Best AI tools for< Accelerate Datasets >
20 - AI tool Sites
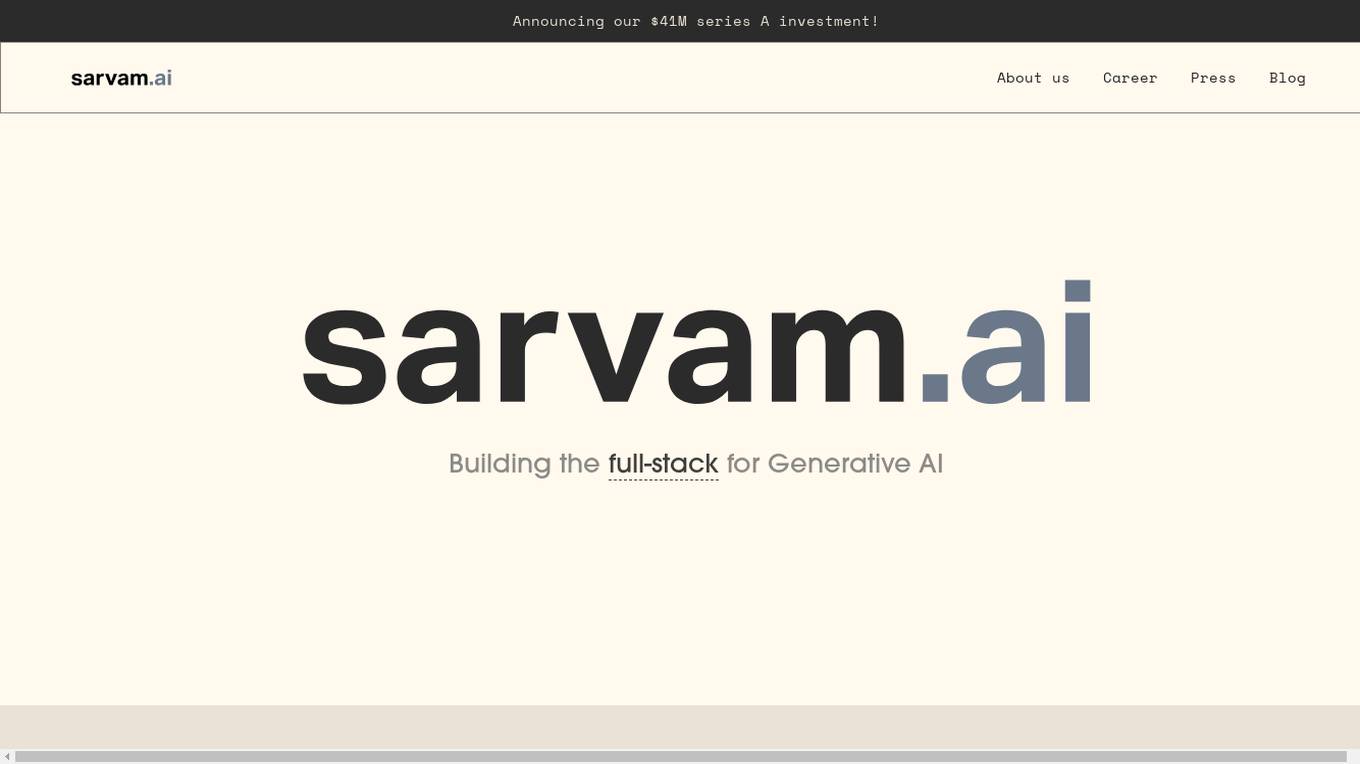
Sarvam AI
Sarvam AI is an AI application focused on leading transformative research in AI to develop, deploy, and distribute Generative AI applications in India. The platform aims to build efficient large language models for India's diverse linguistic culture and enable new GenAI applications through bespoke enterprise models. Sarvam AI is also developing an enterprise-grade platform for developing and evaluating GenAI apps, while contributing to open-source models and datasets to accelerate AI innovation.
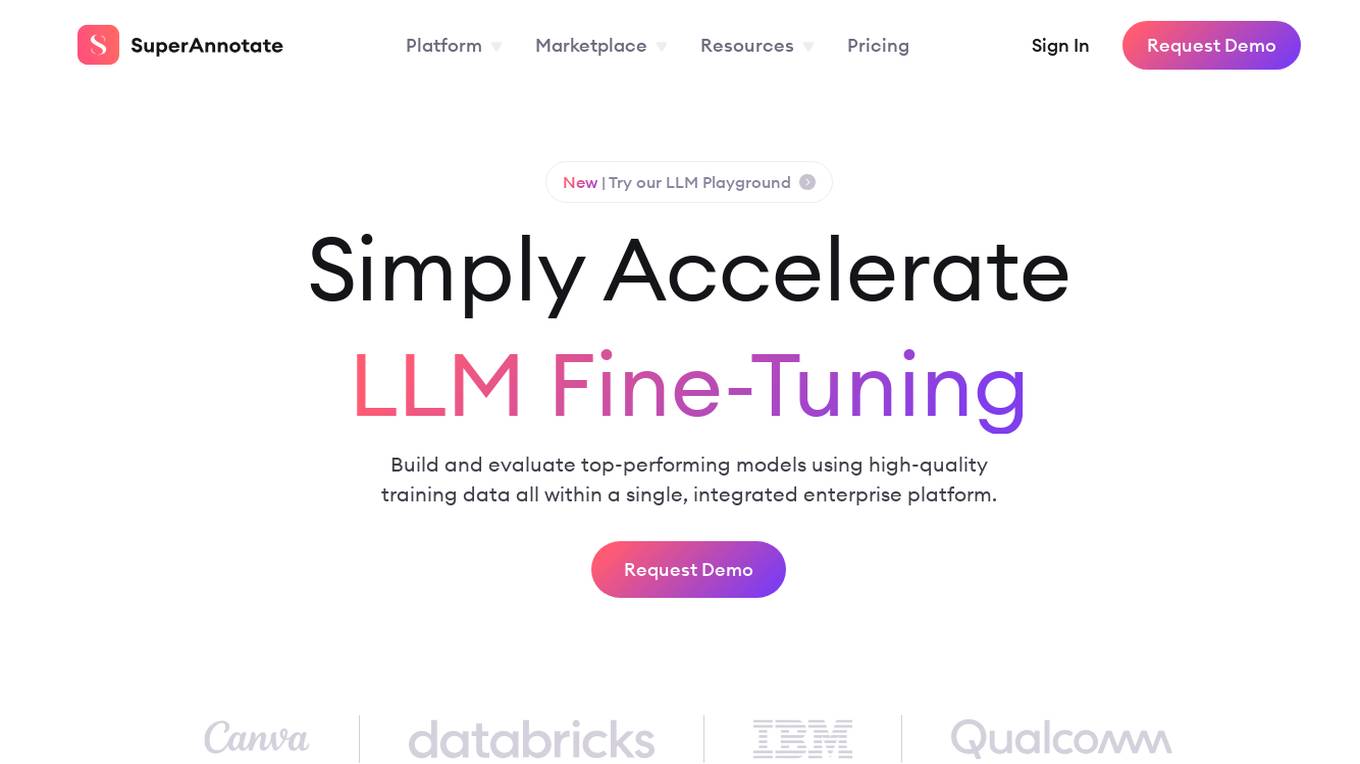
SuperAnnotate
SuperAnnotate is an AI data platform that simplifies and accelerates model-building by unifying the AI pipeline. It enables users to create, curate, and evaluate datasets efficiently, leading to the development of better models faster. The platform offers features like connecting any data source, building customizable UIs, creating high-quality datasets, evaluating models, and deploying models seamlessly. SuperAnnotate ensures global security and privacy measures for data protection.
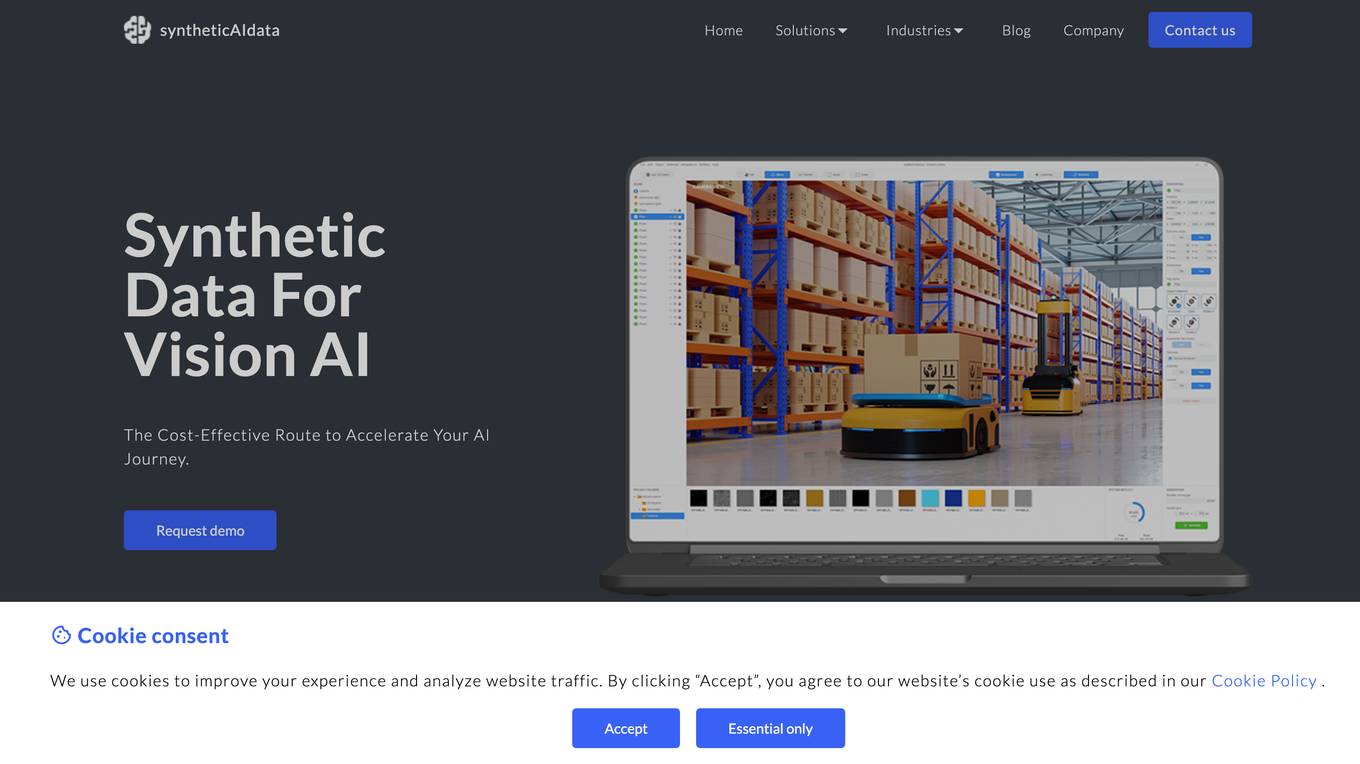
syntheticAIdata
syntheticAIdata is a platform that provides synthetic data for training vision AI models. Synthetic data is generated artificially, and it can be used to augment existing real-world datasets or to create new datasets from scratch. syntheticAIdata's platform is easy to use, and it can be integrated with leading cloud platforms. The company's mission is to make synthetic data accessible to everyone, and to help businesses overcome the challenges of acquiring high-quality data for training their vision AI models.
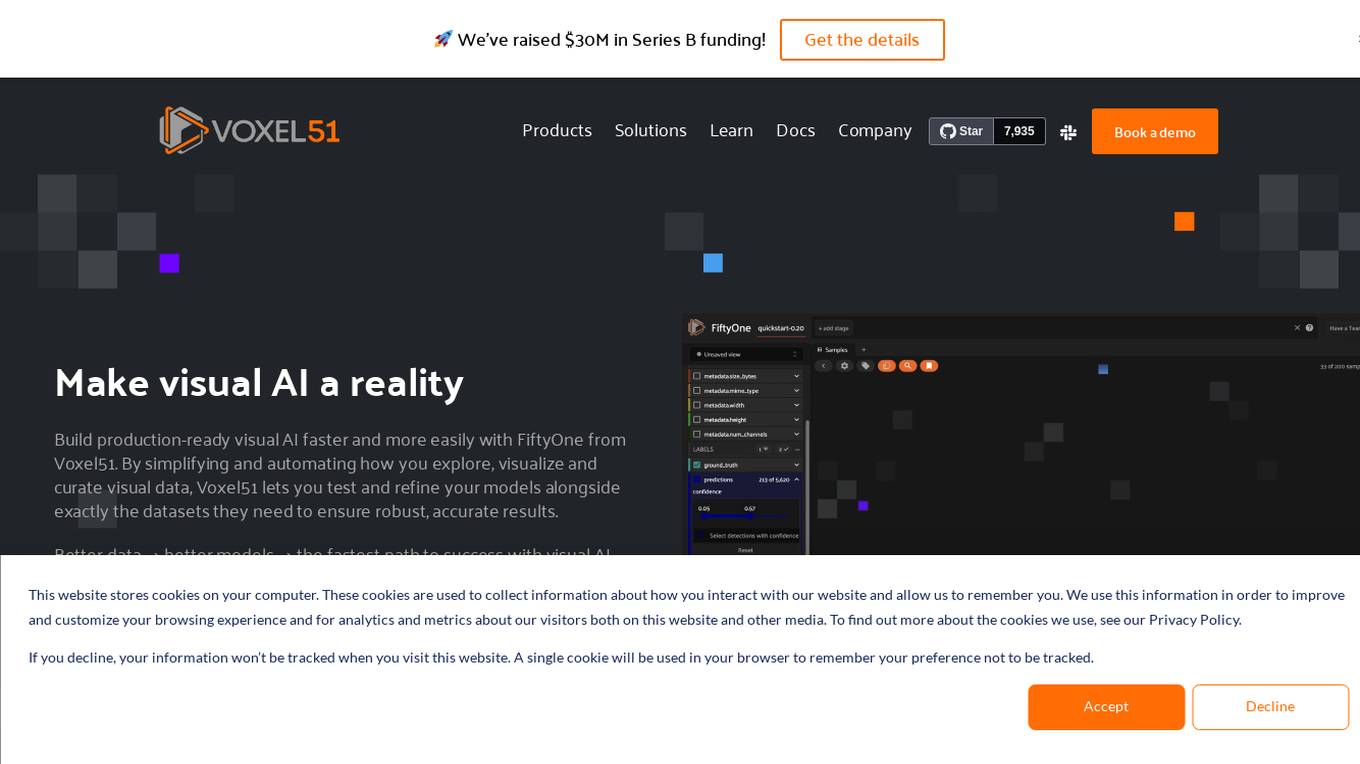
Voxel51
Voxel51 is an AI tool that provides open-source computer vision tools for machine learning. It offers solutions for various industries such as agriculture, aviation, driving, healthcare, manufacturing, retail, robotics, and security. Voxel51's main product, FiftyOne, helps users explore, visualize, and curate visual data to improve model performance and accelerate the development of visual AI applications. The platform is trusted by thousands of users and companies, offering both open-source and enterprise-ready solutions to manage and refine data and models for visual AI.
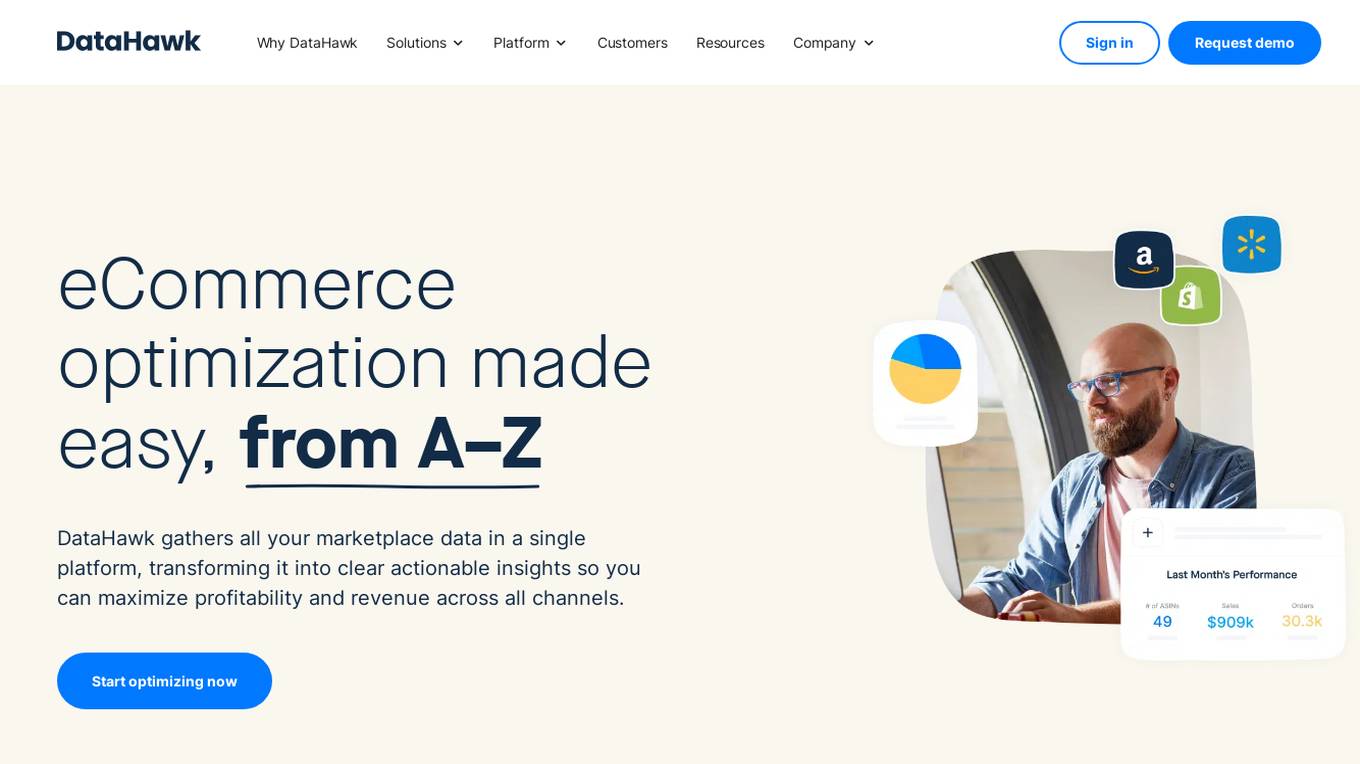
DataHawk
DataHawk is an eCommerce analytics platform powered by AI that helps users optimize their eCommerce operations from A to Z. It provides competitive intelligence, performance analysis, conversion optimization, sales growth, brand image protection, traffic generation, and more. DataHawk offers automated data collection, composable analytics, AI-powered productivity, integrations, exclusive datasets, and professional services. The platform empowers users with end-to-end visibility, comprehensive marketplace data, flexibility, control over operational data, and expert support. With AI capabilities, DataHawk automates data collection, detects anomalies, generates recommended actions, and augments productivity. The platform enhances revenue, RoAS, and time savings for users, supported by dedicated experts and customer success stories.
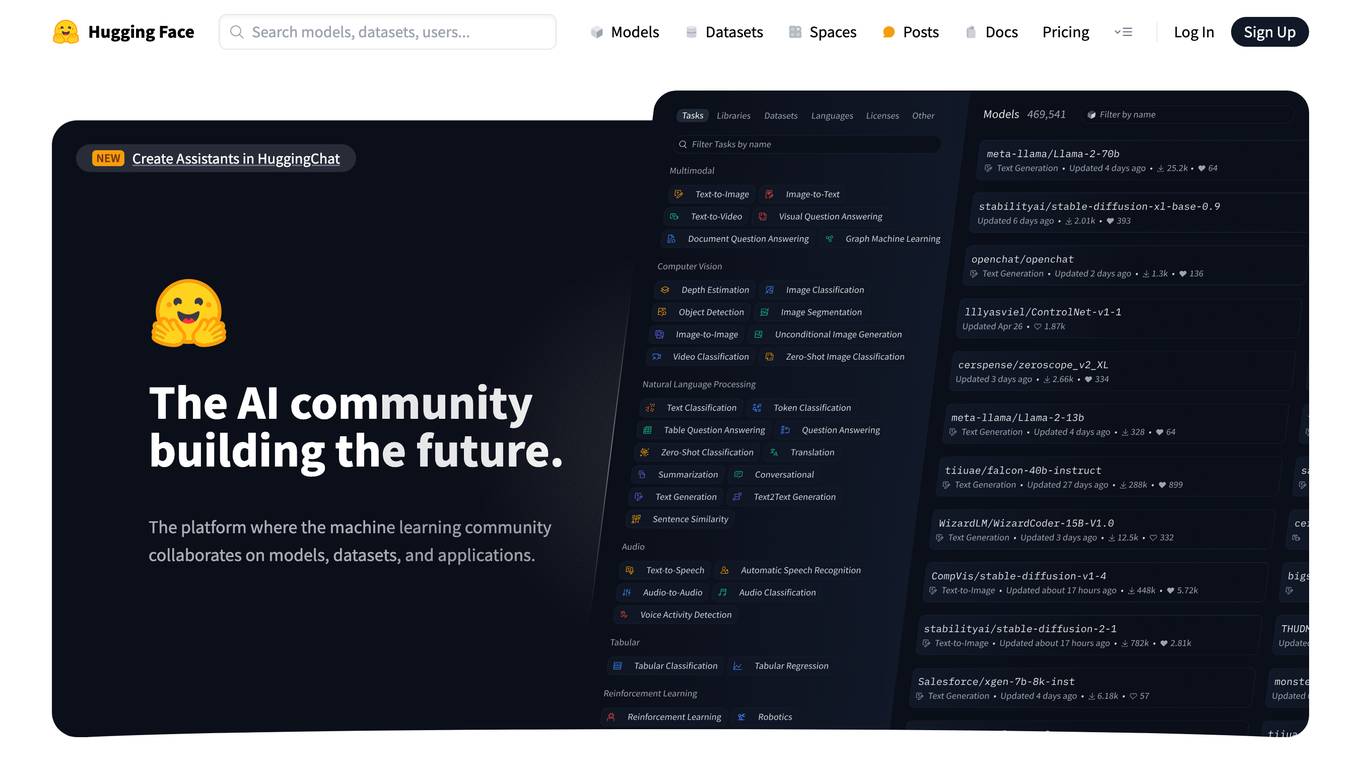
Hugging Face
Hugging Face is an AI community platform where the machine learning community collaborates on models, datasets, and applications. It provides a space for users to create, discover, and collaborate on machine learning projects. The platform offers a wide range of tools and resources to accelerate machine learning development and deployment, including paid compute and enterprise solutions. Hugging Face aims to build the future of AI by fostering collaboration and innovation within the community.
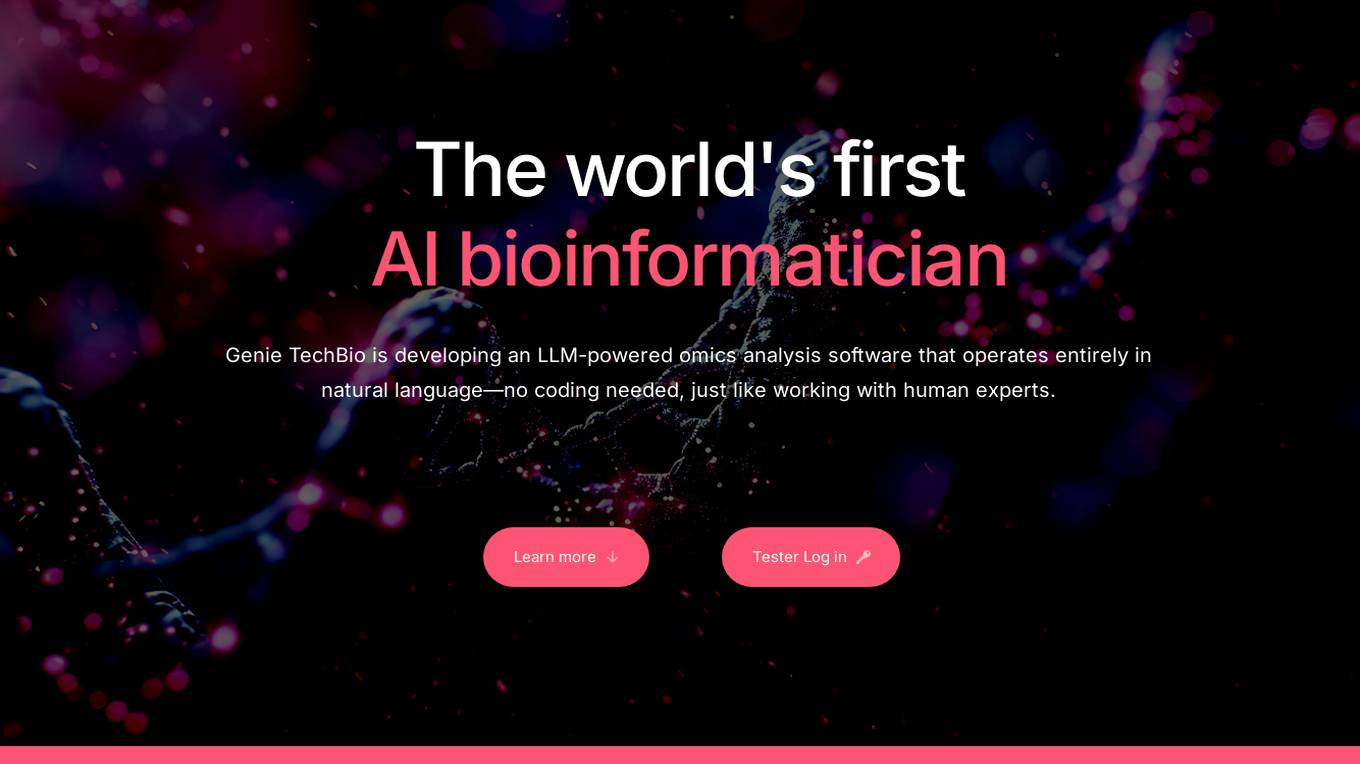
Genie TechBio
Genie TechBio is the world's first AI bioinformatician, offering an LLM-powered omics analysis software that operates entirely in natural language, eliminating the need for coding. Researchers can effortlessly analyze extensive datasets by engaging in a conversation with Genie, receiving recommendations for analysis pipelines, and obtaining results. The tool aims to accelerate biomedical research and empower scientists with newfound data analysis capabilities.
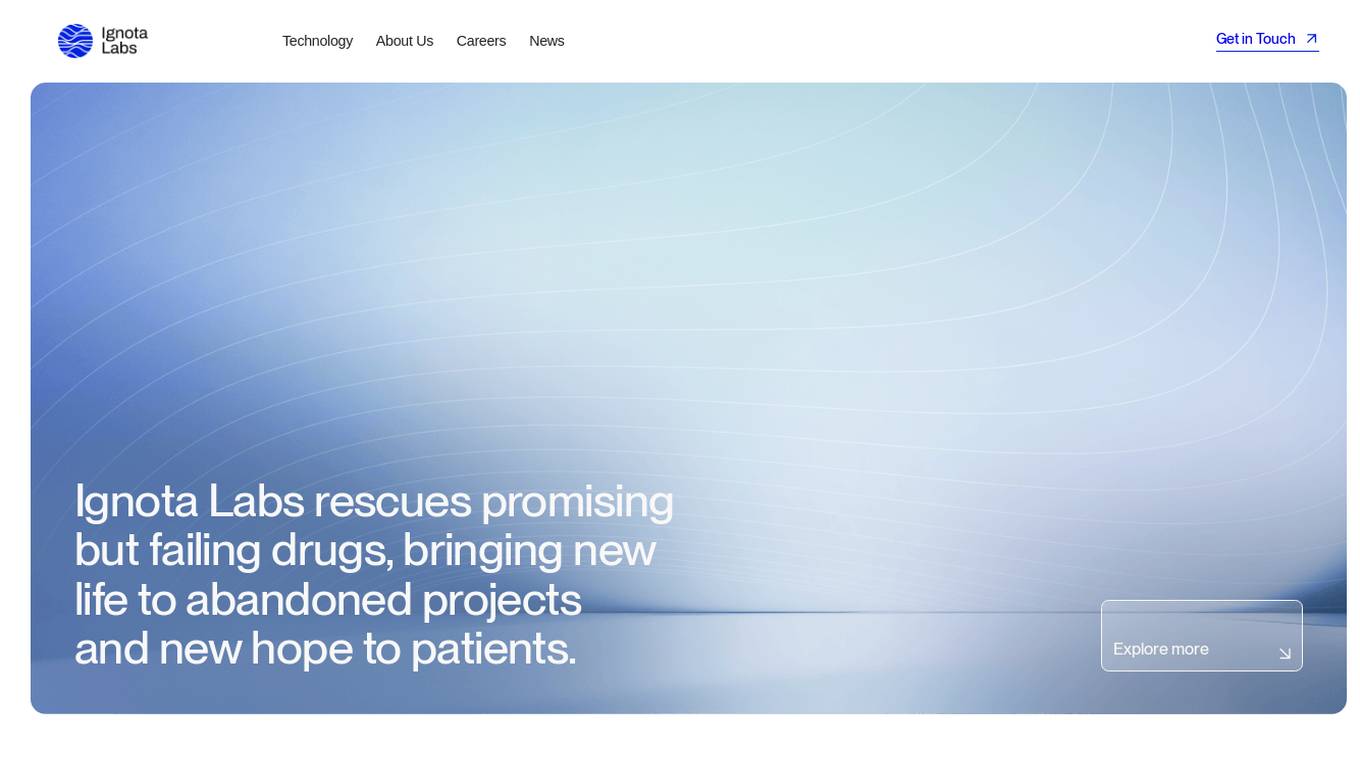
Ignota Labs
Ignota Labs is a technology company focused on rescuing failing drugs and bringing new life to abandoned projects, ultimately providing hope to patients. The company utilizes a proprietary AI model, SAFEPATH, which applies deep learning to bioinformatics and cheminformatics datasets to solve drug safety issues. Ignota Labs aims to identify promising drug targets, address safety problems in clinical trials, and accelerate the delivery of therapeutically effective drugs to patients.
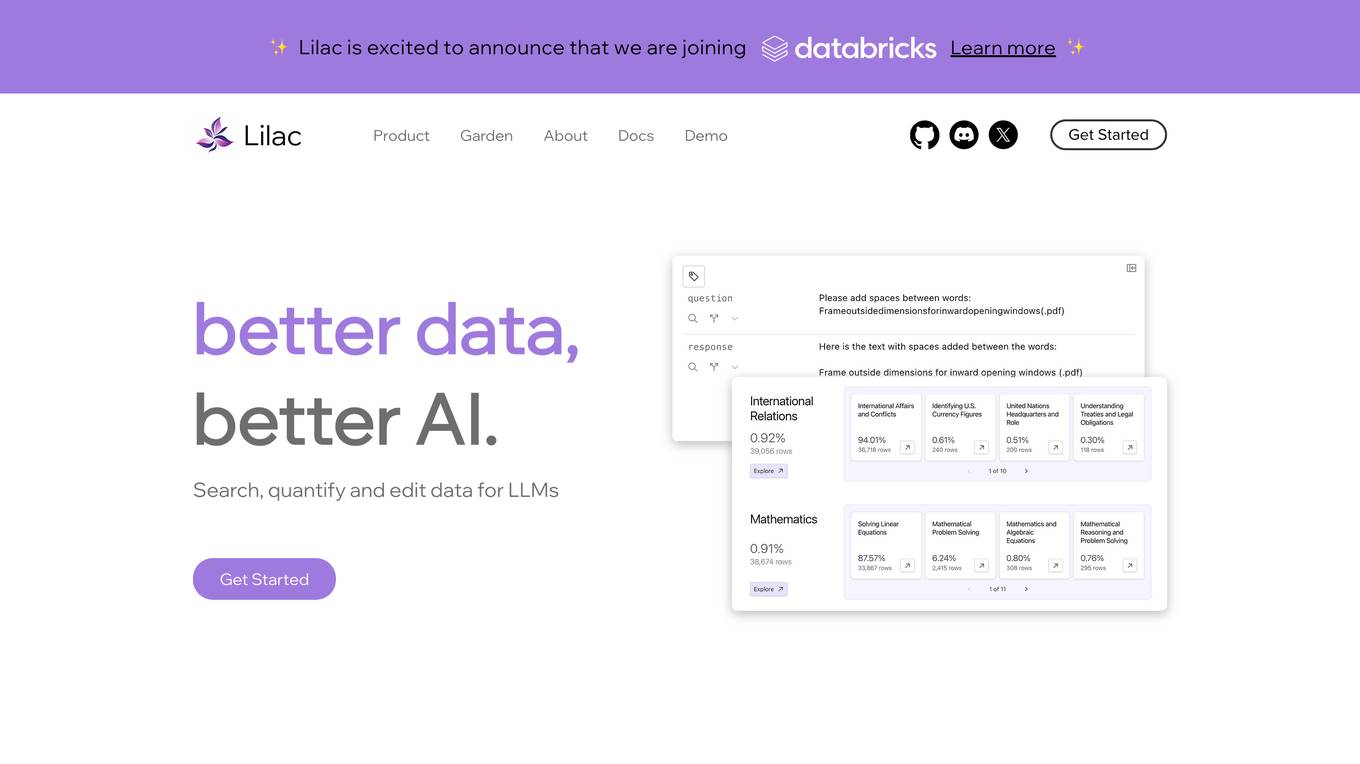
Lilac
Lilac is an AI tool designed to enhance data quality and exploration for AI applications. It offers features such as data search, quantification, editing, clustering, semantic search, field comparison, and fuzzy-concept search. Lilac enables users to accelerate dataset computations and transformations, making it a valuable asset for data scientists and AI practitioners. The tool is trusted by Alignment Lab and is recommended for working with LLM datasets.
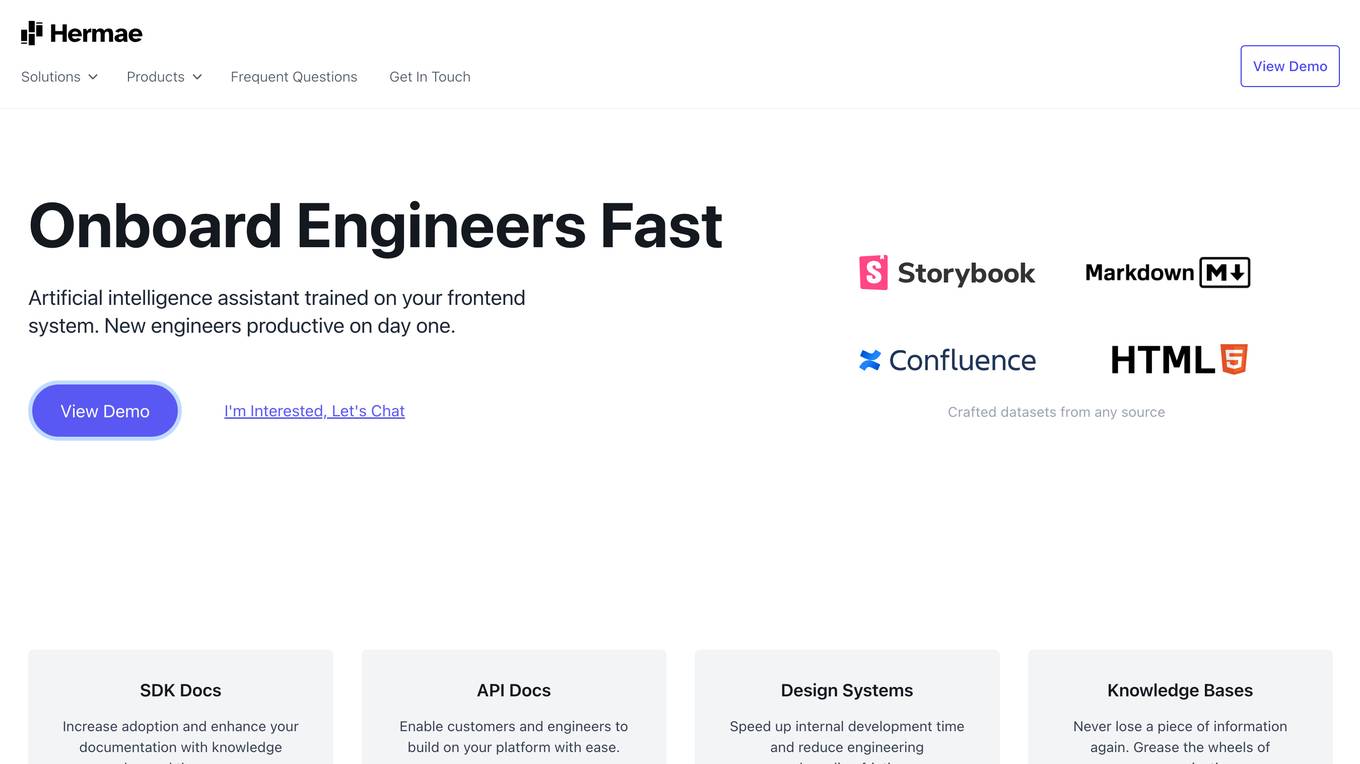
Hermae Solutions
Hermae Solutions offers an AI Assistant for Enterprise Design Systems, providing onboarding acceleration, contractor efficiency, design system adoption support, and knowledge distribution. The AI Documentation Assistant and AI Storybook Assistant help boost frontend productivity and enable continuous training of artificial intelligence profiles. The platform also includes features like crafted datasets, SDK Docs, API Docs, and Assistant Embed for Documentation. Hermae's process simplifies efficiency improvements by gathering information sources, processing data for AI supplementation, customizing integration, and supporting integration success. The AI assistant reduces engineering costs significantly, saving time and money for organizations.
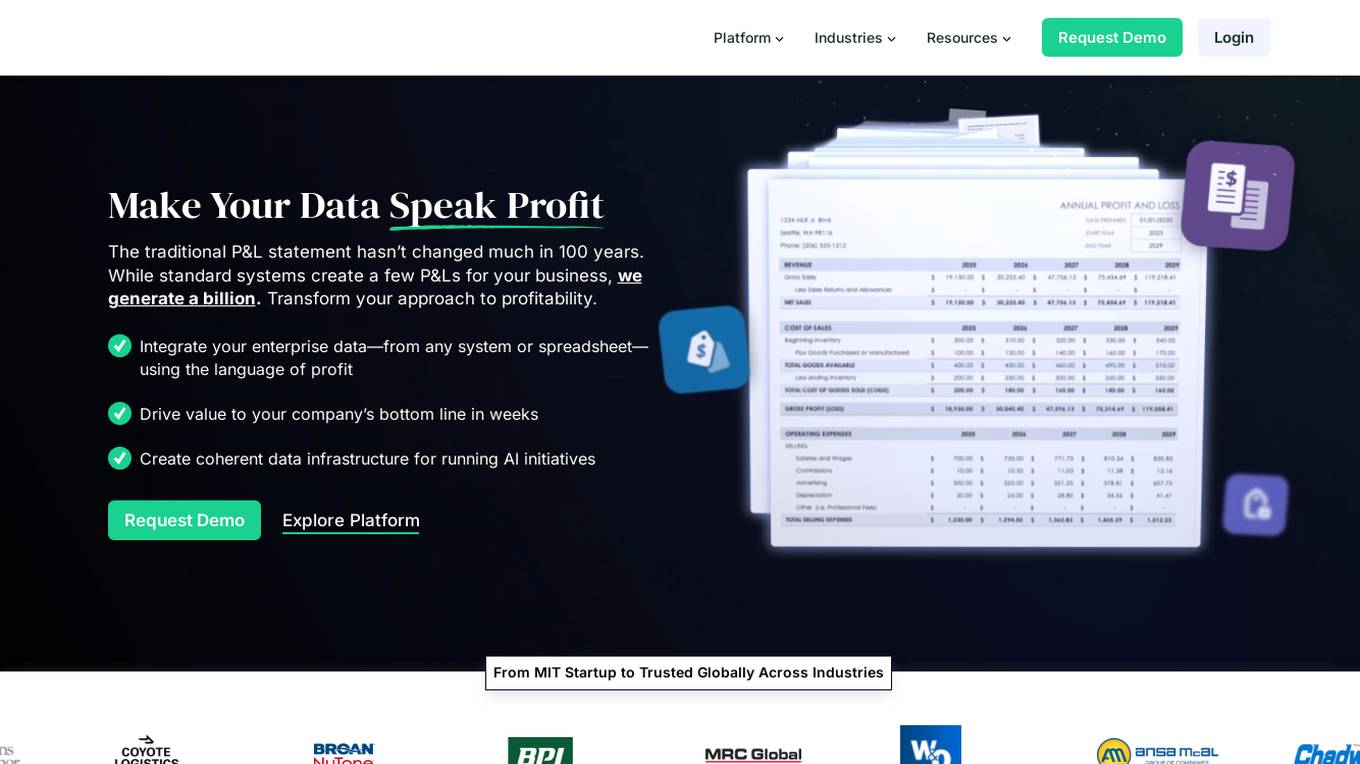
Profit Isle
Profit Isle is an AI application that helps enterprises make data-driven decisions to enhance profitability and drive value to the bottom line. The platform integrates and transforms enterprise data to power AI initiatives, providing actionable insights and recommendations grounded in company data. Profit Isle prioritizes transparency, data governance, and privacy to ensure customers can confidently run AI models and make informed decisions.
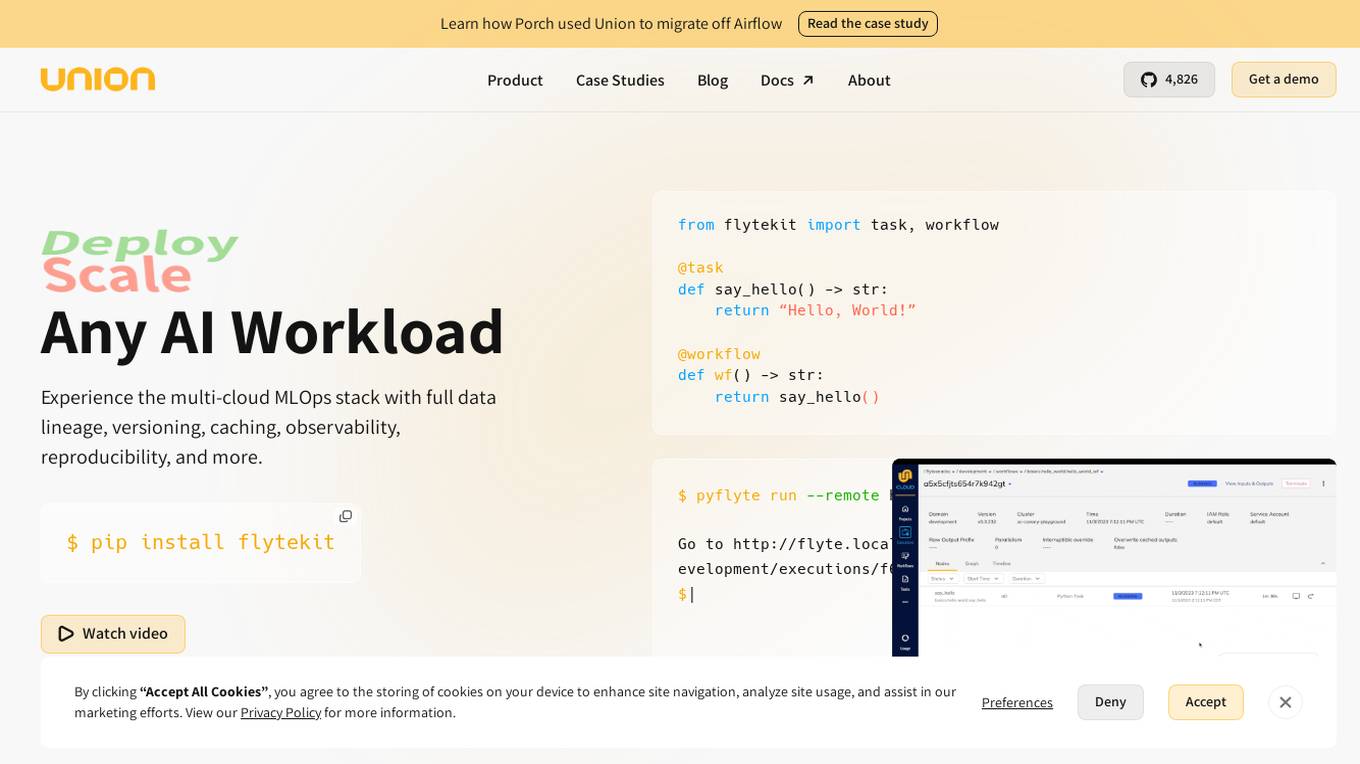
Union.ai
Union.ai is an infrastructure platform designed for AI, ML, and data workloads. It offers a scalable MLOps platform that optimizes resources, reduces costs, and fosters collaboration among team members. Union.ai provides features such as declarative infrastructure, data lineage tracking, accelerated datasets, and more to streamline AI orchestration on Kubernetes. It aims to simplify the management of AI, ML, and data workflows in production environments by addressing complexities and offering cost-effective strategies.
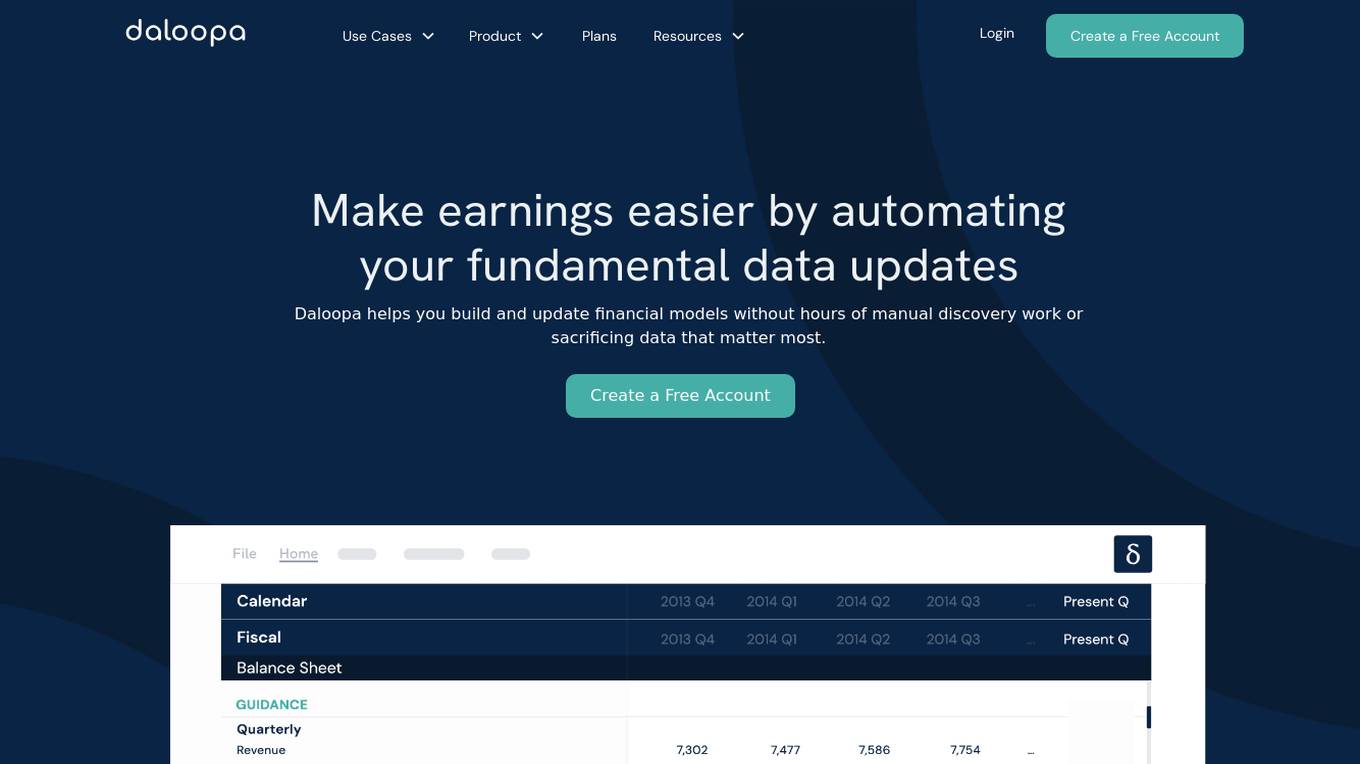
Daloopa
Daloopa is an AI financial modeling tool designed to automate fundamental data updates for financial analysts working in Excel. It helps analysts build and update financial models efficiently by eliminating manual work and providing accurate, auditable data points sourced from thousands of companies. Daloopa leverages AI technology to deliver complete and comprehensive data sets faster than humanly possible, enabling analysts to focus on analysis, insight generation, and idea development to drive better investment decisions.
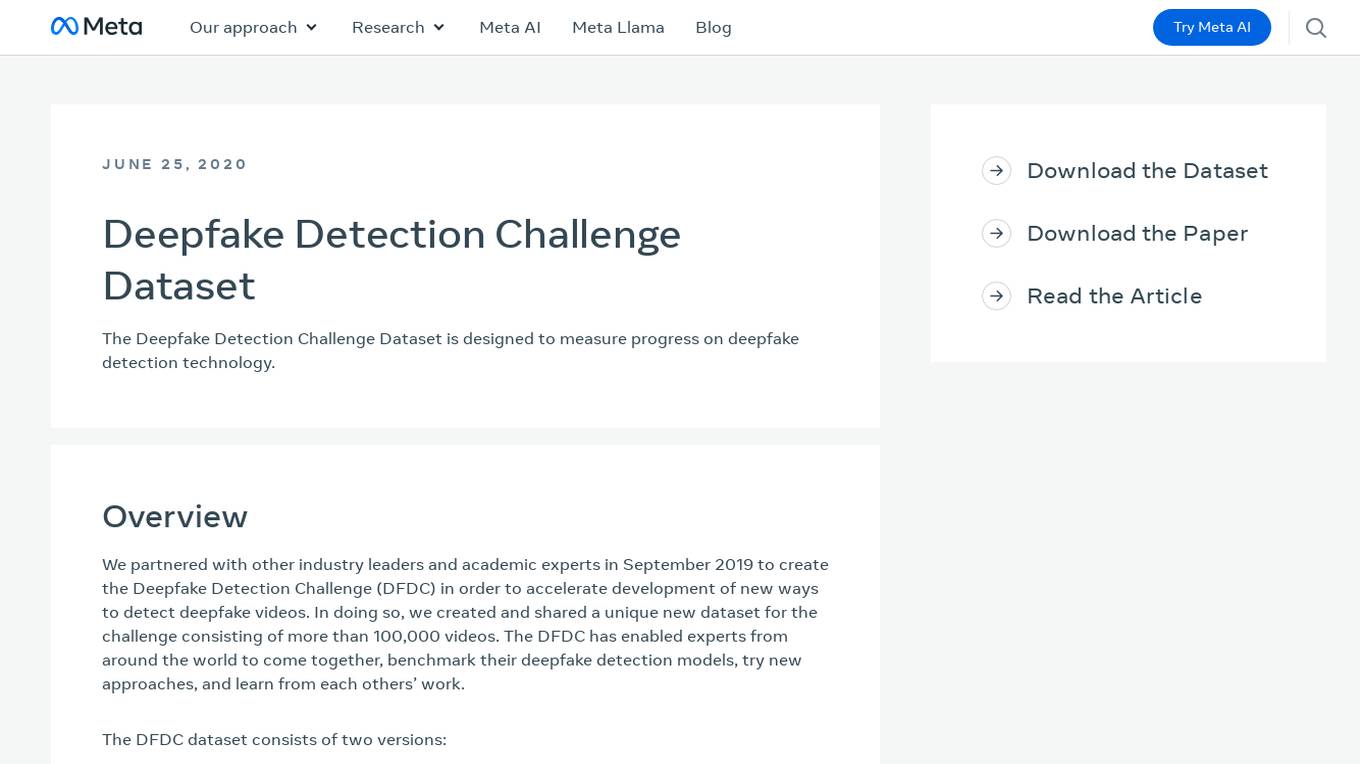
Deepfake Detection Challenge Dataset
The Deepfake Detection Challenge Dataset is a project initiated by Facebook AI to accelerate the development of new ways to detect deepfake videos. The dataset consists of over 100,000 videos and was created in collaboration with industry leaders and academic experts. It includes two versions: a preview dataset with 5k videos and a full dataset with 124k videos, each featuring facial modification algorithms. The dataset was used in a Kaggle competition to create better models for detecting manipulated media. The top-performing models achieved high accuracy on the public dataset but faced challenges when tested against the black box dataset, highlighting the importance of generalization in deepfake detection. The project aims to encourage the research community to continue advancing in detecting harmful manipulated media.
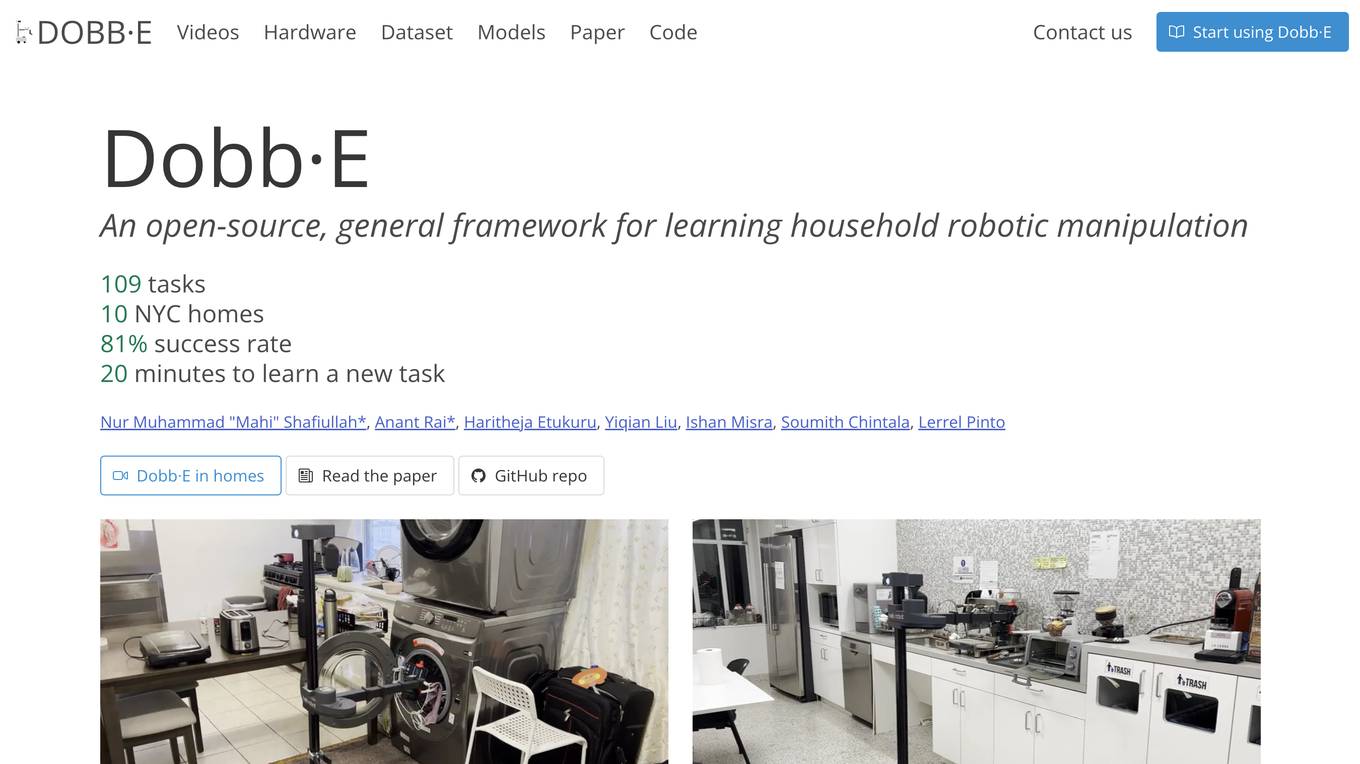
Dobb·E
Dobb·E is an open-source, general framework for learning household robotic manipulation. It aims to create a generalist machine for homes that can adapt and learn from users' needs efficiently. Dobb·E can learn a new task with just five minutes of demonstration, achieving an 81% success rate in various household environments. The project focuses on accelerating research on home robots and making robot assistants a common sight in every home.
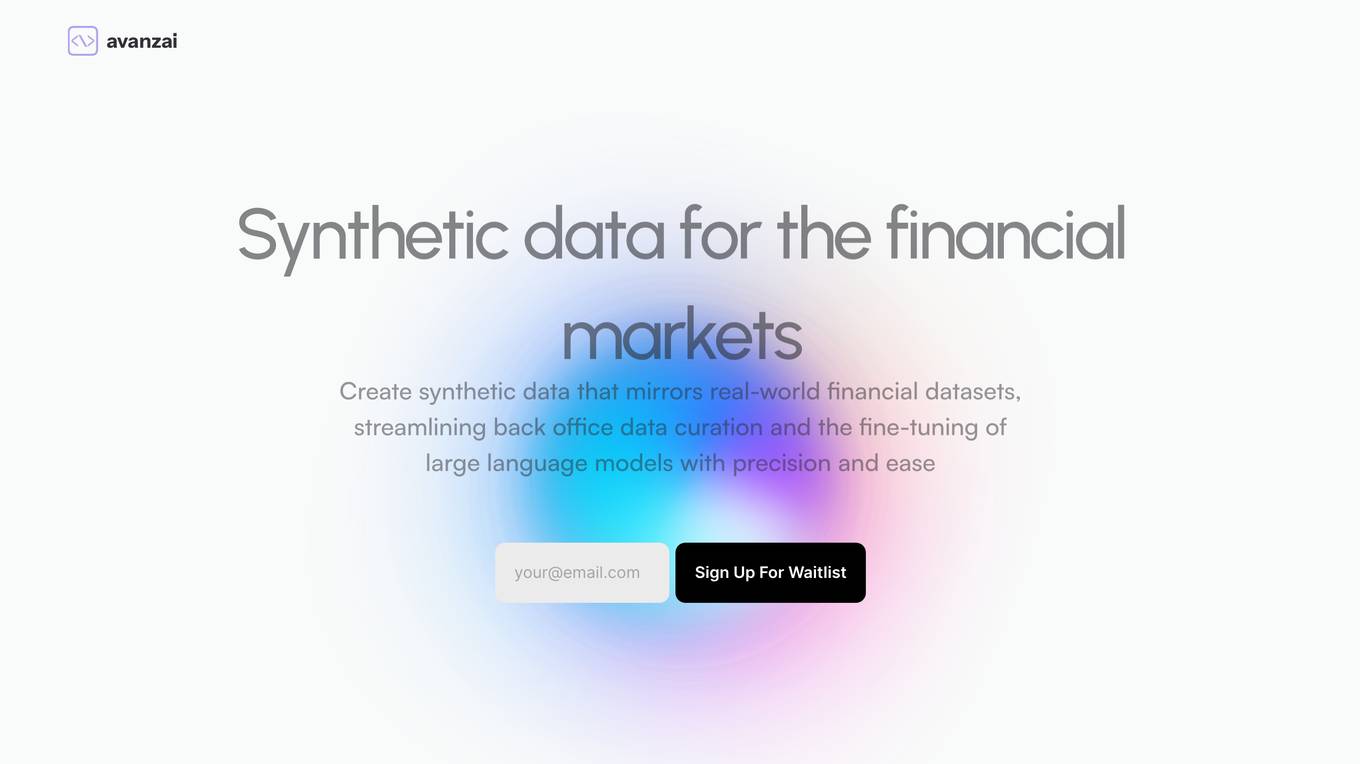
Avanzai
Avanzai is a powerful workflow automation tool designed specifically for financial services. It leverages AI technology to uncover hidden market dynamics, generate interactive charts, and perform complex analysis in plain English. Users can easily build charts that would typically require advanced programming skills in Python or quant tools. Avanzai provides access to macroeconomic data, equity fundamentals, and real-time news, allowing users to customize charts based on their specific needs. The tool accelerates research reports, enhances alternative data analysis, and empowers asset managers to test market hypotheses efficiently.
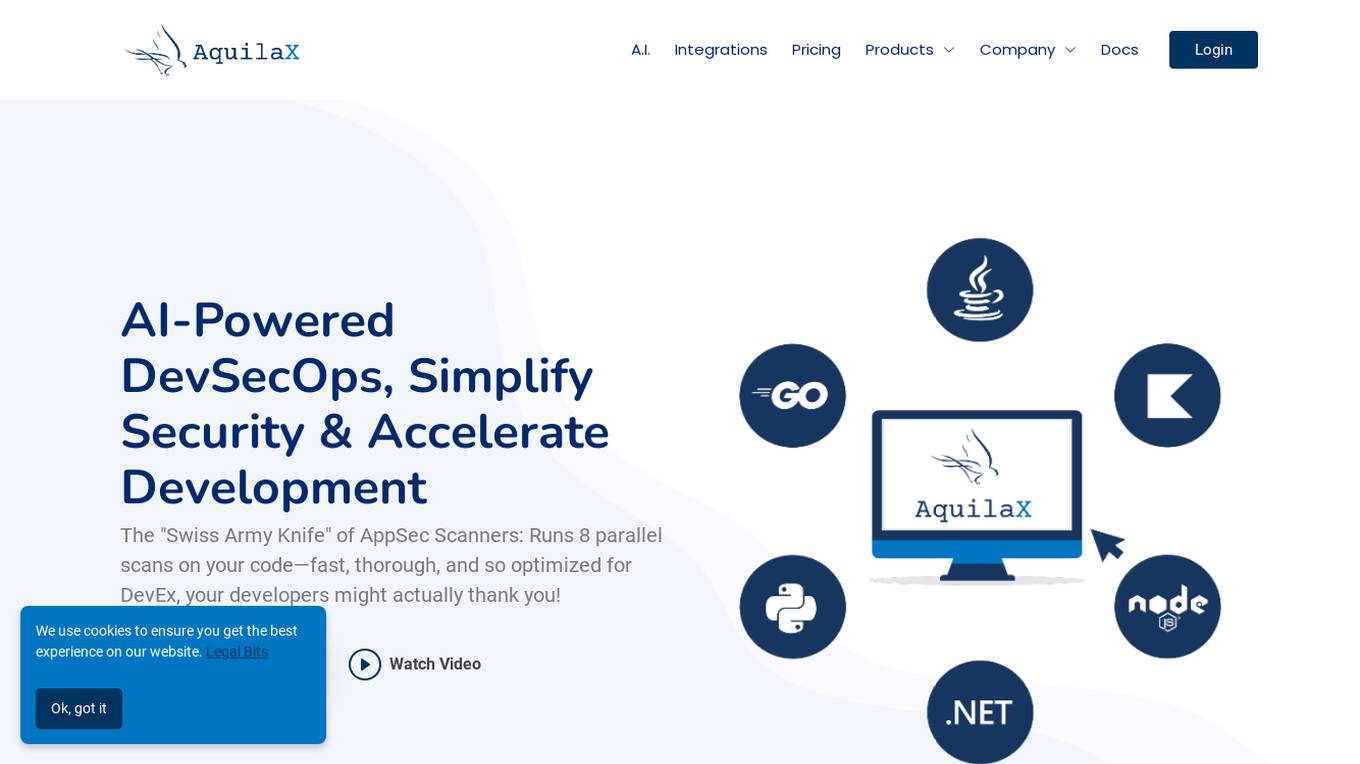
AquilaX
AquilaX is an AI-powered DevSecOps platform that simplifies security and accelerates development processes. It offers a comprehensive suite of security scanning tools, including secret identification, PII scanning, SAST, container scanning, and more. AquilaX is designed to integrate seamlessly into the development workflow, providing fast and accurate results by leveraging AI models trained on extensive datasets. The platform prioritizes developer experience by eliminating noise and false positives, making it a go-to choice for modern Secure-SDLC teams worldwide.
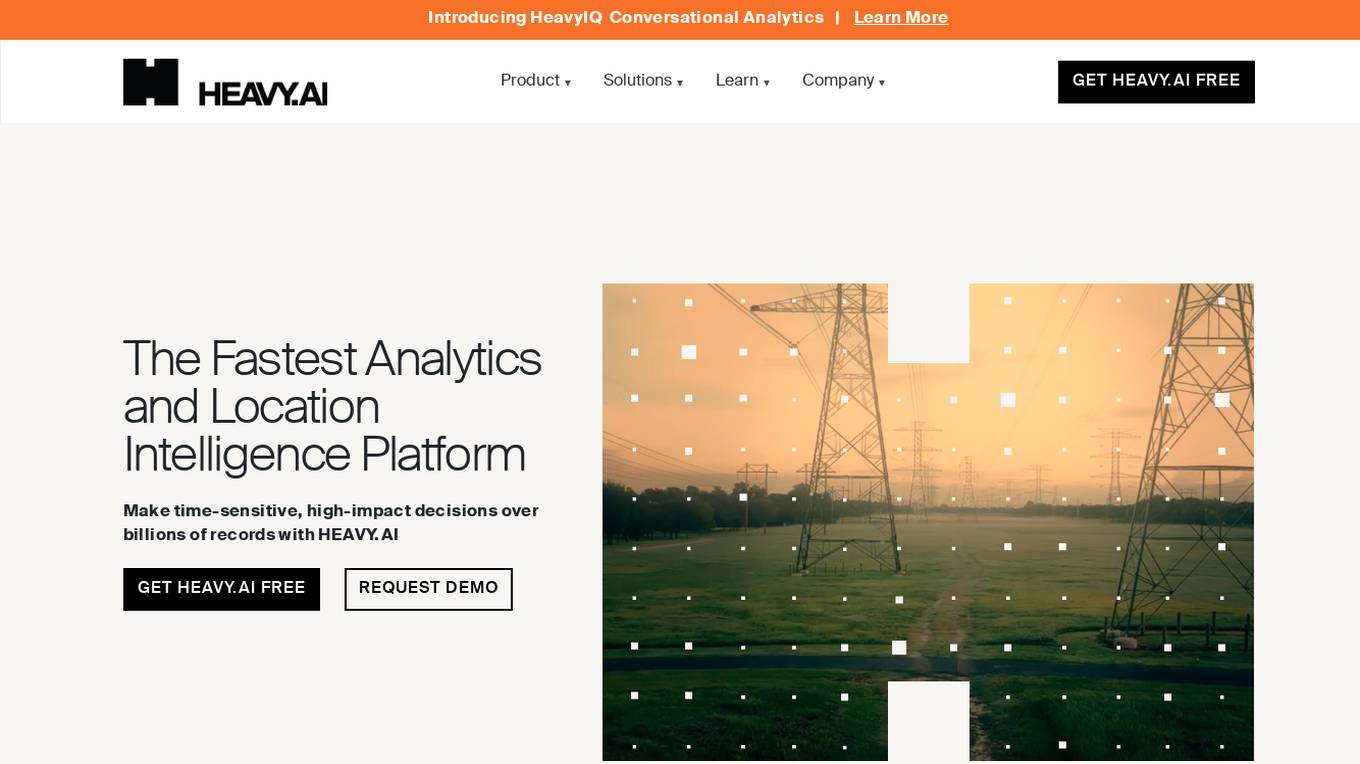
HEAVY.AI
HEAVY.AI is a cutting-edge analytics and location intelligence platform that empowers users to make time-sensitive, high-impact decisions over vast datasets. The platform offers Conversational Analytics, enabling users to ask questions about their data in natural language and view actionable visualizations instantly. With HeavyEco, the platform also supports emergency response efforts by streamlining the management of weather events. HEAVY.AI combines interactive visual analytics, hardware-accelerated SQL, and advanced analytics & data science framework to uncover hidden opportunities and risks within enterprise datasets.
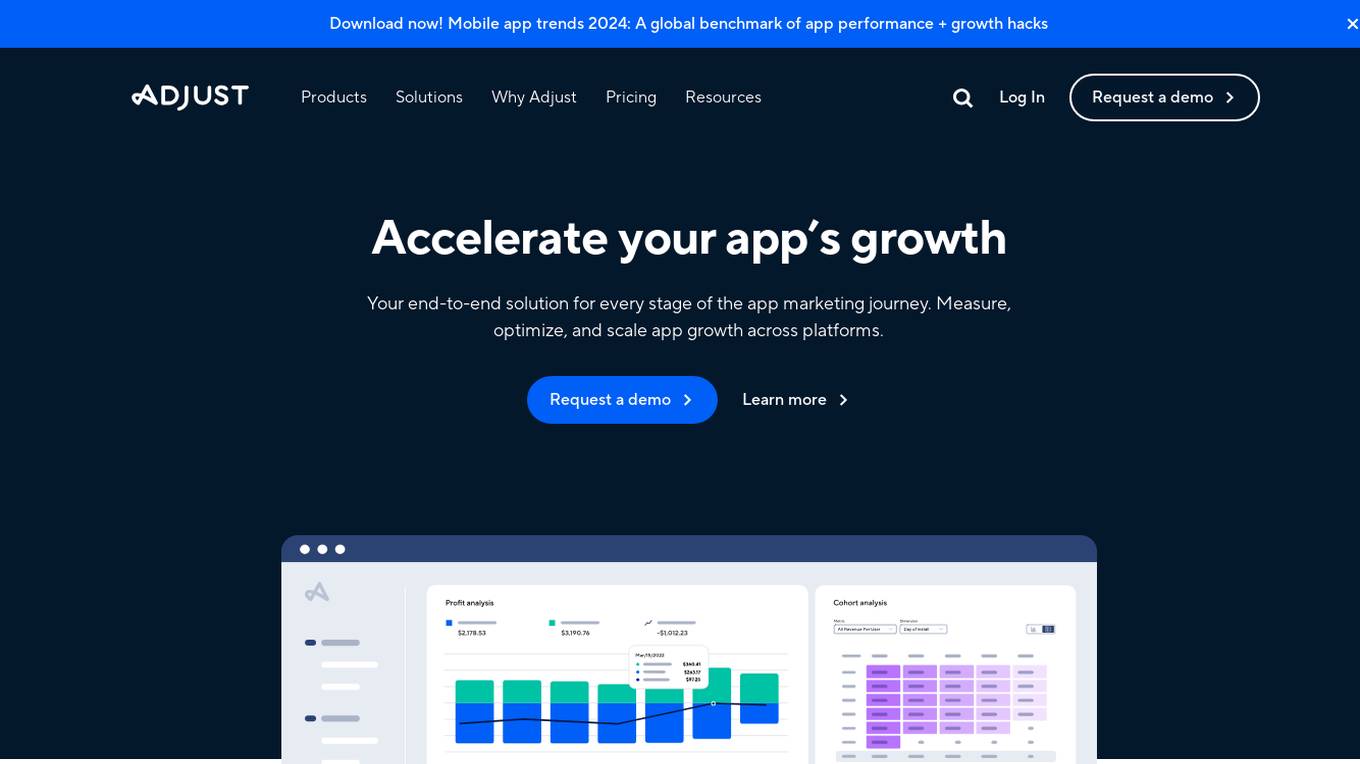
Adjust
Adjust is an AI-driven platform that helps mobile app developers accelerate their app's growth through a comprehensive suite of measurement, analytics, automation, and fraud prevention tools. The platform offers unlimited measurement capabilities across various platforms, powerful analytics and reporting features, AI-driven decision-making recommendations, streamlined operations through automation, and data protection against mobile ad fraud. Adjust also provides solutions for iOS and SKAdNetwork success, CTV and OTT performance enhancement, ROI measurement, fraud prevention, and incrementality analysis. With a focus on privacy and security, Adjust empowers app developers to optimize their marketing strategies and drive tangible growth.
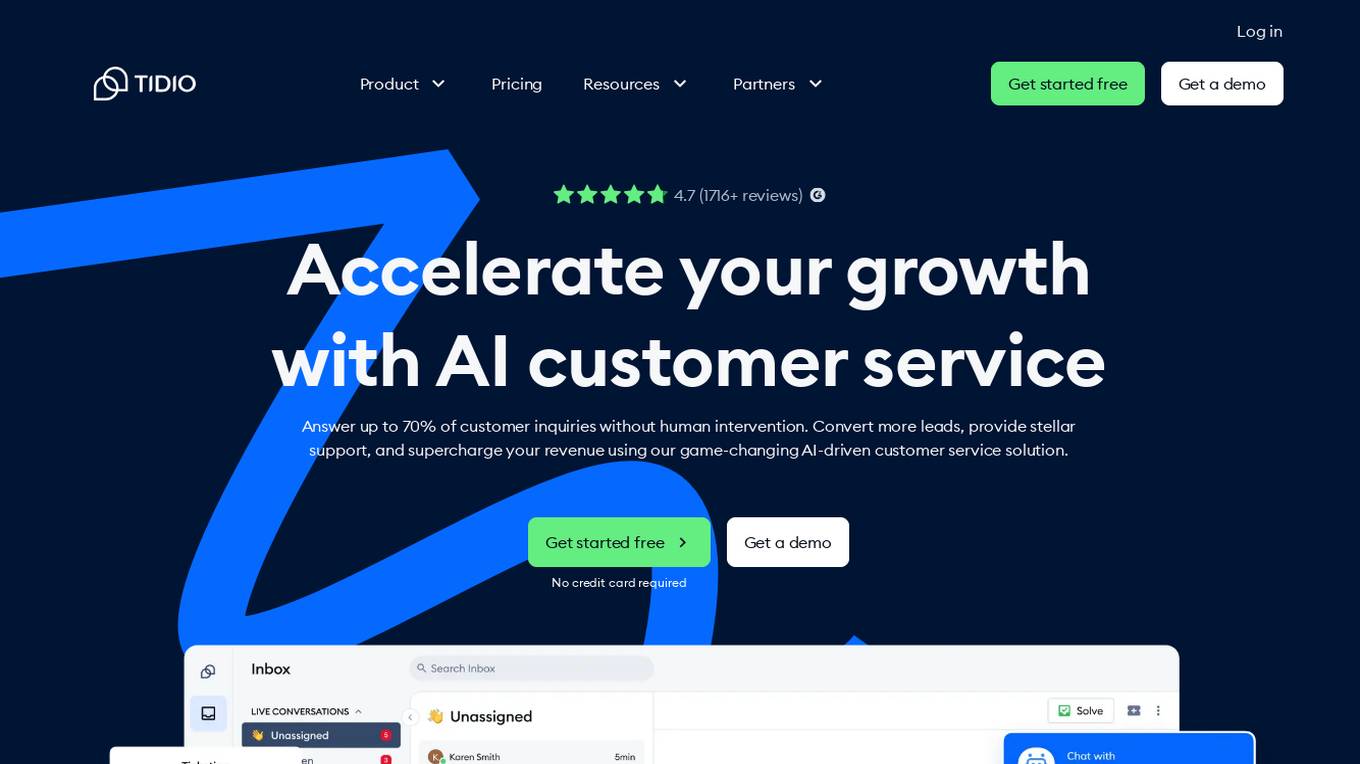
Tidio
Tidio is an AI-powered customer service solution that helps businesses automate support, convert more leads, and increase revenue. With Lyro AI Chatbot, businesses can answer up to 70% of customer inquiries without human intervention, freeing up support agents to focus on high-value requests. Tidio also offers live chat, helpdesk, and automation features to help businesses provide excellent customer support and grow their business.
20 - Open Source AI Tools
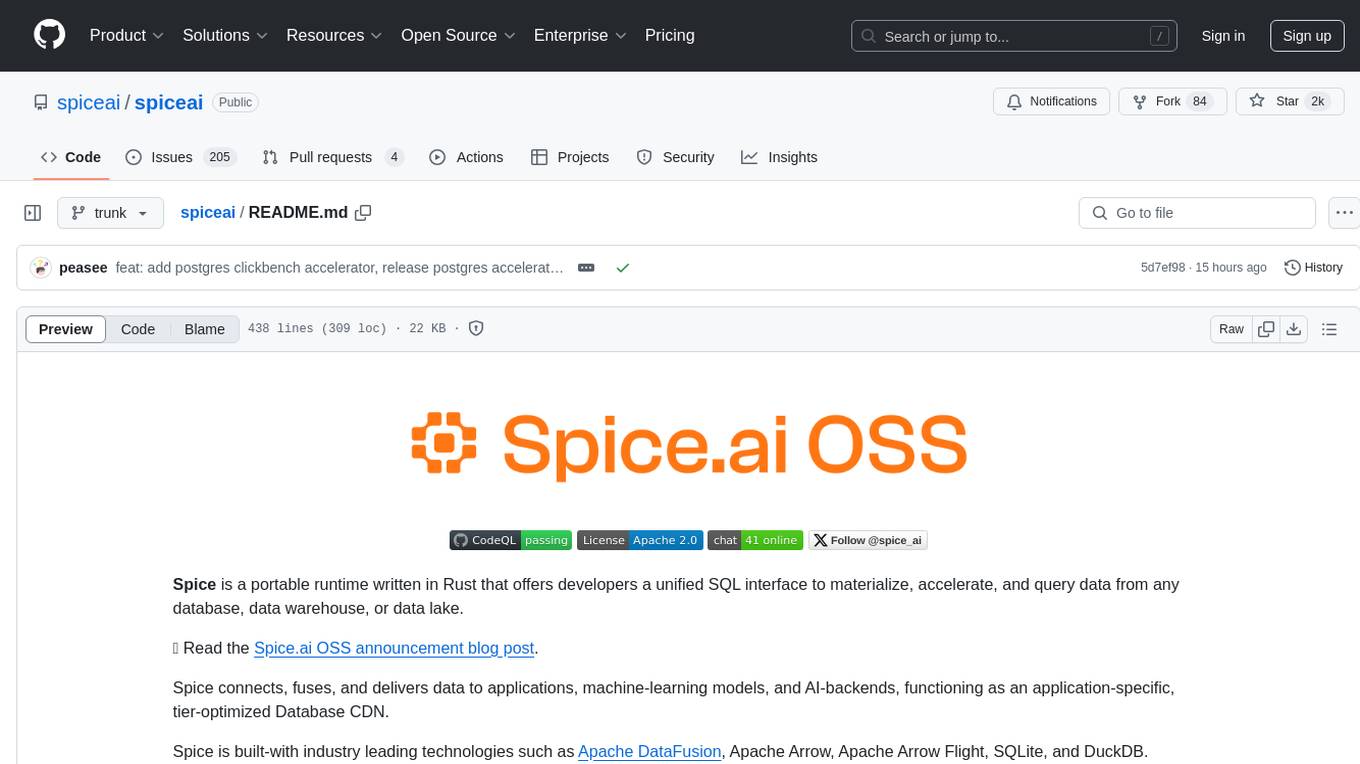
spiceai
Spice is a portable runtime written in Rust that offers developers a unified SQL interface to materialize, accelerate, and query data from any database, data warehouse, or data lake. It connects, fuses, and delivers data to applications, machine-learning models, and AI-backends, functioning as an application-specific, tier-optimized Database CDN. Built with industry-leading technologies such as Apache DataFusion, Apache Arrow, Apache Arrow Flight, SQLite, and DuckDB. Spice makes it fast and easy to query data from one or more sources using SQL, co-locating a managed dataset with applications or machine learning models, and accelerating it with Arrow in-memory, SQLite/DuckDB, or attached PostgreSQL for fast, high-concurrency, low-latency queries.
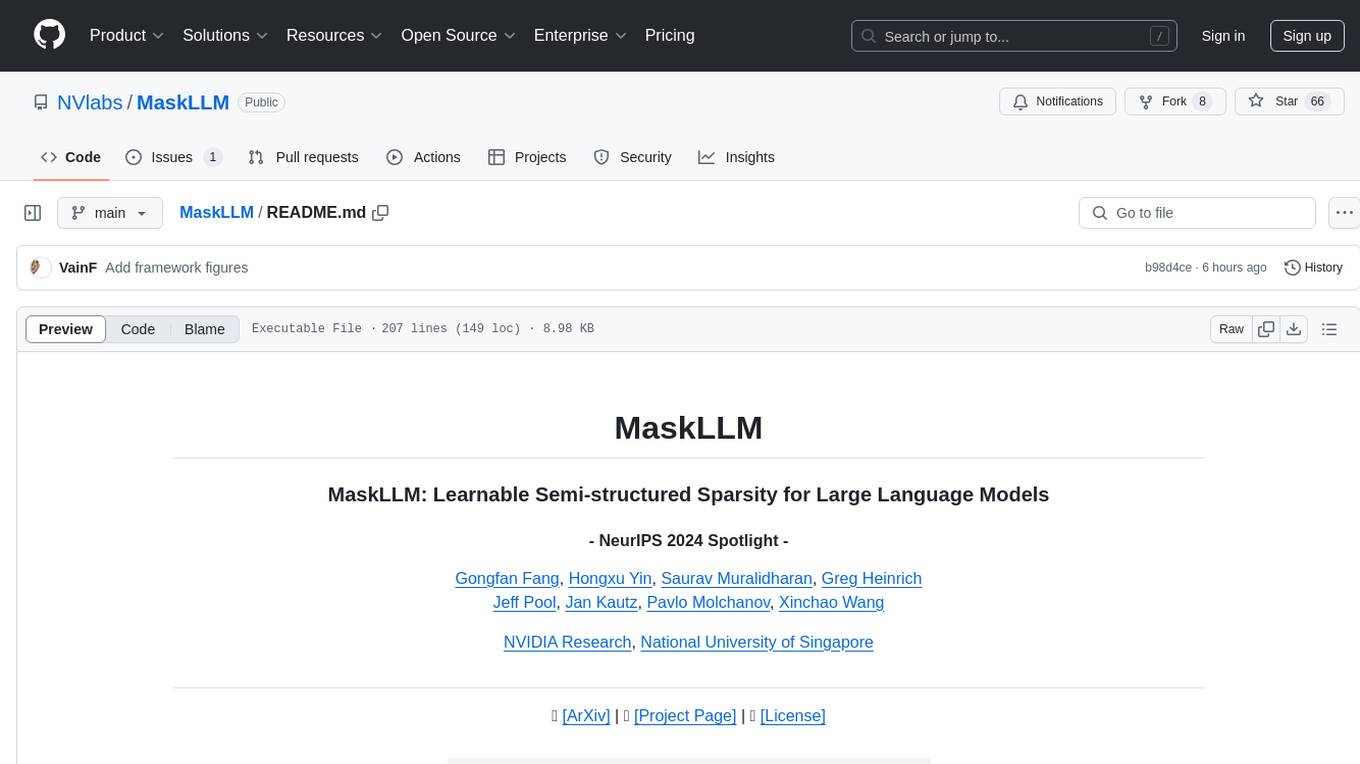
MaskLLM
MaskLLM is a learnable pruning method that establishes Semi-structured Sparsity in Large Language Models (LLMs) to reduce computational overhead during inference. It is scalable and benefits from larger training datasets. The tool provides examples for running MaskLLM with Megatron-LM, preparing LLaMA checkpoints, pre-tokenizing C4 data for Megatron, generating prior masks, training MaskLLM, and evaluating the model. It also includes instructions for exporting sparse models to Huggingface.
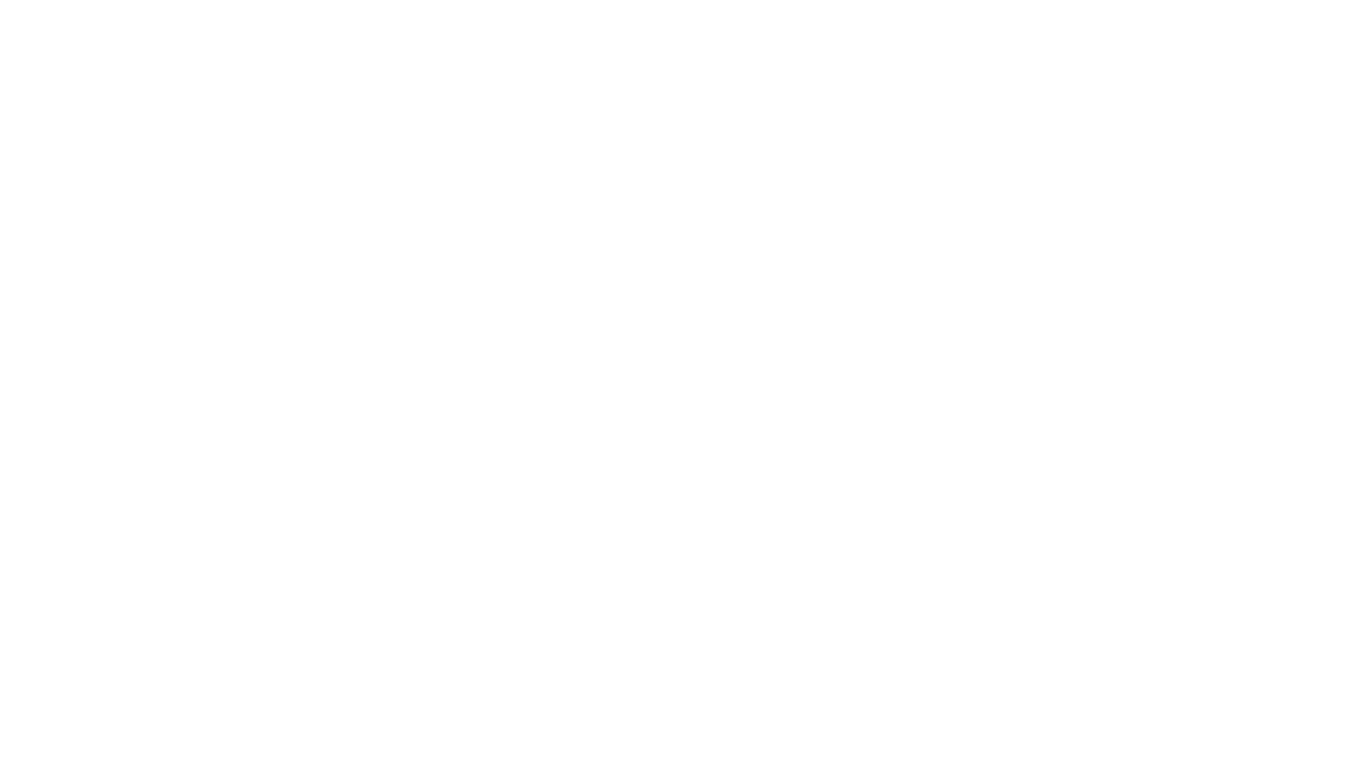
litdata
LitData is a tool designed for blazingly fast, distributed streaming of training data from any cloud storage. It allows users to transform and optimize data in cloud storage environments efficiently and intuitively, supporting various data types like images, text, video, audio, geo-spatial, and multimodal data. LitData integrates smoothly with frameworks such as LitGPT and PyTorch, enabling seamless streaming of data to multiple machines. Key features include multi-GPU/multi-node support, easy data mixing, pause & resume functionality, support for profiling, memory footprint reduction, cache size configuration, and on-prem optimizations. The tool also provides benchmarks for measuring streaming speed and conversion efficiency, along with runnable templates for different data types. LitData enables infinite cloud data processing by utilizing the Lightning.ai platform to scale data processing with optimized machines.
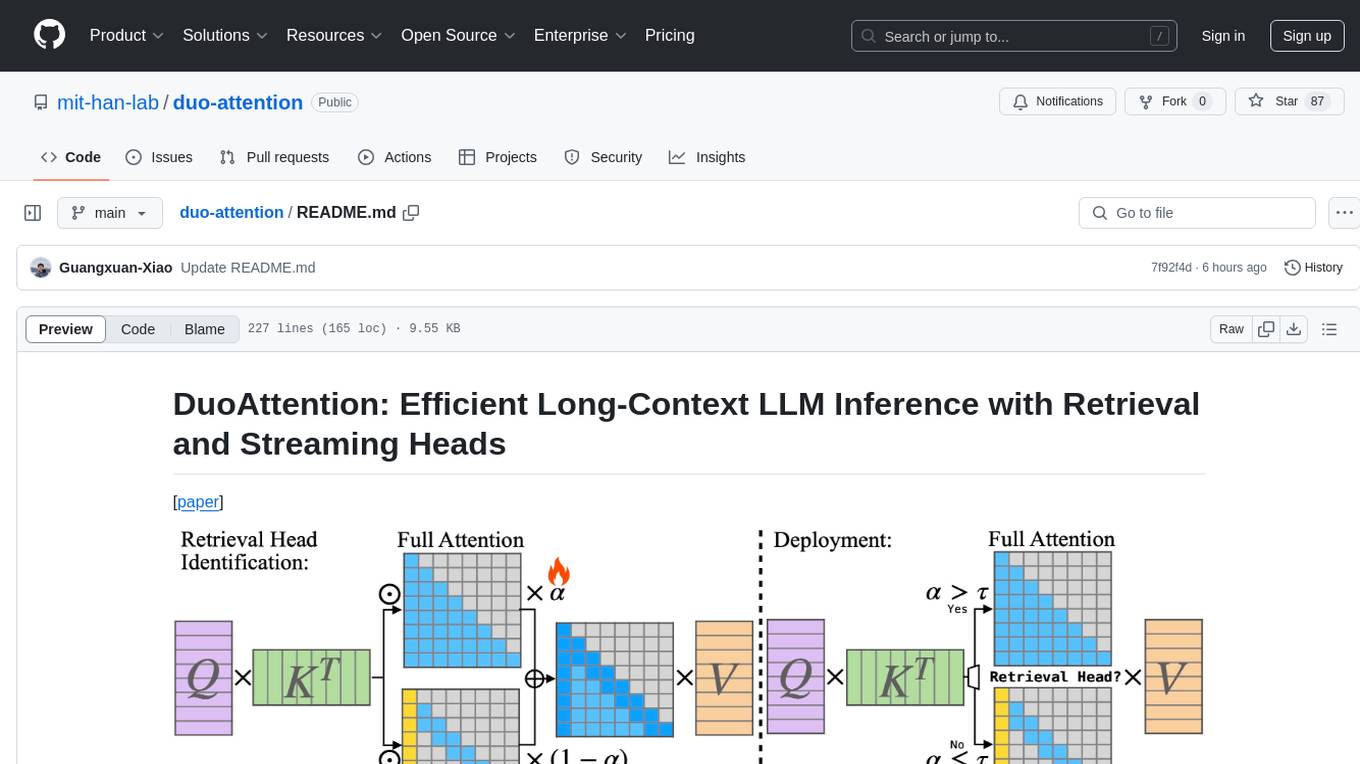
duo-attention
DuoAttention is a framework designed to optimize long-context large language models (LLMs) by reducing memory and latency during inference without compromising their long-context abilities. It introduces a concept of Retrieval Heads and Streaming Heads to efficiently manage attention across tokens. By applying a full Key and Value (KV) cache to retrieval heads and a lightweight, constant-length KV cache to streaming heads, DuoAttention achieves significant reductions in memory usage and decoding time for LLMs. The framework uses an optimization-based algorithm with synthetic data to accurately identify retrieval heads, enabling efficient inference with minimal accuracy loss compared to full attention. DuoAttention also supports quantization techniques for further memory optimization, allowing for decoding of up to 3.3 million tokens on a single GPU.
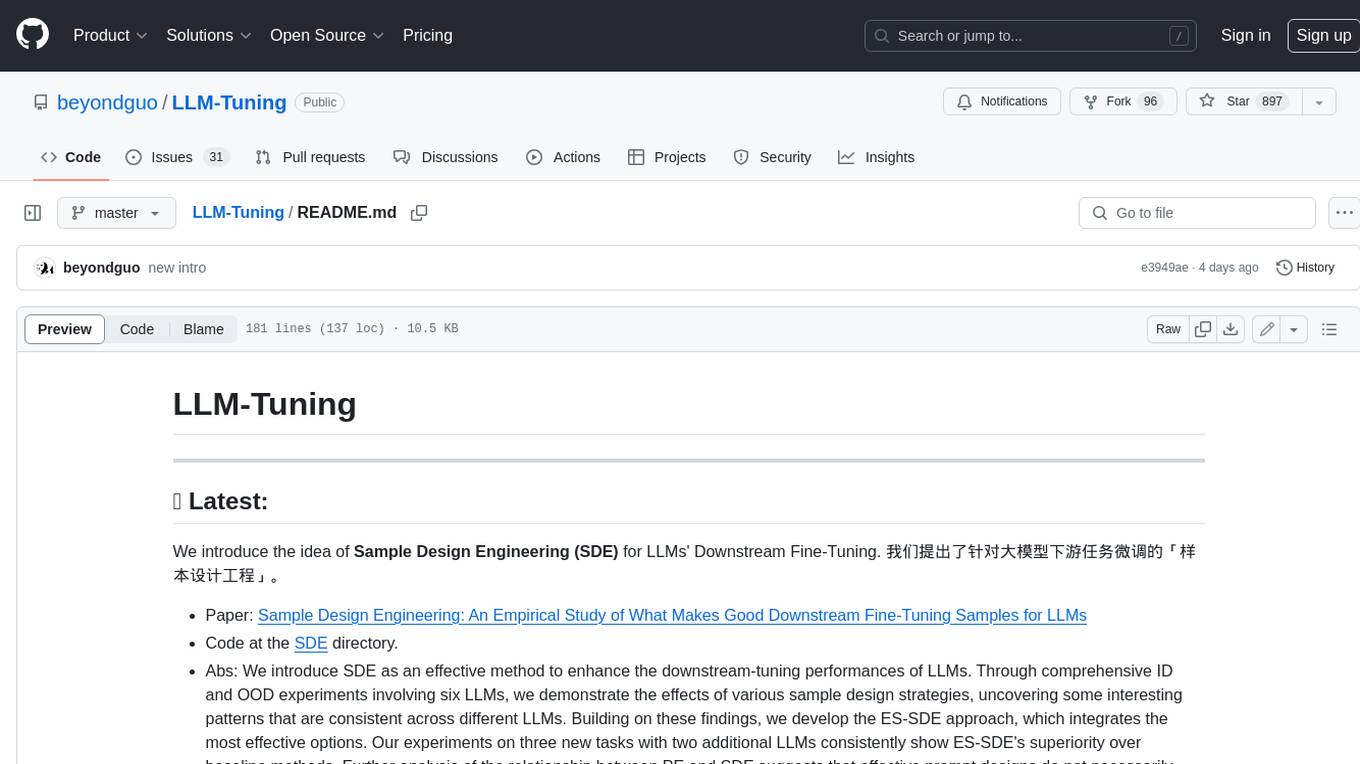
LLM-Tuning
LLM-Tuning is a collection of tools and resources for fine-tuning large language models (LLMs). It includes a library of pre-trained LoRA models, a set of tutorials and examples, and a community forum for discussion and support. LLM-Tuning makes it easy to fine-tune LLMs for a variety of tasks, including text classification, question answering, and dialogue generation. With LLM-Tuning, you can quickly and easily improve the performance of your LLMs on downstream tasks.
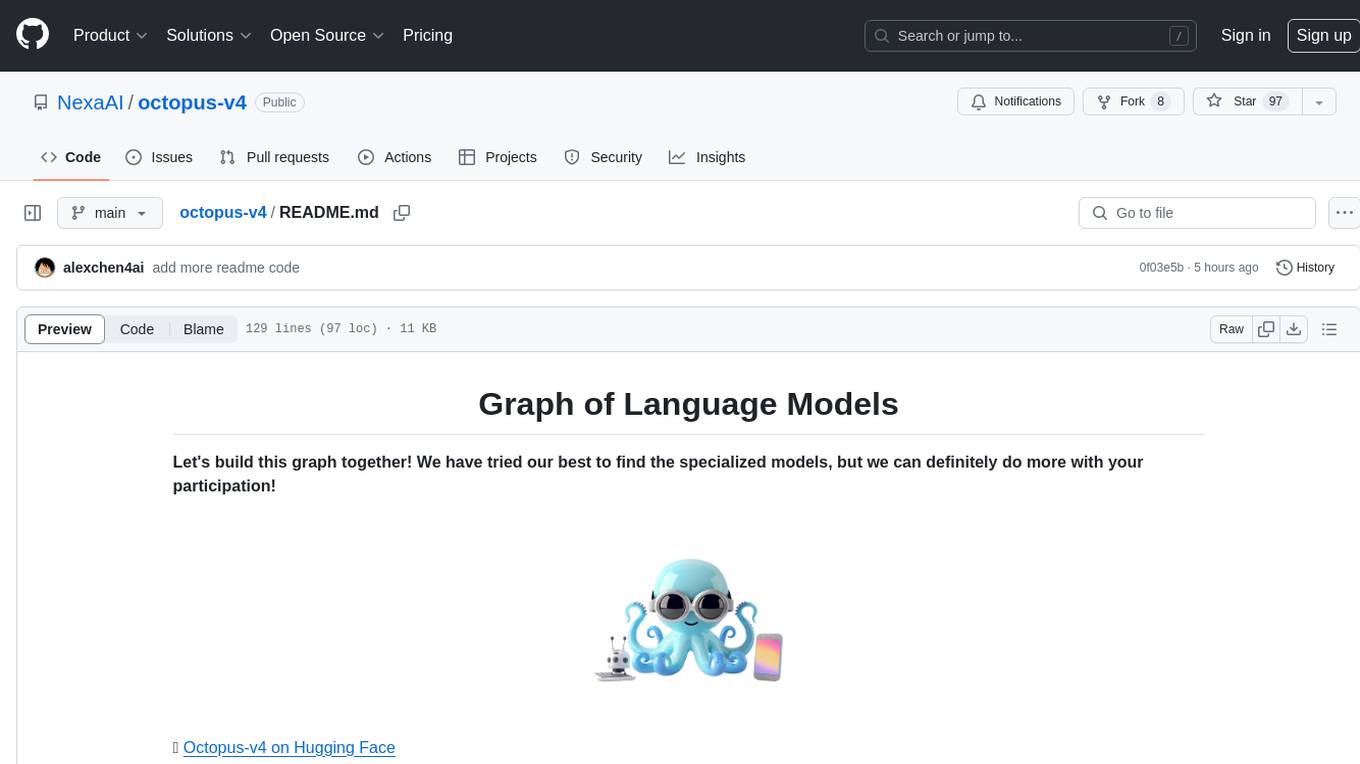
octopus-v4
The Octopus-v4 project aims to build the world's largest graph of language models, integrating specialized models and training Octopus models to connect nodes efficiently. The project focuses on identifying, training, and connecting specialized models. The repository includes scripts for running the Octopus v4 model, methods for managing the graph, training code for specialized models, and inference code. Environment setup instructions are provided for Linux with NVIDIA GPU. The Octopus v4 model helps users find suitable models for tasks and reformats queries for effective processing. The project leverages Language Large Models for various domains and provides benchmark results. Users are encouraged to train and add specialized models following recommended procedures.
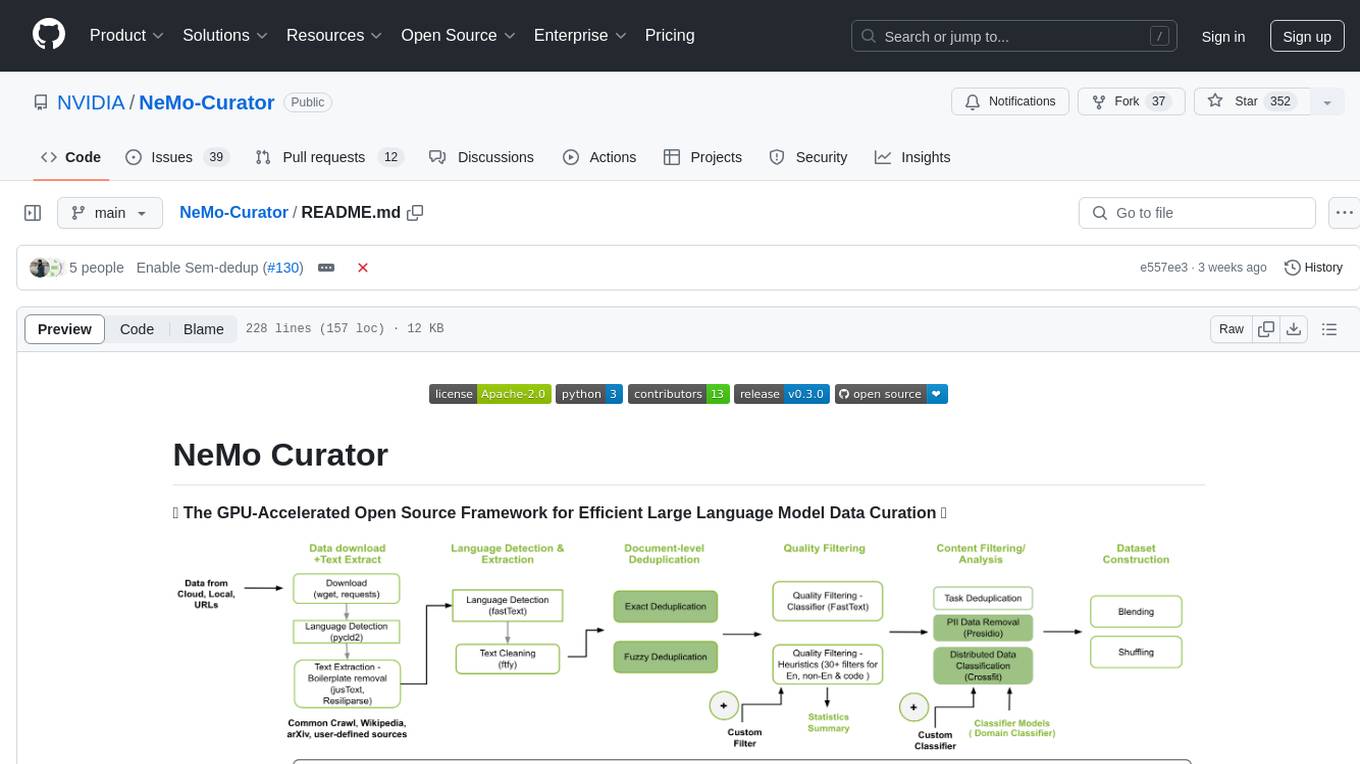
NeMo-Curator
NeMo Curator is a GPU-accelerated open-source framework designed for efficient large language model data curation. It provides scalable dataset preparation for tasks like foundation model pretraining, domain-adaptive pretraining, supervised fine-tuning, and parameter-efficient fine-tuning. The library leverages GPUs with Dask and RAPIDS to accelerate data curation, offering customizable and modular interfaces for pipeline expansion and model convergence. Key features include data download, text extraction, quality filtering, deduplication, downstream-task decontamination, distributed data classification, and PII redaction. NeMo Curator is suitable for curating high-quality datasets for large language model training.
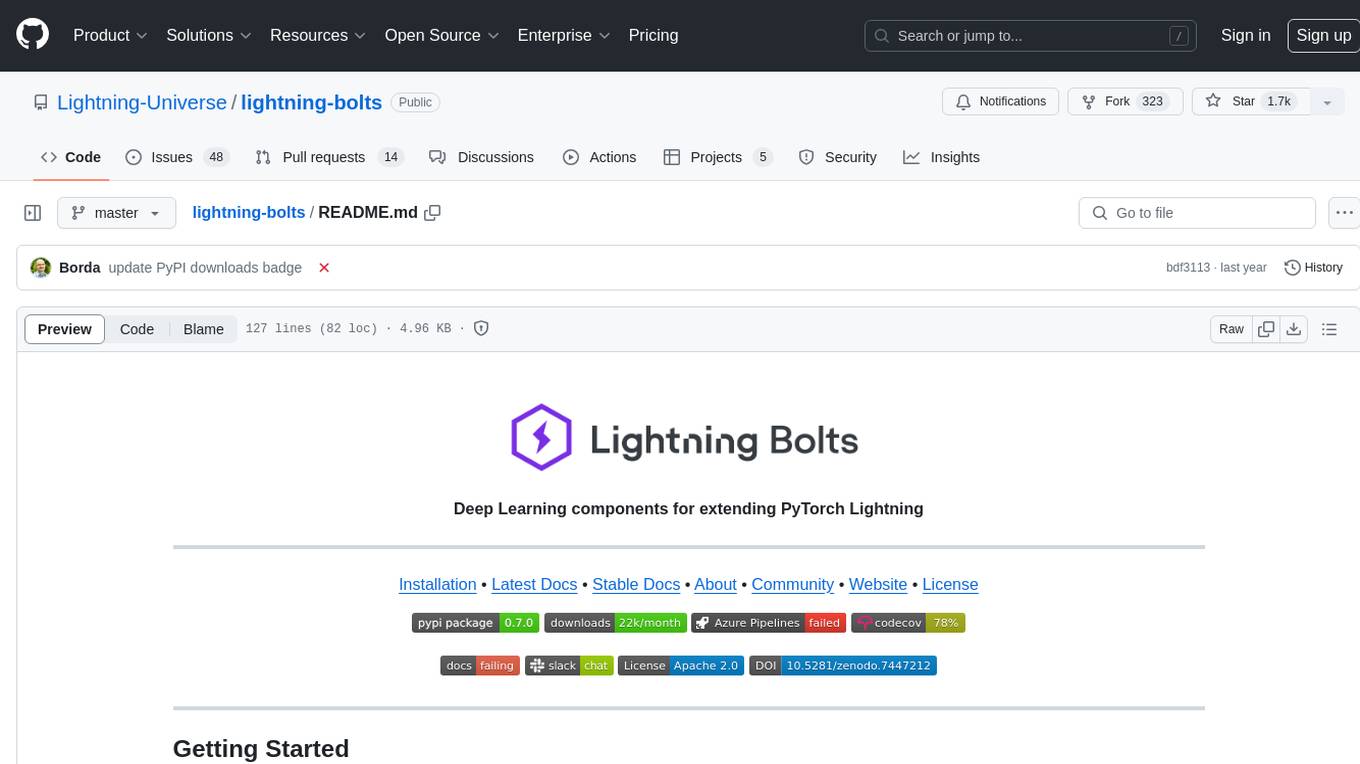
lightning-bolts
Bolts package provides a variety of components to extend PyTorch Lightning, such as callbacks & datasets, for applied research and production. Users can accelerate Lightning training with the Torch ORT Callback to optimize ONNX graph for faster training & inference. Additionally, users can introduce sparsity with the SparseMLCallback to accelerate inference by leveraging the DeepSparse engine. Specific research implementations are encouraged, with contributions that help train SSL models and integrate with Lightning Flash for state-of-the-art models in applied research.
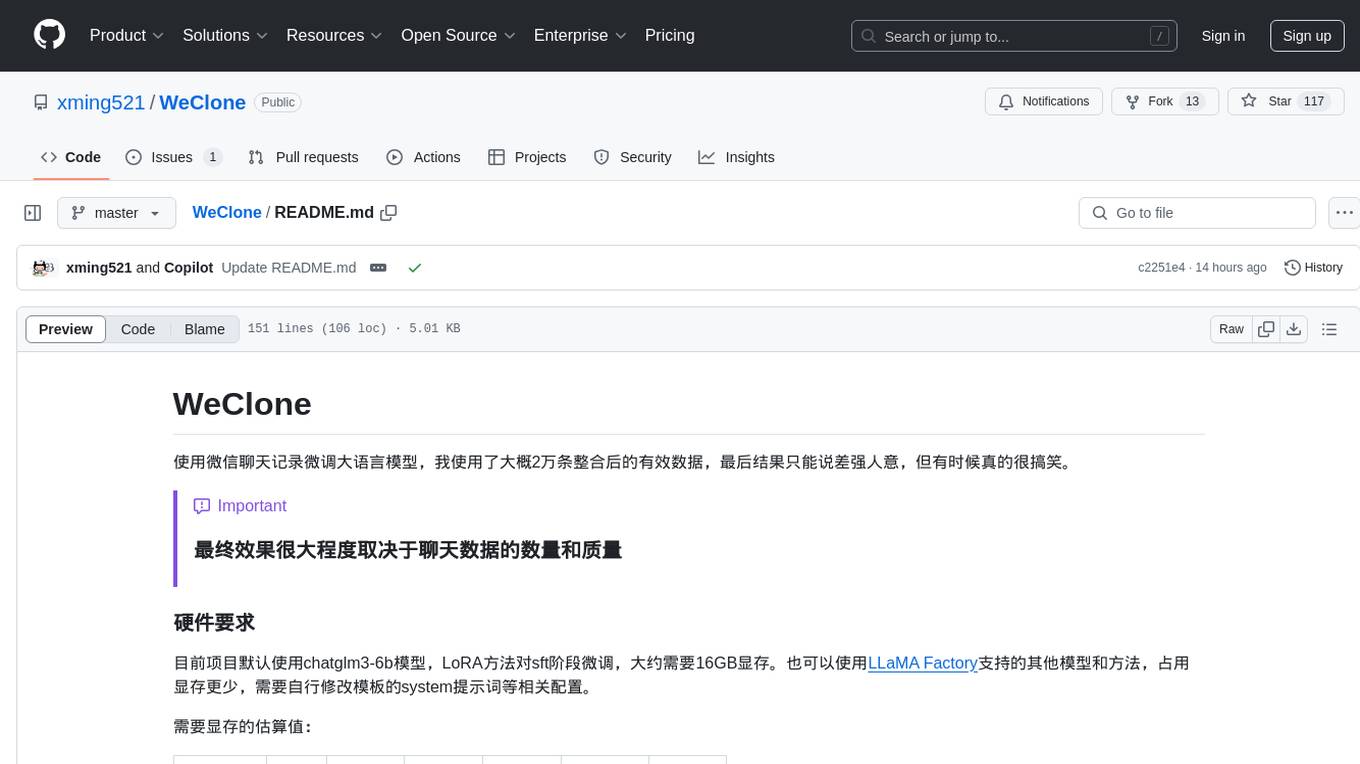
WeClone
WeClone is a tool that fine-tunes large language models using WeChat chat records. It utilizes approximately 20,000 integrated and effective data points, resulting in somewhat satisfactory outcomes that are occasionally humorous. The tool's effectiveness largely depends on the quantity and quality of the chat data provided. It requires a minimum of 16GB of GPU memory for training using the default chatglm3-6b model with LoRA method. Users can also opt for other models and methods supported by LLAMA Factory, which consume less memory. The tool has specific hardware and software requirements, including Python, Torch, Transformers, Datasets, Accelerate, and other optional packages like CUDA and Deepspeed. The tool facilitates environment setup, data preparation, data preprocessing, model downloading, parameter configuration, model fine-tuning, and inference through a browser demo or API service. Additionally, it offers the ability to deploy a WeChat chatbot, although users should be cautious due to the risk of account suspension by WeChat.
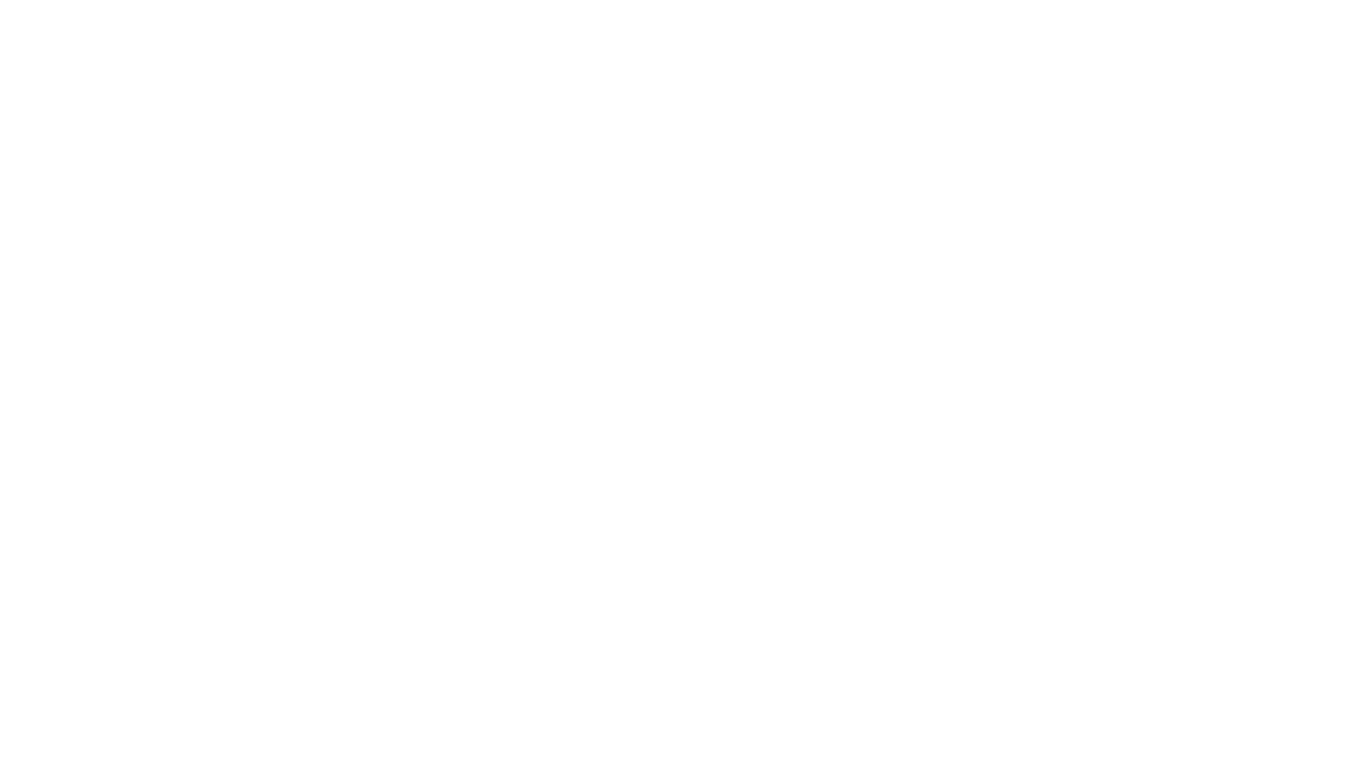
intel-extension-for-transformers
Intel® Extension for Transformers is an innovative toolkit designed to accelerate GenAI/LLM everywhere with the optimal performance of Transformer-based models on various Intel platforms, including Intel Gaudi2, Intel CPU, and Intel GPU. The toolkit provides the below key features and examples: * Seamless user experience of model compressions on Transformer-based models by extending [Hugging Face transformers](https://github.com/huggingface/transformers) APIs and leveraging [Intel® Neural Compressor](https://github.com/intel/neural-compressor) * Advanced software optimizations and unique compression-aware runtime (released with NeurIPS 2022's paper [Fast Distilbert on CPUs](https://arxiv.org/abs/2211.07715) and [QuaLA-MiniLM: a Quantized Length Adaptive MiniLM](https://arxiv.org/abs/2210.17114), and NeurIPS 2021's paper [Prune Once for All: Sparse Pre-Trained Language Models](https://arxiv.org/abs/2111.05754)) * Optimized Transformer-based model packages such as [Stable Diffusion](examples/huggingface/pytorch/text-to-image/deployment/stable_diffusion), [GPT-J-6B](examples/huggingface/pytorch/text-generation/deployment), [GPT-NEOX](examples/huggingface/pytorch/language-modeling/quantization#2-validated-model-list), [BLOOM-176B](examples/huggingface/pytorch/language-modeling/inference#BLOOM-176B), [T5](examples/huggingface/pytorch/summarization/quantization#2-validated-model-list), [Flan-T5](examples/huggingface/pytorch/summarization/quantization#2-validated-model-list), and end-to-end workflows such as [SetFit-based text classification](docs/tutorials/pytorch/text-classification/SetFit_model_compression_AGNews.ipynb) and [document level sentiment analysis (DLSA)](workflows/dlsa) * [NeuralChat](intel_extension_for_transformers/neural_chat), a customizable chatbot framework to create your own chatbot within minutes by leveraging a rich set of [plugins](https://github.com/intel/intel-extension-for-transformers/blob/main/intel_extension_for_transformers/neural_chat/docs/advanced_features.md) such as [Knowledge Retrieval](./intel_extension_for_transformers/neural_chat/pipeline/plugins/retrieval/README.md), [Speech Interaction](./intel_extension_for_transformers/neural_chat/pipeline/plugins/audio/README.md), [Query Caching](./intel_extension_for_transformers/neural_chat/pipeline/plugins/caching/README.md), and [Security Guardrail](./intel_extension_for_transformers/neural_chat/pipeline/plugins/security/README.md). This framework supports Intel Gaudi2/CPU/GPU. * [Inference](https://github.com/intel/neural-speed/tree/main) of Large Language Model (LLM) in pure C/C++ with weight-only quantization kernels for Intel CPU and Intel GPU (TBD), supporting [GPT-NEOX](https://github.com/intel/neural-speed/tree/main/neural_speed/models/gptneox), [LLAMA](https://github.com/intel/neural-speed/tree/main/neural_speed/models/llama), [MPT](https://github.com/intel/neural-speed/tree/main/neural_speed/models/mpt), [FALCON](https://github.com/intel/neural-speed/tree/main/neural_speed/models/falcon), [BLOOM-7B](https://github.com/intel/neural-speed/tree/main/neural_speed/models/bloom), [OPT](https://github.com/intel/neural-speed/tree/main/neural_speed/models/opt), [ChatGLM2-6B](https://github.com/intel/neural-speed/tree/main/neural_speed/models/chatglm), [GPT-J-6B](https://github.com/intel/neural-speed/tree/main/neural_speed/models/gptj), and [Dolly-v2-3B](https://github.com/intel/neural-speed/tree/main/neural_speed/models/gptneox). Support AMX, VNNI, AVX512F and AVX2 instruction set. We've boosted the performance of Intel CPUs, with a particular focus on the 4th generation Intel Xeon Scalable processor, codenamed [Sapphire Rapids](https://www.intel.com/content/www/us/en/products/docs/processors/xeon-accelerated/4th-gen-xeon-scalable-processors.html).
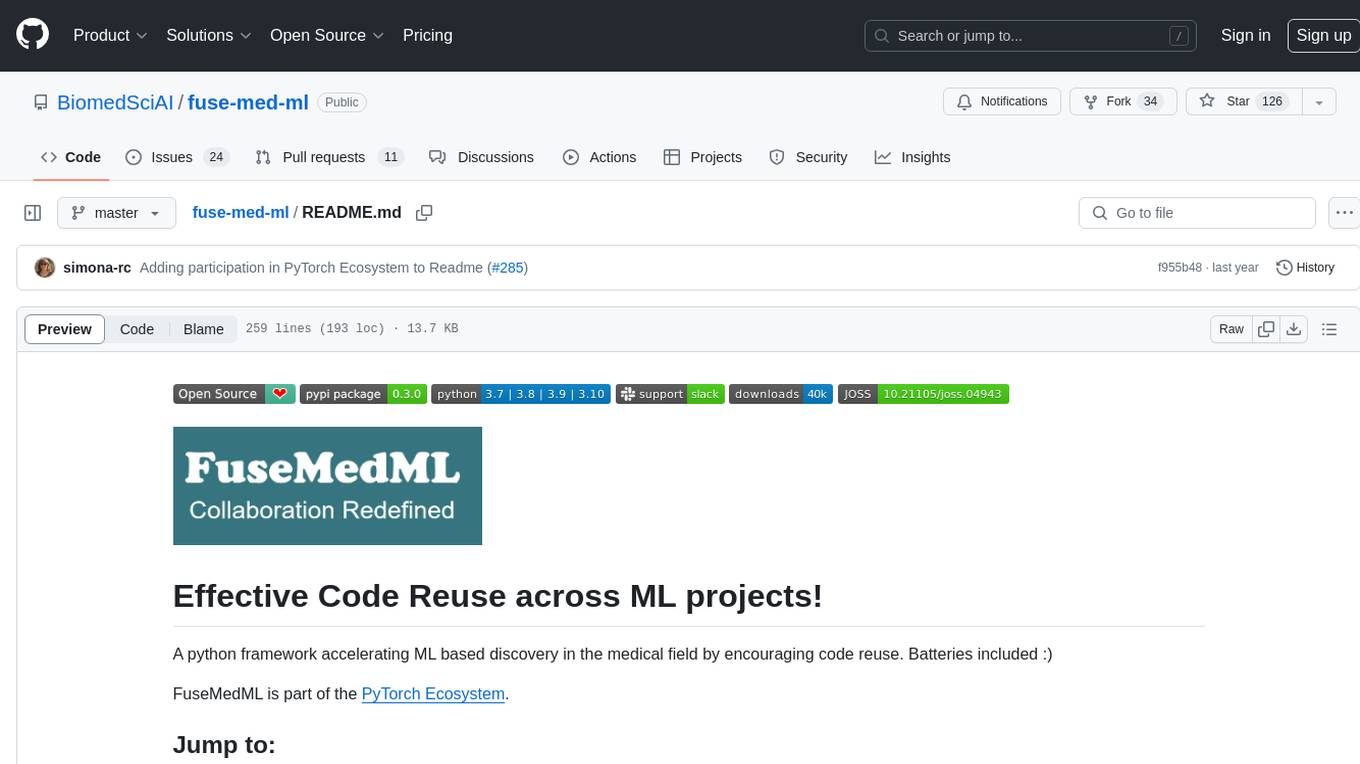
fuse-med-ml
FuseMedML is a Python framework designed to accelerate machine learning-based discovery in the medical field by promoting code reuse. It provides a flexible design concept where data is stored in a nested dictionary, allowing easy handling of multi-modality information. The framework includes components for creating custom models, loss functions, metrics, and data processing operators. Additionally, FuseMedML offers 'batteries included' key components such as fuse.data for data processing, fuse.eval for model evaluation, and fuse.dl for reusable deep learning components. It supports PyTorch and PyTorch Lightning libraries and encourages the creation of domain extensions for specific medical domains.
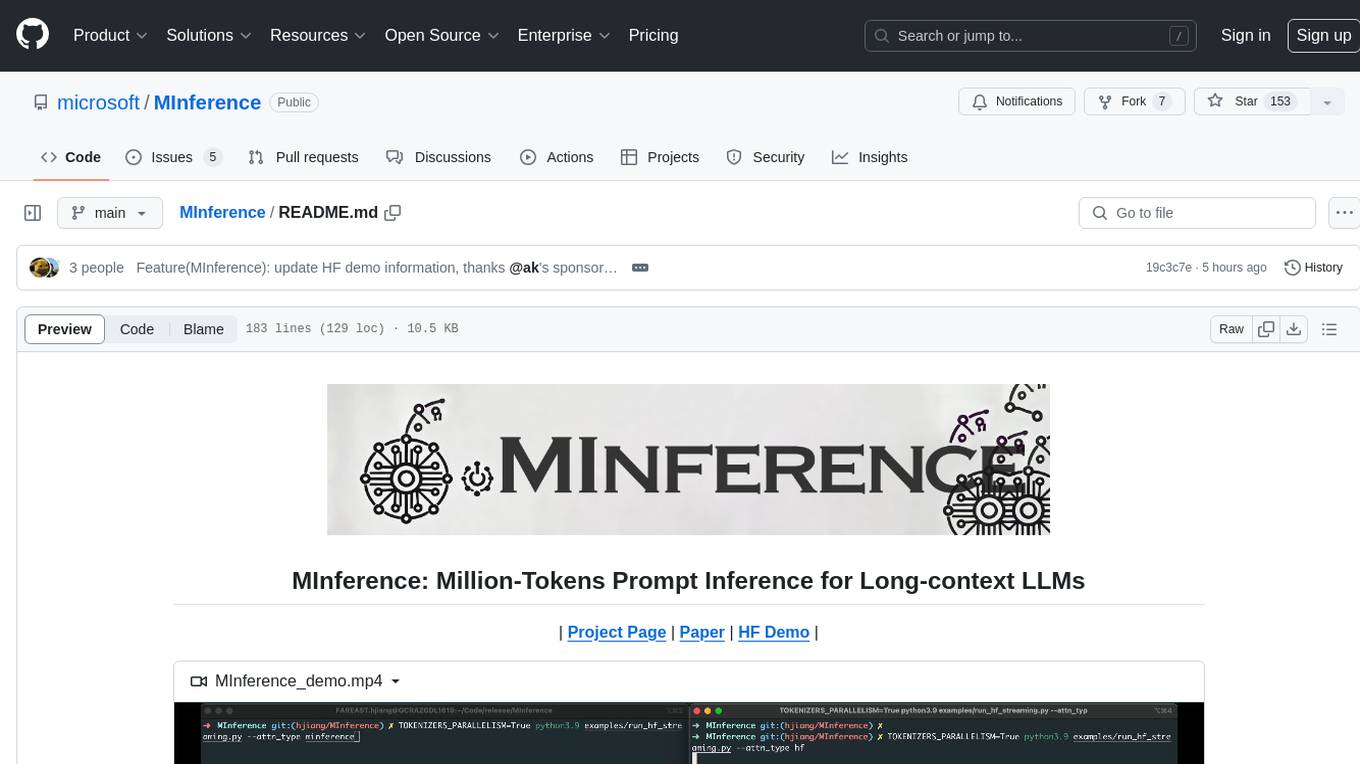
MInference
MInference is a tool designed to accelerate pre-filling for long-context Language Models (LLMs) by leveraging dynamic sparse attention. It achieves up to a 10x speedup for pre-filling on an A100 while maintaining accuracy. The tool supports various decoding LLMs, including LLaMA-style models and Phi models, and provides custom kernels for attention computation. MInference is useful for researchers and developers working with large-scale language models who aim to improve efficiency without compromising accuracy.
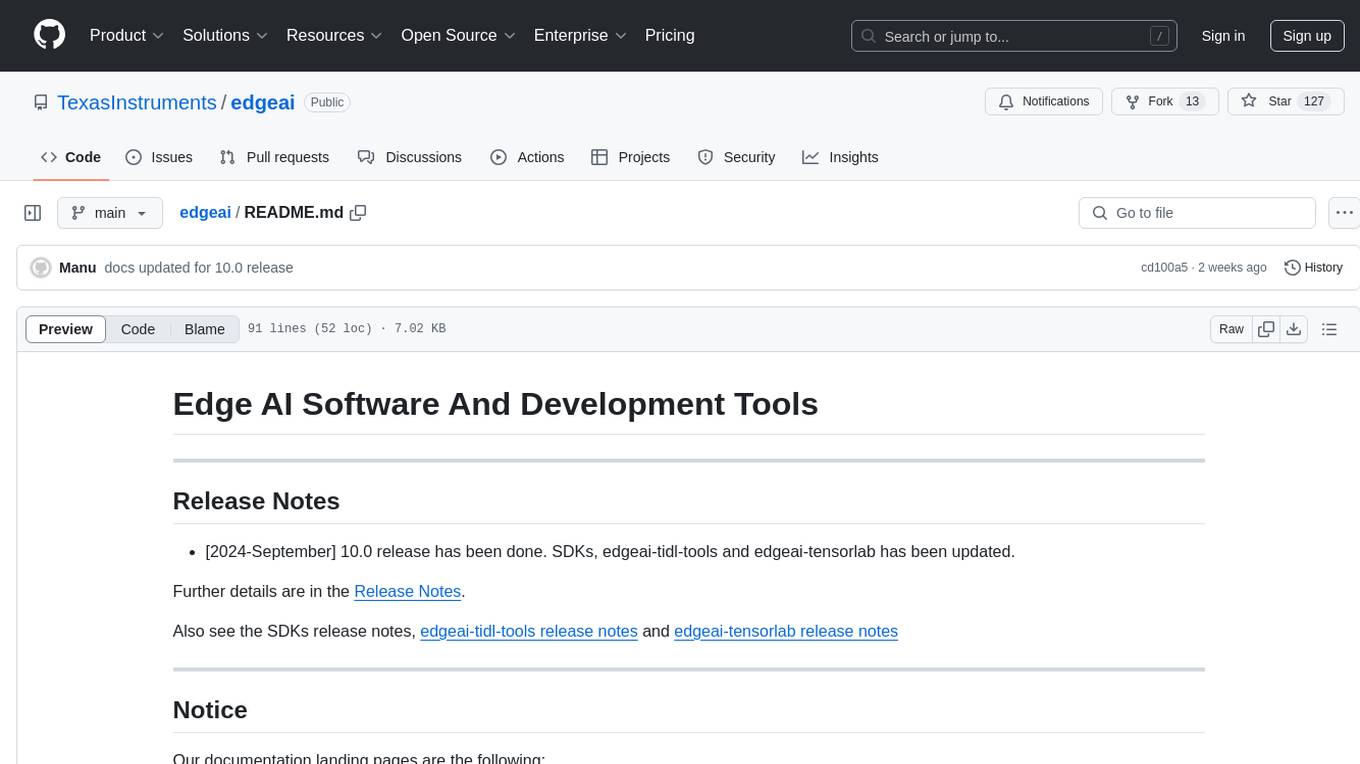
edgeai
Embedded inference of Deep Learning models is quite challenging due to high compute requirements. TI’s Edge AI software product helps optimize and accelerate inference on TI’s embedded devices. It supports heterogeneous execution of DNNs across cortex-A based MPUs, TI’s latest generation C7x DSP, and DNN accelerator (MMA). The solution simplifies the product life cycle of DNN development and deployment by providing a rich set of tools and optimized libraries.
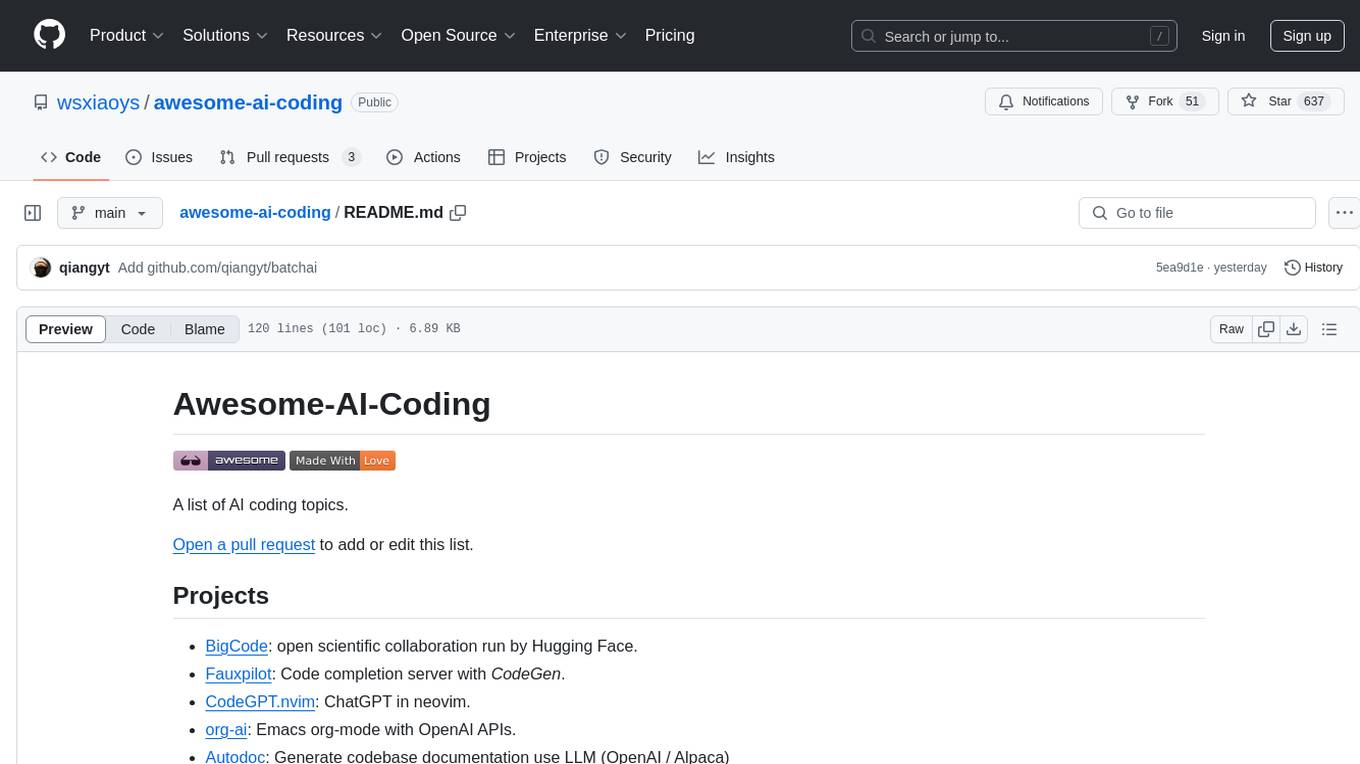
awesome-ai-coding
Awesome-AI-Coding is a curated list of AI coding topics, projects, datasets, LLM models, embedding models, papers, blogs, products, startups, and peer awesome lists related to artificial intelligence in coding. It includes tools for code completion, code generation, code documentation, and code search, as well as AI models and techniques for improving developer productivity. The repository also features information on various AI-powered developer tools, copilots, and related resources in the AI coding domain.
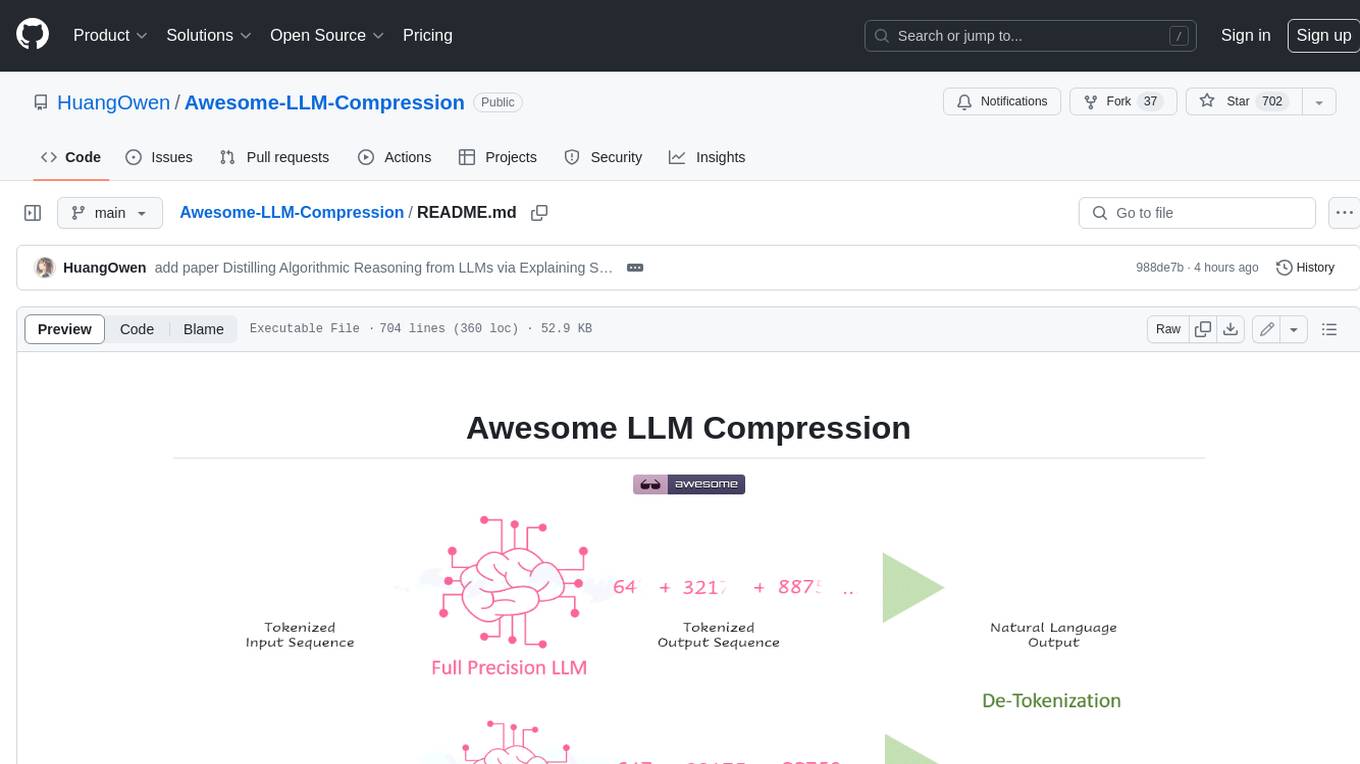
Awesome-LLM-Compression
Awesome LLM compression research papers and tools to accelerate LLM training and inference.
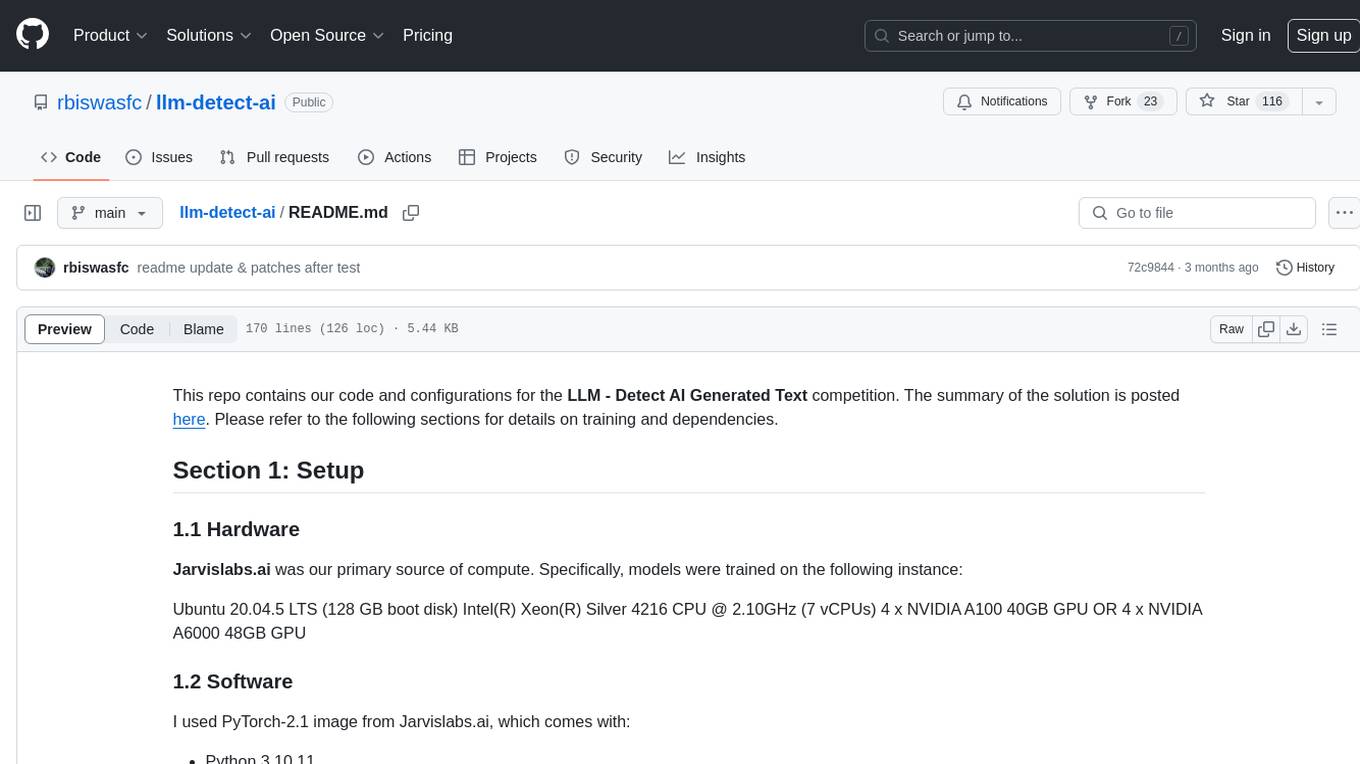
llm-detect-ai
This repository contains code and configurations for the LLM - Detect AI Generated Text competition. It includes setup instructions for hardware, software, dependencies, and datasets. The training section covers scripts and configurations for training LLM models, DeBERTa ranking models, and an embedding model. Text generation section details fine-tuning LLMs using the CLM objective on the PERSUADE corpus to generate student-like essays.
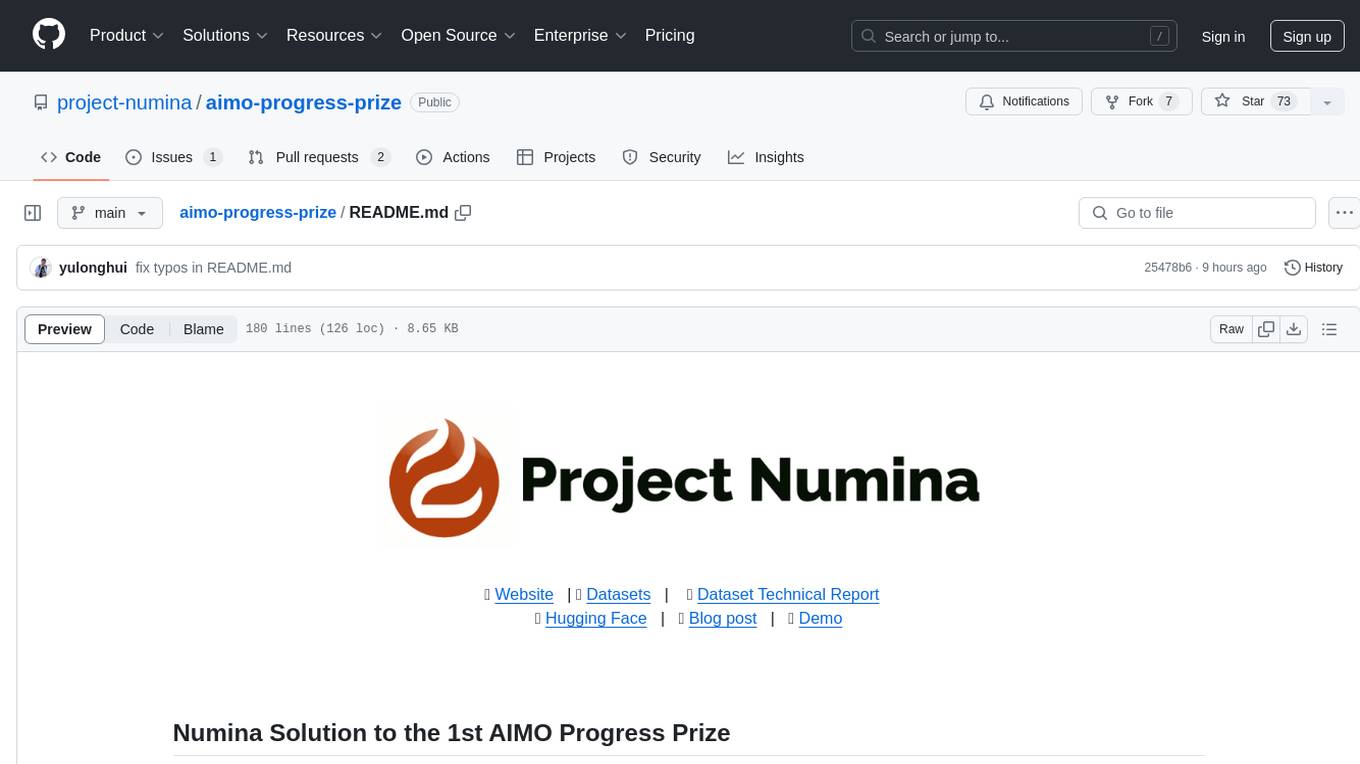
aimo-progress-prize
This repository contains the training and inference code needed to replicate the winning solution to the AI Mathematical Olympiad - Progress Prize 1. It consists of fine-tuning DeepSeekMath-Base 7B, high-quality training datasets, a self-consistency decoding algorithm, and carefully chosen validation sets. The training methodology involves Chain of Thought (CoT) and Tool Integrated Reasoning (TIR) training stages. Two datasets, NuminaMath-CoT and NuminaMath-TIR, were used to fine-tune the models. The models were trained using open-source libraries like TRL, PyTorch, vLLM, and DeepSpeed. Post-training quantization to 8-bit precision was done to improve performance on Kaggle's T4 GPUs. The project structure includes scripts for training, quantization, and inference, along with necessary installation instructions and hardware/software specifications.
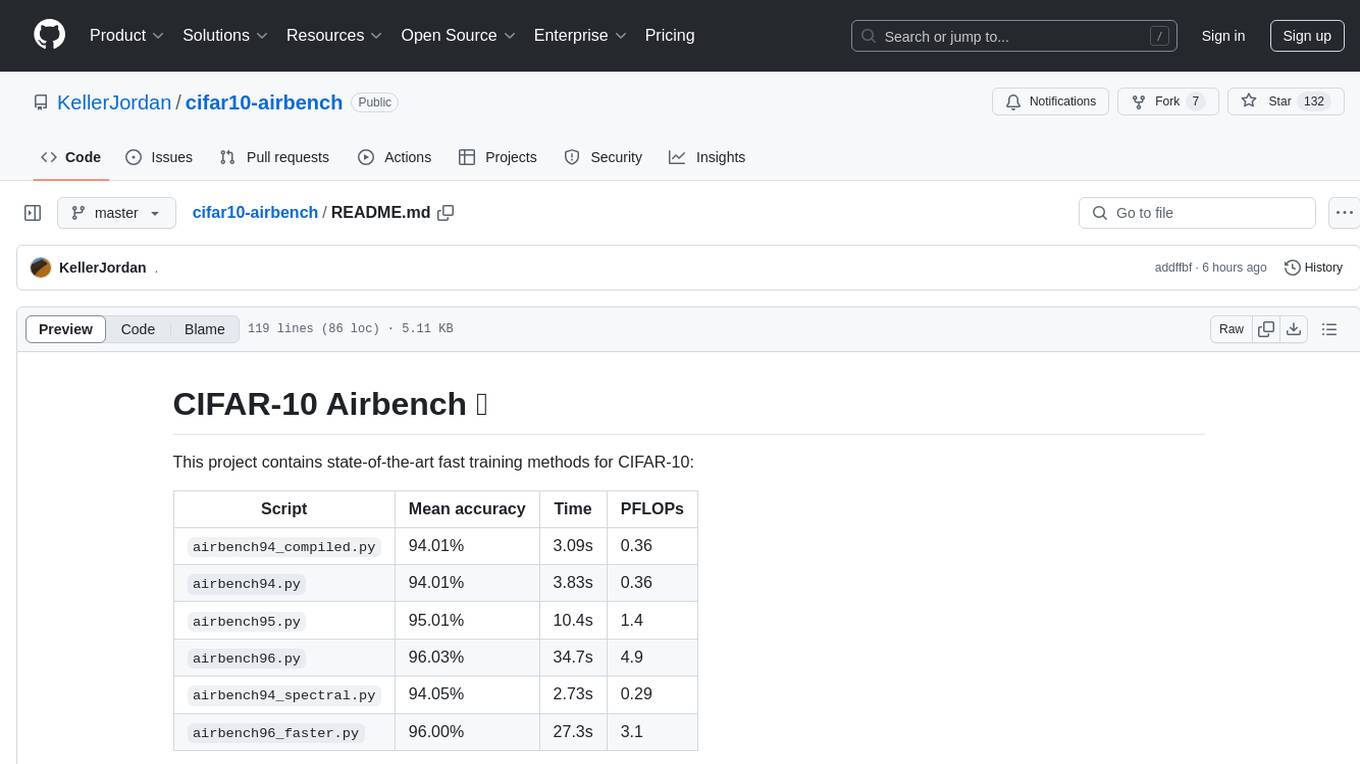
cifar10-airbench
CIFAR-10 Airbench is a project offering fast and stable training baselines for CIFAR-10 dataset, facilitating machine learning research. It provides easily runnable PyTorch scripts for training neural networks with high accuracy levels. The methods used in this project aim to accelerate research on fundamental properties of deep learning. The project includes GPU-accelerated dataloader for custom experiments and trainings, and can be used for data selection and active learning experiments. The training methods provided are faster than standard ResNet training, offering improved performance for research projects.
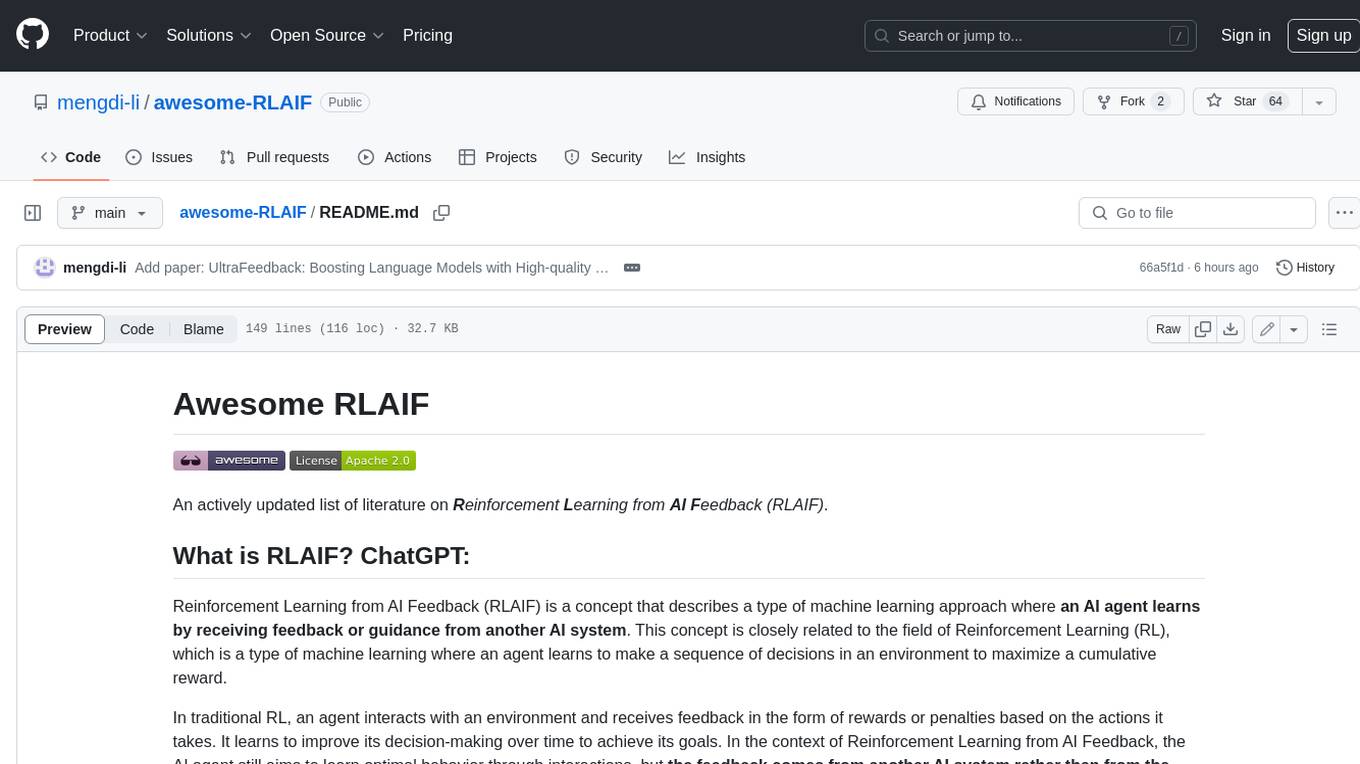
awesome-RLAIF
Reinforcement Learning from AI Feedback (RLAIF) is a concept that describes a type of machine learning approach where **an AI agent learns by receiving feedback or guidance from another AI system**. This concept is closely related to the field of Reinforcement Learning (RL), which is a type of machine learning where an agent learns to make a sequence of decisions in an environment to maximize a cumulative reward. In traditional RL, an agent interacts with an environment and receives feedback in the form of rewards or penalties based on the actions it takes. It learns to improve its decision-making over time to achieve its goals. In the context of Reinforcement Learning from AI Feedback, the AI agent still aims to learn optimal behavior through interactions, but **the feedback comes from another AI system rather than from the environment or human evaluators**. This can be **particularly useful in situations where it may be challenging to define clear reward functions or when it is more efficient to use another AI system to provide guidance**. The feedback from the AI system can take various forms, such as: - **Demonstrations** : The AI system provides demonstrations of desired behavior, and the learning agent tries to imitate these demonstrations. - **Comparison Data** : The AI system ranks or compares different actions taken by the learning agent, helping it to understand which actions are better or worse. - **Reward Shaping** : The AI system provides additional reward signals to guide the learning agent's behavior, supplementing the rewards from the environment. This approach is often used in scenarios where the RL agent needs to learn from **limited human or expert feedback or when the reward signal from the environment is sparse or unclear**. It can also be used to **accelerate the learning process and make RL more sample-efficient**. Reinforcement Learning from AI Feedback is an area of ongoing research and has applications in various domains, including robotics, autonomous vehicles, and game playing, among others.
7 - OpenAI Gpts
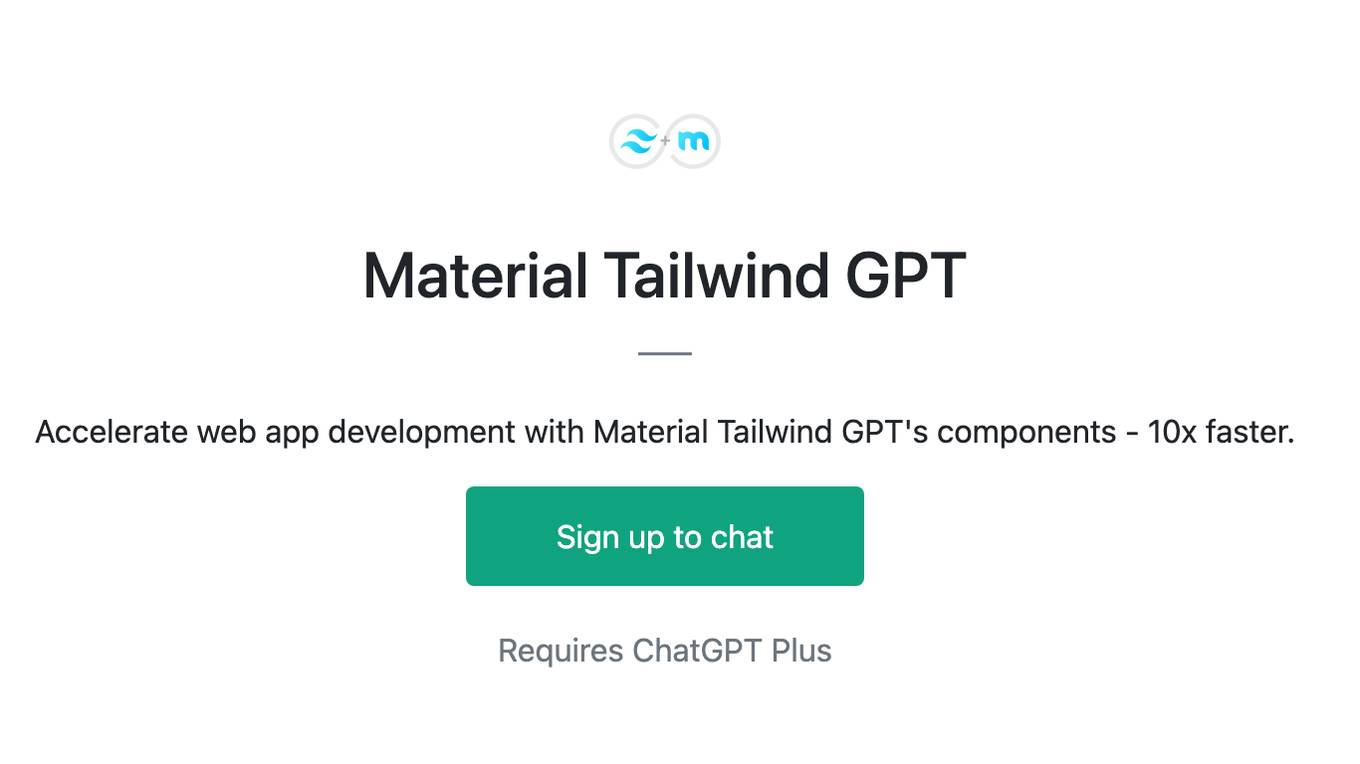
Material Tailwind GPT
Accelerate web app development with Material Tailwind GPT's components - 10x faster.
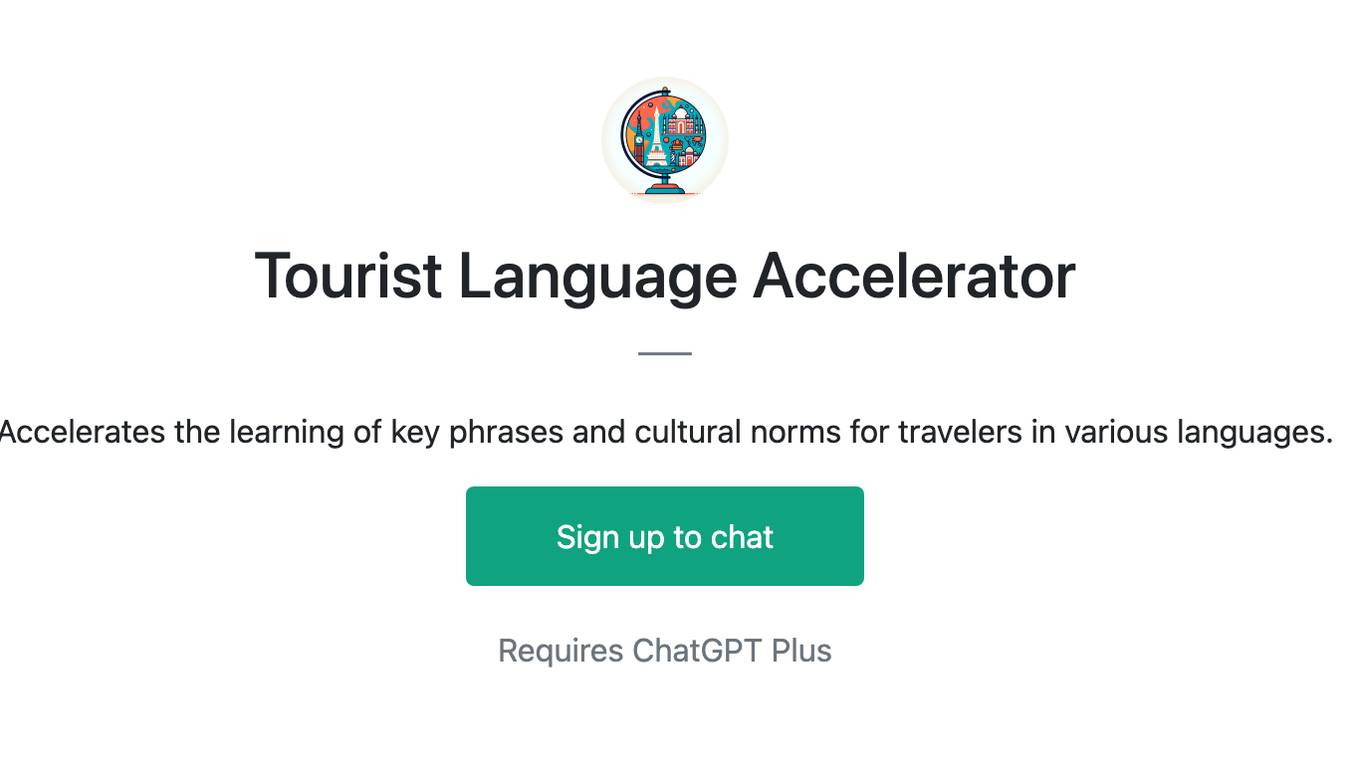
Tourist Language Accelerator
Accelerates the learning of key phrases and cultural norms for travelers in various languages.
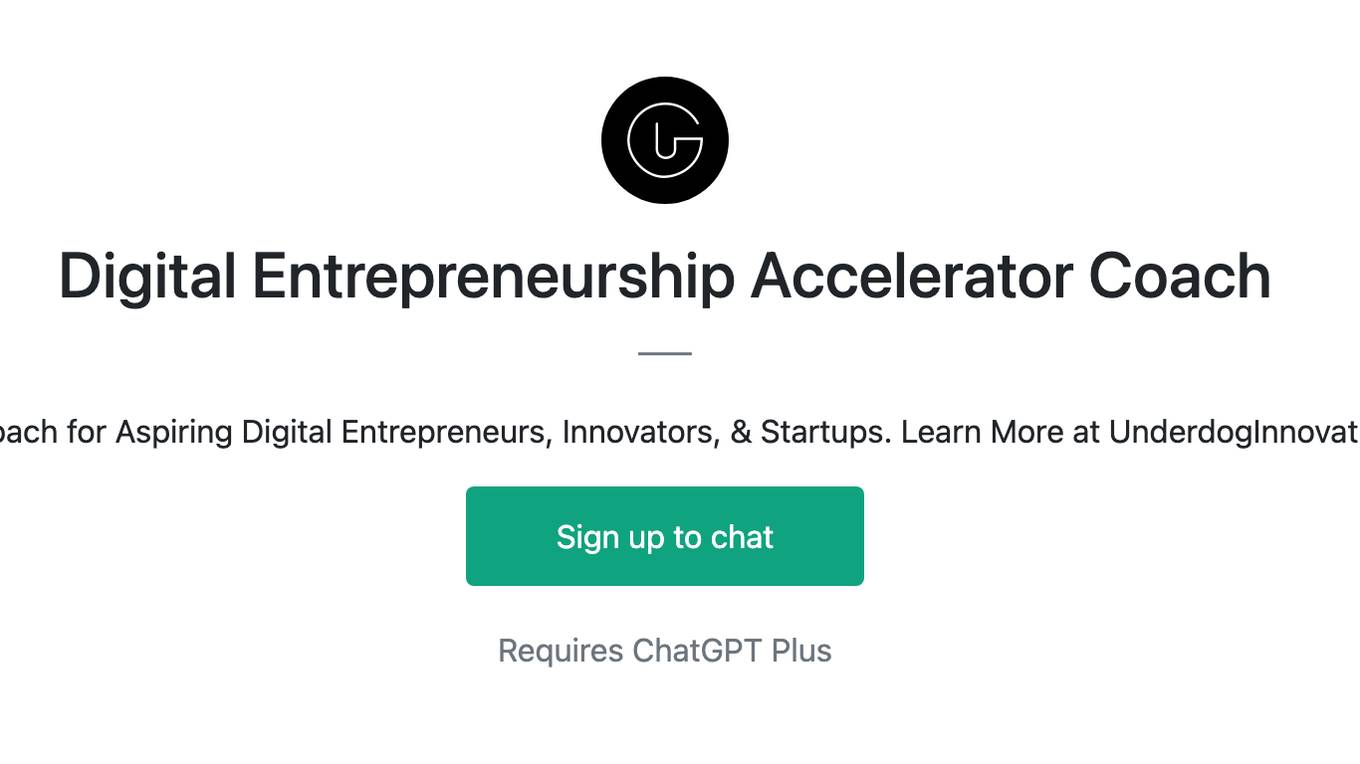
Digital Entrepreneurship Accelerator Coach
The Go-To Coach for Aspiring Digital Entrepreneurs, Innovators, & Startups. Learn More at UnderdogInnovationInc.com.
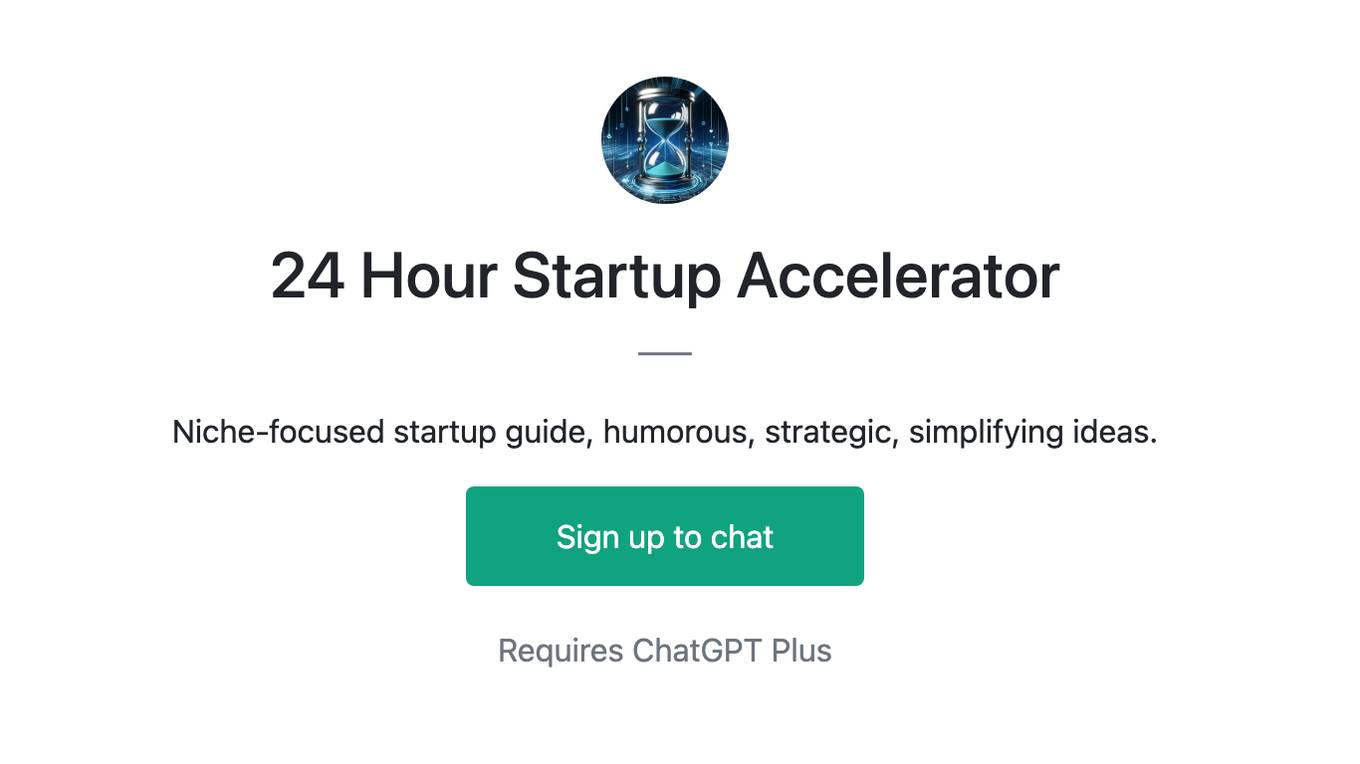
24 Hour Startup Accelerator
Niche-focused startup guide, humorous, strategic, simplifying ideas.
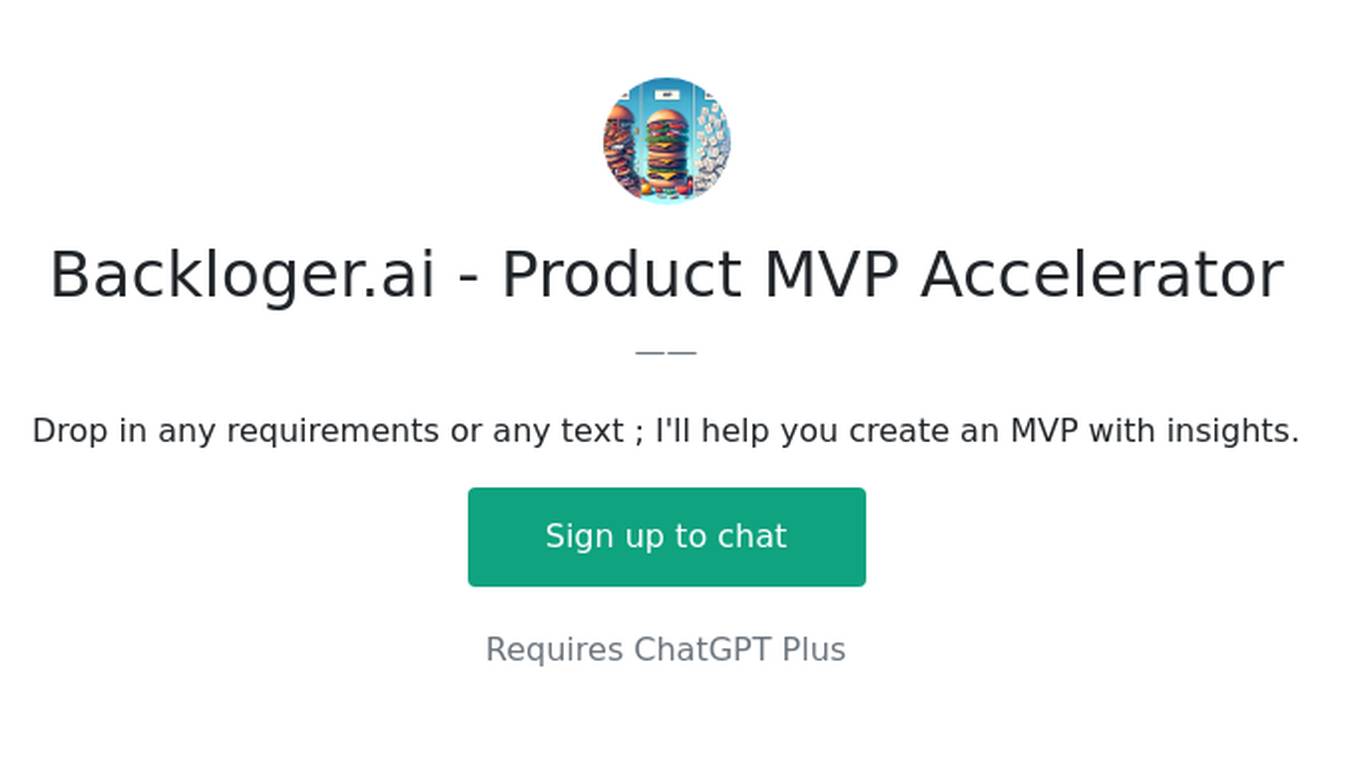
Backloger.ai - Product MVP Accelerator
Drop in any requirements or any text ; I'll help you create an MVP with insights.
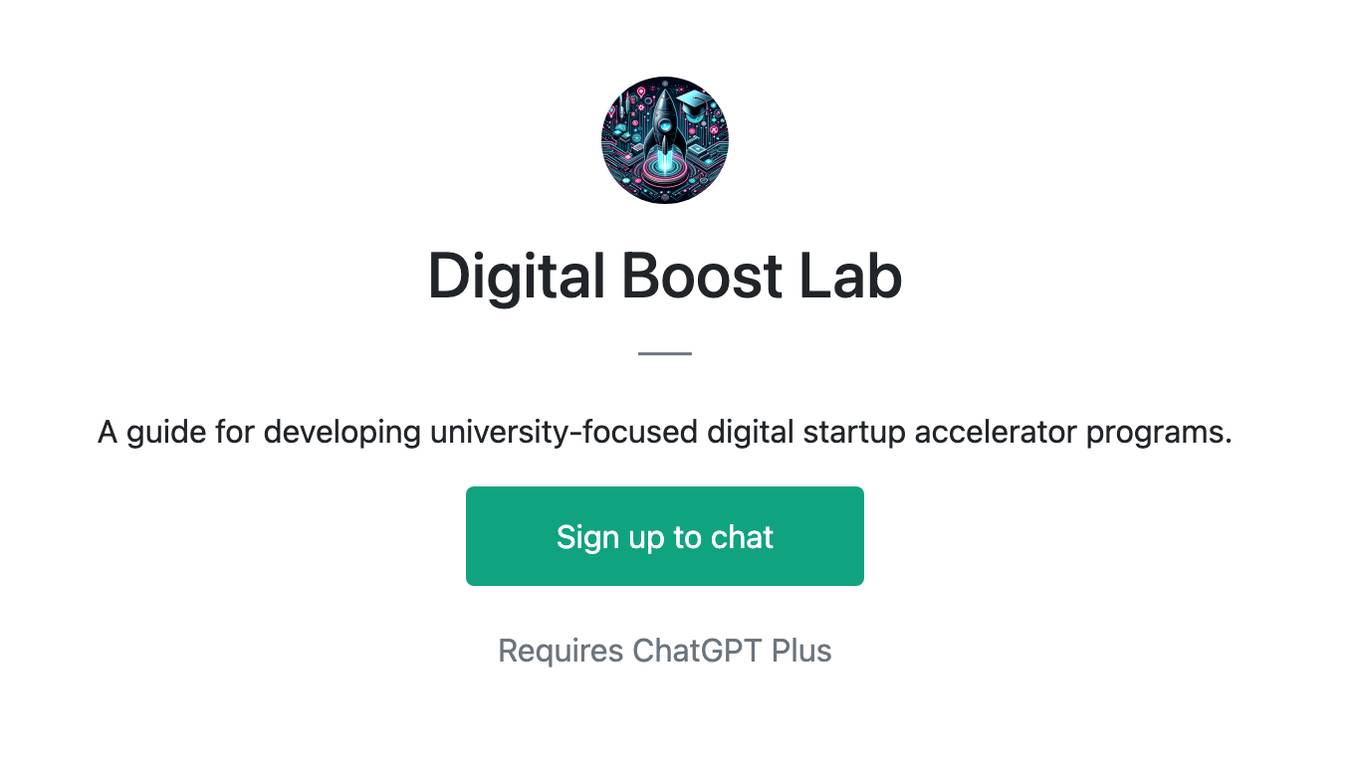
Digital Boost Lab
A guide for developing university-focused digital startup accelerator programs.