Best AI tools for< Deduplicate Documents >
10 - AI tool Sites
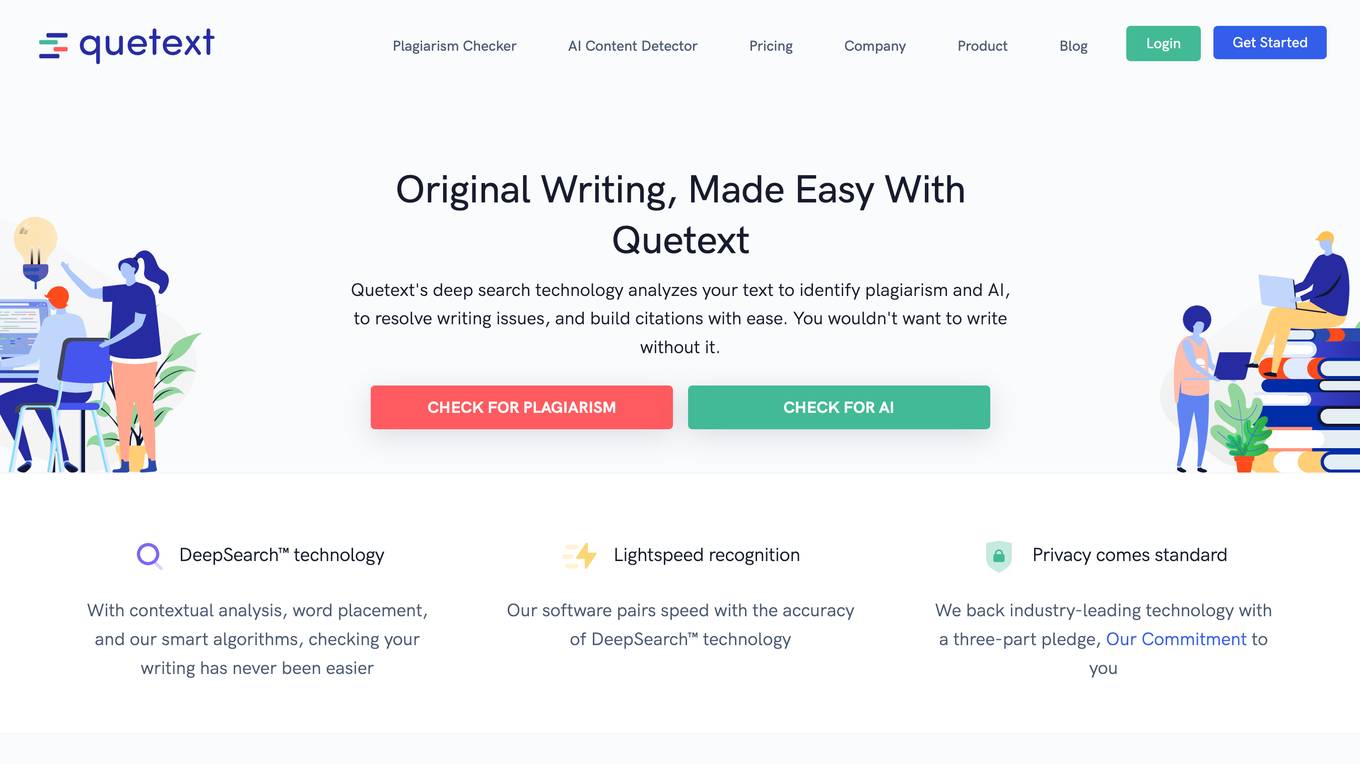
Quetext
Quetext is a plagiarism checker and AI content detector that helps students, teachers, and professionals identify potential plagiarism and AI in their work. With its deep search technology, contextual analysis, and smart algorithms, Quetext makes checking writing easier and more accurate. Quetext also offers a variety of features such as bulk uploads, source exclusion, enhanced citation generator, grammar & spell check, and Deep Search. With its rich and intuitive feedback, Quetext helps users find plagiarism and AI with less stress.
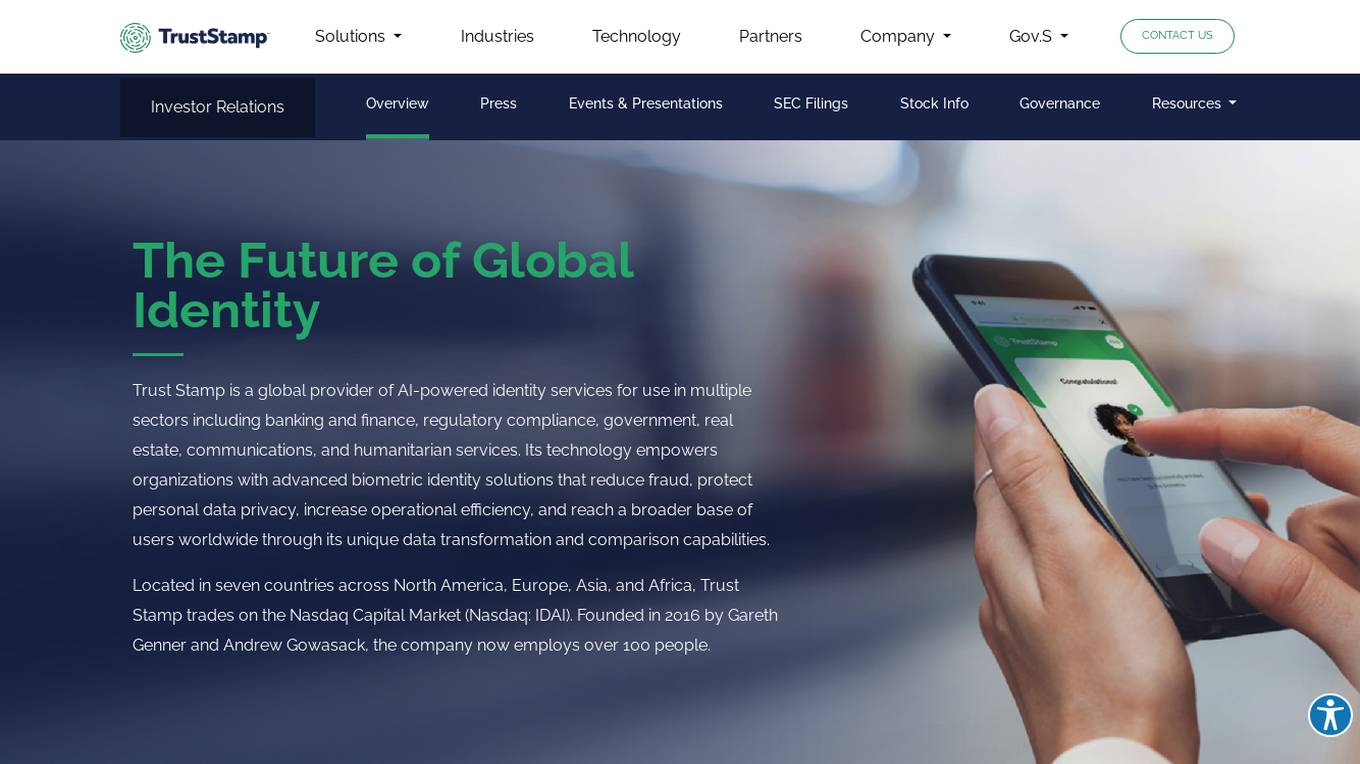
Trust Stamp
Trust Stamp is a global provider of AI-powered identity services offering a full suite of identity tools, including biometric multi-factor authentication, document validation, identity validation, duplicate detection, and geolocation services. The application is designed to empower organizations across various sectors with advanced biometric identity solutions to reduce fraud, protect personal data privacy, increase operational efficiency, and reach a broader user base worldwide through unique data transformation and comparison capabilities. Founded in 2016, Trust Stamp has achieved significant milestones in net sales, gross profit, and strategic partnerships, positioning itself as a leader in the identity verification industry.
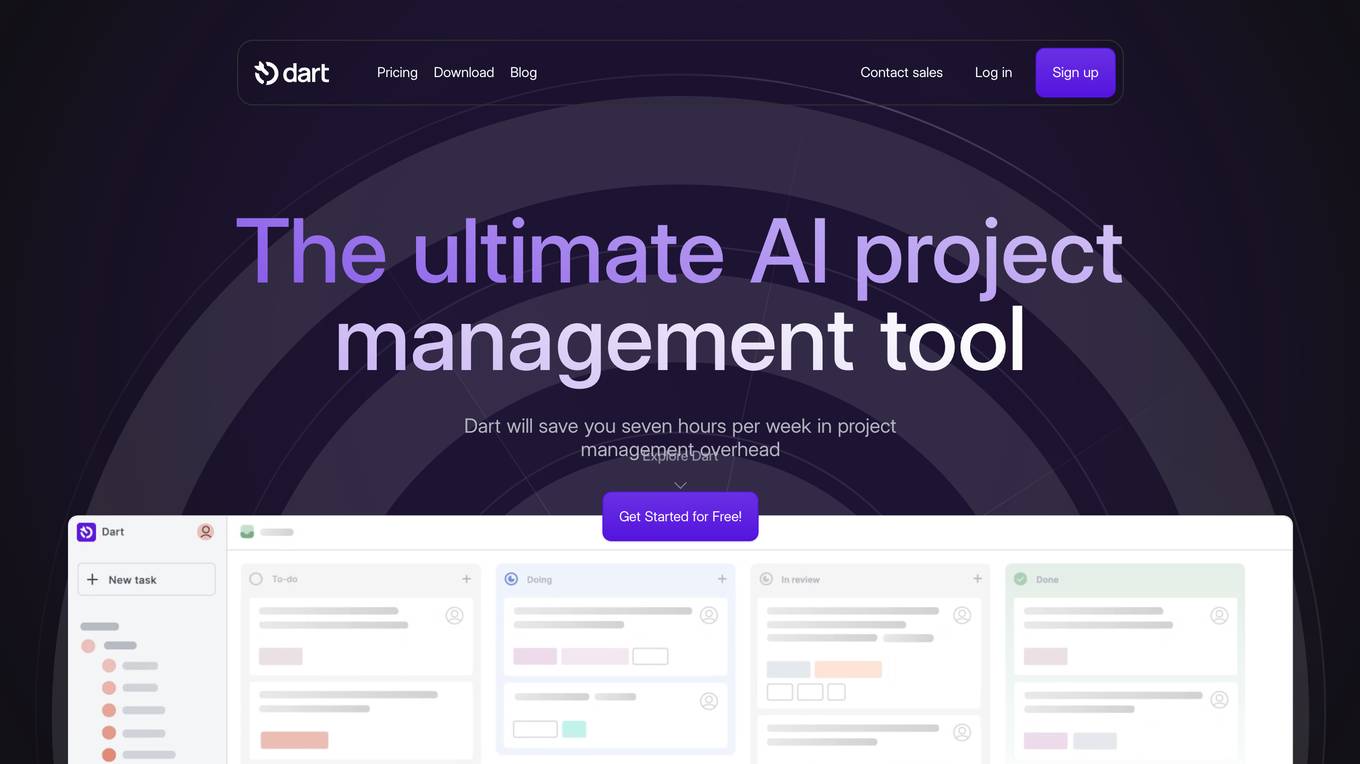
Dart
Dart is an AI project management software designed to empower teams to work smarter, streamline tasks, and achieve more with less effort. It offers features like task execution, subtask generation, project planning, duplicate detection, roadmaps, calendar views, document management, integrations with workplace tools, and more. Dart is used by teams across various roles in organizations to drive focus, innovation, and impact. The tool's AI capabilities automate routine tasks, enhance project reporting, and provide actionable insights for better decision-making.
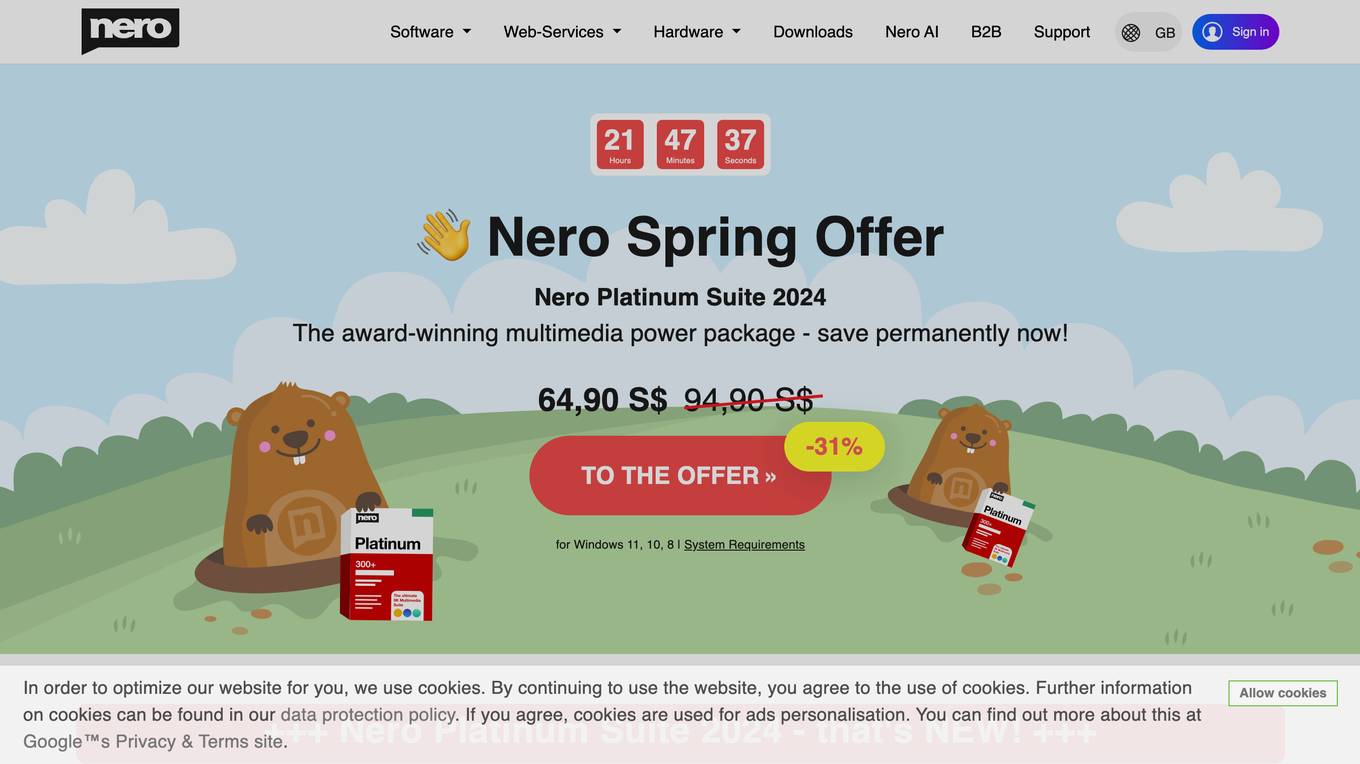
Nero Platinum Suite
Nero Platinum Suite is a comprehensive software collection for Windows PCs that provides a wide range of multimedia capabilities, including burning, managing, optimizing, and editing photos, videos, and music files. It includes various AI-powered features such as the Nero AI Image Upscaler, Nero AI Video Upscaler, and Nero AI Photo Tagger, which enhance and simplify multimedia tasks.
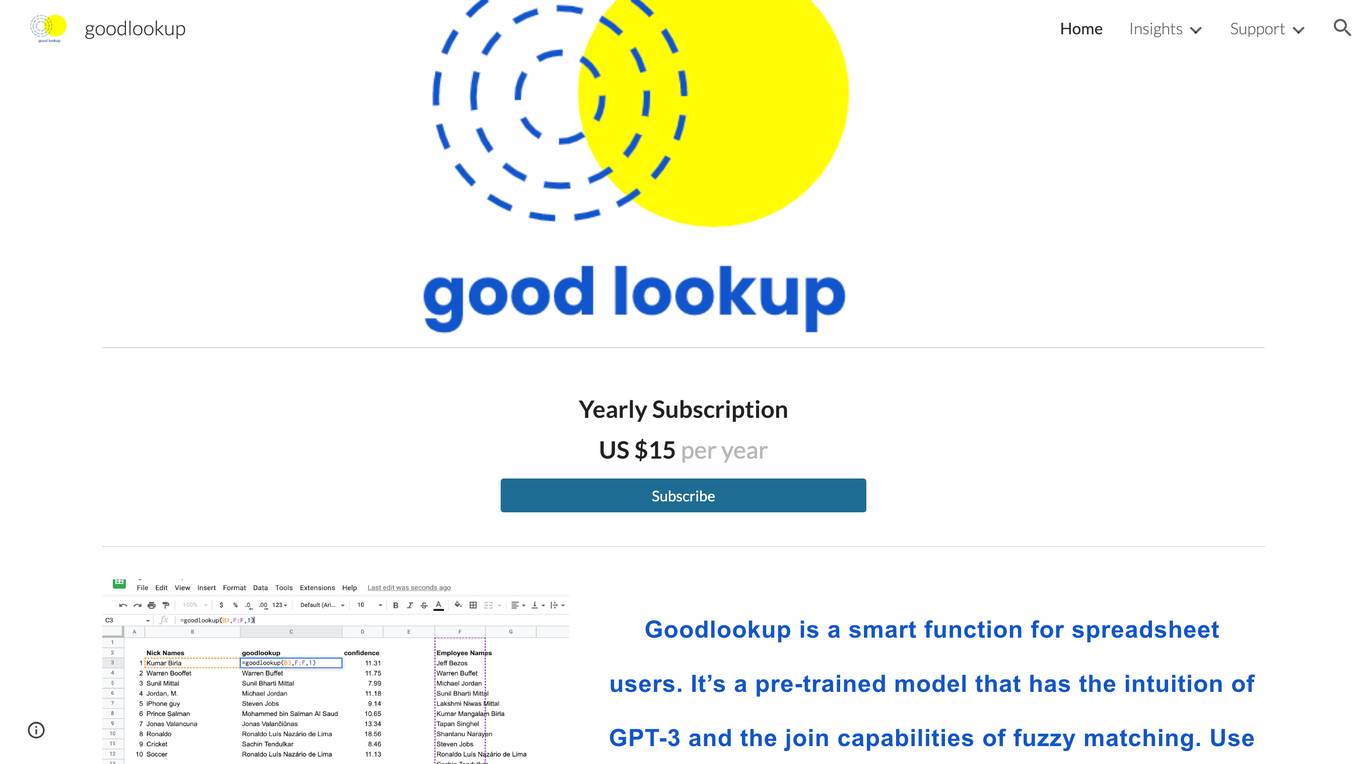
Goodlookup
Goodlookup is a smart function for spreadsheet users that gets very close to semantic understanding. It’s a pre-trained model that has the intuition of GPT-3 and the join capabilities of fuzzy matching. Use it like vlookup or index match to speed up your topic clustering work in google sheets!
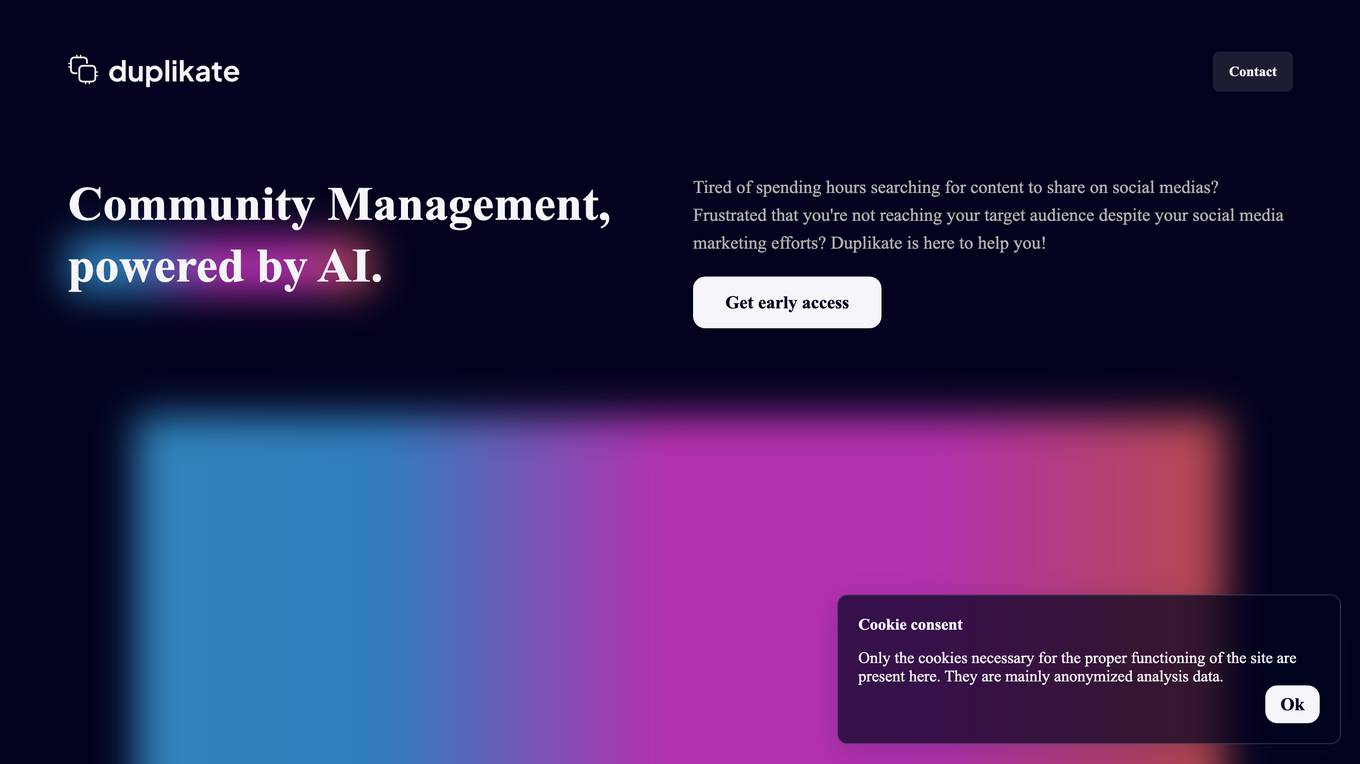
Duplikate
Duplikate is a next-generation AI-powered Community Management tool designed to assist users in managing their social media accounts more efficiently. It helps users save time by retrieving relevant social media posts, categorizing them, and duplicating them with modifications to better suit their audience. The tool is powered by OpenAI and offers features such as post scraping, filtering, and copying, with upcoming features including image generation. Users have praised Duplikate for its ability to streamline content creation, improve engagement, and save time in managing social media accounts.
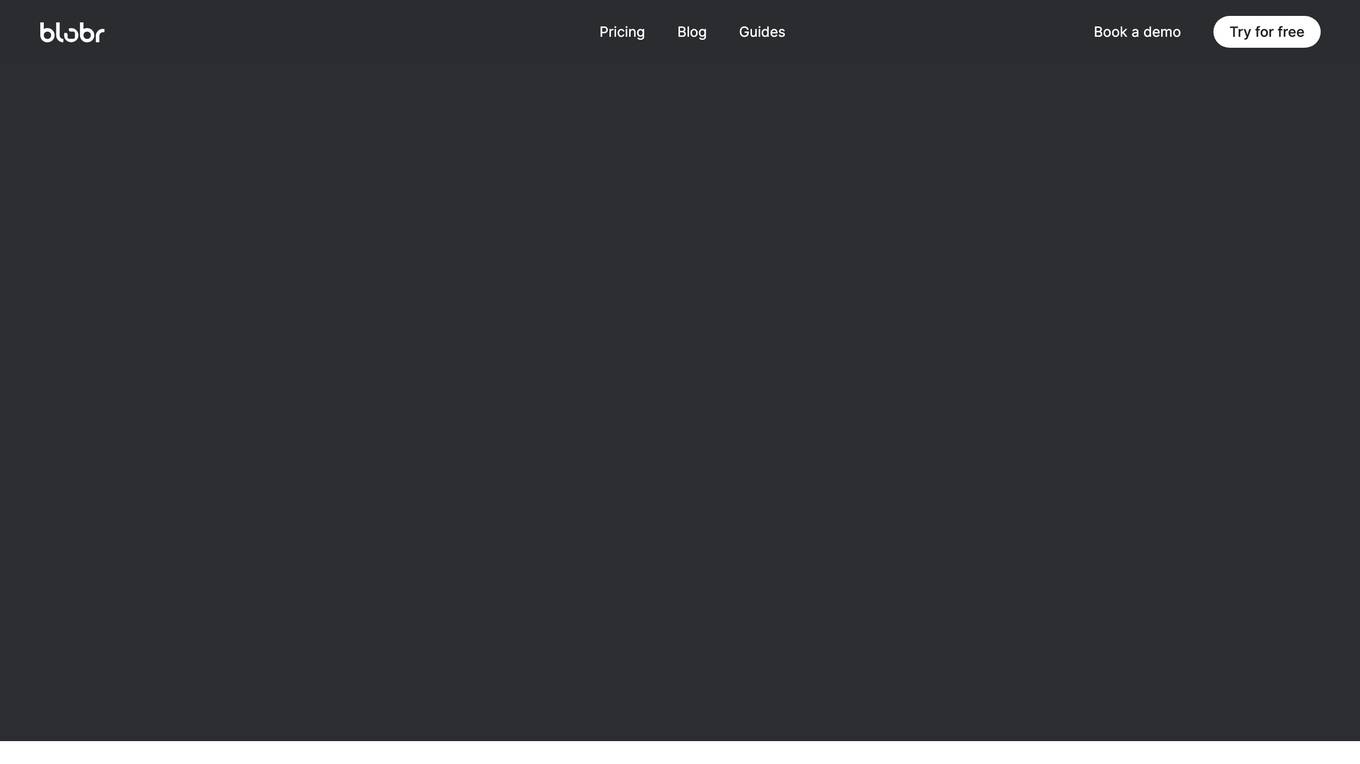
Blobr
Blobr is an AI tool designed to optimize Google Ads spending by providing AI agents that can reduce costs from 10% to 20%. The tool allows users to stay in control and validate changes while offering features such as Negative and Duplicate Keywords Finder, Ad Copy Optimizer, CPC Bid Optimizer, Location, Device & Timing Bid Optimizer, and Keyword Ideas Finder. Blobr helps users maximize every advertising dollar by automating keyword identification, optimizing bids, and improving ad quality scores. It saves time by freeing up teams from repetitive tasks and allows for continuous experimentation with control. Trusted by industry leaders, Blobr's AI agents work in real-time to provide recommendations and insights for better campaign performance.
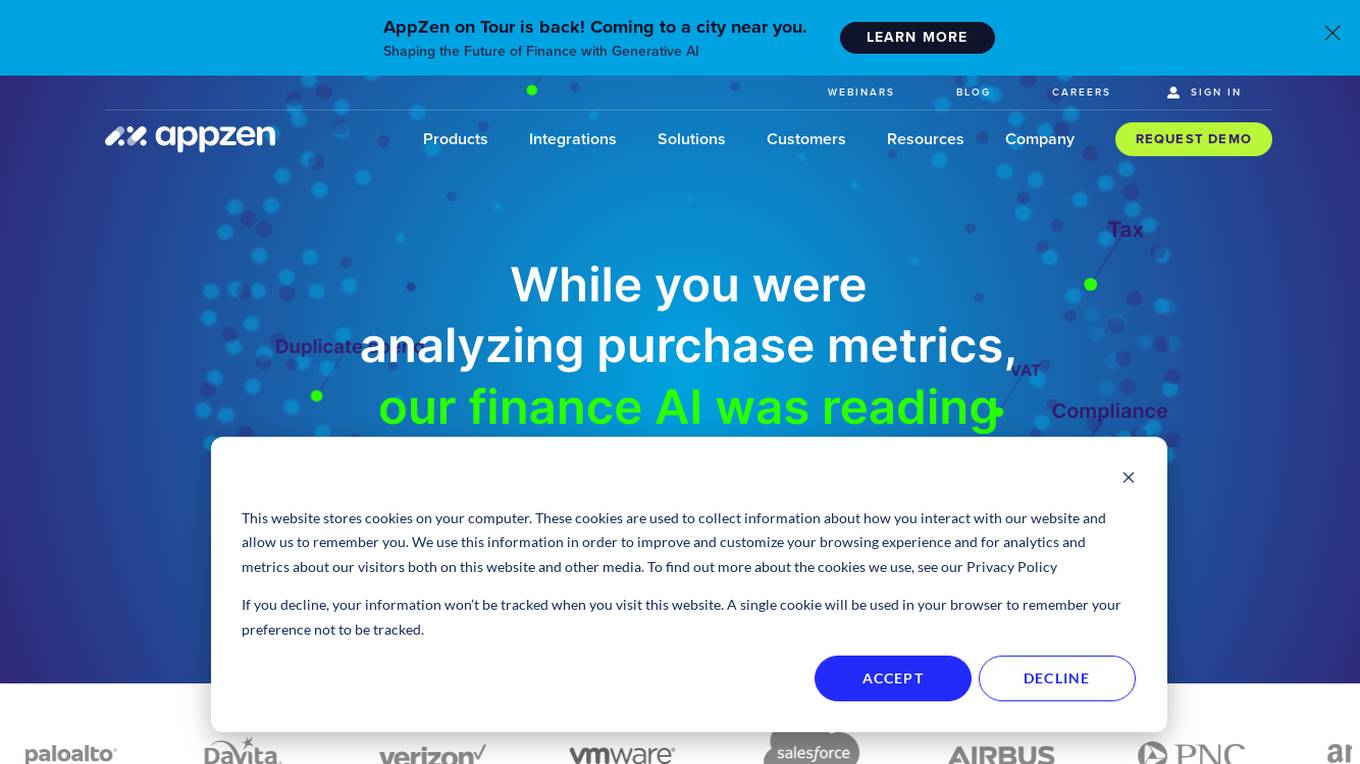
AppZen
AppZen is an AI-powered application designed for modern finance teams to streamline accounts payable processes, automate invoice and expense auditing, and improve compliance. It offers features such as Autonomous AP for invoice automation, Expense Audit for T&E spend management, and Card Audit for analyzing card spend. AppZen's AI learns and understands business practices, ensures compliance, and integrates with existing systems easily. The application helps prevent duplicate spend, fraud, and FCPA violations, making it a valuable tool for finance professionals.
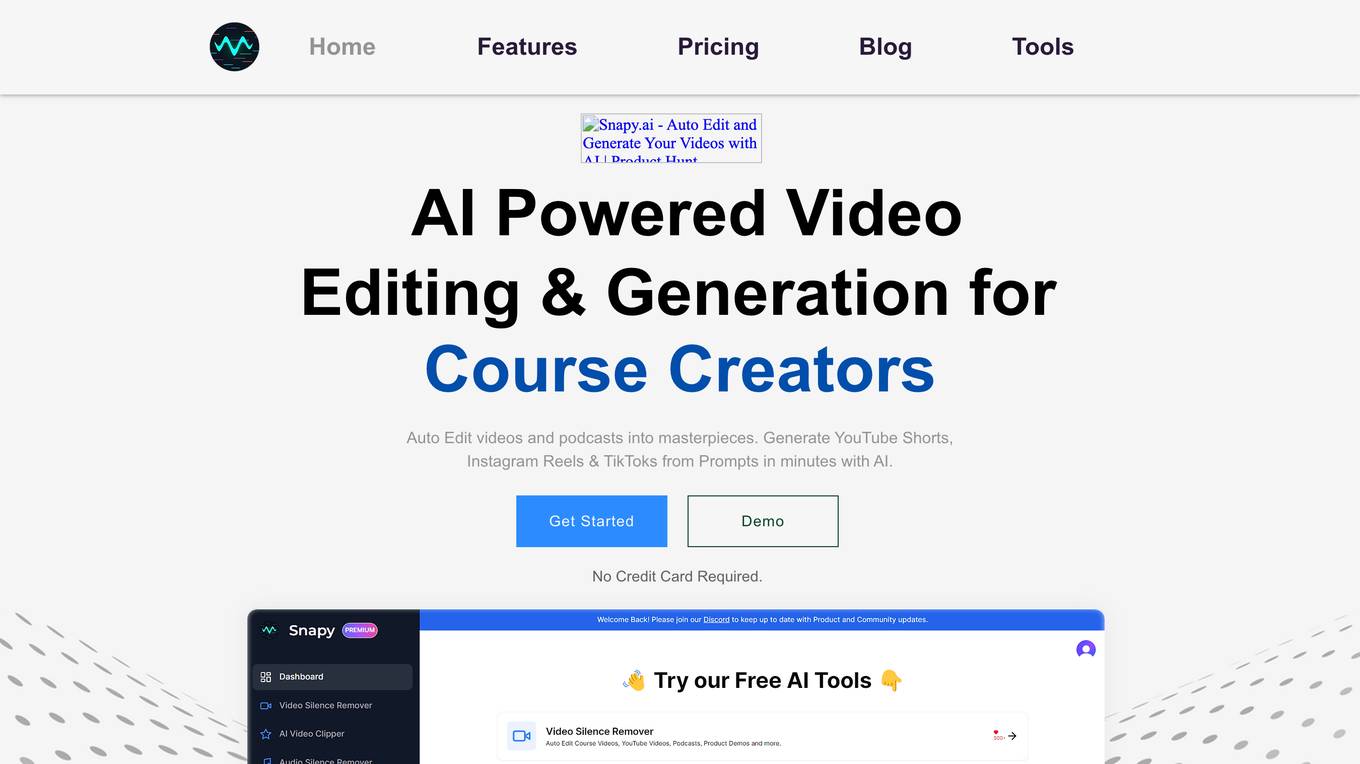
Snapy
Snapy is an AI-powered video editing and generation tool that helps content creators create short videos, edit podcasts, and remove silent parts from videos. It offers a range of features such as turning text prompts into short videos, condensing long videos into engaging short clips, automatically removing silent parts from audio files, and auto-trimming, removing duplicate sentences and filler words, and adding subtitles to short videos. Snapy is designed to save time and effort for content creators, allowing them to publish more content, create more engaging videos, and improve the quality of their audio and video content.
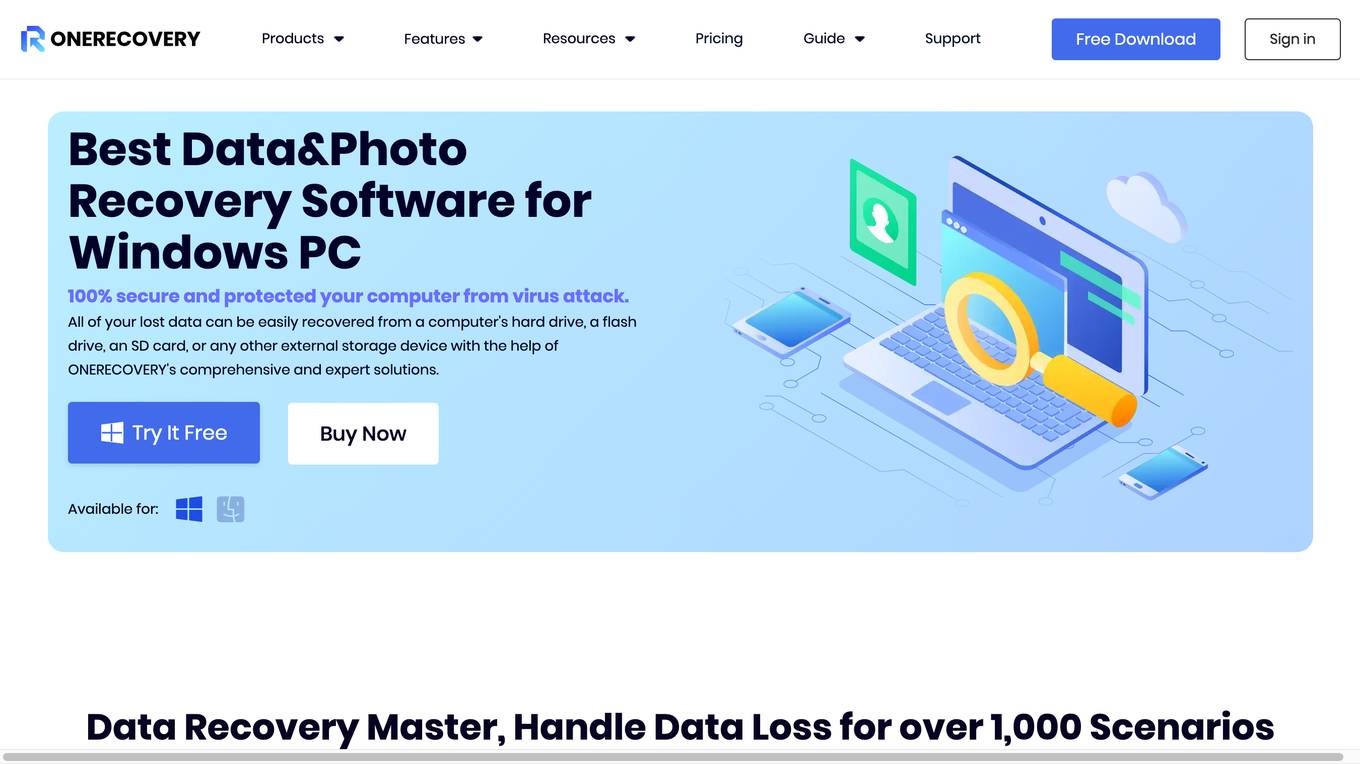
ONERECOVERY
ONERECOVERY is a professional data recovery solution for Windows that offers comprehensive and expert solutions to recover lost data from various storage devices. The software is designed to handle data loss for over 1,000 scenarios, including accidental deletion, formatting errors, virus attacks, and more. ONERECOVERY provides features such as file recovery for Windows and Mac, file duplicate finder, photo and video recovery, hard drive recovery, SD card data recovery, and more. With a user-friendly interface, quick and efficient scanning, and compatibility with diverse operating systems and storage devices, ONERECOVERY is a reliable and secure data recovery tool trusted by millions of users worldwide.
20 - Open Source AI Tools
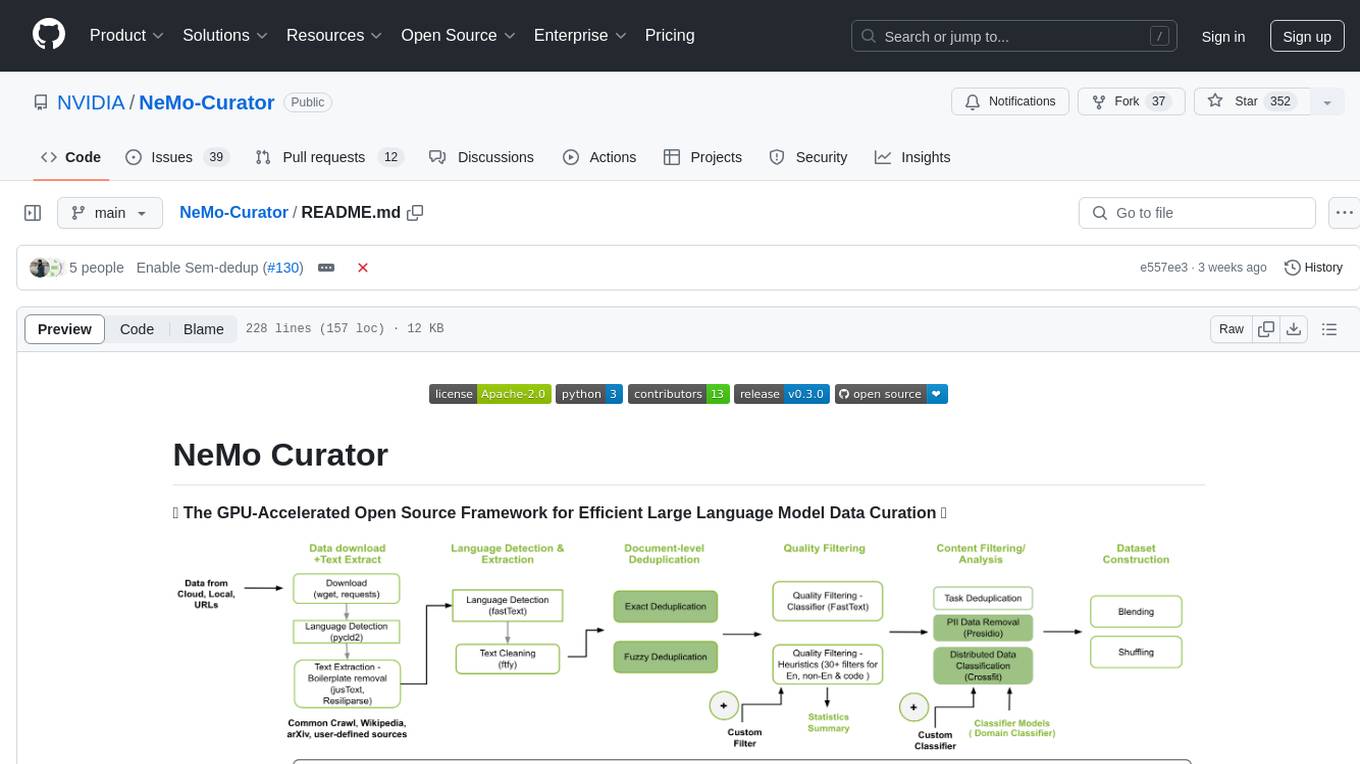
NeMo-Curator
NeMo Curator is a GPU-accelerated open-source framework designed for efficient large language model data curation. It provides scalable dataset preparation for tasks like foundation model pretraining, domain-adaptive pretraining, supervised fine-tuning, and parameter-efficient fine-tuning. The library leverages GPUs with Dask and RAPIDS to accelerate data curation, offering customizable and modular interfaces for pipeline expansion and model convergence. Key features include data download, text extraction, quality filtering, deduplication, downstream-task decontamination, distributed data classification, and PII redaction. NeMo Curator is suitable for curating high-quality datasets for large language model training.
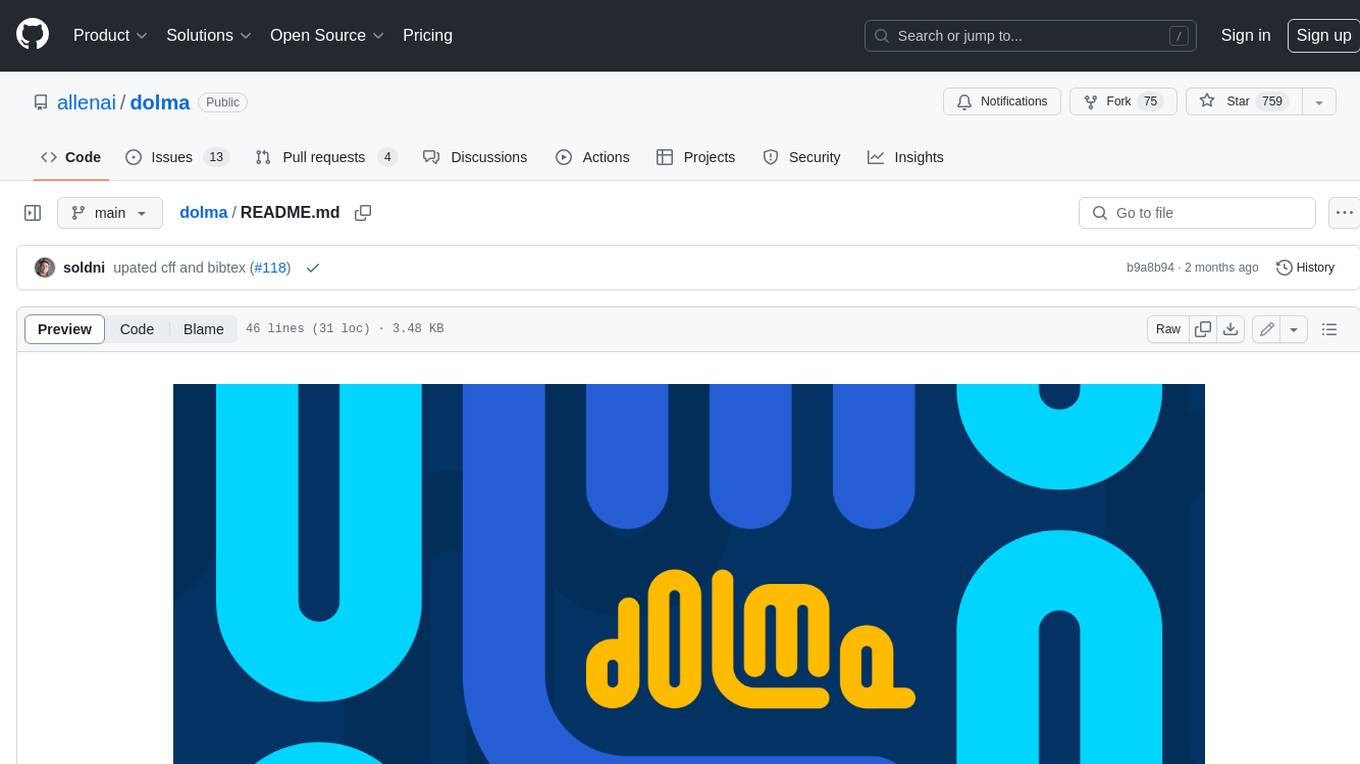
dolma
Dolma is a dataset and toolkit for curating large datasets for (pre)-training ML models. The dataset consists of 3 trillion tokens from a diverse mix of web content, academic publications, code, books, and encyclopedic materials. The toolkit provides high-performance, portable, and extensible tools for processing, tagging, and deduplicating documents. Key features of the toolkit include built-in taggers, fast deduplication, and cloud support.
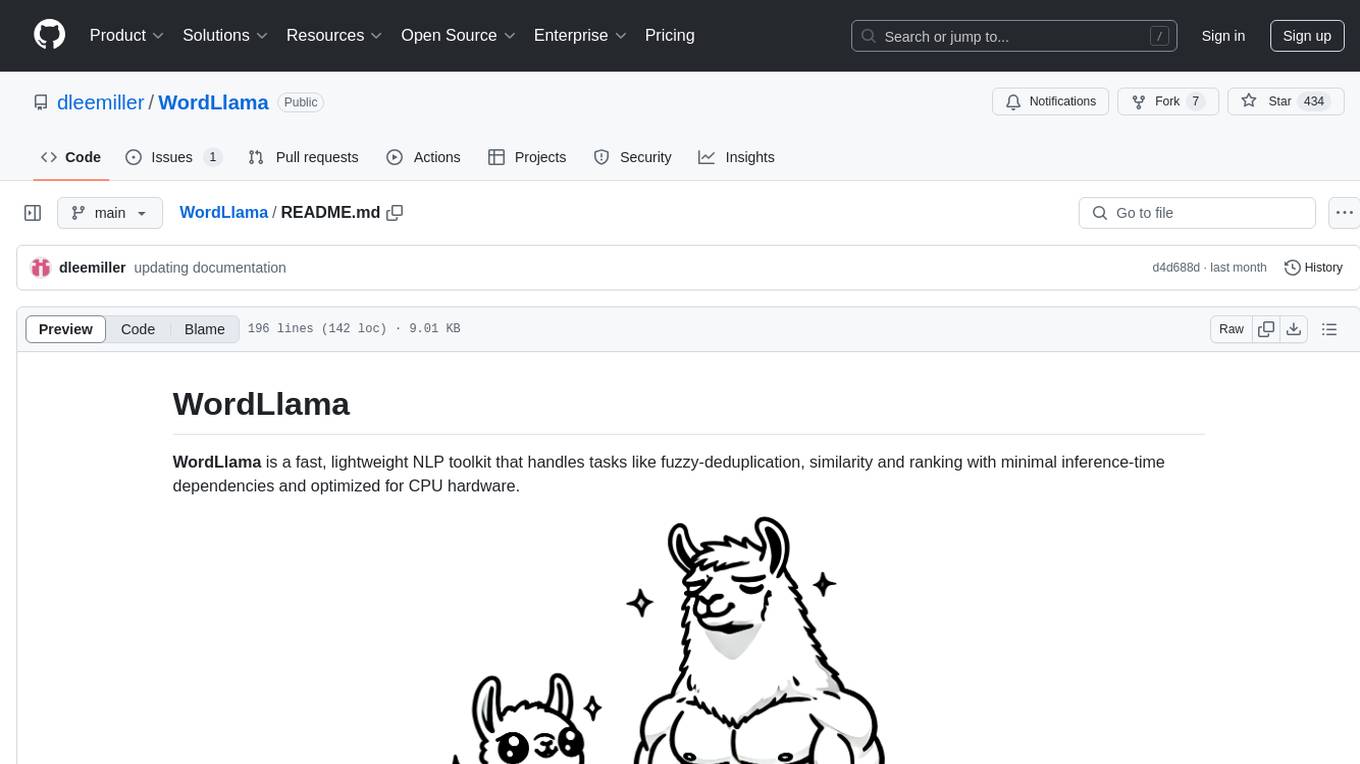
WordLlama
WordLlama is a fast, lightweight NLP toolkit optimized for CPU hardware. It recycles components from large language models to create efficient word representations. It offers features like Matryoshka Representations, low resource requirements, binarization, and numpy-only inference. The tool is suitable for tasks like semantic matching, fuzzy deduplication, ranking, and clustering, making it a good option for NLP-lite tasks and exploratory analysis.
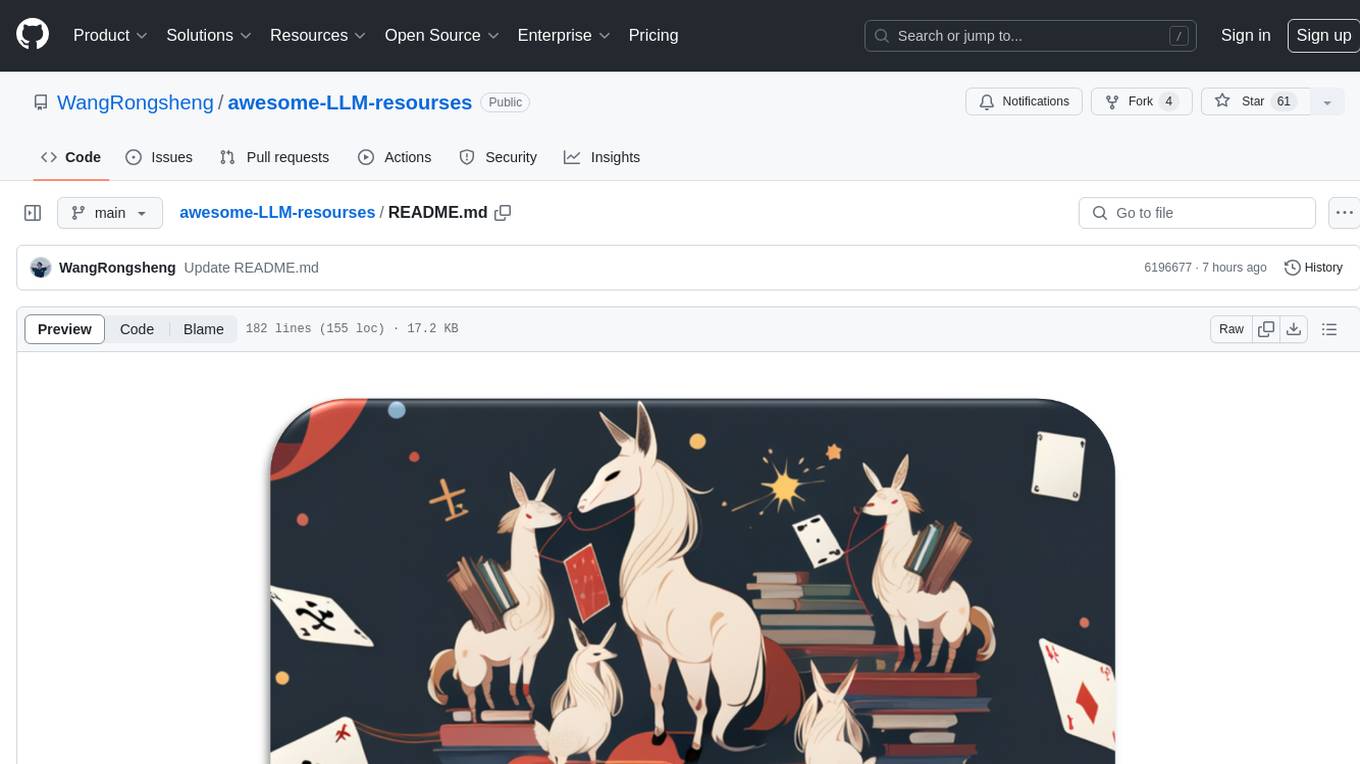
awesome-LLM-resourses
A comprehensive repository of resources for Chinese large language models (LLMs), including data processing tools, fine-tuning frameworks, inference libraries, evaluation platforms, RAG engines, agent frameworks, books, courses, tutorials, and tips. The repository covers a wide range of tools and resources for working with LLMs, from data labeling and processing to model fine-tuning, inference, evaluation, and application development. It also includes resources for learning about LLMs through books, courses, and tutorials, as well as insights and strategies from building with LLMs.
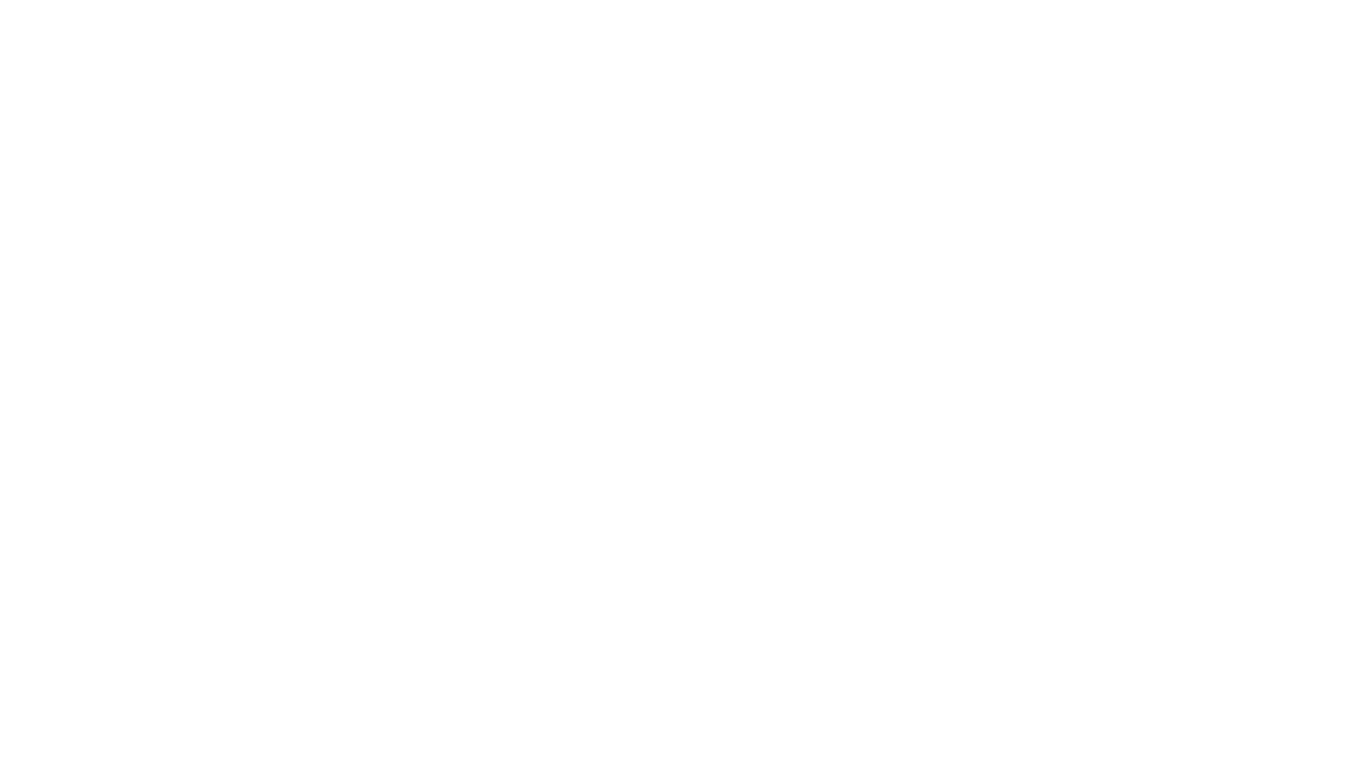
rag-experiment-accelerator
The RAG Experiment Accelerator is a versatile tool that helps you conduct experiments and evaluations using Azure AI Search and RAG pattern. It offers a rich set of features, including experiment setup, integration with Azure AI Search, Azure Machine Learning, MLFlow, and Azure OpenAI, multiple document chunking strategies, query generation, multiple search types, sub-querying, re-ranking, metrics and evaluation, report generation, and multi-lingual support. The tool is designed to make it easier and faster to run experiments and evaluations of search queries and quality of response from OpenAI, and is useful for researchers, data scientists, and developers who want to test the performance of different search and OpenAI related hyperparameters, compare the effectiveness of various search strategies, fine-tune and optimize parameters, find the best combination of hyperparameters, and generate detailed reports and visualizations from experiment results.
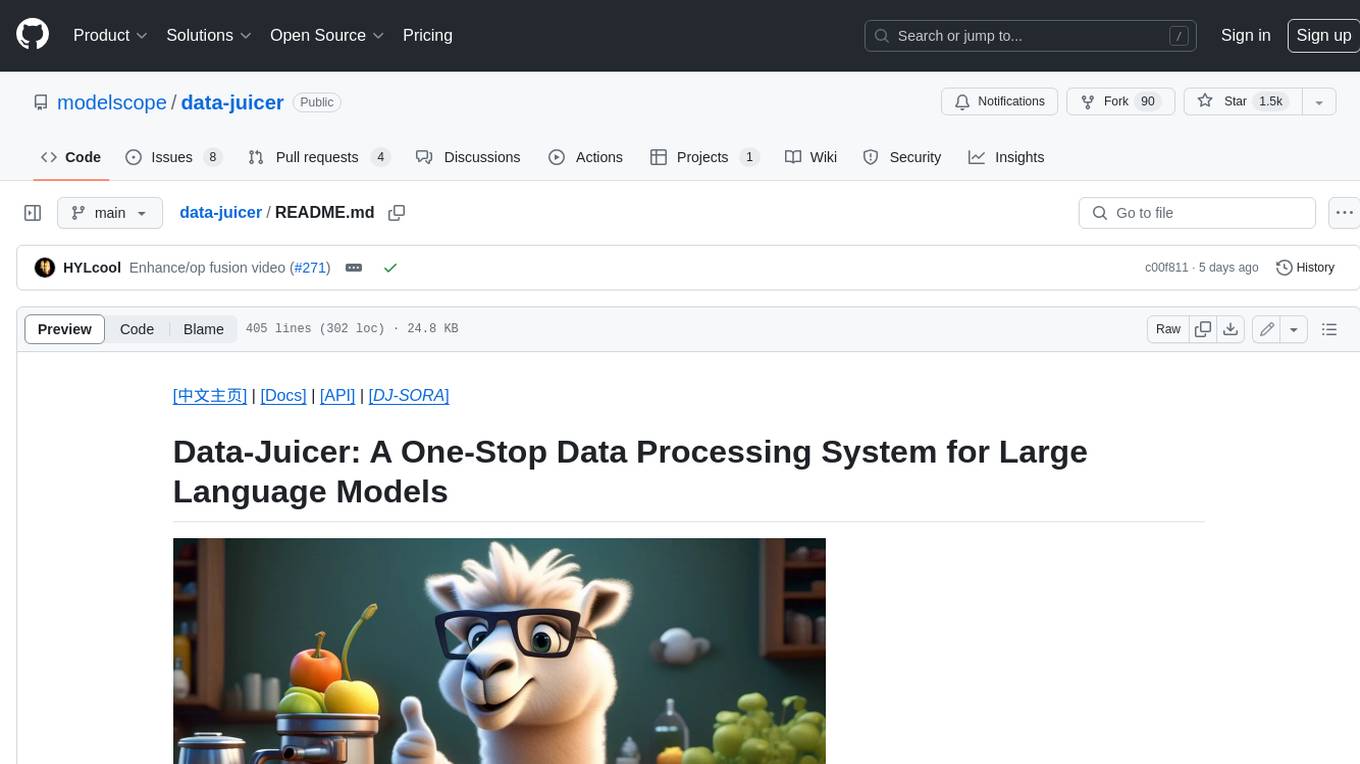
data-juicer
Data-Juicer is a one-stop data processing system to make data higher-quality, juicier, and more digestible for LLMs. It is a systematic & reusable library of 80+ core OPs, 20+ reusable config recipes, and 20+ feature-rich dedicated toolkits, designed to function independently of specific LLM datasets and processing pipelines. Data-Juicer allows detailed data analyses with an automated report generation feature for a deeper understanding of your dataset. Coupled with multi-dimension automatic evaluation capabilities, it supports a timely feedback loop at multiple stages in the LLM development process. Data-Juicer offers tens of pre-built data processing recipes for pre-training, fine-tuning, en, zh, and more scenarios. It provides a speedy data processing pipeline requiring less memory and CPU usage, optimized for maximum productivity. Data-Juicer is flexible & extensible, accommodating most types of data formats and allowing flexible combinations of OPs. It is designed for simplicity, with comprehensive documentation, easy start guides and demo configs, and intuitive configuration with simple adding/removing OPs from existing configs.
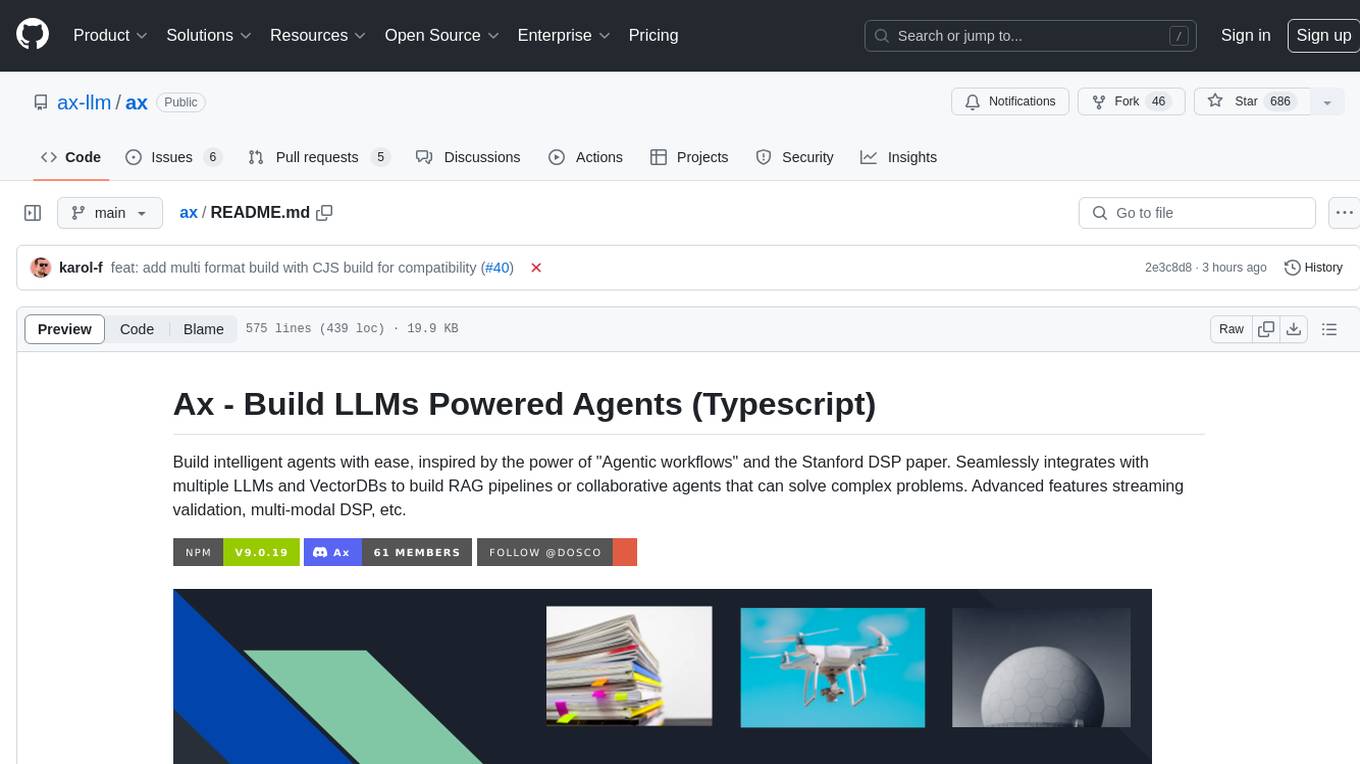
ax
Ax is a Typescript library that allows users to build intelligent agents inspired by agentic workflows and the Stanford DSP paper. It seamlessly integrates with multiple Large Language Models (LLMs) and VectorDBs to create RAG pipelines or collaborative agents capable of solving complex problems. The library offers advanced features such as streaming validation, multi-modal DSP, and automatic prompt tuning using optimizers. Users can easily convert documents of any format to text, perform smart chunking, embedding, and querying, and ensure output validation while streaming. Ax is production-ready, written in Typescript, and has zero dependencies.
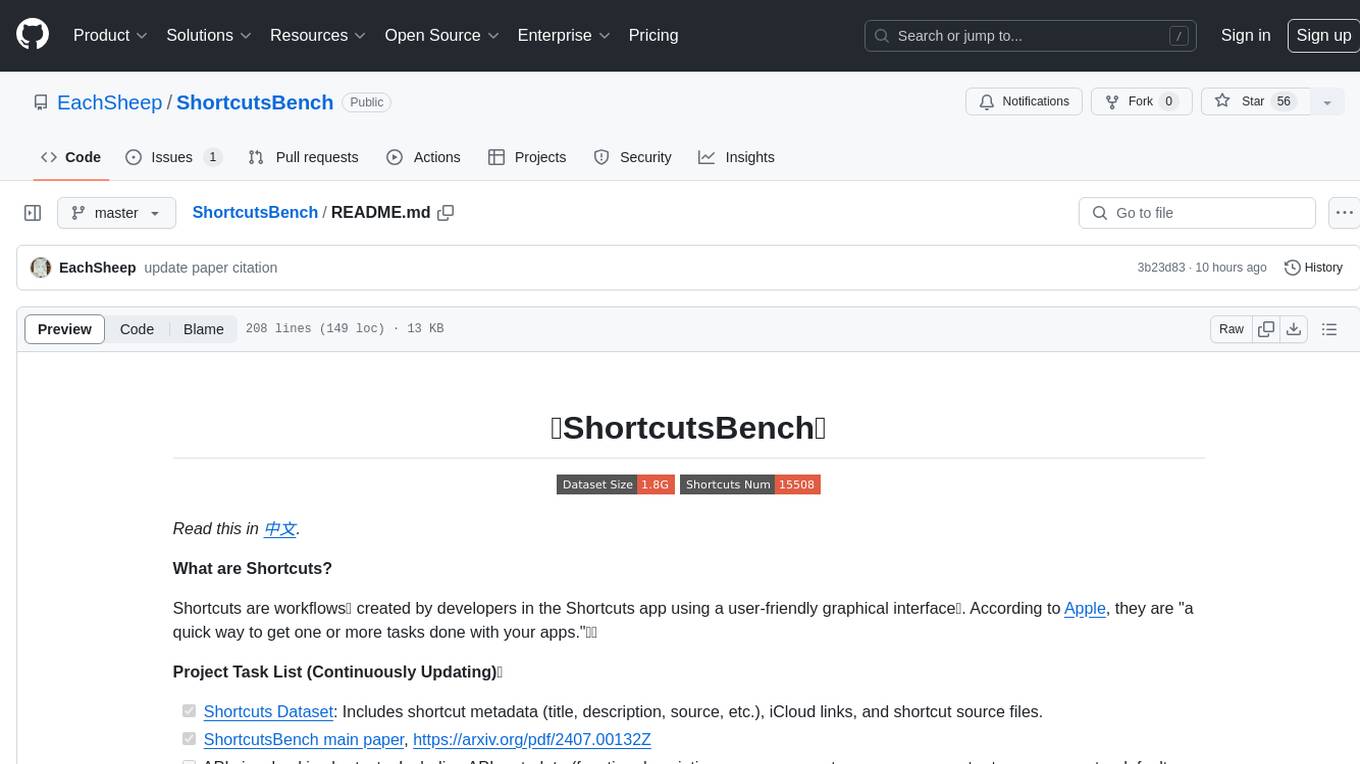
ShortcutsBench
ShortcutsBench is a project focused on collecting and analyzing workflows created in the Shortcuts app, providing a dataset of shortcut metadata, source files, and API information. It aims to study the integration of large language models with Apple devices, particularly focusing on the role of shortcuts in enhancing user experience. The project offers insights for Shortcuts users, enthusiasts, and researchers to explore, customize workflows, and study automated workflows, low-code programming, and API-based agents.
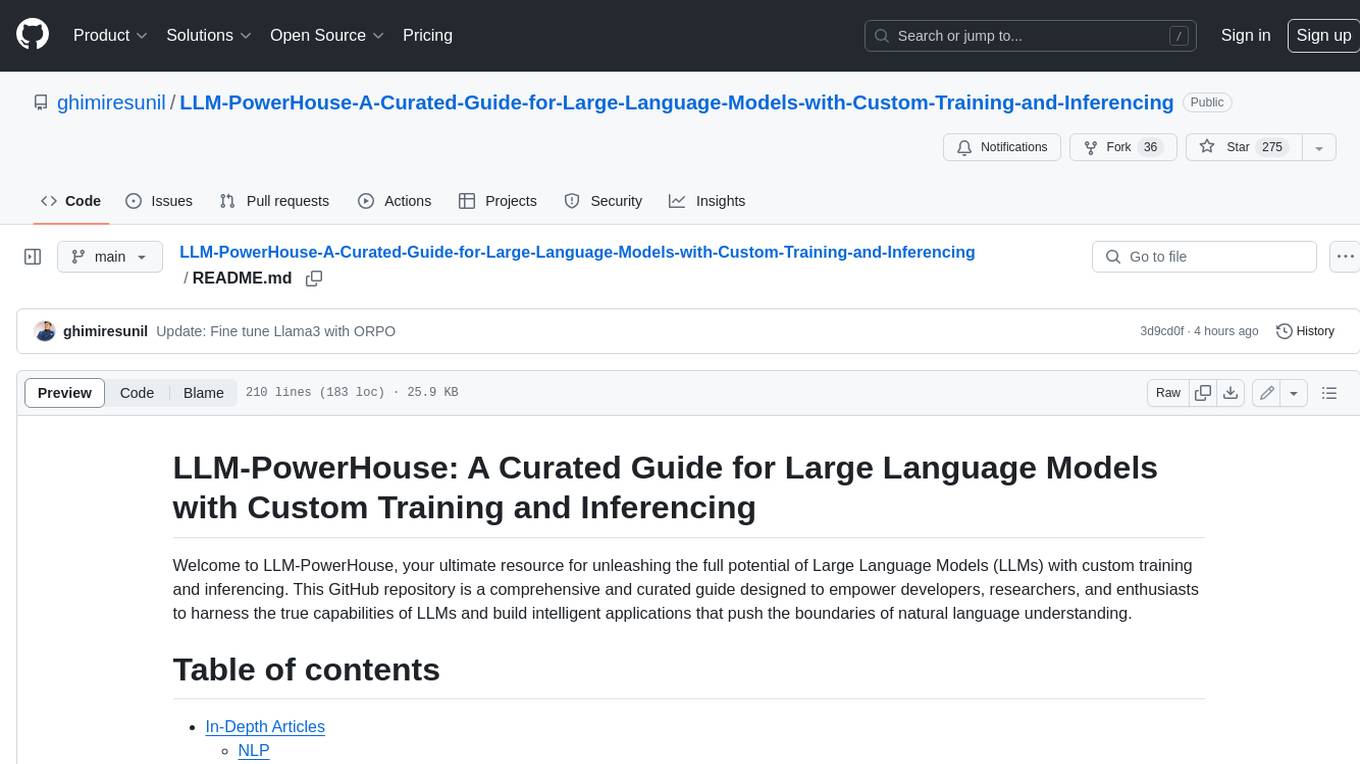
LLM-PowerHouse-A-Curated-Guide-for-Large-Language-Models-with-Custom-Training-and-Inferencing
LLM-PowerHouse is a comprehensive and curated guide designed to empower developers, researchers, and enthusiasts to harness the true capabilities of Large Language Models (LLMs) and build intelligent applications that push the boundaries of natural language understanding. This GitHub repository provides in-depth articles, codebase mastery, LLM PlayLab, and resources for cost analysis and network visualization. It covers various aspects of LLMs, including NLP, models, training, evaluation metrics, open LLMs, and more. The repository also includes a collection of code examples and tutorials to help users build and deploy LLM-based applications.
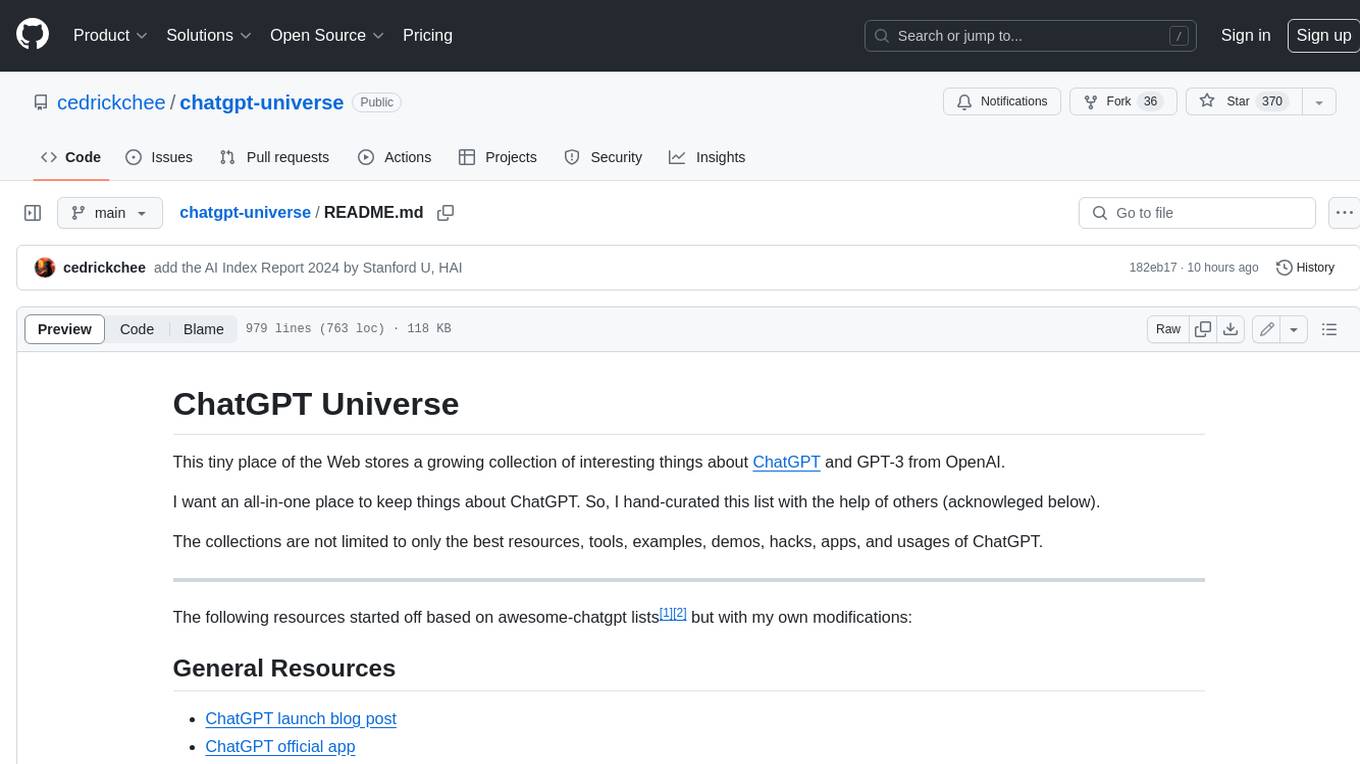
chatgpt-universe
ChatGPT is a large language model that can generate human-like text, translate languages, write different kinds of creative content, and answer your questions in a conversational way. It is trained on a massive amount of text data, and it is able to understand and respond to a wide range of natural language prompts. Here are 5 jobs suitable for this tool, in lowercase letters: 1. content writer 2. chatbot assistant 3. language translator 4. creative writer 5. researcher
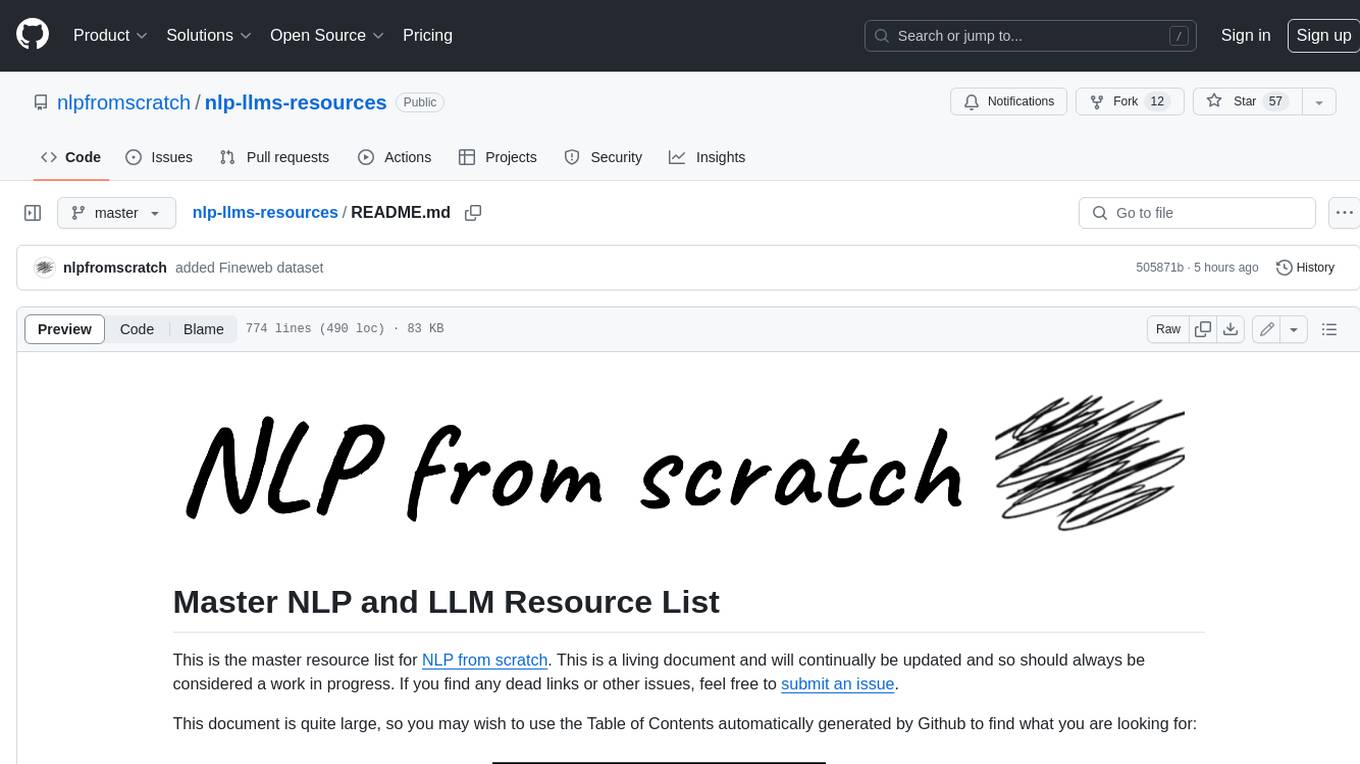
nlp-llms-resources
The 'nlp-llms-resources' repository is a comprehensive resource list for Natural Language Processing (NLP) and Large Language Models (LLMs). It covers a wide range of topics including traditional NLP datasets, data acquisition, libraries for NLP, neural networks, sentiment analysis, optical character recognition, information extraction, semantics, topic modeling, multilingual NLP, domain-specific LLMs, vector databases, ethics, costing, books, courses, surveys, aggregators, newsletters, papers, conferences, and societies. The repository provides valuable information and resources for individuals interested in NLP and LLMs.
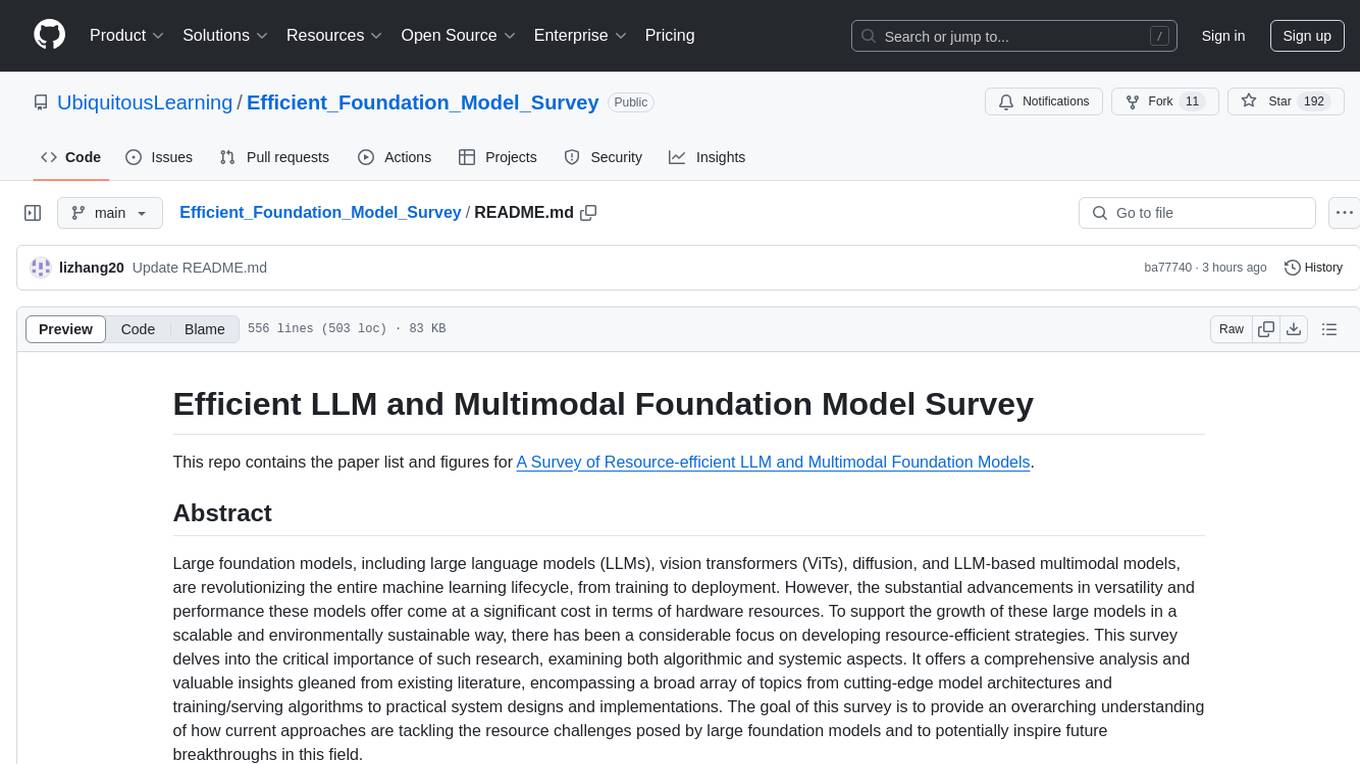
Efficient_Foundation_Model_Survey
Efficient Foundation Model Survey is a comprehensive analysis of resource-efficient large language models (LLMs) and multimodal foundation models. The survey covers algorithmic and systemic innovations to support the growth of large models in a scalable and environmentally sustainable way. It explores cutting-edge model architectures, training/serving algorithms, and practical system designs. The goal is to provide insights on tackling resource challenges posed by large foundation models and inspire future breakthroughs in the field.
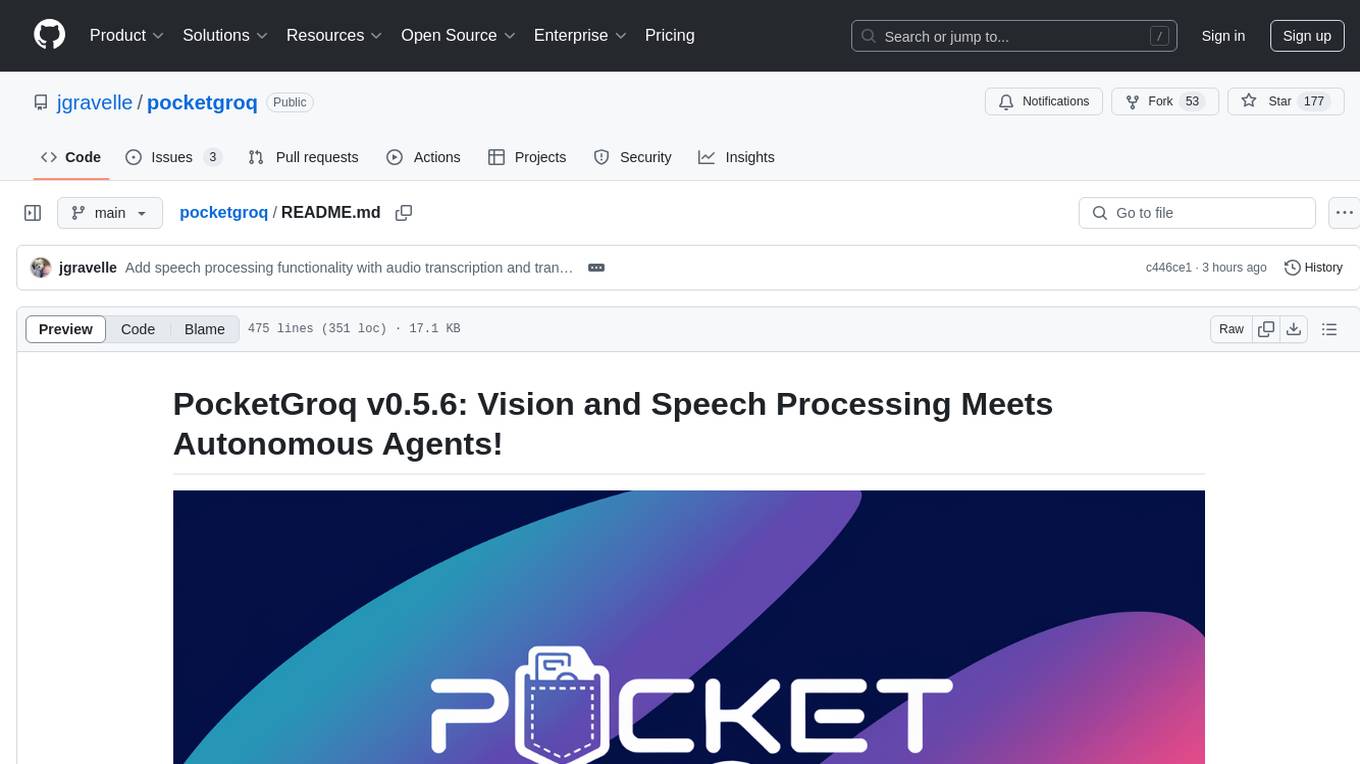
pocketgroq
PocketGroq is a tool that provides advanced functionalities for text generation, web scraping, web search, and AI response evaluation. It includes features like an Autonomous Agent for answering questions, web crawling and scraping capabilities, enhanced web search functionality, and flexible integration with Ollama server. Users can customize the agent's behavior, evaluate responses using AI, and utilize various methods for text generation, conversation management, and Chain of Thought reasoning. The tool offers comprehensive methods for different tasks, such as initializing RAG, error handling, and tool management. PocketGroq is designed to enhance development processes and enable the creation of AI-powered applications with ease.
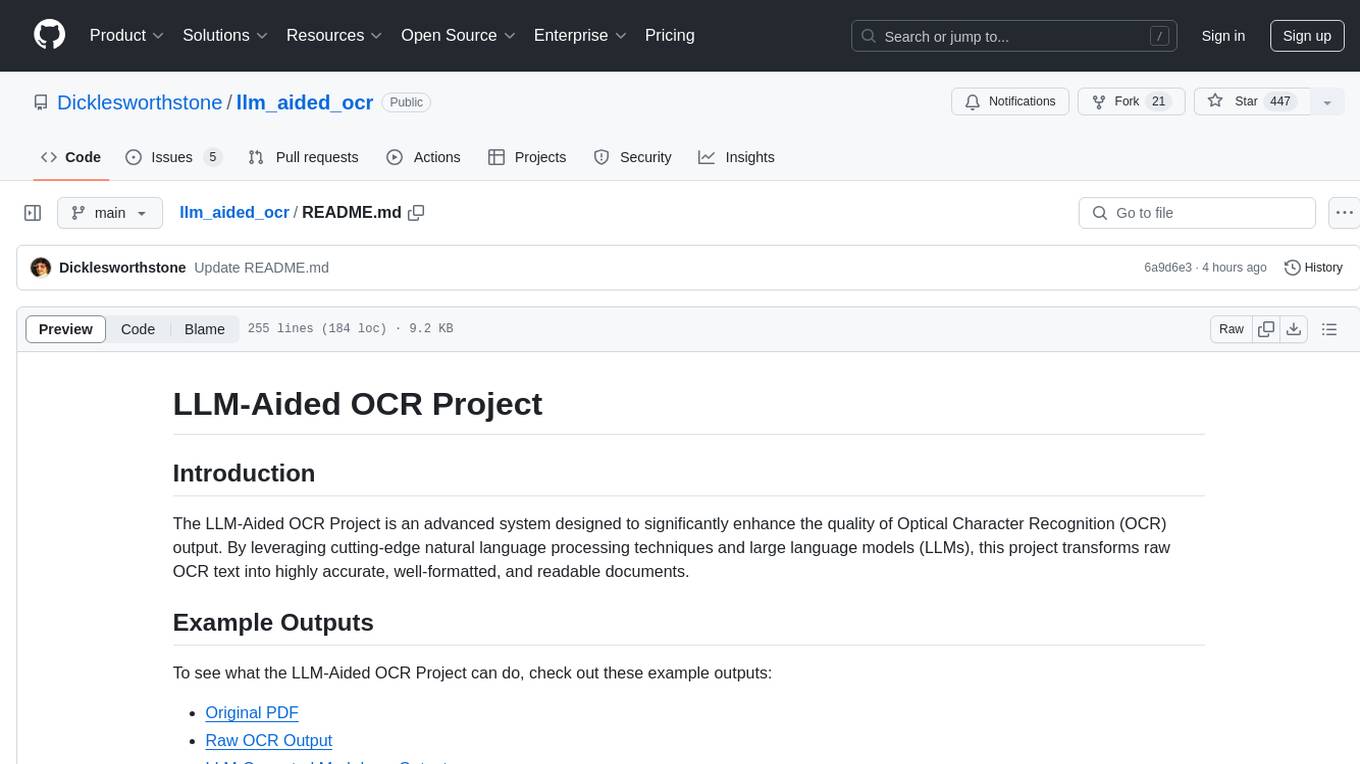
llm_aided_ocr
The LLM-Aided OCR Project is an advanced system that enhances Optical Character Recognition (OCR) output by leveraging natural language processing techniques and large language models. It offers features like PDF to image conversion, OCR using Tesseract, error correction using LLMs, smart text chunking, markdown formatting, duplicate content removal, quality assessment, support for local and cloud-based LLMs, asynchronous processing, detailed logging, and GPU acceleration. The project provides detailed technical overview, text processing pipeline, LLM integration, token management, quality assessment, logging, configuration, and customization. It requires Python 3.12+, Tesseract OCR engine, PDF2Image library, PyTesseract, and optional OpenAI or Anthropic API support for cloud-based LLMs. The installation process involves setting up the project, installing dependencies, and configuring environment variables. Users can place a PDF file in the project directory, update input file path, and run the script to generate post-processed text. The project optimizes processing with concurrent processing, context preservation, and adaptive token management. Configuration settings include choosing between local or API-based LLMs, selecting API provider, specifying models, and setting context size for local LLMs. Output files include raw OCR output and LLM-corrected text. Limitations include performance dependency on LLM quality and time-consuming processing for large documents.
8 - OpenAI Gpts
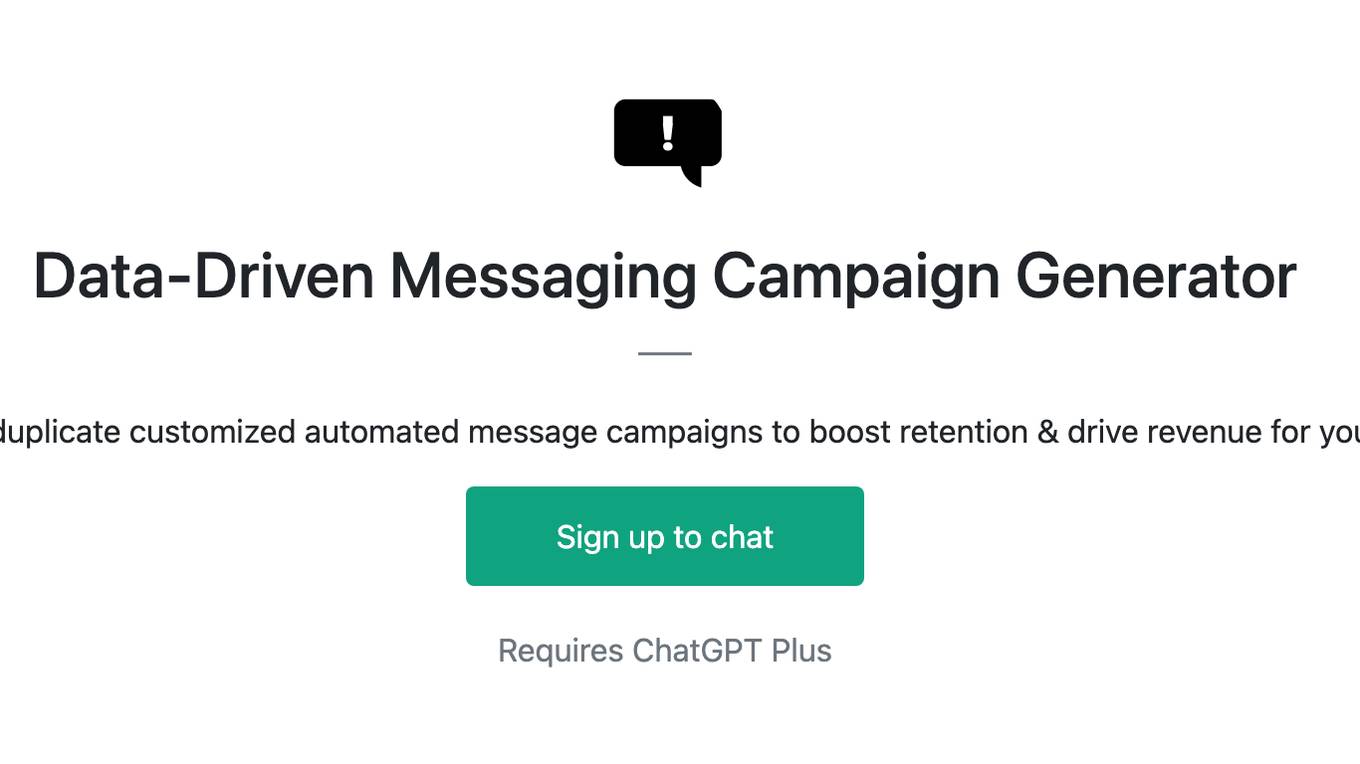
Data-Driven Messaging Campaign Generator
Create, analyze & duplicate customized automated message campaigns to boost retention & drive revenue for your website or app
Plagiarism Checker
Maintain the originality of your work with our Plagiarism Checker. This plagiarism checker identifies duplicate content, ensuring your work's uniqueness and integrity.
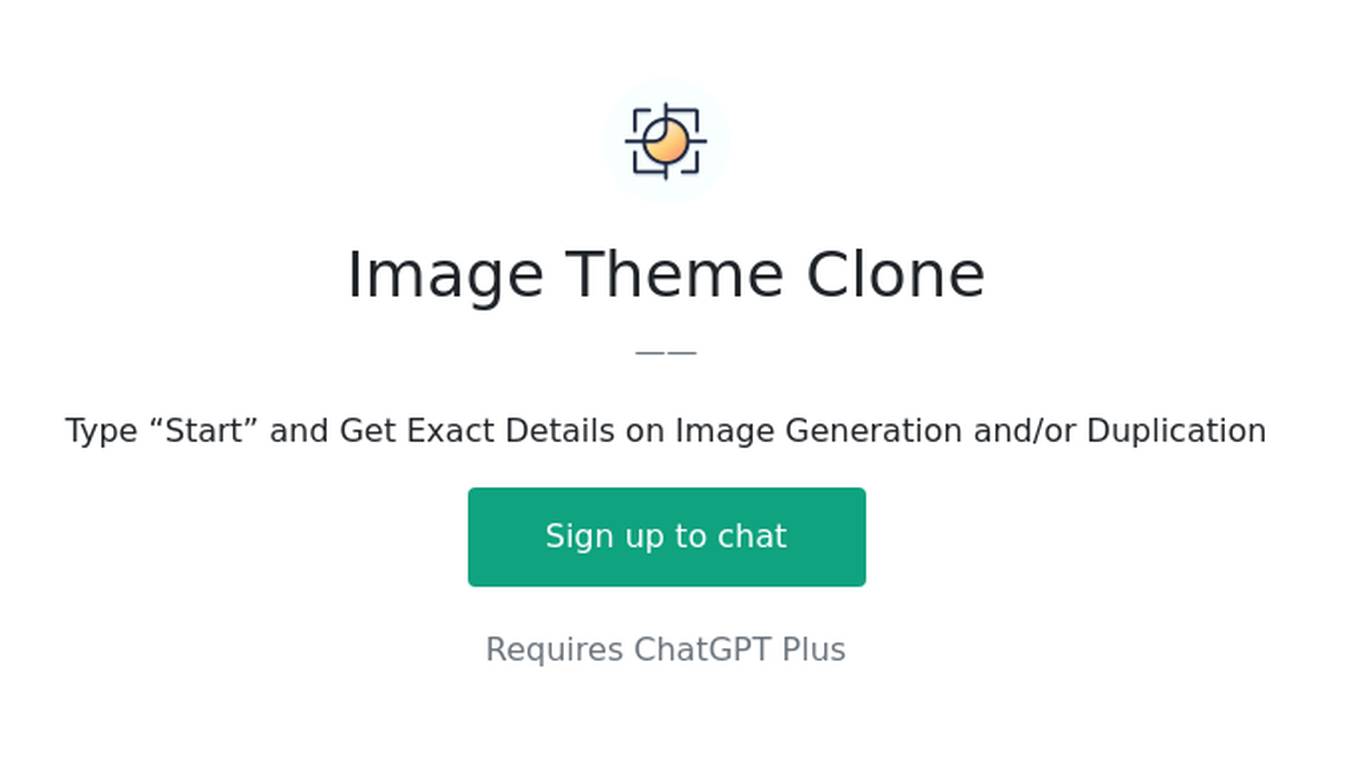
Image Theme Clone
Type “Start” and Get Exact Details on Image Generation and/or Duplication