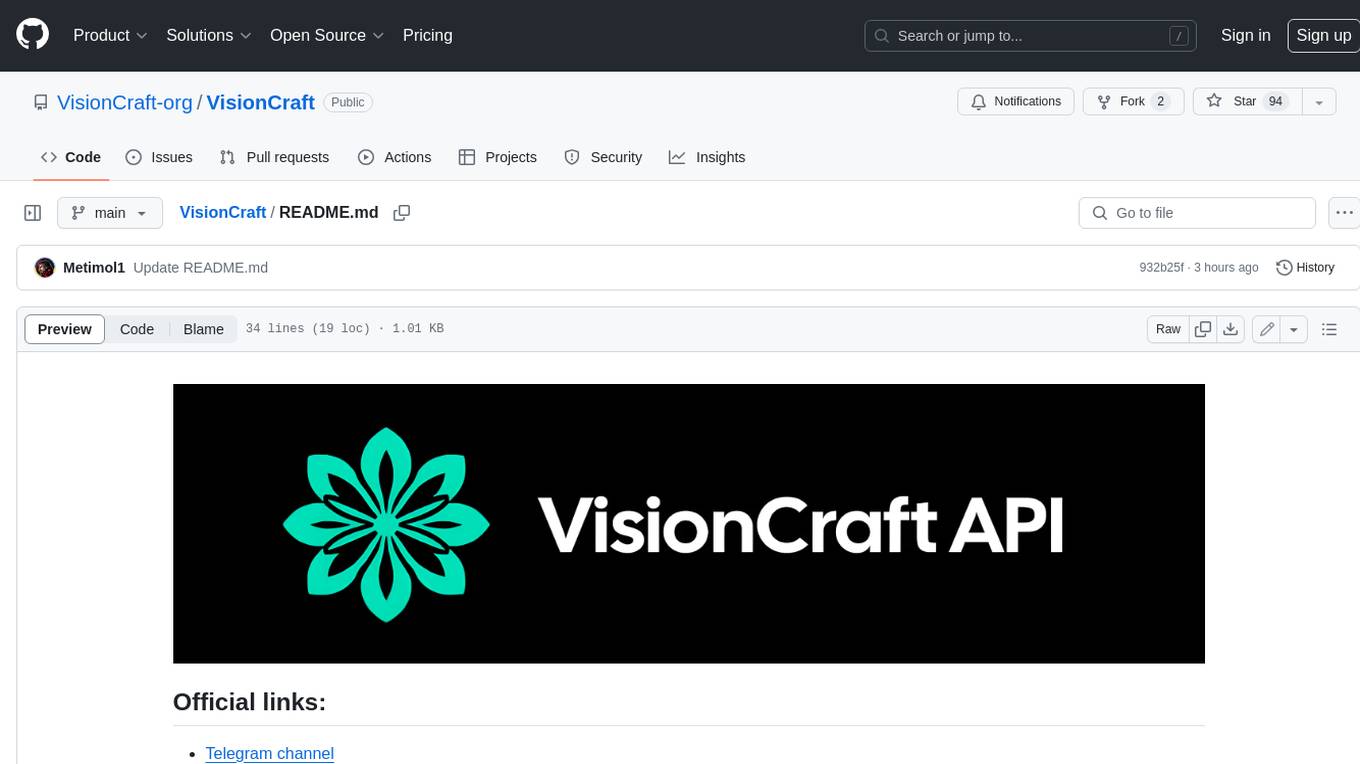
VisionCraft
The VisionCraft API is a free API for using over 100 different AI models. From images to sound.
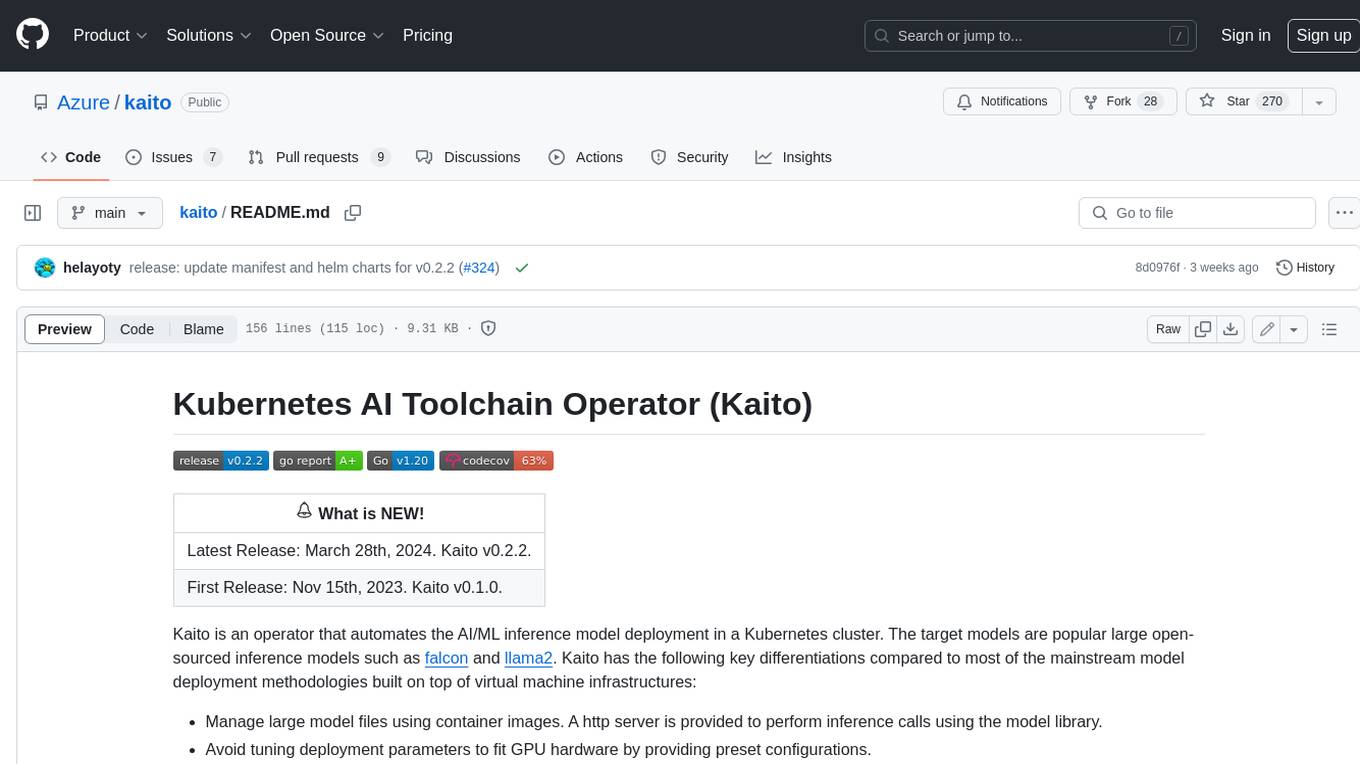
kaito
Kaito is an operator that automates the AI/ML inference model deployment in a Kubernetes cluster. It manages large model files using container images, avoids tuning deployment parameters to fit GPU hardware by providing preset configurations, auto-provisions GPU nodes based on model requirements, and hosts large model images in the public Microsoft Container Registry (MCR) if the license allows. Using Kaito, the workflow of onboarding large AI inference models in Kubernetes is largely simplified.
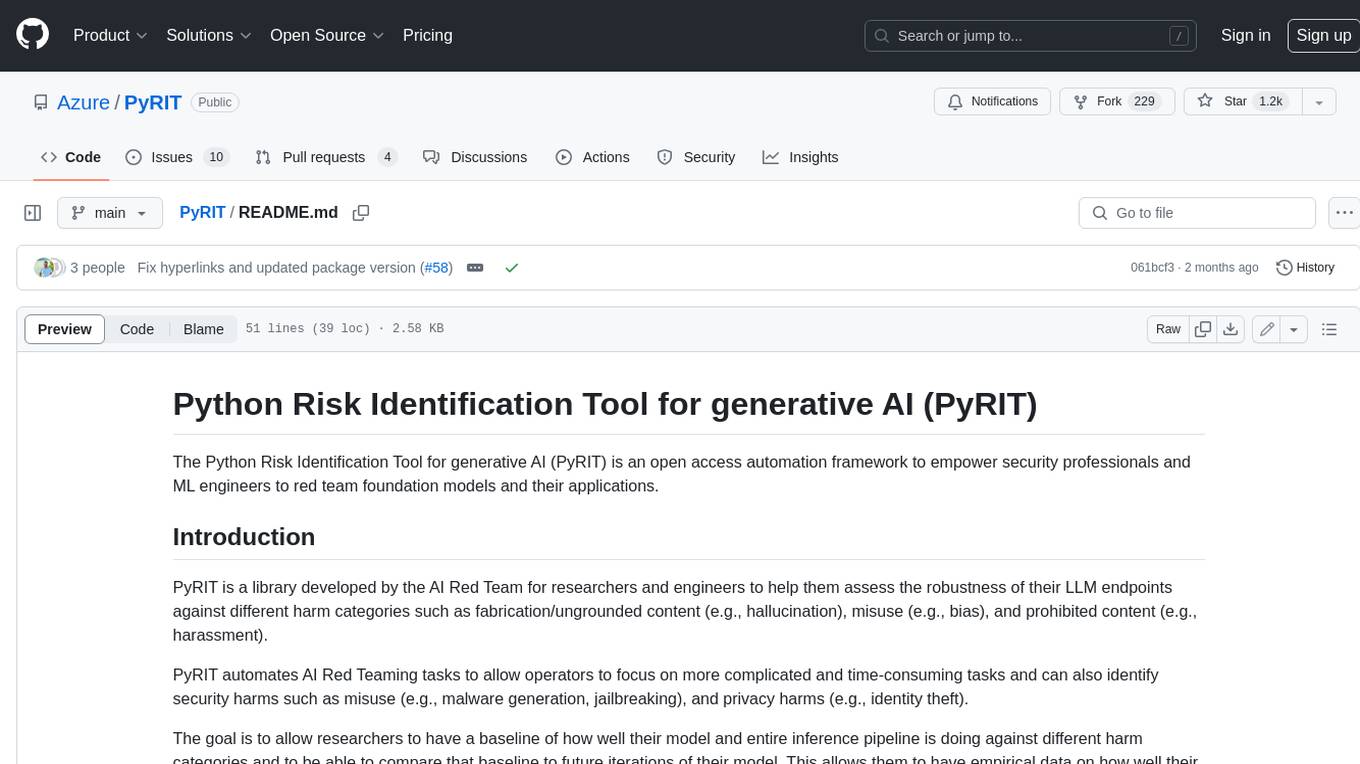
PyRIT
PyRIT is an open access automation framework designed to empower security professionals and ML engineers to red team foundation models and their applications. It automates AI Red Teaming tasks to allow operators to focus on more complicated and time-consuming tasks and can also identify security harms such as misuse (e.g., malware generation, jailbreaking), and privacy harms (e.g., identity theft). The goal is to allow researchers to have a baseline of how well their model and entire inference pipeline is doing against different harm categories and to be able to compare that baseline to future iterations of their model. This allows them to have empirical data on how well their model is doing today, and detect any degradation of performance based on future improvements.
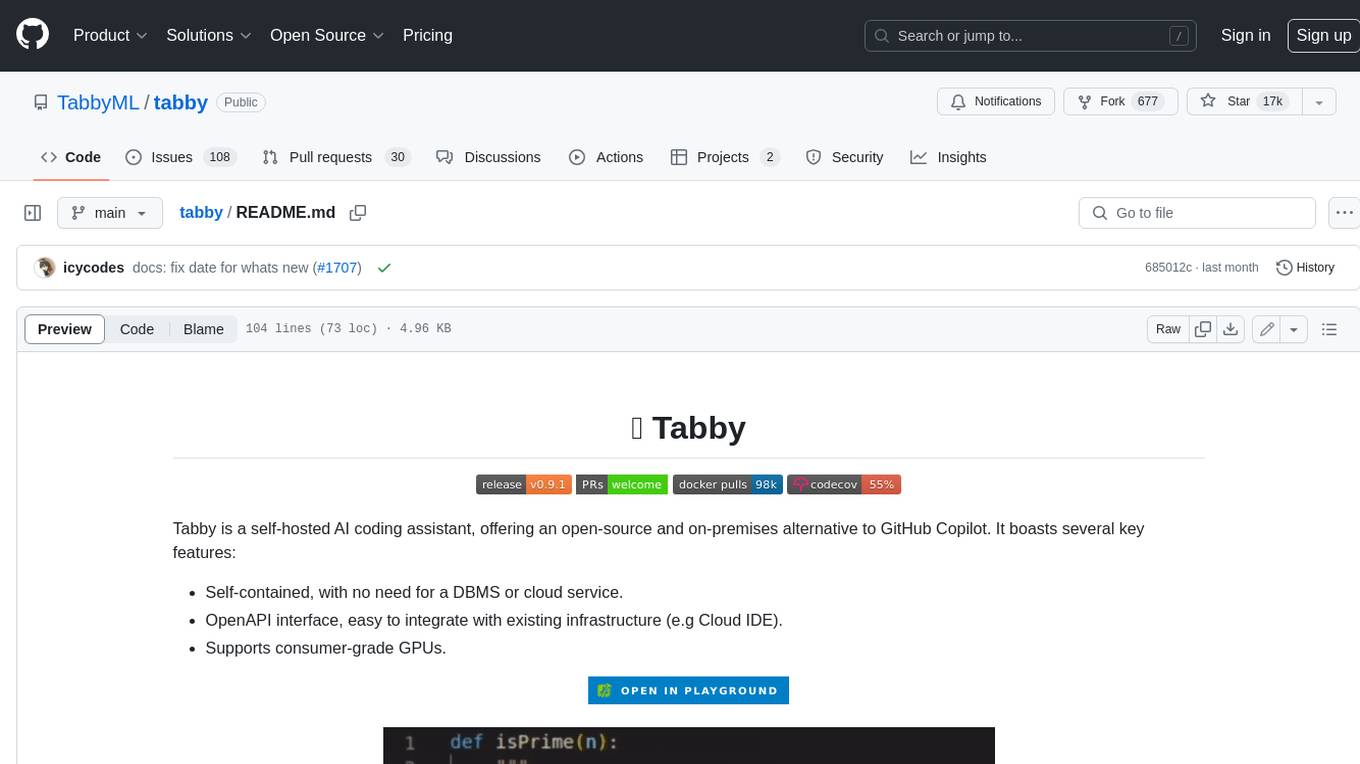
tabby
Tabby is a self-hosted AI coding assistant, offering an open-source and on-premises alternative to GitHub Copilot. It boasts several key features: * Self-contained, with no need for a DBMS or cloud service. * OpenAPI interface, easy to integrate with existing infrastructure (e.g Cloud IDE). * Supports consumer-grade GPUs.
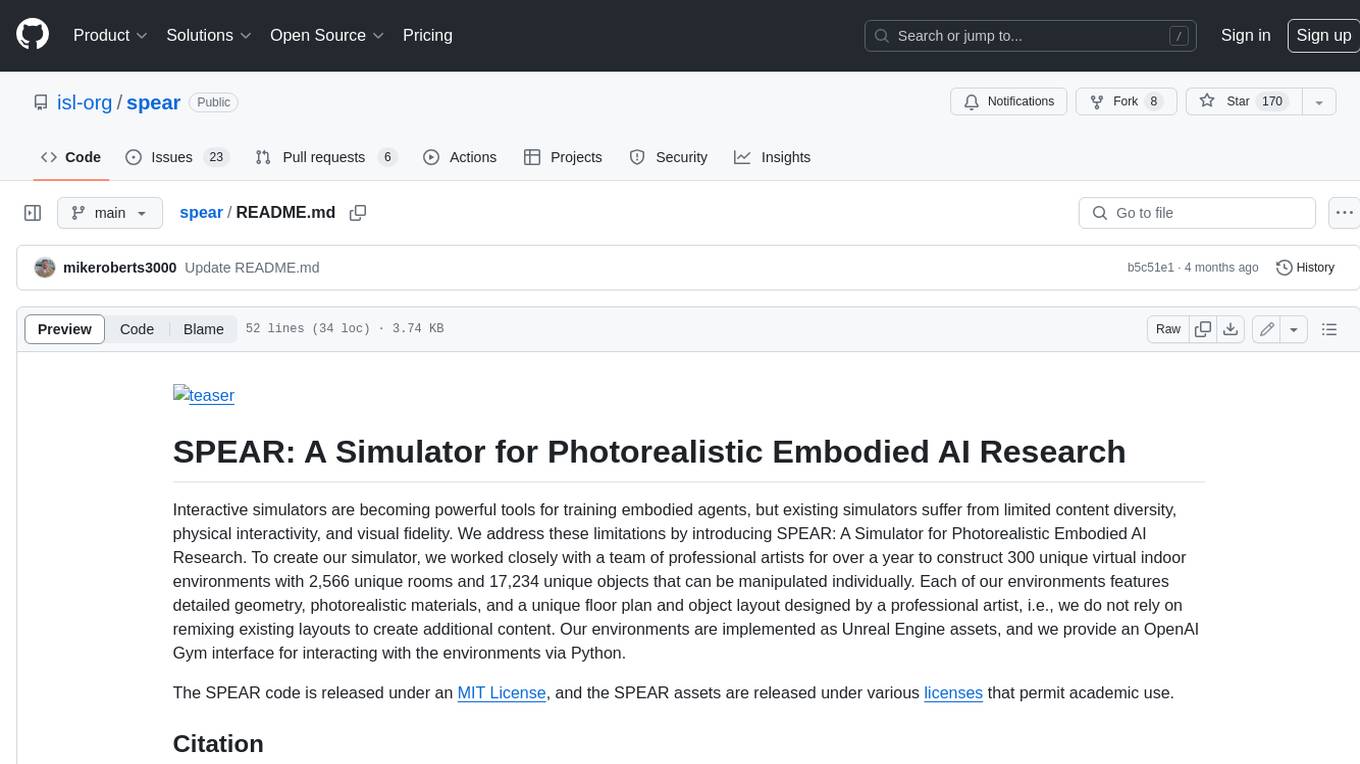
spear
SPEAR (Simulator for Photorealistic Embodied AI Research) is a powerful tool for training embodied agents. It features 300 unique virtual indoor environments with 2,566 unique rooms and 17,234 unique objects that can be manipulated individually. Each environment is designed by a professional artist and features detailed geometry, photorealistic materials, and a unique floor plan and object layout. SPEAR is implemented as Unreal Engine assets and provides an OpenAI Gym interface for interacting with the environments via Python.
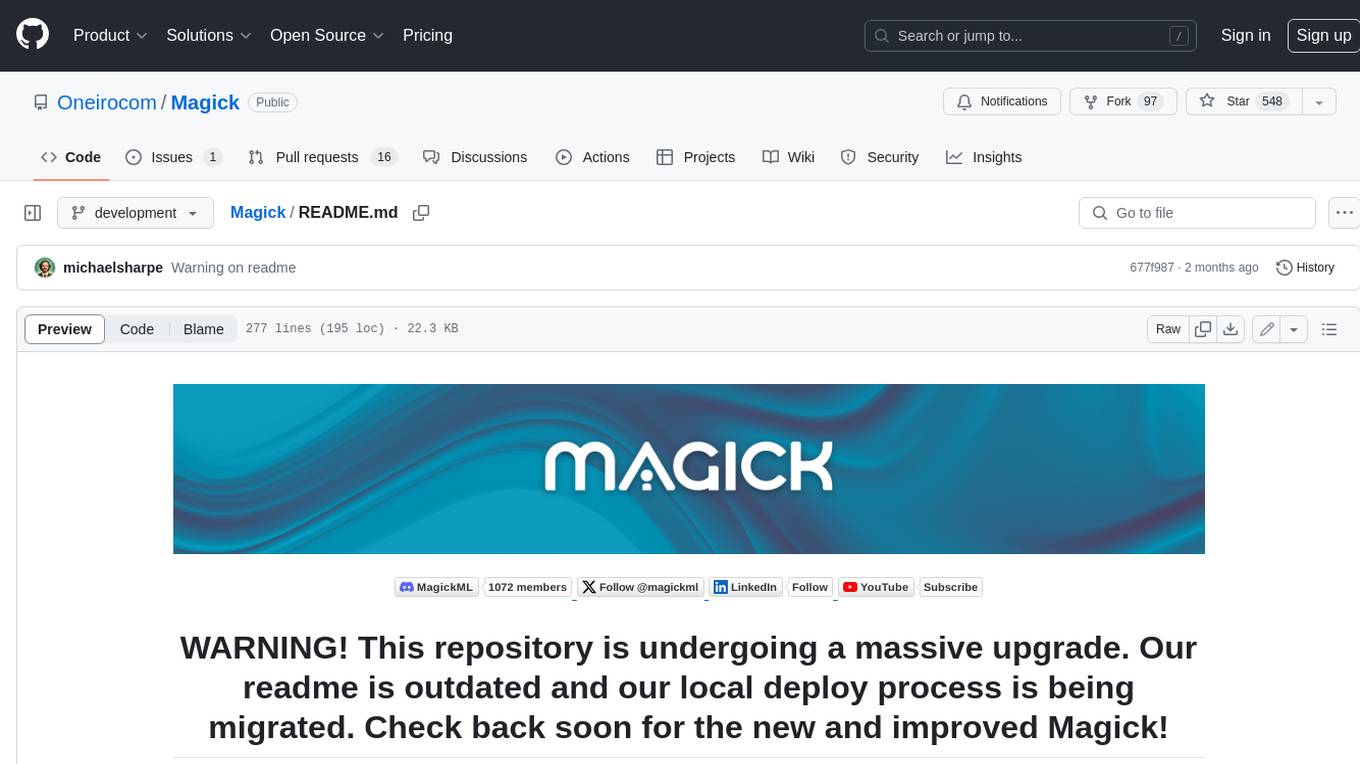
Magick
Magick is a groundbreaking visual AIDE (Artificial Intelligence Development Environment) for no-code data pipelines and multimodal agents. Magick can connect to other services and comes with nodes and templates well-suited for intelligent agents, chatbots, complex reasoning systems and realistic characters.