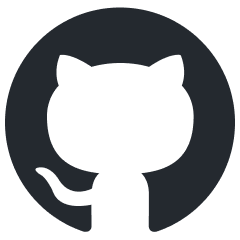
Mastering-NLP-from-Foundations-to-LLMs
Mastering NLP from Foundations to LLMs, Published by Packt
Stars: 86
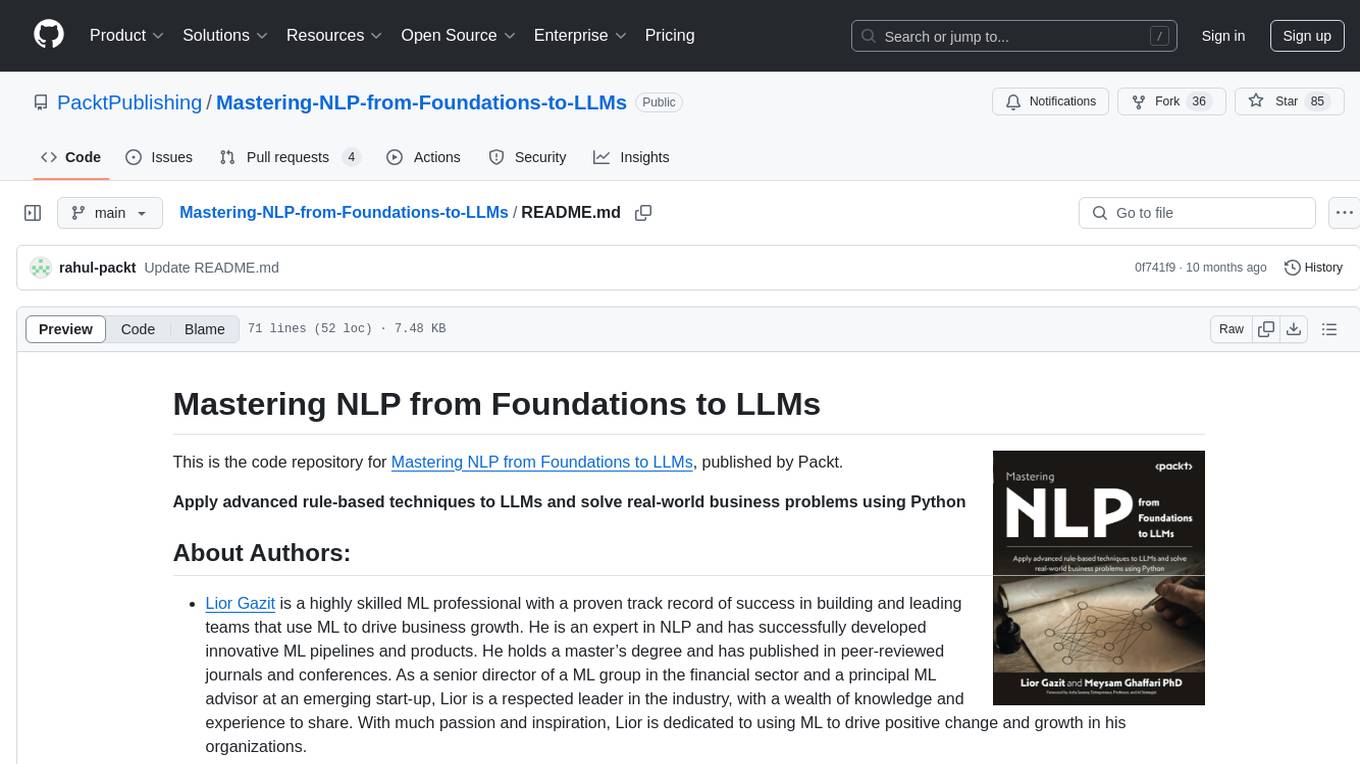
README:
This is the code repository for Mastering NLP from Foundations to LLMs, published by Packt.
Apply advanced rule-based techniques to LLMs and solve real-world business problems using Python
-
Lior Gazit is a highly skilled ML professional with a proven track record of success in building and leading teams that use ML to drive business growth. He is an expert in NLP and has successfully developed innovative ML pipelines and products. He holds a master’s degree and has published in peer-reviewed journals and conferences. As a senior director of a ML group in the financial sector and a principal ML advisor at an emerging start-up, Lior is a respected leader in the industry, with a wealth of knowledge and experience to share. With much passion and inspiration, Lior is dedicated to using ML to drive positive change and growth in his organizations.
-
Meysam Ghaffari is a senior data scientist with a strong background in NLP and deep learning. He currently works at MSKCC, where he specializes in developing and improving ML and NLP models for healthcare problems. He has over nine years of experience in ML and over four years of experience in NLP and deep learning. He received his Ph.D. in computer science from Florida State University, his MS in computer science – artificial intelligence from the Isfahan University of Technology, and his BS in computer science from Iran University of Science and Technology. He also worked as a post-doctoral research associate at the University of Wisconsin-Madison before joining MSKCC.
Enhance your NLP proficiency with modern frameworks like LangChain, explore mathematical foundations and code samples, and gain expert insights into current and future trends
- Learn how to build Python-driven solutions with a focus on NLP, LLMs, RAGs, and GPT
- Master embedding techniques and machine learning principles for real-world applications
- Understand the mathematical foundations of NLP and deep learning designs Purchase of the print or Kindle book includes a free PDF eBook
If you feel this book is for you, get your copy today!
Do you want to master Natural Language Processing (NLP) but don’t know where to begin? This book will give you the right head start. Written by leaders in machine learning and NLP, Mastering NLP from Foundations to LLMs provides an in-depth introduction to techniques. Starting with the mathematical foundations of machine learning (ML), you’ll gradually progress to advanced NLP applications such as large language models (LLMs) and AI applications. You’ll get to grips with linear algebra, optimization, probability, and statistics, which are essential for understanding and implementing machine learning and NLP algorithms. You’ll also explore general machine learning techniques and find out how they relate to NLP. Next, you’ll learn how to preprocess text data, explore methods for cleaning and preparing text for analysis, and understand how to do text classification. You’ll get all of this and more along with complete Python code samples.
By the end of the book, the advanced topics of LLMs’ theory, design, and applications will be discussed along with the future trends in NLP, which will feature expert opinions. You’ll also get to strengthen your practical skills by working on sample real-world NLP business problems and solutions.
- Master the mathematical foundations of machine learning and NLP Implement advanced techniques for preprocessing text data and analysis Design ML-NLP systems in Python
- Model and classify text using traditional machine learning and deep learning methods
- Understand the theory and design of LLMs and their implementation for various applications in AI
- Explore NLP insights, trends, and expert opinions on its future direction and potential
All of the code is organized into folders.
The code will look like the following:
import pandas as pd
import matplotlib.pyplot as plt
# Load the record dict from URL
import requests
import pickle
This book is for deep learning and machine learning researchers, NLP practitioners, ML/NLP educators, and STEM students. Professionals working with text data as part of their projects will also find plenty of useful information in this book. Beginner-level familiarity with machine learning and a basic working knowledge of Python will help you get the best out of this book.
With the following software and hardware list you can run all code files present in the book (Chapter 1-11).
Chapter | Software required | OS required |
---|---|---|
1-11 | Access to a Python environment via one of the following: Accessing Google Colab, which is free and easy from any browser on any device (recommended). A local/cloud development environment of Python with the ability to install public packages and access OpenAI’s API | Windows, macOS or Linux |
1-11 | Sufficient computation resources, as follows: The previously recommended free access to Google Colab includes a free GPU instance. If opting to avoid Google Colab, the local/cloud environment should have a GPU for several code examples |
- Navigating the NLP Landscape: A comprehensive introduction
- Mastering Linear Algebra, Probability, and Statistics for Machine Learning and NLP
- Unleashing Machine Learning Potentials in NLP
- Streamlining Text Preprocessing Techniques for Optimal NLP Performance (Notebooks for chapter 4)
- Empowering Text Classification: Leveraging Traditional Machine Learning Techniques (Notebooks for chapter 5)
- Text Classification Reimagined: Delving Deep into Deep Learning Language Models (Notebooks for chapter 6)
- Demystifying Large Language Models: Theory, Design, and Langchain Implementation
- Accessing the Power of Large Language Models: Advanced Setup and Integration with RAG (Notebooks for chapter 8)
- Exploring the Frontiers: Advanced Applications and Innovations Driven by LLMs (Notebooks for chapter 9)
- Riding the Wave: Analyzing Past, Present, and Future Trends Shaped by LLMs and AI
- Exclusive Industry Insights: Perspectives and Predictions from World Class Experts
For Tasks:
Click tags to check more tools for each tasksFor Jobs:
Alternative AI tools for Mastering-NLP-from-Foundations-to-LLMs
Similar Open Source Tools
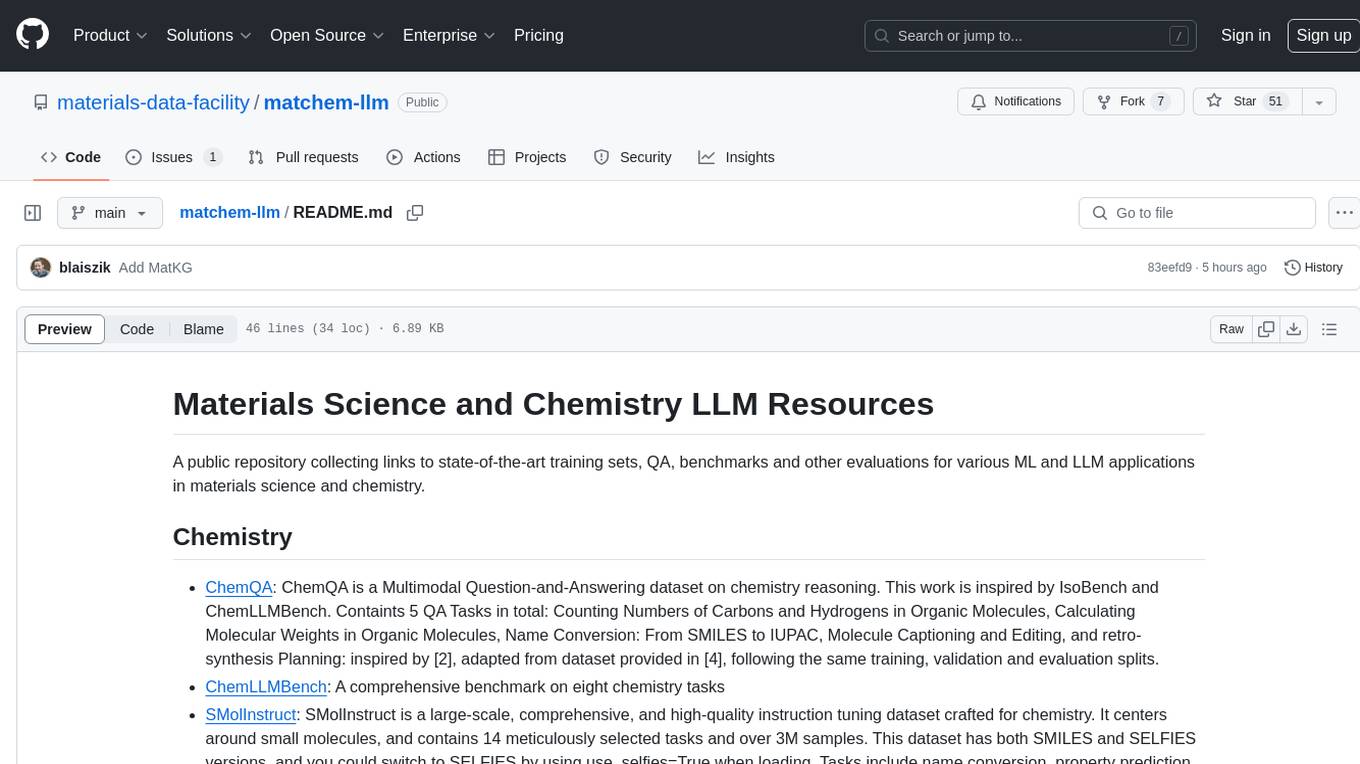
matchem-llm
A public repository collecting links to state-of-the-art training sets, QA, benchmarks and other evaluations for various ML and LLM applications in materials science and chemistry. It includes datasets related to chemistry, materials, multimodal data, and knowledge graphs in the field. The repository aims to provide resources for training and evaluating machine learning models in the materials science and chemistry domains.
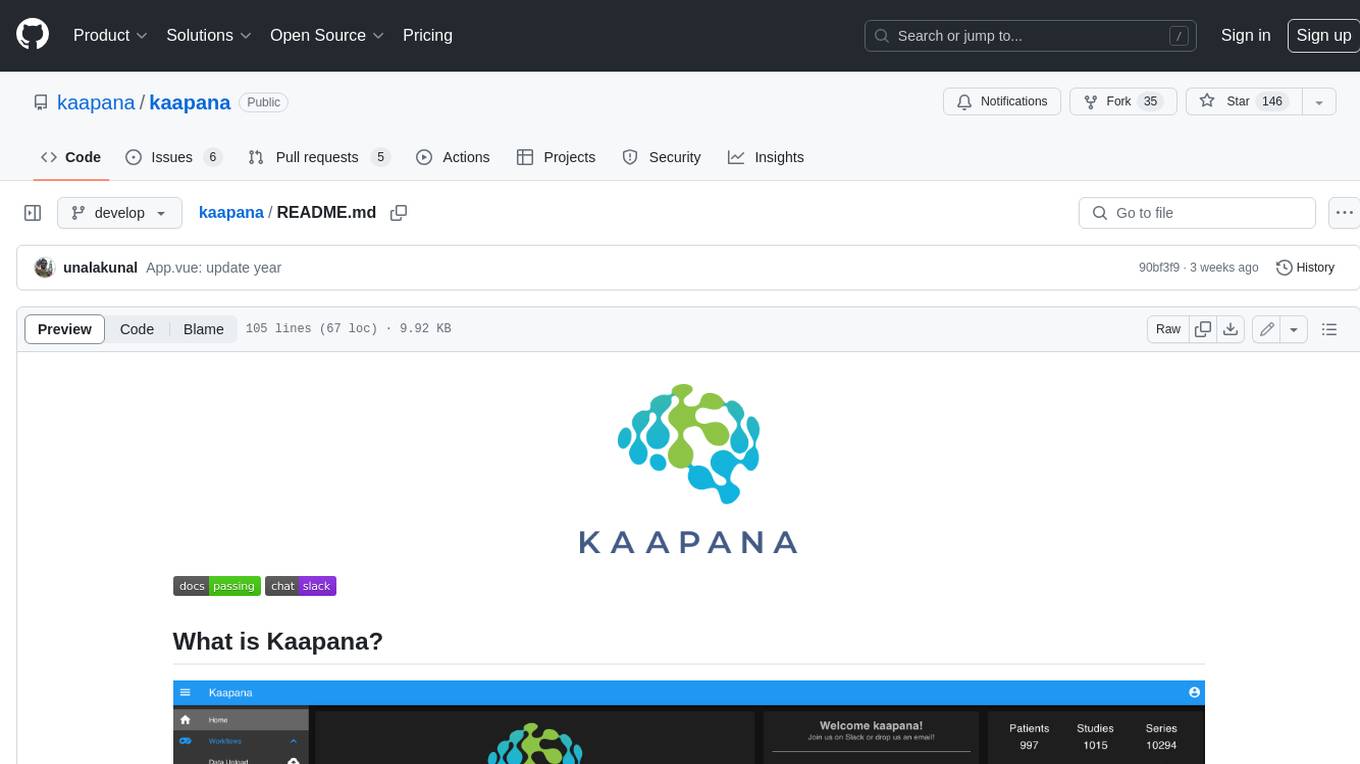
kaapana
Kaapana is an open-source toolkit for state-of-the-art platform provisioning in the field of medical data analysis. The applications comprise AI-based workflows and federated learning scenarios with a focus on radiological and radiotherapeutic imaging. Obtaining large amounts of medical data necessary for developing and training modern machine learning methods is an extremely challenging effort that often fails in a multi-center setting, e.g. due to technical, organizational and legal hurdles. A federated approach where the data remains under the authority of the individual institutions and is only processed on-site is, in contrast, a promising approach ideally suited to overcome these difficulties. Following this federated concept, the goal of Kaapana is to provide a framework and a set of tools for sharing data processing algorithms, for standardized workflow design and execution as well as for performing distributed method development. This will facilitate data analysis in a compliant way enabling researchers and clinicians to perform large-scale multi-center studies. By adhering to established standards and by adopting widely used open technologies for private cloud development and containerized data processing, Kaapana integrates seamlessly with the existing clinical IT infrastructure, such as the Picture Archiving and Communication System (PACS), and ensures modularity and easy extensibility.
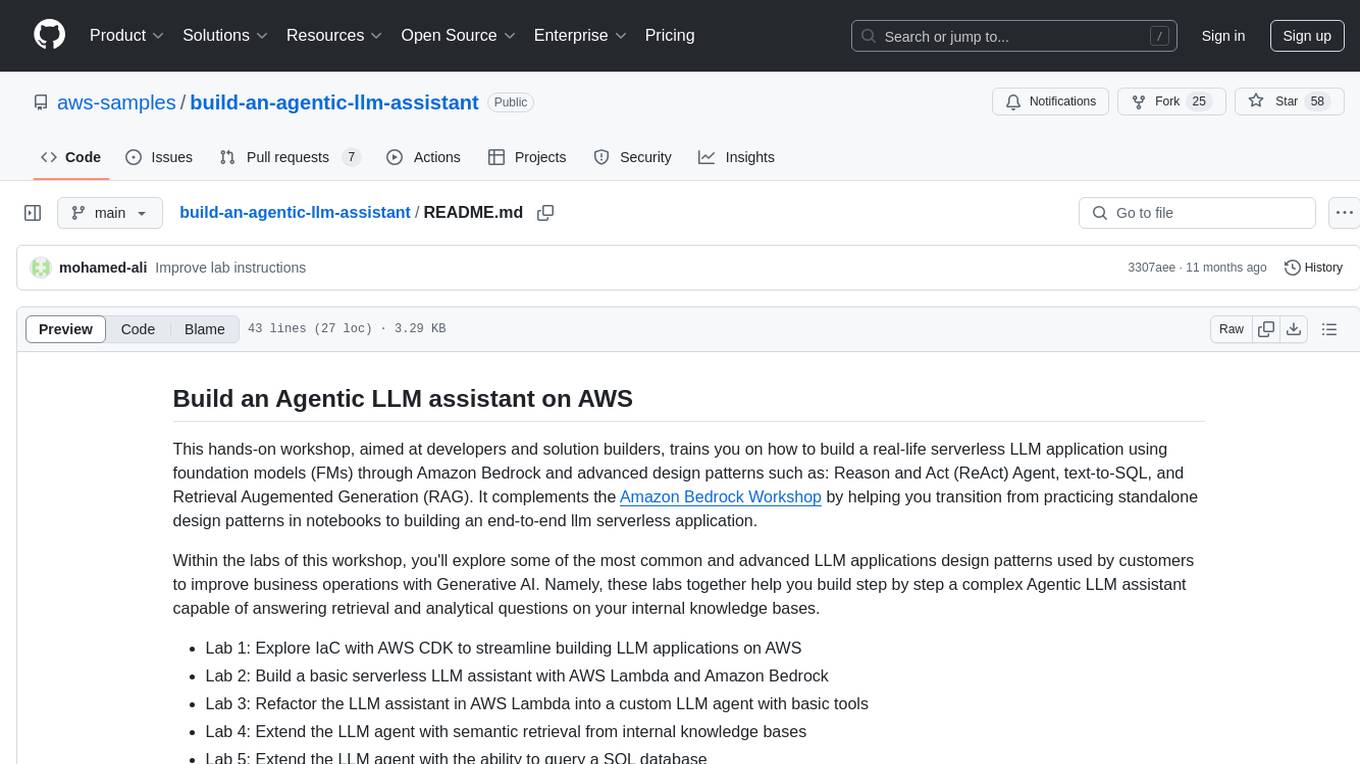
build-an-agentic-llm-assistant
This repository provides a hands-on workshop for developers and solution builders to build a real-life serverless LLM application using foundation models (FMs) through Amazon Bedrock and advanced design patterns such as Reason and Act (ReAct) Agent, text-to-SQL, and Retrieval Augmented Generation (RAG). It guides users through labs to explore common and advanced LLM application design patterns, helping them build a complex Agentic LLM assistant capable of answering retrieval and analytical questions on internal knowledge bases. The repository includes labs on IaC with AWS CDK, building serverless LLM assistants with AWS Lambda and Amazon Bedrock, refactoring LLM assistants into custom agents, extending agents with semantic retrieval, and querying SQL databases. Users need to set up AWS Cloud9, configure model access on Amazon Bedrock, and use Amazon SageMaker Studio environment to run data-pipelines notebooks.
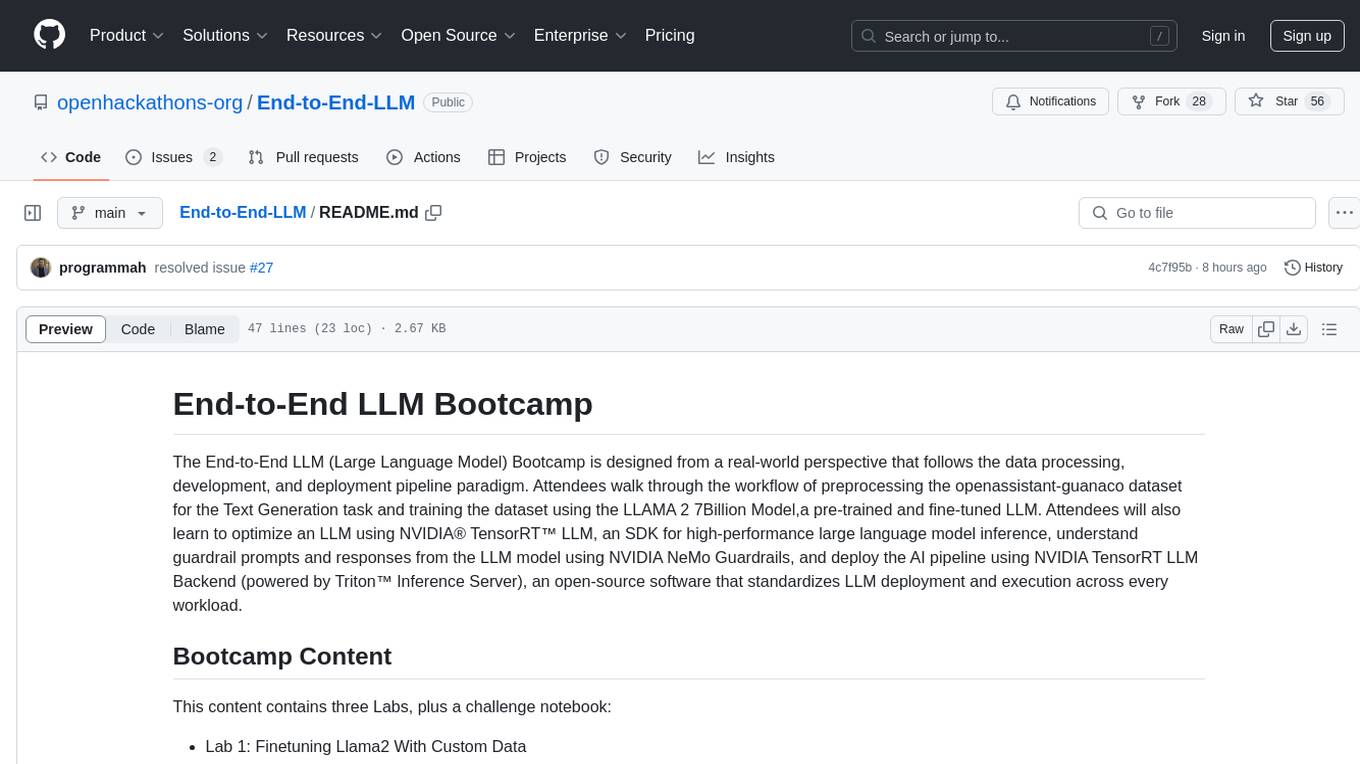
End-to-End-LLM
The End-to-End LLM Bootcamp is a comprehensive training program that covers the entire process of developing and deploying large language models. Participants learn to preprocess datasets, train models, optimize performance using NVIDIA technologies, understand guardrail prompts, and deploy AI pipelines using Triton Inference Server. The bootcamp includes labs, challenges, and practical applications, with a total duration of approximately 7.5 hours. It is designed for individuals interested in working with advanced language models and AI technologies.
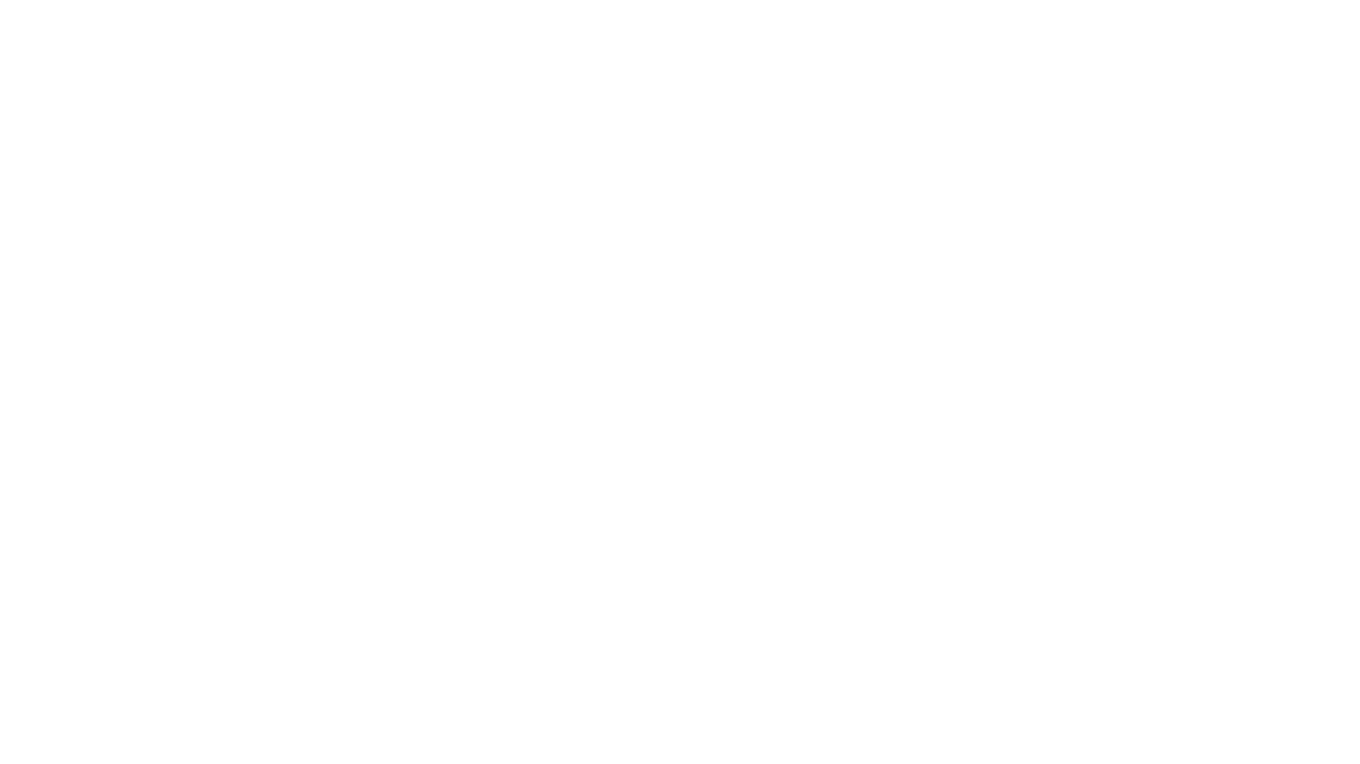
oreilly-hands-on-gpt-llm
This repository contains code for the O'Reilly Live Online Training for Deploying GPT & LLMs. Learn how to use GPT-4, ChatGPT, OpenAI embeddings, and other large language models to build applications for experimenting and production. Gain practical experience in building applications like text generation, summarization, question answering, and more. Explore alternative generative models such as Cohere and GPT-J. Understand prompt engineering, context stuffing, and few-shot learning to maximize the potential of GPT-like models. Focus on deploying models in production with best practices and debugging techniques. By the end of the training, you will have the skills to start building applications with GPT and other large language models.
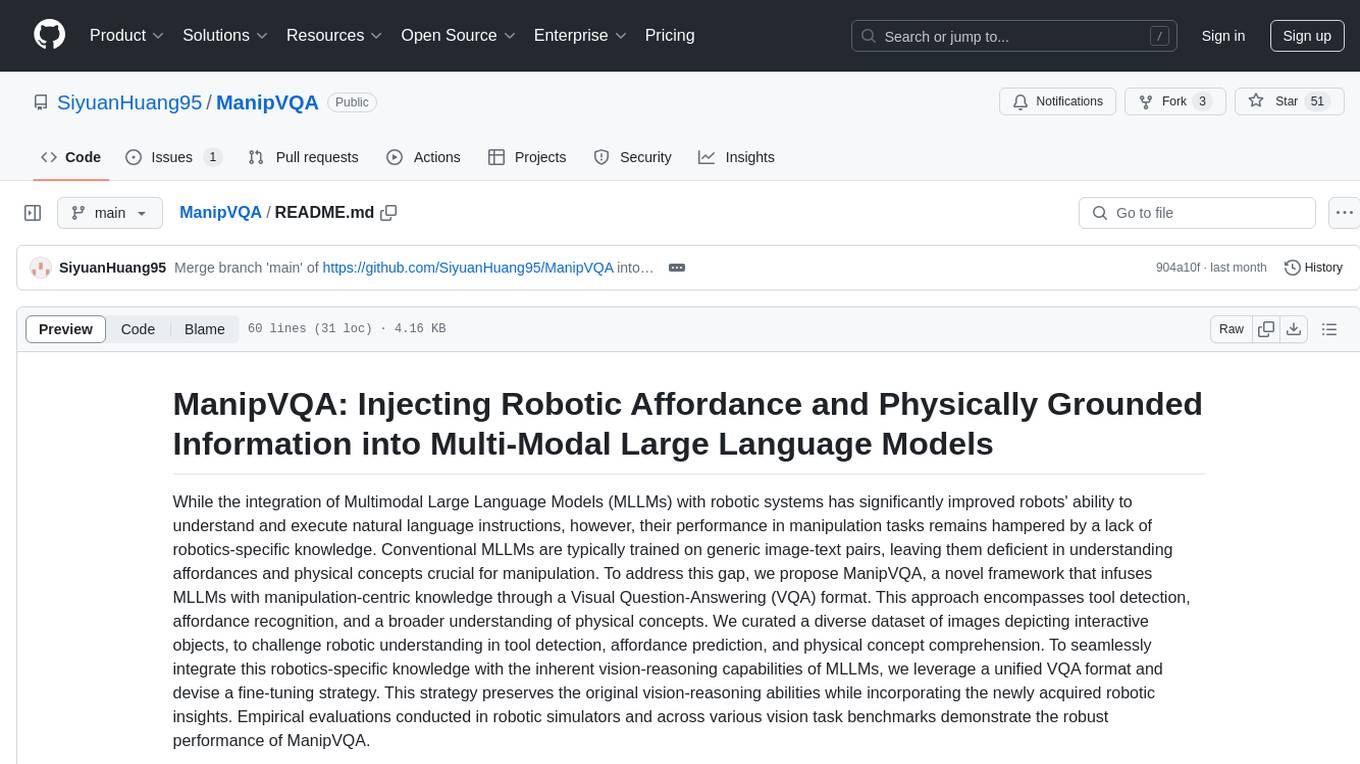
ManipVQA
ManipVQA is a framework that enhances Multimodal Large Language Models (MLLMs) with manipulation-centric knowledge through a Visual Question-Answering (VQA) format. It addresses the deficiency of conventional MLLMs in understanding affordances and physical concepts crucial for manipulation tasks. By infusing robotics-specific knowledge, including tool detection, affordance recognition, and physical concept comprehension, ManipVQA improves the performance of robots in manipulation tasks. The framework involves fine-tuning MLLMs with a curated dataset of interactive objects, enabling robots to understand and execute natural language instructions more effectively.
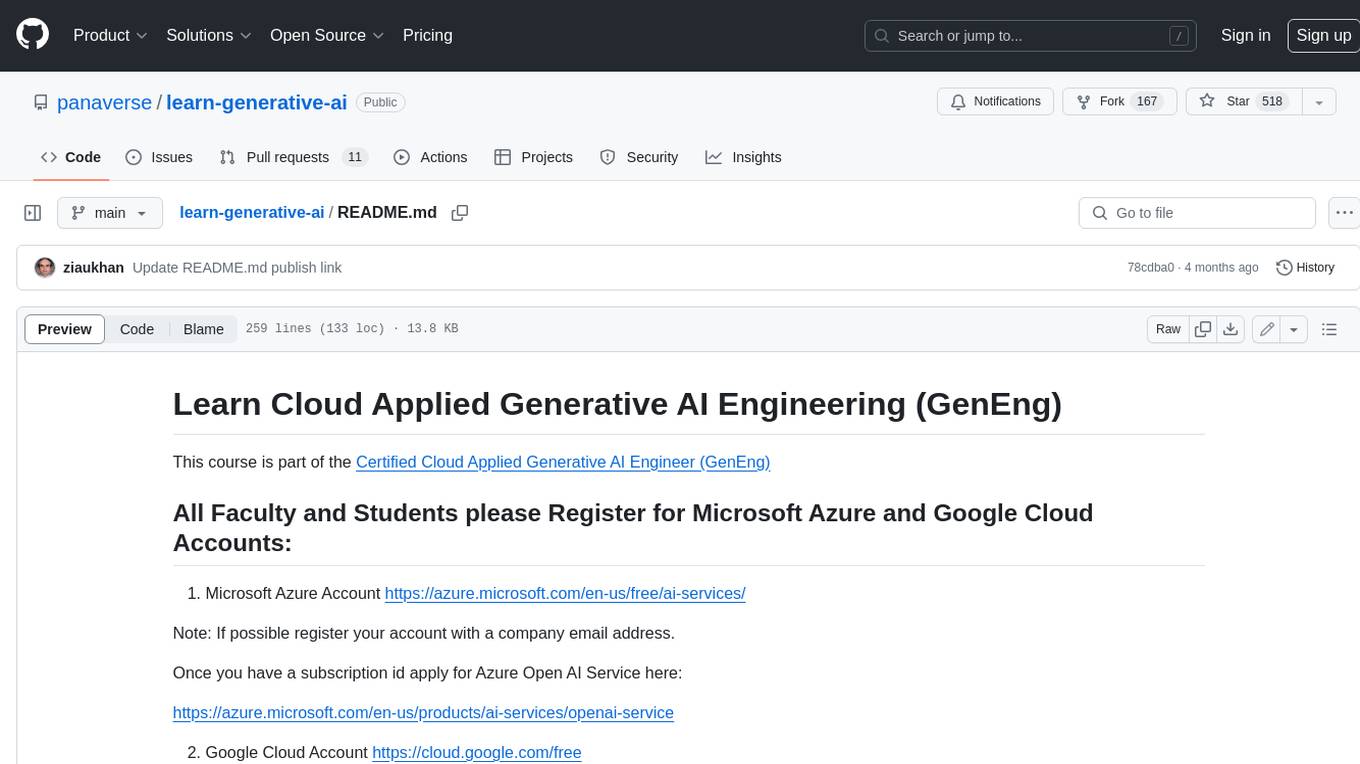
learn-generative-ai
Learn Cloud Applied Generative AI Engineering (GenEng) is a course focusing on the application of generative AI technologies in various industries. The course covers topics such as the economic impact of generative AI, the role of developers in adopting and integrating generative AI technologies, and the future trends in generative AI. Students will learn about tools like OpenAI API, LangChain, and Pinecone, and how to build and deploy Large Language Models (LLMs) for different applications. The course also explores the convergence of generative AI with Web 3.0 and its potential implications for decentralized intelligence.
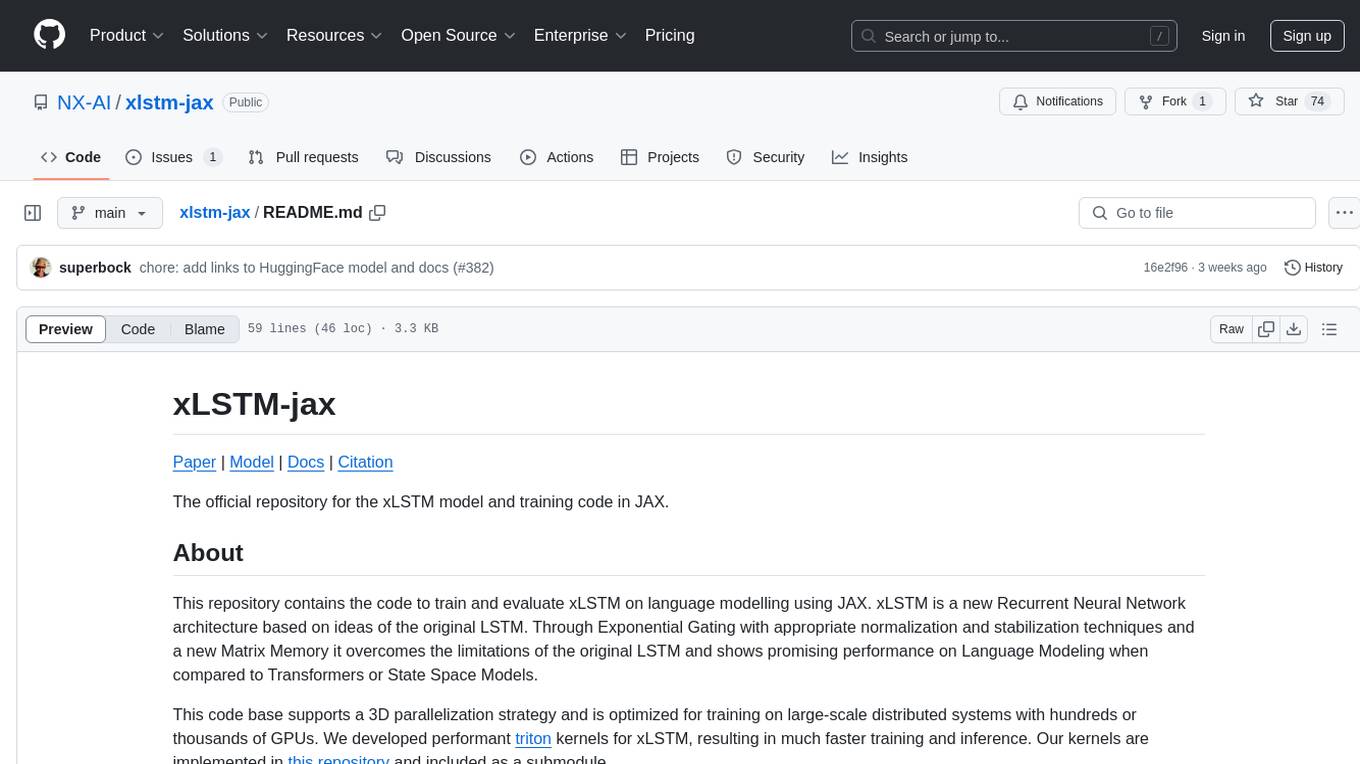
xlstm-jax
The xLSTM-jax repository contains code for training and evaluating the xLSTM model on language modeling using JAX. xLSTM is a Recurrent Neural Network architecture that improves upon the original LSTM through Exponential Gating, normalization, stabilization techniques, and a Matrix Memory. It is optimized for large-scale distributed systems with performant triton kernels for faster training and inference.
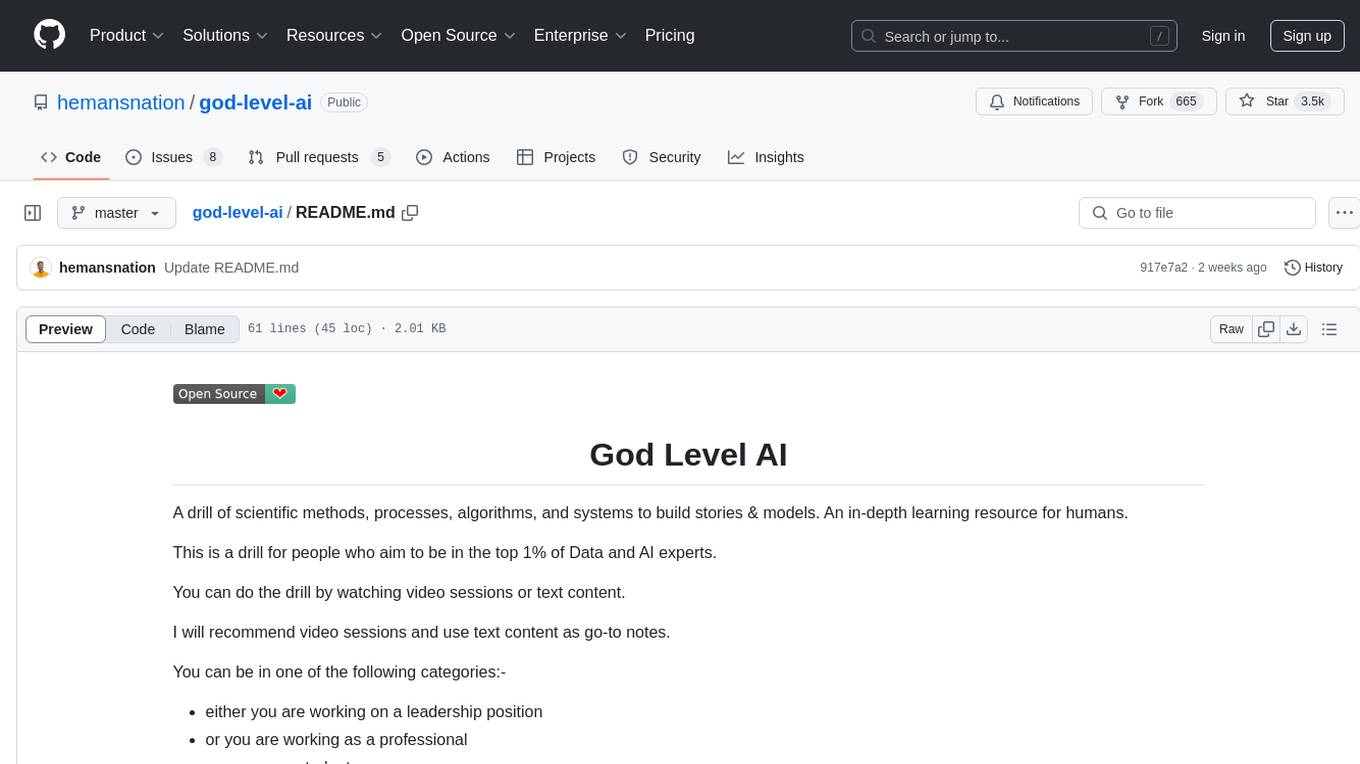
god-level-ai
A drill of scientific methods, processes, algorithms, and systems to build stories & models. An in-depth learning resource for humans. This is a drill for people who aim to be in the top 1% of Data and AI experts. The repository provides a routine for deep and shallow work sessions, covering topics from Python to AI/ML System Design and Personal Branding & Portfolio. It emphasizes the importance of continuous effort and action in the tech field.
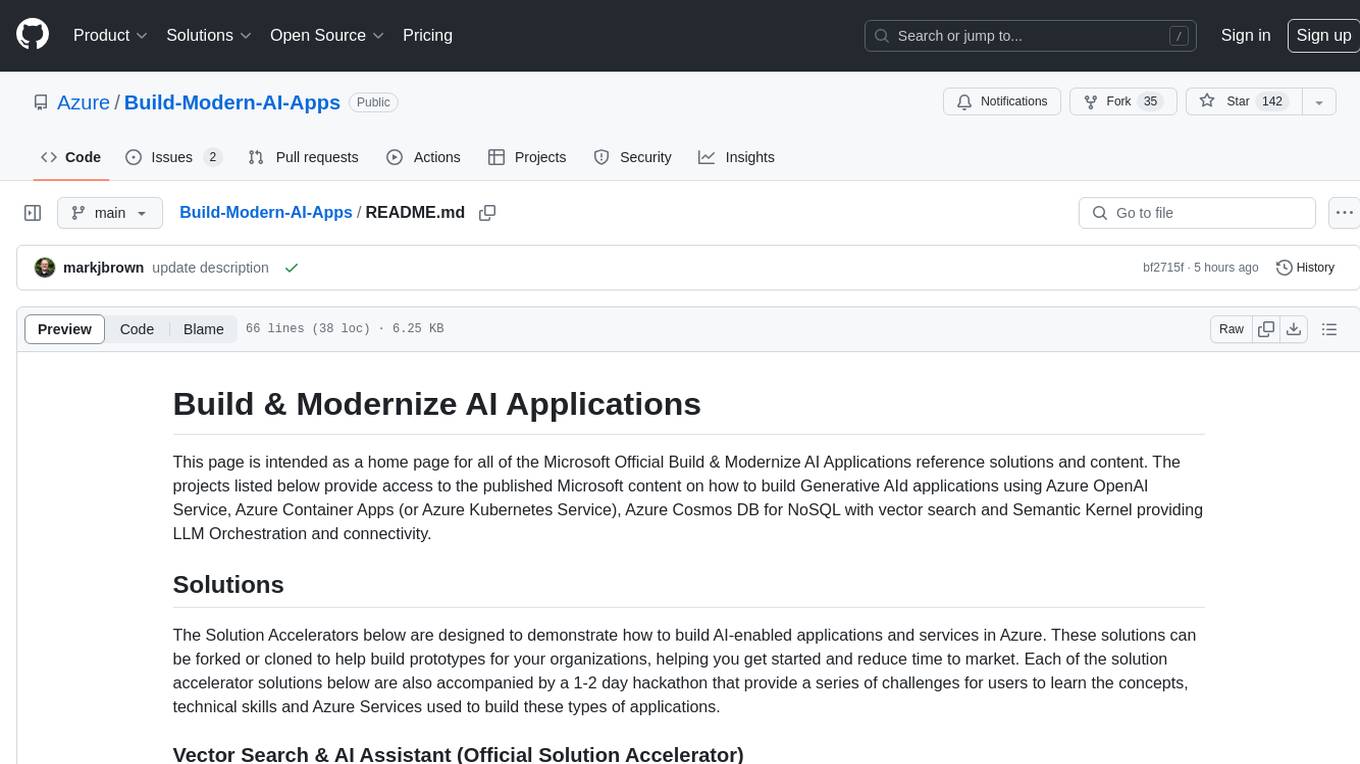
Build-Modern-AI-Apps
This repository serves as a hub for Microsoft Official Build & Modernize AI Applications reference solutions and content. It provides access to projects demonstrating how to build Generative AI applications using Azure services like Azure OpenAI, Azure Container Apps, Azure Kubernetes, and Azure Cosmos DB. The solutions include Vector Search & AI Assistant, Real-Time Payment and Transaction Processing, and Medical Claims Processing. Additionally, there are workshops like the Intelligent App Workshop for Microsoft Copilot Stack, focusing on infusing intelligence into traditional software systems using foundation models and design thinking.
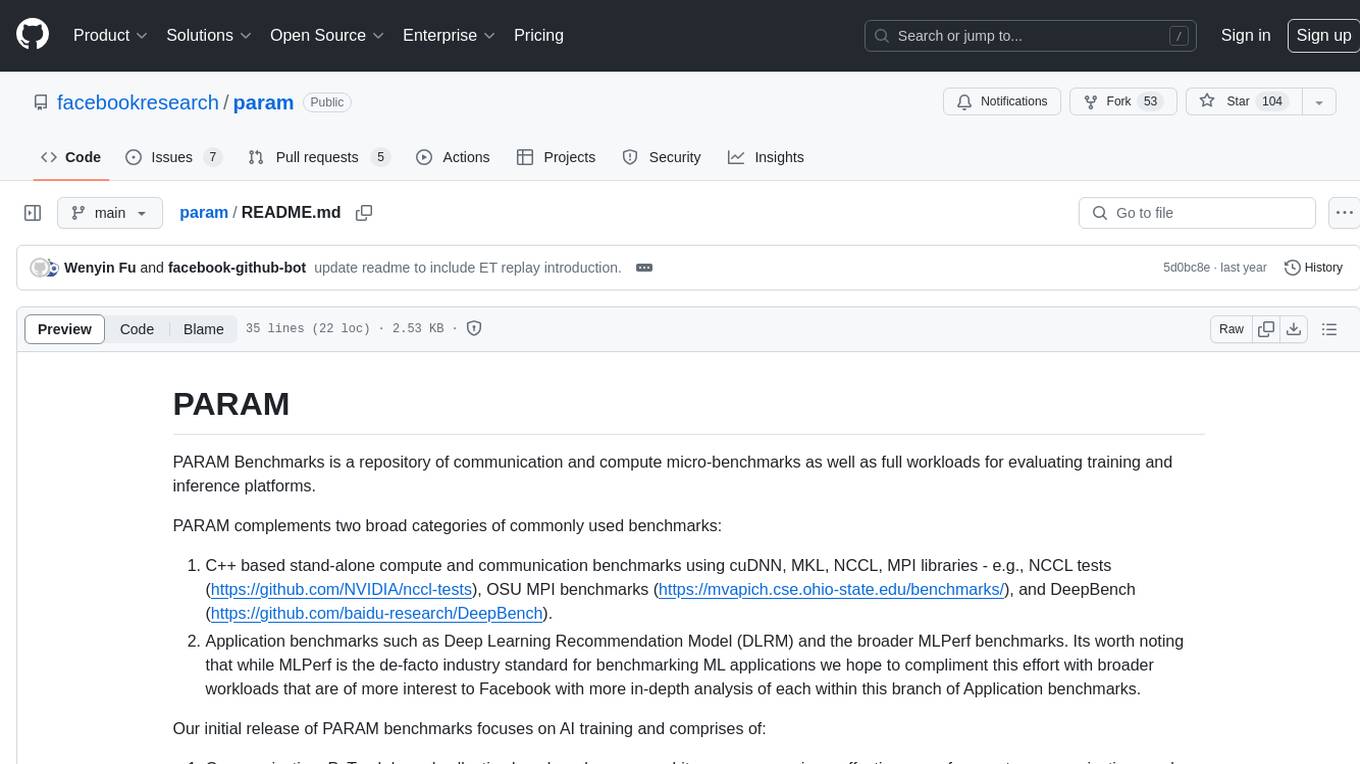
param
PARAM Benchmarks is a repository of communication and compute micro-benchmarks as well as full workloads for evaluating training and inference platforms. It complements commonly used benchmarks by focusing on AI training with PyTorch based collective benchmarks, GEMM, embedding lookup, linear layer, and DLRM communication patterns. The tool bridges the gap between stand-alone C++ benchmarks and PyTorch/Tensorflow based application benchmarks, providing deep insights into system architecture and framework-level overheads.
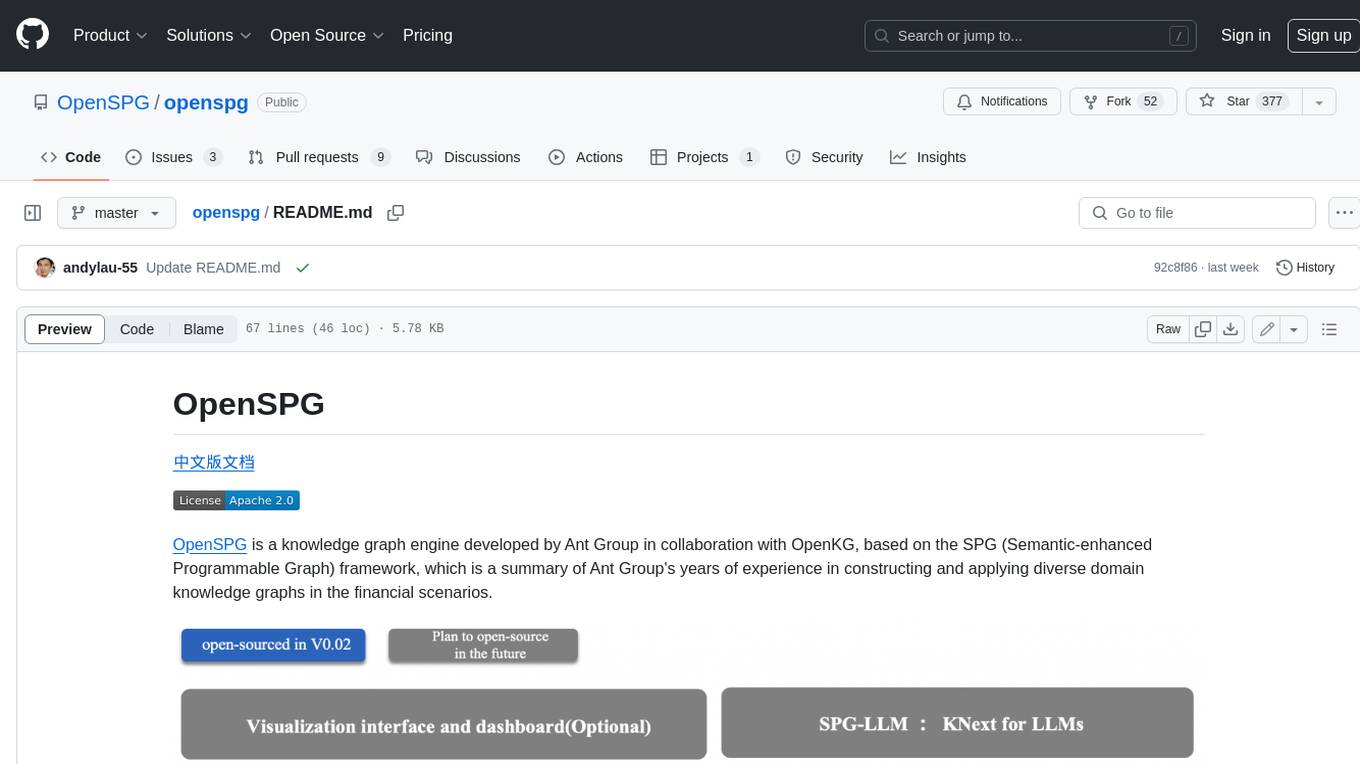
openspg
OpenSPG is a knowledge graph engine developed by Ant Group in collaboration with OpenKG, based on the SPG (Semantic-enhanced Programmable Graph) framework. It provides explicit semantic representations, logical rule definitions, operator frameworks (construction, inference), and other capabilities for domain knowledge graphs. OpenSPG supports pluggable adaptation of basic engines and algorithmic services by various vendors to build customized solutions.
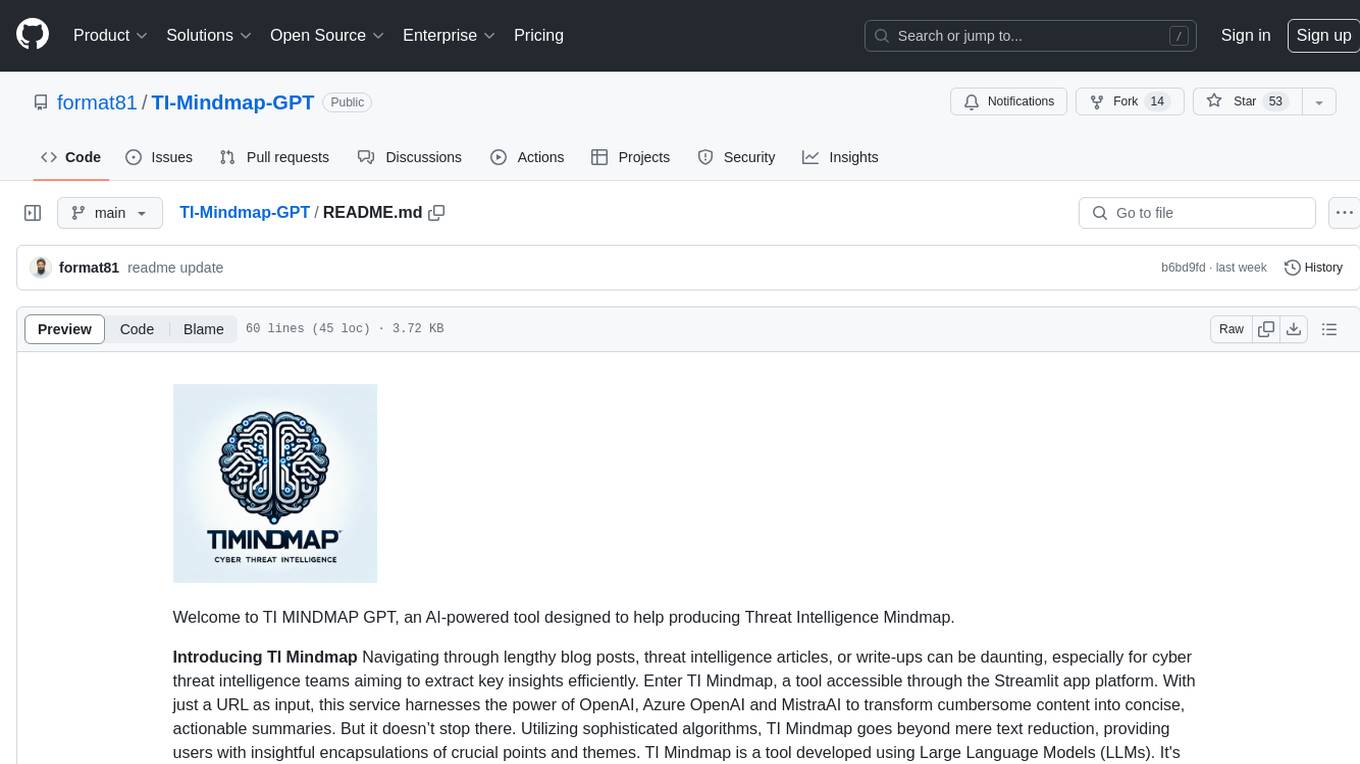
TI-Mindmap-GPT
TI MINDMAP GPT is an AI-powered tool designed to assist cyber threat intelligence teams in quickly synthesizing and visualizing key information from various Threat Intelligence sources. The tool utilizes Large Language Models (LLMs) to transform lengthy content into concise, actionable summaries, going beyond mere text reduction to provide insightful encapsulations of crucial points and themes. Users can leverage their own LLM keys for personalized and efficient information processing, streamlining data analysis and enabling teams to focus on strategic decision-making.
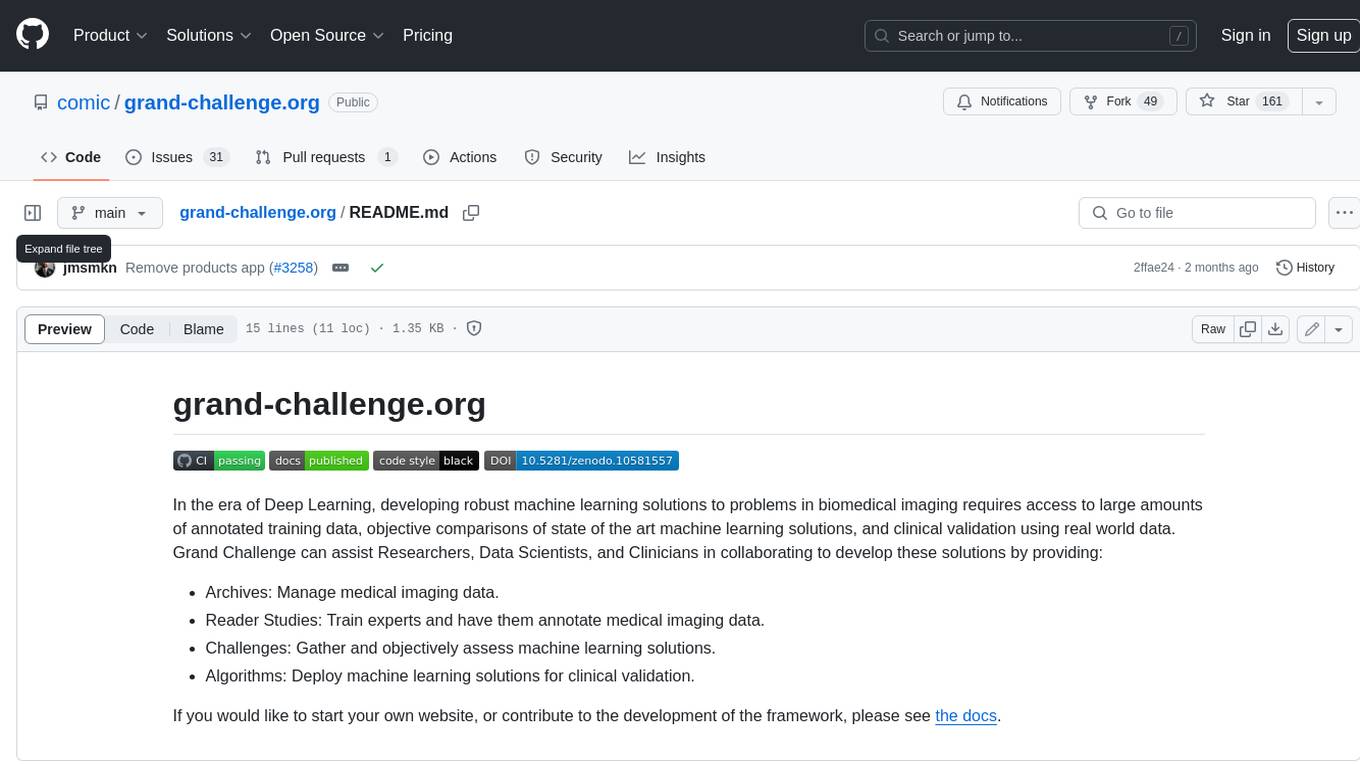
grand-challenge.org
Grand Challenge is a platform that provides access to large amounts of annotated training data, objective comparisons of state-of-the-art machine learning solutions, and clinical validation using real-world data. It assists researchers, data scientists, and clinicians in collaborating to develop robust machine learning solutions to problems in biomedical imaging.
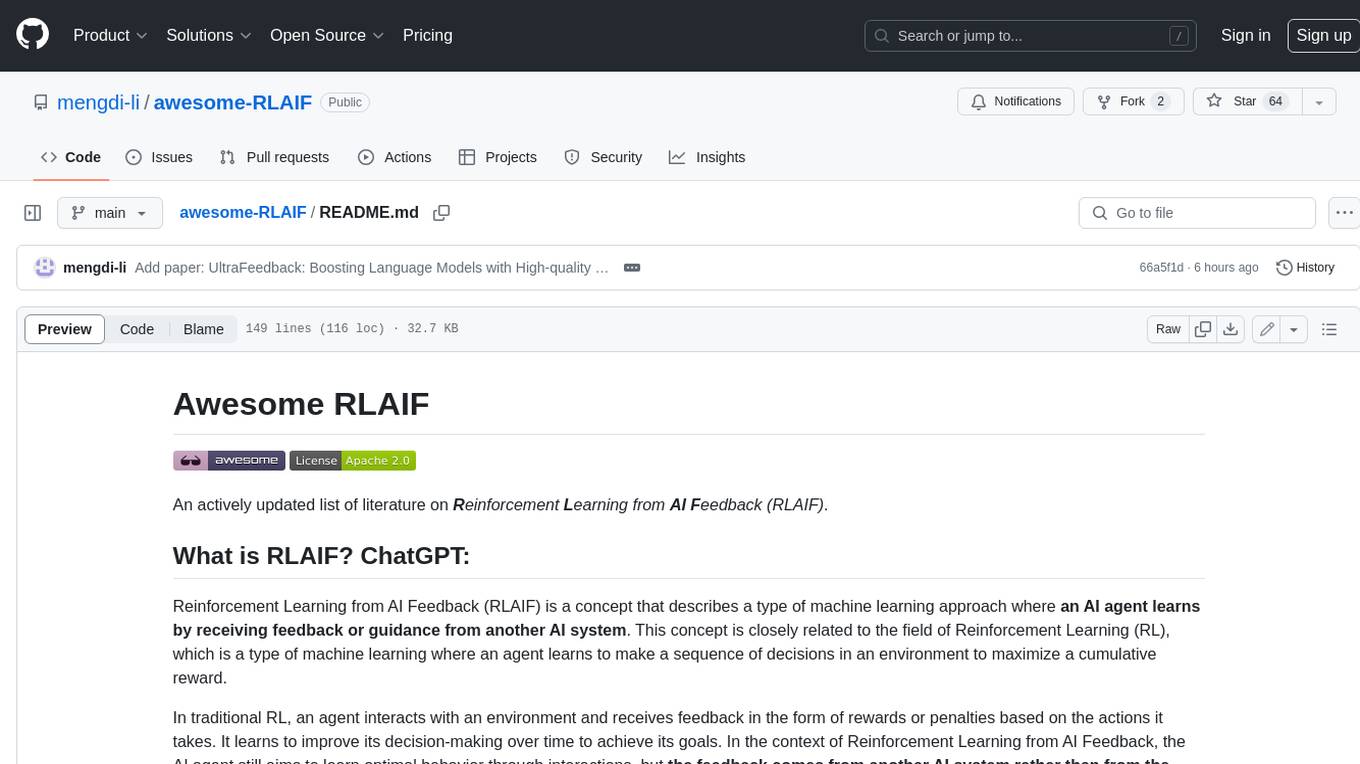
awesome-RLAIF
Reinforcement Learning from AI Feedback (RLAIF) is a concept that describes a type of machine learning approach where **an AI agent learns by receiving feedback or guidance from another AI system**. This concept is closely related to the field of Reinforcement Learning (RL), which is a type of machine learning where an agent learns to make a sequence of decisions in an environment to maximize a cumulative reward. In traditional RL, an agent interacts with an environment and receives feedback in the form of rewards or penalties based on the actions it takes. It learns to improve its decision-making over time to achieve its goals. In the context of Reinforcement Learning from AI Feedback, the AI agent still aims to learn optimal behavior through interactions, but **the feedback comes from another AI system rather than from the environment or human evaluators**. This can be **particularly useful in situations where it may be challenging to define clear reward functions or when it is more efficient to use another AI system to provide guidance**. The feedback from the AI system can take various forms, such as: - **Demonstrations** : The AI system provides demonstrations of desired behavior, and the learning agent tries to imitate these demonstrations. - **Comparison Data** : The AI system ranks or compares different actions taken by the learning agent, helping it to understand which actions are better or worse. - **Reward Shaping** : The AI system provides additional reward signals to guide the learning agent's behavior, supplementing the rewards from the environment. This approach is often used in scenarios where the RL agent needs to learn from **limited human or expert feedback or when the reward signal from the environment is sparse or unclear**. It can also be used to **accelerate the learning process and make RL more sample-efficient**. Reinforcement Learning from AI Feedback is an area of ongoing research and has applications in various domains, including robotics, autonomous vehicles, and game playing, among others.