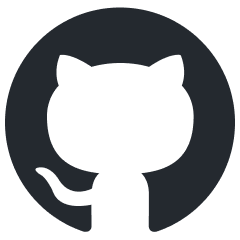
generative-ai
Gemini AI SDK for .NET and ASP.NET Core enables developers to use Google's state-of-the-art generative AI models to build AI-powered features and applications.
Stars: 86
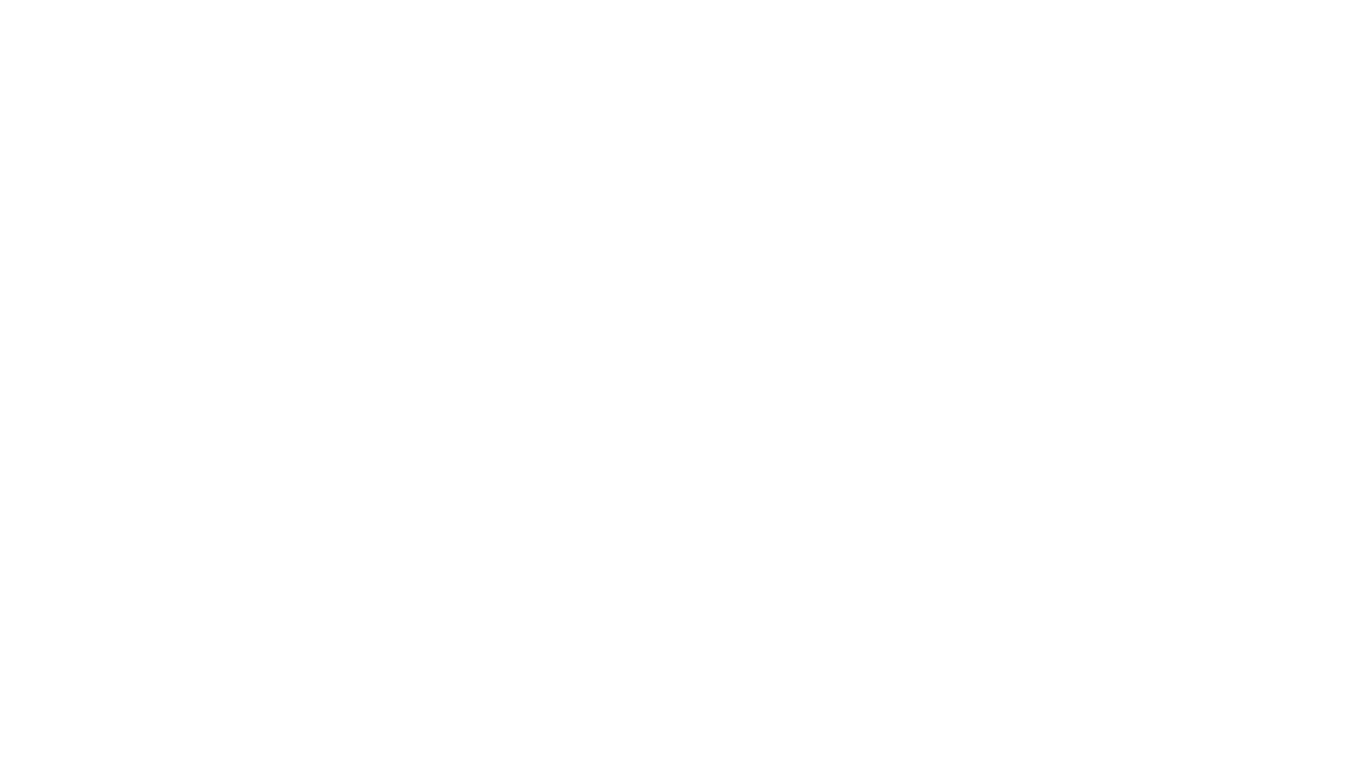
The 'Generative AI' repository provides a C# library for interacting with Google's Generative AI models, specifically the Gemini models. It allows users to access and integrate the Gemini API into .NET applications, supporting functionalities such as listing available models, generating content, creating tuned models, working with large files, starting chat sessions, and more. The repository also includes helper classes and enums for Gemini API aspects. Authentication methods include API key, OAuth, and various authentication modes for Google AI and Vertex AI. The package offers features for both Google AI Studio and Google Cloud Vertex AI, with detailed instructions on installation, usage, and troubleshooting.
README:
Access and integrate the Gemini API into your .NET applications. This SDK allows you to connect to the Gemini API through either Google AI Studio or Vertex AI. The SDK is fully compatible with all Gemini API models and features, including recent additions like improved tool usage (code execution, function calling and integrated Google search grounding), and media generation (Imagen).
Name | Package | Status |
---|---|---|
Client for .NET | Mscc.GenerativeAI |
|
Client for ASP.NET (Core) | Mscc.GenerativeAI.Web |
|
Client for .NET using Google API Client Library | Mscc.GenerativeAI.Google |
|
Client for Microsoft.Extensions.AI and Semantic Kernel | Mscc.GenerativeAI.Microsoft |
|
Read more about Mscc.GenerativeAI.Web and how to add it to your ASP.NET (Core) web applications. Read more about Mscc.GenerativeAI.Google. Read more about Mscc.GenerativeAI.Microsoft and how to use it with Semantic Kernel.
Install the package Mscc.GenerativeAI from NuGet. You can install the package from the command line using either the command line or the NuGet Package Manager Console. Or you add it directly to your .NET project.
Add the package using the dotnet
command line tool in your .NET project folder.
> dotnet add package Mscc.GenerativeAI
Working with Visual Studio use the NuGet Package Manager to install the package Mscc.GenerativeAI.
PM> Install-Package Mscc.GenerativeAI
Alternatively, add the following line to your .csproj
file.
<ItemGroup>
<PackageReference Include="Mscc.GenerativeAI" Version="2.3.4" />
</ItemGroup>
You can then add this code to your sources whenever you need to access any Gemini API provided by Google. This package works for Google AI (Google AI Studio) and Google Cloud Vertex AI.
The provided code defines a C# library for interacting with Google's Generative AI models, specifically the Gemini models. It provides functionalities to:
- List available models: This allows users to see which models are available for use.
- Get information about a specific model: This provides details about a specific model, such as its capabilities and limitations.
- Generate content: This allows users to send prompts to a model and receive generated text in response.
- Generate content stream: This allows users to receive a stream of generated text from a model, which can be useful for real-time applications.
- Generate a grounded answer: This allows users to ask questions and receive answers that are grounded in provided context.
- Generate embeddings: This allows users to convert text into numerical representations that can be used for tasks like similarity search.
- Count tokens: This allows users to estimate the cost of using a model by counting the number of tokens in a prompt or response.
- Start a chat session: This allows users to have a back-and-forth conversation with a model.
-
Create tuned models: This allows users to provide samples for tuning an existing model. Currently, only the
text-bison-001
andgemini-1.0-pro-001
models are supported for tuning - File API: This allows users to upload large files and use them with Gemini 1.5.
The package also defines various helper classes and enums to represent different aspects of the Gemini API, such as model names, request parameters, and response data.
The package supports the following use cases to authenticate.
API | Authentication | Remarks |
---|---|---|
Google AI | Authentication with an API key | |
Google AI | Authentication with OAuth | required for tuned models |
Vertex AI | Authentication with Application Default Credentials (ADC) | |
Vertex AI | Authentication with Credentials by Metadata Server | requires access to a metadata server |
Vertex AI | Authentication with OAuth | using Mscc.GenerativeAI.Google |
Vertex AI | Authentication with Service Account | using Mscc.GenerativeAI.Google |
Vertex AI | Express Mode with an API key |
This applies mainly to the instantiation procedure.
Use of Gemini API in either Google AI or Vertex AI is almost identical. The major difference is the way to instantiate the model handling your prompt.
In the cloud most settings are configured via environment variables (EnvVars). The ease of configuration, their widespread support and the simplicity of environment variables makes them a very interesting option.
Variable Name | Description |
---|---|
GOOGLE_AI_MODEL | The name of the model to use (default is Model.Gemini15Pro) |
GOOGLE_API_KEY | The API key generated in Google AI Studio |
GOOGLE_PROJECT_ID | Project ID in Google Cloud to access the APIs |
GOOGLE_REGION | Region in Google Cloud (default is us-central1) |
GOOGLE_ACCESS_TOKEN | The access token required to use models running in Vertex AI |
GOOGLE_APPLICATION_CREDENTIALS | Path to the application credentials file. |
GOOGLE_WEB_CREDENTIALS | Path to a Web credentials file. |
Using any environment variable provides simplified access to a model.
using Mscc.GenerativeAI;
var model = new GenerativeModel();
Google AI with an API key
using Mscc.GenerativeAI;
// Google AI with an API key
var googleAI = new GoogleAI(apiKey: "your API key");
var model = googleAI.GenerativeModel(model: Model.Gemini15Pro);
Google AI with OAuth. Use gcloud auth application-default print-access-token
to get the access token.
using Mscc.GenerativeAI;
// Google AI with OAuth. Use `gcloud auth application-default print-access-token` to get the access token.
var accessToken = "your access token";
var googleAI = new GoogleAI(accessToken: accessToken);
var model = googleAI.GenerativeModel(model: Model.Gemini15Pro);
Vertex AI with OAuth. Use gcloud auth application-default print-access-token
to get the access token.
using Mscc.GenerativeAI;
// Vertex AI with OAuth. Use `gcloud auth application-default print-access-token` to get the access token.
var vertex = new VertexAI(projectId: projectId, region: region);
var model = vertex.GenerativeModel(model: Model.Gemini15Pro);
model.AccessToken = accessToken;
Vertex AI in express mode using an API key.
using Mscc.GenerativeAI;
// Vertex AI in express mode with an API key.
var vertex = new VertexAI(apiKey: "your API key");
var model = vertex.GenerativeModel(model: Model.Gemini20FlashExperimental);
The ConfigurationFixture
type in the test project implements multiple options to retrieve sensitive information, i.e. API key or access token.
Working with Google AI in your application requires an API key. Get an API key from Google AI Studio.
using Mscc.GenerativeAI;
var apiKey = "your_api_key";
var prompt = "Write a story about a magic backpack.";
var googleAI = new GoogleAI(apiKey: apiKey);
var model = googleAI.GenerativeModel(model: Model.Gemini15Pro);
var response = await model.GenerateContent(prompt);
Console.WriteLine(response.Text);
Use of Vertex AI requires an account on Google Cloud, a project with billing and Vertex AI API enabled.
using Mscc.GenerativeAI;
var projectId = "your_google_project_id"; // the ID of a project, not its name.
var region = "us-central1"; // see documentation for available regions.
var accessToken = "your_access_token"; // use `gcloud auth application-default print-access-token` to get it.
var prompt = "Write a story about a magic backpack.";
var vertex = new VertexAI(projectId: projectId, region: region);
var model = vertex.GenerativeModel(model: Model.Gemini15Pro);
model.AccessToken = accessToken;
var response = await model.GenerateContent(prompt);
Console.WriteLine(response.Text);
Vertex AI in express mode is the fastest way to start building generative AI applications on Google Cloud. Signing up in express mode is quick and easy, and it doesn't require entering any billing information. After you sign up, you can access and use Google Cloud APIs in just a few steps.
using Mscc.GenerativeAI;
var prompt = "Explain bubble sort to me.";
var vertex = new VertexAI(apiKey: "your API key");
var model = vertex.GenerativeModel(model: Model.Gemini20FlashExperimental);
var response = await model.GenerateContent(prompt);
Console.WriteLine(response.Text);
Supported models are accessible via the Model
class. Since release 0.9.0 there is support for the previous PaLM 2 models and their functionalities.
The model can be injected with a system instruction that applies to all further requests. Following is an example how to instruct the model to respond like a pirate.
var apiKey = "your_api_key";
var systemInstruction = new Content("You are a friendly pirate. Speak like one.");
var prompt = "Good morning! How are you?";
IGenerativeAI genAi = new GoogleAI(apiKey);
var model = genAi.GenerativeModel(Model.Gemini15ProLatest, systemInstruction: systemInstruction);
var request = new GenerateContentRequest(prompt);
var response = await model.GenerateContent(request);
Console.WriteLine(response.Text);
The response might look similar to this:
Ahoy there, matey! I be doin' finer than a freshly swabbed poop deck on this fine mornin', how about yerself?
Shimmer me timbers, it's good to see a friendly face!
What brings ye to these here waters?
Gemini generates unstructured text by default, but some applications require structured text. For these use cases, you can constrain Gemini to respond with JSON, a structured data format suitable for automated processing.
You can control the structure of the JSON response by suppling a schema. There are two ways to supply a schema to the model:
- As text in the prompt
- As a structured schema supplied through model configuration
class Recipe {
public string RecipeName { get; set; }
}
// generate structure JSON output
var apiKey = "your_api_key";
var prompt = "List a few popular cookie recipes.";
var googleAi = new GoogleAI(apiKey);
var model = googleAi.GenerativeModel(model: Model.Gemini15ProLatest);
var generationConfig = new GenerationConfig()
{
ResponseMimeType = "application/json",
ResponseSchema = new List<Recipe>()
};
var response = await model.GenerateContent(prompt,
generationConfig: generationConfig);
Console.WriteLine(response?.Text);
}
The output might look like this:
[{"recipeName": "Chocolate Chip Cookies"}, {"recipeName": "Peanut Butter Cookies"}, {"recipeName": "Snickerdoodles"}, {"recipeName": "Oatmeal Raisin Cookies"}, {"recipeName": "Sugar Cookies"}]
To activate Google Search as a tool, set the boolean property UseGoogleSearch
to true, like the following example.
var apiKey = "your_api_key";
var prompt = "When is the next total solar eclipse in Mauritius?";
var genAi = new GoogleAI(apiKey);
var model = genAi.GenerativeModel(Model.Gemini20FlashExperimental);
model.UseGoogleSearch = true;
var response = await model.GenerateContent(prompt);
Console.WriteLine(string.Join(Environment.NewLine,
response.Candidates![0].Content!.Parts
.Select(x => x.Text)
.ToArray()));
More details are described in the API documentation on Search as a tool.
The simplest version is to toggle the boolean property UseGrounding
, like so.
var apiKey = "your_api_key";
var prompt = "What is the current Google stock price?";
var genAi = new GoogleAI(apiKey);
var model = genAi.GenerativeModel(Model.Gemini15Pro002);
model.UseGrounding = true;
var response = await model.GenerateContent(prompt);
Console.WriteLine(response.Text);
In case that you would like to have more control over the Google Search retrieval parameters, use the following approach.
var apiKey = "your_api_key";
var prompt = "Who won Wimbledon this year?";
IGenerativeAI genAi = new GoogleAI(apiKey);
var model = genAi.GenerativeModel(Model.Gemini15Pro002,
tools: [new Tool { GoogleSearchRetrieval =
new(DynamicRetrievalConfigMode.ModeUnspecified, 0.06f) }]);
var response = await model.GenerateContent(prompt);
Console.WriteLine(response.Text);
In either case, the returned Candidates
item type has an additional property GroundingMetadata
which provides the details of the Google Search based grounding
using Mscc.GenerativeAI;
var apiKey = "your_api_key";
var prompt = "Parse the time and city from the airport board shown in this image into a list, in Markdown";
var googleAI = new GoogleAI(apiKey: "your API key");
var model = googleAI.GenerativeModel(model: Model.GeminiVisionPro);
var request = new GenerateContentRequest(prompt);
await request.AddMedia("https://raw.githubusercontent.com/mscraftsman/generative-ai/refs/heads/main/tests/Mscc.GenerativeAI/payload/timetable.png");
var response = await model.GenerateContent(request);
Console.WriteLine(response.Text);
The part of InlineData
is supported by both Google AI and Vertex AI. Whereas the part FileData
is restricted to Vertex AI only.
Gemini enables you to have freeform conversations across multiple turns. You can interact with Gemini Pro using a single-turn prompt and response or chat with it in a multi-turn, continuous conversation, even for code understanding and generation.
using Mscc.GenerativeAI;
var apiKey = "your_api_key";
var googleAI = new GoogleAI(apiKey);
var model = googleAI.GenerativeModel(); // using default model: gemini-1.5-pro
var chat = model.StartChat(); // optionally pass a previous history in the constructor.
// Instead of discarding you could also use the response and access `response.Text`.
_ = await chat.SendMessage("Hello, fancy brainstorming about IT?");
_ = await chat.SendMessage("In one sentence, explain how a computer works to a young child.");
_ = await chat.SendMessage("Okay, how about a more detailed explanation to a high schooler?");
_ = await chat.SendMessage("Lastly, give a thorough definition for a CS graduate.");
// A chat session keeps every response in its history.
chat.History.ForEach(c => Console.WriteLine($"{c.Role}: {c.Text}"));
// Last request/response pair can be removed from the history.
var latest = chat.Rewind();
Console.WriteLine($"{latest.Sent} - {latest.Received}");
With Gemini 1.5 you can create multimodal prompts supporting large files.
The following example uploads one or more files via File API and the created File URIs are used in the GenerateContent
call to generate text.
using Mscc.GenerativeAI;
var apiKey = "your_api_key";
var prompt = "Make a short story from the media resources. The media resources are:";
IGenerativeAI genAi = new GoogleAI(apiKey);
var model = genAi.GenerativeModel(Model.Gemini15Pro);
// Upload your large image(s).
// Instead of discarding you could also use the response and access `response.Text`.
var filePath = Path.Combine(Environment.CurrentDirectory, "verylarge.png");
var displayName = "My very large image";
_ = await model.UploadMedia(filePath, displayName);
// Create the prompt with references to File API resources.
var request = new GenerateContentRequest(prompt);
var files = await model.ListFiles();
foreach (var file in files.Where(x => x.MimeType.StartsWith("image/")))
{
Console.WriteLine($"File: {file.Name}");
request.AddMedia(file);
}
var response = await model.GenerateContent(request);
Console.WriteLine(response.Text);
Read more about Gemini 1.5: Our next-generation model, now available for Private Preview in Google AI Studio.
The Gemini API lets you tune models on your own data. Since it's your data and your tuned models this needs stricter access controls than API-Keys can provide.
Before you can create a tuned model, you'll need to set up OAuth for your project.
using Mscc.GenerativeAI;
var projectId = "your_google_project_id"; // the ID of a project, not its name.
var accessToken = "your_access_token"; // use `gcloud auth application-default print-access-token` to get it.
var googleAI = new GoogleAI(accessToken: accessToken);
var model = googleAI.GenerativeModel(model: Model.Gemini10Pro001);
model.ProjectId = projectId;
var parameters = new HyperParameters() { BatchSize = 2, LearningRate = 0.001f, EpochCount = 3 };
var dataset = new List<TuningExample>
{
new() { TextInput = "1", Output = "2" },
new() { TextInput = "3", Output = "4" },
new() { TextInput = "-3", Output = "-2" },
new() { TextInput = "twenty two", Output = "twenty three" },
new() { TextInput = "two hundred", Output = "two hundred one" },
new() { TextInput = "ninety nine", Output = "one hundred" },
new() { TextInput = "8", Output = "9" },
new() { TextInput = "-98", Output = "-97" },
new() { TextInput = "1,000", Output = "1,001" },
new() { TextInput = "thirteen", Output = "fourteen" },
new() { TextInput = "seven", Output = "eight" },
};
var request = new CreateTunedModelRequest(Model.Gemini10Pro001,
"Simply autogenerated Test model",
dataset,
parameters);
var response = await model.CreateTunedModel(request);
Console.WriteLine($"Name: {response.Name}");
Console.WriteLine($"Model: {response.Metadata.TunedModel} (Steps: {response.Metadata.TotalSteps})");
(This is still work in progress but operational. Future release will provide types to simplify the create request.)
Tuned models appear in your Google AI Studio library.
Read more about Tune Gemini Pro in Google AI Studio or with the Gemini API.
The folders samples and tests contain more examples.
- Sample console application
- ASP.NET Core Minimal web application
- ASP.NET Core MVP web application (work in progress!)
Sometimes you might have authentication warnings HTTP 403 (Forbidden). Especially while working with OAuth-based authentication. You can fix it by re-authenticating through ADC.
gcloud config set project "$PROJECT_ID"
gcloud auth application-default login
gcloud auth application-default set-quota-project "$PROJECT_ID"
Make sure that the required API have been enabled.
# ENABLE APIs
gcloud services enable aiplatform.googleapis.com
In case of long-running streaming requests it can happen that you get a HttpIOException
: The response ended prematurely while waiting for the next frame from the server. (ResponseEnded).
The root cause is the .NET runtime and the solution is to upgrade to the latest version of the .NET runtime.
In case that you cannot upgrade you might disable dynamic window sizing as a workaround:
Either using the environment variable DOTNET_SYSTEM_NET_HTTP_SOCKETSHTTPHANDLER_HTTP2FLOWCONTROL_DISABLEDYNAMICWINDOWSIZING
DOTNET_SYSTEM_NET_HTTP_SOCKETSHTTPHANDLER_HTTP2FLOWCONTROL_DISABLEDYNAMICWINDOWSIZING=true
or setting an AppContext
switch:
AppContext.SetSwitch("System.Net.SocketsHttpHandler.Http2FlowControl.DisableDynamicWindowSizing", true);
Several issues regarding this problem have been reported on GitHub:
- https://github.com/dotnet/runtime/pull/97881
- https://github.com/grpc/grpc-dotnet/issues/2361
- https://github.com/grpc/grpc-dotnet/issues/2358
The repository contains a number of test cases for Google AI and Vertex AI. You will find them in the tests folder. They are part of the [GenerativeAI solution].
To run the tests, either enter the relevant information into the appsettings.json, create a new appsettings.user.json
file with the same JSON structure in the tests
folder, or define the following environment variables
- GOOGLE_API_KEY
- GOOGLE_PROJECT_ID
- GOOGLE_REGION
- GOOGLE_ACCESS_TOKEN (optional: if absent,
gcloud auth application-default print-access-token
is executed)
The test cases should provide more insights and use cases on how to use the Mscc.GenerativeAI package in your .NET projects.
The following link opens an instance of the code repository in Google Project IDX.
This lets you work instantly with the code base without having to install anything.
For support and feedback kindly create issues at the https://github.com/mscraftsman/generative-ai repository.
This project is licensed under the Apache-2.0 License - see the LICENSE file for details.
If you use Mscc.GenerativeAI in your research project, kindly cite as follows
@misc{Mscc.GenerativeAI,
author = {Kirstätter, J and MSCraftsman},
title = {Mscc.GenerativeAI - Gemini AI Client for .NET and ASP.NET Core},
year = {2024},
publisher = {GitHub},
journal = {GitHub repository},
note = {https://github.com/mscraftsman/generative-ai}
}
Created by Jochen Kirstätter.
For Tasks:
Click tags to check more tools for each tasksFor Jobs:
Alternative AI tools for generative-ai
Similar Open Source Tools
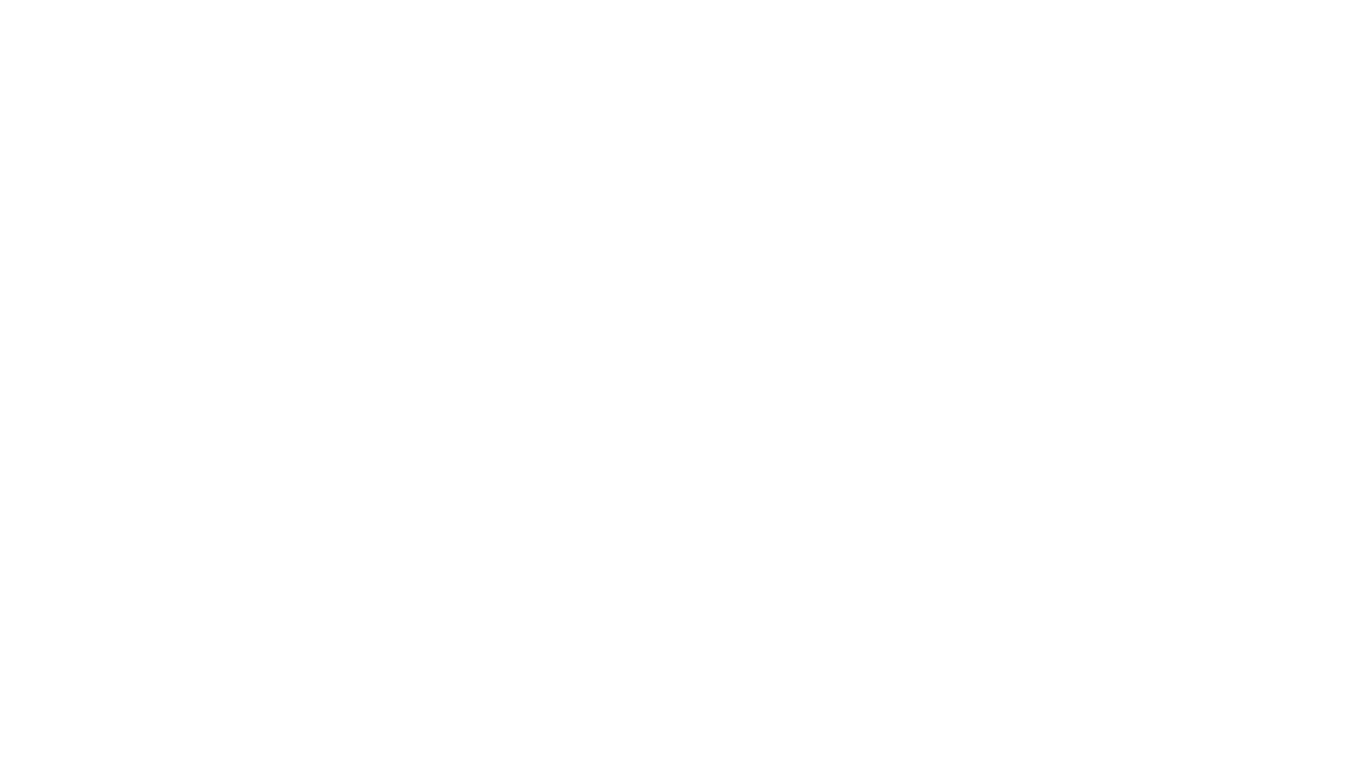
generative-ai
The 'Generative AI' repository provides a C# library for interacting with Google's Generative AI models, specifically the Gemini models. It allows users to access and integrate the Gemini API into .NET applications, supporting functionalities such as listing available models, generating content, creating tuned models, working with large files, starting chat sessions, and more. The repository also includes helper classes and enums for Gemini API aspects. Authentication methods include API key, OAuth, and various authentication modes for Google AI and Vertex AI. The package offers features for both Google AI Studio and Google Cloud Vertex AI, with detailed instructions on installation, usage, and troubleshooting.
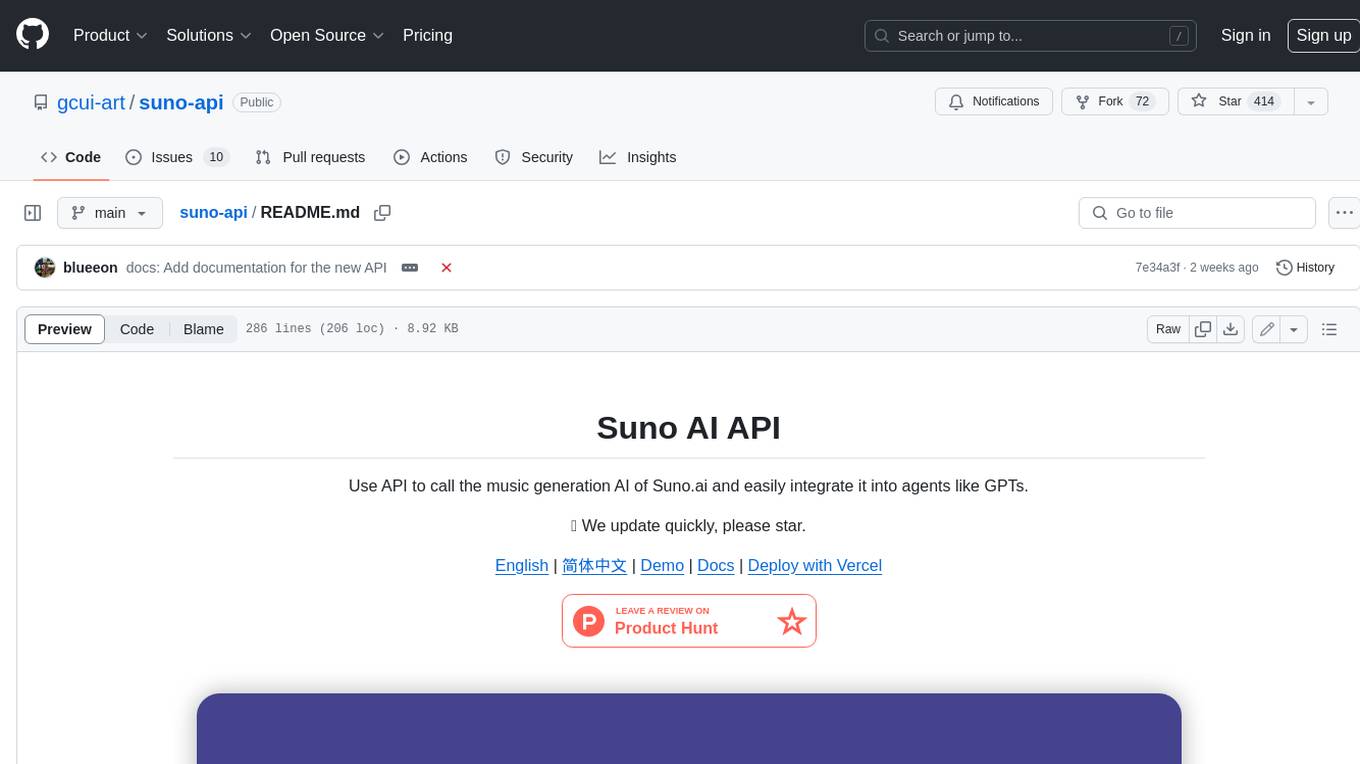
suno-api
Suno AI API is an open-source project that allows developers to integrate the music generation capabilities of Suno.ai into their own applications. The API provides a simple and convenient way to generate music, lyrics, and other audio content using Suno.ai's powerful AI models. With Suno AI API, developers can easily add music generation functionality to their apps, websites, and other projects.
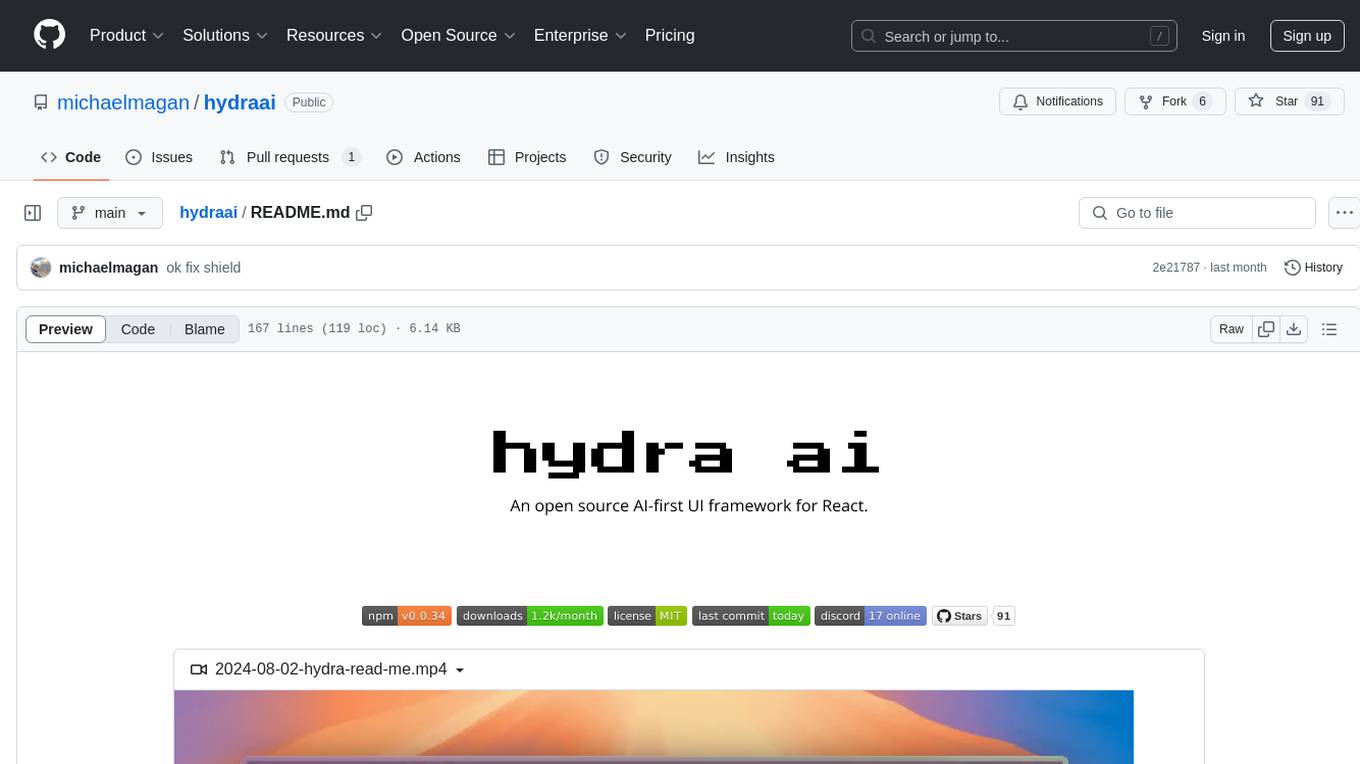
hydraai
Generate React components on-the-fly at runtime using AI. Register your components, and let Hydra choose when to show them in your App. Hydra development is still early, and patterns for different types of components and apps are still being developed. Join the discord to chat with the developers. Expects to be used in a NextJS project. Components that have function props do not work.
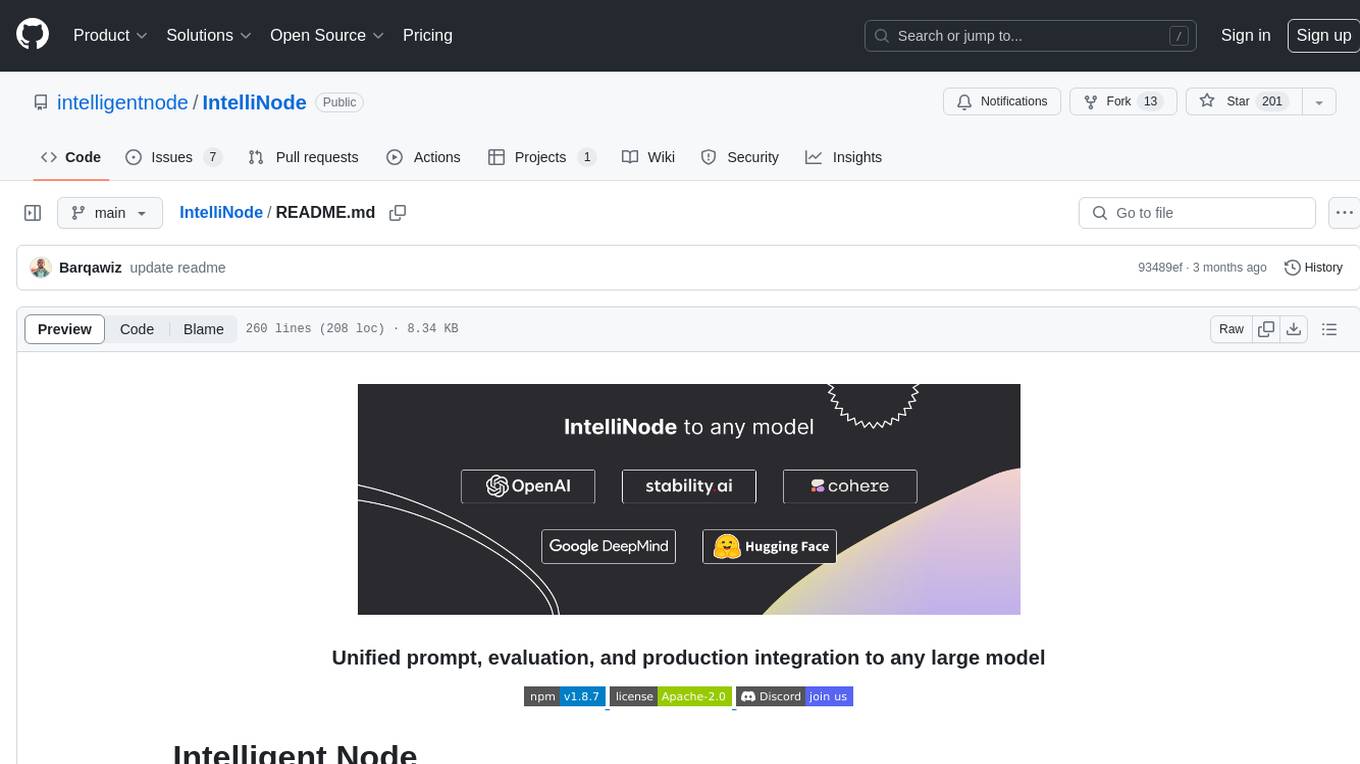
IntelliNode
IntelliNode is a javascript module that integrates cutting-edge AI models like ChatGPT, LLaMA, WaveNet, Gemini, and Stable diffusion into projects. It offers functions for generating text, speech, and images, as well as semantic search, multi-model evaluation, and chatbot capabilities. The module provides a wrapper layer for low-level model access, a controller layer for unified input handling, and a function layer for abstract functionality tailored to various use cases.
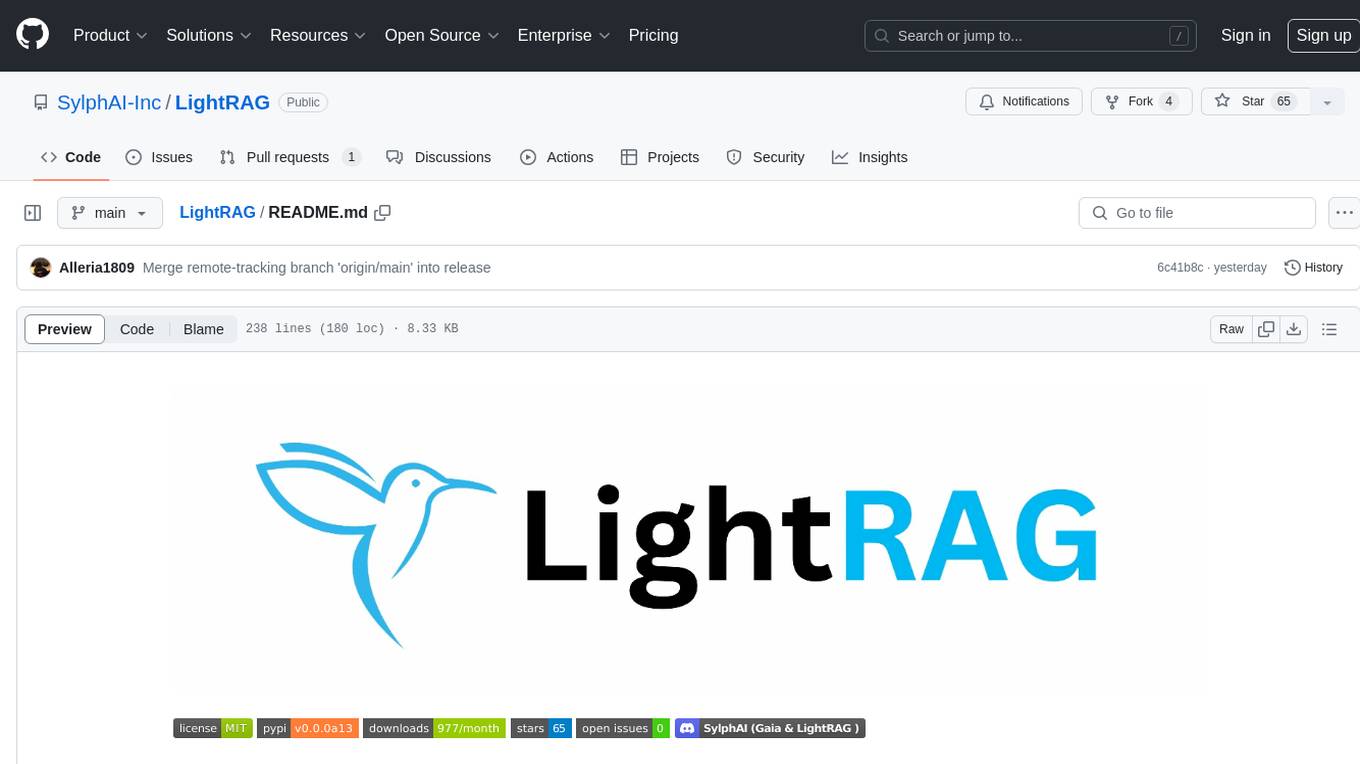
LightRAG
LightRAG is a PyTorch library designed for building and optimizing Retriever-Agent-Generator (RAG) pipelines. It follows principles of simplicity, quality, and optimization, offering developers maximum customizability with minimal abstraction. The library includes components for model interaction, output parsing, and structured data generation. LightRAG facilitates tasks like providing explanations and examples for concepts through a question-answering pipeline.
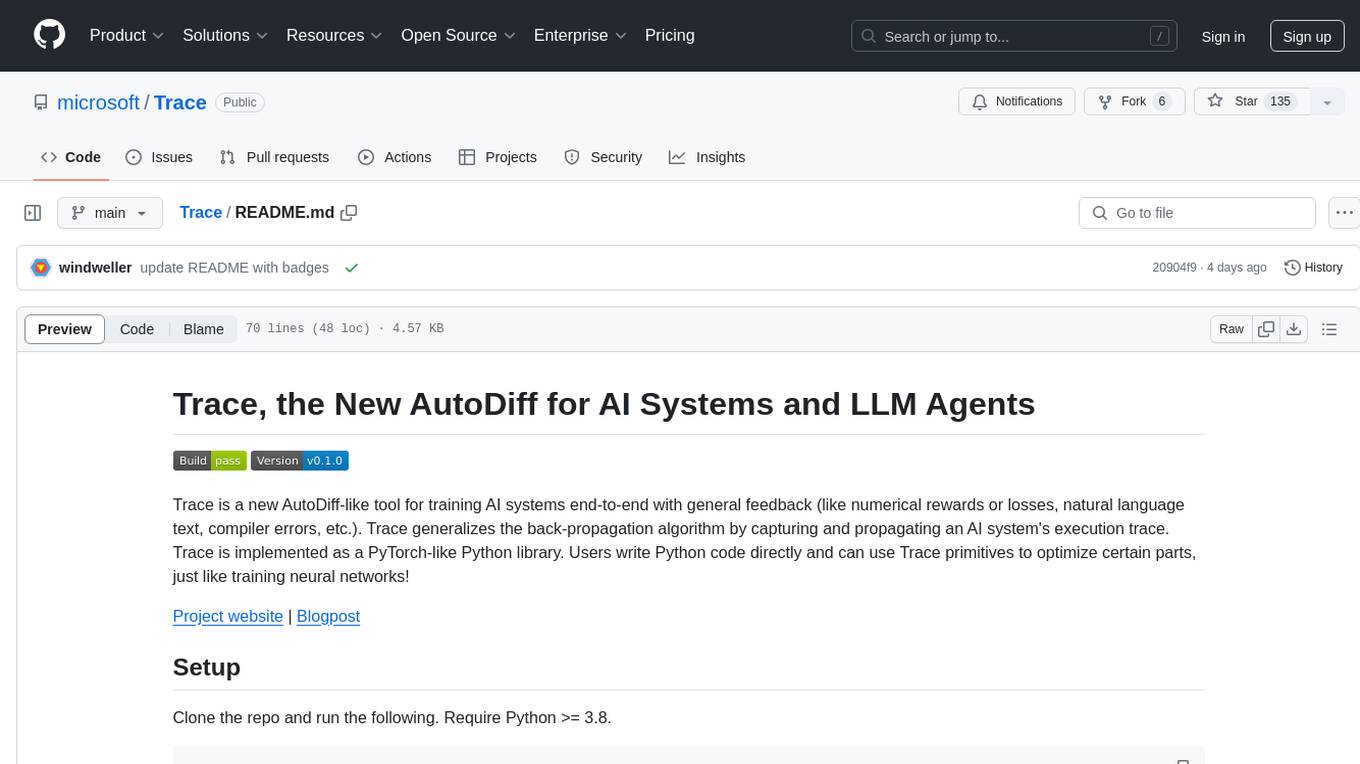
Trace
Trace is a new AutoDiff-like tool for training AI systems end-to-end with general feedback. It generalizes the back-propagation algorithm by capturing and propagating an AI system's execution trace. Implemented as a PyTorch-like Python library, users can write Python code directly and use Trace primitives to optimize certain parts, similar to training neural networks.
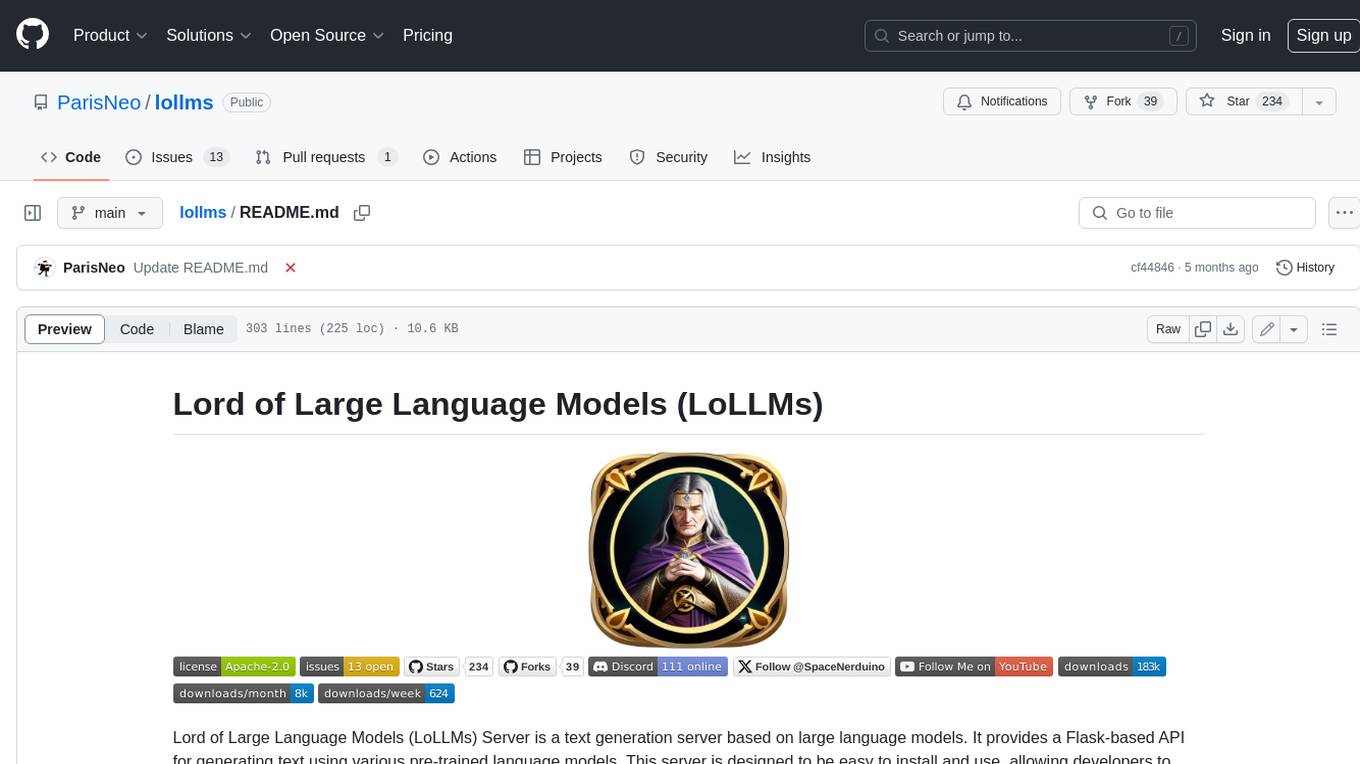
lollms
LoLLMs Server is a text generation server based on large language models. It provides a Flask-based API for generating text using various pre-trained language models. This server is designed to be easy to install and use, allowing developers to integrate powerful text generation capabilities into their applications.
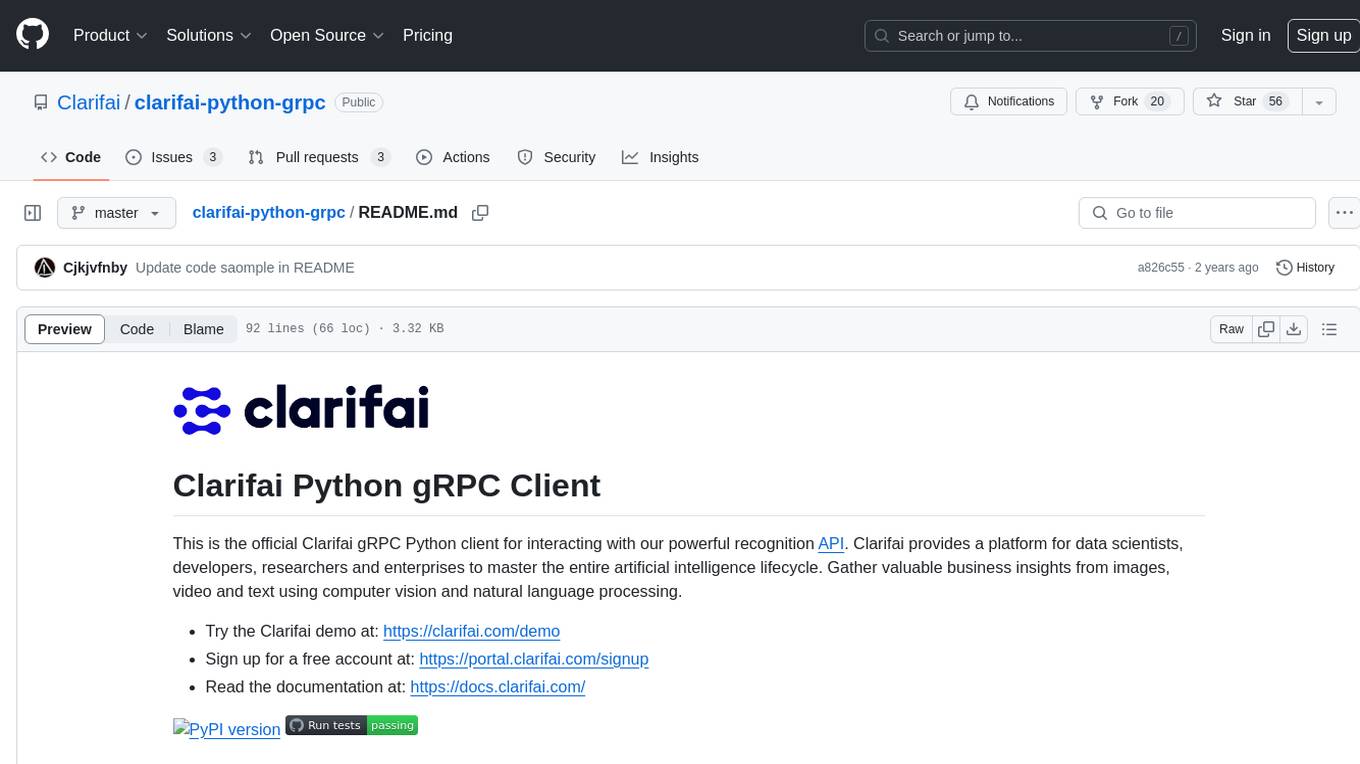
clarifai-python-grpc
This is the official Clarifai gRPC Python client for interacting with their recognition API. Clarifai offers a platform for data scientists, developers, researchers, and enterprises to utilize artificial intelligence for image, video, and text analysis through computer vision and natural language processing. The client allows users to authenticate, predict concepts in images, and access various functionalities provided by the Clarifai API. It follows a versioning scheme that aligns with the backend API updates and includes specific instructions for installation and troubleshooting. Users can explore the Clarifai demo, sign up for an account, and refer to the documentation for detailed information.
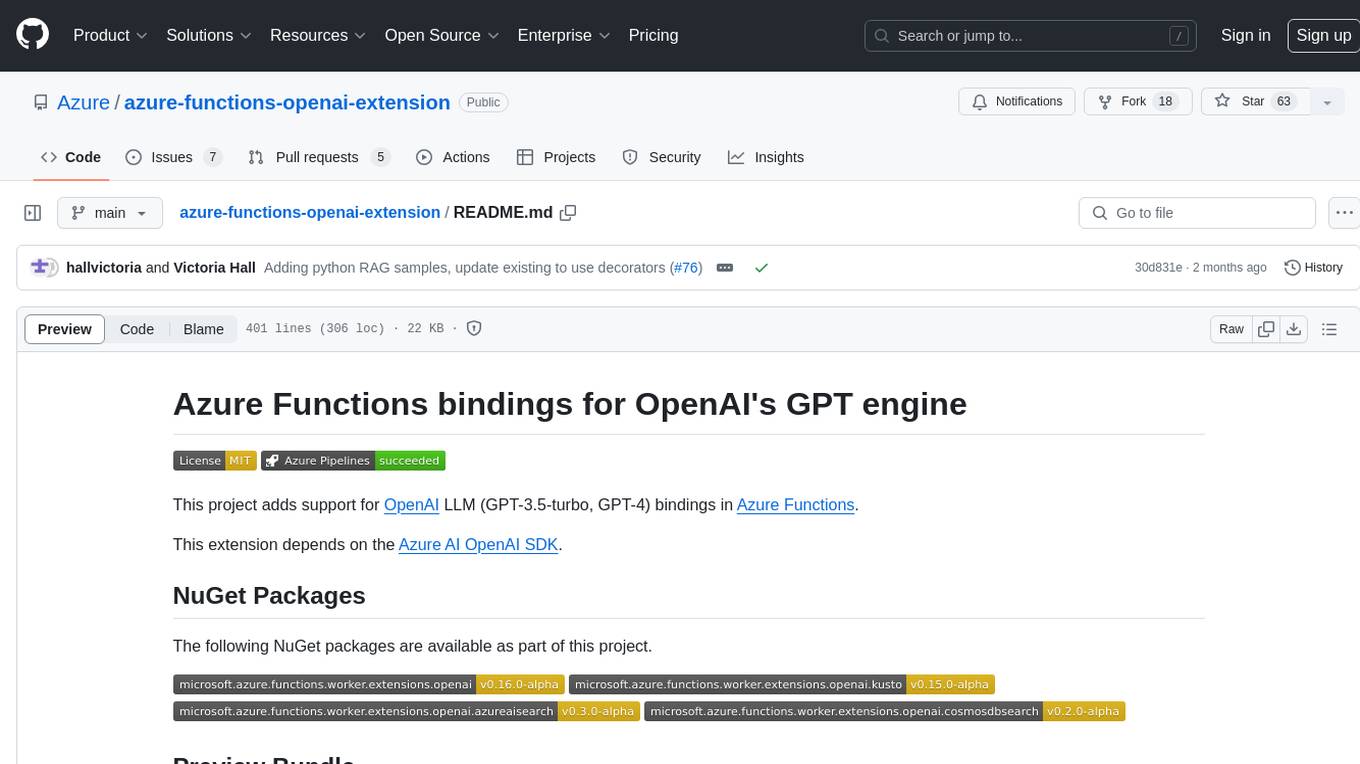
azure-functions-openai-extension
Azure Functions OpenAI Extension is a project that adds support for OpenAI LLM (GPT-3.5-turbo, GPT-4) bindings in Azure Functions. It provides NuGet packages for various functionalities like text completions, chat completions, assistants, embeddings generators, and semantic search. The project requires .NET 6 SDK or greater, Azure Functions Core Tools v4.x, and specific settings in Azure Function or local settings for development. It offers features like text completions, chat completion, assistants with custom skills, embeddings generators for text relatedness, and semantic search using vector databases. The project also includes examples in C# and Python for different functionalities.
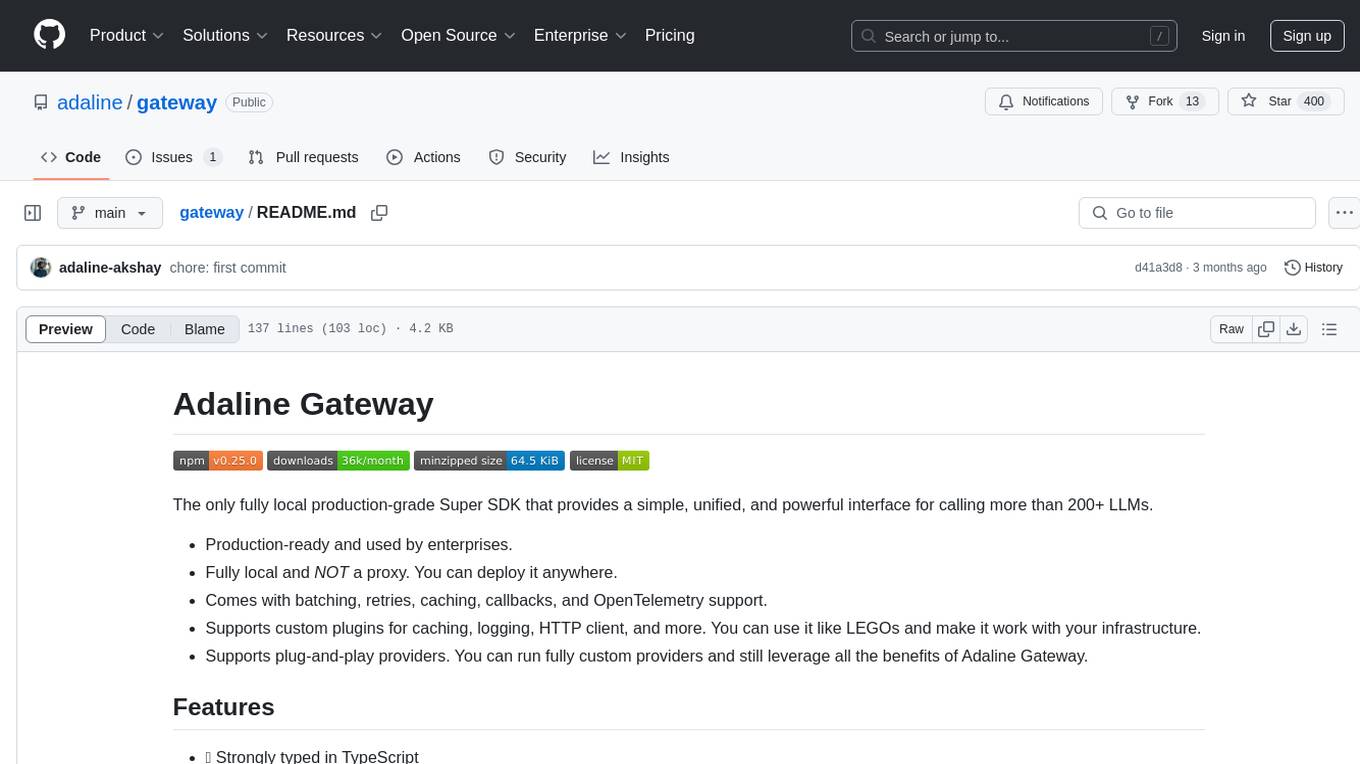
gateway
Adaline Gateway is a fully local production-grade Super SDK that offers a unified interface for calling over 200+ LLMs. It is production-ready, supports batching, retries, caching, callbacks, and OpenTelemetry. Users can create custom plugins and providers for seamless integration with their infrastructure.
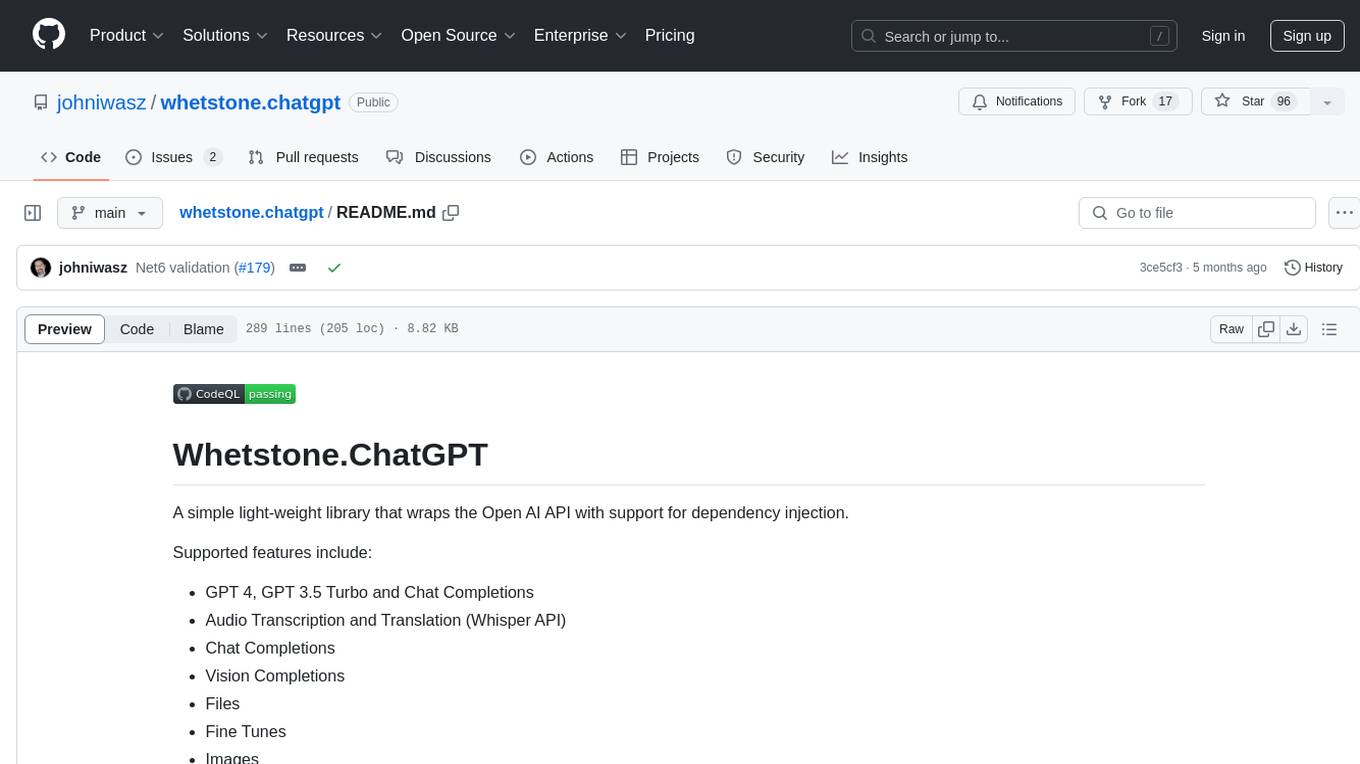
whetstone.chatgpt
Whetstone.ChatGPT is a simple light-weight library that wraps the Open AI API with support for dependency injection. It supports features like GPT 4, GPT 3.5 Turbo, chat completions, audio transcription and translation, vision completions, files, fine tunes, images, embeddings, moderations, and response streaming. The library provides a video walkthrough of a Blazor web app built on it and includes examples such as a command line bot. It offers quickstarts for dependency injection, chat completions, completions, file handling, fine tuning, image generation, and audio transcription.
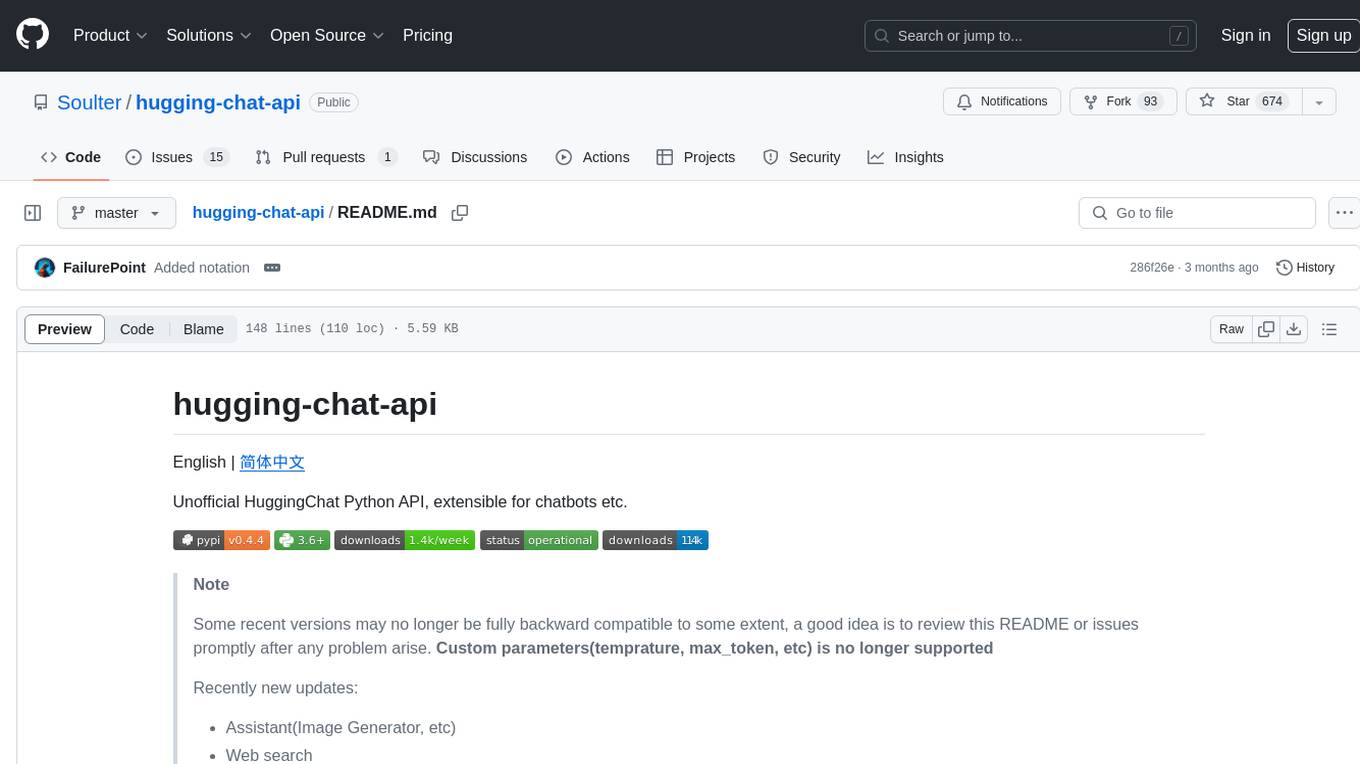
hugging-chat-api
Unofficial HuggingChat Python API for creating chatbots, supporting features like image generation, web search, memorizing context, and changing LLMs. Users can log in, chat with the ChatBot, perform web searches, create new conversations, manage conversations, switch models, get conversation info, use assistants, and delete conversations. The API also includes a CLI mode with various commands for interacting with the tool. Users are advised not to use the application for high-stakes decisions or advice and to avoid high-frequency requests to preserve server resources.
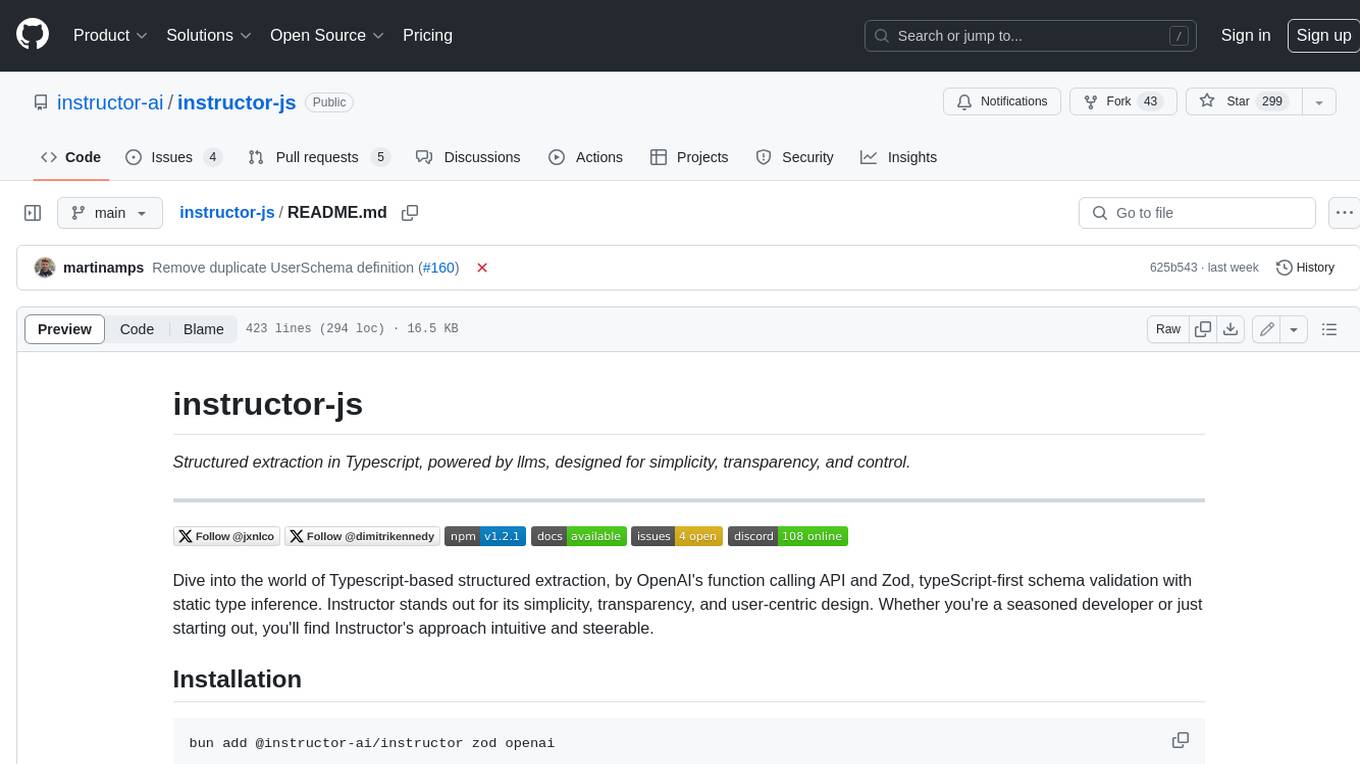
instructor-js
Instructor is a Typescript library for structured extraction in Typescript, powered by llms, designed for simplicity, transparency, and control. It stands out for its simplicity, transparency, and user-centric design. Whether you're a seasoned developer or just starting out, you'll find Instructor's approach intuitive and steerable.
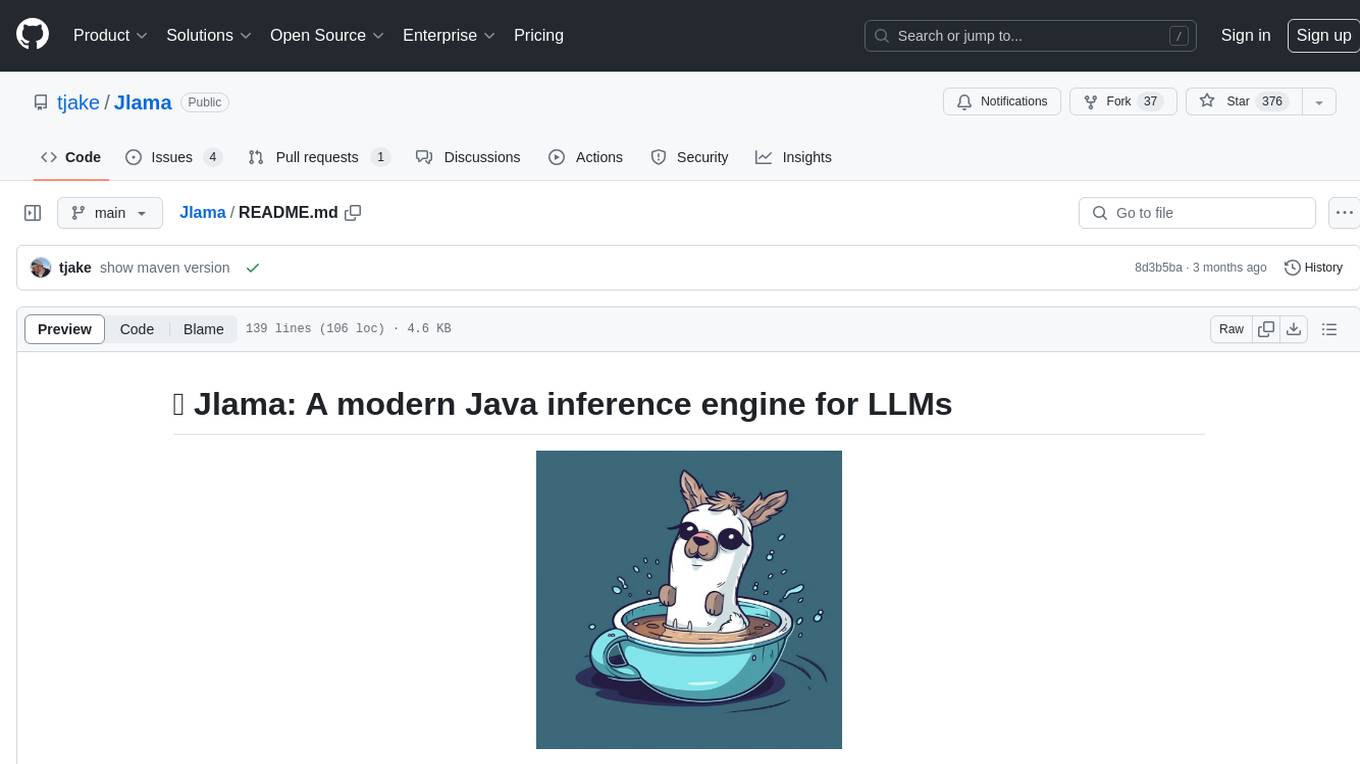
Jlama
Jlama is a modern Java inference engine designed for large language models. It supports various model types such as Gemma, Llama, Mistral, GPT-2, BERT, and more. The tool implements features like Flash Attention, Mixture of Experts, and supports different model quantization formats. Built with Java 21 and utilizing the new Vector API for faster inference, Jlama allows users to add LLM inference directly to their Java applications. The tool includes a CLI for running models, a simple UI for chatting with LLMs, and examples for different model types.
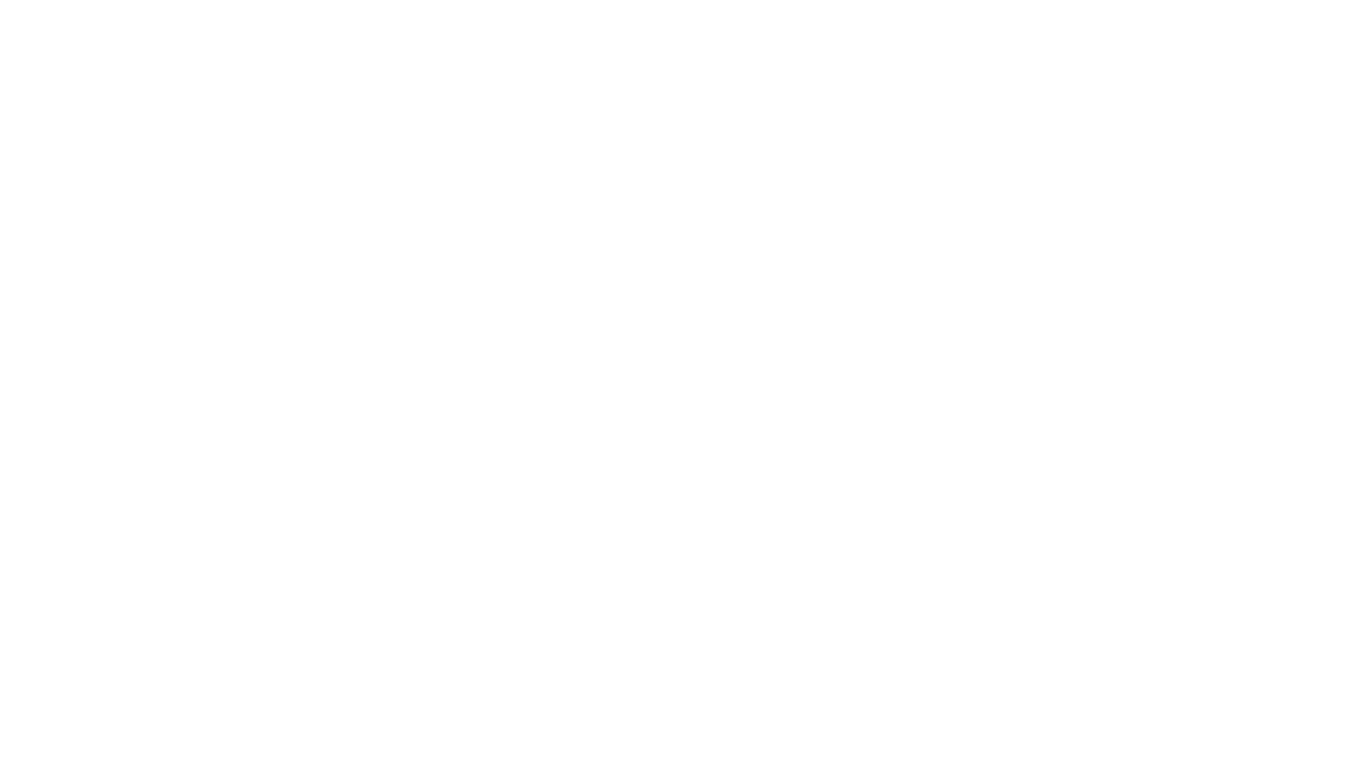
litdata
LitData is a tool designed for blazingly fast, distributed streaming of training data from any cloud storage. It allows users to transform and optimize data in cloud storage environments efficiently and intuitively, supporting various data types like images, text, video, audio, geo-spatial, and multimodal data. LitData integrates smoothly with frameworks such as LitGPT and PyTorch, enabling seamless streaming of data to multiple machines. Key features include multi-GPU/multi-node support, easy data mixing, pause & resume functionality, support for profiling, memory footprint reduction, cache size configuration, and on-prem optimizations. The tool also provides benchmarks for measuring streaming speed and conversion efficiency, along with runnable templates for different data types. LitData enables infinite cloud data processing by utilizing the Lightning.ai platform to scale data processing with optimized machines.
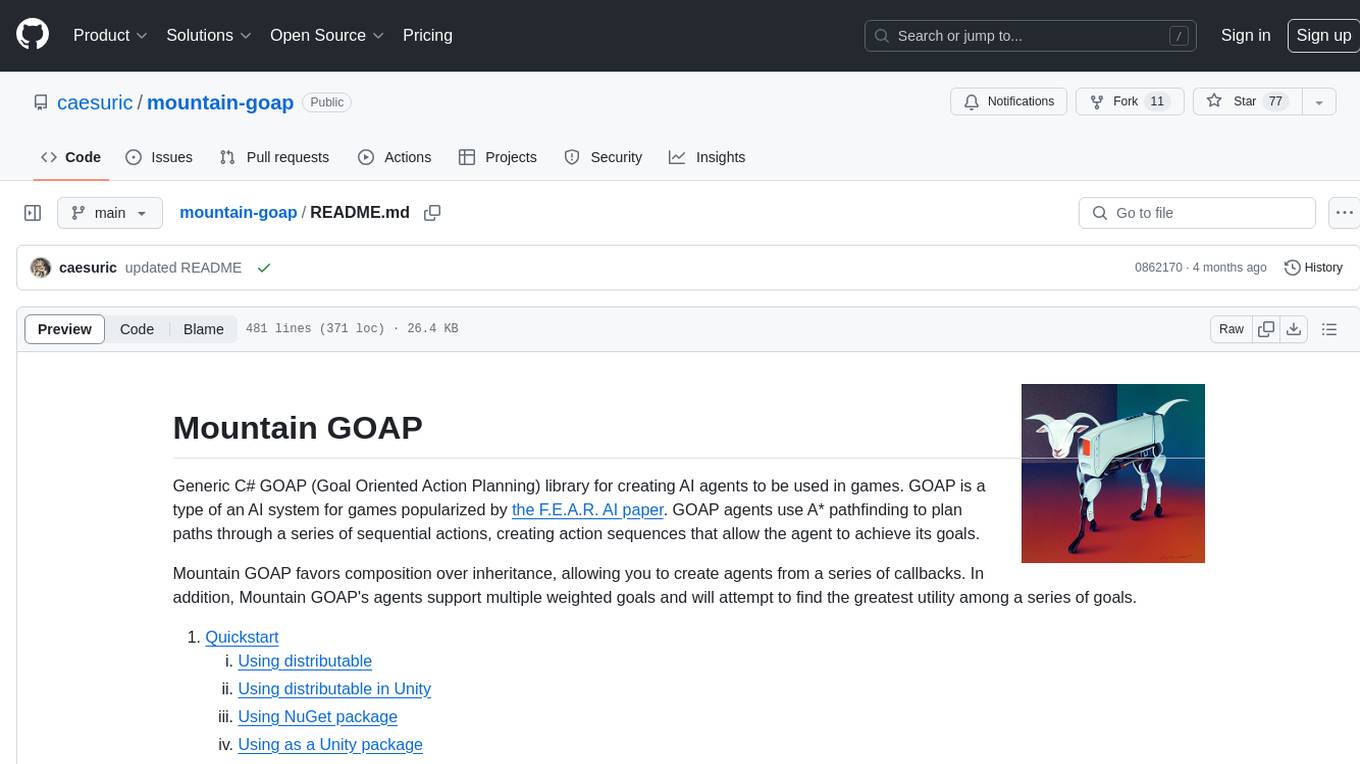
mountain-goap
Mountain GOAP is a generic C# GOAP (Goal Oriented Action Planning) library for creating AI agents in games. It favors composition over inheritance, supports multiple weighted goals, and uses A* pathfinding to plan paths through sequential actions. The library includes concepts like agents, goals, actions, sensors, permutation selectors, cost callbacks, state mutators, state checkers, and a logger. It also features event handling for agent planning and execution. The project structure includes examples, API documentation, and internal classes for planning and execution.
For similar tasks
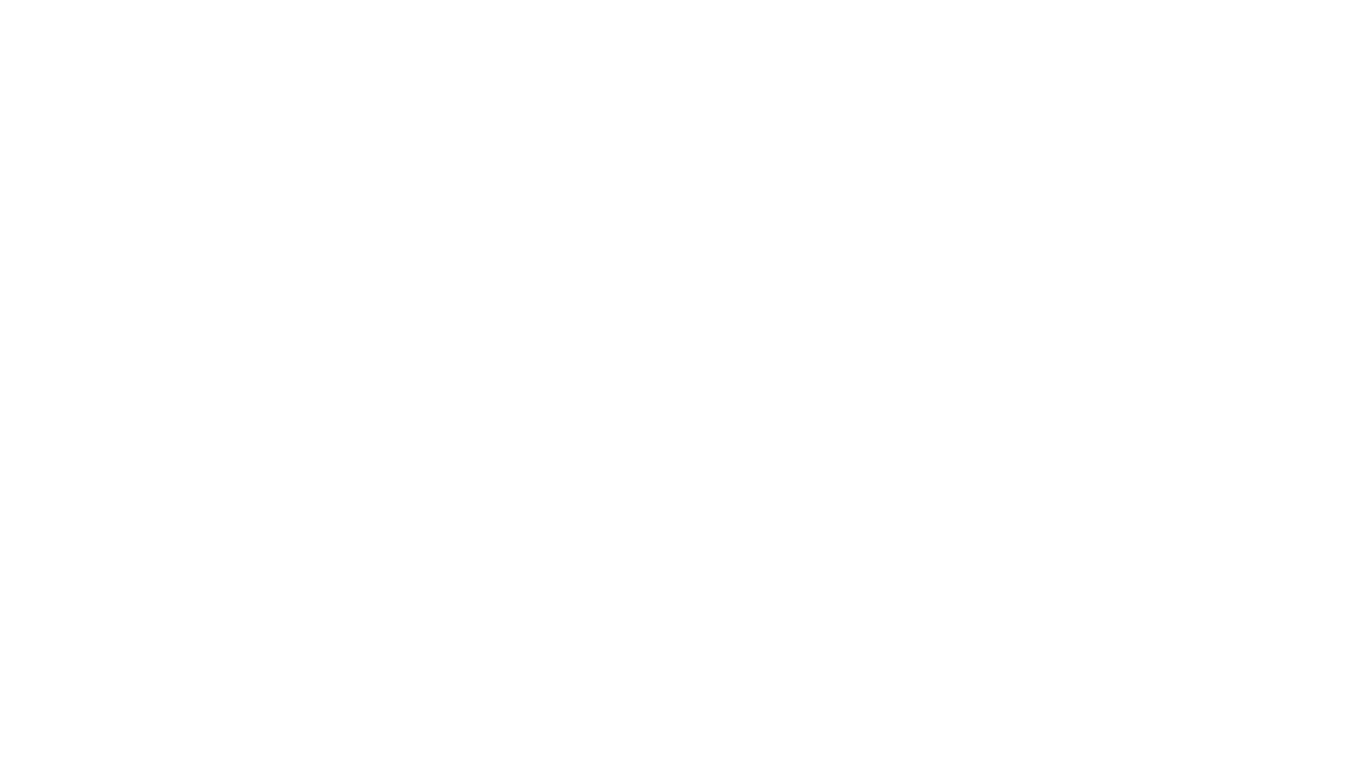
generative-ai
The 'Generative AI' repository provides a C# library for interacting with Google's Generative AI models, specifically the Gemini models. It allows users to access and integrate the Gemini API into .NET applications, supporting functionalities such as listing available models, generating content, creating tuned models, working with large files, starting chat sessions, and more. The repository also includes helper classes and enums for Gemini API aspects. Authentication methods include API key, OAuth, and various authentication modes for Google AI and Vertex AI. The package offers features for both Google AI Studio and Google Cloud Vertex AI, with detailed instructions on installation, usage, and troubleshooting.
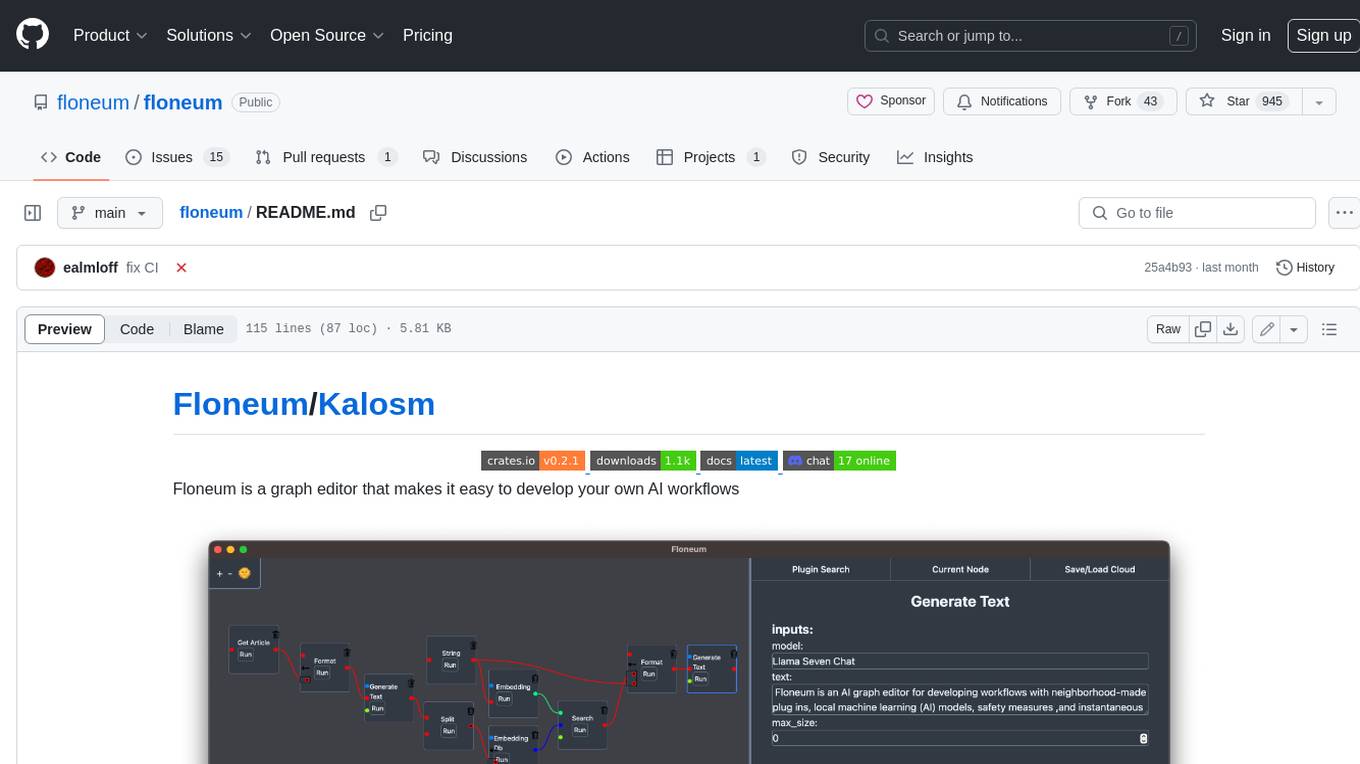
floneum
Floneum is a graph editor that makes it easy to develop your own AI workflows. It uses large language models (LLMs) to run AI models locally, without any external dependencies or even a GPU. This makes it easy to use LLMs with your own data, without worrying about privacy. Floneum also has a plugin system that allows you to improve the performance of LLMs and make them work better for your specific use case. Plugins can be used in any language that supports web assembly, and they can control the output of LLMs with a process similar to JSONformer or guidance.
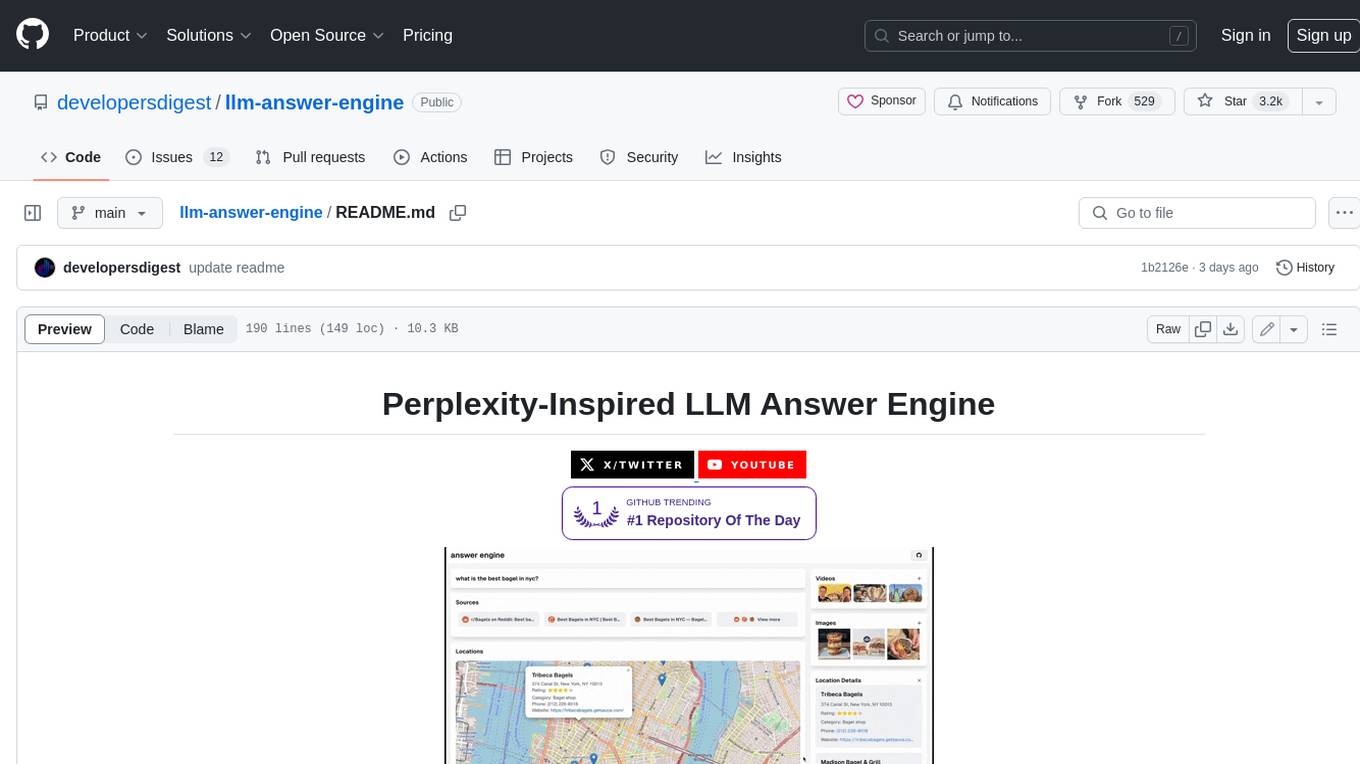
llm-answer-engine
This repository contains the code and instructions needed to build a sophisticated answer engine that leverages the capabilities of Groq, Mistral AI's Mixtral, Langchain.JS, Brave Search, Serper API, and OpenAI. Designed to efficiently return sources, answers, images, videos, and follow-up questions based on user queries, this project is an ideal starting point for developers interested in natural language processing and search technologies.
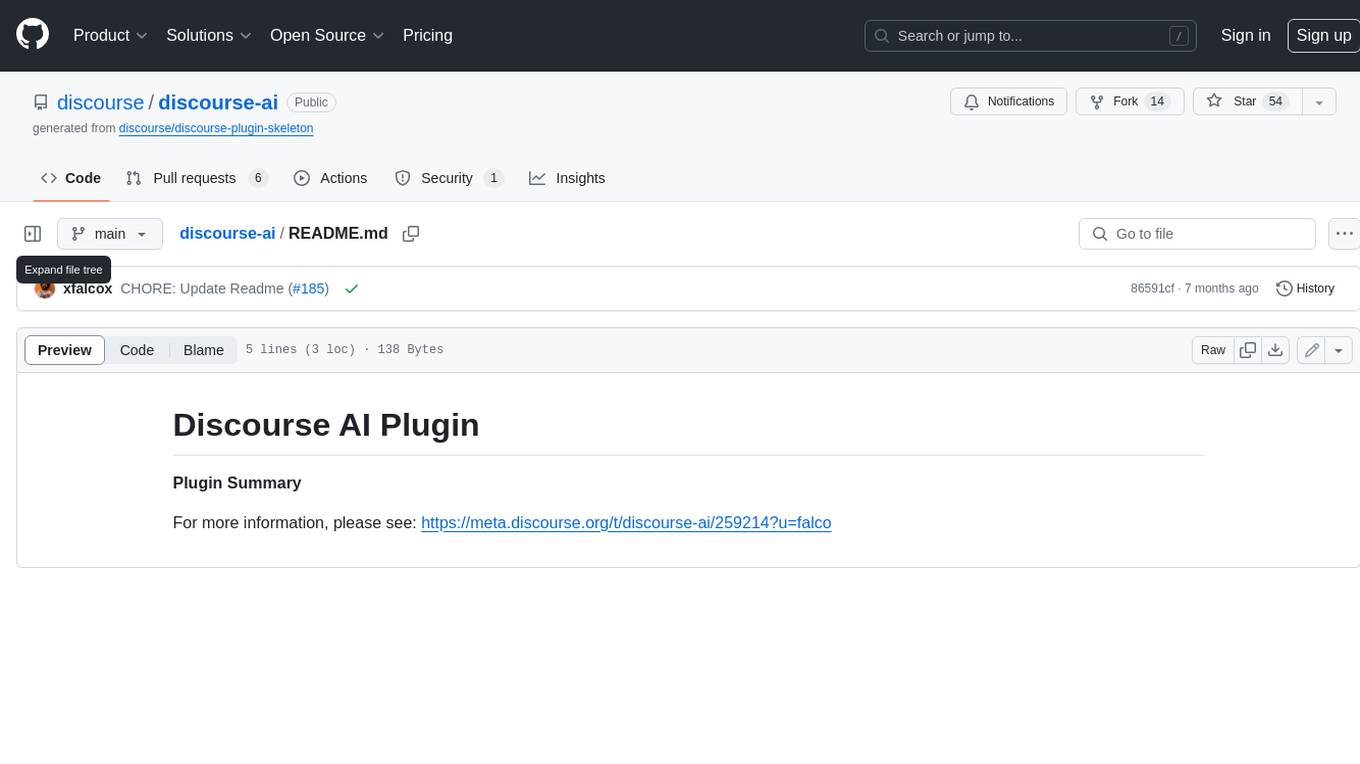
discourse-ai
Discourse AI is a plugin for the Discourse forum software that uses artificial intelligence to improve the user experience. It can automatically generate content, moderate posts, and answer questions. This can free up moderators and administrators to focus on other tasks, and it can help to create a more engaging and informative community.
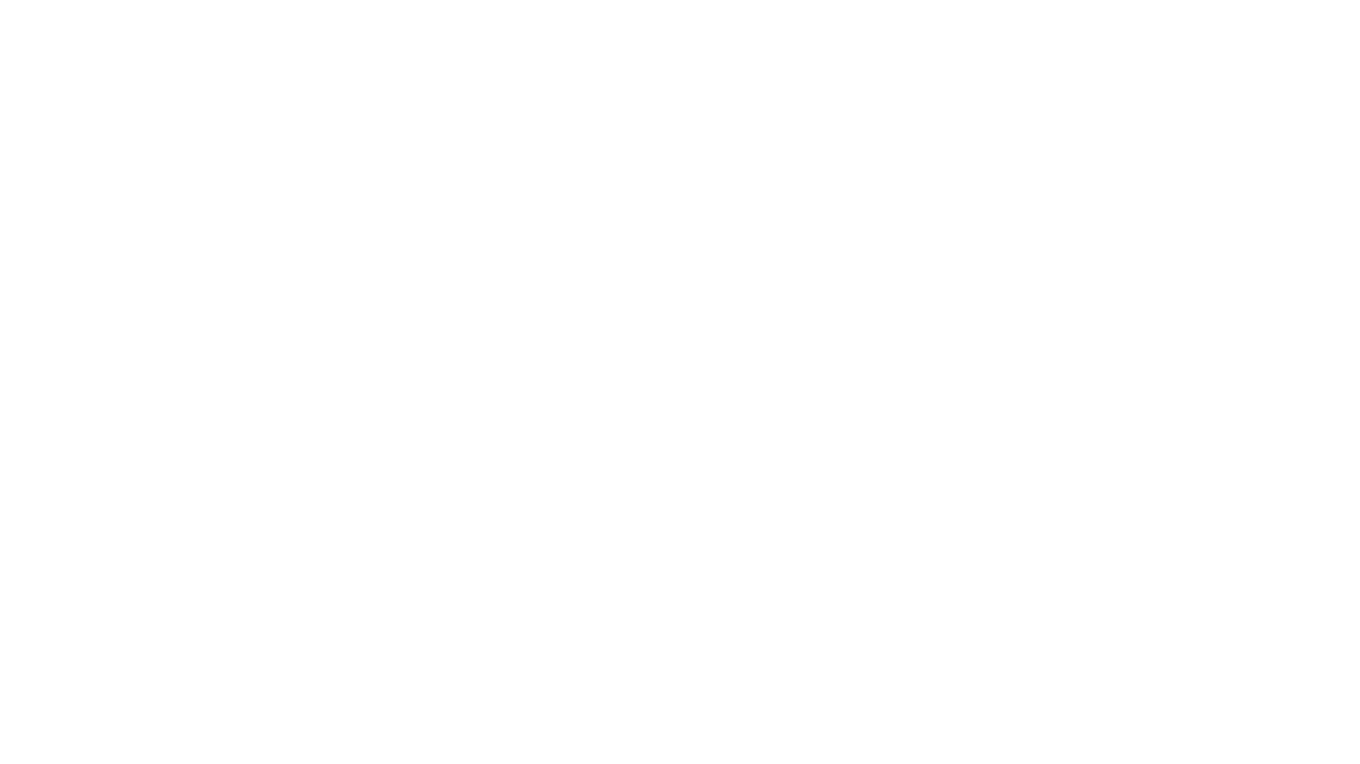
Gemini-API
Gemini-API is a reverse-engineered asynchronous Python wrapper for Google Gemini web app (formerly Bard). It provides features like persistent cookies, ImageFx support, extension support, classified outputs, official flavor, and asynchronous operation. The tool allows users to generate contents from text or images, have conversations across multiple turns, retrieve images in response, generate images with ImageFx, save images to local files, use Gemini extensions, check and switch reply candidates, and control log level.
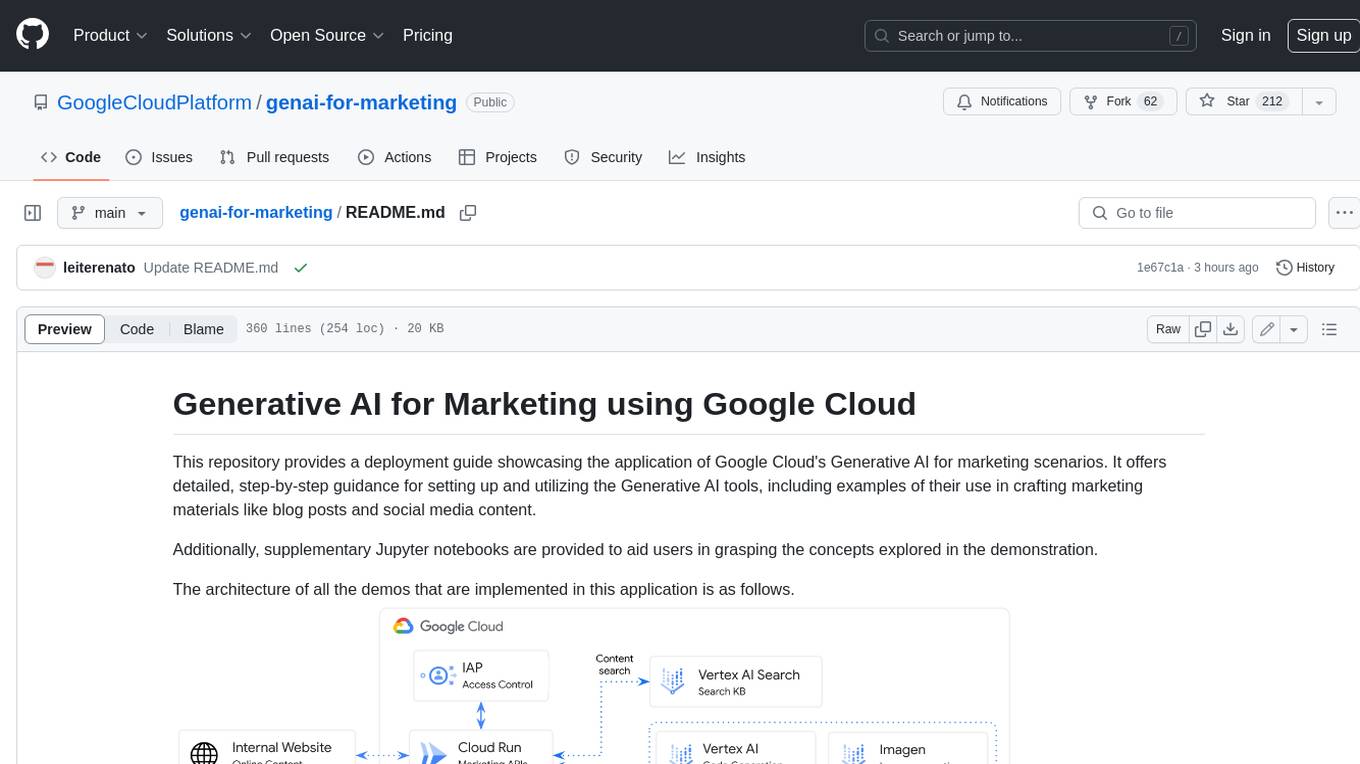
genai-for-marketing
This repository provides a deployment guide for utilizing Google Cloud's Generative AI tools in marketing scenarios. It includes step-by-step instructions, examples of crafting marketing materials, and supplementary Jupyter notebooks. The demos cover marketing insights, audience analysis, trendspotting, content search, content generation, and workspace integration. Users can access and visualize marketing data, analyze trends, improve search experience, and generate compelling content. The repository structure includes backend APIs, frontend code, sample notebooks, templates, and installation scripts.
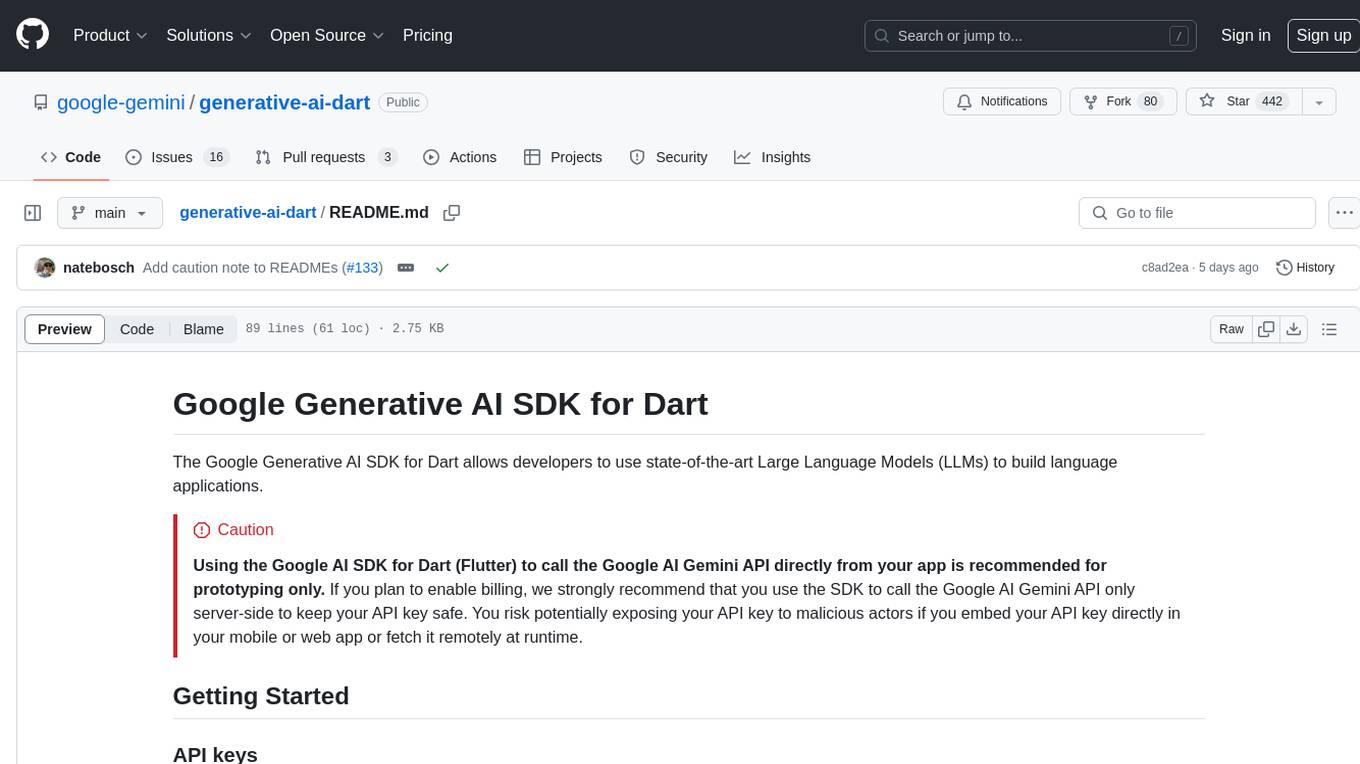
generative-ai-dart
The Google Generative AI SDK for Dart enables developers to utilize cutting-edge Large Language Models (LLMs) for creating language applications. It provides access to the Gemini API for generating content using state-of-the-art models. Developers can integrate the SDK into their Dart or Flutter applications to leverage powerful AI capabilities. It is recommended to use the SDK for server-side API calls to ensure the security of API keys and protect against potential key exposure in mobile or web apps.
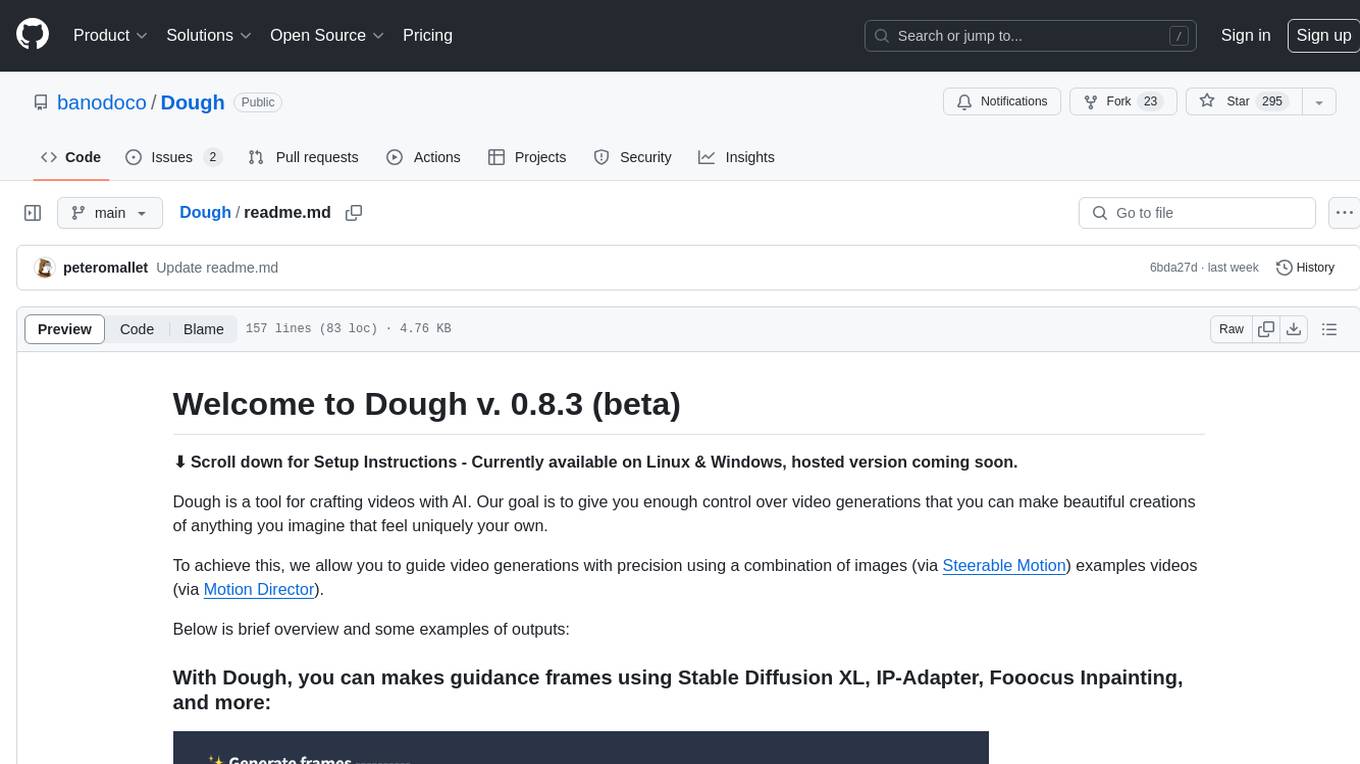
Dough
Dough is a tool for crafting videos with AI, allowing users to guide video generations with precision using images and example videos. Users can create guidance frames, assemble shots, and animate them by defining parameters and selecting guidance videos. The tool aims to help users make beautiful and unique video creations, providing control over the generation process. Setup instructions are available for Linux and Windows platforms, with detailed steps for installation and running the app.
For similar jobs
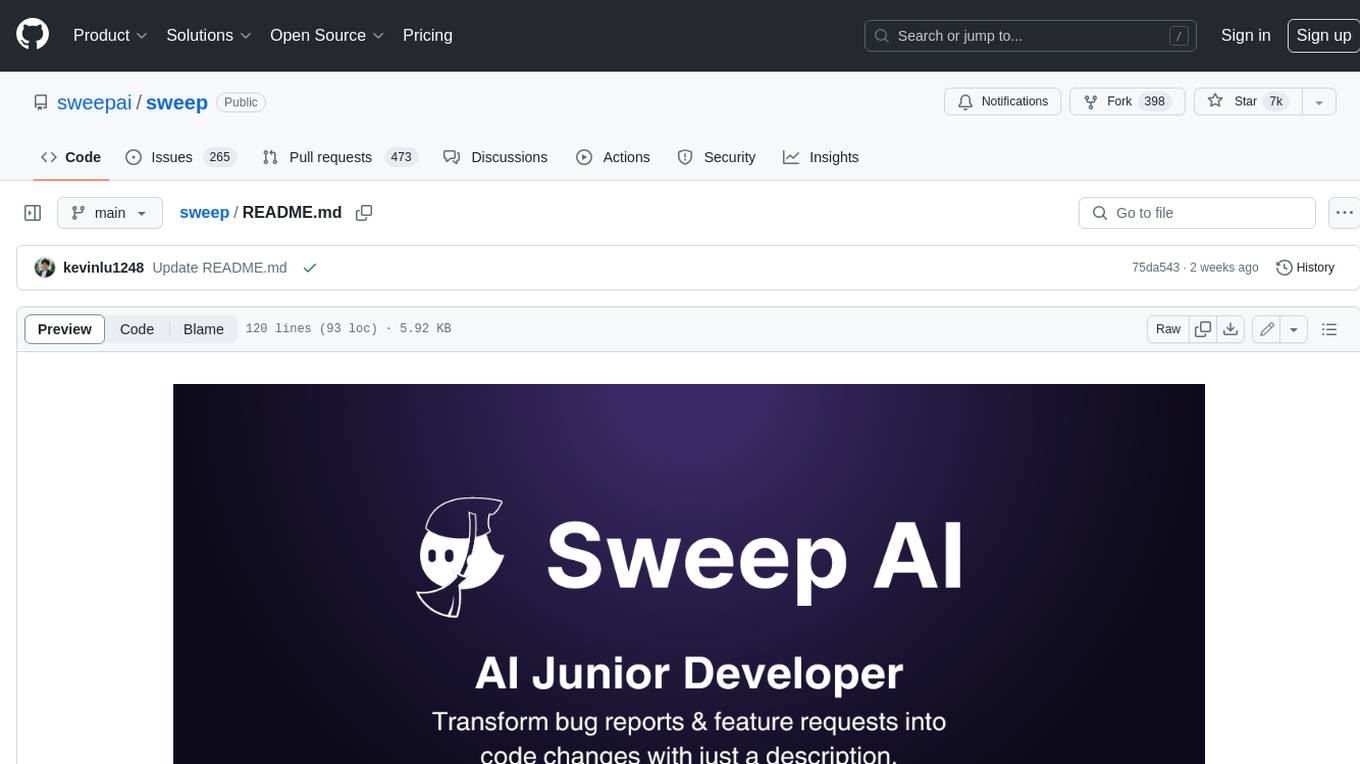
sweep
Sweep is an AI junior developer that turns bugs and feature requests into code changes. It automatically handles developer experience improvements like adding type hints and improving test coverage.
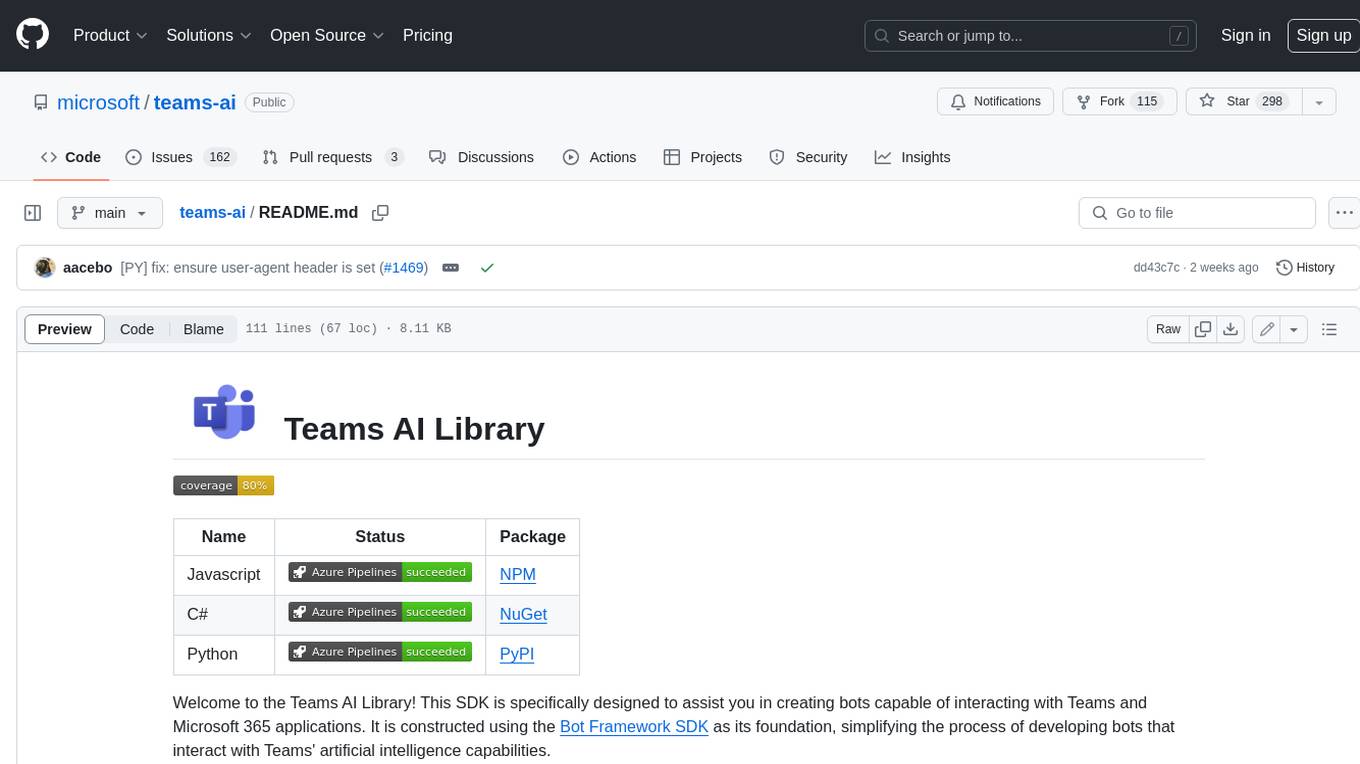
teams-ai
The Teams AI Library is a software development kit (SDK) that helps developers create bots that can interact with Teams and Microsoft 365 applications. It is built on top of the Bot Framework SDK and simplifies the process of developing bots that interact with Teams' artificial intelligence capabilities. The SDK is available for JavaScript/TypeScript, .NET, and Python.
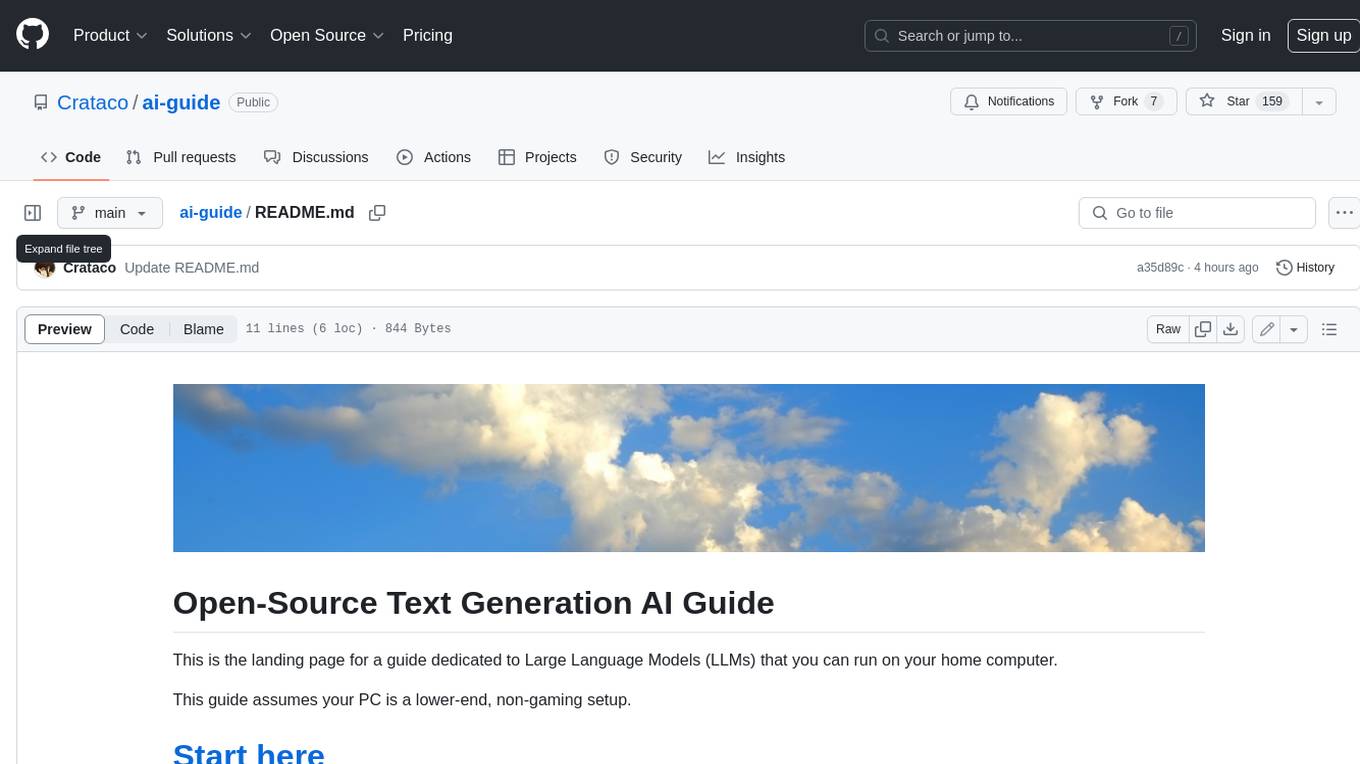
ai-guide
This guide is dedicated to Large Language Models (LLMs) that you can run on your home computer. It assumes your PC is a lower-end, non-gaming setup.
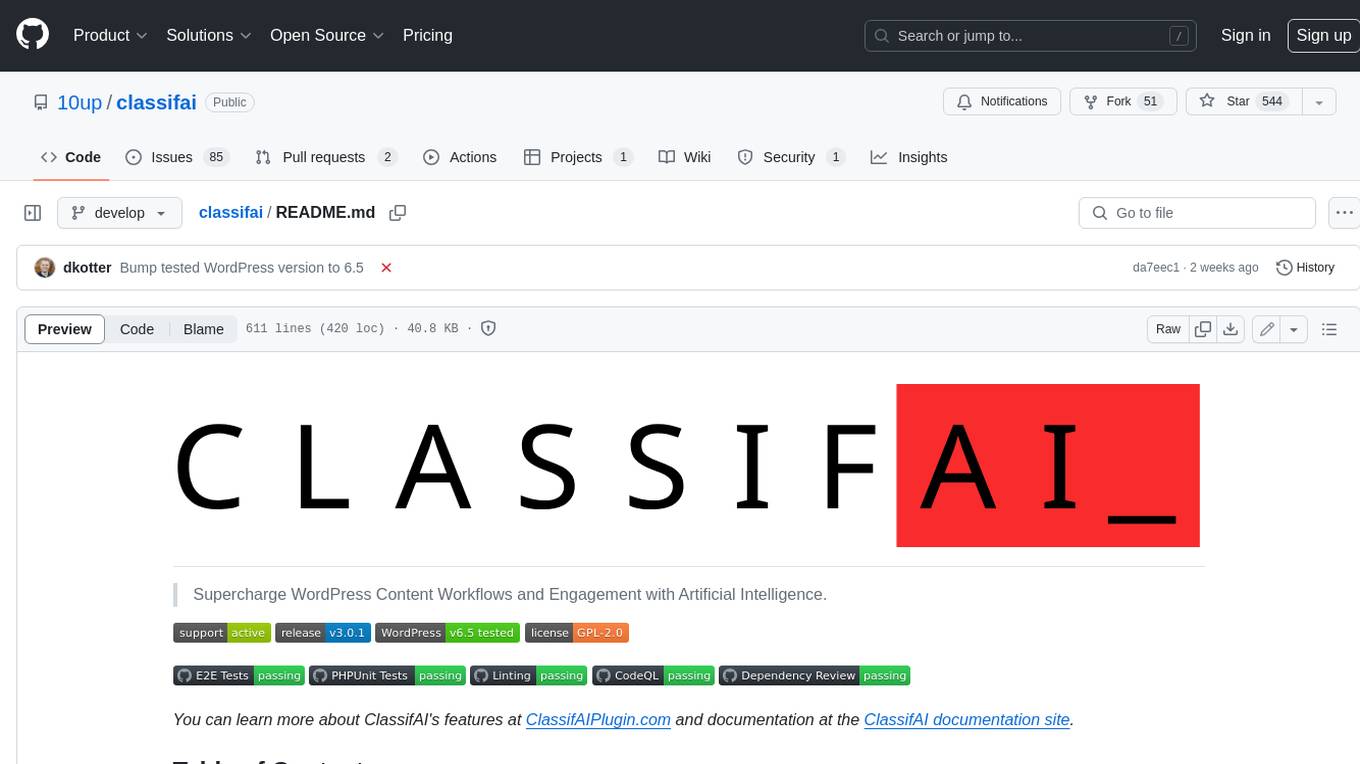
classifai
Supercharge WordPress Content Workflows and Engagement with Artificial Intelligence. Tap into leading cloud-based services like OpenAI, Microsoft Azure AI, Google Gemini and IBM Watson to augment your WordPress-powered websites. Publish content faster while improving SEO performance and increasing audience engagement. ClassifAI integrates Artificial Intelligence and Machine Learning technologies to lighten your workload and eliminate tedious tasks, giving you more time to create original content that matters.
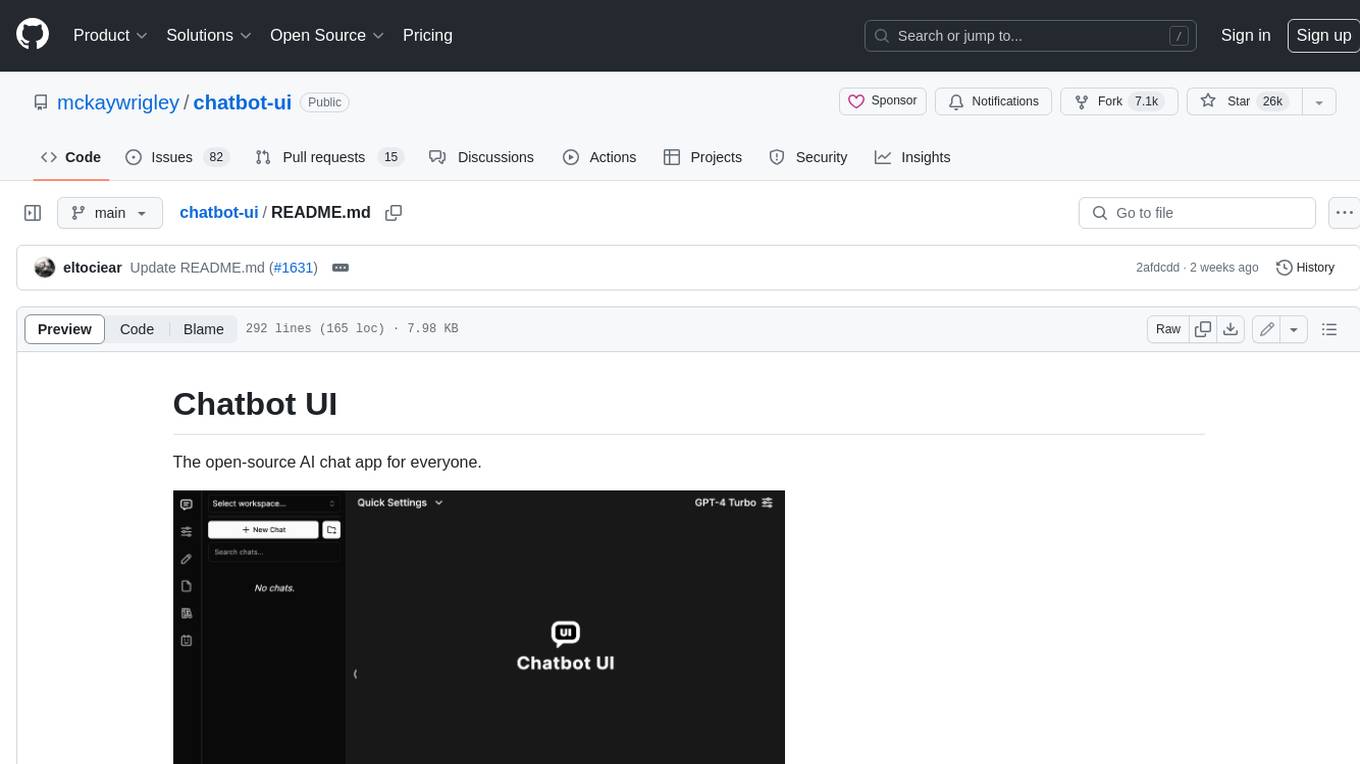
chatbot-ui
Chatbot UI is an open-source AI chat app that allows users to create and deploy their own AI chatbots. It is easy to use and can be customized to fit any need. Chatbot UI is perfect for businesses, developers, and anyone who wants to create a chatbot.
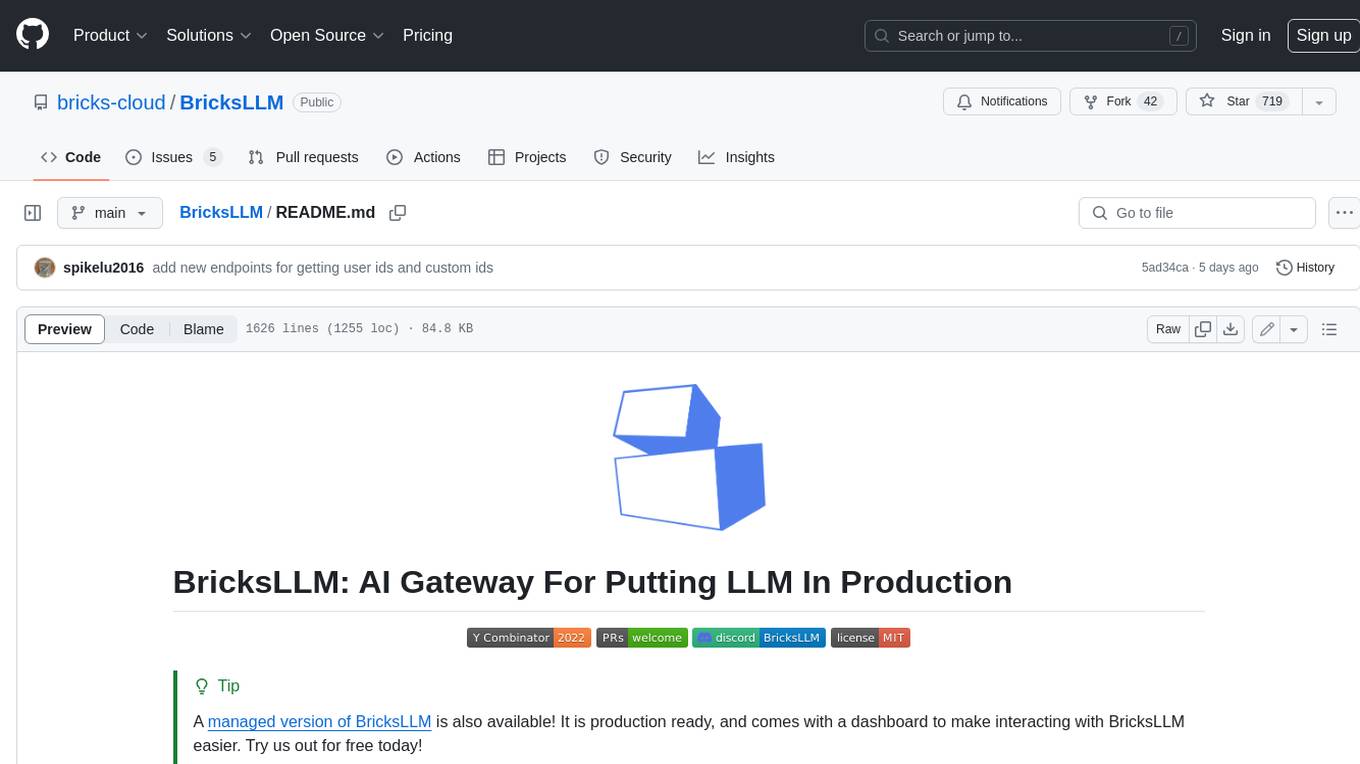
BricksLLM
BricksLLM is a cloud native AI gateway written in Go. Currently, it provides native support for OpenAI, Anthropic, Azure OpenAI and vLLM. BricksLLM aims to provide enterprise level infrastructure that can power any LLM production use cases. Here are some use cases for BricksLLM: * Set LLM usage limits for users on different pricing tiers * Track LLM usage on a per user and per organization basis * Block or redact requests containing PIIs * Improve LLM reliability with failovers, retries and caching * Distribute API keys with rate limits and cost limits for internal development/production use cases * Distribute API keys with rate limits and cost limits for students
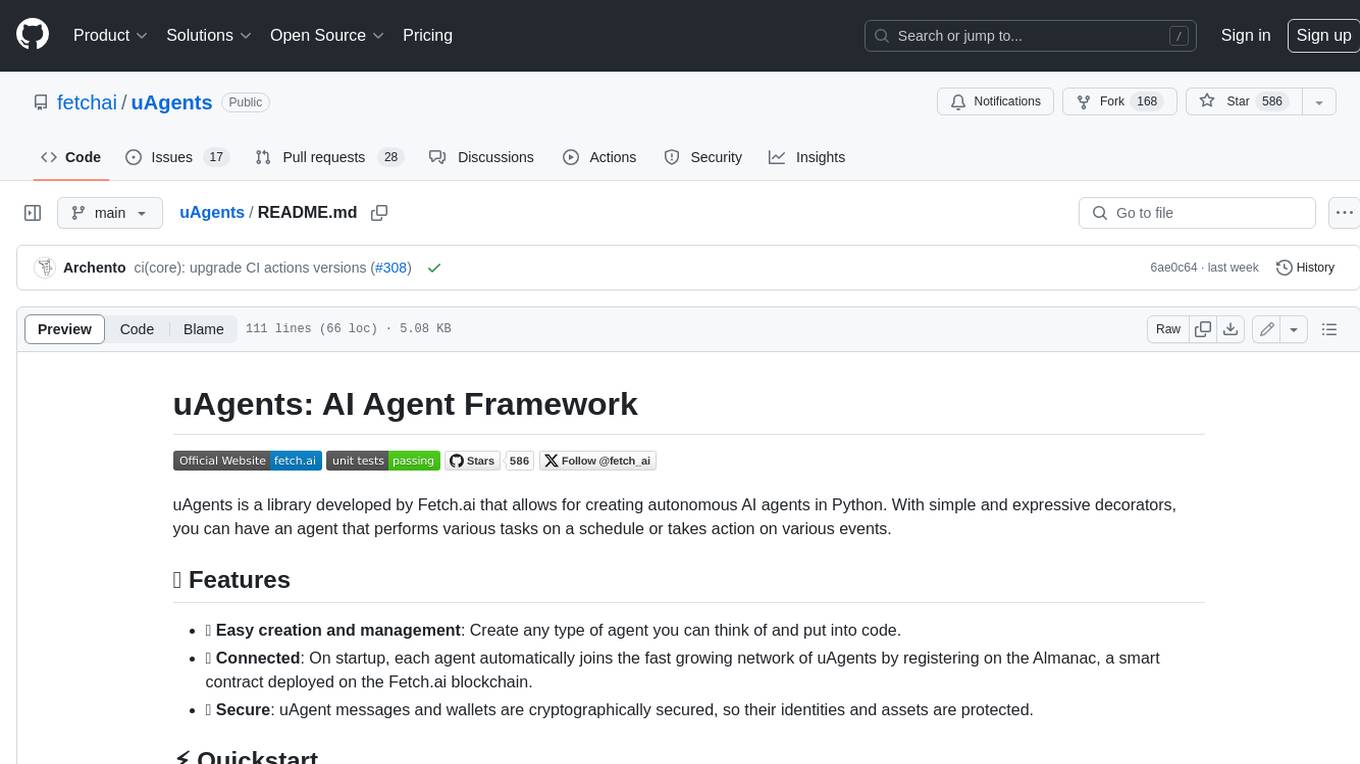
uAgents
uAgents is a Python library developed by Fetch.ai that allows for the creation of autonomous AI agents. These agents can perform various tasks on a schedule or take action on various events. uAgents are easy to create and manage, and they are connected to a fast-growing network of other uAgents. They are also secure, with cryptographically secured messages and wallets.
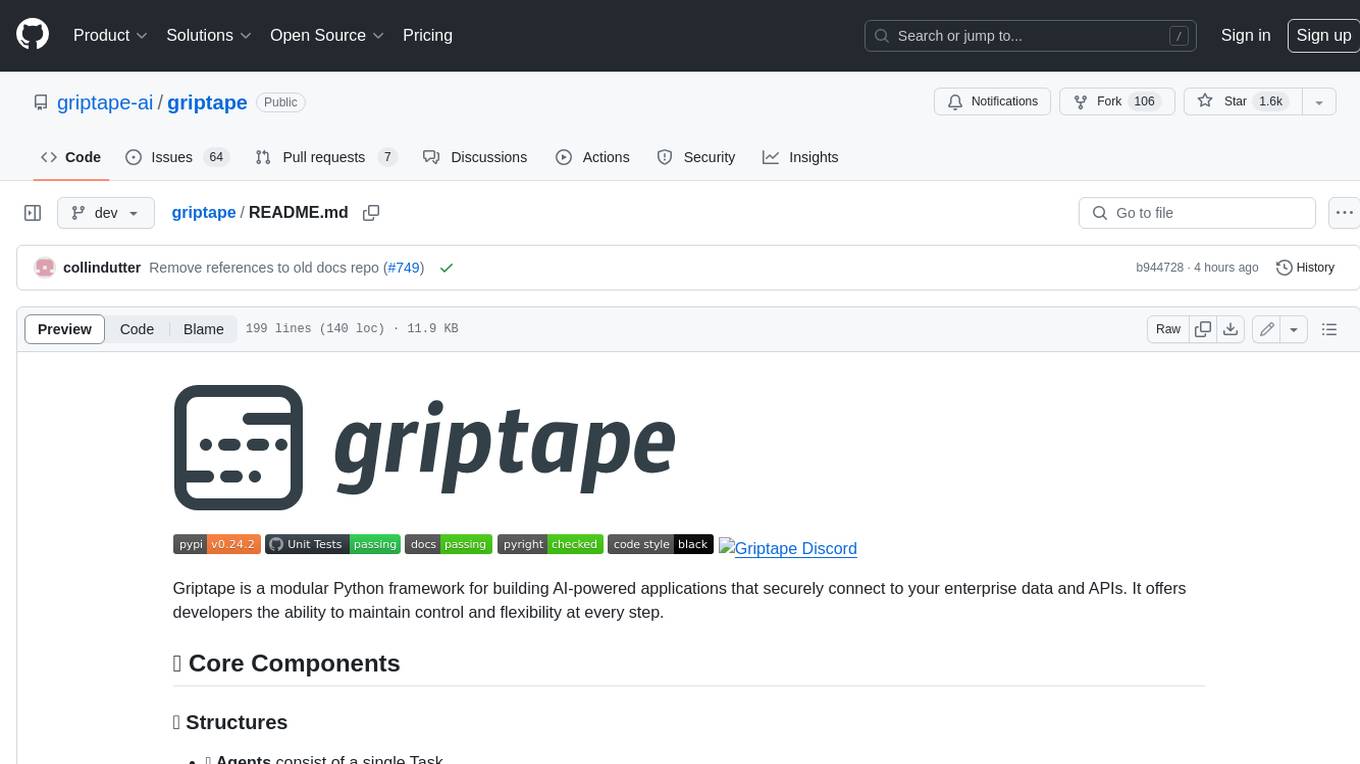
griptape
Griptape is a modular Python framework for building AI-powered applications that securely connect to your enterprise data and APIs. It offers developers the ability to maintain control and flexibility at every step. Griptape's core components include Structures (Agents, Pipelines, and Workflows), Tasks, Tools, Memory (Conversation Memory, Task Memory, and Meta Memory), Drivers (Prompt and Embedding Drivers, Vector Store Drivers, Image Generation Drivers, Image Query Drivers, SQL Drivers, Web Scraper Drivers, and Conversation Memory Drivers), Engines (Query Engines, Extraction Engines, Summary Engines, Image Generation Engines, and Image Query Engines), and additional components (Rulesets, Loaders, Artifacts, Chunkers, and Tokenizers). Griptape enables developers to create AI-powered applications with ease and efficiency.