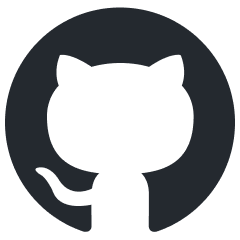
Embodied-AI-Guide
具身智能入门指南 Embodied-AI-Guide
Stars: 1038
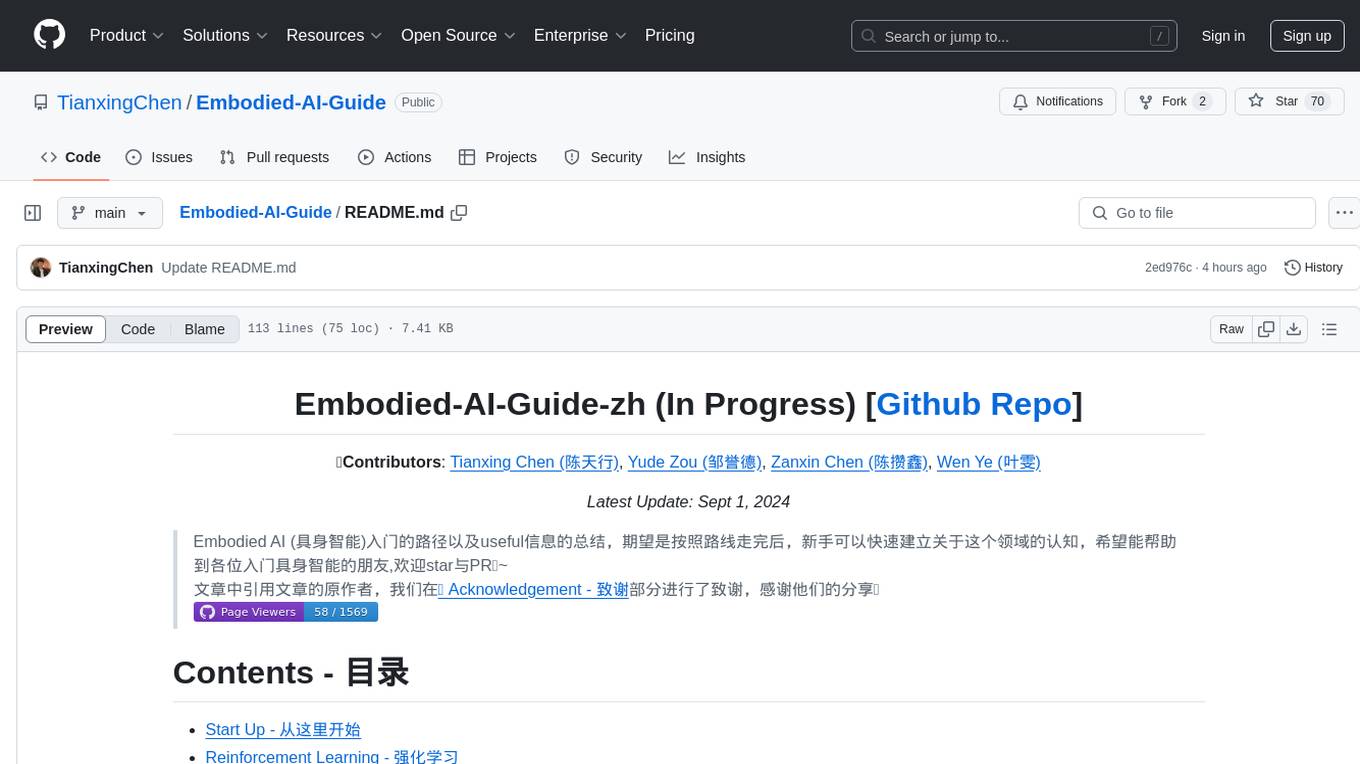
Embodied-AI-Guide is a comprehensive guide for beginners to understand Embodied AI, focusing on the path of entry and useful information in the field. It covers topics such as Reinforcement Learning, Imitation Learning, Large Language Model for Robotics, 3D Vision, Control, Benchmarks, and provides resources for building cognitive understanding. The repository aims to help newcomers quickly establish knowledge in the field of Embodied AI.
README:
Embodied AI(具身智能)入门的路径以及高质量信息的总结,期望是按照路线走完后,新手可以快速建立关于这个领域的认知,希望能帮助到各位入门具身智能的朋友,欢迎点Star、分享与提PR🌟~
【 Embodied-AI-Guide, Latest Update: Dec 29, 2024 】![]()
- 1. Start Up - 从这里开始
- 2. Useful Info - 有利于搭建认知的资料
- 3. Algorithm - 算法
- 4. Hardware - 硬件
- 5. Software - 软件
- 6. Paper Lists - 论文列表
- 7. Acknowledgement - 致谢
具身智能是指一种基于物理身体进行感知和行动的智能系统,其通过智能体与环境的交互获取信息、理解问题、做出决策并实现行动,从而产生智能行为和适应性。
我们希望的是帮助新人快速建立领域认知,所以设计理念是:简要介绍目前具身智能涉及到的主要技术,让大家知道不同的技术能够解决什么问题,未来想要深入发展的时候能够有头绪。
我们是一个由具身初学者组成的团队,希望能够通过我们自己的学习经验,为后来者提供一些帮助,加快具身智能的普及。欢迎更多朋友加入我们的项目,也很欢迎交友、学术合作,有任何问题,可以联系邮箱[email protected]
。
🦉Contributors: 陈天行 (25' 港大PhD), 邹誉德 (25' 上交-浦江实验室联培PhD), 陈思翔 (25' 北大PhD), 叶雯 (25' 中科院自所PhD), 陈攒鑫 (深大本科生), 彭时佳 (深大本科生), 王冠锟 (港中文-华为联培PhD), 吴志杰 (港中文PhD), 朱宇飞 (25' 上科大Ms).
-
社交媒体:
-
可以关注的公众号: 石麻日记 (超高质量!!!), 机器之心, 新智元, 量子位, Xbot具身知识库, 具身智能之心, 自动驾驶之心, 3D视觉工坊, 将门创投, RLCN强化学习研究, CVHub
-
AI领域值得关注的博主列表 [3]: zhihu
-
-
具身智能会投稿的较高质量会议与期刊:RSS, TRO, Science Robotics, IROS, ICRA, ICCV, ECCV, ICRA, AAAI, ICML, CVPR, NIPS, ICLR, IJRR, ACL等。
-
斯坦福机器人学导论:website
-
共建全网最全具身智能知识库 [6]: website
-
社区:
- DeepTimber Robotics Innovations Community, 深木科研交流社区: website
- 宇树具身智能社群: website
- Simulately: Handy information and resources for physics simulators for robot learning research: website
- DeepTimber-地瓜机器人社区: website
- HuggingFace LeRobot (Europe, check the Discord): website
- K-scale labs (US, check the Discord): website
这个部分是关于具身中常用技巧的分享
- 点云降采样: zhihu, 包括随机降采样、均匀降采样、最远点降采样、法线空间降采样等,需要了解清楚每一种降采样的优劣,这个技巧的选择对于3D应用来说是至关重要的。
- 手眼标定:github,手眼标定用于确定相机和机械臂之间以及相机与相机之间的相对位置,大部分Project的开始都需要做一次手眼标定,分为眼在手上和眼在手外。
- URDF生成:CSDN,指导如何通过SolidWorks装配体出发生成机器人URDF文件。
- cuRobo:cuRobocuRobo是Nvidia的一个利用 CUDA 加速的机器人库,提供了一套高效的机器人算法,主要通过并行计算显著提升性能,包括但不限于IK,碰撞检测,路径规划等。
- IKFast:IKFast,经典IK库。
- mplib:mplib,Maniskill Benchmark以及Sapien仿真平台的IK库。
以下是部分具身智能中常用的基础模型, 计算机视觉中发展的非常好的工具可以直接赋能具身智能的下游应用。
-
CLIP: website, 来自OpenAI的研究, 最基本的应用是可以计算图像与语言描述的相似度, 中间层的视觉特征对各种下游应用非常有帮助。
-
DINO: DINO repo, DINO-v2 repo, 来自Meta的研究, 可以提供图像的高层视觉特征, 对corresponding之类的信息提取非常有帮助, 比如不同个体之间的鼻子都有类似的几何特征, 这个时候不同图像中关于不同鼻子的视觉特征值可能是近似的。
-
SAM: website, 来自Meta的研究, 可以基于提示点或者框, 对图像的物体进行分割。
-
SAM2: website, 来自Meta的研究, SAM的升级版, 可以在视频层面持续对物体进行分割追踪。
-
Grounding-DINO: repo, 在线尝试, 这个DINO与上面Meta的DINO没有关系, 是一个由IDEA研究院(做了很多不错开源项目的机构)开发集成的图像目标检测的框架,很多时候需要对目标物体进行检测的时候可以考虑使用。
-
Grounded-SAM: repo, 比Grounding-DINO多了一个分割功能, 也就是支持检测后分割, 也有很多下游应用, 具体可以翻一下README。
-
FoundationPose: website, 来自Nvidia的研究, 物体姿态追踪模型。
-
Stable Diffusion: repo, website, 22年的文生图模型, 现在虽然不是SOTA了, 但是依然可以作为不错的应用, 例如中间层特征支持下游应用、生成Goal Image (目标状态) 等等。
-
Point Transformer (v3): repo, 点云特征提取的工作。
-
RDT-1B: website, 清华朱军老师团队的工作, 机器人双臂操作的基础模型, 具有强大的few-shot能力。
-
SigLIP: huggingface, 类似CLIP。
机器人学习 Robot Learning 的发展: zhihu
- 推荐直接跟着李宏毅老师一套走: bilibili上课+刷蘑菇书巩固+gymnasium动手实践, 重点了解一下PPO。
- 《模仿学习简洁教程》 - 南京大学LAMDA: PDF
- Supervised Policy Learning for Real Robots, RSS 2024 Workshop 教程:真实机器人的监督策略学习, bilibili
- Robotics+LLM系列通过大语言模型控制机器人 [2]: zhihu
- Embodied Agent wiki: website
- Lilian Weng 个人博客 - AI Agent 系统综述 [5]: 中文: website 英文: website
CS231n (斯坦福计算机视觉课程): website, 该课程对深度学习在计算机视觉的应用有较为全面的介绍。因为已经在具体实现某个论文的算法了,所以这个阶段可以不用做作业,只需要看课程视频和课程讲义即可。
第一阶段:学习最基础的3DV知识,追求广度,了解一些基础的概念和算法
第二阶段:细分方向,追求深度,上手一些项目
- 如果对传统图形学感兴趣,可以看下面两门(闫令琪老师开的课,讲得特别好):
- 如果对motion synthesis/computer animation感兴趣,可以看:
- GAMES105 - 计算机角色动画基础: website
- GAMES105 - 计算机角色动画基础: website
- 如何对三维重建感兴趣,可以看下面两门:
- 三维预训练最新综述:
- Advances in 3D pre-training and downstream tasks: a survey: PDF
- Advances in 3D pre-training and downstream tasks: a survey: PDF
- 3DGS在具身上的综述:
- 3D Gaussian Splatting in Robotics: A Survey: PDF
- 3D Gaussian Splatting in Robotics: A Survey: PDF
- SkinGPT-4 for dermatological diagnosis: website
- PneumoLLM for pneumoconiosis diagnosis: website
- BiomedGPT: website
- LLAVA-Med: website
- RoboNurse-VLA: website
Coming Soon...
具身智能硬件方面涵盖多个技术栈,如嵌入式软硬件设计,机械设计,机器人硬件系统设计,这部分知识比较繁杂,适合想要专注此方向的人
关于硬件部分的学习,最好从实践出发!
- 嵌入式学习路线:CSDN
- 51单片机:BiliBili经典江科大自动协出品
- Stm32单片机:BiliBili经典江科大自动协出品
- Stm32电机驱动:BiliBili野火
- 野火Stm32标准库:BiliBili野火
- 正点原子Stm32:BiliBili正点原子
- 韦东山嵌入式Linux:BiliBili韦东山
- SoildWorks教学:BiliBili
Coming Soon !
公司 | 主营产品 | Others |
---|---|---|
松灵AgileX |
pipper机械臂 移动底盘 |
面向教育科研 |
宇树Unitree |
Go2机器狗 通用人形H1 通用人形G1 |
许多产出使用宇树的机器人作为硬件基础 |
方舟无限ARX |
X5机械臂 X7双臂平台 R5机械臂 |
适合复现很多经典的工作,eg. aloha RoboTwin松灵底盘+方舟臂 |
波士顿动力 |
spot机器狗 Atlas通用人形 |
具身智能本体制造商,从液压驱动转向电机驱动 |
灵心巧手 | ||
灵巧智能DexRobot | Dexhand 021灵巧手 | 19自由度量产灵巧手 |
银河通用 | 已完成多轮融资 | |
星海图Galaxea | A1机械臂 | |
World Labs | 专注于空间智能,致力于打造大型世界模型(LWM),以感知、生成并与 3D 世界进行交互。 相关介绍 | |
星动纪元 |
Star1人形 XHAND1灵巧手 |
|
加速进化 | Booster T1人形 | |
青龙机器人 | ||
科技云深处 |
绝影X30四足机器人 Dr.01人形机器人 |
|
松应科技) | 具身智能仿真平台供应商 | |
光轮智能 | 具身智能数据平台 | |
智元机器人 |
A2人形机器人 A2-D数据采集机器人(轮式人形) |
|
Nvidia | 具身智能基建公司 | |
求之科技 | ||
穹彻智能 | ||
优必选机器人 |
具身智能常用benchmark总结 [1]: zhihu
常见仿真器wiki: wiki
- Awesome Humanoid Robot Learning - Yanjie Ze: repo
- Paper Reading List - DeepTimber Community: repo
- Paper List - Yanjie Ze: repo
- Paper List For EmbodiedAI - Tianxing Chen: repo
- SOTA Paper Rating - Weiyang Jin: website
- Awesome-LLM-Robotics: A repo contains a curative list of papers using Large Language/Multi-Modal Models for Robotics/RL: website
本文转载/引用了一些博主的文章,我们对他们的知识分享表示感谢,引用列表如下: [1] 知乎 穆尧, [2] 知乎 东林钟声, Github Yunlong Dong, [3] 知乎 强化学徒, [4] 知乎 Biang哥, [5] OpenAI Lilian Weng, [6] B站 木木具身, [7] Github Zhuoheng Li, [8] 知乎 Flood Sung, [9] Github Sida Peng
This repository is released under the MIT license. See LICENSE for additional details.
For Tasks:
Click tags to check more tools for each tasksFor Jobs:
Alternative AI tools for Embodied-AI-Guide
Similar Open Source Tools
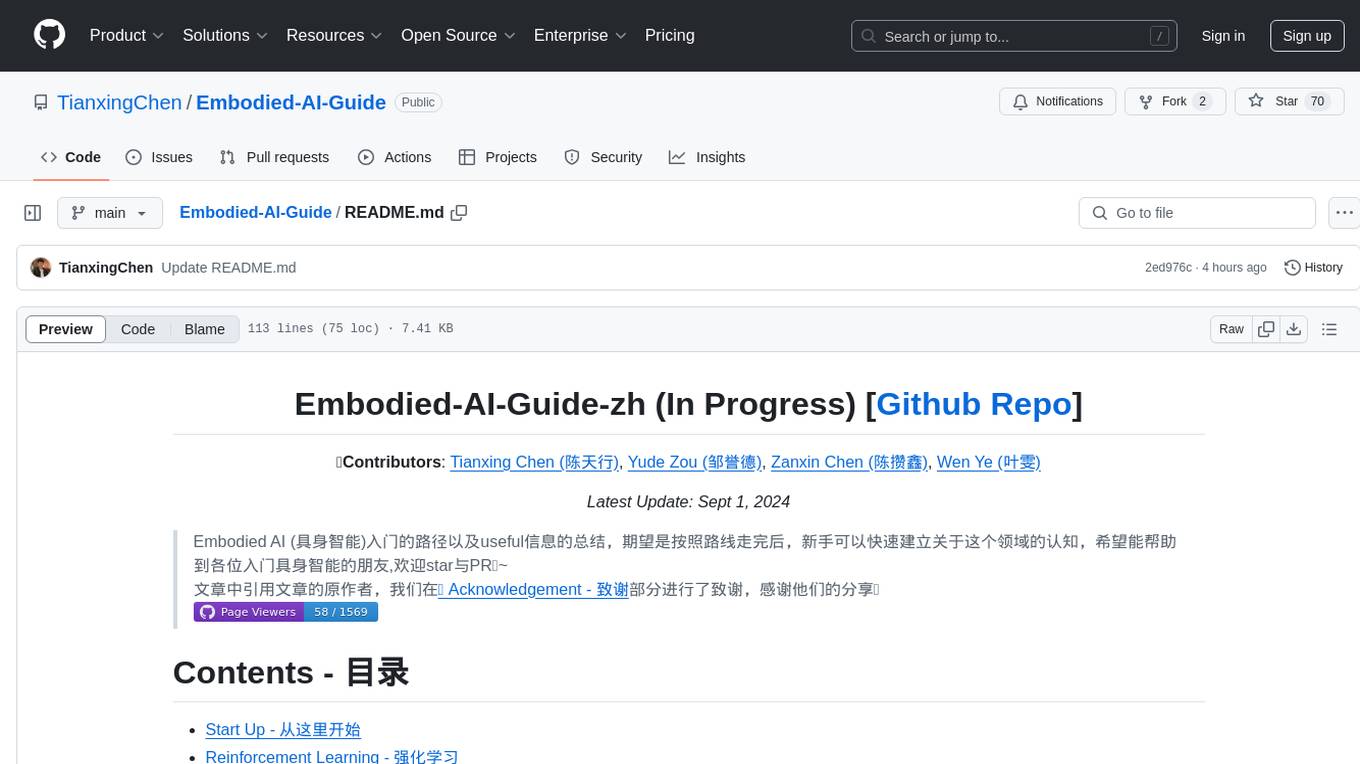
Embodied-AI-Guide
Embodied-AI-Guide is a comprehensive guide for beginners to understand Embodied AI, focusing on the path of entry and useful information in the field. It covers topics such as Reinforcement Learning, Imitation Learning, Large Language Model for Robotics, 3D Vision, Control, Benchmarks, and provides resources for building cognitive understanding. The repository aims to help newcomers quickly establish knowledge in the field of Embodied AI.
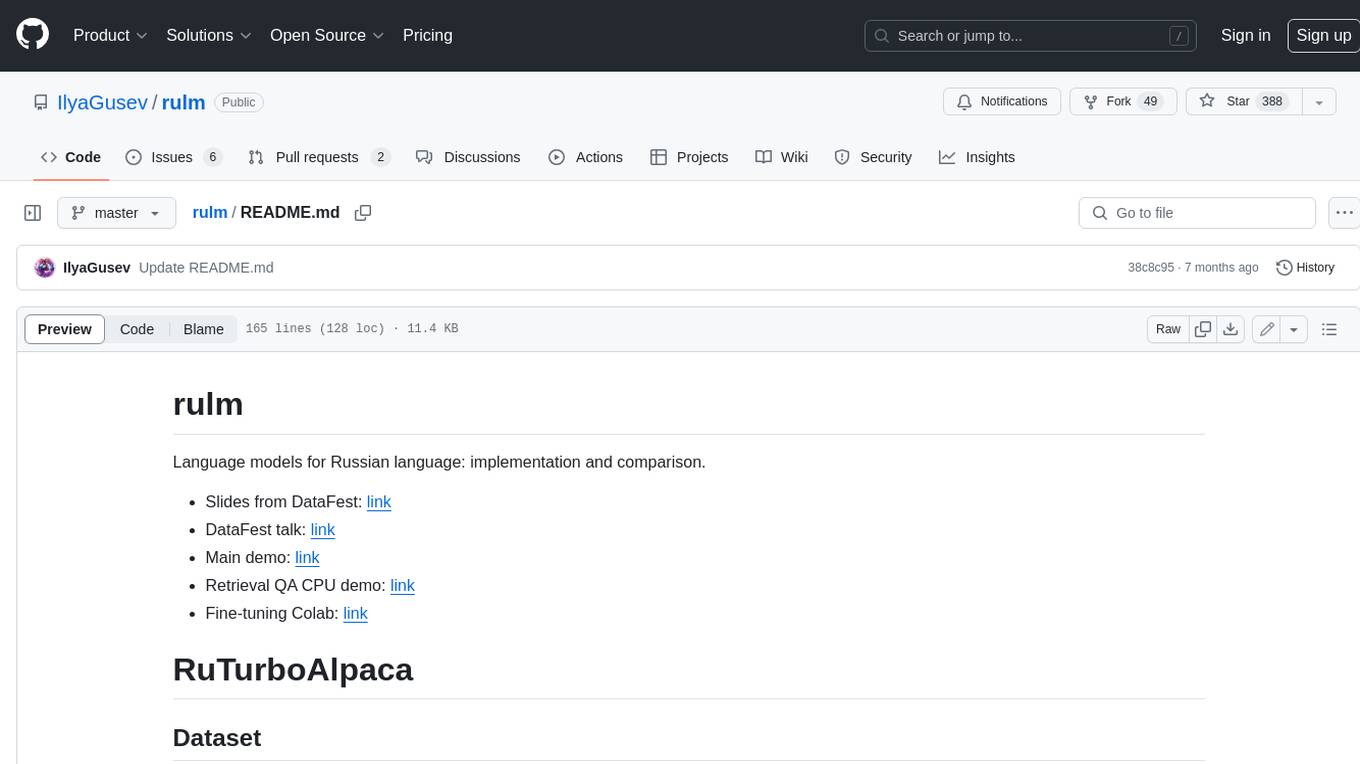
rulm
This repository contains language models for the Russian language, as well as their implementation and comparison. The models are trained on a dataset of ChatGPT-generated instructions and chats in Russian. They can be used for a variety of tasks, including question answering, text generation, and translation.
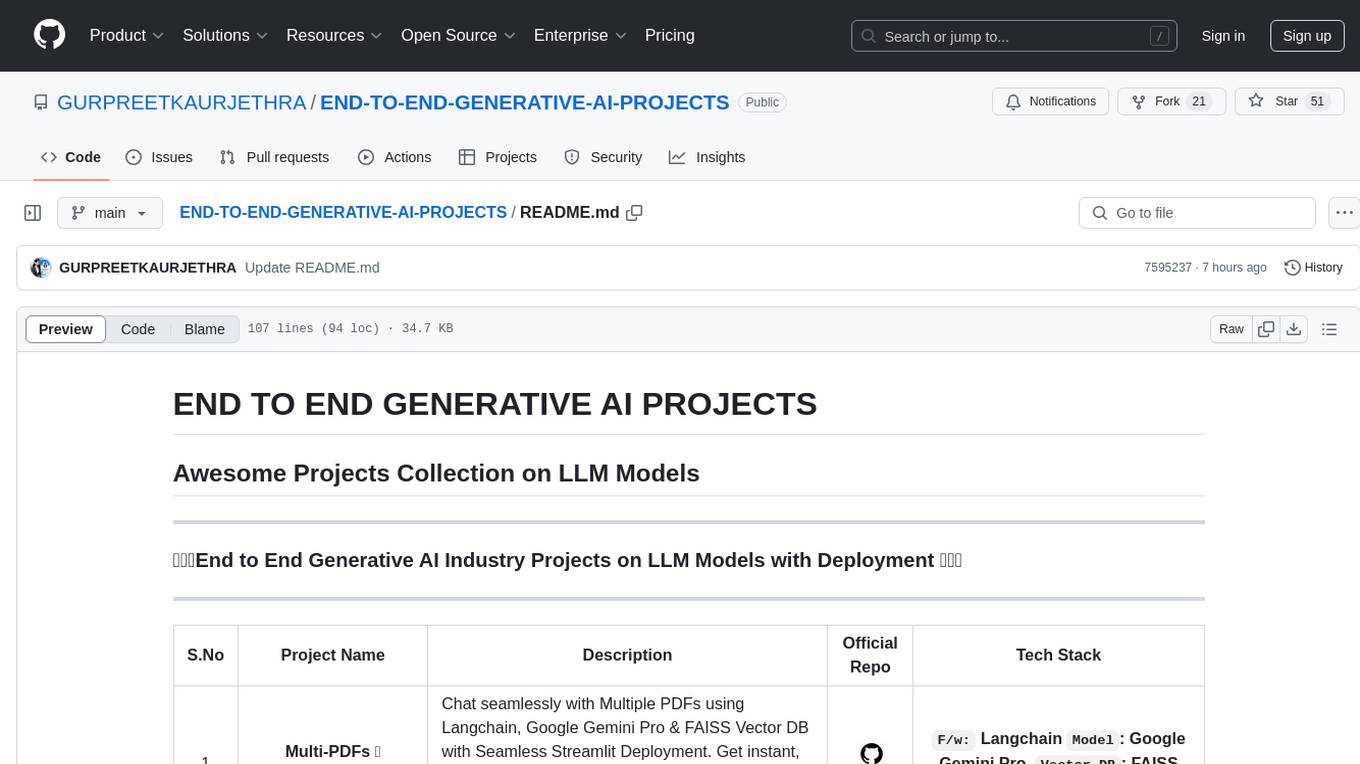
END-TO-END-GENERATIVE-AI-PROJECTS
The 'END TO END GENERATIVE AI PROJECTS' repository is a collection of awesome industry projects utilizing Large Language Models (LLM) for various tasks such as chat applications with PDFs, image to speech generation, video transcribing and summarizing, resume tracking, text to SQL conversion, invoice extraction, medical chatbot, financial stock analysis, and more. The projects showcase the deployment of LLM models like Google Gemini Pro, HuggingFace Models, OpenAI GPT, and technologies such as Langchain, Streamlit, LLaMA2, LLaMAindex, and more. The repository aims to provide end-to-end solutions for different AI applications.
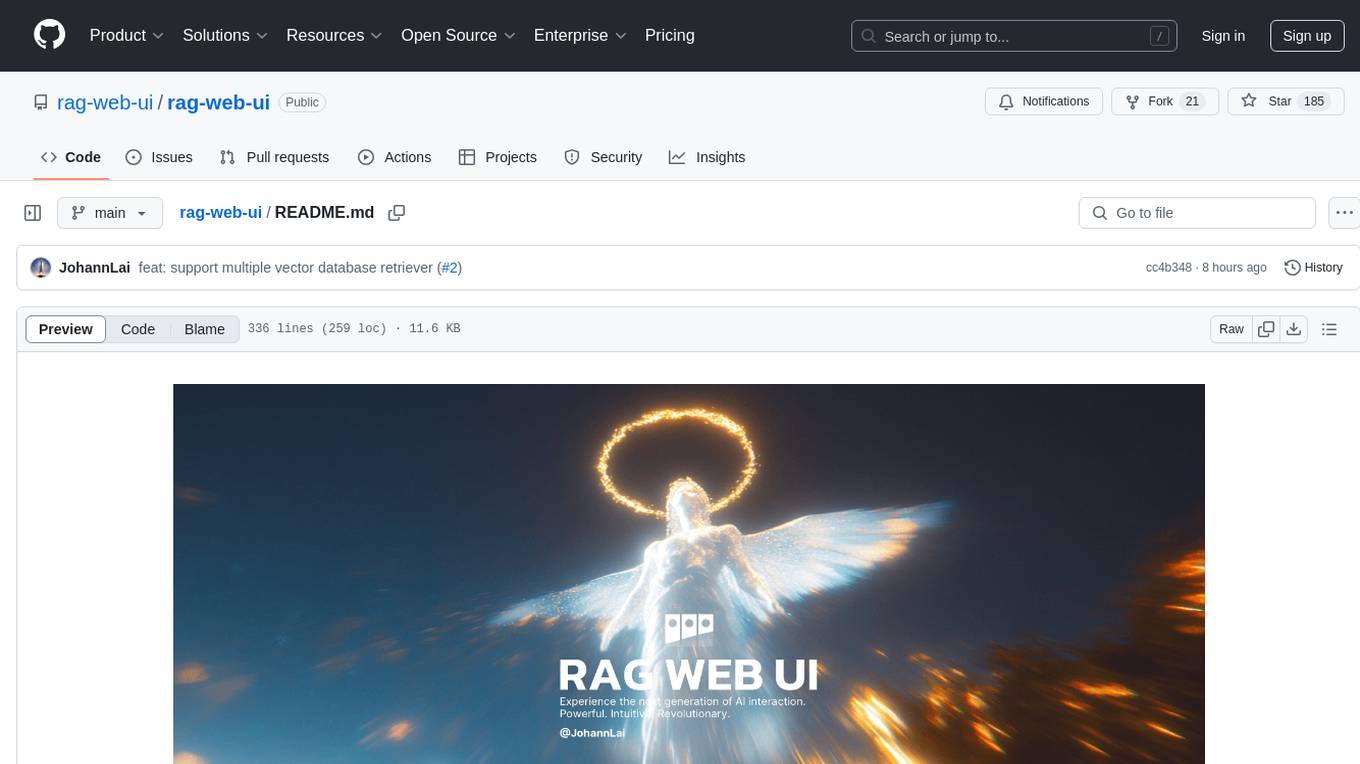
rag-web-ui
RAG Web UI is an intelligent dialogue system based on RAG (Retrieval-Augmented Generation) technology. It helps enterprises and individuals build intelligent Q&A systems based on their own knowledge bases. By combining document retrieval and large language models, it delivers accurate and reliable knowledge-based question-answering services. The system is designed with features like intelligent document management, advanced dialogue engine, and a robust architecture. It supports multiple document formats, async document processing, multi-turn contextual dialogue, and reference citations in conversations. The architecture includes a backend stack with Python FastAPI, MySQL + ChromaDB, MinIO, Langchain, JWT + OAuth2 for authentication, and a frontend stack with Next.js, TypeScript, Tailwind CSS, Shadcn/UI, and Vercel AI SDK for AI integration. Performance optimization includes incremental document processing, streaming responses, vector database performance tuning, and distributed task processing. The project is licensed under the Apache-2.0 License and is intended for learning and sharing RAG knowledge only, not for commercial purposes.
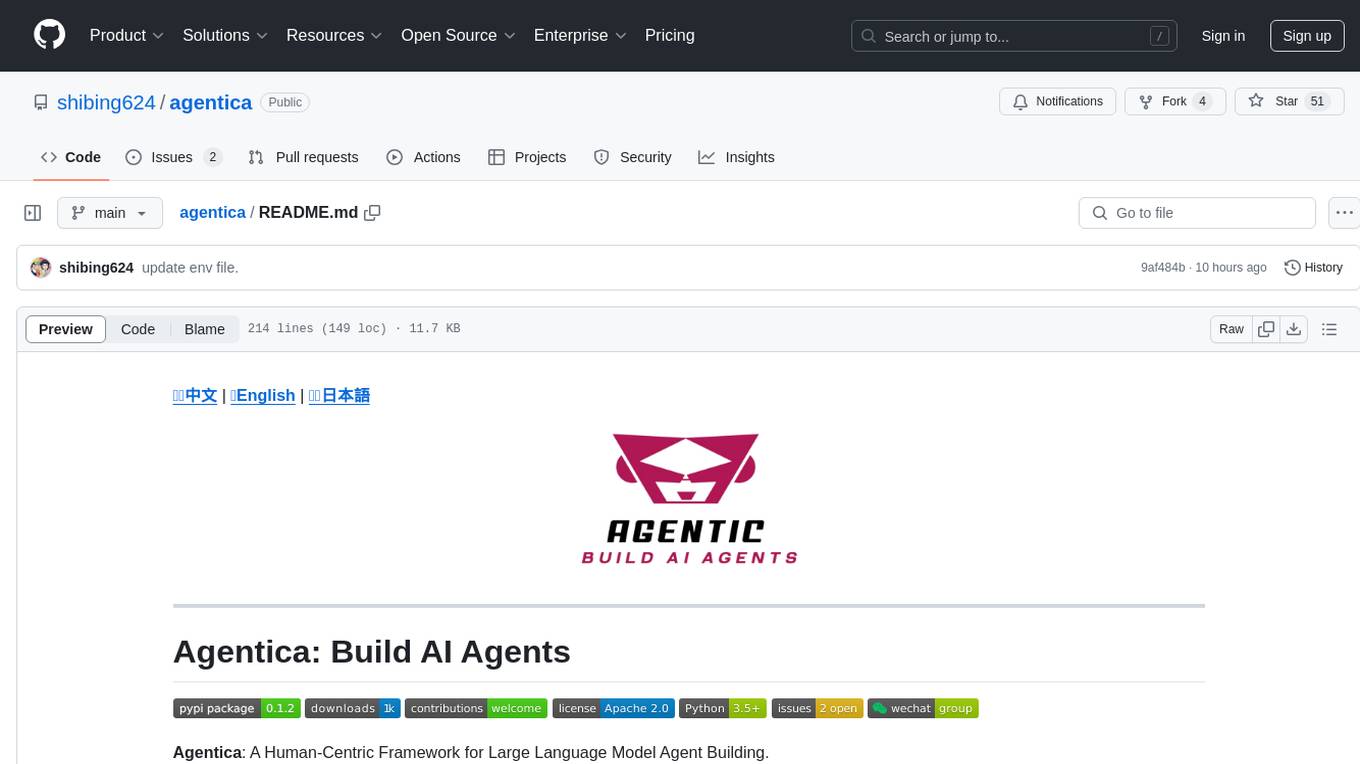
agentica
Agentica is a human-centric framework for building large language model agents. It provides functionalities for planning, memory management, tool usage, and supports features like reflection, planning and execution, RAG, multi-agent, multi-role, and workflow. The tool allows users to quickly code and orchestrate agents, customize prompts, and make API calls to various services. It supports API calls to OpenAI, Azure, Deepseek, Moonshot, Claude, Ollama, and Together. Agentica aims to simplify the process of building AI agents by providing a user-friendly interface and a range of functionalities for agent development.
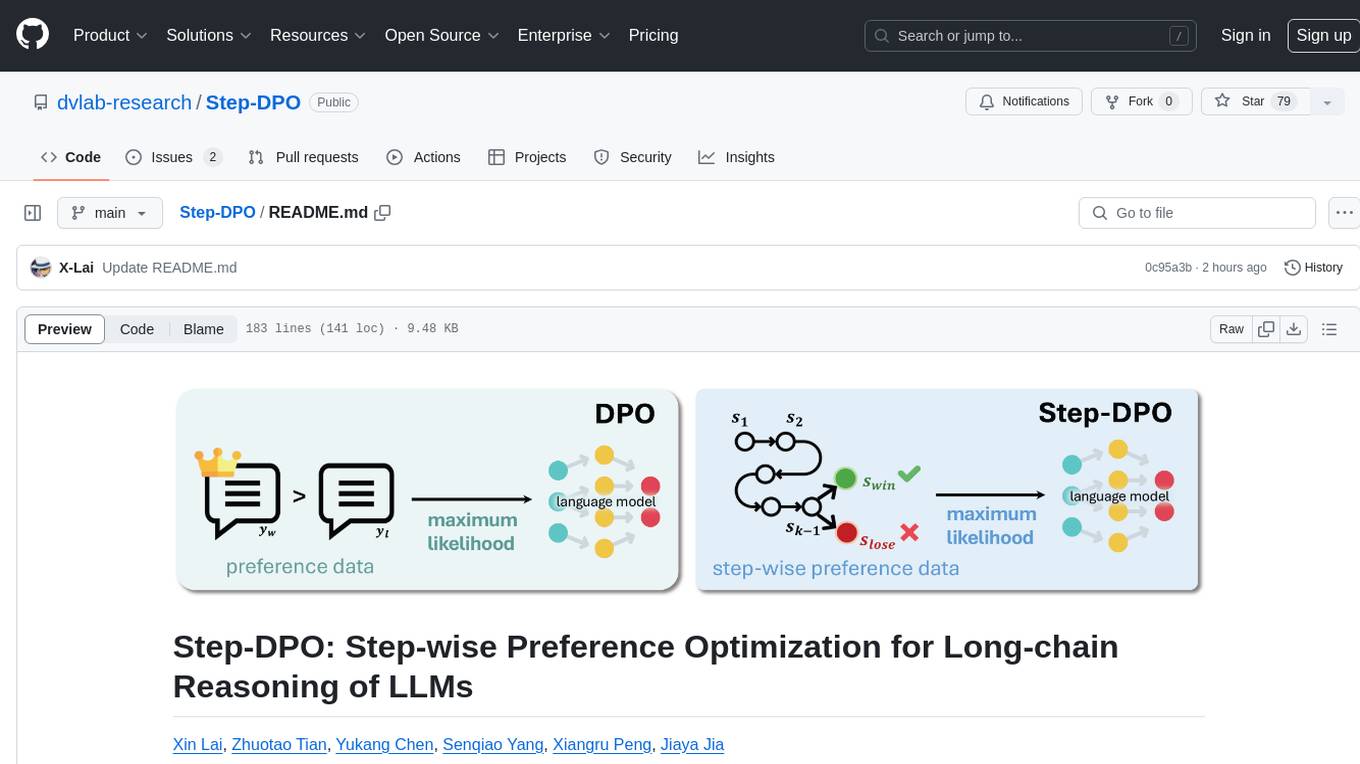
Step-DPO
Step-DPO is a method for enhancing long-chain reasoning ability of LLMs with a data construction pipeline creating a high-quality dataset. It significantly improves performance on math and GSM8K tasks with minimal data and training steps. The tool fine-tunes pre-trained models like Qwen2-7B-Instruct with Step-DPO, achieving superior results compared to other models. It provides scripts for training, evaluation, and deployment, along with examples and acknowledgements.
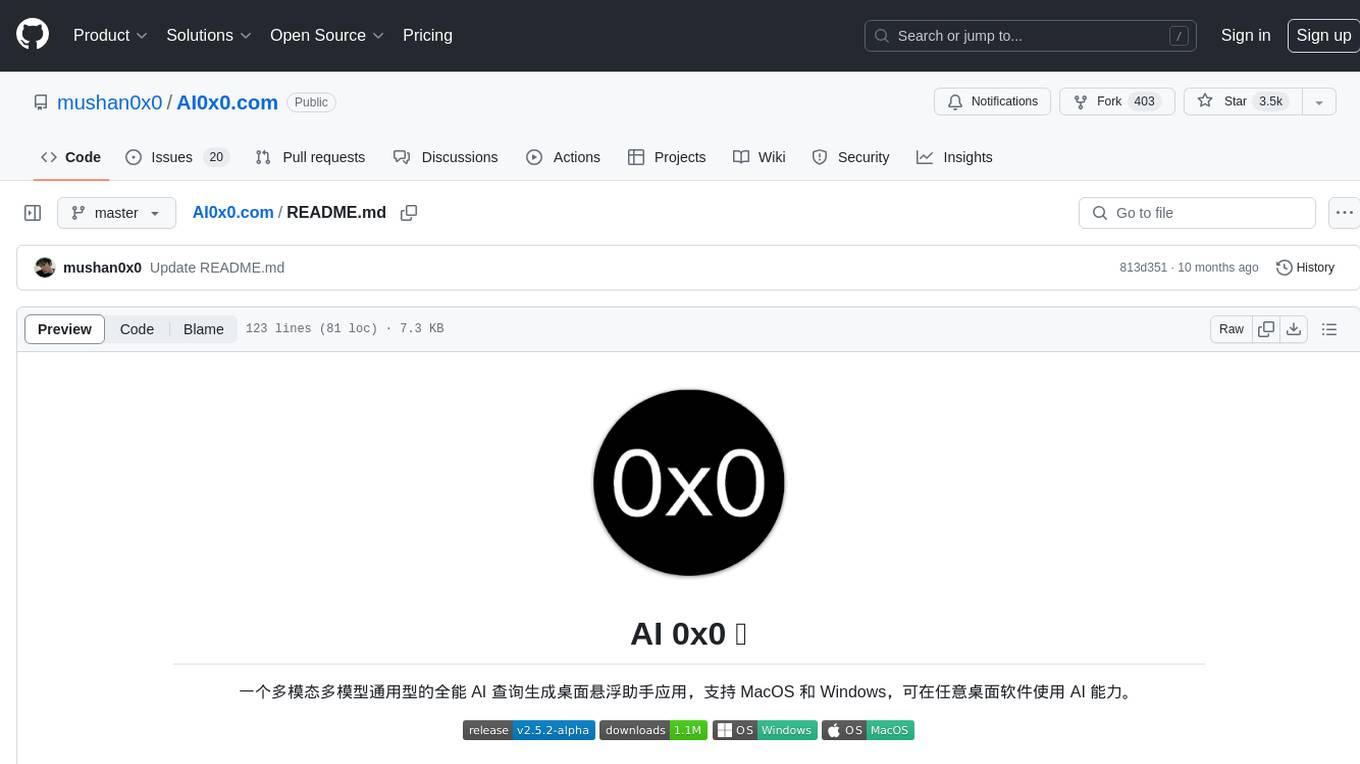
AI0x0.com
AI 0x0 is a versatile AI query generation desktop floating assistant application that supports MacOS and Windows. It allows users to utilize AI capabilities in any desktop software to query and generate text, images, audio, and video data, helping them work more efficiently. The application features a dynamic desktop floating ball, floating dialogue bubbles, customizable presets, conversation bookmarking, preset packages, network acceleration, query mode, input mode, mouse navigation, deep customization of ChatGPT Next Web, support for full-format libraries, online search, voice broadcasting, voice recognition, voice assistant, application plugins, multi-model support, online text and image generation, image recognition, frosted glass interface, light and dark theme adaptation for each language model, and free access to all language models except Chat0x0 with a key.
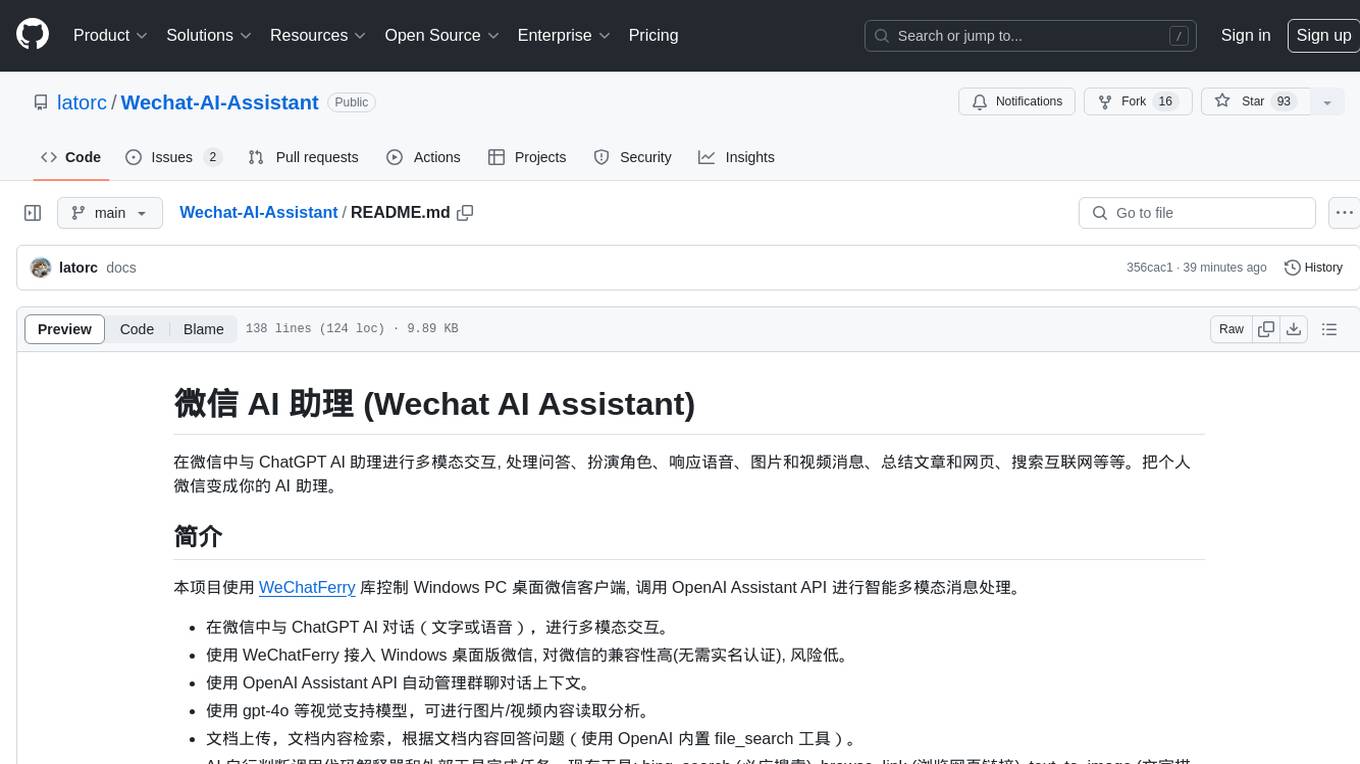
Wechat-AI-Assistant
Wechat AI Assistant is a project that enables multi-modal interaction with ChatGPT AI assistant within WeChat. It allows users to engage in conversations, role-playing, respond to voice messages, analyze images and videos, summarize articles and web links, and search the internet. The project utilizes the WeChatFerry library to control the Windows PC desktop WeChat client and leverages the OpenAI Assistant API for intelligent multi-modal message processing. Users can interact with ChatGPT AI in WeChat through text or voice, access various tools like bing_search, browse_link, image_to_text, text_to_image, text_to_speech, video_analysis, and more. The AI autonomously determines which code interpreter and external tools to use to complete tasks. Future developments include file uploads for AI to reference content, integration with other APIs, and login support for enterprise WeChat and WeChat official accounts.
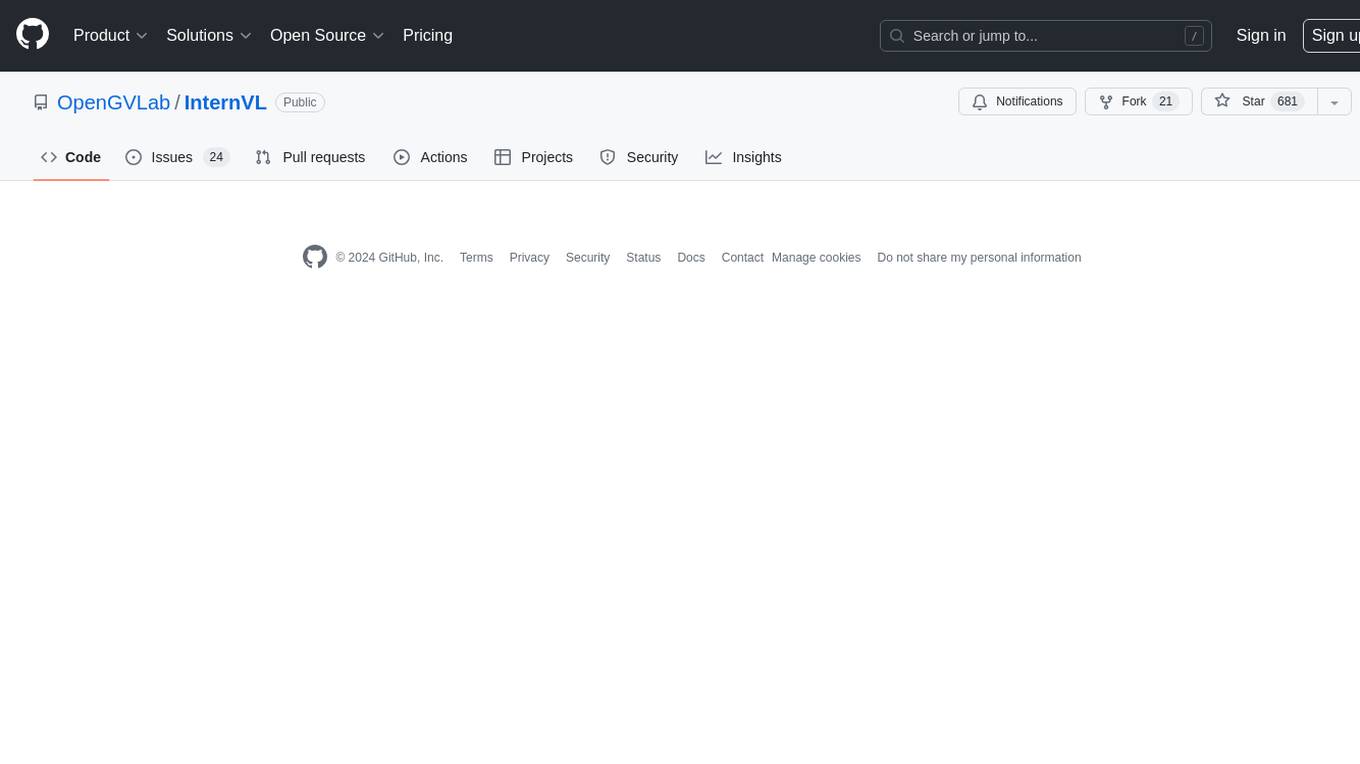
InternVL
InternVL scales up the ViT to _**6B parameters**_ and aligns it with LLM. It is a vision-language foundation model that can perform various tasks, including: **Visual Perception** - Linear-Probe Image Classification - Semantic Segmentation - Zero-Shot Image Classification - Multilingual Zero-Shot Image Classification - Zero-Shot Video Classification **Cross-Modal Retrieval** - English Zero-Shot Image-Text Retrieval - Chinese Zero-Shot Image-Text Retrieval - Multilingual Zero-Shot Image-Text Retrieval on XTD **Multimodal Dialogue** - Zero-Shot Image Captioning - Multimodal Benchmarks with Frozen LLM - Multimodal Benchmarks with Trainable LLM - Tiny LVLM InternVL has been shown to achieve state-of-the-art results on a variety of benchmarks. For example, on the MMMU image classification benchmark, InternVL achieves a top-1 accuracy of 51.6%, which is higher than GPT-4V and Gemini Pro. On the DocVQA question answering benchmark, InternVL achieves a score of 82.2%, which is also higher than GPT-4V and Gemini Pro. InternVL is open-sourced and available on Hugging Face. It can be used for a variety of applications, including image classification, object detection, semantic segmentation, image captioning, and question answering.
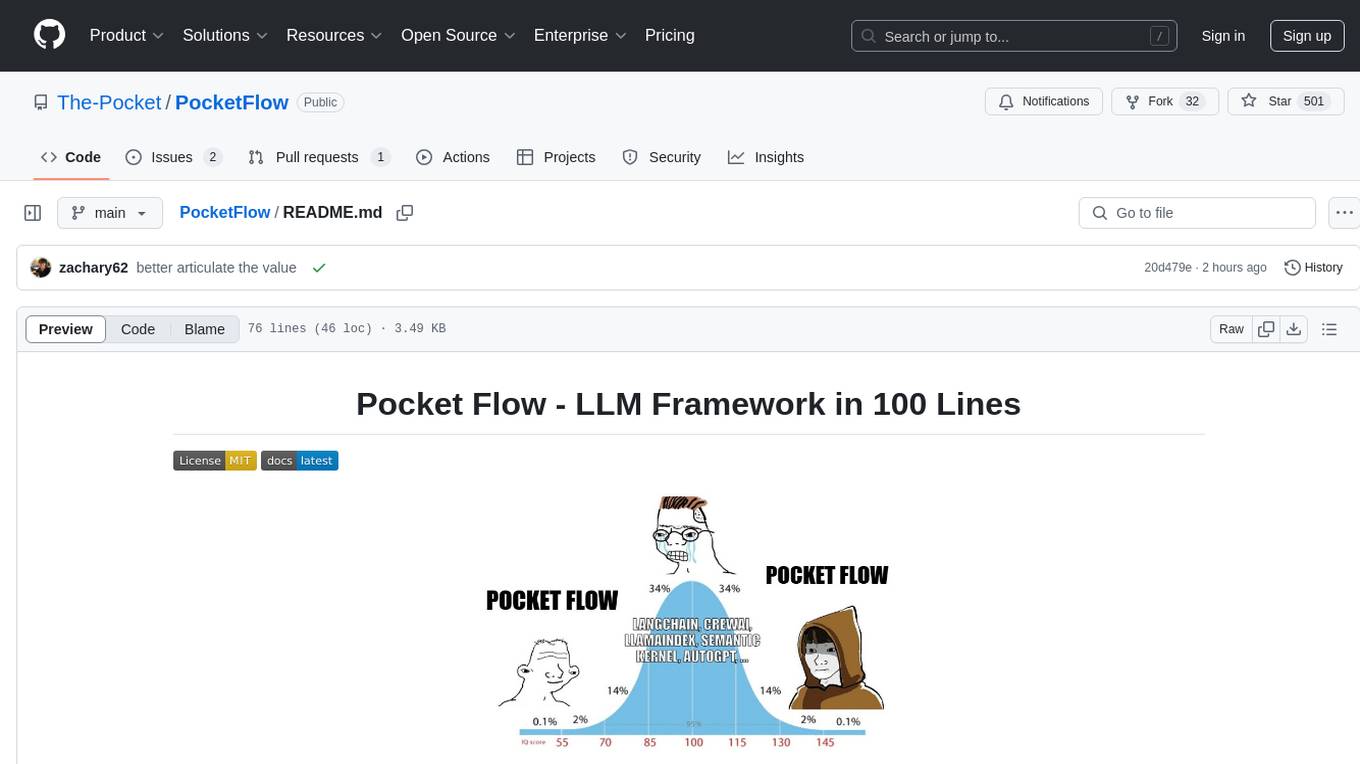
PocketFlow
Pocket Flow is a 100-line minimalist LLM framework designed for (Multi-)Agents, Workflow, RAG, etc. It provides a core abstraction for LLM projects by focusing on computation and communication through a graph structure and shared store. The framework aims to support the development of LLM Agents, such as Cursor AI, by offering a minimal and low-level approach that is well-suited for understanding and usage. Users can install Pocket Flow via pip or by copying the source code, and detailed documentation is available on the project website.
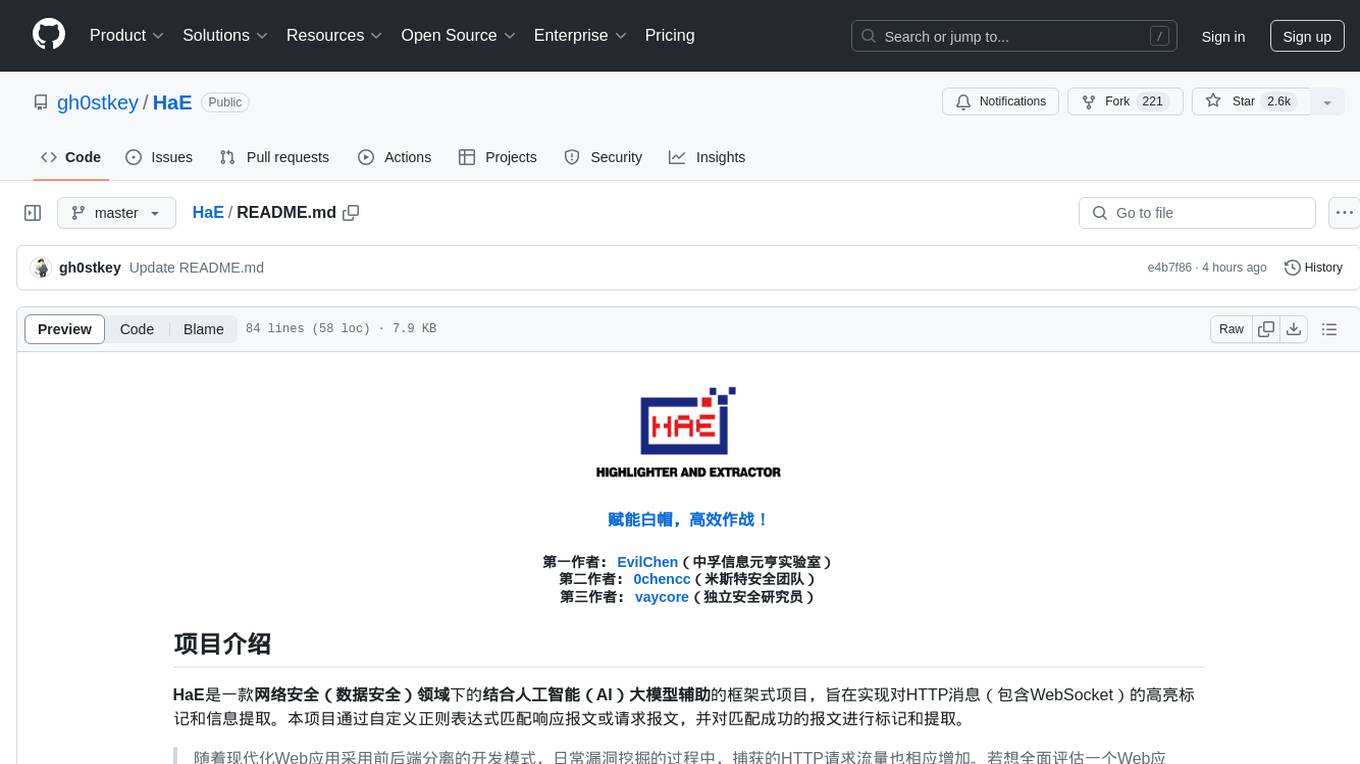
HaE
HaE is a framework project in the field of network security (data security) that combines artificial intelligence (AI) large models to achieve highlighting and information extraction of HTTP messages (including WebSocket). It aims to reduce testing time, focus on valuable and meaningful messages, and improve vulnerability discovery efficiency. The project provides a clear and visual interface design, simple interface interaction, and centralized data panel for querying and extracting information. It also features built-in color upgrade algorithm, one-click export/import of data, and integration of AI large models API for optimized data processing.
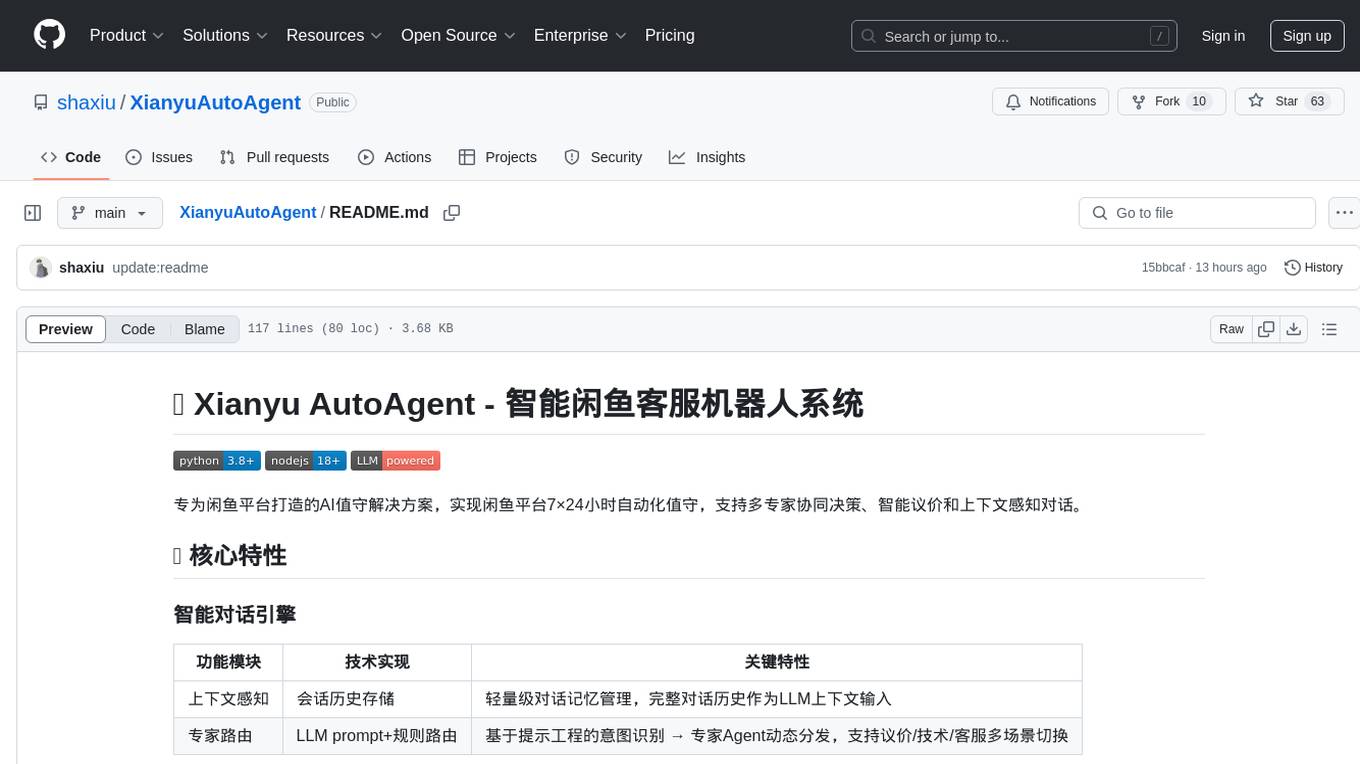
XianyuAutoAgent
Xianyu AutoAgent is an AI customer service robot system specifically designed for the Xianyu platform, providing 24/7 automated customer service, supporting multi-expert collaborative decision-making, intelligent bargaining, and context-aware conversations. The system includes intelligent conversation engine with features like context awareness and expert routing, business function matrix with modules like core engine, bargaining system, technical support, and operation monitoring. It requires Python 3.8+ and NodeJS 18+ for installation and operation. Users can customize prompts for different experts and contribute to the project through issues or pull requests.
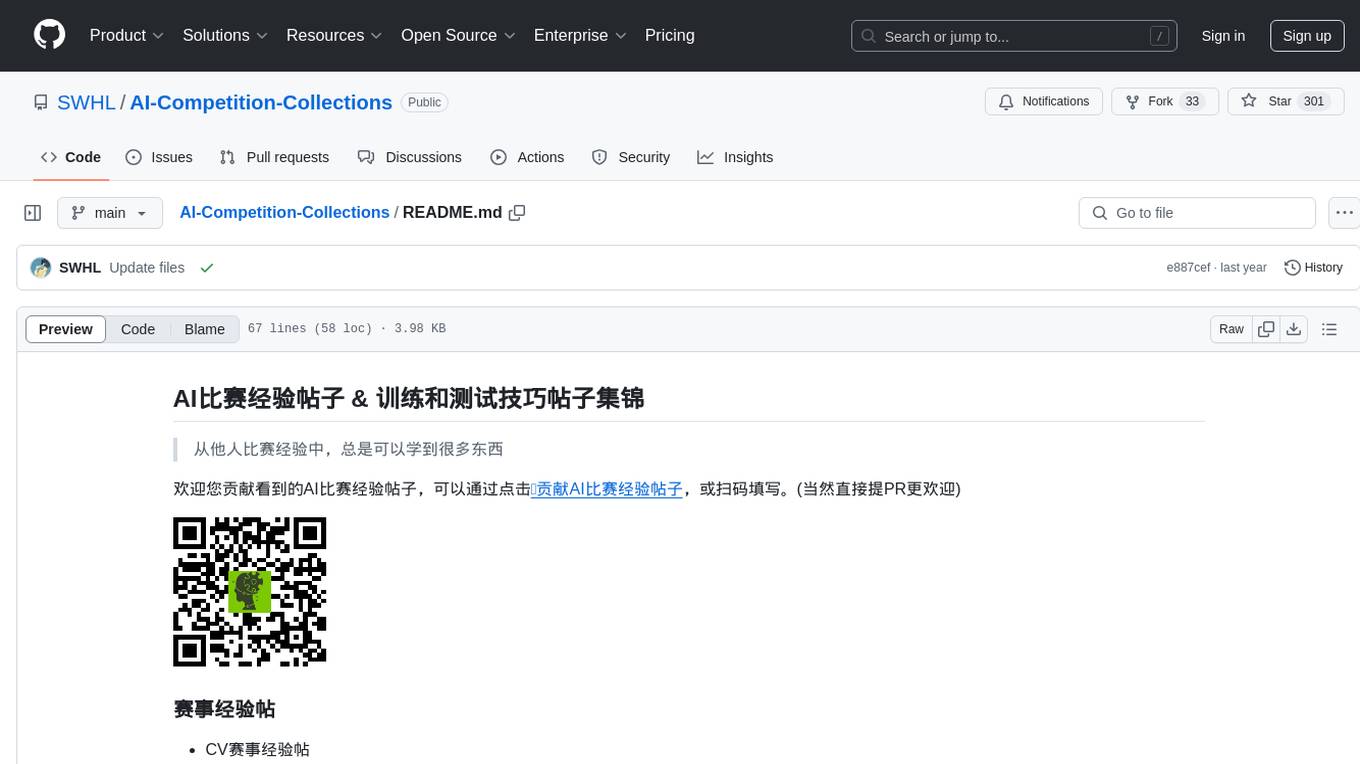
AI-Competition-Collections
AI-Competition-Collections is a repository that collects and curates various experiences and tips from AI competitions. It includes posts on competition experiences in computer vision, NLP, speech, and other AI-related fields. The repository aims to provide valuable insights and techniques for individuals participating in AI competitions, covering topics such as image classification, object detection, OCR, adversarial attacks, and more.
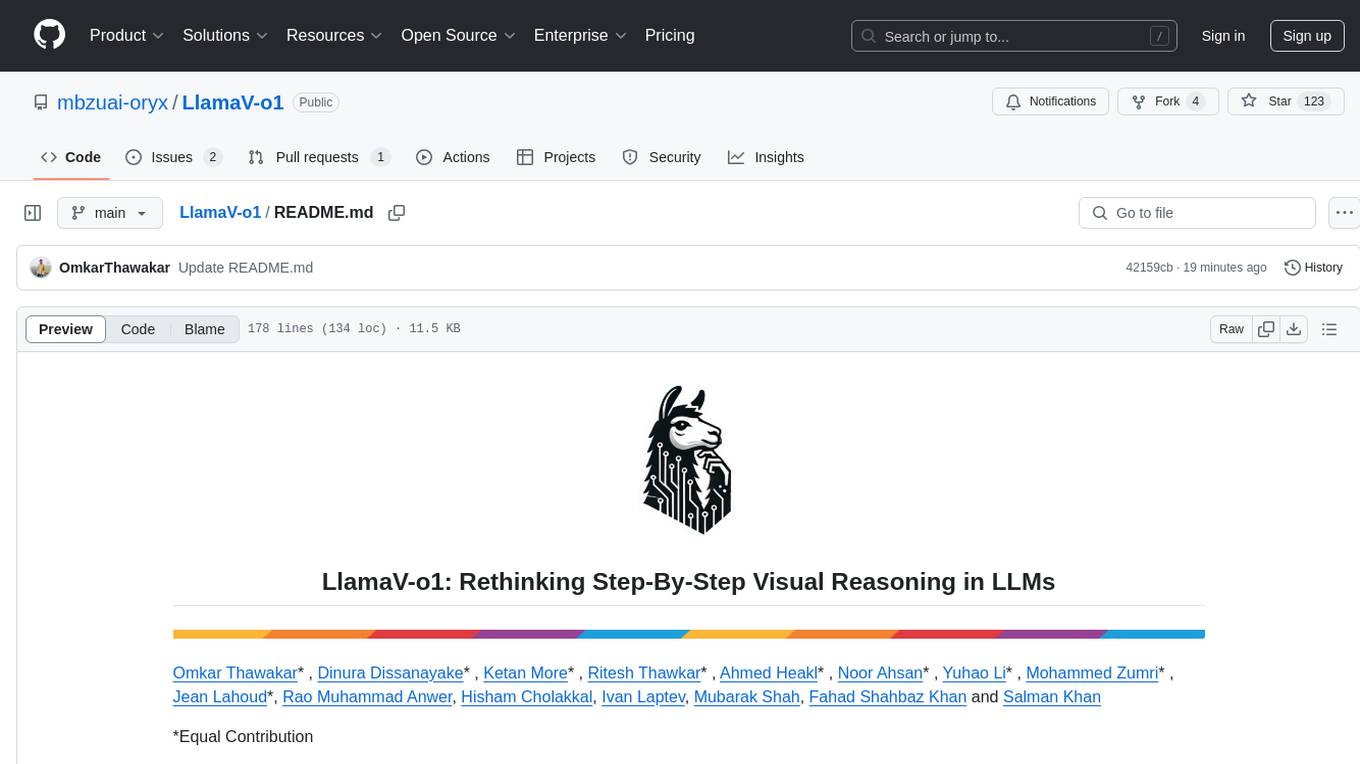
LlamaV-o1
LlamaV-o1 is a Large Multimodal Model designed for spontaneous reasoning tasks. It outperforms various existing models on multimodal reasoning benchmarks. The project includes a Step-by-Step Visual Reasoning Benchmark, a novel evaluation metric, and a combined Multi-Step Curriculum Learning and Beam Search Approach. The model achieves superior performance in complex multi-step visual reasoning tasks in terms of accuracy and efficiency.
For similar tasks
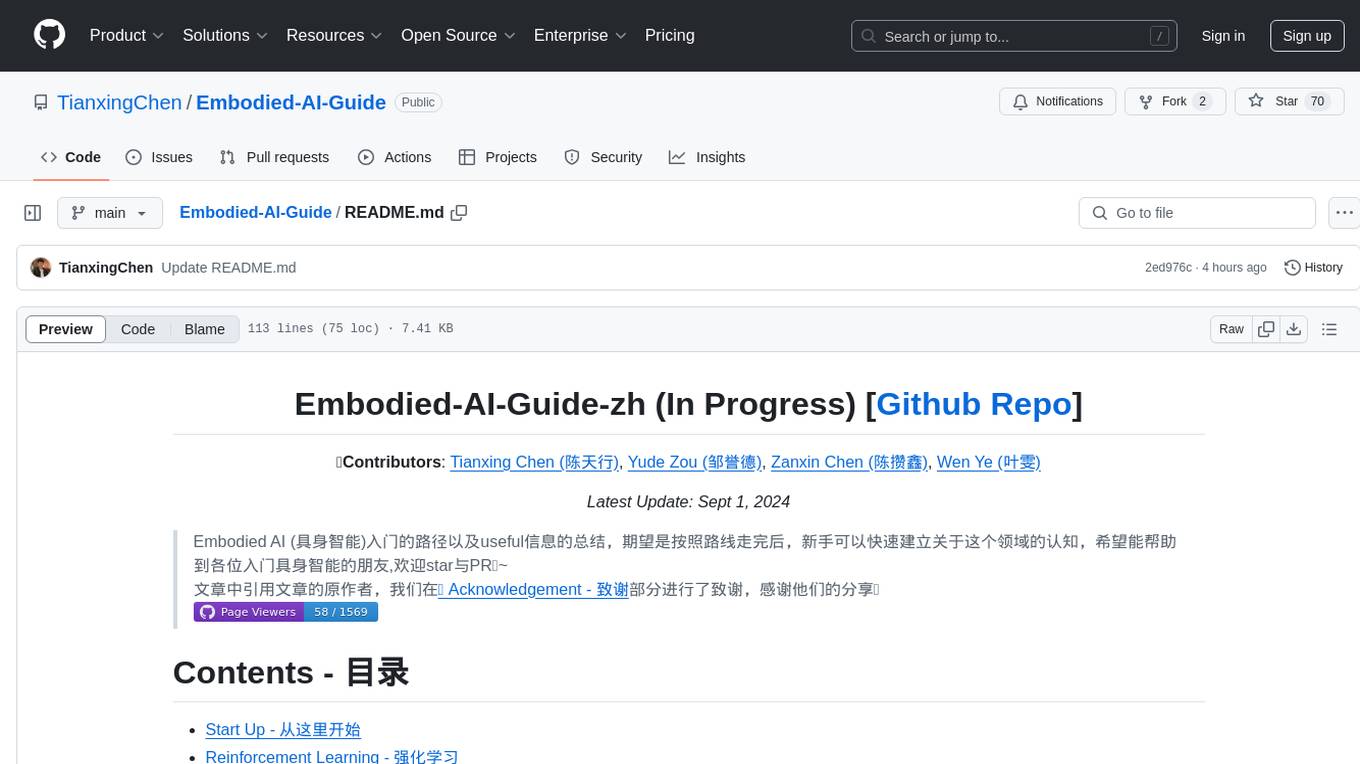
Embodied-AI-Guide
Embodied-AI-Guide is a comprehensive guide for beginners to understand Embodied AI, focusing on the path of entry and useful information in the field. It covers topics such as Reinforcement Learning, Imitation Learning, Large Language Model for Robotics, 3D Vision, Control, Benchmarks, and provides resources for building cognitive understanding. The repository aims to help newcomers quickly establish knowledge in the field of Embodied AI.
For similar jobs
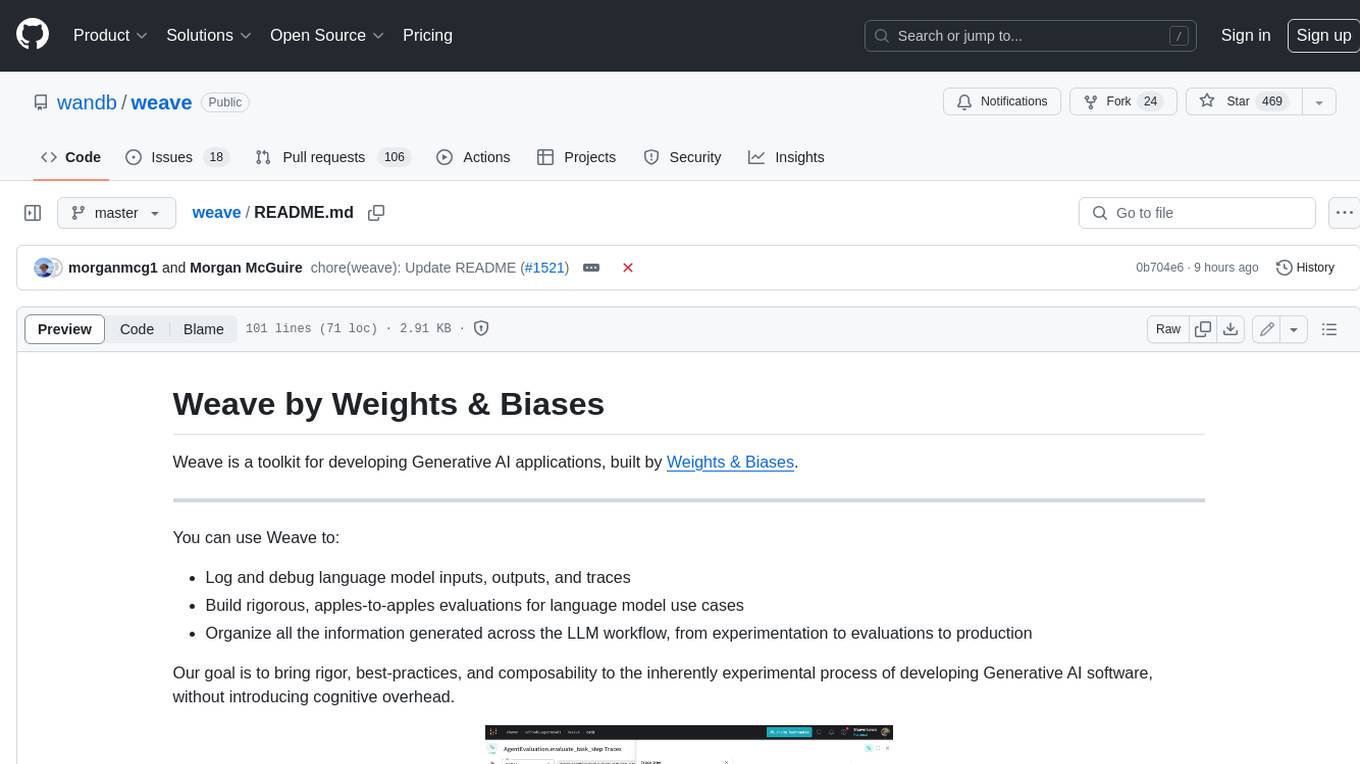
weave
Weave is a toolkit for developing Generative AI applications, built by Weights & Biases. With Weave, you can log and debug language model inputs, outputs, and traces; build rigorous, apples-to-apples evaluations for language model use cases; and organize all the information generated across the LLM workflow, from experimentation to evaluations to production. Weave aims to bring rigor, best-practices, and composability to the inherently experimental process of developing Generative AI software, without introducing cognitive overhead.
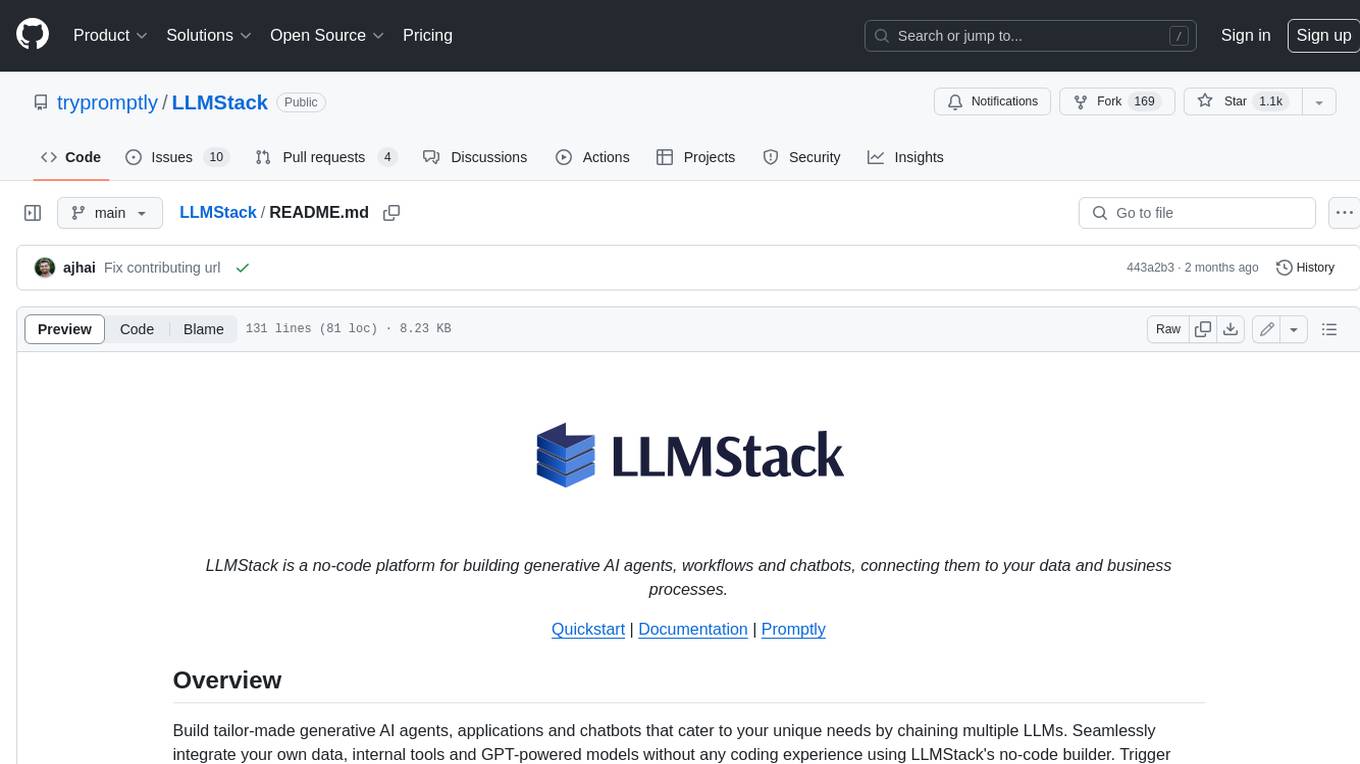
LLMStack
LLMStack is a no-code platform for building generative AI agents, workflows, and chatbots. It allows users to connect their own data, internal tools, and GPT-powered models without any coding experience. LLMStack can be deployed to the cloud or on-premise and can be accessed via HTTP API or triggered from Slack or Discord.
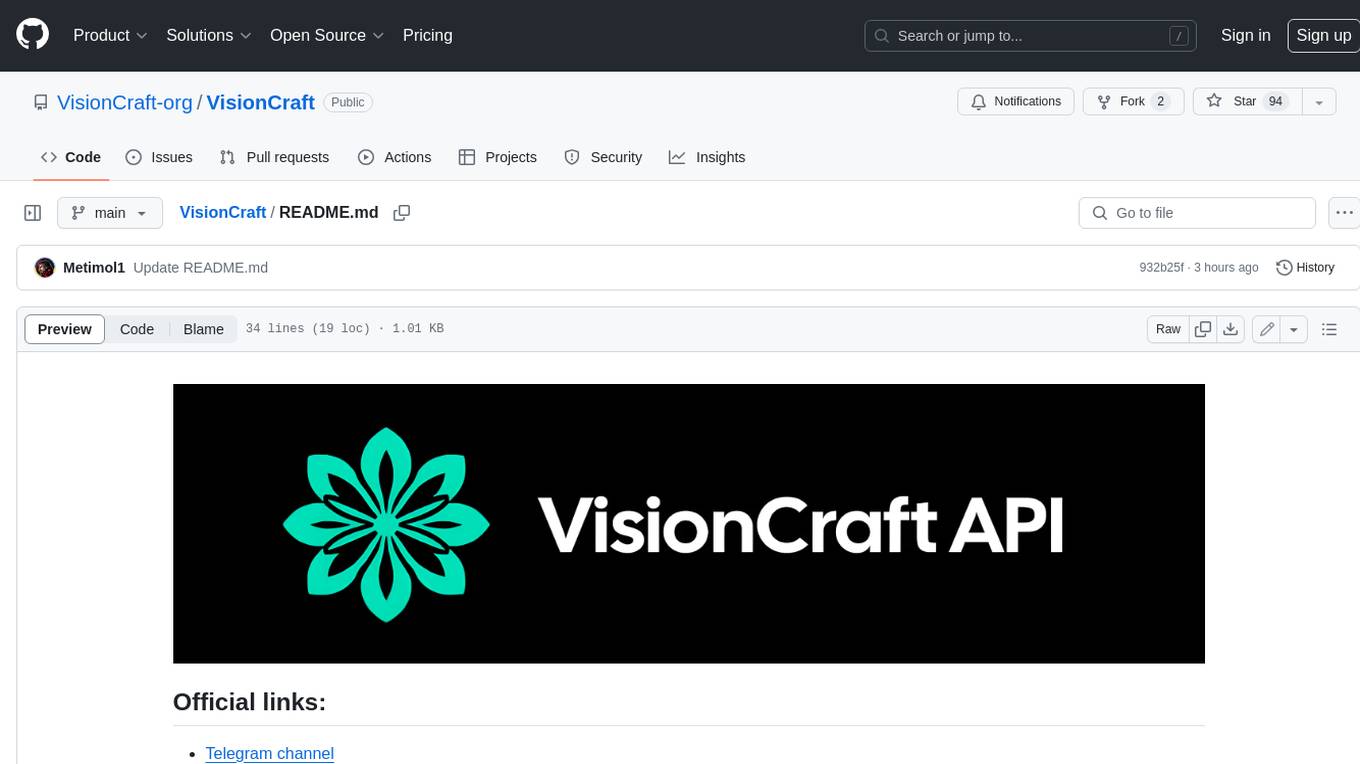
VisionCraft
The VisionCraft API is a free API for using over 100 different AI models. From images to sound.
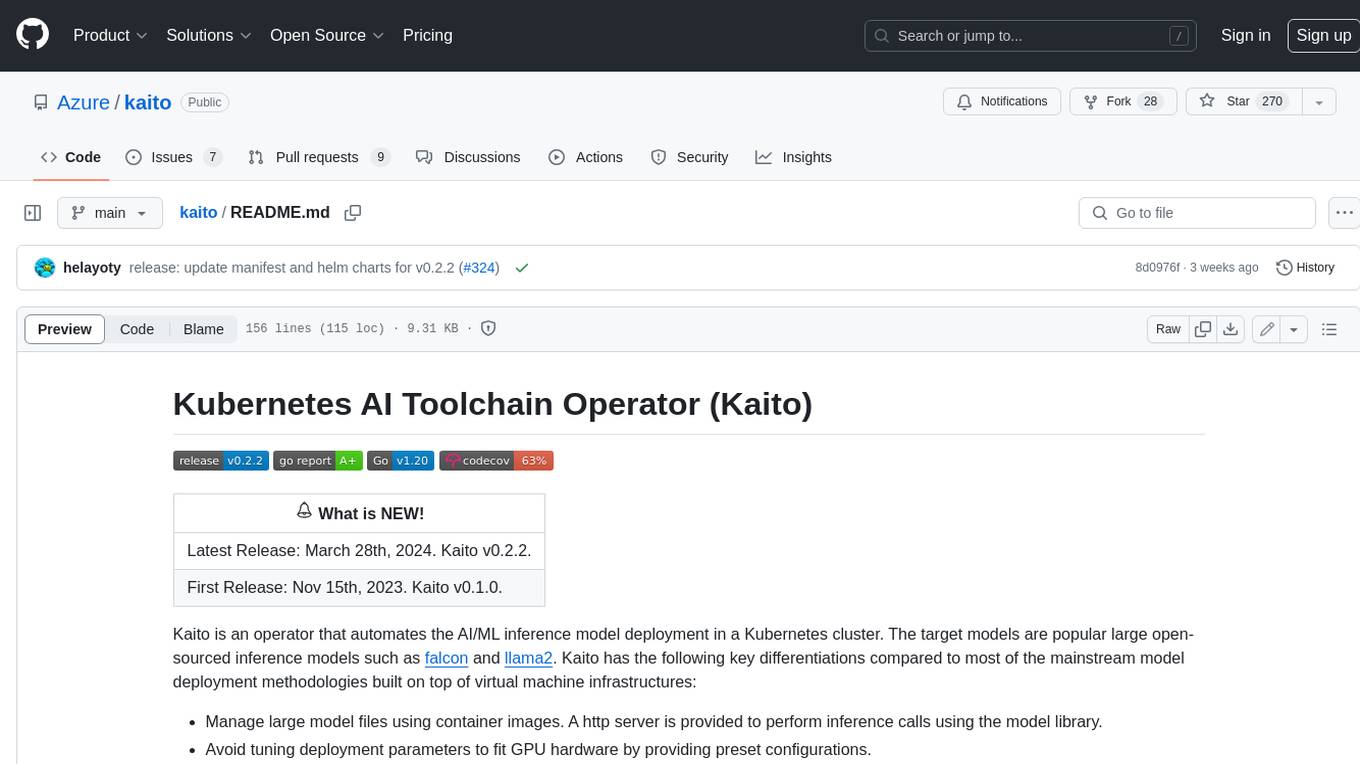
kaito
Kaito is an operator that automates the AI/ML inference model deployment in a Kubernetes cluster. It manages large model files using container images, avoids tuning deployment parameters to fit GPU hardware by providing preset configurations, auto-provisions GPU nodes based on model requirements, and hosts large model images in the public Microsoft Container Registry (MCR) if the license allows. Using Kaito, the workflow of onboarding large AI inference models in Kubernetes is largely simplified.
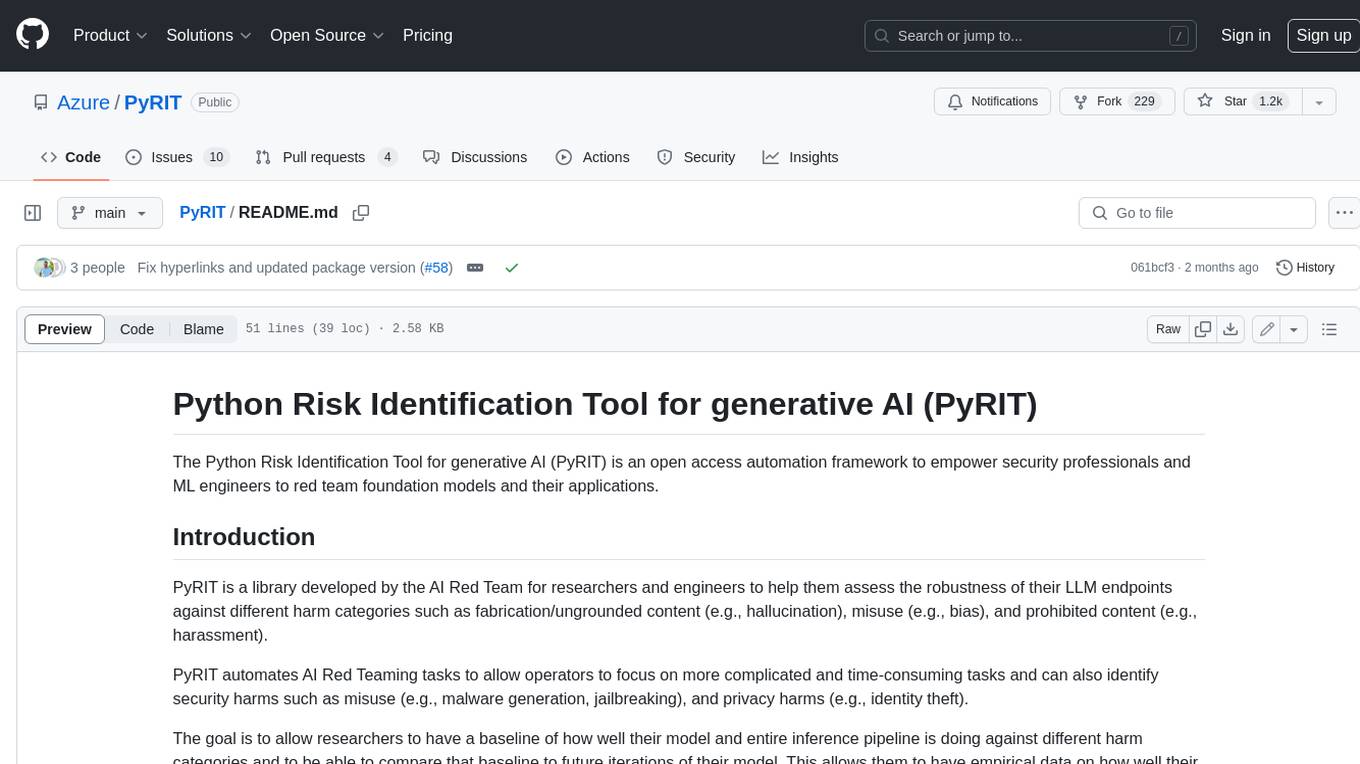
PyRIT
PyRIT is an open access automation framework designed to empower security professionals and ML engineers to red team foundation models and their applications. It automates AI Red Teaming tasks to allow operators to focus on more complicated and time-consuming tasks and can also identify security harms such as misuse (e.g., malware generation, jailbreaking), and privacy harms (e.g., identity theft). The goal is to allow researchers to have a baseline of how well their model and entire inference pipeline is doing against different harm categories and to be able to compare that baseline to future iterations of their model. This allows them to have empirical data on how well their model is doing today, and detect any degradation of performance based on future improvements.
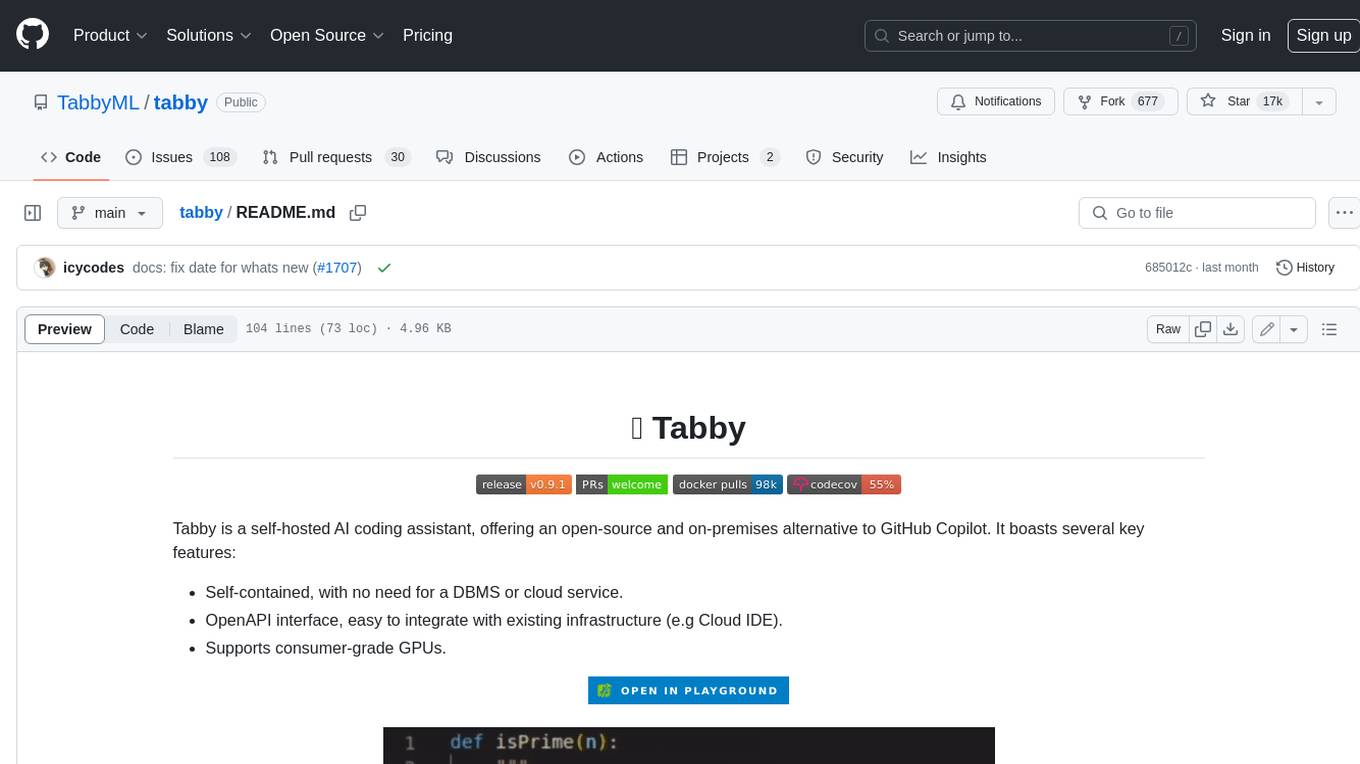
tabby
Tabby is a self-hosted AI coding assistant, offering an open-source and on-premises alternative to GitHub Copilot. It boasts several key features: * Self-contained, with no need for a DBMS or cloud service. * OpenAPI interface, easy to integrate with existing infrastructure (e.g Cloud IDE). * Supports consumer-grade GPUs.
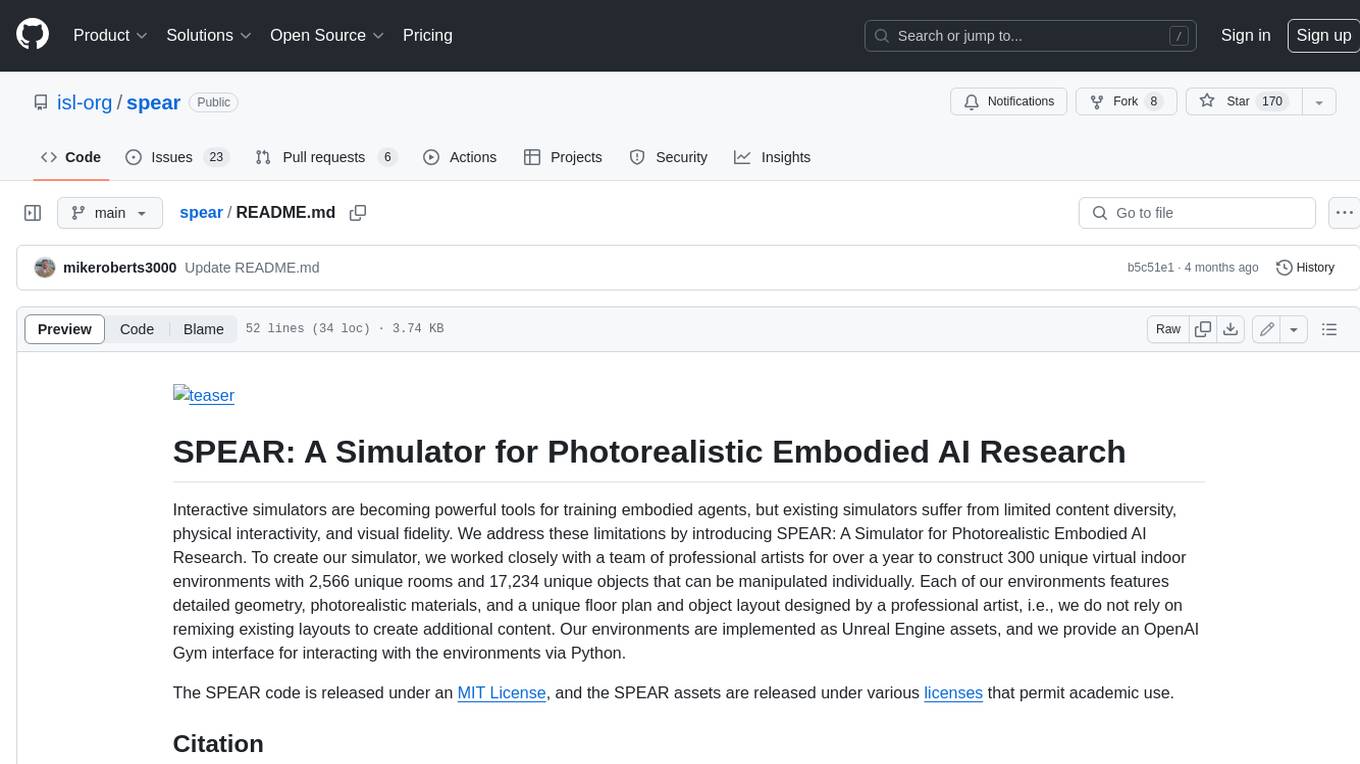
spear
SPEAR (Simulator for Photorealistic Embodied AI Research) is a powerful tool for training embodied agents. It features 300 unique virtual indoor environments with 2,566 unique rooms and 17,234 unique objects that can be manipulated individually. Each environment is designed by a professional artist and features detailed geometry, photorealistic materials, and a unique floor plan and object layout. SPEAR is implemented as Unreal Engine assets and provides an OpenAI Gym interface for interacting with the environments via Python.
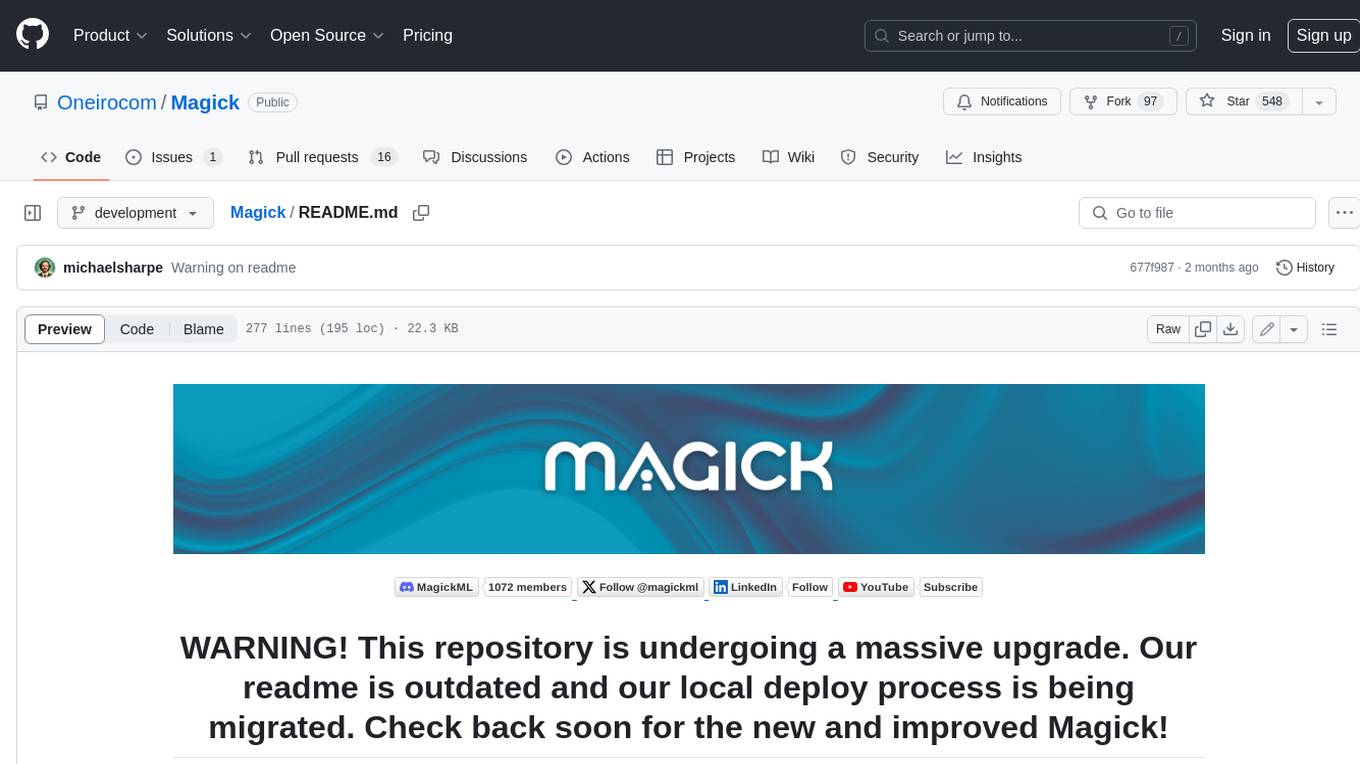
Magick
Magick is a groundbreaking visual AIDE (Artificial Intelligence Development Environment) for no-code data pipelines and multimodal agents. Magick can connect to other services and comes with nodes and templates well-suited for intelligent agents, chatbots, complex reasoning systems and realistic characters.