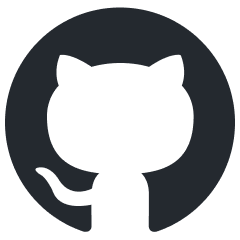
dcai-course
Introduction to Data-Centric AI, MIT IAP 2023 🤖
Stars: 93
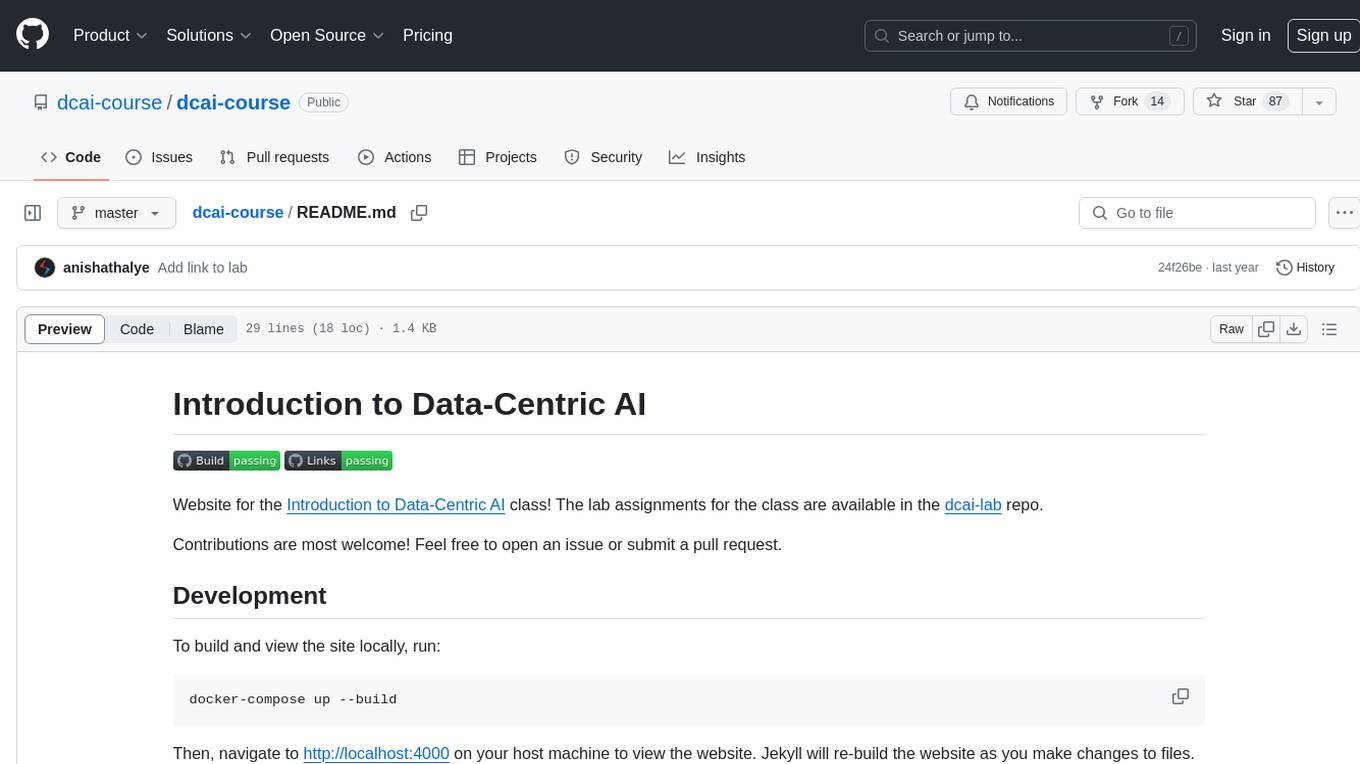
This repository serves as the website for the Introduction to Data-Centric AI class. It contains lab assignments and resources for the course. Users can contribute by opening issues or submitting pull requests. The website can be built locally using Docker and Jekyll. The design is based on Missing Semester. All contents, including source code, lecture notes, and videos, are licensed under CC BY-NC-SA 4.0.
README:
Website for the Introduction to Data-Centric AI class! The lab assignments for the class are available in the dcai-lab repo.
Contributions are most welcome! Feel free to open an issue or submit a pull request.
To build and view the site locally, run:
docker-compose up --build
Then, navigate to http://localhost:4000 on your host machine to view the website. Jekyll will re-build the website as you make changes to files.
The design for this class website is based on Missing Semester. Used with permission.
All the contents in this course, including the website source code, lecture notes, exercises, and lecture videos are licensed under Attribution-NonCommercial-ShareAlike 4.0 International CC BY-NC-SA 4.0. See here for more information on contributions or translations.
For Tasks:
Click tags to check more tools for each tasksFor Jobs:
Alternative AI tools for dcai-course
Similar Open Source Tools
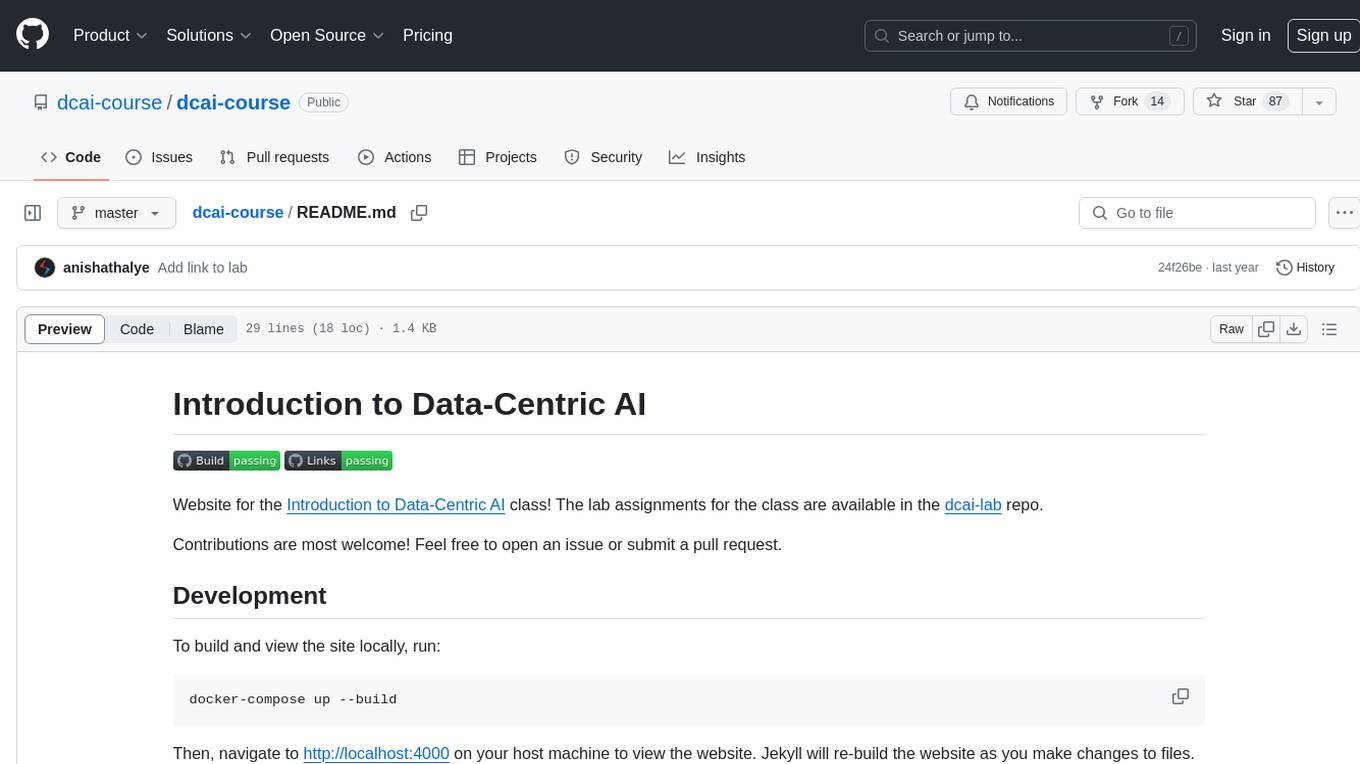
dcai-course
This repository serves as the website for the Introduction to Data-Centric AI class. It contains lab assignments and resources for the course. Users can contribute by opening issues or submitting pull requests. The website can be built locally using Docker and Jekyll. The design is based on Missing Semester. All contents, including source code, lecture notes, and videos, are licensed under CC BY-NC-SA 4.0.
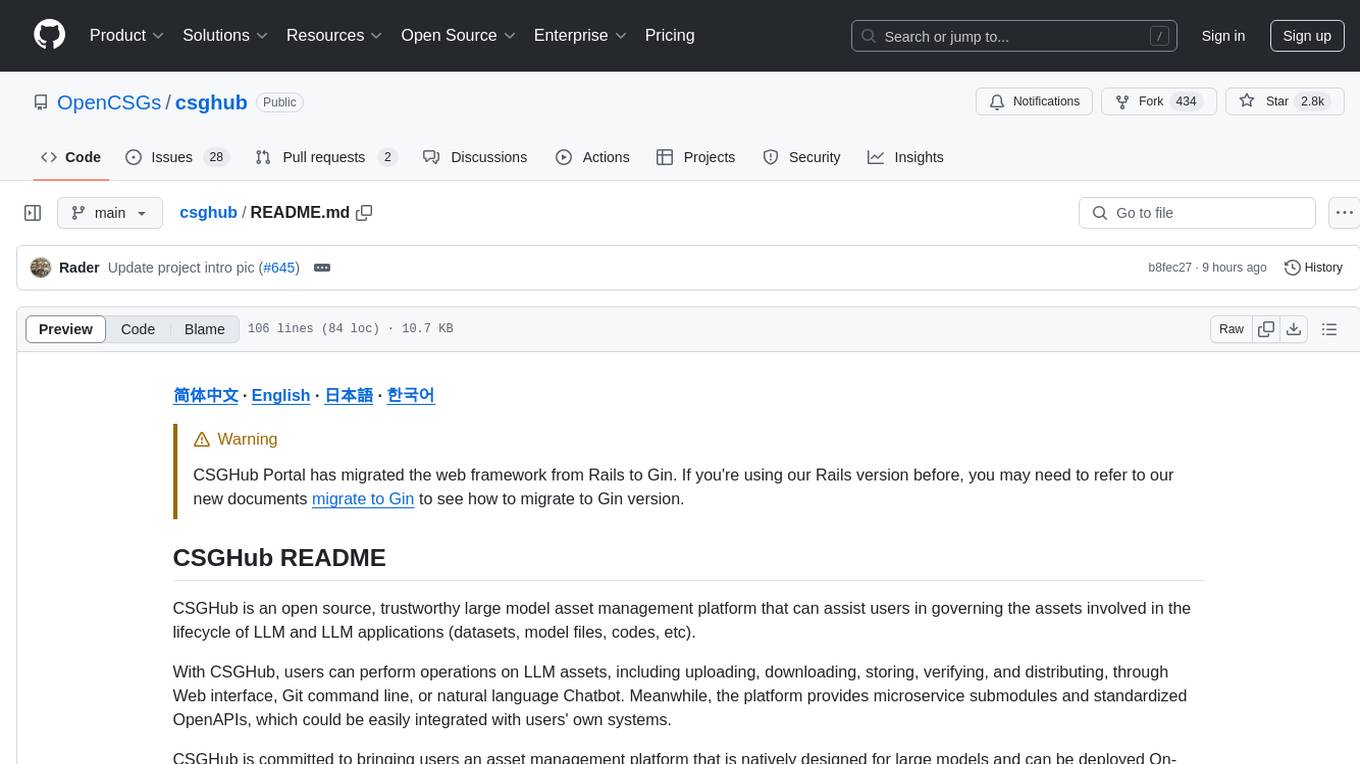
csghub
CSGHub is an open source platform for managing large model assets, including datasets, model files, and codes. It offers functionalities similar to a privatized Huggingface, managing assets in a manner akin to how OpenStack Glance manages virtual machine images. Users can perform operations such as uploading, downloading, storing, verifying, and distributing assets through various interfaces. The platform provides microservice submodules and standardized OpenAPIs for easy integration with users' systems. CSGHub is designed for large models and can be deployed On-Premise for offline operation.
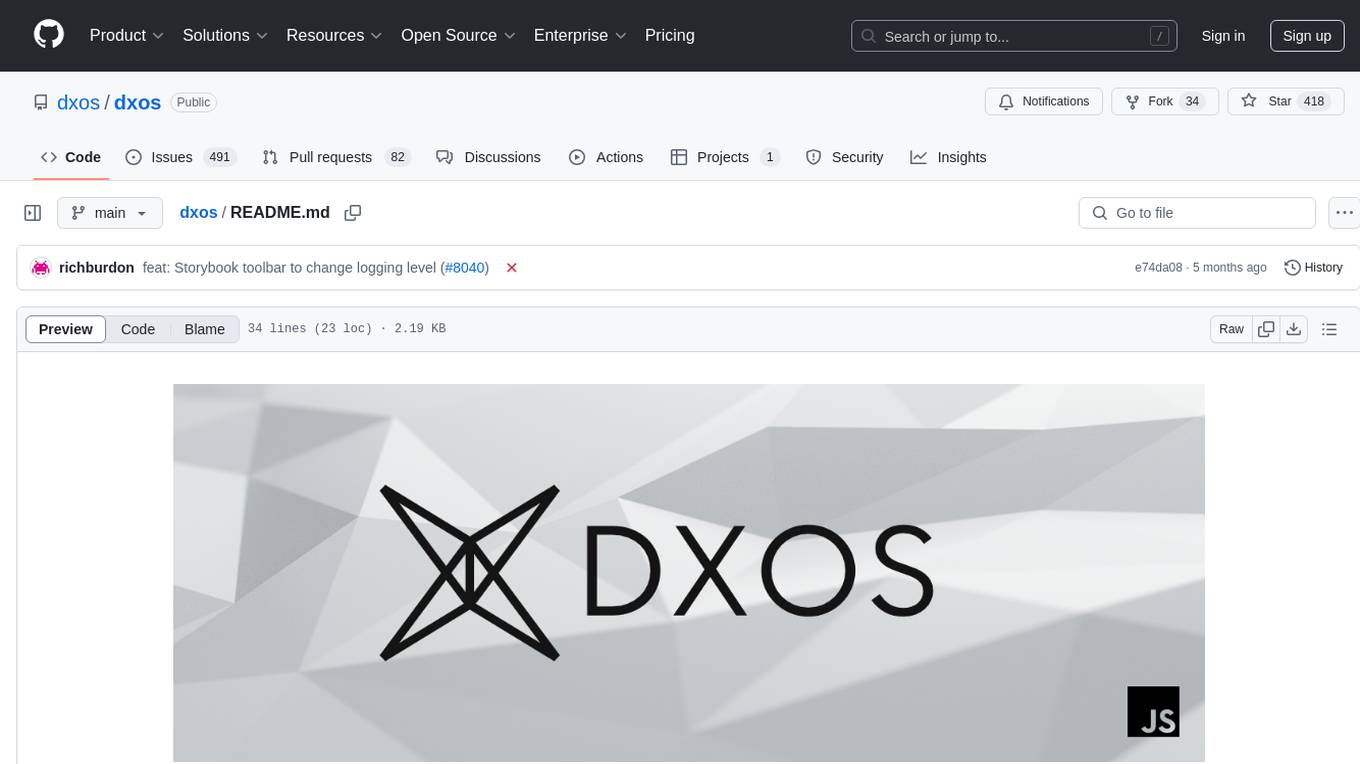
dxos
DXOS is an open-source platform that offers Composer, an extensible app platform for developers to organize and sync their knowledge across devices. It enables real-time or offline collaboration with others, emphasizing a local-first and private approach. The DXOS SDK facilitates peer-to-peer collaboration for local-first apps without relying on central sync servers.
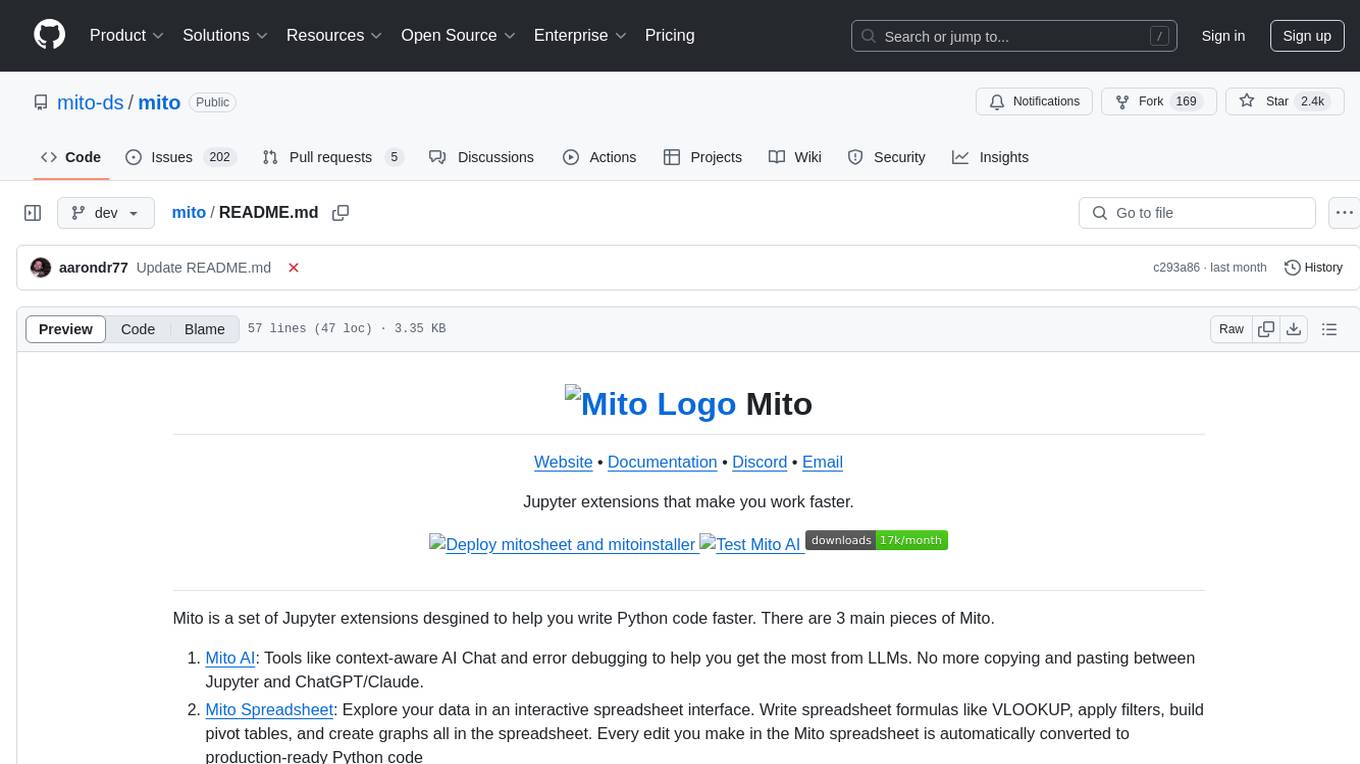
mito
Mito is a set of Jupyter extensions designed to help users write Python code faster. It consists of Mito AI, providing tools like context-aware AI Chat and error debugging; Mito Spreadsheet, enabling data exploration with interactive spreadsheet features; and Mito for Streamlit and Dash, allowing easy integration of spreadsheets into dashboards with minimal code. Mito is open source and community-driven, with options to purchase Mito Pro for further development support.
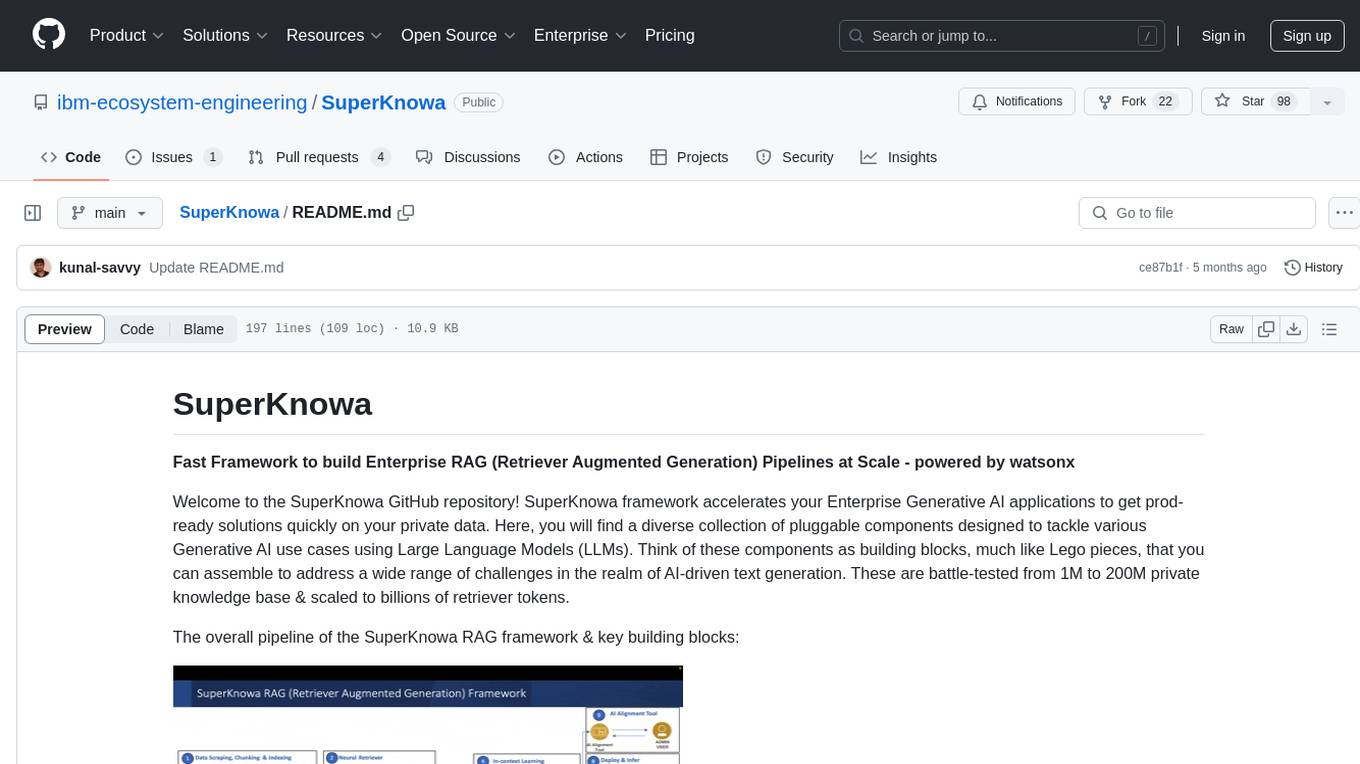
SuperKnowa
SuperKnowa is a fast framework to build Enterprise RAG (Retriever Augmented Generation) Pipelines at Scale, powered by watsonx. It accelerates Enterprise Generative AI applications to get prod-ready solutions quickly on private data. The framework provides pluggable components for tackling various Generative AI use cases using Large Language Models (LLMs), allowing users to assemble building blocks to address challenges in AI-driven text generation. SuperKnowa is battle-tested from 1M to 200M private knowledge base & scaled to billions of retriever tokens.
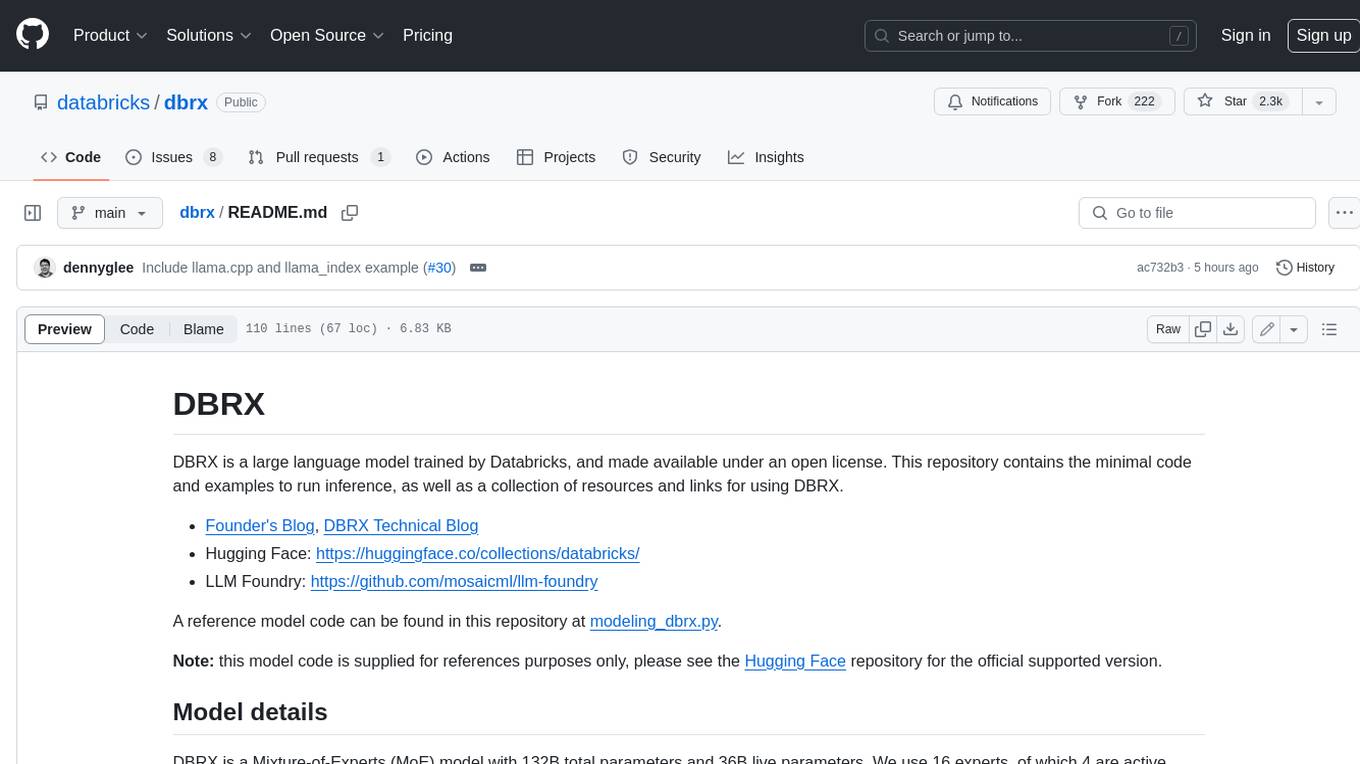
dbrx
DBRX is a large language model trained by Databricks and made available under an open license. It is a Mixture-of-Experts (MoE) model with 132B total parameters and 36B live parameters, using 16 experts, of which 4 are active during training or inference. DBRX was pre-trained for 12T tokens of text and has a context length of 32K tokens. The model is available in two versions: a base model and an Instruct model, which is finetuned for instruction following. DBRX can be used for a variety of tasks, including text generation, question answering, summarization, and translation.
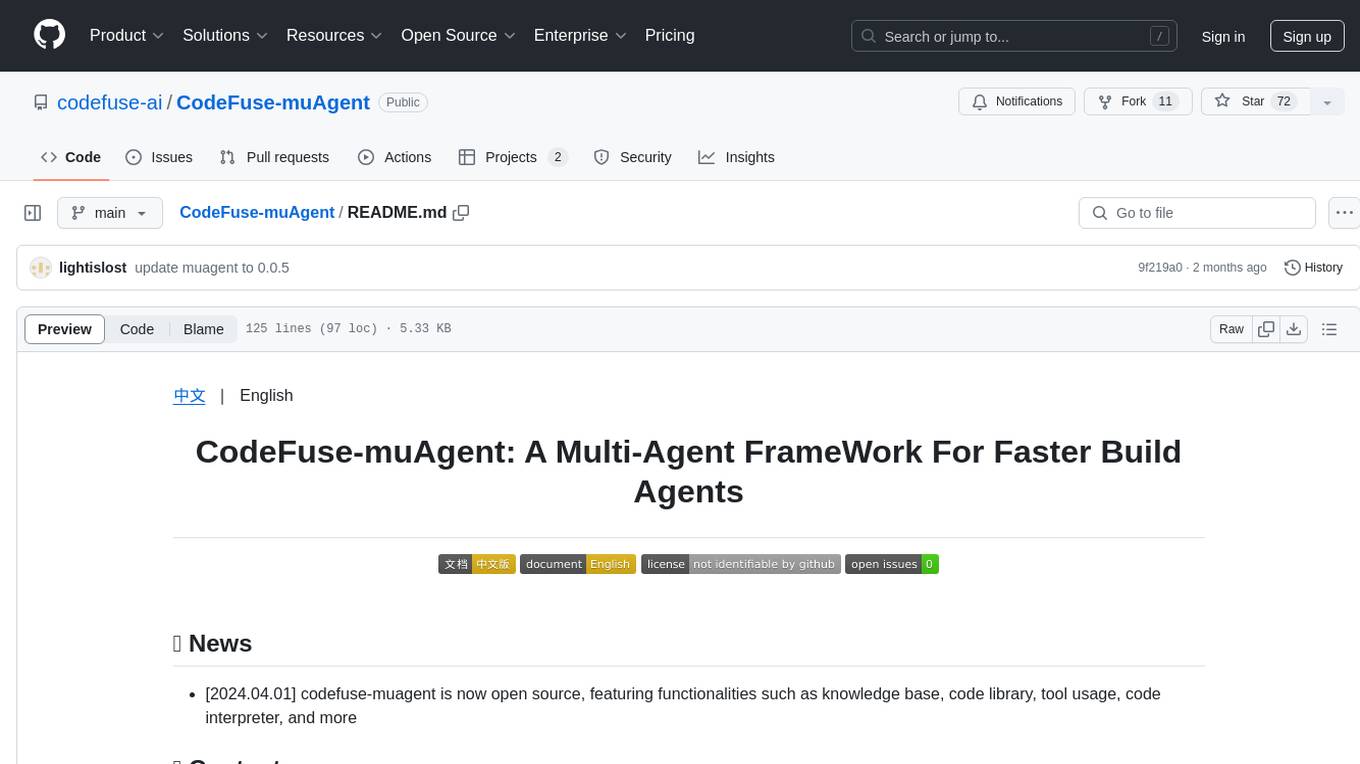
CodeFuse-muAgent
CodeFuse-muAgent is a Multi-Agent framework designed to streamline Standard Operating Procedure (SOP) orchestration for agents. It integrates toolkits, code libraries, knowledge bases, and sandbox environments for rapid construction of complex Multi-Agent interactive applications. The framework enables efficient execution and handling of multi-layered and multi-dimensional tasks.
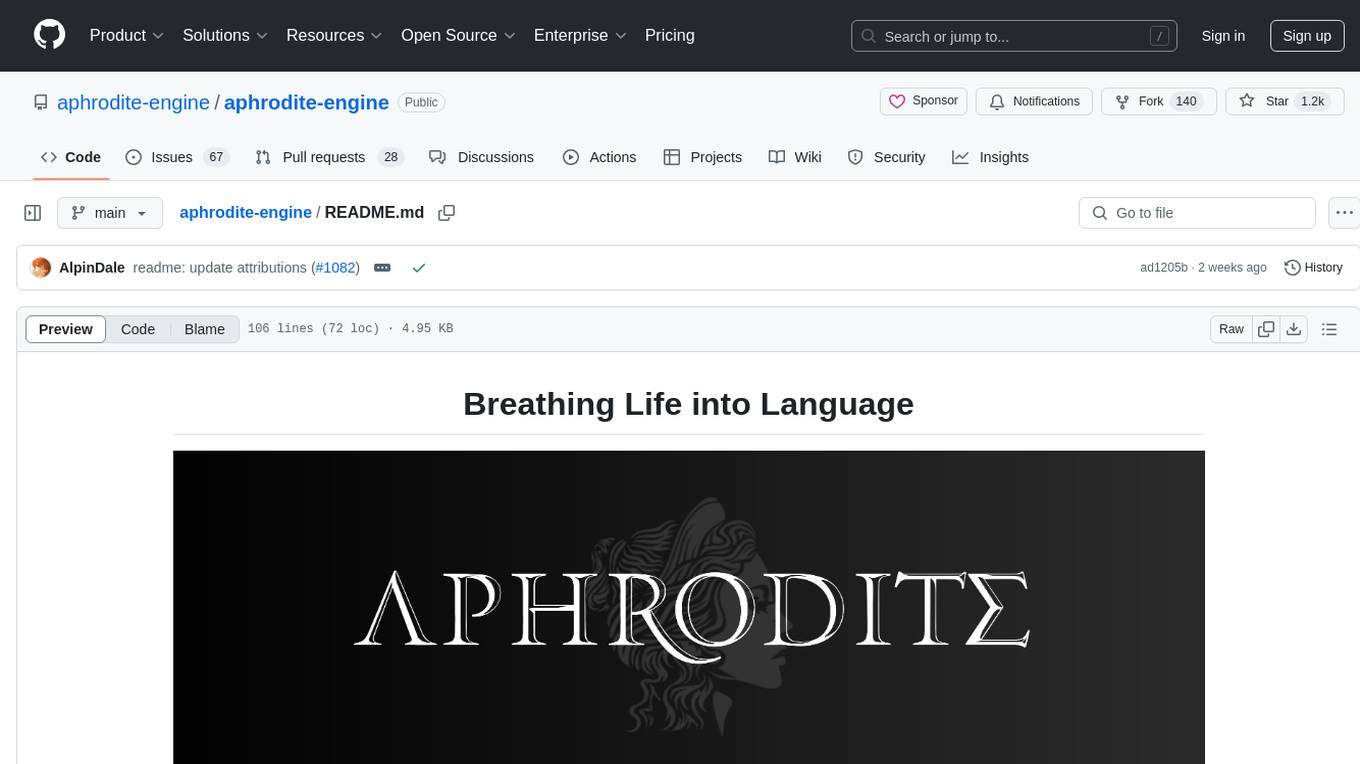
aphrodite-engine
Aphrodite is an inference engine optimized for serving HuggingFace-compatible models at scale. It leverages vLLM's Paged Attention technology to deliver high-performance model inference for multiple concurrent users. The engine supports continuous batching, efficient key/value management, optimized CUDA kernels, quantization support, distributed inference, and modern samplers. It can be easily installed and launched, with Docker support for deployment. Aphrodite requires Linux or Windows OS, Python 3.8 to 3.12, and CUDA >= 11. It is designed to utilize 90% of GPU VRAM but offers options to limit memory usage. Contributors are welcome to enhance the engine.
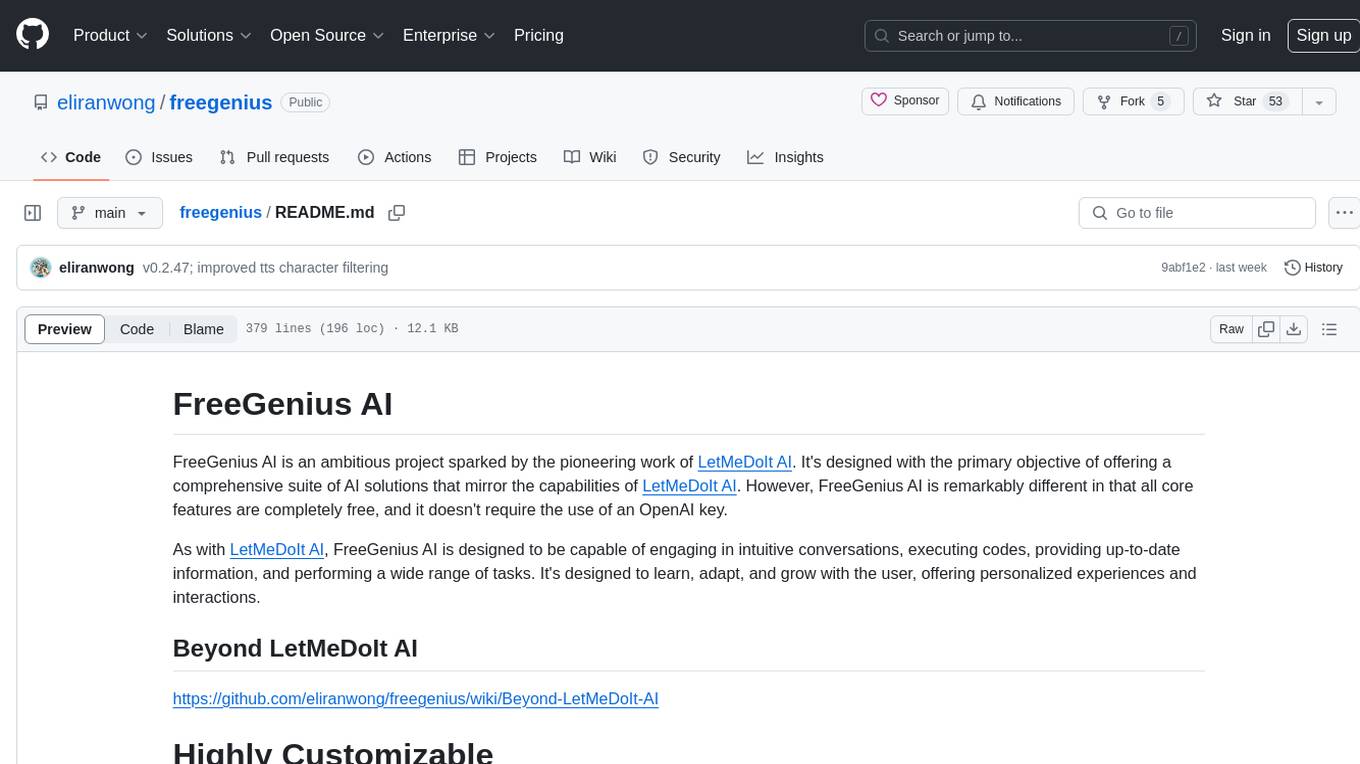
freegenius
FreeGenius AI is an ambitious project offering a comprehensive suite of AI solutions that mirror the capabilities of LetMeDoIt AI. It is designed to engage in intuitive conversations, execute codes, provide up-to-date information, and perform various tasks. The tool is free, customizable, and provides access to real-time data and device information. It aims to support offline and online backends, open-source large language models, and optional API keys. Users can use FreeGenius AI for tasks like generating tweets, analyzing audio, searching financial data, checking weather, and creating maps.
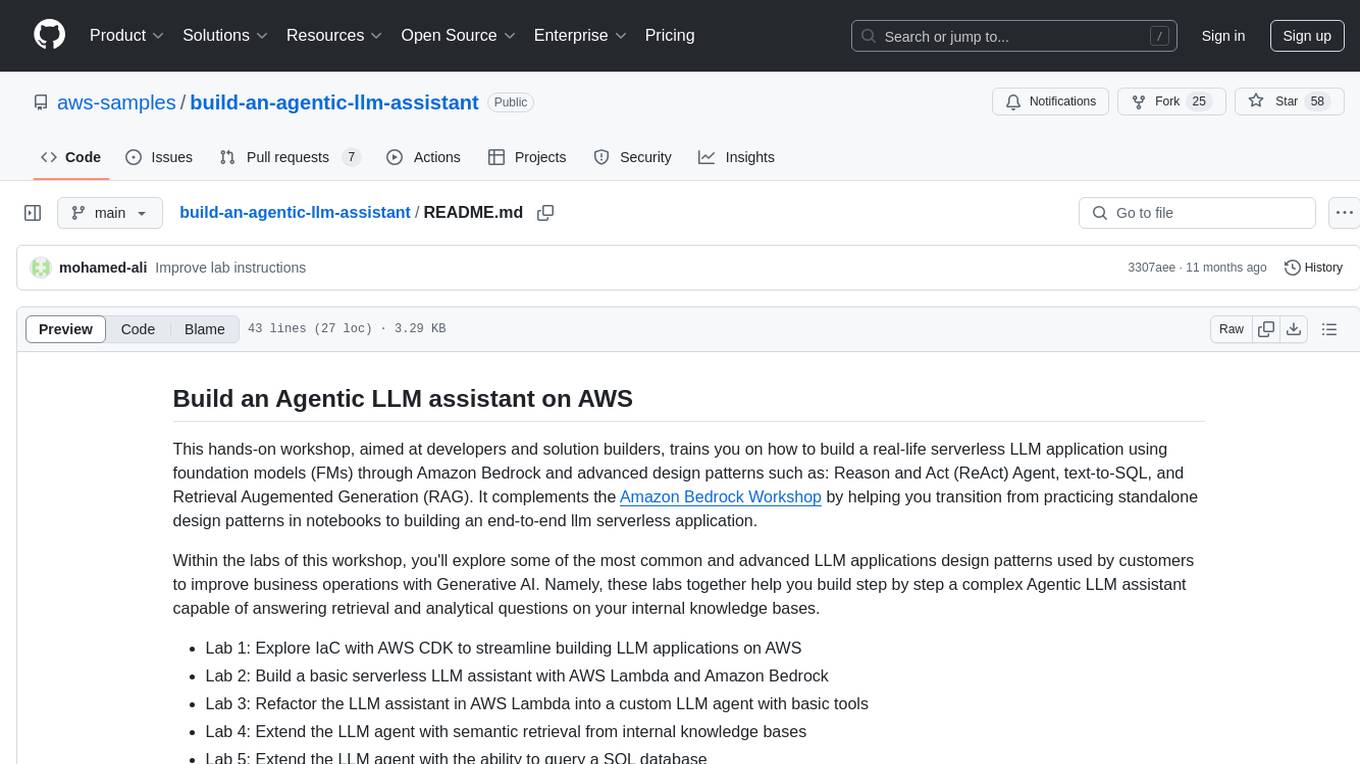
build-an-agentic-llm-assistant
This repository provides a hands-on workshop for developers and solution builders to build a real-life serverless LLM application using foundation models (FMs) through Amazon Bedrock and advanced design patterns such as Reason and Act (ReAct) Agent, text-to-SQL, and Retrieval Augmented Generation (RAG). It guides users through labs to explore common and advanced LLM application design patterns, helping them build a complex Agentic LLM assistant capable of answering retrieval and analytical questions on internal knowledge bases. The repository includes labs on IaC with AWS CDK, building serverless LLM assistants with AWS Lambda and Amazon Bedrock, refactoring LLM assistants into custom agents, extending agents with semantic retrieval, and querying SQL databases. Users need to set up AWS Cloud9, configure model access on Amazon Bedrock, and use Amazon SageMaker Studio environment to run data-pipelines notebooks.
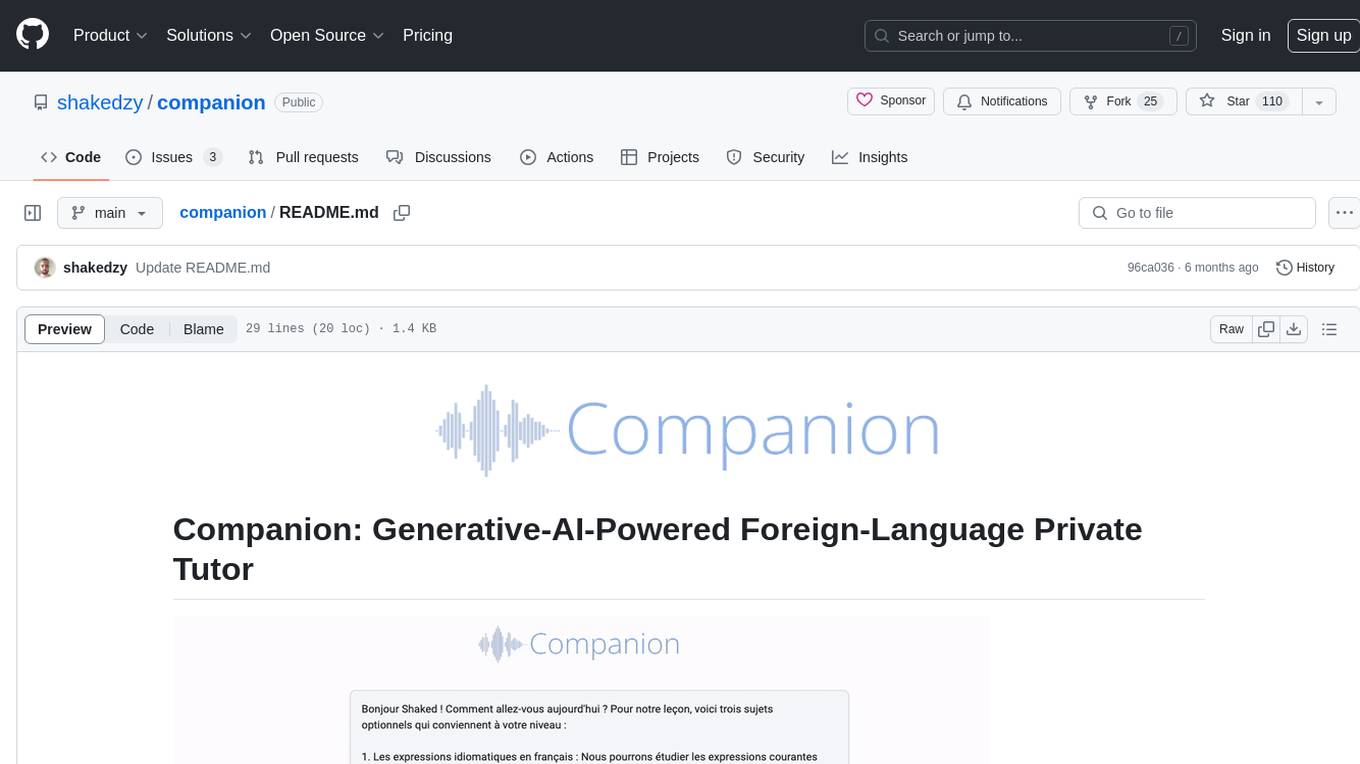
companion
Companion is a generative AI-powered tool that serves as a private tutor for learning a new foreign language. It utilizes OpenAI ChatGPT & Whisper and Google Text-to-Speech & Translate to enable users to write, talk, read, and listen in both their native language and the selected foreign language. The tool is designed to correct any mistakes made by the user and can be run locally or as a cloud service, making it accessible on mobile devices. Companion is distributed for non-commercial usage, but users should be aware that some of the APIs and services it relies on may incur charges based on usage.
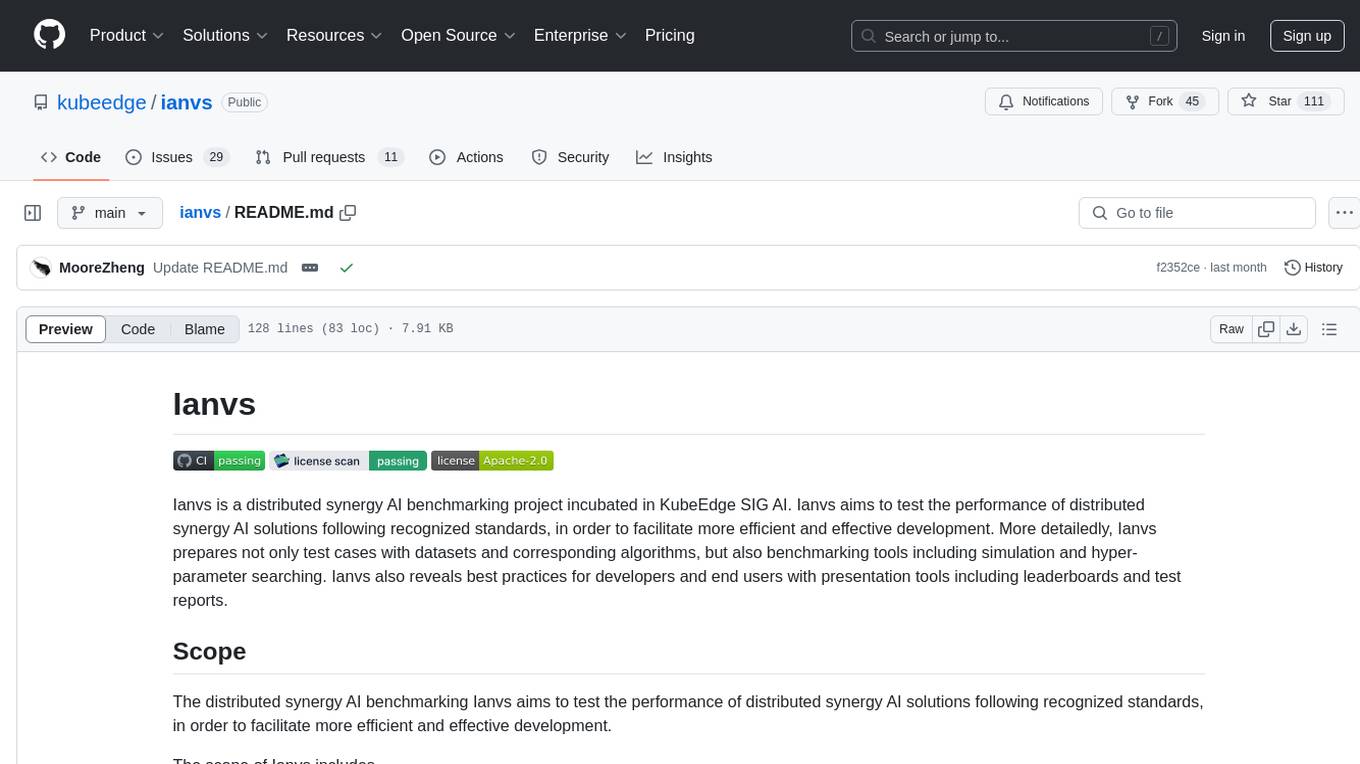
ianvs
Ianvs is a distributed synergy AI benchmarking project incubated in KubeEdge SIG AI. It aims to test the performance of distributed synergy AI solutions following recognized standards, providing end-to-end benchmark toolkits, test environment management tools, test case control tools, and benchmark presentation tools. It also collaborates with other organizations to establish comprehensive benchmarks and related applications. The architecture includes critical components like Test Environment Manager, Test Case Controller, Generation Assistant, Simulation Controller, and Story Manager. Ianvs documentation covers quick start, guides, dataset descriptions, algorithms, user interfaces, stories, and roadmap.
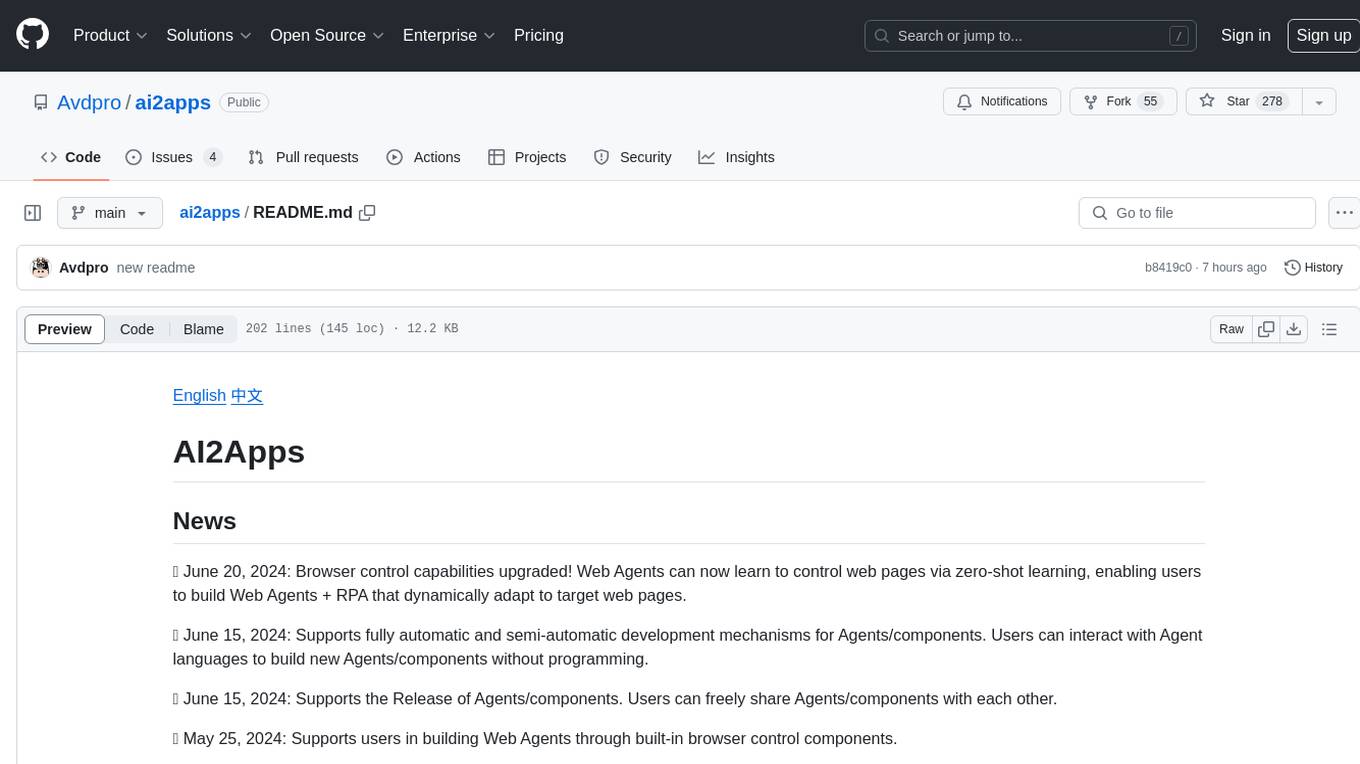
ai2apps
AI2Apps is a visual IDE for building LLM-based AI agent applications, enabling developers to efficiently create AI agents through drag-and-drop, with features like design-to-development for rapid prototyping, direct packaging of agents into apps, powerful debugging capabilities, enhanced user interaction, efficient team collaboration, flexible deployment, multilingual support, simplified product maintenance, and extensibility through plugins.
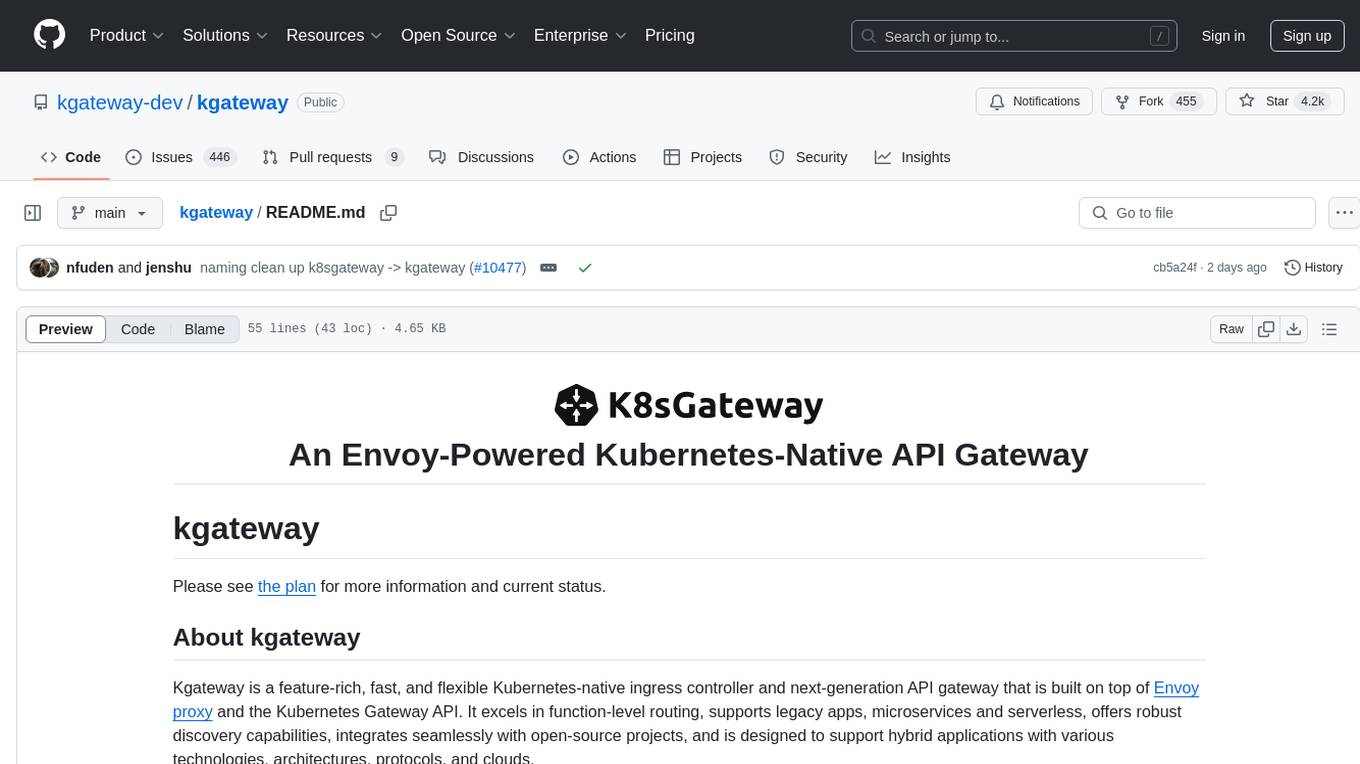
kgateway
Kgateway is a feature-rich, fast, and flexible Kubernetes-native API gateway built on top of Envoy proxy and the Kubernetes Gateway API. It excels in function-level routing, supports legacy apps, microservices, and serverless, offers robust discovery capabilities, integrates seamlessly with open-source projects, and is designed to support hybrid applications with various technologies, architectures, protocols, and clouds.
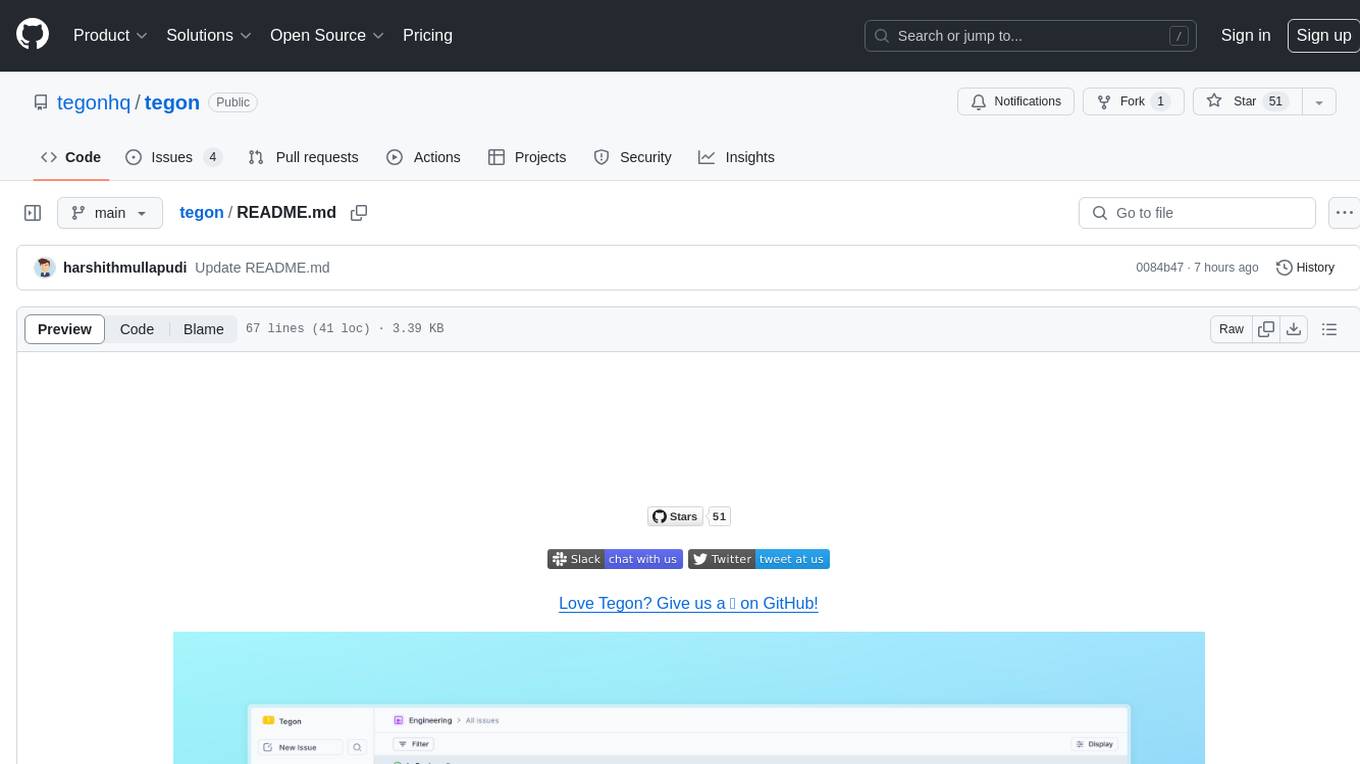
tegon
Tegon is an open-source AI-First issue tracking tool designed for engineering teams. It aims to simplify task management by leveraging AI and integrations to automate task creation, prioritize tasks, and enhance bug resolution. Tegon offers features like issues tracking, automatic title generation, AI-generated labels and assignees, custom views, and upcoming features like sprints and task prioritization. It integrates with GitHub, Slack, and Sentry to streamline issue tracking processes. Tegon also plans to introduce AI Agents like PR Agent and Bug Agent to enhance product management and bug resolution. Contributions are welcome, and the product is licensed under the MIT License.
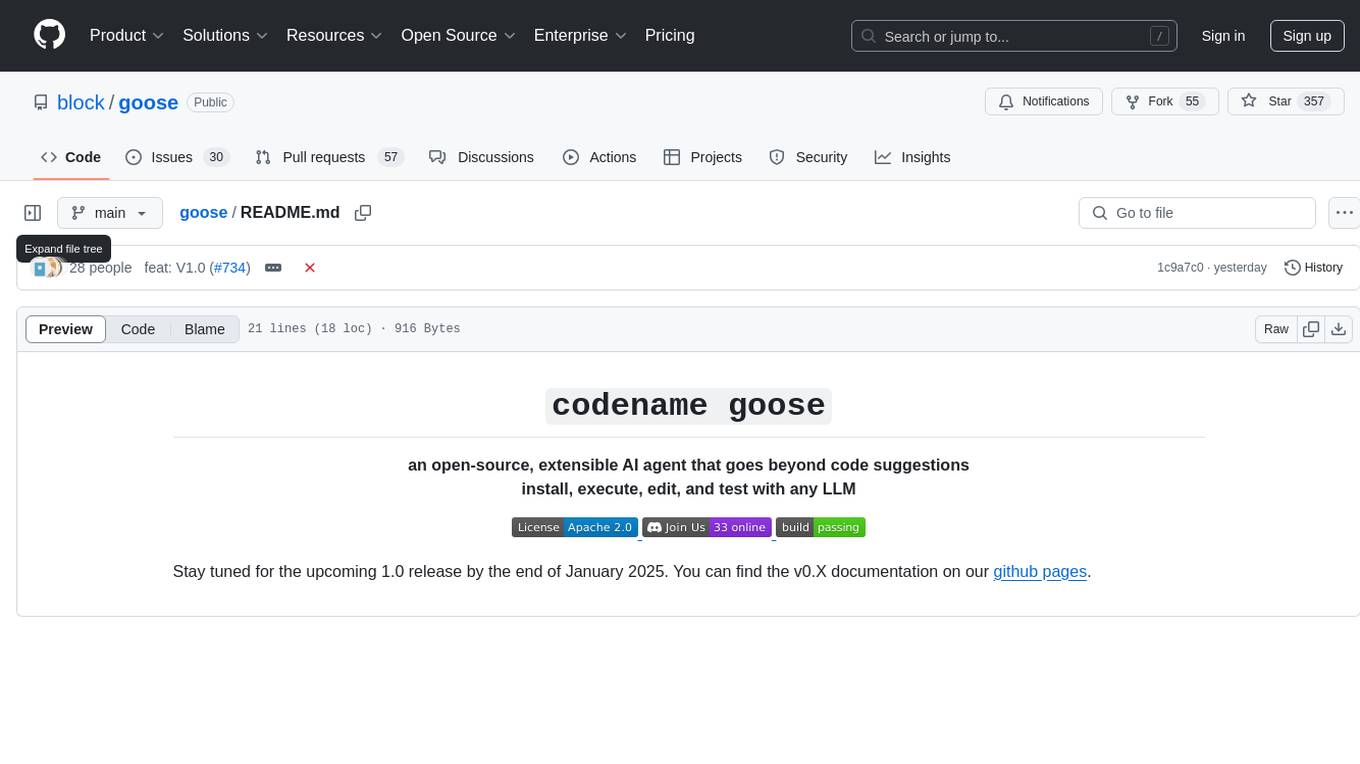
goose
Codename Goose is an open-source, extensible AI agent designed to provide functionalities beyond code suggestions. Users can install, execute, edit, and test with any LLM. The tool aims to enhance the coding experience by offering advanced features and capabilities. Stay updated for the upcoming 1.0 release scheduled by the end of January 2025. Explore the v0.X documentation available on the project's GitHub pages.
For similar tasks
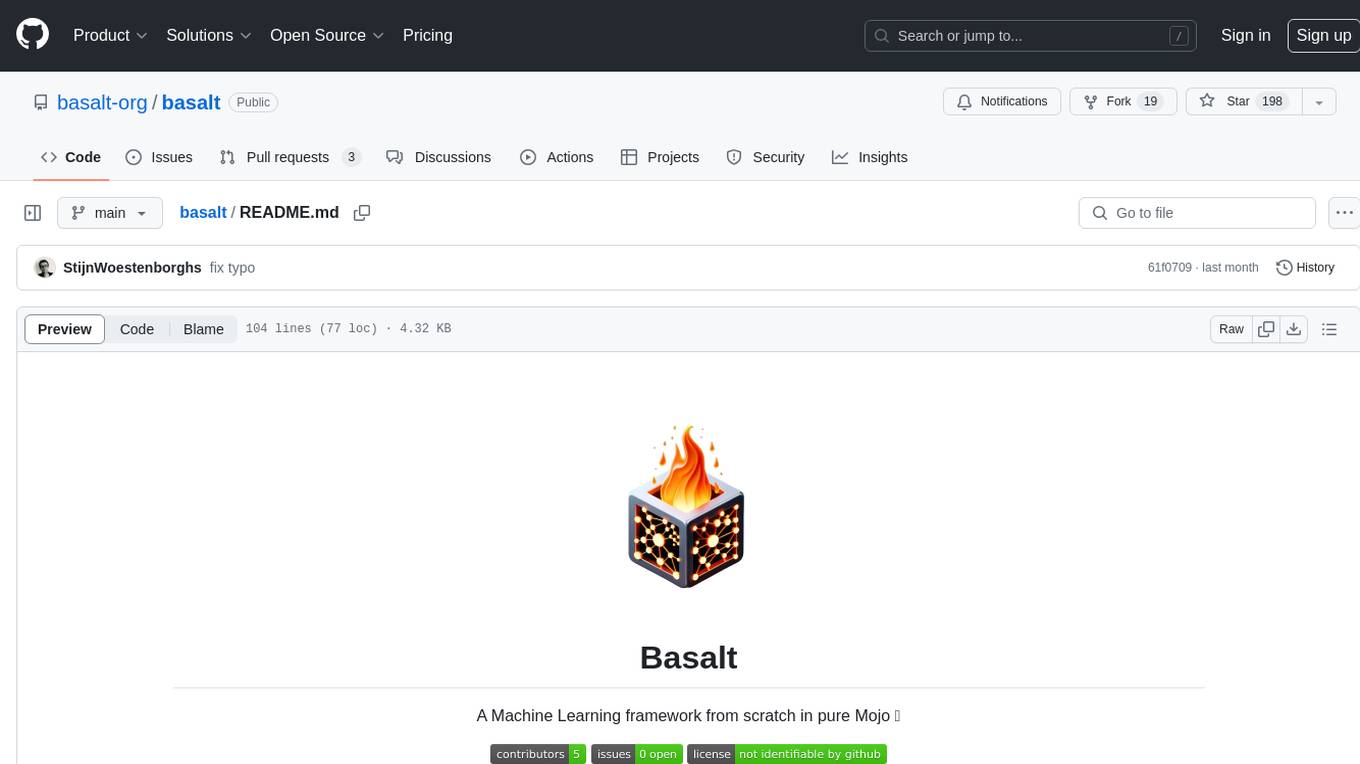
basalt
Basalt is a lightweight and flexible CSS framework designed to help developers quickly build responsive and modern websites. It provides a set of pre-designed components and utilities that can be easily customized to create unique and visually appealing web interfaces. With Basalt, developers can save time and effort by leveraging its modular structure and responsive design principles to create professional-looking websites with ease.
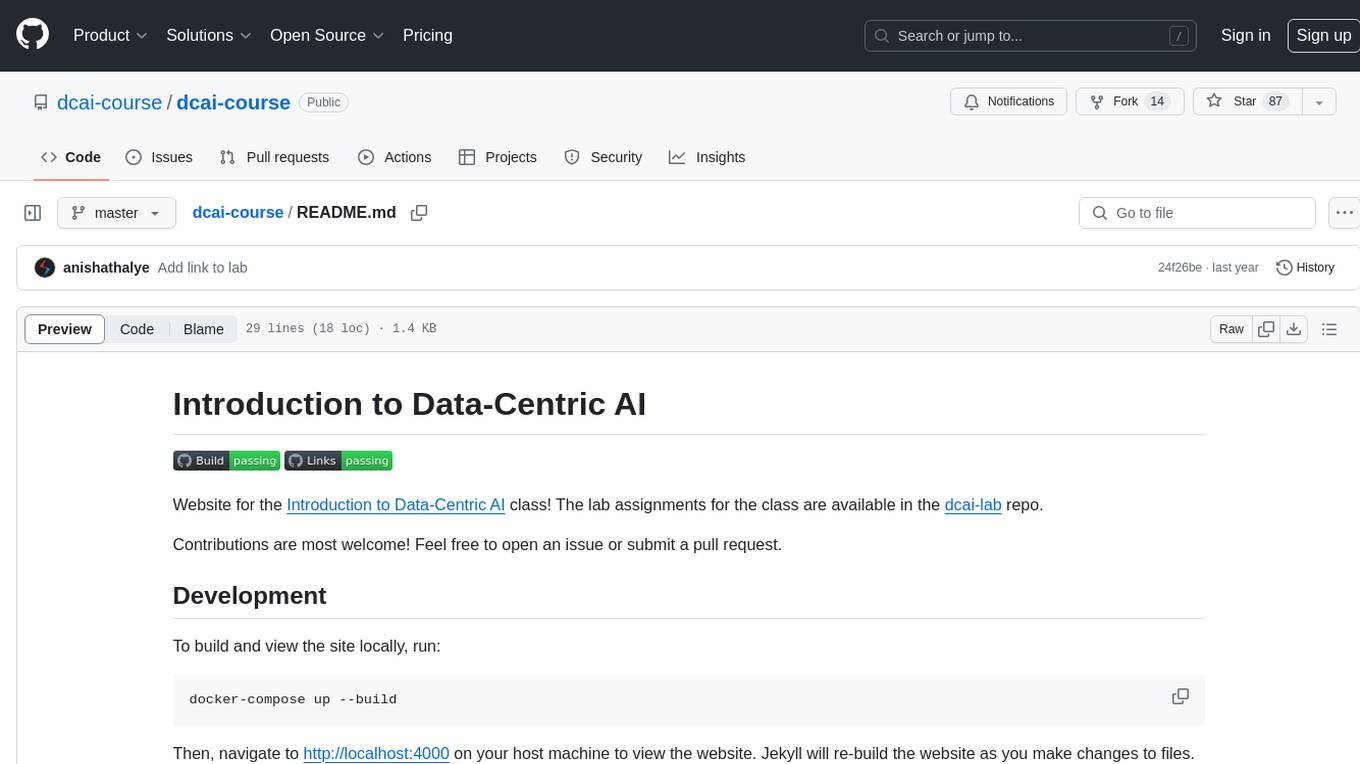
dcai-course
This repository serves as the website for the Introduction to Data-Centric AI class. It contains lab assignments and resources for the course. Users can contribute by opening issues or submitting pull requests. The website can be built locally using Docker and Jekyll. The design is based on Missing Semester. All contents, including source code, lecture notes, and videos, are licensed under CC BY-NC-SA 4.0.
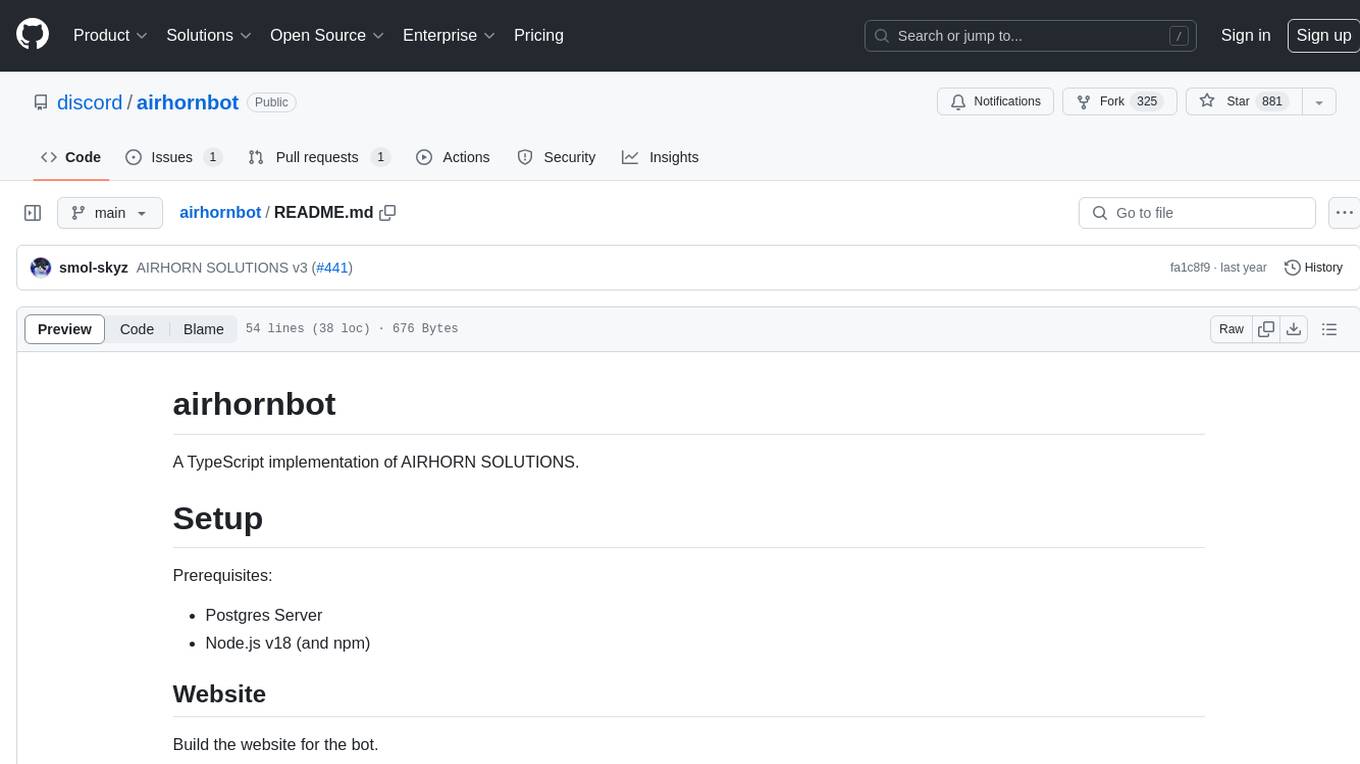
airhornbot
airhornbot is a TypeScript implementation of AIRHORN SOLUTIONS. It includes a website and a bot with a web server process. The setup requires a Postgres Server and Node.js v18. The website can be built using npm commands, and the bot can be built and run using npx commands after setting up the environment variables in the .env file.
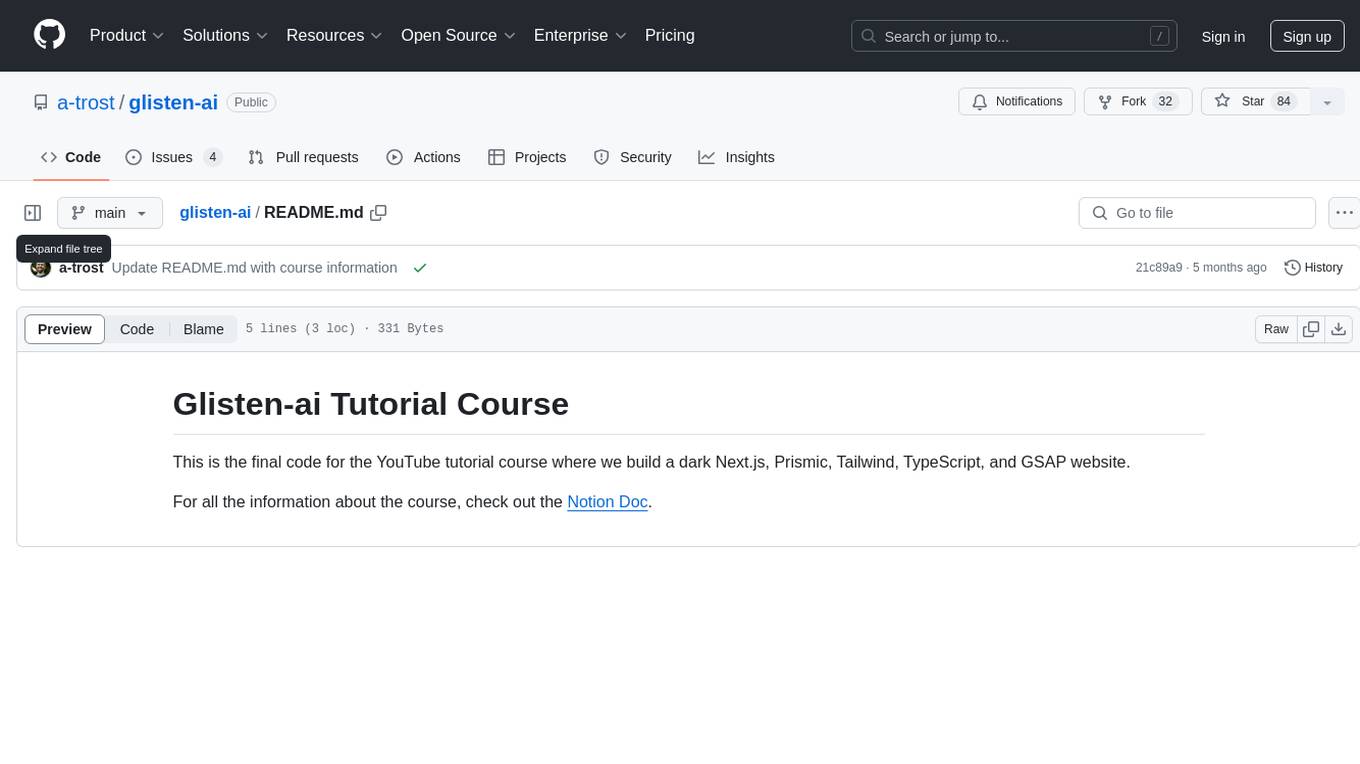
glisten-ai
Glisten-ai Tutorial Course is the final code for a YouTube tutorial course demonstrating the creation of a dark Next.js, Prismic, Tailwind, TypeScript, and GSAP website. The repository contains the code used in the tutorial, providing a practical example for building websites using these technologies.
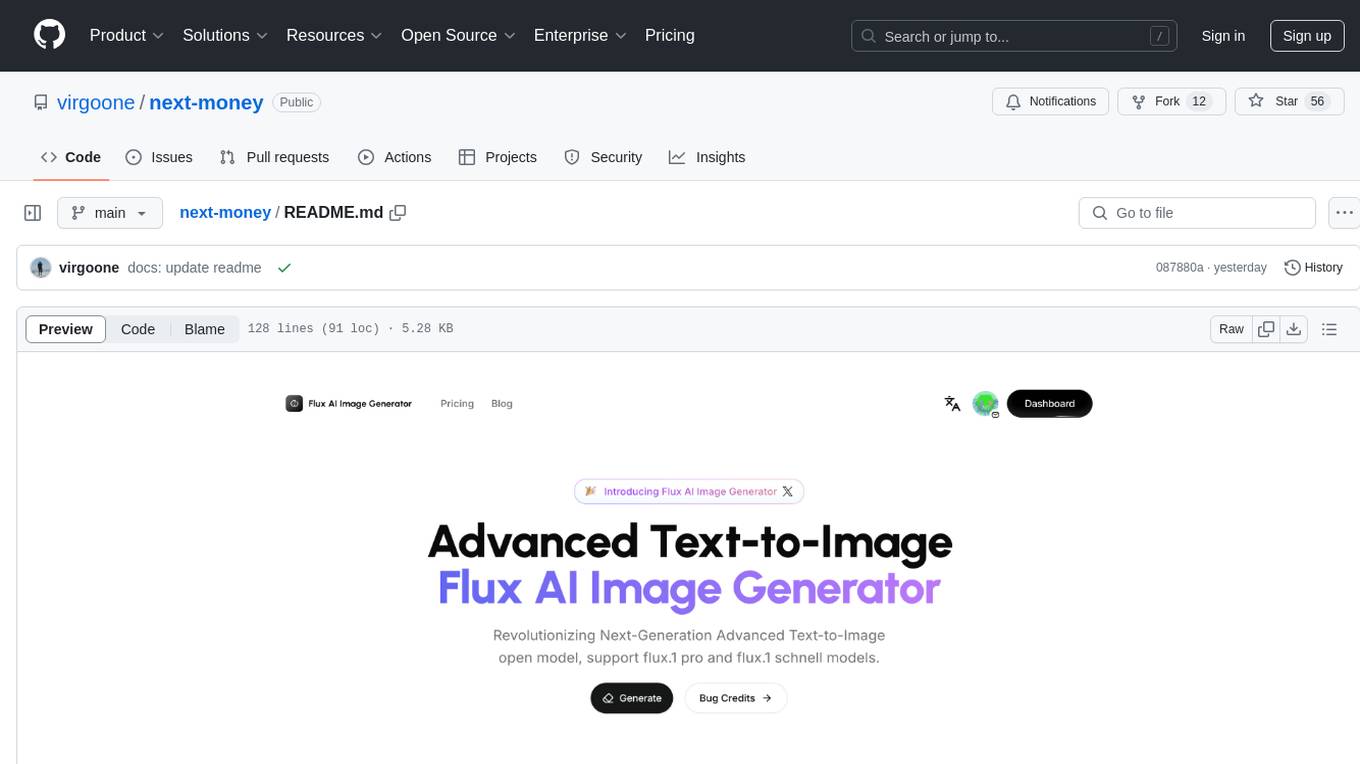
next-money
Next Money Stripe Starter is a SaaS Starter project that empowers your next project with a stack of Next.js, Prisma, Supabase, Clerk Auth, Resend, React Email, Shadcn/ui, and Stripe. It seamlessly integrates these technologies to accelerate your development and SaaS journey. The project includes frameworks, platforms, UI components, hooks and utilities, code quality tools, and miscellaneous features to enhance the development experience. Created by @koyaguo in 2023 and released under the MIT license.
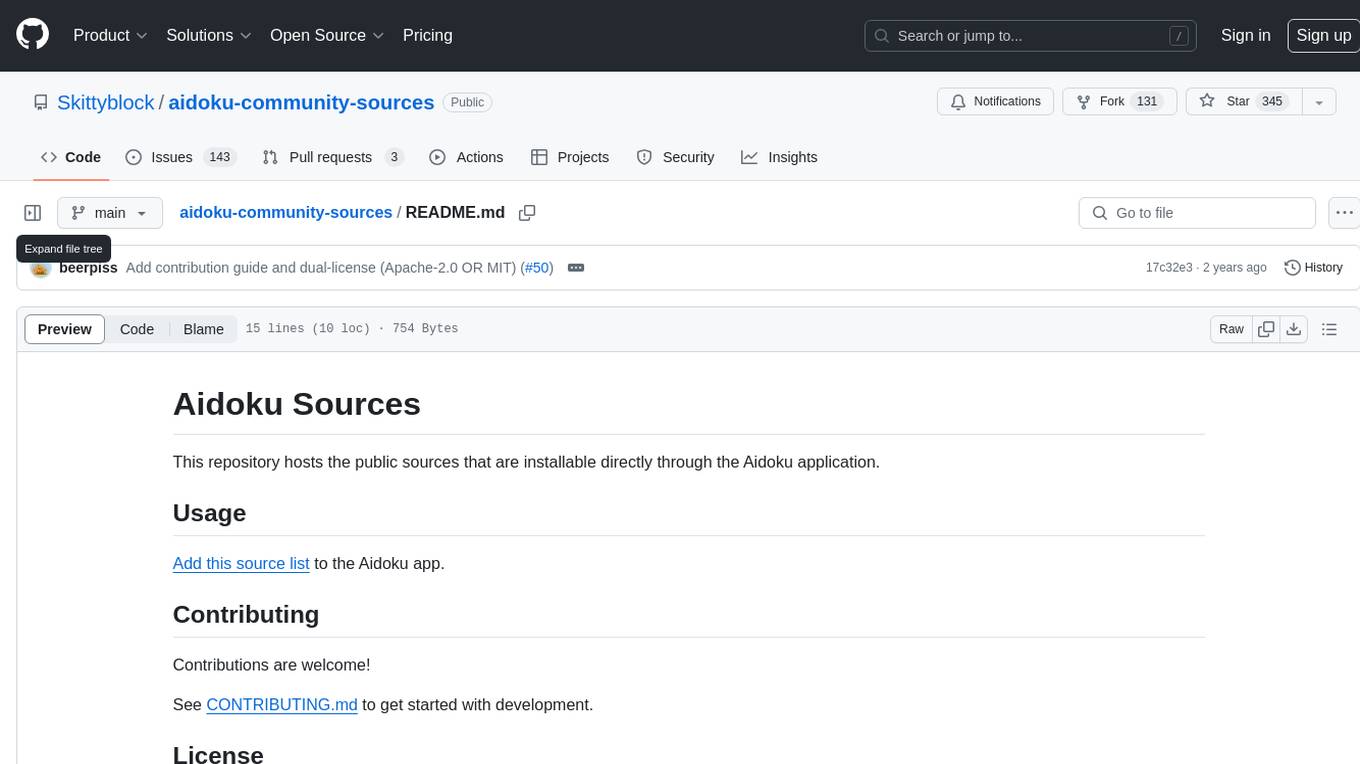
aidoku-community-sources
Aidoku Sources is a repository containing public sources that can be directly installed through the Aidoku application. Users can add this source list to the Aidoku app to access additional content. Contributions to the repository are welcome, and it is licensed under either the Apache License, version 2.0, or the MIT license.
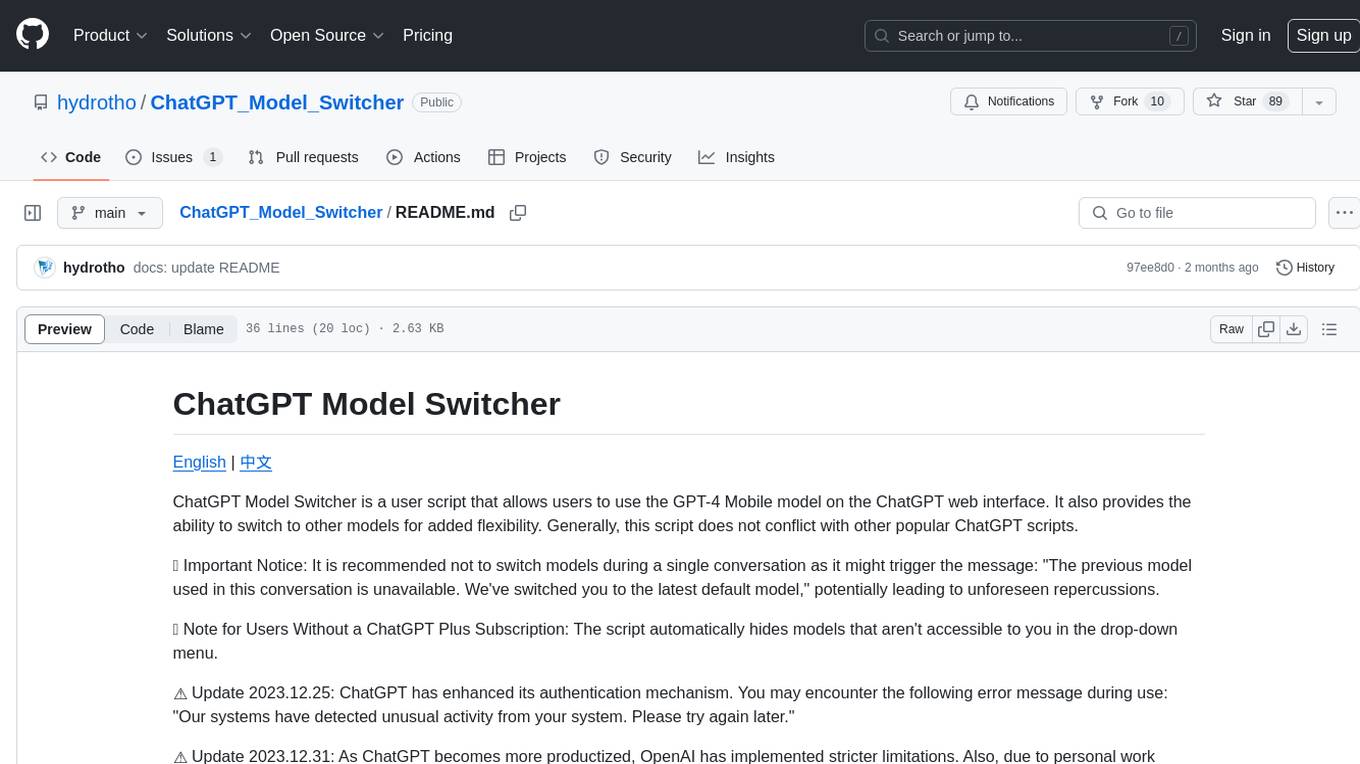
ChatGPT_Model_Switcher
ChatGPT Model Switcher is a user script that enables users to utilize the GPT-4 Mobile model on the ChatGPT web interface and switch to other models for added flexibility. It ensures compatibility with multiple mirror sites and hides inaccessible models for non-subscribers. However, recent updates have introduced stricter limitations due to enhanced authentication mechanisms and personal constraints, potentially affecting the project's ability to override usage limits. Users are encouraged to contribute to the project if capable.
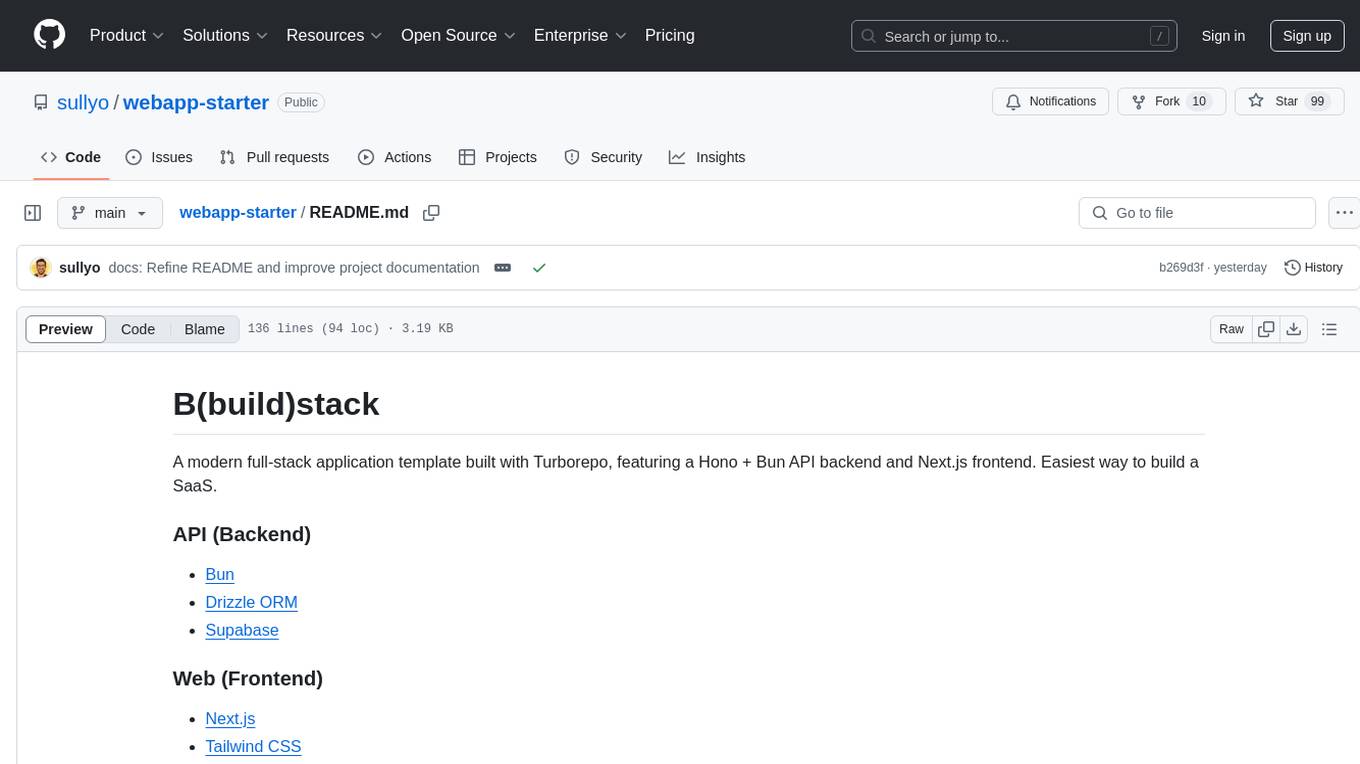
webapp-starter
webapp-starter is a modern full-stack application template built with Turborepo, featuring a Hono + Bun API backend and Next.js frontend. It provides an easy way to build a SaaS product. The backend utilizes technologies like Bun, Drizzle ORM, and Supabase, while the frontend is built with Next.js, Tailwind CSS, Shadcn/ui, and Clerk. Deployment can be done using Vercel and Render. The project structure includes separate directories for API backend and Next.js frontend, along with shared packages for the main database. Setup involves installing dependencies, configuring environment variables, and setting up services like Bun, Supabase, and Clerk. Development can be done using 'turbo dev' command, and deployment instructions are provided for Vercel and Render. Contributions are welcome through pull requests.
For similar jobs
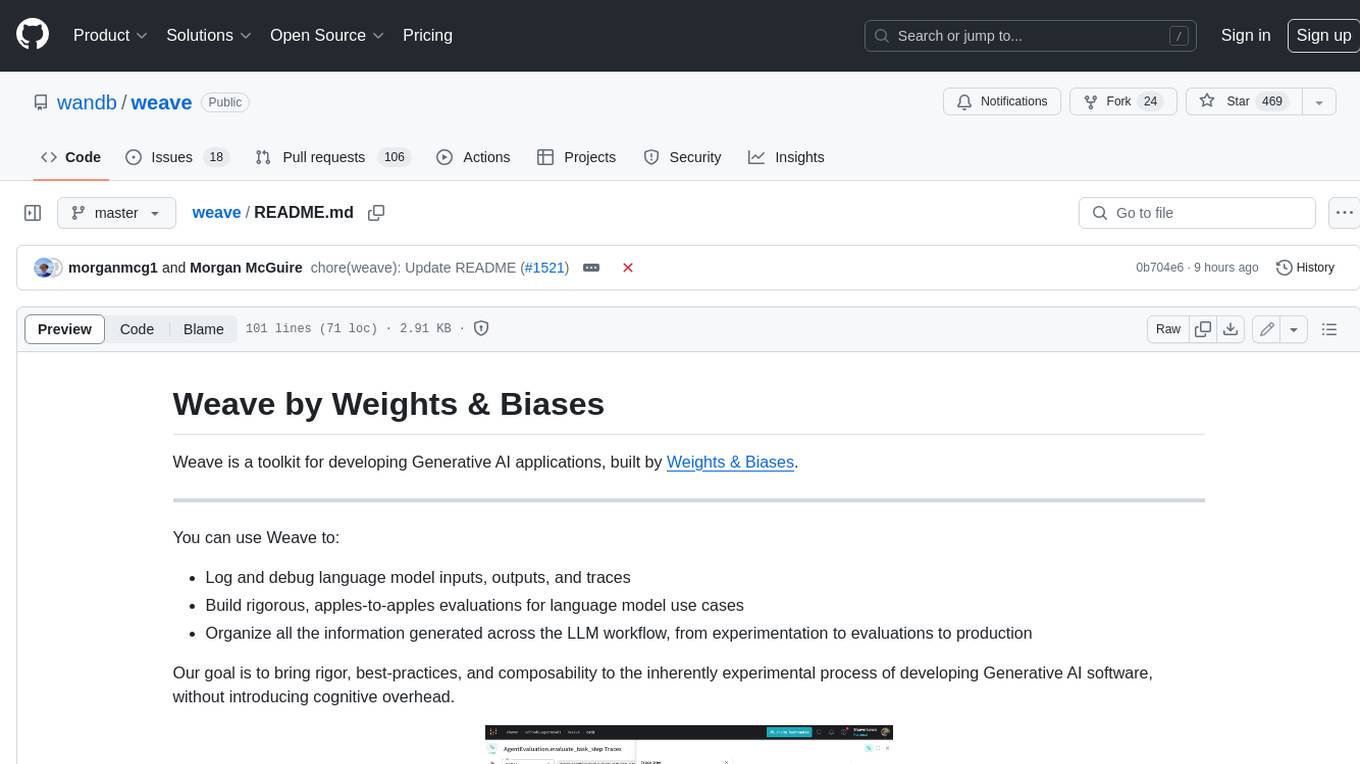
weave
Weave is a toolkit for developing Generative AI applications, built by Weights & Biases. With Weave, you can log and debug language model inputs, outputs, and traces; build rigorous, apples-to-apples evaluations for language model use cases; and organize all the information generated across the LLM workflow, from experimentation to evaluations to production. Weave aims to bring rigor, best-practices, and composability to the inherently experimental process of developing Generative AI software, without introducing cognitive overhead.
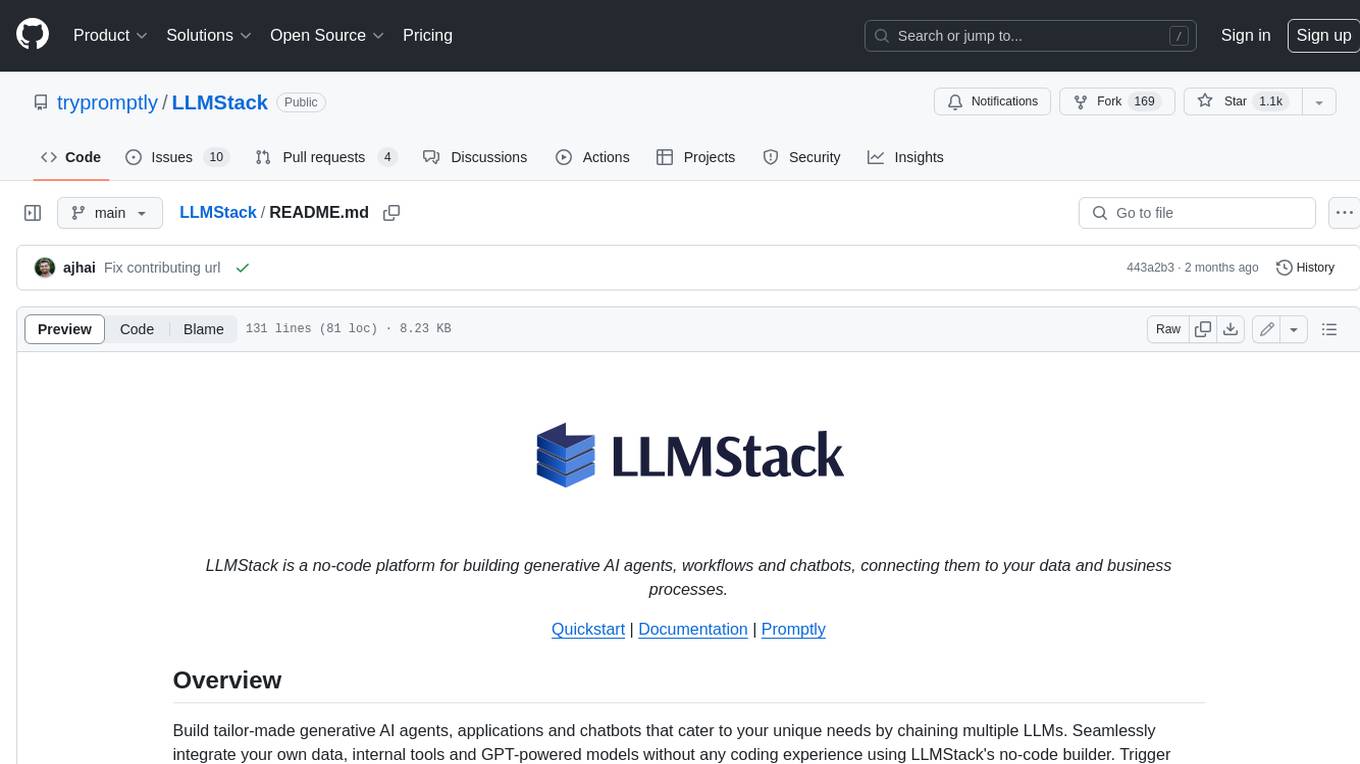
LLMStack
LLMStack is a no-code platform for building generative AI agents, workflows, and chatbots. It allows users to connect their own data, internal tools, and GPT-powered models without any coding experience. LLMStack can be deployed to the cloud or on-premise and can be accessed via HTTP API or triggered from Slack or Discord.
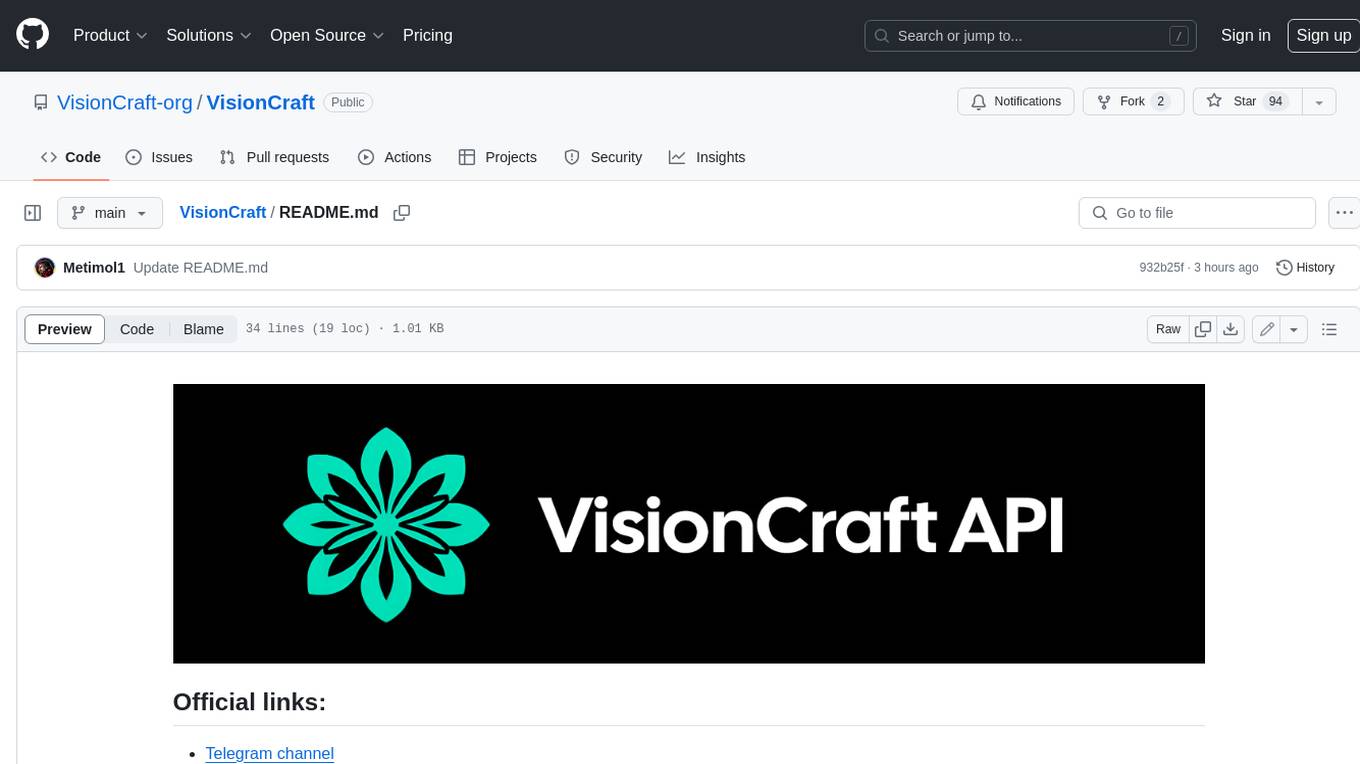
VisionCraft
The VisionCraft API is a free API for using over 100 different AI models. From images to sound.
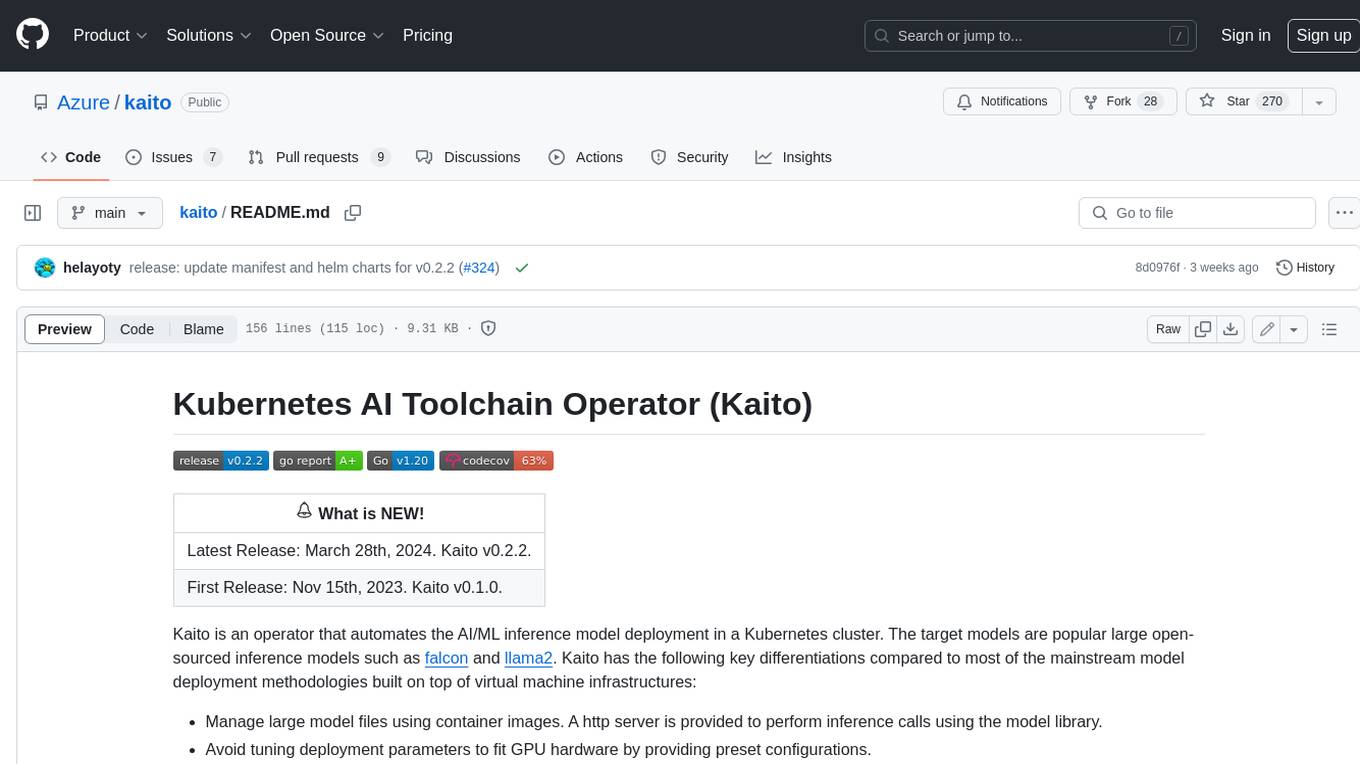
kaito
Kaito is an operator that automates the AI/ML inference model deployment in a Kubernetes cluster. It manages large model files using container images, avoids tuning deployment parameters to fit GPU hardware by providing preset configurations, auto-provisions GPU nodes based on model requirements, and hosts large model images in the public Microsoft Container Registry (MCR) if the license allows. Using Kaito, the workflow of onboarding large AI inference models in Kubernetes is largely simplified.
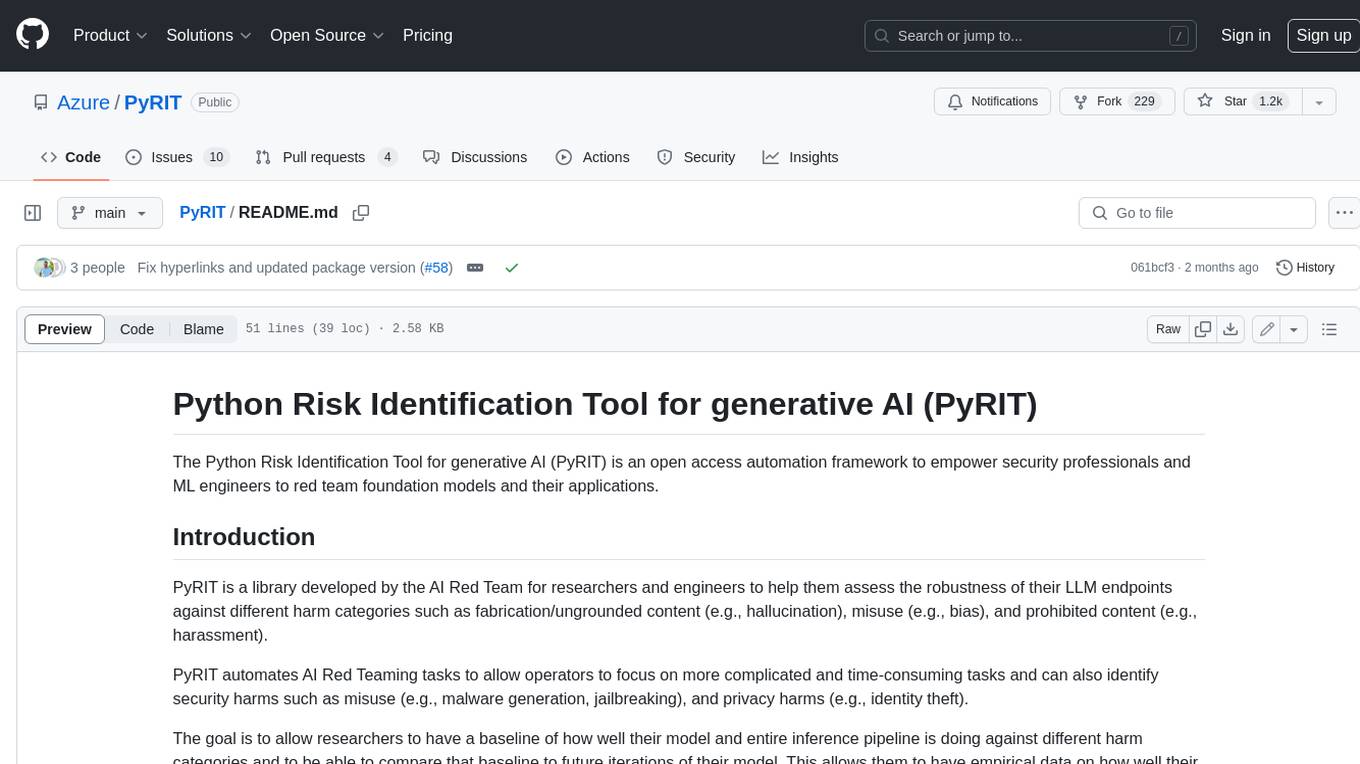
PyRIT
PyRIT is an open access automation framework designed to empower security professionals and ML engineers to red team foundation models and their applications. It automates AI Red Teaming tasks to allow operators to focus on more complicated and time-consuming tasks and can also identify security harms such as misuse (e.g., malware generation, jailbreaking), and privacy harms (e.g., identity theft). The goal is to allow researchers to have a baseline of how well their model and entire inference pipeline is doing against different harm categories and to be able to compare that baseline to future iterations of their model. This allows them to have empirical data on how well their model is doing today, and detect any degradation of performance based on future improvements.
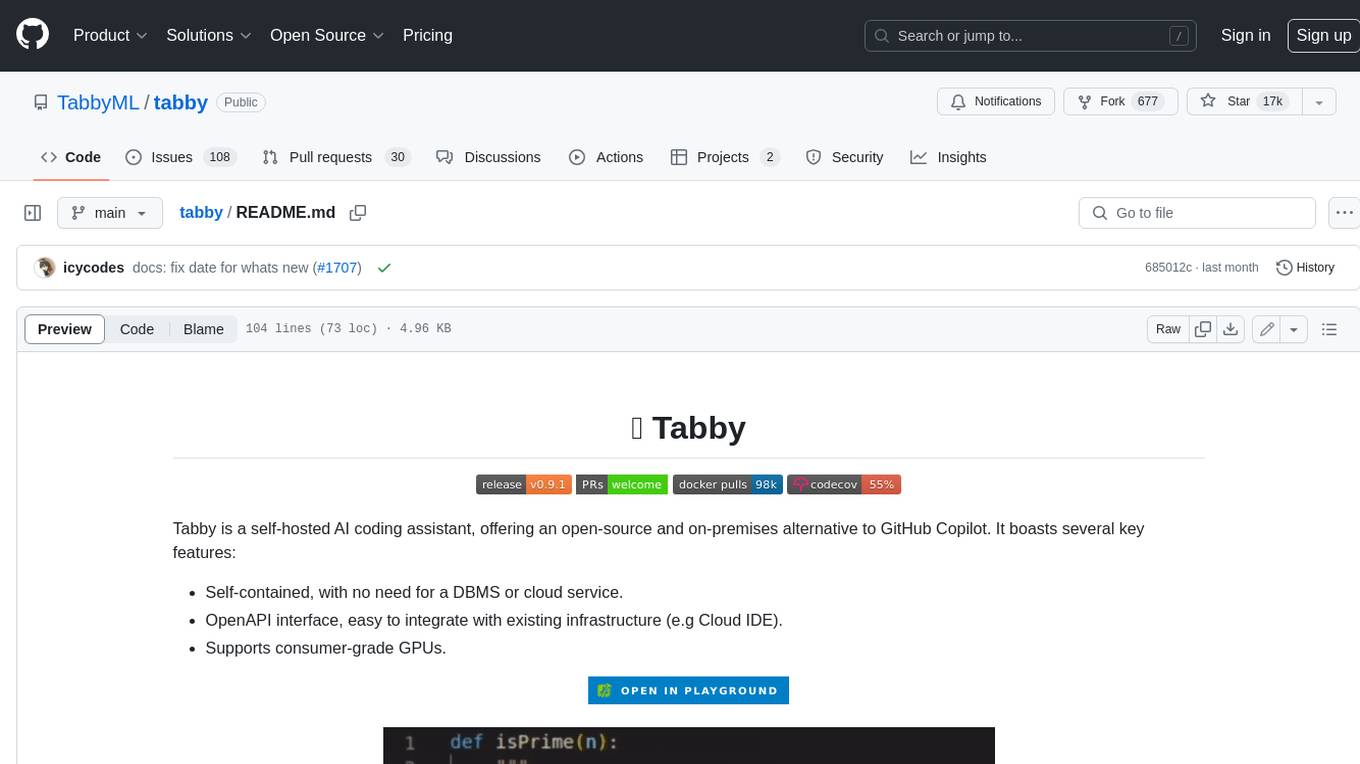
tabby
Tabby is a self-hosted AI coding assistant, offering an open-source and on-premises alternative to GitHub Copilot. It boasts several key features: * Self-contained, with no need for a DBMS or cloud service. * OpenAPI interface, easy to integrate with existing infrastructure (e.g Cloud IDE). * Supports consumer-grade GPUs.
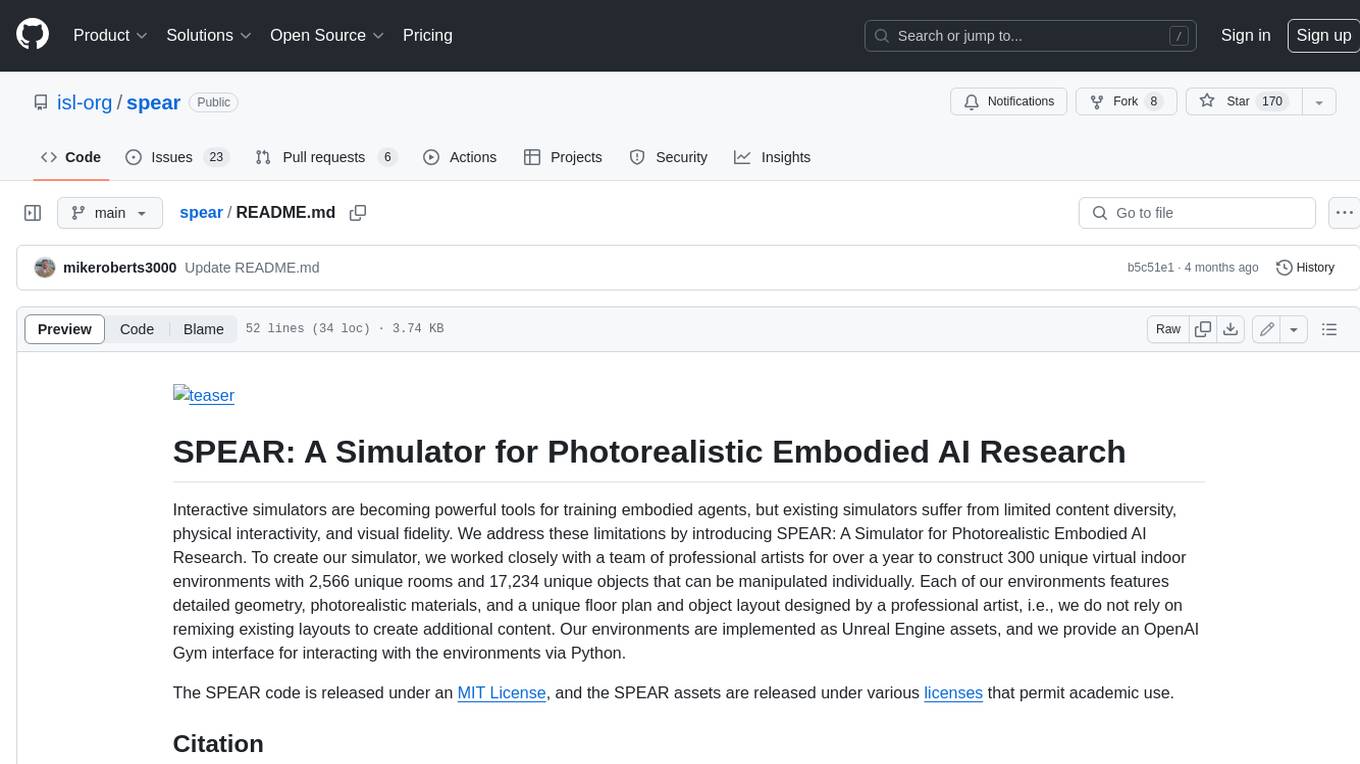
spear
SPEAR (Simulator for Photorealistic Embodied AI Research) is a powerful tool for training embodied agents. It features 300 unique virtual indoor environments with 2,566 unique rooms and 17,234 unique objects that can be manipulated individually. Each environment is designed by a professional artist and features detailed geometry, photorealistic materials, and a unique floor plan and object layout. SPEAR is implemented as Unreal Engine assets and provides an OpenAI Gym interface for interacting with the environments via Python.
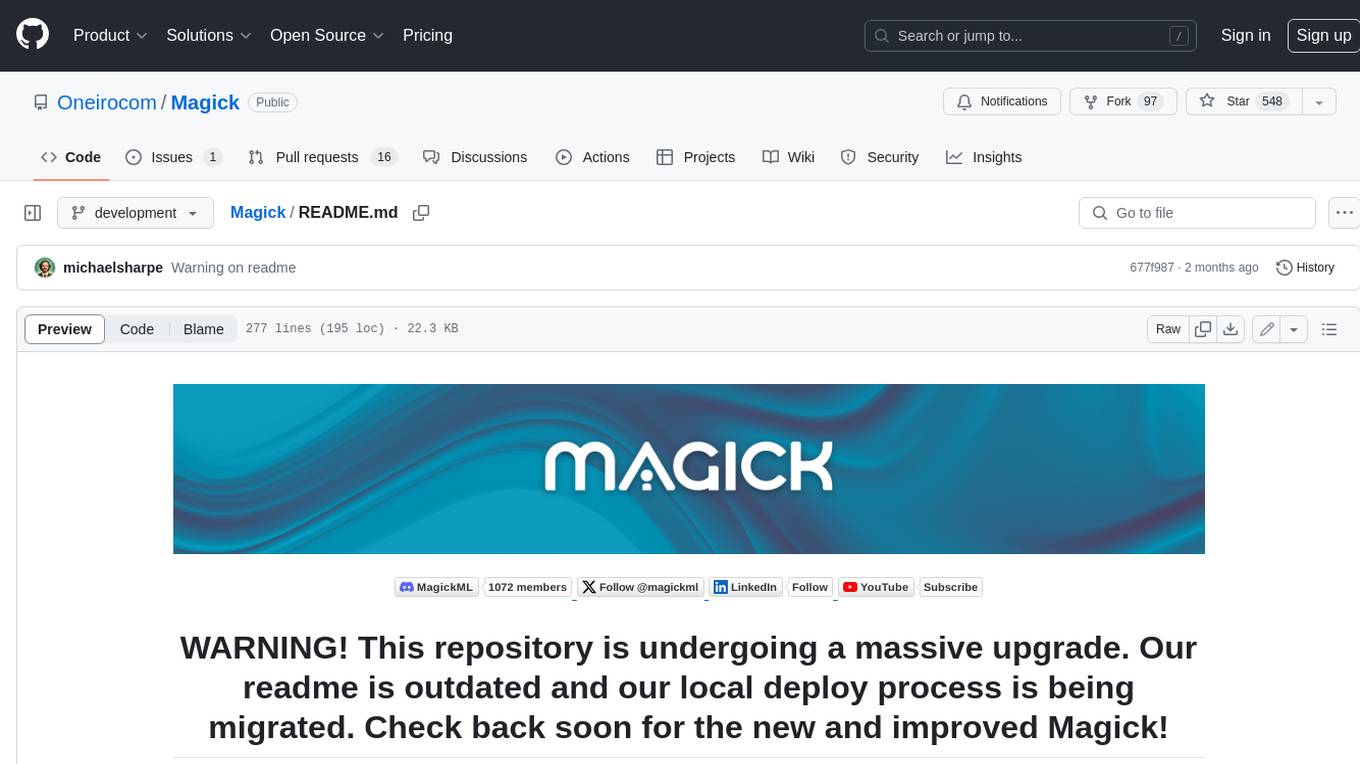
Magick
Magick is a groundbreaking visual AIDE (Artificial Intelligence Development Environment) for no-code data pipelines and multimodal agents. Magick can connect to other services and comes with nodes and templates well-suited for intelligent agents, chatbots, complex reasoning systems and realistic characters.