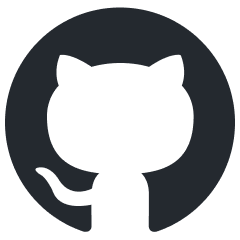
iLLM-TSC
This repository contains the code for the paper“iLLM-TSC: Integration reinforcement learning and large language model for traffic signal control policy improvement”
Stars: 54
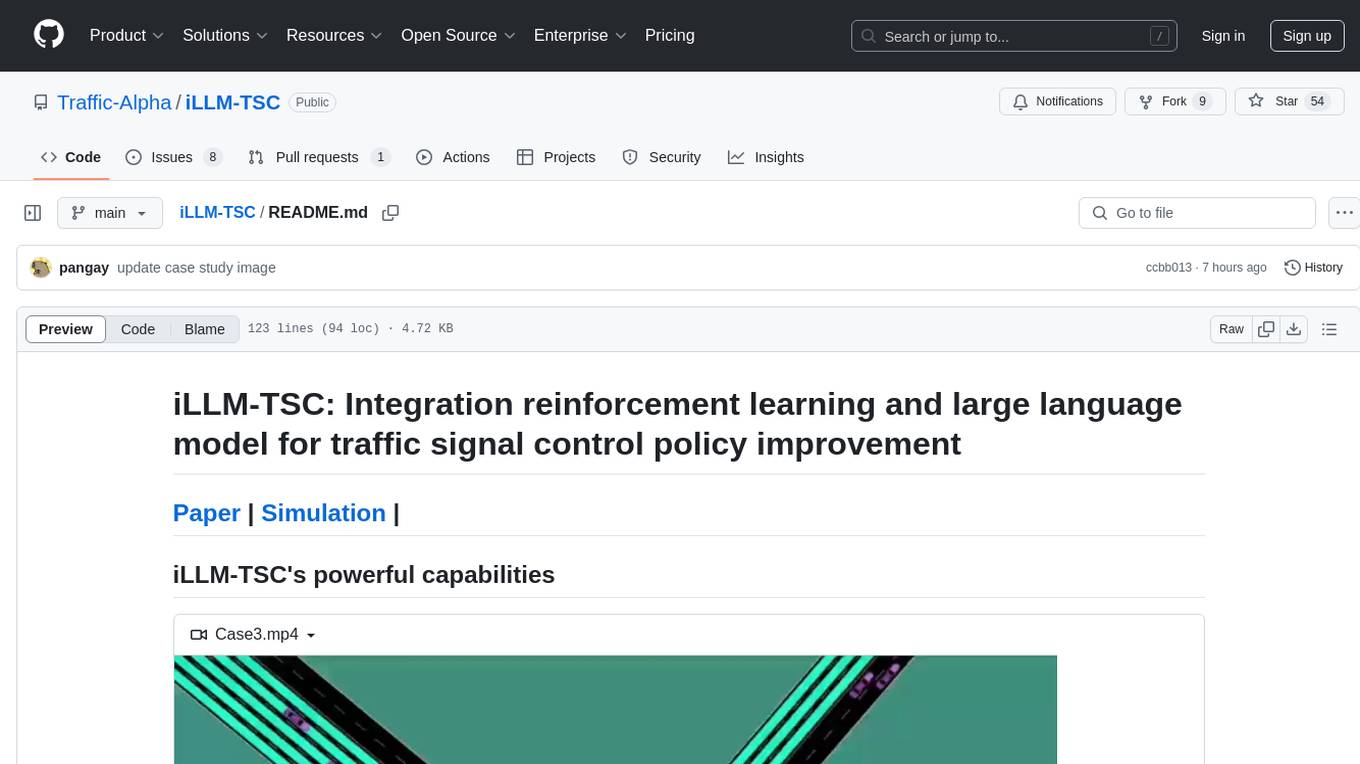
README:
iLLM-TSC: Integration reinforcement learning and large language model for traffic signal control policy improvement
Paper | Simulation |
https://github.com/Traffic-Alpha/TSC-HARLA/assets/75999557/92d6ff7f-cc5b-42ba-9feb-046022e70ad9
We propose a framework that utilizes LLM to support RL models. This framework refines RL decisions based on real-world contexts and provides reasonable actions when RL agents make erroneous decisions.
- Case1: LLM think that the action taken by the RL Agent was unreasonable and gave a reasonable explanation and recommended actions.
- Case 2: LLM considers that the movement made by the RL Agent is not the movement with the highest current mean occupancy but it is reasonable, after which LLM gives an explanation and recommendation.
- Case 3: An ambulance needs to pass through the intersection, but the RL Agent does not take into account that the ambulance needs to be prioritized. LLM modifies the RL Agent’s action to prioritize the ambulance to pass through the intersection.
Install TransSimHub
The simulation environment we used is TransSimHub, which is based on SUMO and can be used for TSC, V2X and UAM simulation. More information is available via docs.
You can install TransSimHub by cloning the GitHub repository. Follow these steps:
git clone https://github.com/Traffic-Alpha/TransSimHub.git
cd TransSimHub
pip install -e .
After the installation is complete, you can use the following Python command to check if TransSimHub is installed and view its version:
import tshub
print(tshub.__version__)
You can install HARLA by cloning the GitHub repository. Follow these steps:
git clone https://github.com/Traffic-Alpha/iLLM-TSC
cd iLLM-TSC
pip install -r requirements.txt
After completing the above Install steps
, you can use this program locally.
The first thing you need to do is train a RL model. You can do it with the following code:
cd iLLM-TSC
python sb3_ppo.py
The training results are shown in the figure, and model weight has been uploaded in models.
The effect of the RL model can be tested with the following code:
python eval_rl_agent.py
Before you can use LLM, you need to have your own KEY and fill it in the utils/config.yaml
.
OPENAI_PROXY:
OPENAI_API_KEY:
The entire framework can be used with the following code.
python rl_llm_tsc.py
Evaluation Rule: To make fair evaluation and comparison among different models, make sure you use the same LLM evaluation model (we use GPT4) for all the models you want to evaluate. Using a different scoring model or API updating might lead to different results.
All assets and code in this repository are under the Apache 2.0 license unless specified otherwise. The language data is under CC BY-NC-SA 4.0. Other datasets (including nuScenes) inherit their own distribution licenses. Please consider citing our project if it helps your research.
@article{pang2024illm,
title={iLLM-TSC: Integration reinforcement learning and large language model for traffic signal control policy improvement},
author={Pang, Aoyu and Wang, Maonan and Pun, Man-On and Chen, Chung Shue and Xiong, Xi},
journal={arXiv preprint arXiv:2407.06025},
year={2024}
}
@article{wang2024llm,
title={LLM-Assisted Light: Leveraging Large Language Model Capabilities for Human-Mimetic Traffic Signal Control in Complex Urban Environments},
author={Wang, Maonan and Pang, Aoyu and Kan, Yuheng and Pun, Man-On and Chen, Chung Shue and Huang, Bo},
journal={arXiv preprint arXiv:2403.08337},
year={2024}
}
iLLM-TSC just explores the combination of RL and LLM, more work will be updated in TSC-LLM, welcome to star!
- Yufei Teng: Thanks for editing the video.
- Thank you to everyone who pays attention to our work. Hope our work can help you.
For Tasks:
Click tags to check more tools for each tasksFor Jobs:
Alternative AI tools for iLLM-TSC
Similar Open Source Tools
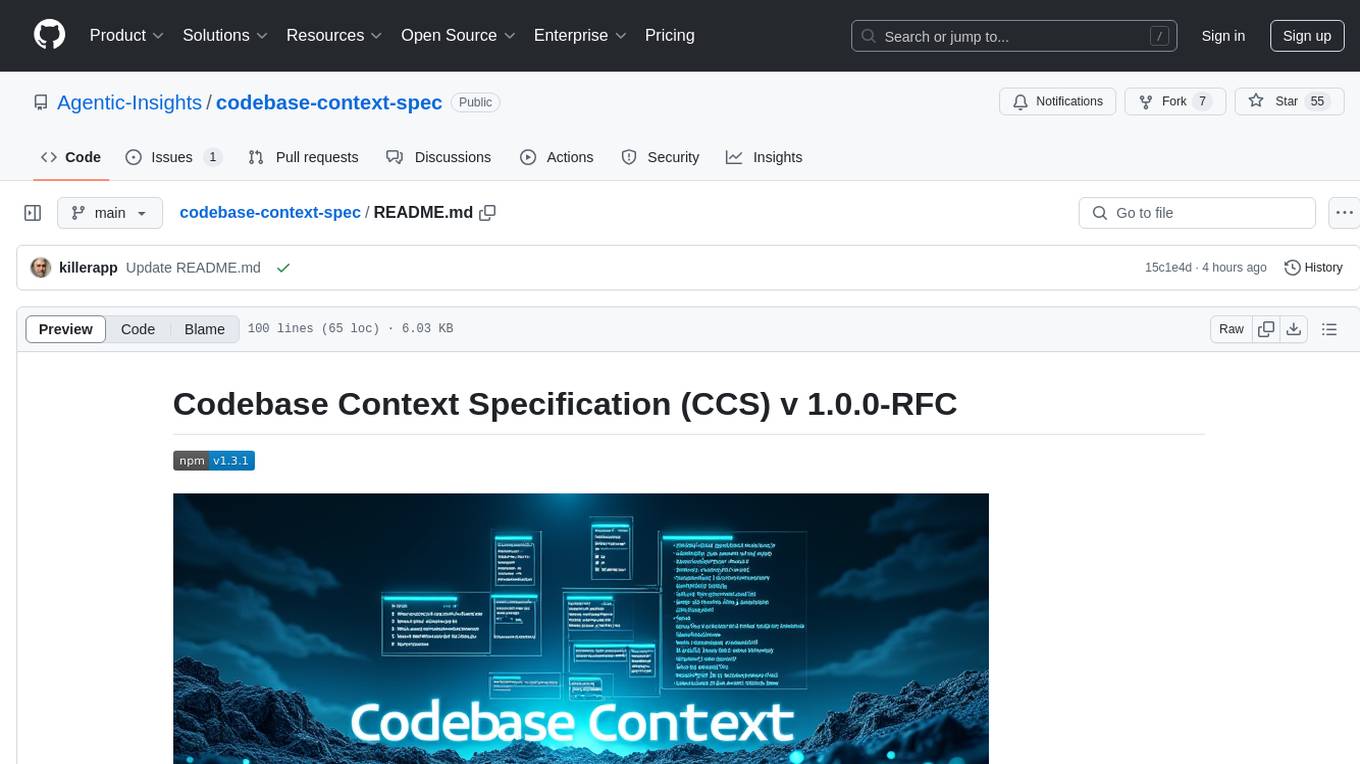
codebase-context-spec
The Codebase Context Specification (CCS) project aims to standardize embedding contextual information within codebases to enhance understanding for both AI and human developers. It introduces a convention similar to `.env` and `.editorconfig` files but focused on documenting code for both AI and humans. By providing structured contextual metadata, collaborative documentation guidelines, and standardized context files, developers can improve code comprehension, collaboration, and development efficiency. The project includes a linter for validating context files and provides guidelines for using the specification with AI assistants. Tooling recommendations suggest creating memory systems, IDE plugins, AI model integrations, and agents for context creation and utilization. Future directions include integration with existing documentation systems, dynamic context generation, and support for explicit context overriding.
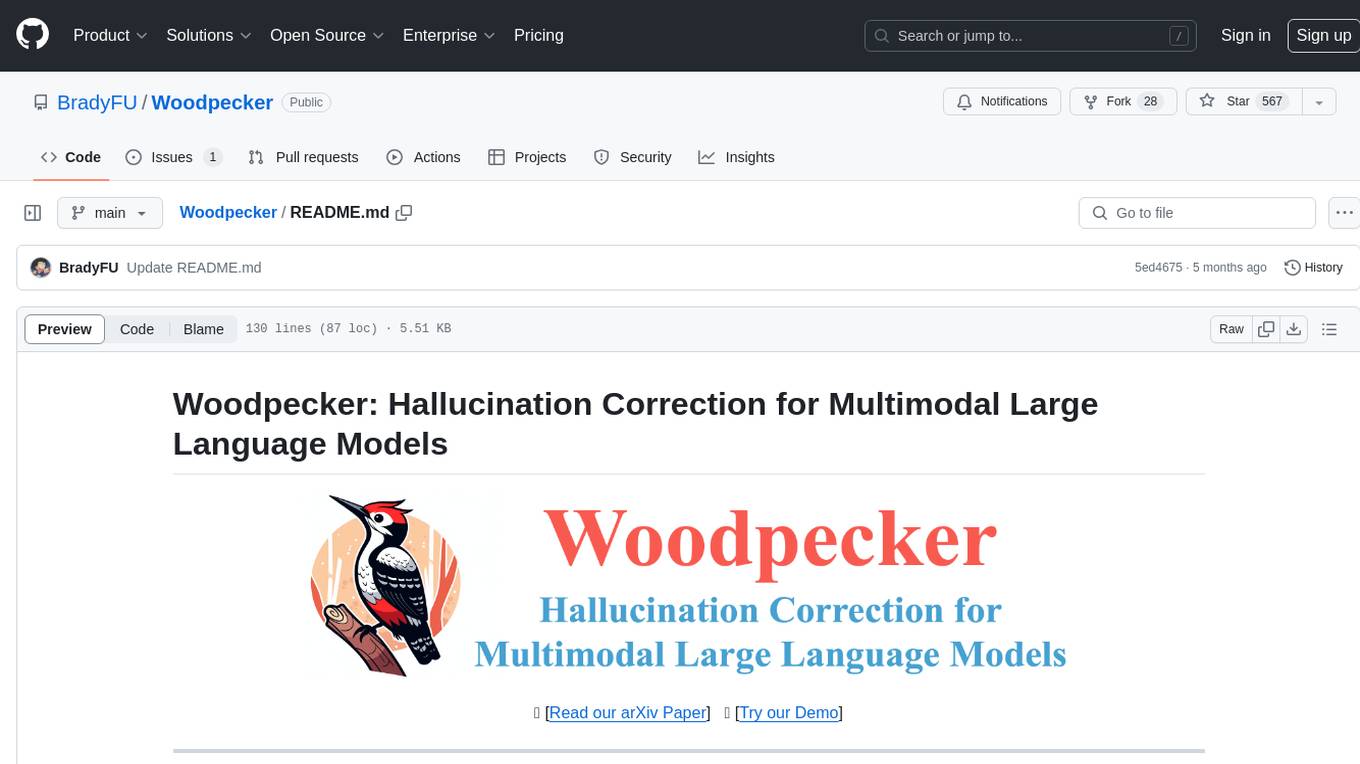
Woodpecker
Woodpecker is a tool designed to correct hallucinations in Multimodal Large Language Models (MLLMs) by introducing a training-free method that picks out and corrects inconsistencies between generated text and image content. It consists of five stages: key concept extraction, question formulation, visual knowledge validation, visual claim generation, and hallucination correction. Woodpecker can be easily integrated with different MLLMs and provides interpretable results by accessing intermediate outputs of the stages. The tool has shown significant improvements in accuracy over baseline models like MiniGPT-4 and mPLUG-Owl.
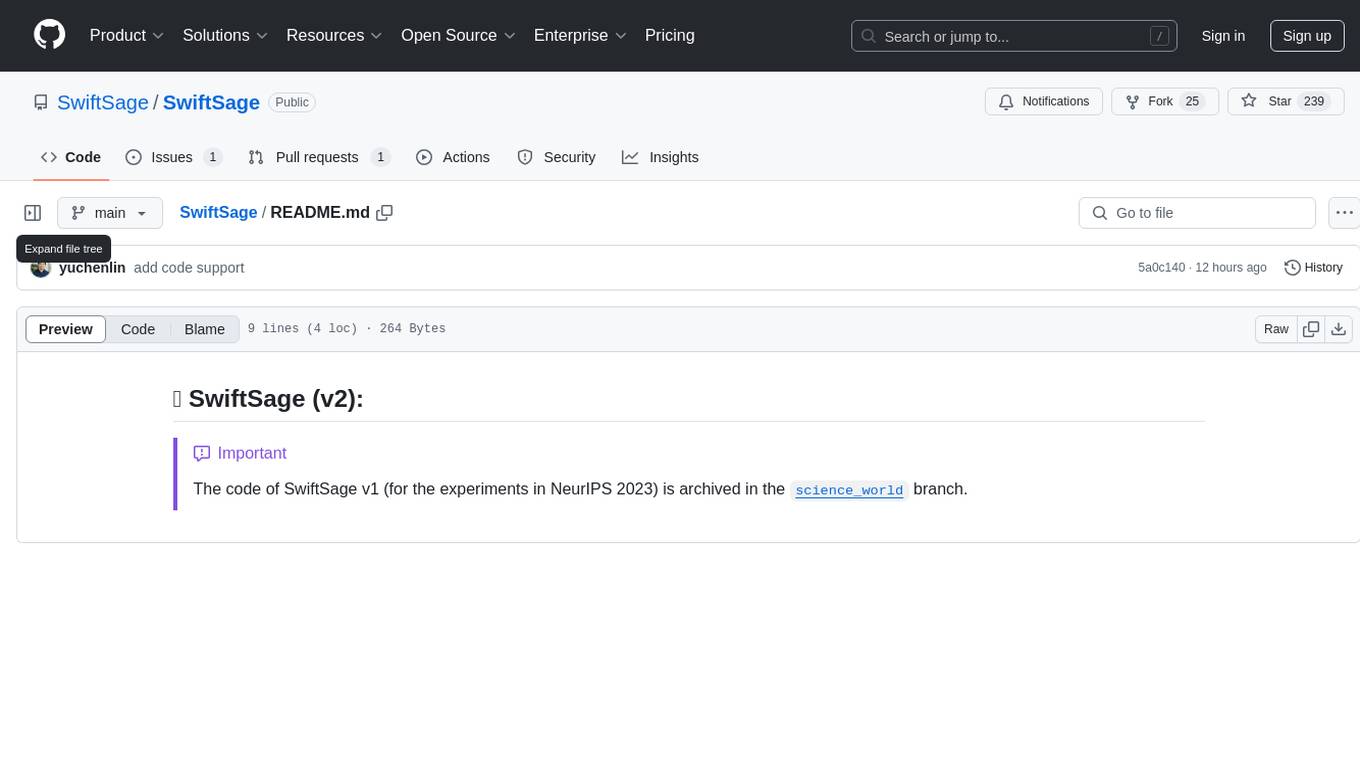
SwiftSage
SwiftSage is a tool designed for conducting experiments in the field of machine learning and artificial intelligence. It provides a platform for researchers and developers to implement and test various algorithms and models. The tool is particularly useful for exploring new ideas and conducting experiments in a controlled environment. SwiftSage aims to streamline the process of developing and testing machine learning models, making it easier for users to iterate on their ideas and achieve better results. With its user-friendly interface and powerful features, SwiftSage is a valuable tool for anyone working in the field of AI and ML.
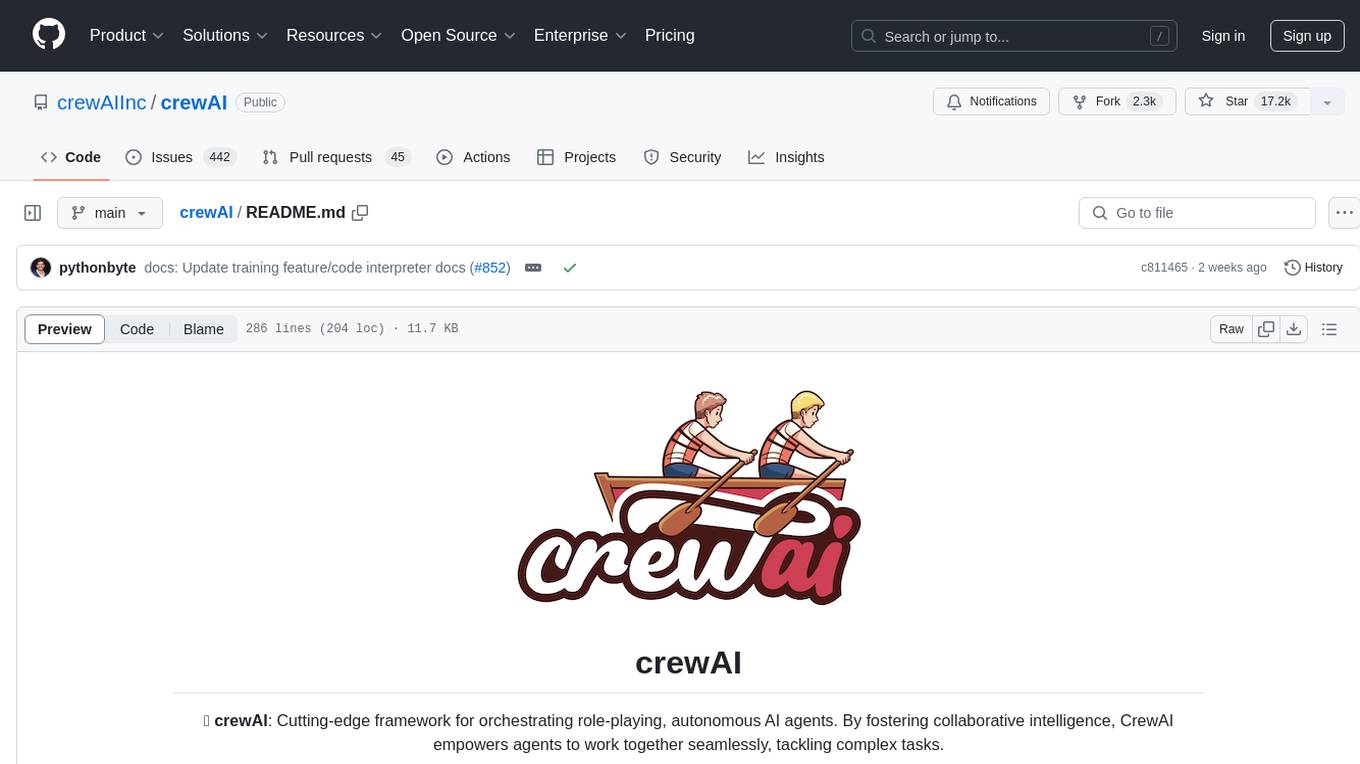
crewAI
CrewAI is a cutting-edge framework designed to orchestrate role-playing autonomous AI agents. By fostering collaborative intelligence, CrewAI empowers agents to work together seamlessly, tackling complex tasks. It enables AI agents to assume roles, share goals, and operate in a cohesive unit, much like a well-oiled crew. Whether you're building a smart assistant platform, an automated customer service ensemble, or a multi-agent research team, CrewAI provides the backbone for sophisticated multi-agent interactions. With features like role-based agent design, autonomous inter-agent delegation, flexible task management, and support for various LLMs, CrewAI offers a dynamic and adaptable solution for both development and production workflows.
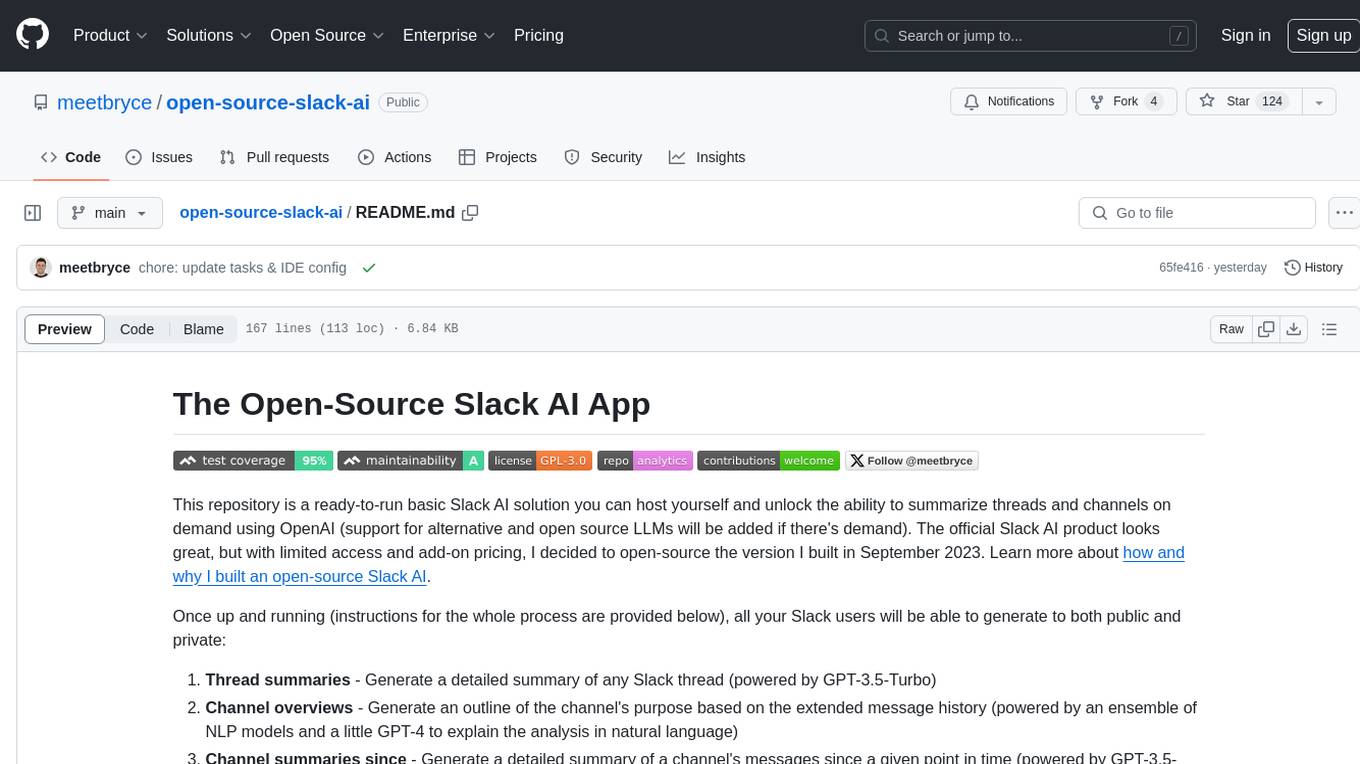
open-source-slack-ai
This repository provides a ready-to-run basic Slack AI solution that allows users to summarize threads and channels using OpenAI. Users can generate thread summaries, channel overviews, channel summaries since a specific time, and full channel summaries. The tool is powered by GPT-3.5-Turbo and an ensemble of NLP models. It requires Python 3.8 or higher, an OpenAI API key, Slack App with associated API tokens, Poetry package manager, and ngrok for local development. Users can customize channel and thread summaries, run tests with coverage using pytest, and contribute to the project for future enhancements.
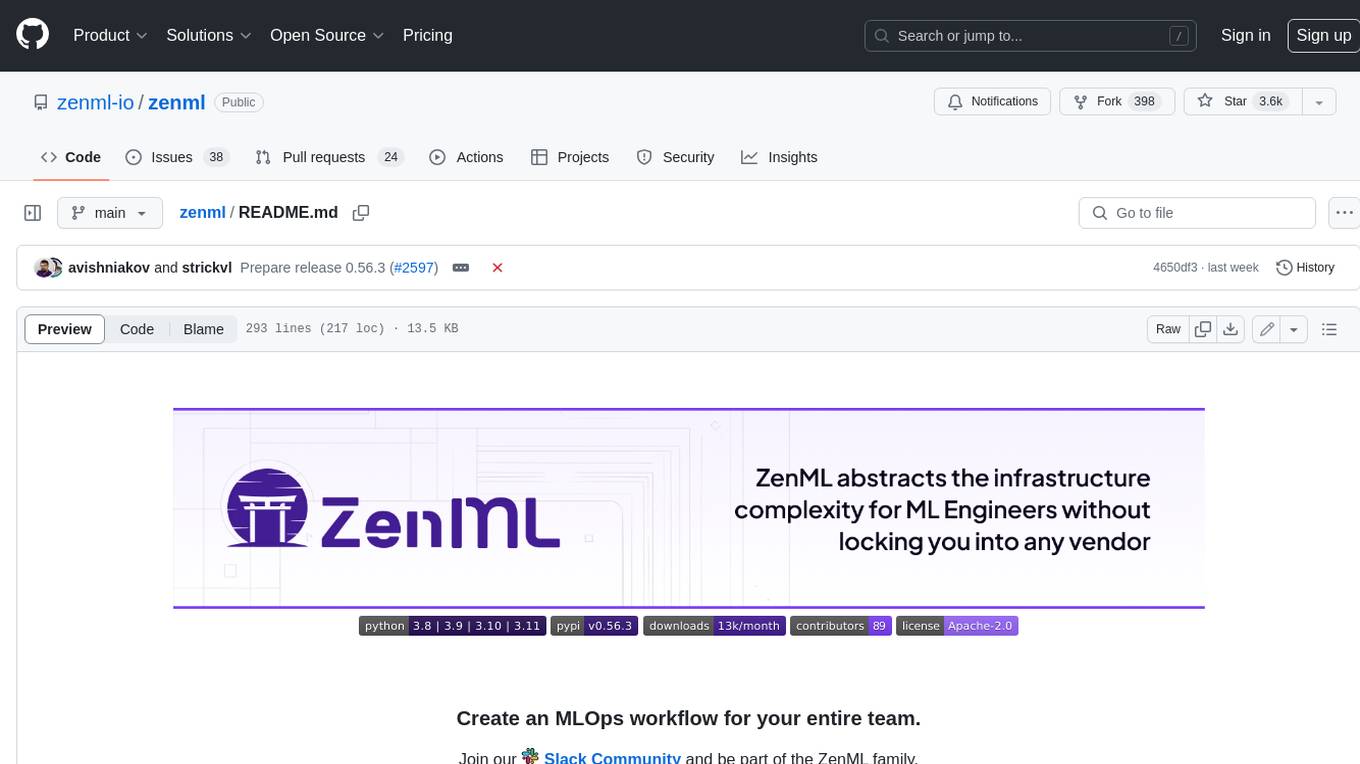
zenml
ZenML is an extensible, open-source MLOps framework for creating portable, production-ready machine learning pipelines. By decoupling infrastructure from code, ZenML enables developers across your organization to collaborate more effectively as they develop to production.
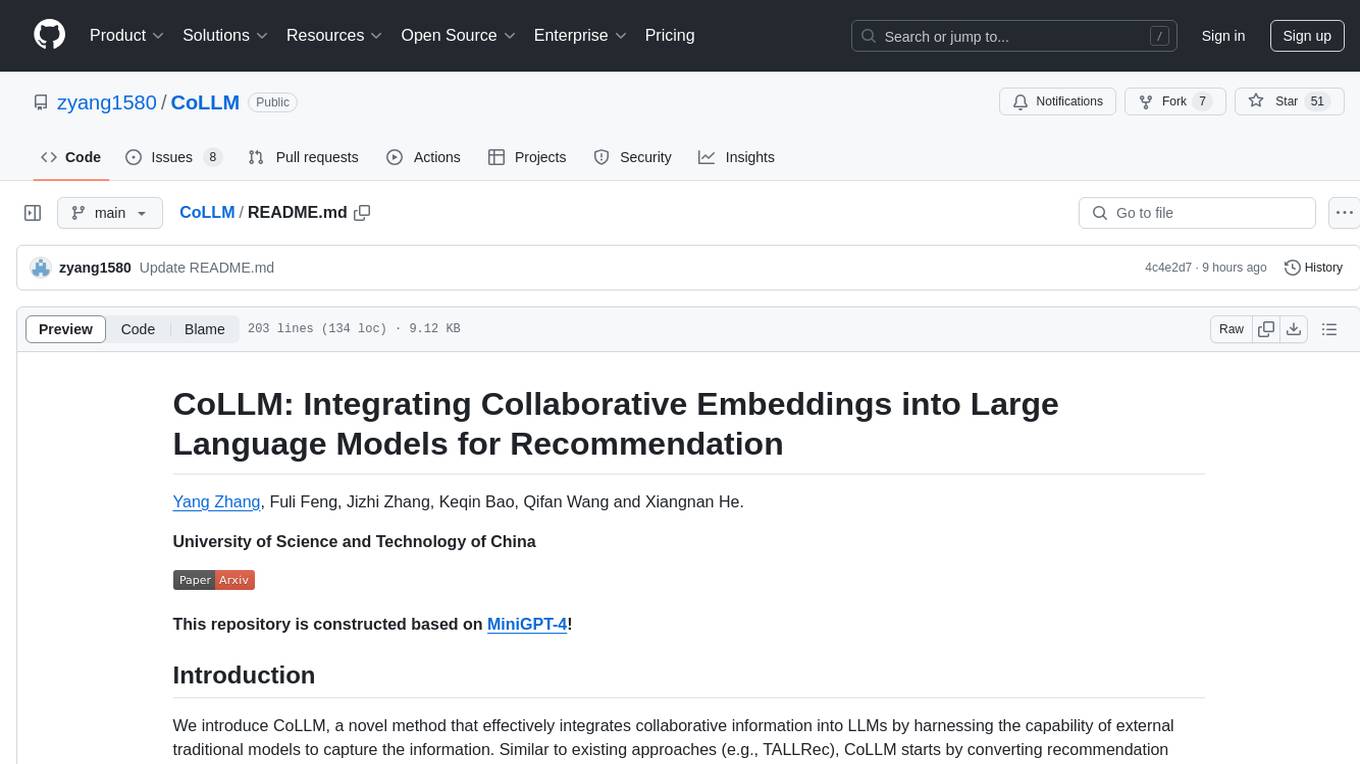
CoLLM
CoLLM is a novel method that integrates collaborative information into Large Language Models (LLMs) for recommendation. It converts recommendation data into language prompts, encodes them with both textual and collaborative information, and uses a two-step tuning method to train the model. The method incorporates user/item ID fields in prompts and employs a conventional collaborative model to generate user/item representations. CoLLM is built upon MiniGPT-4 and utilizes pretrained Vicuna weights for training.
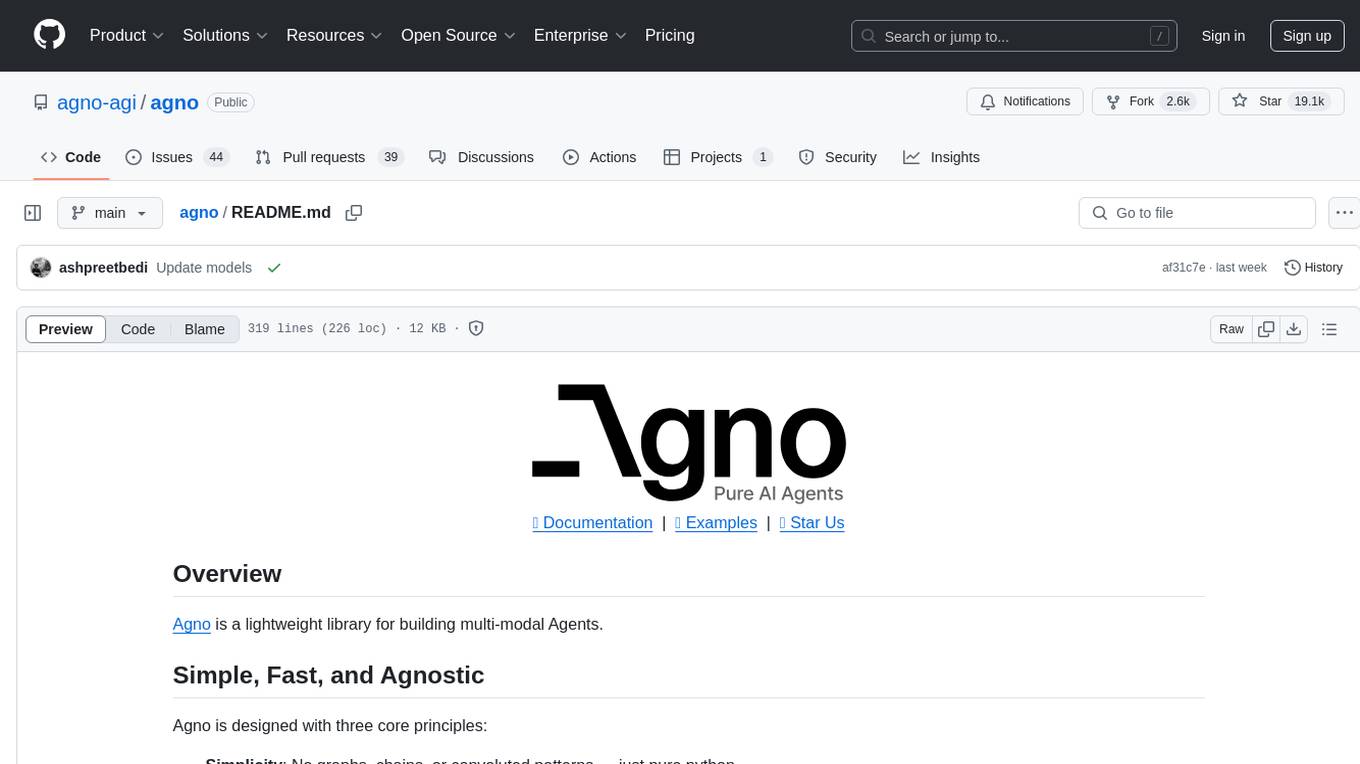
agno
Agno is a lightweight library for building multi-modal Agents. It is designed with core principles of simplicity, uncompromising performance, and agnosticism, allowing users to create blazing fast agents with minimal memory footprint. Agno supports any model, any provider, and any modality, making it a versatile container for AGI. Users can build agents with lightning-fast agent creation, model agnostic capabilities, native support for text, image, audio, and video inputs and outputs, memory management, knowledge stores, structured outputs, and real-time monitoring. The library enables users to create autonomous programs that use language models to solve problems, improve responses, and achieve tasks with varying levels of agency and autonomy.
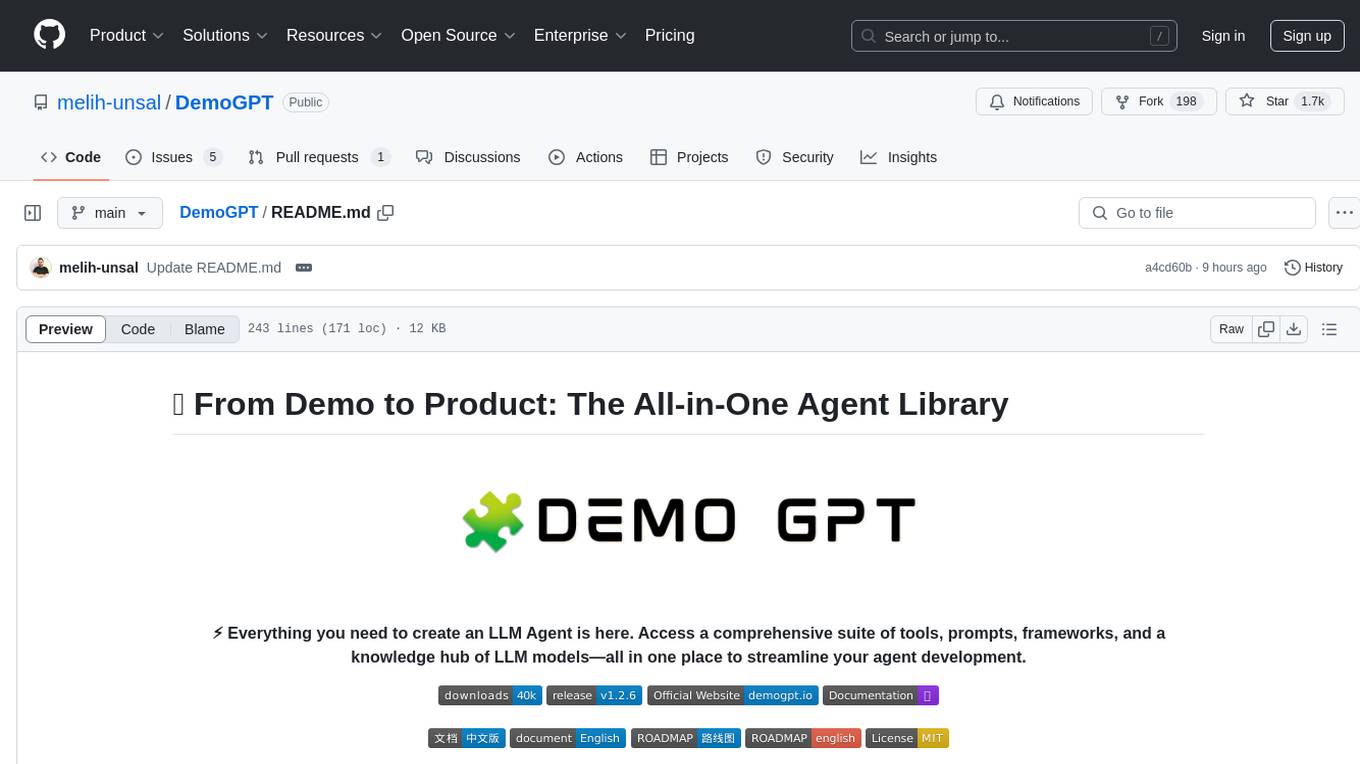
DemoGPT
DemoGPT is an all-in-one agent library that provides tools, prompts, frameworks, and LLM models for streamlined agent development. It leverages GPT-3.5-turbo to generate LangChain code, creating interactive Streamlit applications. The tool is designed for creating intelligent, interactive, and inclusive solutions in LLM-based application development. It offers model flexibility, iterative development, and a commitment to user engagement. Future enhancements include integrating Gorilla for autonomous API usage and adding a publicly available database for refining the generation process.
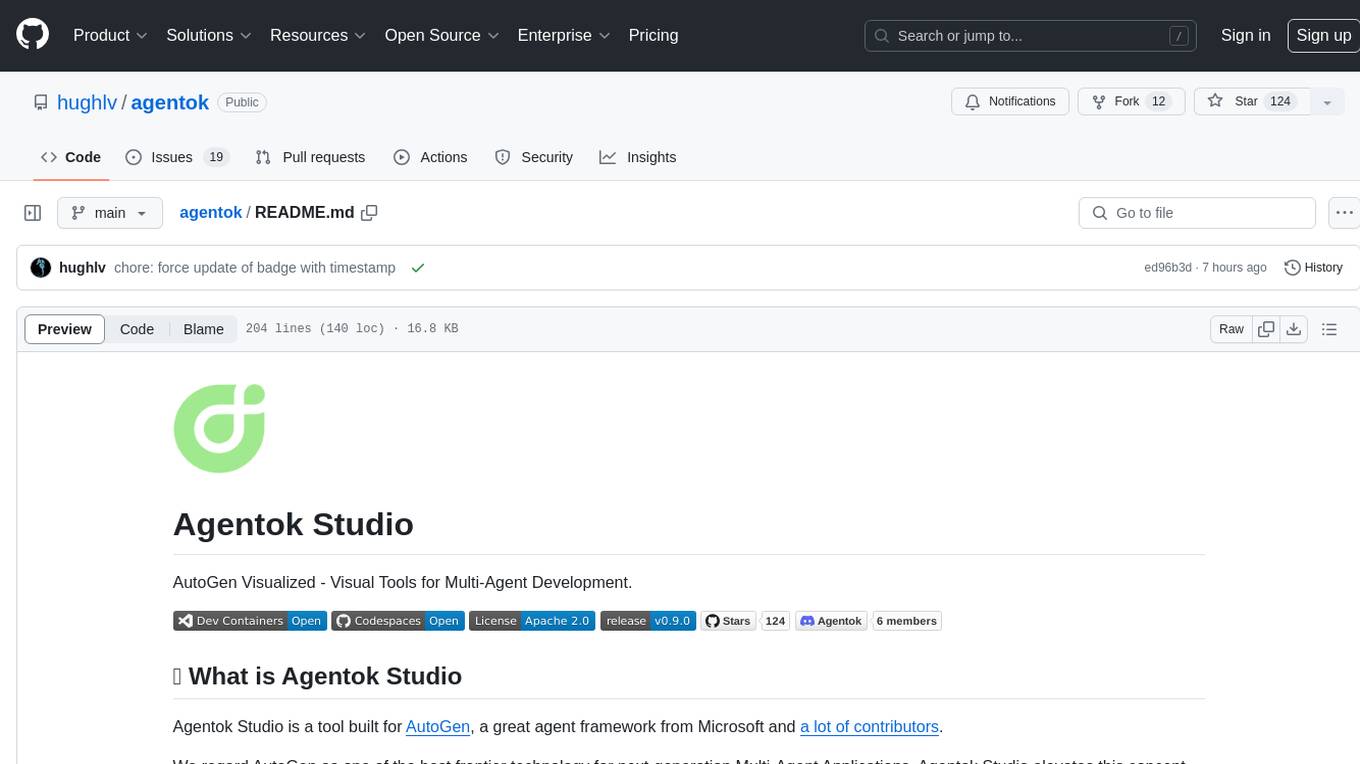
agentok
Agentok Studio is a visual tool built for AutoGen, a cutting-edge agent framework from Microsoft and various contributors. It offers intuitive visual tools to simplify the construction and management of complex agent-based workflows. Users can create workflows visually as graphs, chat with agents, and share flow templates. The tool is designed to streamline the development process for creators and developers working on next-generation Multi-Agent Applications.
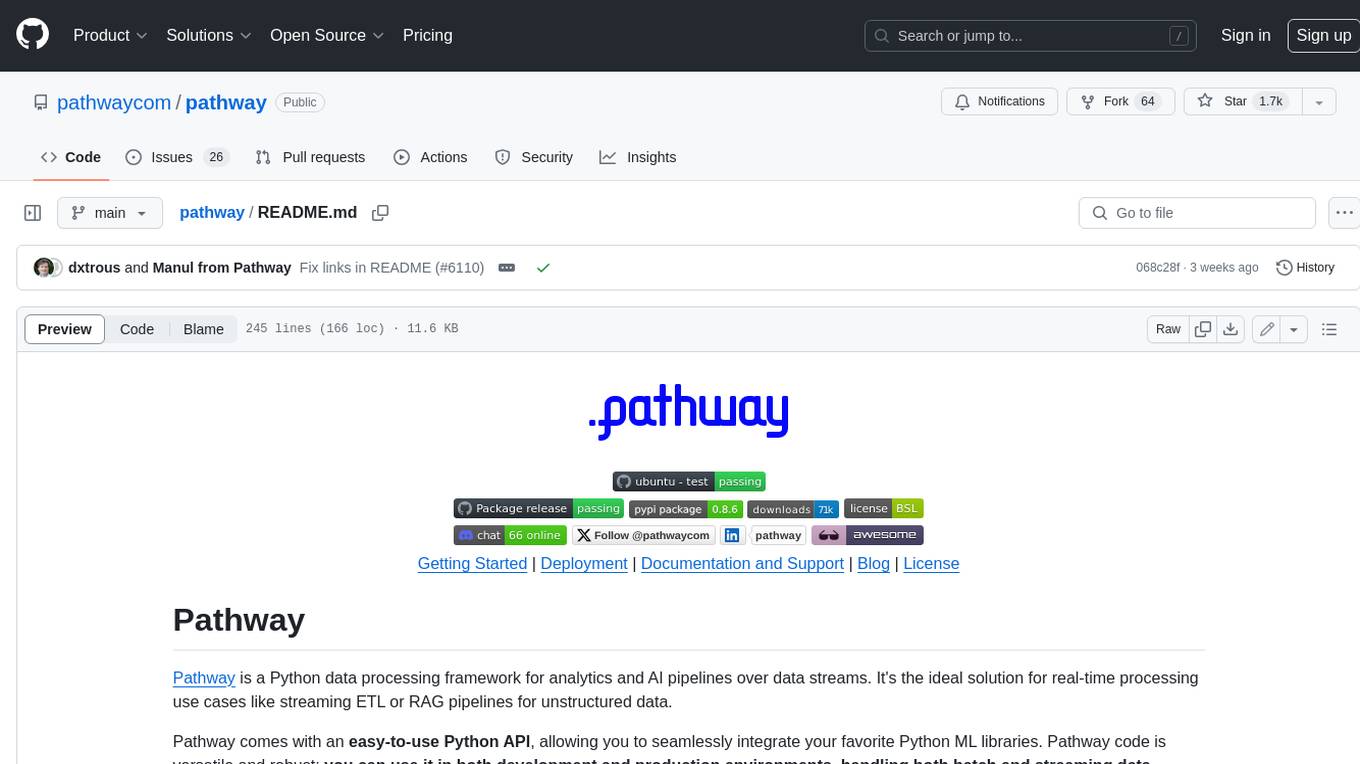
pathway
Pathway is a Python data processing framework for analytics and AI pipelines over data streams. It's the ideal solution for real-time processing use cases like streaming ETL or RAG pipelines for unstructured data. Pathway comes with an **easy-to-use Python API** , allowing you to seamlessly integrate your favorite Python ML libraries. Pathway code is versatile and robust: **you can use it in both development and production environments, handling both batch and streaming data effectively**. The same code can be used for local development, CI/CD tests, running batch jobs, handling stream replays, and processing data streams. Pathway is powered by a **scalable Rust engine** based on Differential Dataflow and performs incremental computation. Your Pathway code, despite being written in Python, is run by the Rust engine, enabling multithreading, multiprocessing, and distributed computations. All the pipeline is kept in memory and can be easily deployed with **Docker and Kubernetes**. You can install Pathway with pip: `pip install -U pathway` For any questions, you will find the community and team behind the project on Discord.
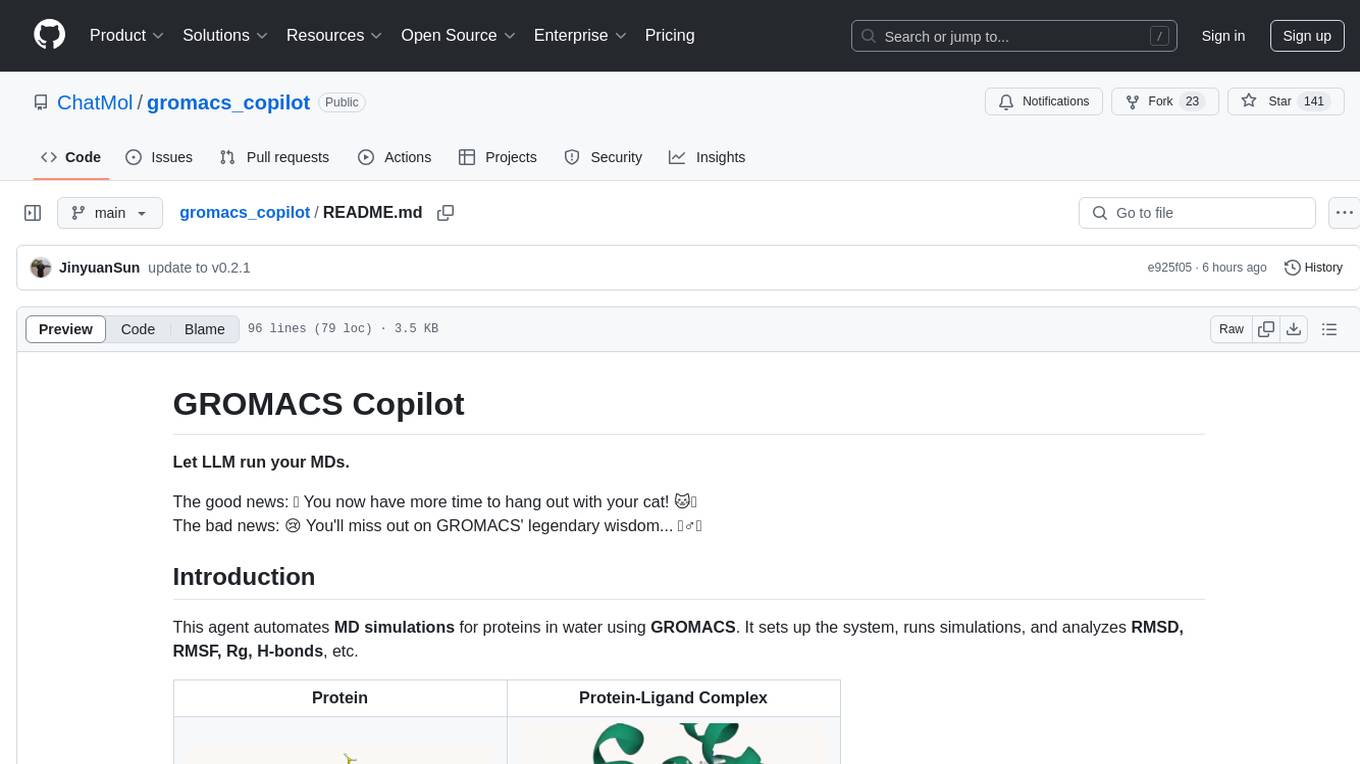
gromacs_copilot
GROMACS Copilot is an agent designed to automate molecular dynamics simulations for proteins in water using GROMACS. It handles system setup, simulation execution, and result analysis automatically, providing outputs such as RMSD, RMSF, Rg, and H-bonds. Users can interact with the agent through prompts and API keys from DeepSeek and OpenAI. The tool aims to simplify the process of running MD simulations, allowing users to focus on other tasks while it handles the technical aspects of the simulations.
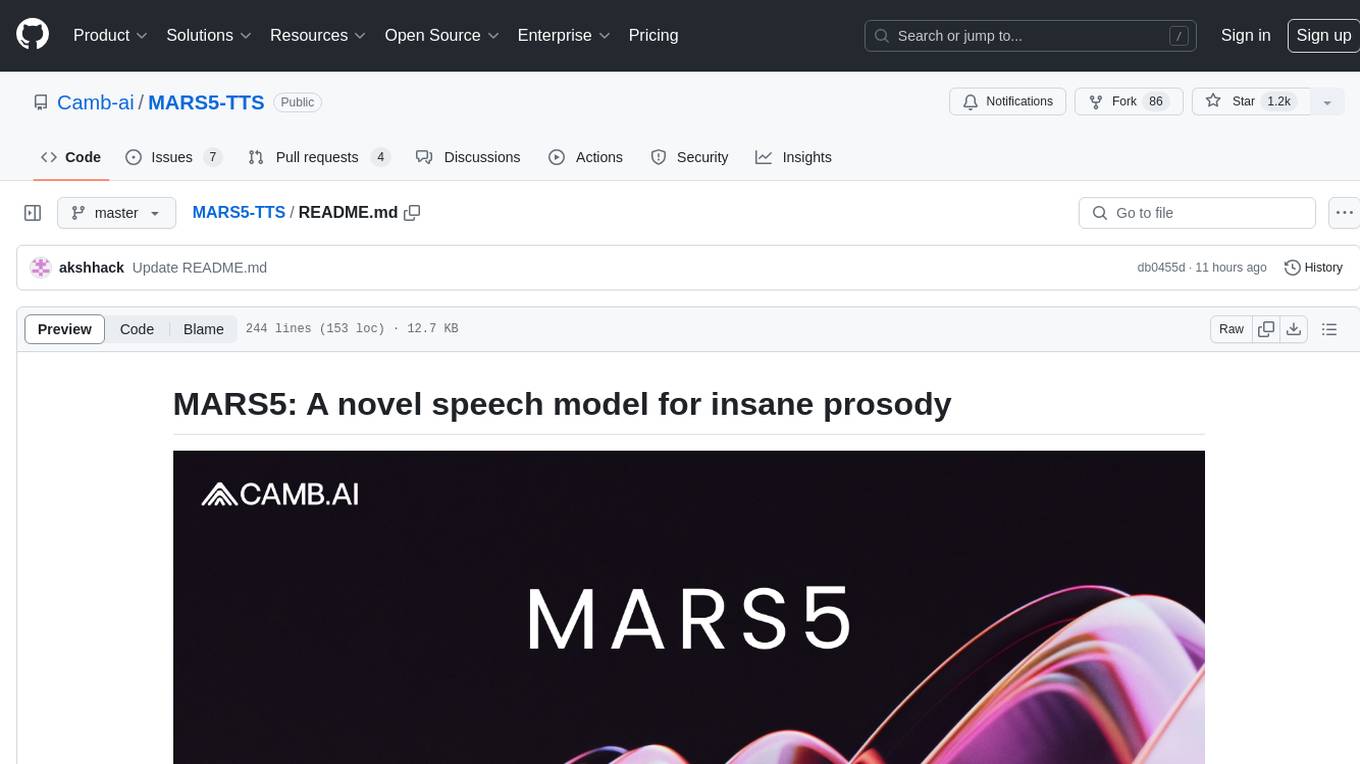
MARS5-TTS
MARS5 is a novel English speech model (TTS) developed by CAMB.AI, featuring a two-stage AR-NAR pipeline with a unique NAR component. The model can generate speech for various scenarios like sports commentary and anime with just 5 seconds of audio and a text snippet. It allows steering prosody using punctuation and capitalization in the transcript. Speaker identity is specified using an audio reference file, enabling 'deep clone' for improved quality. The model can be used via torch.hub or HuggingFace, supporting both shallow and deep cloning for inference. Checkpoints are provided for AR and NAR models, with hardware requirements of 750M+450M params on GPU. Contributions to improve model stability, performance, and reference audio selection are welcome.
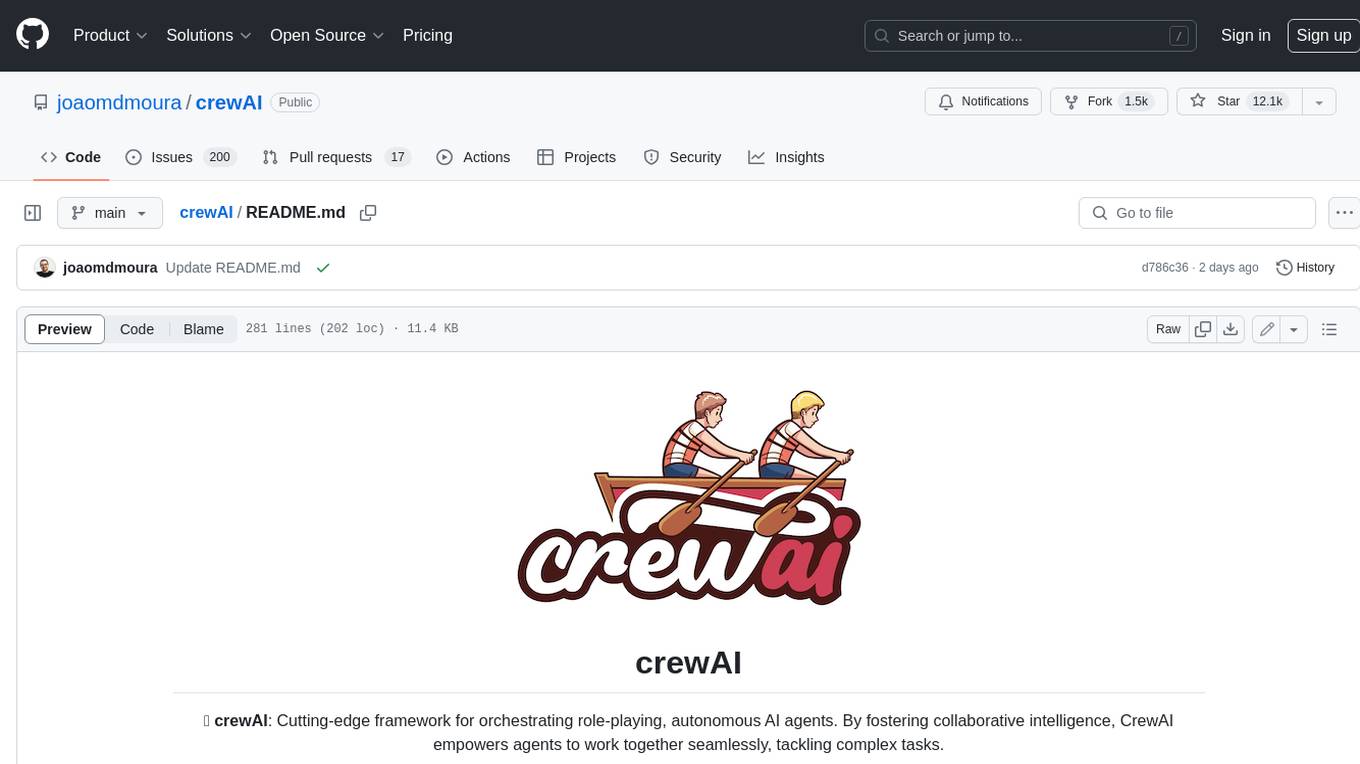
crewAI
crewAI is a cutting-edge framework for orchestrating role-playing, autonomous AI agents. By fostering collaborative intelligence, CrewAI empowers agents to work together seamlessly, tackling complex tasks. It provides a flexible and structured approach to AI collaboration, enabling users to define agents with specific roles, goals, and tools, and assign them tasks within a customizable process. crewAI supports integration with various LLMs, including OpenAI, and offers features such as autonomous task delegation, flexible task management, and output parsing. It is open-source and welcomes contributions, with a focus on improving the library based on usage data collected through anonymous telemetry.
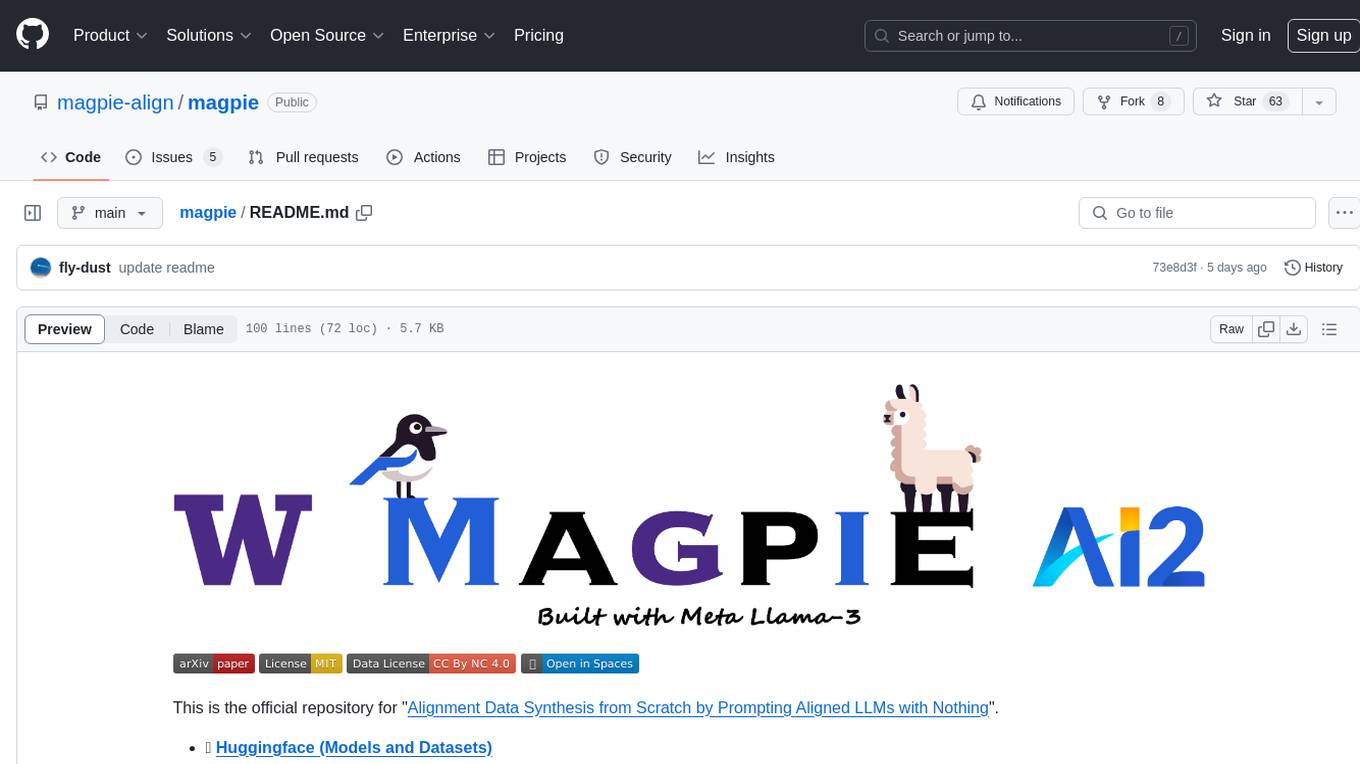
magpie
This is the official repository for 'Alignment Data Synthesis from Scratch by Prompting Aligned LLMs with Nothing'. Magpie is a tool designed to synthesize high-quality instruction data at scale by extracting it directly from an aligned Large Language Models (LLMs). It aims to democratize AI by generating large-scale alignment data and enhancing the transparency of model alignment processes. Magpie has been tested on various model families and can be used to fine-tune models for improved performance on alignment benchmarks such as AlpacaEval, ArenaHard, and WildBench.