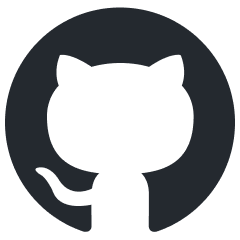
verl
verl: Volcano Engine Reinforcement Learning for LLMs
Stars: 4051
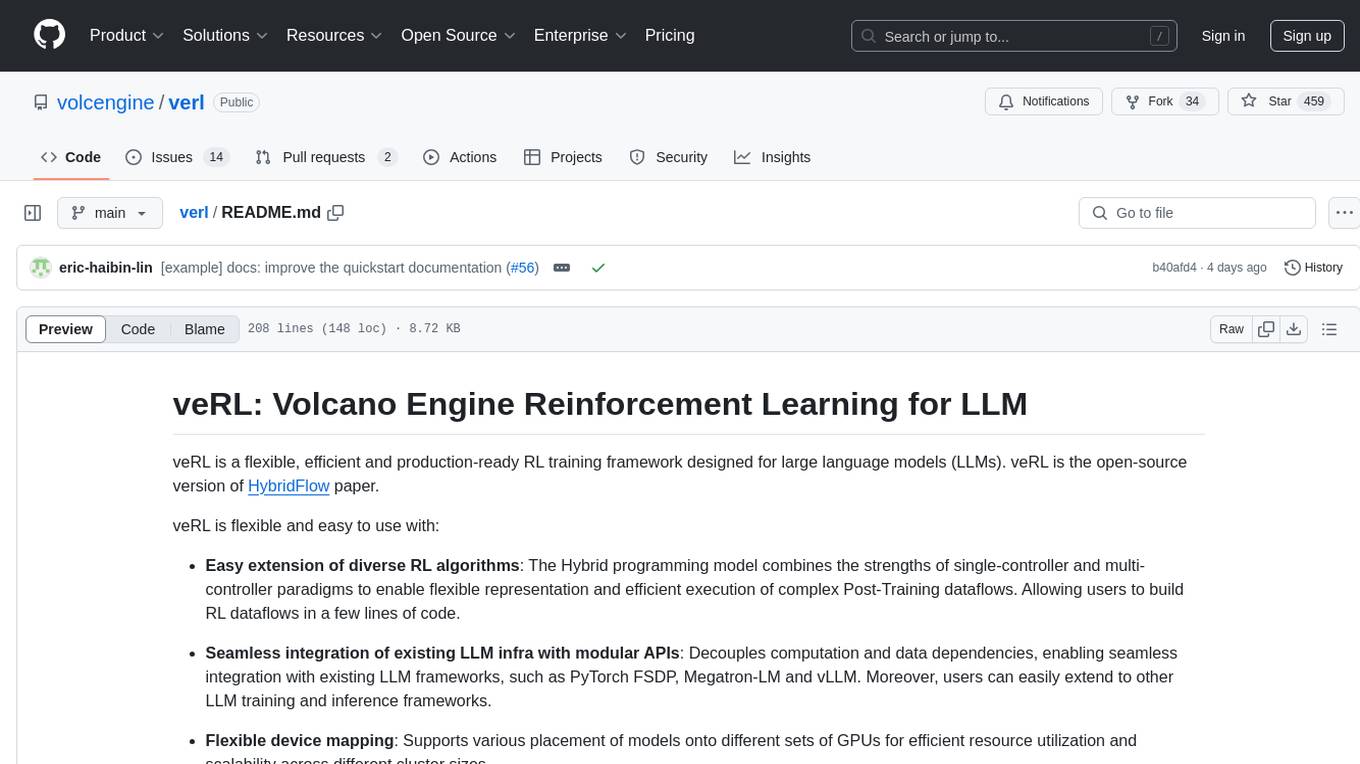
veRL is a flexible and efficient reinforcement learning training framework designed for large language models (LLMs). It allows easy extension of diverse RL algorithms, seamless integration with existing LLM infrastructures, and flexible device mapping. The framework achieves state-of-the-art throughput and efficient actor model resharding with 3D-HybridEngine. It supports popular HuggingFace models and is suitable for users working with PyTorch FSDP, Megatron-LM, and vLLM backends.
README:
verl is a flexible, efficient and production-ready RL training library for large language models (LLMs).
verl is the open-source version of HybridFlow: A Flexible and Efficient RLHF Framework paper.
verl is flexible and easy to use with:
-
Easy extension of diverse RL algorithms: The Hybrid programming model combines the strengths of single-controller and multi-controller paradigms to enable flexible representation and efficient execution of complex Post-Training dataflows. Allowing users to build RL dataflows in a few lines of code.
-
Seamless integration of existing LLM infra with modular APIs: Decouples computation and data dependencies, enabling seamless integration with existing LLM frameworks, such as PyTorch FSDP, Megatron-LM and vLLM. Moreover, users can easily extend to other LLM training and inference frameworks.
-
Flexible device mapping: Supports various placement of models onto different sets of GPUs for efficient resource utilization and scalability across different cluster sizes.
-
Readily integration with popular HuggingFace models
verl is fast with:
-
State-of-the-art throughput: By seamlessly integrating existing SOTA LLM training and inference frameworks, verl achieves high generation and training throughput.
-
Efficient actor model resharding with 3D-HybridEngine: Eliminates memory redundancy and significantly reduces communication overhead during transitions between training and generation phases.
| Documentation | Paper | Slack | Wechat | Twitter
- [2025/3] We will present verl(HybridFlow) at EuroSys 2025. See you in in Rotterdam!
- [2025/2] verl v0.2.0.post1 is released! See release note for details.
- [2025/2] We presented verl in the Bytedance/NVIDIA/Anyscale Ray Meetup. See you in San Jose!
- [2025/1] Doubao-1.5-pro is released with SOTA-level performance on LLM & VLM. The RL scaling preview model is trained using verl, reaching OpenAI O1-level performance on math benchmarks (70.0 pass@1 on AIME).
- [2024/12] The team presented Post-training LLMs: From Algorithms to Infrastructure at NeurIPS 2024. Slides and video available.
- [2024/12] verl is presented at Ray Forward 2024. Slides available here.
- [2024/10] verl is presented at Ray Summit. Youtube video available.
- [2024/08] HybridFlow (verl) is accepted to EuroSys 2025.
- FSDP and Megatron-LM for training.
- vLLM and TGI for rollout generation, SGLang support coming soon.
- huggingface models support
- Supervised fine-tuning
- Reinforcement learning from human feedback with PPO, GRPO, ReMax, Reinforce++, RLOO, etc
- Support model-based reward and function-based reward (verifiable reward)
- flash-attention, sequence packing, long context support via DeepSpeed Ulysses, LoRA, Liger-kernel
- scales up to 70B models and hundreds of GPUs
- experiment tracking with wandb, swanlab and mlflow
- Reward model training
- DPO training
- DeepSeek integration with Megatron v0.11
- SGLang integration
- vision language model RL
Quickstart:
Running a PPO example step-by-step:
- Data and Reward Preparation
- Understanding the PPO Example
Reproducible algorithm baselines:
For code explanation and advance usage (extension):
- PPO Trainer and Workers
- Advance Usage and Extension
Blogs from the community
Checkout this Jupyter Notebook to get started with PPO training with a single 24GB L4 GPU (FREE GPU quota provided by Lighting Studio)!
The performance is essential for on-policy RL algorithm. We write a detailed performance tuning guide to allow people tune the performance. See here for more details.
We have released a testing version of veRL that supports vLLM>=0.7.0. Please refer to this document for installation guide and more information.
If you find the project helpful, please cite:
- HybridFlow: A Flexible and Efficient RLHF Framework
- A Framework for Training Large Language Models for Code Generation via Proximal Policy Optimization
@article{sheng2024hybridflow,
title = {HybridFlow: A Flexible and Efficient RLHF Framework},
author = {Guangming Sheng and Chi Zhang and Zilingfeng Ye and Xibin Wu and Wang Zhang and Ru Zhang and Yanghua Peng and Haibin Lin and Chuan Wu},
year = {2024},
journal = {arXiv preprint arXiv: 2409.19256}
}
verl is inspired by the design of Nemo-Aligner, Deepspeed-chat and OpenRLHF. The project is adopted and supported by Anyscale, Bytedance, LMSys.org, Shanghai AI Lab, Tsinghua University, UC Berkeley, UCLA, UIUC, and University of Hong Kong.
- TinyZero: a reproduction of DeepSeek R1 Zero recipe for reasoning tasks
- PRIME: Process reinforcement through implicit rewards
- RAGEN: a general-purpose reasoning agent training framework
- Logic-RL: a reproduction of DeepSeek R1 Zero on 2K Tiny Logic Puzzle Dataset.
- deepscaler: iterative context scaling with GRPO
- critic-rl: LLM critics for code generation
- Easy-R1: Multi-modal RL training framework
- self-rewarding-reasoning-LLM: self-rewarding and correction with generative reward models
- Search-R1: RL with reasoning and searching (tool-call) interleaved LLMs
- DQO: Enhancing multi-Step reasoning abilities of language models through direct Q-function optimization
- FIRE: Flaming-hot initiation with regular execution sampling for large language models
- Code-R1: Reproducing R1 for Code with Reliable Rewards
Contributions from the community are welcome! Please checkout our roadmap and release plan.
We use yapf (Google style) to enforce strict code formatting when reviewing PRs. To reformat you code locally, make sure you installed latest yapf
pip3 install yapf --upgrade
Then, make sure you are at top level of verl repo and run
bash scripts/format.sh
We are HIRING! Send us an email if you are interested in internship/FTE opportunities in MLSys/LLM reasoning/multimodal alignment.
For Tasks:
Click tags to check more tools for each tasksFor Jobs:
Alternative AI tools for verl
Similar Open Source Tools
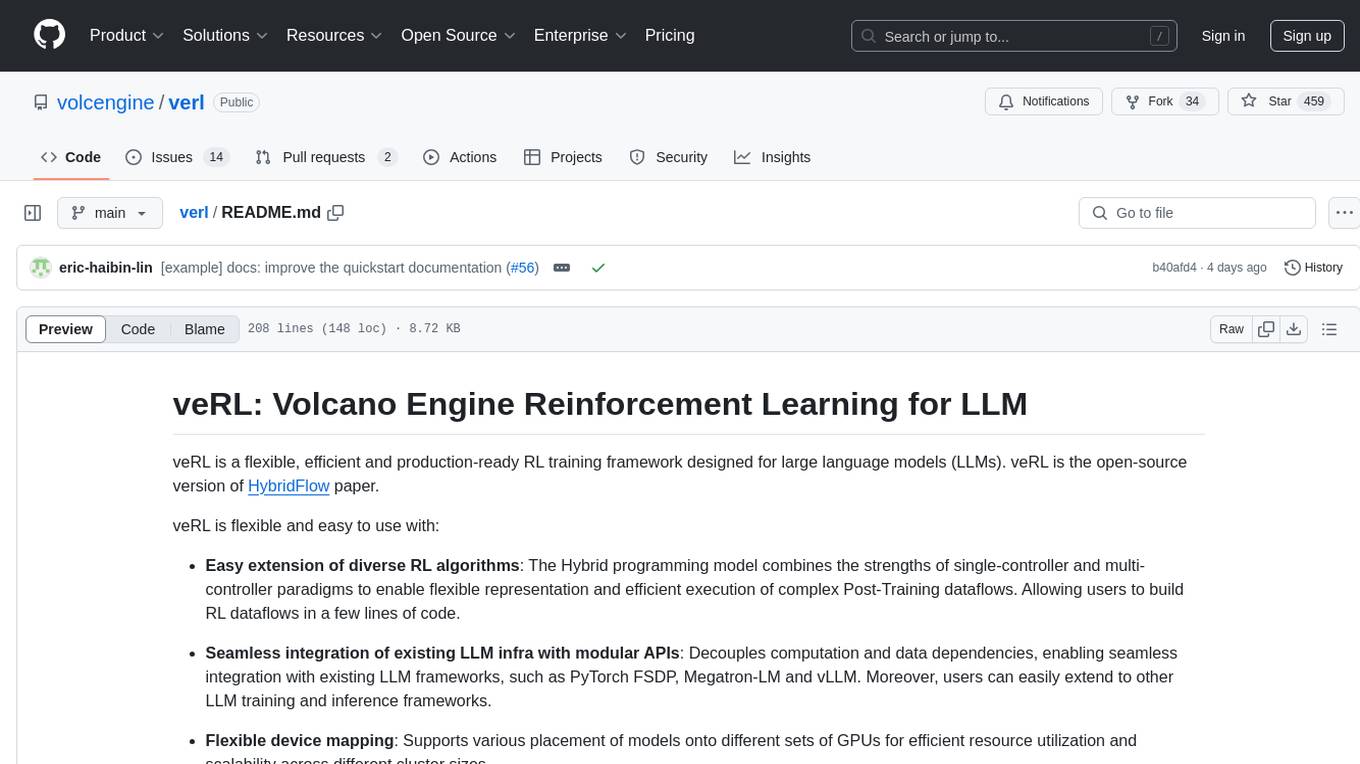
verl
veRL is a flexible and efficient reinforcement learning training framework designed for large language models (LLMs). It allows easy extension of diverse RL algorithms, seamless integration with existing LLM infrastructures, and flexible device mapping. The framework achieves state-of-the-art throughput and efficient actor model resharding with 3D-HybridEngine. It supports popular HuggingFace models and is suitable for users working with PyTorch FSDP, Megatron-LM, and vLLM backends.
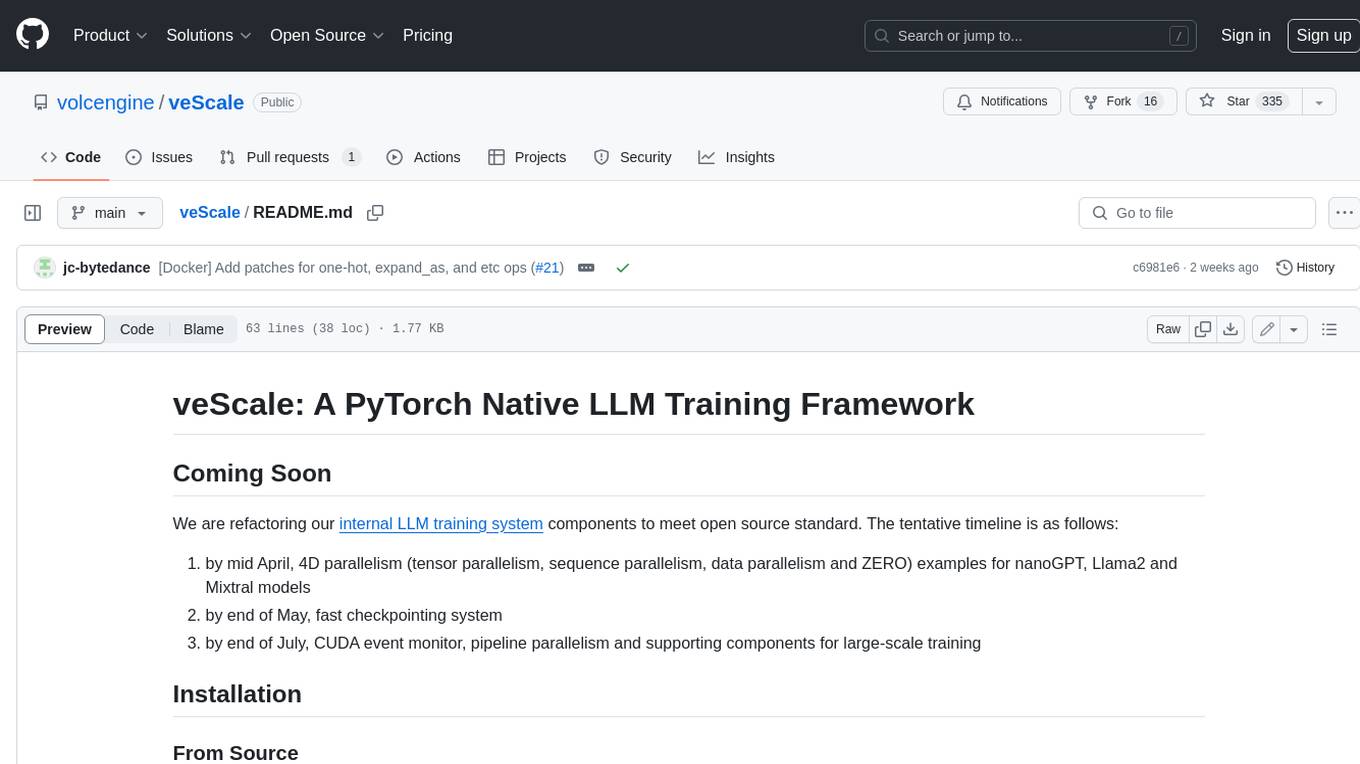
veScale
veScale is a PyTorch Native LLM Training Framework. It provides a set of tools and components to facilitate the training of large language models (LLMs) using PyTorch. veScale includes features such as 4D parallelism, fast checkpointing, and a CUDA event monitor. It is designed to be scalable and efficient, and it can be used to train LLMs on a variety of hardware platforms.
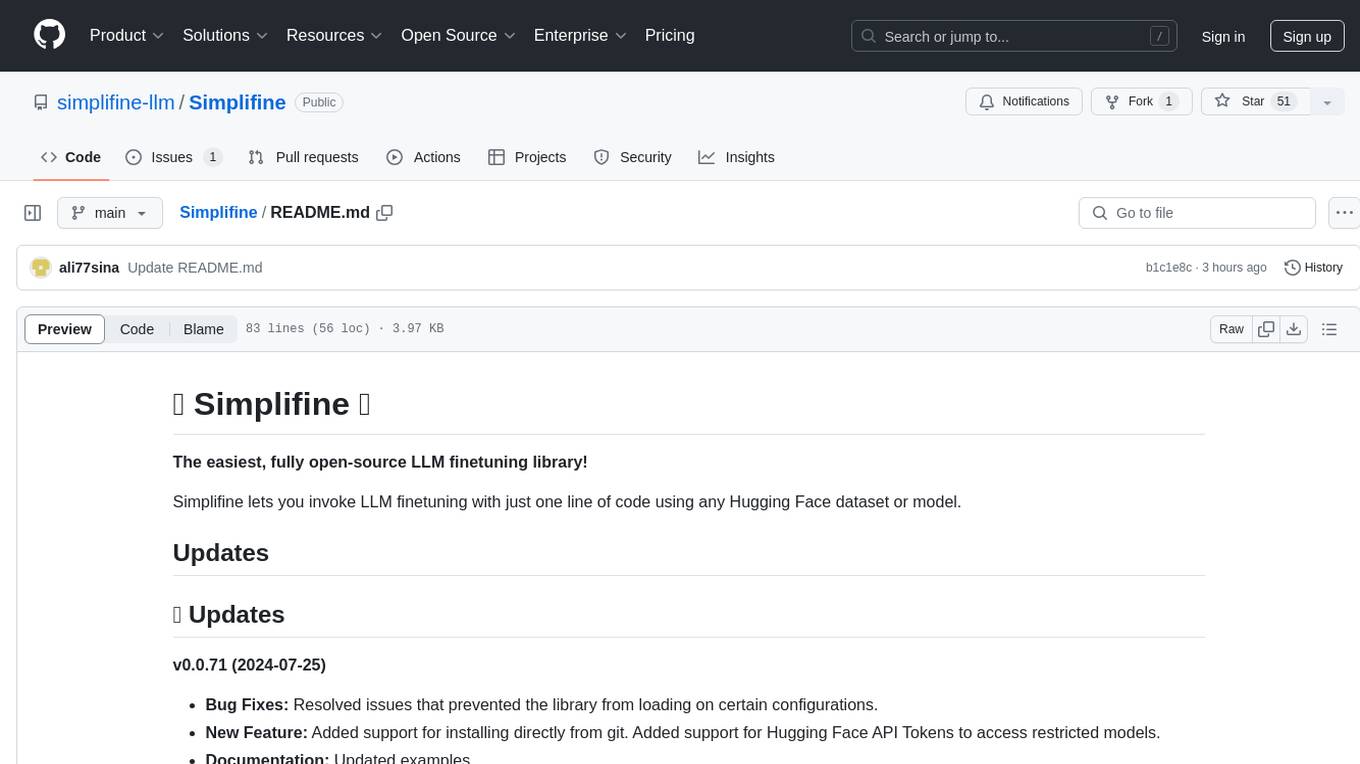
Simplifine
Simplifine is an open-source library designed for easy LLM finetuning, enabling users to perform tasks such as supervised fine tuning, question-answer finetuning, contrastive loss for embedding tasks, multi-label classification finetuning, and more. It provides features like WandB logging, in-built evaluation tools, automated finetuning parameters, and state-of-the-art optimization techniques. The library offers bug fixes, new features, and documentation updates in its latest version. Users can install Simplifine via pip or directly from GitHub. The project welcomes contributors and provides comprehensive documentation and support for users.
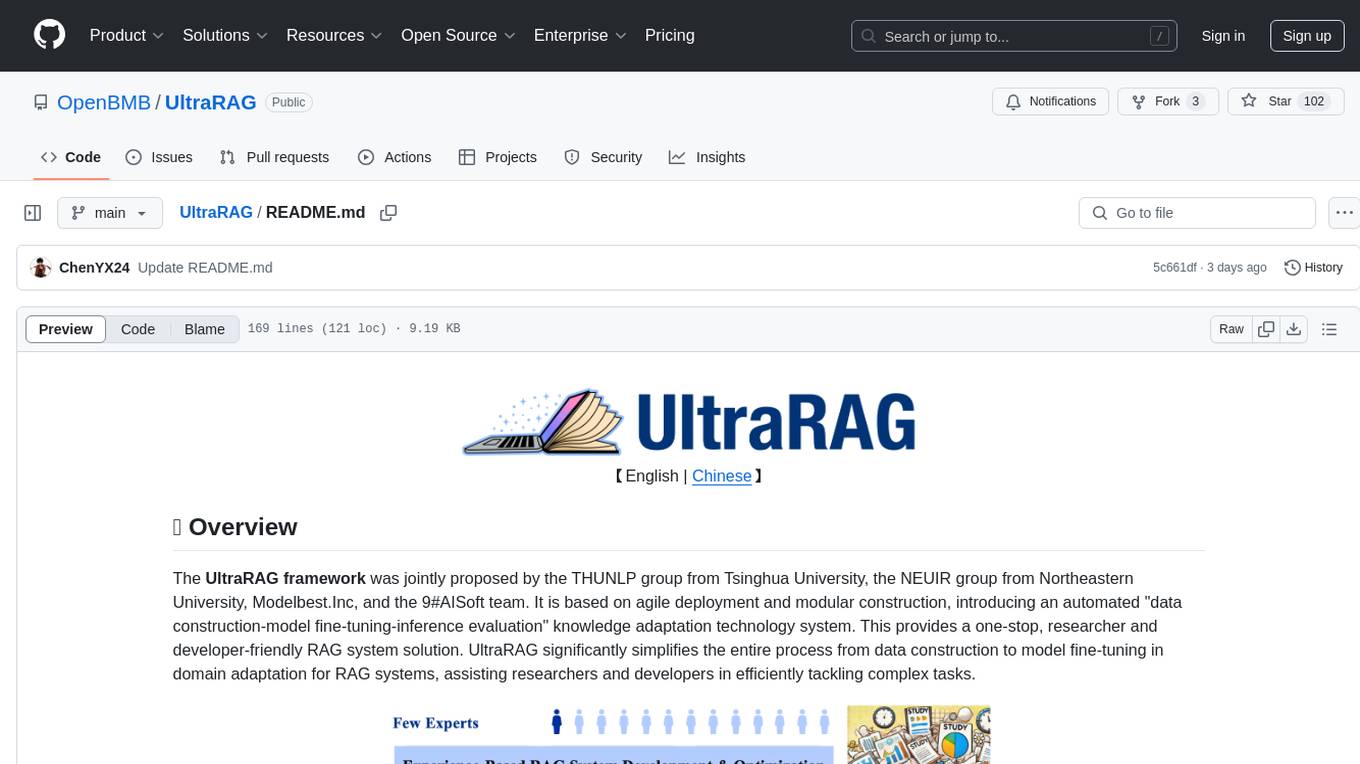
UltraRAG
The UltraRAG framework is a researcher and developer-friendly RAG system solution that simplifies the process from data construction to model fine-tuning in domain adaptation. It introduces an automated knowledge adaptation technology system, supporting no-code programming, one-click synthesis and fine-tuning, multidimensional evaluation, and research-friendly exploration work integration. The architecture consists of Frontend, Service, and Backend components, offering flexibility in customization and optimization. Performance evaluation in the legal field shows improved results compared to VanillaRAG, with specific metrics provided. The repository is licensed under Apache-2.0 and encourages citation for support.
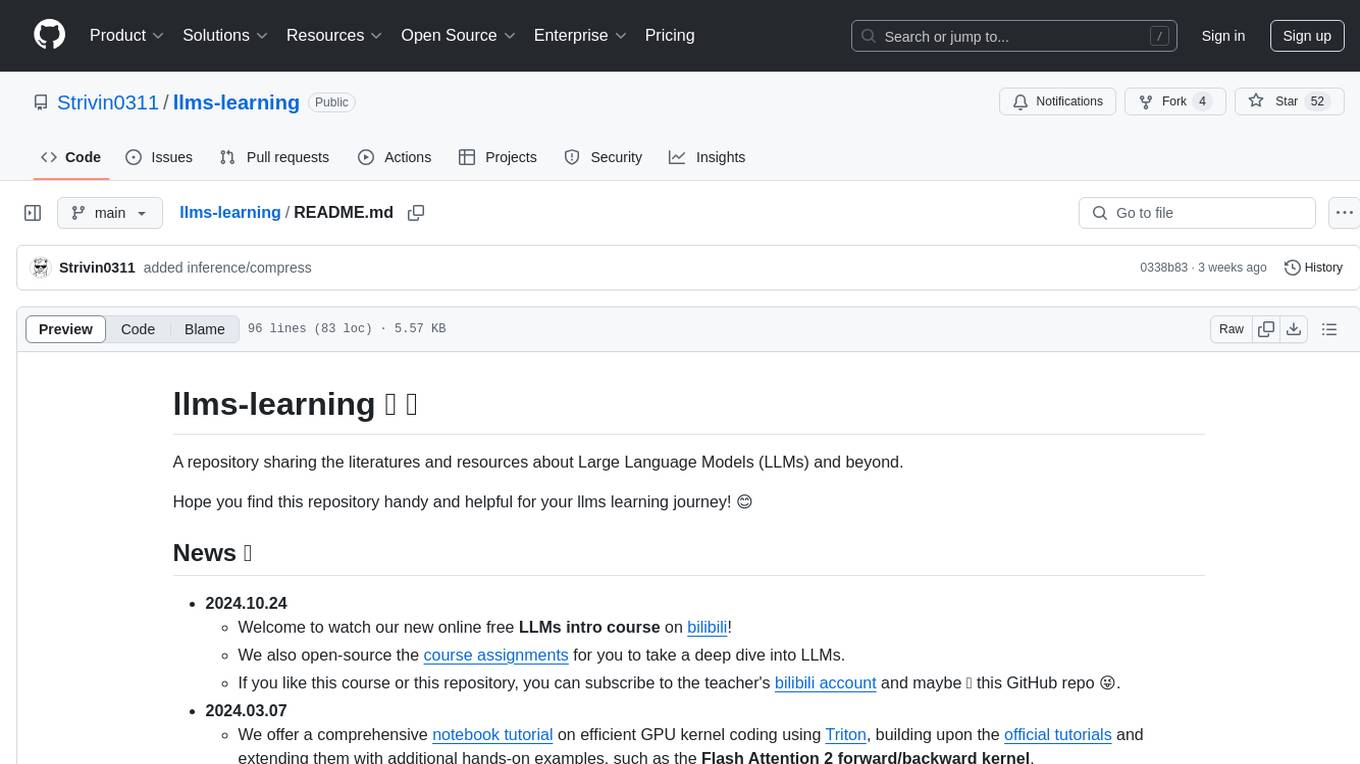
llms-learning
A repository sharing literatures and resources about Large Language Models (LLMs) and beyond. It includes tutorials, notebooks, course assignments, development stages, modeling, inference, training, applications, study, and basics related to LLMs. The repository covers various topics such as language models, transformers, state space models, multi-modal language models, training recipes, applications in autonomous driving, code, math, embodied intelligence, and more. The content is organized by different categories and provides comprehensive information on LLMs and related topics.
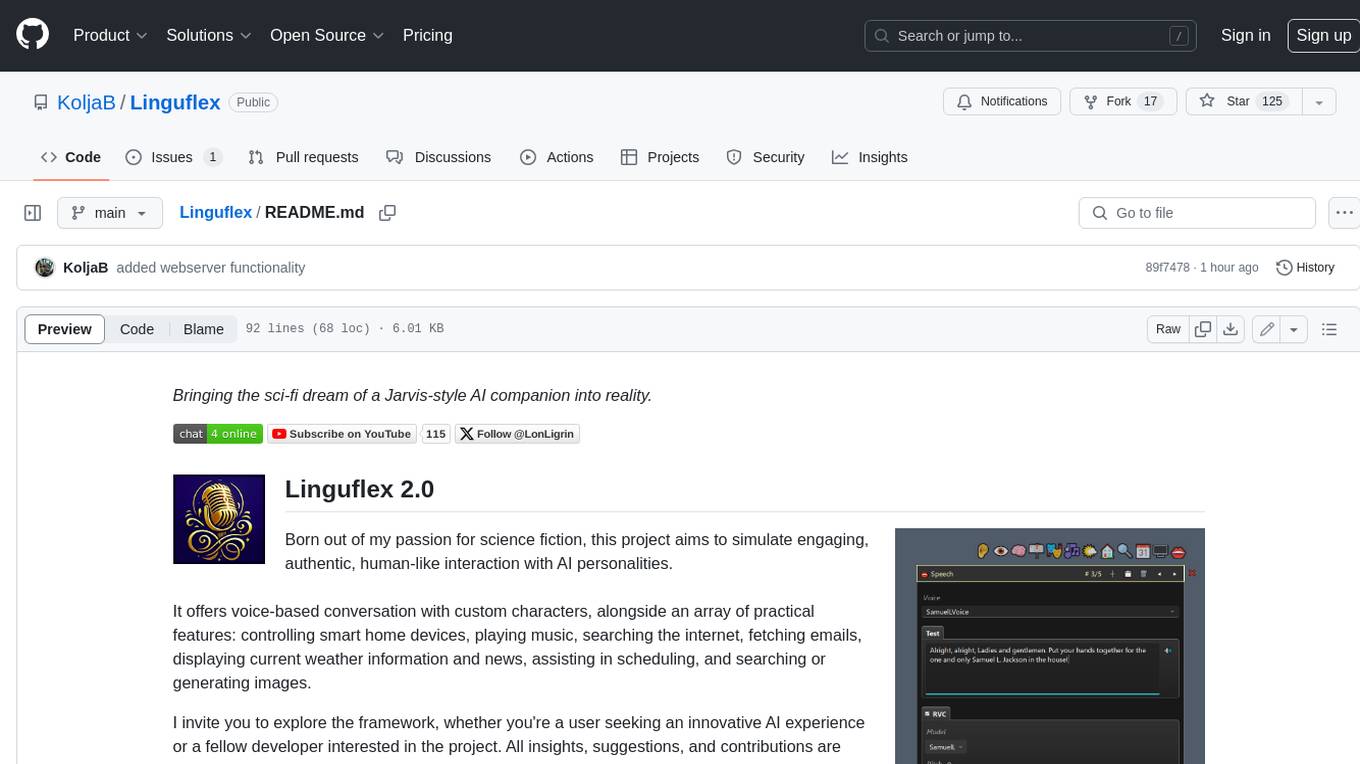
Linguflex
Linguflex is a project that aims to simulate engaging, authentic, human-like interaction with AI personalities. It offers voice-based conversation with custom characters, alongside an array of practical features such as controlling smart home devices, playing music, searching the internet, fetching emails, displaying current weather information and news, assisting in scheduling, and searching or generating images.
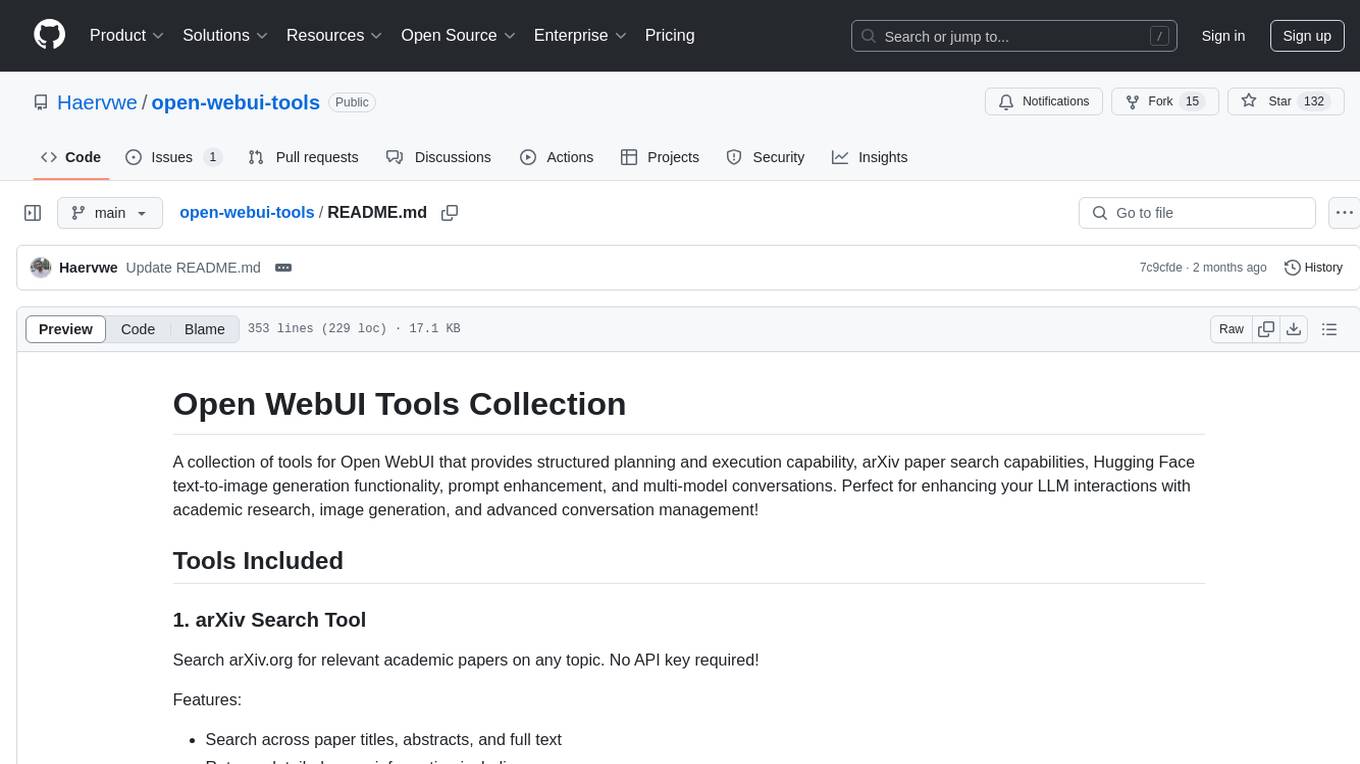
open-webui-tools
Open WebUI Tools Collection is a set of tools for structured planning, arXiv paper search, Hugging Face text-to-image generation, prompt enhancement, and multi-model conversations. It enhances LLM interactions with academic research, image generation, and conversation management. Tools include arXiv Search Tool and Hugging Face Image Generator. Function Pipes like Planner Agent offer autonomous plan generation and execution. Filters like Prompt Enhancer improve prompt quality. Installation and configuration instructions are provided for each tool and pipe.
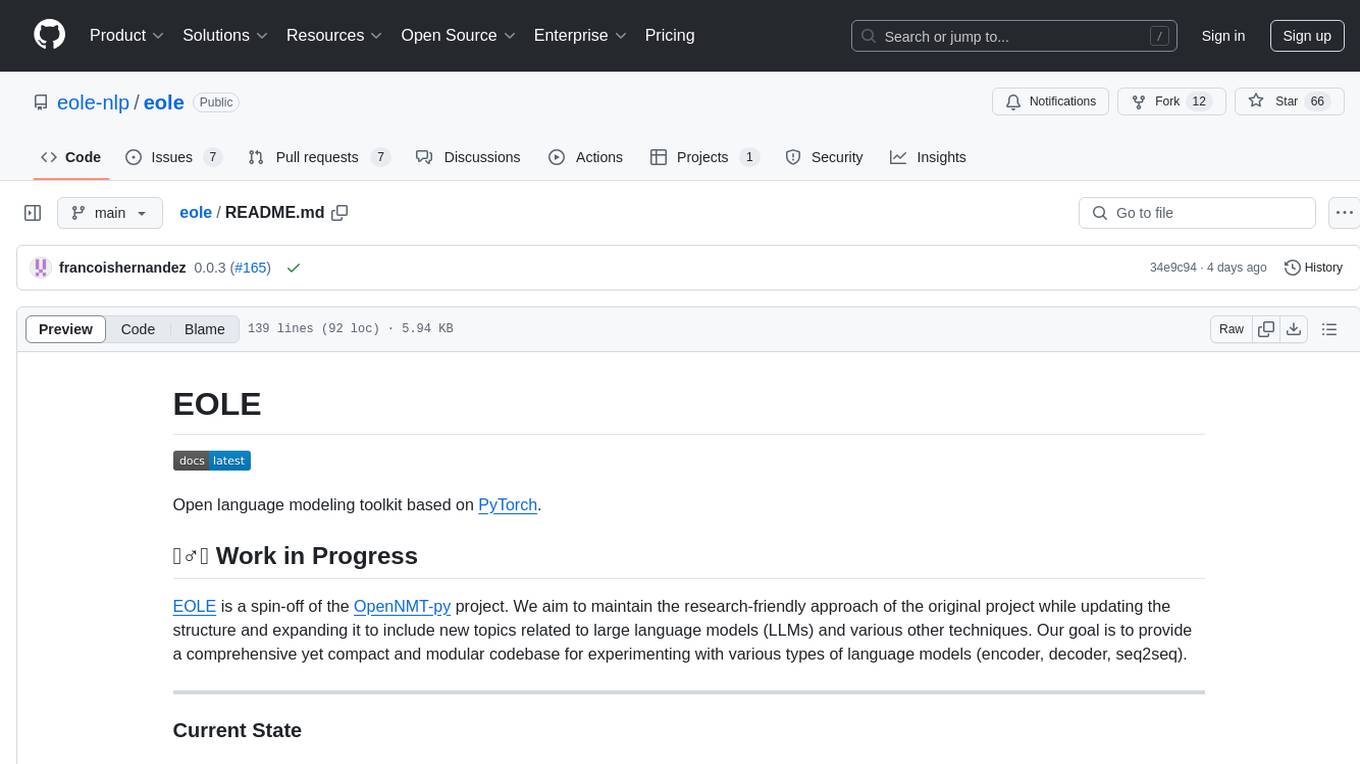
eole
EOLE is an open language modeling toolkit based on PyTorch. It aims to provide a research-friendly approach with a comprehensive yet compact and modular codebase for experimenting with various types of language models. The toolkit includes features such as versatile training and inference, dynamic data transforms, comprehensive large language model support, advanced quantization, efficient finetuning, flexible inference, and tensor parallelism. EOLE is a work in progress with ongoing enhancements in configuration management, command line entry points, reproducible recipes, core API simplification, and plans for further simplification, refactoring, inference server development, additional recipes, documentation enhancement, test coverage improvement, logging enhancements, and broader model support.
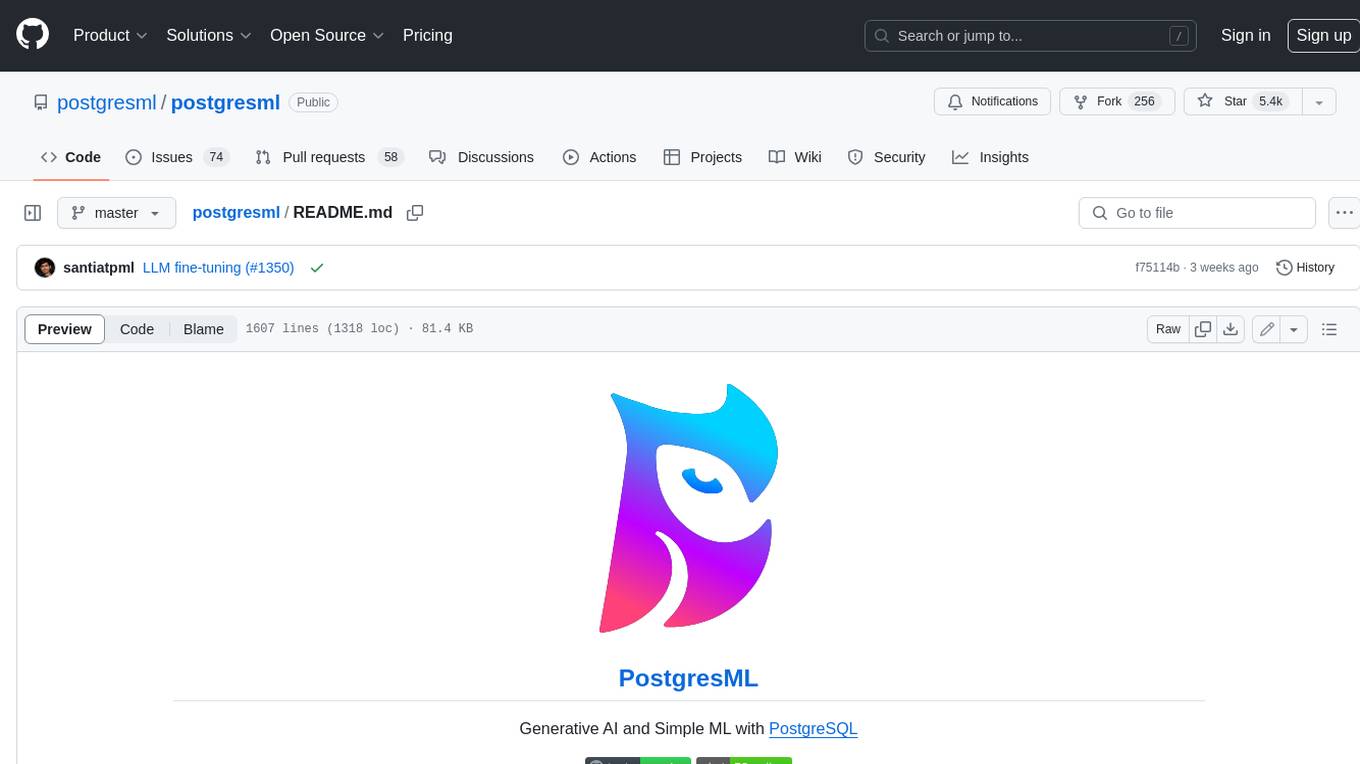
postgresml
PostgresML is a powerful Postgres extension that seamlessly combines data storage and machine learning inference within your database. It enables running machine learning and AI operations directly within PostgreSQL, leveraging GPU acceleration for faster computations, integrating state-of-the-art large language models, providing built-in functions for text processing, enabling efficient similarity search, offering diverse ML algorithms, ensuring high performance, scalability, and security, supporting a wide range of NLP tasks, and seamlessly integrating with existing PostgreSQL tools and client libraries.
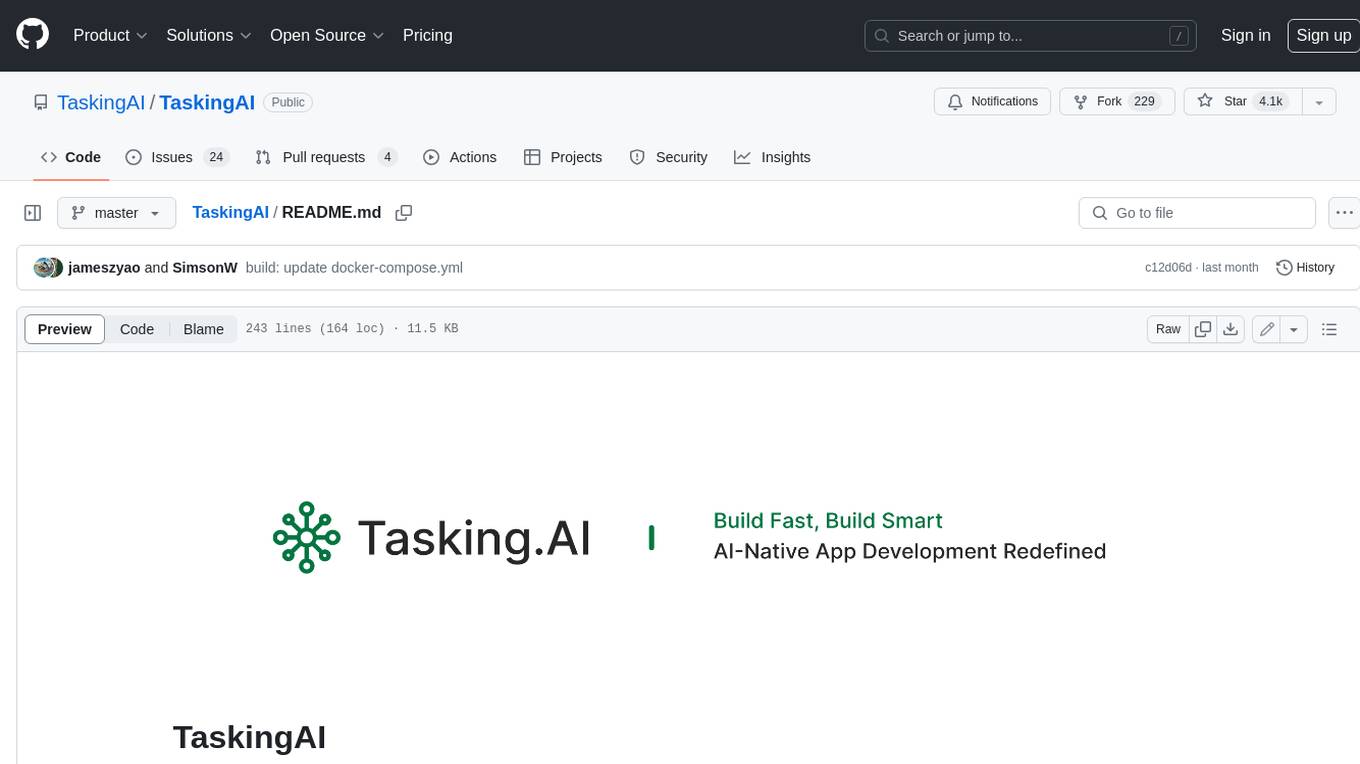
TaskingAI
TaskingAI brings Firebase's simplicity to **AI-native app development**. The platform enables the creation of GPTs-like multi-tenant applications using a wide range of LLMs from various providers. It features distinct, modular functions such as Inference, Retrieval, Assistant, and Tool, seamlessly integrated to enhance the development process. TaskingAI’s cohesive design ensures an efficient, intelligent, and user-friendly experience in AI application development.
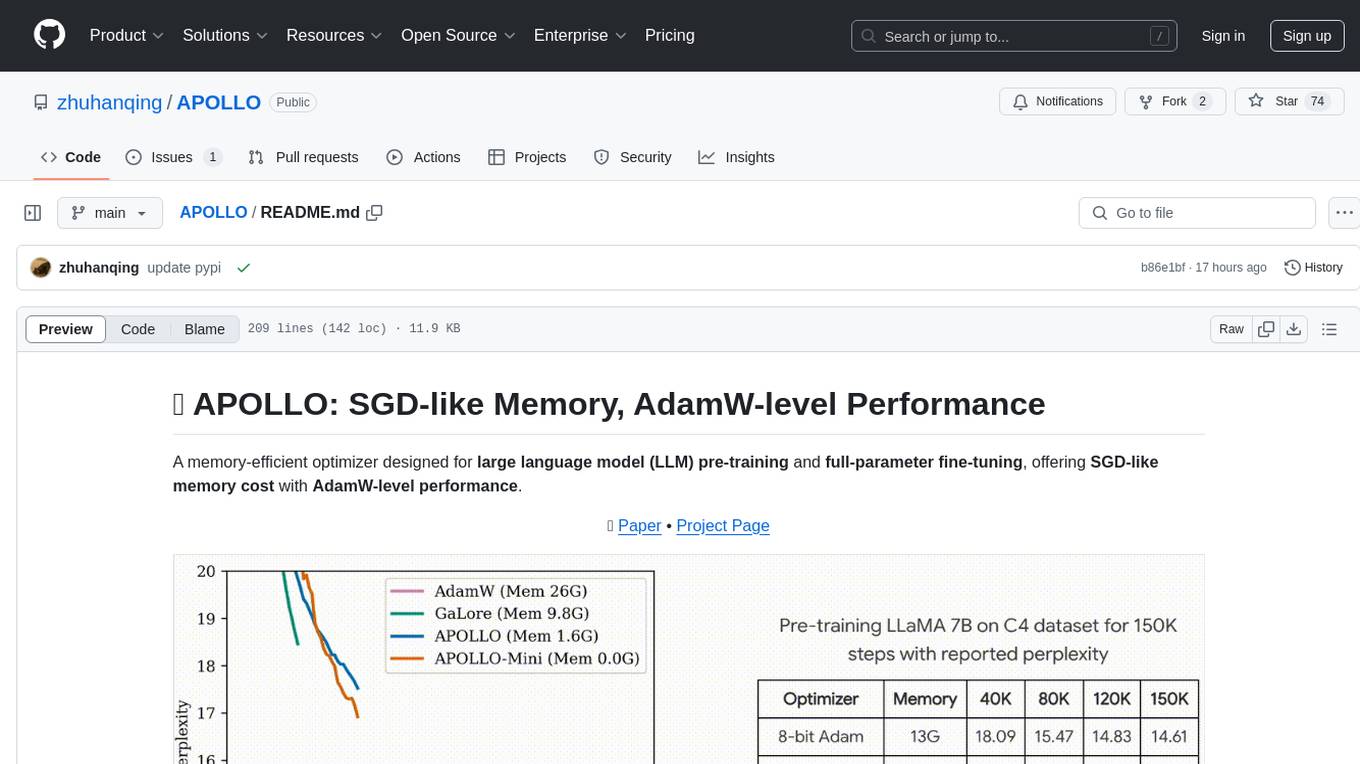
APOLLO
APOLLO is a memory-efficient optimizer designed for large language model (LLM) pre-training and full-parameter fine-tuning. It offers SGD-like memory cost with AdamW-level performance. The optimizer integrates low-rank approximation and optimizer state redundancy reduction to achieve significant memory savings while maintaining or surpassing the performance of Adam(W). Key contributions include structured learning rate updates for LLM training, approximated channel-wise gradient scaling in a low-rank auxiliary space, and minimal-rank tensor-wise gradient scaling. APOLLO aims to optimize memory efficiency during training large language models.
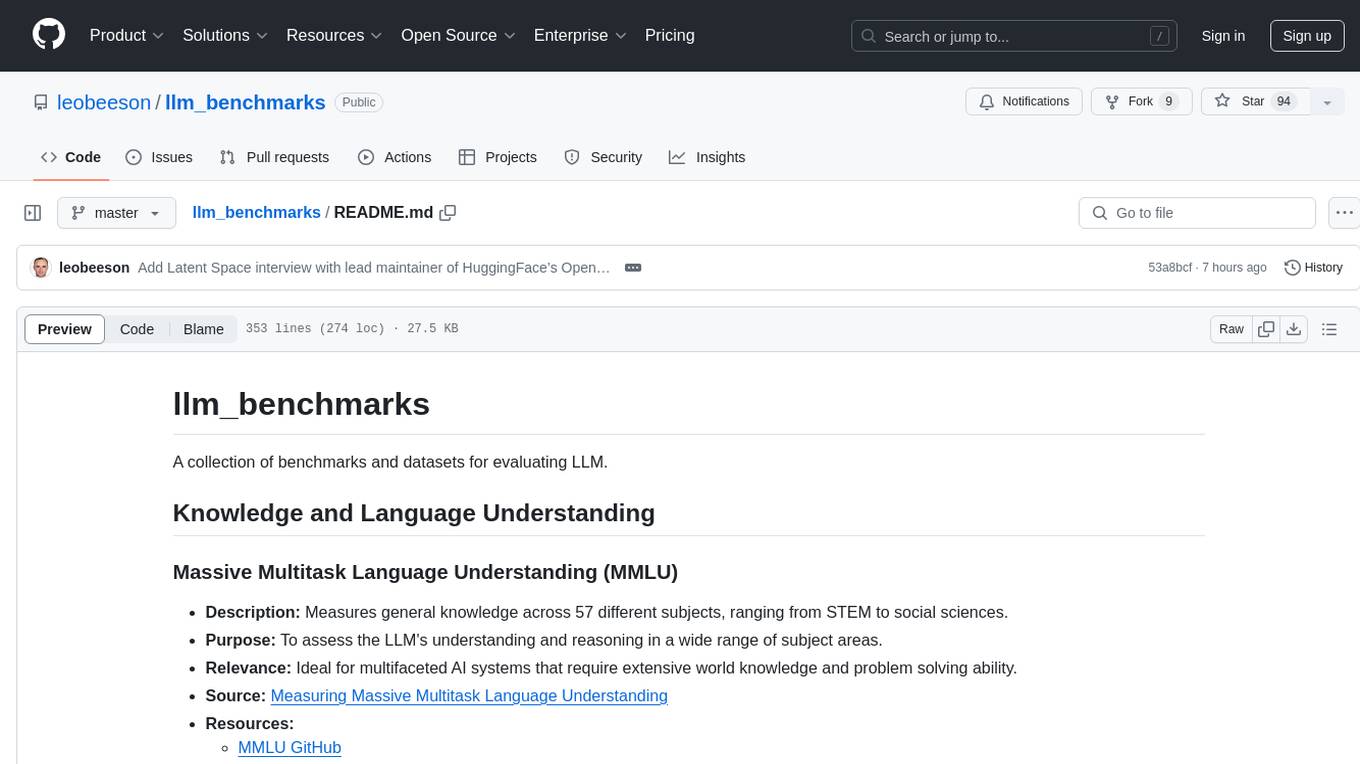
llm_benchmarks
llm_benchmarks is a collection of benchmarks and datasets for evaluating Large Language Models (LLMs). It includes various tasks and datasets to assess LLMs' knowledge, reasoning, language understanding, and conversational abilities. The repository aims to provide comprehensive evaluation resources for LLMs across different domains and applications, such as education, healthcare, content moderation, coding, and conversational AI. Researchers and developers can leverage these benchmarks to test and improve the performance of LLMs in various real-world scenarios.
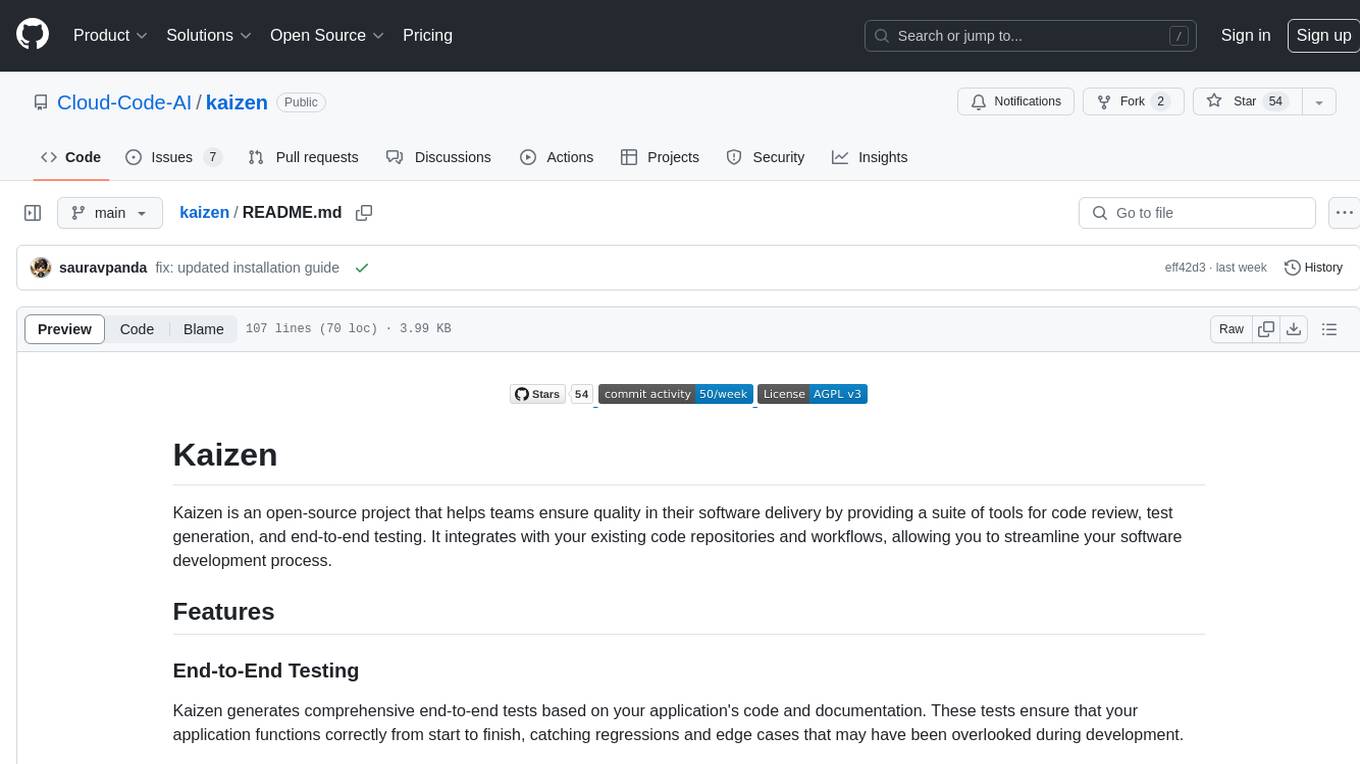
kaizen
Kaizen is an open-source project that helps teams ensure quality in their software delivery by providing a suite of tools for code review, test generation, and end-to-end testing. It integrates with your existing code repositories and workflows, allowing you to streamline your software development process. Kaizen generates comprehensive end-to-end tests, provides UI testing and review, and automates code review with insightful feedback. The file structure includes components for API server, logic, actors, generators, LLM integrations, documentation, and sample code. Getting started involves installing the Kaizen package, generating tests for websites, and executing tests. The tool also runs an API server for GitHub App actions. Contributions are welcome under the AGPL License.
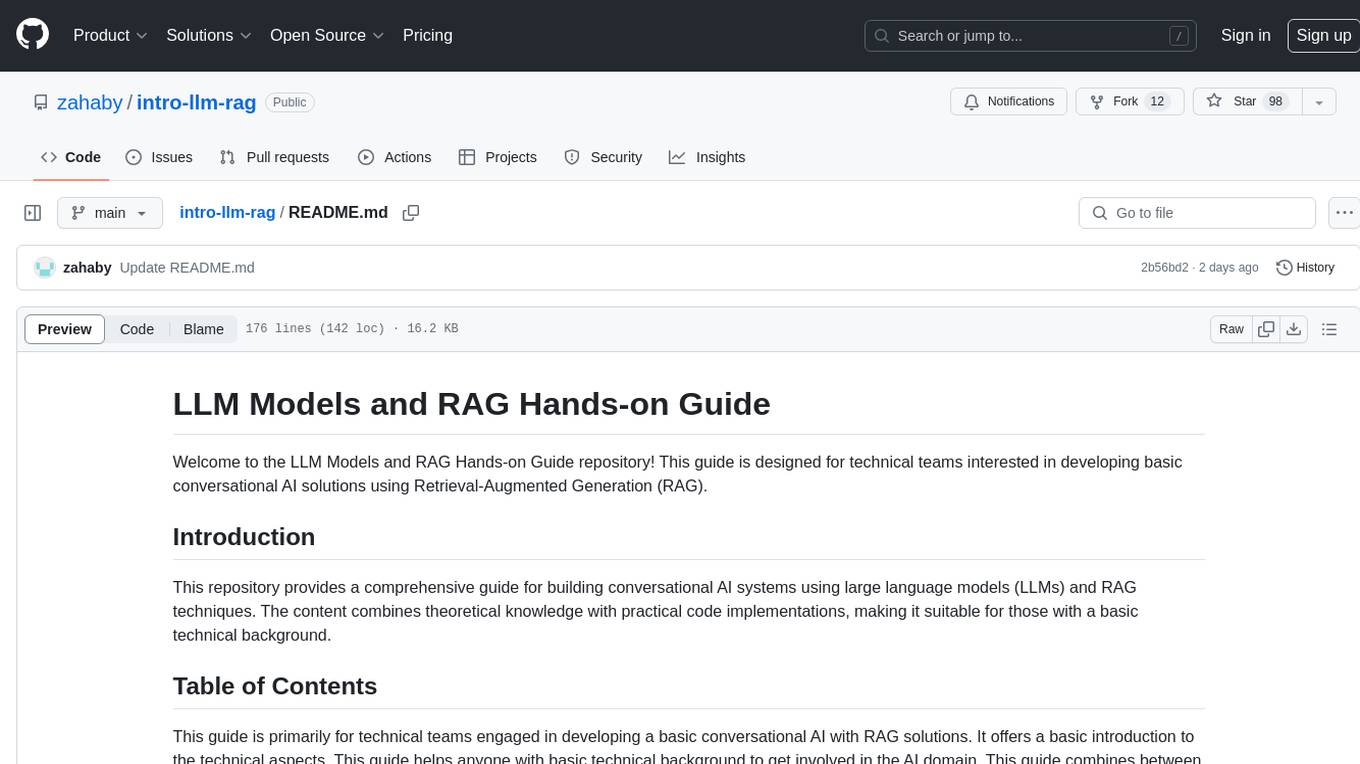
intro-llm-rag
This repository serves as a comprehensive guide for technical teams interested in developing conversational AI solutions using Retrieval-Augmented Generation (RAG) techniques. It covers theoretical knowledge and practical code implementations, making it suitable for individuals with a basic technical background. The content includes information on large language models (LLMs), transformers, prompt engineering, embeddings, vector stores, and various other key concepts related to conversational AI. The repository also provides hands-on examples for two different use cases, along with implementation details and performance analysis.
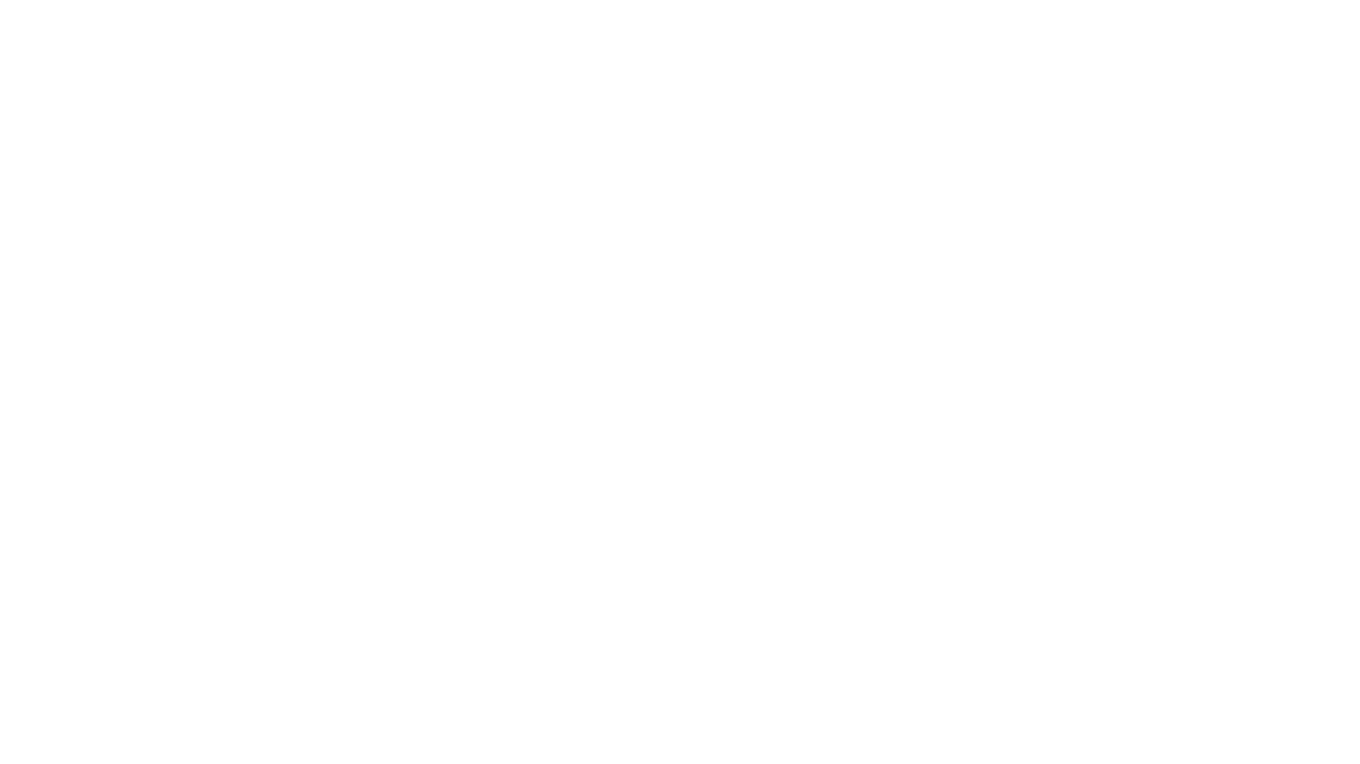
RetouchGPT
RetouchGPT is a novel framework designed for interactive face retouching using Large Language Models (LLMs). It leverages instruction-driven imperfection prediction and LLM-based embedding to guide the retouching process. The tool allows users to interactively modify imperfection features in face images, achieving high-fidelity retouching results. RetouchGPT outperforms existing methods by integrating textual and visual features to accurately identify imperfections and replace them with normal skin features.
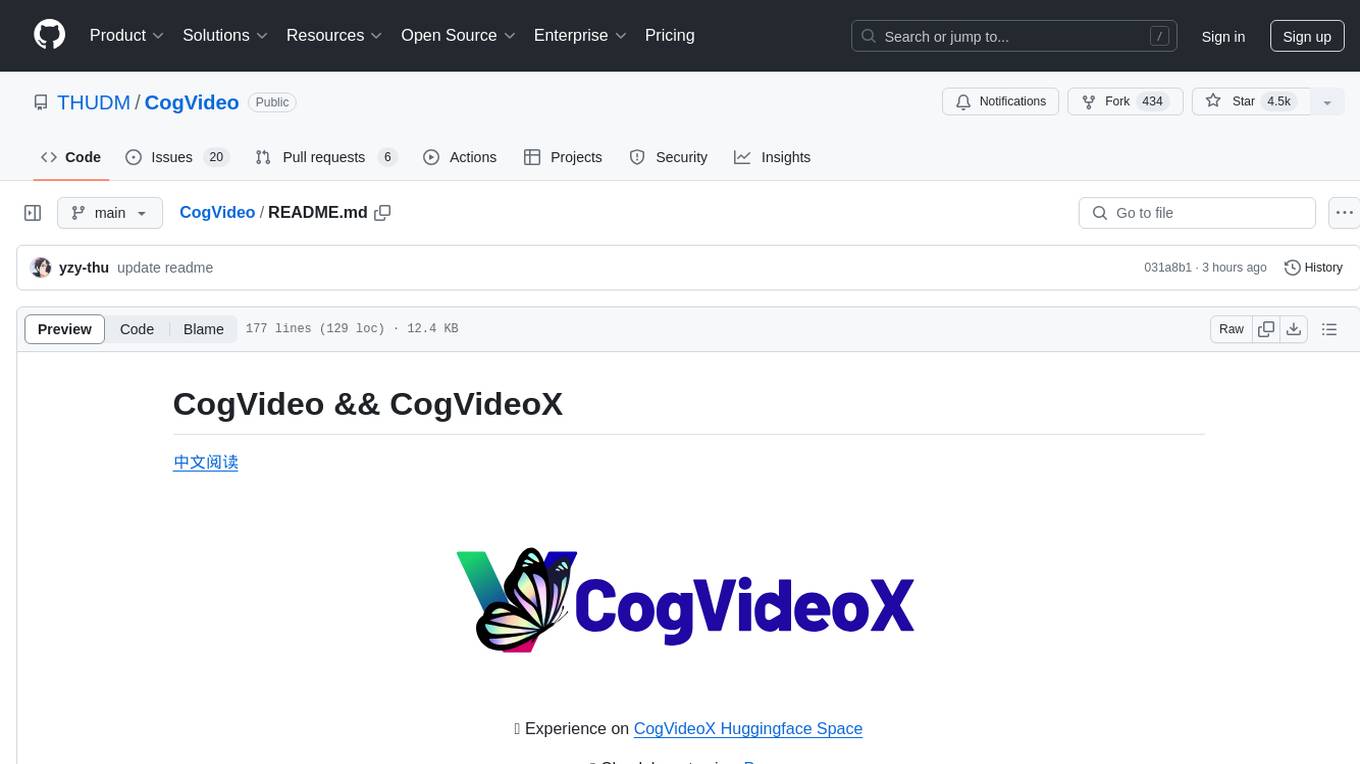
CogVideo
CogVideo is an open-source repository that provides pretrained text-to-video models for generating videos based on input text. It includes models like CogVideoX-2B and CogVideo, offering powerful video generation capabilities. The repository offers tools for inference, fine-tuning, and model conversion, along with demos showcasing the model's capabilities through CLI, web UI, and online experiences. CogVideo aims to facilitate the creation of high-quality videos from textual descriptions, catering to a wide range of applications.
For similar tasks
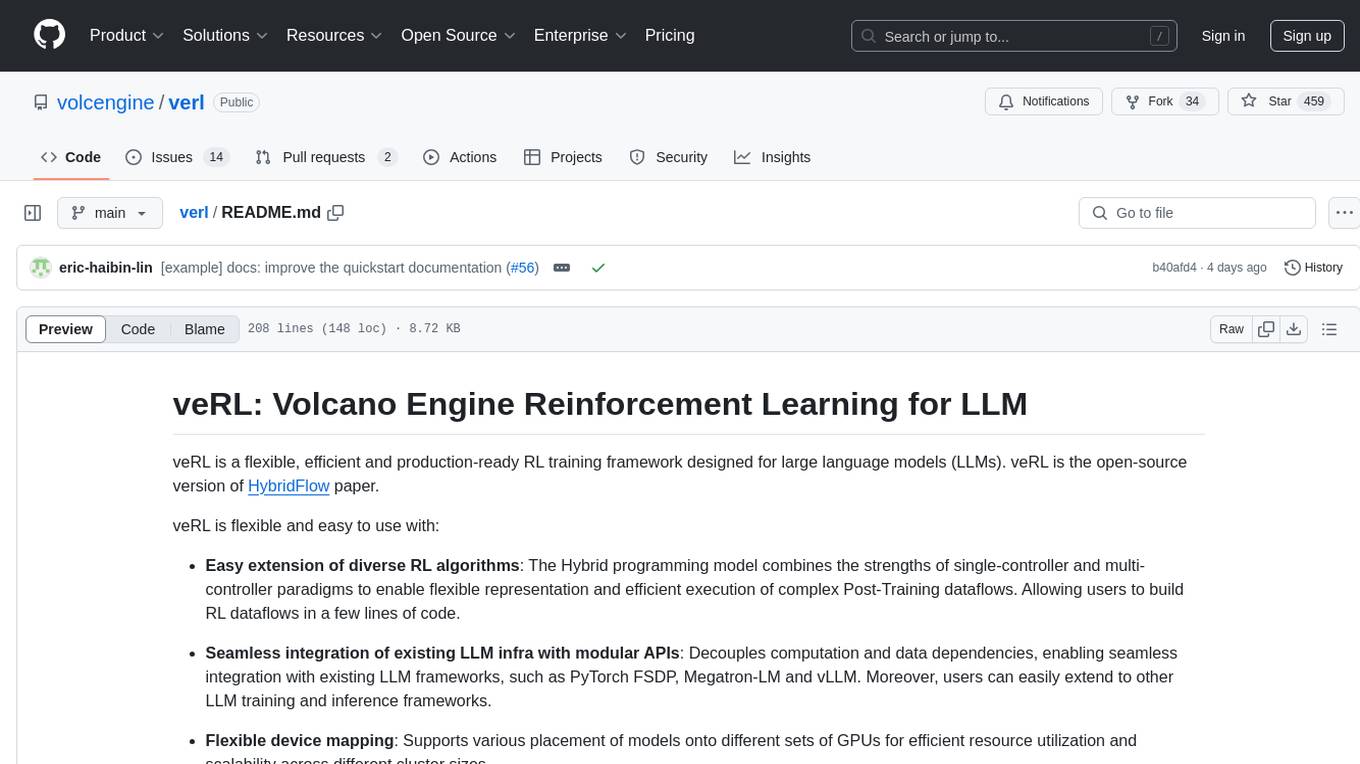
verl
veRL is a flexible and efficient reinforcement learning training framework designed for large language models (LLMs). It allows easy extension of diverse RL algorithms, seamless integration with existing LLM infrastructures, and flexible device mapping. The framework achieves state-of-the-art throughput and efficient actor model resharding with 3D-HybridEngine. It supports popular HuggingFace models and is suitable for users working with PyTorch FSDP, Megatron-LM, and vLLM backends.
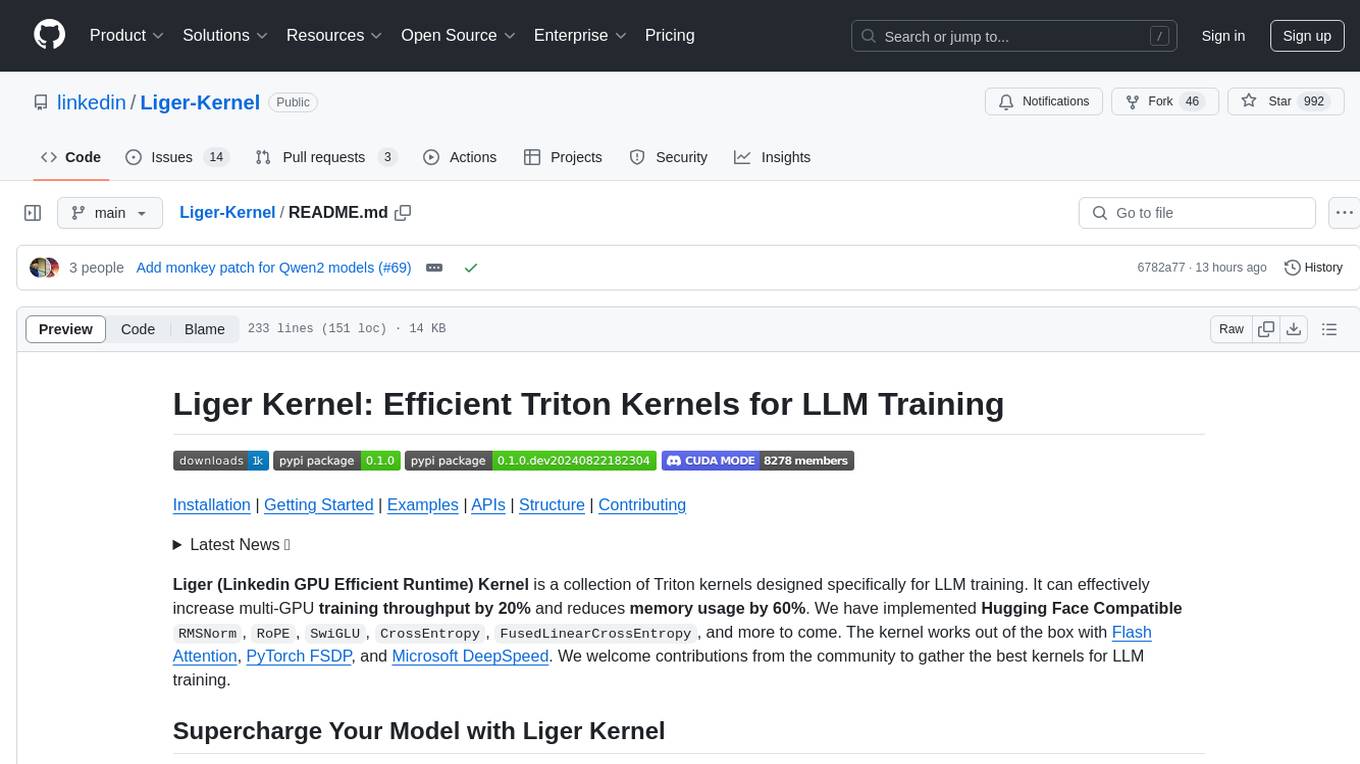
Liger-Kernel
Liger Kernel is a collection of Triton kernels designed for LLM training, increasing training throughput by 20% and reducing memory usage by 60%. It includes Hugging Face Compatible modules like RMSNorm, RoPE, SwiGLU, CrossEntropy, and FusedLinearCrossEntropy. The tool works with Flash Attention, PyTorch FSDP, and Microsoft DeepSpeed, aiming to enhance model efficiency and performance for researchers, ML practitioners, and curious novices.
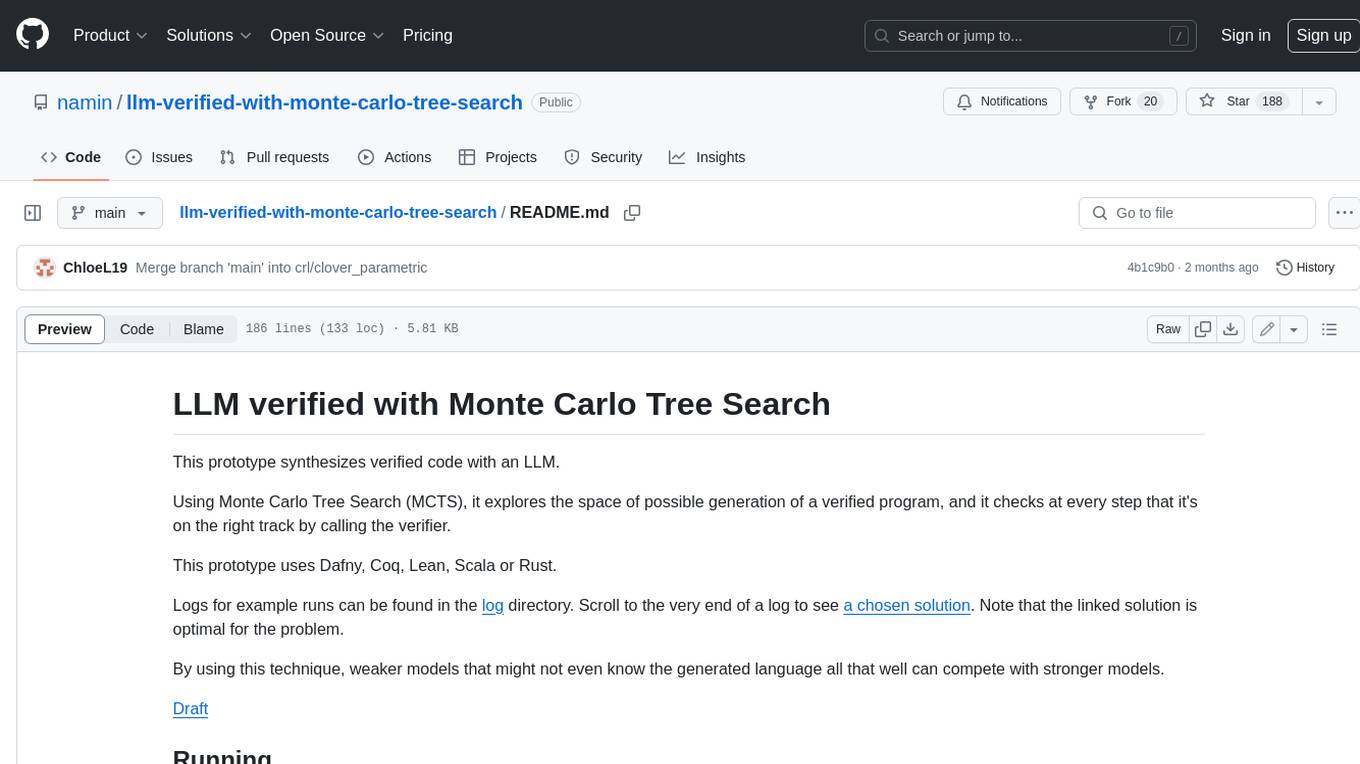
llm-verified-with-monte-carlo-tree-search
This prototype synthesizes verified code with an LLM using Monte Carlo Tree Search (MCTS). It explores the space of possible generation of a verified program and checks at every step that it's on the right track by calling the verifier. This prototype uses Dafny, Coq, Lean, Scala, or Rust. By using this technique, weaker models that might not even know the generated language all that well can compete with stronger models.
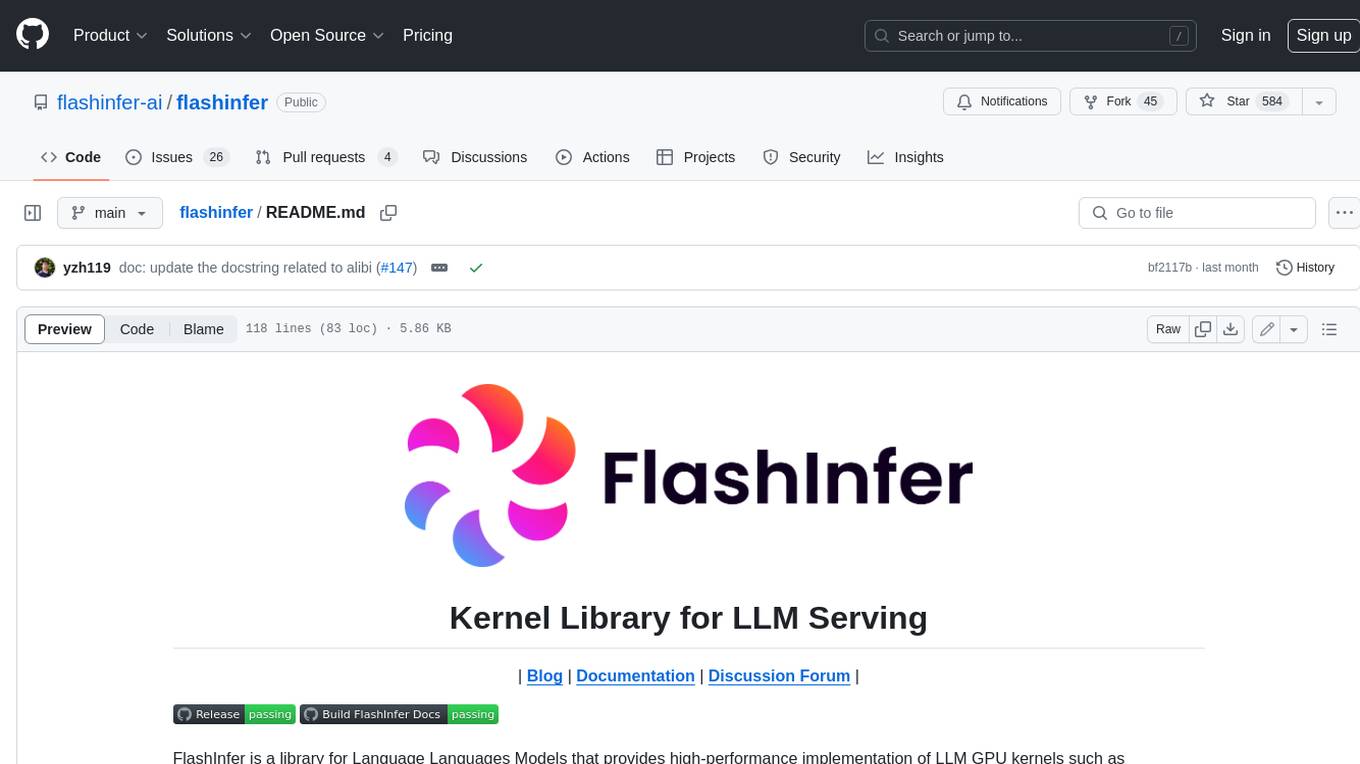
flashinfer
FlashInfer is a library for Language Languages Models that provides high-performance implementation of LLM GPU kernels such as FlashAttention, PageAttention and LoRA. FlashInfer focus on LLM serving and inference, and delivers state-the-art performance across diverse scenarios.
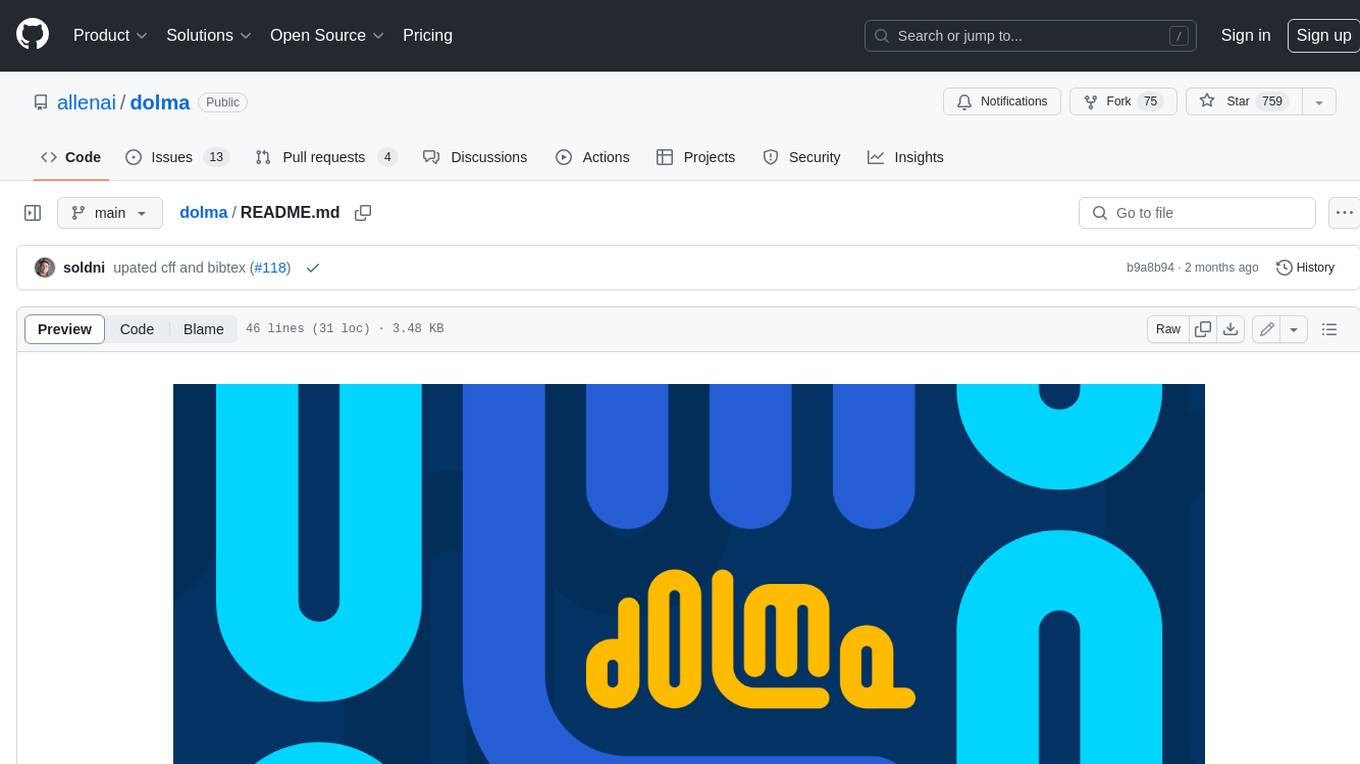
dolma
Dolma is a dataset and toolkit for curating large datasets for (pre)-training ML models. The dataset consists of 3 trillion tokens from a diverse mix of web content, academic publications, code, books, and encyclopedic materials. The toolkit provides high-performance, portable, and extensible tools for processing, tagging, and deduplicating documents. Key features of the toolkit include built-in taggers, fast deduplication, and cloud support.
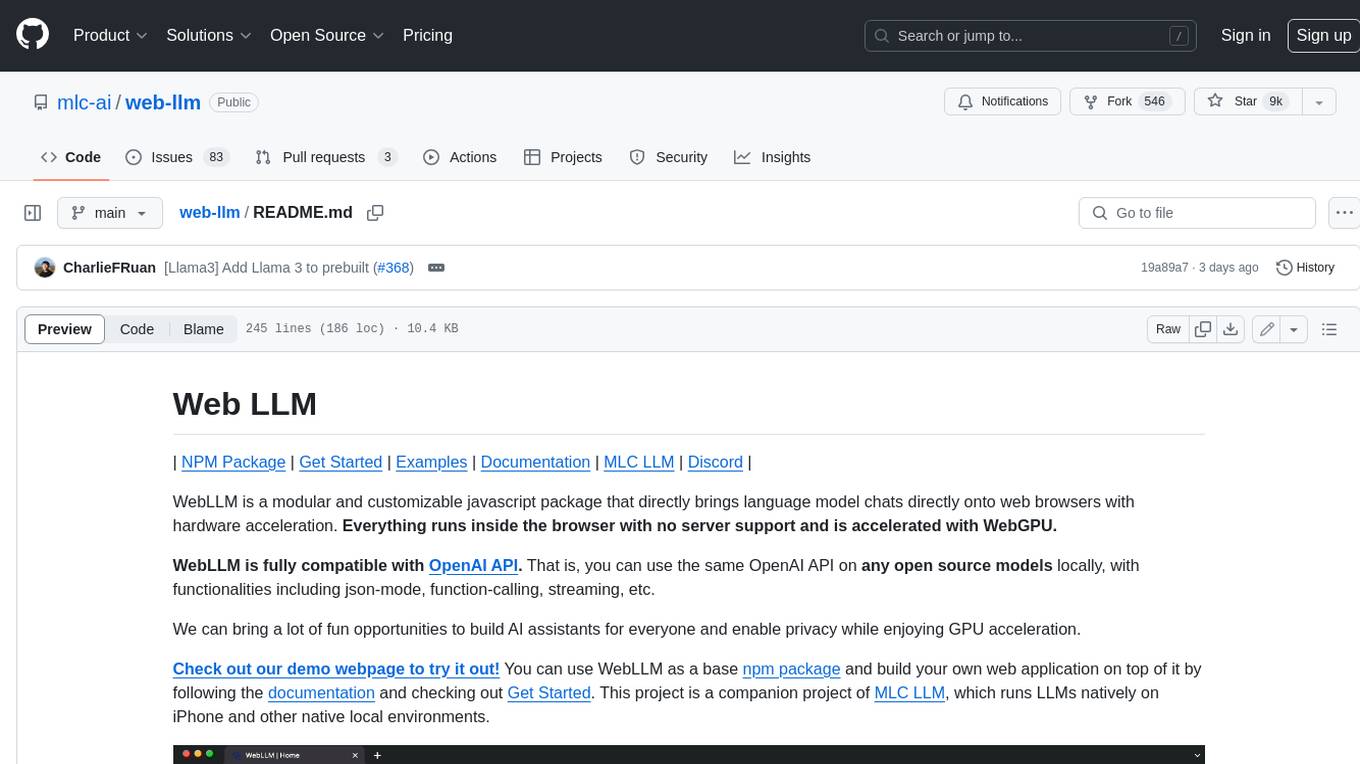
web-llm
WebLLM is a modular and customizable javascript package that directly brings language model chats directly onto web browsers with hardware acceleration. Everything runs inside the browser with no server support and is accelerated with WebGPU. WebLLM is fully compatible with OpenAI API. That is, you can use the same OpenAI API on any open source models locally, with functionalities including json-mode, function-calling, streaming, etc. We can bring a lot of fun opportunities to build AI assistants for everyone and enable privacy while enjoying GPU acceleration.
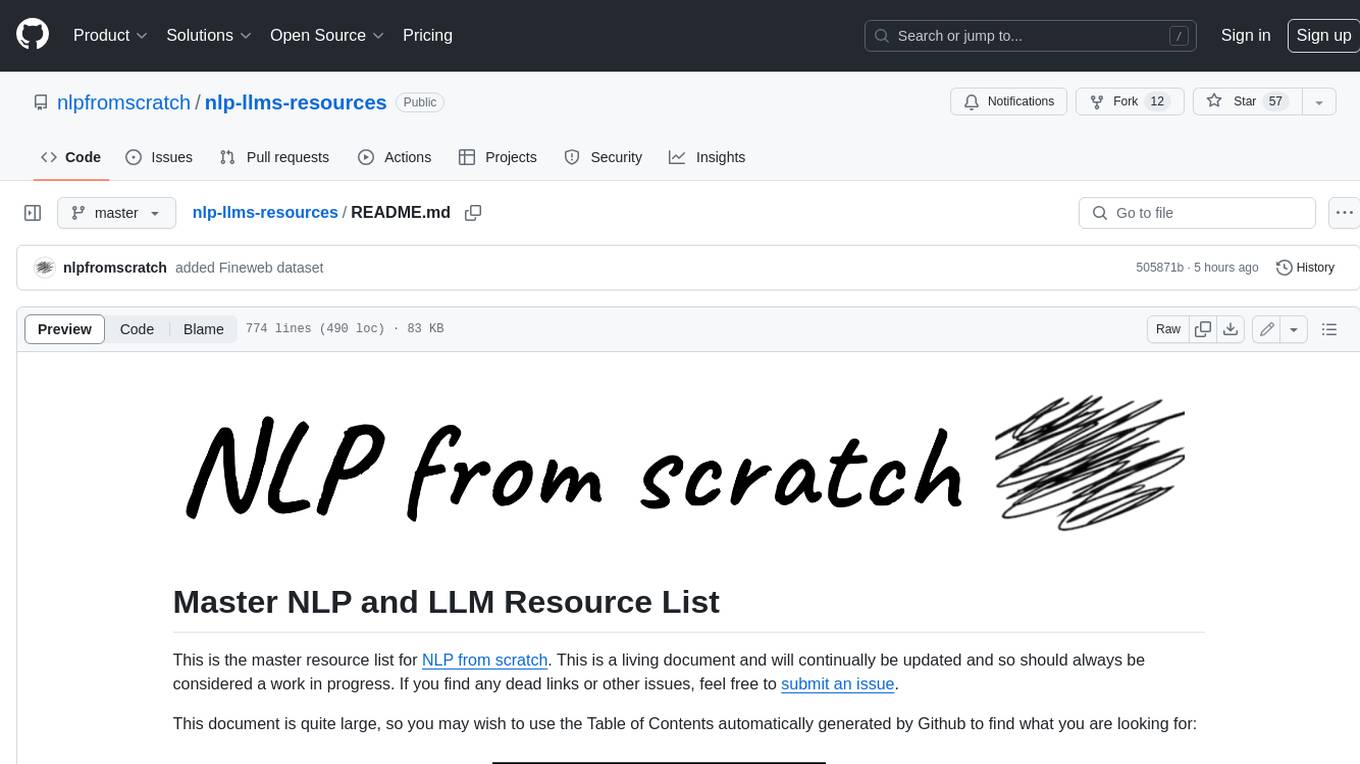
nlp-llms-resources
The 'nlp-llms-resources' repository is a comprehensive resource list for Natural Language Processing (NLP) and Large Language Models (LLMs). It covers a wide range of topics including traditional NLP datasets, data acquisition, libraries for NLP, neural networks, sentiment analysis, optical character recognition, information extraction, semantics, topic modeling, multilingual NLP, domain-specific LLMs, vector databases, ethics, costing, books, courses, surveys, aggregators, newsletters, papers, conferences, and societies. The repository provides valuable information and resources for individuals interested in NLP and LLMs.
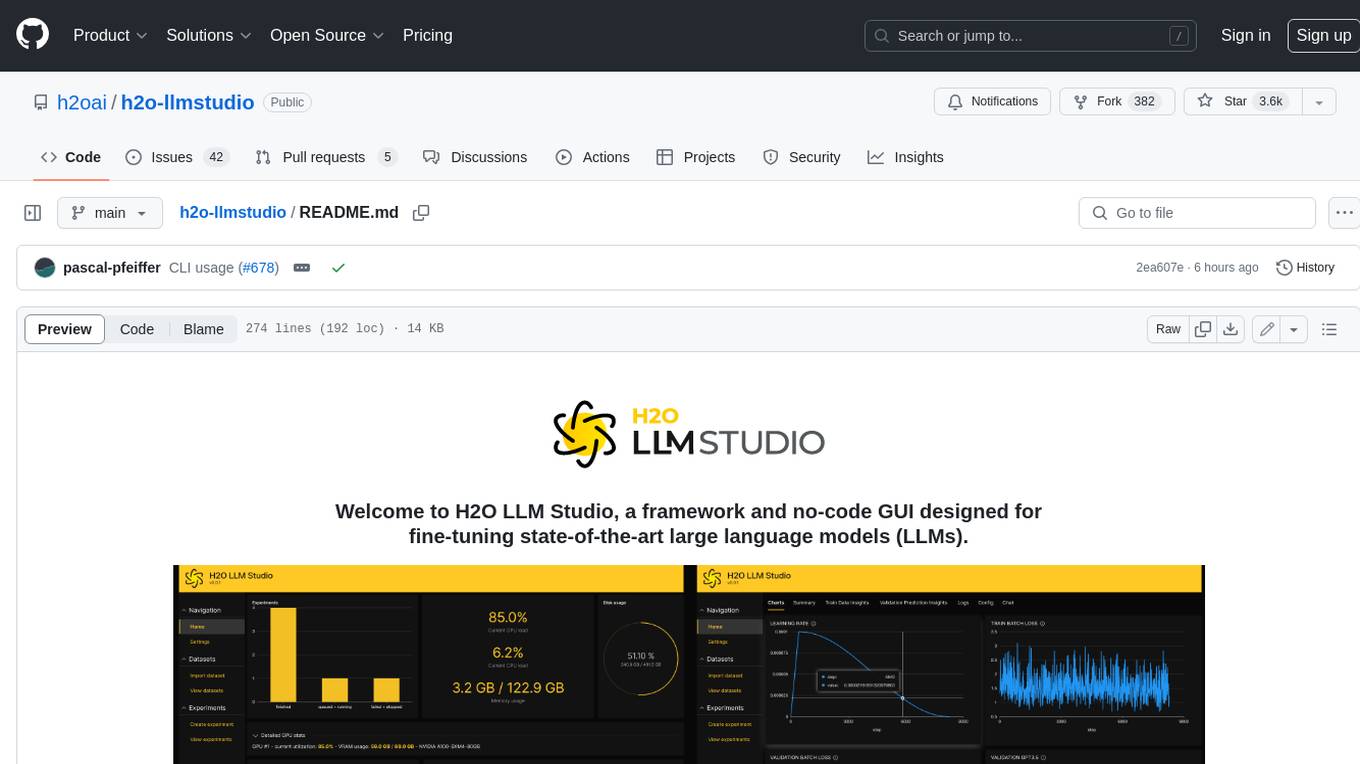
h2o-llmstudio
H2O LLM Studio is a framework and no-code GUI designed for fine-tuning state-of-the-art large language models (LLMs). With H2O LLM Studio, you can easily and effectively fine-tune LLMs without the need for any coding experience. The GUI is specially designed for large language models, and you can finetune any LLM using a large variety of hyperparameters. You can also use recent finetuning techniques such as Low-Rank Adaptation (LoRA) and 8-bit model training with a low memory footprint. Additionally, you can use Reinforcement Learning (RL) to finetune your model (experimental), use advanced evaluation metrics to judge generated answers by the model, track and compare your model performance visually, and easily export your model to the Hugging Face Hub and share it with the community.
For similar jobs
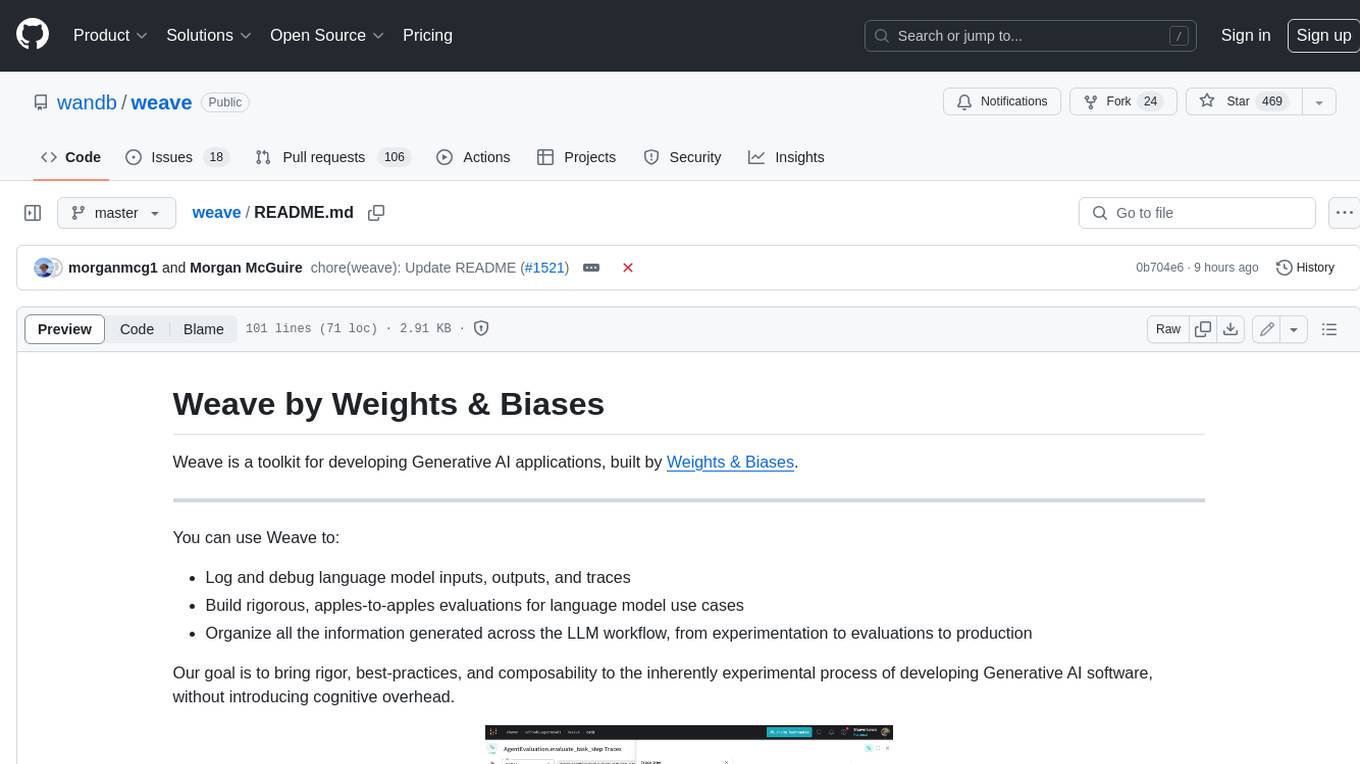
weave
Weave is a toolkit for developing Generative AI applications, built by Weights & Biases. With Weave, you can log and debug language model inputs, outputs, and traces; build rigorous, apples-to-apples evaluations for language model use cases; and organize all the information generated across the LLM workflow, from experimentation to evaluations to production. Weave aims to bring rigor, best-practices, and composability to the inherently experimental process of developing Generative AI software, without introducing cognitive overhead.
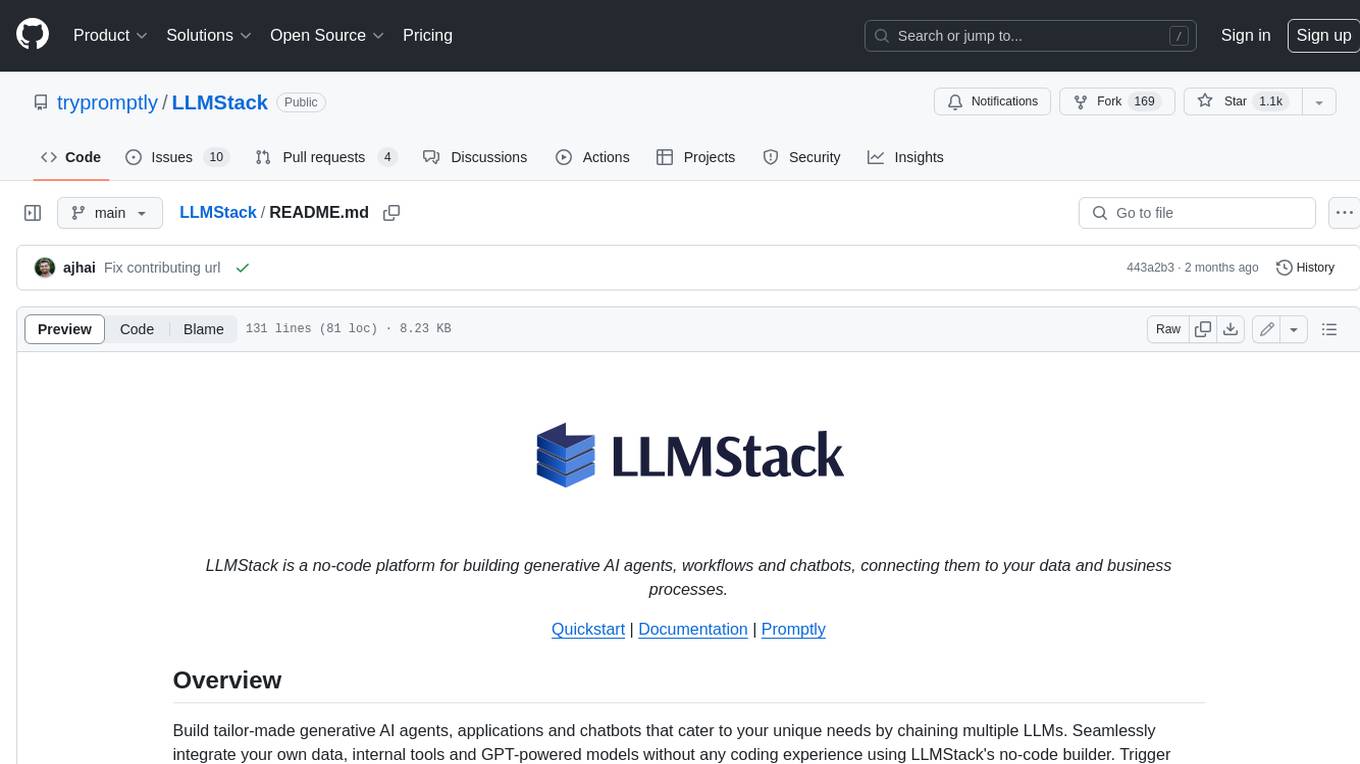
LLMStack
LLMStack is a no-code platform for building generative AI agents, workflows, and chatbots. It allows users to connect their own data, internal tools, and GPT-powered models without any coding experience. LLMStack can be deployed to the cloud or on-premise and can be accessed via HTTP API or triggered from Slack or Discord.
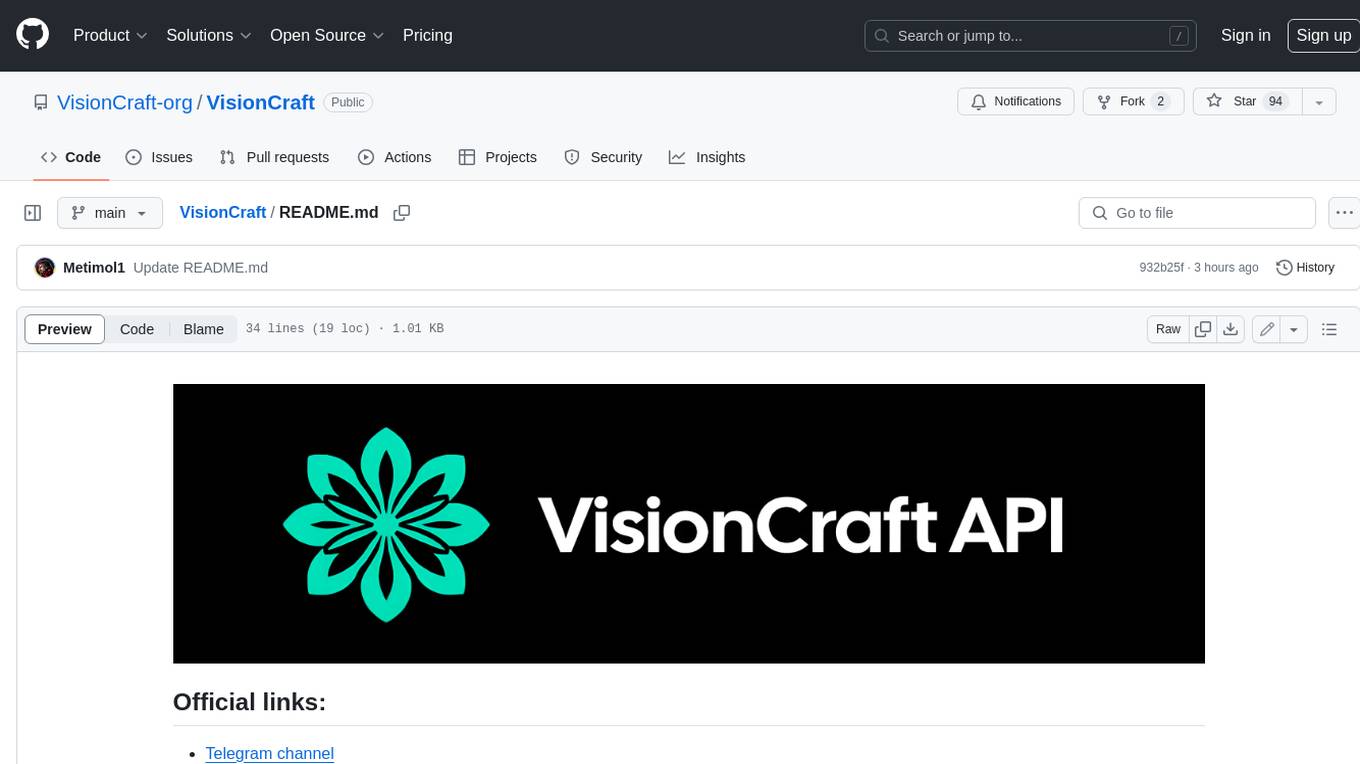
VisionCraft
The VisionCraft API is a free API for using over 100 different AI models. From images to sound.
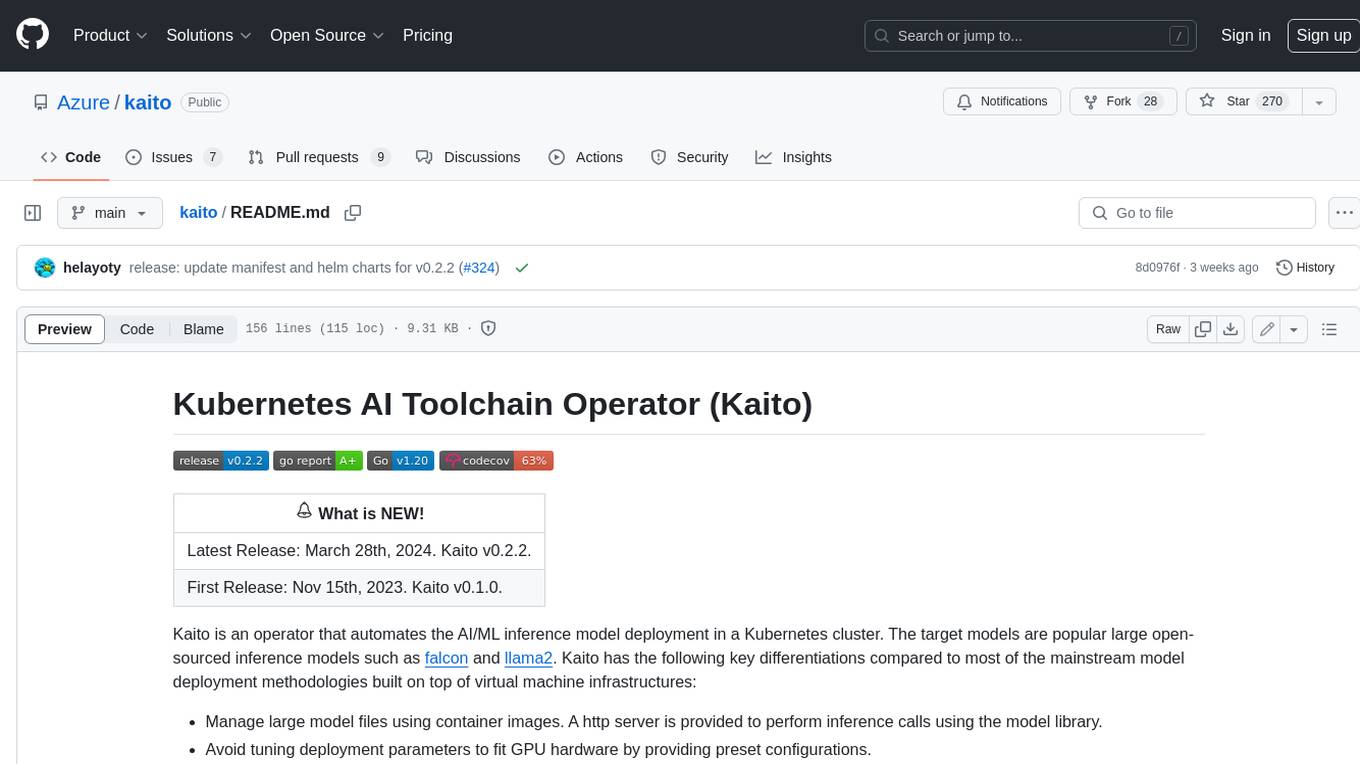
kaito
Kaito is an operator that automates the AI/ML inference model deployment in a Kubernetes cluster. It manages large model files using container images, avoids tuning deployment parameters to fit GPU hardware by providing preset configurations, auto-provisions GPU nodes based on model requirements, and hosts large model images in the public Microsoft Container Registry (MCR) if the license allows. Using Kaito, the workflow of onboarding large AI inference models in Kubernetes is largely simplified.
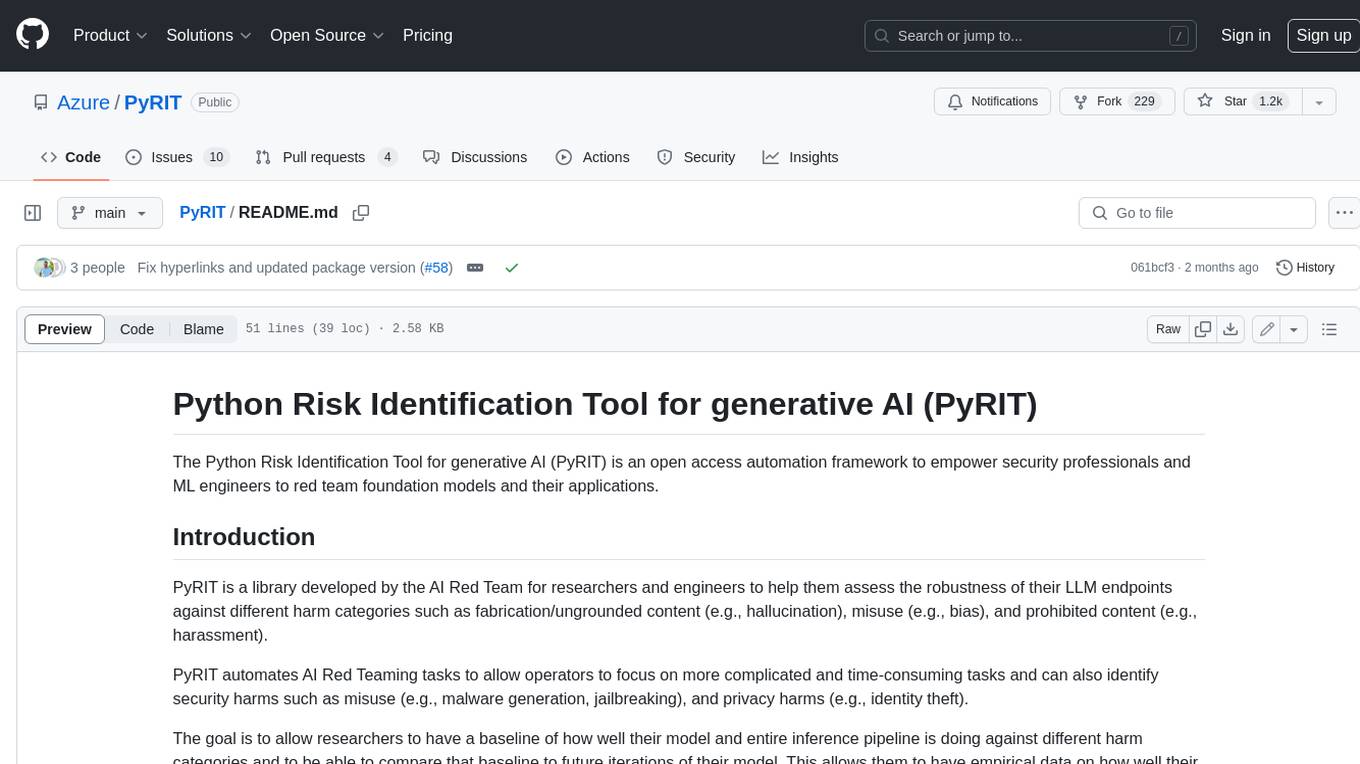
PyRIT
PyRIT is an open access automation framework designed to empower security professionals and ML engineers to red team foundation models and their applications. It automates AI Red Teaming tasks to allow operators to focus on more complicated and time-consuming tasks and can also identify security harms such as misuse (e.g., malware generation, jailbreaking), and privacy harms (e.g., identity theft). The goal is to allow researchers to have a baseline of how well their model and entire inference pipeline is doing against different harm categories and to be able to compare that baseline to future iterations of their model. This allows them to have empirical data on how well their model is doing today, and detect any degradation of performance based on future improvements.
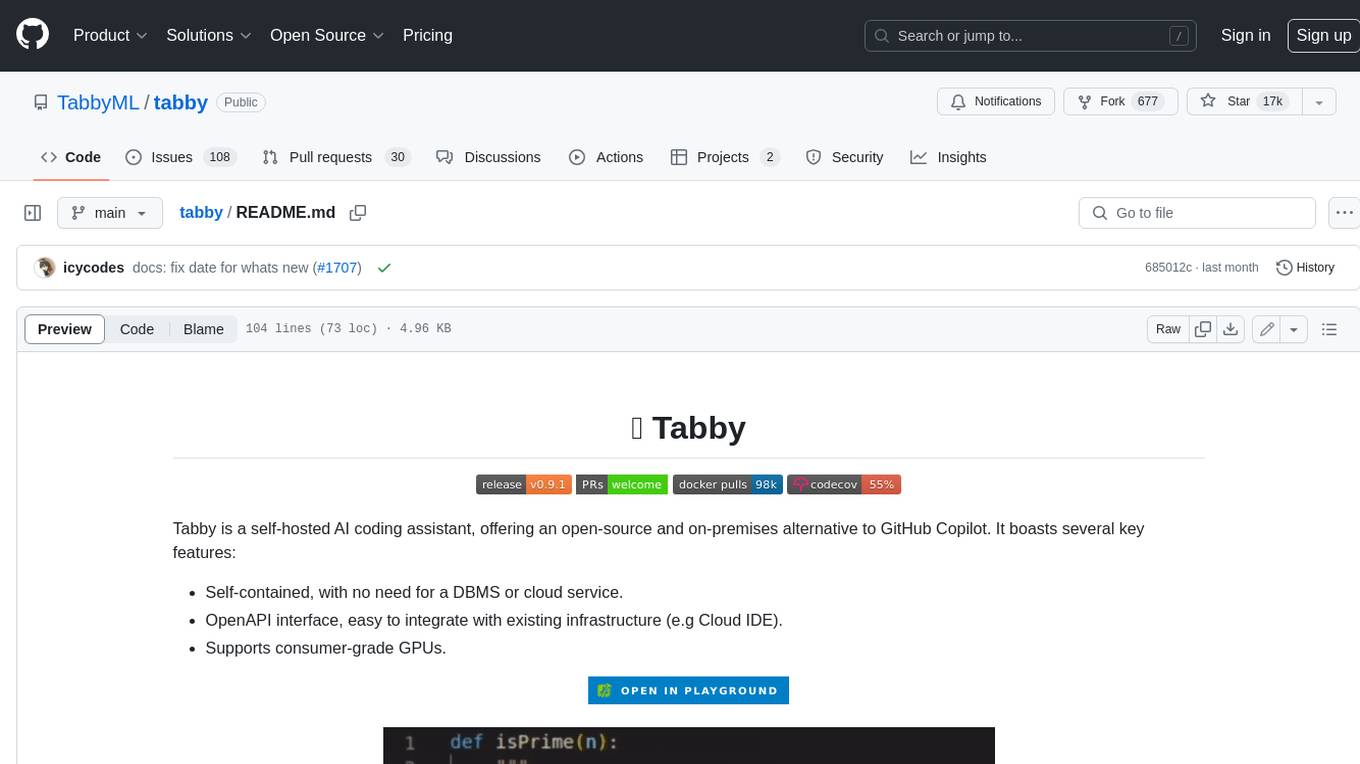
tabby
Tabby is a self-hosted AI coding assistant, offering an open-source and on-premises alternative to GitHub Copilot. It boasts several key features: * Self-contained, with no need for a DBMS or cloud service. * OpenAPI interface, easy to integrate with existing infrastructure (e.g Cloud IDE). * Supports consumer-grade GPUs.
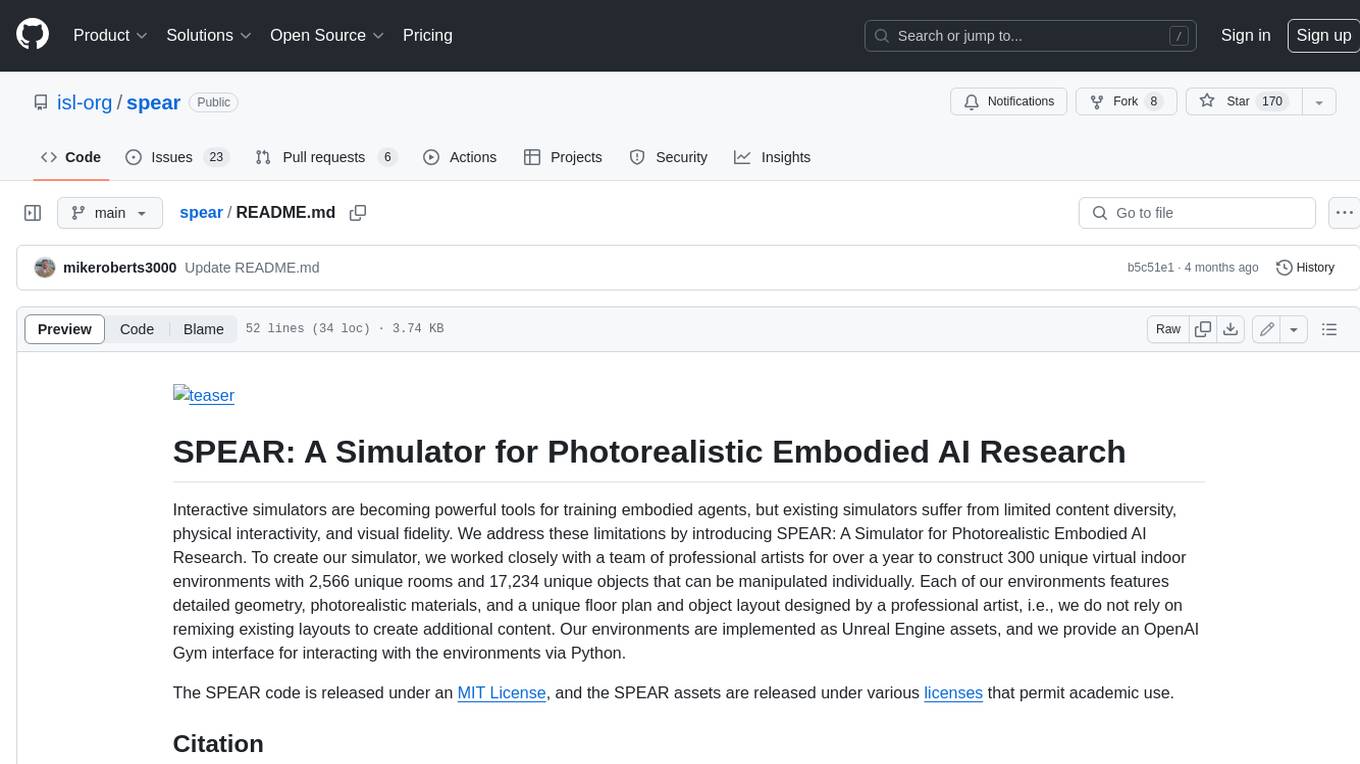
spear
SPEAR (Simulator for Photorealistic Embodied AI Research) is a powerful tool for training embodied agents. It features 300 unique virtual indoor environments with 2,566 unique rooms and 17,234 unique objects that can be manipulated individually. Each environment is designed by a professional artist and features detailed geometry, photorealistic materials, and a unique floor plan and object layout. SPEAR is implemented as Unreal Engine assets and provides an OpenAI Gym interface for interacting with the environments via Python.
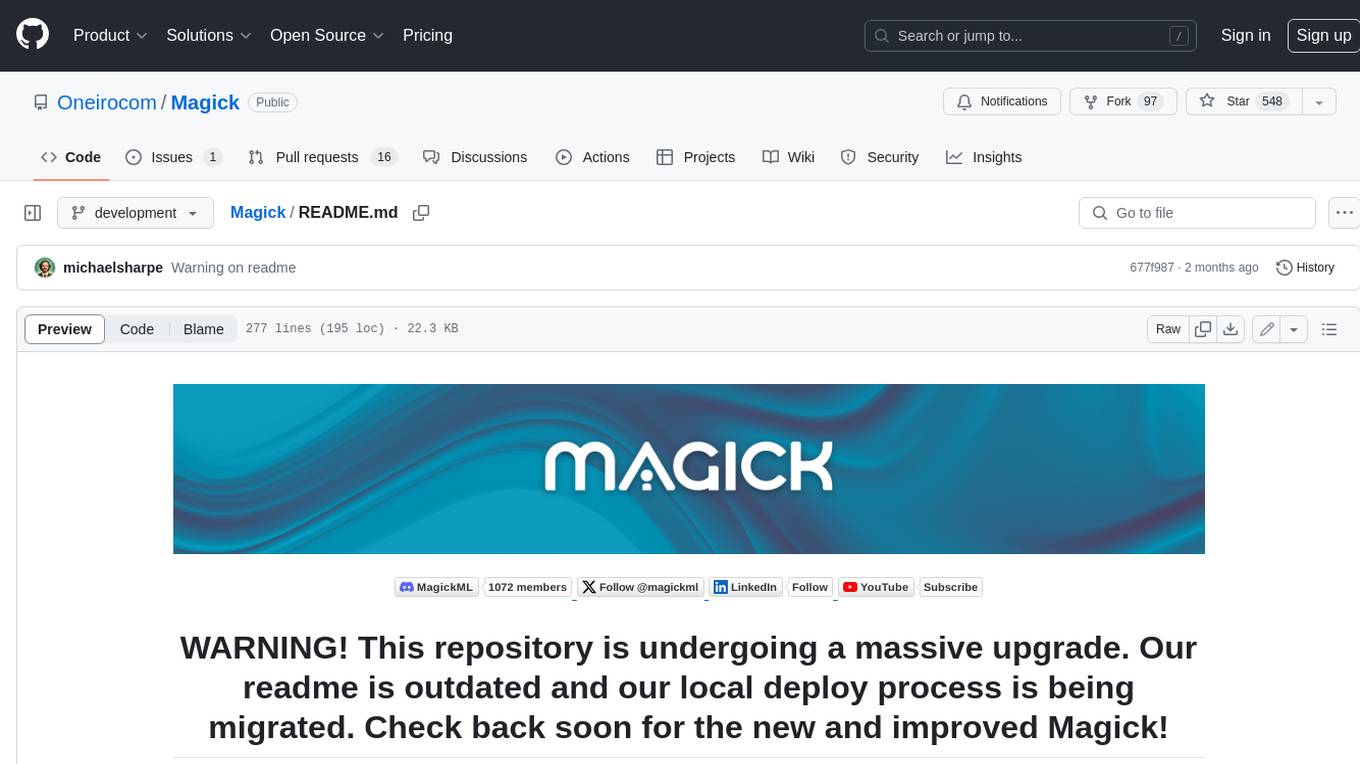
Magick
Magick is a groundbreaking visual AIDE (Artificial Intelligence Development Environment) for no-code data pipelines and multimodal agents. Magick can connect to other services and comes with nodes and templates well-suited for intelligent agents, chatbots, complex reasoning systems and realistic characters.