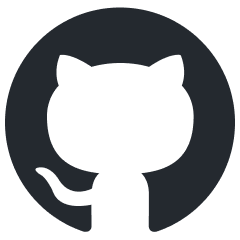
cosmos-predict1
Cosmos-Predict1 is a collection of general-purpose world foundation models for Physical AI that can be fine-tuned into customized world models for downstream applications.
Stars: 115
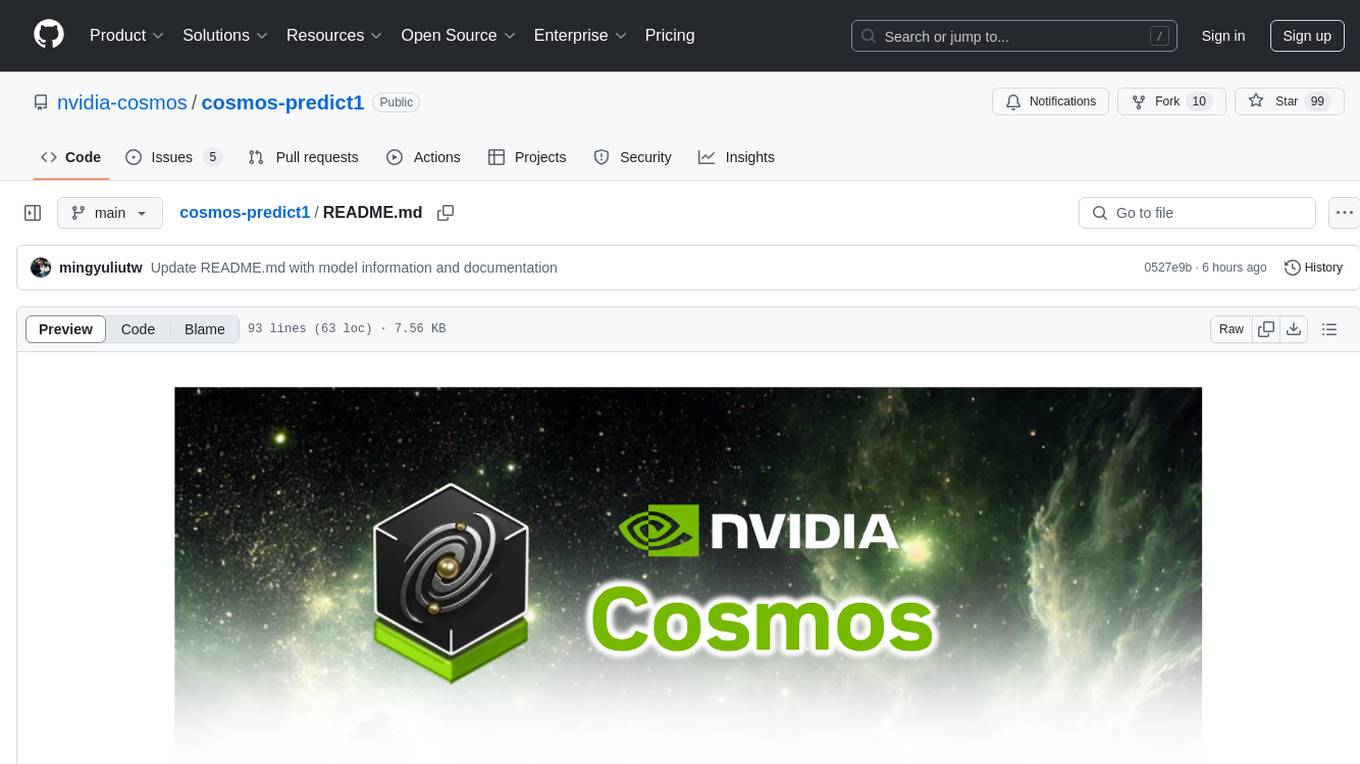
README:
Cosmos-Predict1 is a key branch of Cosmos World Foundation Models (WFMs) specialized for future state prediction, often referred to as world models. The tree main branches of Cosmos WFMs are cosmos-predict, cosmos-transfer, and cosmos-reason. We visualize the architecture of Cosmos-Predict1 in the following figure.
Cosmos-Predict1 includes the following:
- Diffusion-based world foundation models for Text2World and Video2World generation, where a user can generate visual simulation based on text prompts and video prompts.
- Autoregressive-based world foundation models for Video2World generation, where a user can generate visual simulation based on video prompts and optional text prompts.
- Image and video tokenizers for tokenizing videos into continuous tokens (latent vectors) and discrete tokens (integers) efficiently and effectively.
- Post-training scripts for helping Physical AI builders post-train pre-trained Cosmos-Predict1 for their applications.
- Pre-training scripts for helping Physical AI builders train their WFMs from scratch.
Your browser does not support the video tag.
Your browser does not support the video tag.
We provide a comphrehensive set of examples to illustrate how to perform inference, post-training, etc, with Cosmos-Predict1. Click a relevant example below and start your Cosmos journey.
Please refer to INSTALL.md for general instructions on environment setup.
- Inference with diffusion-based Text2World models [with multi-GPU support]
- Inference with diffusion-based Video2World models [with multi-GPU support]
- Inference with autoregressive-based base models [with multi-GPU support]
- Inference with autoregressive-based Video2World models [with multi-GPU support]
- Inference with tokenizer models
- Post-train diffusion-based Text2World models using custom datasets [with multi-node support]
- Post-train diffusion-based Video2World models using custom datasets [with multi-node support]
- Post-train diffusion-based Text2World models using custom multi-view datasets [with multi-node support]
- Post-train diffusion-based Video2World models using custom multi-view datasets) [with multi-node support]
- Post-train autoregressive-based base models using custom datasets [with multi-node support]
- Post-train tokenizers using custom datasets [with multi-node support]
- Inference with post-trained multi-view diffusion-based Text2World models) [with multi-GPU support]
- Inference with post-trained multi-view diffusion-based Video2World models) [with multi-GPU support]
Cosmos-Predict1 include the following models
Diffusion models
- Cosmos-Predict1-7B-Text2World: Text to visual world generation
- Cosmos-Predict1-14B-Text2World: Text to visual world generation
- Cosmos-Predict1-7B-Video2World: Video + Text based future visual world generation
- Cosmos-Predict1-14B-Video2World: Video + Text based future visual world generation
Autoregressive models
- Cosmos-Predict1-4B: Future visual world generation
- Cosmos-Predict1-12B: Future visual world generation
- Cosmos-Predict1-5B-Video2World: Video + Text based future visual world generation
- Cosmos-Predict1-13B-Video2World: Video + Text based future visual world generation
Tokenizers
- Cosmos-Tokenize1-CV8×8×8-720p: Continuous Video Tokenizer with 8x8x8 spatio-temporal compression with, 121 frames context
- Cosmos-Tokenize1-DV8×16×16-720p: Discrete Video Tokenizer with 8x16x16 spatio-temporal compression, and 49 frames context
- Cosmos-Tokenize1-CI8×8-360p: Continuous Image Tokenizer with 8x8 spatial compression with low-resolution support
- Cosmos-Tokenize1-CI16x16-360p: Continuous Image Tokenizer with 16x16 spatial compression with low-resolution support
- Cosmos-Tokenize1-CV4×8×8-360p: Continuous Video Tokenizer with 4x8x8 spatio-temporal compression with low-resolution support
- Cosmos-Tokenize1-DI8×8-360p: Discrete Image Tokenizer with 8x8 spatial compression with low-resolution support
- Cosmos-Tokenize1-DI16x16-360p: Discrete Image Tokenizer with 16x16 spatial compression with low-resolution support
- Cosmos-Tokenize1-DV4×8×8-360p: Discrete Video Tokenizer with 4x8x8 spatio-temporal compression with low-resolution support
This project will download and install additional third-party open source software projects. Review the license terms of these open source projects before use.
NVIDIA Cosmos source code is released under the Apache 2 License.
NVIDIA Cosmos models are released under the NVIDIA Open Model License. For a custom license (such as exemption of guardrail), please contact [email protected].
For Tasks:
Click tags to check more tools for each tasksFor Jobs:
Alternative AI tools for cosmos-predict1
Similar Open Source Tools
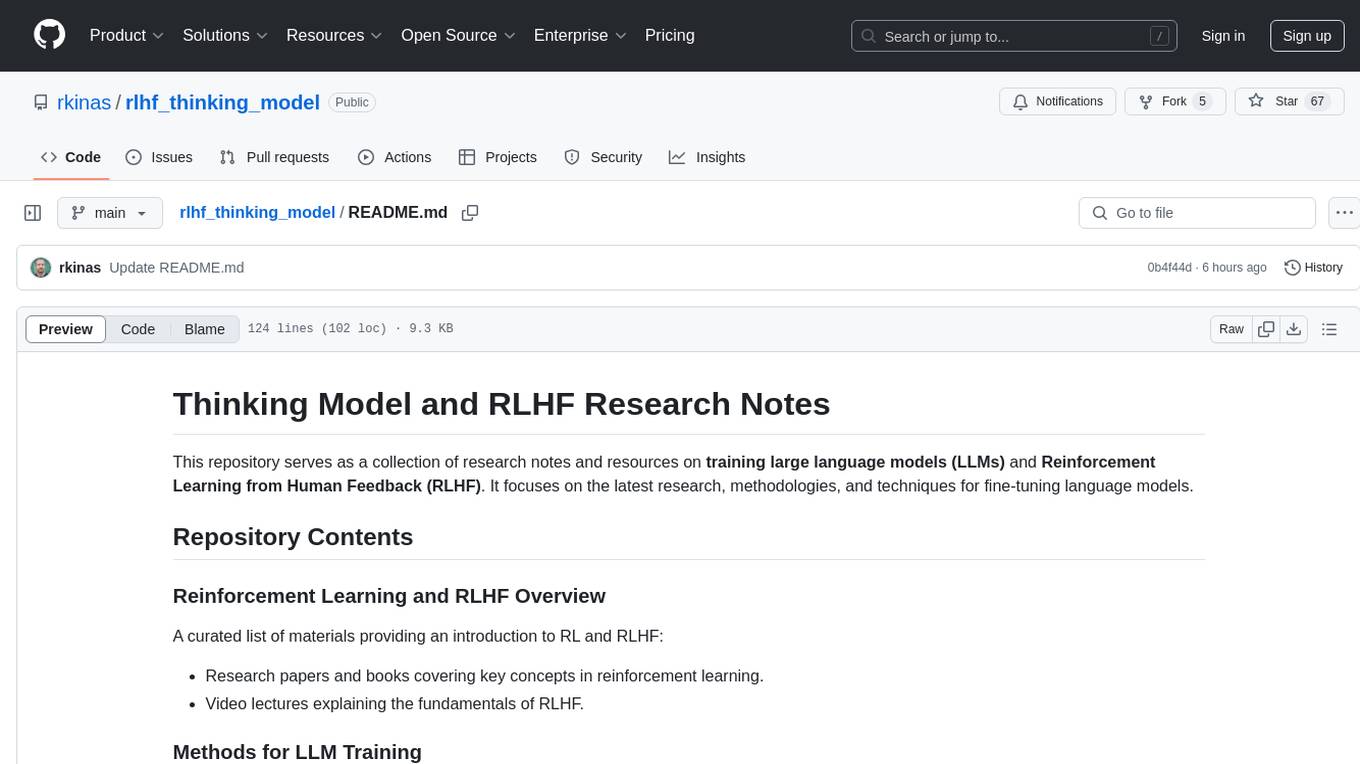
rlhf_thinking_model
This repository is a collection of research notes and resources focusing on training large language models (LLMs) and Reinforcement Learning from Human Feedback (RLHF). It includes methodologies, techniques, and state-of-the-art approaches for optimizing preferences and model alignment in LLM training. The purpose is to serve as a reference for researchers and engineers interested in reinforcement learning, large language models, model alignment, and alternative RL-based methods.
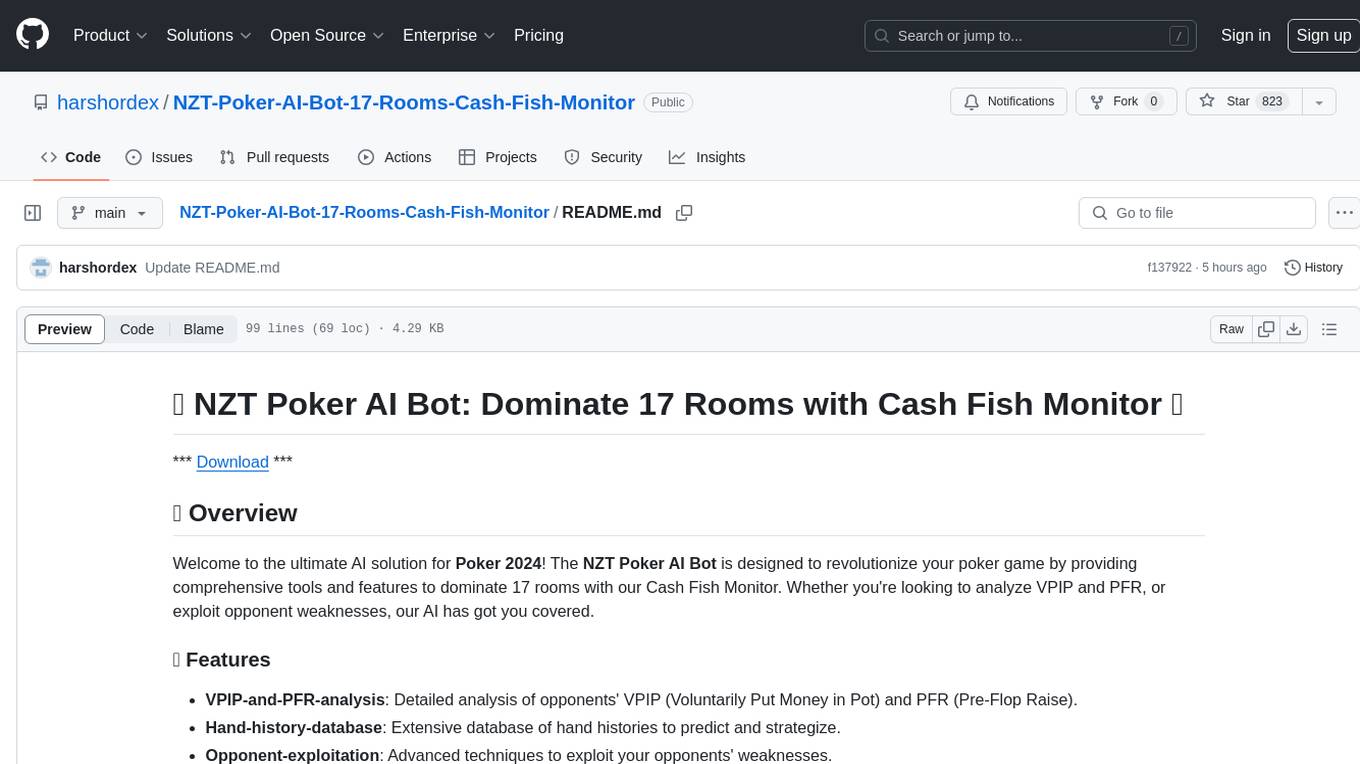
NZT-Poker-AI-Bot-17-Rooms-Cash-Fish-Monitor
The NZT Poker AI Bot is an advanced tool designed to revolutionize poker gameplay by providing comprehensive features to dominate 17 rooms with the Cash Fish Monitor. It offers detailed analysis of opponents' VPIP and PFR, extensive hand history database, opponent exploitation techniques, data-driven intelligence, player profiles, advanced neural network technology, expert training, continuous refinement, and cutting-edge algorithms for maximizing poker profits. Created by a team of poker experts, this AI tool continuously adapts to the latest poker strategies and utilizes state-of-the-art technology to enhance decision-making prowess.
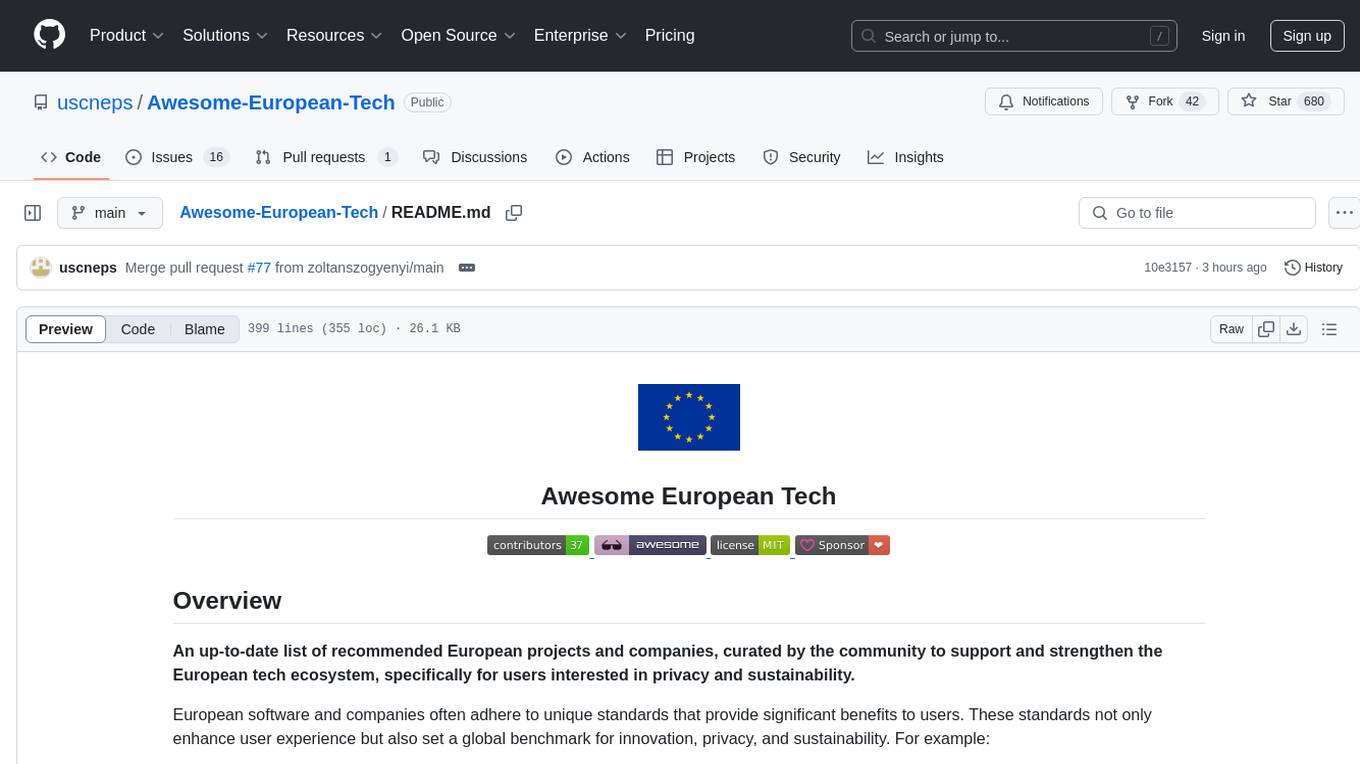
Awesome-European-Tech
Awesome European Tech is an up-to-date list of recommended European projects and companies curated by the community to support and strengthen the European tech ecosystem. It focuses on privacy and sustainability, showcasing companies that adhere to GDPR compliance and sustainability standards. The project aims to highlight and support European startups and projects excelling in privacy, sustainability, and innovation to contribute to a more diverse, resilient, and interconnected global tech landscape.
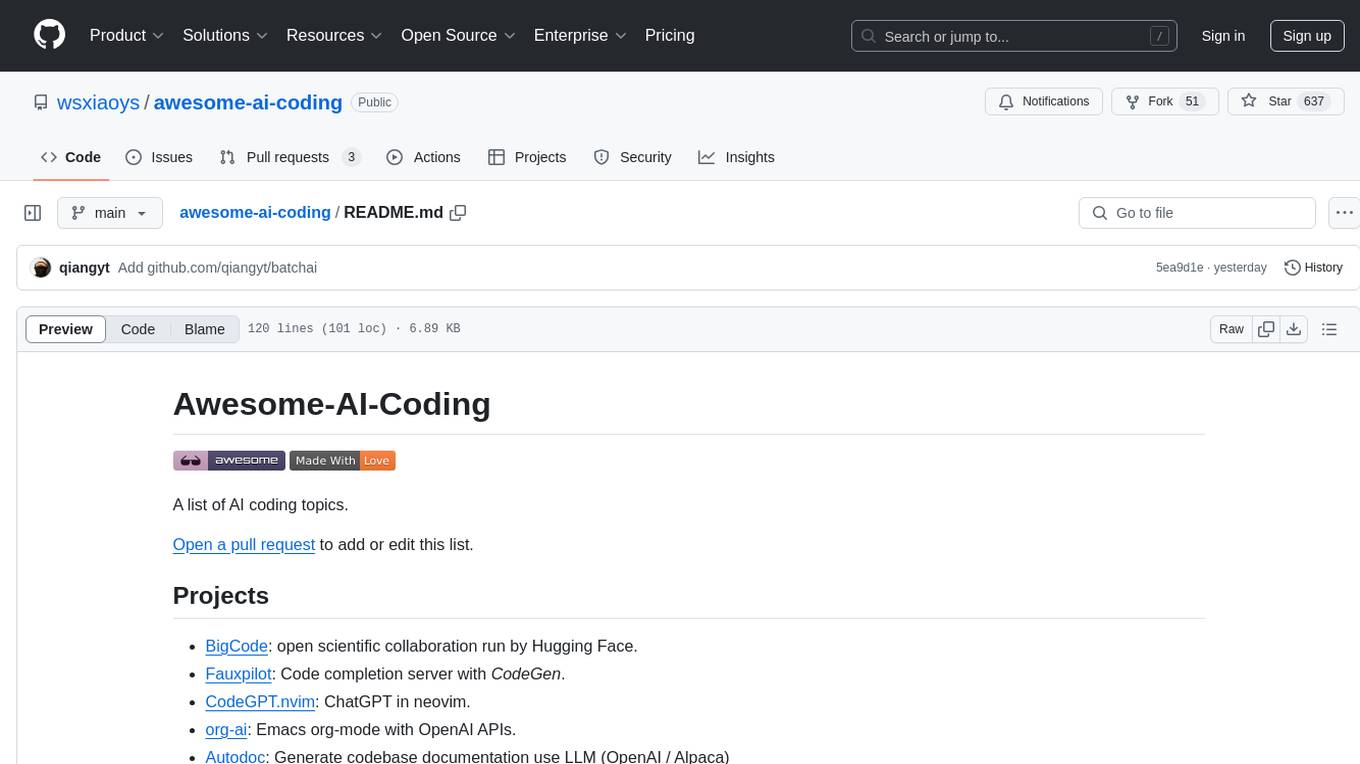
awesome-ai-coding
Awesome-AI-Coding is a curated list of AI coding topics, projects, datasets, LLM models, embedding models, papers, blogs, products, startups, and peer awesome lists related to artificial intelligence in coding. It includes tools for code completion, code generation, code documentation, and code search, as well as AI models and techniques for improving developer productivity. The repository also features information on various AI-powered developer tools, copilots, and related resources in the AI coding domain.
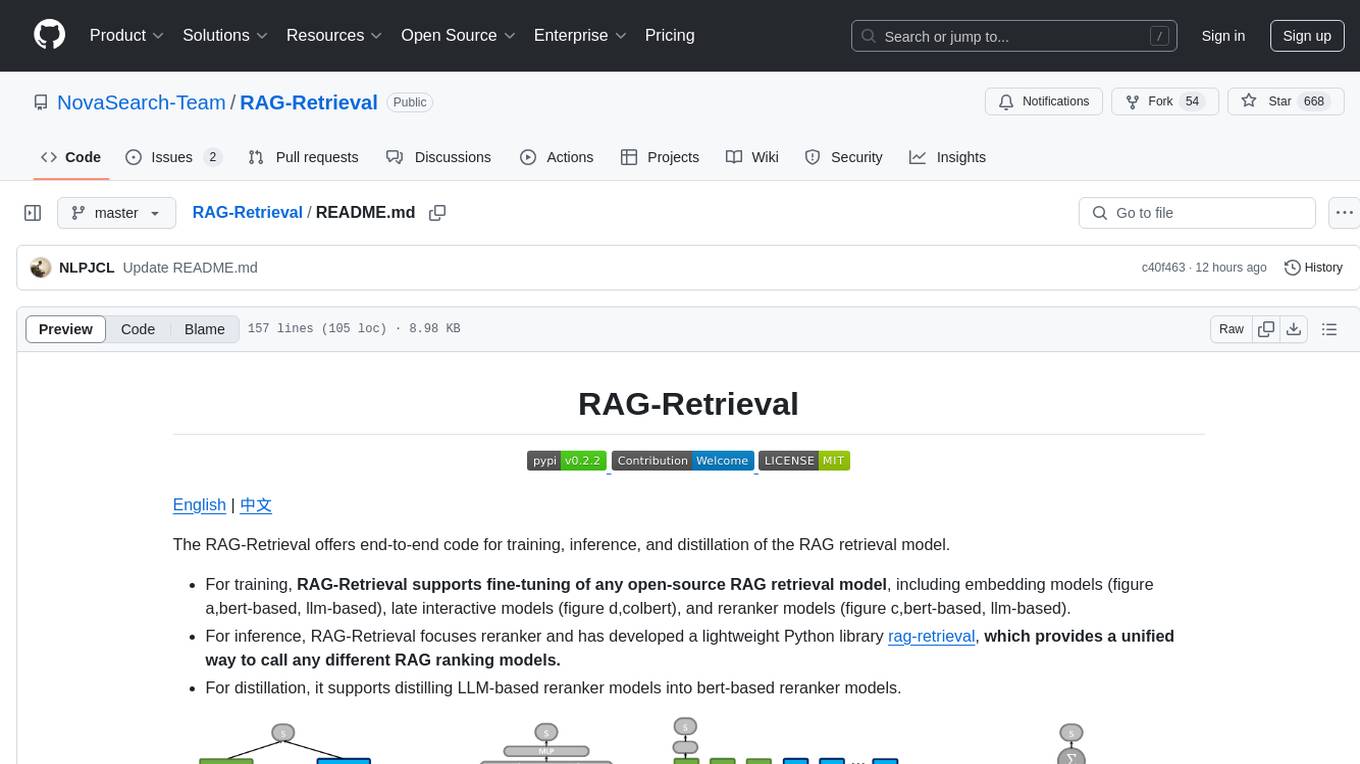
RAG-Retrieval
RAG-Retrieval is an end-to-end code repository that provides training, inference, and distillation capabilities for the RAG retrieval model. It supports fine-tuning of various open-source RAG retrieval models, including embedding models, late interactive models, and reranker models. The repository offers a lightweight Python library for calling different RAG ranking models and allows distillation of LLM-based reranker models into bert-based reranker models. It includes features such as support for end-to-end fine-tuning, distillation of large models, advanced algorithms like MRL, multi-GPU training strategy, and a simple code structure for easy modifications.
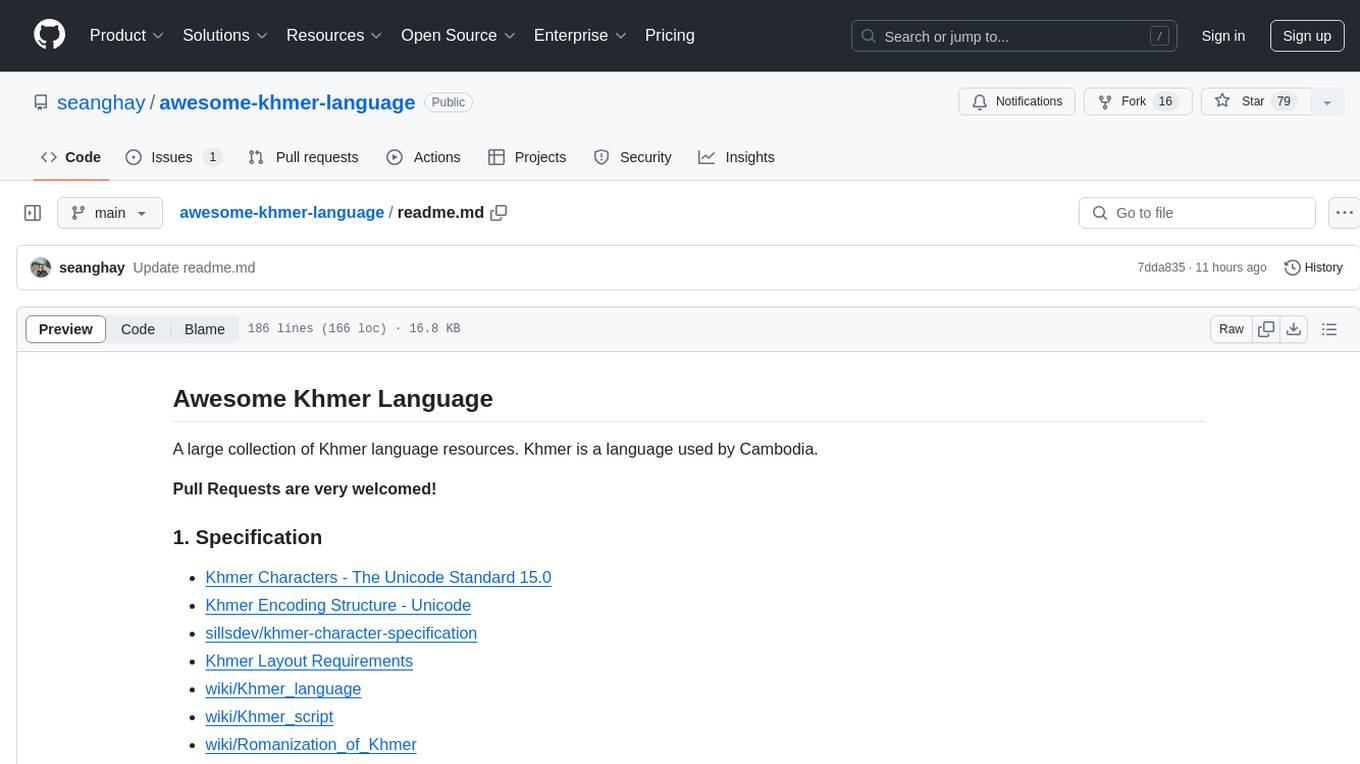
awesome-khmer-language
Awesome Khmer Language is a comprehensive collection of resources for the Khmer language, including tools, datasets, research papers, projects/models, blogs/slides, and miscellaneous items. It covers a wide range of topics related to Khmer language processing, such as character normalization, word segmentation, part-of-speech tagging, optical character recognition, text-to-speech, and more. The repository aims to support the development of natural language processing applications for the Khmer language by providing a diverse set of resources and tools for researchers and developers.
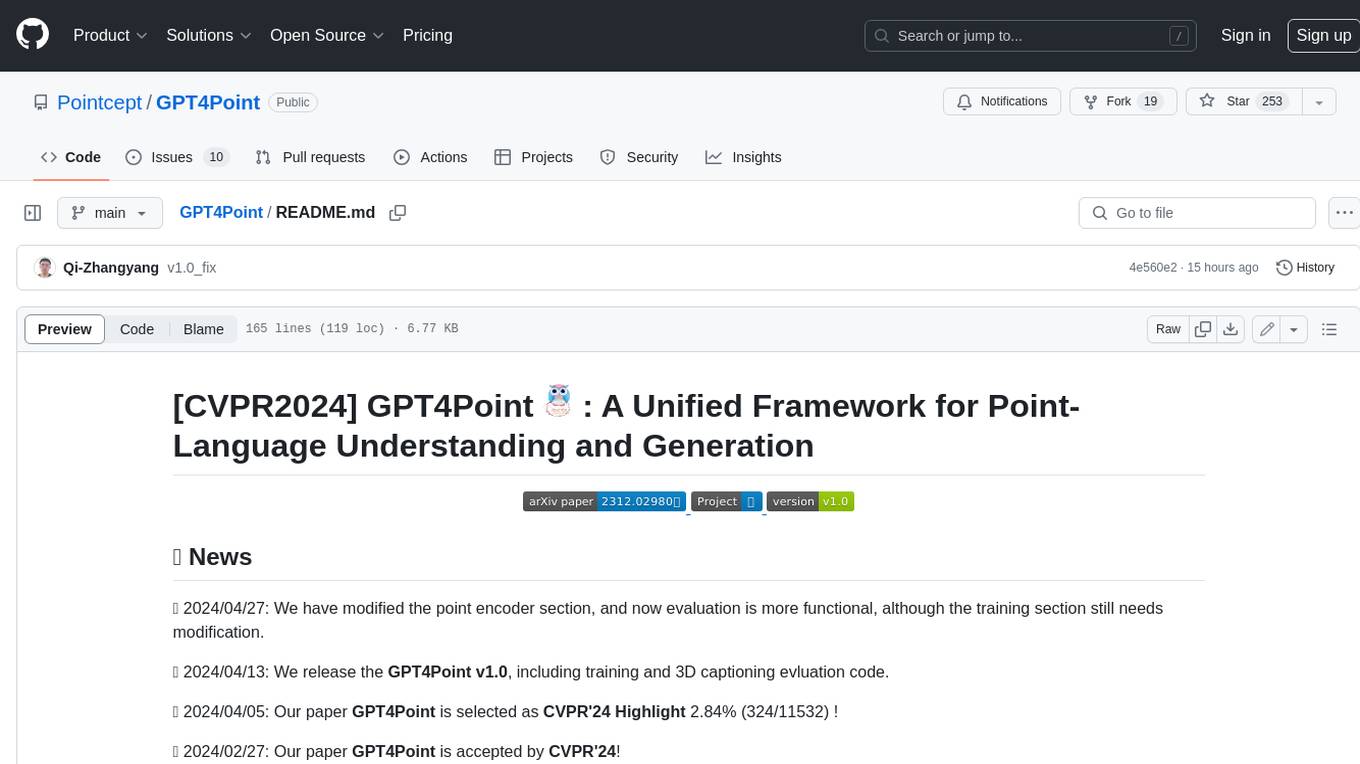
GPT4Point
GPT4Point is a unified framework for point-language understanding and generation. It aligns 3D point clouds with language, providing a comprehensive solution for tasks such as 3D captioning and controlled 3D generation. The project includes an automated point-language dataset annotation engine, a novel object-level point cloud benchmark, and a 3D multi-modality model. Users can train and evaluate models using the provided code and datasets, with a focus on improving models' understanding capabilities and facilitating the generation of 3D objects.
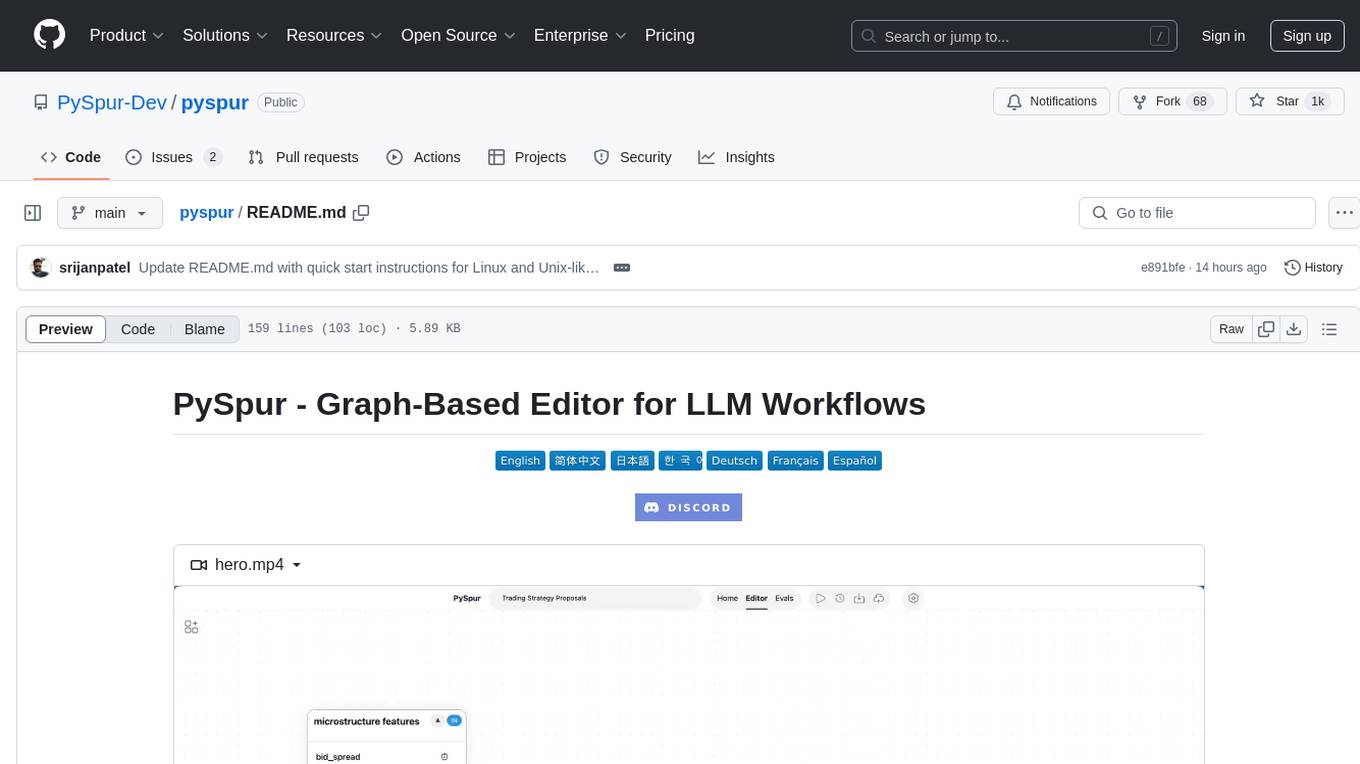
pyspur
PySpur is a graph-based editor designed for LLM (Large Language Models) workflows. It offers modular building blocks, node-level debugging, and performance evaluation. The tool is easy to hack, supports JSON configs for workflow graphs, and is lightweight with minimal dependencies. Users can quickly set up PySpur by cloning the repository, creating a .env file, starting docker services, and accessing the portal. PySpur can also work with local models served using Ollama, with steps provided for configuration. The roadmap includes features like canvas, async/batch execution, support for Ollama, new nodes, pipeline optimization, templates, code compilation, multimodal support, and more.
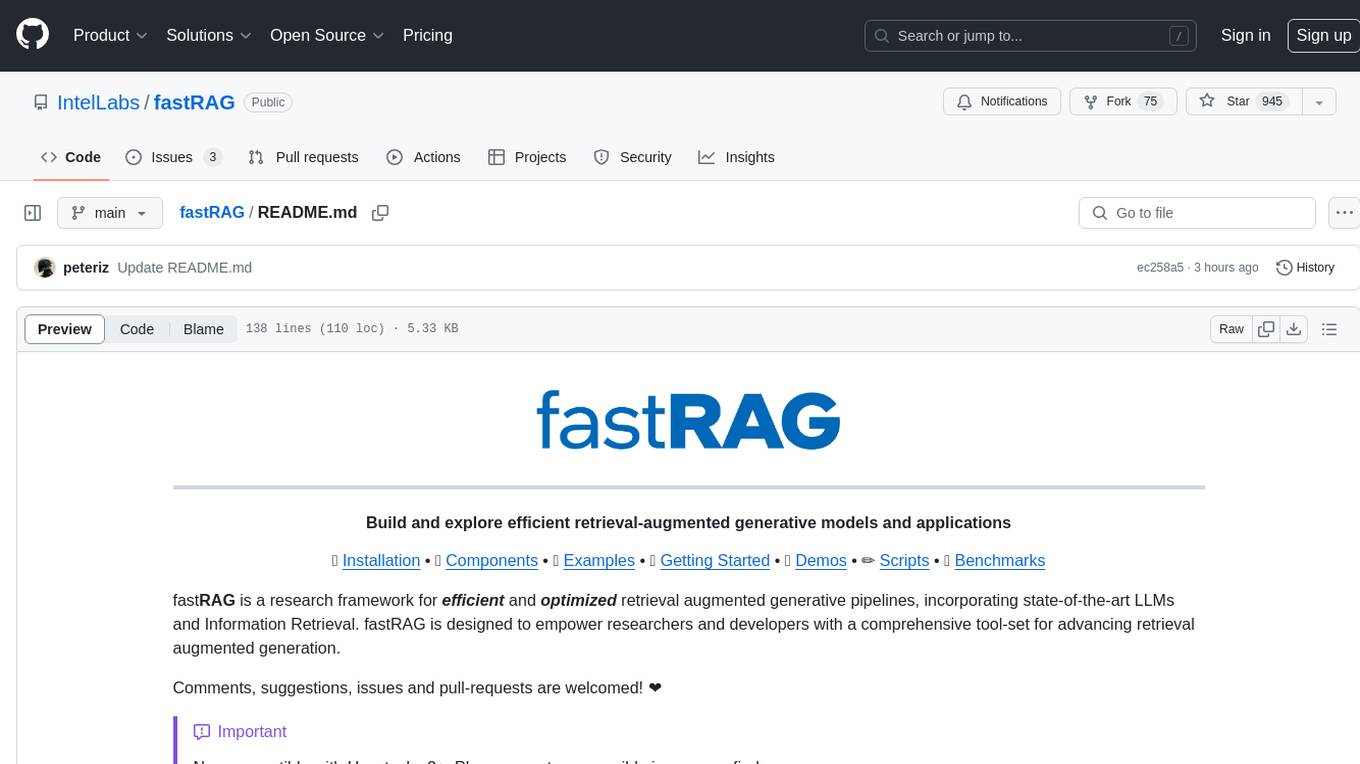
fastRAG
fastRAG is a research framework designed to build and explore efficient retrieval-augmented generative models. It incorporates state-of-the-art Large Language Models (LLMs) and Information Retrieval to empower researchers and developers with a comprehensive tool-set for advancing retrieval augmented generation. The framework is optimized for Intel hardware, customizable, and includes key features such as optimized RAG pipelines, efficient components, and RAG-efficient components like ColBERT and Fusion-in-Decoder (FiD). fastRAG supports various unique components and backends for running LLMs, making it a versatile tool for research and development in the field of retrieval-augmented generation.
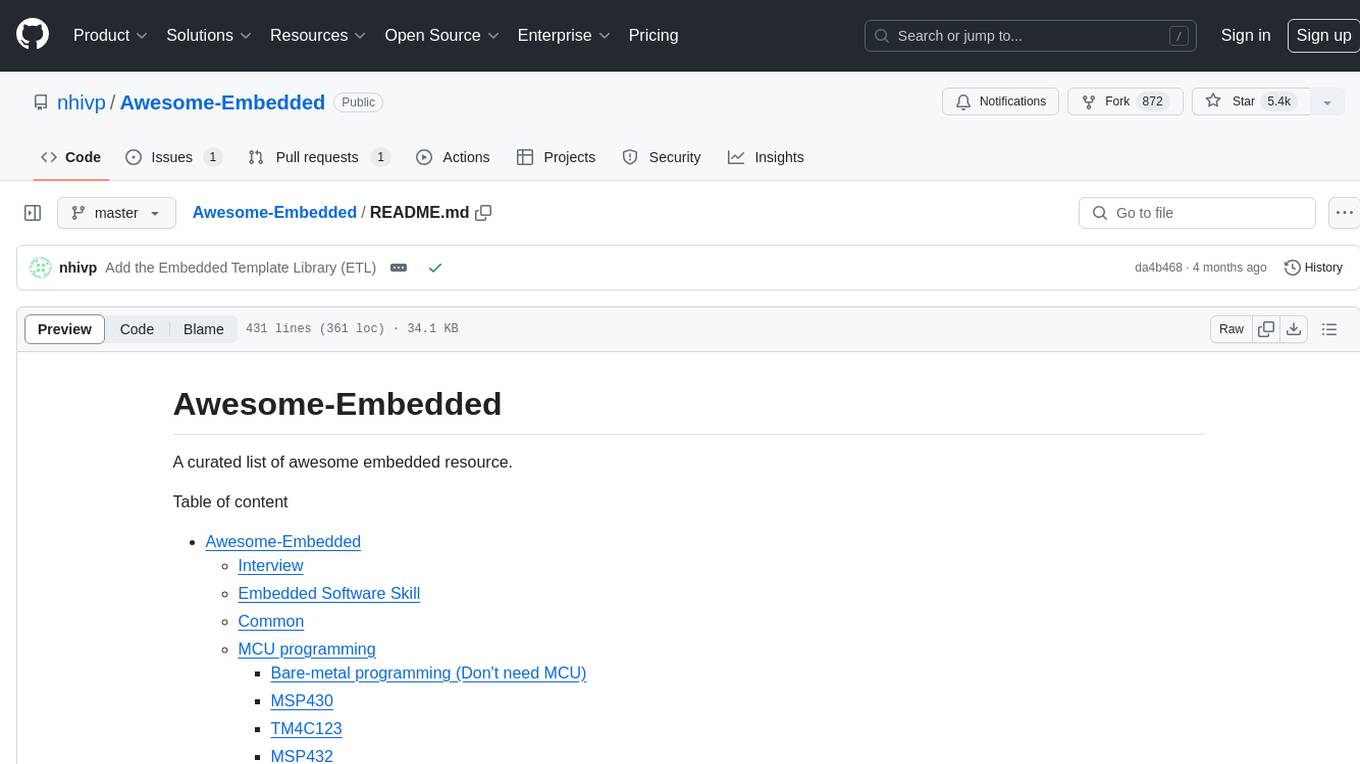
Awesome-Embedded
Awesome-Embedded is a curated list of resources for embedded systems enthusiasts. It covers a wide range of topics including MCU programming, RTOS, Linux kernel development, assembly programming, machine learning & AI on MCU, utilities, tips & tricks, and more. The repository provides valuable information, tutorials, and tools for individuals interested in embedded systems development.
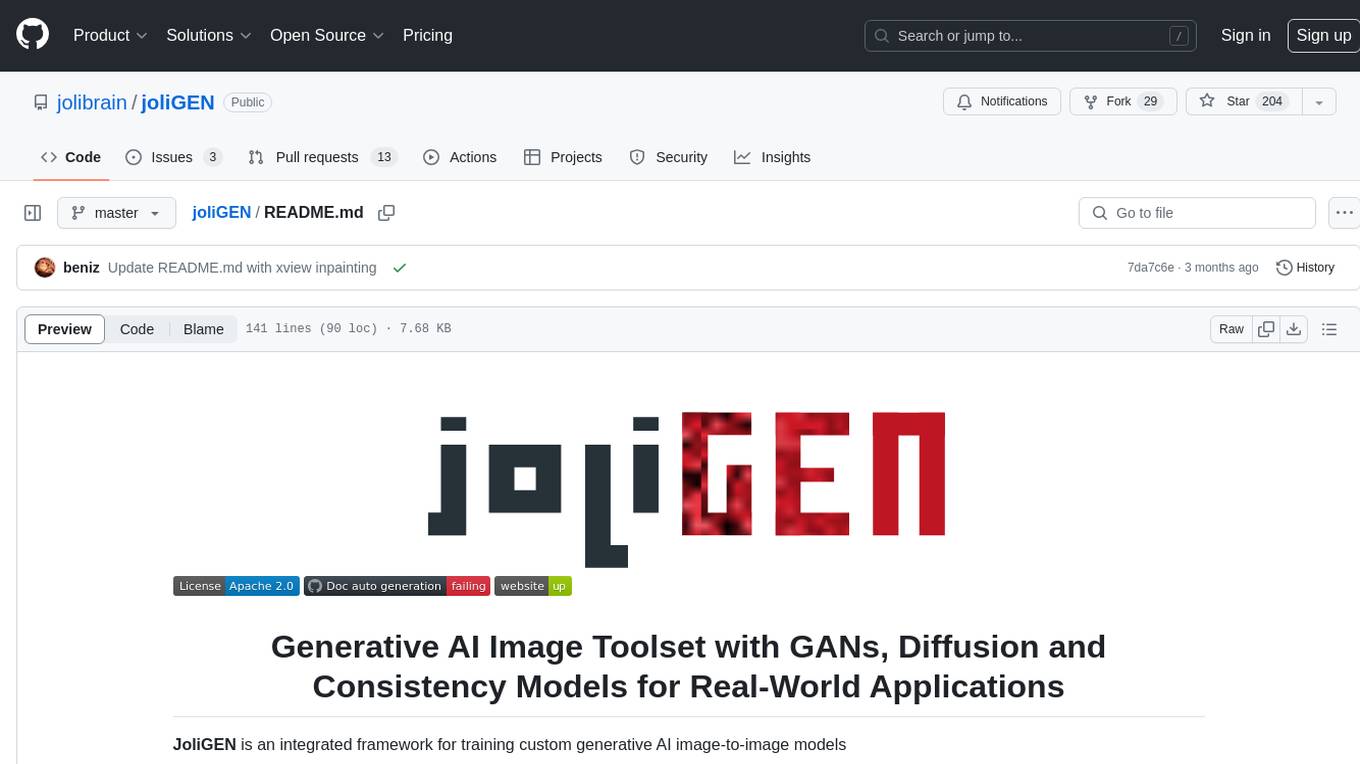
joliGEN
JoliGEN is an integrated framework for training custom generative AI image-to-image models. It implements GAN, Diffusion, and Consistency models for various image translation tasks, including domain and style adaptation with conservation of semantics. The tool is designed for real-world applications such as Controlled Image Generation, Augmented Reality, Dataset Smart Augmentation, and Synthetic to Real transforms. JoliGEN allows for fast and stable training with a REST API server for simplified deployment. It offers a wide range of options and parameters with detailed documentation available for models, dataset formats, and data augmentation.
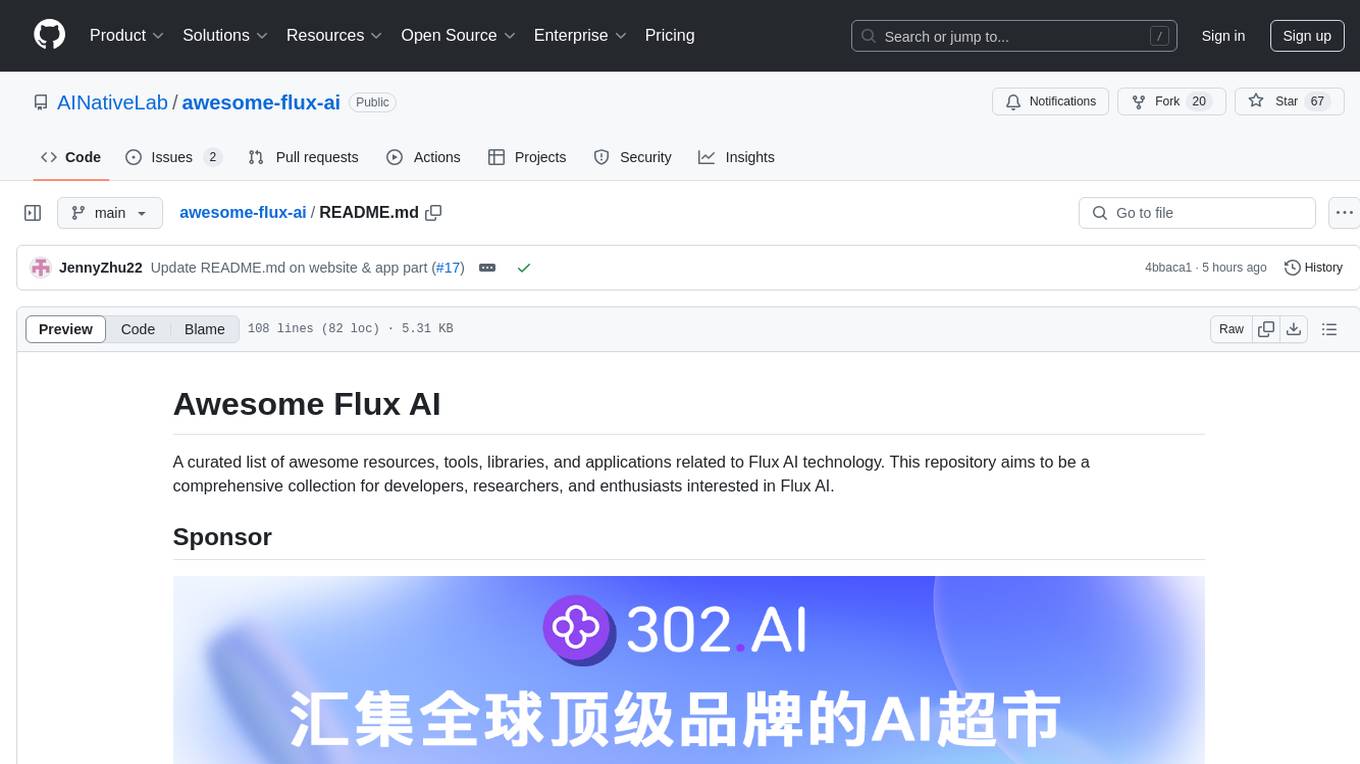
awesome-flux-ai
Awesome Flux AI is a curated list of resources, tools, libraries, and applications related to Flux AI technology. It serves as a comprehensive collection for developers, researchers, and enthusiasts interested in Flux AI. The platform offers open-source text-to-image AI models developed by Black Forest Labs, aiming to advance generative deep learning models for media, creativity, efficiency, and diversity.
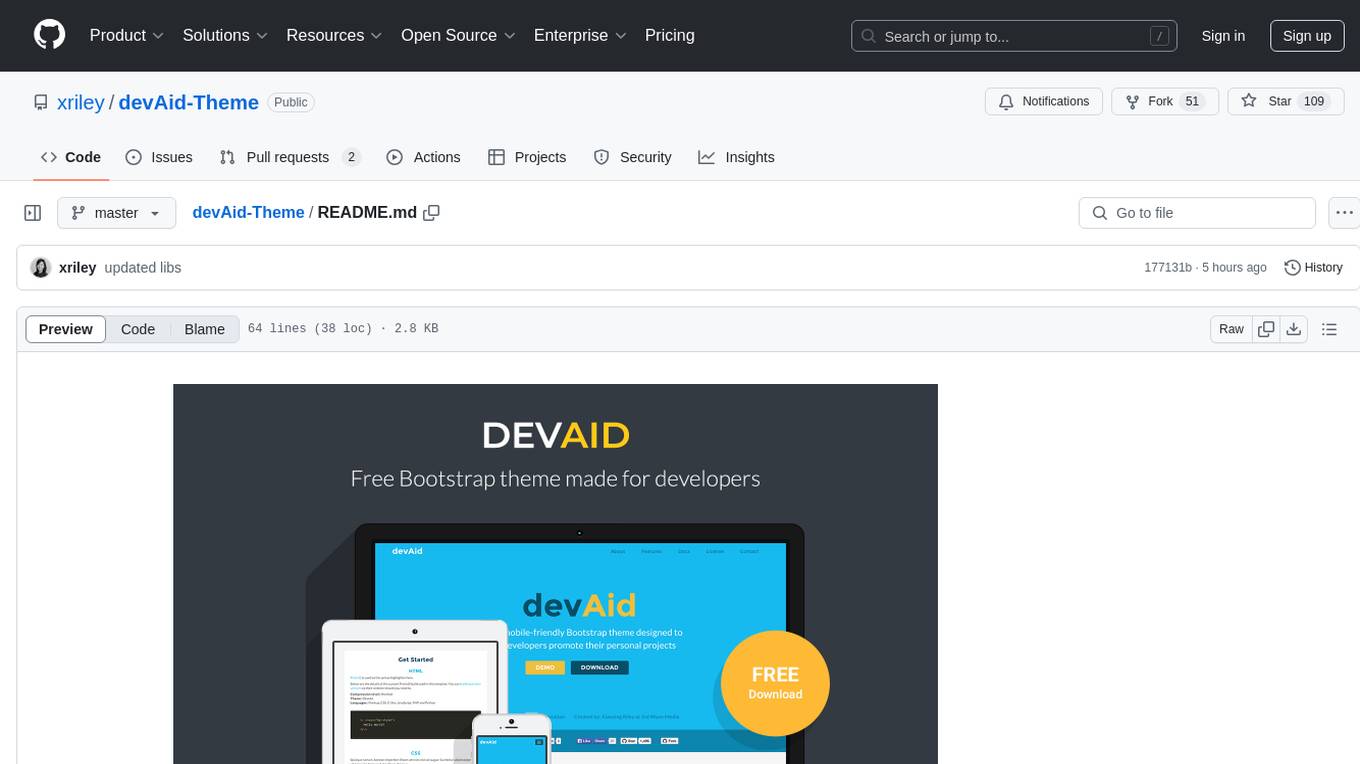
devAid-Theme
devAid-Theme is a free Bootstrap theme designed to help developers promote their personal projects. It comes with 4 colour schemes and includes source SCSS files for easy styling customizations. The theme is fully responsive, built on Bootstrap 5, and includes FontAwesome icons. Author Xiaoying Riley offers the template for free with the requirement to keep the footer attribution link. Commercial licenses are available for those who wish to remove the attribution link. The theme is suitable for developers looking to showcase their side projects with a professional and modern design.
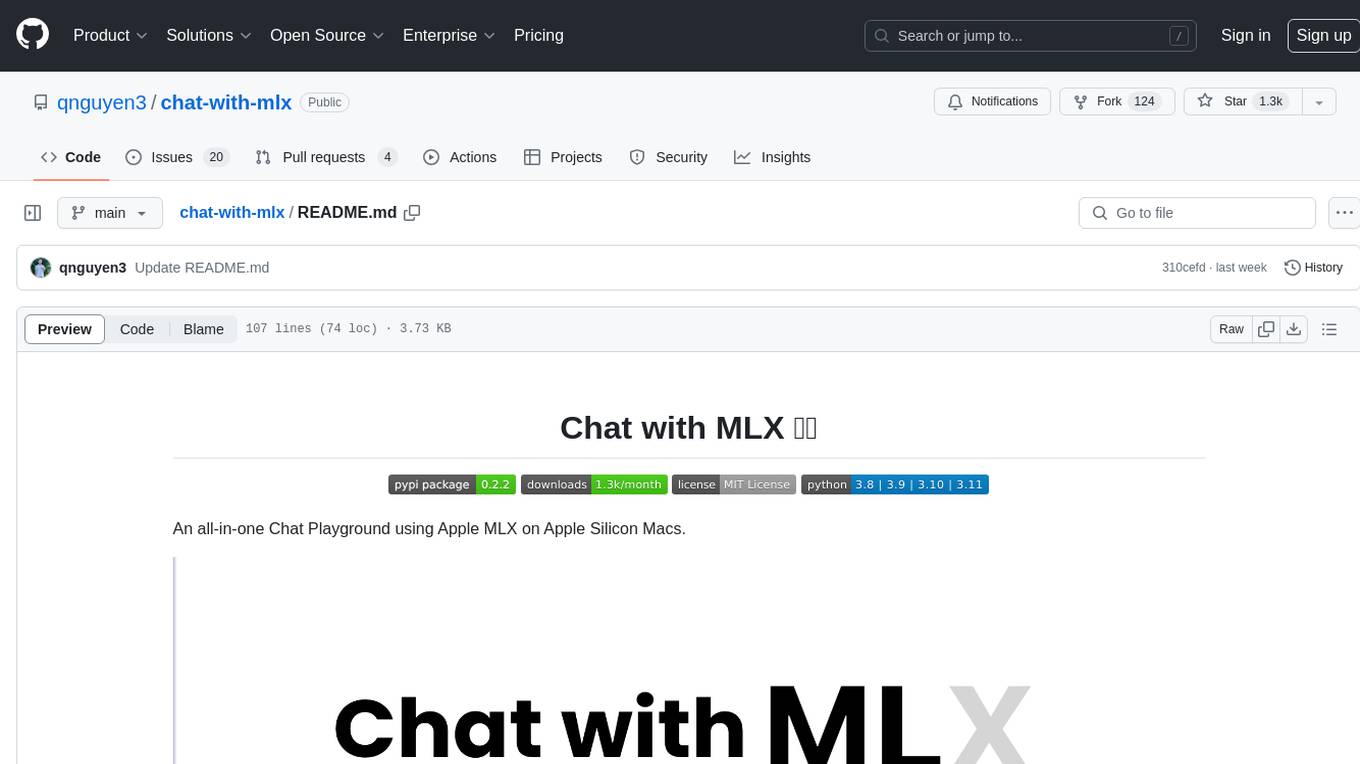
chat-with-mlx
Chat with MLX is an all-in-one Chat Playground using Apple MLX on Apple Silicon Macs. It provides privacy-enhanced AI for secure conversations with various models, easy integration of HuggingFace and MLX Compatible Open-Source Models, and comes with default models like Llama-3, Phi-3, Yi, Qwen, Mistral, Codestral, Mixtral, StableLM. The tool is designed for developers and researchers working with machine learning models on Apple Silicon.