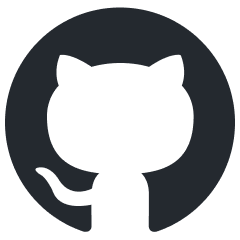
flyte
Scalable and flexible workflow orchestration platform that seamlessly unifies data, ML and analytics stacks.
Stars: 5920
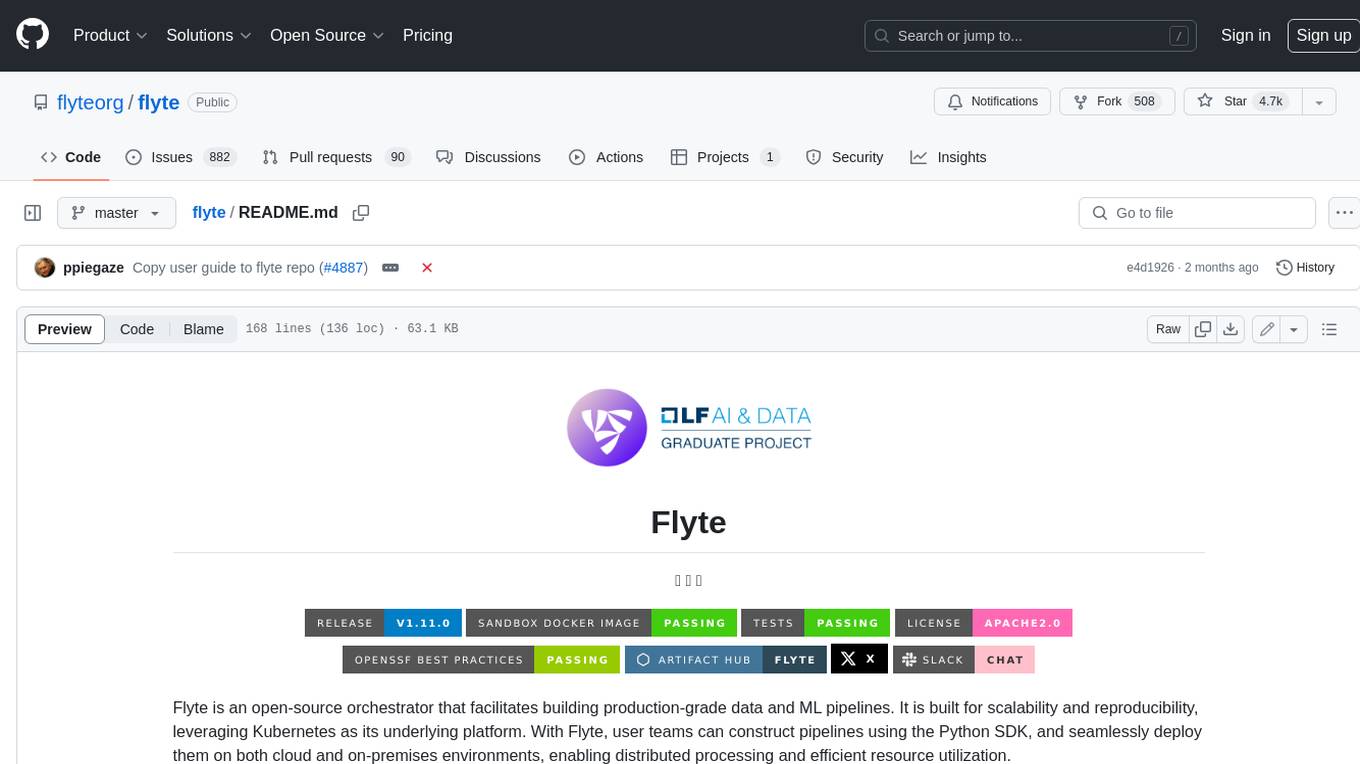
Flyte is an open-source orchestrator that facilitates building production-grade data and ML pipelines. It is built for scalability and reproducibility, leveraging Kubernetes as its underlying platform. With Flyte, user teams can construct pipelines using the Python SDK, and seamlessly deploy them on both cloud and on-premises environments, enabling distributed processing and efficient resource utilization.
README:
๐๏ธ ๐ ๐
Flyte is an open-source orchestrator that facilitates building production-grade data and ML pipelines. It is built for scalability and reproducibility, leveraging Kubernetes as its underlying platform. With Flyte, user teams can construct pipelines using the Python SDK, and seamlessly deploy them on both cloud and on-premises environments, enabling distributed processing and efficient resource utilization.
Write code in Python or any other language and leverage a robust type engine.
Either locally or on a remote cluster, execute your models with ease.
- Install Flyte's Python SDK
pip install flytekit
- Create a workflow (see example)
- Run it locally with:
pyflyte run hello_world.py hello_world_wf
Ready to try a Flyte cluster?
- Create a new sandbox cluster, running as a Docker container:
flytectl demo start
- Now execute your workflows on the cluster:
pyflyte run --remote hello_world.py hello_world_wf
Do you want to see more but don't want to install anything?
Head over to https://sandbox.union.ai/. It allows you to experiment with Flyte's capabilities from a hosted Jupyter notebook.
Ready to productionize?
Go to the Deployment guide for instructions to install Flyte on different environments
- Fine-tune Code Llama on the Flyte codebase
- Forecast sales with Horovod and Spark
- Nucleotide Sequence Querying with BLASTX
๐ Strongly typed interfaces: Validate your data at every step of the workflow by defining data guardrails using Flyte types.
๐ Any language: Write code in any language using raw containers, or choose Python, Java, Scala or JavaScript SDKs to develop your Flyte workflows.
๐ Immutability: Immutable executions help ensure reproducibility by preventing any changes to the state of an execution.
๐งฌ Data lineage: Track the movement and transformation of data throughout the lifecycle of your data and ML workflows.
๐ Map tasks: Achieve parallel code execution with minimal configuration using map tasks.
๐ Multi-tenancy: Multiple users can share the same platform while maintaining their own distinct data and configurations.
๐ Dynamic workflows: Build flexible and adaptable workflows that can change and evolve as needed, making it easier to respond to changing requirements.
โฏ๏ธ Wait for external inputs before proceeding with the execution.
๐ณ Branching: Selectively execute branches of your workflow based on static or dynamic data produced by other tasks or input data.
๐ Data visualization: Visualize data, monitor models and view training history through plots.
๐ FlyteFile & FlyteDirectory: Transfer files and directories between local and cloud storage.
๐๏ธ Structured dataset: Convert dataframes between types and enforce column-level type checking using the abstract 2D representation provided by Structured Dataset.
๐ก๏ธ Recover from failures: Recover only the failed tasks.
๐ Rerun a single task: Rerun workflows at the most granular level without modifying the previous state of a data/ML workflow.
๐ Cache outputs: Cache task outputs by passing cache=True
to the task decorator.
๐ฉ Intra-task checkpointing: Checkpoint progress within a task execution.
โฐ Timeout: Define a timeout period, after which the task is marked as failure.
๐ญ Dev to prod: As simple as changing your domain from development or staging to production.
๐ธ Spot or preemptible instances: Schedule your workflows on spot instances by setting interruptible
to True
in the task decorator.
โ๏ธ Cloud-native deployment: Deploy Flyte on AWS, GCP, Azure and other cloud services.
๐
Scheduling: Schedule your data and ML workflows to run at a specific time.
๐ข Notifications: Stay informed about changes to your workflow's state by configuring notifications through Slack, PagerDuty or email.
โ๏ธ Timeline view: Evaluate the duration of each of your Flyte tasks and identify potential bottlenecks.
๐จ GPU acceleration: Enable and control your tasksโ GPU demands by requesting resources in the task decorator.
๐ณ Dependency isolation via containers: Maintain separate sets of dependencies for your tasks so no dependency conflicts arise.
๐ Parallelism: Flyte tasks are inherently parallel to optimize resource consumption and improve performance.
๐พ Allocate resources dynamically at the task level.
Join the likes of LinkedIn, Spotify, Freenome, Pachama, Warner Bros. and many others in adopting Flyte for mission-critical use cases. For a full list of adopters and information on how to add your organization or project, please visit our ADOPTERS page.
๐ฅ Monthly community sync: Happening the first Tuesday of every month, this is where the Flyte team provides updates on the project, and community members can share their progress and ask questions.
๐ฌ Slack: Join the Flyte community on Slack to chat with other users, ask questions, and get help.
๐น Youtube: Tune into panel discussions, customer success stories, community updates and feature deep dives.
๐ Blog: Here, you can find tutorials and feature deep dives to help you learn more about Flyte.
๐ก RFCs: RFCs are used for proposing new ideas and features to improve Flyte. You can refer to them to stay updated on the latest developments and contribute to the growth of the platform.
There are many ways to get involved in Flyte, including:
- Submitting bugs and feature requests for various components.
- Reviewing the documentation and submitting pull requests for anything from fixing typos to adding new content.
- Speaking or writing about Flyte or any other ecosystem integration and letting us know!
- Taking on a
help wanted
orgood-first-issue
and following the CONTRIBUTING guide to submit changes to the codebase. - Upvoting popular feature requests to show your support.
Flyte is available under the Apache License 2.0. Use it wisely.
For Tasks:
Click tags to check more tools for each tasksFor Jobs:
Alternative AI tools for flyte
Similar Open Source Tools
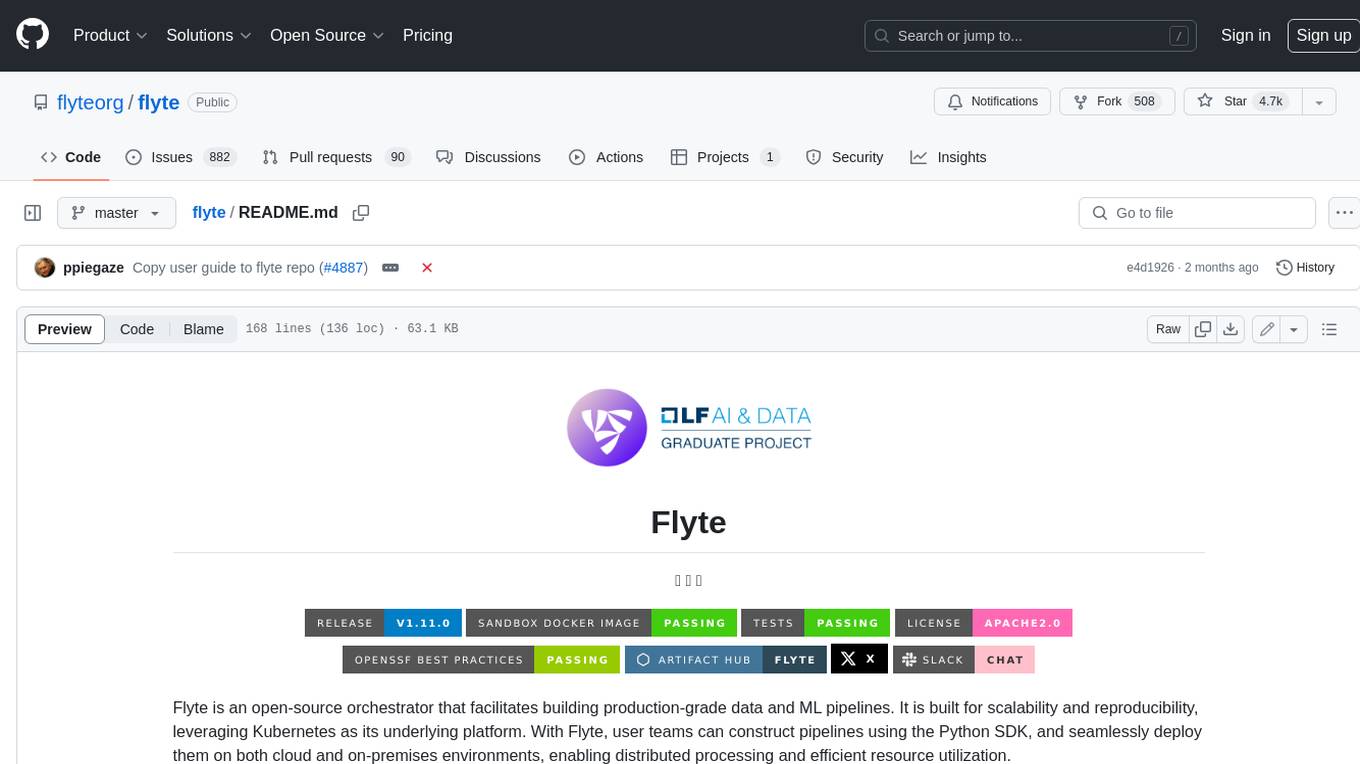
flyte
Flyte is an open-source orchestrator that facilitates building production-grade data and ML pipelines. It is built for scalability and reproducibility, leveraging Kubernetes as its underlying platform. With Flyte, user teams can construct pipelines using the Python SDK, and seamlessly deploy them on both cloud and on-premises environments, enabling distributed processing and efficient resource utilization.
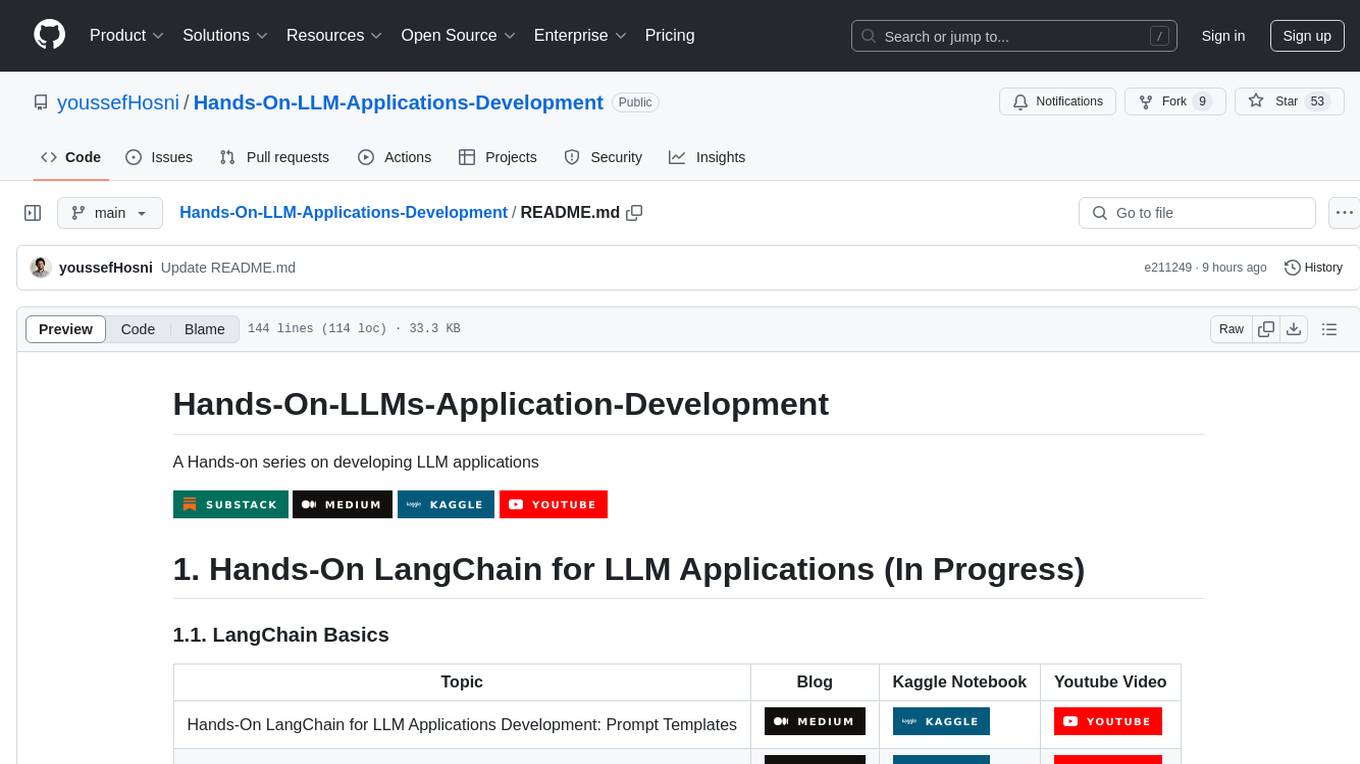
Hands-On-LLM-Applications-Development
Hands-On-LLM-Applications-Development is a repository focused on developing applications using Large Language Models (LLMs). The repository provides hands-on tutorials, guides, and resources for building various applications such as LangChain for LLM applications, Retrieval Augmented Generation (RAG) with LangChain, building LLM agents with LangGraph, and advanced LangChain with OpenAI. It covers topics like prompt engineering for LLMs, building applications using HuggingFace open-source models, LLM fine-tuning, and advanced RAG applications.
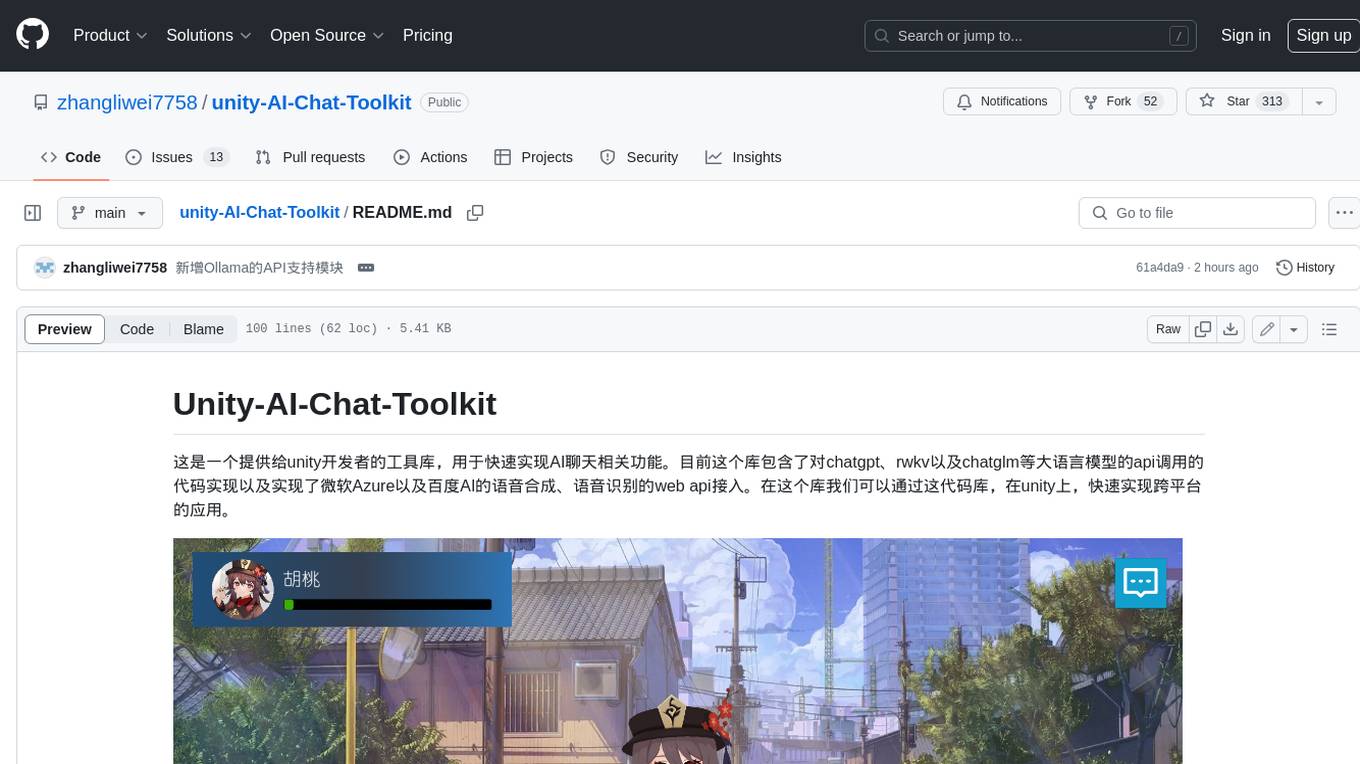
unity-AI-Chat-Toolkit
The Unity-AI-Chat-Toolkit is a toolset for Unity developers to quickly implement AI chat-related functions. Currently, this library includes code implementations for API calls to large language models such as ChatGPT, RKV, and ChatGLM, as well as web API access to Microsoft Azure and Baidu AI for speech synthesis and speech recognition. With this library, we can quickly implement cross-platform applications on Unity.
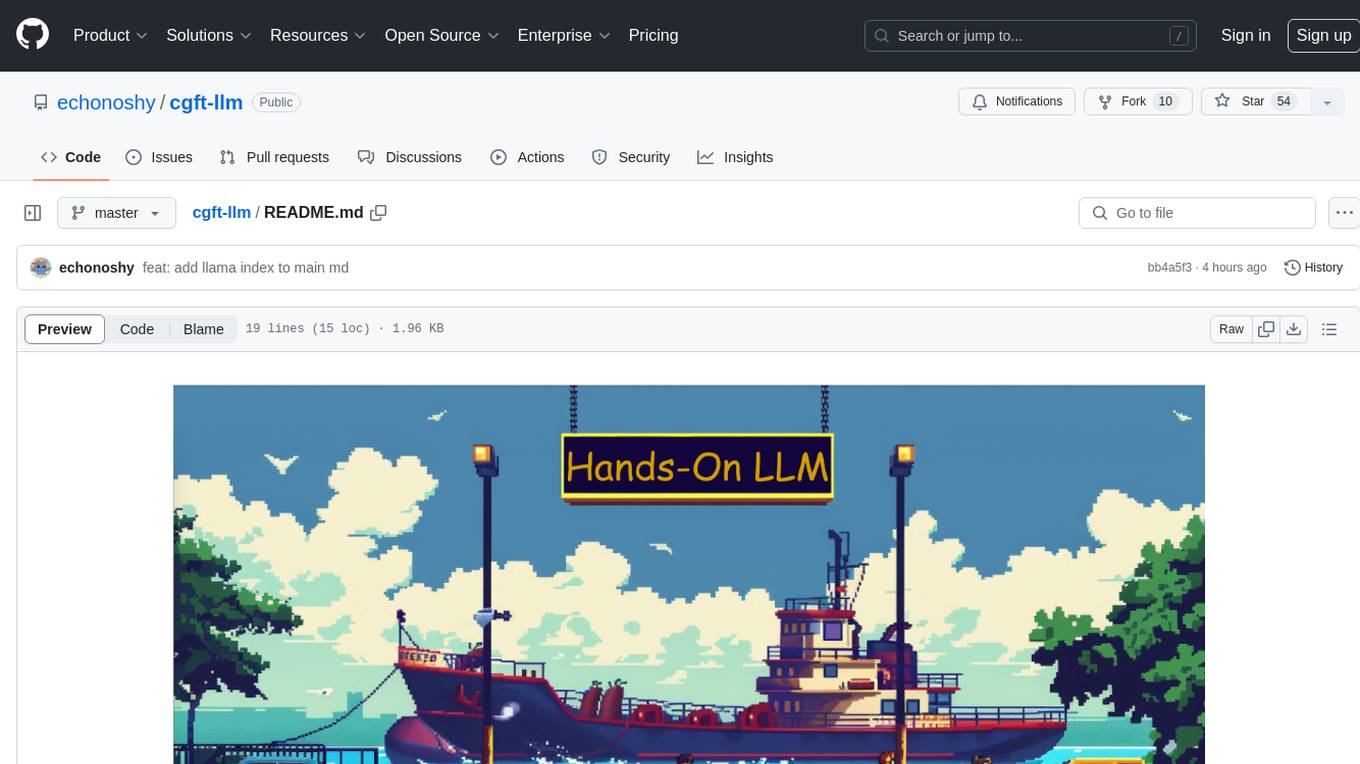
cgft-llm
The cgft-llm repository is a collection of video tutorials and documentation for implementing large models. It provides guidance on topics such as fine-tuning llama3 with llama-factory, lightweight deployment and quantization using llama.cpp, speech generation with ChatTTS, introduction to Ollama for large model deployment, deployment tools for vllm and paged attention, and implementing RAG with llama-index. Users can find detailed code documentation and video tutorials for each project in the repository.
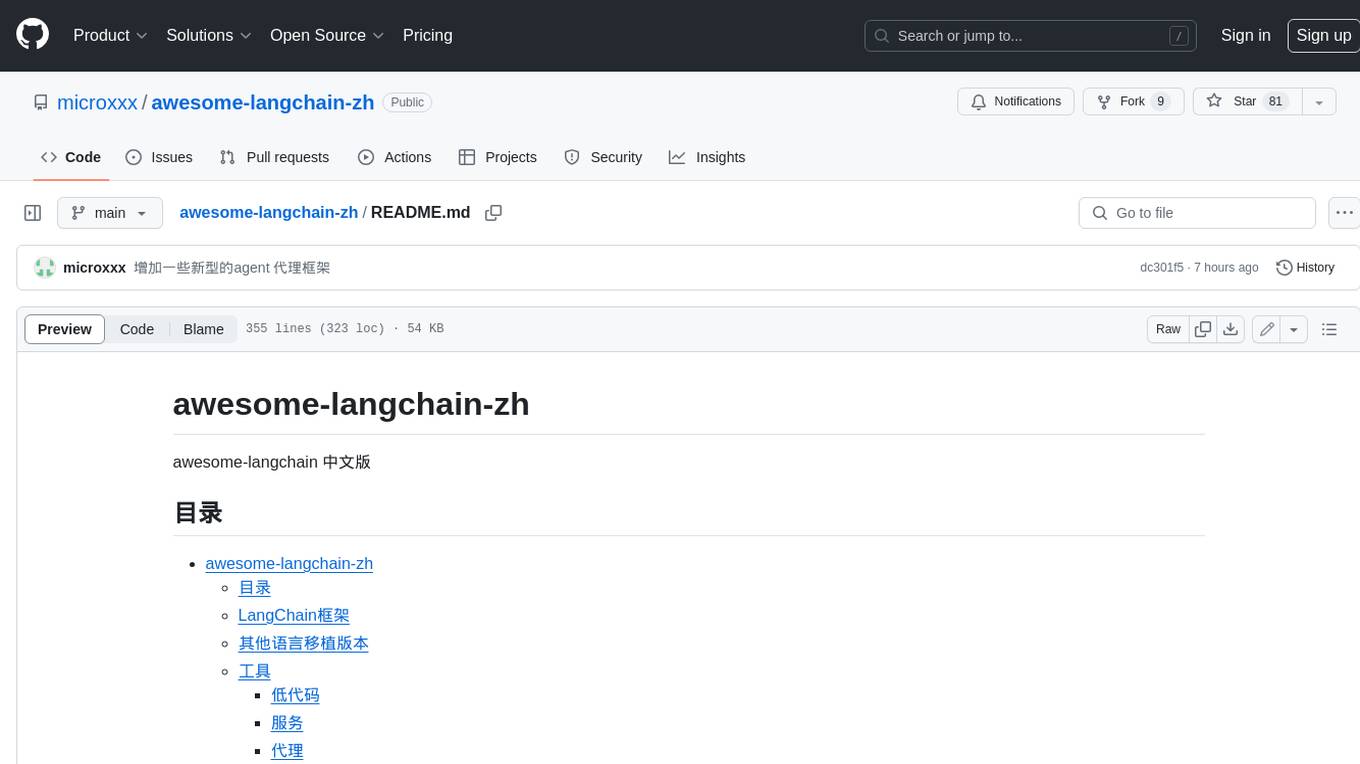
awesome-langchain-zh
The awesome-langchain-zh repository is a collection of resources related to LangChain, a framework for building AI applications using large language models (LLMs). The repository includes sections on the LangChain framework itself, other language ports of LangChain, tools for low-code development, services, agents, templates, platforms, open-source projects related to knowledge management and chatbots, as well as learning resources such as notebooks, videos, and articles. It also covers other LLM frameworks and provides additional resources for exploring and working with LLMs. The repository serves as a comprehensive guide for developers and AI enthusiasts interested in leveraging LangChain and LLMs for various applications.
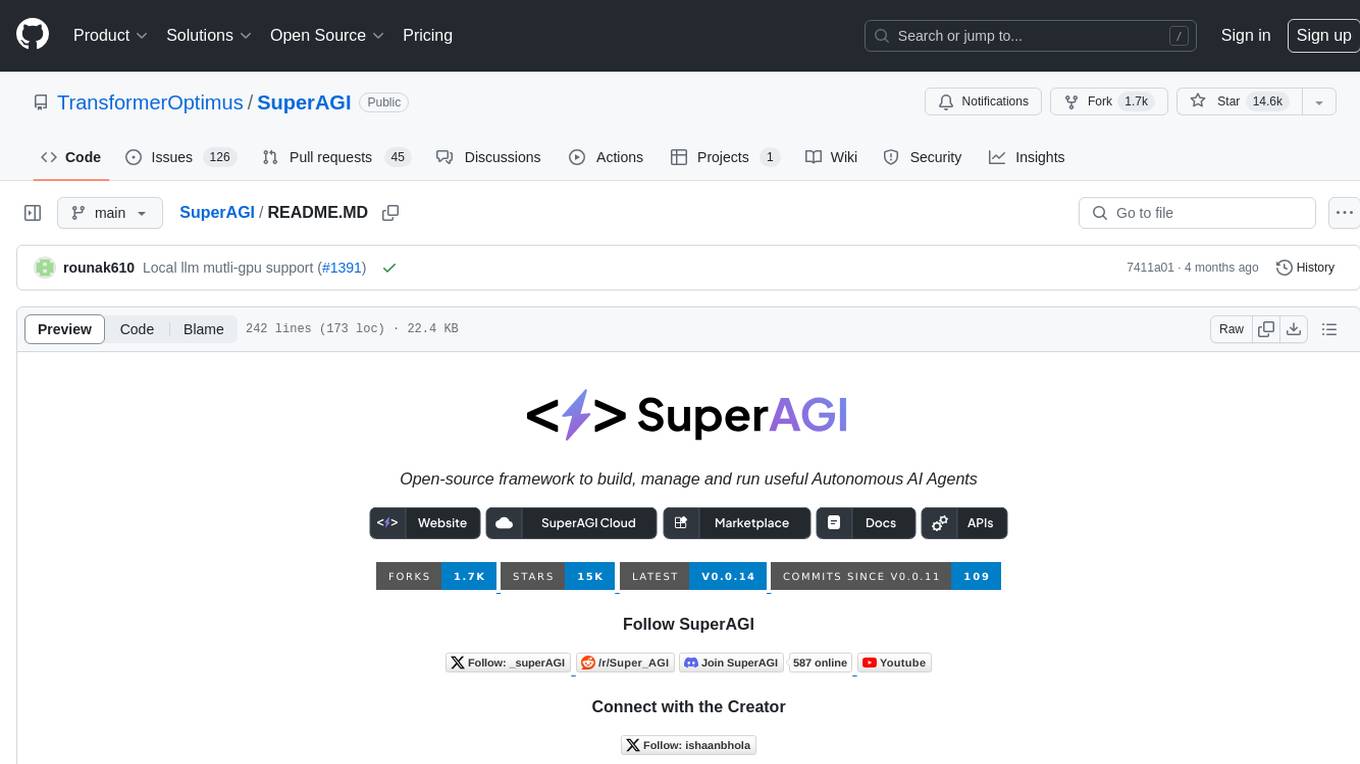
SuperAGI
SuperAGI is an open-source framework designed to build, manage, and run autonomous AI agents. It enables developers to create production-ready and scalable agents, extend agent capabilities with toolkits, and interact with agents through a graphical user interface. The framework allows users to connect to multiple Vector DBs, optimize token usage, store agent memory, utilize custom fine-tuned models, and automate tasks with predefined steps. SuperAGI also provides a marketplace for toolkits that enable agents to interact with external systems and third-party plugins.
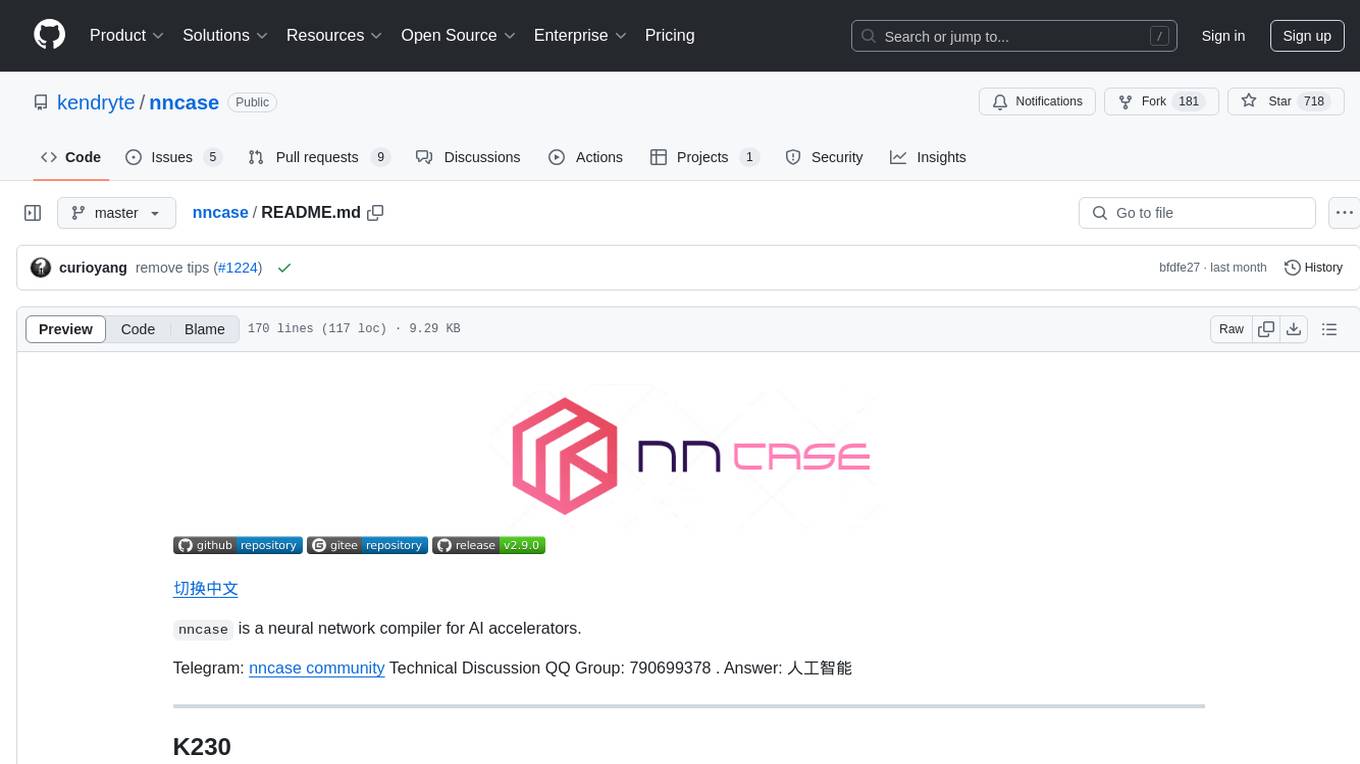
nncase
nncase is a neural network compiler for AI accelerators that supports multiple inputs and outputs, static memory allocation, operators fusion and optimizations, float and quantized uint8 inference, post quantization from float model with calibration dataset, and flat model with zero copy loading. It can be installed via pip and supports TFLite, Caffe, and ONNX ops. Users can compile nncase from source using Ninja or make. The tool is suitable for tasks like image classification, object detection, image segmentation, pose estimation, and more.
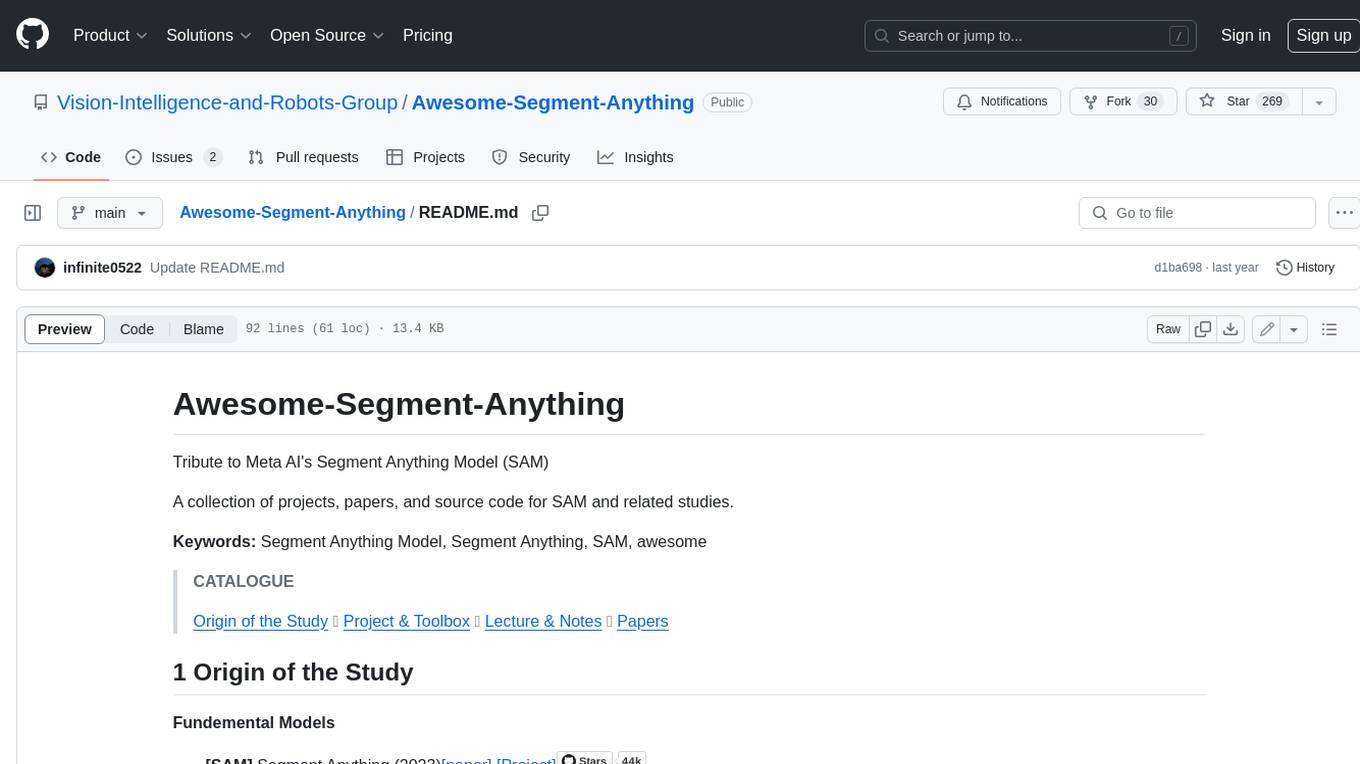
Awesome-Segment-Anything
The Segment Anything Model (SAM) is a powerful tool that allows users to segment any object in an image with just a few clicks. This makes it a great tool for a variety of tasks, such as object detection, tracking, and editing. SAM is also very easy to use, making it a great option for both beginners and experienced users.
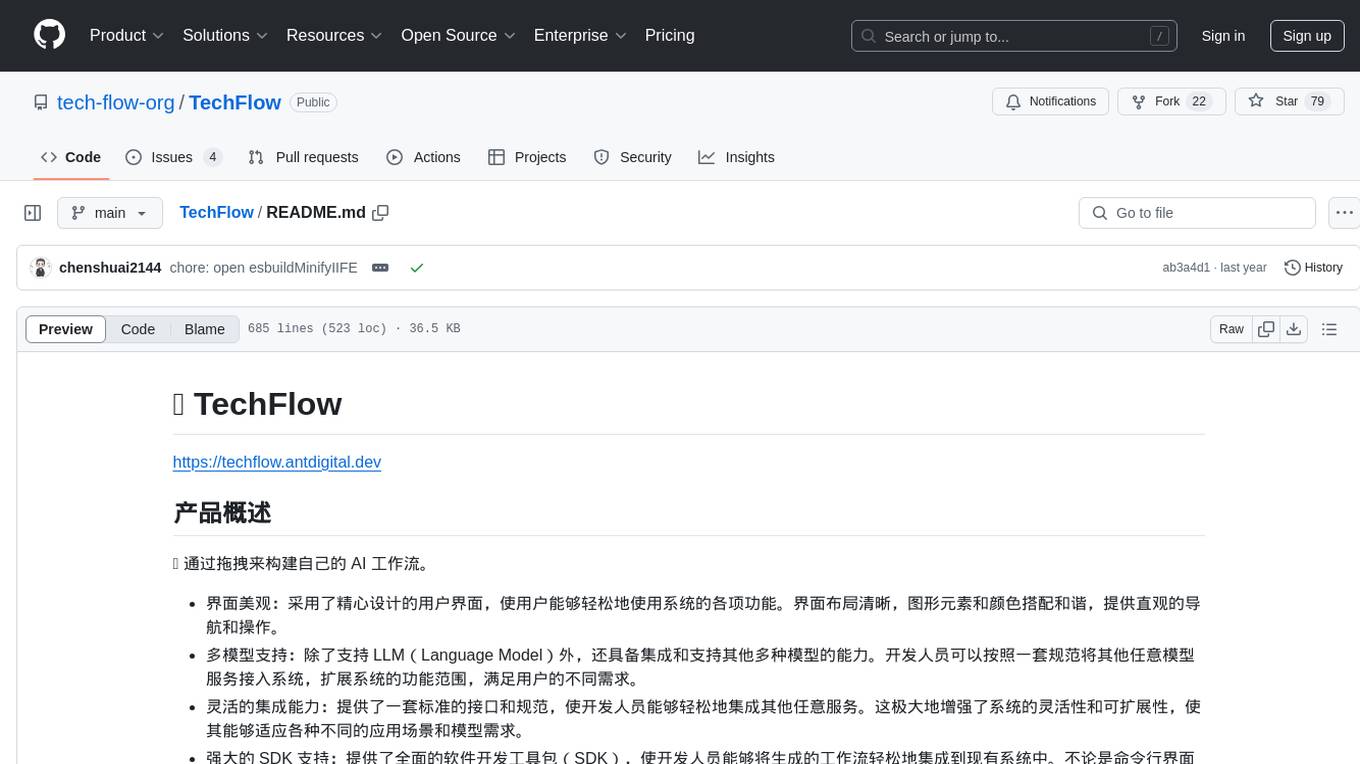
TechFlow
TechFlow is a platform that allows users to build their own AI workflows through drag-and-drop functionality. It features a visually appealing interface with clear layout and intuitive navigation. TechFlow supports multiple models beyond Language Models (LLM) and offers flexible integration capabilities. It provides a powerful SDK for developers to easily integrate generated workflows into existing systems, enhancing flexibility and scalability. The platform aims to embed AI capabilities as modules into existing functionalities to enhance business competitiveness.
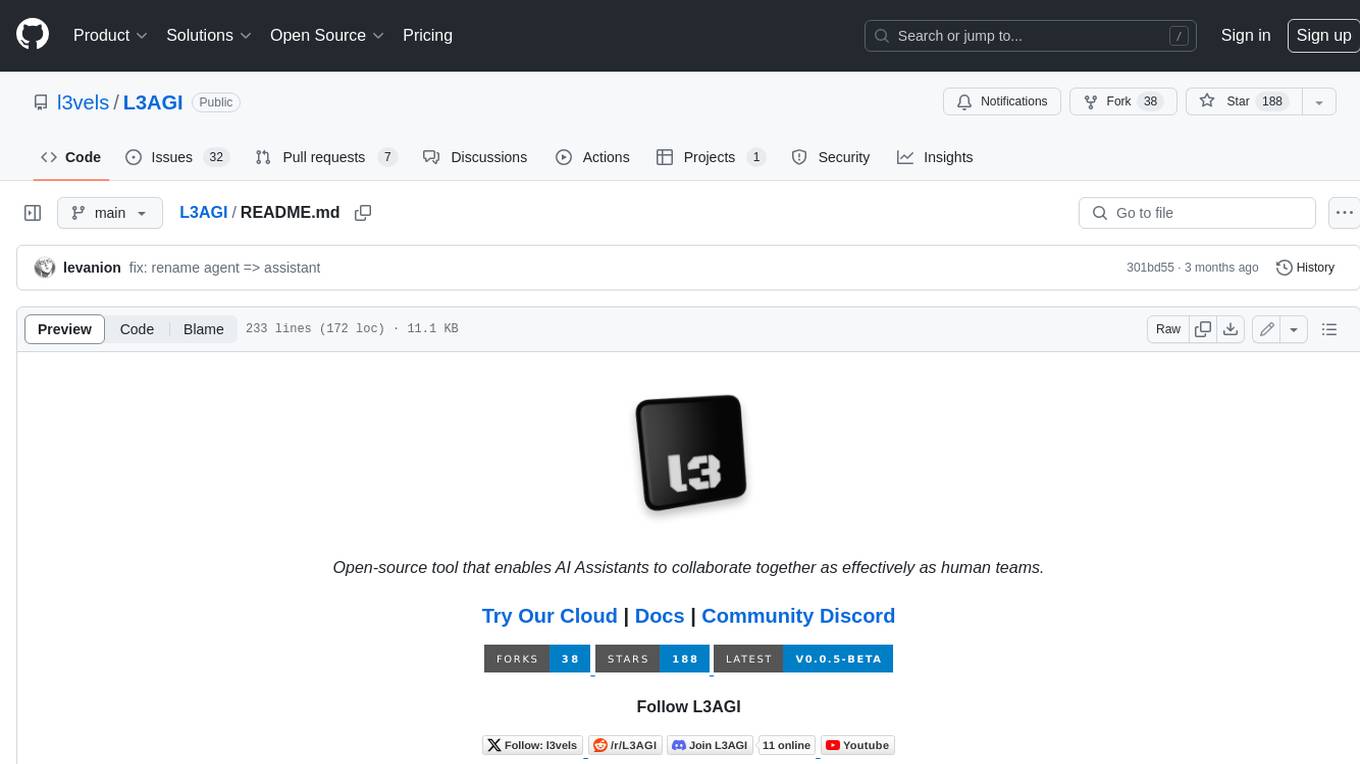
L3AGI
L3AGI is an open-source tool that enables AI Assistants to collaborate together as effectively as human teams. It provides a robust set of functionalities that empower users to design, supervise, and execute both autonomous AI Assistants and Teams of Assistants. Key features include the ability to create and manage Teams of AI Assistants, design and oversee standalone AI Assistants, equip AI Assistants with the ability to retain and recall information, connect AI Assistants to an array of data sources for efficient information retrieval and processing, and employ curated sets of tools for specific tasks. L3AGI also offers a user-friendly interface, APIs for integration with other systems, and a vibrant community for support and collaboration.
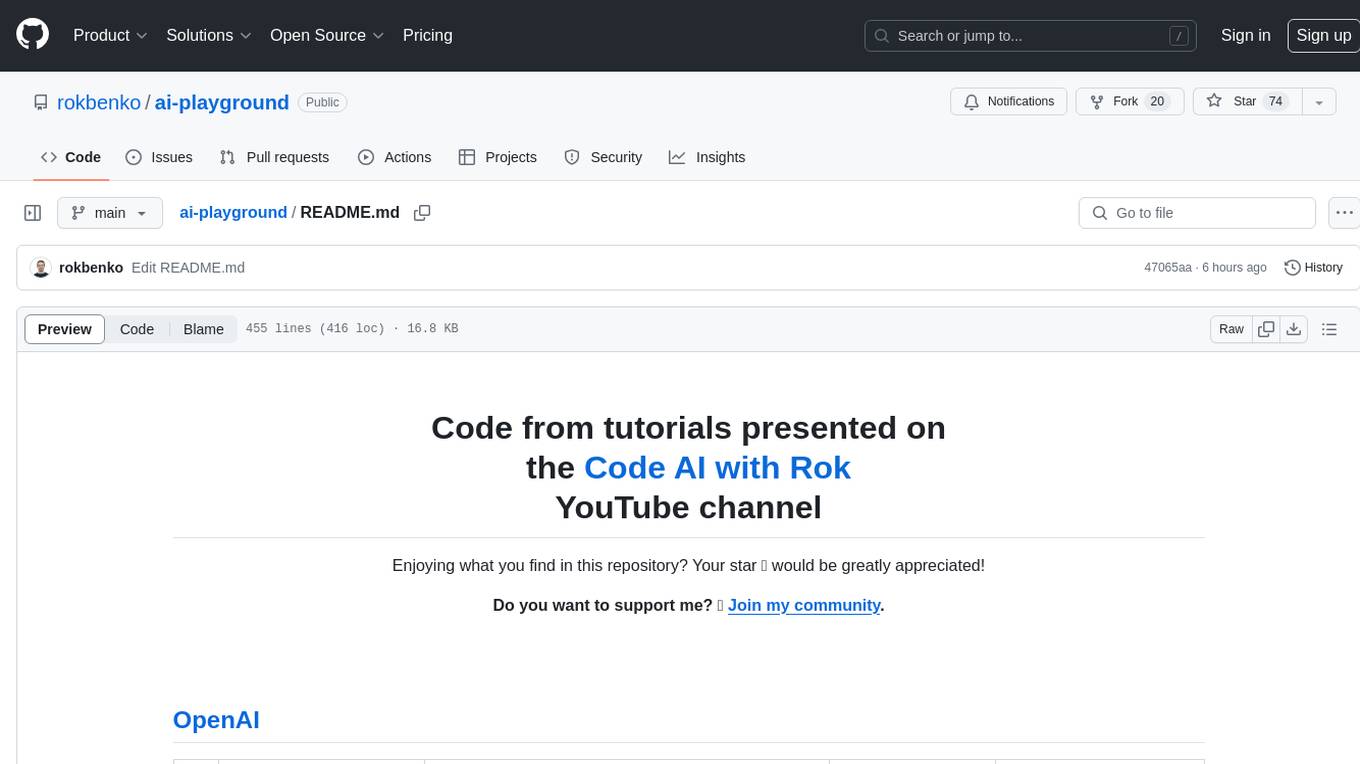
ai-playground
The ai-playground repository contains code from tutorials presented on the Code AI with Rok YouTube channel. It includes tutorials on using the OpenAI Assistants API v1 beta to build personal math tutors, customer support chatbots, and more. Additionally, there are tutorials on using Gemini Pro API, Snowflake Cortex LLM functions, LlamaIndex chat streaming app, Fetch.ai uAgents, Milvus Standalone, spaCy for NER, and more. The repository aims to provide practical examples and guides for developers interested in AI-related projects and tools.
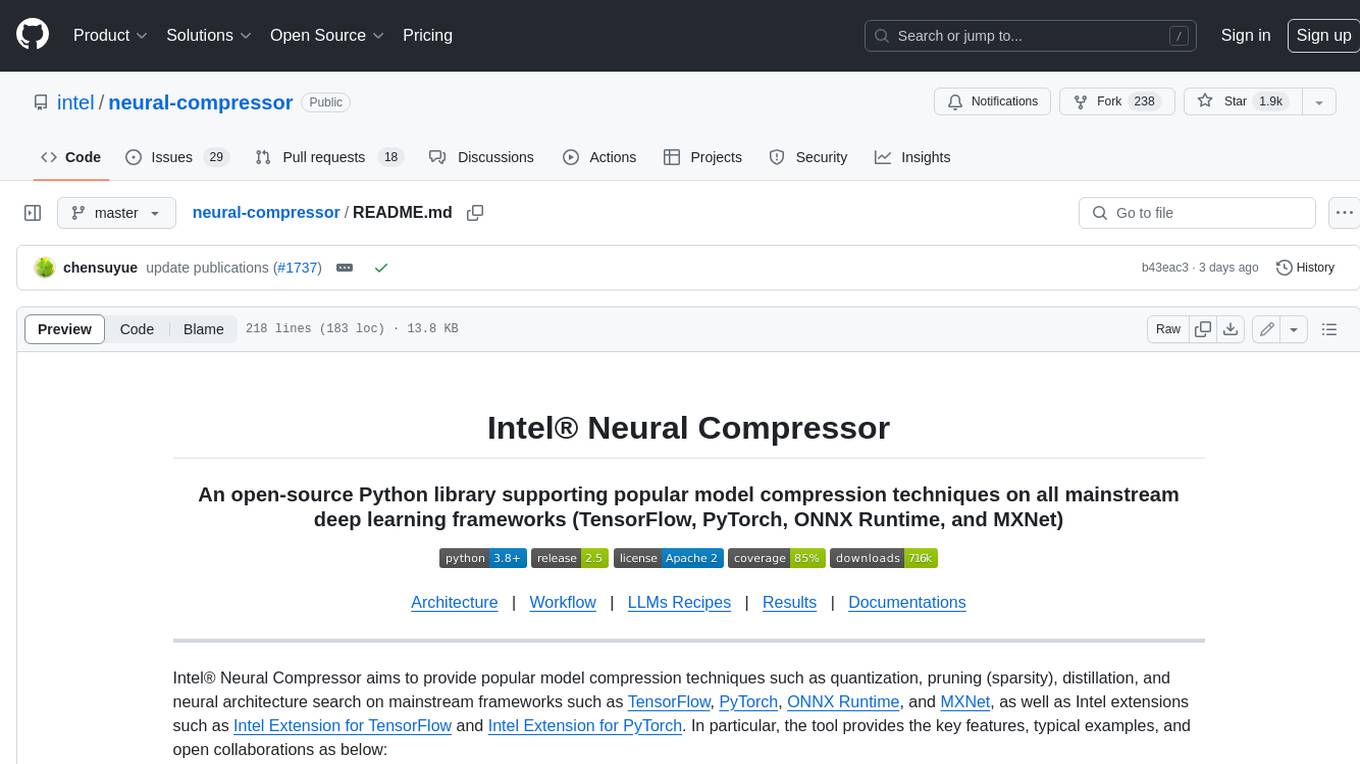
neural-compressor
Intelยฎ Neural Compressor is an open-source Python library that supports popular model compression techniques such as quantization, pruning (sparsity), distillation, and neural architecture search on mainstream frameworks such as TensorFlow, PyTorch, ONNX Runtime, and MXNet. It provides key features, typical examples, and open collaborations, including support for a wide range of Intel hardware, validation of popular LLMs, and collaboration with cloud marketplaces, software platforms, and open AI ecosystems.
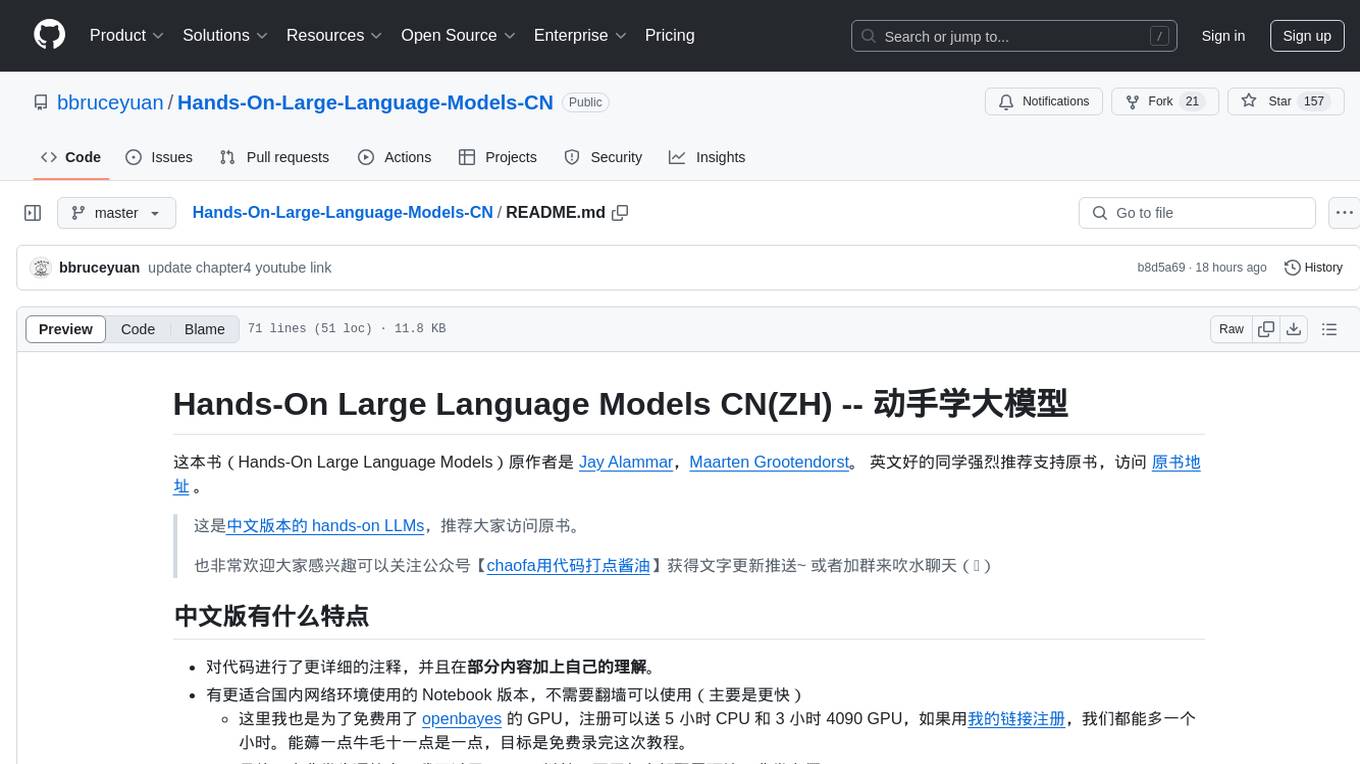
Hands-On-Large-Language-Models-CN
Hands-On Large Language Models CN(ZH) is a Chinese version of the book 'Hands-On Large Language Models' by Jay Alammar and Maarten Grootendorst. It provides detailed code annotations and additional insights, offers Notebook versions suitable for Chinese network environments, utilizes openbayes for free GPU access, allows convenient environment setup with vscode, and includes accompanying Chinese language videos on platforms like Bilibili and YouTube. The book covers various chapters on topics like Tokens and Embeddings, Transformer LLMs, Text Classification, Text Clustering, Prompt Engineering, Text Generation, Semantic Search, Multimodal LLMs, Text Embedding Models, Fine-tuning Models, and more.
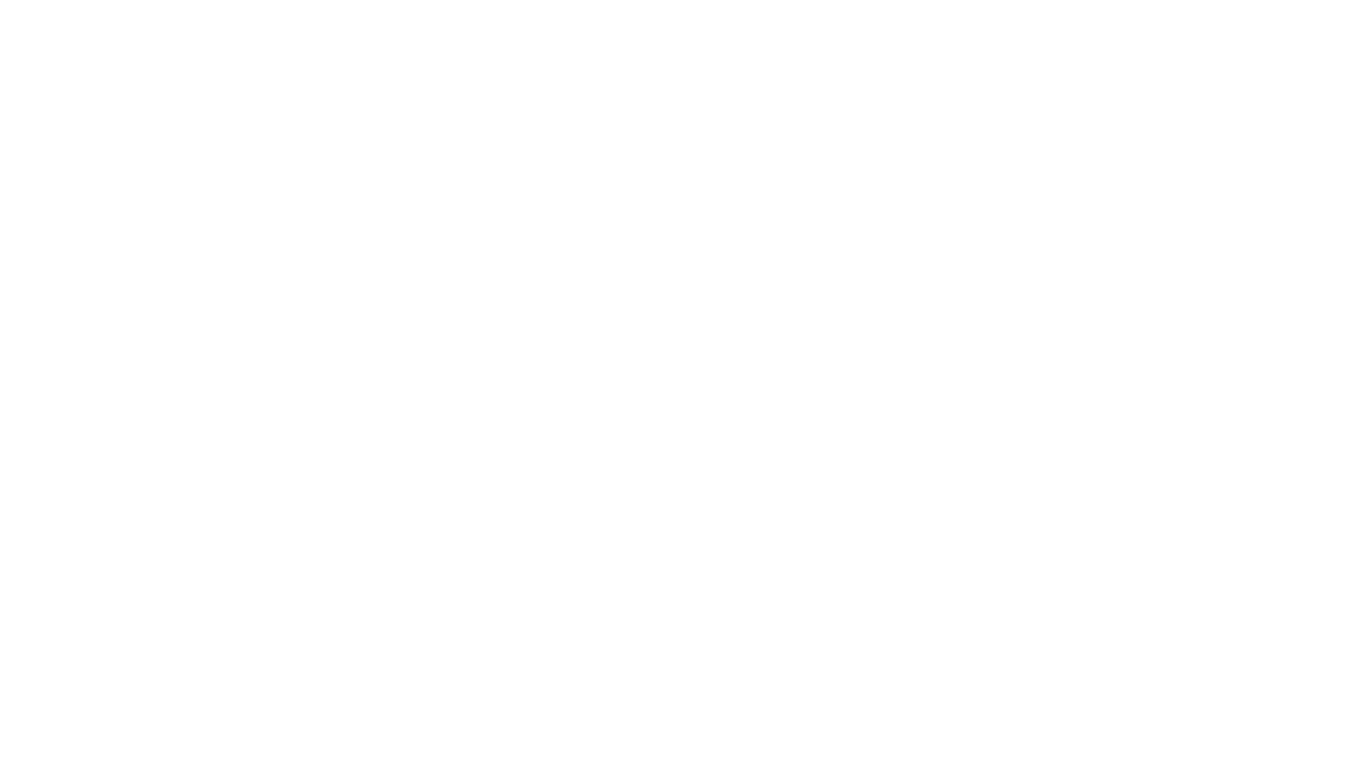
intel-extension-for-transformers
Intelยฎ Extension for Transformers is an innovative toolkit designed to accelerate GenAI/LLM everywhere with the optimal performance of Transformer-based models on various Intel platforms, including Intel Gaudi2, Intel CPU, and Intel GPU. The toolkit provides the below key features and examples: * Seamless user experience of model compressions on Transformer-based models by extending [Hugging Face transformers](https://github.com/huggingface/transformers) APIs and leveraging [Intelยฎ Neural Compressor](https://github.com/intel/neural-compressor) * Advanced software optimizations and unique compression-aware runtime (released with NeurIPS 2022's paper [Fast Distilbert on CPUs](https://arxiv.org/abs/2211.07715) and [QuaLA-MiniLM: a Quantized Length Adaptive MiniLM](https://arxiv.org/abs/2210.17114), and NeurIPS 2021's paper [Prune Once for All: Sparse Pre-Trained Language Models](https://arxiv.org/abs/2111.05754)) * Optimized Transformer-based model packages such as [Stable Diffusion](examples/huggingface/pytorch/text-to-image/deployment/stable_diffusion), [GPT-J-6B](examples/huggingface/pytorch/text-generation/deployment), [GPT-NEOX](examples/huggingface/pytorch/language-modeling/quantization#2-validated-model-list), [BLOOM-176B](examples/huggingface/pytorch/language-modeling/inference#BLOOM-176B), [T5](examples/huggingface/pytorch/summarization/quantization#2-validated-model-list), [Flan-T5](examples/huggingface/pytorch/summarization/quantization#2-validated-model-list), and end-to-end workflows such as [SetFit-based text classification](docs/tutorials/pytorch/text-classification/SetFit_model_compression_AGNews.ipynb) and [document level sentiment analysis (DLSA)](workflows/dlsa) * [NeuralChat](intel_extension_for_transformers/neural_chat), a customizable chatbot framework to create your own chatbot within minutes by leveraging a rich set of [plugins](https://github.com/intel/intel-extension-for-transformers/blob/main/intel_extension_for_transformers/neural_chat/docs/advanced_features.md) such as [Knowledge Retrieval](./intel_extension_for_transformers/neural_chat/pipeline/plugins/retrieval/README.md), [Speech Interaction](./intel_extension_for_transformers/neural_chat/pipeline/plugins/audio/README.md), [Query Caching](./intel_extension_for_transformers/neural_chat/pipeline/plugins/caching/README.md), and [Security Guardrail](./intel_extension_for_transformers/neural_chat/pipeline/plugins/security/README.md). This framework supports Intel Gaudi2/CPU/GPU. * [Inference](https://github.com/intel/neural-speed/tree/main) of Large Language Model (LLM) in pure C/C++ with weight-only quantization kernels for Intel CPU and Intel GPU (TBD), supporting [GPT-NEOX](https://github.com/intel/neural-speed/tree/main/neural_speed/models/gptneox), [LLAMA](https://github.com/intel/neural-speed/tree/main/neural_speed/models/llama), [MPT](https://github.com/intel/neural-speed/tree/main/neural_speed/models/mpt), [FALCON](https://github.com/intel/neural-speed/tree/main/neural_speed/models/falcon), [BLOOM-7B](https://github.com/intel/neural-speed/tree/main/neural_speed/models/bloom), [OPT](https://github.com/intel/neural-speed/tree/main/neural_speed/models/opt), [ChatGLM2-6B](https://github.com/intel/neural-speed/tree/main/neural_speed/models/chatglm), [GPT-J-6B](https://github.com/intel/neural-speed/tree/main/neural_speed/models/gptj), and [Dolly-v2-3B](https://github.com/intel/neural-speed/tree/main/neural_speed/models/gptneox). Support AMX, VNNI, AVX512F and AVX2 instruction set. We've boosted the performance of Intel CPUs, with a particular focus on the 4th generation Intel Xeon Scalable processor, codenamed [Sapphire Rapids](https://www.intel.com/content/www/us/en/products/docs/processors/xeon-accelerated/4th-gen-xeon-scalable-processors.html).
For similar tasks
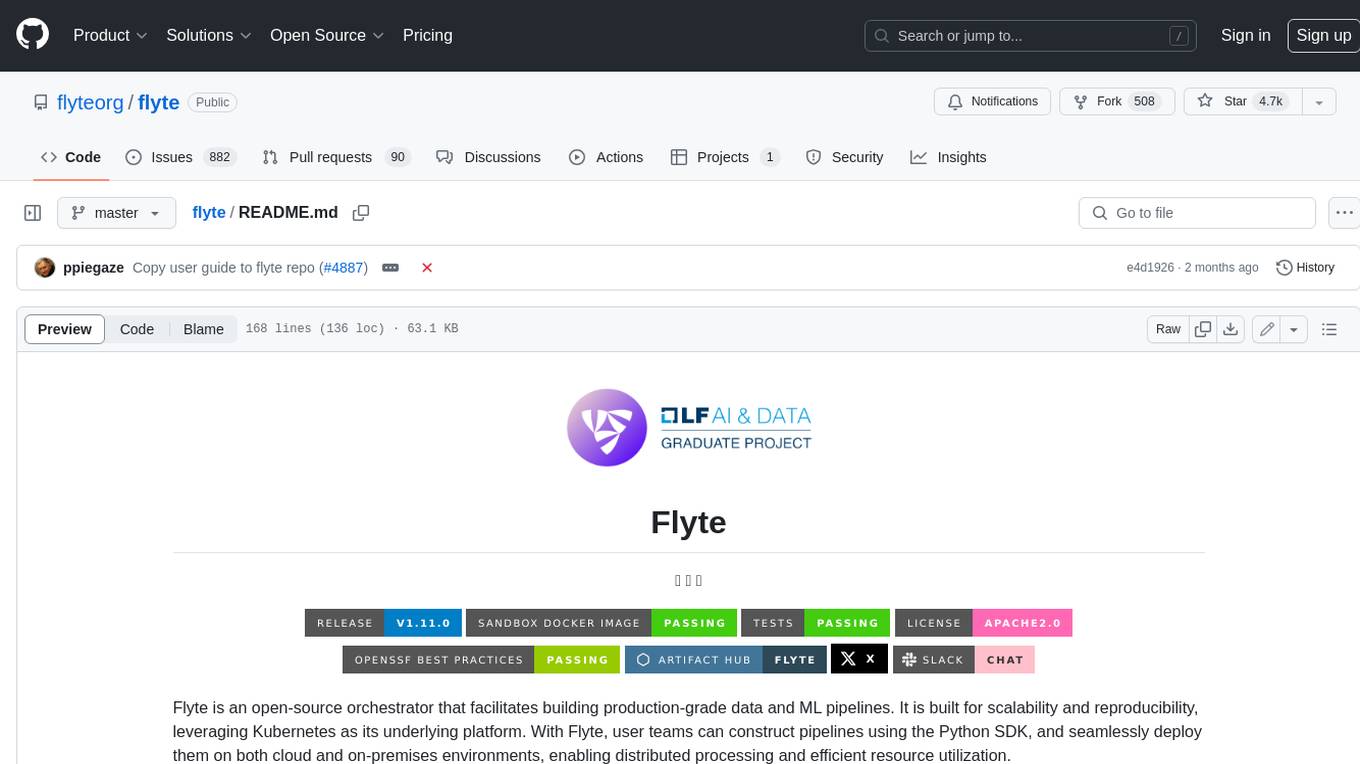
flyte
Flyte is an open-source orchestrator that facilitates building production-grade data and ML pipelines. It is built for scalability and reproducibility, leveraging Kubernetes as its underlying platform. With Flyte, user teams can construct pipelines using the Python SDK, and seamlessly deploy them on both cloud and on-premises environments, enabling distributed processing and efficient resource utilization.
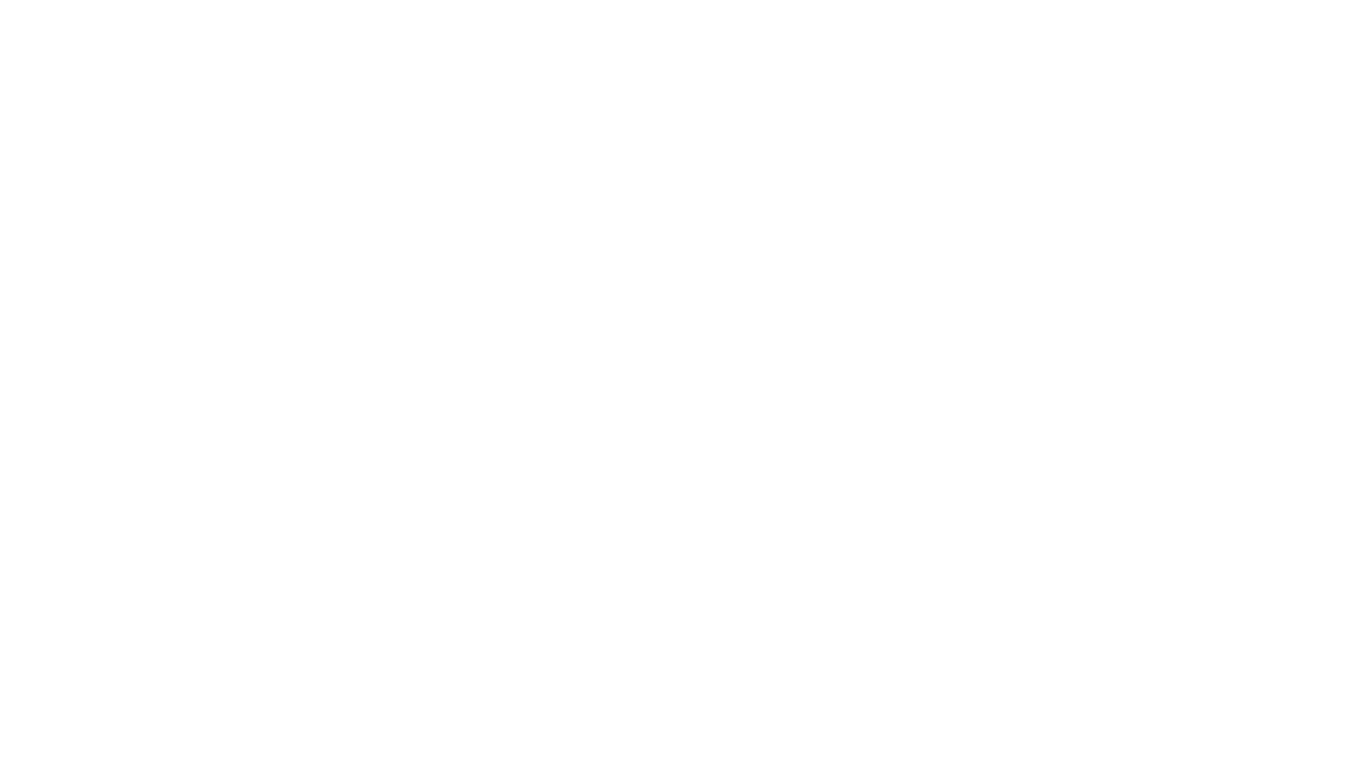
project_alice
Alice is an agentic workflow framework that integrates task execution and intelligent chat capabilities. It provides a flexible environment for creating, managing, and deploying AI agents for various purposes, leveraging a microservices architecture with MongoDB for data persistence. The framework consists of components like APIs, agents, tasks, and chats that interact to produce outputs through files, messages, task results, and URL references. Users can create, test, and deploy agentic solutions in a human-language framework, making it easy to engage with by both users and agents. The tool offers an open-source option, user management, flexible model deployment, and programmatic access to tasks and chats.
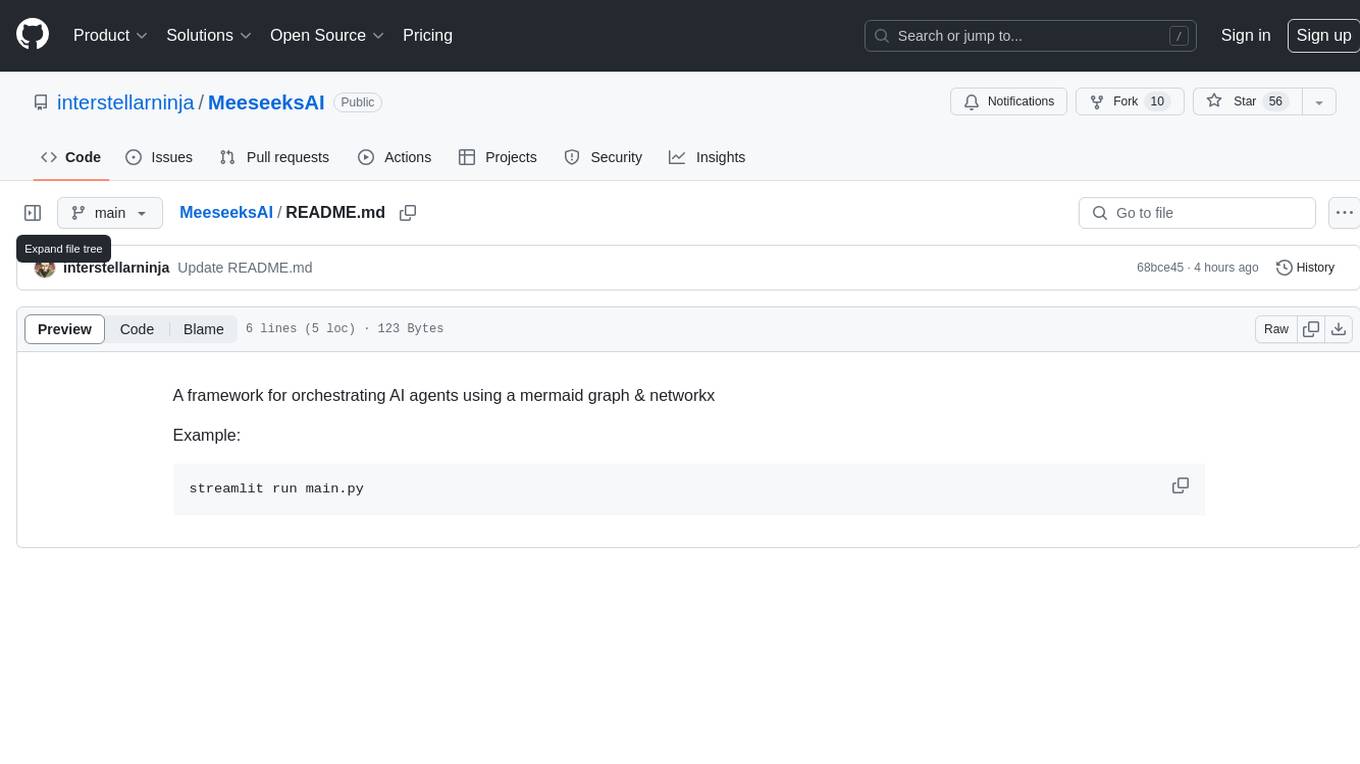
MeeseeksAI
MeeseeksAI is a framework designed to orchestrate AI agents using a mermaid graph and networkx. It provides a structured approach to managing and coordinating multiple AI agents within a system. The framework allows users to define the interactions and dependencies between agents through a visual representation, making it easier to understand and modify the behavior of the AI system. By leveraging the power of networkx, MeeseeksAI enables efficient graph-based computations and optimizations, enhancing the overall performance of AI workflows. With its intuitive design and flexible architecture, MeeseeksAI simplifies the process of building and deploying complex AI systems, empowering users to create sophisticated agent interactions with ease.
For similar jobs
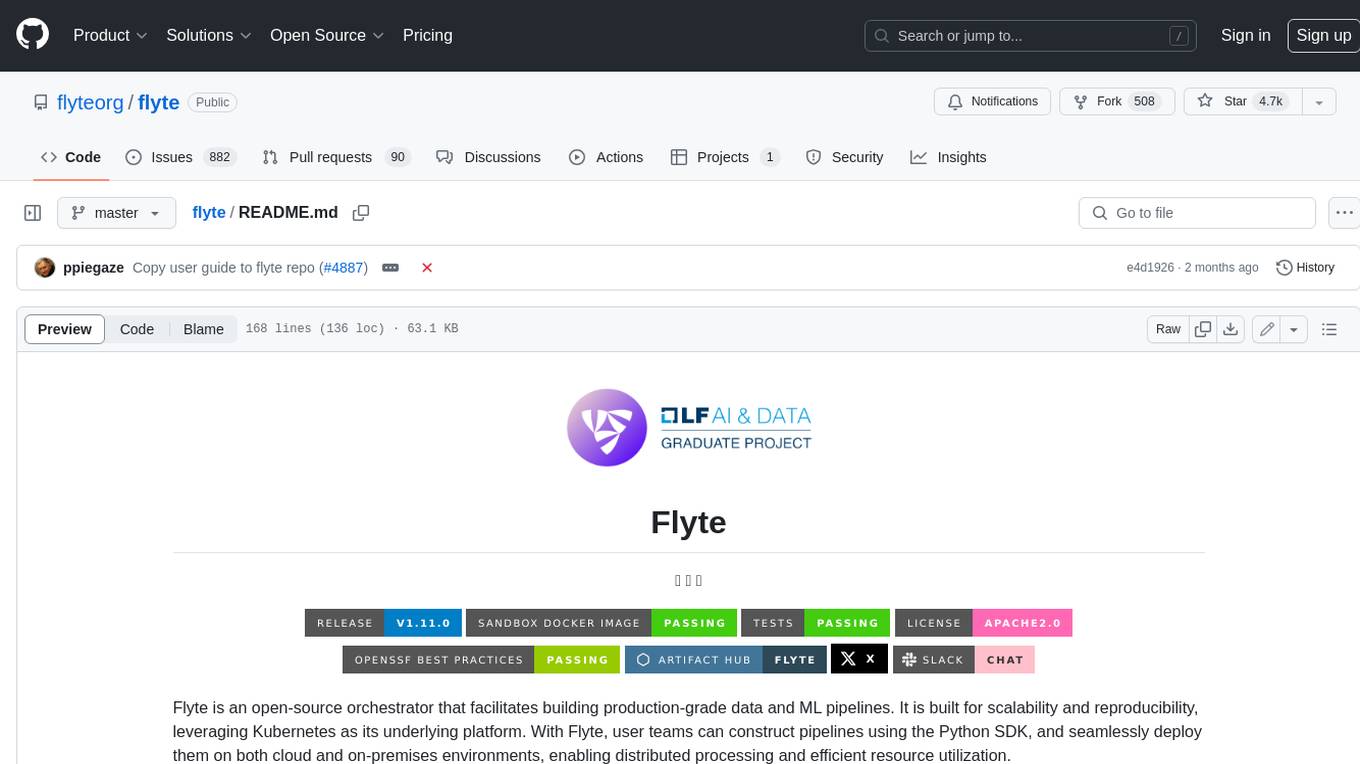
flyte
Flyte is an open-source orchestrator that facilitates building production-grade data and ML pipelines. It is built for scalability and reproducibility, leveraging Kubernetes as its underlying platform. With Flyte, user teams can construct pipelines using the Python SDK, and seamlessly deploy them on both cloud and on-premises environments, enabling distributed processing and efficient resource utilization.