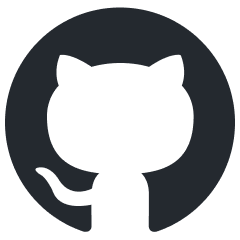
spiceai
A data query and AI-inference compute engine written in Rust using Apache Arrow and DataFusion for secure, fast, agentic AI applications.
Stars: 1970
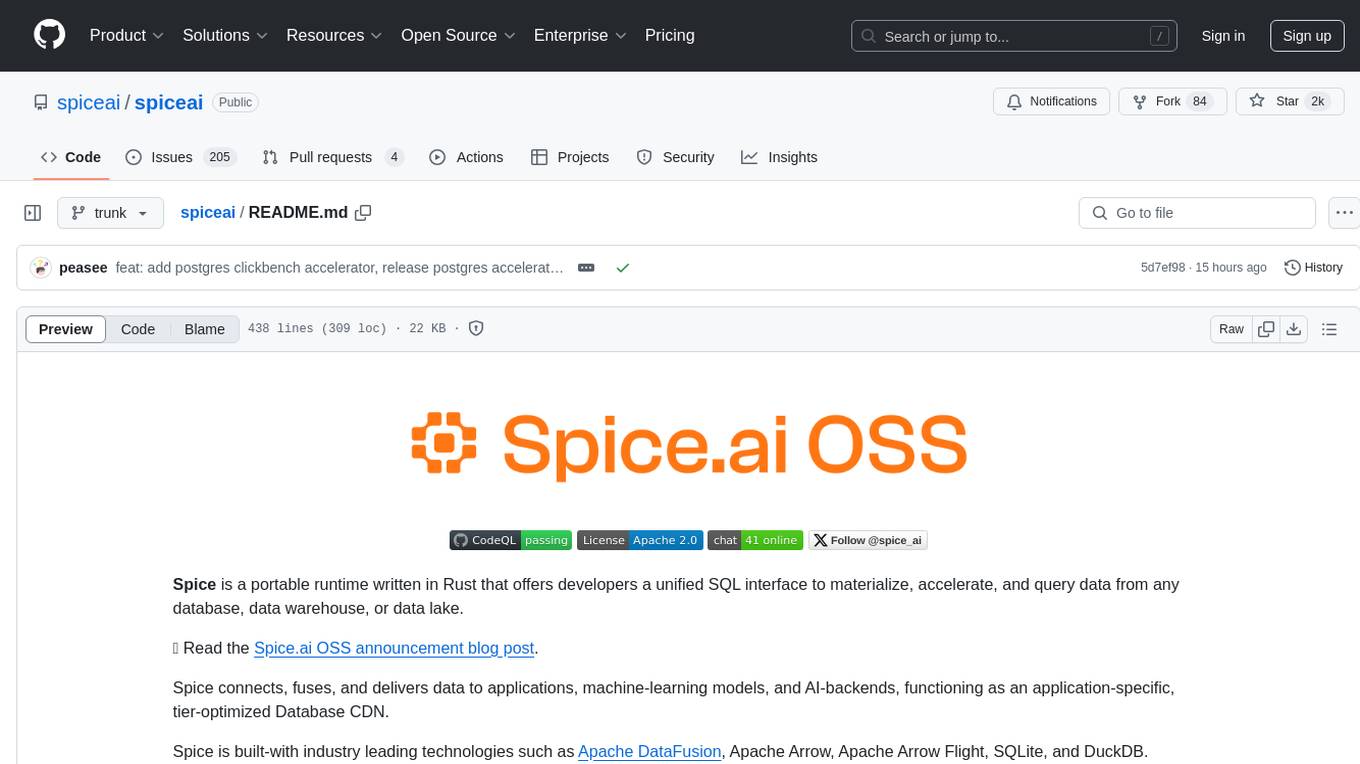
Spice is a portable runtime written in Rust that offers developers a unified SQL interface to materialize, accelerate, and query data from any database, data warehouse, or data lake. It connects, fuses, and delivers data to applications, machine-learning models, and AI-backends, functioning as an application-specific, tier-optimized Database CDN. Built with industry-leading technologies such as Apache DataFusion, Apache Arrow, Apache Arrow Flight, SQLite, and DuckDB. Spice makes it fast and easy to query data from one or more sources using SQL, co-locating a managed dataset with applications or machine learning models, and accelerating it with Arrow in-memory, SQLite/DuckDB, or attached PostgreSQL for fast, high-concurrency, low-latency queries.
README:
Spice is a portable runtime written in Rust that offers developers a unified SQL interface to materialize, accelerate, and query data from any database, data warehouse, or data lake.
π£ Read the Spice.ai OSS announcement blog post.
Spice connects, fuses, and delivers data to applications, machine-learning models, and AI-backends, functioning as an application-specific, tier-optimized Database CDN.
Spice is built-with industry leading technologies such as Apache DataFusion, Apache Arrow, Apache Arrow Flight, SQLite, and DuckDB.
π Read the MaterializedView interview on Spice.ai
π₯ Watch the CMU Databases Accelerating Data and AI with Spice.ai Open-Source
Spice makes it fast and easy to query data from one or more sources using SQL. You can co-locate a managed dataset with your application or machine learning model, and accelerate it with Arrow in-memory, SQLite/DuckDB, or with attached PostgreSQL for fast, high-concurrency, low-latency queries. Accelerated engines give you flexibility and control over query cost and performance.
-
Application-focused: Spice is designed to integrate at the application level; 1:1 or 1:N application to Spice mapping, whereas most other data systems are designed for multiple applications to share a single database or data warehouse. It's not uncommon to have many Spice instances, even down to one for each tenant or customer.
-
Dual-Engine Acceleration: Spice supports both OLAP (Arrow/DuckDB) and OLTP (SQLite/PostgreSQL) databases at the dataset level, unlike other systems that only support one type.
-
Separation of Materialization and Storage/Compute: Spice separates storage and compute, allowing you to keep data close to its source and bring a materialized working set next to your application, dashboard, or data/ML pipeline.
-
Edge to Cloud Native. Spice is designed to be deployed anywhere, from a standalone instance to a Kubernetes container sidecar, microservice, or cluster at the Edge/POP, On-Prem, or in public clouds. You can also chain Spice instances and deploy them across multiple infrastructure tiers.
Spice | Trino/Presto | Dremio | Clickhouse | |
---|---|---|---|---|
Primary Use-Case | Data & AI Applications | Big Data Analytics | Interactive Analytics | Real-Time Analytics |
Typical Deployment | Colocated with application | Cloud Cluster | Cloud Cluster | On-Prem/Cloud Cluster |
Application-to-Data System | One-to-One/Many | Many-to-One | Many-to-One | Many-to-One |
Query Federation | Native with query push-down | Supported with push-down | Supported with limited push-down | Limited |
Materialization | Arrow/SQLite/DuckDB/PostgreSQL | Intermediate Storage | Reflections (Iceberg) | Views & MergeTree |
Query Result Caching | Supported | Supported | Supported | Supported |
Typical Configuration | Single-Binary/Sidecar/Microservice | Coodinator+Executor w/ Zookeeper | Coodinator+Executor w/ Zookeeper | Clickhouse Keeper+Nodes |
1. Faster applications and frontends. Accelerate and co-locate datasets with applications and frontends, to serve more concurrent queries and users with faster page loads and data updates. Try the CQRS sample app
2. Faster dashboards, analytics, and BI. Faster, more responsive dashboards without massive compute costs. Watch the Apache Superset demo
3. Faster data pipelines, machine learning training and inferencing. Co-locate datasets in pipelines where the data is needed to minimize data-movement and improve query performance.
4. Easily query many data sources. Federated SQL query across databases, data warehouses, and data lakes using Data Connectors.
-
Is Spice a cache? No, however you can think of Spice data materialization like an active cache or data prefetcher. A cache would fetch data on a cache-miss while Spice prefetches and materializes filtered data on an interval or as new data becomes available. In addition to materialization Spice supports results caching.
-
Is Spice a CDN for databases? Yes, you can think of Spice like a CDN for different data sources. Using CDN concepts, Spice enables you to ship (load) a working set of your database (or data lake, or data warehouse) where it's most frequently accessed, like from a data application or for AI-inference.
-
Where is the AI? Spice provides a unified API for both data and AI/ML with a high-performance bus between the two. However, because the first step in AI-readiness is data-readiness, the Getting Started content is focused on data. Spice has endpoints and APIs for model deployment and inference including LLMs, accelerated embeddings, and an AI-gateway for providers like OpenAI and Anthropic. Read more about the vision to enable development of intelligent AI-driven applications.
https://github.com/spiceai/spiceai/assets/80174/7735ee94-3f4a-4983-a98e-fe766e79e03a
Currently supported data connectors for upstream datasets. More coming soon.
Name | Description | Status | Protocol/Format |
---|---|---|---|
duckdb |
DuckDB | Release Candidate | |
github |
GitHub | Release Candidate | |
graphql |
GraphQL | Release Candidate | JSON |
mysql |
MySQL | Release Candidate | |
postgres |
PostgreSQL | Release Candidate | |
s3 |
S3 | Release Candidate | Parquet, CSV |
databricks (mode: delta_lake) |
Databricks | Release Candidate | S3/Delta Lake |
file |
File | Release Candidate | Parquet, CSV |
databricks (mode: spark_connect) |
Databricks | Beta | Spark Connect |
delta_lake |
Delta Lake | Beta | Delta Lake |
flightsql |
FlightSQL | Beta | Arrow Flight SQL |
mssql |
Microsoft SQL Server | Beta | Tabular Data Stream (TDS) |
odbc |
ODBC | Beta | ODBC |
spiceai |
Spice.ai | Beta | Arrow Flight |
abfs |
Azure BlobFS | Alpha | Parquet, CSV |
clickhouse |
Clickhouse | Alpha | |
debezium |
Debezium CDC | Alpha | Kafka + JSON |
dremio |
Dremio | Alpha | Arrow Flight |
ftp , sftp
|
FTP/SFTP | Alpha | Parquet, CSV |
http , https
|
HTTP(s) | Alpha | Parquet, CSV |
iceberg |
Apache Iceberg | Alpha | Parquet |
localpod |
Local dataset replication | Alpha | |
sharepoint |
Microsoft SharePoint | Alpha | Unstructured UTF-8 documents |
snowflake |
Snowflake | Alpha | Arrow |
spark |
Spark | Alpha | Spark Connect |
Currently supported data stores for local materialization/acceleration. More coming soon.
Name | Description | Status | Engine Modes |
---|---|---|---|
arrow |
In-Memory Arrow Records | Release Candidate | memory |
duckdb |
Embedded DuckDB | Release Candidate |
memory , file
|
postgres |
Attached PostgreSQL | Release Candidate | |
sqlite |
Embedded SQLite | Release Candidate |
memory , file
|
https://github.com/spiceai/spiceai/assets/88671039/85cf9a69-46e7-412e-8b68-22617dcbd4e0
Step 1. Install the Spice CLI:
On macOS, Linux, and WSL:
curl https://install.spiceai.org | /bin/bash
Or using brew
:
brew install spiceai/spiceai/spice
On Windows:
curl -L "https://install.spiceai.org/Install.ps1" -o Install.ps1 && PowerShell -ExecutionPolicy Bypass -File ./Install.ps1
Step 2. Initialize a new Spice app with the spice init
command:
spice init spice_qs
A spicepod.yaml
file is created in the spice_qs
directory. Change to that directory:
cd spice_qs
Step 3. Start the Spice runtime:
spice run
Example output will be shown as follows:
Spice.ai runtime starting...
2024-08-05T13:02:40.247484Z INFO runtime::flight: Spice Runtime Flight listening on 127.0.0.1:50051
2024-08-05T13:02:40.247490Z INFO runtime::metrics_server: Spice Runtime Metrics listening on 127.0.0.1:9090
2024-08-05T13:02:40.247949Z INFO runtime: Initialized results cache; max size: 128.00 MiB, item ttl: 1s
2024-08-05T13:02:40.248611Z INFO runtime::http: Spice Runtime HTTP listening on 127.0.0.1:8090
2024-08-05T13:02:40.252356Z INFO runtime::opentelemetry: Spice Runtime OpenTelemetry listening on 127.0.0.1:50052
The runtime is now started and ready for queries.
Step 4. In a new terminal window, add the spiceai/quickstart
Spicepod. A Spicepod is a package of configuration defining datasets and ML models.
spice add spiceai/quickstart
The spicepod.yaml
file will be updated with the spiceai/quickstart
dependency.
version: v1beta1
kind: Spicepod
name: spice_qs
dependencies:
- spiceai/quickstart
The spiceai/quickstart
Spicepod will add a taxi_trips
data table to the runtime which is now available to query by SQL.
2024-08-05T13:04:56.742779Z INFO runtime: Dataset taxi_trips registered (s3://spiceai-demo-datasets/taxi_trips/2024/), acceleration (arrow, 10s refresh), results cache enabled.
2024-08-05T13:04:56.744062Z INFO runtime::accelerated_table::refresh_task: Loading data for dataset taxi_trips
2024-08-05T13:05:03.556169Z INFO runtime::accelerated_table::refresh_task: Loaded 2,964,624 rows (421.71 MiB) for dataset taxi_trips in 6s 812ms.
Step 5. Start the Spice SQL REPL:
spice sql
The SQL REPL inferface will be shown:
Welcome to the Spice.ai SQL REPL! Type 'help' for help.
show tables; -- list available tables
sql>
Enter show tables;
to display the available tables for query:
sql> show tables;
+---------------+--------------+---------------+------------+
| table_catalog | table_schema | table_name | table_type |
+---------------+--------------+---------------+------------+
| spice | public | taxi_trips | BASE TABLE |
| spice | runtime | query_history | BASE TABLE |
| spice | runtime | metrics | BASE TABLE |
+---------------+--------------+---------------+------------+
Time: 0.022671708 seconds. 3 rows.
Enter a query to display the longest taxi trips:
SELECT trip_distance, total_amount FROM taxi_trips ORDER BY trip_distance DESC LIMIT 10;
Output:
+---------------+--------------+
| trip_distance | total_amount |
+---------------+--------------+
| 312722.3 | 22.15 |
| 97793.92 | 36.31 |
| 82015.45 | 21.56 |
| 72975.97 | 20.04 |
| 71752.26 | 49.57 |
| 59282.45 | 33.52 |
| 59076.43 | 23.17 |
| 58298.51 | 18.63 |
| 51619.36 | 24.2 |
| 44018.64 | 52.43 |
+---------------+--------------+
Time: 0.045150667 seconds. 10 rows.
Using the Docker image locally:
docker pull spiceai/spiceai
In a Dockerfile:
from spiceai/spiceai:latest
Using Helm:
helm repo add spiceai https://helm.spiceai.org
helm install spiceai spiceai/spiceai
The Spice.ai Cookbook is a collection of recipes and examples for using Spice. Find it at https://github.com/spiceai/cookbook.
You can use any number of predefined datasets available from the Spice.ai Cloud Platform in the Spice runtime.
A list of publicly available datasets from the Spice.ai Cloud Platform can be found on Spicerack: https://spicerack.org/.
In order to access public datasets from Spice.ai, you will first need to create an account with Spice.ai by selecting the free tier membership.
Navigate to spice.ai and create a new account by clicking on Try for Free.
After creating an account, you will need to create an app in order to create to an API key.
You will now be able to access datasets from Spice.ai. For this demonstration, we will be using the taxi_trips
dataset from the https://spice.ai/spiceai/quickstart Spice.ai app.
Step 1. Initialize a new project.
# Initialize a new Spice app
spice init spice_app
# Change to app directory
cd spice_app
Step 2. Log in and authenticate from the command line using the spice login
command. A pop up browser window will prompt you to authenticate:
spice login
Step 3. Start the runtime:
# Start the runtime
spice run
Step 4. Configure the dataset:
In a new terminal window, configure a new dataset using the spice dataset configure
command:
spice dataset configure
Enter a dataset name that will be used to reference the dataset in queries. This name does not need to match the name in the dataset source.
dataset name: (spice_app) taxi_trips
Enter the description of the dataset:
description: Taxi trips dataset
Enter the location of the dataset:
from: spice.ai/spiceai/quickstart/datasets/taxi_trips
Select y
when prompted whether to accelerate the data:
Locally accelerate (y/n)? y
You should see the following output from your runtime terminal:
2024-12-16T05:12:45.803694Z INFO runtime::init::dataset: Dataset taxi_trips registered (spice.ai/spiceai/quickstart/datasets/taxi_trips), acceleration (arrow, 10s refresh), results cache enabled.
2024-12-16T05:12:45.805494Z INFO runtime::accelerated_table::refresh_task: Loading data for dataset taxi_trips
2024-12-16T05:13:24.218345Z INFO runtime::accelerated_table::refresh_task: Loaded 2,964,624 rows (8.41 GiB) for dataset taxi_trips in 38s 412ms.
Step 5. In a new terminal window, use the Spice SQL REPL to query the dataset
spice sql
SELECT tpep_pickup_datetime, passenger_count, trip_distance from taxi_trips LIMIT 10;
The output displays the results of the query along with the query execution time:
+----------------------+-----------------+---------------+
| tpep_pickup_datetime | passenger_count | trip_distance |
+----------------------+-----------------+---------------+
| 2024-01-11T12:55:12 | 1 | 0.0 |
| 2024-01-11T12:55:12 | 1 | 0.0 |
| 2024-01-11T12:04:56 | 1 | 0.63 |
| 2024-01-11T12:18:31 | 1 | 1.38 |
| 2024-01-11T12:39:26 | 1 | 1.01 |
| 2024-01-11T12:18:58 | 1 | 5.13 |
| 2024-01-11T12:43:13 | 1 | 2.9 |
| 2024-01-11T12:05:41 | 1 | 1.36 |
| 2024-01-11T12:20:41 | 1 | 1.11 |
| 2024-01-11T12:37:25 | 1 | 2.04 |
+----------------------+-----------------+---------------+
Time: 0.00538925 seconds. 10 rows.
You can experiment with the time it takes to generate queries when using non-accelerated datasets. You can change the acceleration setting from true
to false
in the datasets.yaml file.
Comprehensive documentation is available at docs.spiceai.org.
Spice.ai is designed to be extensible with extension points documented at EXTENSIBILITY.md. Build custom Data Connectors, Data Accelerators, Catalog Connectors, Secret Stores, Models, or Embeddings.
π See the Roadmap to v1.0-stable for upcoming features.
We greatly appreciate and value your support! You can help Spice in a number of ways:
- Build an app with Spice.ai and send us feedback and suggestions at [email protected] or on Discord, X, or LinkedIn.
- File an issue if you see something not quite working correctly.
- Join our team (Weβre hiring!)
- Contribute code or documentation to the project (see CONTRIBUTING.md).
- Follow our blog at blog.spiceai.org
βοΈ star this repo! Thank you for your support! π
For Tasks:
Click tags to check more tools for each tasksFor Jobs:
Alternative AI tools for spiceai
Similar Open Source Tools
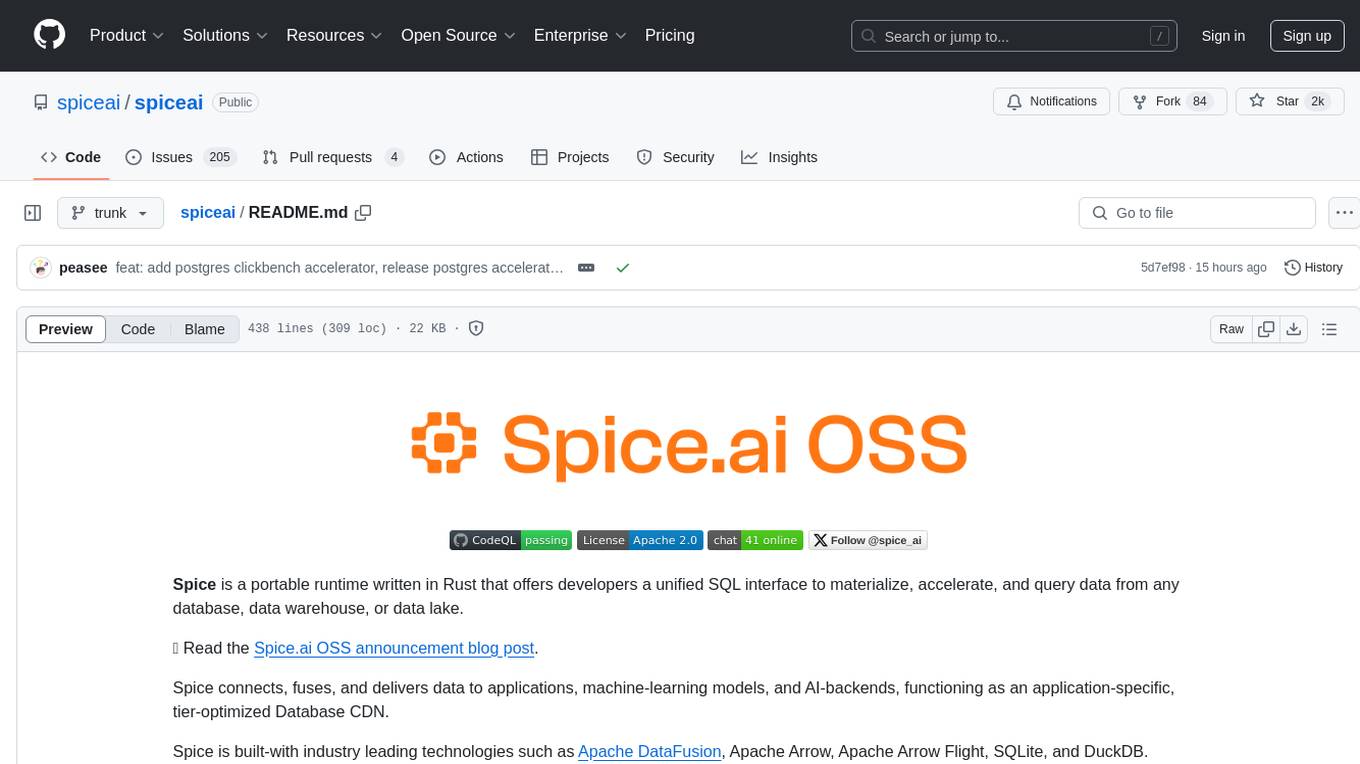
spiceai
Spice is a portable runtime written in Rust that offers developers a unified SQL interface to materialize, accelerate, and query data from any database, data warehouse, or data lake. It connects, fuses, and delivers data to applications, machine-learning models, and AI-backends, functioning as an application-specific, tier-optimized Database CDN. Built with industry-leading technologies such as Apache DataFusion, Apache Arrow, Apache Arrow Flight, SQLite, and DuckDB. Spice makes it fast and easy to query data from one or more sources using SQL, co-locating a managed dataset with applications or machine learning models, and accelerating it with Arrow in-memory, SQLite/DuckDB, or attached PostgreSQL for fast, high-concurrency, low-latency queries.
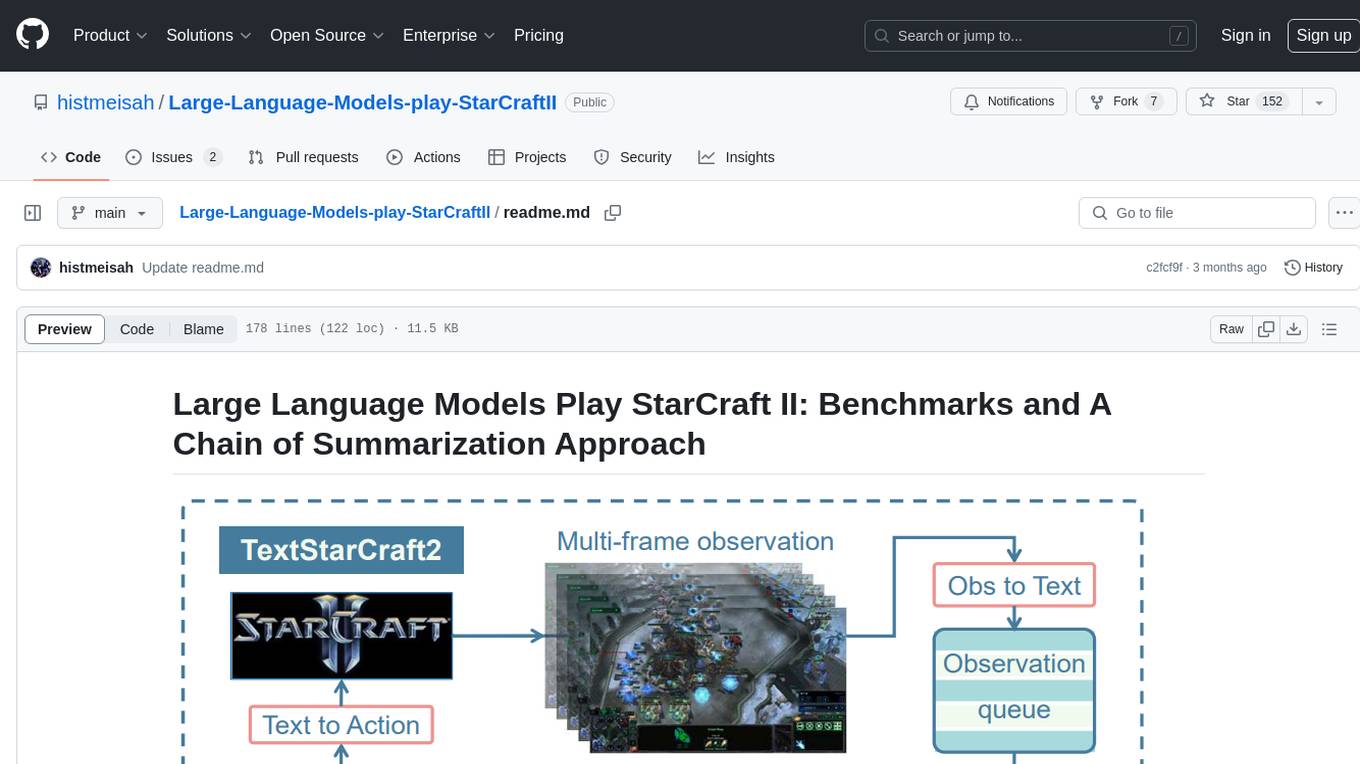
Large-Language-Models-play-StarCraftII
Large Language Models Play StarCraft II is a project that explores the capabilities of large language models (LLMs) in playing the game StarCraft II. The project introduces TextStarCraft II, a textual environment for the game, and a Chain of Summarization method for analyzing game information and making strategic decisions. Through experiments, the project demonstrates that LLM agents can defeat the built-in AI at a challenging difficulty level. The project provides benchmarks and a summarization approach to enhance strategic planning and interpretability in StarCraft II gameplay.
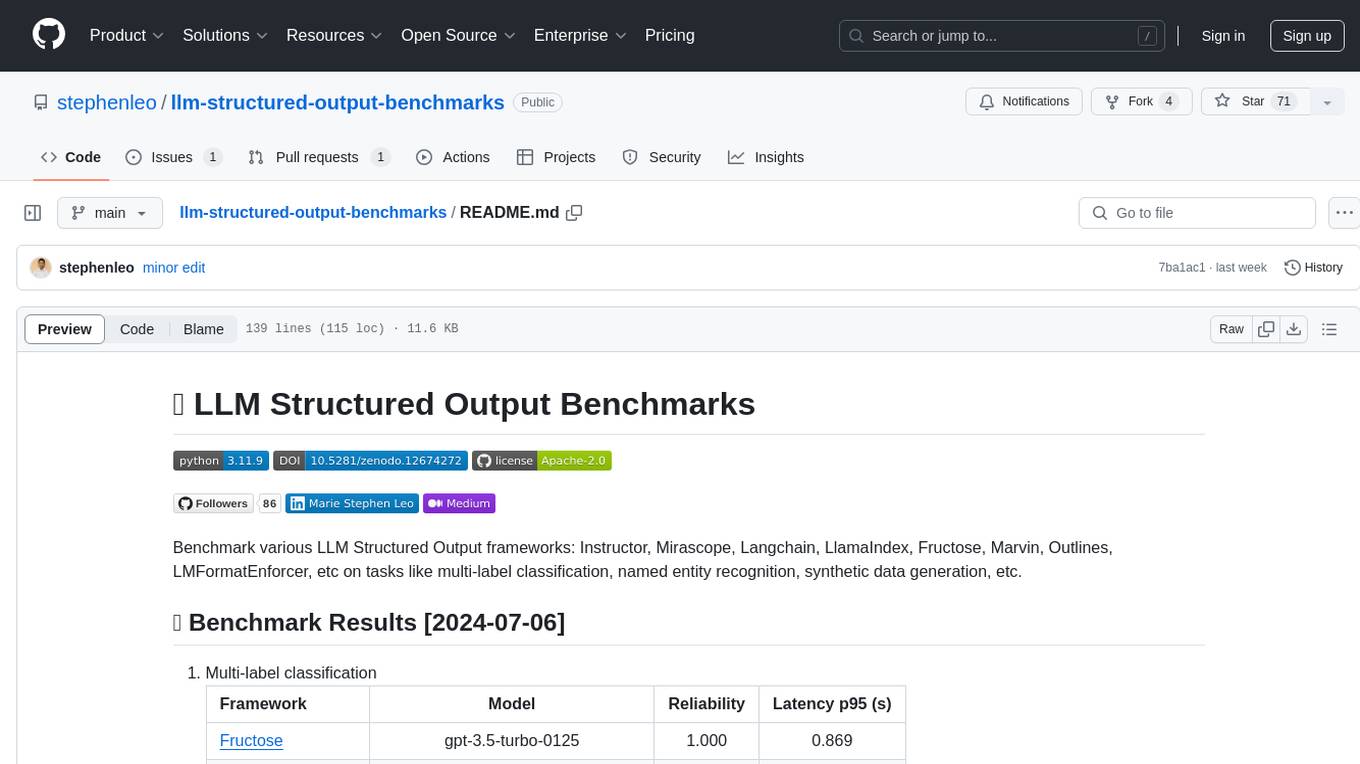
llm-structured-output-benchmarks
Benchmark various LLM Structured Output frameworks like Instructor, Mirascope, Langchain, LlamaIndex, Fructose, Marvin, Outlines, LMFormatEnforcer, etc on tasks like multi-label classification, named entity recognition, synthetic data generation. The tool provides benchmark results, methodology, instructions to run the benchmark, add new data, and add a new framework. It also includes a roadmap for framework-related tasks, contribution guidelines, citation information, and feedback request.
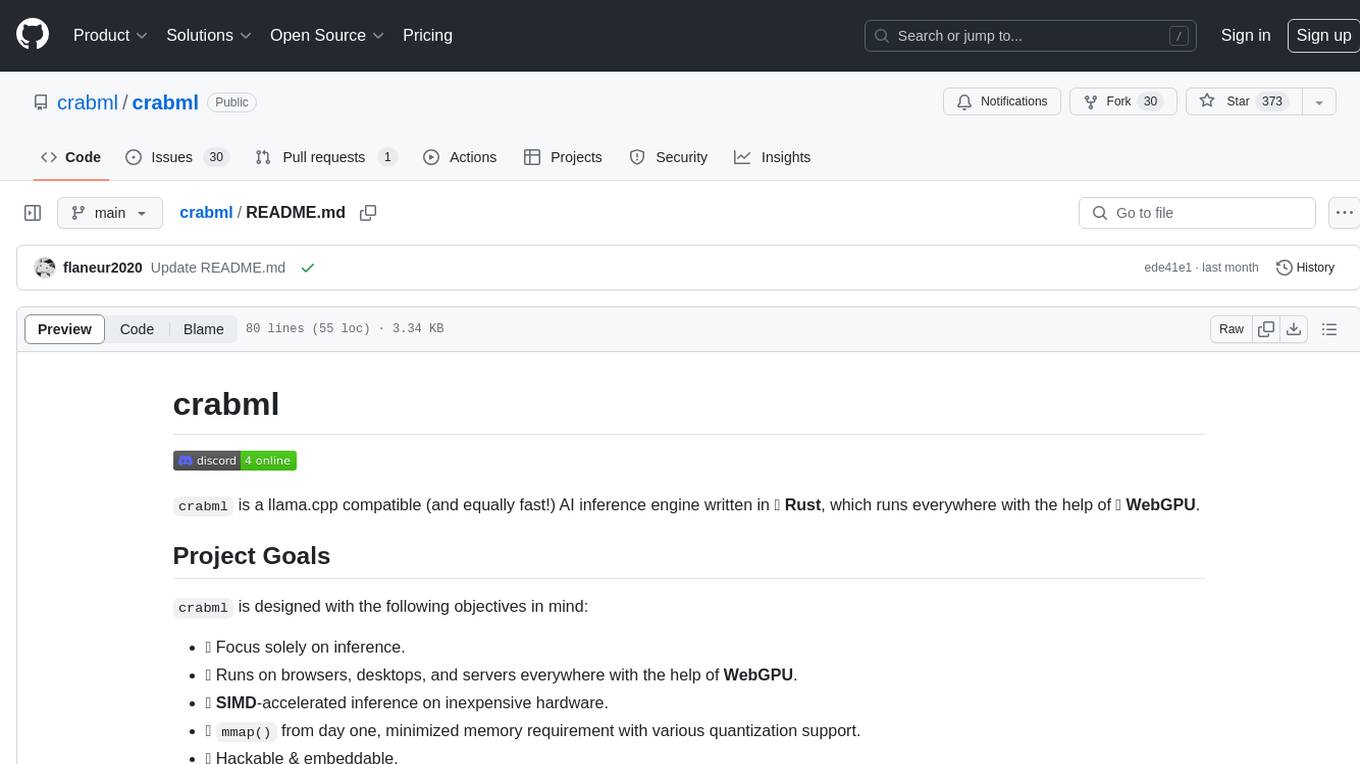
crabml
Crabml is a llama.cpp compatible AI inference engine written in Rust, designed for efficient inference on various platforms with WebGPU support. It focuses on running inference tasks with SIMD acceleration and minimal memory requirements, supporting multiple models and quantization methods. The project is hackable, embeddable, and aims to provide high-performance AI inference capabilities.
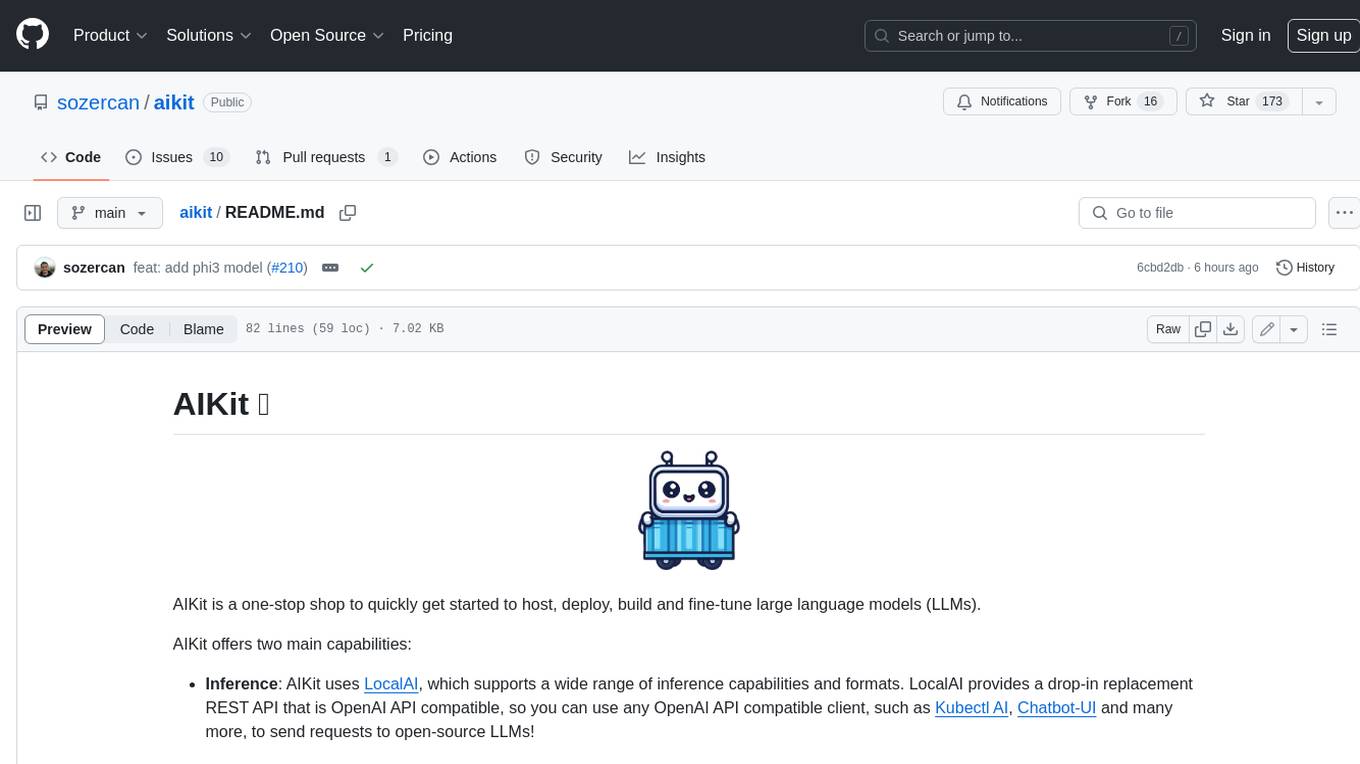
aikit
AIKit is a one-stop shop to quickly get started to host, deploy, build and fine-tune large language models (LLMs). AIKit offers two main capabilities: Inference: AIKit uses LocalAI, which supports a wide range of inference capabilities and formats. LocalAI provides a drop-in replacement REST API that is OpenAI API compatible, so you can use any OpenAI API compatible client, such as Kubectl AI, Chatbot-UI and many more, to send requests to open-source LLMs! Fine Tuning: AIKit offers an extensible fine tuning interface. It supports Unsloth for fast, memory efficient, and easy fine-tuning experience.
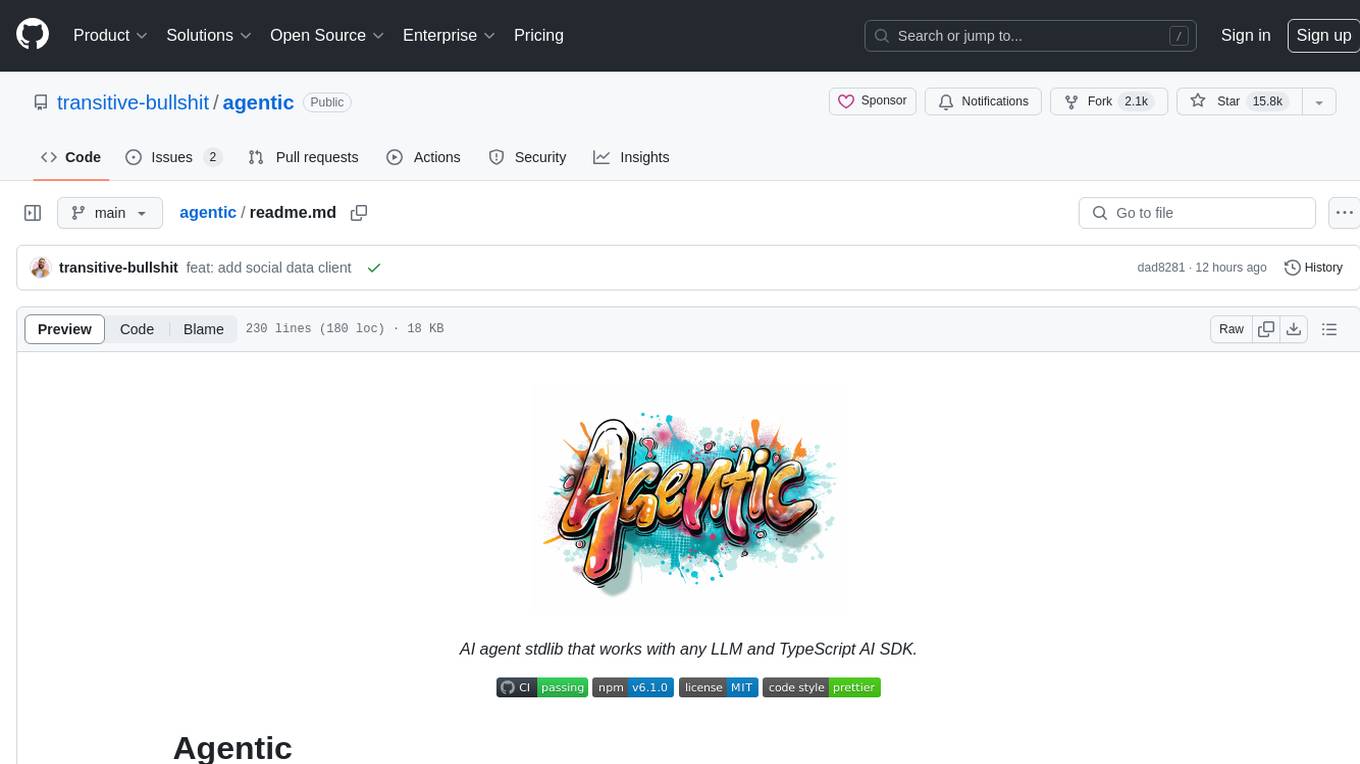
agentic
Agentic is a standard AI functions/tools library optimized for TypeScript and LLM-based apps, compatible with major AI SDKs. It offers a set of thoroughly tested AI functions that can be used with favorite AI SDKs without writing glue code. The library includes various clients for services like Bing web search, calculator, Clearbit data resolution, Dexa podcast questions, and more. It also provides compound tools like SearchAndCrawl and supports multiple AI SDKs such as OpenAI, Vercel AI SDK, LangChain, LlamaIndex, Firebase Genkit, and Dexa Dexter. The goal is to create minimal clients with strongly-typed TypeScript DX, composable AIFunctions via AIFunctionSet, and compatibility with major TS AI SDKs.
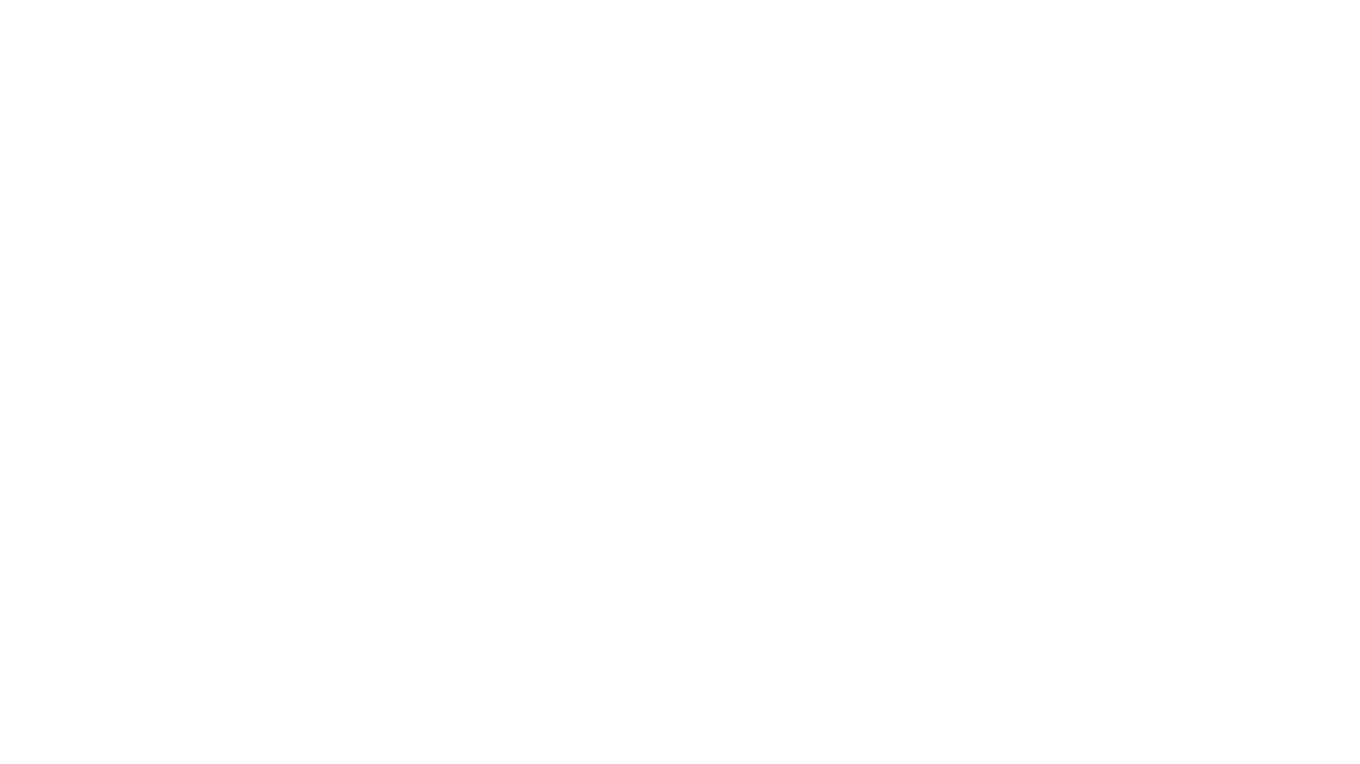
graphrag-visualizer
GraphRAG Visualizer is an application designed to visualize Microsoft GraphRAG artifacts by uploading parquet files generated from the GraphRAG indexing pipeline. Users can view and analyze data in 2D or 3D graphs, display data tables, search for specific nodes or relationships, and process artifacts locally for data security and privacy.
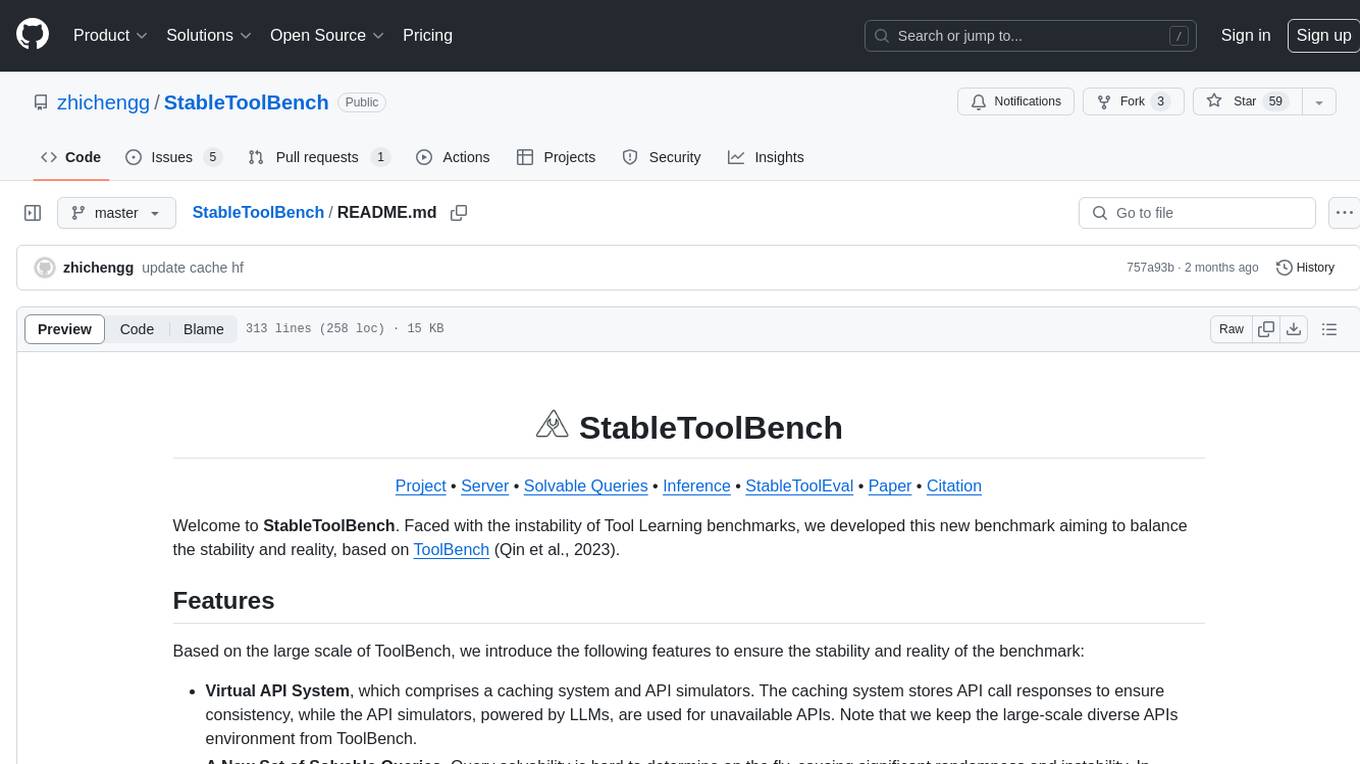
StableToolBench
StableToolBench is a new benchmark developed to address the instability of Tool Learning benchmarks. It aims to balance stability and reality by introducing features such as a Virtual API System with caching and API simulators, a new set of solvable queries determined by LLMs, and a Stable Evaluation System using GPT-4. The Virtual API Server can be set up either by building from source or using a prebuilt Docker image. Users can test the server using provided scripts and evaluate models with Solvable Pass Rate and Solvable Win Rate metrics. The tool also includes model experiments results comparing different models' performance.
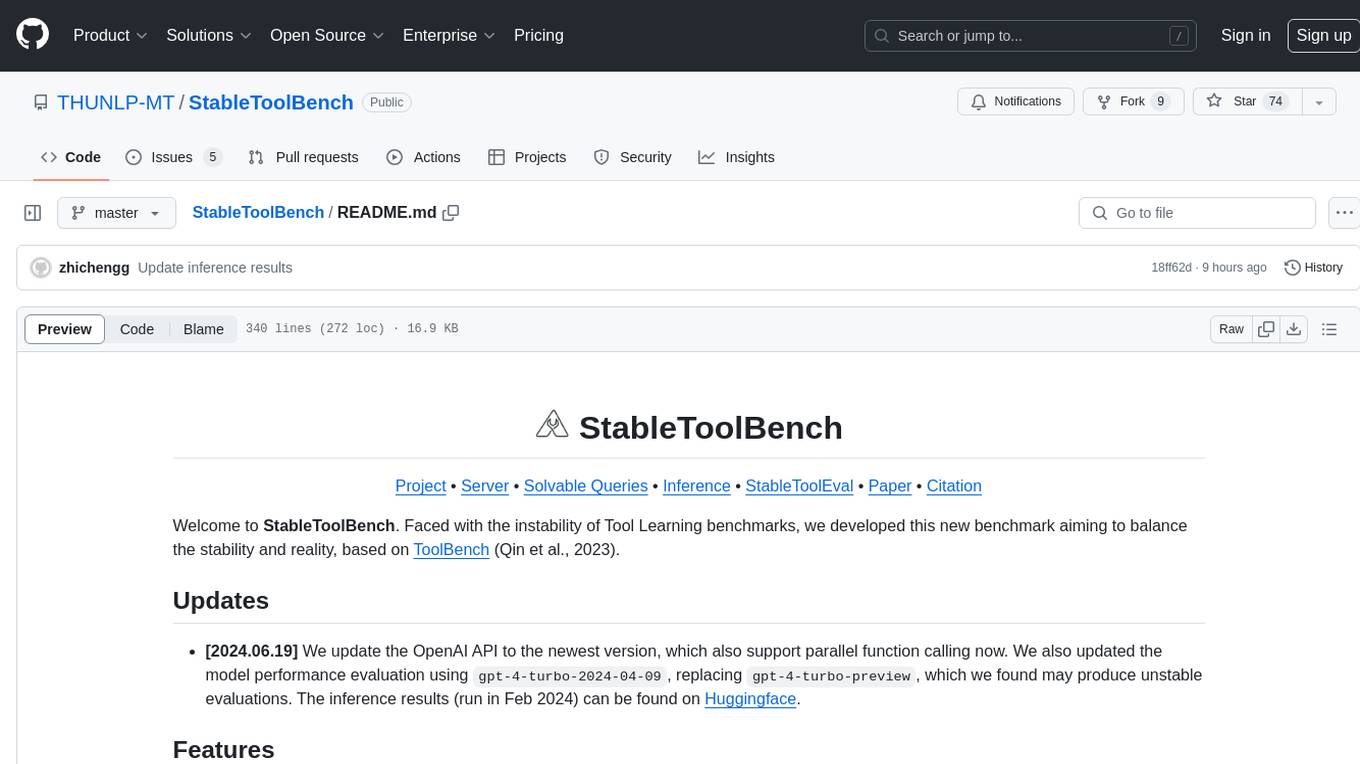
StableToolBench
StableToolBench is a new benchmark developed to address the instability of Tool Learning benchmarks. It aims to balance stability and reality by introducing features like Virtual API System, Solvable Queries, and Stable Evaluation System. The benchmark ensures consistency through a caching system and API simulators, filters queries based on solvability using LLMs, and evaluates model performance using GPT-4 with metrics like Solvable Pass Rate and Solvable Win Rate.
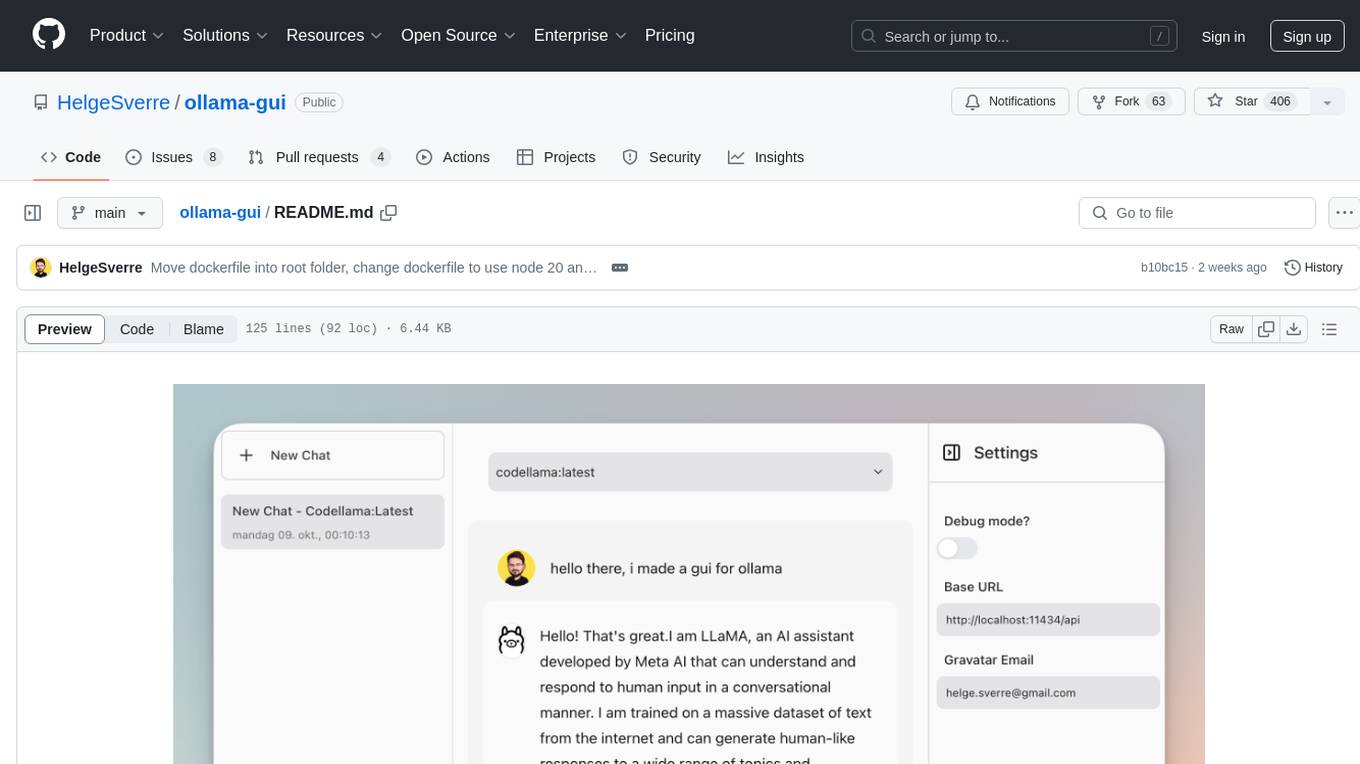
ollama-gui
Ollama GUI is a web interface for ollama.ai, a tool that enables running Large Language Models (LLMs) on your local machine. It provides a user-friendly platform for chatting with LLMs and accessing various models for text generation. Users can easily interact with different models, manage chat history, and explore available models through the web interface. The tool is built with Vue.js, Vite, and Tailwind CSS, offering a modern and responsive design for seamless user experience.
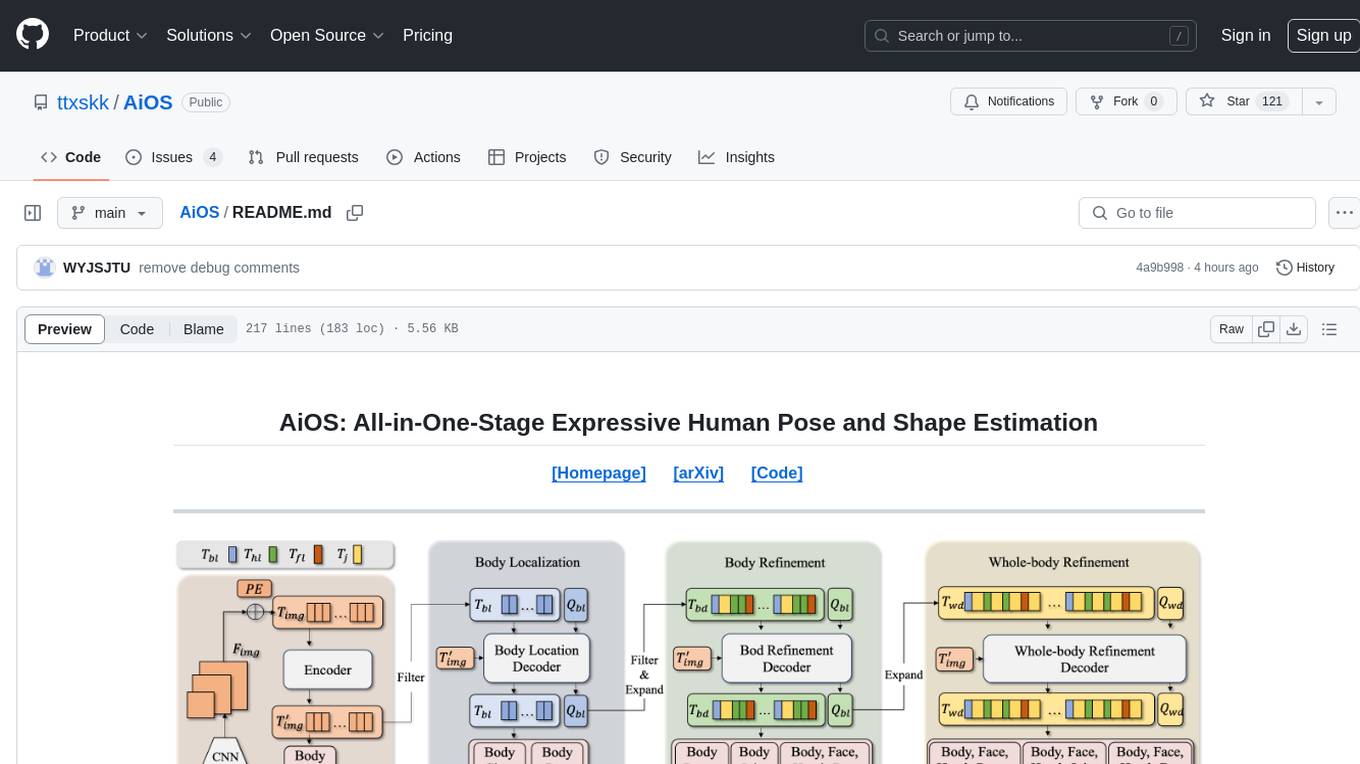
AiOS
AiOS is a tool for human pose and shape estimation, performing human localization and SMPL-X estimation in a progressive manner. It consists of body localization, body refinement, and whole-body refinement stages. Users can download datasets for evaluation, SMPL-X body models, and AiOS checkpoint. Installation involves creating a conda virtual environment, installing PyTorch, torchvision, Pytorch3D, MMCV, and other dependencies. Inference requires placing the video for inference and pretrained models in specific directories. Test results are provided for NMVE, NMJE, MVE, and MPJPE on datasets like BEDLAM and AGORA. Users can run scripts for AGORA validation, AGORA test leaderboard, and BEDLAM leaderboard. The tool acknowledges codes from MMHuman3D, ED-Pose, and SMPLer-X.
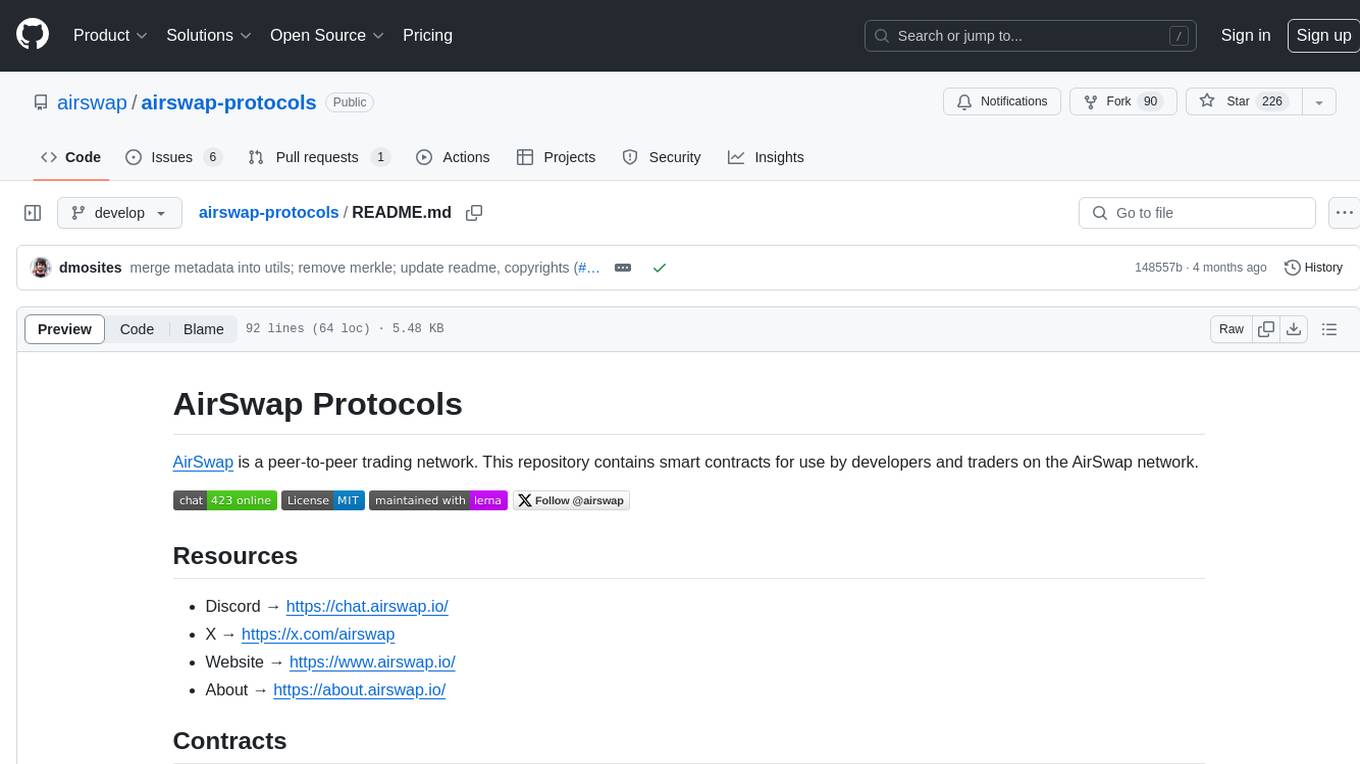
airswap-protocols
AirSwap Protocols is a repository containing smart contracts for developers and traders on the AirSwap peer-to-peer trading network. It includes various packages for functionalities like server registry, atomic token swap, staking, rewards pool, batch token and order calls, libraries, and utils. The repository follows a branching and release process for contracts and tools, with steps for regular development process and individual package features or patches. Users can deploy and verify contracts using specific commands with network flags.
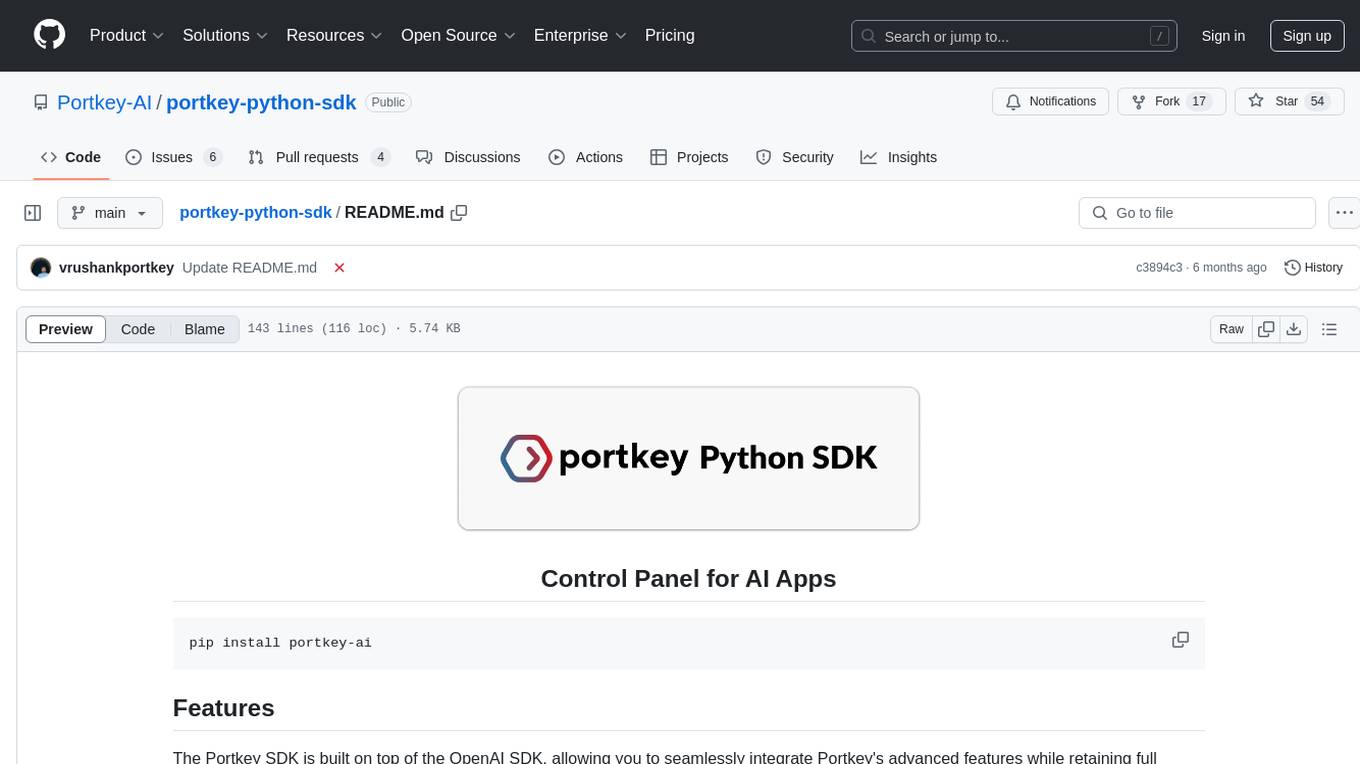
portkey-python-sdk
The Portkey Python SDK is a control panel for AI apps that allows seamless integration of Portkey's advanced features with OpenAI methods. It provides features such as AI gateway for unified API signature, interoperability, automated fallbacks & retries, load balancing, semantic caching, virtual keys, request timeouts, observability with logging, requests tracing, custom metadata, feedback collection, and analytics. Users can make requests to OpenAI using Portkey SDK and also use async functionality. The SDK is compatible with OpenAI SDK methods and offers Portkey-specific methods like feedback and prompts. It supports various providers and encourages contributions through Github issues or direct contact via email or Discord.
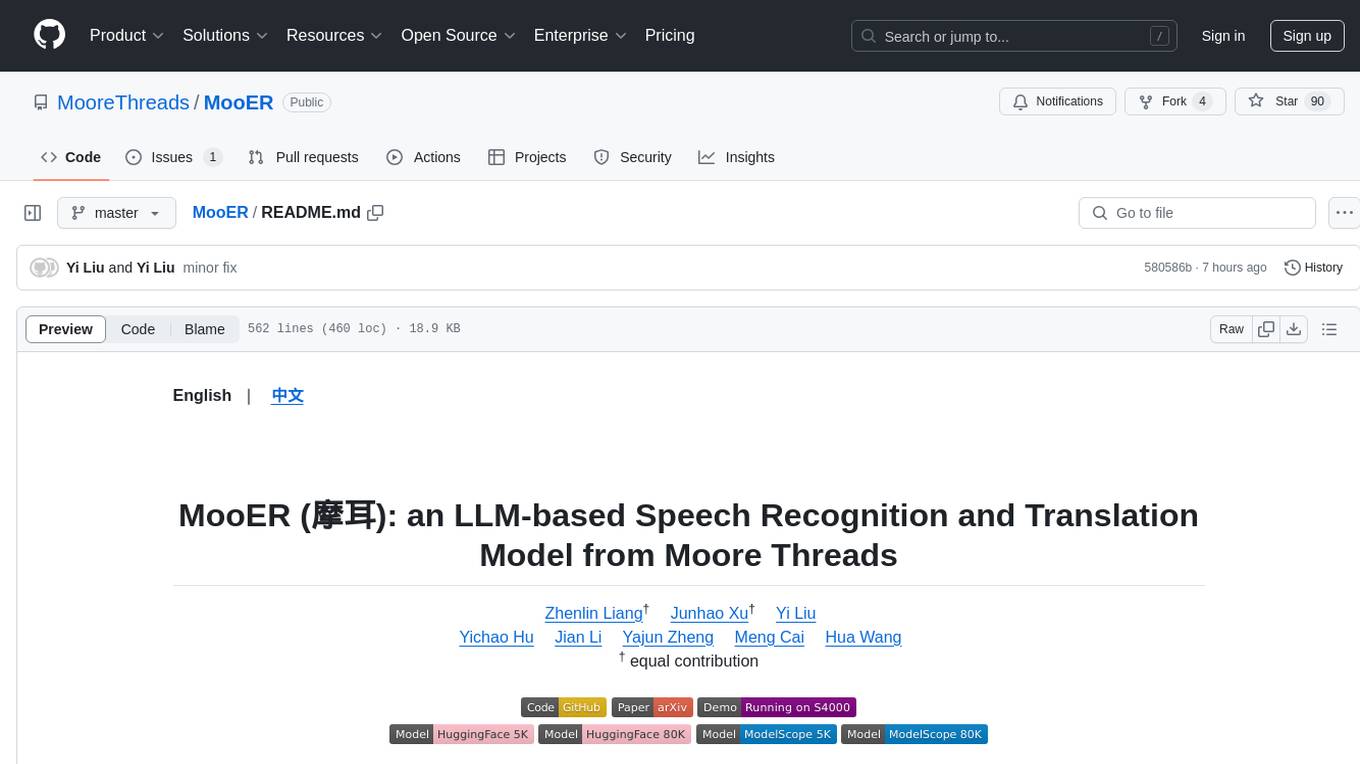
MooER
MooER (ζ©θ³) is an LLM-based speech recognition and translation model developed by Moore Threads. It allows users to transcribe speech into text (ASR) and translate speech into other languages (AST) in an end-to-end manner. The model was trained using 5K hours of data and is now also available with an 80K hours version. MooER is the first LLM-based speech model trained and inferred using domestic GPUs. The repository includes pretrained models, inference code, and a Gradio demo for a better user experience.
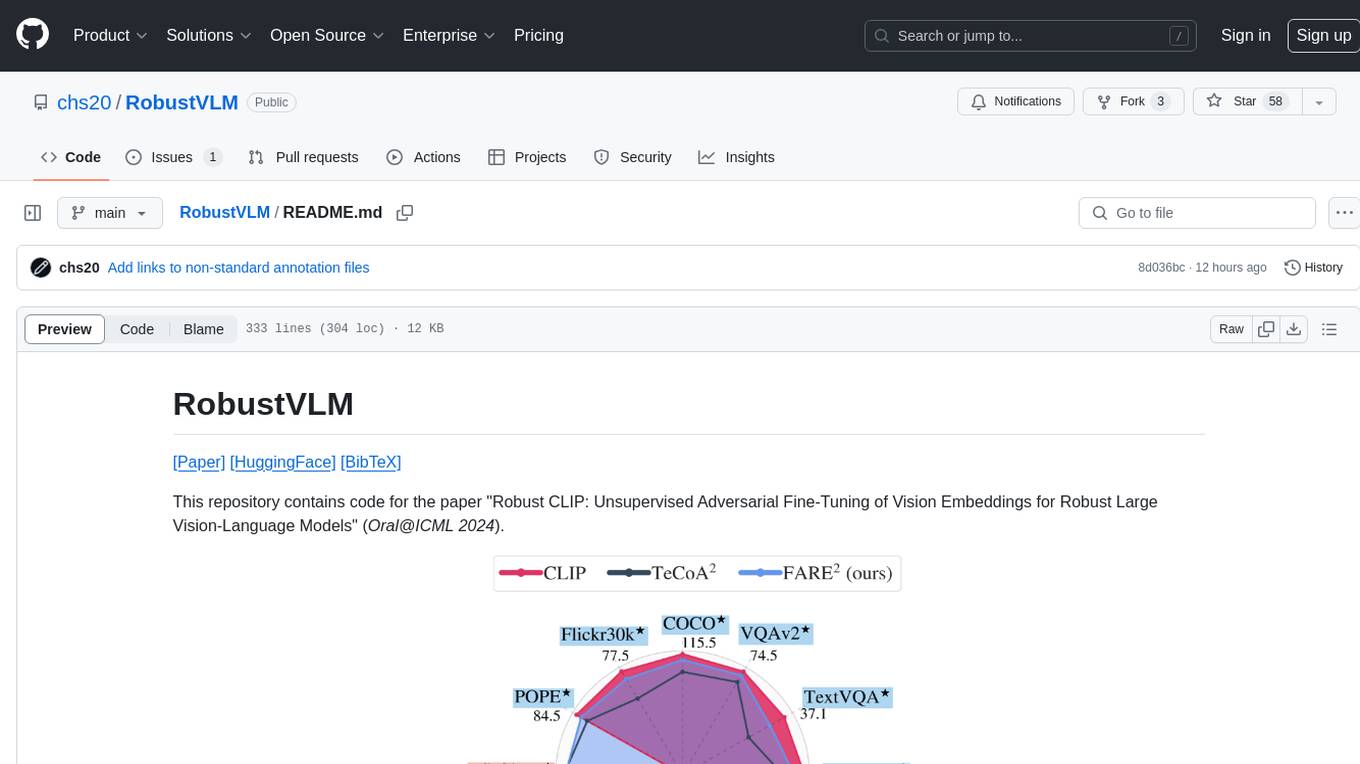
RobustVLM
This repository contains code for the paper 'Robust CLIP: Unsupervised Adversarial Fine-Tuning of Vision Embeddings for Robust Large Vision-Language Models'. It focuses on fine-tuning CLIP in an unsupervised manner to enhance its robustness against visual adversarial attacks. By replacing the vision encoder of large vision-language models with the fine-tuned CLIP models, it achieves state-of-the-art adversarial robustness on various vision-language tasks. The repository provides adversarially fine-tuned ViT-L/14 CLIP models and offers insights into zero-shot classification settings and clean accuracy improvements.
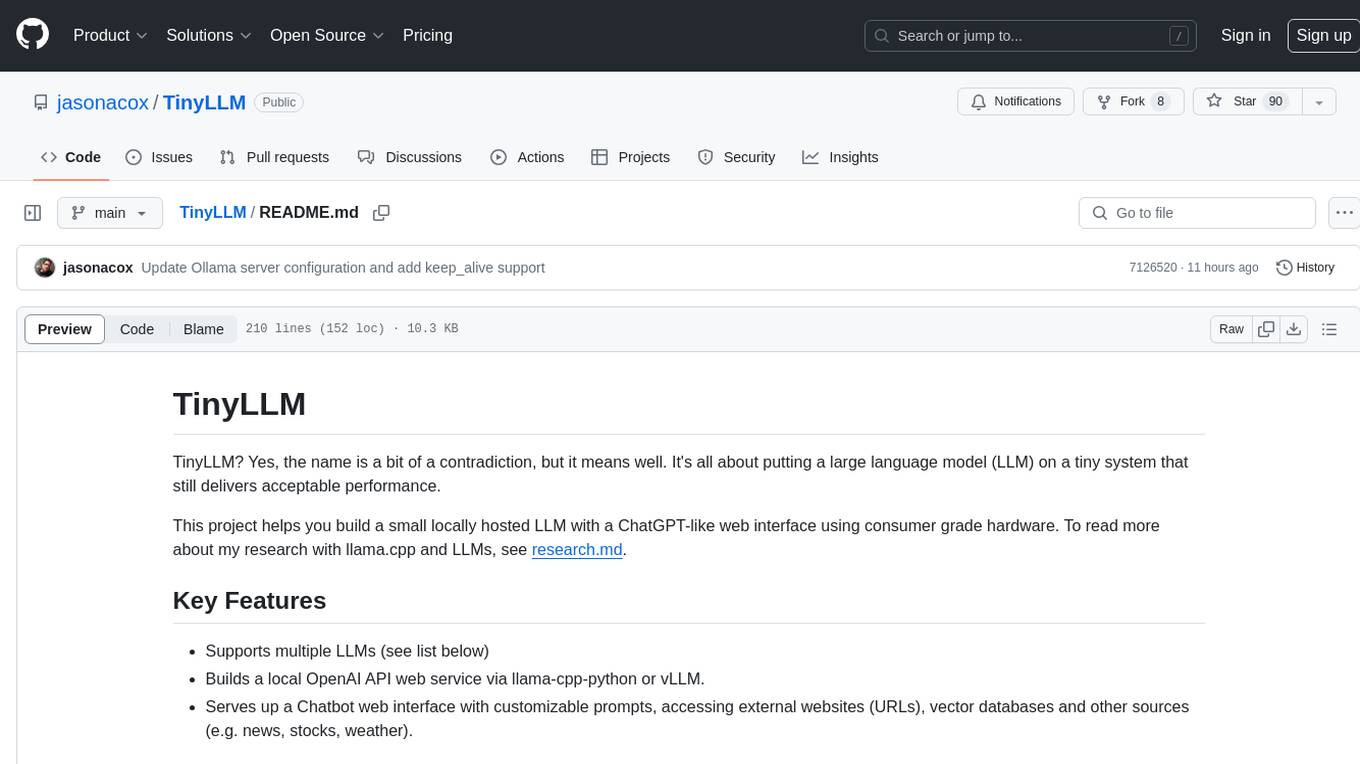
TinyLLM
TinyLLM is a project that helps build a small locally hosted language model with a web interface using consumer-grade hardware. It supports multiple language models, builds a local OpenAI API web service, and serves a Chatbot web interface with customizable prompts. The project requires specific hardware and software configurations for optimal performance. Users can run a local language model using inference servers like vLLM, llama-cpp-python, and Ollama. The Chatbot feature allows users to interact with the language model through a web-based interface, supporting features like summarizing websites, displaying news headlines, stock prices, weather conditions, and using vector databases for queries.
For similar tasks
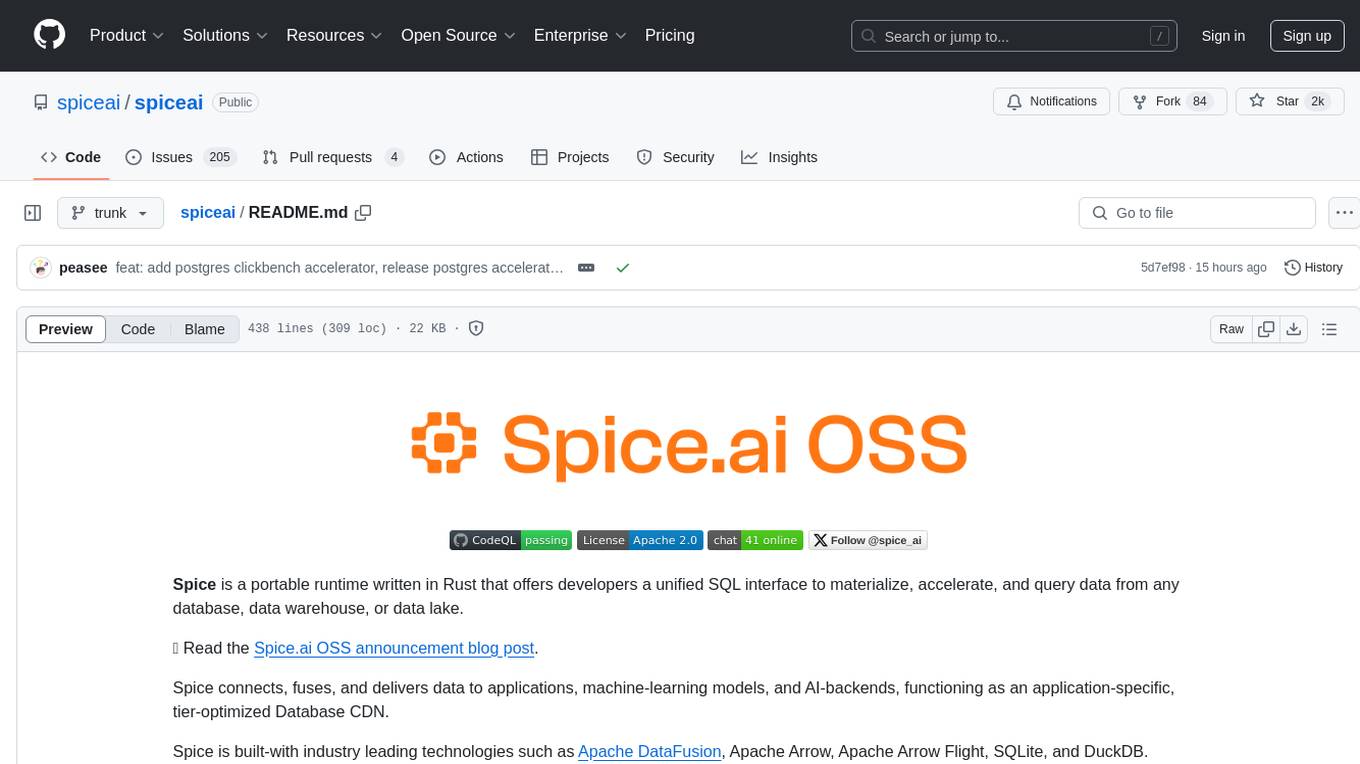
spiceai
Spice is a portable runtime written in Rust that offers developers a unified SQL interface to materialize, accelerate, and query data from any database, data warehouse, or data lake. It connects, fuses, and delivers data to applications, machine-learning models, and AI-backends, functioning as an application-specific, tier-optimized Database CDN. Built with industry-leading technologies such as Apache DataFusion, Apache Arrow, Apache Arrow Flight, SQLite, and DuckDB. Spice makes it fast and easy to query data from one or more sources using SQL, co-locating a managed dataset with applications or machine learning models, and accelerating it with Arrow in-memory, SQLite/DuckDB, or attached PostgreSQL for fast, high-concurrency, low-latency queries.
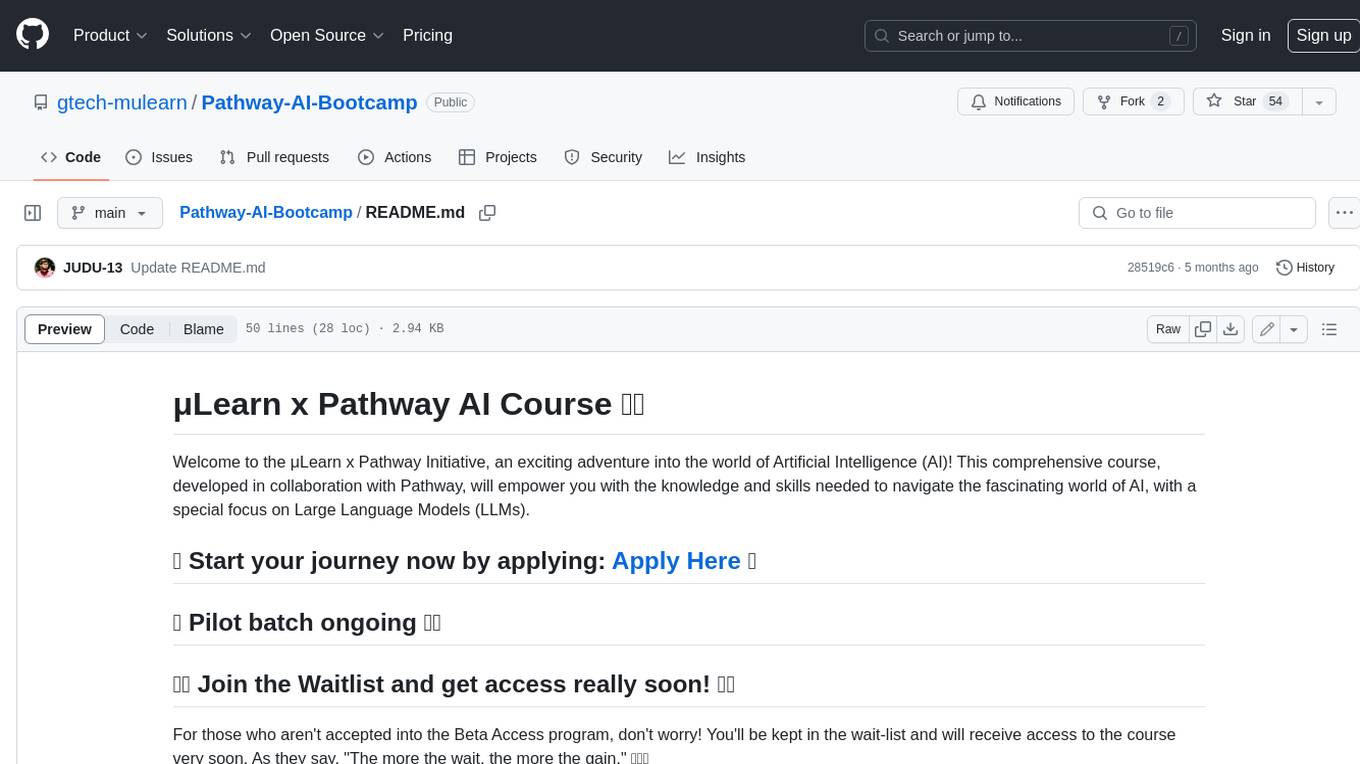
Pathway-AI-Bootcamp
Welcome to the ΞΌLearn x Pathway Initiative, an exciting adventure into the world of Artificial Intelligence (AI)! This comprehensive course, developed in collaboration with Pathway, will empower you with the knowledge and skills needed to navigate the fascinating world of AI, with a special focus on Large Language Models (LLMs).
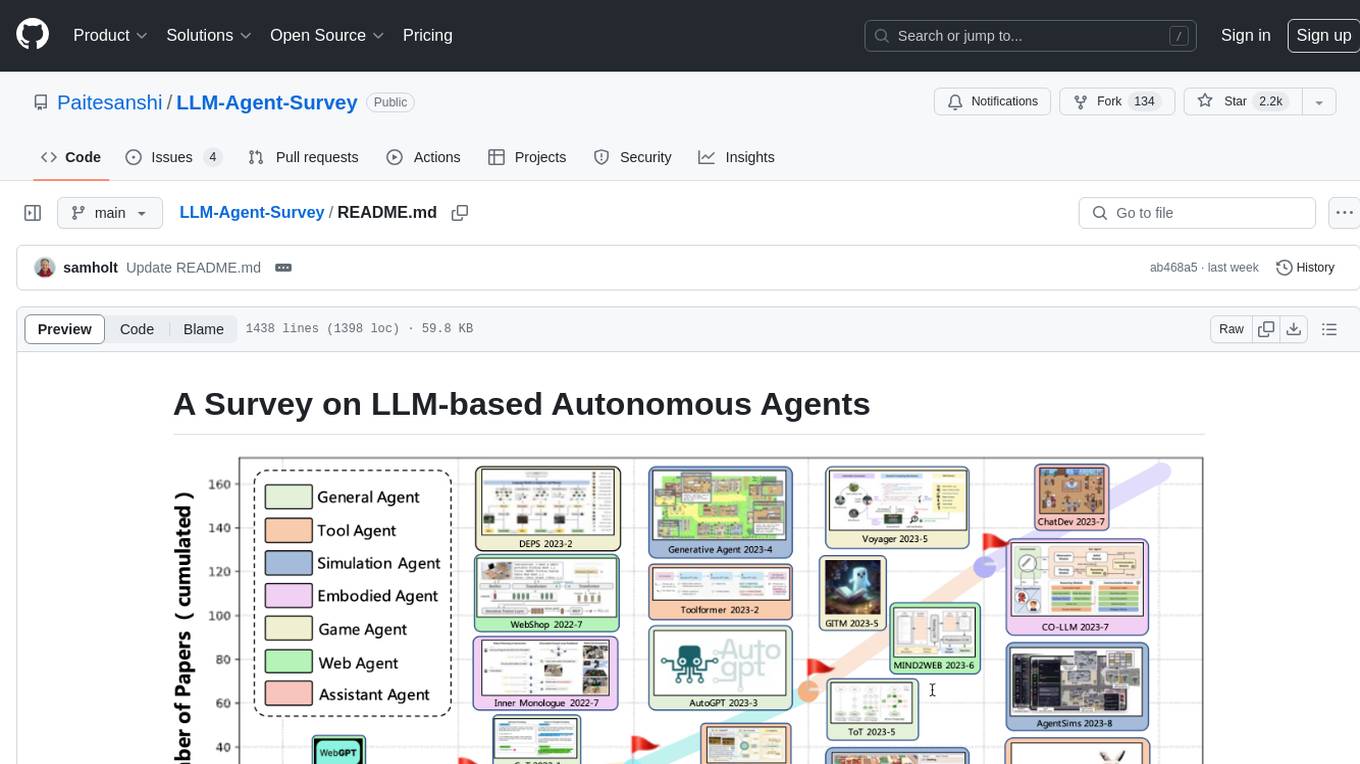
LLM-Agent-Survey
Autonomous agents are designed to achieve specific objectives through self-guided instructions. With the emergence and growth of large language models (LLMs), there is a growing trend in utilizing LLMs as fundamental controllers for these autonomous agents. This repository conducts a comprehensive survey study on the construction, application, and evaluation of LLM-based autonomous agents. It explores essential components of AI agents, application domains in natural sciences, social sciences, and engineering, and evaluation strategies. The survey aims to be a resource for researchers and practitioners in this rapidly evolving field.
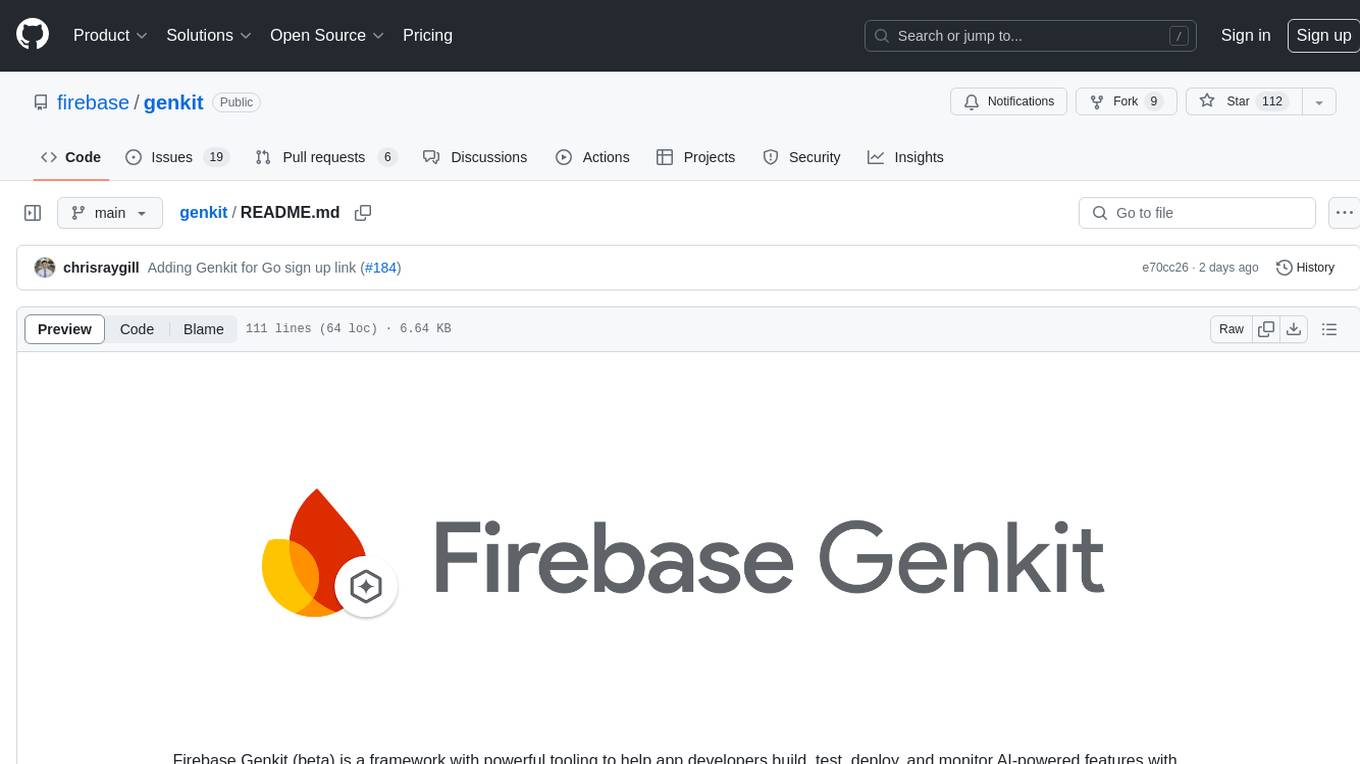
genkit
Firebase Genkit (beta) is a framework with powerful tooling to help app developers build, test, deploy, and monitor AI-powered features with confidence. Genkit is cloud optimized and code-centric, integrating with many services that have free tiers to get started. It provides unified API for generation, context-aware AI features, evaluation of AI workflow, extensibility with plugins, easy deployment to Firebase or Google Cloud, observability and monitoring with OpenTelemetry, and a developer UI for prototyping and testing AI features locally. Genkit works seamlessly with Firebase or Google Cloud projects through official plugins and templates.
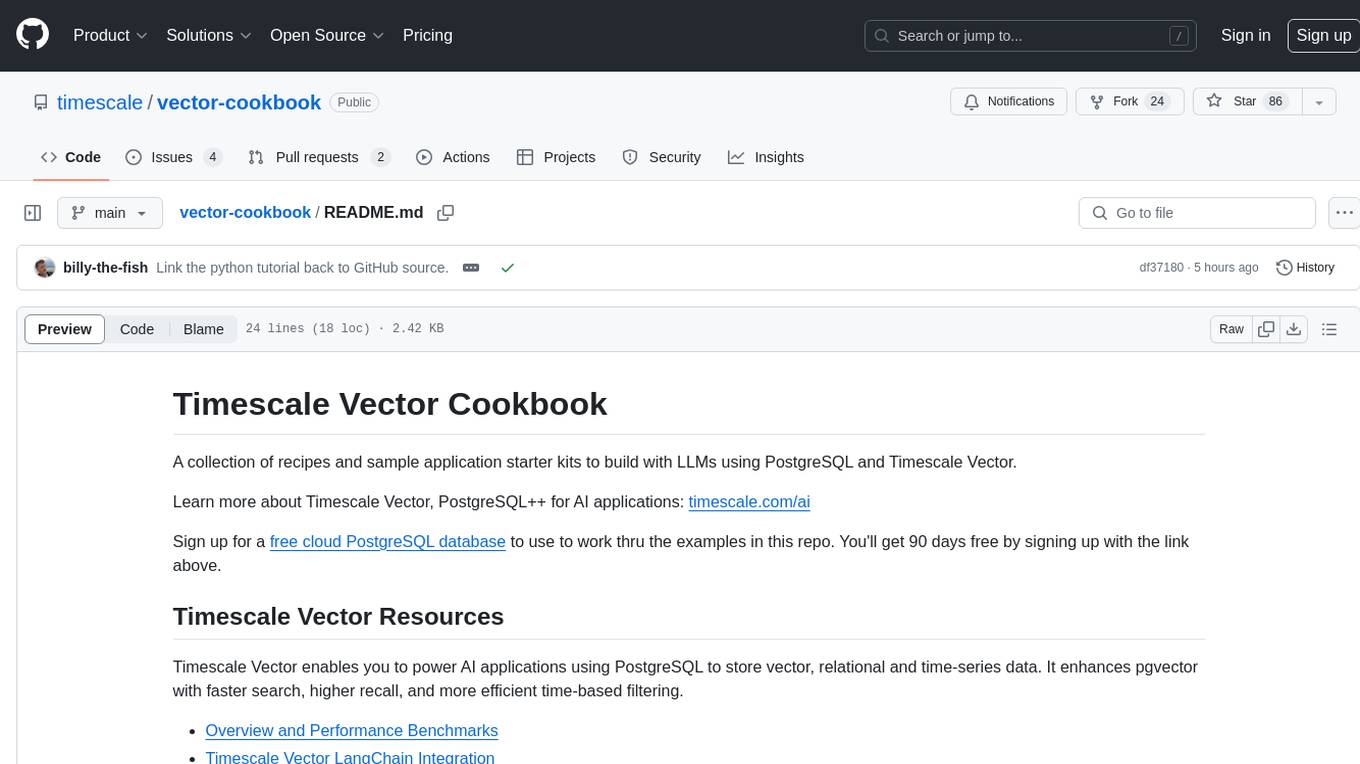
vector-cookbook
The Vector Cookbook is a collection of recipes and sample application starter kits for building AI applications with LLMs using PostgreSQL and Timescale Vector. Timescale Vector enhances PostgreSQL for AI applications by enabling the storage of vector, relational, and time-series data with faster search, higher recall, and more efficient time-based filtering. The repository includes resources, sample applications like TSV Time Machine, and guides for creating, storing, and querying OpenAI embeddings with PostgreSQL and pgvector. Users can learn about Timescale Vector, explore performance benchmarks, and access Python client libraries and tutorials.
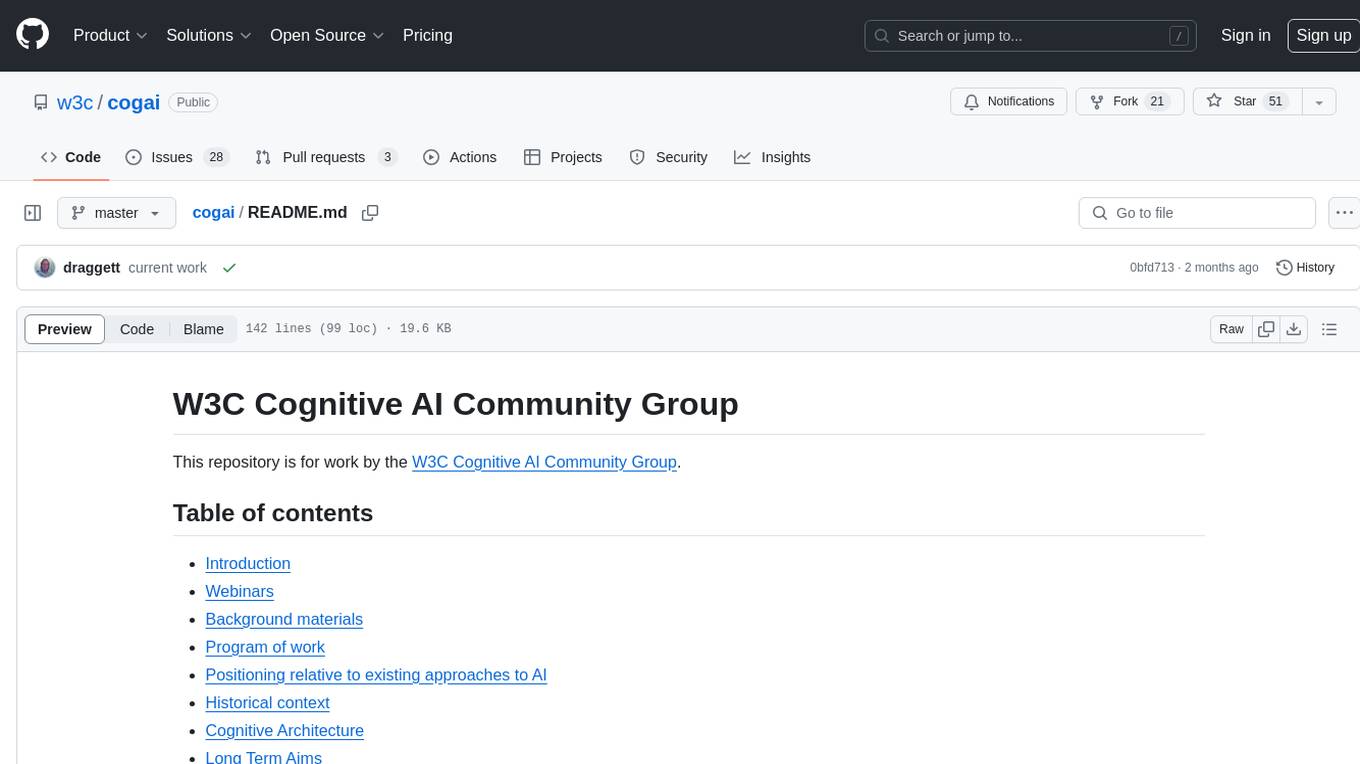
cogai
The W3C Cognitive AI Community Group focuses on advancing Cognitive AI through collaboration on defining use cases, open source implementations, and application areas. The group aims to demonstrate the potential of Cognitive AI in various domains such as customer services, healthcare, cybersecurity, online learning, autonomous vehicles, manufacturing, and web search. They work on formal specifications for chunk data and rules, plausible knowledge notation, and neural networks for human-like AI. The group positions Cognitive AI as a combination of symbolic and statistical approaches inspired by human thought processes. They address research challenges including mimicry, emotional intelligence, natural language processing, and common sense reasoning. The long-term goal is to develop cognitive agents that are knowledgeable, creative, collaborative, empathic, and multilingual, capable of continual learning and self-awareness.
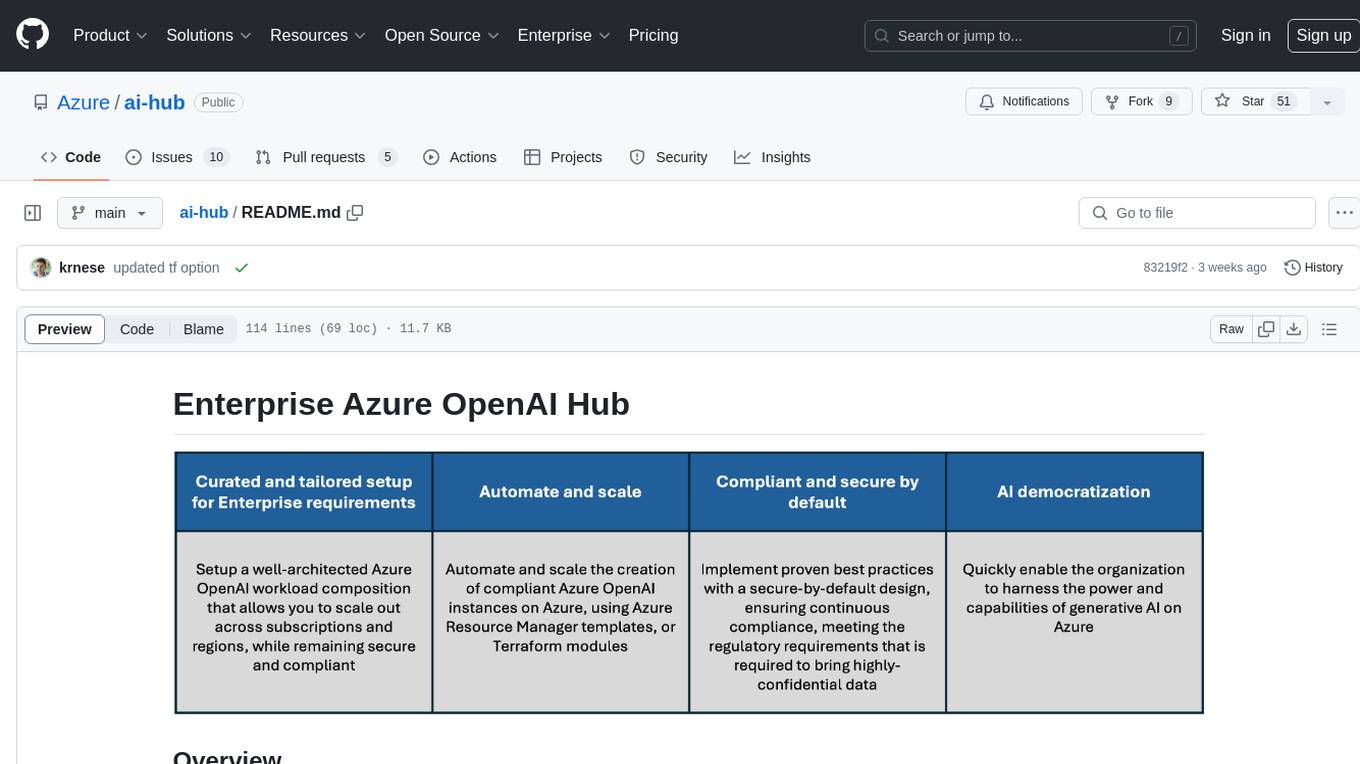
ai-hub
The Enterprise Azure OpenAI Hub is a comprehensive repository designed to guide users through the world of Generative AI on the Azure platform. It offers a structured learning experience to accelerate the transition from concept to production in an Enterprise context. The hub empowers users to explore various use cases with Azure services, ensuring security and compliance. It provides real-world examples and playbooks for practical insights into solving complex problems and developing cutting-edge AI solutions. The repository also serves as a library of proven patterns, aligning with industry standards and promoting best practices for secure and compliant AI development.
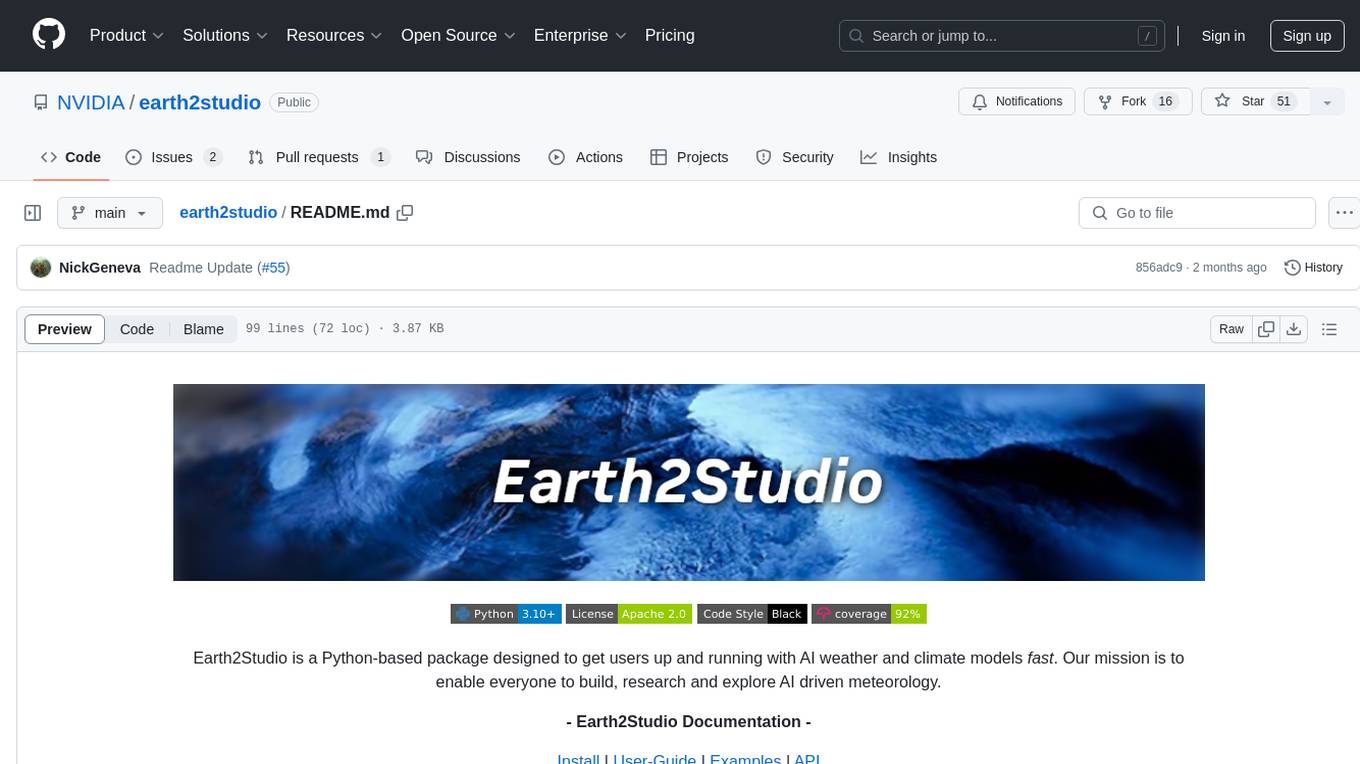
earth2studio
Earth2Studio is a Python-based package designed to enable users to quickly get started with AI weather and climate models. It provides access to pre-trained models, diagnostic tools, data sources, IO utilities, perturbation methods, and sample workflows for building custom weather prediction workflows. The package aims to empower users to explore AI-driven meteorology through modular components and seamless integration with other Nvidia packages like Modulus.
For similar jobs
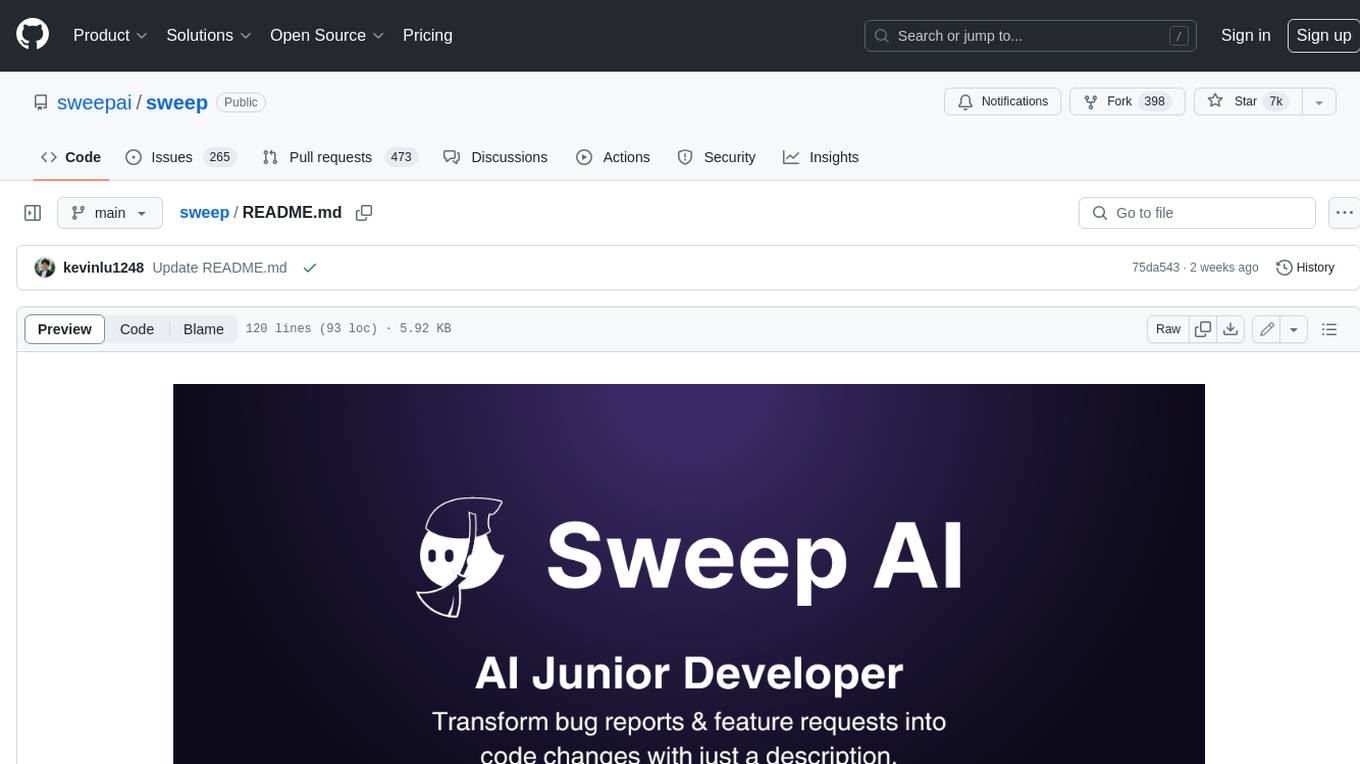
sweep
Sweep is an AI junior developer that turns bugs and feature requests into code changes. It automatically handles developer experience improvements like adding type hints and improving test coverage.
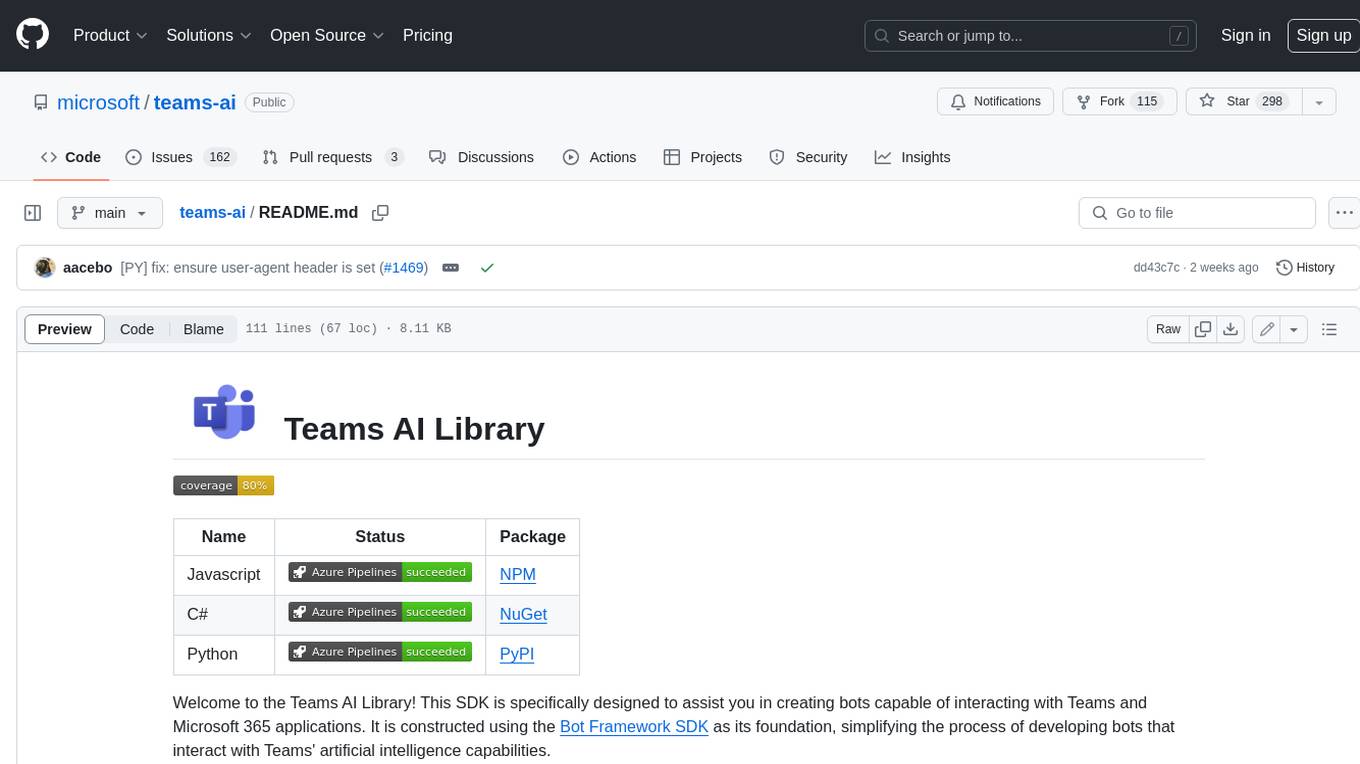
teams-ai
The Teams AI Library is a software development kit (SDK) that helps developers create bots that can interact with Teams and Microsoft 365 applications. It is built on top of the Bot Framework SDK and simplifies the process of developing bots that interact with Teams' artificial intelligence capabilities. The SDK is available for JavaScript/TypeScript, .NET, and Python.
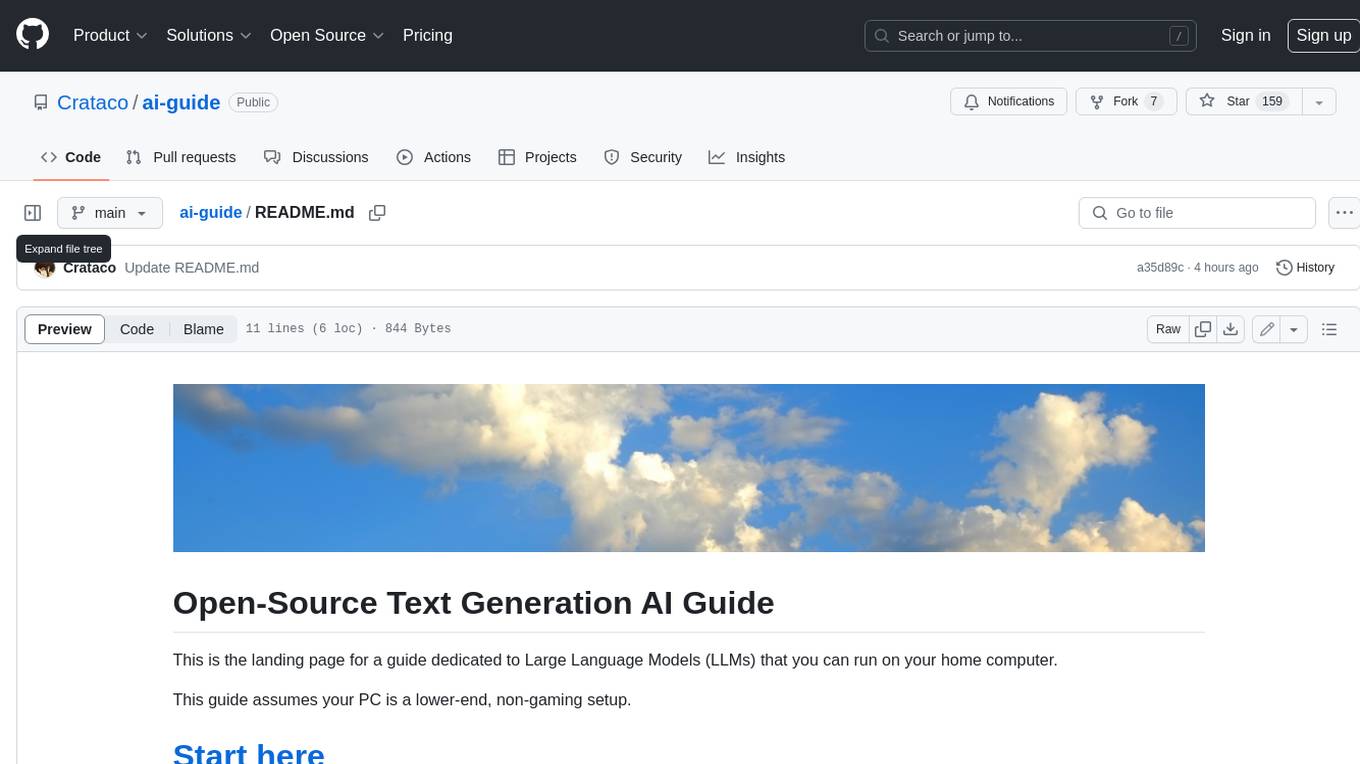
ai-guide
This guide is dedicated to Large Language Models (LLMs) that you can run on your home computer. It assumes your PC is a lower-end, non-gaming setup.
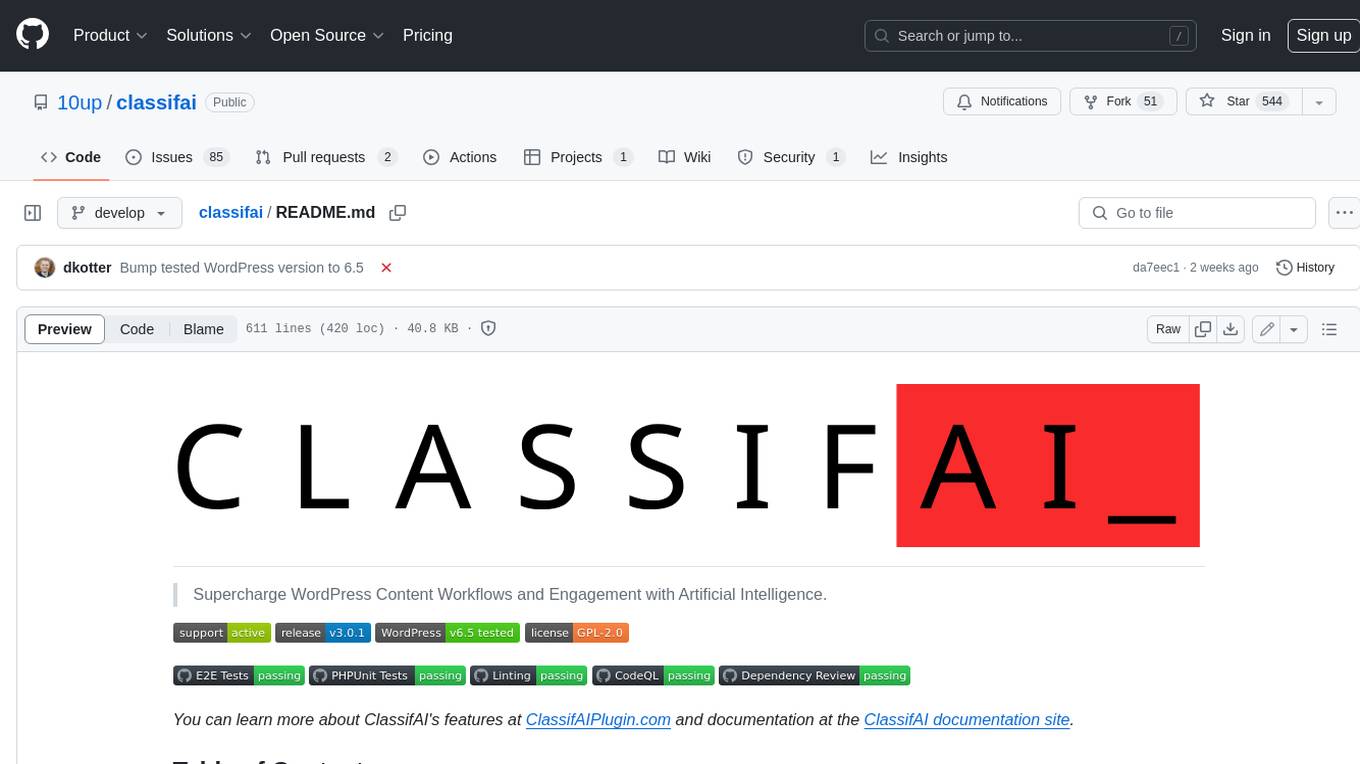
classifai
Supercharge WordPress Content Workflows and Engagement with Artificial Intelligence. Tap into leading cloud-based services like OpenAI, Microsoft Azure AI, Google Gemini and IBM Watson to augment your WordPress-powered websites. Publish content faster while improving SEO performance and increasing audience engagement. ClassifAI integrates Artificial Intelligence and Machine Learning technologies to lighten your workload and eliminate tedious tasks, giving you more time to create original content that matters.
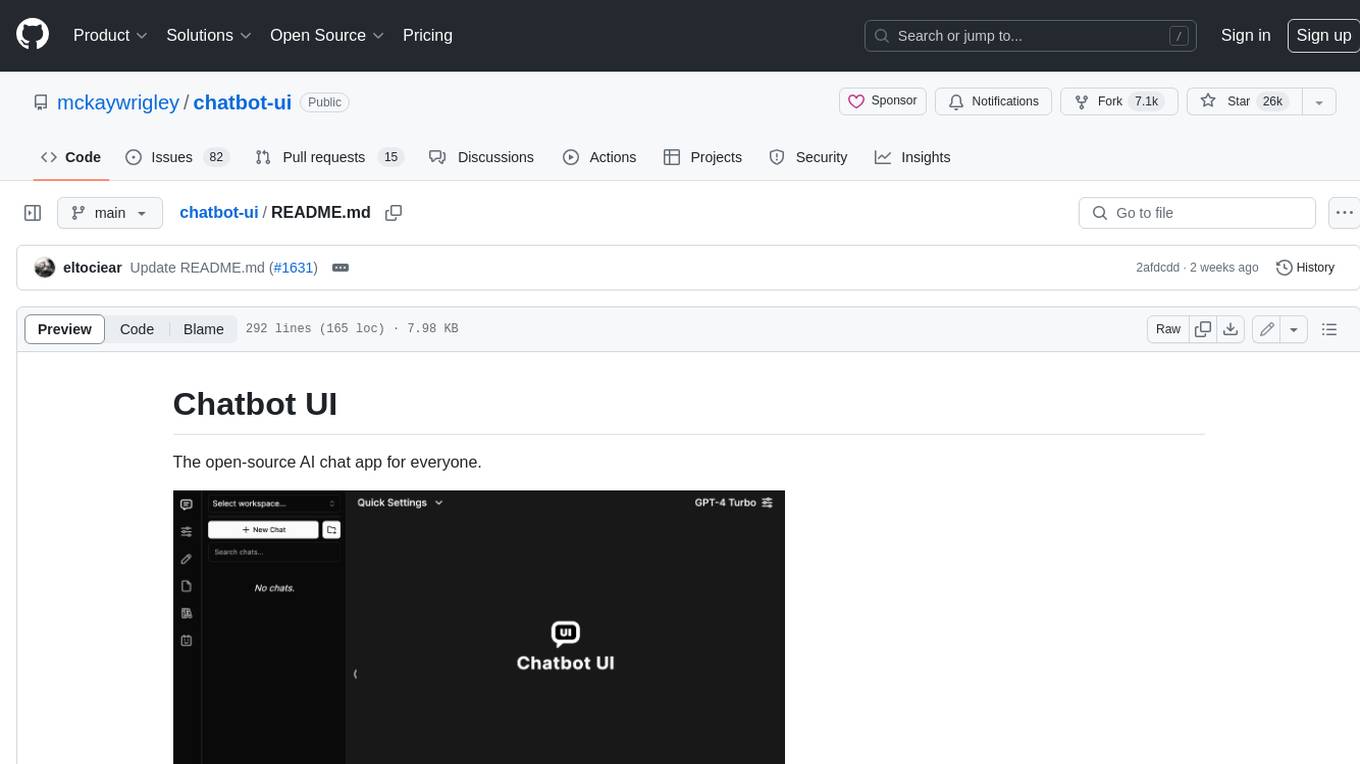
chatbot-ui
Chatbot UI is an open-source AI chat app that allows users to create and deploy their own AI chatbots. It is easy to use and can be customized to fit any need. Chatbot UI is perfect for businesses, developers, and anyone who wants to create a chatbot.
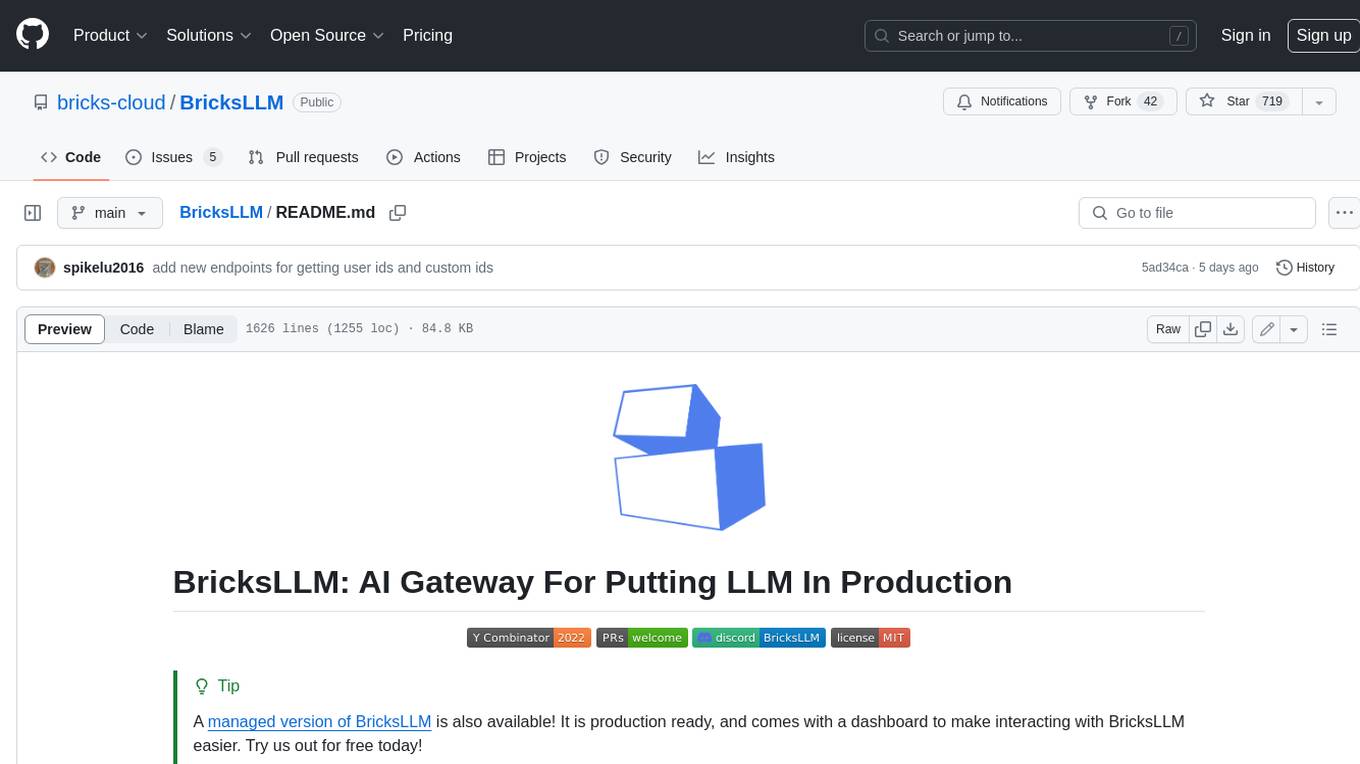
BricksLLM
BricksLLM is a cloud native AI gateway written in Go. Currently, it provides native support for OpenAI, Anthropic, Azure OpenAI and vLLM. BricksLLM aims to provide enterprise level infrastructure that can power any LLM production use cases. Here are some use cases for BricksLLM: * Set LLM usage limits for users on different pricing tiers * Track LLM usage on a per user and per organization basis * Block or redact requests containing PIIs * Improve LLM reliability with failovers, retries and caching * Distribute API keys with rate limits and cost limits for internal development/production use cases * Distribute API keys with rate limits and cost limits for students
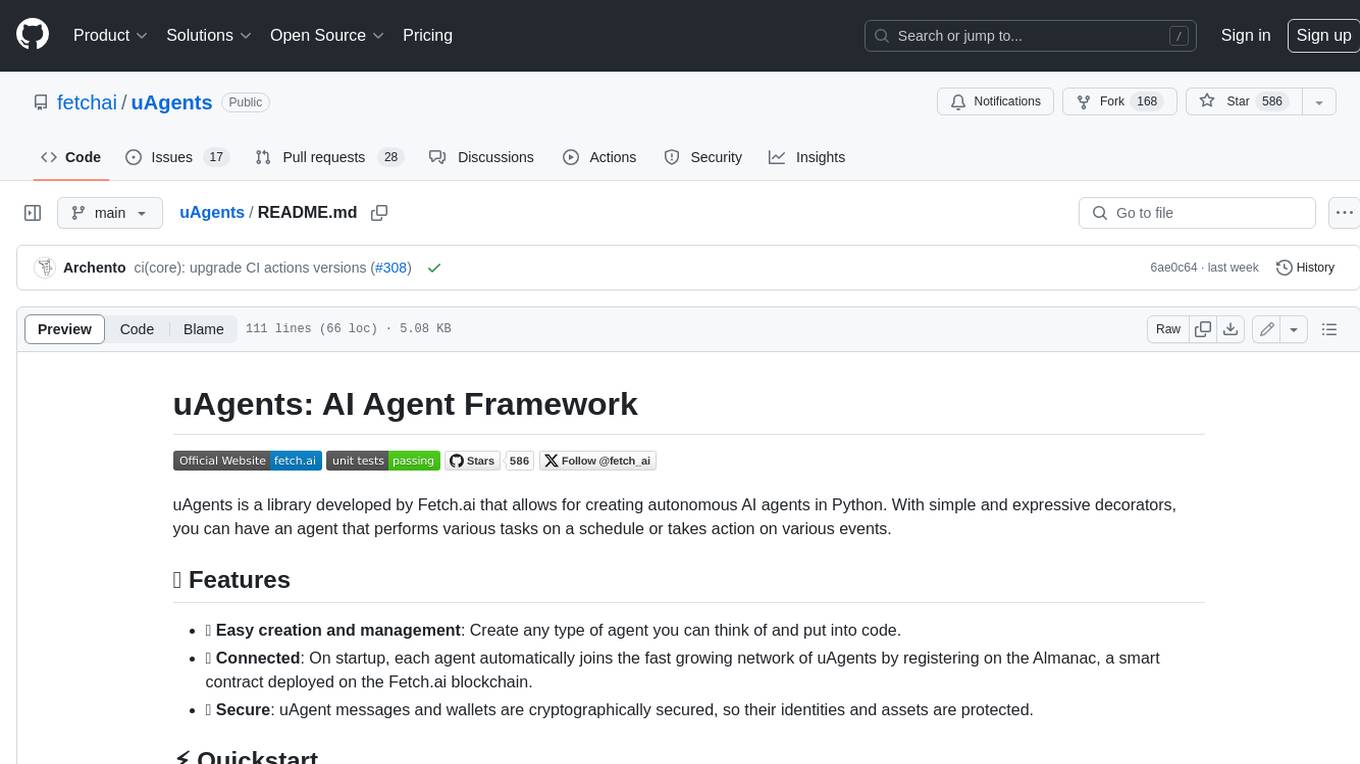
uAgents
uAgents is a Python library developed by Fetch.ai that allows for the creation of autonomous AI agents. These agents can perform various tasks on a schedule or take action on various events. uAgents are easy to create and manage, and they are connected to a fast-growing network of other uAgents. They are also secure, with cryptographically secured messages and wallets.
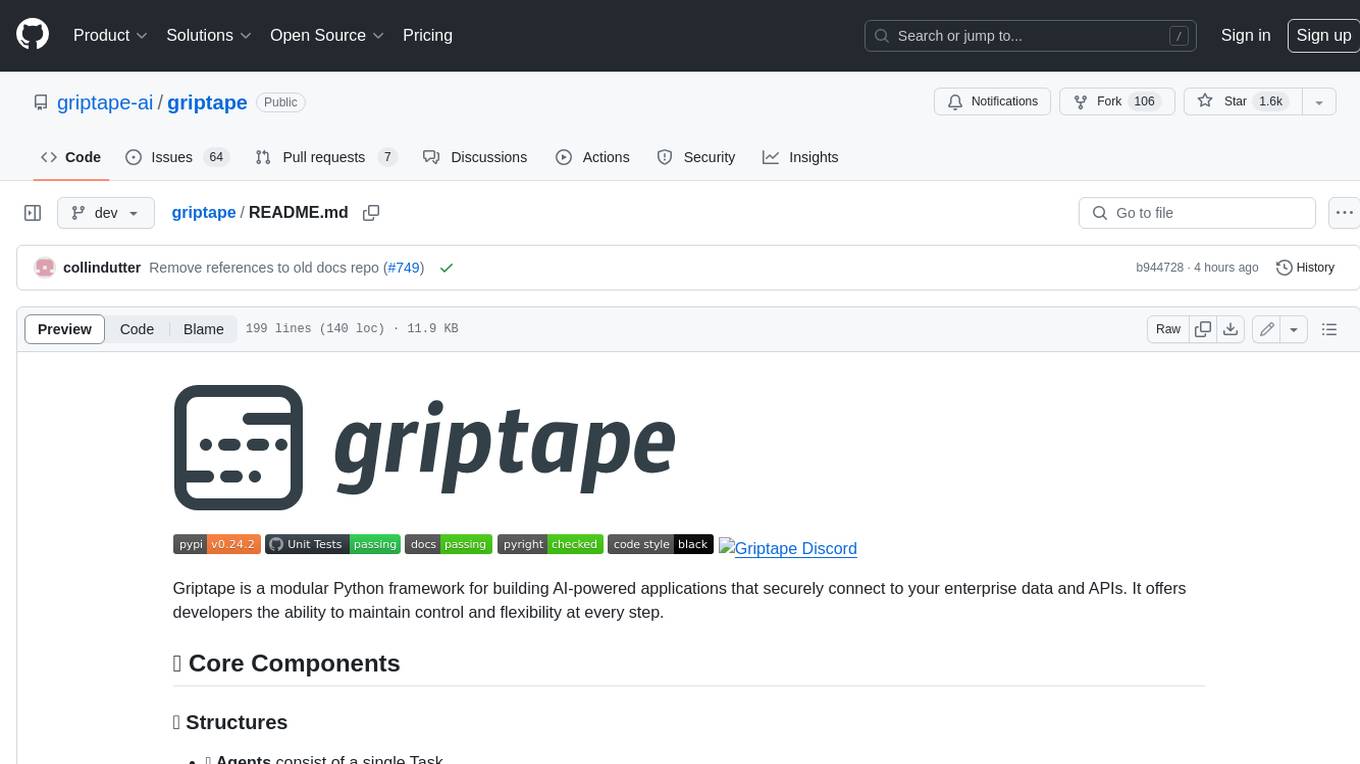
griptape
Griptape is a modular Python framework for building AI-powered applications that securely connect to your enterprise data and APIs. It offers developers the ability to maintain control and flexibility at every step. Griptape's core components include Structures (Agents, Pipelines, and Workflows), Tasks, Tools, Memory (Conversation Memory, Task Memory, and Meta Memory), Drivers (Prompt and Embedding Drivers, Vector Store Drivers, Image Generation Drivers, Image Query Drivers, SQL Drivers, Web Scraper Drivers, and Conversation Memory Drivers), Engines (Query Engines, Extraction Engines, Summary Engines, Image Generation Engines, and Image Query Engines), and additional components (Rulesets, Loaders, Artifacts, Chunkers, and Tokenizers). Griptape enables developers to create AI-powered applications with ease and efficiency.