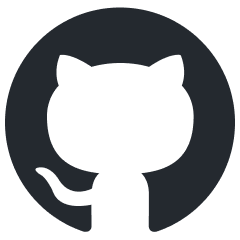
sports-betting
Collection of sports betting AI tools.
Stars: 415
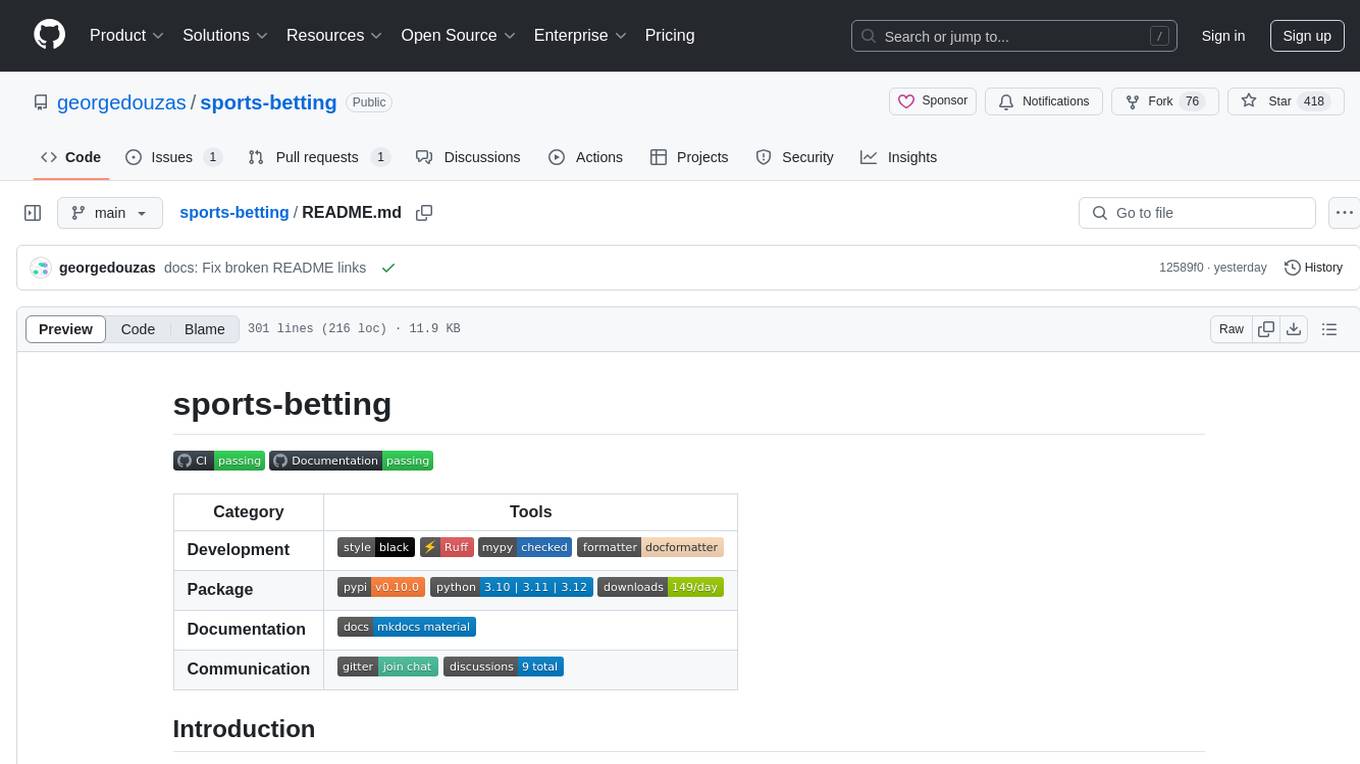
Sports-betting is a Python library for implementing betting strategies and analyzing sports data. It provides tools for collecting, processing, and visualizing sports data to make informed betting decisions. The library includes modules for scraping data from sports websites, calculating odds, simulating betting strategies, and evaluating performance. With sports-betting, users can automate betting processes, test different strategies, and improve their betting outcomes.
README:
Category | Tools |
---|---|
Development |
|
Package |
|
Documentation | |
Communication |
|
The sports-betting package
is a handy set of tools for creating, testing, and using sports betting models. It comes
with a Python API, a CLI, and even a GUI built with Reflex to keep things simple:
The main components of sports-betting
are dataloaders and bettors objects:
- Dataloaders download and prepare data suitable for predictive modelling.
- Bettors provide an easy way to backtest betting strategies and predict the value bets of future events.
sports-betting
comes with a GUI that provides a intuitive way to interact with the library. It supports the following
functionalitites:
- Easily upload, create, or update dataloaders to handle historical and fixtures data.
- Develop and test betting models with tools for backtesting and identifying value bets.
To launch the GUI, simply run the command sportsbet-gui
. Once started, you’ll see the initial screen:
Explore the functionality with guidance from the built-in bot, which streams helpful messages along the way.
The sports-betting
package makes it easy to download sports betting data:
from sportsbet.datasets import SoccerDataLoader
dataloader = SoccerDataLoader(param_grid={'league': ['Italy'], 'year': [2020]})
X_train, Y_train, O_train = dataloader.extract_train_data(odds_type='market_maximum')
X_fix, Y_fix, O_fix = dataloader.extract_fixtures_data()
X_train
are the historical/training data and X_fix
are the test/fixtures data. The historical data can be used to backtest the
performance of a bettor model:
from sportsbet.evaluation import ClassifierBettor, backtest
from sklearn.dummy import DummyClassifier
bettor = ClassifierBettor(DummyClassifier())
backtest(bettor, X_train, Y_train, O_train)
We can use the trained bettor model to predict the value bets using the fixtures data:
bettor.fit(X_train, Y_train)
bettor.bet(X_fix, O_fix)
You can think of any sports betting event as a random experiment with unknown probabilities for the various outcomes. Even for the most unlikely outcome, for example scoring more than 10 goals in a soccer match, a small probability is still assigned. The bookmaker estimates this probability P and offers the corresponding odds O. In theory, if the bookmaker offers the so-called fair odds O = 1 / P in the long run, neither the bettor nor the bookmaker would make any money.
The bookmaker's strategy is to adjust the odds in their favor using the over-round of probabilities. In practice, it offers odds less than the estimated fair odds. The important point here is that the bookmaker still has to estimate the probabilities of outcomes and provide odds that guarantee them long-term profit.
On the other hand, the bettor can also estimate the probabilities and compare them to the odds the bookmaker offers. If the estimated probability of an outcome is higher than the implied probability from the provided odds, then the bet is called a value bet.
The only long-term betting strategy that makes sense is to select value bets. However, you have to remember that neither the bettor nor the bookmaker can access the actual probabilities of outcomes. Therefore, identifying a value bet from the side of the bettor is still an estimation. The bettor or the bookmaker might be wrong, or both of them.
Another essential point is that bookmakers can access resources that the typical bettor is rare to access. For instance, they have
more data, computational power, and teams of experts working on predictive models. You may assume that trying to beat them is
pointless, but this is not necessarily correct. The bookmakers have multiple factors to consider when they offer their adjusted
odds. This is the reason there is a considerable variation among the offered odds. The bettor should aim to systematically
estimate the value bets, backtest their performance, and not create arbitrarily accurate predictive models. This is a realistic
goal, and sports-betting
can help by providing appropriate tools.
For user installation, sports-betting
is currently available on the PyPi's repository, and you can install it via pip
:
pip install sports-betting
If you have Node.js version v22.0.0 or higher, you can optionally install the GUI:
pip install sports-betting[gui]
Development installation requires to clone the repository and then use PDM to install the project as well as the main and development dependencies:
git clone https://github.com/georgedouzas/sports-betting.git
cd sports-betting
pdm install
You can access sports-betting
through the GUI application, the Python API, or the CLI. However, it’s a good idea to
get familiar with the Python API since you’ll need it to create configuration files for the CLI or load custom betting
models into the GUI. sports-betting
supports all common sports betting needs i.e. fetching historical and fixtures
data as well as backtesting of betting strategies and prediction of value bets.
Launch the GUI app with the command sportsbet-gui
.
Here are a few things you can do with the GUI:
- Configure the dataloader:
- Create a new betting model:
Run the model to get predictions:
Assume we would like to backtest the following scenario and use the bettor object to predict value bets:
- Selection of data
- First and second division of German, Italian and French leagues for the years 2021-2024
- Maximum odds of the market in order to backtest our betting strategy
- Configuration of betting strategy
- 5-fold time ordered cross-validation
- Initial cash of 10000 euros
- Stake of 50 euros for each bet
- Use match odds (home win, away win and draw) as betting markets
- Logistic regression classifier to predict probabilities and value bets
# Selection of data
from sportsbet.datasets import SoccerDataLoader
leagues = ['Germany', 'Italy', 'France']
divisions = [1, 2]
years = [2021, 2022, 2023, 2024]
odds_type = 'market_maximum'
dataloader = SoccerDataLoader({'league': leagues, 'year': years, 'division': divisions})
X_train, Y_train, O_train = dataloader.extract_train_data(odds_type=odds_type)
X_fix, _, O_fix = dataloader.extract_fixtures_data()
# Configuration of betting strategy
from sklearn.model_selection import TimeSeriesSplit
from sklearn.compose import make_column_transformer
from sklearn.linear_model import LogisticRegression
from sklearn.impute import SimpleImputer
from sklearn.pipeline import make_pipeline
from sklearn.preprocessing import OneHotEncoder
from sklearn.multioutput import MultiOutputClassifier
from sportsbet.evaluation import ClassifierBettor, backtest
tscv = TimeSeriesSplit(5)
init_cash = 10000.0
stake = 50.0
betting_markets = ['home_win__full_time_goals', 'draw__full_time_goals', 'away_win__full_time_goals']
classifier = make_pipeline(
make_column_transformer(
(OneHotEncoder(handle_unknown='ignore'), ['league', 'home_team', 'away_team']), remainder='passthrough'
),
SimpleImputer(),
MultiOutputClassifier(LogisticRegression(solver='liblinear', random_state=7, class_weight='balanced', C=50)),
)
bettor = ClassifierBettor(classifier, betting_markets=betting_markets, stake=stake, init_cash=init_cash)
# Apply backtesting and get results
backtesting_results = backtest(bettor, X_train, Y_train, O_train, cv=tscv)
# Get value bets for upcoming betting events
bettor.fit(X_train, Y_train)
bettor.bet(X_fix, O_fix)
The command sportsbet
provides various sub-commands to download data and predict the value bets. For any sub-command you may
add the --help
flag to get more information about its usage.
In order to use the commands, a configuration file is required. You can find examples of such configuration files in
sports-betting/configs/
. The configuration file should have a Python file extension and contain a few variables. The variables
DATALOADER_CLASS
and PARAM_GRID
are mandatory while the rest are optional.
The following variables configure the data extraction:
-
DATALOADER_CLASS
: The dataloader class to use. -
PARAM_GRID
: The parameters grid to select the type of information that the data includes. -
DROP_NA_THRES
: The parameterdrop_na_thres
of the dataloader'sextract_train_data
. -
ODDS_TYPE
: The parameterodds_type
of the dataloader'sextract_train_data
.
The following variables configure the betting process:
-
BETTOR
: A bettor object. -
CV
: The parametercv
of the functionbacktest
. -
N_JOBS
: The parametern_jobs
of the functionbacktest
. -
VERBOSE
: The parameterverbose
of the functionbacktest
.
Once these variables are provided, we can select the appropriate commands to select any of the sports-betting
's functionalities.
Show available parameters for dataloaders:
sportsbet dataloader params -c config.py
Show available odds types:
sportsbet dataloader odds-types -c config.py
Extract training data and save them as CSV files:
sportsbet dataloader training -c config.py -d /path/to/directory
Extract fixtures data and save them as CSV files:
sportsbet dataloader fixtures -c config.py -d /path/to/directory
Backtest the bettor and save the results as CSV file:
sportsbet bettor backtest -c config.py -d /path/to/directory
Get the value bets and save them as CSV file:
sportsbet bettor bet -c config.py -d /path/to/directory
For Tasks:
Click tags to check more tools for each tasksFor Jobs:
Alternative AI tools for sports-betting
Similar Open Source Tools
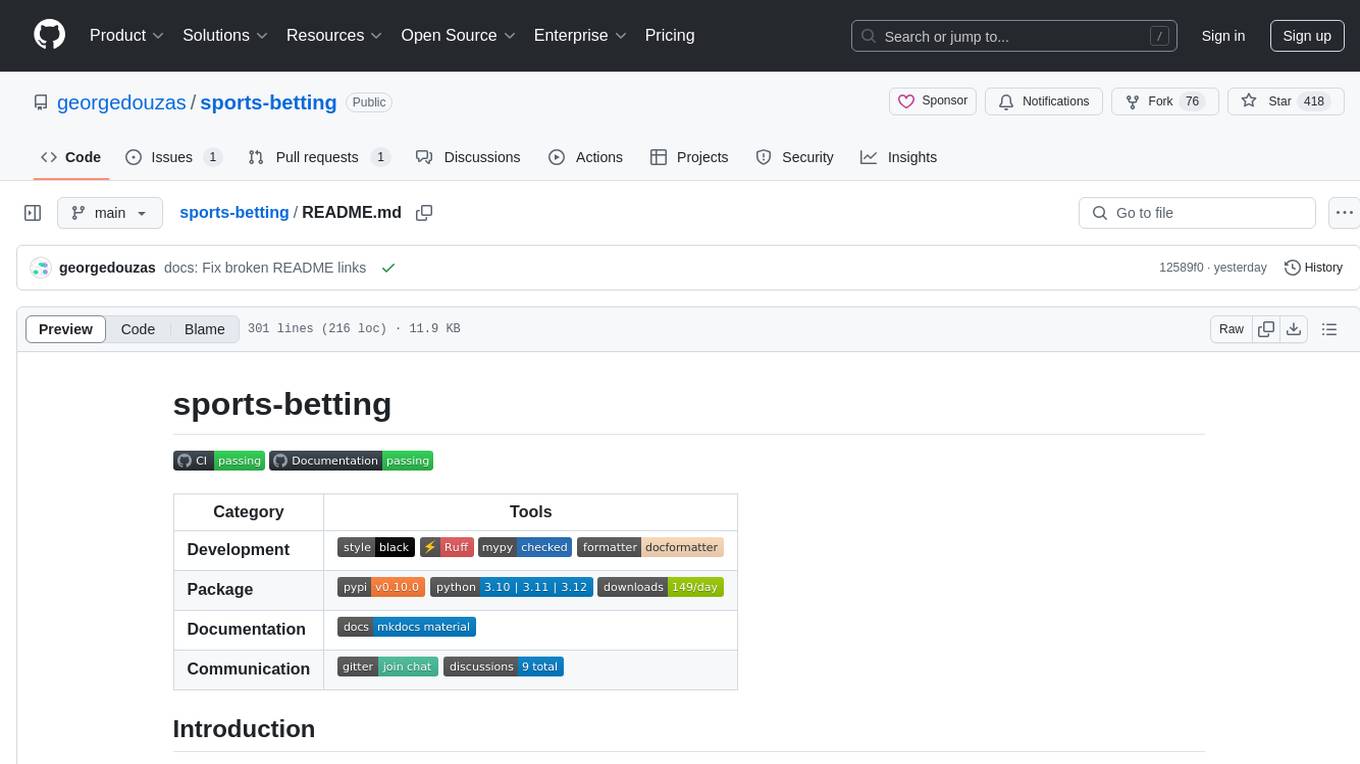
sports-betting
Sports-betting is a Python library for implementing betting strategies and analyzing sports data. It provides tools for collecting, processing, and visualizing sports data to make informed betting decisions. The library includes modules for scraping data from sports websites, calculating odds, simulating betting strategies, and evaluating performance. With sports-betting, users can automate betting processes, test different strategies, and improve their betting outcomes.
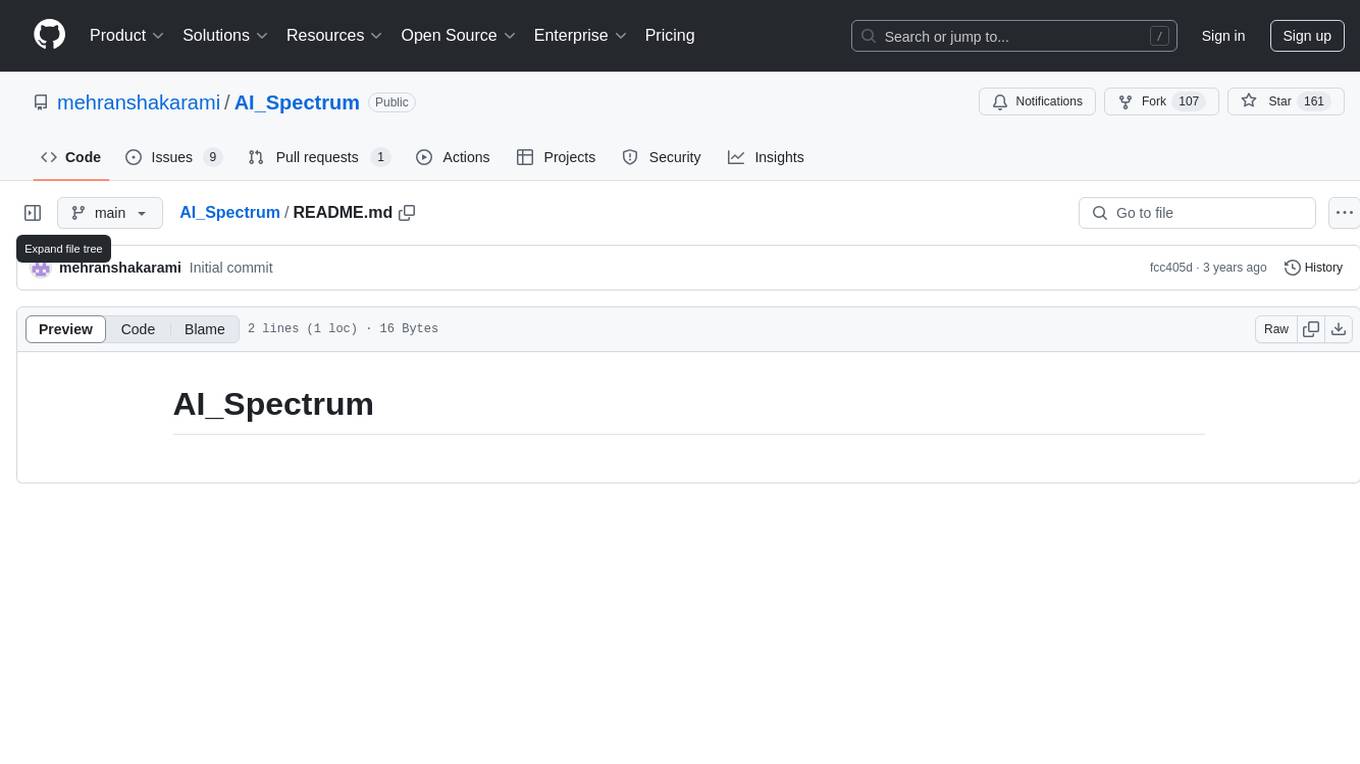
AI_Spectrum
AI_Spectrum is a versatile machine learning library that provides a wide range of tools and algorithms for building and deploying AI models. It offers a user-friendly interface for data preprocessing, model training, and evaluation. With AI_Spectrum, users can easily experiment with different machine learning techniques and optimize their models for various tasks. The library is designed to be flexible and scalable, making it suitable for both beginners and experienced data scientists.
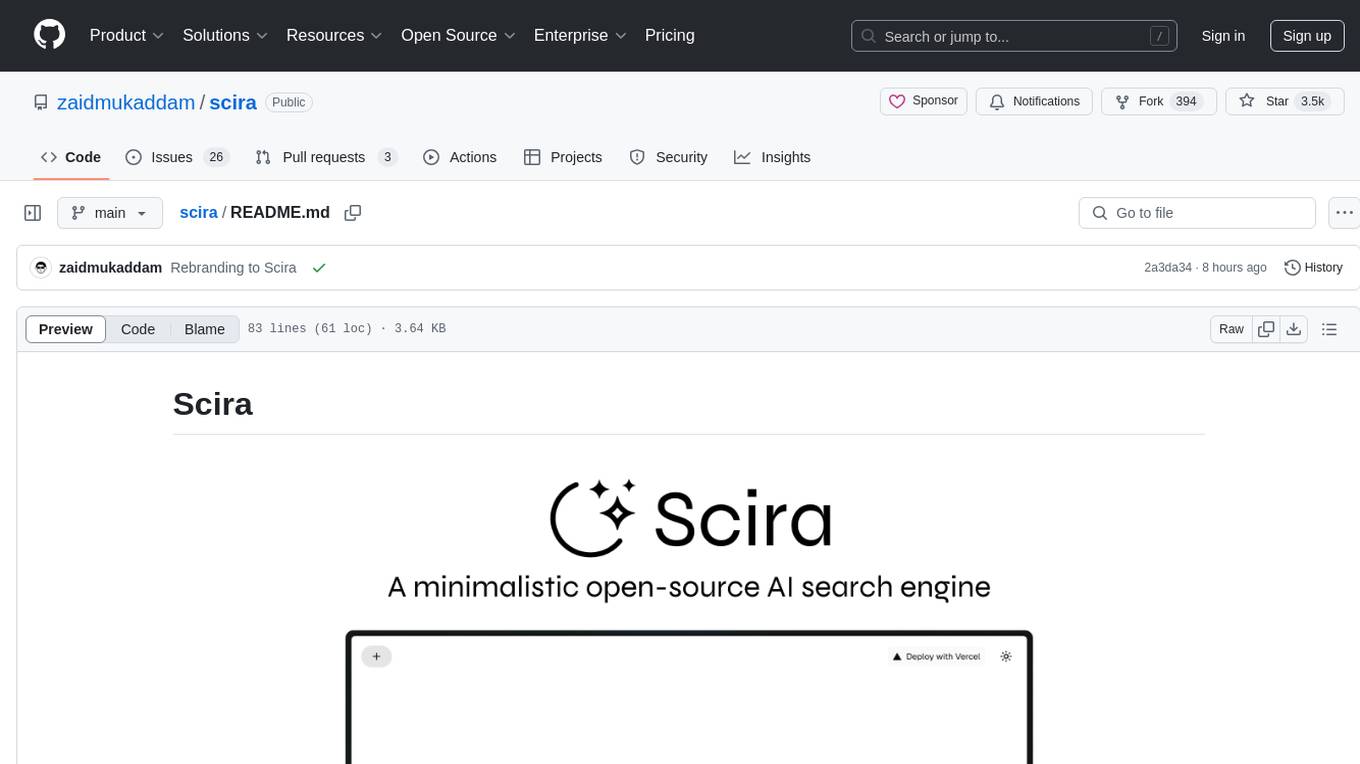
scira
Scira is a powerful open-source tool for analyzing and visualizing data. It provides a user-friendly interface for data exploration, cleaning, and modeling. With Scira, users can easily import datasets, perform statistical analysis, create insightful visualizations, and generate reports. The tool supports various data formats and offers a wide range of statistical functions and visualization options. Whether you are a data scientist, researcher, or student, Scira can help you uncover valuable insights from your data and communicate your findings effectively.
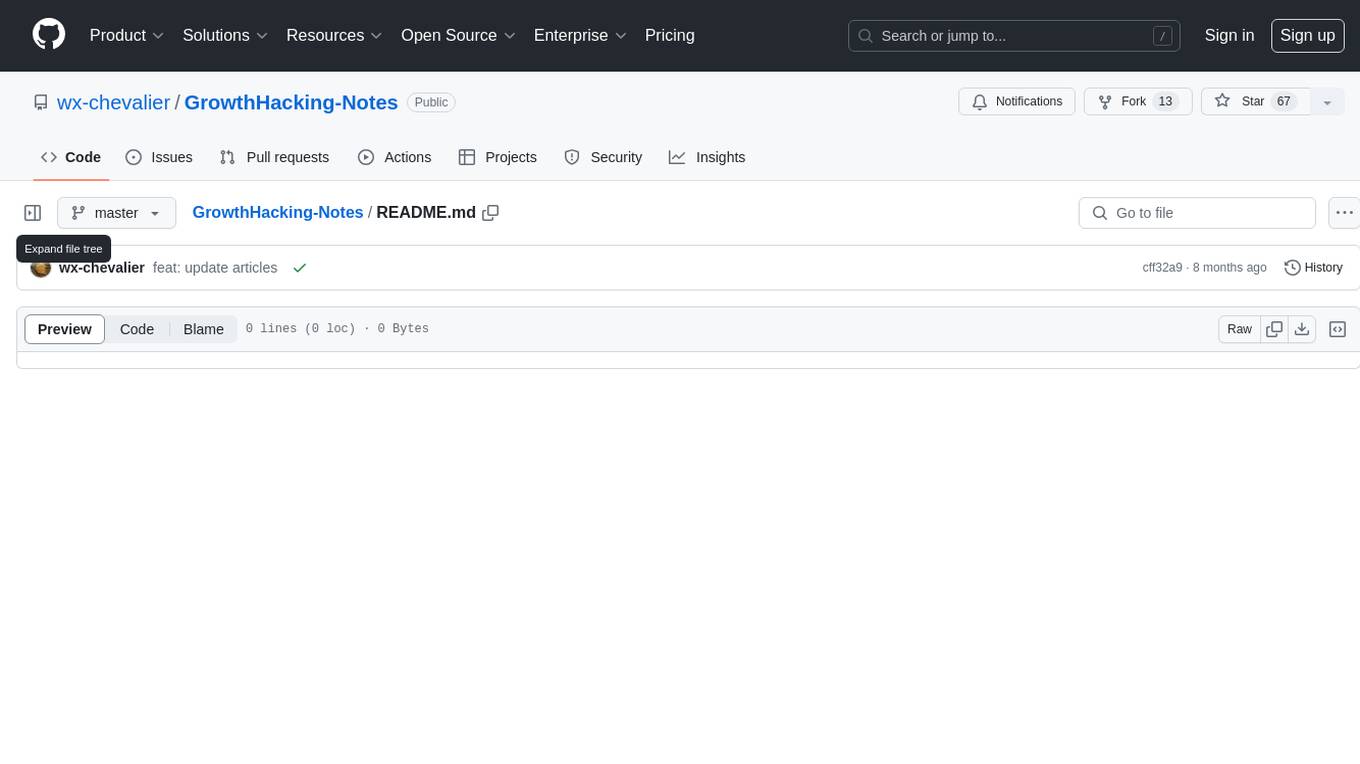
GrowthHacking-Notes
GrowthHacking-Notes is a repository containing detailed notes, strategies, and resources related to growth hacking. It provides valuable insights and tips for individuals and businesses looking to accelerate their growth through innovative marketing techniques and data-driven strategies. The repository covers various topics such as user acquisition, retention, conversion optimization, and more, making it a comprehensive resource for anyone interested in growth hacking.
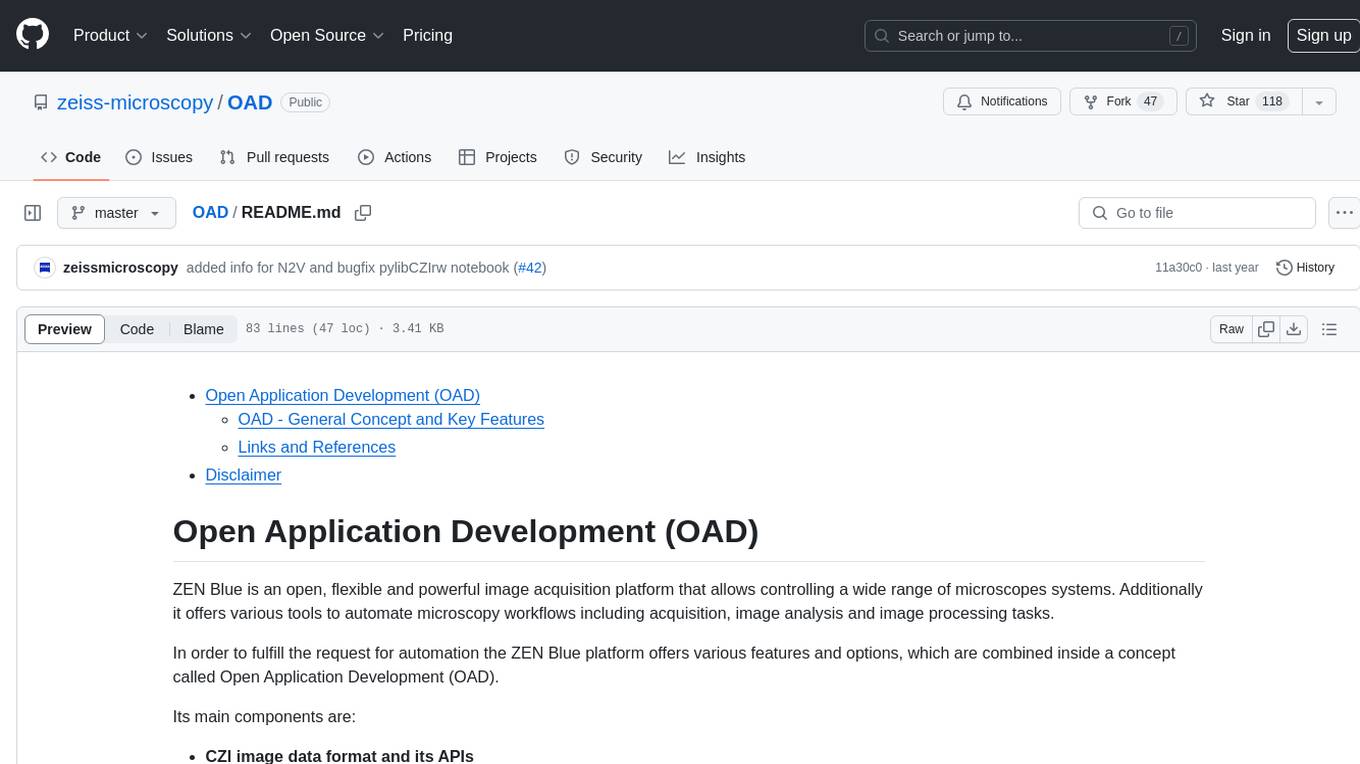
OAD
OAD is a powerful open-source tool for analyzing and visualizing data. It provides a user-friendly interface for exploring datasets, generating insights, and creating interactive visualizations. With OAD, users can easily import data from various sources, clean and preprocess data, perform statistical analysis, and create customizable visualizations to communicate findings effectively. Whether you are a data scientist, analyst, or researcher, OAD can help you streamline your data analysis workflow and uncover valuable insights from your data.
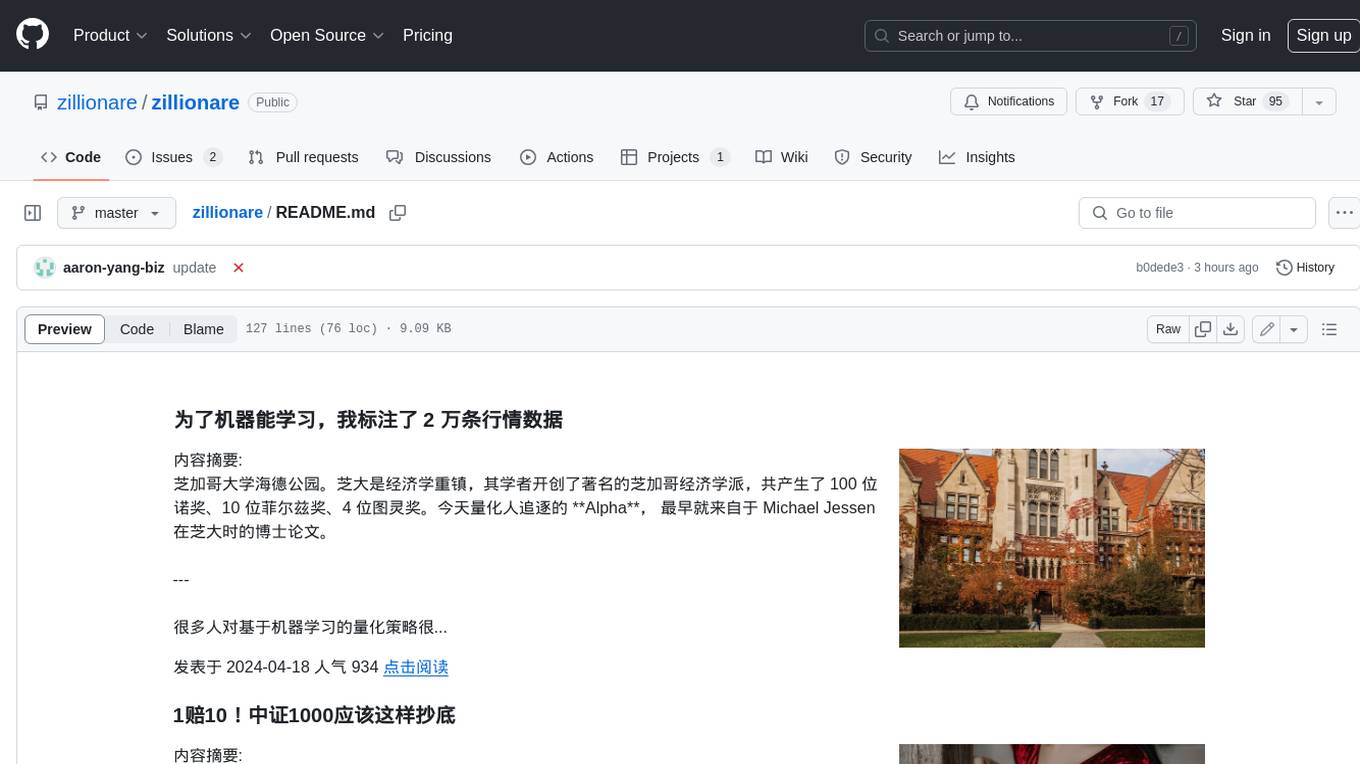
zillionare
This repository contains a collection of articles and tutorials on quantitative finance, including topics such as machine learning, statistical arbitrage, and risk management. The articles are written in a clear and concise style, and they are suitable for both beginners and experienced practitioners. The repository also includes a number of Jupyter notebooks that demonstrate how to use Python for quantitative finance.
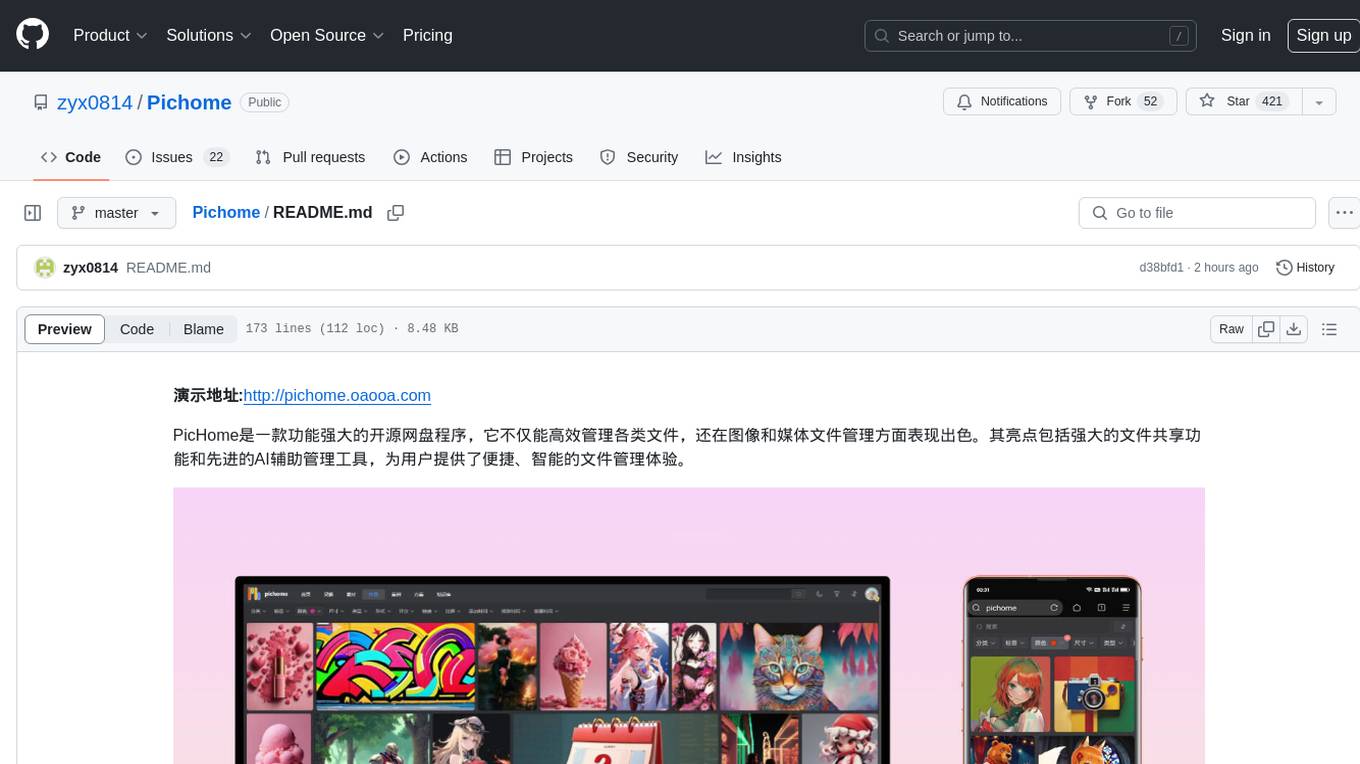
Pichome
PicHome is a powerful open-source cloud storage program that efficiently manages various types of files and excels in image and media file management. Its highlights include robust file sharing features and advanced AI-assisted management tools, providing users with a convenient and intelligent file management experience. The program offers diverse list modes, customizable file information display, enhanced quick file preview, advanced tagging, custom cover and preview images, multiple preview images, and multi-library management. Additionally, PicHome features strong file sharing capabilities, allowing users to share entire libraries, create personalized showcase web pages, and build complete data sharing websites. The AI-assisted management aspect includes AI file renaming, tagging, description writing, batch annotation, and file Q&A services, all aimed at improving file management efficiency. PicHome supports a wide range of file formats and can be applied in various scenarios such as e-commerce, gaming, design, development, enterprises, schools, labs, media, and entertainment institutions.
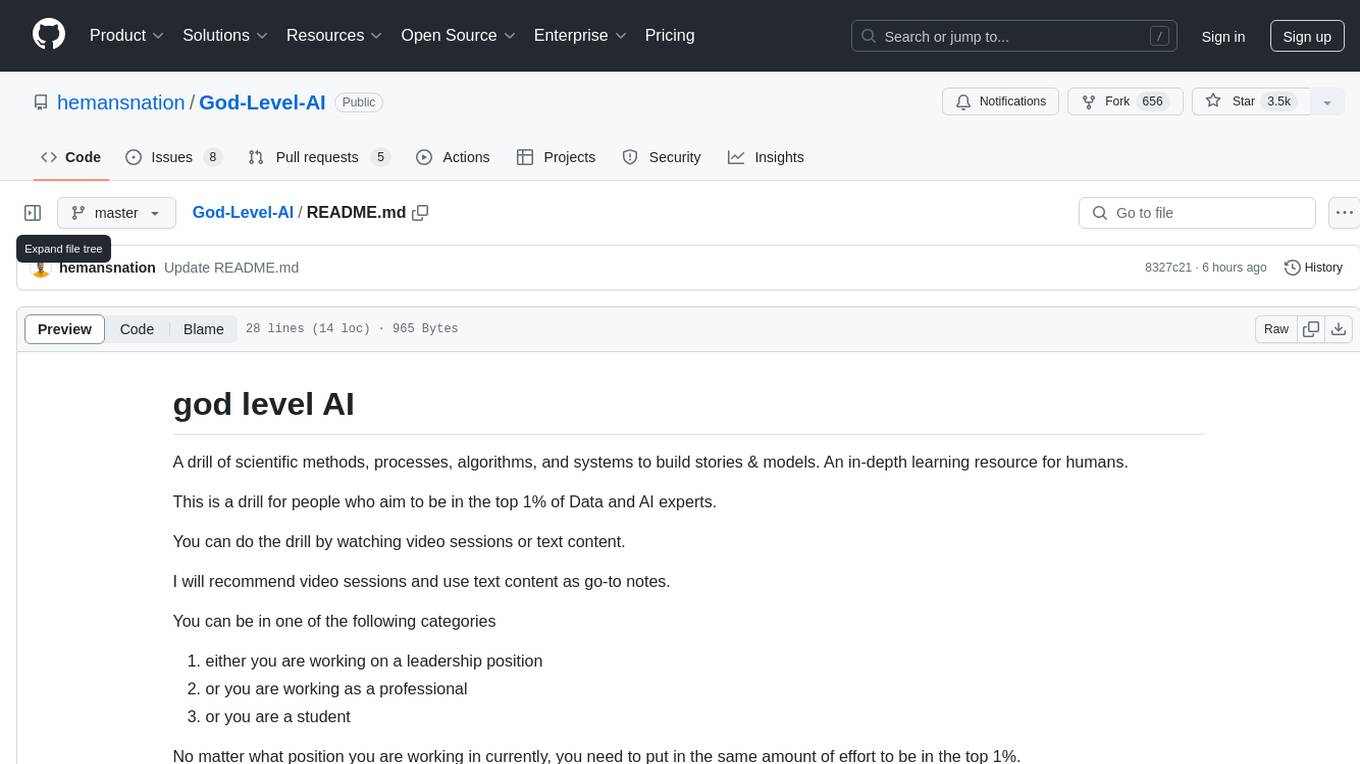
God-Level-AI
A drill of scientific methods, processes, algorithms, and systems to build stories & models. An in-depth learning resource for humans. This repository is designed for individuals aiming to excel in the field of Data and AI, providing video sessions and text content for learning. It caters to those in leadership positions, professionals, and students, emphasizing the need for dedicated effort to achieve excellence in the tech field. The content covers various topics with a focus on practical application.
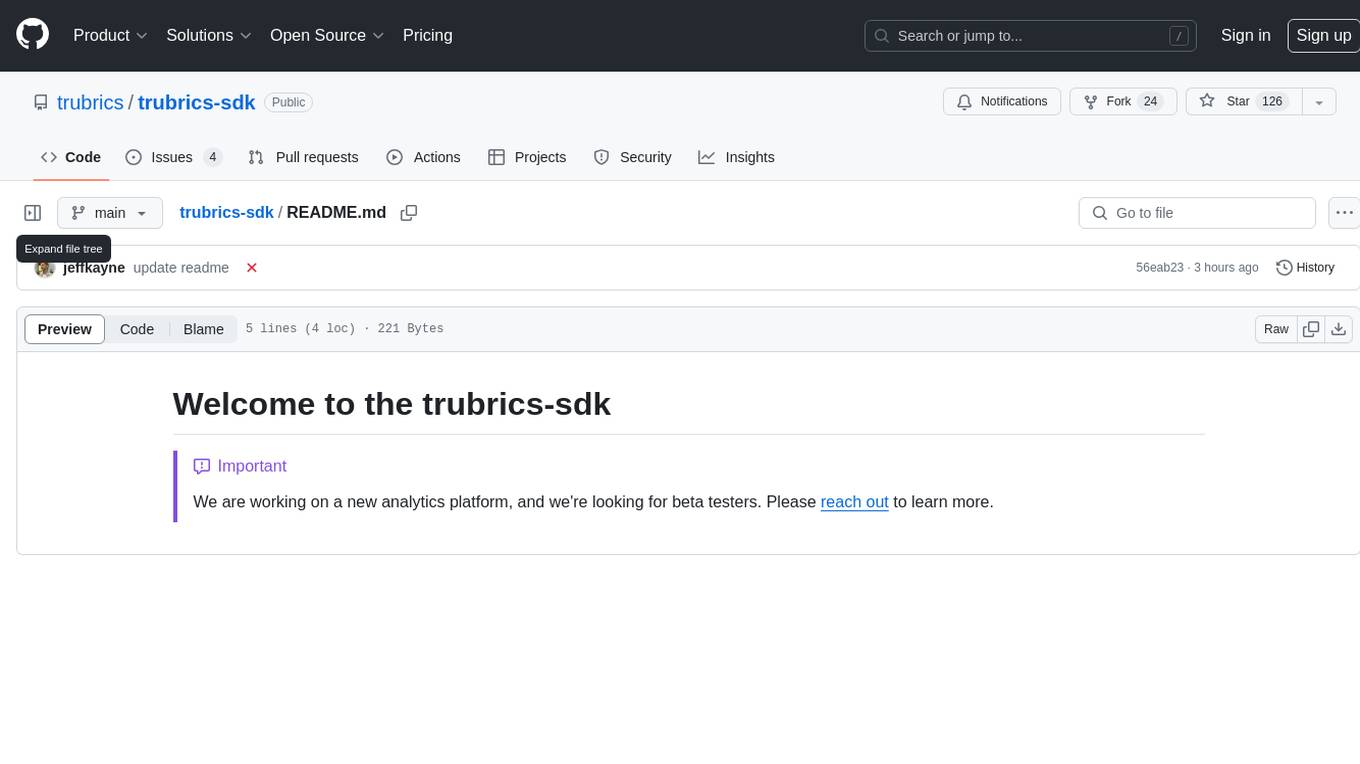
trubrics-sdk
Trubrics-sdk is a software development kit designed to facilitate the integration of analytics features into applications. It provides a set of tools and functionalities that enable developers to easily incorporate analytics capabilities, such as data collection, analysis, and reporting, into their software products. The SDK streamlines the process of implementing analytics solutions, allowing developers to focus on building and enhancing their applications' functionality and user experience. By leveraging trubrics-sdk, developers can quickly and efficiently integrate robust analytics features, gaining valuable insights into user behavior and application performance.
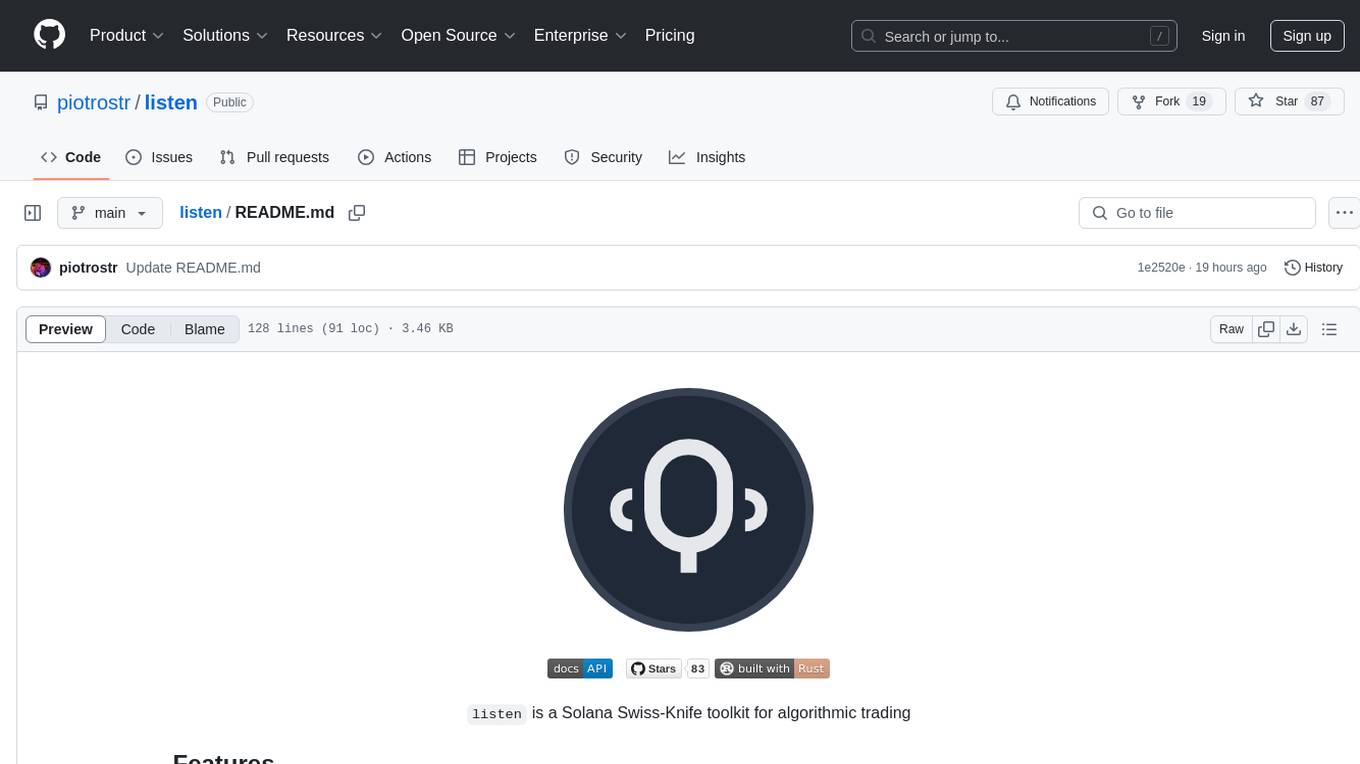
listen
Listen is a Solana Swiss-Knife toolkit for algorithmic trading, offering real-time transaction monitoring, multi-DEX swap execution, fast transactions with Jito MEV bundles, price tracking, token management utilities, and performance monitoring. It includes tools for grabbing data from unofficial APIs and works with the $arc rig framework for AI Agents to interact with the Solana blockchain. The repository provides miscellaneous tools for analysis and data retrieval, with the core functionality in the `src` directory.
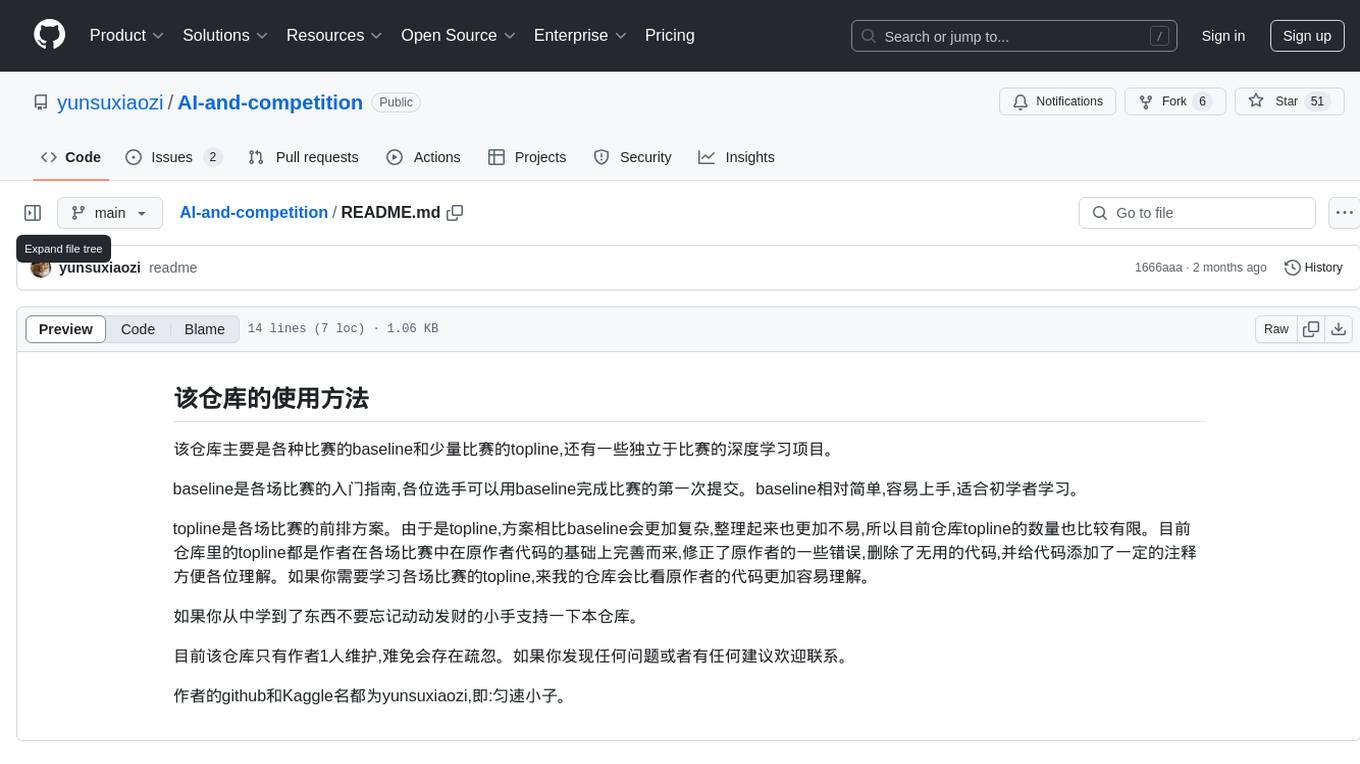
AI-and-competition
This repository provides baselines for various competitions, a few top solutions for some competitions, and independent deep learning projects. Baselines serve as entry guides for competitions, suitable for beginners to make their first submission. Top solutions are more complex and refined versions of baselines, with limited quantity but enhanced quality. The repository is maintained by a single author, yunsuxiaozi, offering code improvements and annotations for better understanding. Users can support the repository by learning from it and providing feedback.
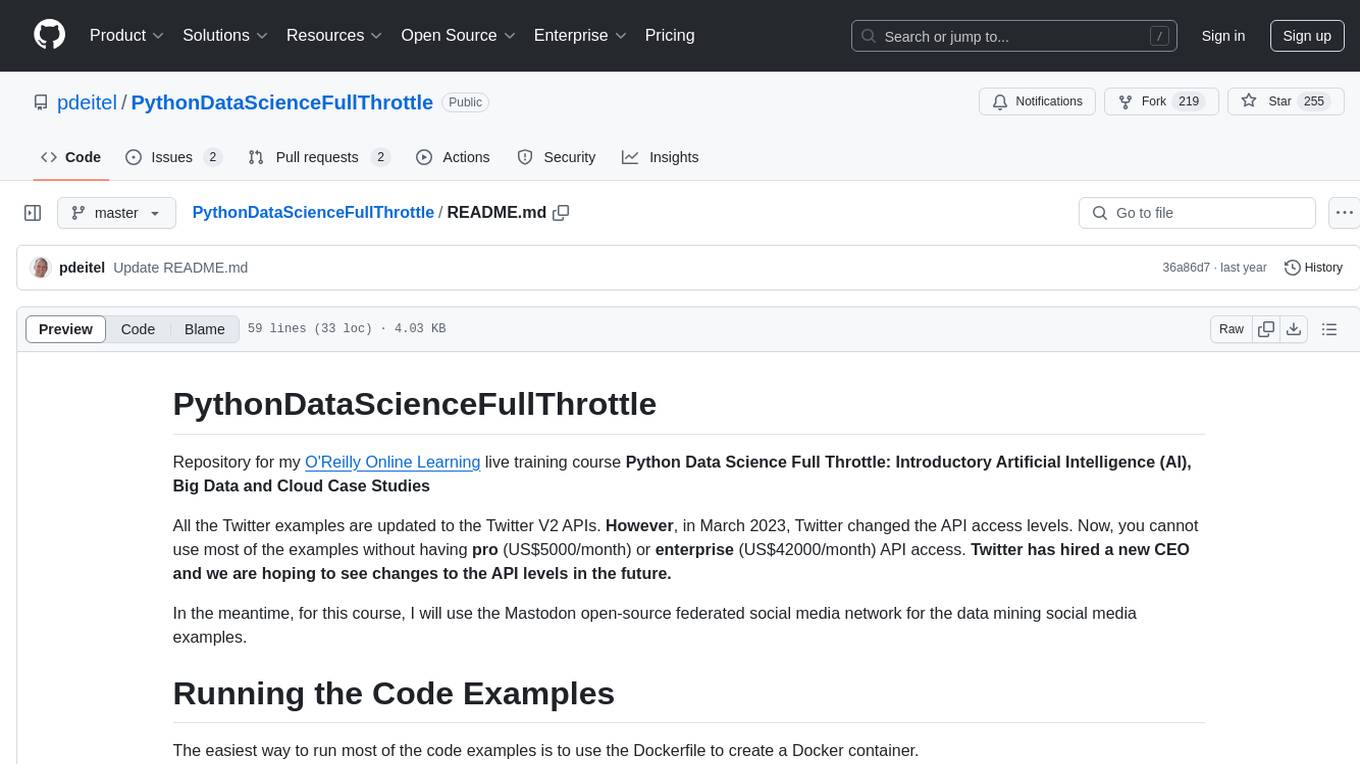
PythonDataScienceFullThrottle
PythonDataScienceFullThrottle is a comprehensive repository containing various Python scripts, libraries, and tools for data science enthusiasts. It includes a wide range of functionalities such as data preprocessing, visualization, machine learning algorithms, and statistical analysis. The repository aims to provide a one-stop solution for individuals looking to dive deep into the world of data science using Python.
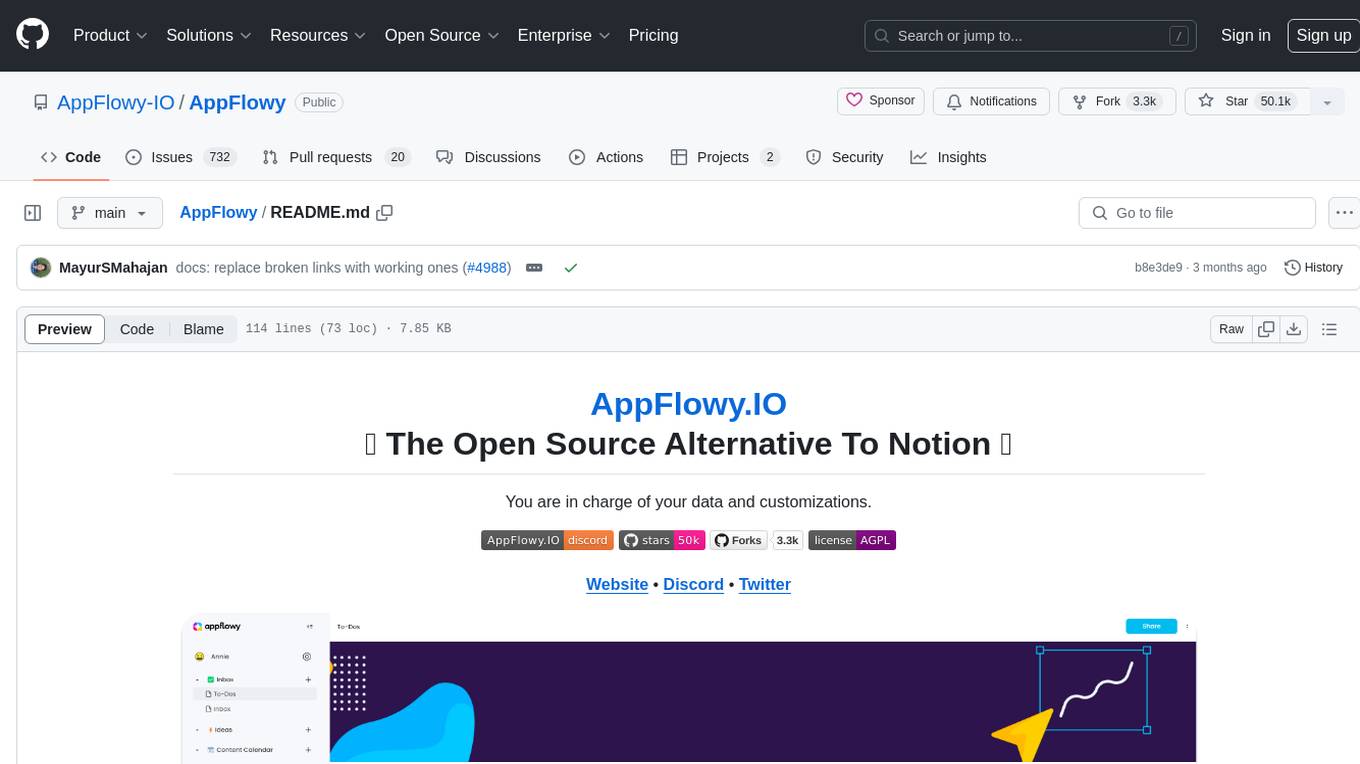
AppFlowy
AppFlowy.IO is an open-source alternative to Notion, providing users with control over their data and customizations. It aims to offer functionality, data security, and cross-platform native experience to individuals, as well as building blocks and collaboration infra services to enterprises and hackers. The tool is built with Flutter and Rust, supporting multiple platforms and emphasizing long-term maintainability. AppFlowy prioritizes data privacy, reliable native experience, and community-driven extensibility, aiming to democratize the creation of complex workplace management tools.
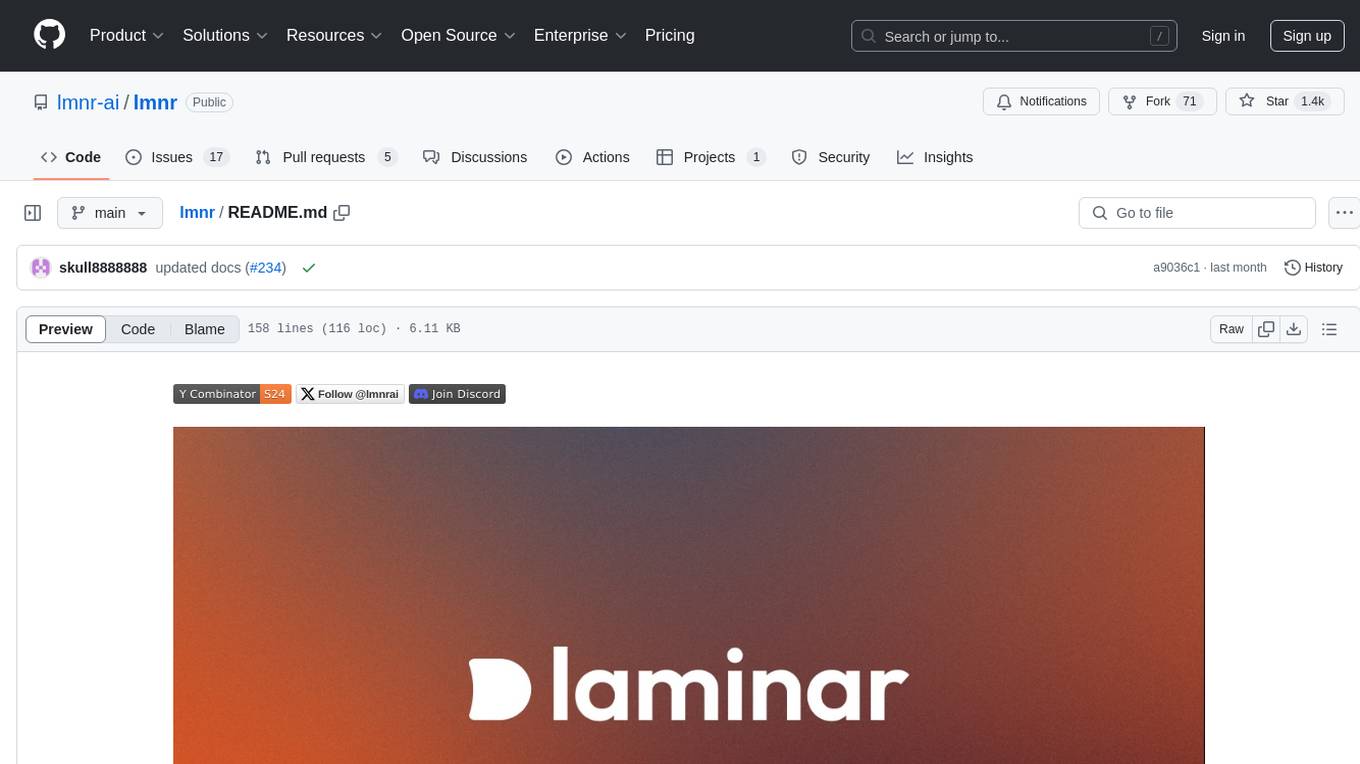
lmnr
Laminar is an all-in-one open-source platform designed for engineering AI products. It allows users to trace, evaluate, label, and analyze LLM data efficiently. The platform offers features such as automatic tracing of common AI frameworks and SDKs, local and online evaluations, simple UI for data labeling, dataset management, and scalability with gRPC communication. Laminar is built with a modern open-source stack including RabbitMQ, Postgres, Clickhouse, and Qdrant for semantic similarity search. It provides fast and beautiful dashboards for traces, evaluations, and labels, making it a comprehensive tool for AI product development.
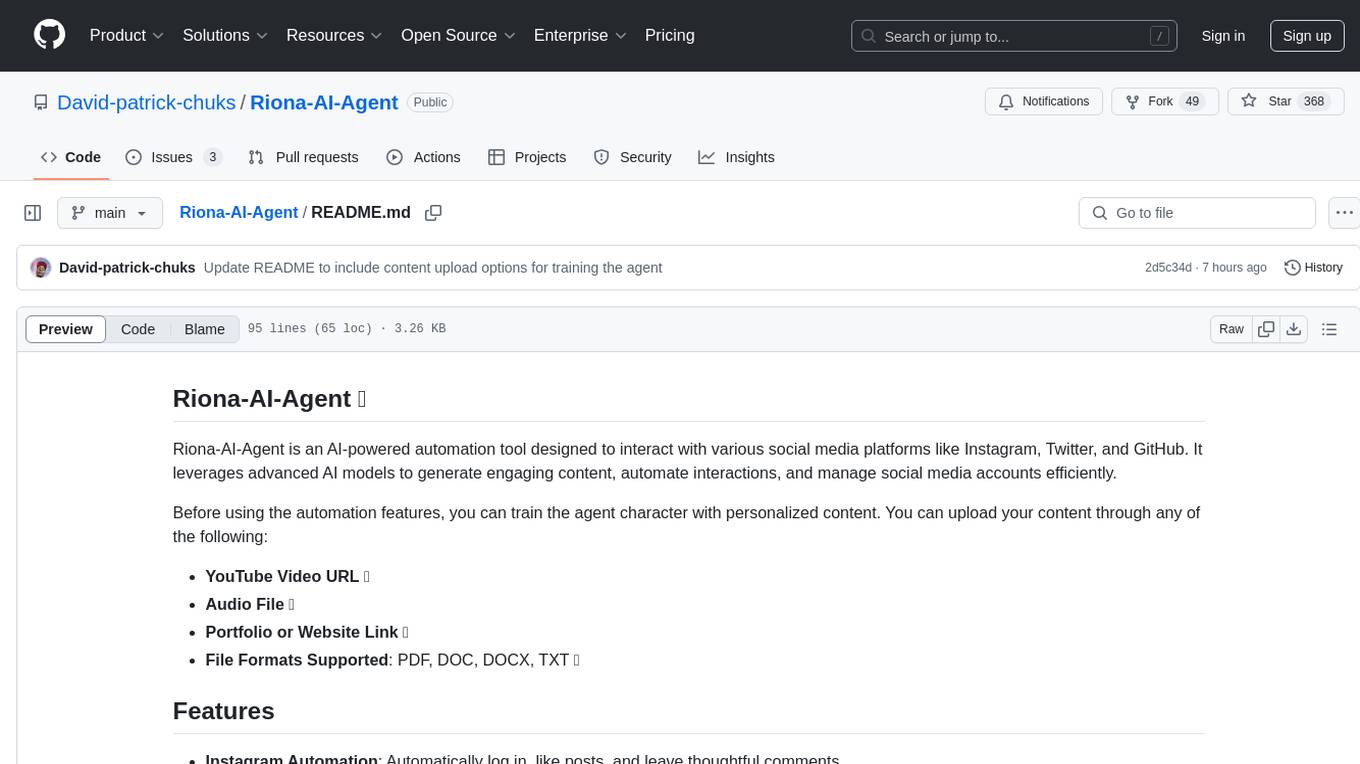
Riona-AI-Agent
Riona-AI-Agent is a versatile AI chatbot designed to assist users in various tasks. It utilizes natural language processing and machine learning algorithms to understand user queries and provide accurate responses. The chatbot can be integrated into websites, applications, and messaging platforms to enhance user experience and streamline communication. With its customizable features and easy deployment, Riona-AI-Agent is suitable for businesses, developers, and individuals looking to automate customer support, provide information, and engage with users in a conversational manner.
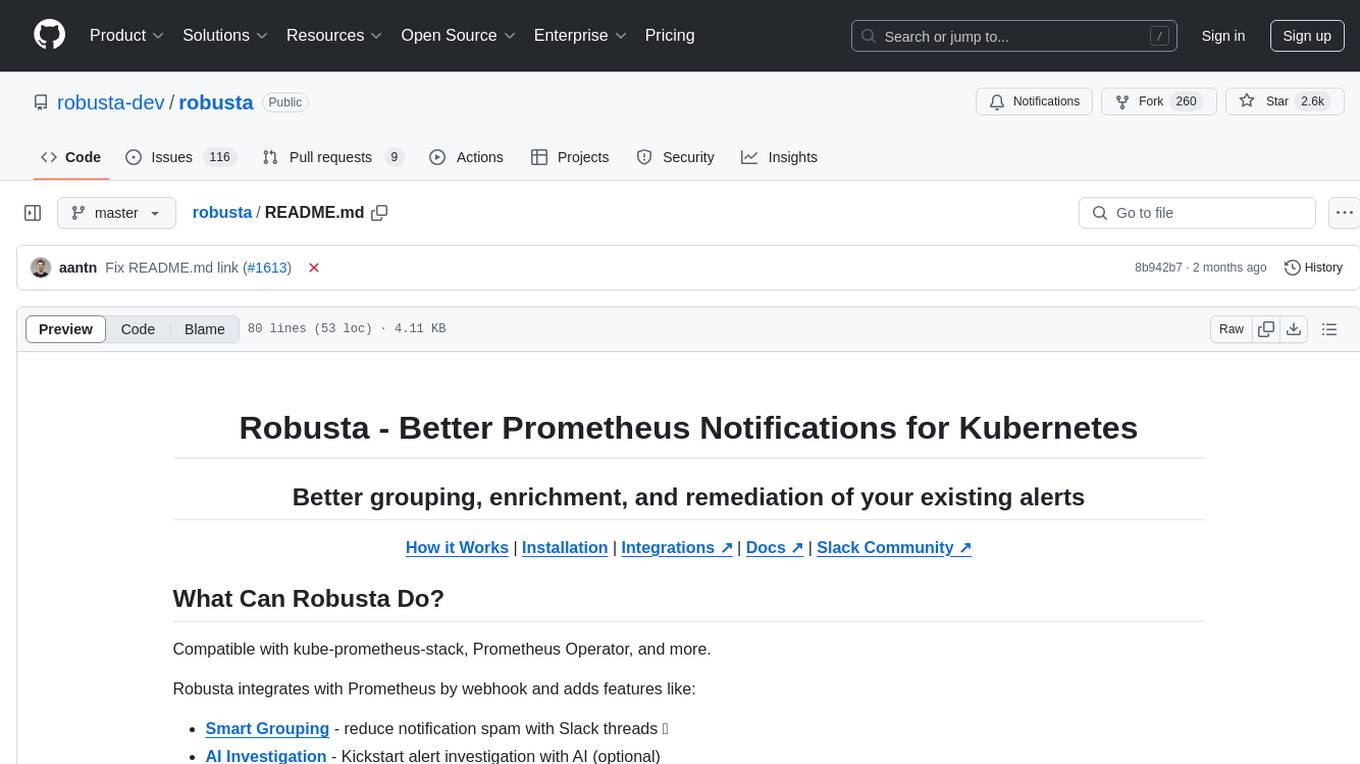
robusta
Robusta is a tool designed to enhance Prometheus notifications for Kubernetes environments. It offers features such as smart grouping to reduce notification spam, AI investigation for alert analysis, alert enrichment with additional data like pod logs, self-healing capabilities for defining auto-remediation rules, advanced routing options, problem detection without PromQL, change-tracking for Kubernetes resources, auto-resolve functionality, and integration with various external systems like Slack, Teams, and Jira. Users can utilize Robusta with or without Prometheus, and it can be installed alongside existing Prometheus setups or as part of an all-in-one Kubernetes observability stack.
For similar tasks
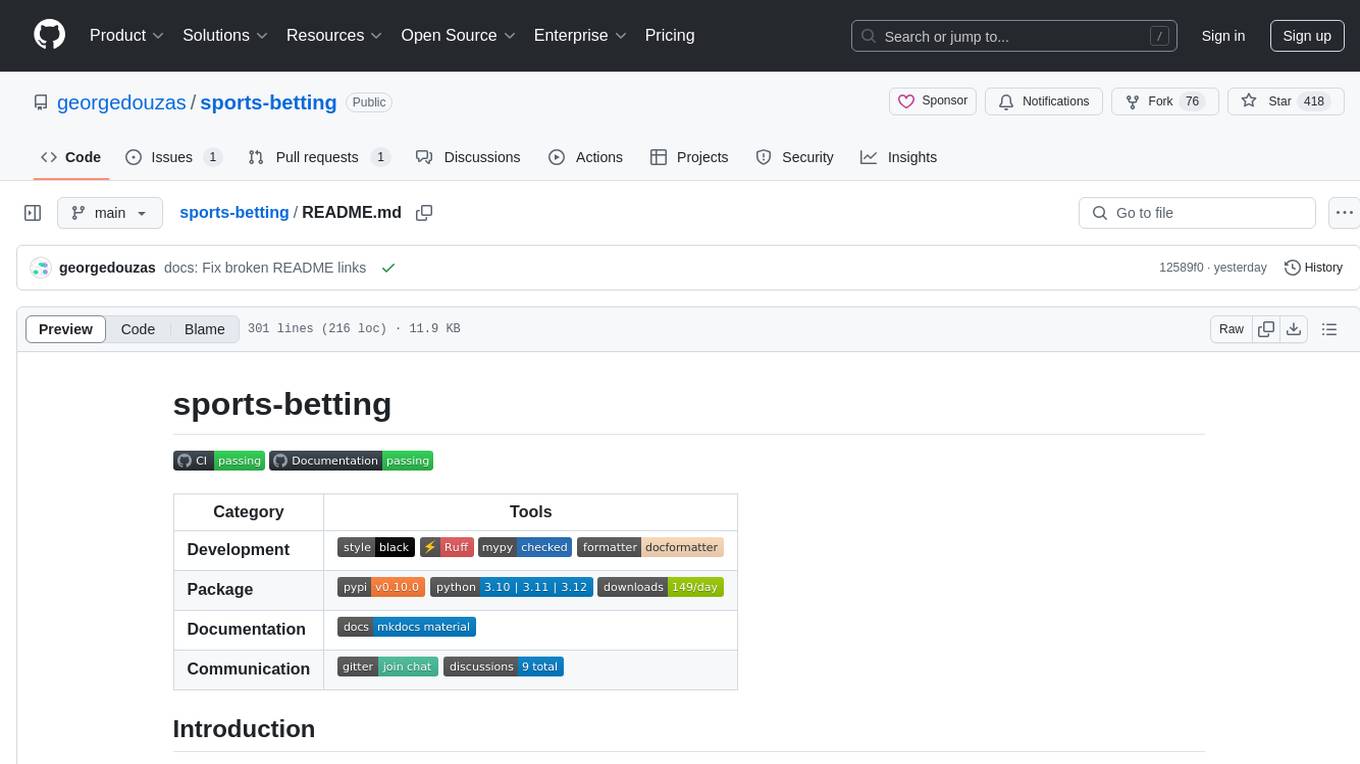
sports-betting
Sports-betting is a Python library for implementing betting strategies and analyzing sports data. It provides tools for collecting, processing, and visualizing sports data to make informed betting decisions. The library includes modules for scraping data from sports websites, calculating odds, simulating betting strategies, and evaluating performance. With sports-betting, users can automate betting processes, test different strategies, and improve their betting outcomes.
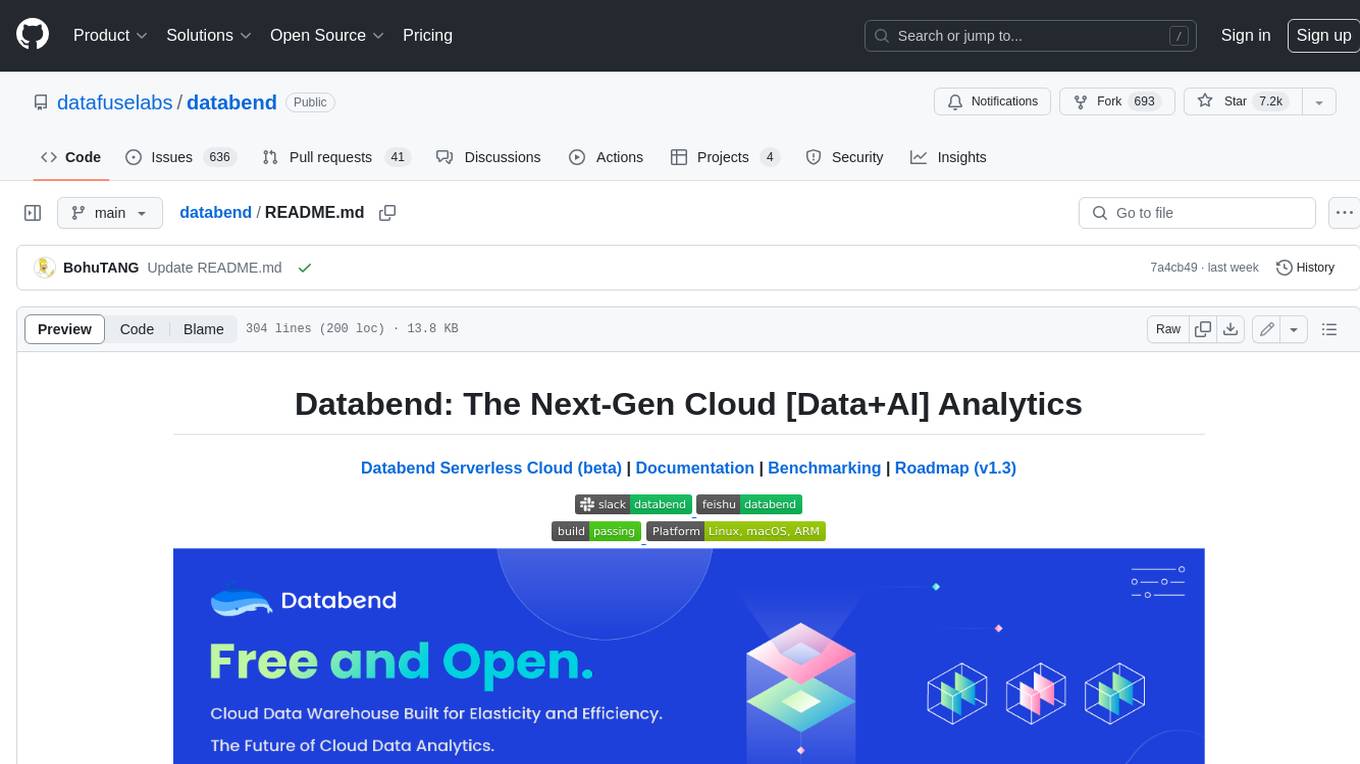
databend
Databend is an open-source cloud data warehouse that serves as a cost-effective alternative to Snowflake. With its focus on fast query execution and data ingestion, it's designed for complex analysis of the world's largest datasets.
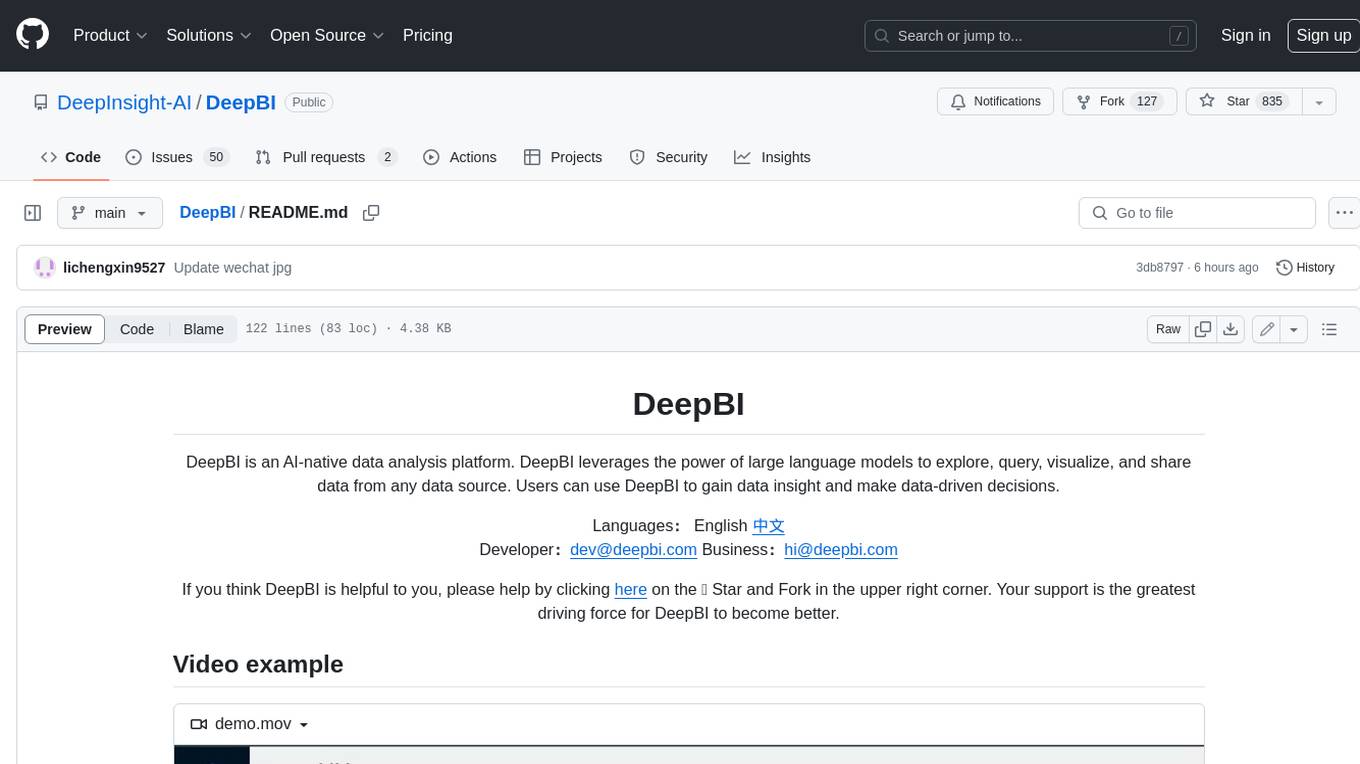
DeepBI
DeepBI is an AI-native data analysis platform that leverages the power of large language models to explore, query, visualize, and share data from any data source. Users can use DeepBI to gain data insight and make data-driven decisions.
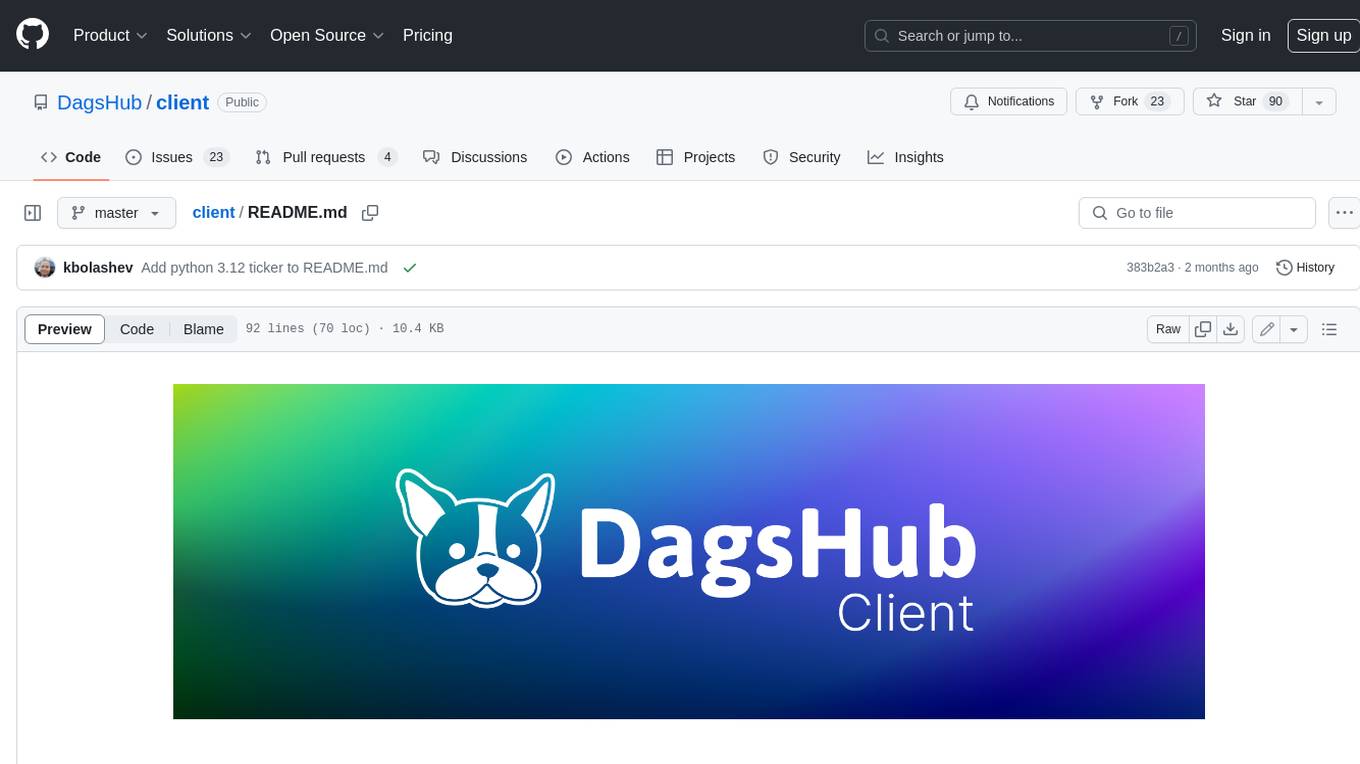
client
DagsHub is a platform for machine learning and data science teams to build, manage, and collaborate on their projects. With DagsHub you can: 1. Version code, data, and models in one place. Use the free provided DagsHub storage or connect it to your cloud storage 2. Track Experiments using Git, DVC or MLflow, to provide a fully reproducible environment 3. Visualize pipelines, data, and notebooks in and interactive, diff-able, and dynamic way 4. Label your data directly on the platform using Label Studio 5. Share your work with your team members 6. Stream and upload your data in an intuitive and easy way, while preserving versioning and structure. DagsHub is built firmly around open, standard formats for your project. In particular: * Git * DVC * MLflow * Label Studio * Standard data formats like YAML, JSON, CSV Therefore, you can work with DagsHub regardless of your chosen programming language or frameworks.
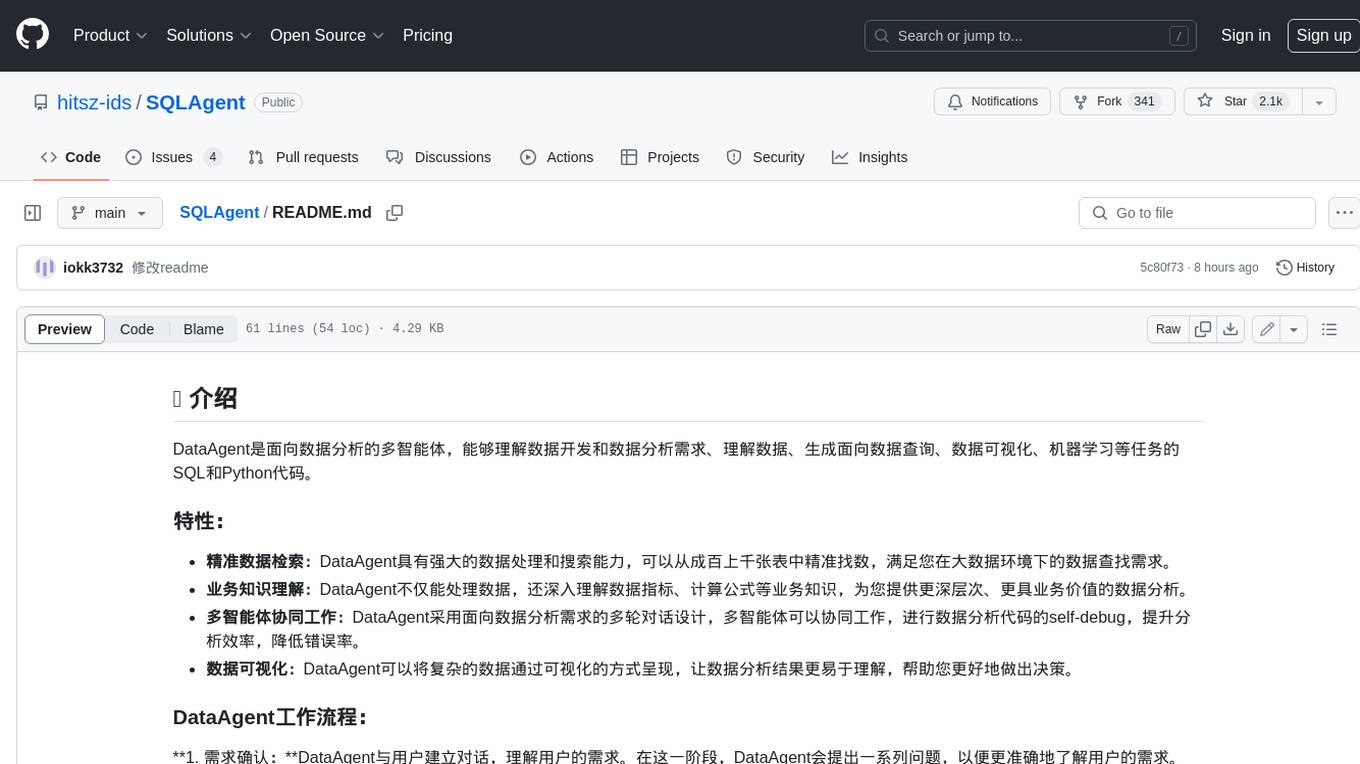
SQLAgent
DataAgent is a multi-agent system for data analysis, capable of understanding data development and data analysis requirements, understanding data, and generating SQL and Python code for tasks such as data query, data visualization, and machine learning.
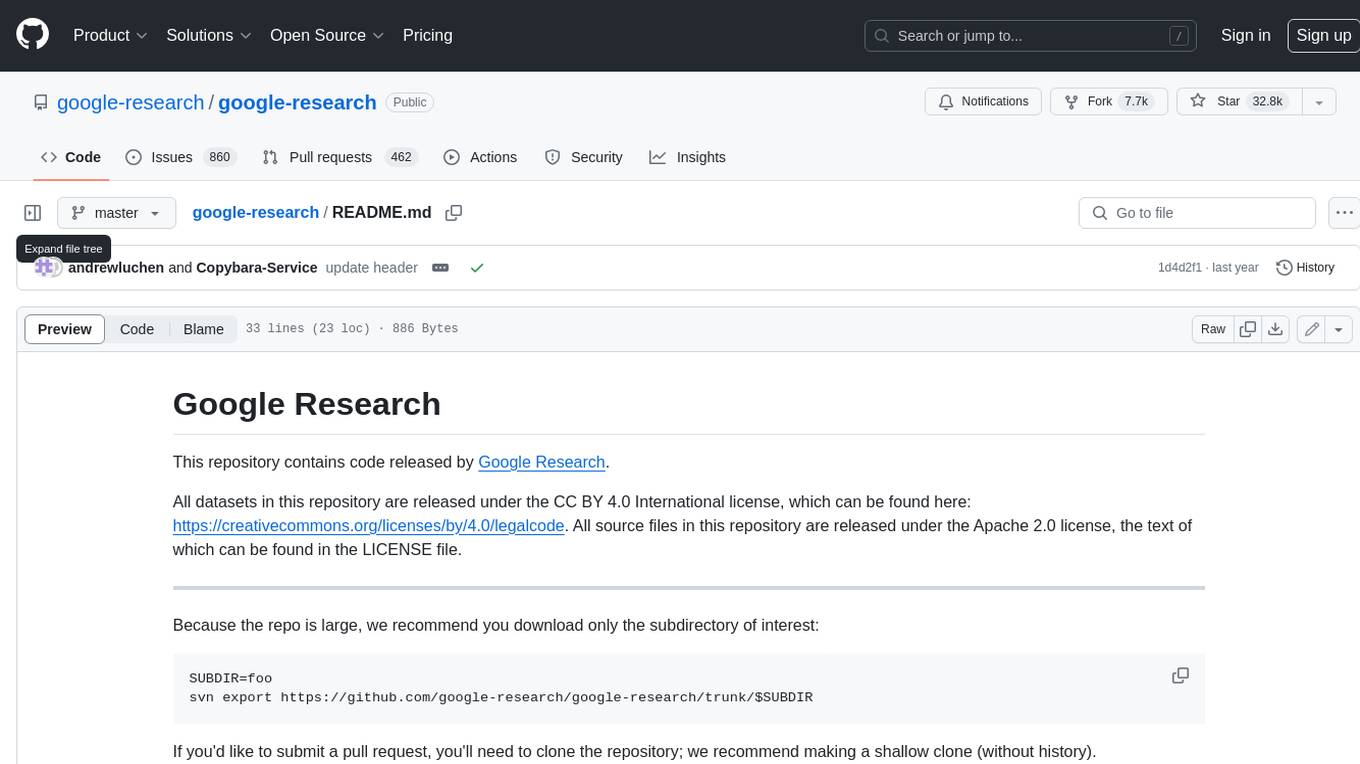
google-research
This repository contains code released by Google Research. All datasets in this repository are released under the CC BY 4.0 International license, which can be found here: https://creativecommons.org/licenses/by/4.0/legalcode. All source files in this repository are released under the Apache 2.0 license, the text of which can be found in the LICENSE file.
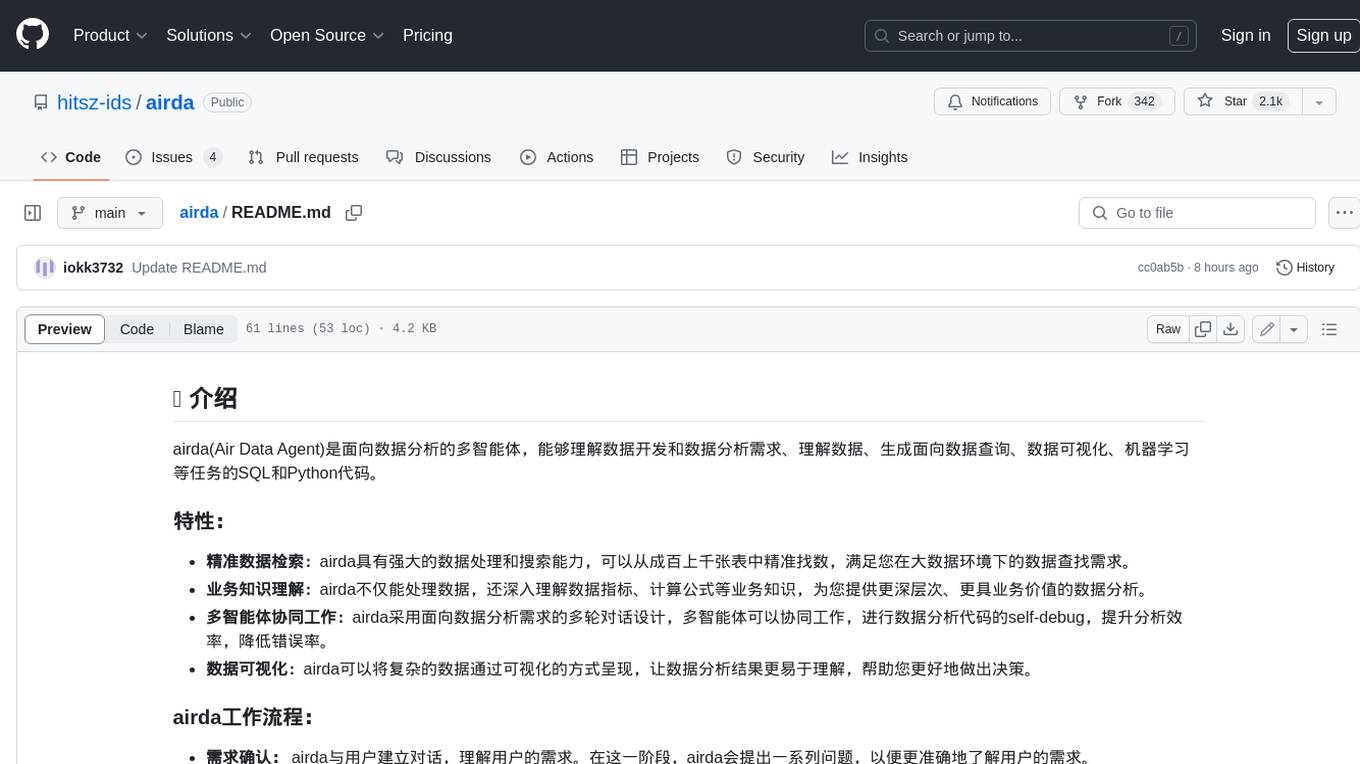
airda
airda(Air Data Agent) is a multi-agent system for data analysis, which can understand data development and data analysis requirements, understand data, and generate SQL and Python code for data query, data visualization, machine learning and other tasks.
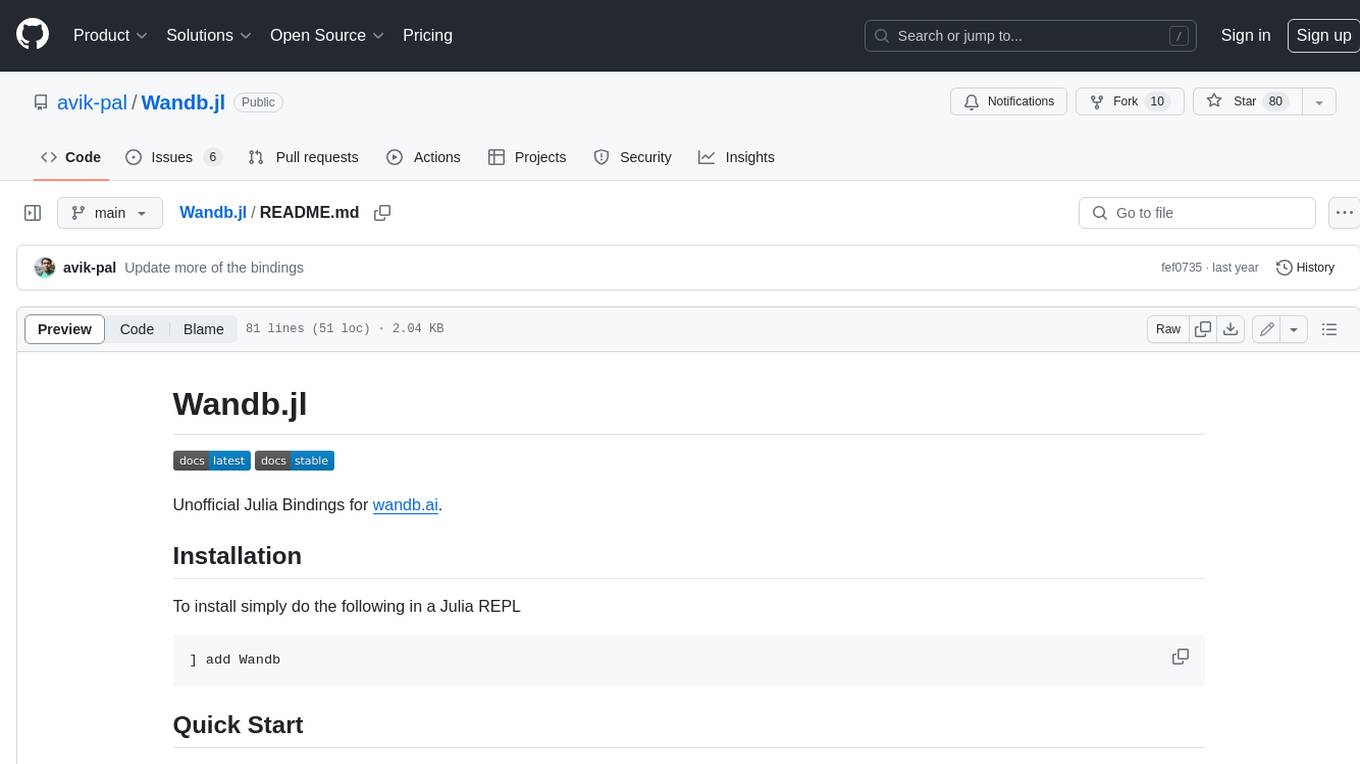
Wandb.jl
Unofficial Julia Bindings for wandb.ai. Wandb is a platform for tracking and visualizing machine learning experiments. It provides a simple and consistent way to log metrics, parameters, and other data from your experiments, and to visualize them in a variety of ways. Wandb.jl provides a convenient way to use Wandb from Julia.
For similar jobs
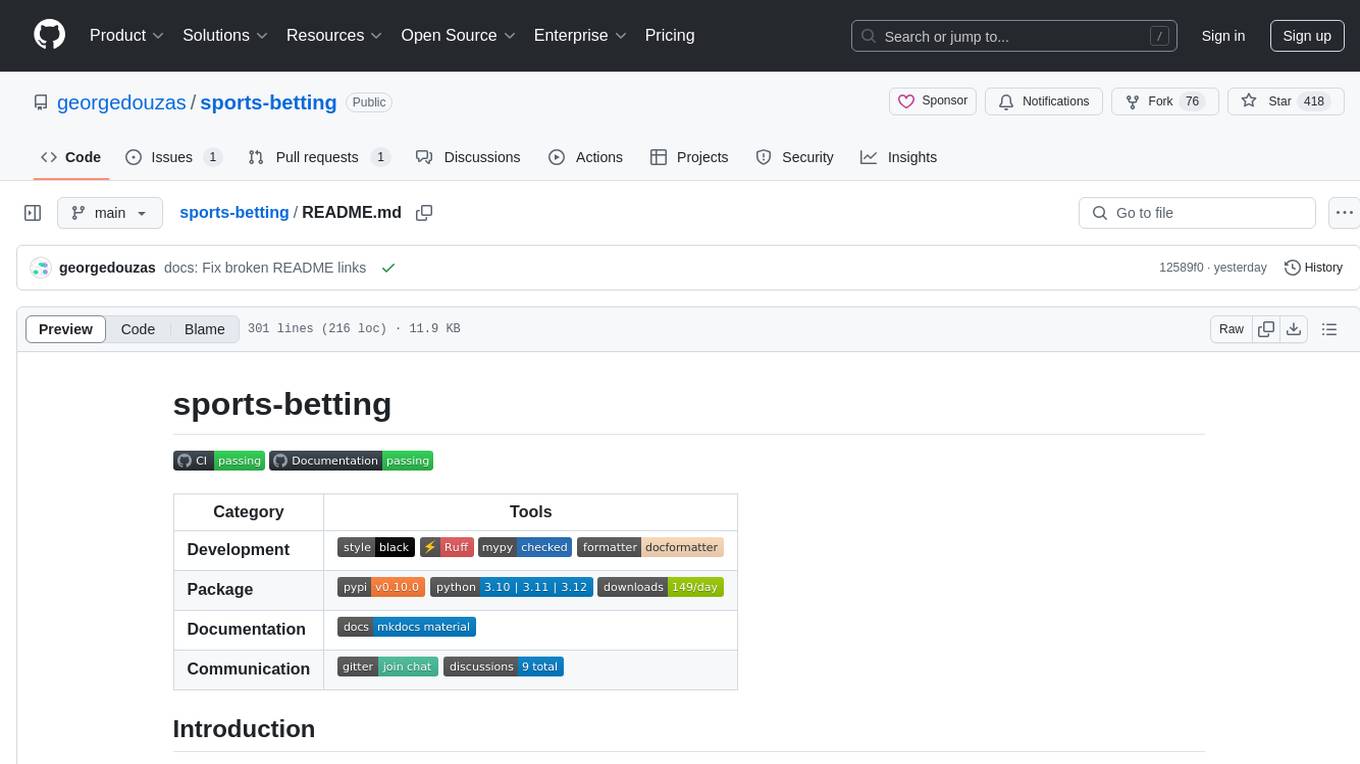
sports-betting
Sports-betting is a Python library for implementing betting strategies and analyzing sports data. It provides tools for collecting, processing, and visualizing sports data to make informed betting decisions. The library includes modules for scraping data from sports websites, calculating odds, simulating betting strategies, and evaluating performance. With sports-betting, users can automate betting processes, test different strategies, and improve their betting outcomes.
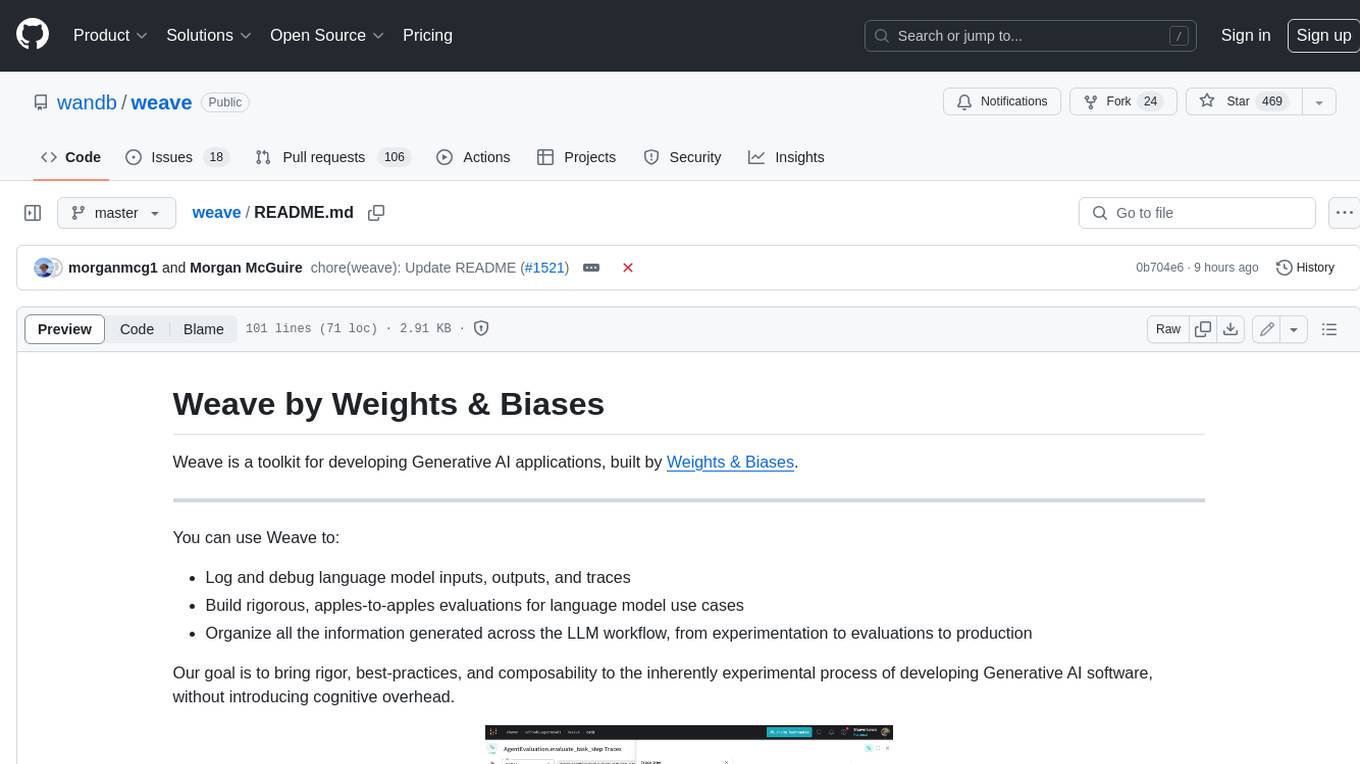
weave
Weave is a toolkit for developing Generative AI applications, built by Weights & Biases. With Weave, you can log and debug language model inputs, outputs, and traces; build rigorous, apples-to-apples evaluations for language model use cases; and organize all the information generated across the LLM workflow, from experimentation to evaluations to production. Weave aims to bring rigor, best-practices, and composability to the inherently experimental process of developing Generative AI software, without introducing cognitive overhead.
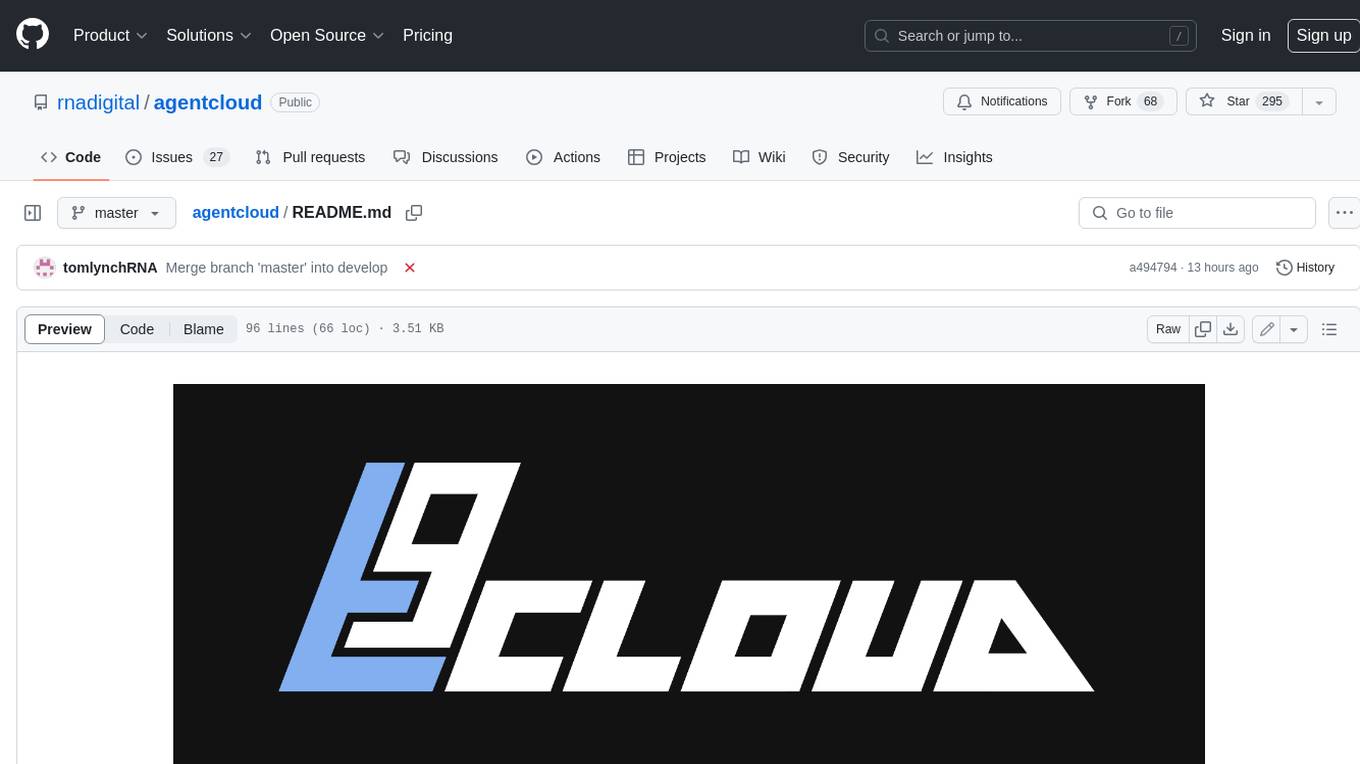
agentcloud
AgentCloud is an open-source platform that enables companies to build and deploy private LLM chat apps, empowering teams to securely interact with their data. It comprises three main components: Agent Backend, Webapp, and Vector Proxy. To run this project locally, clone the repository, install Docker, and start the services. The project is licensed under the GNU Affero General Public License, version 3 only. Contributions and feedback are welcome from the community.
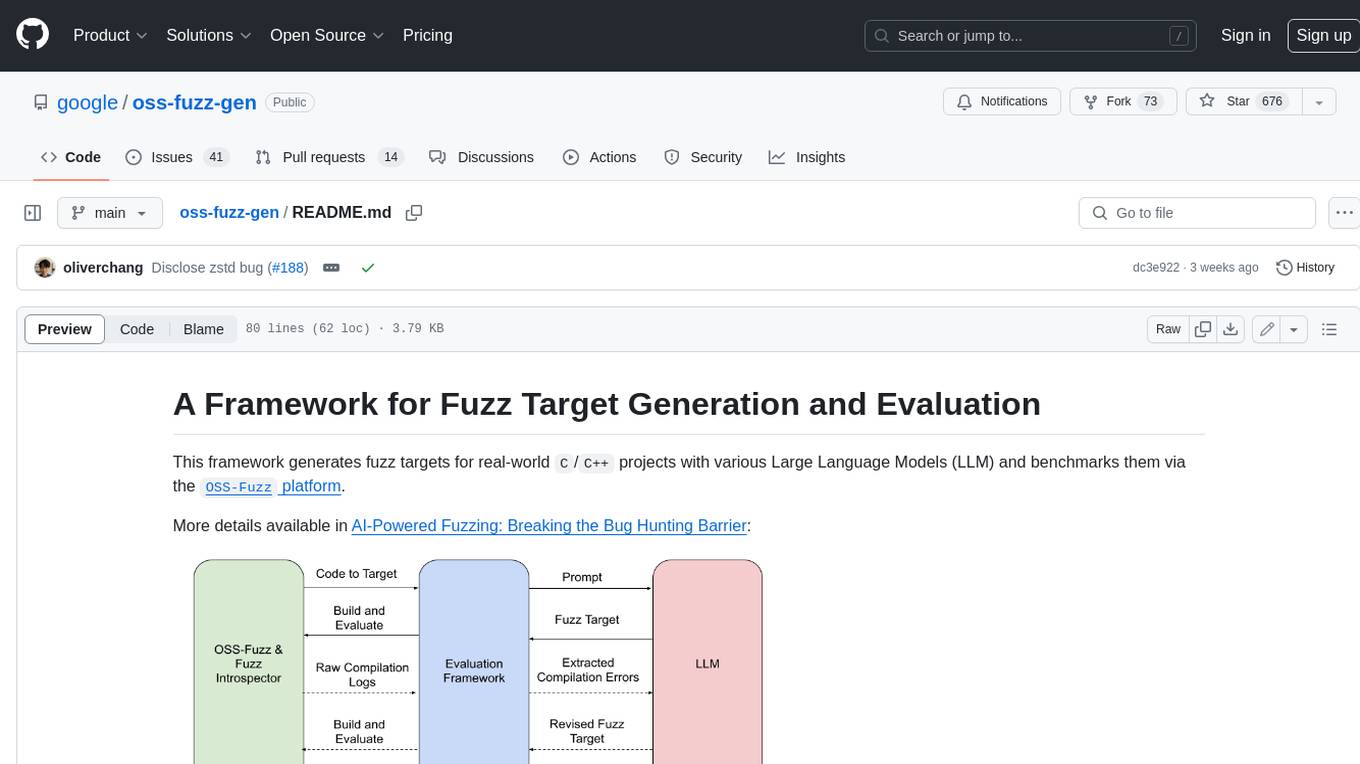
oss-fuzz-gen
This framework generates fuzz targets for real-world `C`/`C++` projects with various Large Language Models (LLM) and benchmarks them via the `OSS-Fuzz` platform. It manages to successfully leverage LLMs to generate valid fuzz targets (which generate non-zero coverage increase) for 160 C/C++ projects. The maximum line coverage increase is 29% from the existing human-written targets.
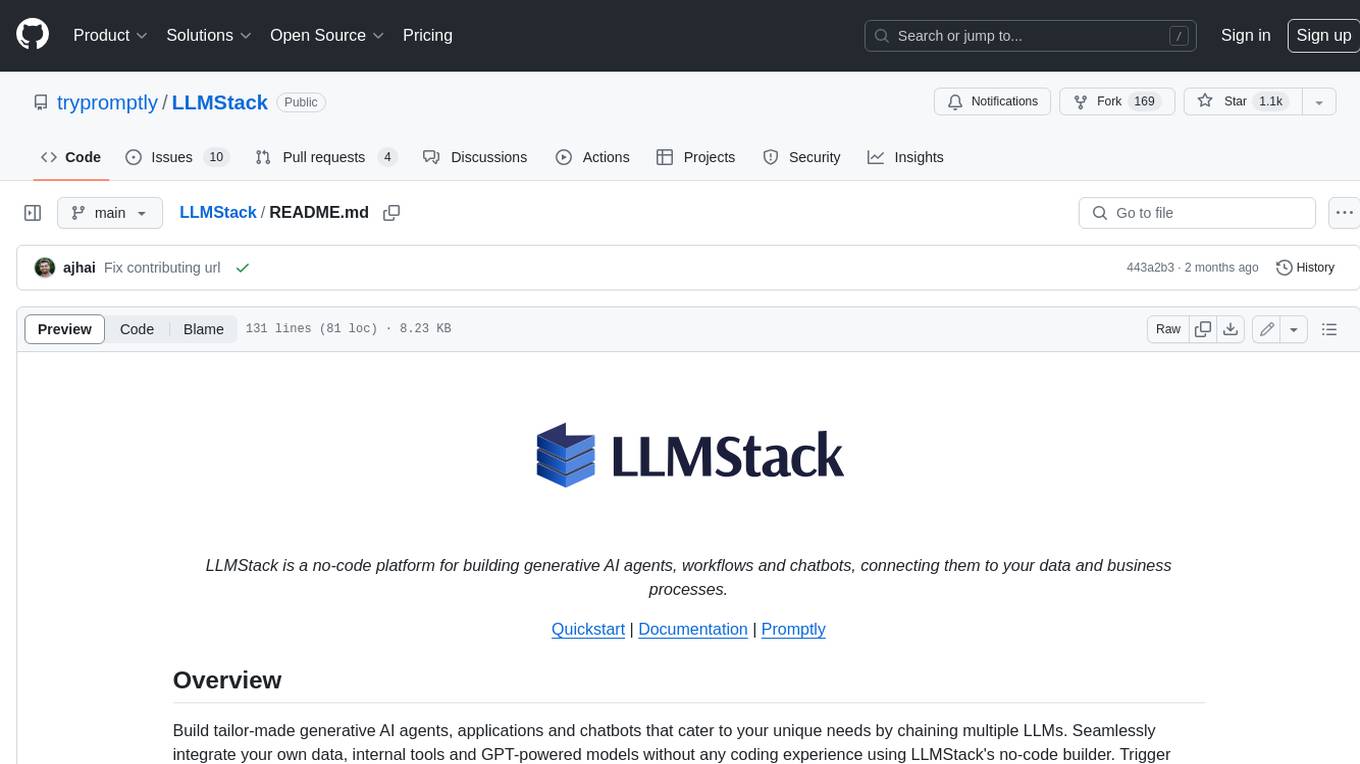
LLMStack
LLMStack is a no-code platform for building generative AI agents, workflows, and chatbots. It allows users to connect their own data, internal tools, and GPT-powered models without any coding experience. LLMStack can be deployed to the cloud or on-premise and can be accessed via HTTP API or triggered from Slack or Discord.
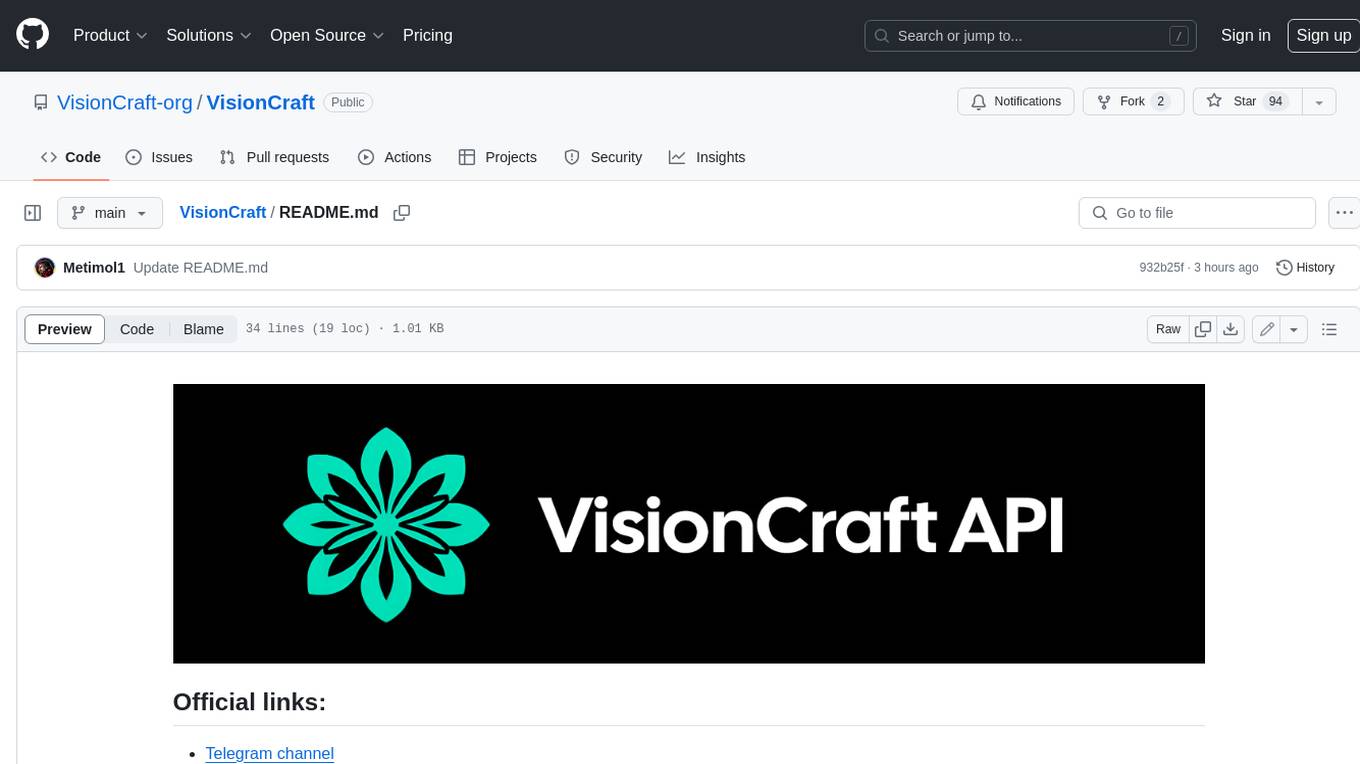
VisionCraft
The VisionCraft API is a free API for using over 100 different AI models. From images to sound.
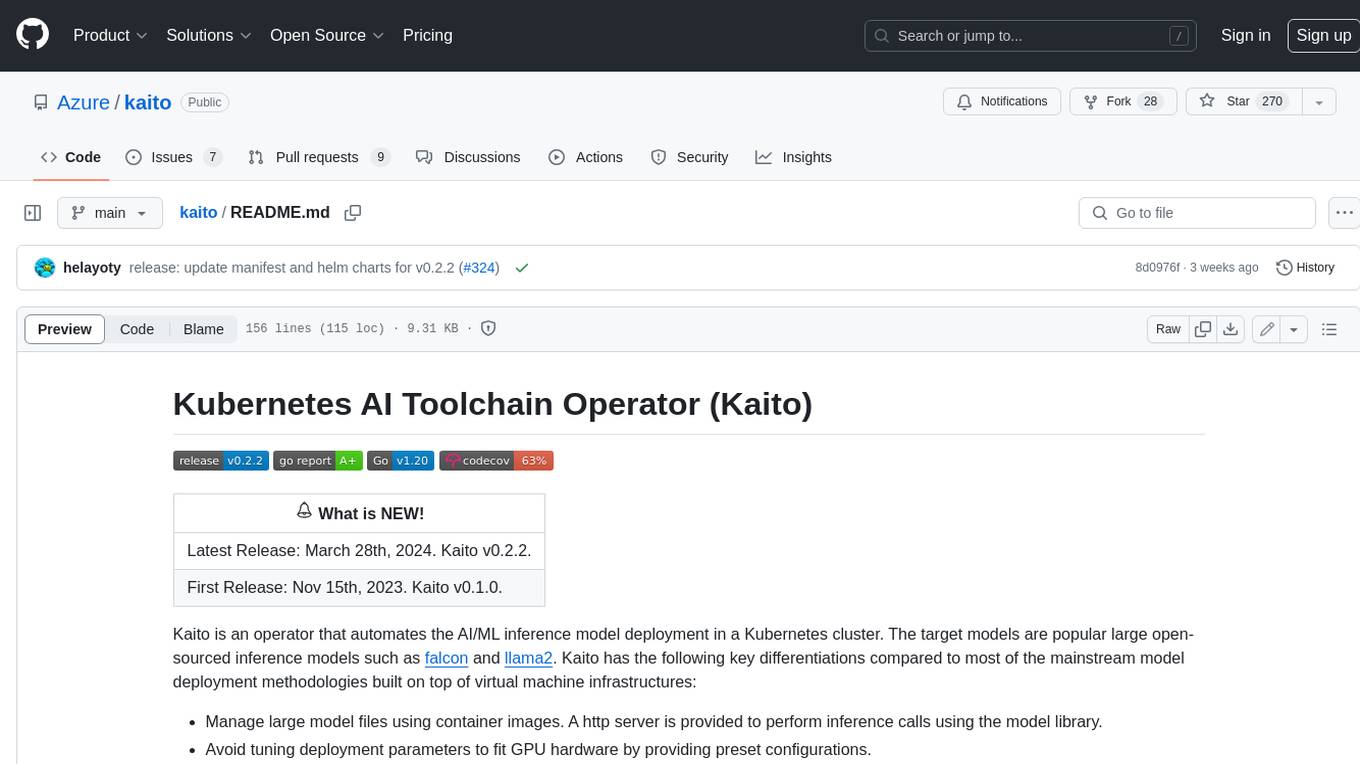
kaito
Kaito is an operator that automates the AI/ML inference model deployment in a Kubernetes cluster. It manages large model files using container images, avoids tuning deployment parameters to fit GPU hardware by providing preset configurations, auto-provisions GPU nodes based on model requirements, and hosts large model images in the public Microsoft Container Registry (MCR) if the license allows. Using Kaito, the workflow of onboarding large AI inference models in Kubernetes is largely simplified.
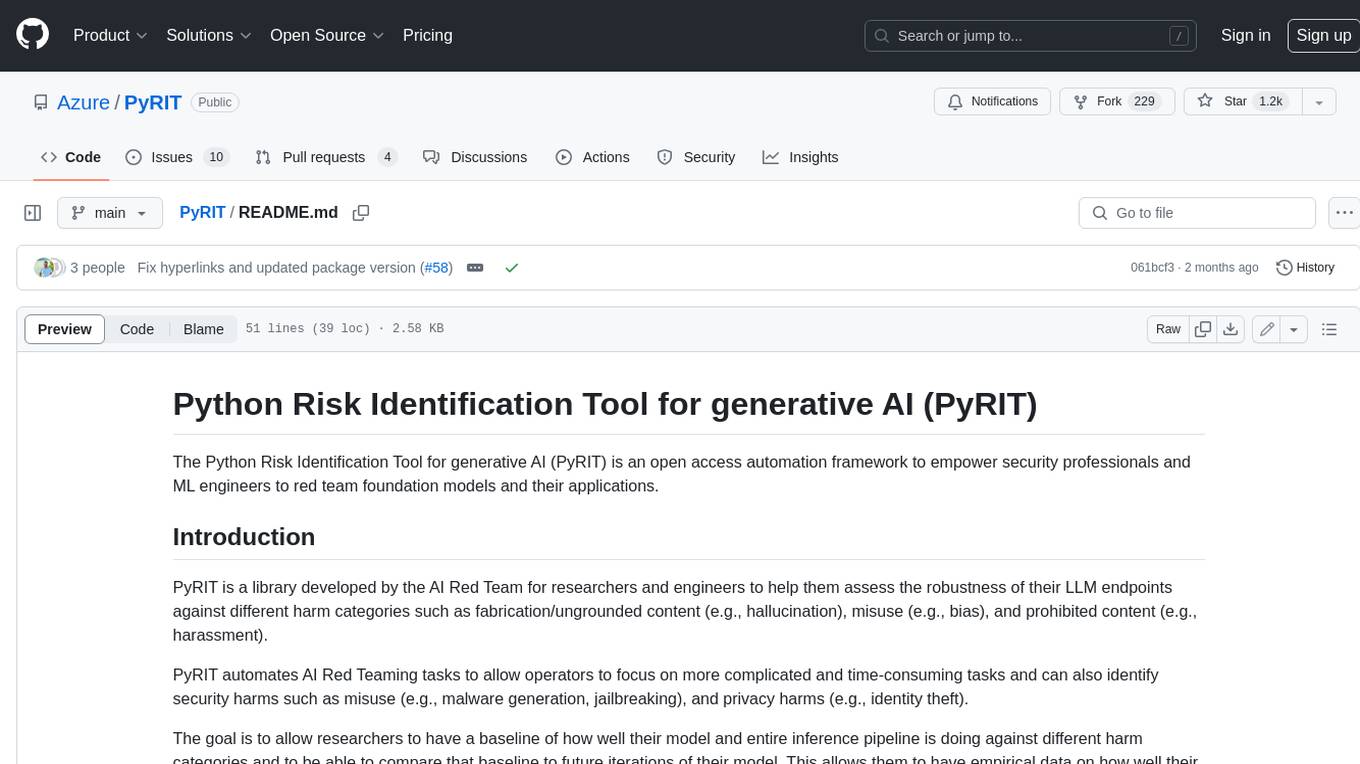
PyRIT
PyRIT is an open access automation framework designed to empower security professionals and ML engineers to red team foundation models and their applications. It automates AI Red Teaming tasks to allow operators to focus on more complicated and time-consuming tasks and can also identify security harms such as misuse (e.g., malware generation, jailbreaking), and privacy harms (e.g., identity theft). The goal is to allow researchers to have a baseline of how well their model and entire inference pipeline is doing against different harm categories and to be able to compare that baseline to future iterations of their model. This allows them to have empirical data on how well their model is doing today, and detect any degradation of performance based on future improvements.