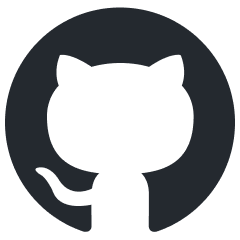
awesome-llm-understanding-mechanism
awesome papers in LLM interpretability
Stars: 376
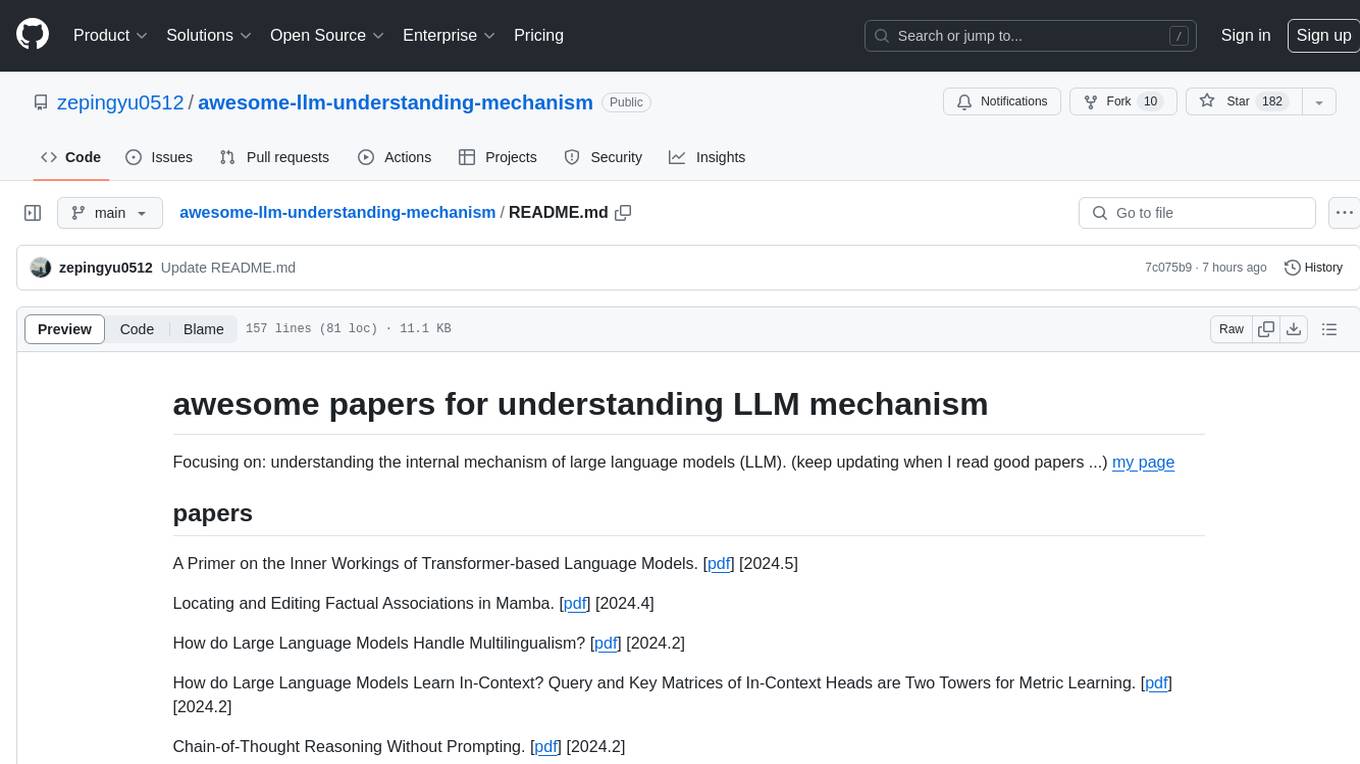
This repository is a collection of papers focused on understanding the internal mechanism of large language models (LLM). It includes research on topics such as how LLMs handle multilingualism, learn in-context, and handle factual associations. The repository aims to provide insights into the inner workings of transformer-based language models through a curated list of papers and surveys.
README:
This list focuses on understanding the internal mechanism of large language models (LLM). Works in this list are accepted by top conferences (e.g. ICML, NeurIPS, ICLR, ACL, EMNLP, NAACL), or written by top research institutions.
Other paper lists focuses on SAE and neuron.
Paper recommendation (accepted by conferences): please contact me.
-
Interpreting Arithmetic Mechanism in Large Language Models through Comparative Neuron Analysis
- [EMNLP 2024] [2024.9] [neuron] [arithmetic] [fine-tune]
-
NNsight and NDIF: Democratizing Access to Open-Weight Foundation Model Internals
- [2024.7]
-
Scaling and evaluating sparse autoencoders
- [OpenAI] [2024.6] [SAE]
-
- [EMNLP 2024] [2024.6] [in-context learning]
-
Hopping Too Late: Exploring the Limitations of Large Language Models on Multi-Hop Queries
- [EMNLP 2024] [2024.6] [knowledge] [reasoning]
-
Neuron-Level Knowledge Attribution in Large Language Models
- [EMNLP 2024] [2024.6] [neuron] [knowledge]
-
Knowledge Circuits in Pretrained Transformers
- [NeurIPS 2024] [2024.5] [circuit] [knowledge]
-
Locating and Editing Factual Associations in Mamba
- [COLM 2024] [2024.4] [causal] [knowledge]
-
Have Faith in Faithfulness: Going Beyond Circuit Overlap When Finding Model Mechanisms
- [COLM 2024] [2024.3] [circuit]
-
Diffusion Lens: Interpreting Text Encoders in Text-to-Image Pipelines
- [ACL 2024] [2024.3] [logit lens] [multimodal]
-
Chain-of-Thought Reasoning Without Prompting
- [Deepmind] [2024.2] [chain-of-thought]
-
Backward Lens: Projecting Language Model Gradients into the Vocabulary Space
- [EMNLP 2024] [2024.2] [logit lens]
-
Fine-Tuning Enhances Existing Mechanisms: A Case Study on Entity Tracking
- [ICLR 2024] [2024.2] [fine-tune]
-
TruthX: Alleviating Hallucinations by Editing Large Language Models in Truthful Space
- [ACL 2024] [2024.2] [hallucination]
-
Understanding and Patching Compositional Reasoning in LLMs
- [ACL 2024] [2024.2] [reasoning]
-
Do Large Language Models Latently Perform Multi-Hop Reasoning?
- [ACL 2024] [2024.2] [knowledge] [reasoning]
-
Long-form evaluation of model editing
- [NAACL 2024] [2024.2] [model editing]
-
A Mechanistic Understanding of Alignment Algorithms: A Case Study on DPO and Toxicity
- [ICML 2024] [2024.1] [toxicity] [fine-tune]
-
What does the Knowledge Neuron Thesis Have to do with Knowledge?
- [ICLR 2024] [2023.11] [knowledge] [neuron]
-
Mechanistically analyzing the effects of fine-tuning on procedurally defined tasks
- [ICLR 2024] [2023.11] [fine-tune]
-
Scaling Monosemanticity: Extracting Interpretable Features from Claude 3 Sonnet
- [Anthropic] [2023.10] [SAE]
-
Interpreting CLIP's Image Representation via Text-Based Decomposition
- [ICLR 2024] [2023.10] [multimodal]
-
Towards Best Practices of Activation Patching in Language Models: Metrics and Methods
- [ICLR 2024] [2023.10] [causal] [circuit]
-
Fact Finding: Attempting to Reverse-Engineer Factual Recall on the Neuron Level
- [Deepmind] [2023.12] [neuron]
-
Successor Heads: Recurring, Interpretable Attention Heads In The Wild
- [ICLR 2024] [2023.12] [circuit]
-
Towards Monosemanticity: Decomposing Language Models With Dictionary Learning
- [Anthropic] [2023.10] [SAE]
-
Impact of Co-occurrence on Factual Knowledge of Large Language Models
- [EMNLP 2023] [2023.10] [knowledge]
-
Function vectors in large language models
- [ICLR 2024] [2023.10] [in-context learning]
-
Neurons in Large Language Models: Dead, N-gram, Positional
- [ACL 2024] [2023.9] [neuron]
-
Sparse Autoencoders Find Highly Interpretable Features in Language Models
- [ICLR 2024] [2023.9] [SAE]
-
Do Machine Learning Models Memorize or Generalize?
- [2023.8] [grokking]
-
Overthinking the Truth: Understanding how Language Models Process False Demonstrations
- [TACL 2024] [2023.7] [circuit]
-
Evaluating the ripple effects of knowledge editing in language models
- [2023.7] [knowledge] [model editing]
-
Inference-Time Intervention: Eliciting Truthful Answers from a Language Model
- [NeurIPS 2023] [2023.6] [hallucination]
-
VISIT: Visualizing and Interpreting the Semantic Information Flow of Transformers
- [EMNLP 2023] [2023.5] [logit lens]
-
Finding Neurons in a Haystack: Case Studies with Sparse Probing
- [TMLR 2024] [2023.5] [neuron]
-
Label Words are Anchors: An Information Flow Perspective for Understanding In-Context Learning
- [EMNLP 2023] [2023.5] [in-context learning]
-
- [ICLR 2024] [2023.5] [chain-of-thought]
-
What In-Context Learning "Learns" In-Context: Disentangling Task Recognition and Task Learning
- [ACL 2023] [2023.5] [in-context learning]
-
Language models can explain neurons in language models
- [OpenAI] [2023.5] [neuron]
-
- [EMNLP 2023] [2023.5] [causal] [arithmetic]
-
Dissecting Recall of Factual Associations in Auto-Regressive Language Models
- [EMNLP 2023] [2023.4] [causal] [knowledge]
-
The Internal State of an LLM Knows When It's Lying
- [EMNLP 2023] [2023.4] [hallucination]
-
Are Emergent Abilities of Large Language Models a Mirage?
- [NeurIPS 2023] [2023.4] [grokking]
-
Towards automated circuit discovery for mechanistic interpretability
- [NeurIPS 2023] [2023.4] [circuit]
-
- [NeurIPS 2023] [2023.4] [circuit] [arithmetic]
-
Larger language models do in-context learning differently
- [Google Research] [2023.3] [in-context learning]
-
- [NeurIPs 2023] [2023.1] [knowledge] [model editing]
-
Towards Understanding Chain-of-Thought Prompting: An Empirical Study of What Matters
- [ACL 2023] [2022.12] [chain-of-thought]
-
Interpretability in the Wild: a Circuit for Indirect Object Identification in GPT-2 small
- [ICLR 2023] [2022.11] [arithmetic] [circuit]
-
Inverse scaling can become U-shaped
- [EMNLP 2023] [2022.11] [grokking]
-
Mass-Editing Memory in a Transformer
- [ICLR 2023] [2022.10] [model editing]
-
Polysemanticity and Capacity in Neural Networks
- [2022.10] [neuron] [SAE]
-
Analyzing Transformers in Embedding Space
- [ACL 2023] [2022.9] [logit lens]
-
- [Anthropic] [2022.9] [neuron] [SAE]
-
Text and Patterns: For Effective Chain of Thought, It Takes Two to Tango
- [Google Research] [2022.9] [chain-of-thought]
-
Emergent Abilities of Large Language Models
- [Google Research] [2022.6] [grokking]
-
Towards Tracing Factual Knowledge in Language Models Back to the Training Data
- [EMNLP 2022] [2022.5] [knowledge] [data]
-
Ground-Truth Labels Matter: A Deeper Look into Input-Label Demonstrations
- [EMNLP 2022] [2022.5] [in-context learning]
-
Large Language Models are Zero-Shot Reasoners
- [NeurIPS 2022] [2022.5] [chain-of-thought]
-
Scaling Laws and Interpretability of Learning from Repeated Data
- [Anthropic] [2022.5] [grokking] [data]
-
Transformer Feed-Forward Layers Build Predictions by Promoting Concepts in the Vocabulary Space
- [EMNLP 2022] [2022.3] [neuron] [logit lens]
-
In-context Learning and Induction Heads
- [Anthropic] [2022.3] [circuit] [in-context learning]
-
Locating and Editing Factual Associations in GPT
- [NeurIPS 2022] [2022.2] [causal] [knowledge]
-
Rethinking the Role of Demonstrations: What Makes In-Context Learning Work?
- [EMNLP 2022] [2022.2] [in-context learning]
-
Grokking: Generalization Beyond Overfitting on Small Algorithmic Datasets
- [OpenAI & Google] [2022.1] [grokking]
-
Chain-of-Thought Prompting Elicits Reasoning in Large Language Models
- [NeurIPS 2022] [2022.1] [chain-of-thought]
-
A Mathematical Framework for Transformer Circuits
- [Anthropic] [2021.12] [circuit]
-
Towards a Unified View of Parameter-Efficient Transfer Learning
- [ICLR 2022] [2021.10] [fine-tune]
-
Deduplicating Training Data Makes Language Models Better
- [ACL 2022] [2021.7] [fine-tune] [data]
-
Fantastically Ordered Prompts and Where to Find Them: Overcoming Few-Shot Prompt Order Sensitivity
- [ACL 2022] [2021.4] [in-context learning]
-
Calibrate Before Use: Improving Few-Shot Performance of Language Models
- [ICML 2021] [2021.2] [in-context learning]
-
Transformer Feed-Forward Layers Are Key-Value Memories
- [EMNLP 2021] [2020.12] [neuron]
-
Mechanistic Interpretability for AI Safety A Review
- [2024.8] [safety]
-
A Practical Review of Mechanistic Interpretability for Transformer-Based Language Models
- [2024.7] [interpretability]
-
Internal Consistency and Self-Feedback in Large Language Models: A Survey
- [2024.7]
-
A Primer on the Inner Workings of Transformer-based Language Models
- [2024.5] [interpretability]
-
Usable XAI: 10 strategies towards exploiting explainability in the LLM era
- [2024.3] [interpretability]
-
A Comprehensive Overview of Large Language Models
- [2023.12] [LLM]
-
- [2023.11] [hallucination]
-
A Survey of Large Language Models
- [2023.11] [LLM]
-
Explainability for Large Language Models: A Survey
- [2023.11] [interpretability]
-
A Survey of Chain of Thought Reasoning: Advances, Frontiers and Future
- [2023.10] [chain of thought]
-
Instruction tuning for large language models: A survey
- [2023.10] [instruction tuning]
-
- [2023.9] [instruction tuning]
-
Siren’s Song in the AI Ocean: A Survey on Hallucination in Large Language Models
- [2023.9] [hallucination]
-
Reasoning with language model prompting: A survey
- [2023.9] [reasoning]
-
Toward Transparent AI: A Survey on Interpreting the Inner Structures of Deep Neural Networks
- [2023.8] [interpretability]
-
A Survey on In-context Learning
- [2023.6] [in-context learning]
-
Scaling Down to Scale Up: A Guide to Parameter-Efficient Fine-Tuning
- [2023.3] [parameter-efficient fine-tuning]
-
https://github.com/ruizheliUOA/Awesome-Interpretability-in-Large-Language-Models (interpretability)
-
https://github.com/cooperleong00/Awesome-LLM-Interpretability?tab=readme-ov-file (interpretability)
-
https://github.com/JShollaj/awesome-llm-interpretability (interpretability)
-
https://github.com/IAAR-Shanghai/Awesome-Attention-Heads (attention)
-
https://github.com/zjunlp/KnowledgeEditingPapers (model editing)
From Insights to Actions: The Impact of Interpretability and Analysis Research on NLP
For Tasks:
Click tags to check more tools for each tasksFor Jobs:
Alternative AI tools for awesome-llm-understanding-mechanism
Similar Open Source Tools
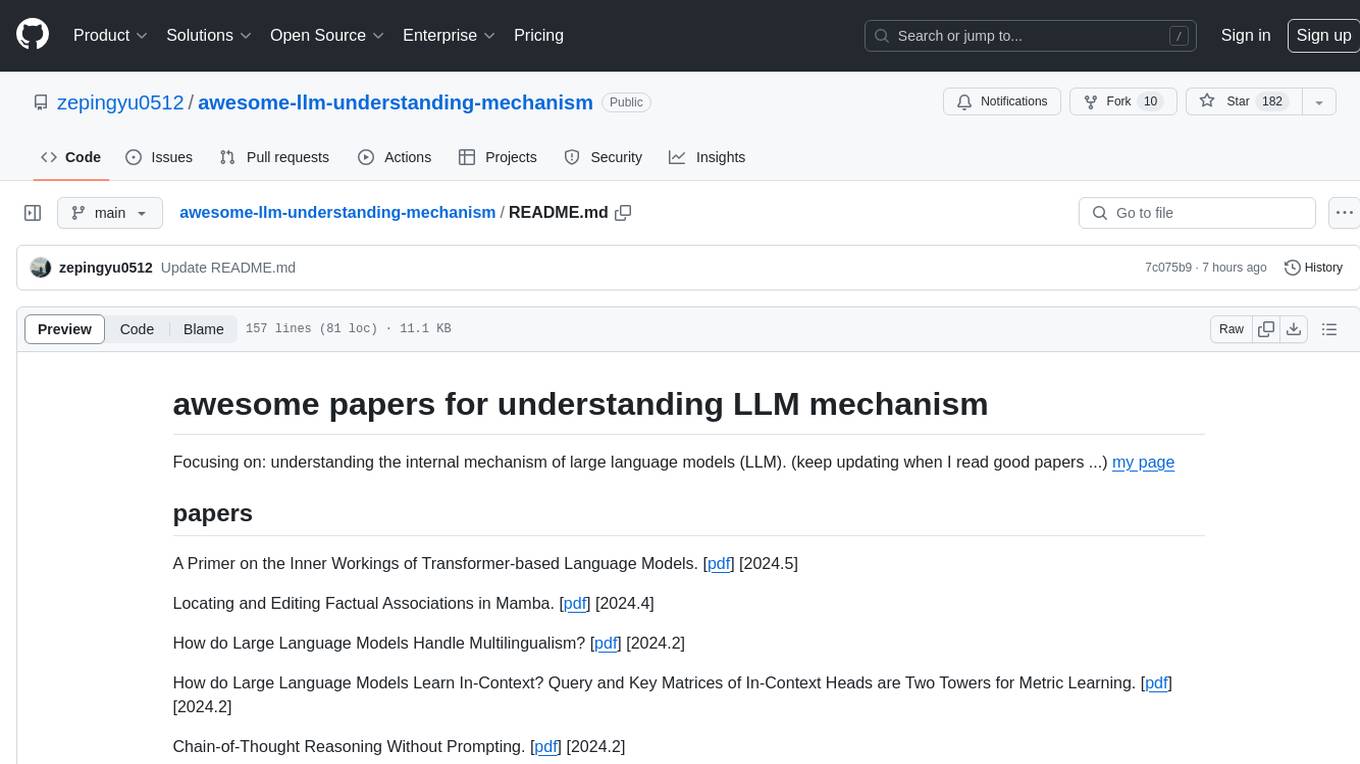
awesome-llm-understanding-mechanism
This repository is a collection of papers focused on understanding the internal mechanism of large language models (LLM). It includes research on topics such as how LLMs handle multilingualism, learn in-context, and handle factual associations. The repository aims to provide insights into the inner workings of transformer-based language models through a curated list of papers and surveys.
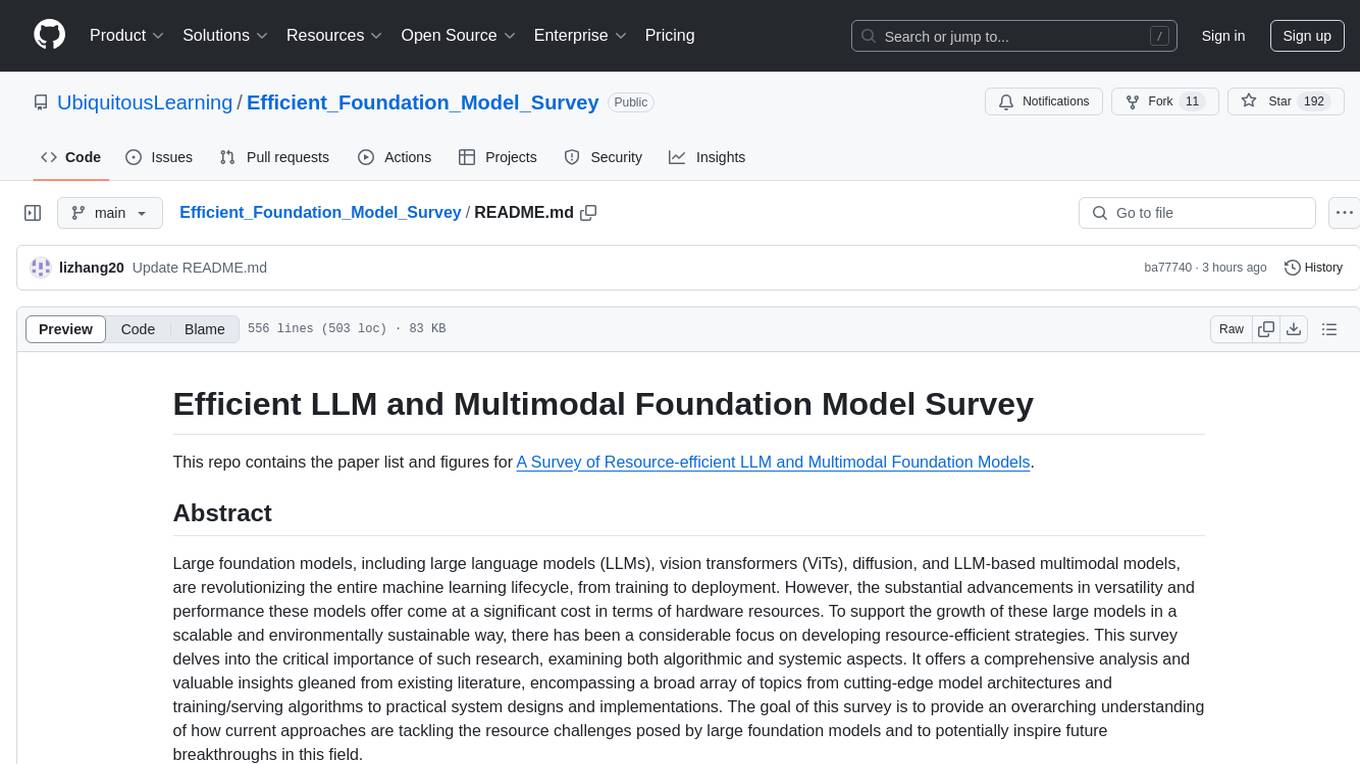
Efficient_Foundation_Model_Survey
Efficient Foundation Model Survey is a comprehensive analysis of resource-efficient large language models (LLMs) and multimodal foundation models. The survey covers algorithmic and systemic innovations to support the growth of large models in a scalable and environmentally sustainable way. It explores cutting-edge model architectures, training/serving algorithms, and practical system designs. The goal is to provide insights on tackling resource challenges posed by large foundation models and inspire future breakthroughs in the field.
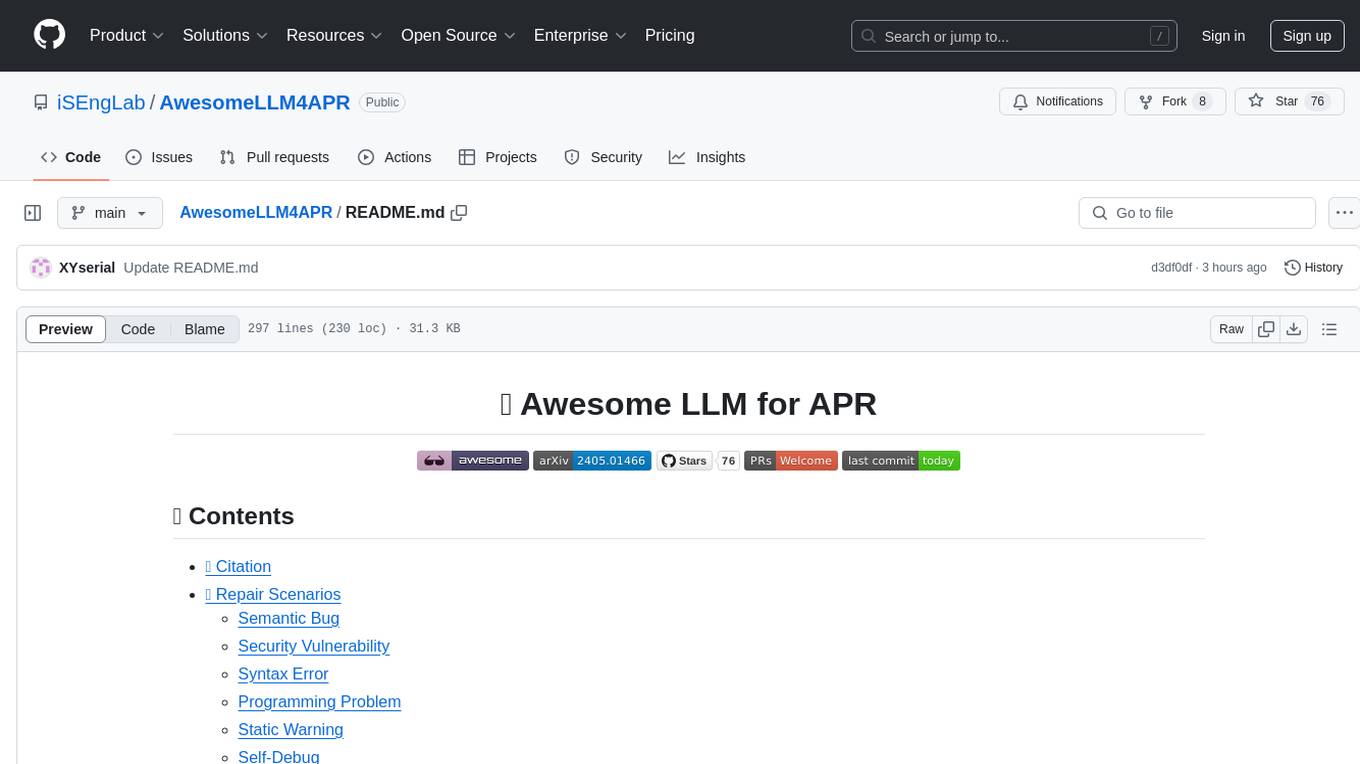
AwesomeLLM4APR
Awesome LLM for APR is a repository dedicated to exploring the capabilities of Large Language Models (LLMs) in Automated Program Repair (APR). It provides a comprehensive collection of research papers, tools, and resources related to using LLMs for various scenarios such as repairing semantic bugs, security vulnerabilities, syntax errors, programming problems, static warnings, self-debugging, type errors, web UI tests, smart contracts, hardware bugs, performance bugs, API misuses, crash bugs, test case repairs, formal proofs, GitHub issues, code reviews, motion planners, human studies, and patch correctness assessments. The repository serves as a valuable reference for researchers and practitioners interested in leveraging LLMs for automated program repair.
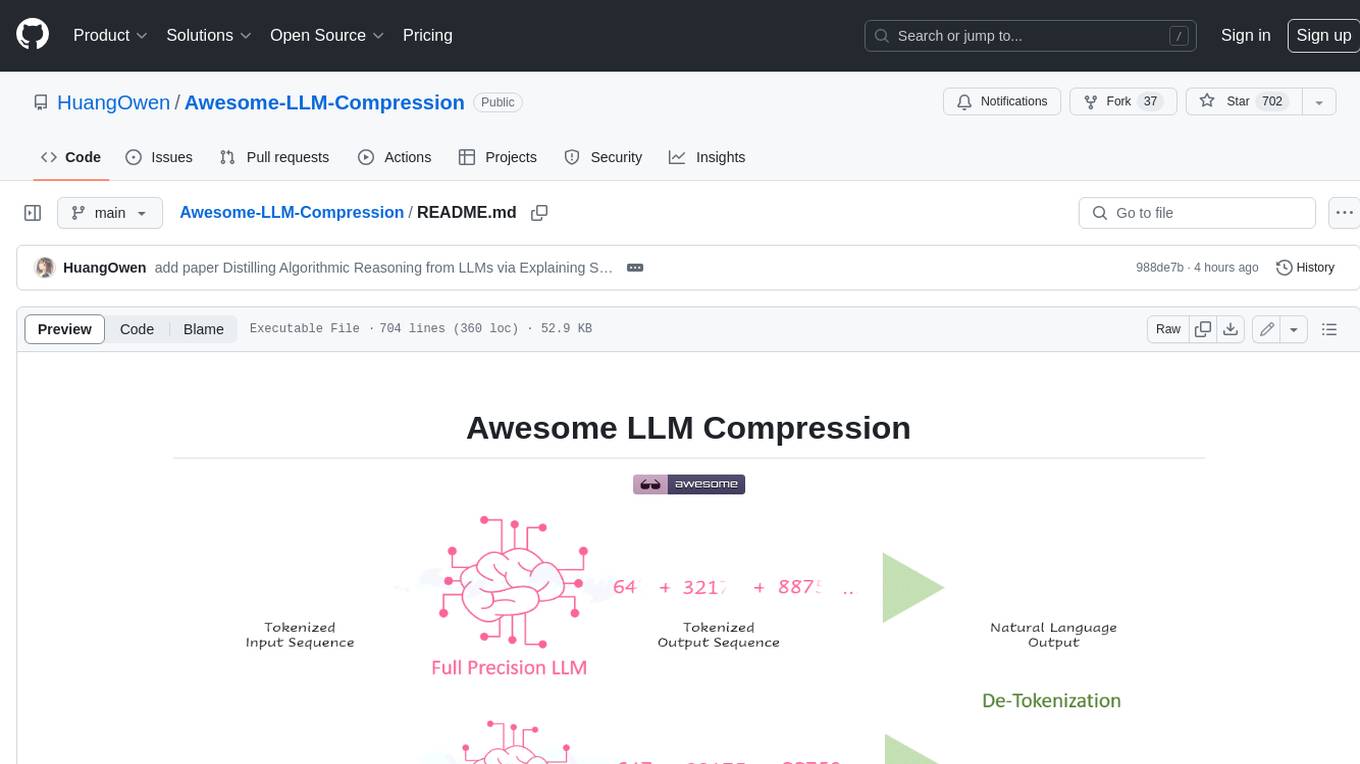
Awesome-LLM-Compression
Awesome LLM compression research papers and tools to accelerate LLM training and inference.
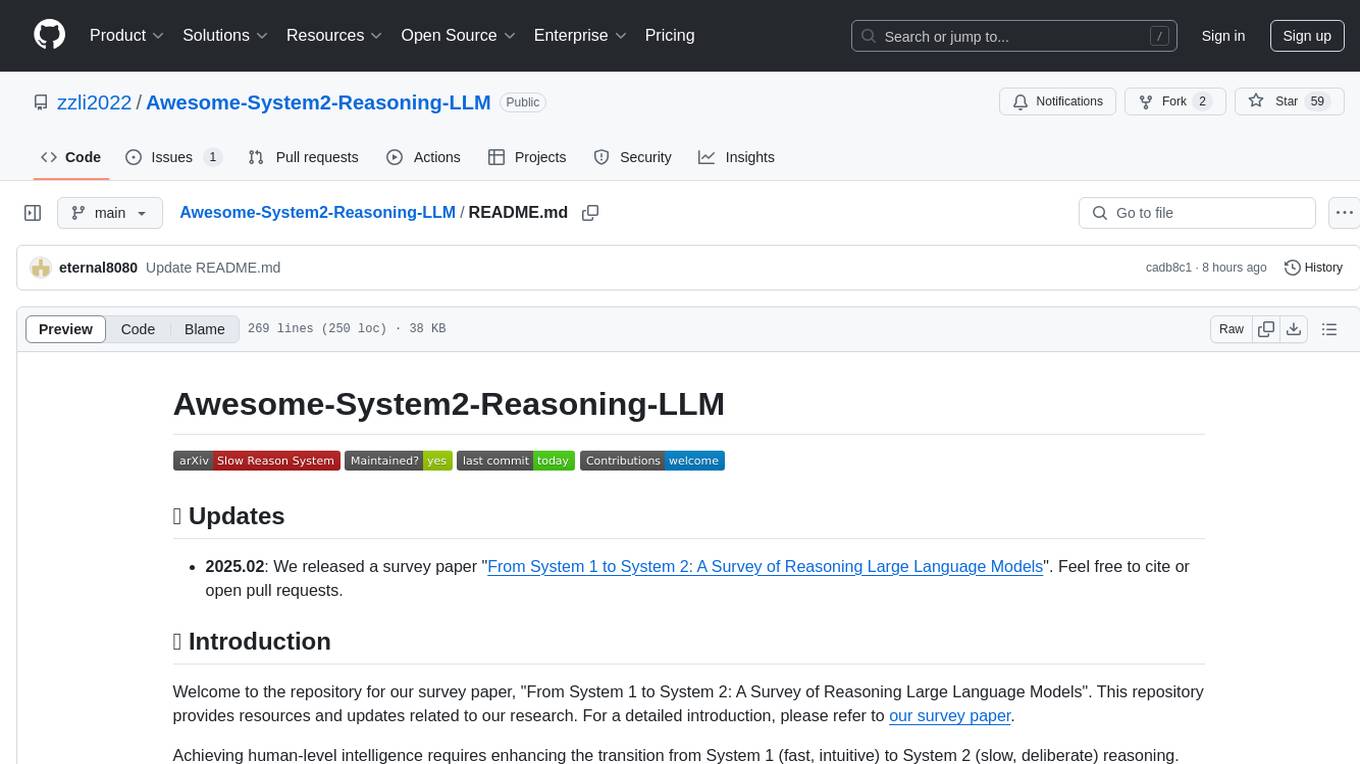
Awesome-System2-Reasoning-LLM
The Awesome-System2-Reasoning-LLM repository is dedicated to a survey paper titled 'From System 1 to System 2: A Survey of Reasoning Large Language Models'. It explores the development of reasoning Large Language Models (LLMs), their foundational technologies, benchmarks, and future directions. The repository provides resources and updates related to the research, tracking the latest developments in the field of reasoning LLMs.
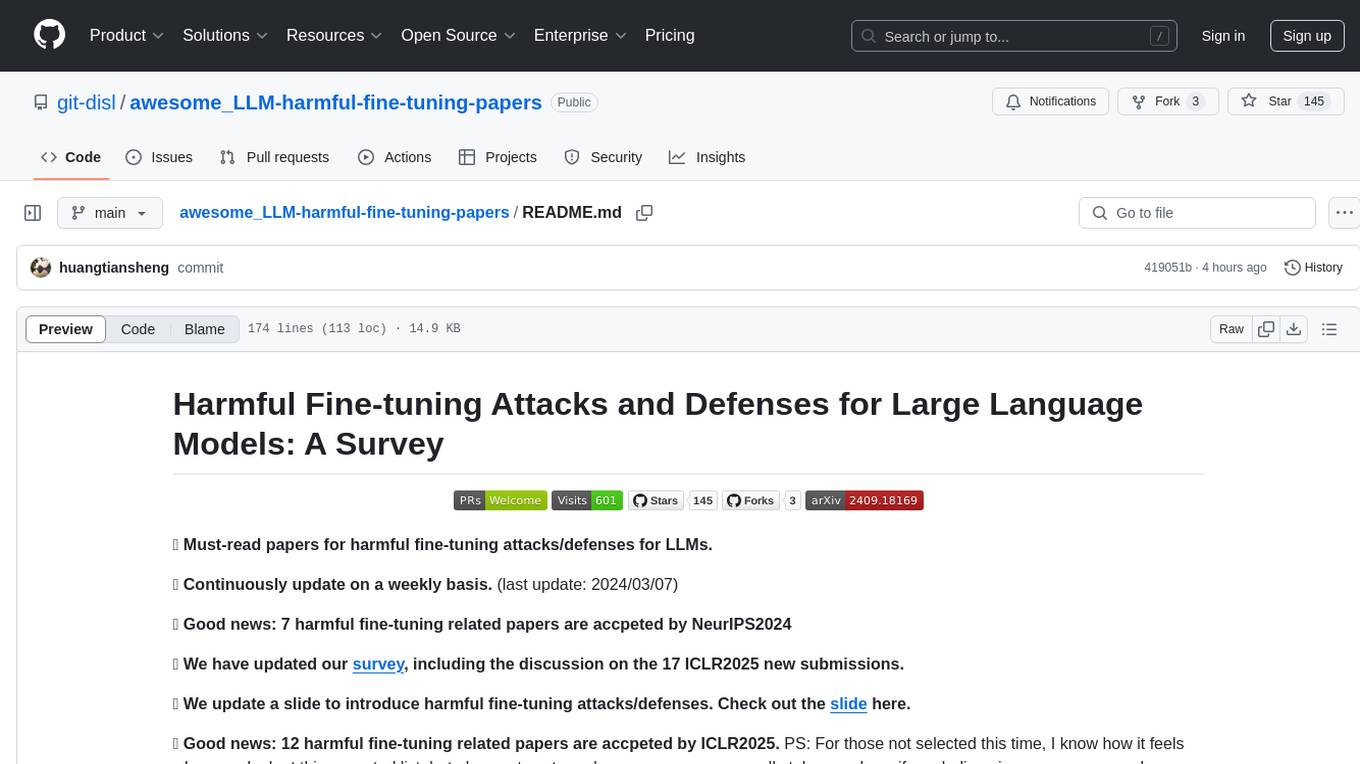
awesome_LLM-harmful-fine-tuning-papers
This repository is a comprehensive survey of harmful fine-tuning attacks and defenses for large language models (LLMs). It provides a curated list of must-read papers on the topic, covering various aspects such as alignment stage defenses, fine-tuning stage defenses, post-fine-tuning stage defenses, mechanical studies, benchmarks, and attacks/defenses for federated fine-tuning. The repository aims to keep researchers updated on the latest developments in the field and offers insights into the vulnerabilities and safeguards related to fine-tuning LLMs.
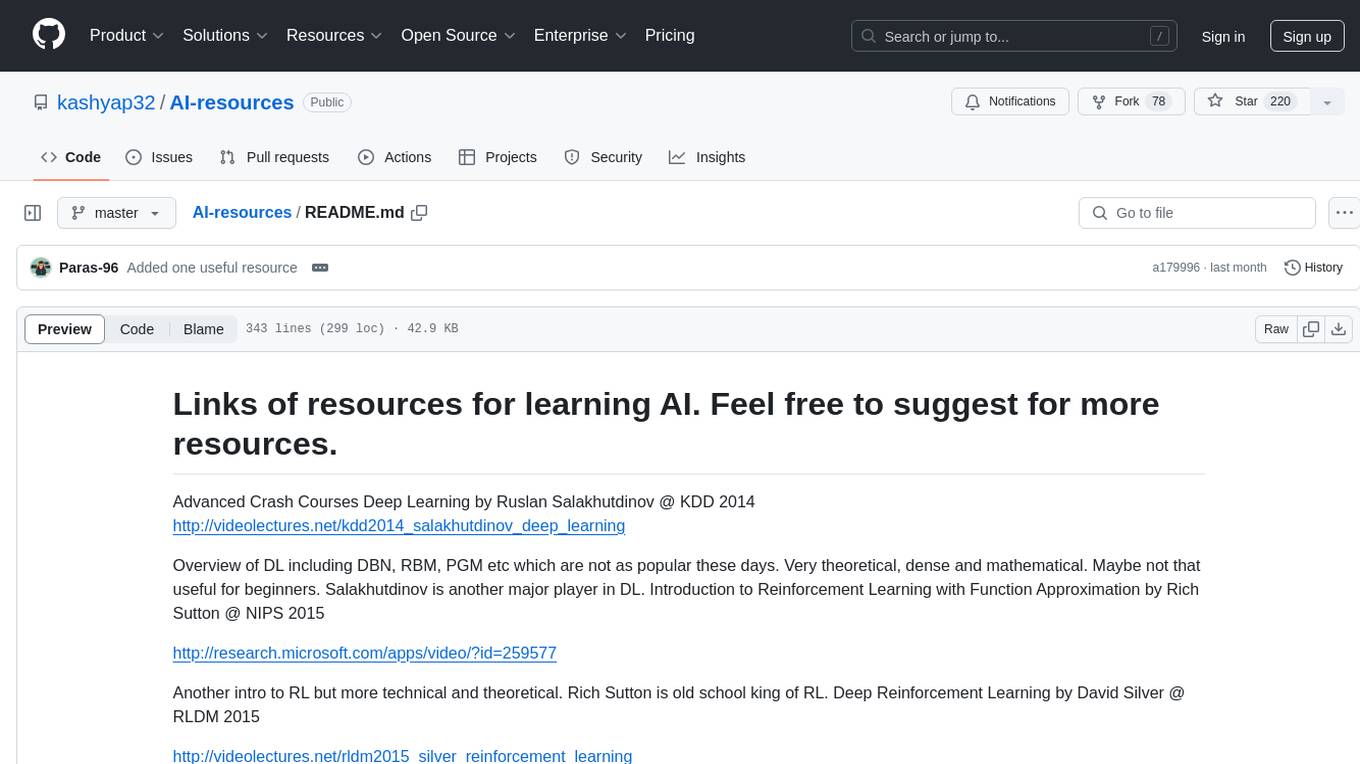
AI-resources
AI-resources is a repository containing links to various resources for learning Artificial Intelligence. It includes video lectures, courses, tutorials, and open-source libraries related to deep learning, reinforcement learning, machine learning, and more. The repository categorizes resources for beginners, average users, and advanced users/researchers, providing a comprehensive collection of materials to enhance knowledge and skills in AI.
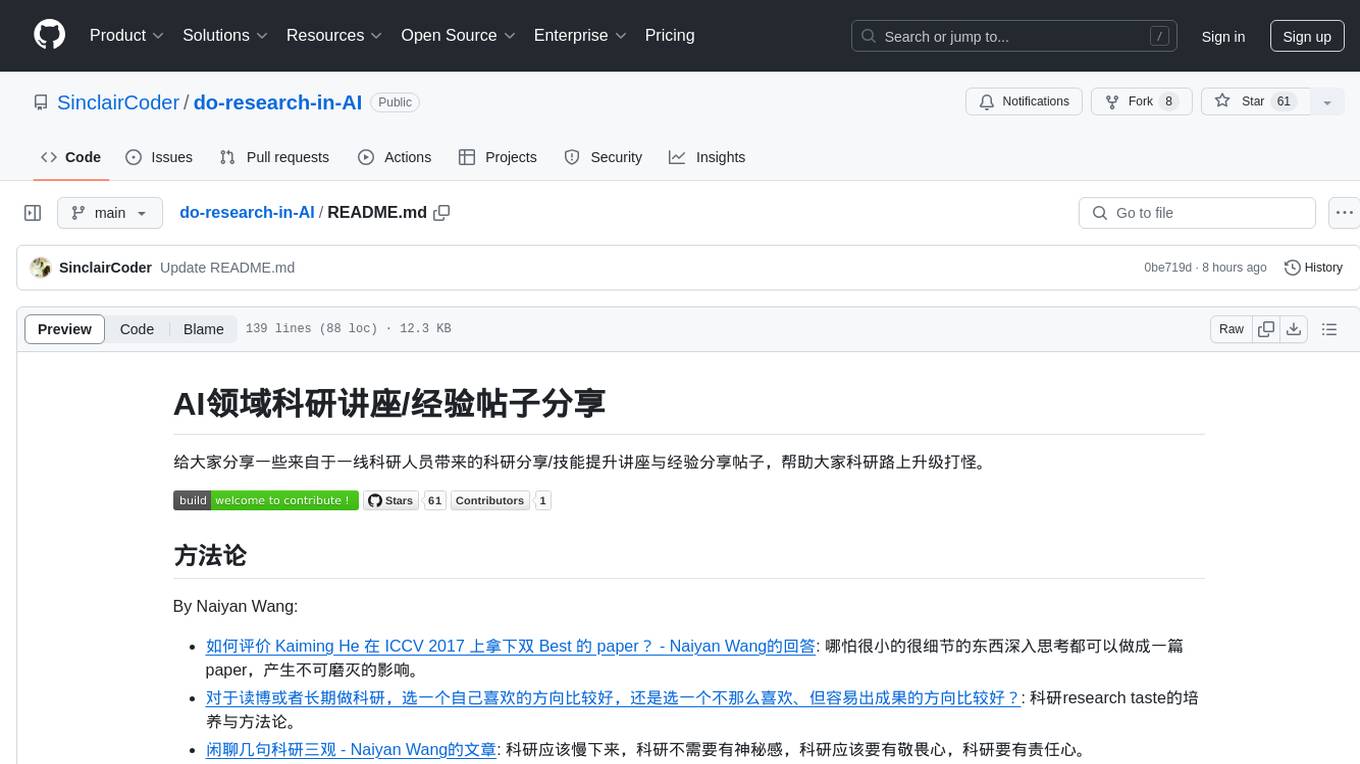
do-research-in-AI
This repository is a collection of research lectures and experience sharing posts from frontline researchers in the field of AI. It aims to help individuals upgrade their research skills and knowledge through insightful talks and experiences shared by experts. The content covers various topics such as evaluating research papers, choosing research directions, research methodologies, and tips for writing high-quality scientific papers. The repository also includes discussions on academic career paths, research ethics, and the emotional aspects of research work. Overall, it serves as a valuable resource for individuals interested in advancing their research capabilities in the field of AI.
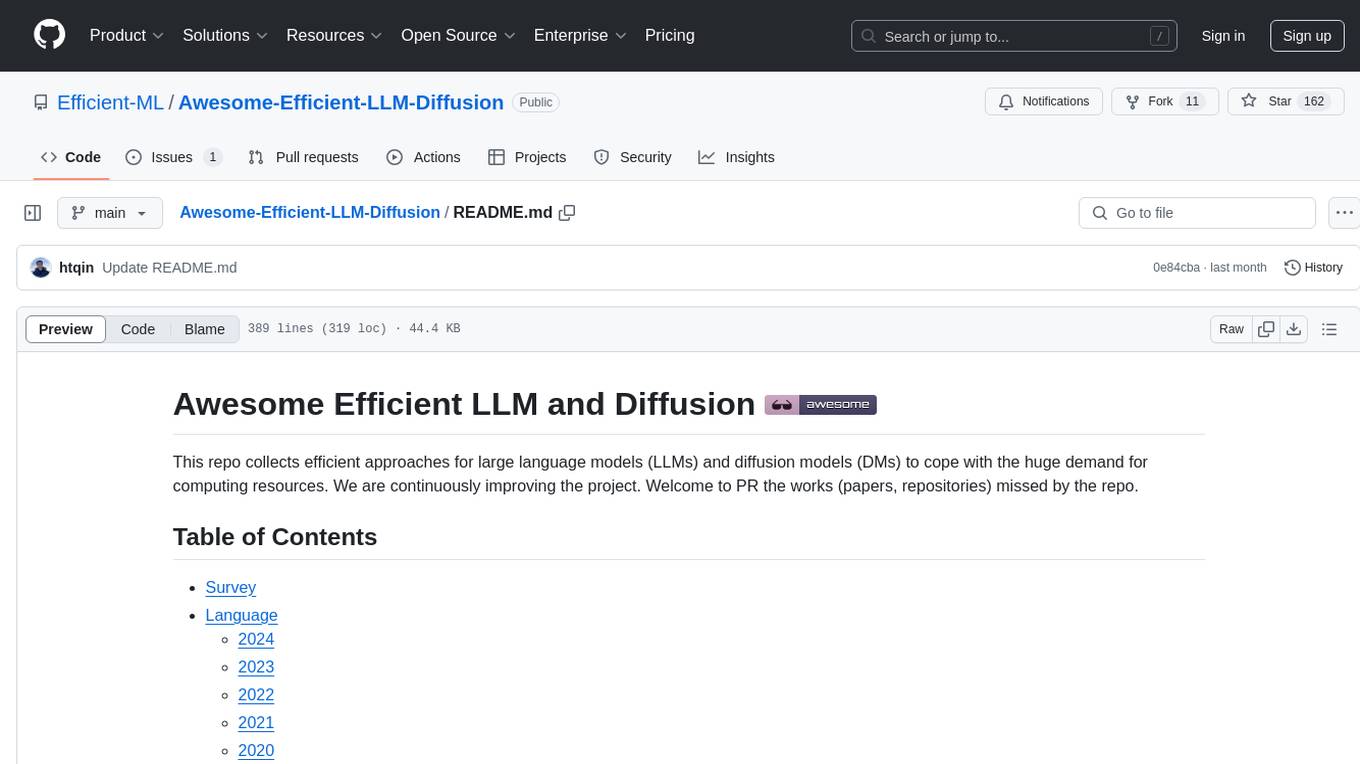
Awesome-Efficient-AIGC
This repository, Awesome Efficient AIGC, collects efficient approaches for AI-generated content (AIGC) to cope with its huge demand for computing resources. It includes efficient Large Language Models (LLMs), Diffusion Models (DMs), and more. The repository is continuously improving and welcomes contributions of works like papers and repositories that are missed by the collection.
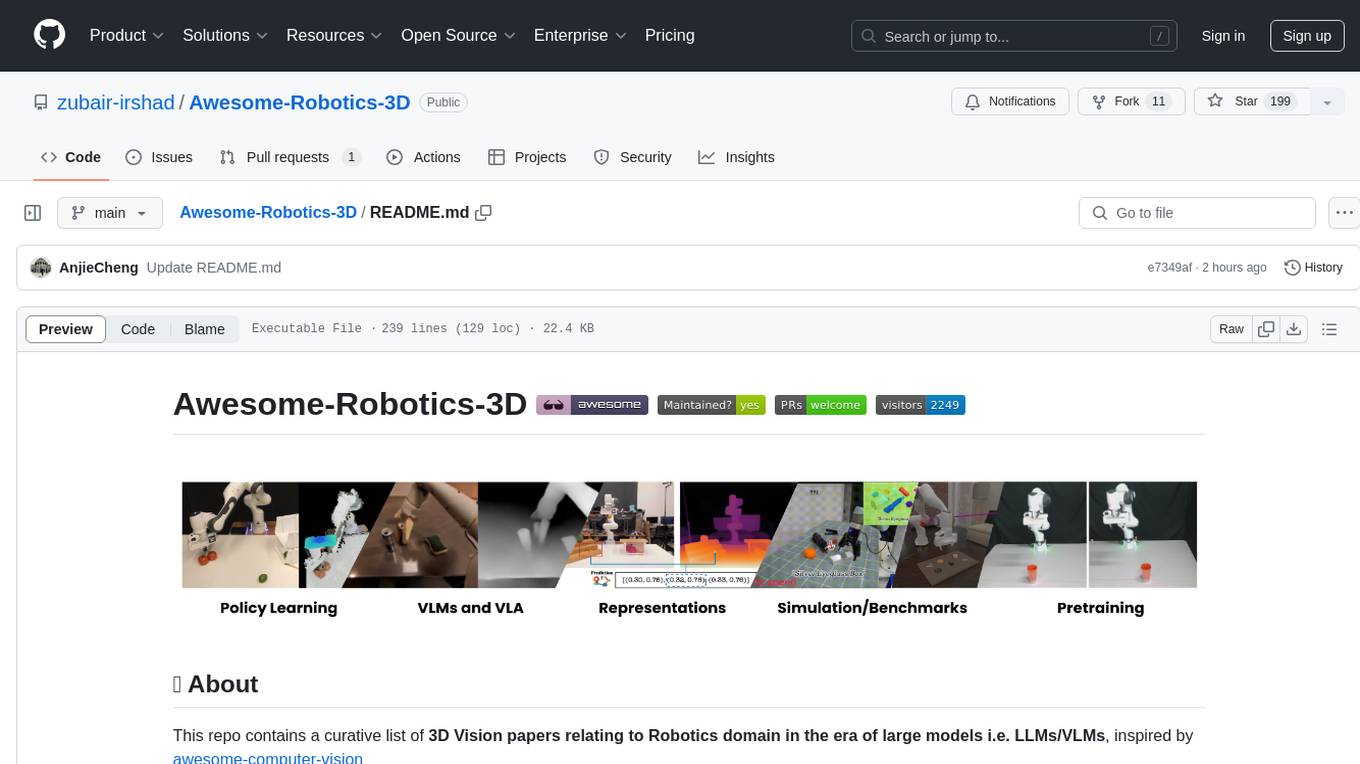
Awesome-Robotics-3D
Awesome-Robotics-3D is a curated list of 3D Vision papers related to Robotics domain, focusing on large models like LLMs/VLMs. It includes papers on Policy Learning, Pretraining, VLM and LLM, Representations, and Simulations, Datasets, and Benchmarks. The repository is maintained by Zubair Irshad and welcomes contributions and suggestions for adding papers. It serves as a valuable resource for researchers and practitioners in the field of Robotics and Computer Vision.
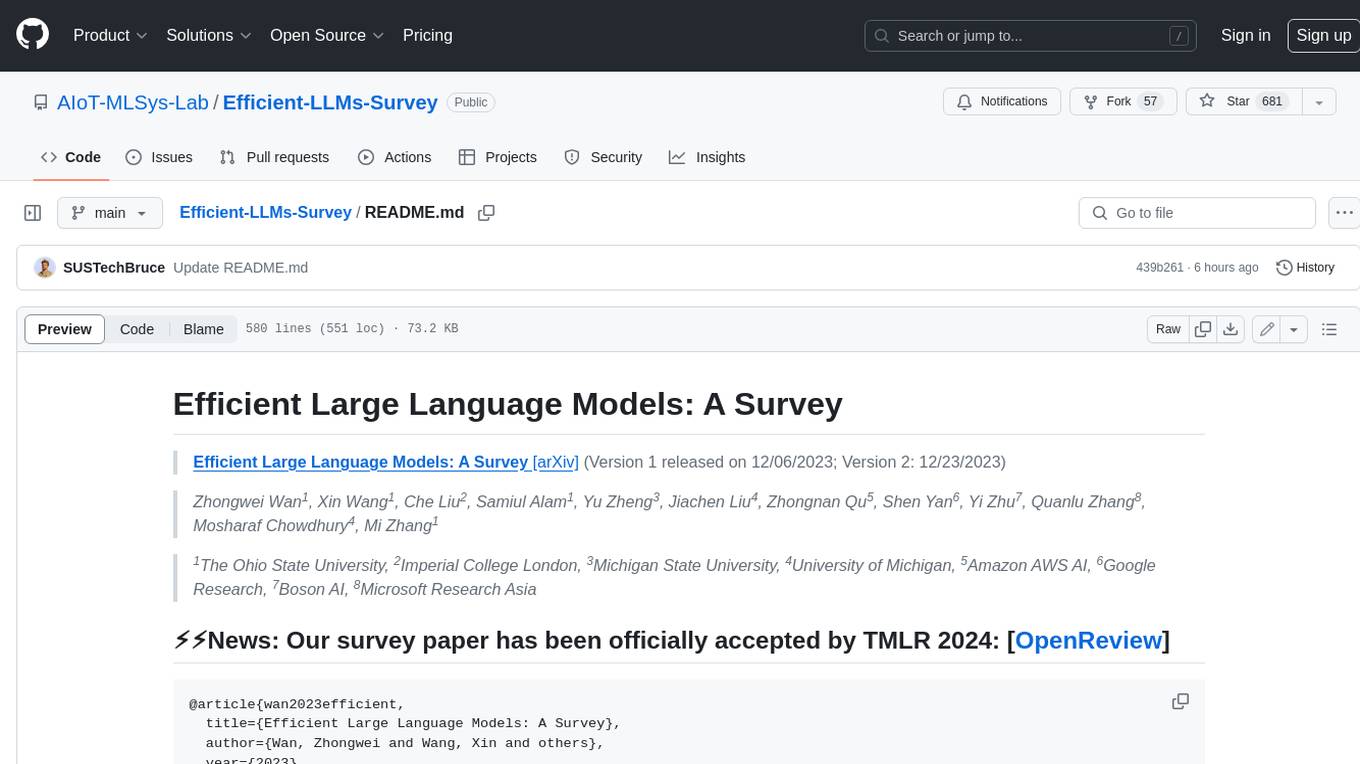
Efficient-LLMs-Survey
This repository provides a systematic and comprehensive review of efficient LLMs research. We organize the literature in a taxonomy consisting of three main categories, covering distinct yet interconnected efficient LLMs topics from **model-centric** , **data-centric** , and **framework-centric** perspective, respectively. We hope our survey and this GitHub repository can serve as valuable resources to help researchers and practitioners gain a systematic understanding of the research developments in efficient LLMs and inspire them to contribute to this important and exciting field.
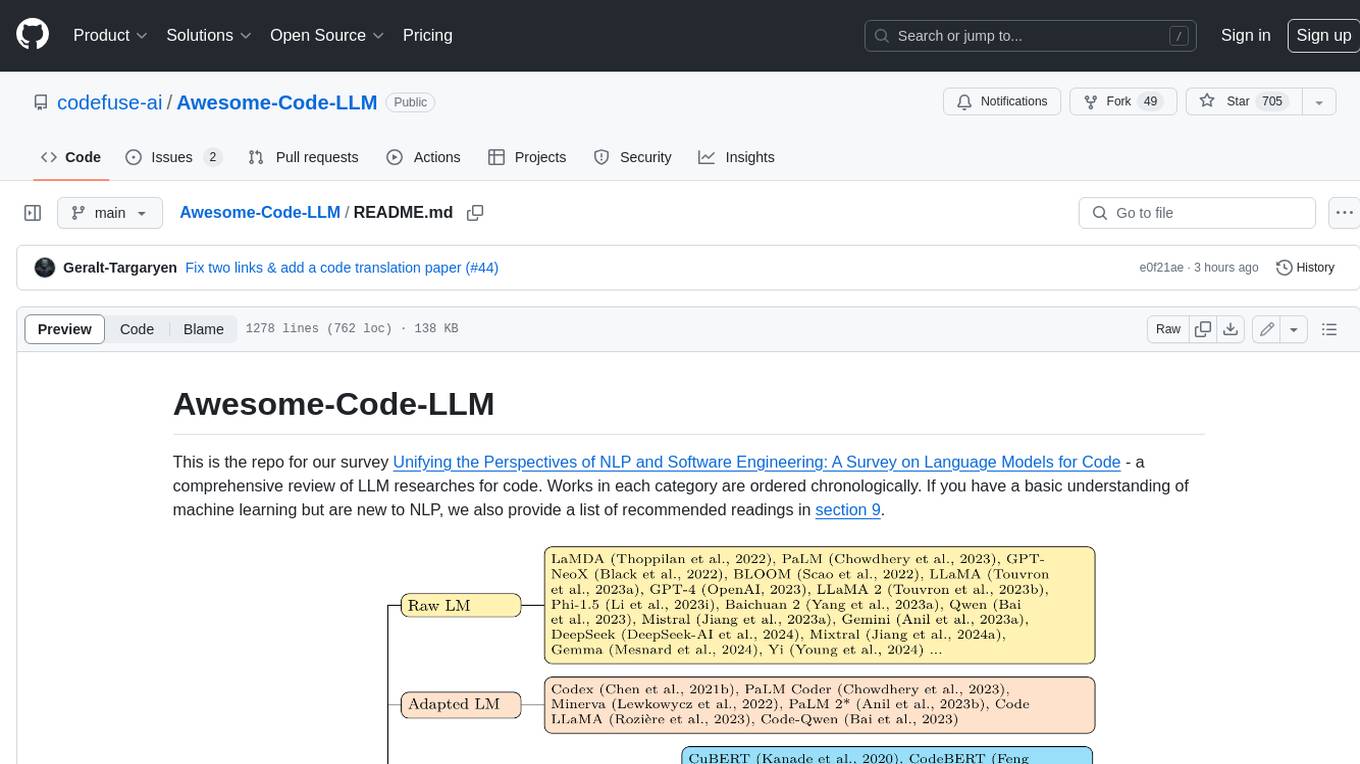
Awesome-Code-LLM
Analyze the following text from a github repository (name and readme text at end) . Then, generate a JSON object with the following keys and provide the corresponding information for each key, in lowercase letters: 'description' (detailed description of the repo, must be less than 400 words,Ensure that no line breaks and quotation marks.),'for_jobs' (List 5 jobs suitable for this tool,in lowercase letters), 'ai_keywords' (keywords of the tool,user may use those keyword to find the tool,in lowercase letters), 'for_tasks' (list of 5 specific tasks user can use this tool to do,in lowercase letters), 'answer' (in english languages)
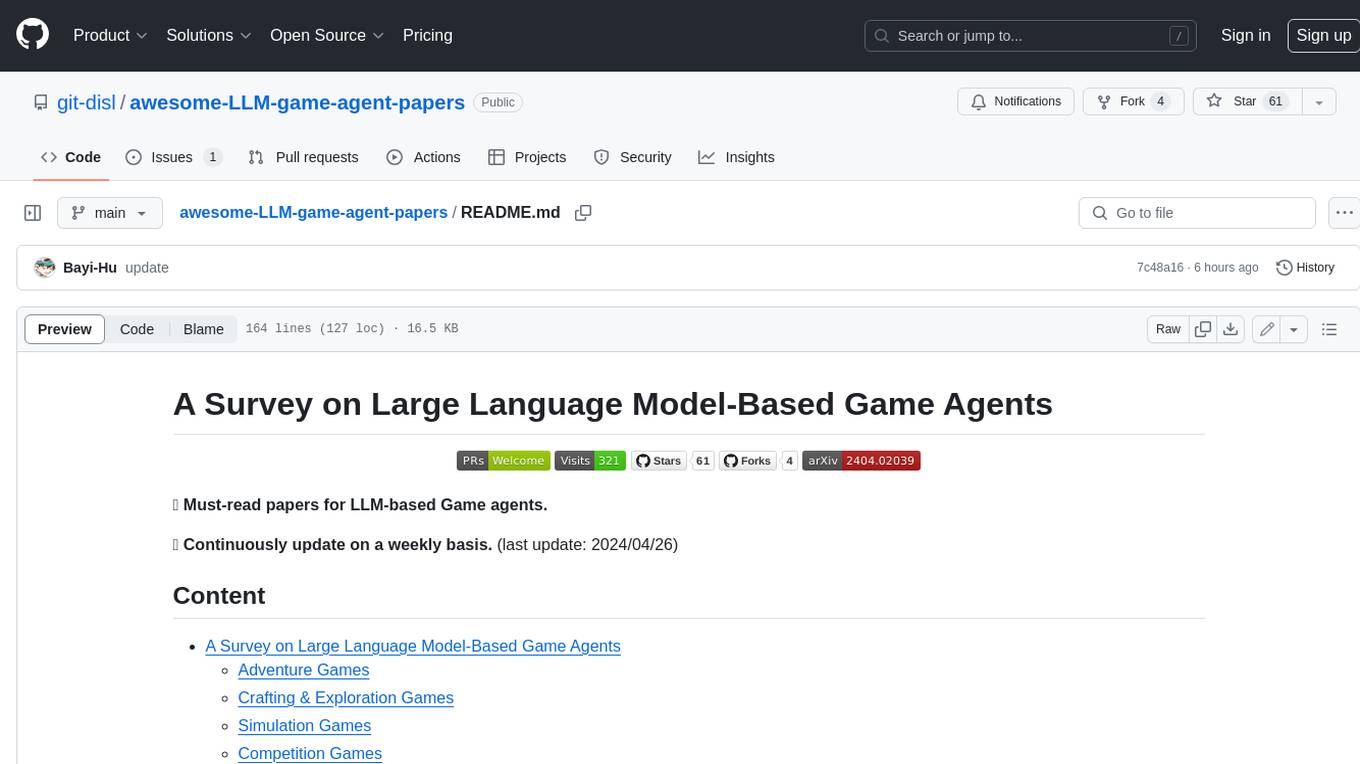
awesome-LLM-game-agent-papers
This repository provides a comprehensive survey of research papers on large language model (LLM)-based game agents. LLMs are powerful AI models that can understand and generate human language, and they have shown great promise for developing intelligent game agents. This survey covers a wide range of topics, including adventure games, crafting and exploration games, simulation games, competition games, cooperation games, communication games, and action games. For each topic, the survey provides an overview of the state-of-the-art research, as well as a discussion of the challenges and opportunities for future work.
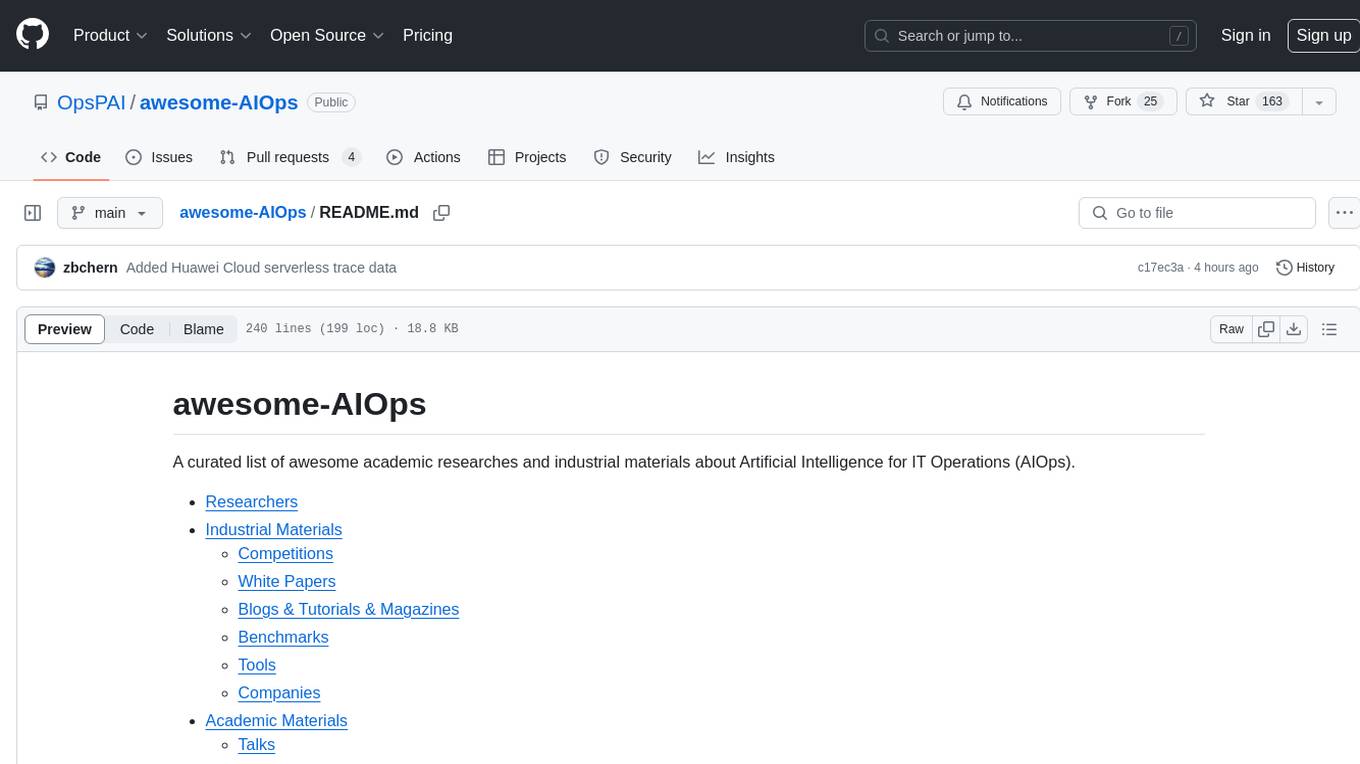
awesome-AIOps
awesome-AIOps is a curated list of academic researches and industrial materials related to Artificial Intelligence for IT Operations (AIOps). It includes resources such as competitions, white papers, blogs, tutorials, benchmarks, tools, companies, academic materials, talks, workshops, papers, and courses covering various aspects of AIOps like anomaly detection, root cause analysis, incident management, microservices, dependency tracing, and more.
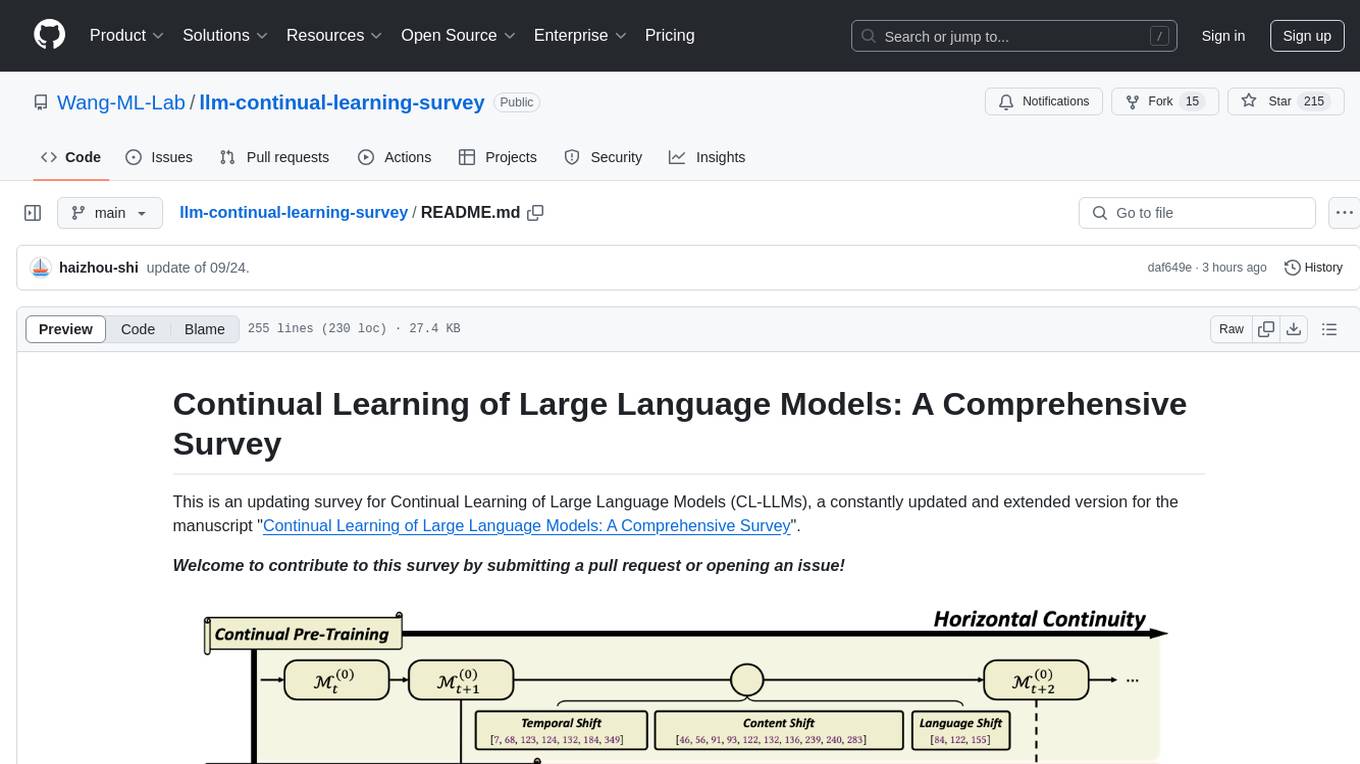
llm-continual-learning-survey
This repository is an updating survey for Continual Learning of Large Language Models (CL-LLMs), providing a comprehensive overview of various aspects related to the continual learning of large language models. It covers topics such as continual pre-training, domain-adaptive pre-training, continual fine-tuning, model refinement, model alignment, multimodal LLMs, and miscellaneous aspects. The survey includes a collection of relevant papers, each focusing on different areas within the field of continual learning of large language models.
For similar tasks
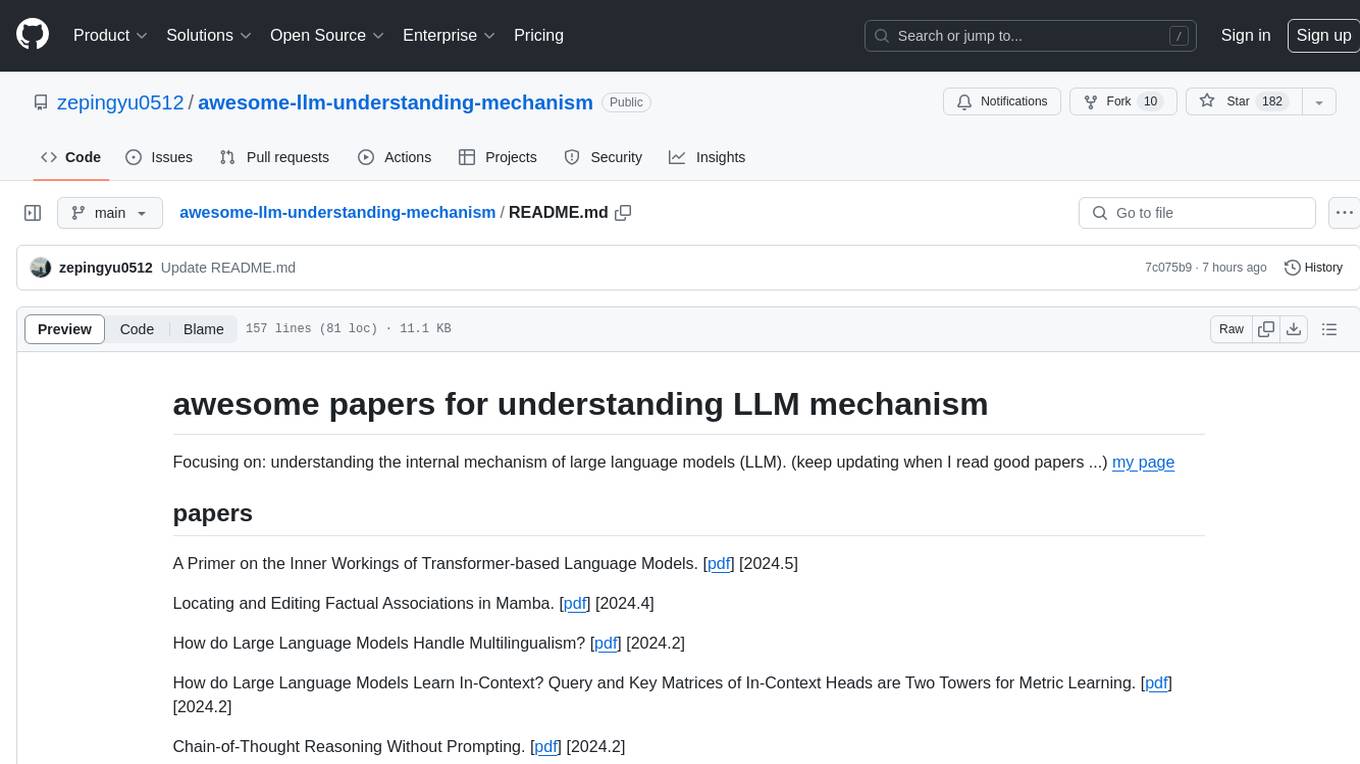
awesome-llm-understanding-mechanism
This repository is a collection of papers focused on understanding the internal mechanism of large language models (LLM). It includes research on topics such as how LLMs handle multilingualism, learn in-context, and handle factual associations. The repository aims to provide insights into the inner workings of transformer-based language models through a curated list of papers and surveys.
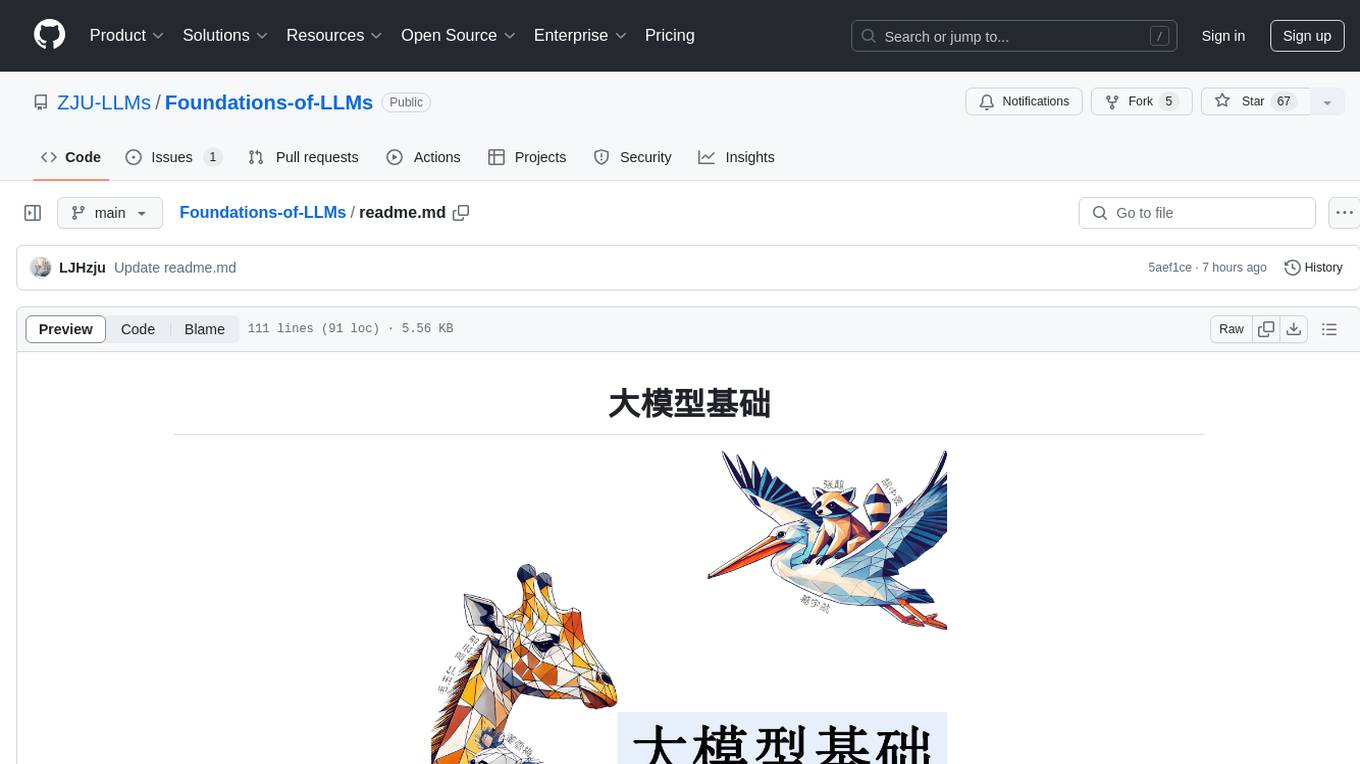
Foundations-of-LLMs
Foundations-of-LLMs is a comprehensive book aimed at readers interested in large language models, providing systematic explanations of foundational knowledge and introducing cutting-edge technologies. The book covers traditional language models, evolution of large language model architectures, prompt engineering, parameter-efficient fine-tuning, model editing, and retrieval-enhanced generation. Each chapter uses an animal as a theme to explain specific technologies, enhancing readability. The content is based on the author team's exploration and understanding of the field, with continuous monthly updates planned. The book includes a 'Paper List' for each chapter to track the latest advancements in related technologies.
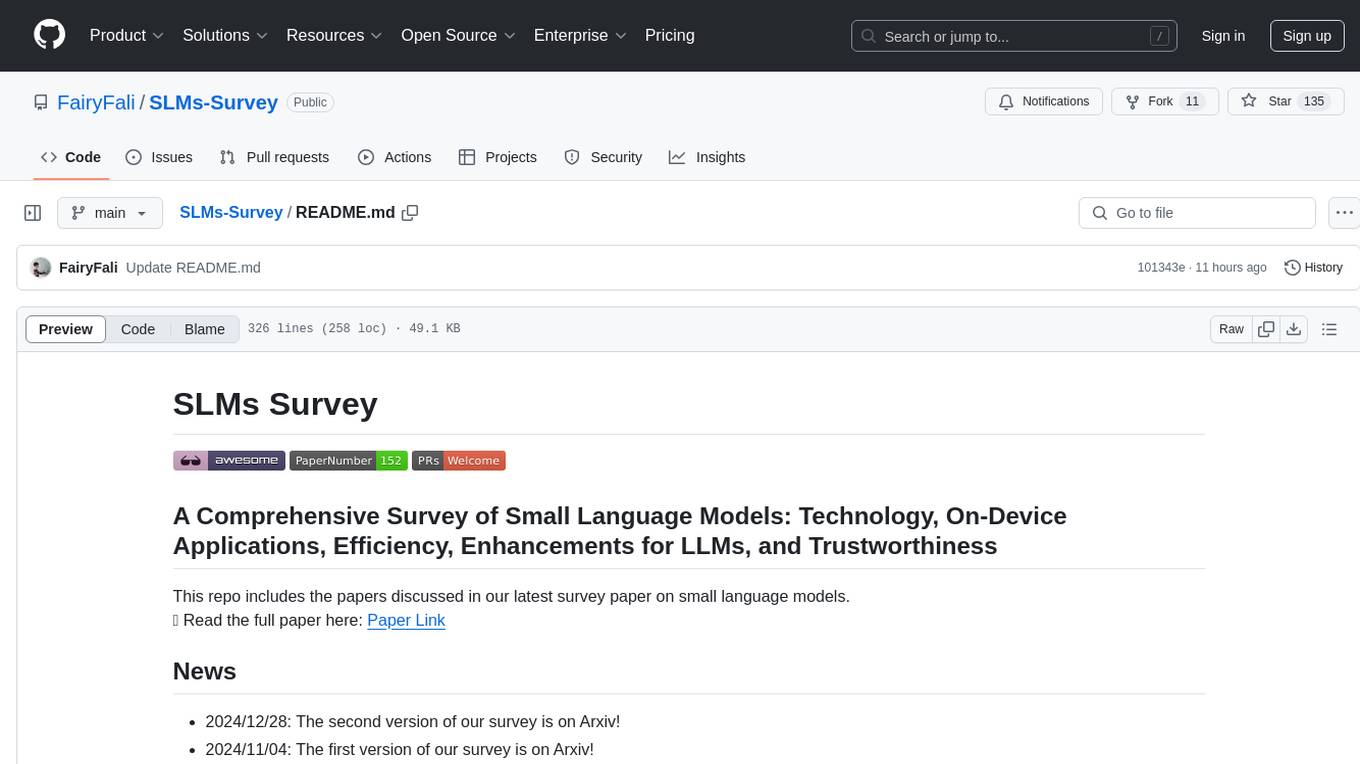
SLMs-Survey
SLMs-Survey is a comprehensive repository that includes papers and surveys on small language models. It covers topics such as technology, on-device applications, efficiency, enhancements for LLMs, and trustworthiness. The repository provides a detailed overview of existing SLMs, their architecture, enhancements, and specific applications in various domains. It also includes information on SLM deployment optimization techniques and the synergy between SLMs and LLMs.
For similar jobs
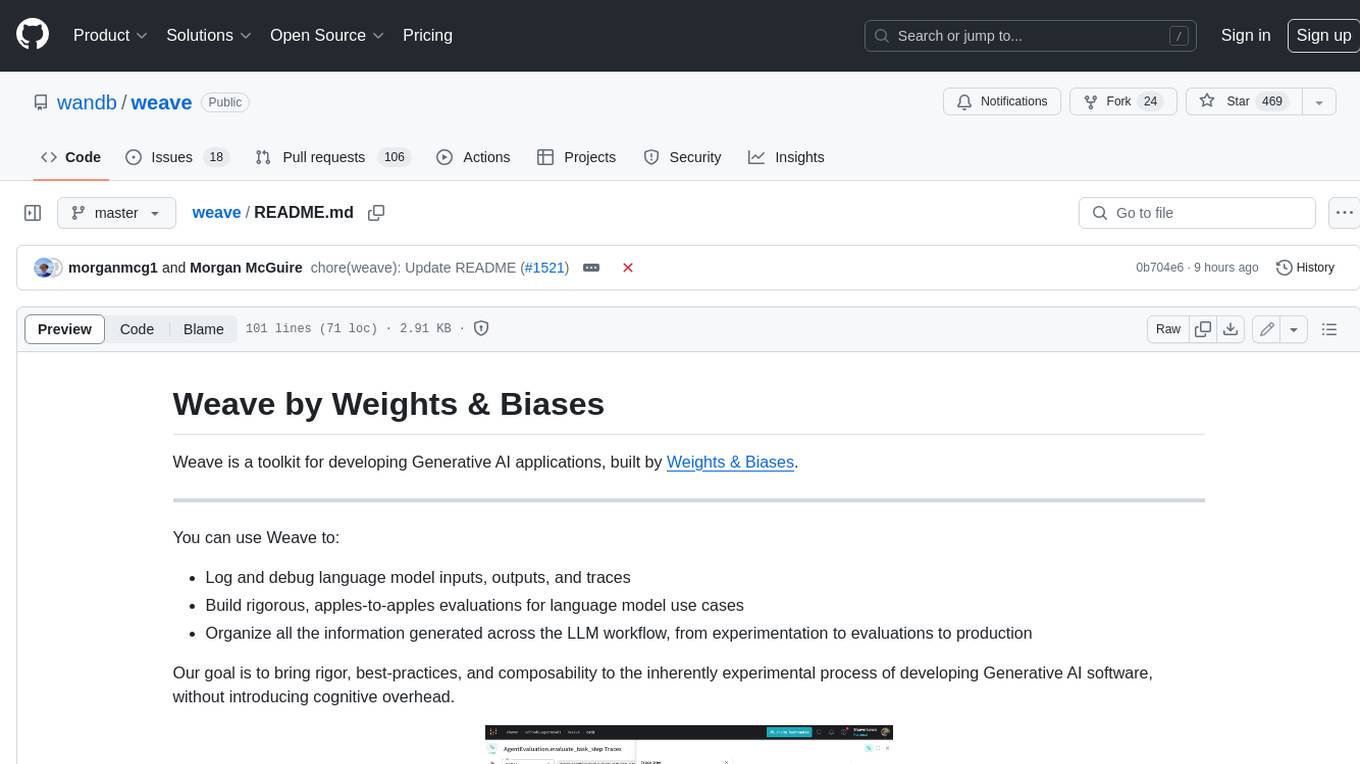
weave
Weave is a toolkit for developing Generative AI applications, built by Weights & Biases. With Weave, you can log and debug language model inputs, outputs, and traces; build rigorous, apples-to-apples evaluations for language model use cases; and organize all the information generated across the LLM workflow, from experimentation to evaluations to production. Weave aims to bring rigor, best-practices, and composability to the inherently experimental process of developing Generative AI software, without introducing cognitive overhead.
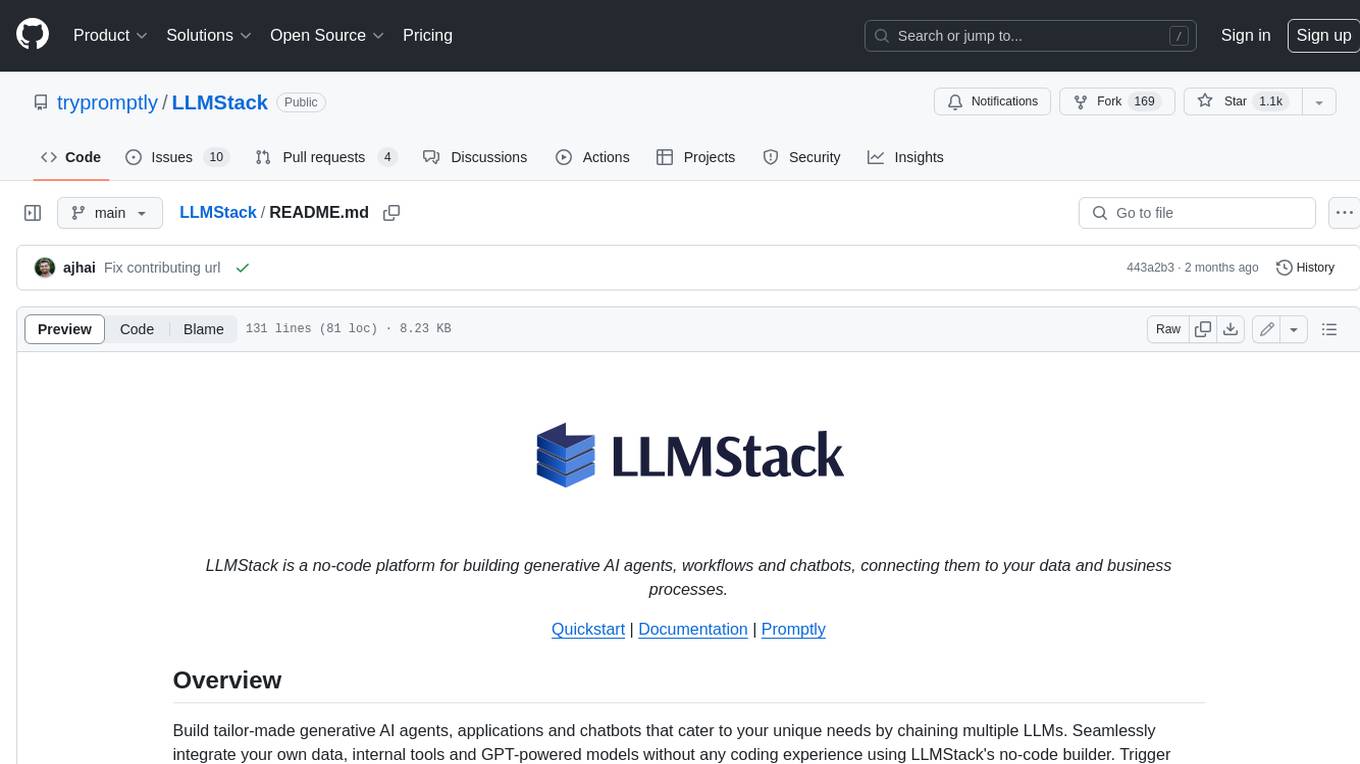
LLMStack
LLMStack is a no-code platform for building generative AI agents, workflows, and chatbots. It allows users to connect their own data, internal tools, and GPT-powered models without any coding experience. LLMStack can be deployed to the cloud or on-premise and can be accessed via HTTP API or triggered from Slack or Discord.
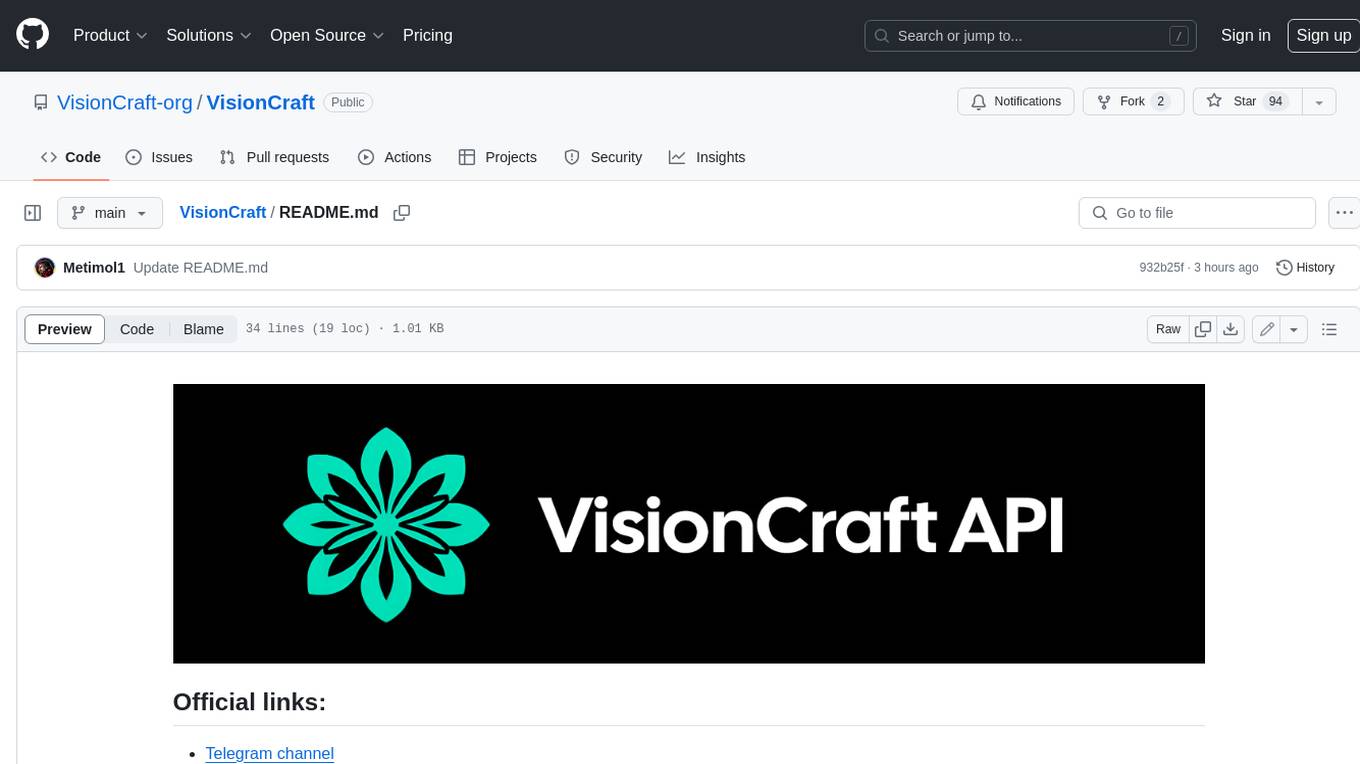
VisionCraft
The VisionCraft API is a free API for using over 100 different AI models. From images to sound.
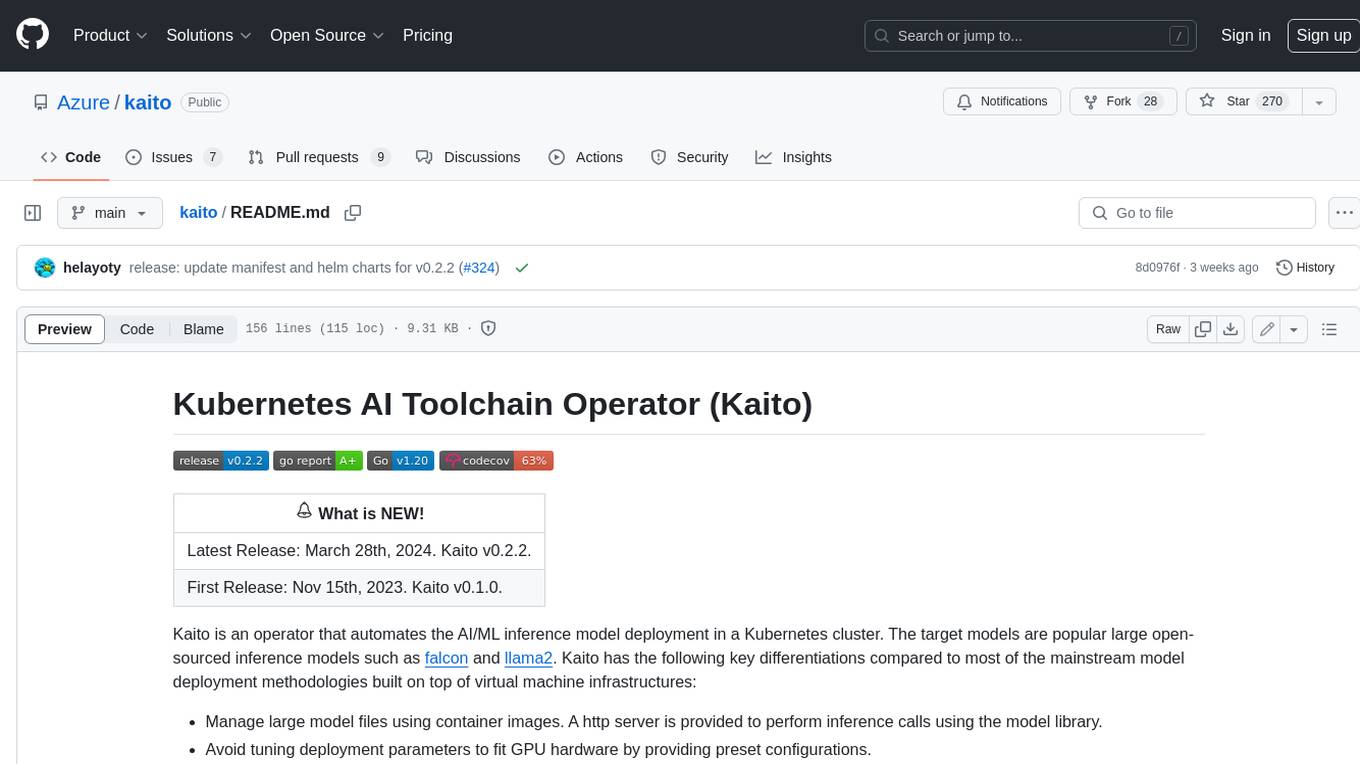
kaito
Kaito is an operator that automates the AI/ML inference model deployment in a Kubernetes cluster. It manages large model files using container images, avoids tuning deployment parameters to fit GPU hardware by providing preset configurations, auto-provisions GPU nodes based on model requirements, and hosts large model images in the public Microsoft Container Registry (MCR) if the license allows. Using Kaito, the workflow of onboarding large AI inference models in Kubernetes is largely simplified.
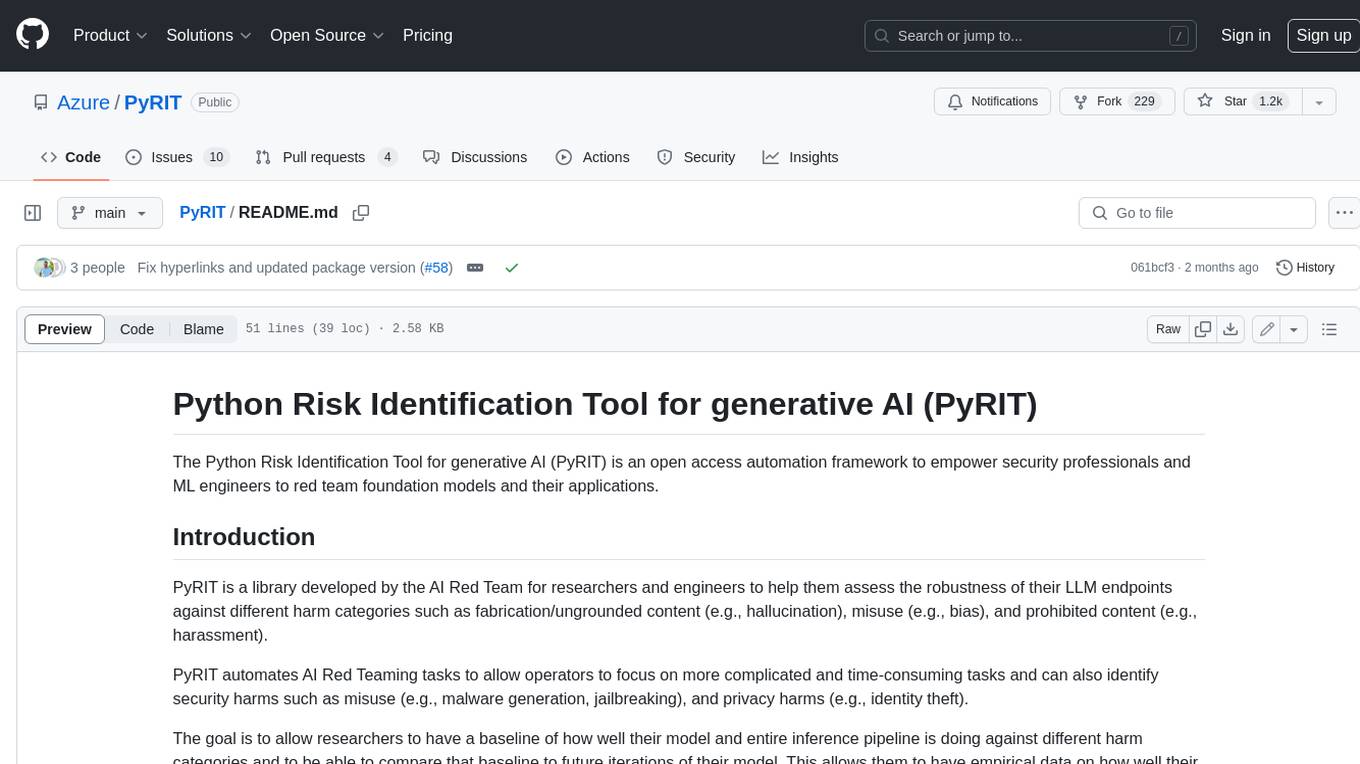
PyRIT
PyRIT is an open access automation framework designed to empower security professionals and ML engineers to red team foundation models and their applications. It automates AI Red Teaming tasks to allow operators to focus on more complicated and time-consuming tasks and can also identify security harms such as misuse (e.g., malware generation, jailbreaking), and privacy harms (e.g., identity theft). The goal is to allow researchers to have a baseline of how well their model and entire inference pipeline is doing against different harm categories and to be able to compare that baseline to future iterations of their model. This allows them to have empirical data on how well their model is doing today, and detect any degradation of performance based on future improvements.
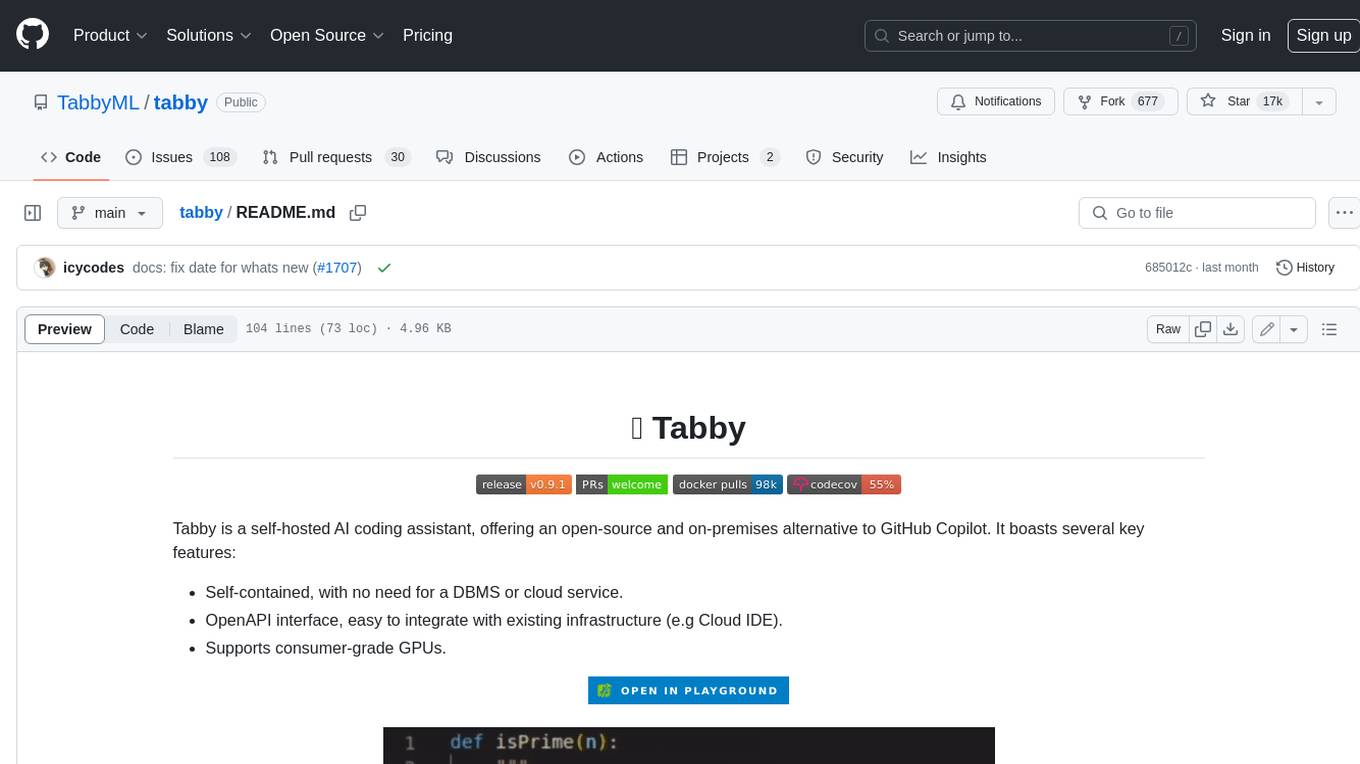
tabby
Tabby is a self-hosted AI coding assistant, offering an open-source and on-premises alternative to GitHub Copilot. It boasts several key features: * Self-contained, with no need for a DBMS or cloud service. * OpenAPI interface, easy to integrate with existing infrastructure (e.g Cloud IDE). * Supports consumer-grade GPUs.
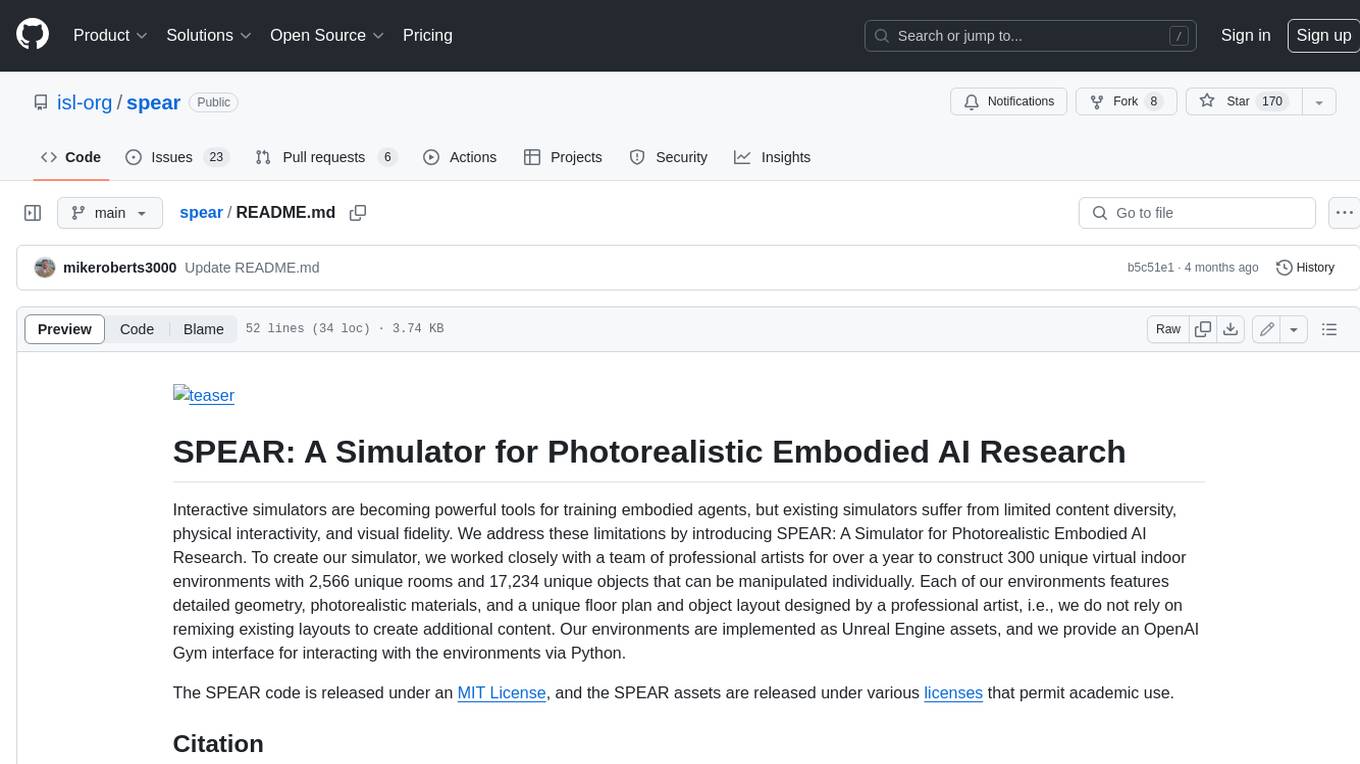
spear
SPEAR (Simulator for Photorealistic Embodied AI Research) is a powerful tool for training embodied agents. It features 300 unique virtual indoor environments with 2,566 unique rooms and 17,234 unique objects that can be manipulated individually. Each environment is designed by a professional artist and features detailed geometry, photorealistic materials, and a unique floor plan and object layout. SPEAR is implemented as Unreal Engine assets and provides an OpenAI Gym interface for interacting with the environments via Python.
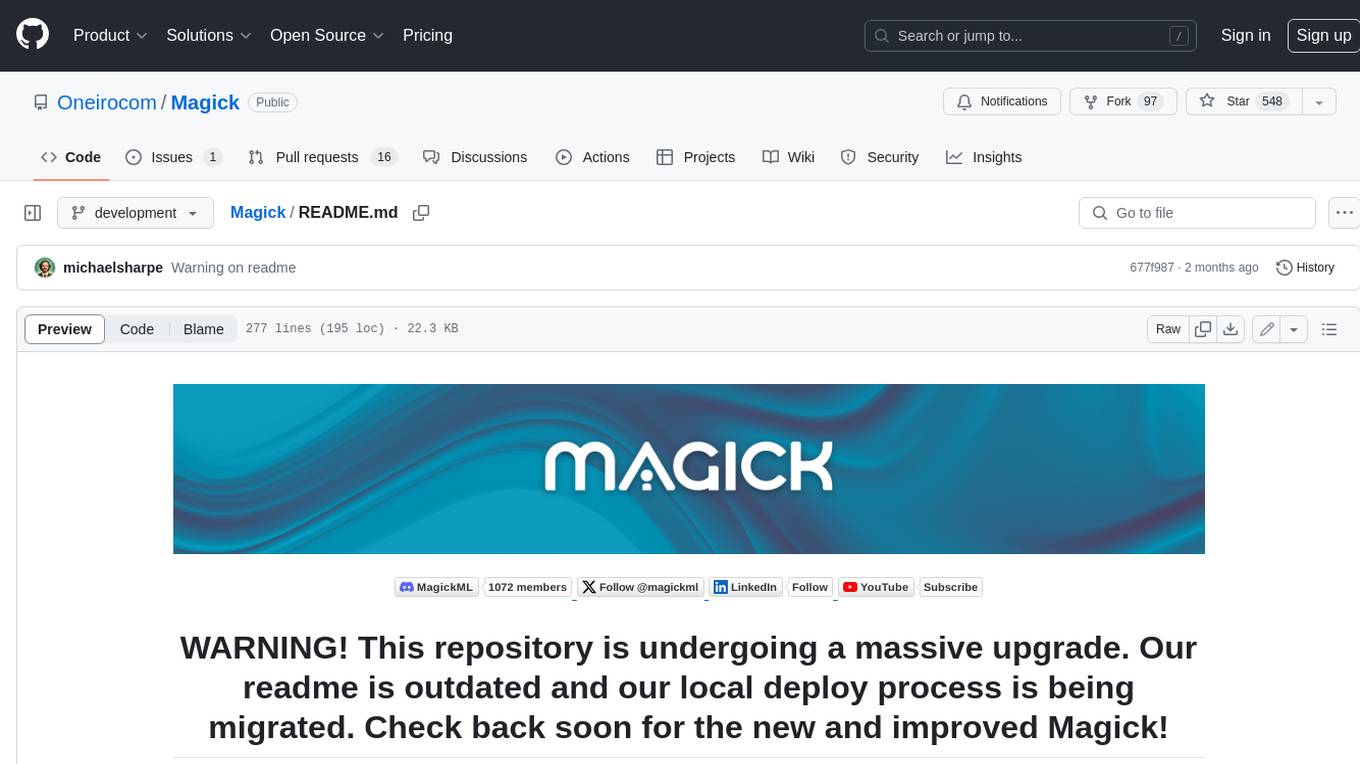
Magick
Magick is a groundbreaking visual AIDE (Artificial Intelligence Development Environment) for no-code data pipelines and multimodal agents. Magick can connect to other services and comes with nodes and templates well-suited for intelligent agents, chatbots, complex reasoning systems and realistic characters.