AI tools for codespell
Related Tools:
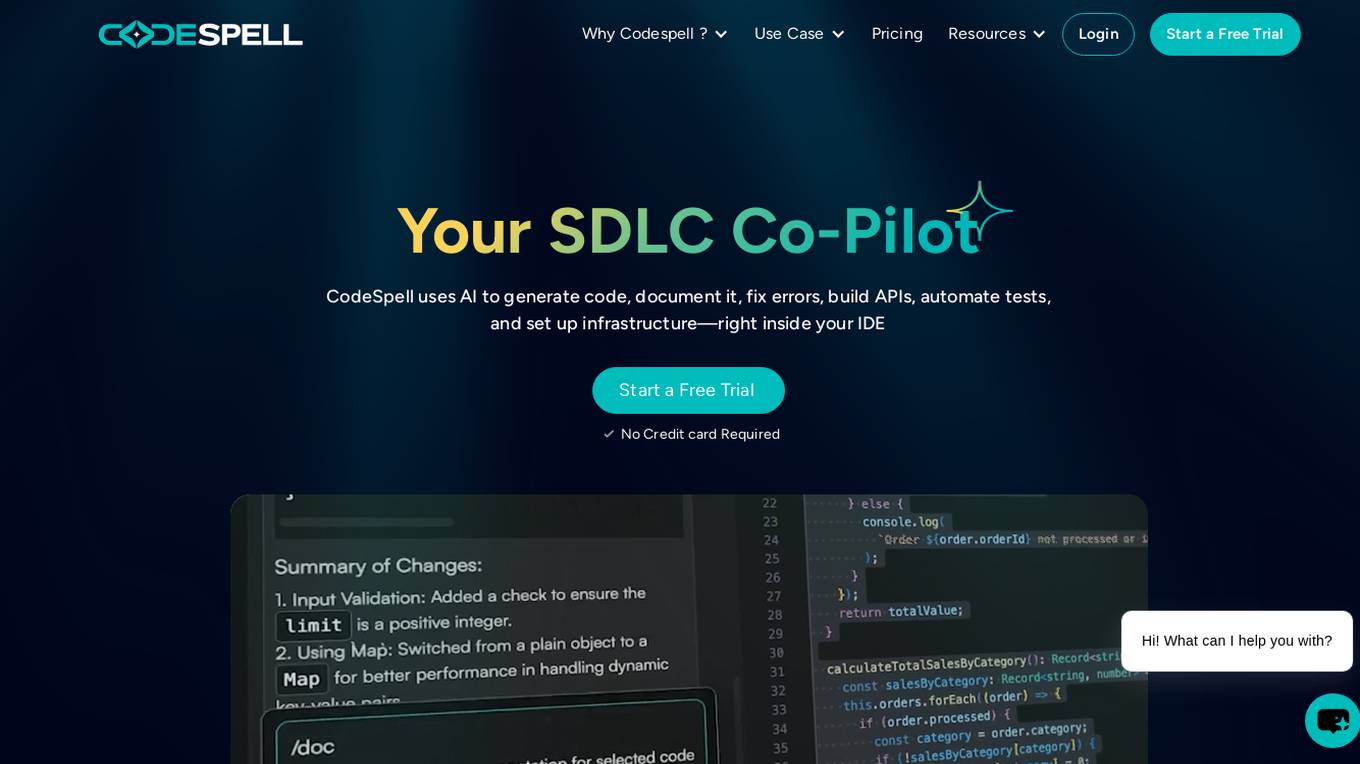
CodeSpell
CodeSpell is an AI-powered code completion tool designed to streamline the software development life cycle (SDLC). It assists developers in generating code, documenting it, fixing errors, building APIs, automating tests, and setting up infrastructure directly within their integrated development environment (IDE). CodeSpell's unique feature, Design Studio, automates project setup by generating scaffolding, APIs, and infrastructure scripts tailored to the user's stack, reducing manual coding effort and accelerating development. The tool is compatible with popular IDEs and supports code generation in any language. CodeSpell aims to transition the software industry from AI-assisted code generation to an AI-driven landscape.
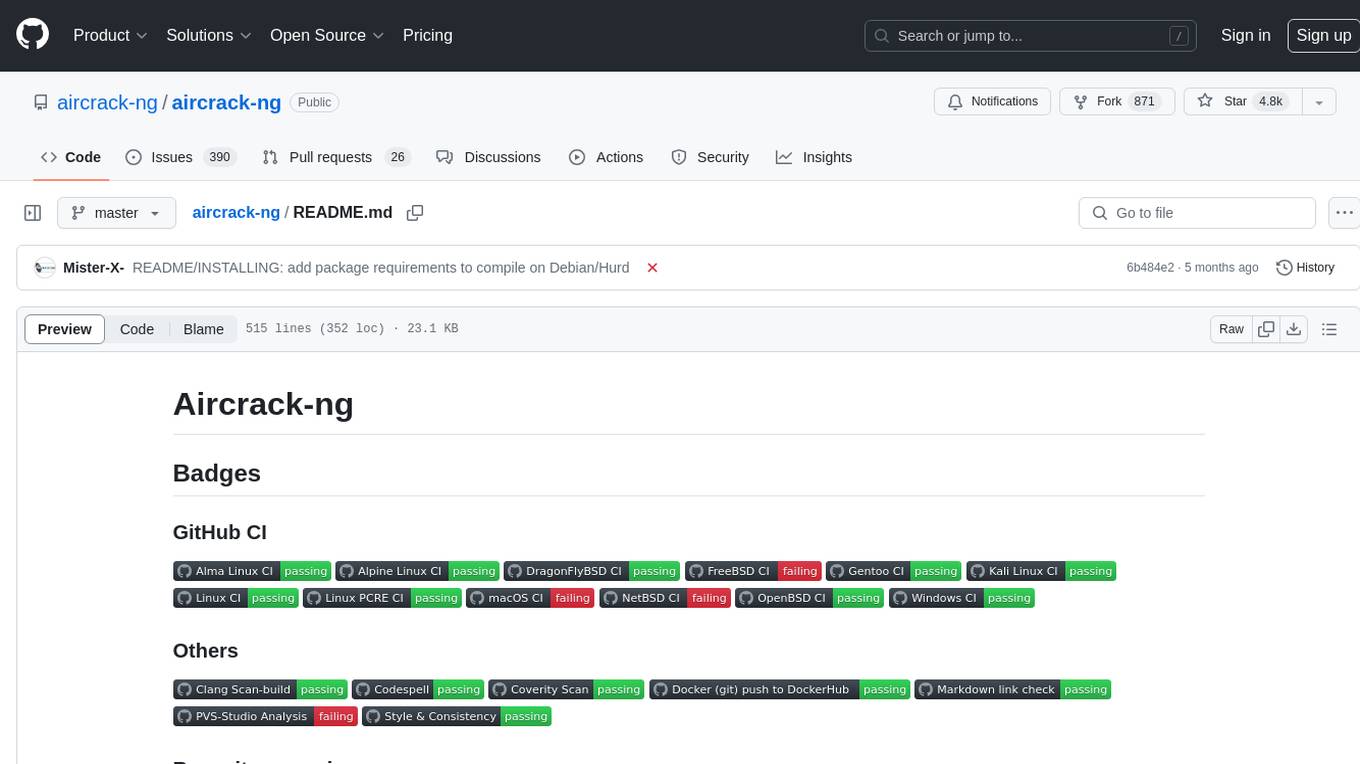
aircrack-ng
Aircrack-ng is a comprehensive suite of tools designed to evaluate the security of WiFi networks. It covers various aspects of WiFi security, including monitoring, attacking (replay attacks, deauthentication, fake access points), testing WiFi cards and driver capabilities, and cracking WEP and WPA PSK. The tools are command line-based, allowing for extensive scripting and have been utilized by many GUIs. Aircrack-ng primarily works on Linux but also supports Windows, macOS, FreeBSD, OpenBSD, NetBSD, Solaris, and eComStation 2.
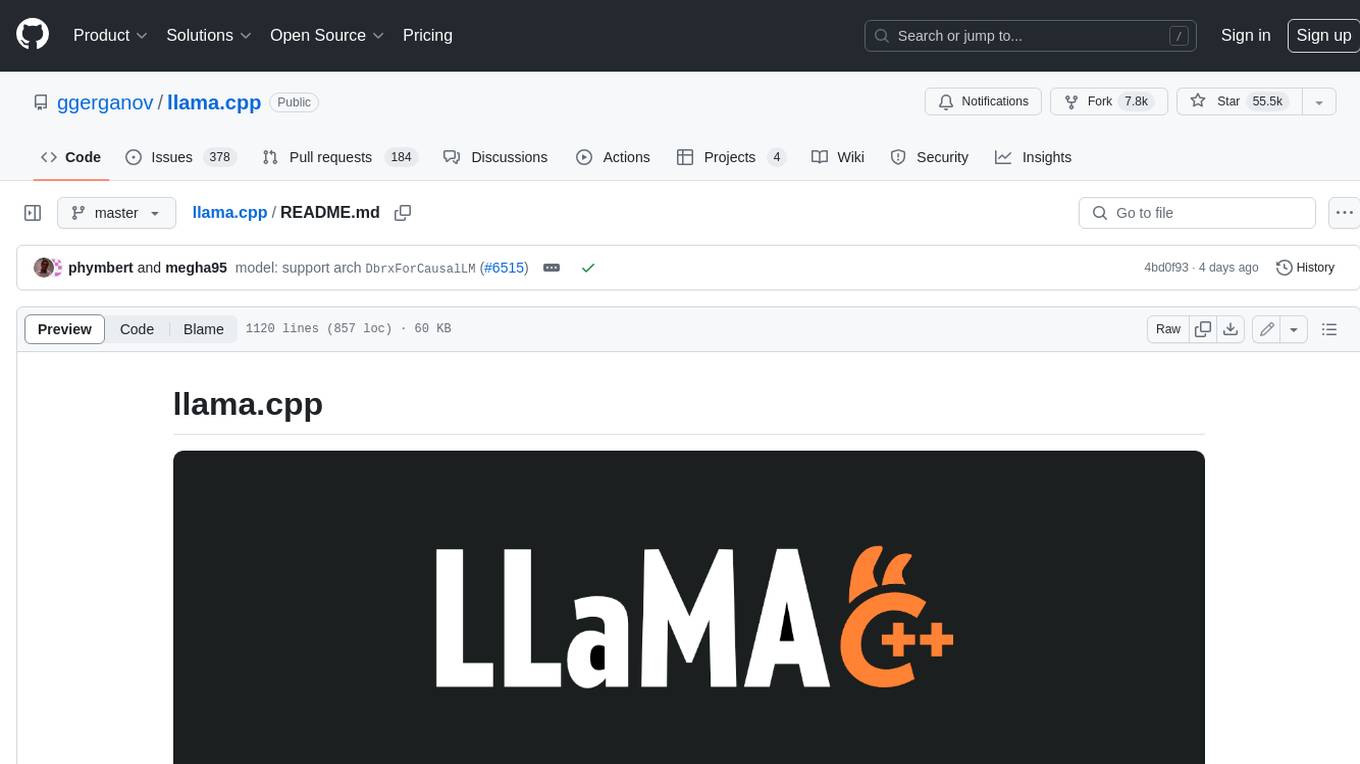
llama.cpp
llama.cpp is a C++ implementation of LLaMA, a large language model from Meta. It provides a command-line interface for inference and can be used for a variety of tasks, including text generation, translation, and question answering. llama.cpp is highly optimized for performance and can be run on a variety of hardware, including CPUs, GPUs, and TPUs.
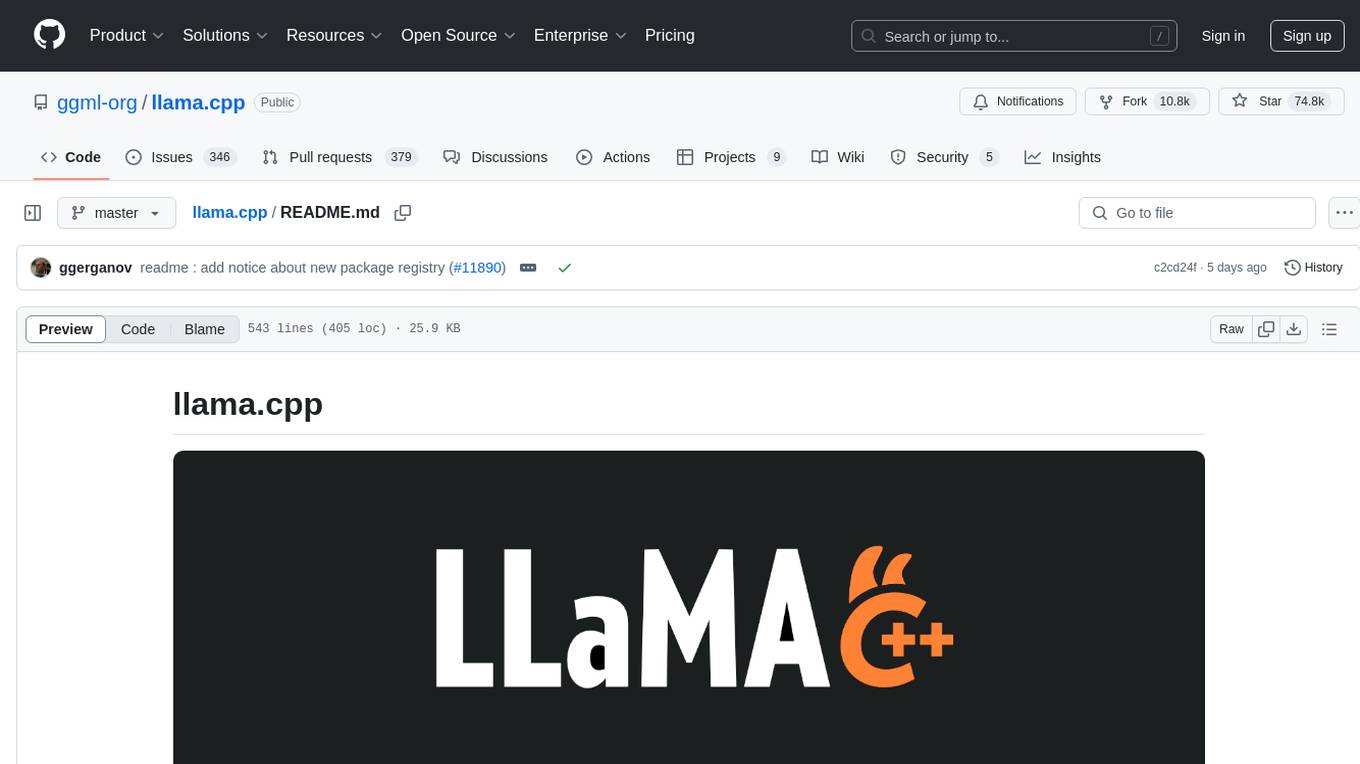
llama.cpp
The main goal of llama.cpp is to enable LLM inference with minimal setup and state-of-the-art performance on a wide range of hardware - locally and in the cloud. It provides a Plain C/C++ implementation without any dependencies, optimized for Apple silicon via ARM NEON, Accelerate and Metal frameworks, and supports various architectures like AVX, AVX2, AVX512, and AMX. It offers integer quantization for faster inference, custom CUDA kernels for NVIDIA GPUs, Vulkan and SYCL backend support, and CPU+GPU hybrid inference. llama.cpp is the main playground for developing new features for the ggml library, supporting various models and providing tools and infrastructure for LLM deployment.
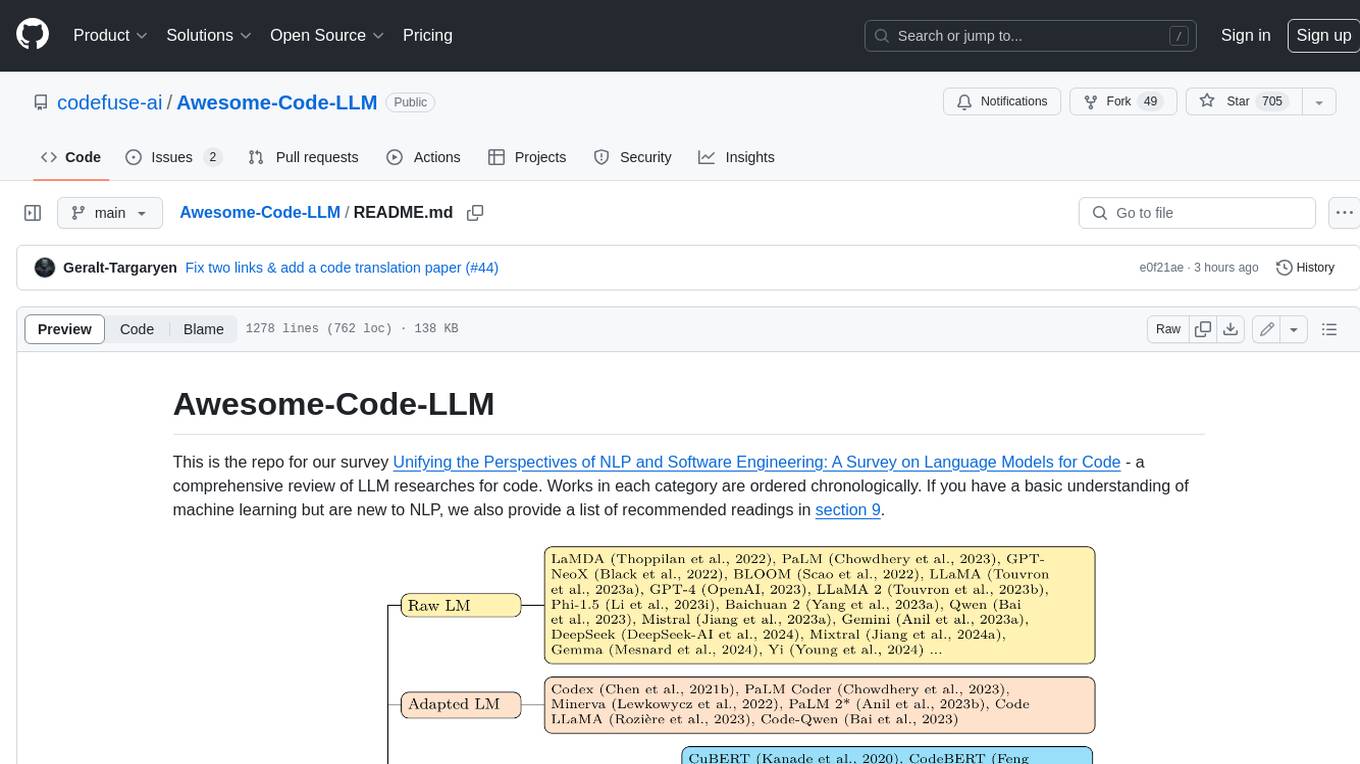
Awesome-Code-LLM
Analyze the following text from a github repository (name and readme text at end) . Then, generate a JSON object with the following keys and provide the corresponding information for each key, in lowercase letters: 'description' (detailed description of the repo, must be less than 400 words,Ensure that no line breaks and quotation marks.),'for_jobs' (List 5 jobs suitable for this tool,in lowercase letters), 'ai_keywords' (keywords of the tool,user may use those keyword to find the tool,in lowercase letters), 'for_tasks' (list of 5 specific tasks user can use this tool to do,in lowercase letters), 'answer' (in english languages)
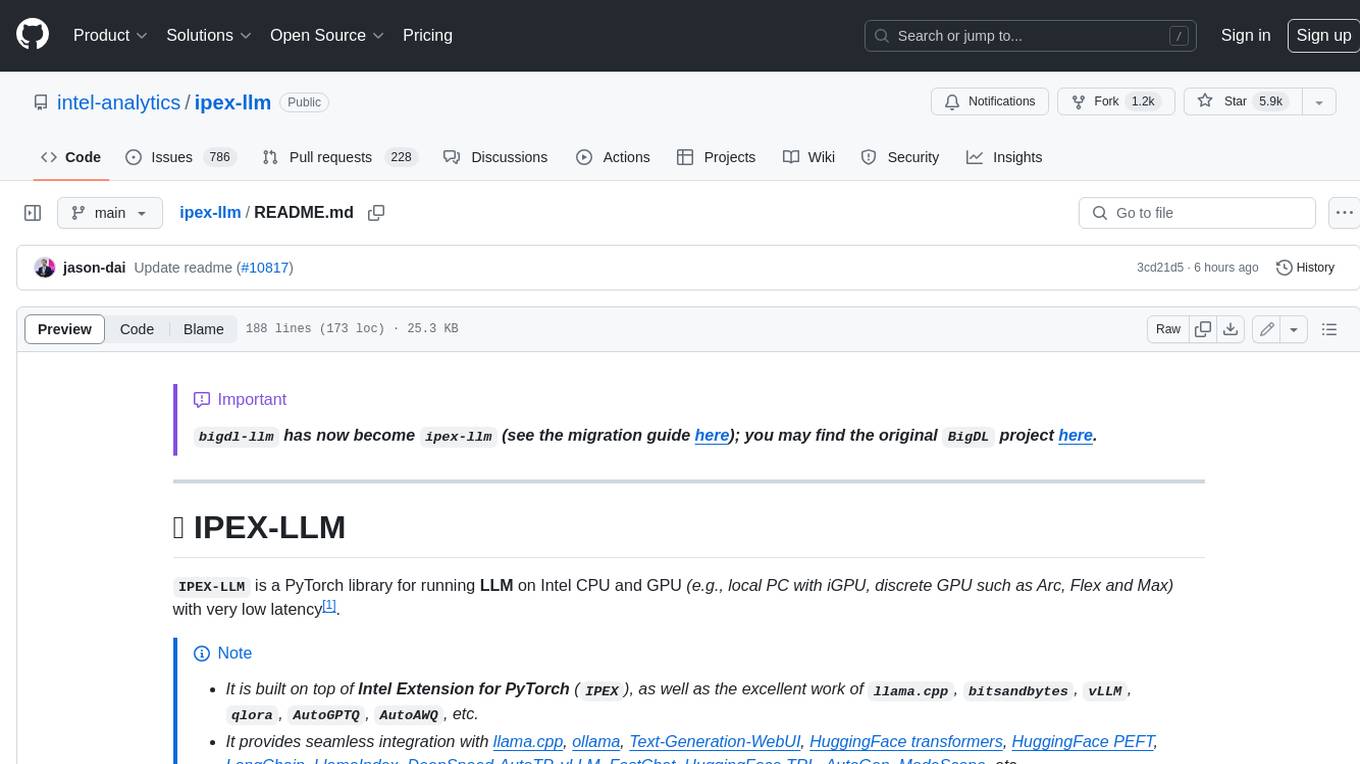
ipex-llm
IPEX-LLM is a PyTorch library for running Large Language Models (LLMs) on Intel CPUs and GPUs with very low latency. It provides seamless integration with various LLM frameworks and tools, including llama.cpp, ollama, Text-Generation-WebUI, HuggingFace transformers, and more. IPEX-LLM has been optimized and verified on over 50 LLM models, including LLaMA, Mistral, Mixtral, Gemma, LLaVA, Whisper, ChatGLM, Baichuan, Qwen, and RWKV. It supports a range of low-bit inference formats, including INT4, FP8, FP4, INT8, INT2, FP16, and BF16, as well as finetuning capabilities for LoRA, QLoRA, DPO, QA-LoRA, and ReLoRA. IPEX-LLM is actively maintained and updated with new features and optimizations, making it a valuable tool for researchers, developers, and anyone interested in exploring and utilizing LLMs.
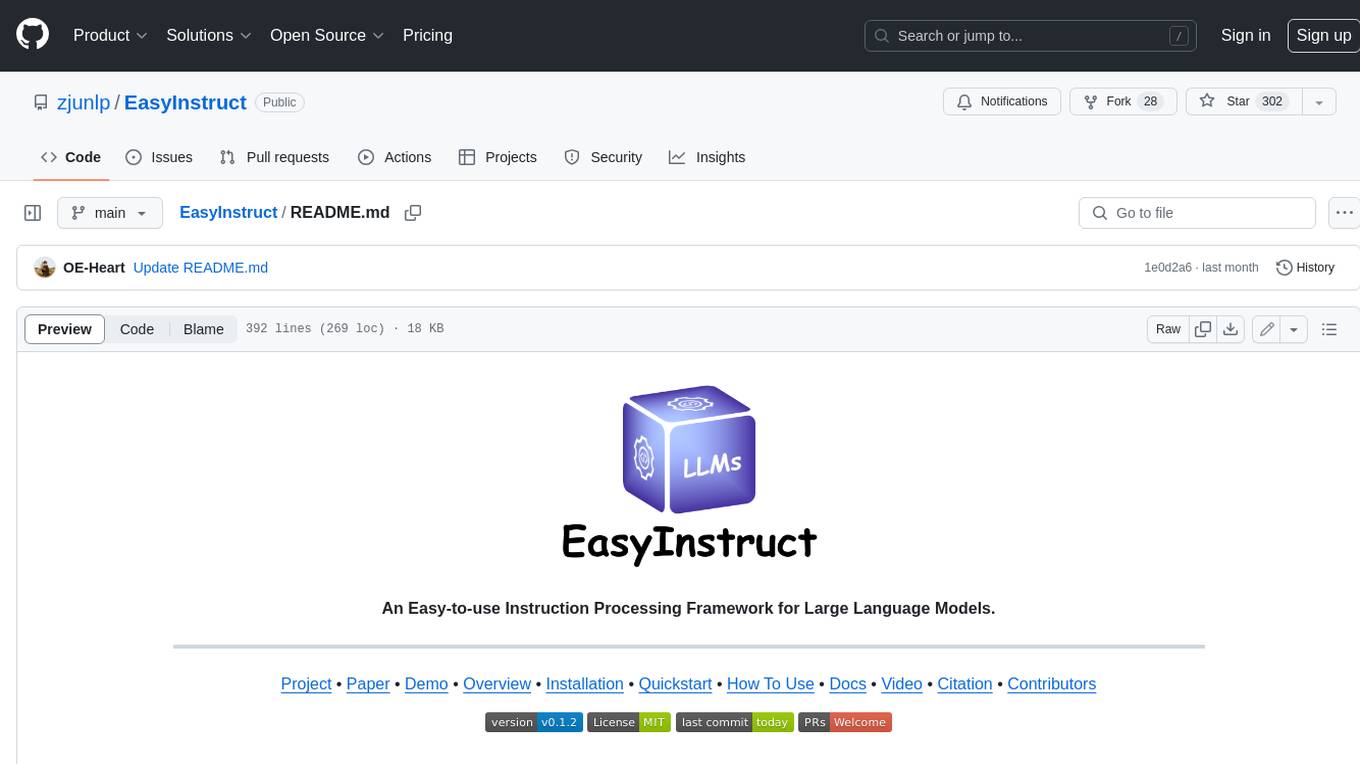
EasyInstruct
EasyInstruct is a Python package proposed as an easy-to-use instruction processing framework for Large Language Models (LLMs) like GPT-4, LLaMA, ChatGLM in your research experiments. EasyInstruct modularizes instruction generation, selection, and prompting, while also considering their combination and interaction.