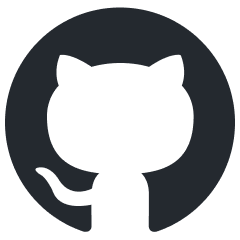
bmf
Cross-platform, customizable multimedia/video processing framework. With strong GPU acceleration, heterogeneous design, multi-language support, easy to use, multi-framework compatible and high performance, the framework is ideal for transcoding, AI inference, algorithm integration, live video streaming, and more.
Stars: 841
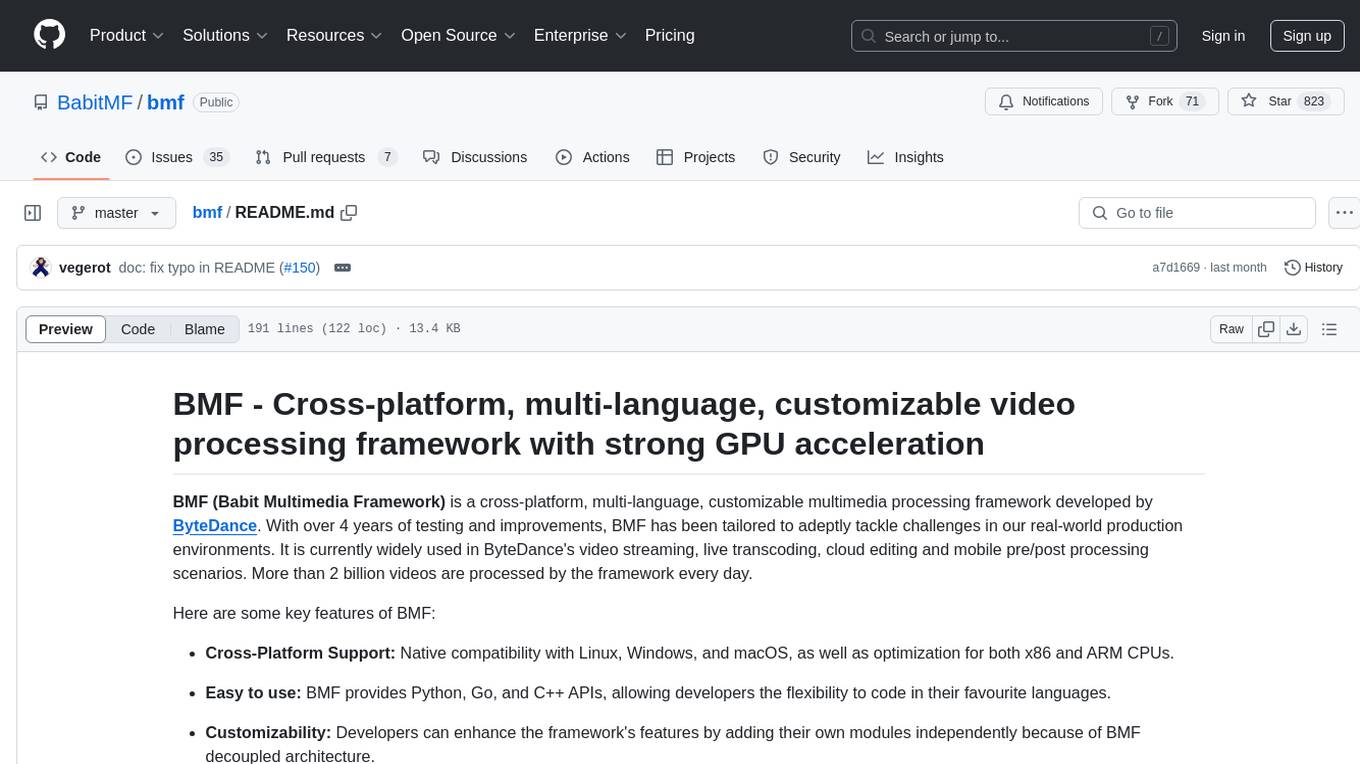
BMF (Babit Multimedia Framework) is a cross-platform, multi-language, customizable multimedia processing framework developed by ByteDance. It offers native compatibility with Linux, Windows, and macOS, Python, Go, and C++ APIs, and high performance with strong GPU acceleration. BMF allows developers to enhance its features independently and provides efficient data conversion across popular frameworks and hardware devices. BMFLite is a client-side lightweight framework used in apps like Douyin/Xigua, serving over one billion users daily. BMF is widely used in video streaming, live transcoding, cloud editing, and mobile pre/post processing scenarios.
README:
BMF - Cross-platform, multi-language, customizable video processing framework with strong GPU acceleration
BMF (Babit Multimedia Framework) is a cross-platform, multi-language, customizable multimedia processing framework developed by ByteDance. With over 4 years of testing and improvements, BMF has been tailored to adeptly tackle challenges in our real-world production environments. It is currently widely used in ByteDance's video streaming, live transcoding, cloud editing and mobile pre/post processing scenarios. More than 2 billion videos are processed by the framework every day.
Here are some key features of BMF:
-
Cross-Platform Support: Native compatibility with Linux, Windows, and macOS, as well as optimization for both x86 and ARM CPUs.
-
Easy to use: BMF provides Python, Go, and C++ APIs, allowing developers the flexibility to code in their favourite languages.
-
Customizability: Developers can enhance the framework's features by adding their own modules independently because of BMF decoupled architecture.
-
High performance: BMF has a powerful scheduler and strong support for heterogeneous acceleration hardware. Moreover, NVIDIA has been cooperating with us to develop a highly optimized GPU pipeline for video transcoding and AI inference.
-
Efficient data conversion: BMF offers seamless data format conversions across popular frameworks (FFmpeg/Numpy/PyTorch/OpenCV/TensorRT), conversion between hardware devices (CPU/GPU), and color space and pixel format conversion.
BMFLite is a client-side cross-platform, lightweight, more efficient client-side multimedia processing framework. So far, the BMFLite client-side algorithm is used in apps such as Douyin/Xigua, serving more than one billion users in live streaming/video playing/pictures/cloud games and other scenarios, and processing videos and pictures trillions of times every day.
Dive deeper into BMF's capabilities on our website for more details.
In this section, we will directly showcase the capabilities of the BMF framework around six dimensions: Transcode, Edit, Meeting/Broadcaster, GPU acceleration, AI Inference, and client-side Framework. For all the demos provided below, corresponding implementations and documentation are available on Google Colab, allowing you to experience them intuitively.
This demo describes step-by-step how to use BMF to develop a transcoding program, including video transcoding, audio transcoding, and image transcoding. In it, you can familiarize yourself with how to use BMF and how to use FFmpeg-compatible options to achieve the capabilities you need.
If you want to have a quick experiment, you can try it on
The Edit Demo will show you how to implement a high-complexity audio and video editing pipeline through the BMF framework. We have implemented two Python modules, video_concat and video_overlay, and combined various atomic capabilities to construct a complex BMF Graph.
If you want to have a quick experiment, you can try it on
This demo uses BMF framework to construct a simple broadcast service. The service provides an API that enables dynamic video source pulling, video layout control, audio mixing, and ultimately streaming the output to an RTMP server. This demo showcases the modularity of BMF, multi-language development, and the ability to dynamically adjust the pipeline.
Below is a screen recording demonstrating the operation of broadcaster:
The video frame extraction acceleration demo shows:
-
BMF flexible capability of:
- Multi-language programming, we can see multi-language modules work together in the demo
- Ability to extend easily, there are new C++, Python modules added simply
- FFmpeg ability is fully compatible
-
Hardware acceleration quickly enablement and CPU/GPU pipeline support
- Heterogeneous pipeline is supported in BMF, such as process between CPU and GPU
- Useful hardware color space conversion in BMF
If you want to have a quick experiment, you can try it on
The GPU transcoding and filter module demo shows:
- Common video/image filters in BMF accelerated by GPU
- How to write GPU modules in BMF
The demo builds a transcoding pipeline which fully runs on GPU:
decode->scale->flip->rotate->crop->blur->encode
If you want to have a quick experiment, you can try it on
The prototype of how to build a video preprocessing for LLM training data in Bytedance, which serves billions of clip processing each day.
The input video will be split according to scene change, and subtitles in the video will be detected and cropped by OCR module, and the video quality will be assessed by BMF provided aesthetic module. After that, the finalized video clips will be encoded as output.
If you want to have a quick experiment, you can try it on
This demo shows how to integrate the state of art AI algorithms into the BMF video processing pipeline. The famous open source colorization algorithm DeOldify is wrapped as a BMF pyhton module in less than 100 lines of codes. The final effect is illustrated below, with the original video on the left side and the colored video on the right.
If you want to have a quick experiment, you can try it on
This demo implements the super-resolution inference process of Real-ESRGAN as a BMF module, showcasing a BMF pipeline that combines decoding, super-resolution inference and encoding.
If you want to have a quick experiment, you can try it on
This demo shows how to invoke our aesthetic assessment model using bmf. Our deep learning model Aesmode has achieved a binary classification accuracy of 83.8% on AVA dataset, reaching the level of academic SOTA, and can be directly used to evaluate the aesthetic degree of videos by means of frame extraction processing.
If you want to have a quick experiment, you can try it on
This Demo shows a full-link face detect pipeline based on TensorRT acceleration, which internally uses the TensorRT-accelerated Onnx model to process the input video. It uses the NMS algorithm to filter repeated candidate boxes to form an output, which can be used to process a Face Detection Task efficiently.
If you want to have a quick experiment, you can try it on
This case illustrates the procedures of integrating an external algorithm module into the BMFLite framework and management of its execution.
This example implements the denoise algorithm as a BMF module, showcasing a BMF pipeline that combines video capture, noise reduction and rendering.
-
- Install
-
Create a Graph
- one of transcode example with 3 languages
- Use Module Directly
- Create a Module
-
APIs
The project has an Apache 2.0 License. Third party components and dependencies remain under their own licenses.
Contributions are welcomed. Please follow the guidelines.
We use GitHub issues to track and resolve problems. If you have any questions, please feel free to join the discussion and work with us to find a solution.
The decoder, encoder and filter reference ffmpeg cmdline tool. They are wrapped as BMF's built-in modules under the LGPL license.
The project also draws inspiration from other popular frameworks, such as ffmpeg-python and mediapipe. Our website is using the project from docsy based on hugo.
Here, we'd like to express our sincerest thanks to the developers of the above projects!
For Tasks:
Click tags to check more tools for each tasksFor Jobs:
Alternative AI tools for bmf
Similar Open Source Tools
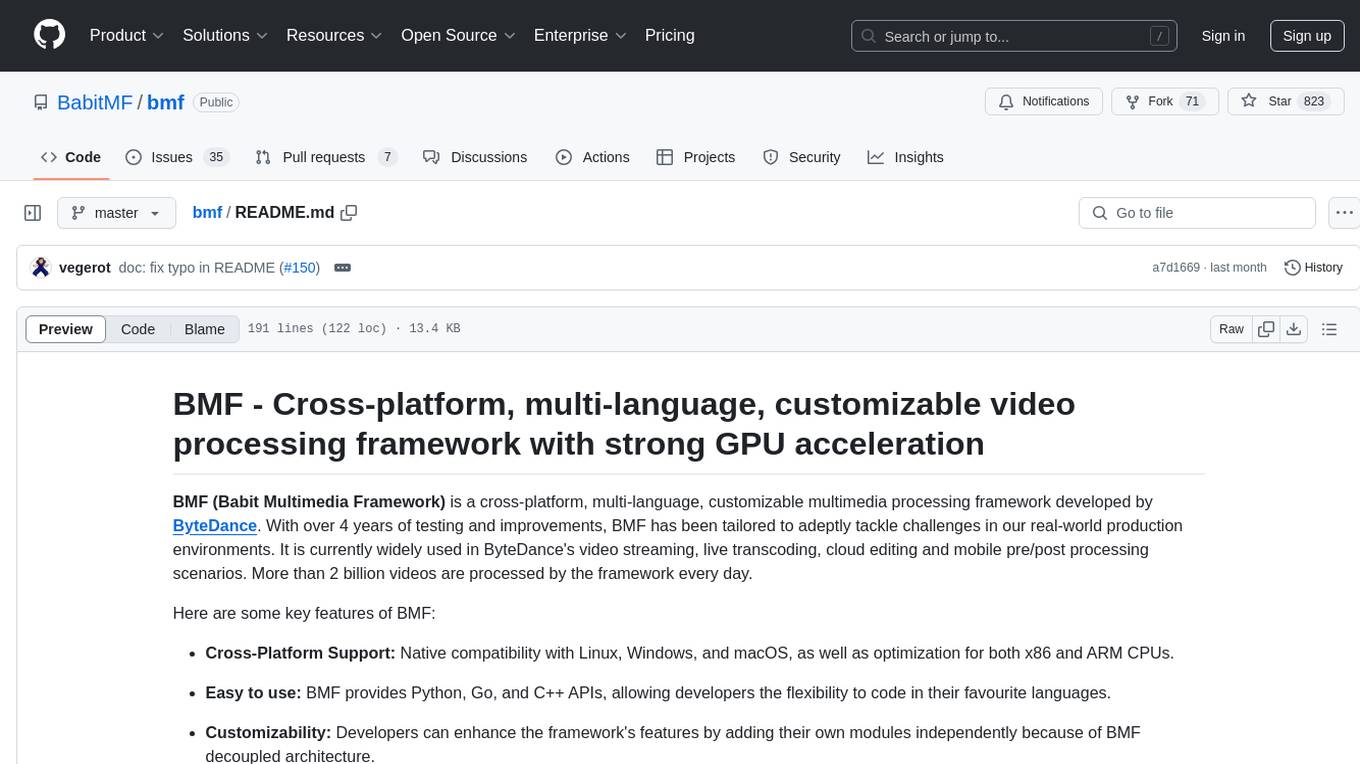
bmf
BMF (Babit Multimedia Framework) is a cross-platform, multi-language, customizable multimedia processing framework developed by ByteDance. It offers native compatibility with Linux, Windows, and macOS, Python, Go, and C++ APIs, and high performance with strong GPU acceleration. BMF allows developers to enhance its features independently and provides efficient data conversion across popular frameworks and hardware devices. BMFLite is a client-side lightweight framework used in apps like Douyin/Xigua, serving over one billion users daily. BMF is widely used in video streaming, live transcoding, cloud editing, and mobile pre/post processing scenarios.
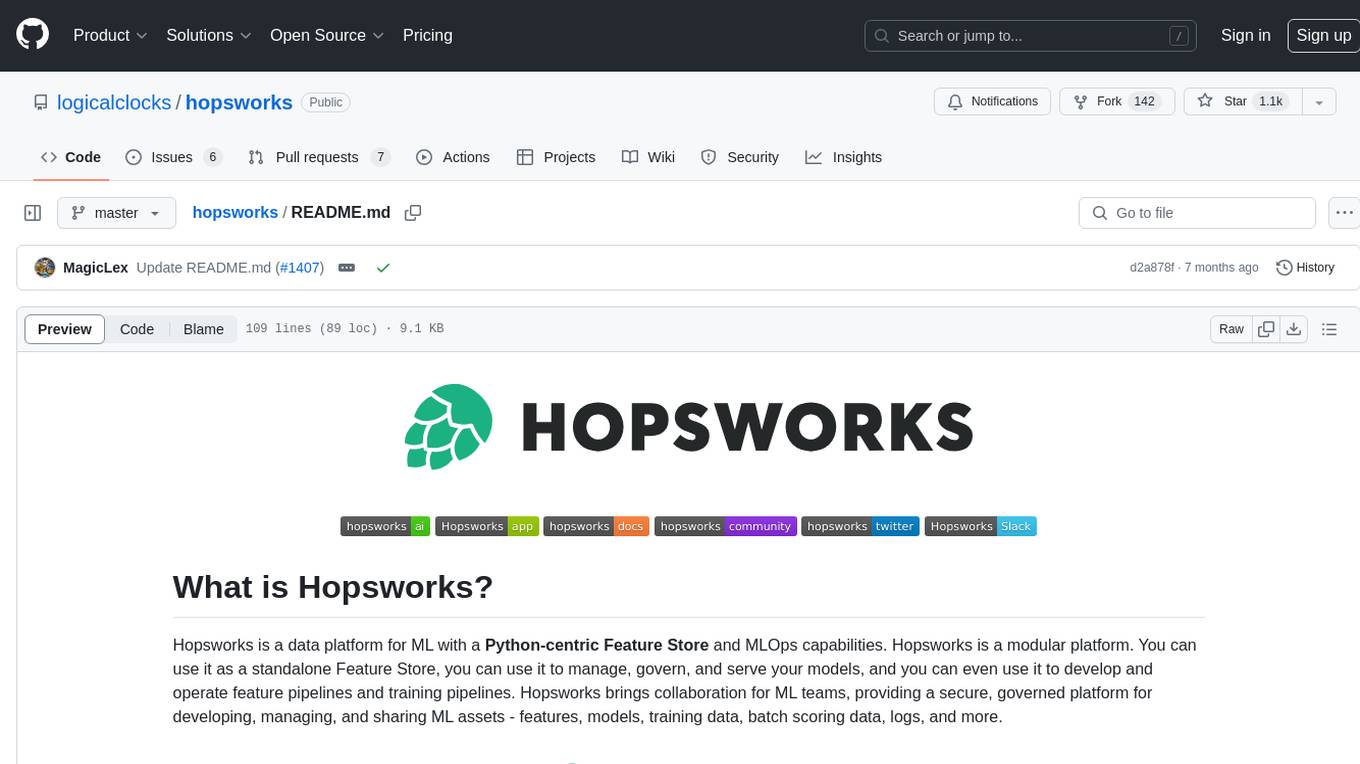
hopsworks
Hopsworks is a data platform for ML with a Python-centric Feature Store and MLOps capabilities. It provides collaboration for ML teams, offering a secure, governed platform for developing, managing, and sharing ML assets. Hopsworks supports project-based multi-tenancy, team collaboration, development tools for Data Science, and is available on any platform including managed cloud services and on-premise installations. The platform enables end-to-end responsibility from raw data to managed features and models, supports versioning, lineage, and provenance, and facilitates the complete MLOps life cycle.
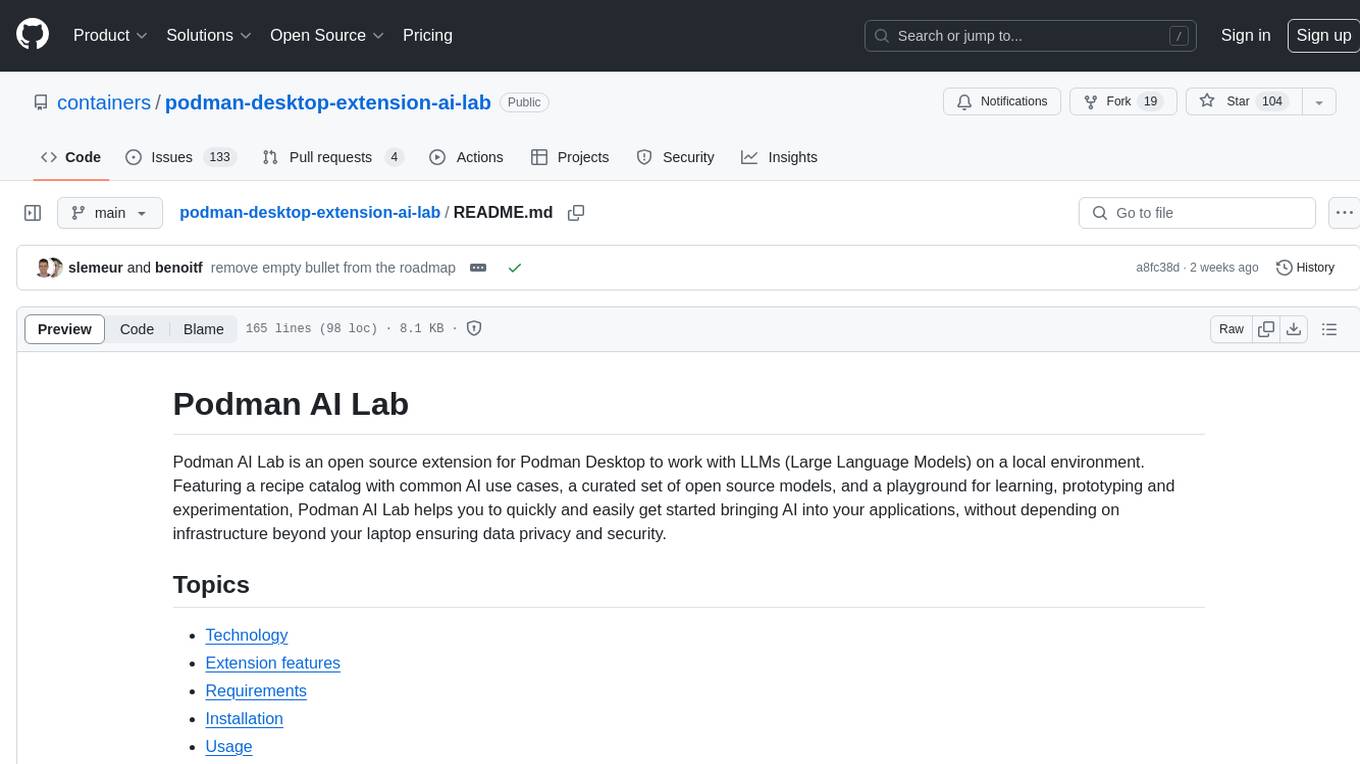
podman-desktop-extension-ai-lab
Podman AI Lab is an open source extension for Podman Desktop designed to work with Large Language Models (LLMs) on a local environment. It features a recipe catalog with common AI use cases, a curated set of open source models, and a playground for learning, prototyping, and experimentation. Users can quickly and easily get started bringing AI into their applications without depending on external infrastructure, ensuring data privacy and security.
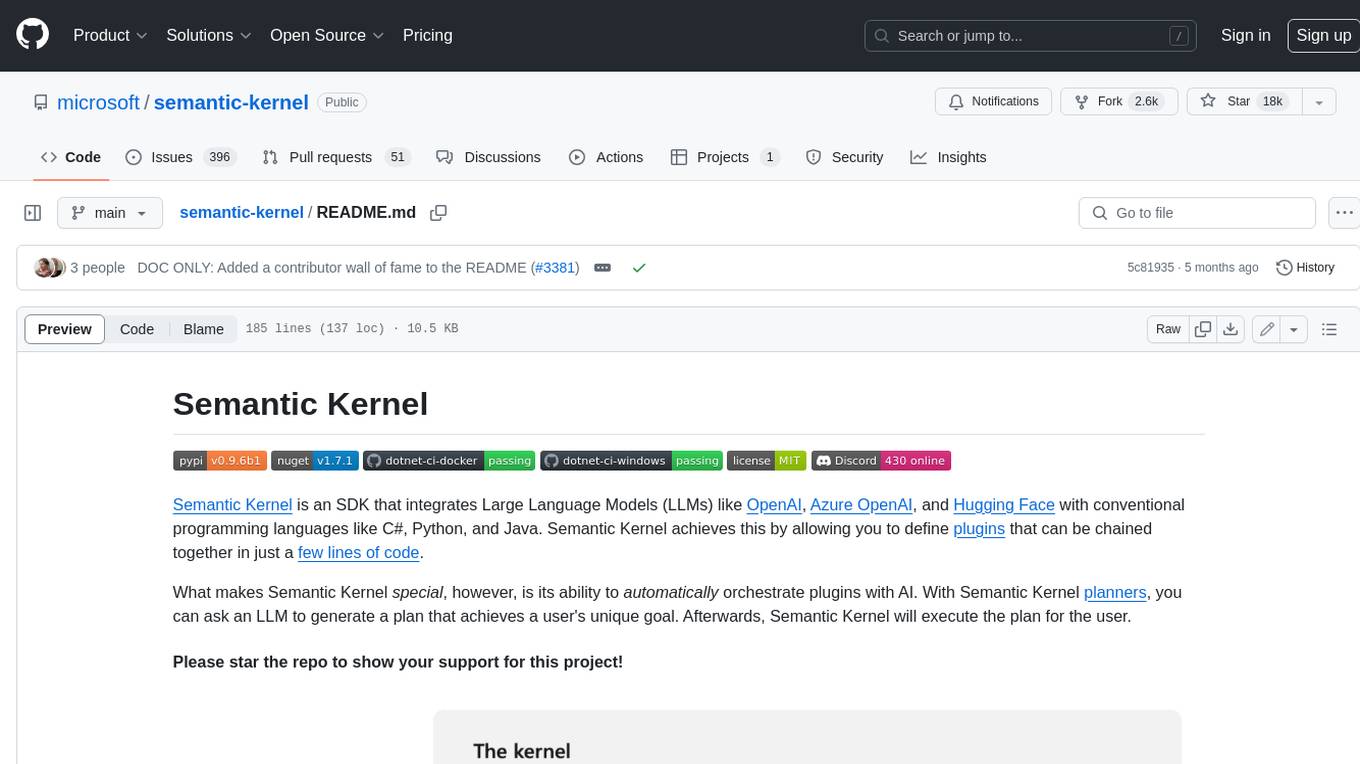
semantic-kernel
Semantic Kernel is an SDK that integrates Large Language Models (LLMs) like OpenAI, Azure OpenAI, and Hugging Face with conventional programming languages like C#, Python, and Java. Semantic Kernel achieves this by allowing you to define plugins that can be chained together in just a few lines of code. What makes Semantic Kernel _special_ , however, is its ability to _automatically_ orchestrate plugins with AI. With Semantic Kernel planners, you can ask an LLM to generate a plan that achieves a user's unique goal. Afterwards, Semantic Kernel will execute the plan for the user.
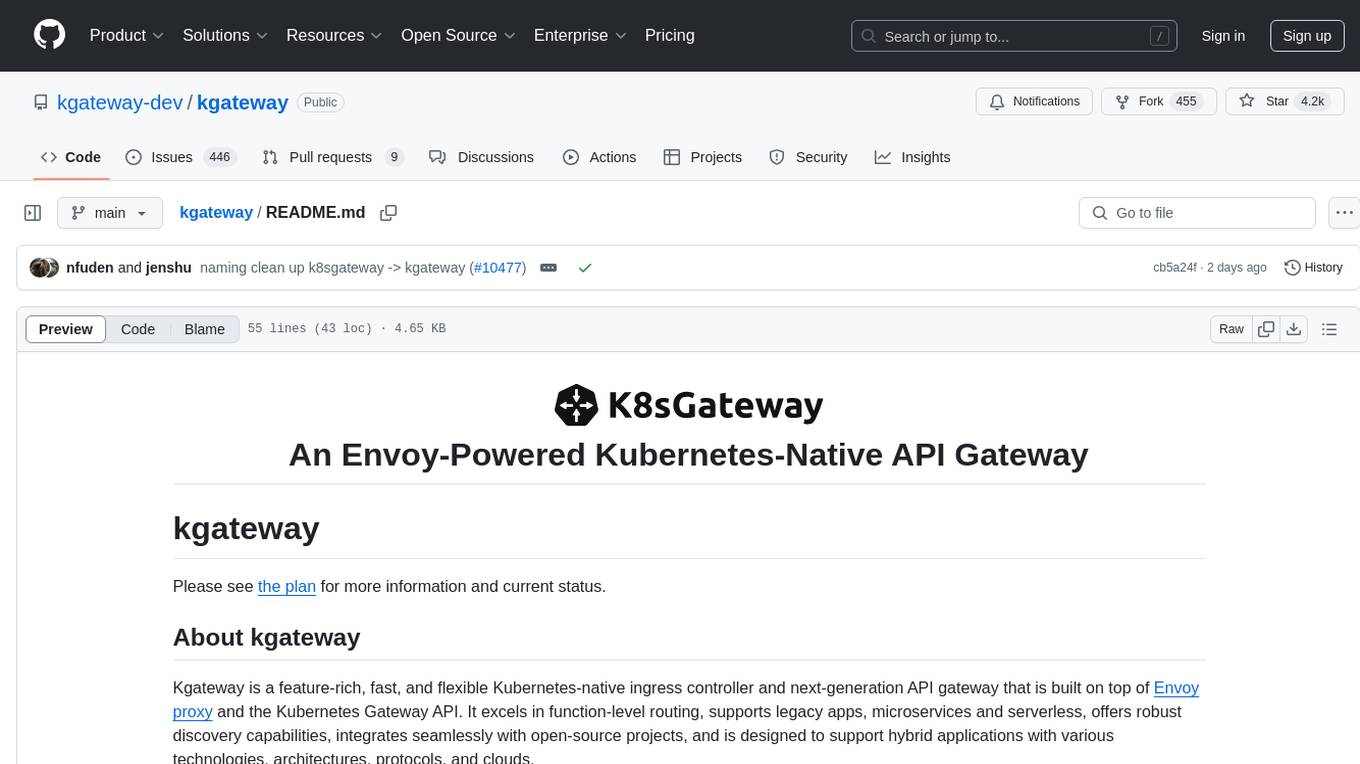
kgateway
Kgateway is a feature-rich, fast, and flexible Kubernetes-native API gateway built on top of Envoy proxy and the Kubernetes Gateway API. It excels in function-level routing, supports legacy apps, microservices, and serverless, offers robust discovery capabilities, integrates seamlessly with open-source projects, and is designed to support hybrid applications with various technologies, architectures, protocols, and clouds.
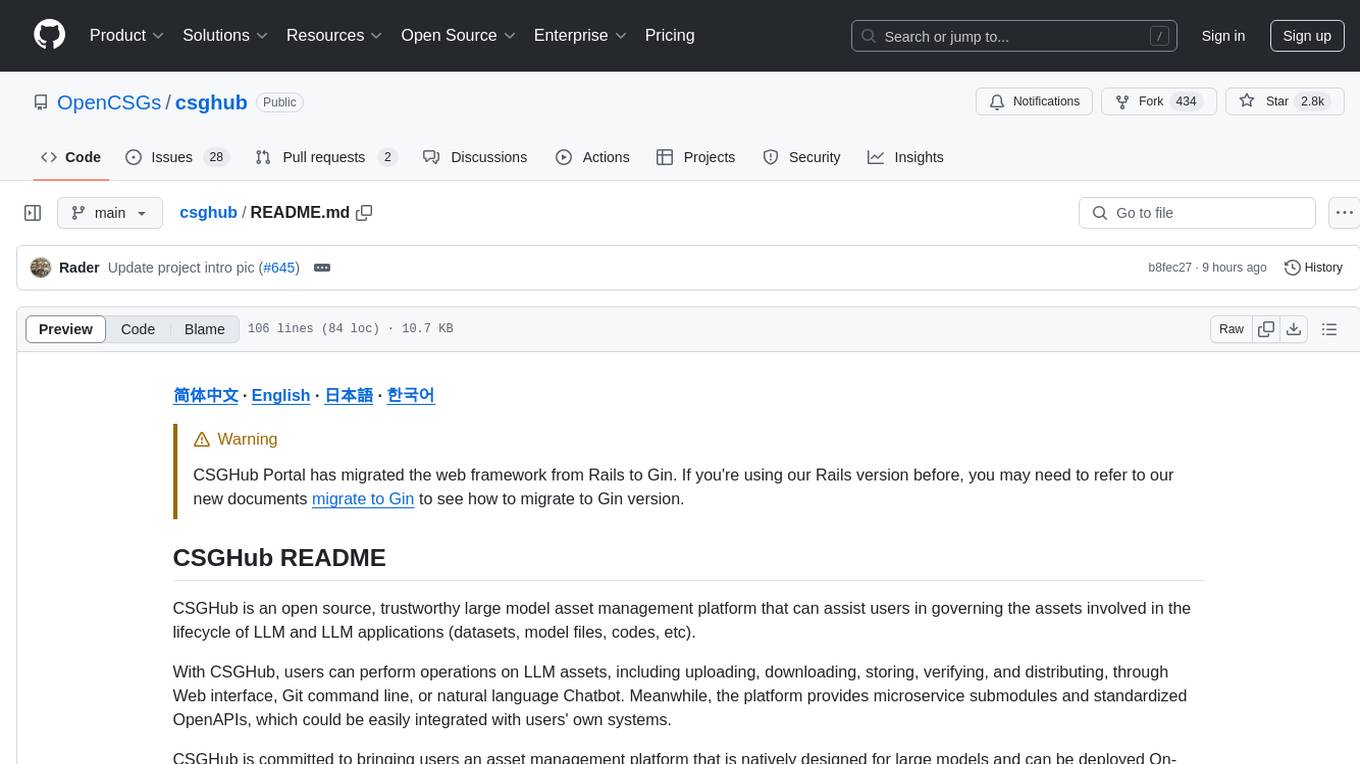
csghub
CSGHub is an open source platform for managing large model assets, including datasets, model files, and codes. It offers functionalities similar to a privatized Huggingface, managing assets in a manner akin to how OpenStack Glance manages virtual machine images. Users can perform operations such as uploading, downloading, storing, verifying, and distributing assets through various interfaces. The platform provides microservice submodules and standardized OpenAPIs for easy integration with users' systems. CSGHub is designed for large models and can be deployed On-Premise for offline operation.
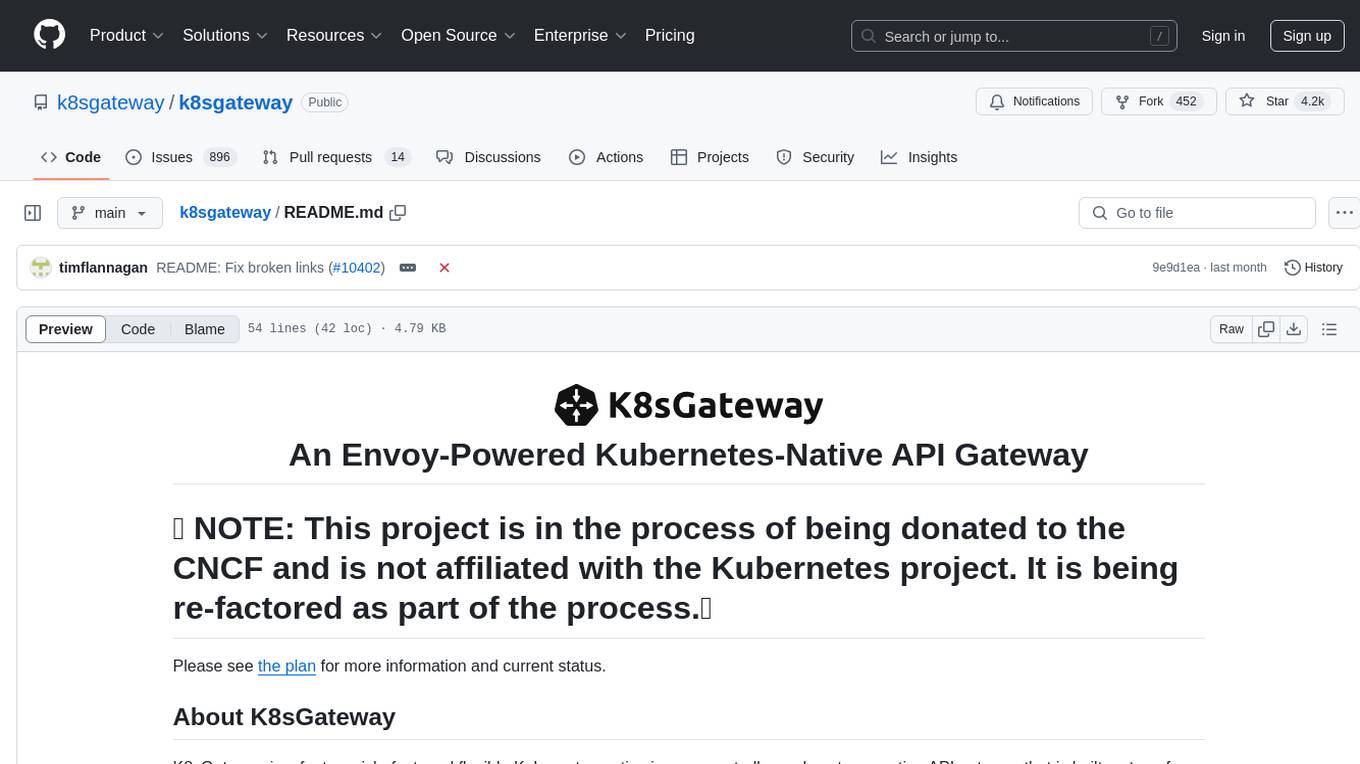
k8sgateway
K8sGateway is a feature-rich, fast, and flexible Kubernetes-native API gateway built on Envoy proxy and Kubernetes Gateway API. It excels in function-level routing, supports legacy apps, microservices, and serverless. It offers robust discovery capabilities, seamless integration with open-source projects, and supports hybrid applications with various technologies, architectures, protocols, and clouds.
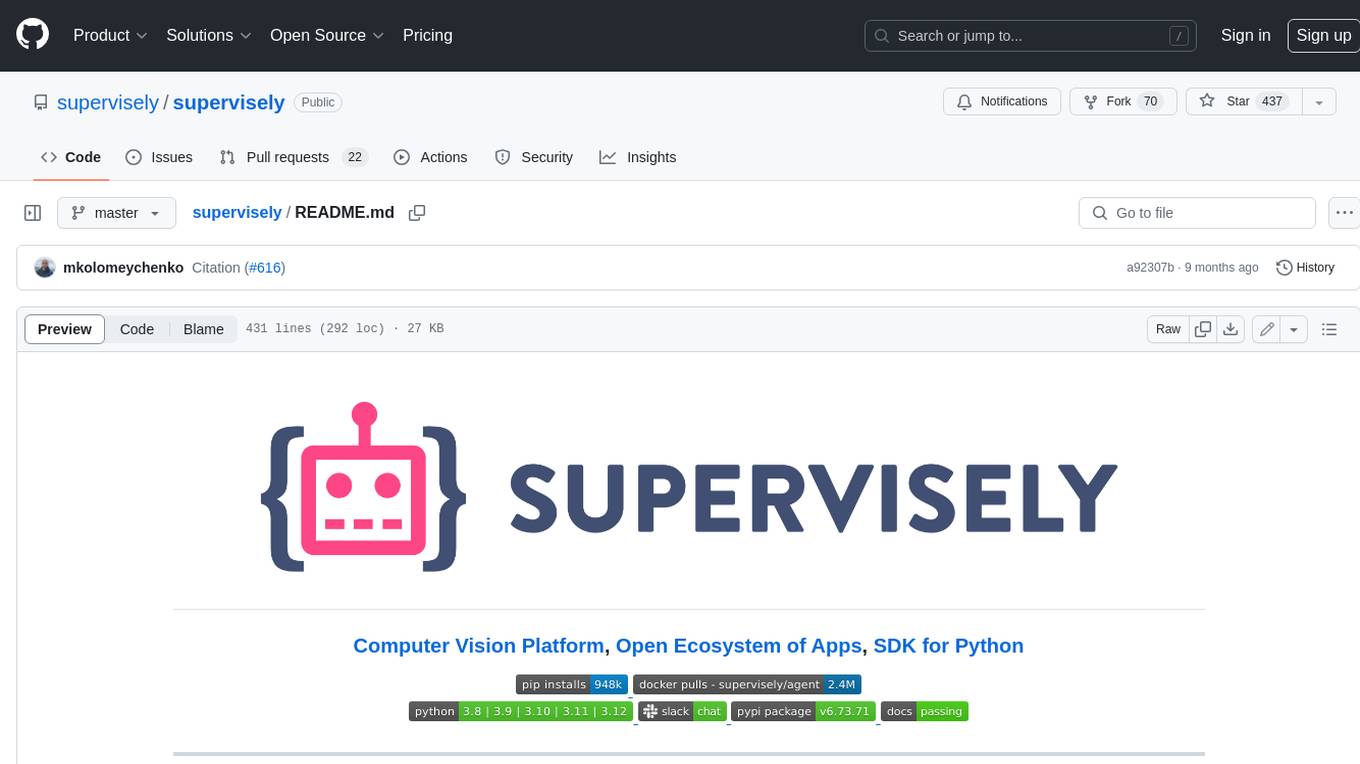
supervisely
Supervisely is a computer vision platform that provides a range of tools and services for developing and deploying computer vision solutions. It includes a data labeling platform, a model training platform, and a marketplace for computer vision apps. Supervisely is used by a variety of organizations, including Fortune 500 companies, research institutions, and government agencies.
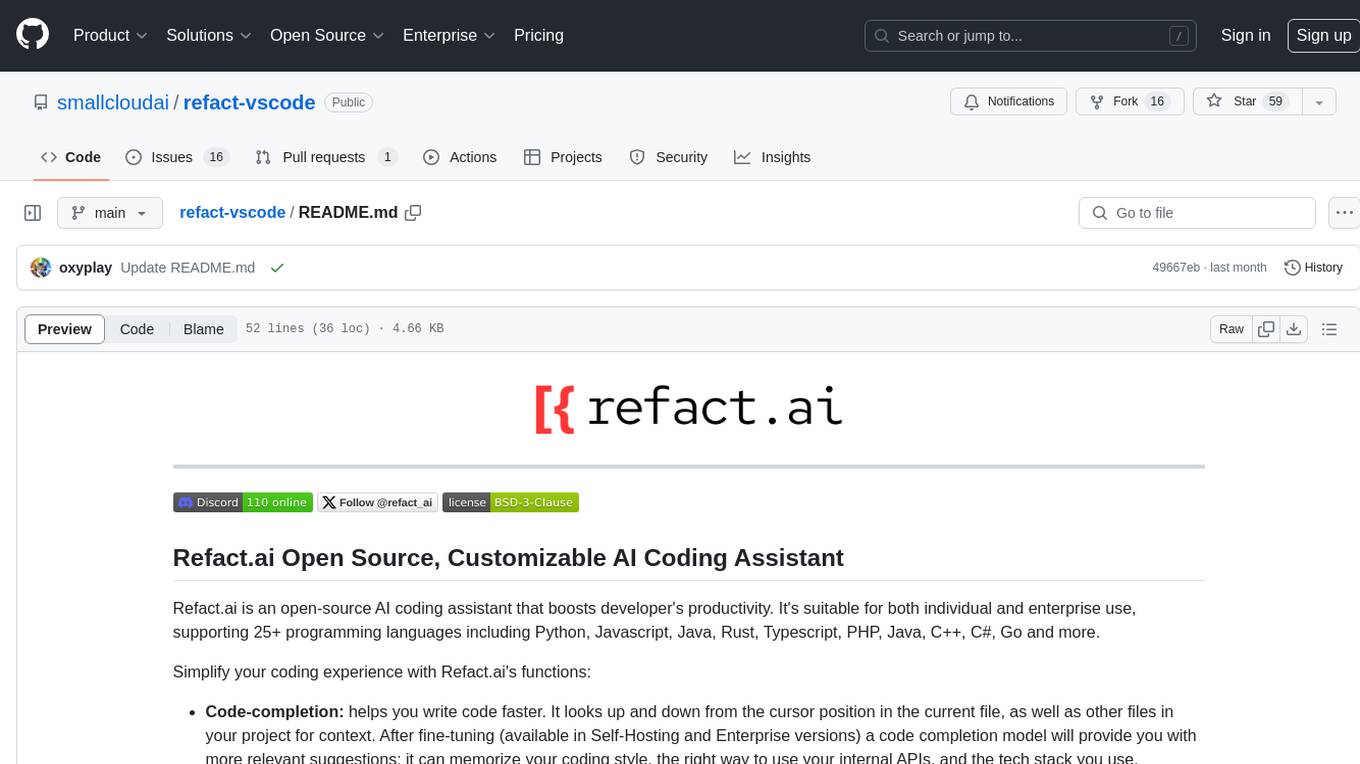
refact-vscode
Refact.ai is an open-source AI coding assistant that boosts developer's productivity. It supports 25+ programming languages and offers features like code completion, AI Toolbox for code explanation and refactoring, integrated in-IDE chat, and self-hosting or cloud version. The Enterprise plan provides enhanced customization, security, fine-tuning, user statistics, efficient inference, priority support, and access to 20+ LLMs for up to 50 engineers per GPU.
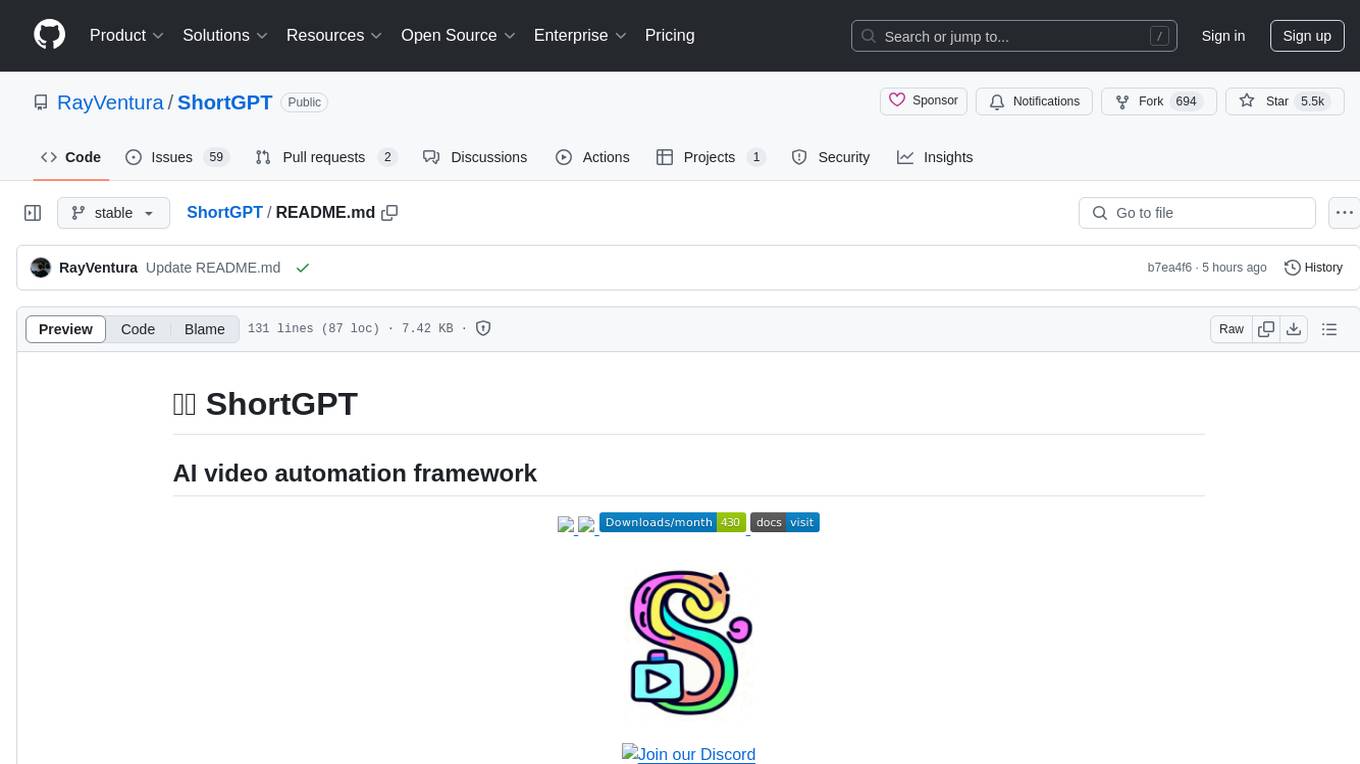
ShortGPT
ShortGPT is a powerful framework for automating content creation, simplifying video creation, footage sourcing, voiceover synthesis, and editing tasks. It offers features like automated editing framework, scripts and prompts, voiceover support in multiple languages, caption generation, asset sourcing, and persistency of editing variables. The tool is designed for youtube automation, Tiktok creativity program automation, and offers customization options for efficient and creative content creation.
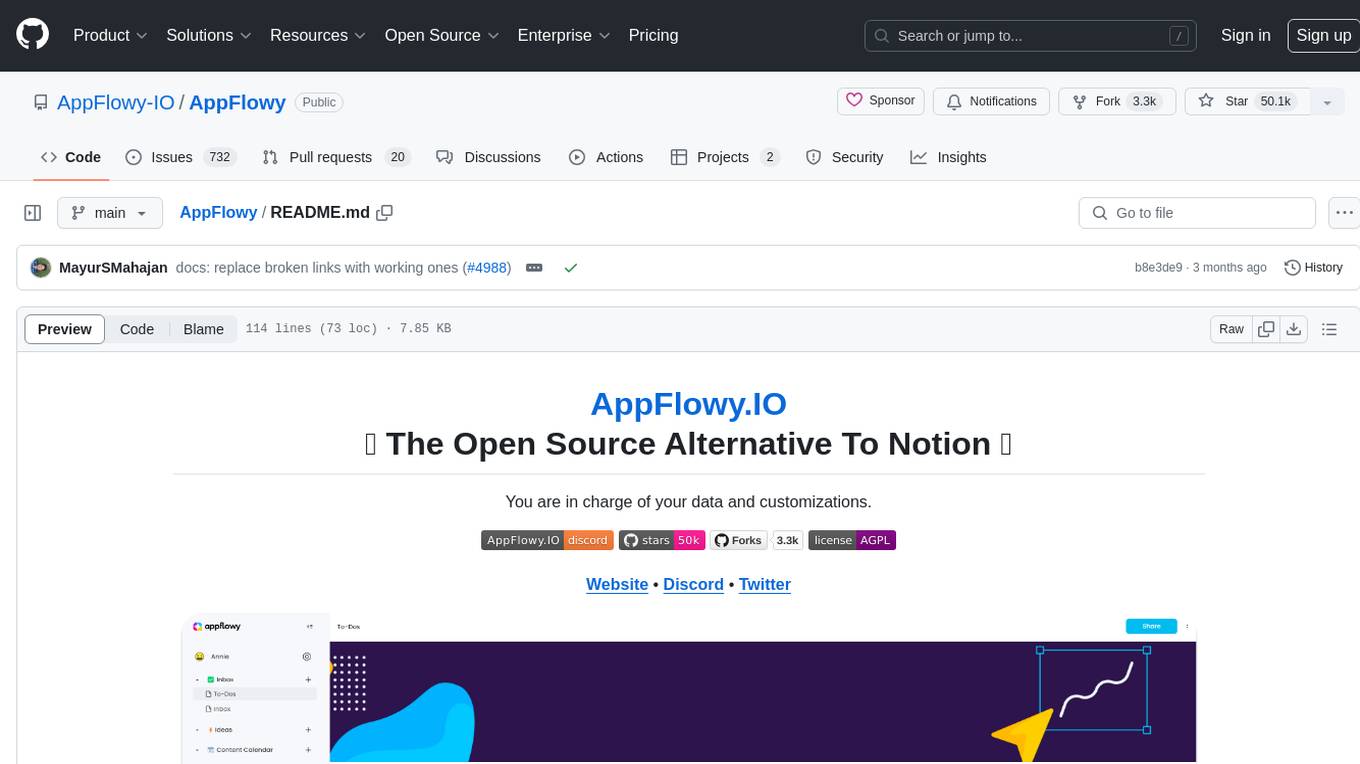
AppFlowy
AppFlowy.IO is an open-source alternative to Notion, providing users with control over their data and customizations. It aims to offer functionality, data security, and cross-platform native experience to individuals, as well as building blocks and collaboration infra services to enterprises and hackers. The tool is built with Flutter and Rust, supporting multiple platforms and emphasizing long-term maintainability. AppFlowy prioritizes data privacy, reliable native experience, and community-driven extensibility, aiming to democratize the creation of complex workplace management tools.
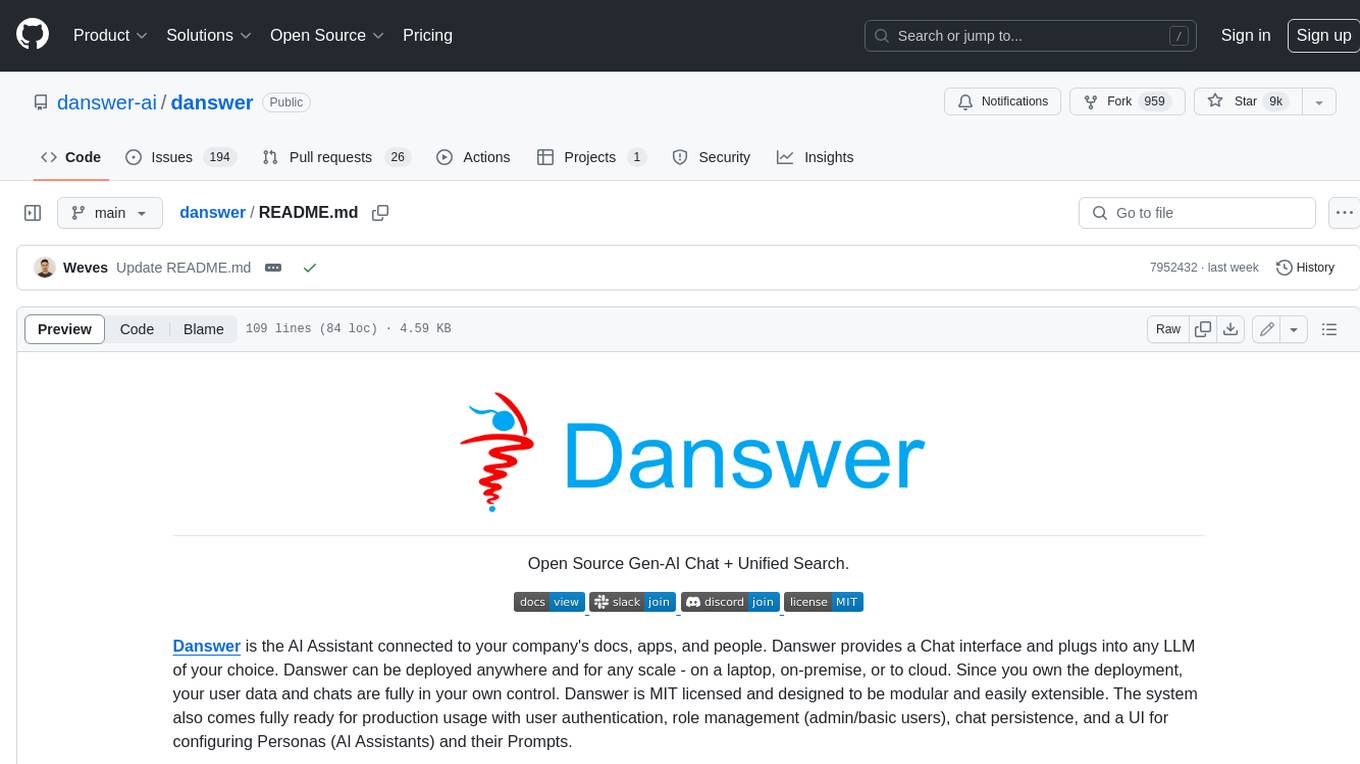
danswer
Danswer is an open-source Gen-AI Chat and Unified Search tool that connects to your company's docs, apps, and people. It provides a Chat interface and plugs into any LLM of your choice. Danswer can be deployed anywhere and for any scale - on a laptop, on-premise, or to cloud. Since you own the deployment, your user data and chats are fully in your own control. Danswer is MIT licensed and designed to be modular and easily extensible. The system also comes fully ready for production usage with user authentication, role management (admin/basic users), chat persistence, and a UI for configuring Personas (AI Assistants) and their Prompts. Danswer also serves as a Unified Search across all common workplace tools such as Slack, Google Drive, Confluence, etc. By combining LLMs and team specific knowledge, Danswer becomes a subject matter expert for the team. Imagine ChatGPT if it had access to your team's unique knowledge! It enables questions such as "A customer wants feature X, is this already supported?" or "Where's the pull request for feature Y?"
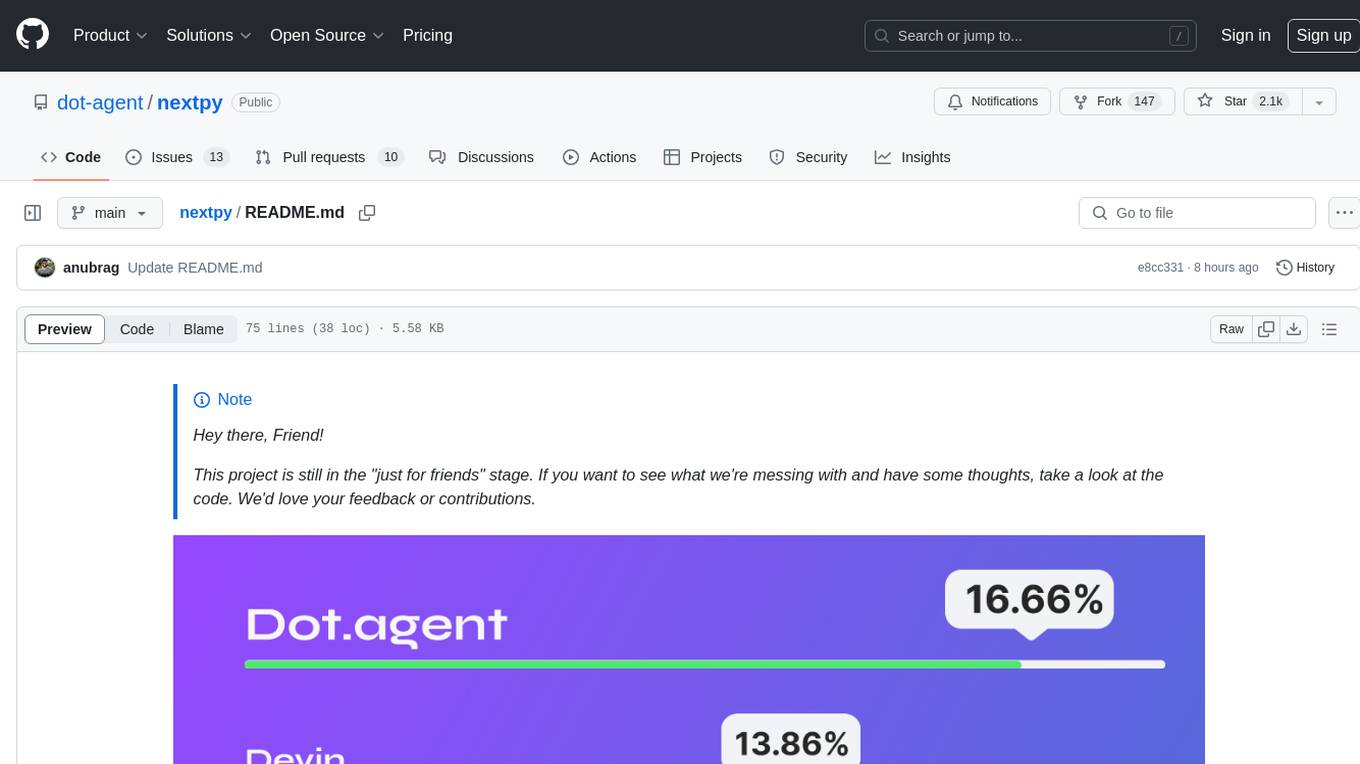
nextpy
Nextpy is a cutting-edge software development framework optimized for AI-based code generation. It provides guardrails for defining AI system boundaries, structured outputs for prompt engineering, a powerful prompt engine for efficient processing, better AI generations with precise output control, modularity for multiplatform and extensible usage, developer-first approach for transferable knowledge, and containerized & scalable deployment options. It offers 4-10x faster performance compared to Streamlit apps, with a focus on cooperation within the open-source community and integration of key components from various projects.
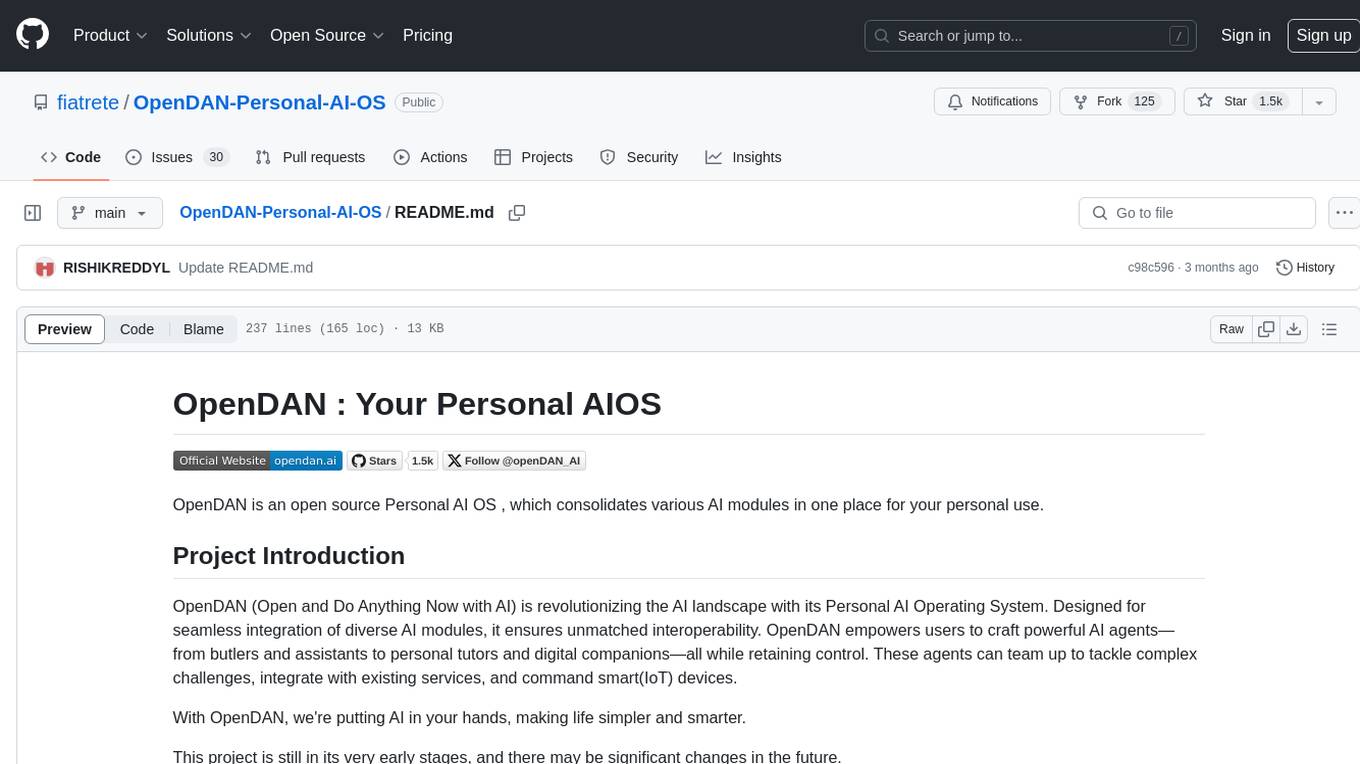
OpenDAN-Personal-AI-OS
OpenDAN is an open source Personal AI OS that consolidates various AI modules for personal use. It empowers users to create powerful AI agents like assistants, tutors, and companions. The OS allows agents to collaborate, integrate with services, and control smart devices. OpenDAN offers features like rapid installation, AI agent customization, connectivity via Telegram/Email, building a local knowledge base, distributed AI computing, and more. It aims to simplify life by putting AI in users' hands. The project is in early stages with ongoing development and future plans for user and kernel mode separation, home IoT device control, and an official OpenDAN SDK release.
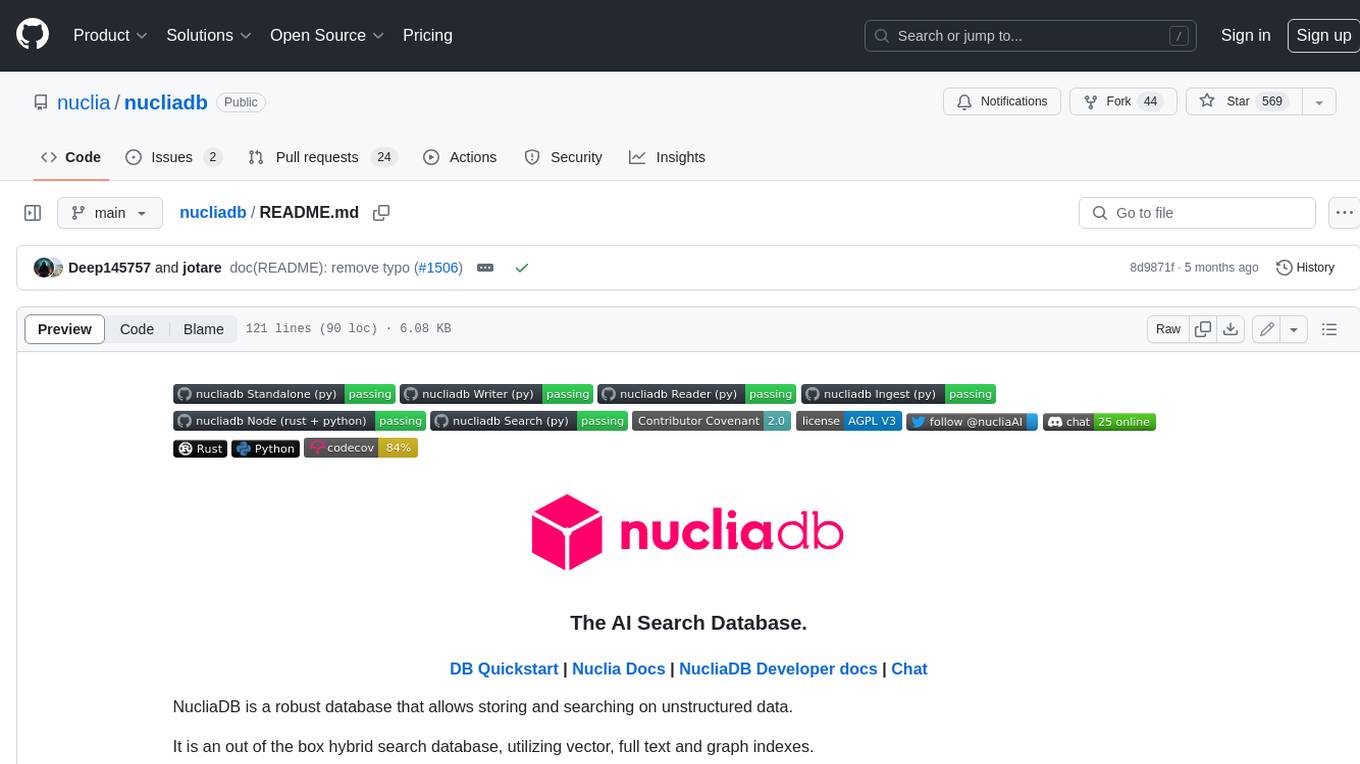
nucliadb
NucliaDB is a robust database that allows storing and searching on unstructured data. It is an out of the box hybrid search database, utilizing vector, full text and graph indexes. NucliaDB is written in Rust and Python. We designed it to index large datasets and provide multi-teanant support. When utilizing NucliaDB with Nuclia cloud, you are able to the power of an NLP database without the hassle of data extraction, enrichment and inference. We do all the hard work for you.
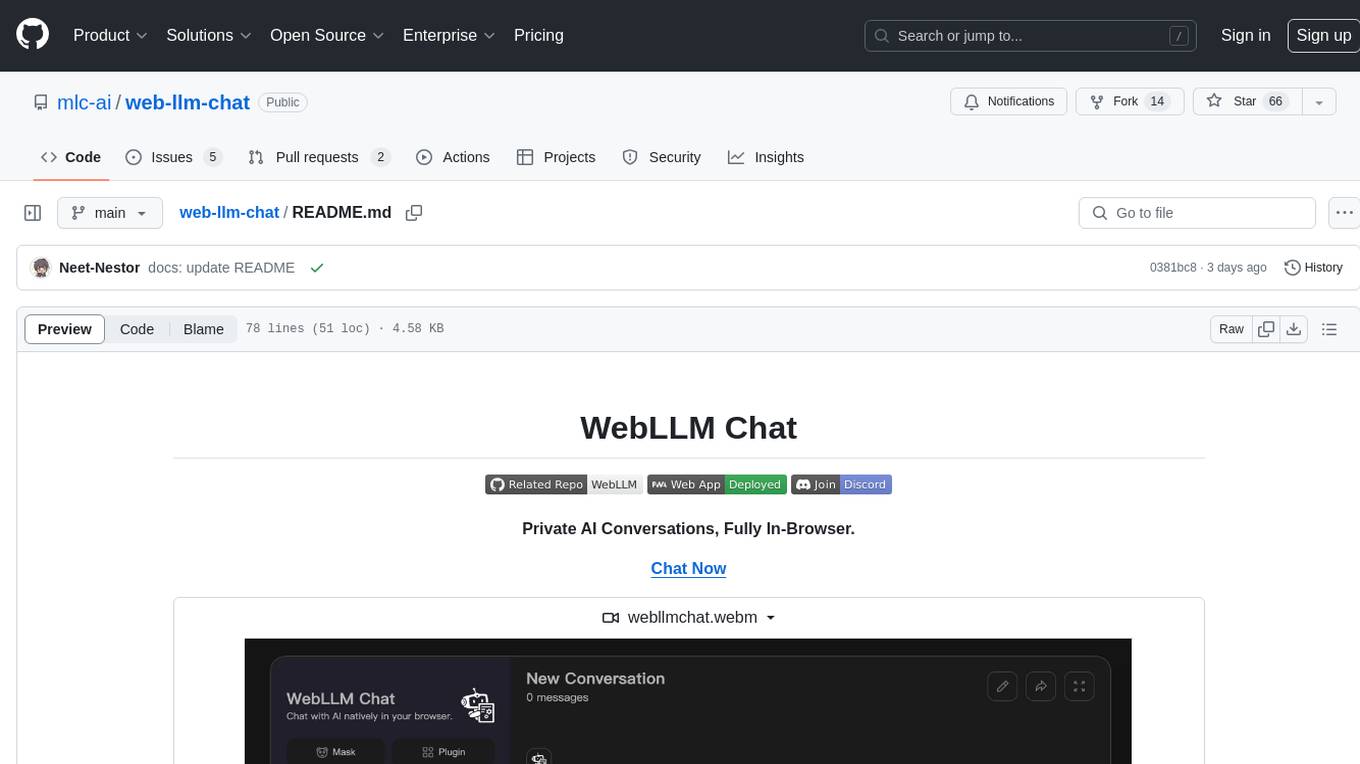
web-llm-chat
WebLLM Chat is a private AI chat interface that combines WebLLM with a user-friendly design, leveraging WebGPU to run large language models natively in your browser. It offers browser-native AI experience with WebGPU acceleration, guaranteed privacy as all data processing happens locally, offline accessibility, user-friendly interface with markdown support, and open-source customization. The project aims to democratize AI technology by making powerful tools accessible directly to end-users, enhancing the chatting experience and broadening the scope for deployment of self-hosted and customizable language models.
For similar tasks
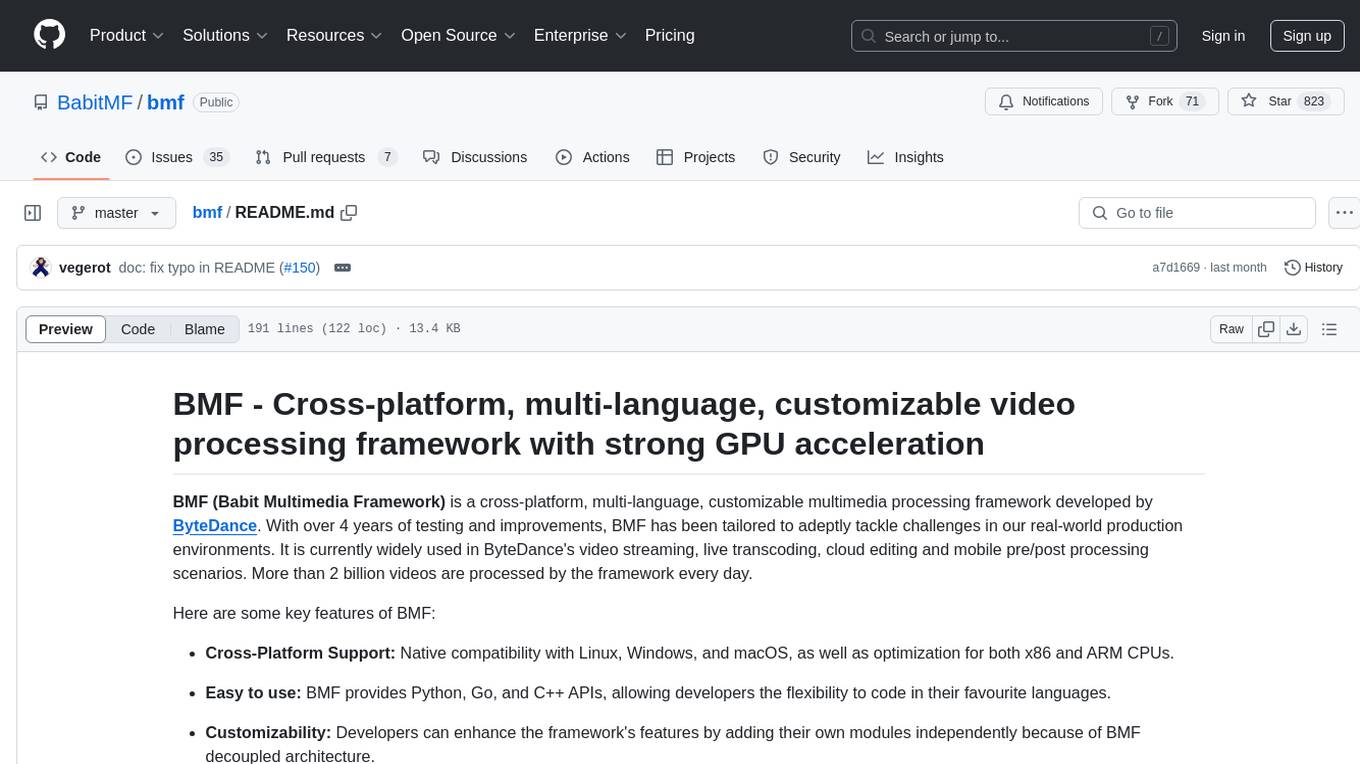
bmf
BMF (Babit Multimedia Framework) is a cross-platform, multi-language, customizable multimedia processing framework developed by ByteDance. It offers native compatibility with Linux, Windows, and macOS, Python, Go, and C++ APIs, and high performance with strong GPU acceleration. BMF allows developers to enhance its features independently and provides efficient data conversion across popular frameworks and hardware devices. BMFLite is a client-side lightweight framework used in apps like Douyin/Xigua, serving over one billion users daily. BMF is widely used in video streaming, live transcoding, cloud editing, and mobile pre/post processing scenarios.
For similar jobs
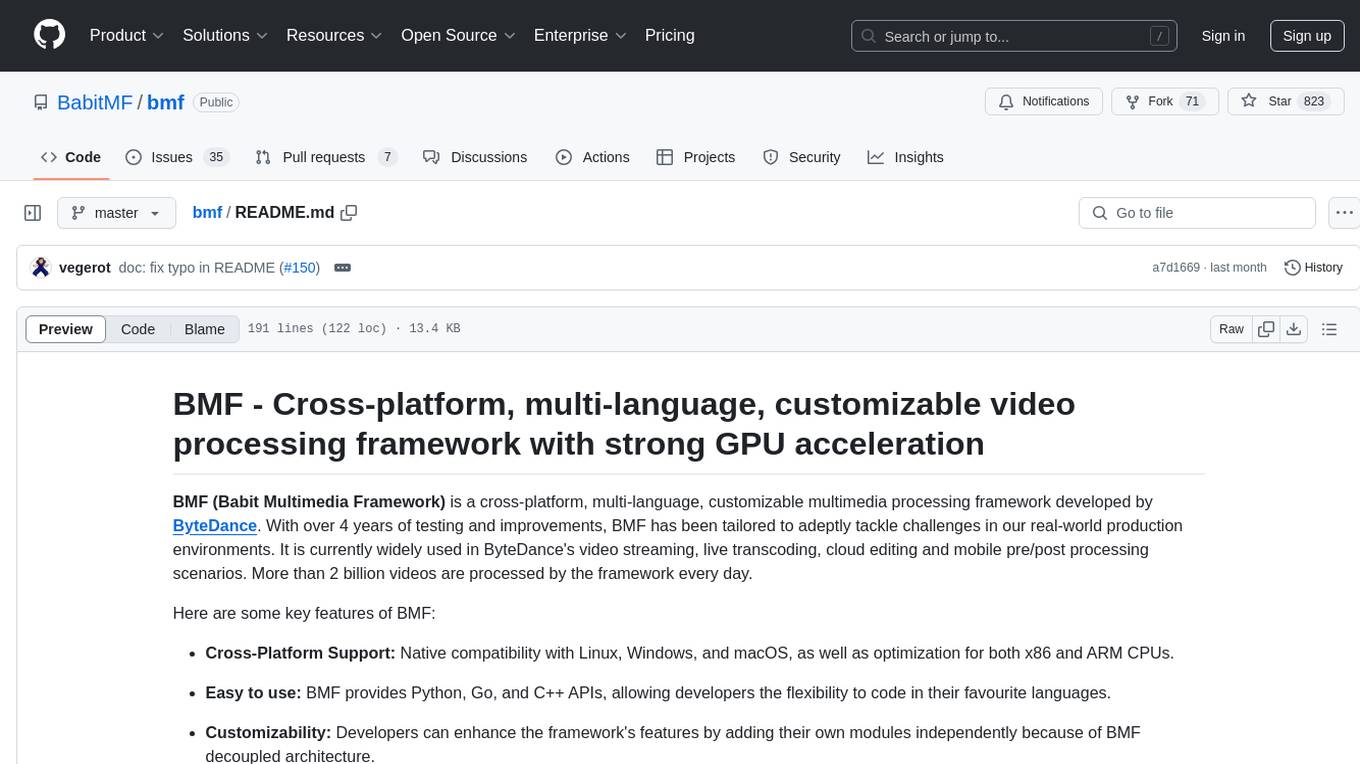
bmf
BMF (Babit Multimedia Framework) is a cross-platform, multi-language, customizable multimedia processing framework developed by ByteDance. It offers native compatibility with Linux, Windows, and macOS, Python, Go, and C++ APIs, and high performance with strong GPU acceleration. BMF allows developers to enhance its features independently and provides efficient data conversion across popular frameworks and hardware devices. BMFLite is a client-side lightweight framework used in apps like Douyin/Xigua, serving over one billion users daily. BMF is widely used in video streaming, live transcoding, cloud editing, and mobile pre/post processing scenarios.