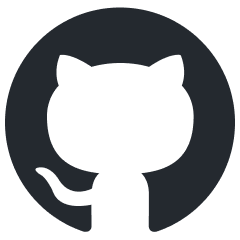
AI-Security-and-Privacy-Events
A curated list of academic events on AI Security & Privacy
Stars: 124
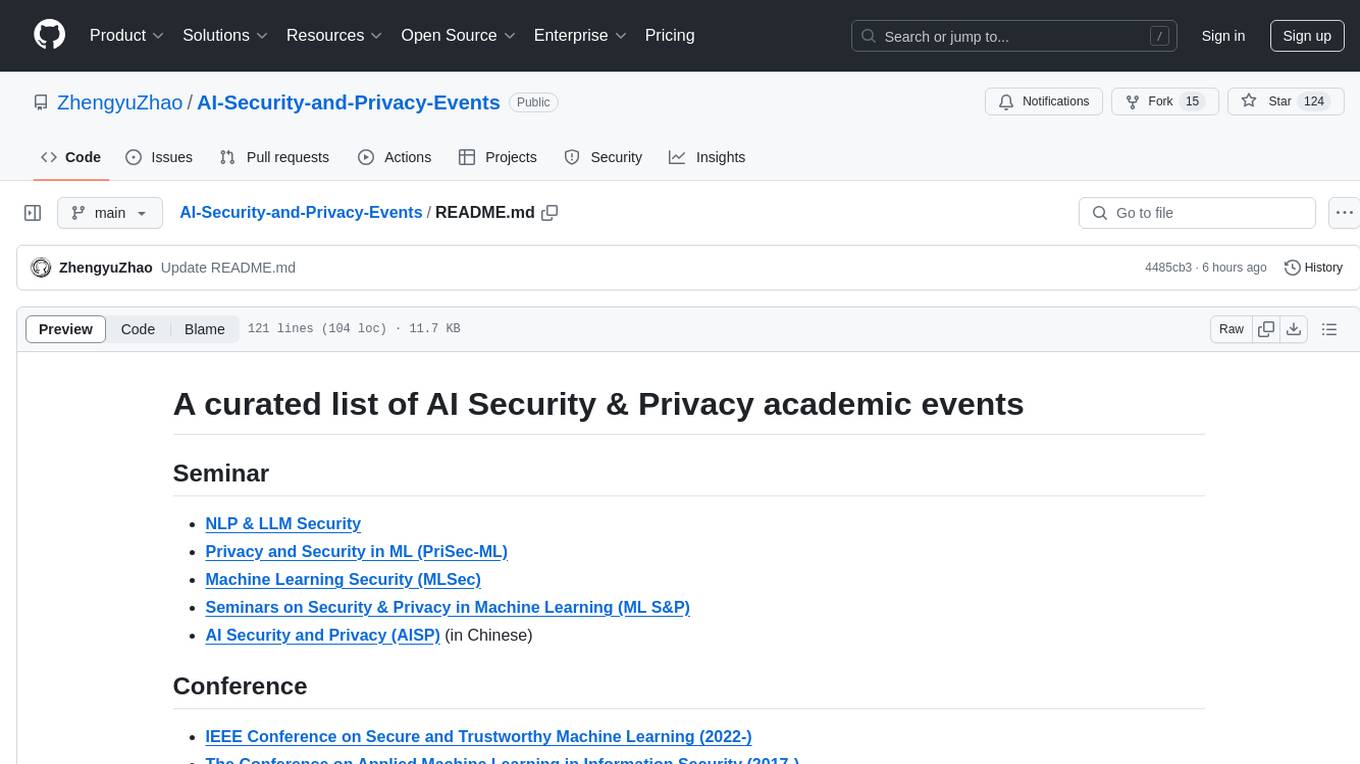
AI-Security-and-Privacy-Events is a curated list of academic events focusing on AI security and privacy. It includes seminars, conferences, workshops, tutorials, special sessions, and covers various topics such as NLP & LLM Security, Privacy and Security in ML, Machine Learning Security, AI System with Confidential Computing, Adversarial Machine Learning, and more.
README:
- NLP & LLM Security
- Privacy and Security in ML (PriSec-ML)
- Machine Learning Security (MLSec)
- Seminars on Security & Privacy in Machine Learning (ML S&P)
- AI Security and Privacy (AISP) (in Chinese)
- IEEE Conference on Secure and Trustworthy Machine Learning (2022-)
- The Conference on Applied Machine Learning in Information Security (2017-)
-
- Red Teaming GenAI: What Can We Learn from Adversaries? (NeurIPS 2024)
- Safe Generative AI (NeurIPS 2024)
- Towards Safe & Trustworthy Agents (NeurIPS 2024)
- Socially Responsible Language Modelling Research (NeurIPS 2024)
- Next Generation of AI Safety (ICML 2024)
- Trustworthy Multi-modal Foundation Models and AI Agents (ICML 2024)
- Secure and Trustworthy Large Language Models (ICLR 2024)
- Reliable and Responsible Foundation Models (ICLR 2024)
- Privacy Regulation and Protection in Machine Learning (ICLR 2024)
- Responsible Language Models (AAAI 2024)
- Privacy-Preserving Artificial Intelligence (AAAI 2020-2024)
- Practical Deep Learning in the Wild (CAI 2024, AAAI 2022-2023)
- Backdoors in Deep Learning: The Good, the Bad, and the Ugly (NeurIPS 2023)
- Trustworthy and Reliable Large-Scale Machine Learning Models (ICLR 2023)
- Backdoor Attacks and Defenses in Machine Learning (ICLR 2023)
- Privacy, Accountability, Interpretability, Robustness, Reasoning on Structured Data (ICLR 2022)
- Security and Safety in Machine Learning Systems (ICLR 2021)
- Robust and Reliable Machine Learning in the Real World (ICLR 2021)
- Towards Trustworthy ML: Rethinking Security and Privacy for ML (ICLR 2020)
- Safe Machine Learning: Specification, Robustness and Assurance (ICLR 2019)
- New Frontiers in Adversarial Machine Learning (ICML 2022-2023)
- Theory and Practice of Differential Privacy (ICML 2021-2022)
- Uncertainty & Robustness in Deep Learning (ICML 2020-2021)
- A Blessing in Disguise: The Prospects and Perils of Adversarial Machine Learning (ICML 2021)
- Security and Privacy of Machine Learning (ICML 2019)
- Socially Responsible Machine Learning (NeurIPS 2022, ICLR 2022, ICML 2021)
- ML Safety (NeurIPS 2022)
- Privacy in Machine Learning (NeurIPS 2021)
- Dataset Curation and Security (NeurIPS 2020)
- Security in Machine Learning (NeurIPS 2018)
- Machine Learning and Computer Security (NeurIPS 2017)
- Adversarial Training (NeurIPS 2016)
- Reliable Machine Learning in the Wild (NeurIPS 2016)
- Adversarial Learning Methods for Machine Learning and Data Mining (KDD 2019-2022)
- Privacy Preserving Machine Learning (FOCS 2022, CCS 2021, NeurIPS 2020, CCS 2019, NeurIPS 2018)
- SafeAI (AAAI 2019-2022)
- Adversarial Machine Learning and Beyond (AAAI 2022)
- Towards Robust, Secure and Efficient Machine Learning (AAAI2021)
- AISafety (IJCAI 2019-2022)
-
- The Dark Side of Generative AIs and Beyond (ECCV 2024)
- Trust What You learN (ECCV 2024)
- Privacy for Vision & Imaging (ECCV 2024)
- Adversarial Machine Learning on Computer Vision (CVPR 2024, CVPR 2023, CVPR 2022, CVPR 2020)
- Secure and Safe Autonomous Driving (CVPR 2023)
- Adversarial Robustness in the Real World (ICCV 2023, ECCV 2022, ICCV 2021, CVPR 2021, ECCV 2020, CVPR 2020, CVPR 2019)
- The Bright and Dark Sides of Computer Vision: Challenges and Opportunities for Privacy and Security (CVPR 2021, ECCV 2020, CVPR 2019, CVPR 2018, CVPR 2017)
- Responsible Computer Vision (ECCV 2022)
- Safe Artificial Intelligence for Automated Driving (ECCV 2022)
- Adversarial Learning for Multimedia (ACMMM 2021)
- Adversarial Machine Learning towards Advanced Vision Systems (ACCV 2022)
-
- Trustworthy Natural Language Processing (2021-2024)
- Privacy in Natural Language Processing (ACL 2024, NAACL 2022, NAACL 2021, EMNLP 2020, WSDM 2020)
- BlackboxNLP (2018-2024)
-
- Online Misinformation- and Harm-Aware Recommender Systems (RecSys 2021, RecSys 2020)
- Adversarial Machine Learning for Recommendation and Search (CIKM 2021)
-
- Quantitative Reasoning About Data Privacy in Machine Learning (ICML 2022)
- Foundational Robustness of Foundation Models (NeurIPS 2022)
- Adversarial Robustness - Theory and Practice (NeurIPS 2018)
- Towards Adversarial Learning: from Evasion Attacks to Poisoning Attacks (KDD 2022)
- Adversarial Robustness in Deep Learning: From Practices to Theories (KDD 2021)
- Adversarial Attacks and Defenses: Frontiers, Advances and Practice (KDD 2020)
- Adversarial Robustness of Deep Learning: Theory, Algorithms, and Applications (ICDM 2020)
- Adversarial Machine Learning for Good (AAAI 2022)
- Adversarial Machine Learning (AAAI 2018)
-
- Adversarial Machine Learning in Computer Vision (CVPR 2021)
- Practical Adversarial Robustness in Deep Learning: Problems and Solutions (CVPR 2021)
- Adversarial Robustness of Deep Learning Models (ECCV 2020)
- Deep Learning for Privacy in Multimedia (ACMMM 2020)
-
- Vulnerabilities of Large Language Models to Adversarial Attacks (ACL 2024)
- Robustness and Adversarial Examples in Natural Language Processing (EMNLP 2021)
- Deep Adversarial Learning for NLP (NAACL 2019)
-
- Adversarial Machine Learning in Recommender Systems (ECIR 2021, RecSys 2020, WSDM 2020)
- Special Track on Safe and Robust AI (AAAI 2023)
- Special Session on Adversarial Learning for Multimedia Understanding and Retrieval (ICMR 2022)
- Special Session on Adversarial Attack and Defense (APSIPA 2022)
- Special Session on Information Security meets Adversarial Examples (WIFS 2019)
For Tasks:
Click tags to check more tools for each tasksFor Jobs:
Alternative AI tools for AI-Security-and-Privacy-Events
Similar Open Source Tools
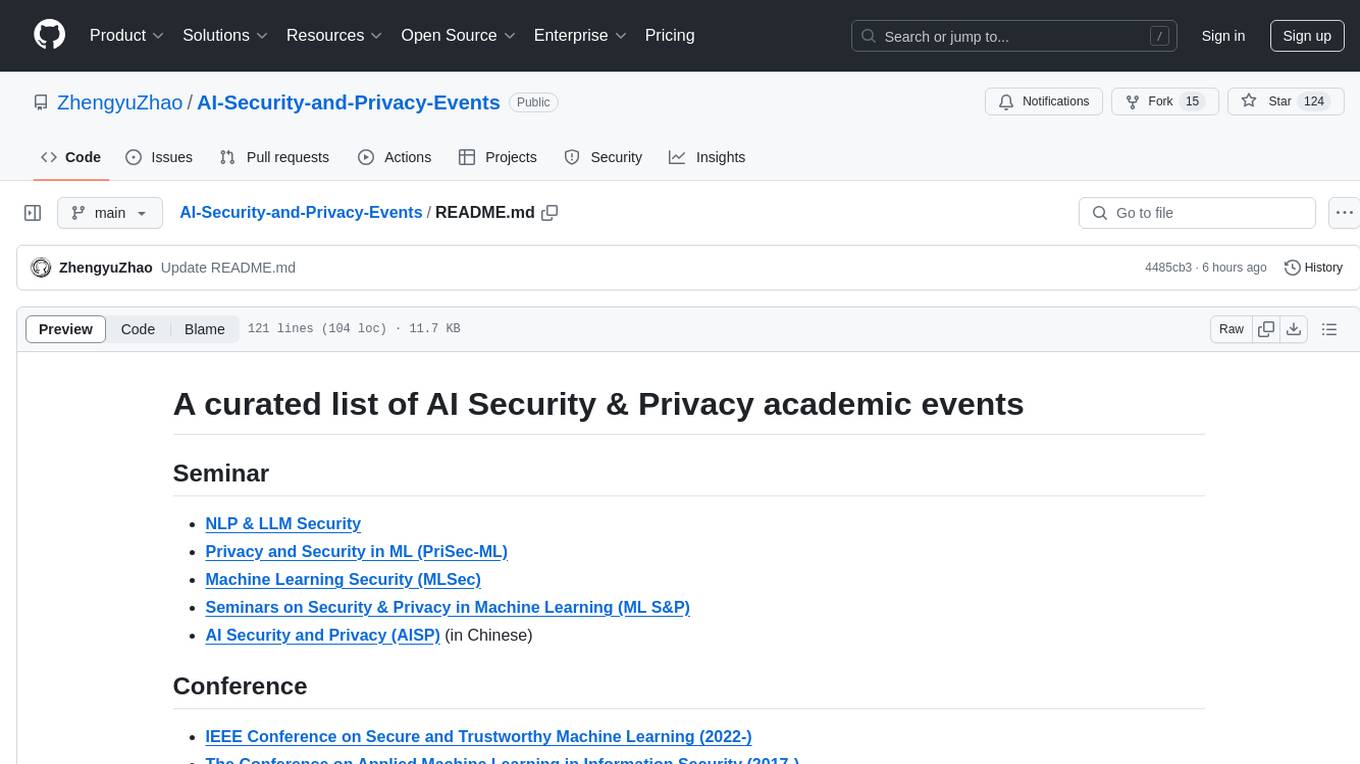
AI-Security-and-Privacy-Events
AI-Security-and-Privacy-Events is a curated list of academic events focusing on AI security and privacy. It includes seminars, conferences, workshops, tutorials, special sessions, and covers various topics such as NLP & LLM Security, Privacy and Security in ML, Machine Learning Security, AI System with Confidential Computing, Adversarial Machine Learning, and more.
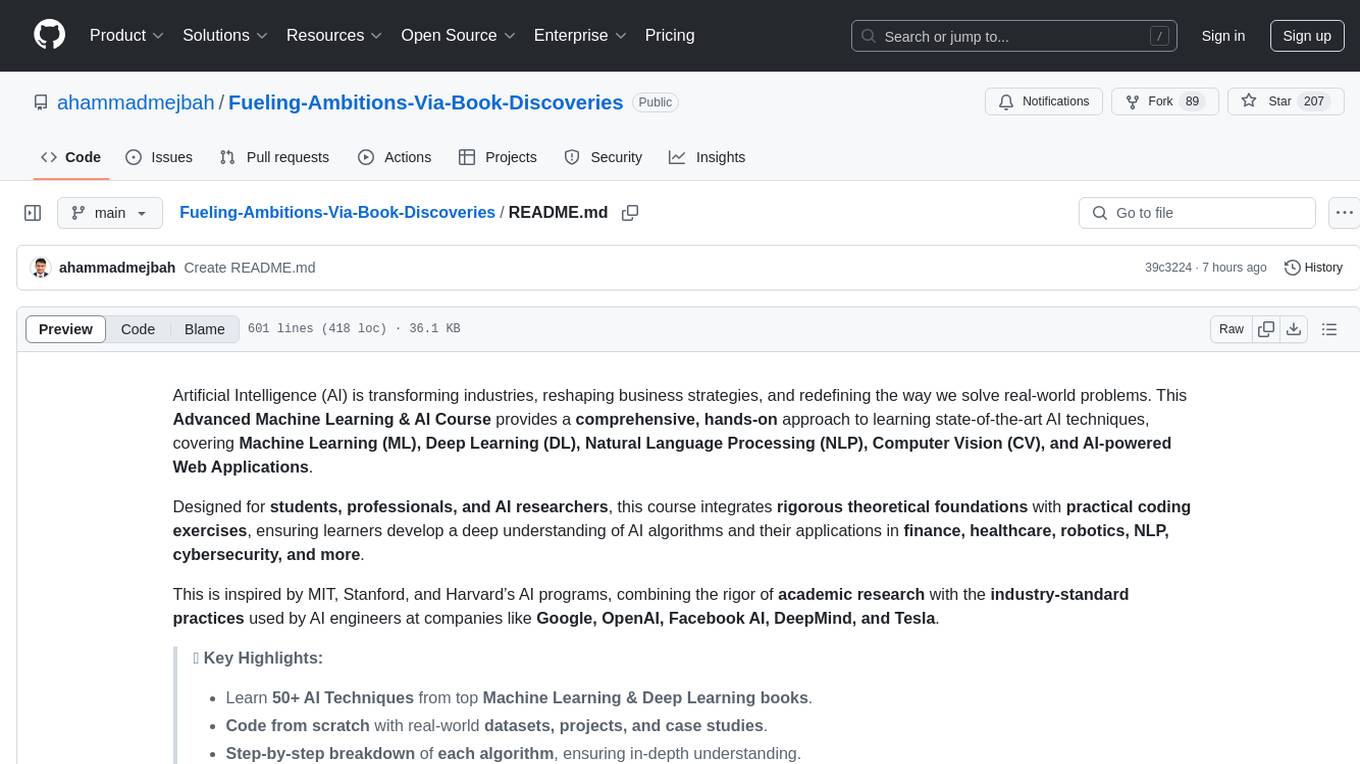
Fueling-Ambitions-Via-Book-Discoveries
Fueling-Ambitions-Via-Book-Discoveries is an Advanced Machine Learning & AI Course designed for students, professionals, and AI researchers. The course integrates rigorous theoretical foundations with practical coding exercises, ensuring learners develop a deep understanding of AI algorithms and their applications in finance, healthcare, robotics, NLP, cybersecurity, and more. Inspired by MIT, Stanford, and Harvard’s AI programs, it combines academic research rigor with industry-standard practices used by AI engineers at companies like Google, OpenAI, Facebook AI, DeepMind, and Tesla. Learners can learn 50+ AI techniques from top Machine Learning & Deep Learning books, code from scratch with real-world datasets, projects, and case studies, and focus on ML Engineering & AI Deployment using Django & Streamlit. The course also offers industry-relevant projects to build a strong AI portfolio.
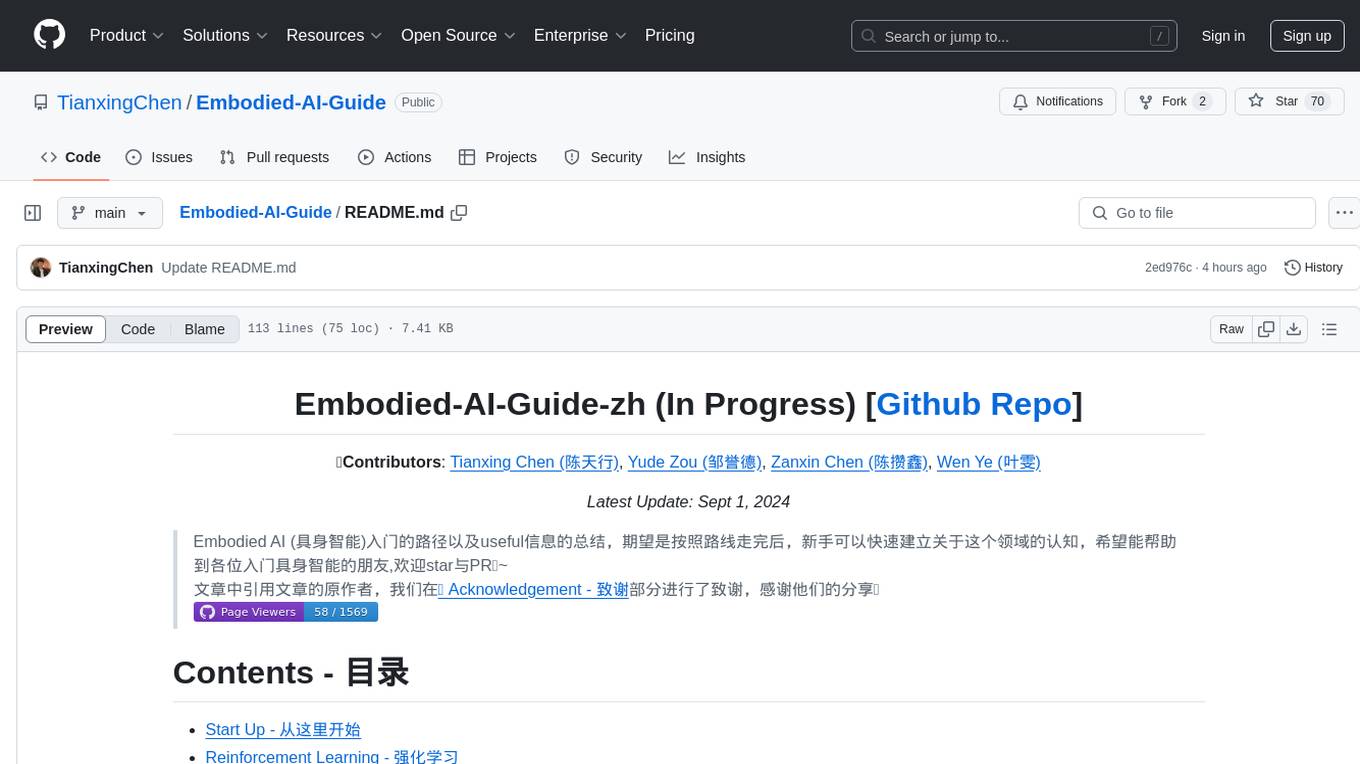
Embodied-AI-Guide
Embodied-AI-Guide is a comprehensive guide for beginners to understand Embodied AI, focusing on the path of entry and useful information in the field. It covers topics such as Reinforcement Learning, Imitation Learning, Large Language Model for Robotics, 3D Vision, Control, Benchmarks, and provides resources for building cognitive understanding. The repository aims to help newcomers quickly establish knowledge in the field of Embodied AI.
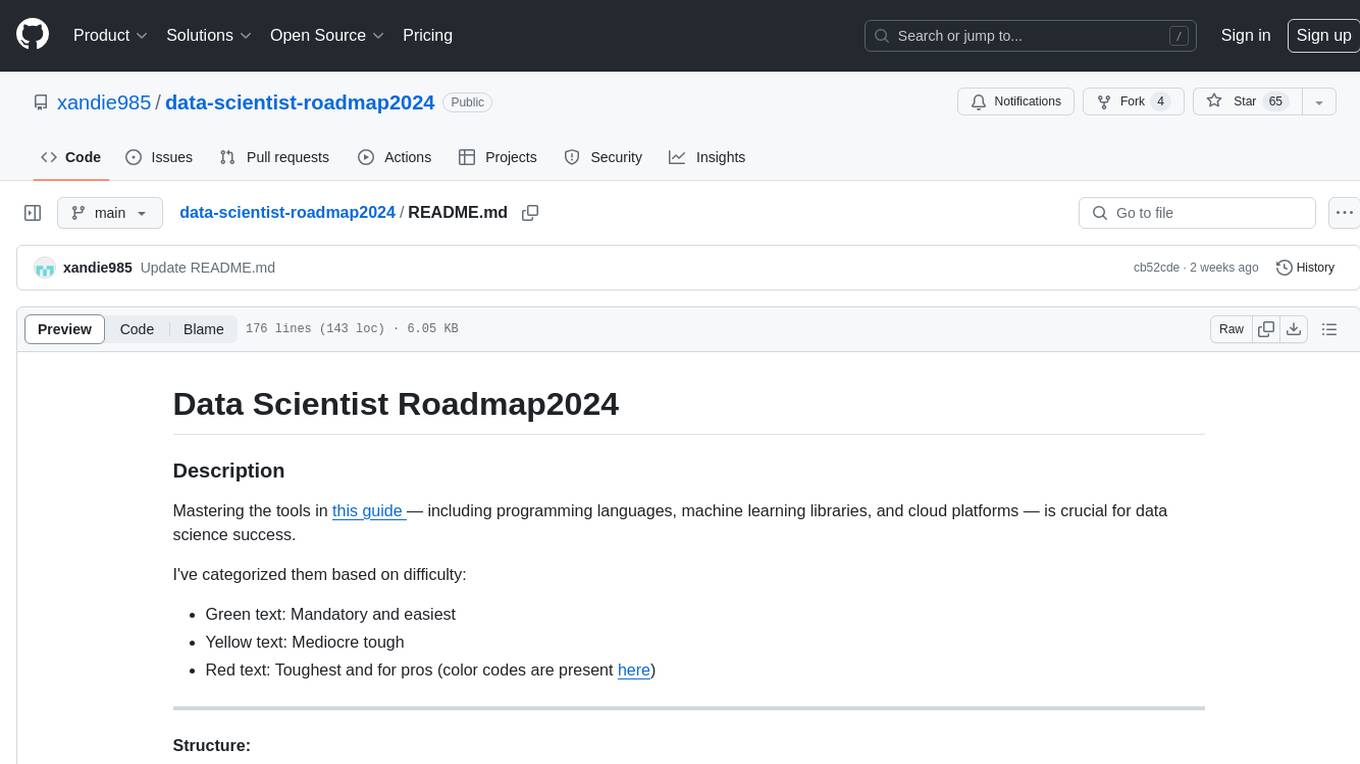
data-scientist-roadmap2024
The Data Scientist Roadmap2024 provides a comprehensive guide to mastering essential tools for data science success. It includes programming languages, machine learning libraries, cloud platforms, and concepts categorized by difficulty. The roadmap covers a wide range of topics from programming languages to machine learning techniques, data visualization tools, and DevOps/MLOps tools. It also includes web development frameworks and specific concepts like supervised and unsupervised learning, NLP, deep learning, reinforcement learning, and statistics. Additionally, it delves into DevOps tools like Airflow and MLFlow, data visualization tools like Tableau and Matplotlib, and other topics such as ETL processes, optimization algorithms, and financial modeling.
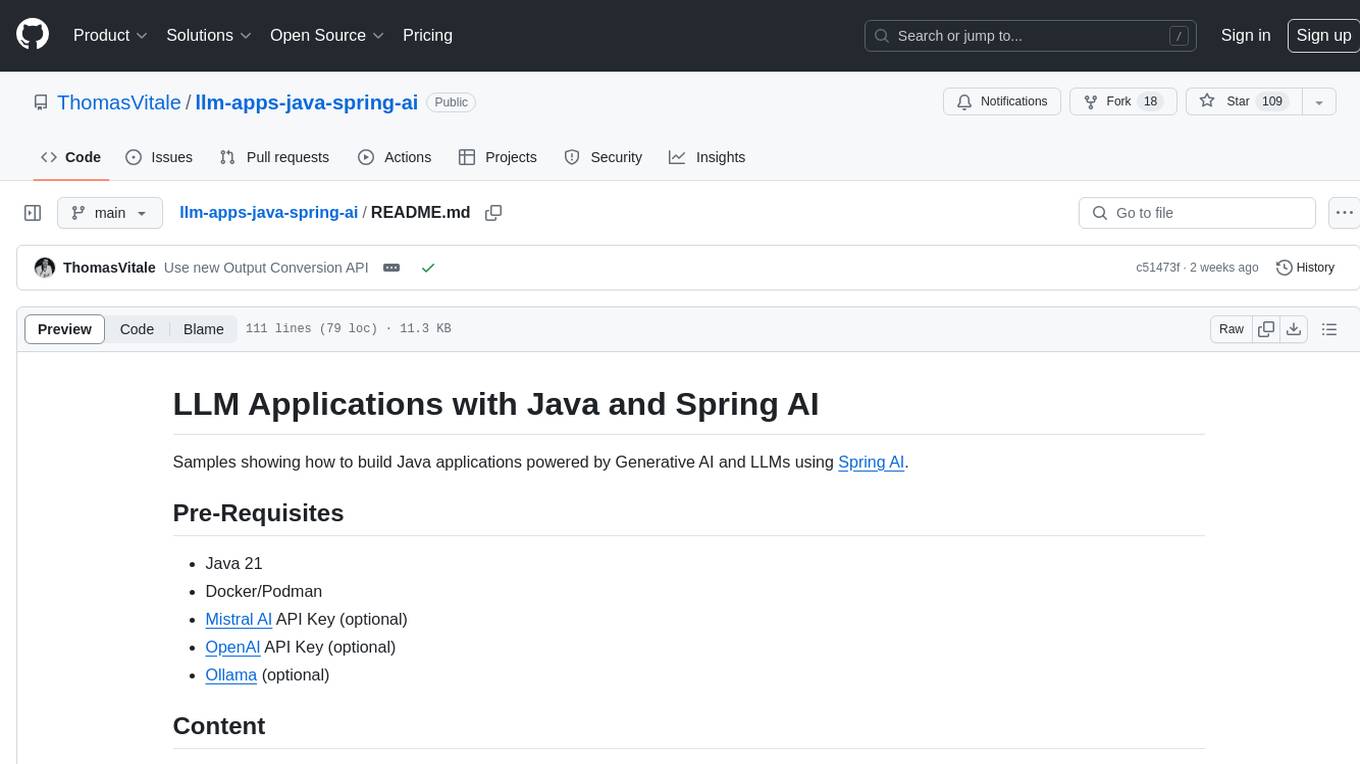
llm-apps-java-spring-ai
The 'LLM Applications with Java and Spring AI' repository provides samples demonstrating how to build Java applications powered by Generative AI and Large Language Models (LLMs) using Spring AI. It includes projects for question answering, chat completion models, prompts, templates, multimodality, output converters, embedding models, document ETL pipeline, function calling, image models, and audio models. The repository also lists prerequisites such as Java 21, Docker/Podman, Mistral AI API Key, OpenAI API Key, and Ollama. Users can explore various use cases and projects to leverage LLMs for text generation, vector transformation, document processing, and more.
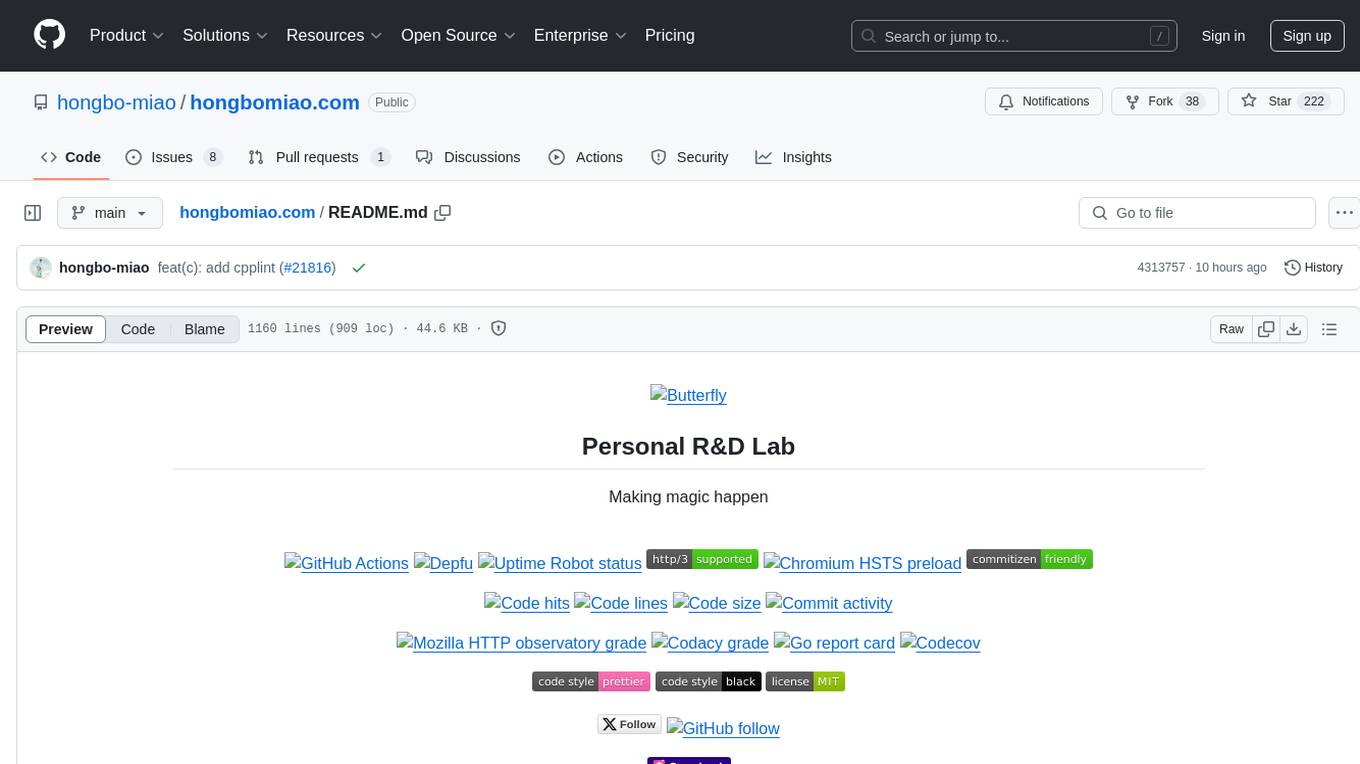
hongbomiao.com
hongbomiao.com is a personal research and development (R&D) lab that facilitates the sharing of knowledge. The repository covers a wide range of topics including web development, mobile development, desktop applications, API servers, cloud native technologies, data processing, machine learning, computer vision, embedded systems, simulation, database management, data cleaning, data orchestration, testing, ops, authentication, authorization, security, system tools, reverse engineering, Ethereum, hardware, network, guidelines, design, bots, and more. It provides detailed information on various tools, frameworks, libraries, and platforms used in these domains.
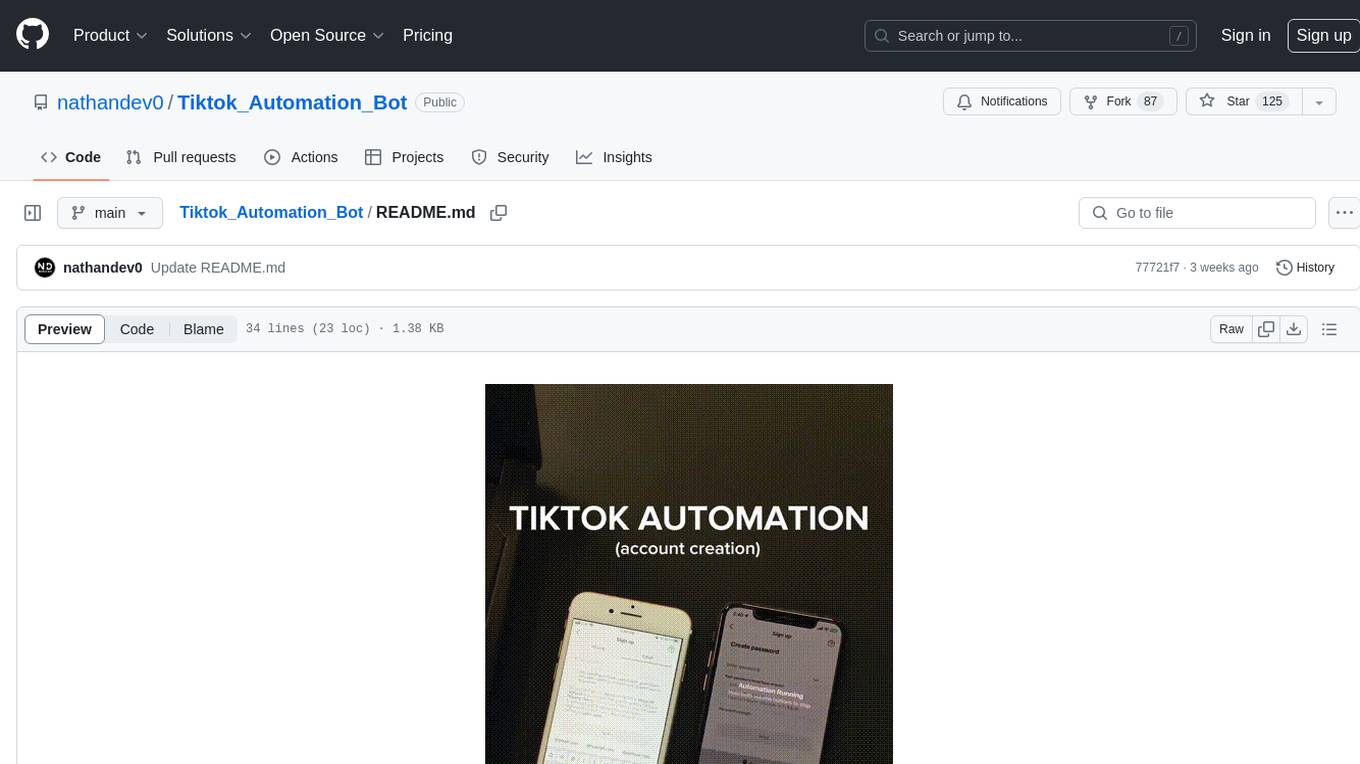
Tiktok_Automation_Bot
TikTok Automation Bot is an Appium-based tool for automating TikTok account creation and video posting on real devices. It offers functionalities such as automated account creation and video posting, along with integrations like Crane tweak, SMSActivate service, and IPQualityScore service. The tool also provides device and automation management system, anti-bot system for human behavior modeling, and IP rotation system for different IP addresses. It is designed to simplify the process of managing TikTok accounts and posting videos efficiently.
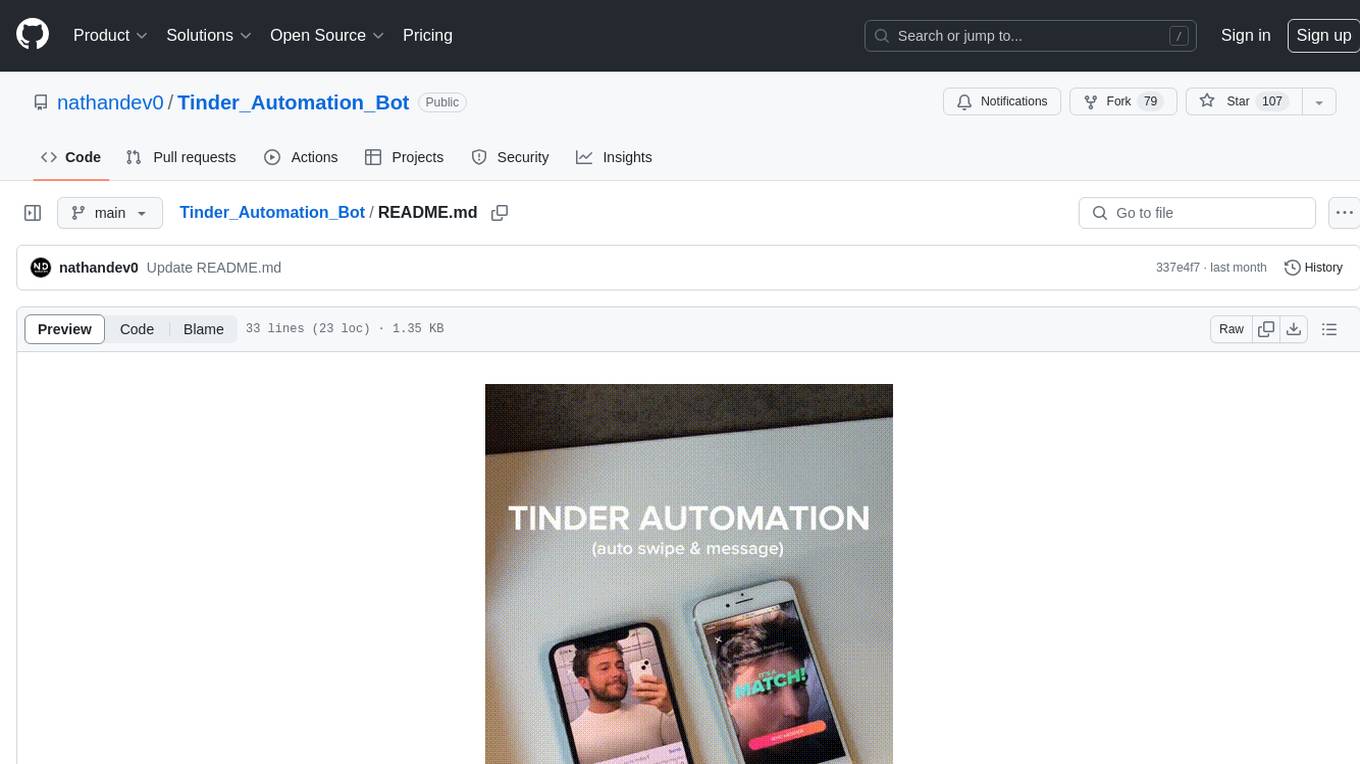
Tinder_Automation_Bot
Tinder Automation Bot is an Appium-based tool designed for automated Tinder account creation and swiping on real devices. It offers functionalities such as automated account creation and swiping, along with integrations like Crane tweak and SMSPool service. The tool also provides features like device and automation management system, anti-bot system for human behavior modeling, IP rotation system for different IP addresses, and GPS location spoofing for different GPS coordinates. It is part of a series of automation bots including TikTok, Bumble, and Badoo automation bots.
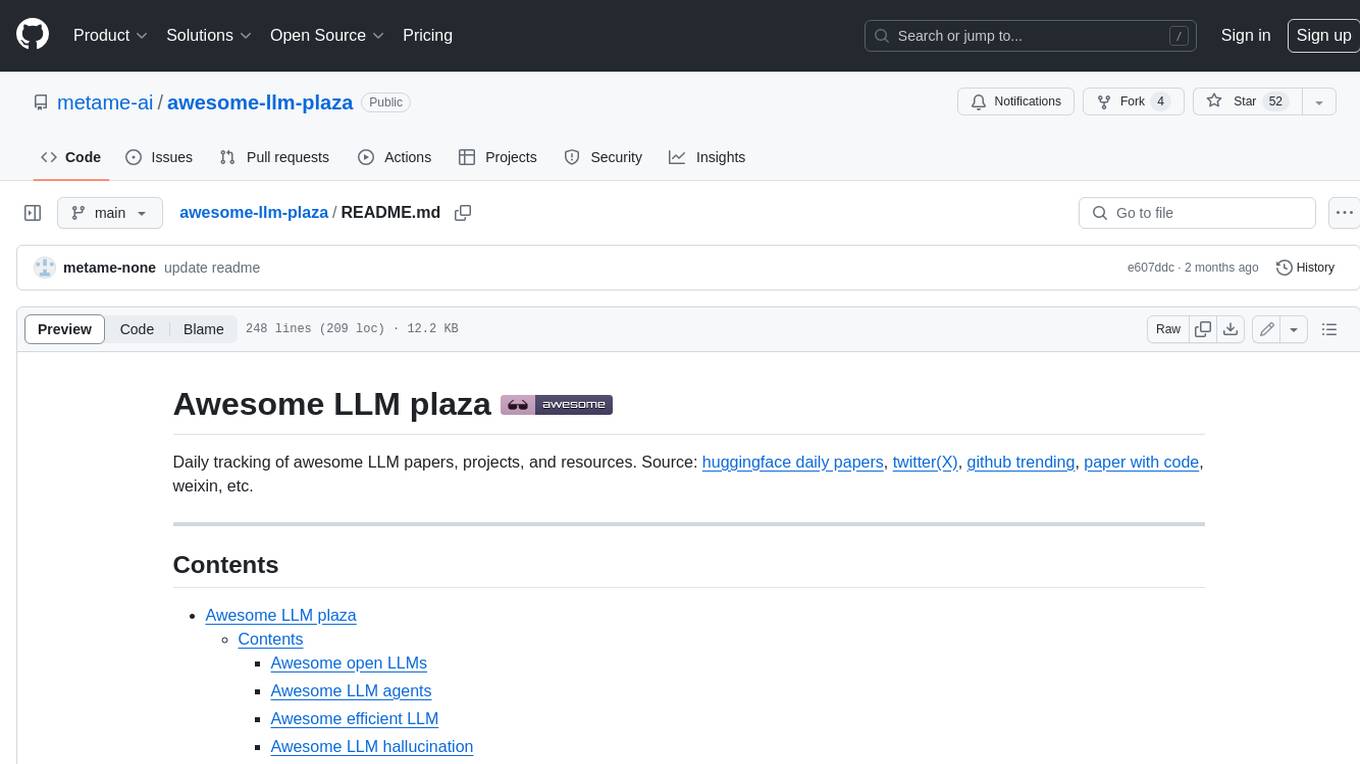
awesome-llm-plaza
Awesome LLM plaza is a curated list of awesome LLM papers, projects, and resources. It is updated daily and includes resources from a variety of sources, including huggingface daily papers, twitter, github trending, paper with code, weixin, etc.
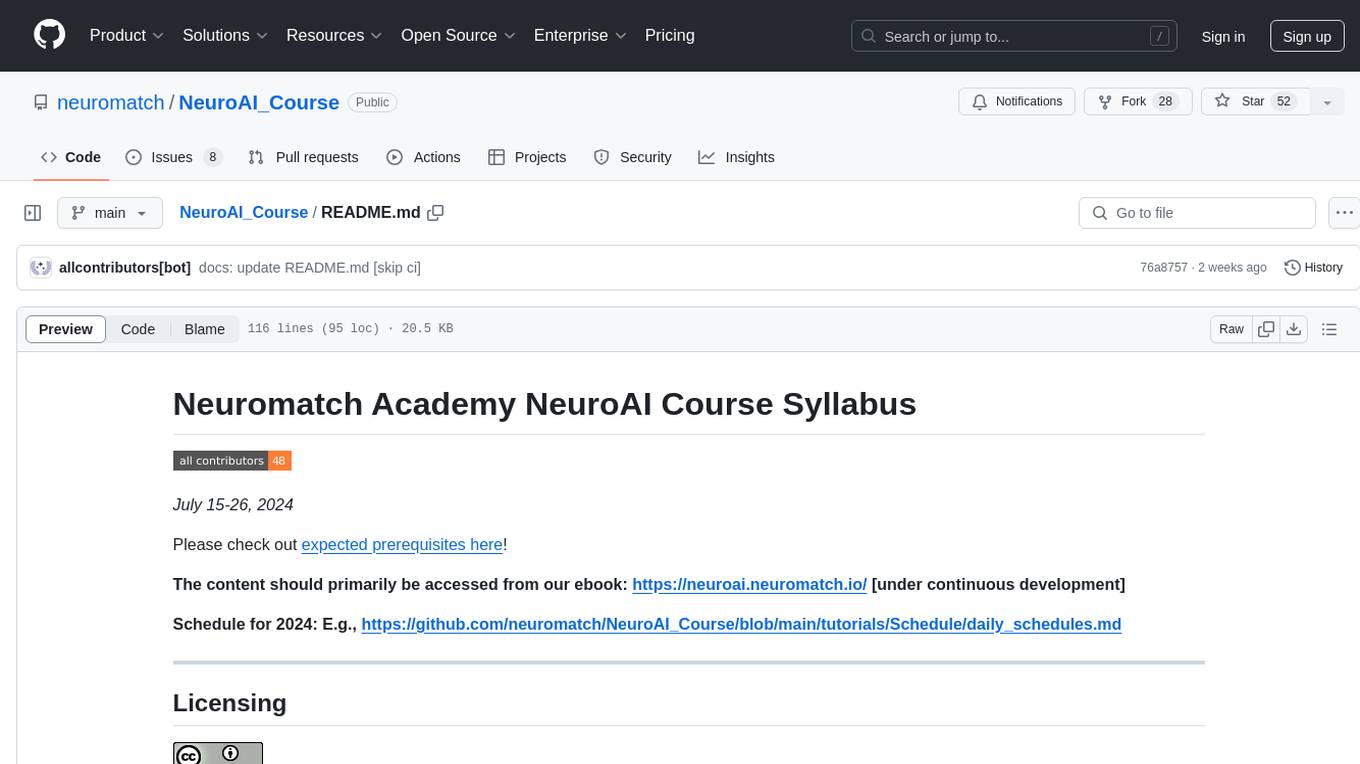
NeuroAI_Course
Neuromatch Academy NeuroAI Course Syllabus is a repository that contains the schedule and licensing information for the NeuroAI course. The course is designed to provide participants with a comprehensive understanding of artificial intelligence in neuroscience. It covers various topics related to AI applications in neuroscience, including machine learning, data analysis, and computational modeling. The content is primarily accessed from the ebook provided in the repository, and the course is scheduled for July 15-26, 2024. The repository is shared under a Creative Commons Attribution 4.0 International License and software elements are additionally licensed under the BSD (3-Clause) License. Contributors to the project are acknowledged and welcomed to contribute further.
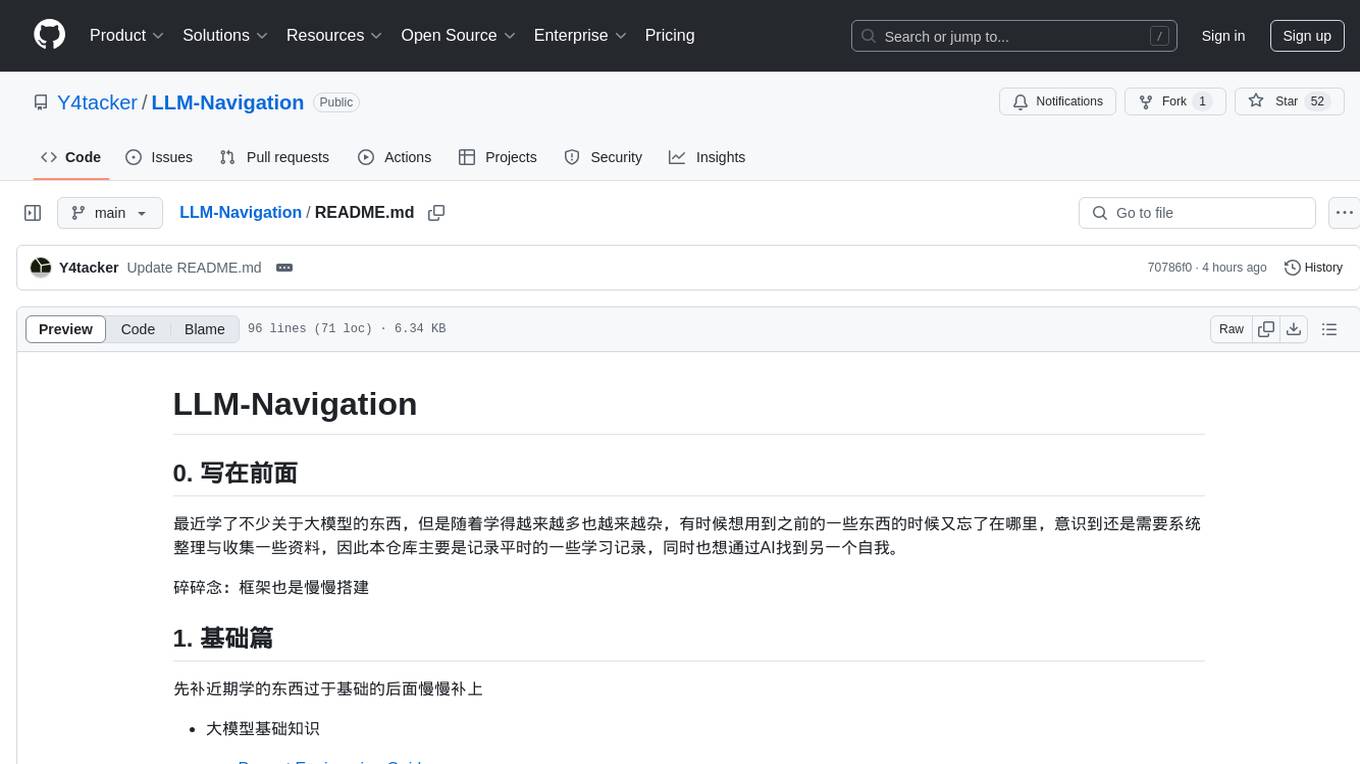
LLM-Navigation
LLM-Navigation is a repository dedicated to documenting learning records related to large models, including basic knowledge, prompt engineering, building effective agents, model expansion capabilities, security measures against prompt injection, and applications in various fields such as AI agent control, browser automation, financial analysis, 3D modeling, and tool navigation using MCP servers. The repository aims to organize and collect information for personal learning and self-improvement through AI exploration.
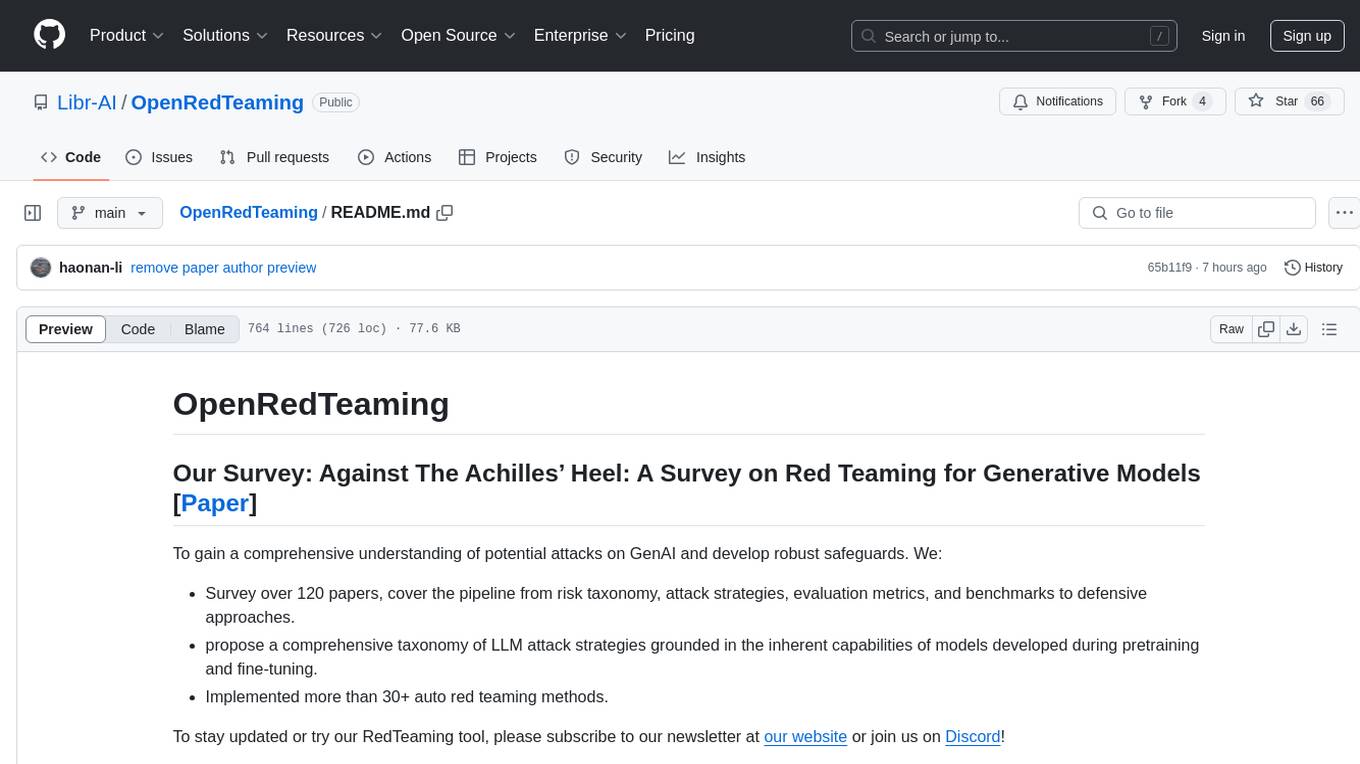
OpenRedTeaming
OpenRedTeaming is a repository focused on red teaming for generative models, specifically large language models (LLMs). The repository provides a comprehensive survey on potential attacks on GenAI and robust safeguards. It covers attack strategies, evaluation metrics, benchmarks, and defensive approaches. The repository also implements over 30 auto red teaming methods. It includes surveys, taxonomies, attack strategies, and risks related to LLMs. The goal is to understand vulnerabilities and develop defenses against adversarial attacks on large language models.
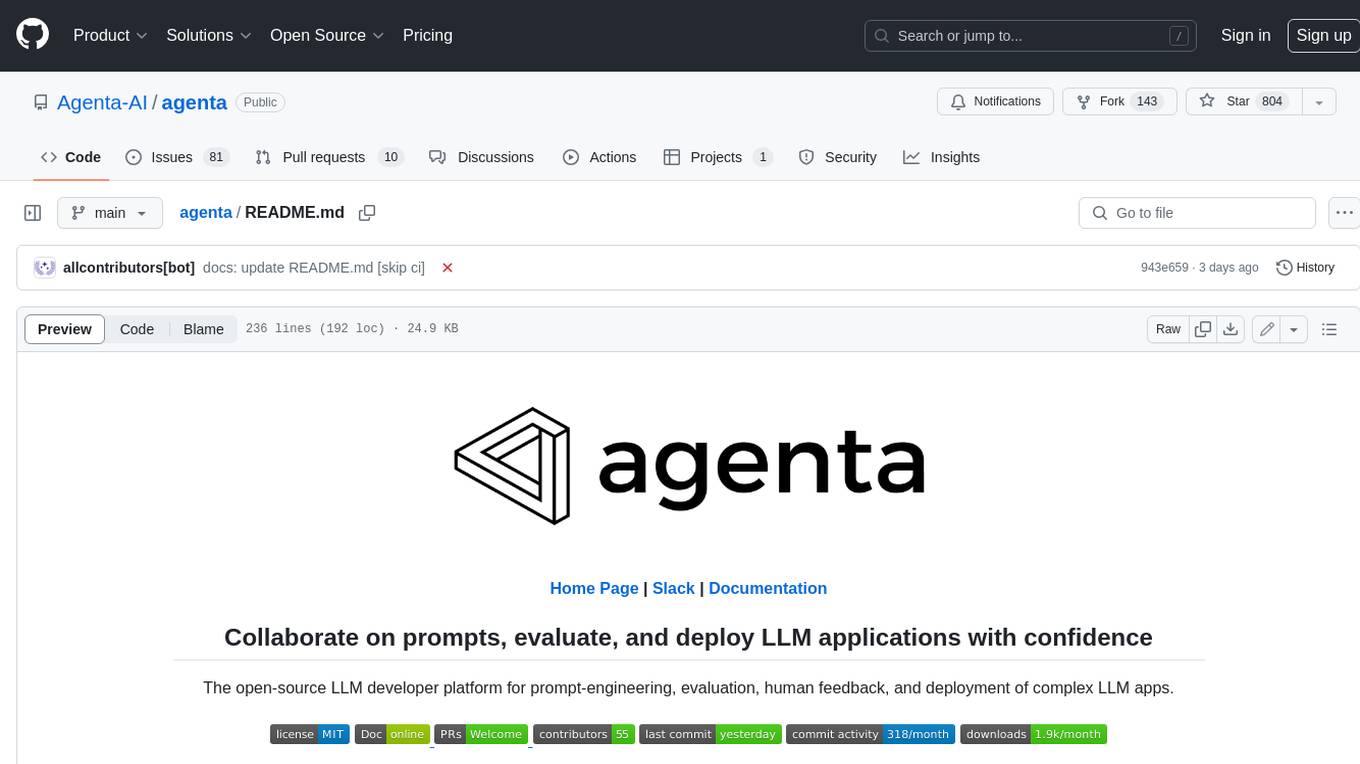
agenta
Agenta is an open-source LLM developer platform for prompt engineering, evaluation, human feedback, and deployment of complex LLM applications. It provides tools for prompt engineering and management, evaluation, human annotation, and deployment, all without imposing any restrictions on your choice of framework, library, or model. Agenta allows developers and product teams to collaborate in building production-grade LLM-powered applications in less time.
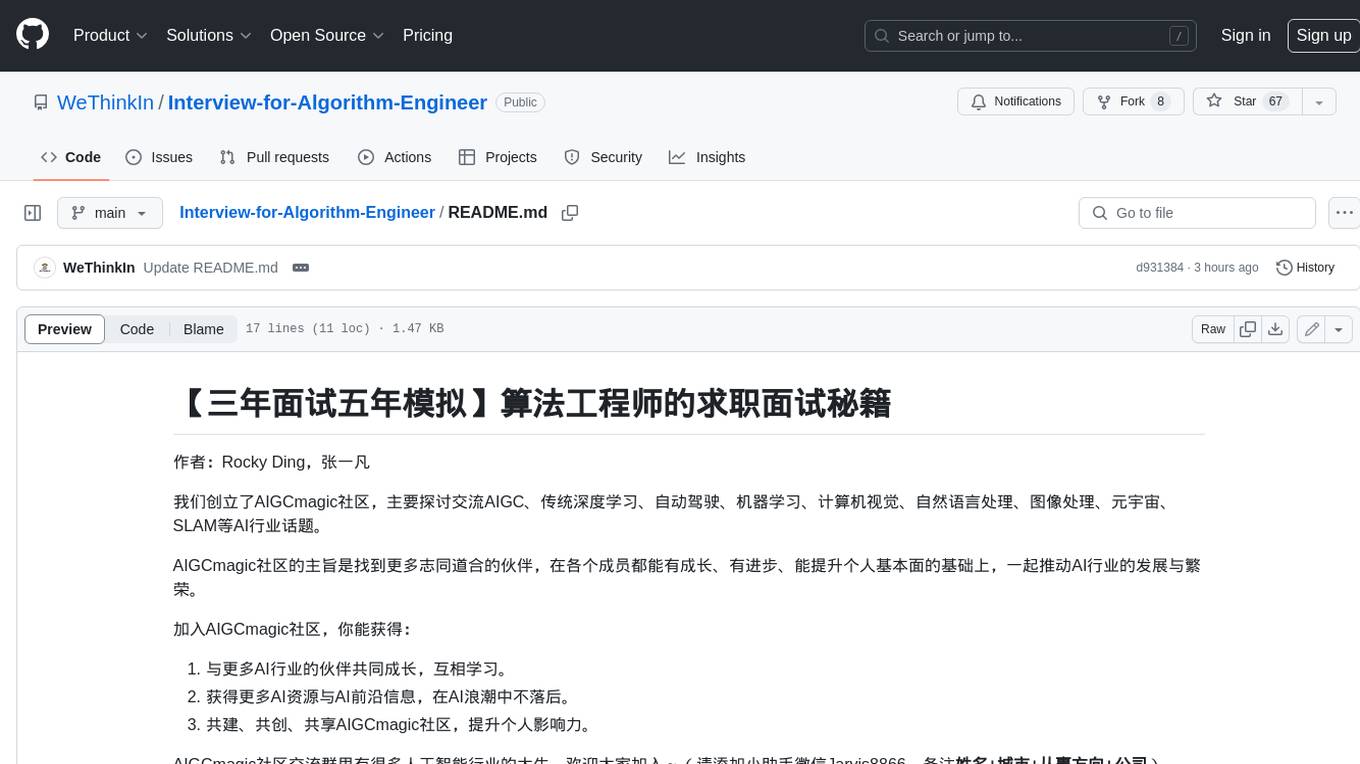
Interview-for-Algorithm-Engineer
This repository provides a collection of interview questions and answers for algorithm engineers. The questions are organized by topic, and each question includes a detailed explanation of the answer. This repository is a valuable resource for anyone preparing for an algorithm engineering interview.
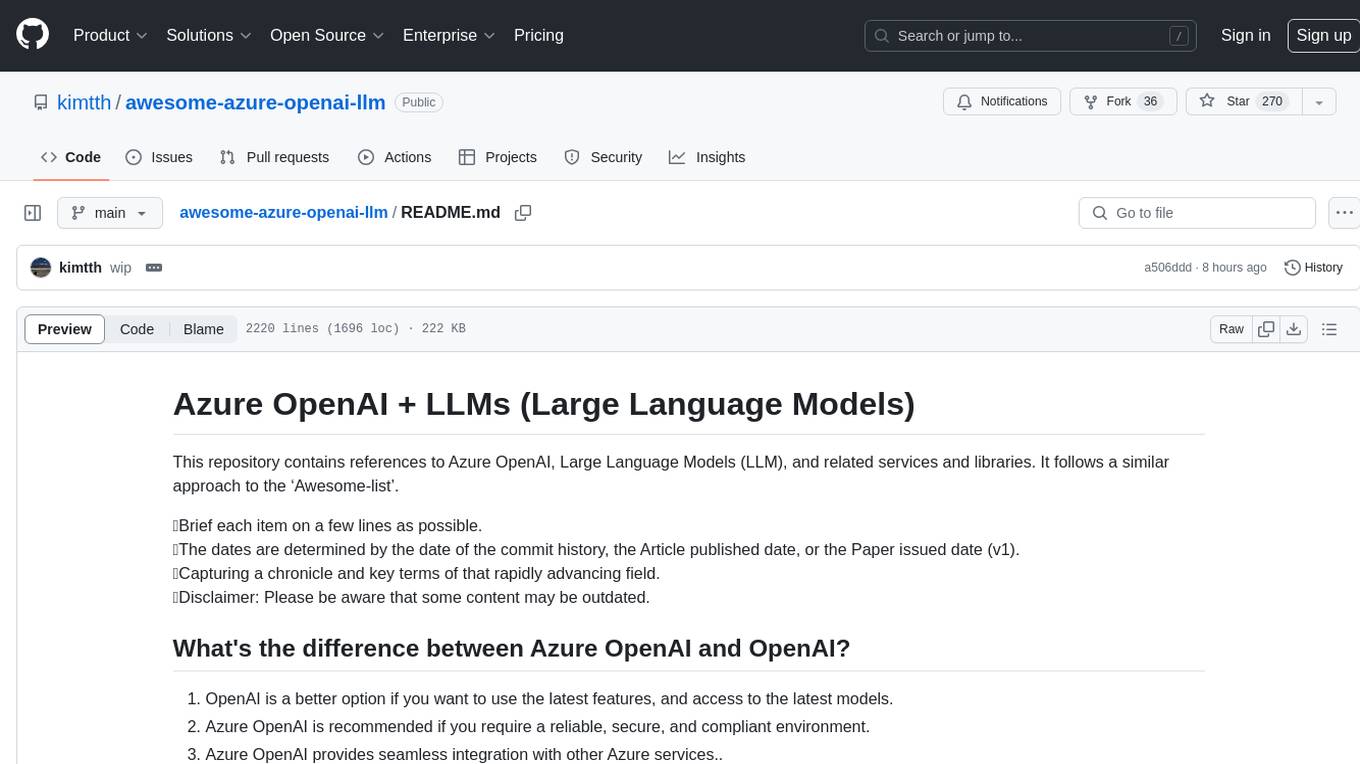
awesome-azure-openai-llm
This repository is a collection of references to Azure OpenAI, Large Language Models (LLM), and related services and libraries. It provides information on various topics such as RAG, Azure OpenAI, LLM applications, agent design patterns, semantic kernel, prompting, finetuning, challenges & abilities, LLM landscape, surveys & references, AI tools & extensions, datasets, and evaluations. The content covers a wide range of topics related to AI, machine learning, and natural language processing, offering insights into the latest advancements in the field.
For similar tasks
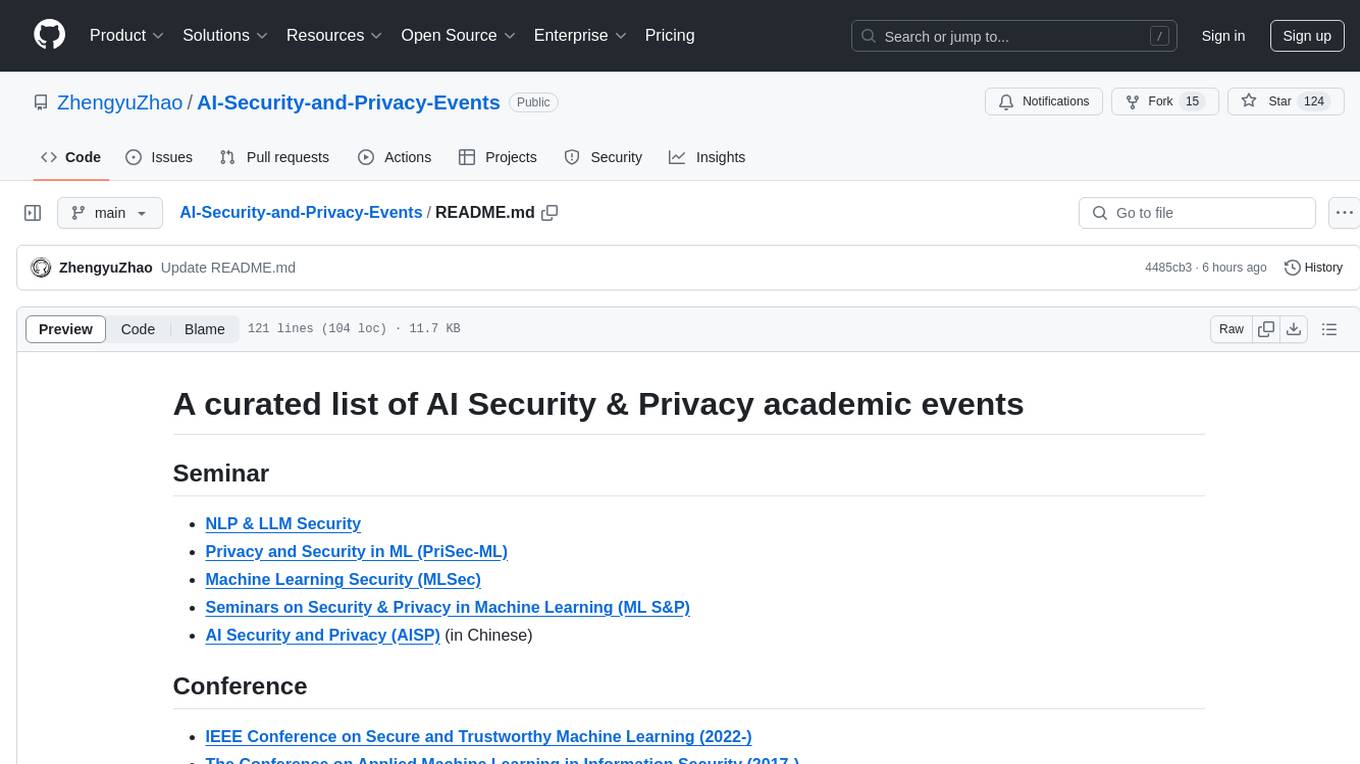
AI-Security-and-Privacy-Events
AI-Security-and-Privacy-Events is a curated list of academic events focusing on AI security and privacy. It includes seminars, conferences, workshops, tutorials, special sessions, and covers various topics such as NLP & LLM Security, Privacy and Security in ML, Machine Learning Security, AI System with Confidential Computing, Adversarial Machine Learning, and more.
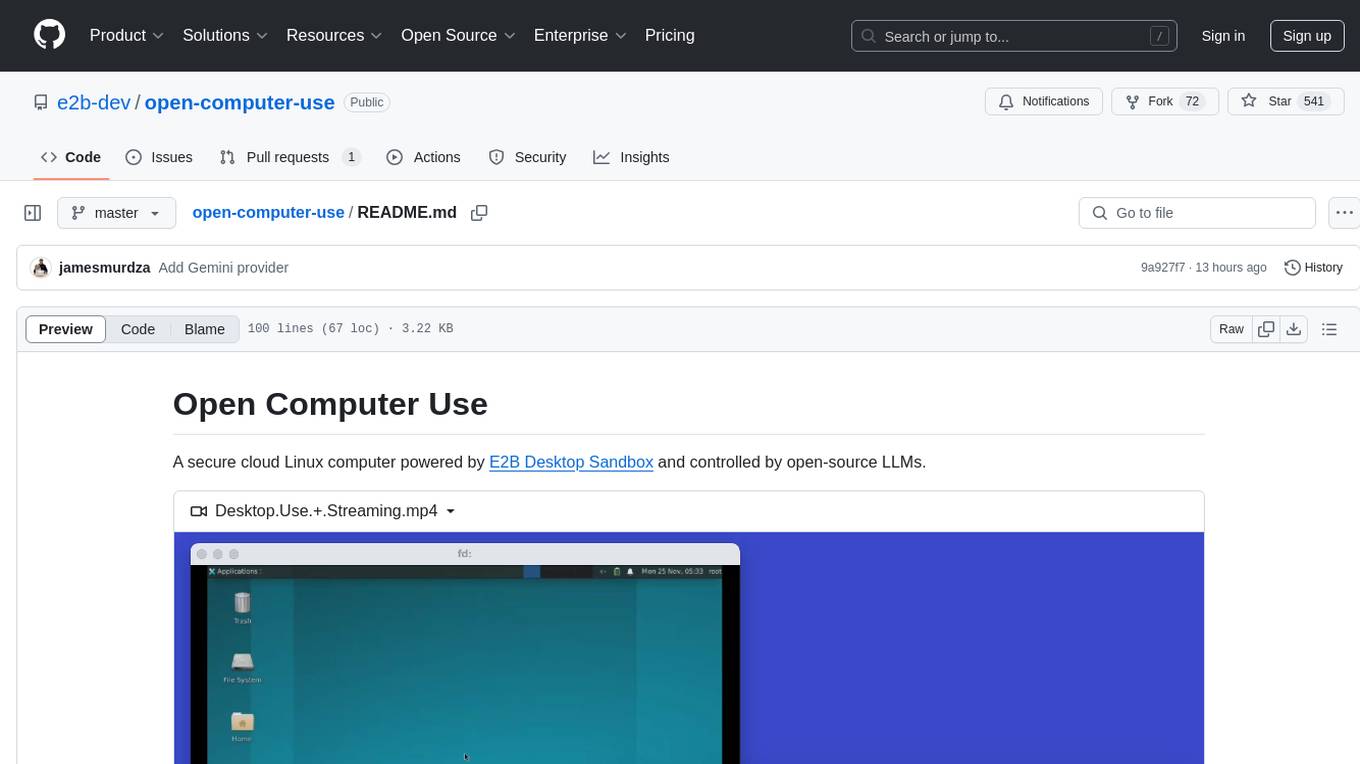
open-computer-use
Open Computer Use is a secure cloud Linux computer powered by E2B Desktop Sandbox and controlled by open-source LLMs. It allows users to operate the computer via keyboard, mouse, and shell commands, live stream the display of the sandbox on the client computer, and pause or prompt the agent at any time. The tool is designed to work with any operating system and supports integration with various LLMs and providers following the OpenAI API specification.
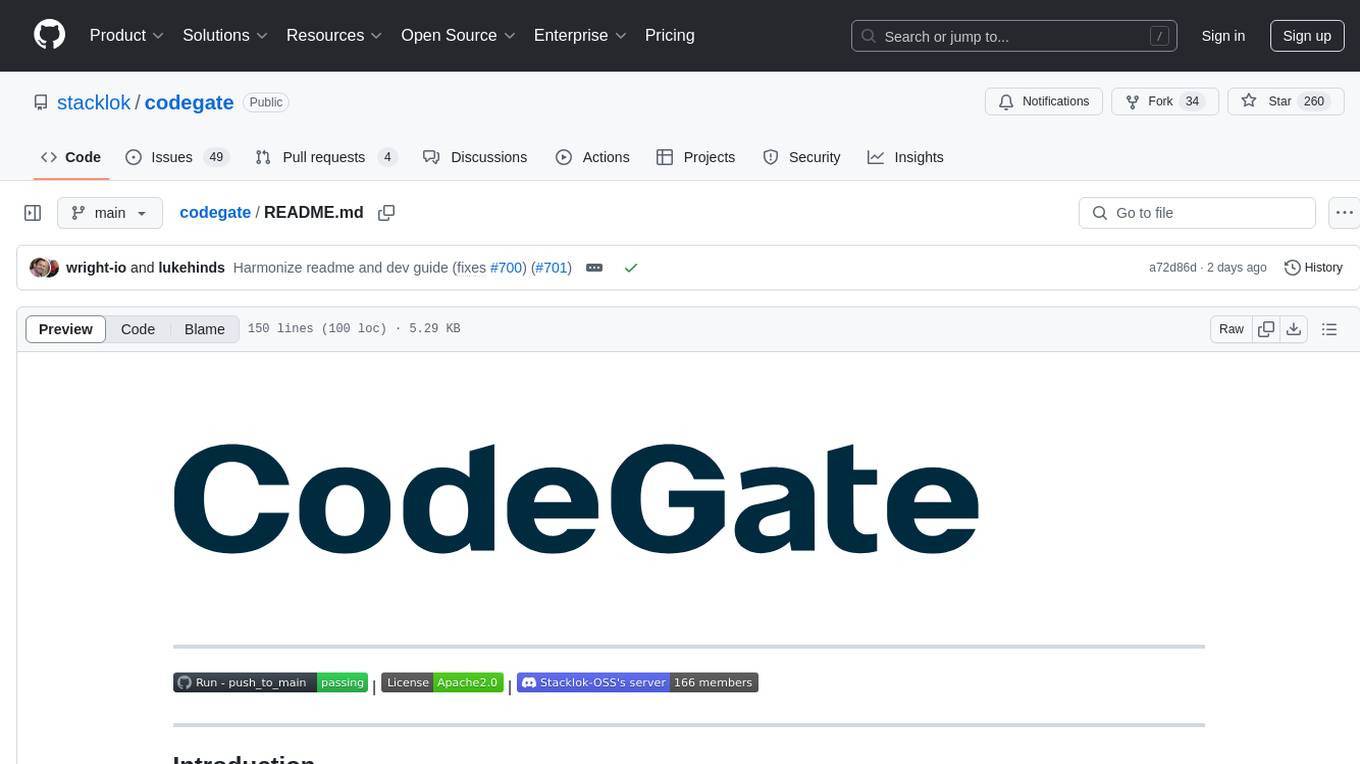
codegate
CodeGate is a local gateway that enhances the safety of AI coding assistants by ensuring AI-generated recommendations adhere to best practices, safeguarding code integrity, and protecting individual privacy. Developed by Stacklok, CodeGate allows users to confidently leverage AI in their development workflow without compromising security or productivity. It works seamlessly with coding assistants, providing real-time security analysis of AI suggestions. CodeGate is designed with privacy at its core, keeping all data on the user's machine and offering complete control over data.
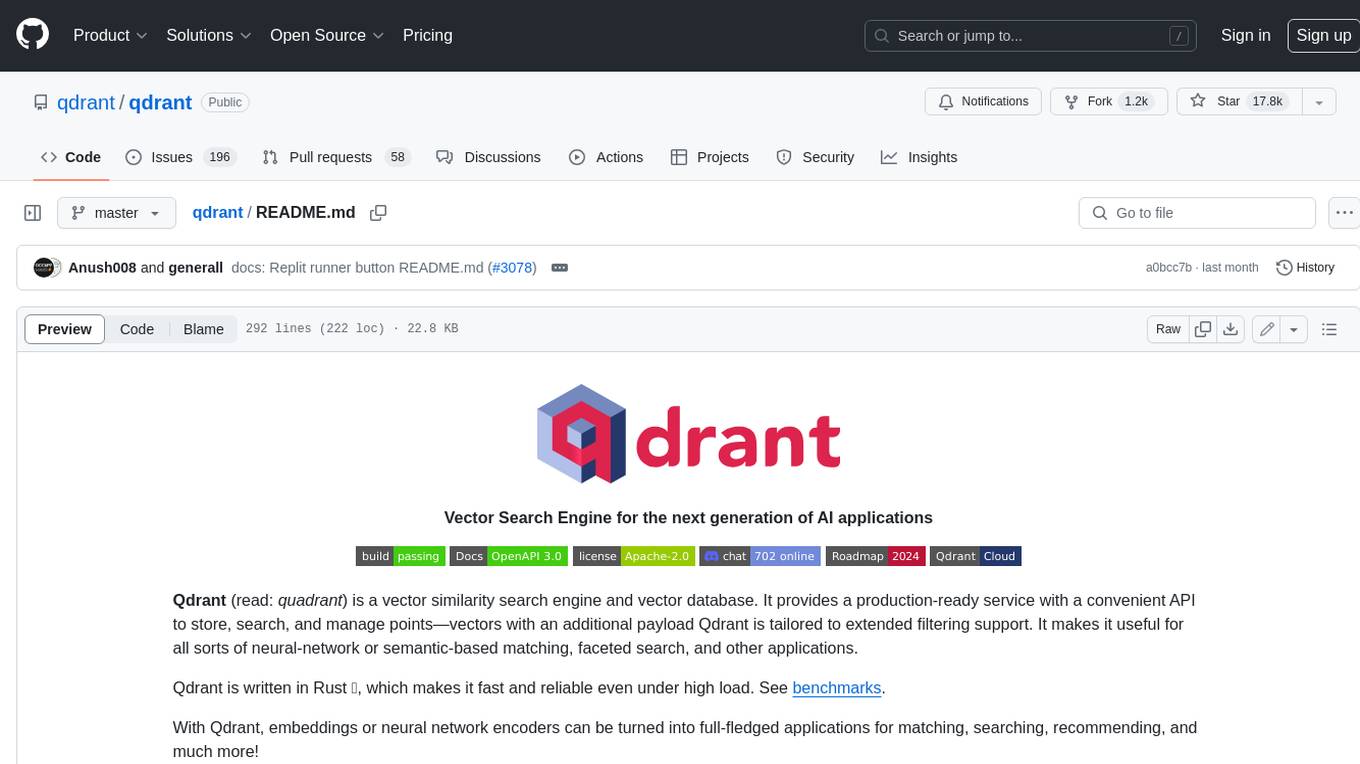
qdrant
Qdrant is a vector similarity search engine and vector database. It is written in Rust, which makes it fast and reliable even under high load. Qdrant can be used for a variety of applications, including: * Semantic search * Image search * Product recommendations * Chatbots * Anomaly detection Qdrant offers a variety of features, including: * Payload storage and filtering * Hybrid search with sparse vectors * Vector quantization and on-disk storage * Distributed deployment * Highlighted features such as query planning, payload indexes, SIMD hardware acceleration, async I/O, and write-ahead logging Qdrant is available as a fully managed cloud service or as an open-source software that can be deployed on-premises.
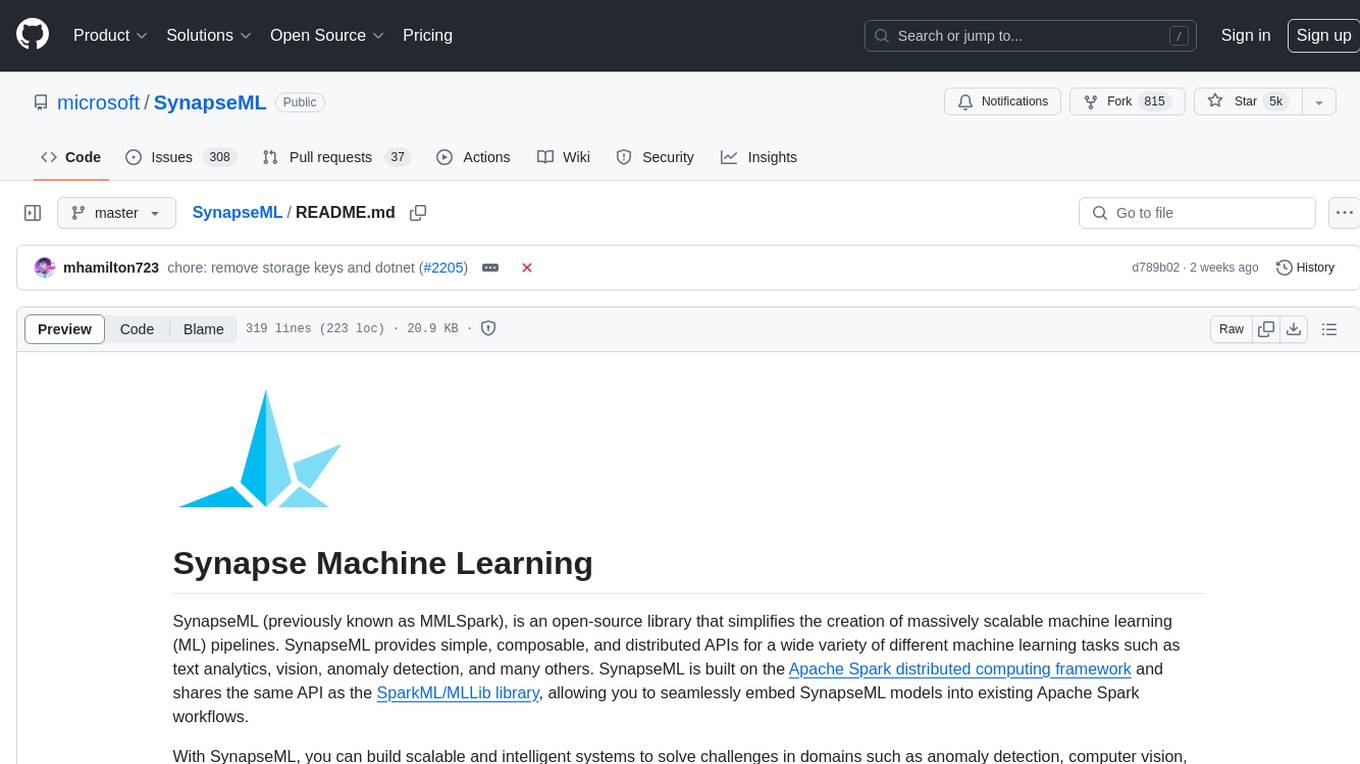
SynapseML
SynapseML (previously known as MMLSpark) is an open-source library that simplifies the creation of massively scalable machine learning (ML) pipelines. It provides simple, composable, and distributed APIs for various machine learning tasks such as text analytics, vision, anomaly detection, and more. Built on Apache Spark, SynapseML allows seamless integration of models into existing workflows. It supports training and evaluation on single-node, multi-node, and resizable clusters, enabling scalability without resource wastage. Compatible with Python, R, Scala, Java, and .NET, SynapseML abstracts over different data sources for easy experimentation. Requires Scala 2.12, Spark 3.4+, and Python 3.8+.
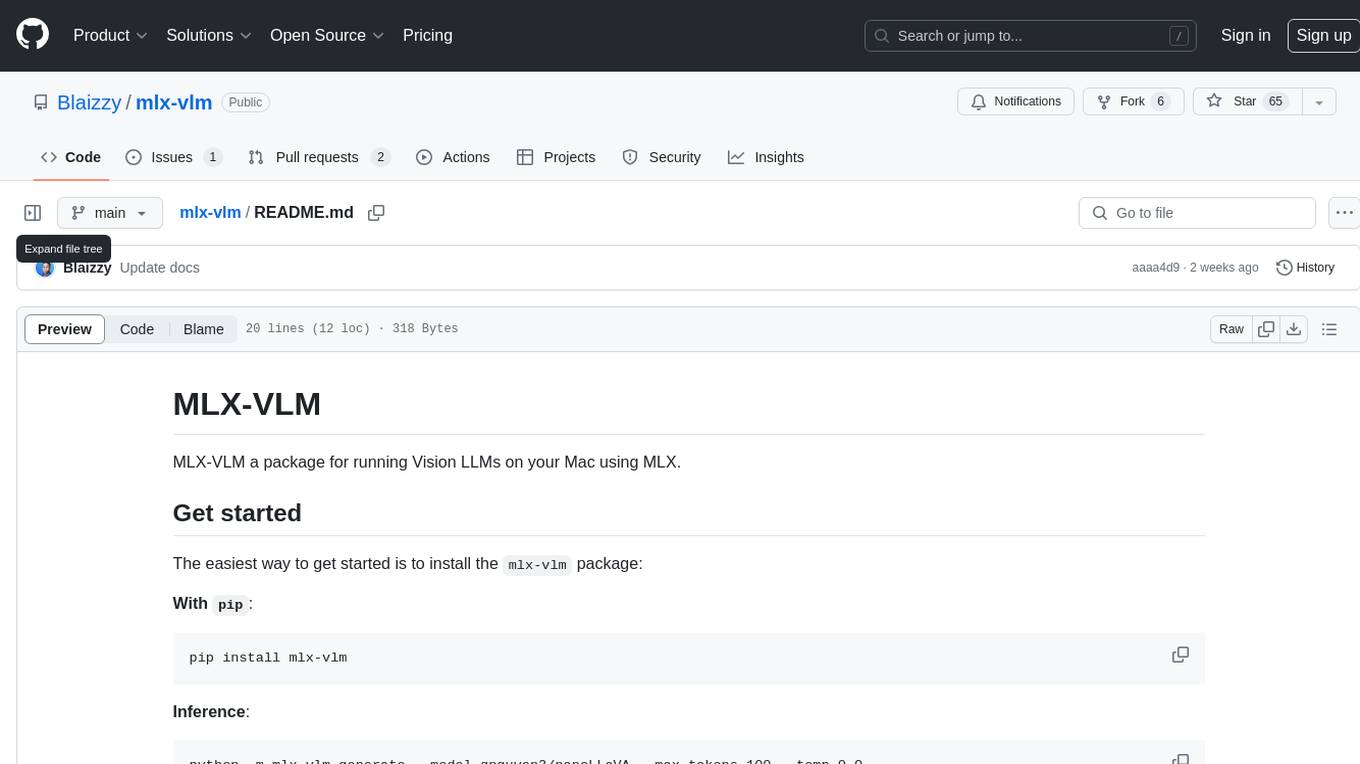
mlx-vlm
MLX-VLM is a package designed for running Vision LLMs on Mac systems using MLX. It provides a convenient way to install and utilize the package for processing large language models related to vision tasks. The tool simplifies the process of running LLMs on Mac computers, offering a seamless experience for users interested in leveraging MLX for vision-related projects.
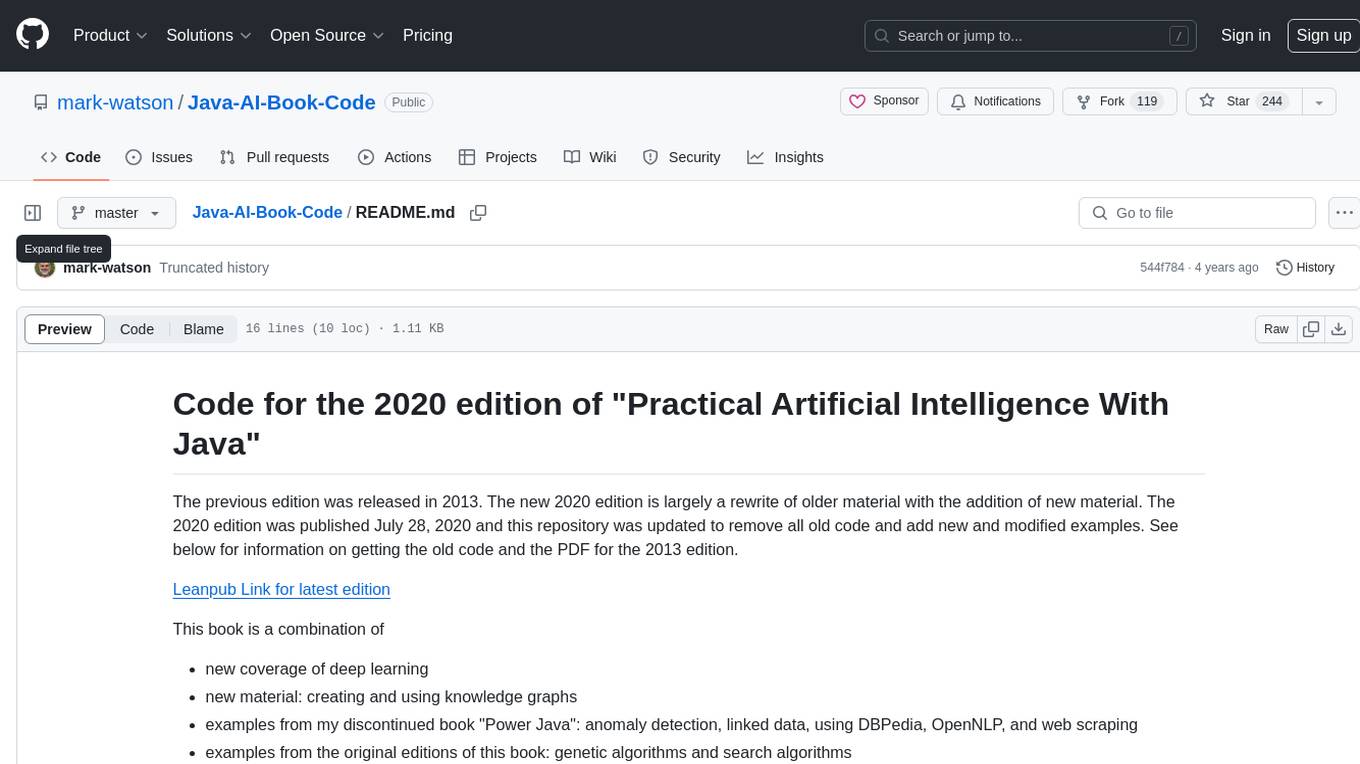
Java-AI-Book-Code
The Java-AI-Book-Code repository contains code examples for the 2020 edition of 'Practical Artificial Intelligence With Java'. It is a comprehensive update of the previous 2013 edition, featuring new content on deep learning, knowledge graphs, anomaly detection, linked data, genetic algorithms, search algorithms, and more. The repository serves as a valuable resource for Java developers interested in AI applications and provides practical implementations of various AI techniques and algorithms.
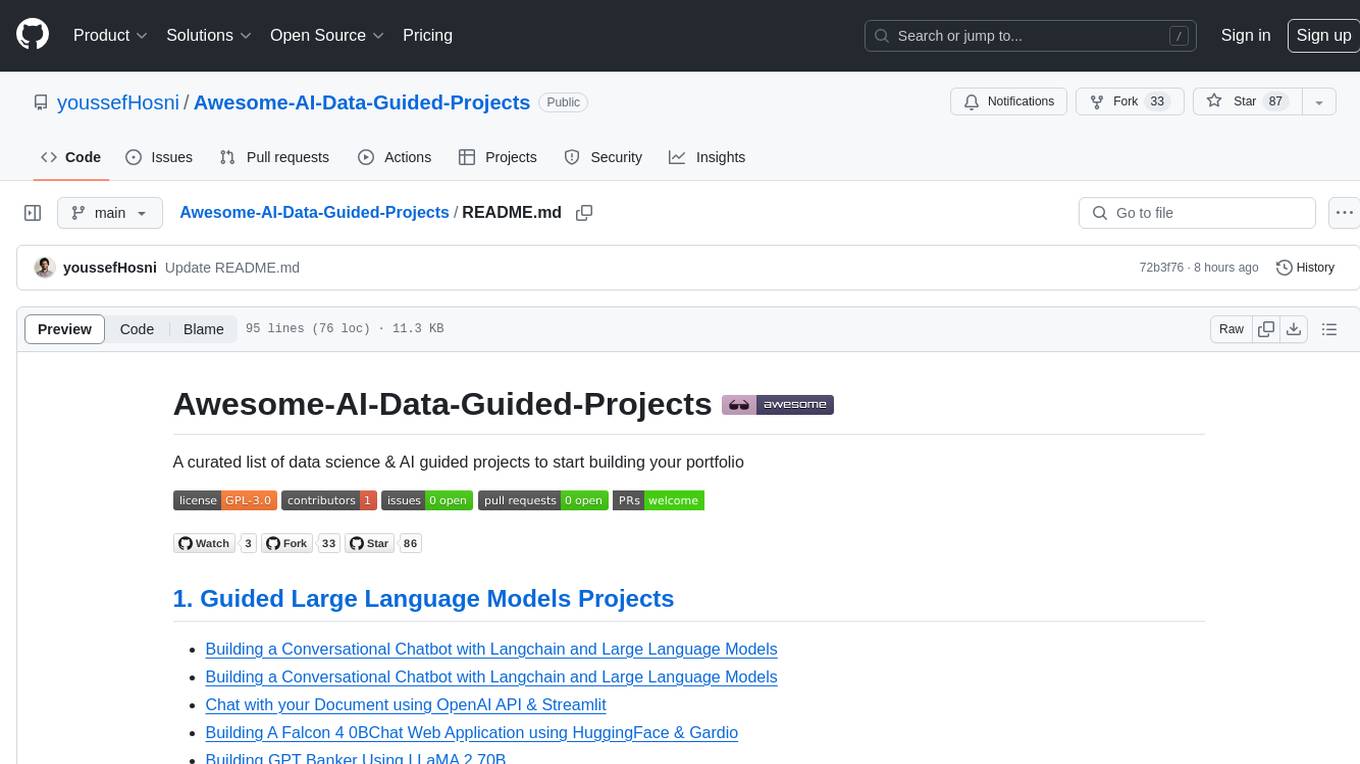
Awesome-AI-Data-Guided-Projects
A curated list of data science & AI guided projects to start building your portfolio. The repository contains guided projects covering various topics such as large language models, time series analysis, computer vision, natural language processing (NLP), and data science. Each project provides detailed instructions on how to implement specific tasks using different tools and technologies.
For similar jobs
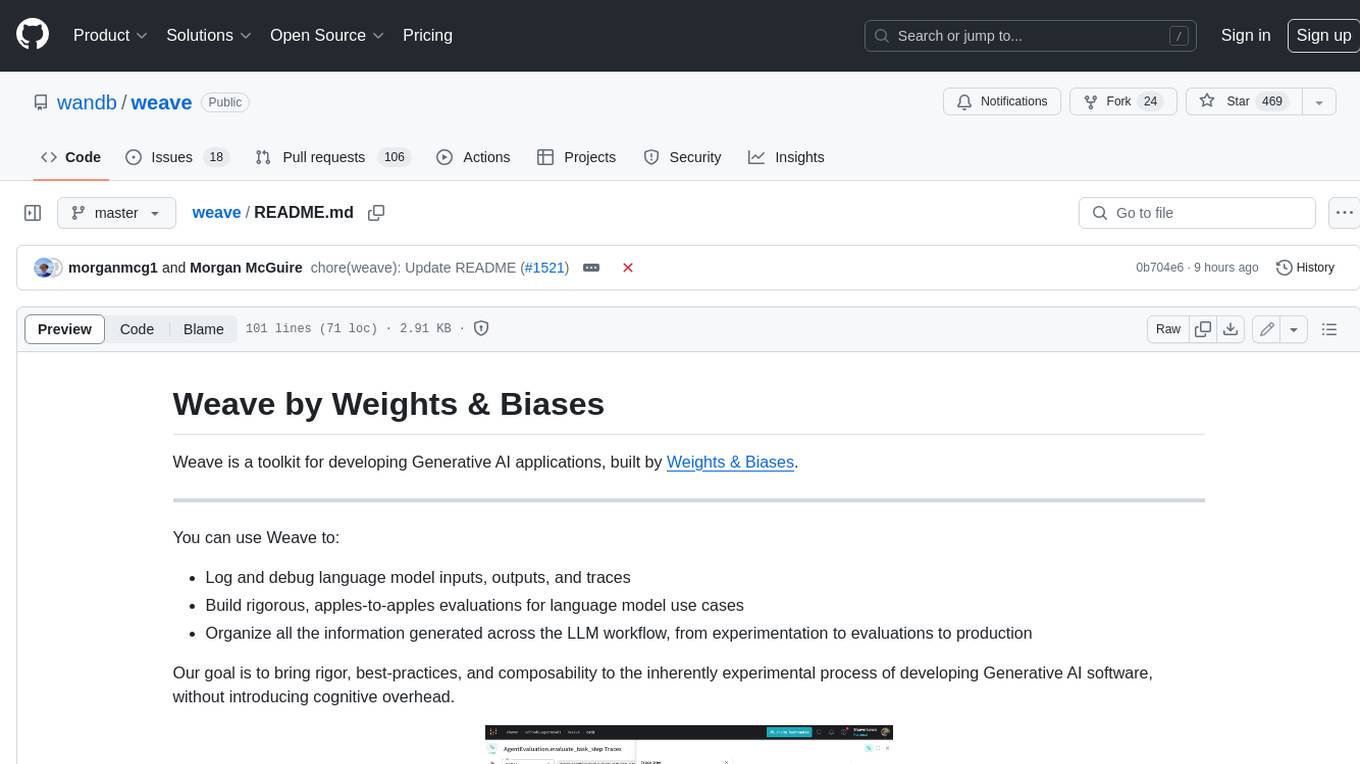
weave
Weave is a toolkit for developing Generative AI applications, built by Weights & Biases. With Weave, you can log and debug language model inputs, outputs, and traces; build rigorous, apples-to-apples evaluations for language model use cases; and organize all the information generated across the LLM workflow, from experimentation to evaluations to production. Weave aims to bring rigor, best-practices, and composability to the inherently experimental process of developing Generative AI software, without introducing cognitive overhead.
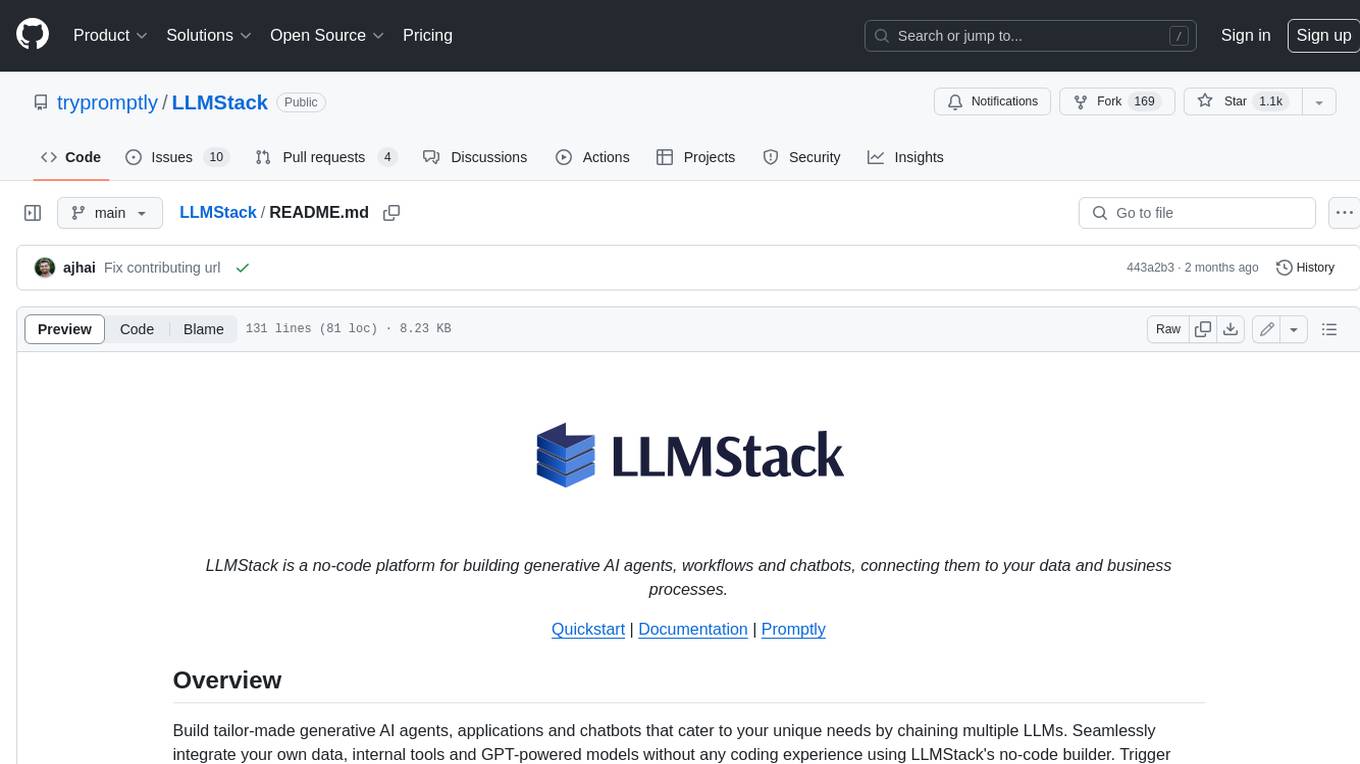
LLMStack
LLMStack is a no-code platform for building generative AI agents, workflows, and chatbots. It allows users to connect their own data, internal tools, and GPT-powered models without any coding experience. LLMStack can be deployed to the cloud or on-premise and can be accessed via HTTP API or triggered from Slack or Discord.
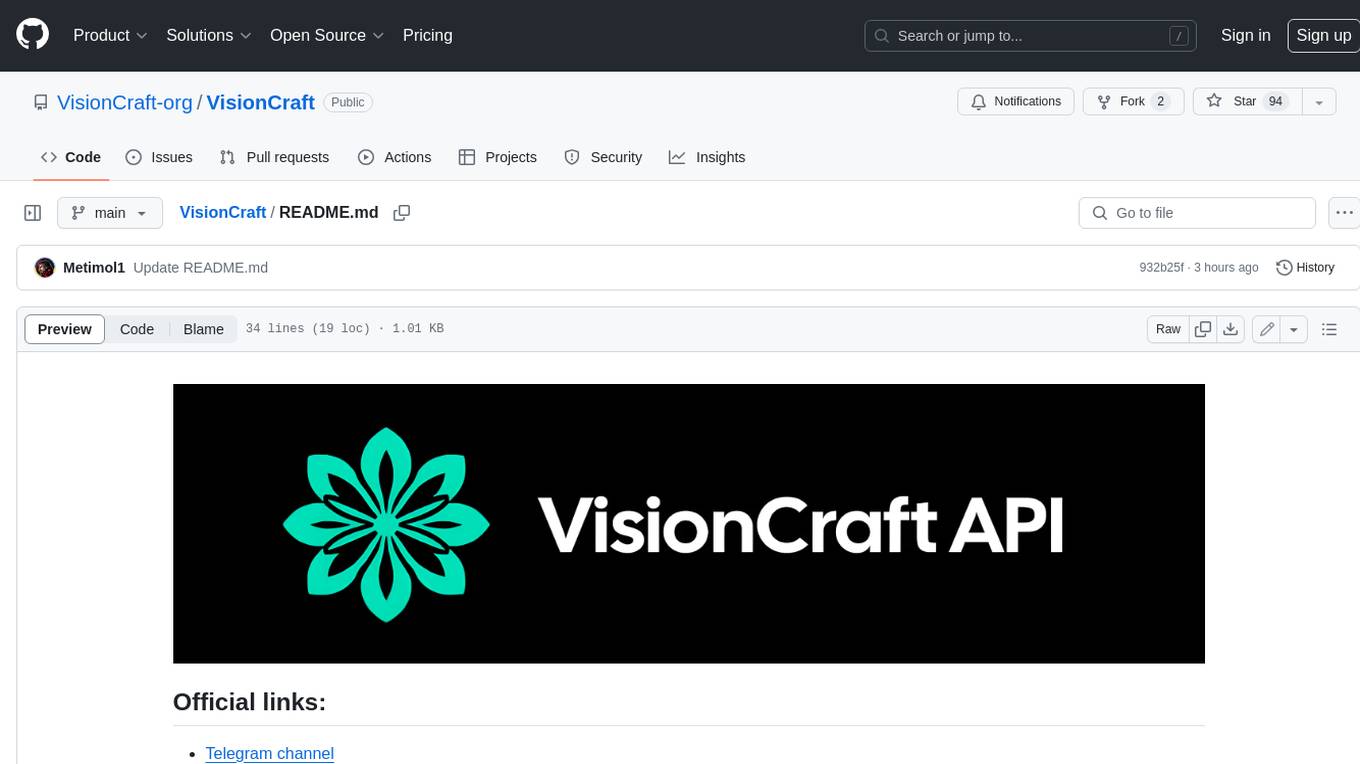
VisionCraft
The VisionCraft API is a free API for using over 100 different AI models. From images to sound.
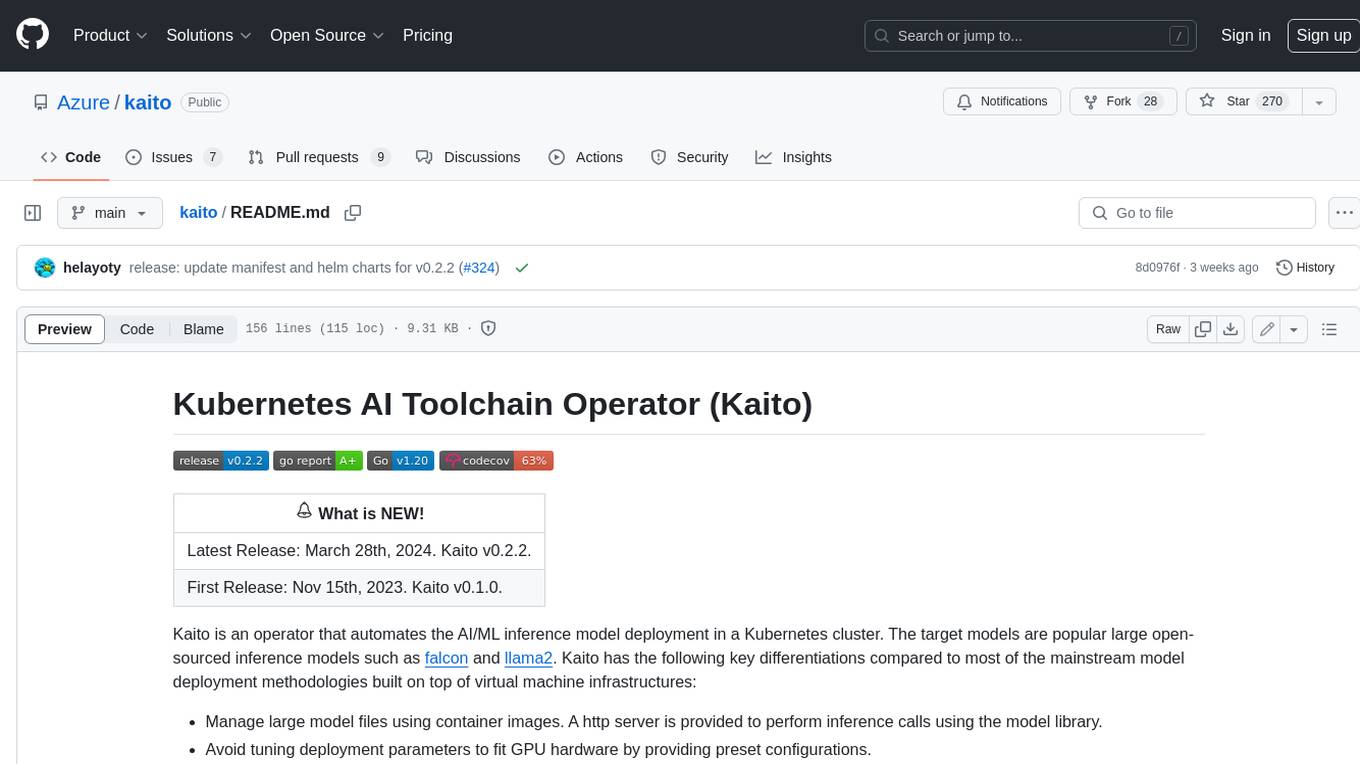
kaito
Kaito is an operator that automates the AI/ML inference model deployment in a Kubernetes cluster. It manages large model files using container images, avoids tuning deployment parameters to fit GPU hardware by providing preset configurations, auto-provisions GPU nodes based on model requirements, and hosts large model images in the public Microsoft Container Registry (MCR) if the license allows. Using Kaito, the workflow of onboarding large AI inference models in Kubernetes is largely simplified.
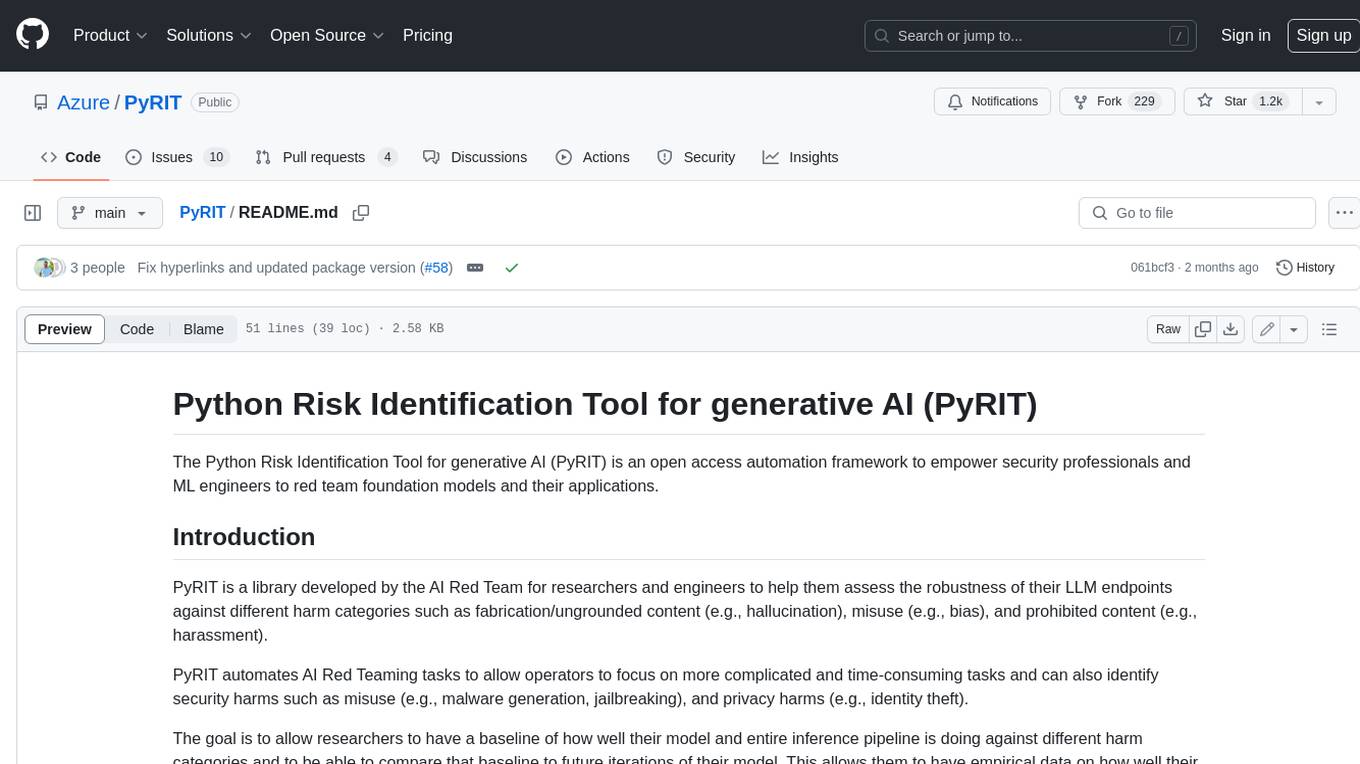
PyRIT
PyRIT is an open access automation framework designed to empower security professionals and ML engineers to red team foundation models and their applications. It automates AI Red Teaming tasks to allow operators to focus on more complicated and time-consuming tasks and can also identify security harms such as misuse (e.g., malware generation, jailbreaking), and privacy harms (e.g., identity theft). The goal is to allow researchers to have a baseline of how well their model and entire inference pipeline is doing against different harm categories and to be able to compare that baseline to future iterations of their model. This allows them to have empirical data on how well their model is doing today, and detect any degradation of performance based on future improvements.
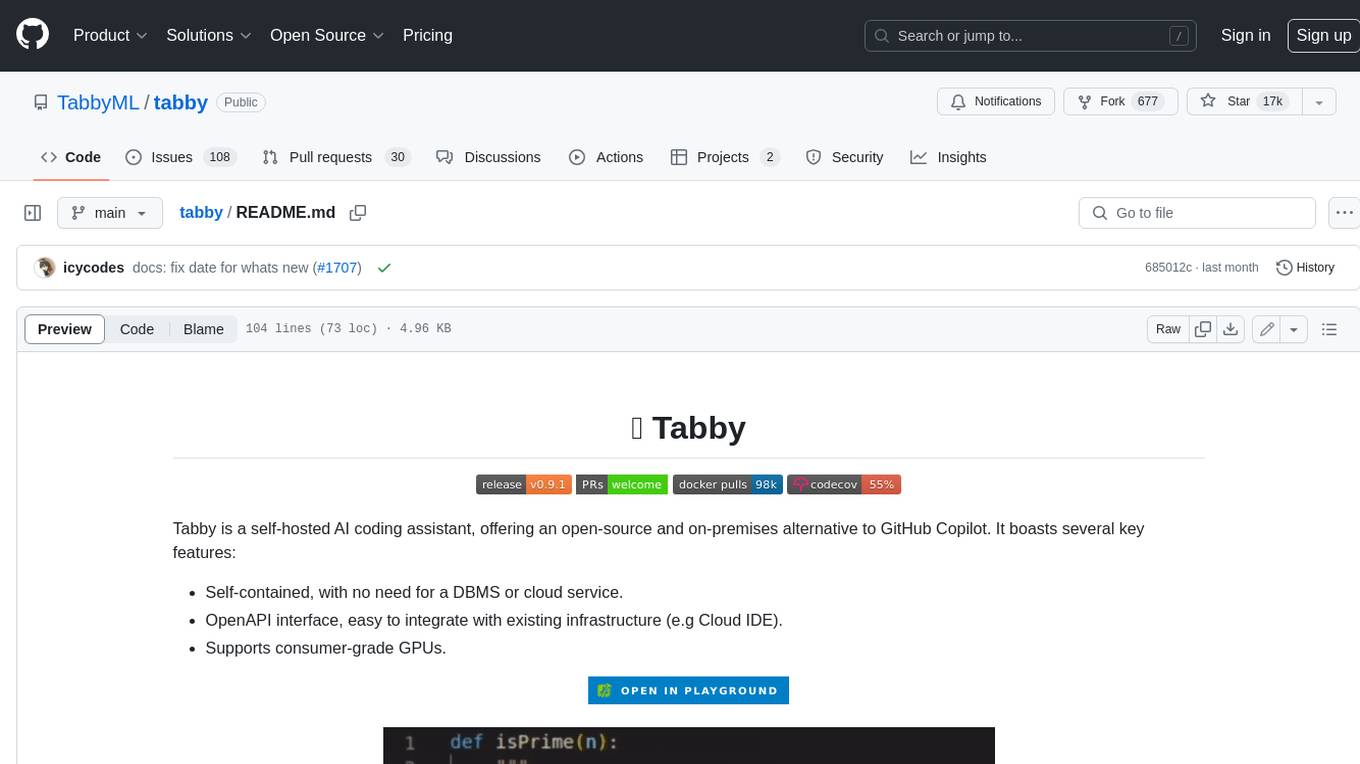
tabby
Tabby is a self-hosted AI coding assistant, offering an open-source and on-premises alternative to GitHub Copilot. It boasts several key features: * Self-contained, with no need for a DBMS or cloud service. * OpenAPI interface, easy to integrate with existing infrastructure (e.g Cloud IDE). * Supports consumer-grade GPUs.
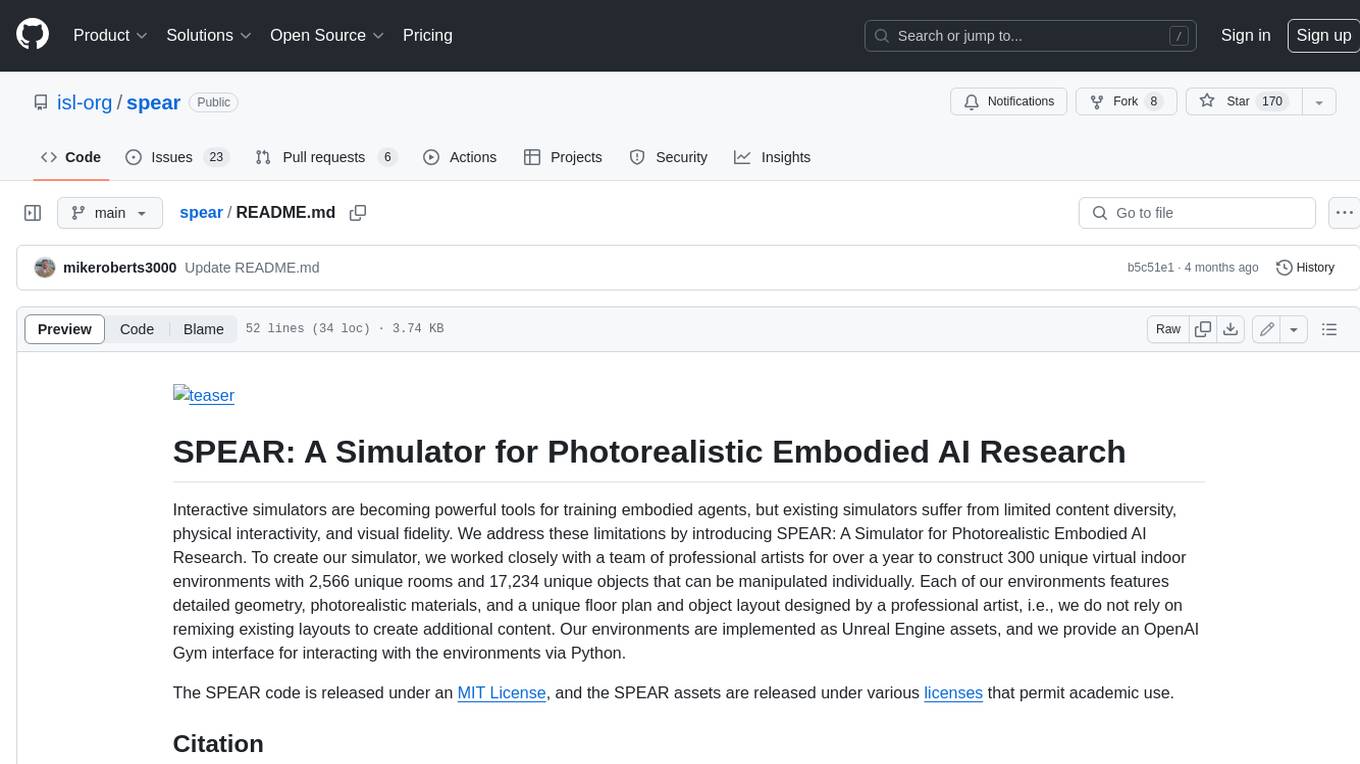
spear
SPEAR (Simulator for Photorealistic Embodied AI Research) is a powerful tool for training embodied agents. It features 300 unique virtual indoor environments with 2,566 unique rooms and 17,234 unique objects that can be manipulated individually. Each environment is designed by a professional artist and features detailed geometry, photorealistic materials, and a unique floor plan and object layout. SPEAR is implemented as Unreal Engine assets and provides an OpenAI Gym interface for interacting with the environments via Python.
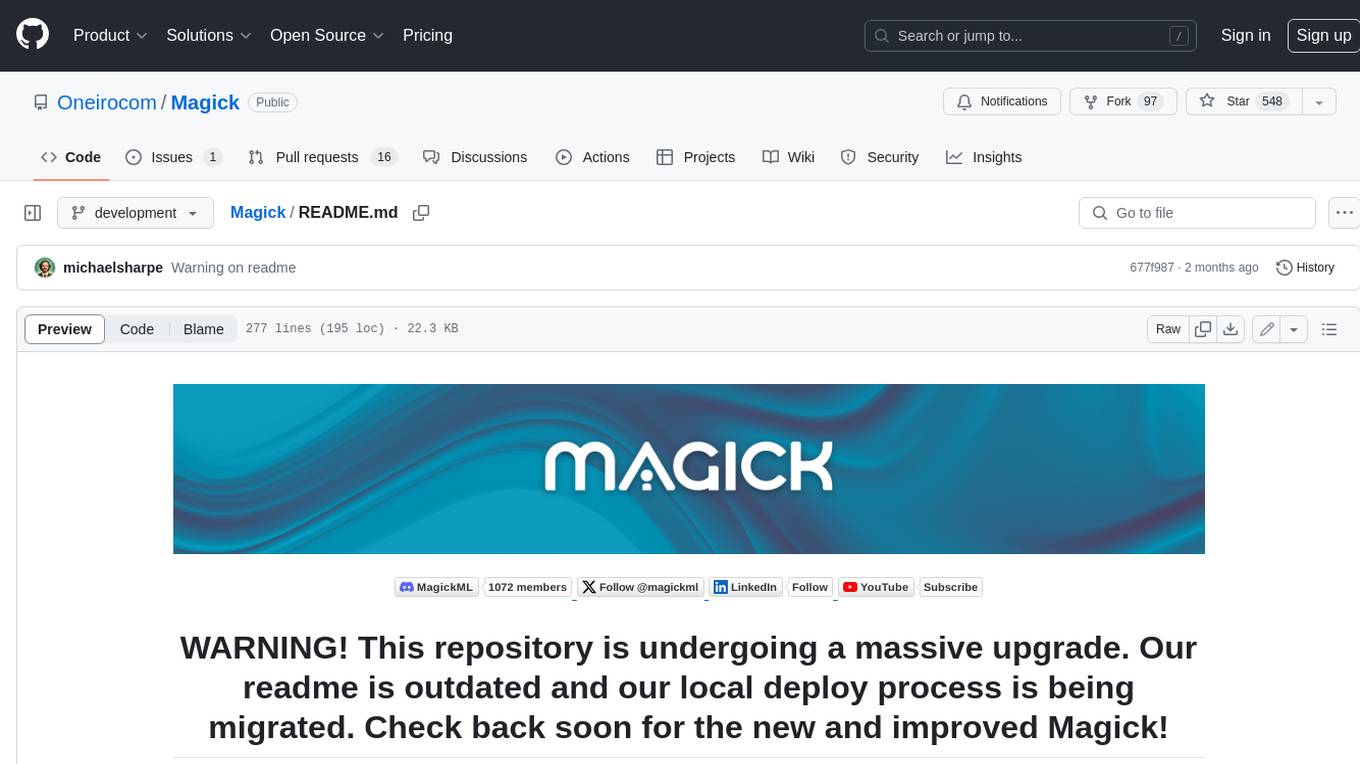
Magick
Magick is a groundbreaking visual AIDE (Artificial Intelligence Development Environment) for no-code data pipelines and multimodal agents. Magick can connect to other services and comes with nodes and templates well-suited for intelligent agents, chatbots, complex reasoning systems and realistic characters.