AI tools for Evidently
Related Tools:
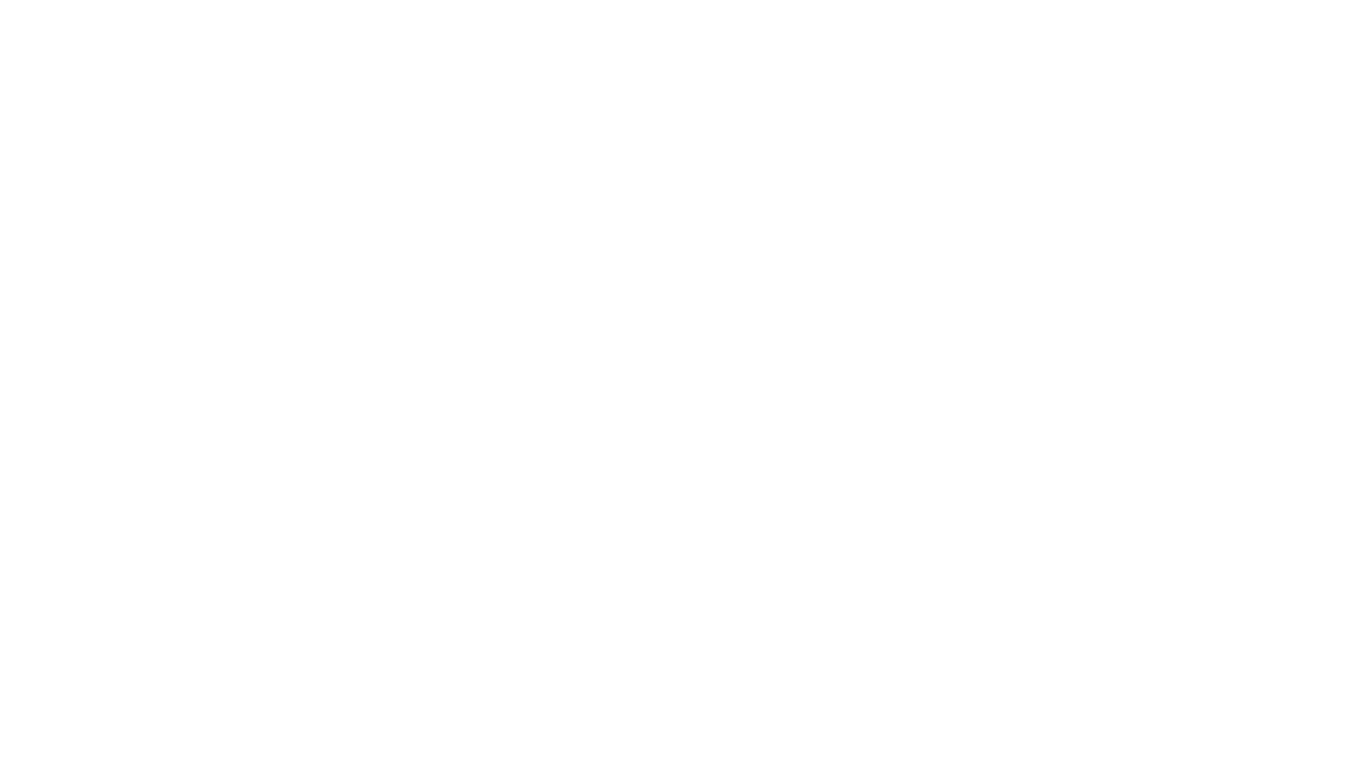
Evidently AI
Evidently AI is an open-source machine learning (ML) monitoring and observability platform that helps data scientists and ML engineers evaluate, test, and monitor ML models from validation to production. It provides a centralized hub for ML in production, including data quality monitoring, data drift monitoring, ML model performance monitoring, and NLP and LLM monitoring. Evidently AI's features include customizable reports, structured checks for data and models, and a Python library for ML monitoring. It is designed to be easy to use, with a simple setup process and a user-friendly interface. Evidently AI is used by over 2,500 data scientists and ML engineers worldwide, and it has been featured in publications such as Forbes, VentureBeat, and TechCrunch.
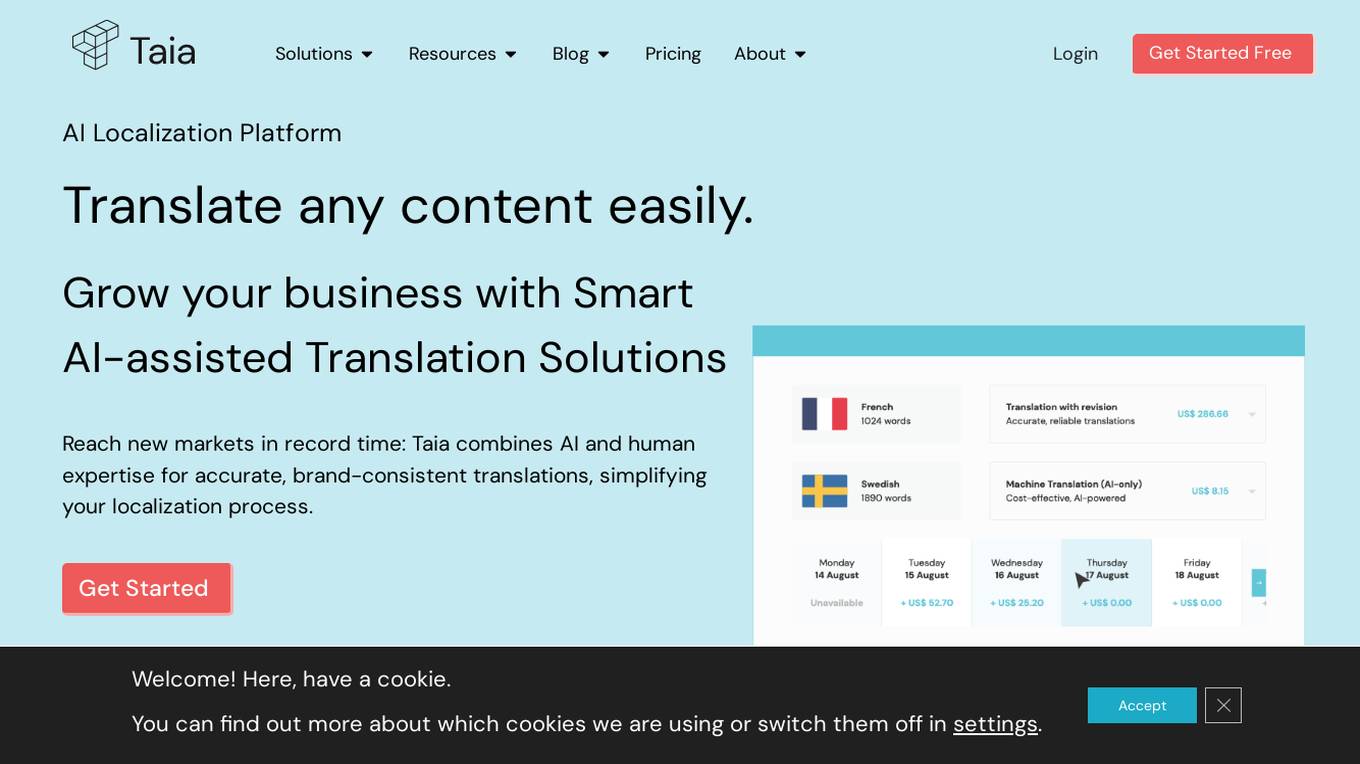
Taia Translations
Taia Translations is an AI-powered platform that offers human-perfected services for document translation, website localization, subtitling and transcription, software localization, financial translations, and content marketing localization. The platform combines AI and human expertise to provide accurate and brand-consistent translations, simplifying the localization process for businesses. Taia's Translation Process includes instant translation quotes, transparent pricing, project management, DIY & AI translation tools, and on-time delivery. The platform also offers resources such as success stories, client comparisons, and references. Taia's dedication to efficient localization is evident in its commitment to quality, speed, and customer satisfaction.
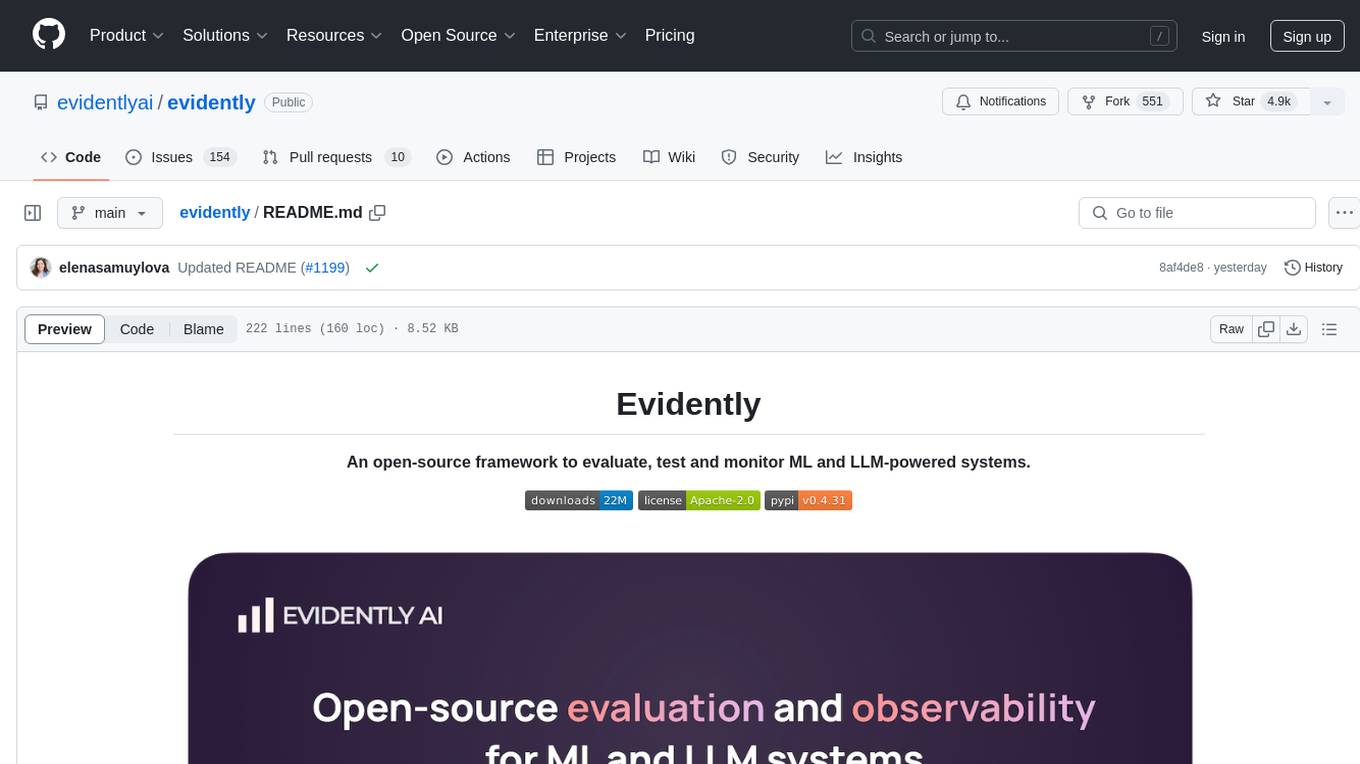
evidently
Evidently is an open-source Python library designed for evaluating, testing, and monitoring machine learning (ML) and large language model (LLM) powered systems. It offers a wide range of functionalities, including working with tabular, text data, and embeddings, supporting predictive and generative systems, providing over 100 built-in metrics for data drift detection and LLM evaluation, allowing for custom metrics and tests, enabling both offline evaluations and live monitoring, and offering an open architecture for easy data export and integration with existing tools. Users can utilize Evidently for one-off evaluations using Reports or Test Suites in Python, or opt for real-time monitoring through the Dashboard service.
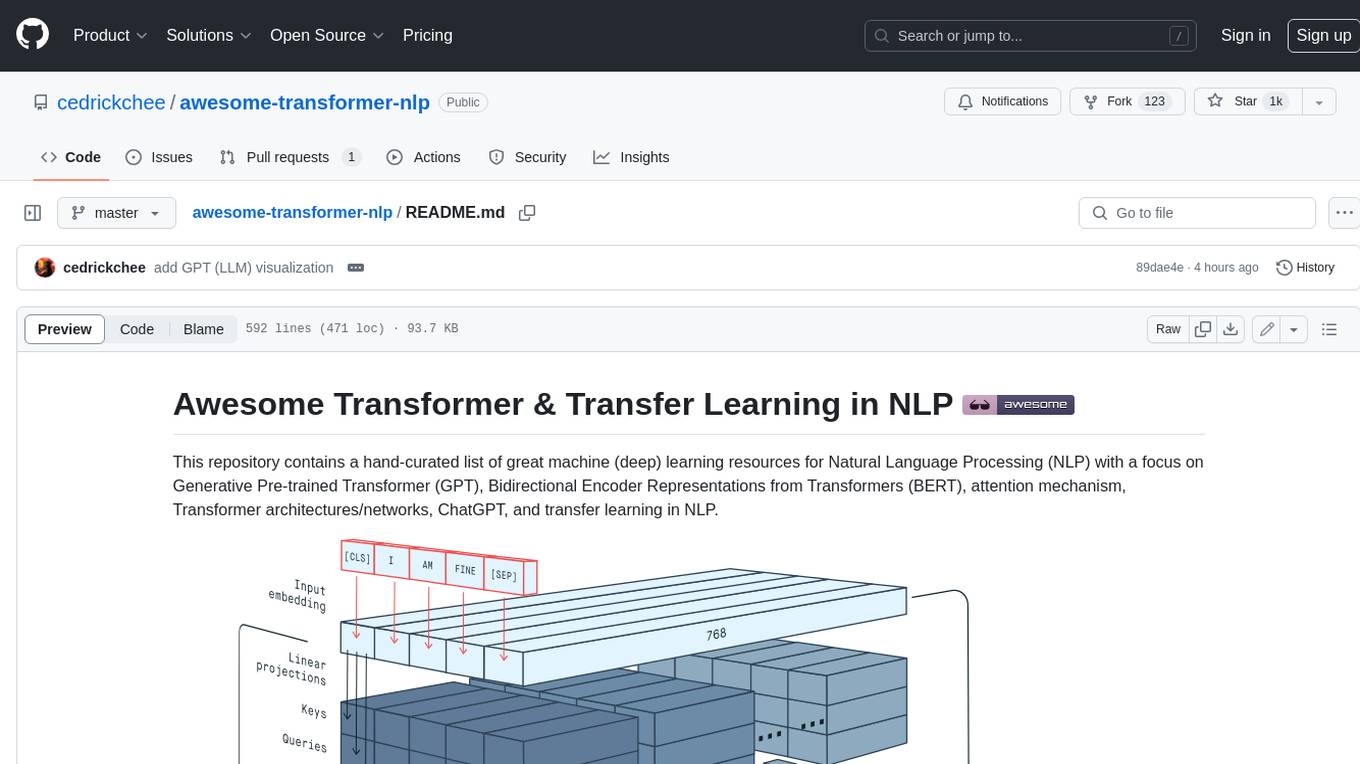
awesome-transformer-nlp
This repository contains a hand-curated list of great machine (deep) learning resources for Natural Language Processing (NLP) with a focus on Generative Pre-trained Transformer (GPT), Bidirectional Encoder Representations from Transformers (BERT), attention mechanism, Transformer architectures/networks, Chatbot, and transfer learning in NLP.
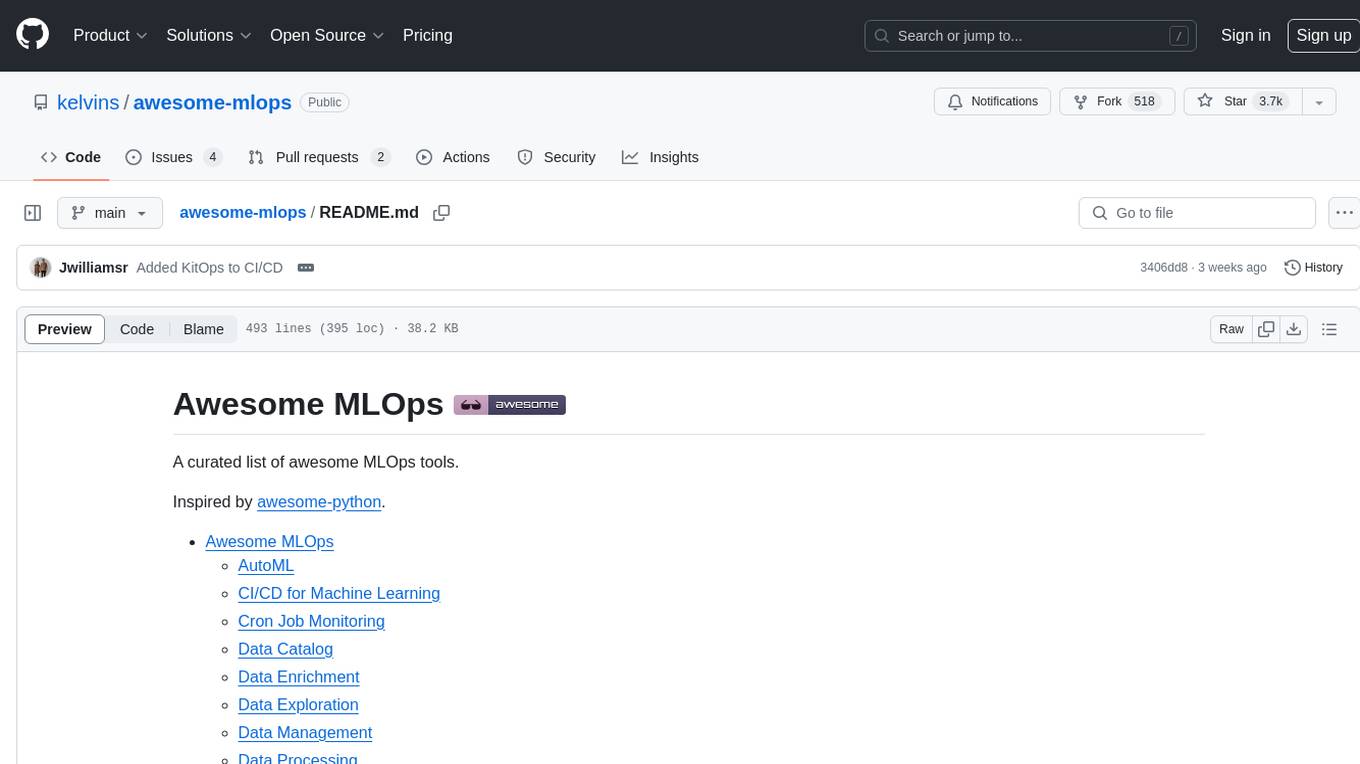
awesome-mlops
Awesome MLOps is a curated list of tools related to Machine Learning Operations, covering areas such as AutoML, CI/CD for Machine Learning, Data Cataloging, Data Enrichment, Data Exploration, Data Management, Data Processing, Data Validation, Data Visualization, Drift Detection, Feature Engineering, Feature Store, Hyperparameter Tuning, Knowledge Sharing, Machine Learning Platforms, Model Fairness and Privacy, Model Interpretability, Model Lifecycle, Model Serving, Model Testing & Validation, Optimization Tools, Simplification Tools, Visual Analysis and Debugging, and Workflow Tools. The repository provides a comprehensive collection of tools and resources for individuals and teams working in the field of MLOps.
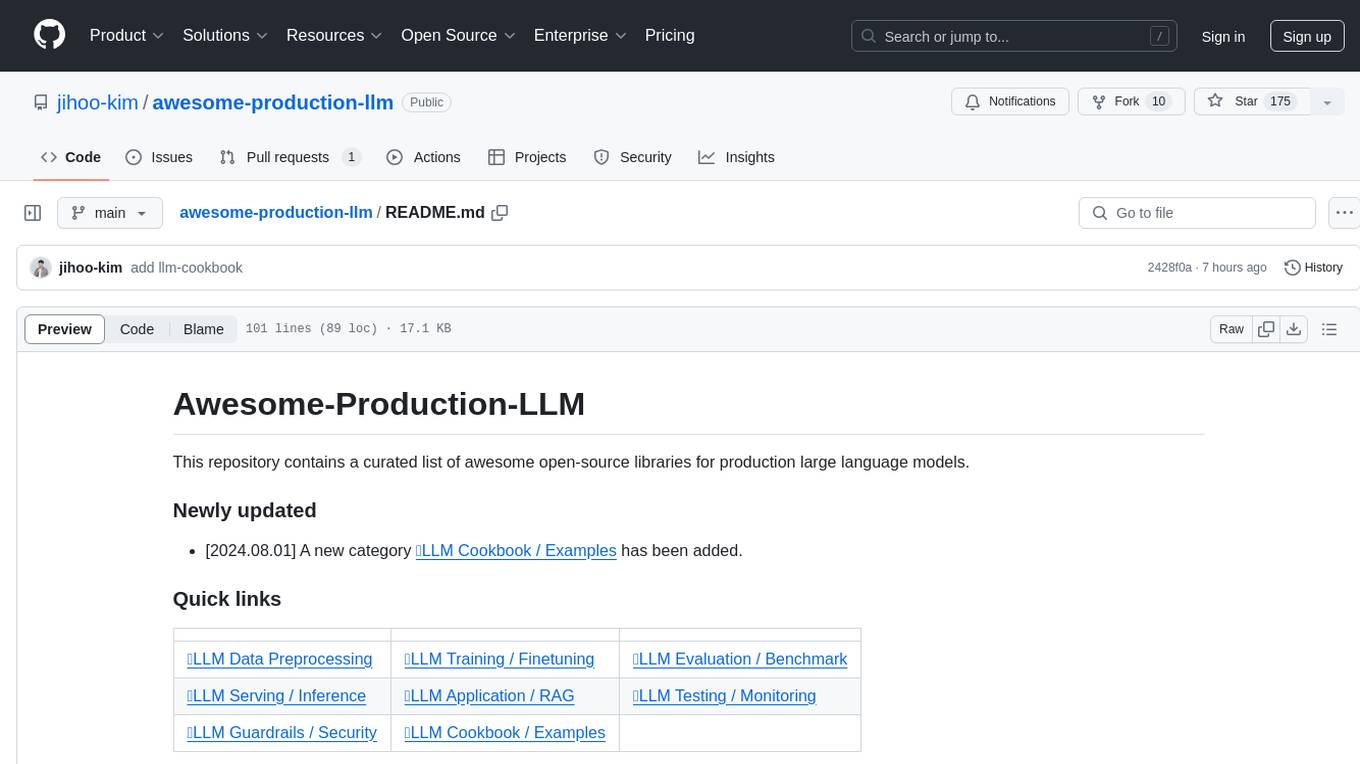
awesome-production-llm
This repository is a curated list of open-source libraries for production large language models. It includes tools for data preprocessing, training/finetuning, evaluation/benchmarking, serving/inference, application/RAG, testing/monitoring, and guardrails/security. The repository also provides a new category called LLM Cookbook/Examples for showcasing examples and guides on using various LLM APIs.
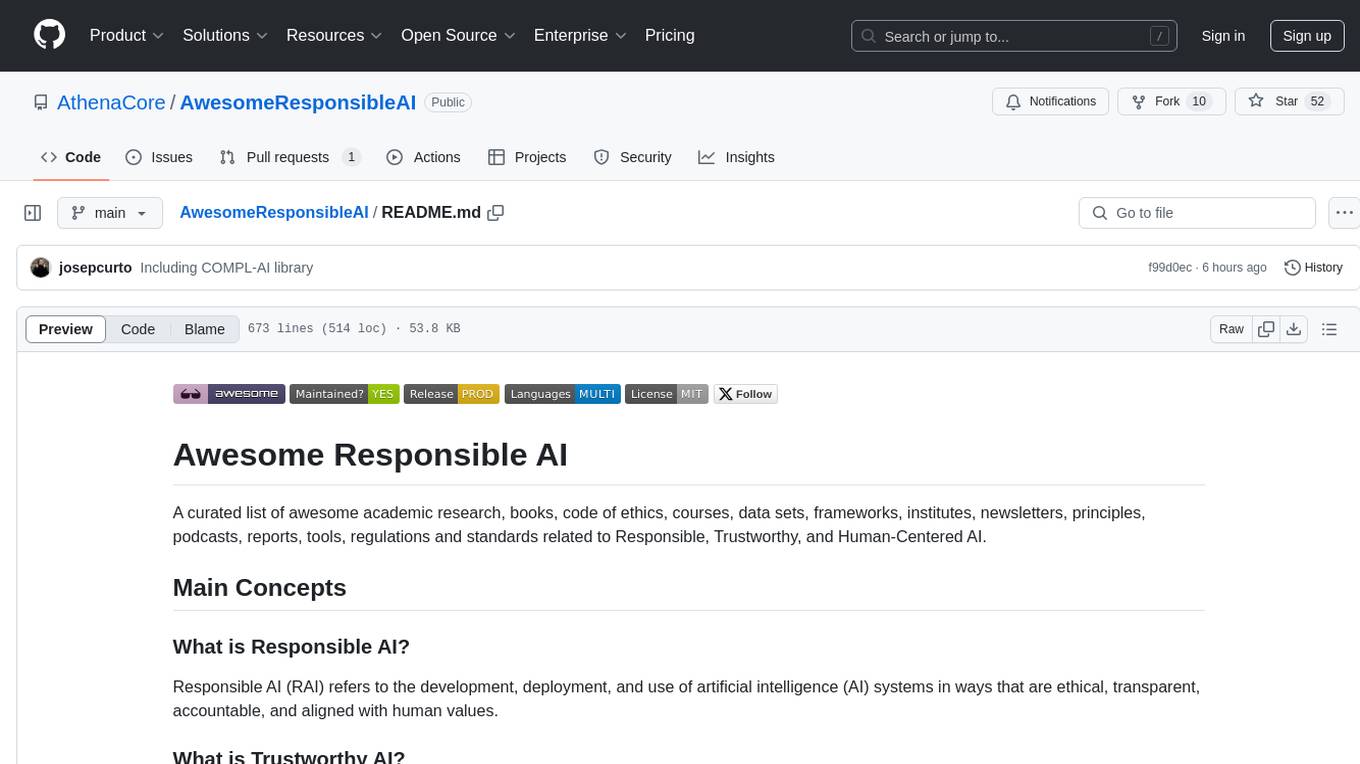
AwesomeResponsibleAI
Awesome Responsible AI is a curated list of academic research, books, code of ethics, courses, data sets, frameworks, institutes, newsletters, principles, podcasts, reports, tools, regulations, and standards related to Responsible, Trustworthy, and Human-Centered AI. It covers various concepts such as Responsible AI, Trustworthy AI, Human-Centered AI, Responsible AI frameworks, AI Governance, and more. The repository provides a comprehensive collection of resources for individuals interested in ethical, transparent, and accountable AI development and deployment.
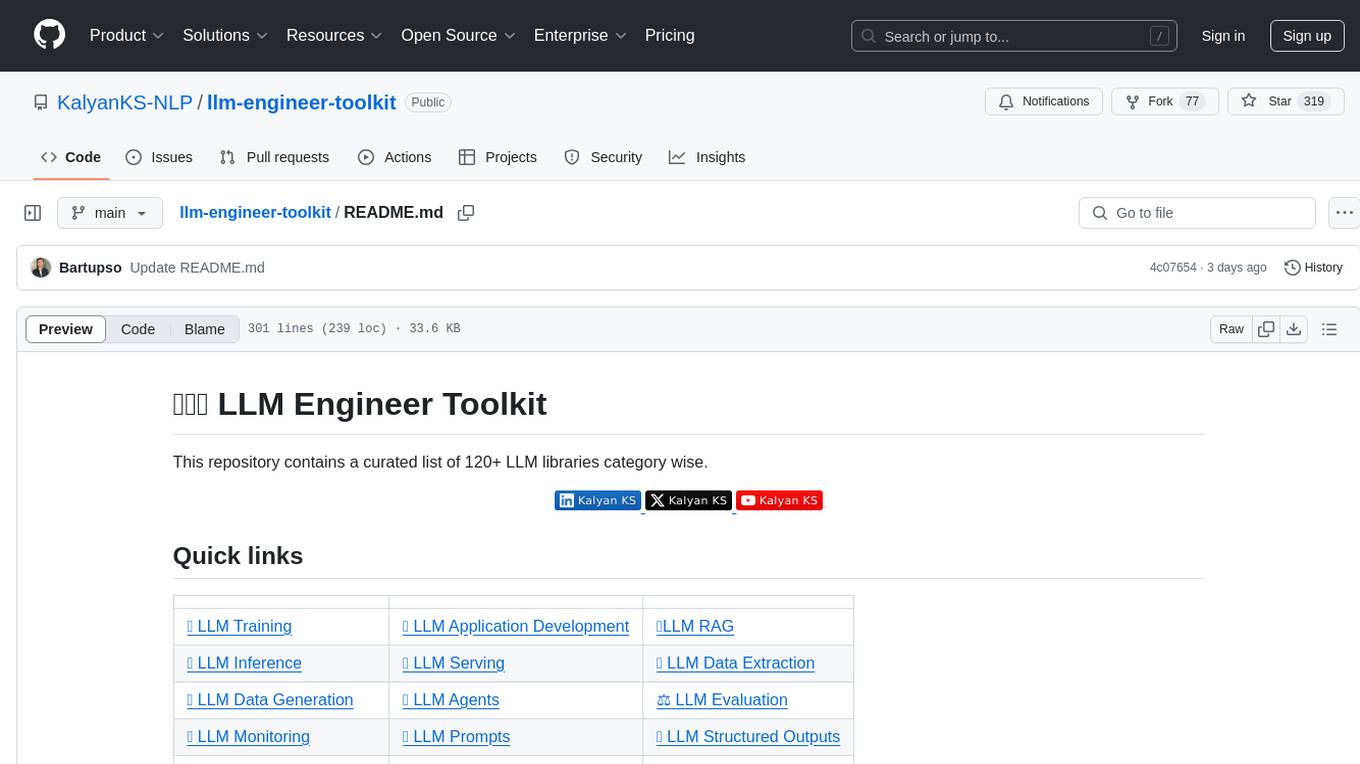
llm-engineer-toolkit
The LLM Engineer Toolkit is a curated repository containing over 120 LLM libraries categorized for various tasks such as training, application development, inference, serving, data extraction, data generation, agents, evaluation, monitoring, prompts, structured outputs, safety, security, embedding models, and other miscellaneous tools. It includes libraries for fine-tuning LLMs, building applications powered by LLMs, serving LLM models, extracting data, generating synthetic data, creating AI agents, evaluating LLM applications, monitoring LLM performance, optimizing prompts, handling structured outputs, ensuring safety and security, embedding models, and more. The toolkit covers a wide range of tools and frameworks to streamline the development, deployment, and optimization of large language models.
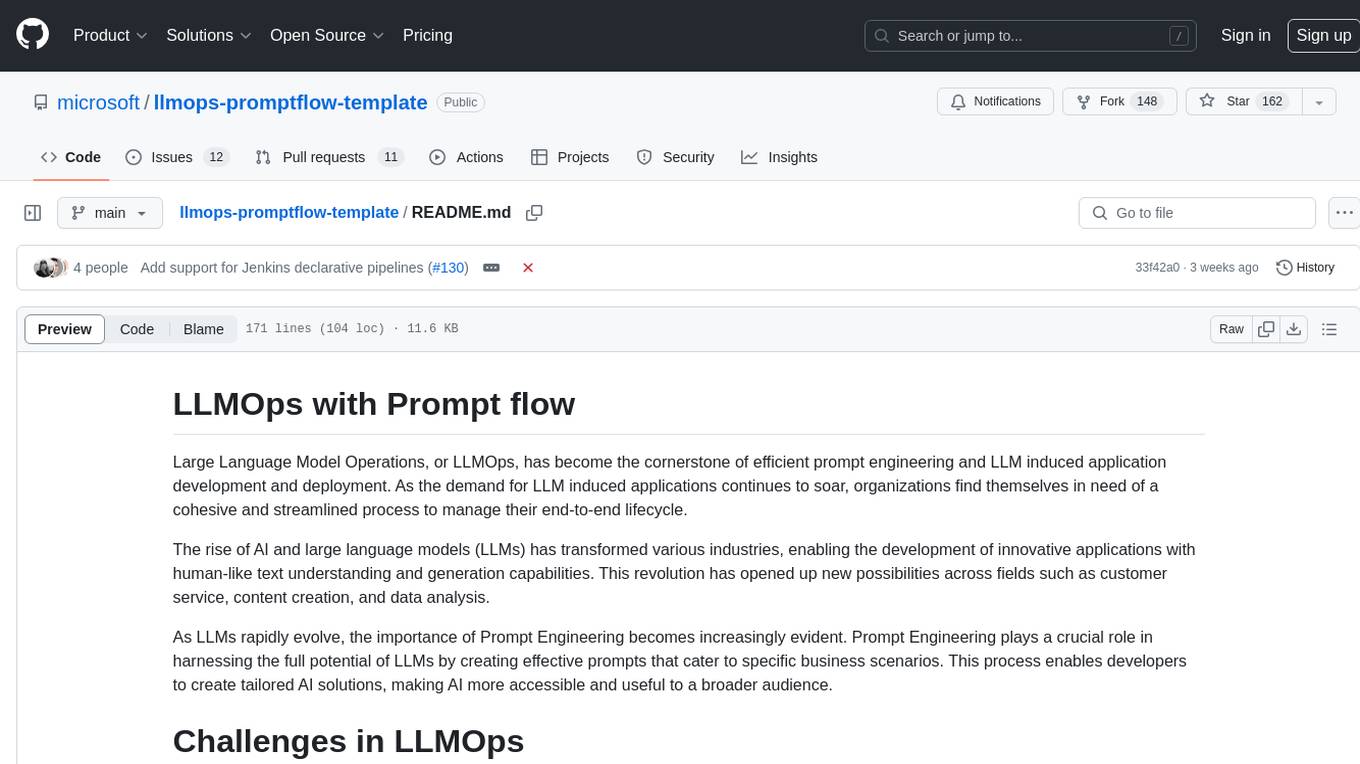
llmops-promptflow-template
LLMOps with Prompt flow is a template and guidance for building LLM-infused apps using Prompt flow. It provides centralized code hosting, lifecycle management, variant and hyperparameter experimentation, A/B deployment, many-to-many dataset/flow relationships, multiple deployment targets, comprehensive reporting, BYOF capabilities, configuration-based development, local prompt experimentation and evaluation, endpoint testing, and optional Human-in-loop validation. The tool is customizable to suit various application needs.
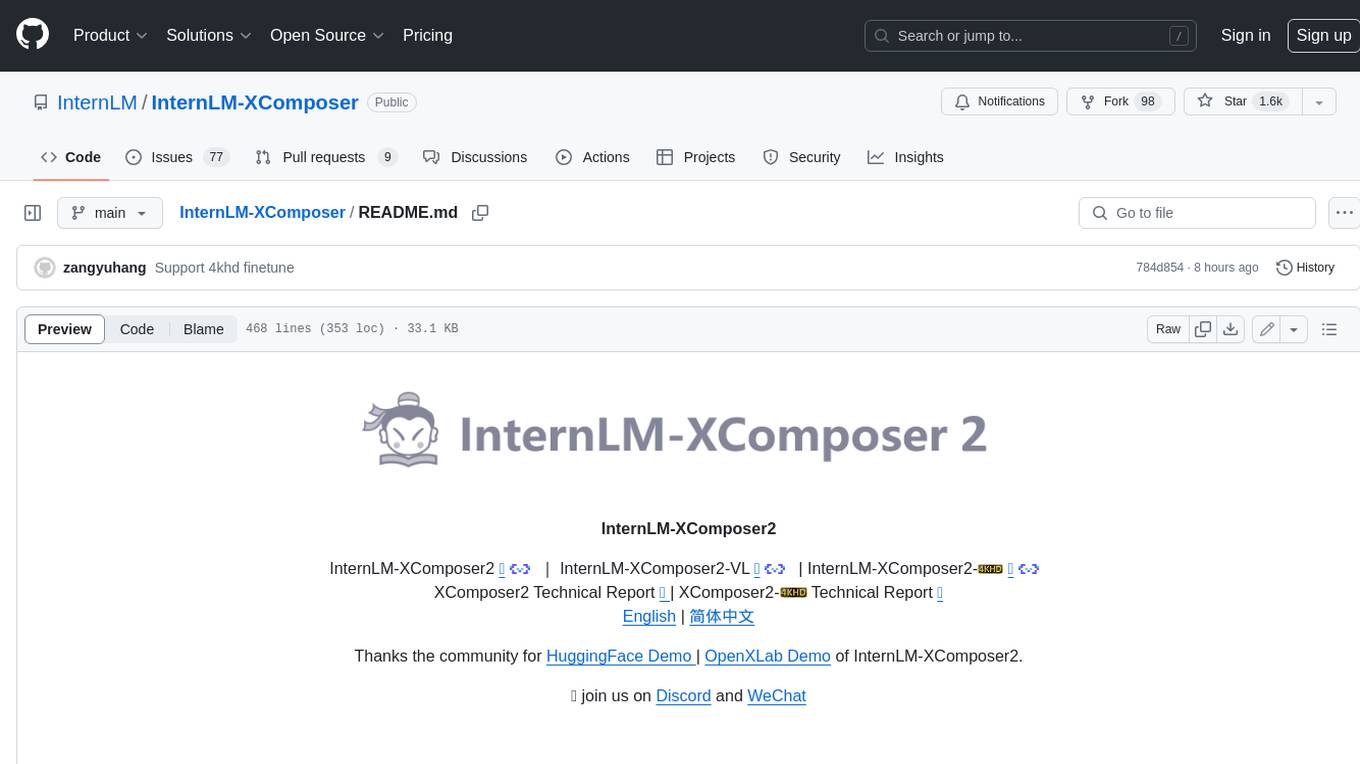
InternLM-XComposer
InternLM-XComposer2 is a groundbreaking vision-language large model (VLLM) based on InternLM2-7B excelling in free-form text-image composition and comprehension. It boasts several amazing capabilities and applications: * **Free-form Interleaved Text-Image Composition** : InternLM-XComposer2 can effortlessly generate coherent and contextual articles with interleaved images following diverse inputs like outlines, detailed text requirements and reference images, enabling highly customizable content creation. * **Accurate Vision-language Problem-solving** : InternLM-XComposer2 accurately handles diverse and challenging vision-language Q&A tasks based on free-form instructions, excelling in recognition, perception, detailed captioning, visual reasoning, and more. * **Awesome performance** : InternLM-XComposer2 based on InternLM2-7B not only significantly outperforms existing open-source multimodal models in 13 benchmarks but also **matches or even surpasses GPT-4V and Gemini Pro in 6 benchmarks** We release InternLM-XComposer2 series in three versions: * **InternLM-XComposer2-4KHD-7B** 🤗: The high-resolution multi-task trained VLLM model with InternLM-7B as the initialization of the LLM for _High-resolution understanding_ , _VL benchmarks_ and _AI assistant_. * **InternLM-XComposer2-VL-7B** 🤗 : The multi-task trained VLLM model with InternLM-7B as the initialization of the LLM for _VL benchmarks_ and _AI assistant_. **It ranks as the most powerful vision-language model based on 7B-parameter level LLMs, leading across 13 benchmarks.** * **InternLM-XComposer2-VL-1.8B** 🤗 : A lightweight version of InternLM-XComposer2-VL based on InternLM-1.8B. * **InternLM-XComposer2-7B** 🤗: The further instruction tuned VLLM for _Interleaved Text-Image Composition_ with free-form inputs. Please refer to Technical Report and 4KHD Technical Reportfor more details.
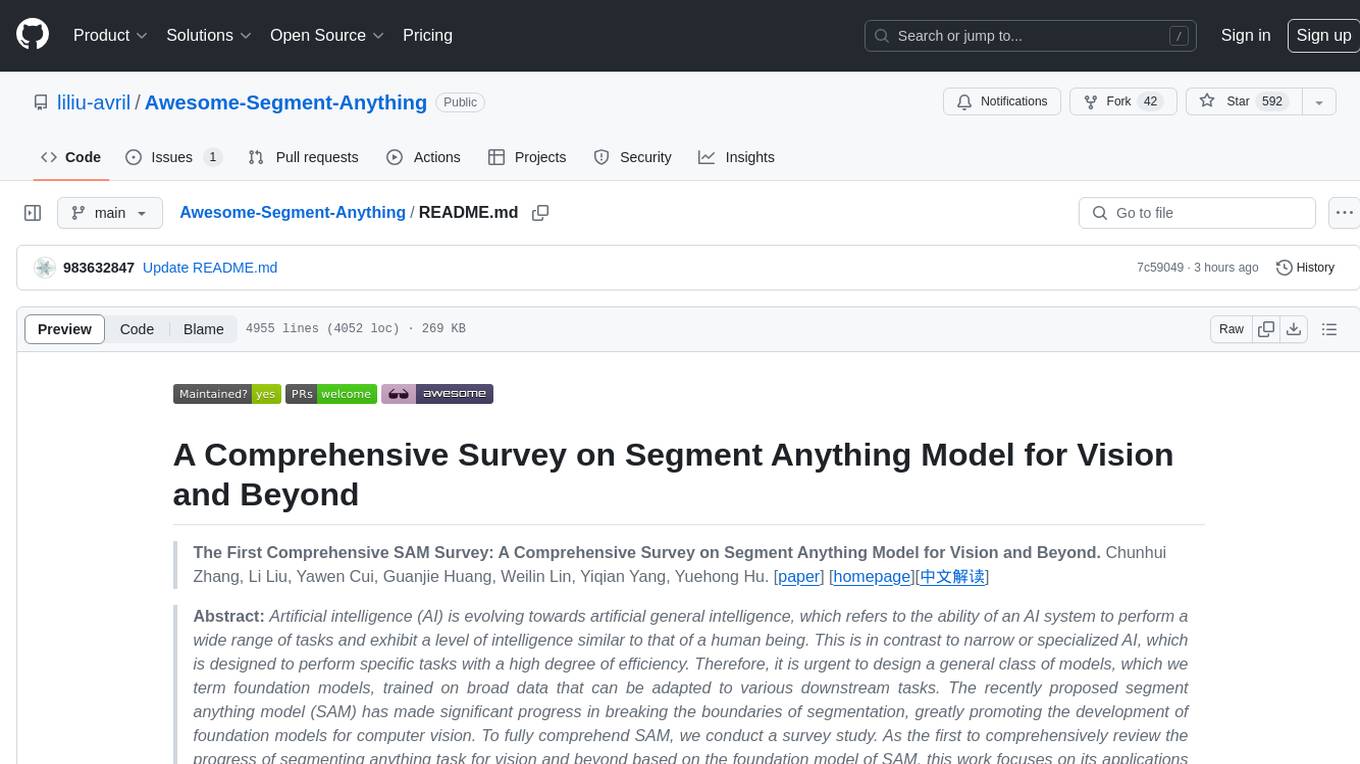
Awesome-Segment-Anything
Awesome-Segment-Anything is a powerful tool for segmenting and extracting information from various types of data. It provides a user-friendly interface to easily define segmentation rules and apply them to text, images, and other data formats. The tool supports both supervised and unsupervised segmentation methods, allowing users to customize the segmentation process based on their specific needs. With its versatile functionality and intuitive design, Awesome-Segment-Anything is ideal for data analysts, researchers, content creators, and anyone looking to efficiently extract valuable insights from complex datasets.
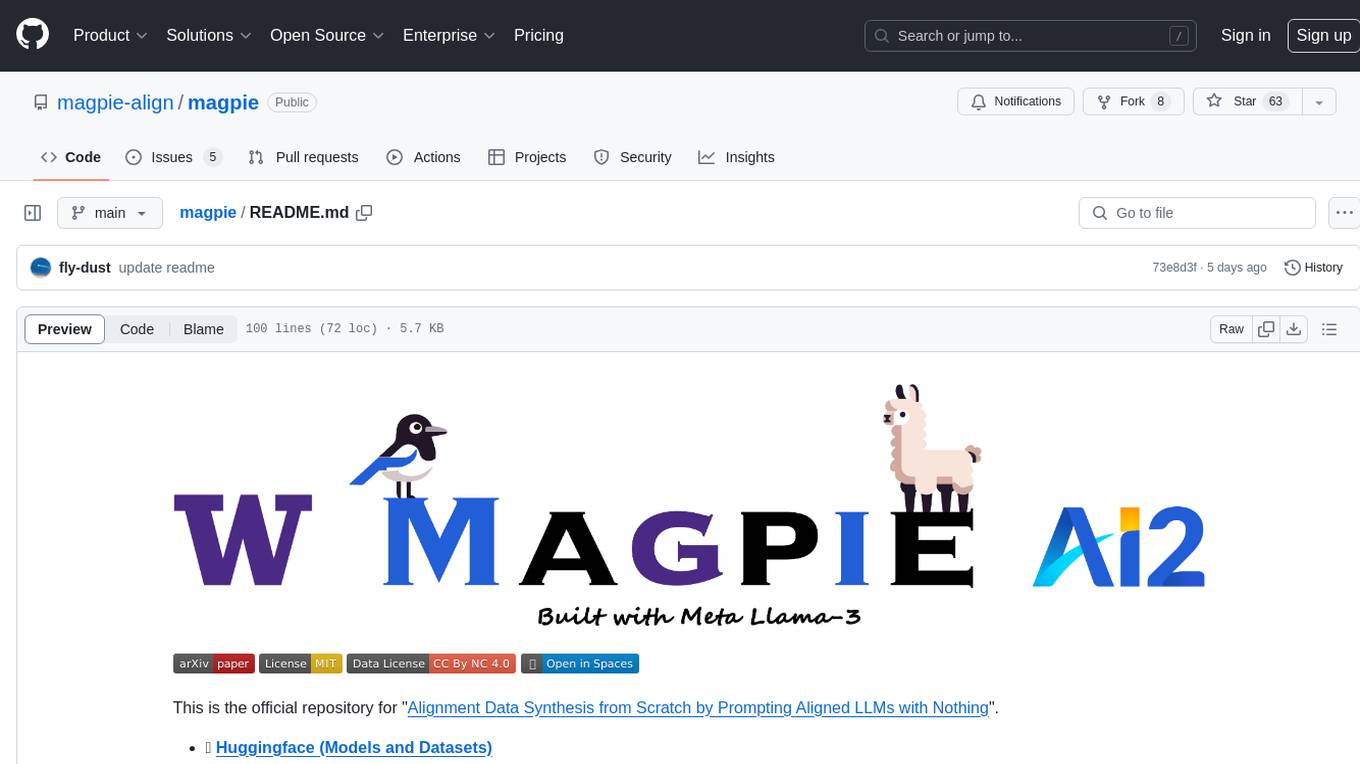
magpie
This is the official repository for 'Alignment Data Synthesis from Scratch by Prompting Aligned LLMs with Nothing'. Magpie is a tool designed to synthesize high-quality instruction data at scale by extracting it directly from an aligned Large Language Models (LLMs). It aims to democratize AI by generating large-scale alignment data and enhancing the transparency of model alignment processes. Magpie has been tested on various model families and can be used to fine-tune models for improved performance on alignment benchmarks such as AlpacaEval, ArenaHard, and WildBench.
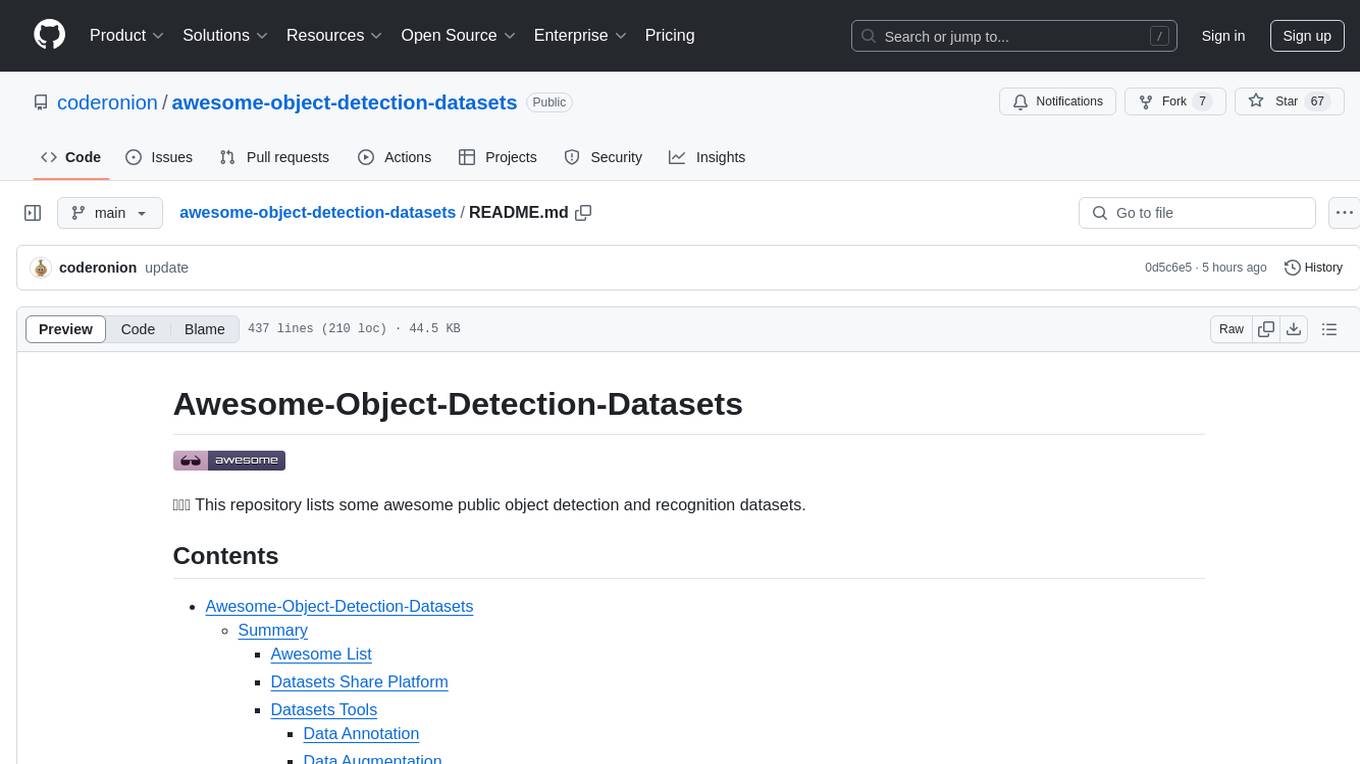
awesome-object-detection-datasets
This repository is a curated list of awesome public object detection and recognition datasets. It includes a wide range of datasets related to object detection and recognition tasks, such as general detection and recognition datasets, autonomous driving datasets, adverse weather datasets, person detection datasets, anti-UAV datasets, optical aerial imagery datasets, low-light image datasets, infrared image datasets, SAR image datasets, multispectral image datasets, 3D object detection datasets, vehicle-to-everything field datasets, super-resolution field datasets, and face detection and recognition datasets. The repository also provides information on tools for data annotation, data augmentation, and data management related to object detection tasks.