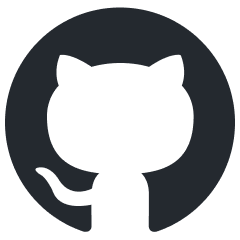
AI-Guide-and-Demos-zh_CN
这是一份入门AI/LLM大模型的逐步指南,包含教程和演示代码,带你从API走进本地大模型部署和微调,代码文件会提供Kaggle或Colab在线版本,即便没有显卡也可以进行学习。项目中还开设了一个小型的代码游乐场🎡,你可以尝试在里面实验一些有意思的AI脚本。同时,包含李宏毅 (HUNG-YI LEE)2024生成式人工智能导论课程的完整中文镜像作业。
Stars: 1576
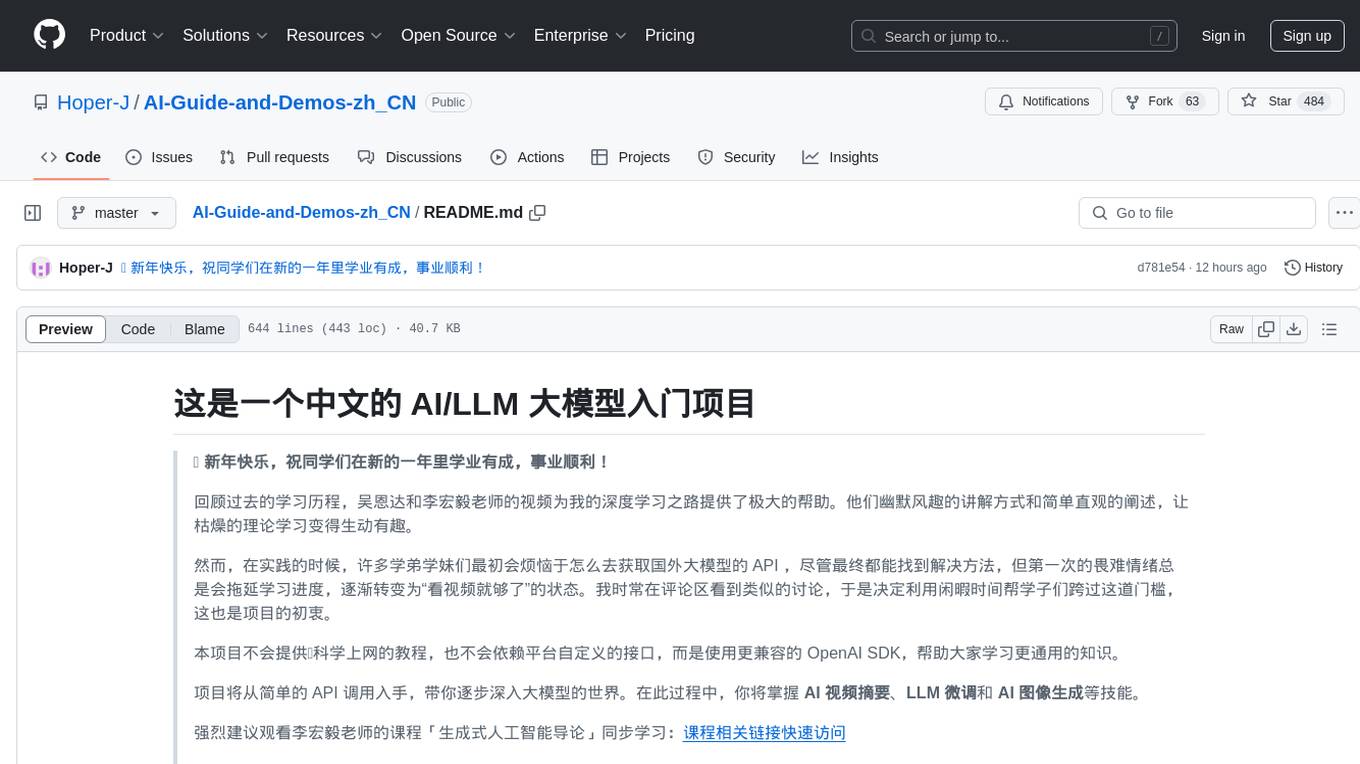
This is a Chinese AI/LLM introductory project that aims to help students overcome the initial difficulties of accessing foreign large models' APIs. The project uses the OpenAI SDK to provide a more compatible learning experience. It covers topics such as AI video summarization, LLM fine-tuning, and AI image generation. The project also offers a CodePlayground for easy setup and one-line script execution to experience the charm of AI. It includes guides on API usage, LLM configuration, building AI applications with Gradio, customizing prompts for better model performance, understanding LoRA, and more.
README:
回顾过去的学习历程,吴恩达和李宏毅老师的视频为我的深度学习之路提供了极大的帮助。他们幽默风趣的讲解方式和简单直观的阐述,让枯燥的理论学习变得生动有趣。
然而,在实践的时候,许多学弟学妹们最初会烦恼于怎么去获取国外大模型的 API ,尽管最终都能找到解决方法,但第一次的畏难情绪总是会拖延学习进度,逐渐转变为“看视频就够了”的状态。我时常在评论区看到类似的讨论,于是决定利用闲暇时间帮学子们跨过这道门槛,这也是项目的初衷。
本项目不会提供🪜科学上网的教程,也不会依赖平台自定义的接口,而是使用更兼容的 OpenAI SDK,帮助大家学习更通用的知识。
项目将从简单的 API 调用入手,带你逐步深入大模型的世界。在此过程中,你将掌握 AI 视频摘要、LLM 微调和 AI 图像生成等技能。
强烈建议观看李宏毅老师的课程「生成式人工智能导论」同步学习:课程相关链接快速访问
现在,项目还开设了🎡CodePlayground,你可以按照文档配置好环境,使用一行代码运行脚本,体验 AI 的魅力。
📑论文随笔位于 PaperNotes,将逐步上传大模型相关的基础论文。
🚀 基础镜像已经准备好,如果你还没有配置好属于自己的深度学习环境,不妨尝试一下 Docker。
祝你旅途愉快!
-
Tag 说明:
-
---
: 基础知识,根据需要进行观看,也可以暂时跳过。其中的代码文件结果都会在文章中示出,但仍建议动手运行代码。可能会有显存要求。 -
API
: 文章仅使用大模型的 API,不受设备限制,无 GPU 也可运行。- Kaggle 目前不允许使用 Gradio,故部分交互文件不提供相关链接(这一类文件可以本地运行)。
-
LLM
: 大型语言模型相关的实践,代码文件可能有显存要求。 -
SD
: Stable Diffusion,文生图相关的实践,代码文件有显存要求。
-
-
Online 在线链接说明:
- 与 Code 内容一致,如果提供了 Kaggle 和 Colab,则三选一运行。
- 如果仅提供了 Colab,说明不受显卡限制可以本地运行,此时不能科学上网的同学可以下载
File
的代码,学习效果一致。 - 运行时请不要忘记打开对应在线平台的 GPU。
- Kaggle:
Setting
->Accelerator
->选择 GPU
。 - Colab:
代码执行程序
->更改运行时类型
->选择 GPU
。
- Kaggle:
当前模块置顶,以方便大家索引更新。部分内容将直接由之前的文章(导论部分)重组得来,故存在重复,此模块将暂时专注于 DeepSeek API 的使用,是 OpenAI SDK 相关的通用知识,也可以作为导论 API 部分的拓展。
Guide | Tag | Describe | File | Online |
---|---|---|---|---|
DeepSeek API 的获取与对话示例 | API | 获取 DeepSeek API 的 N 种方法及其单轮对话样例: - DeepSeek 官方 - 硅基流动 - 阿里云百炼 - 百度智能云 - 字节火山引擎 |
Code |
Kaggle Colab |
DeepSeek 联网满血版使用指南 | API | 通过 API 绕开 DeepSeek 网页对话的卡顿,提供两种配置方案: - Cherry Studio【推荐】 - Chatbox |
||
DeepSeek API 输出解析 - OpenAI SDK | API | 关于 OpenAI SDK 的通用知识,以 DeepSeek 聊天/推理模型为例进行演示: - 认识 API 的返回字段 - 打印模型回复和每次对话的用量信息 |
Code |
Kaggle Colab |
└─流式输出解析 | API | API 解析 - 流式输出篇 - 认识 chunk 的结构 - 处理各平台聊天/推理模型的流式输出 |
Code |
Kaggle Colab |
DeepSeek API 多轮对话 - OpenAI SDK | API | DeepSeek API 的多轮对话示例 - 非流式输出篇: - 认识单轮对话和多轮对话时 messages 的差异- 尝试封装对话类 |
Code |
Kaggle Colab |
└─统一模型对话逻辑与流式输出 | API | - 统一聊天模型和推理模型对话类 - 引入流式输出处理 【代码文件】 - 使用 APIConfigManager 进行各平台配置,不再分散逻辑 |
Code |
Kaggle Colab |
Guide | Tag | Describe | File | Online |
---|---|---|---|---|
00. 大模型 API 获取步骤 | API | 带你一步步的获取 API: - 阿里(通义千问)。 - 智谱。 - DeepSeek。 |
||
01. 初识 LLM API:环境配置与多轮对话演示 | API | 这是一段入门的配置和演示,对话代码修改自开发文档。 | Code |
Kaggle Colab |
02. 简单入门:通过 API 与 Gradio 构建 AI 应用 | API | 指导如何去使用 Gradio 搭建一个简单的 AI 应用。 | Code | Colab |
03. 进阶指南:自定义 Prompt 提升大模型解题能力 | API | 你将学习自定义一个 Prompt 来提升大模型解数学题的能力,其中一样会提供 Gradio 和非 Gradio 两个版本,并展示代码细节。 | Code |
Kaggle Colab |
04. 认识 LoRA:从线性层到注意力机制 | --- | 在正式进入实践之前,你需要知道 LoRA 的基础概念,这篇文章会带你从线性层的 LoRA 实现到注意力机制。 | ||
05. 理解 Hugging Face 的 AutoModel 系列:不同任务的自动模型加载类 |
--- | 我们即将用到的模块是 Hugging Face 中的 AutoModel,这篇文章一样是一个前置知识,你将了解到如何查看模型的参数和配置信息,以及如何使用 inspect 库进一步查看对应的源码。 |
Code |
Kaggle Colab |
06. 开始实践:部署你的第一个语言模型 | LLM | 实现非常入门的语言模型部署,项目到现在为止都不会有 GPU 的硬性要求,你可以继续学习。 |
Code app_fastapi.py app_flask.py |
|
07. 探究模型参数与显存的关系以及不同精度造成的影响 | --- | 了解模型参数和显存的对应关系并掌握不同精度的导入方式会使得你对模型的选择更加称手。 | ||
08. 尝试微调 LLM:让它会写唐诗 | LLM | 这篇文章与 03. 进阶指南:自定义 Prompt 提升大模型解题能力一样,本质上是专注于“用”而非“写”,你可以像之前一样,对整体的流程有了一个了解,尝试调整超参数部分来查看对微调的影响。 | Code |
Kaggle Colab |
09. 深入理解 Beam Search:原理, 示例与代码实现 | --- | 从示例到代码演示,讲解 Beam Search 的数学原理,这应该能解决一些之前阅读的困惑,最终提供一个简单的使用 Hugging Face Transformers 库的示例(如果跳过了之前的文章的话可以尝试它)。 | Code |
Kaggle Colab |
10. Top-K vs Top-P:生成式模型中的采样策略与 Temperature 的影响 | --- | 进一步向你展示其他的生成策略。 | Code |
Kaggle Colab |
11. DPO 微调示例:根据人类偏好优化 LLM 大语言模型 | LLM | 一个使用 DPO 微调的示例。 | Code |
Kaggle Colab |
12. Inseq 特征归因:可视化解释 LLM 的输出 | LLM | 翻译和文本生成(填空)任务的可视化示例。 | Code |
Kaggle Colab |
13. 了解人工智能可能存在的偏见 | LLM | 不需要理解代码,可以当作休闲时的一次有趣探索。 | Code |
Kaggle Colab |
14. PEFT:在大模型中快速应用 LoRA | --- | 学习如何在导入模型后增加 LoRA 层。 | Code |
Kaggle Colab |
15. 用 API 实现 AI 视频摘要:动手制作属于你的 AI 视频助手 | API & LLM | 你将了解到常见的 AI 视频总结小助手背后的原理,并动手实现 AI 视频摘要。 |
Code - 完整版 Code - 精简版 🎡脚本 |
Kaggle Colab |
16. 用 LoRA 微调 Stable Diffusion:拆开炼丹炉,动手实现你的第一次 AI 绘画 | SD | 使用 LoRA 进行文生图模型的微调,现在你也能够为别人提供属于你的 LoRA 文件。 |
Code Code - 精简版 🎡 脚本 |
Kaggle Colab |
17. 浅谈 RTN 模型量化:非对称 vs 对称.md | --- | 更进一步地了解 RTN 模型量化的行为,文章以 INT8 为例进行讲解。 | Code |
Kaggle Colab |
18. 模型量化技术概述及 GGUF/GGML 文件格式解析 | --- | 这是一个概述文章,或许可以解决一些你在使用 GGUF/GGML 时的疑惑。 | ||
19a. 从加载到对话:使用 Transformers 本地运行量化 LLM 大模型(GPTQ & AWQ) 19b. 从加载到对话:使用 Llama-cpp-python 本地运行量化 LLM 大模型(GGUF) |
LLM | 你将在自己的电脑上部署一个拥有 70 亿(7B)参数的量化模型,注意,这篇文章没有显卡要求。 19 a 使用 Transformers,涉及 GPTQ 和 AWQ 格式的模型加载。 19 b 使用 Llama-cpp-python,涉及 GGUF 格式的模型加载。 另外,你还将完成本地的大模型对话交互功能。 |
Code - a Code - b 🎡脚本 |
Kaggle - a Colab - a Kaggle - b Colab - b |
20. RAG 入门实践:从文档拆分到向量数据库与问答构建 | LLM | RAG 的相关实践。 了解文本分块的递归工作原理。 |
Code |
Kaggle Colab |
21. BPE vs WordPiece:理解 Tokenizer 的工作原理与子词分割方法 | --- | Tokenizer 的基本操作。 了解常见的子词分割方法:BPE 和 WordPiece。 了解注意力掩码(Attention Mask)和词元类型 ID (Token Type IDs)。 |
Code |
Kaggle Colab |
22a. 微调 LLM:实现抽取式问答 22b. 作业 - Bert 微调抽取式问答 |
LLM | 微调预训练模型以实现下游任务:抽取式问答。 可以先尝试作业 22b 再阅读 22a,但并不强制要求。 |
BERT 论文精读 Code - 完整 Code - 作业 |
Kaggle - 完整 Colab - 完整 Kaggle - 作业 Colab - 作业 |
[!TIP]
如果你更喜欢拉取仓库到本地进行阅读
.md
,那么在出现公式报错的时候,请使用Ctrl+F
或者Command+F
,搜索\\_
并全部替换为\_
。
Guide | Describe |
---|---|
a. 使用 HFD 加快 Hugging Face 模型和数据集的下载 | 如果你觉得模型下载实在是太慢了,可以参考这篇文章进行配置。 遇到代理相关的 443 错误,也可以试着查看这篇文章。 |
b. 命令行基础指令速查(Linux/Mac适用) | 一份命令行的指令速查,基本包含当前仓库的涉及的所有指令,在感到疑惑时去查看它。 |
c. 一些问题的解决方法 | 这里会解决一些项目运行过程中可能遇到的问题。 - 如何拉取远程仓库覆盖本地的一切修改? - 怎么查看和删除 Hugging Face 下载的文件,怎么修改保存路径? - 在线平台 Kaggle/Colab 怎么开启 GPU? |
d. 如何加载 GGUF 模型(分片/Shared/Split/00001-of-0000...的解决方法) | - 了解 Transformers 关于 GGUF 的新特性。 - 使用 Transformers/Llama-cpp-python/Ollama 加载 GGUF 格式的模型文件。 - 学会合并分片的 GGUF 文件。 - 解决 LLama-cpp-python 无法 offload 的问题。 |
e. 数据增强:torchvision.transforms 常用方法解析 | - 了解常用的图像数据增强方法。 Code | Kaggle | Colab |
f. 交叉熵损失函数 nn.CrossEntropyLoss() 详解和要点提醒(PyTorch) | - 了解交叉熵损失的数学原理及 PyTorch 实现。 - 了解初次使用时需要注意的地方。 |
g. 嵌入层 nn.Embedding() 详解和要点提醒(PyTorch) | - 了解嵌入层和词嵌入的概念。 - 使用预训练模型可视化 Embedding。 Code | Kaggle | Colab |
h. 使用 Docker 快速配置深度学习环境(Linux) h. Docker 基础命令介绍和常见报错解决 |
- 使用两行命令配置好深度学习环境 - Docker 基础命令介绍 - 解决使用时的三个常见报错 |
i. Epoch、Batch 和 Step 之间的关系以及梯度累积 | 基础文章,可以在任意时候进行阅读 - Epoch、Batch、Step 三者之间的关系 - SGD、BGD、MBGD 方法的区别 - 梯度累积的使用 |
文件夹解释:
-
Demos
所有的代码文件都将存放在其中。
-
data
存放代码中可能用到的小型数据,不需要关注这个文件夹。
-
-
GenAI_PDF
这里是【生成式人工智能导论】课程的作业PDF文件,我上传了它们,因为其最初保存在 Google Drive 中。
-
Guide
所有的指导文件都将存放在其中。
-
assets
这里是 .md 文件用到的图片,不需要关注这个文件夹。
-
-
PaperNotes
论文随笔。
-
README.md
- 目录索引。
-
对比学习论文随笔 1:正负样本
- 涉及使用正负样本思想且优化目标一致的基础论文
- Transformer 论文精读
-
BERT 论文精读
- 预训练任务 MLM 和 NSP
- BERT 模型的输入和输出,以及一些与 Transformer 不同的地方
- 以 $\text{BERT}_\text{BASE}$ 为例,计算模型的总参数量
- 作业 - BERT 微调抽取式问答
- GPT 论文精读
-
README.md
生成式人工智能导论学习资源
中文镜像版的制作与分享已经获得李宏毅老师的授权,感谢老师对于知识的无私分享!
- HW1,2不涉及代码相关知识,你可以通过访问对应的作业PDF来了解其中的内容:HW1 | HW2。
- HW3: 引导文章 | 代码中文镜像 | 中文 Colab | 英文 Colab | 作业PDF
- HW4: 引导文章 | 代码中文镜像 | 中文 Colab | 英文 Colab | Kaggle | 作业PDF
- HW5: 引导文章 | 代码中文镜像 | 中文 Colab | 英文 Colab | Kaggle | 作业PDF
- HW6: 引导文章 | 代码中文镜像 | 中文 Colab | 英文 Colab | Kaggle | 作业PDF
- HW7: 引导文章 | 代码中文镜像 | 中文 Colab | 英文 Colab | Kaggle | 作业PDF
- HW8: 引导文章 | 代码中文镜像 | 中文 Colab | 英文 Colab | Kaggle | 作业PDF
- HW9: 引导文章 | 代码中文镜像 | 中文 Colab | 英文 Colab | Kaggle | 作业PDF
- HW10: 引导文章 | 代码中文镜像 | 中文 Colab | 英文 Colab | Kaggle | 作业PDF
P.S. 中文镜像将完全实现作业代码的所有功能(本地运行),Kaggle 是国内可直连的在线平台,中文 Colab 和 Kaggle 内容一致,英文 Colab 链接对应于原作业,选择其中一个完成学习即可。
根据实际需求,从下方选择一种方式来准备学习环境,点击 ►
或文字展开。
如果倾向于使用在线平台学习,或者受到显卡性能的限制,可以选择以下平台:
-
Kaggle(国内直连,推荐):阅读文章《Kaggle:免费 GPU 使用指南,Colab 的理想替代方案》进行了解。
-
Colab(需要🪜科学上网)
项目中的代码文件在两个平台是同步的。
安装基础软件
- Git:用于克隆代码仓库。
- Wget 和 Curl:用于下载脚本和文件。
- Conda:用于创建和管理虚拟环境。
- pip:用于安装 Python 依赖包。
-
Linux (Ubuntu):
sudo apt-get update sudo apt-get install git
-
macOS:
-
先安装 Homebrew:
/bin/bash -c "$(curl -fsSL https://raw.githubusercontent.com/Homebrew/install/HEAD/install.sh)"
然后运行:
brew install git
-
-
Windows:
从 Git for Windows 下载并安装。
-
Linux (Ubuntu):
sudo apt-get update sudo apt-get install wget curl
-
macOS:
brew install wget curl
-
Windows:
从 Wget for Windows 和 Curl 官方网站 下载并安装。
访问 Anaconda 官方网站,输入邮箱地址后检查邮箱,你应该能看到:
点击 Download Now
,选择合适的版本并下载(Anaconda 和 Miniconda 都可以):
-
Linux (Ubuntu):
-
安装 Anaconda
访问 repo.anaconda.com 进行版本选择。
# 下载 Anaconda 安装脚本(以最新版本为例) wget https://repo.anaconda.com/archive/Anaconda3-2024.10-1-Linux-x86_64.sh # 运行安装脚本 bash Anaconda3-2024.10-1-Linux-x86_64.sh # 按照提示完成安装(先回车,空格一直翻页,翻到最后输入 yes,回车) # 安装完成后,刷新环境变量或者重新打开终端 source ~/.bashrc
-
安装 Miniconda(推荐)
访问 repo.anaconda.com/miniconda 进行版本选择。Miniconda 是一个精简版的 Anaconda,只包含 Conda 和 Python。
# 下载 Miniconda 安装脚本 wget https://repo.anaconda.com/miniconda/Miniconda3-latest-Linux-x86_64.sh # 运行安装脚本 bash Miniconda3-latest-Linux-x86_64.sh # 按照提示完成安装(先回车,空格一直翻页,翻到最后输入 yes,回车) # 安装完成后,刷新环境变量或者重新打开终端 source ~/.bashrc
-
-
macOS:
对应替换 Linux 命令中的网址。
-
安装 Anaconda
访问 repo.anaconda.com 进行版本选择。
-
安装 Miniconda(推荐)
访问 repo.anaconda.com/miniconda 进行版本选择。
-
在终端中输入以下命令,如果显示版本信息,则说明安装成功。
conda --version
cat <<'EOF' > ~/.condarc
channels:
- defaults
show_channel_urls: true
default_channels:
- https://mirror.nju.edu.cn/anaconda/pkgs/main
- https://mirror.nju.edu.cn/anaconda/pkgs/r
- https://mirror.nju.edu.cn/anaconda/pkgs/msys2
custom_channels:
conda-forge: https://mirror.nju.edu.cn/anaconda/cloud
pytorch: https://mirror.nju.edu.cn/anaconda/cloud
EOF
[!note]
很多去年可用的镜像源已经不可用,目前其余镜像站配置可以参考南大这个非常 nice 的文档:镜像使用帮助。
注意:如果已经安装了 Anaconda 或 Miniconda,系统中会包含 pip
,无需额外安装。
-
Linux (Ubuntu):
sudo apt-get update sudo apt-get install python3-pip
-
macOS:
brew install python3
-
Windows:
-
下载并安装 Python,确保勾选“Add Python to PATH”选项。
-
打开命令提示符,输入:
python -m ensurepip --upgrade
-
在终端中输入以下命令,如果显示版本信息,则说明安装成功。
pip --version
pip config set global.index-url https://mirrors.aliyun.com/pypi/simple
通过以下命令拉取项目:
git clone https://github.com/Hoper-J/AI-Guide-and-Demos-zh_CN.git
cd AI-Guide-and-Demos-zh_CN
版本不限制,可以更高:
conda create -n aigc python=3.9
按y
回车以继续,等创建完成后,激活虚拟环境:
conda activate aigc
接下来需要进行基础的依赖安装,参考 PyTorch 官网,以 CUDA 11.8 为例(如果显卡不支持11.8,需要更换命令),二选一进行安装:
# pip
pip3 install torch torchvision torchaudio --index-url https://download.pytorch.org/whl/cu118
# conda
conda install pytorch torchvision torchaudio pytorch-cuda=11.8 -c pytorch -c nvidia
现在我们成功配置好了所有需要的环境,准备开始学习 :) 其余依赖在每个文章中会单独列出。
[!note]
Docker 镜像已经预装了依赖,不用重新安装。
先安装 jupyter-lab
,这比 jupyter notebook
好用很多。
pip install jupyterlab
安装完成后,执行下面的命令:
jupyter-lab
现在你将可以通过弹出的链接进行访问,一般位于 8888 端口。对于图形化界面,Windows/Linux 摁住 Ctrl
,mac 按住 Command
,然后点击链接可以直接跳转。至此,你将获得项目的全貌:
没有安装 Docker 的同学可以阅读文章《使用 Docker 快速配置深度学习环境(Linux)》,建议初学者阅读《Docker 基础命令介绍和常见报错解决》。
所有版本都预装了 sudo
、pip
、conda
、wget
、curl
和 vim
等常用工具,且已经配置好 pip
和 conda
的国内镜像源。同时,集成了 zsh
和一些实用的命令行插件(命令自动补全、语法高亮、以及目录跳转工具 z
)。此外,已预装 jupyter notebook
和 jupyter lab
,设置了其中的默认终端为 zsh
,方便进行深度学习开发,并优化了容器内的中文显示,避免出现乱码问题。其中还预配置了 Hugging Face 的国内镜像地址。
-
base 版本:占用约 16GB 存储空间,基于
pytorch/pytorch:2.5.1-cuda11.8-cudnn9-devel
,默认python
版本为 3.11.10,可以通过conda install python==版本号
直接修改版本。 - dl 版本:占用约 20GB 存储空间,在 base 基础上,额外安装了深度学习框架和常用工具,具体查看安装清单。
base
基础环境:
- python 3.11.10
- torch 2.5.1 + cuda 11.8 + cudnn 9
Apt 安装:
-
wget
、curl
:命令行下载工具 -
vim
、nano
:文本编辑器 -
git
:版本控制工具 -
git-lfs
:Git LFS(大文件存储) -
zip
、unzip
:文件压缩和解压工具 -
htop
:系统监控工具 -
tmux
、screen
:会话管理工具 -
build-essential
:编译工具(如gcc
、g++
) -
iputils-ping
、iproute2
、net-tools
:网络工具(提供ping
、ip
、ifconfig
、netstat
等命令) -
ssh
:远程连接工具 -
rsync
:文件同步工具 -
tree
:显示文件和目录树 -
lsof
:查看当前系统打开的文件 -
aria2
:多线程下载工具 -
libssl-dev
:OpenSSL 开发库
pip 安装:
-
jupyter notebook
、jupyter lab
:交互式开发环境 -
virtualenv
:Python 虚拟环境管理工具,可以直接用 conda -
tensorboard
:深度学习训练可视化工具 -
ipywidgets
:Jupyter 小部件库,用以正确显示进度条
插件:
-
zsh-autosuggestions
:命令自动补全 -
zsh-syntax-highlighting
:语法高亮 -
z
:快速跳转目录
dl
dl(Deep Learning)版本在 base 基础上,额外安装了深度学习可能用到的基础工具和库:
Apt 安装:
-
ffmpeg
:音视频处理工具 -
libgl1-mesa-glx
:图形库依赖(解决一些深度学习框架图形相关问题)
pip 安装:
-
数据科学库:
-
numpy
、scipy
:数值计算和科学计算 -
pandas
:数据分析 -
matplotlib
、seaborn
:数据可视化 -
scikit-learn
:机器学习工具
-
-
深度学习框架:
-
tensorflow
、tensorflow-addons
:另一种流行的深度学习框架 -
tf-keras
:Keras 接口的 TensorFlow 实现
-
-
NLP 相关库:
-
transformers
、datasets
:Hugging Face 提供的 NLP 工具 -
nltk
、spacy
:自然语言处理工具
-
如果需要额外的库,可以通过以下命令手动安装:
pip install --timeout 120 <替换成库名>
这里 --timeout 120
设置了 120 秒的超时时间,确保在网络不佳的情况下仍然有足够的时间进行安装。如果不进行设置,在国内的环境下可能会遇到安装包因下载超时而失败的情况。
注意,所有镜像都不会提前拉取仓库。
假设你已经安装并配置好了 Docker,那么只需两行命令即可完成深度学习的环境配置,对于当前项目,你可以查看完版本说明后进行选择,二者对应的 image_name:tag
如下:
-
base:
hoperj/quickstart:base-torch2.5.1-cuda11.8-cudnn9-devel
-
dl:
hoperj/quickstart:dl-torch2.5.1-cuda11.8-cudnn9-devel
拉取命令为:
docker pull <image_name:tag>
下面以 dl 版为例进行命令演示,选择其中一种方式完成。
docker pull dockerpull.org/hoperj/quickstart:dl-torch2.5.1-cuda11.8-cudnn9-devel
docker pull hoperj/quickstart:dl-torch2.5.1-cuda11.8-cudnn9-devel
可以通过百度云盘下载文件(阿里云盘不支持分享大的压缩文件)。
同名文件内容相同,
.tar.gz
为压缩版本,下载后通过以下命令解压:gzip -d dl.tar.gz
假设 dl.tar
被下载到了 ~/Downloads
中,那么切换至对应目录:
cd ~/Downloads
然后加载镜像:
docker load -i dl.tar
此模式下,容器会直接使用主机的网络配置,所有端口都等同于主机的端口,无需单独映射。如果只需映射指定端口,将
--network host
替换为-p port:port
。
docker run --gpus all -it --name ai --network host hoperj/quickstart:dl-torch2.5.1-cuda11.8-cudnn9-devel /bin/zsh
对于需要使用代理的同学,增加 -e
来设置环境变量,也可以参考拓展文章a:
假设代理的 HTTP/HTTPS 端口号为 7890, SOCKS5 为 7891:
-e http_proxy=http://127.0.0.1:7890
-e https_proxy=http://127.0.0.1:7890
-e all_proxy=socks5://127.0.0.1:7891
融入到之前的命令中:
docker run --gpus all -it \
--name ai \
--network host \
-e http_proxy=http://127.0.0.1:7890 \
-e https_proxy=http://127.0.0.1:7890 \
-e all_proxy=socks5://127.0.0.1:7891 \
hoperj/quickstart:dl-torch2.5.1-cuda11.8-cudnn9-devel \
/bin/zsh
[!tip]
常用操作提前看:
- 启动容器:
docker start <容器名>
- 运行容器:
docker exec -it <容器名> /bin/zsh
- 容器内退出:
Ctrl + D
或exit
。- 停止容器:
docker stop <容器名>
- 删除容器:
docker rm <容器名>
git clone https://github.com/Hoper-J/AI-Guide-and-Demos-zh_CN.git
cd AI-Guide-and-Demos-zh_CN
jupyter lab --ip=0.0.0.0 --port=8888 --no-browser --allow-root
对于图形化界面,Windows/Linux 摁住 Ctrl
,mac 按住 Command
,然后点击链接可以直接跳转。
感谢你的STAR🌟,希望这一切对你有所帮助。
For Tasks:
Click tags to check more tools for each tasksFor Jobs:
Alternative AI tools for AI-Guide-and-Demos-zh_CN
Similar Open Source Tools
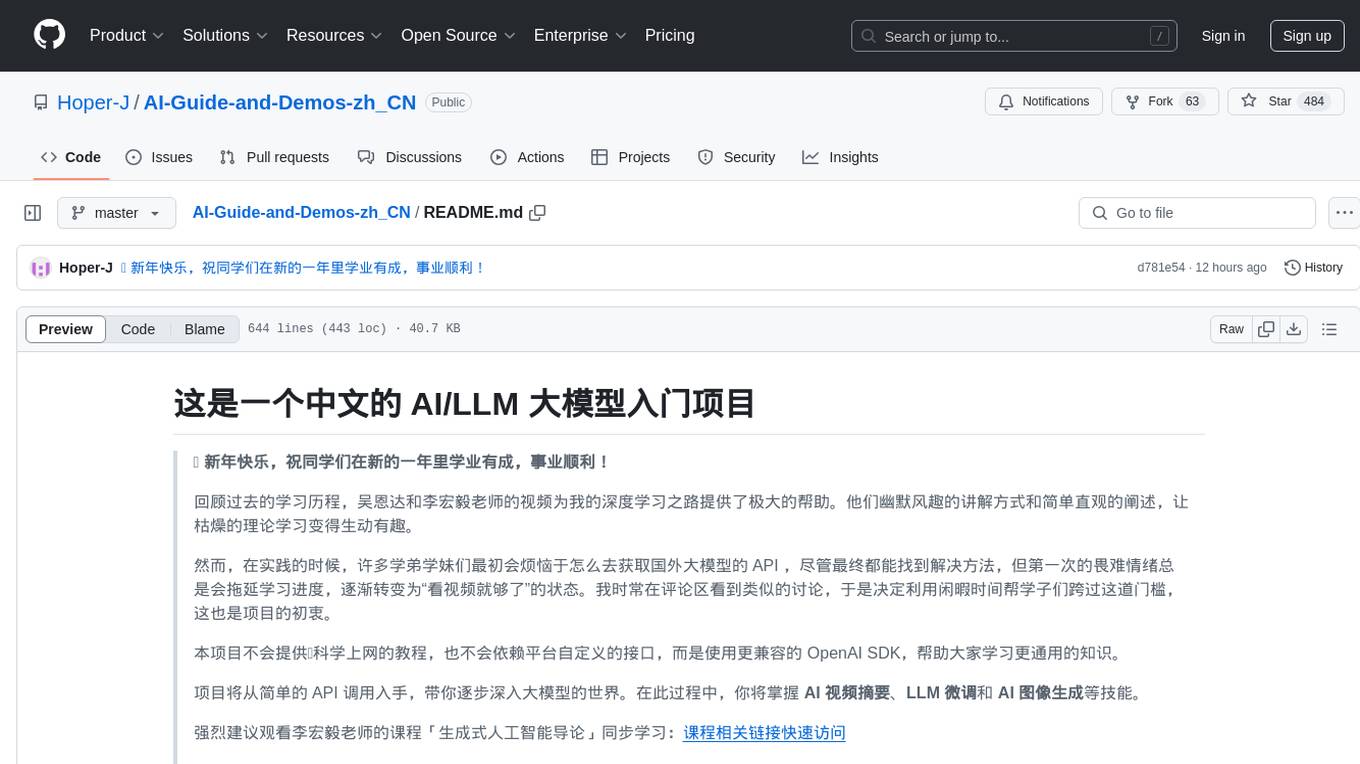
AI-Guide-and-Demos-zh_CN
This is a Chinese AI/LLM introductory project that aims to help students overcome the initial difficulties of accessing foreign large models' APIs. The project uses the OpenAI SDK to provide a more compatible learning experience. It covers topics such as AI video summarization, LLM fine-tuning, and AI image generation. The project also offers a CodePlayground for easy setup and one-line script execution to experience the charm of AI. It includes guides on API usage, LLM configuration, building AI applications with Gradio, customizing prompts for better model performance, understanding LoRA, and more.
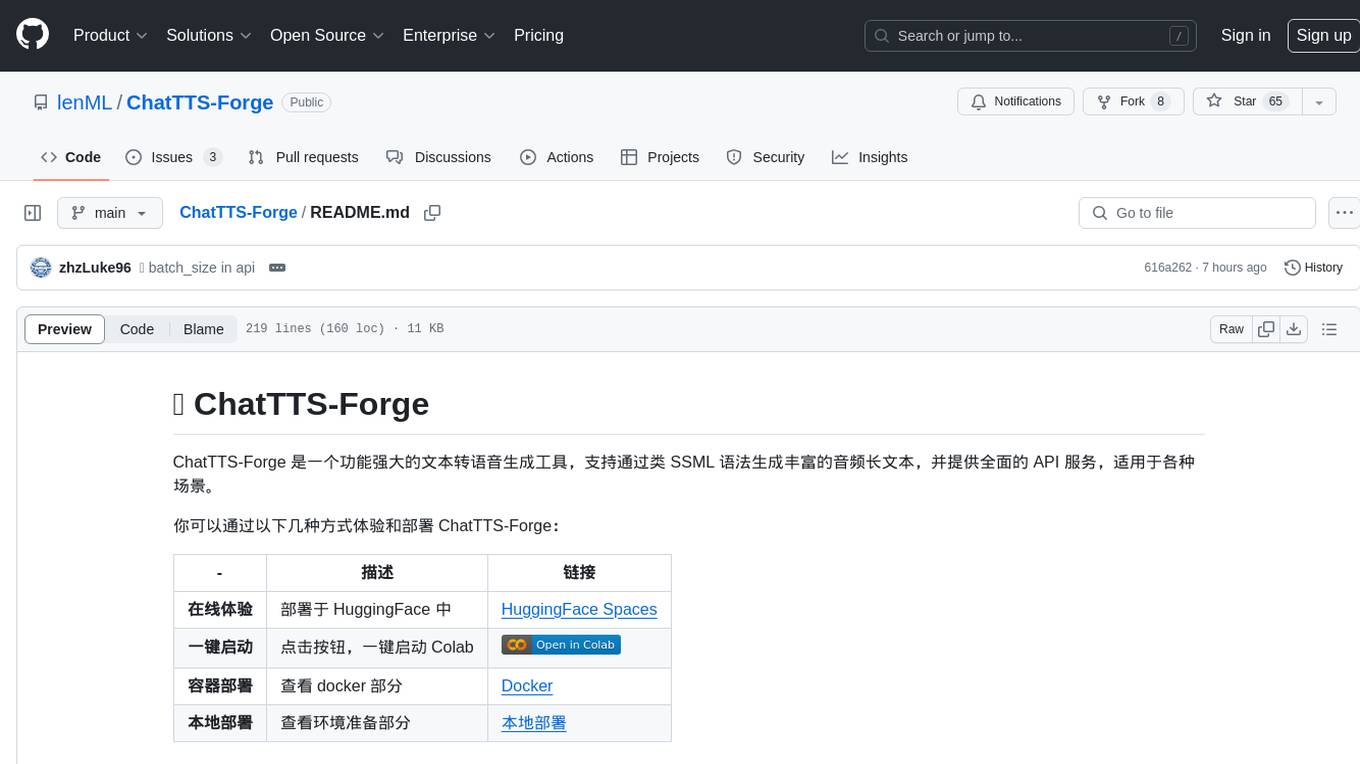
ChatTTS-Forge
ChatTTS-Forge is a powerful text-to-speech generation tool that supports generating rich audio long texts using a SSML-like syntax and provides comprehensive API services, suitable for various scenarios. It offers features such as batch generation, support for generating super long texts, style prompt injection, full API services, user-friendly debugging GUI, OpenAI-style API, Google-style API, support for SSML-like syntax, speaker management, style management, independent refine API, text normalization optimized for ChatTTS, and automatic detection and processing of markdown format text. The tool can be experienced and deployed online through HuggingFace Spaces, launched with one click on Colab, deployed using containers, or locally deployed after cloning the project, preparing models, and installing necessary dependencies.
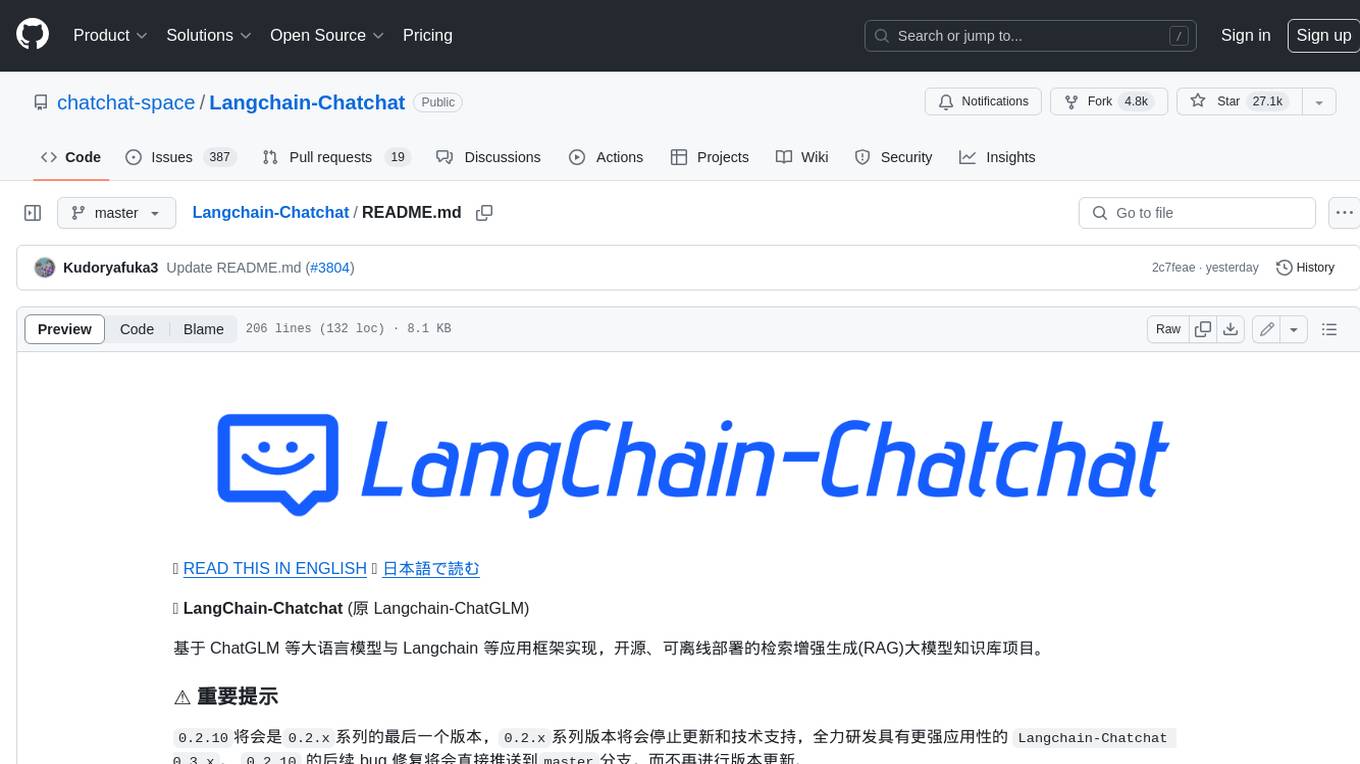
Langchain-Chatchat
LangChain-Chatchat is an open-source, offline-deployable retrieval-enhanced generation (RAG) large model knowledge base project based on large language models such as ChatGLM and application frameworks such as Langchain. It aims to establish a knowledge base Q&A solution that is friendly to Chinese scenarios, supports open-source models, and can run offline.
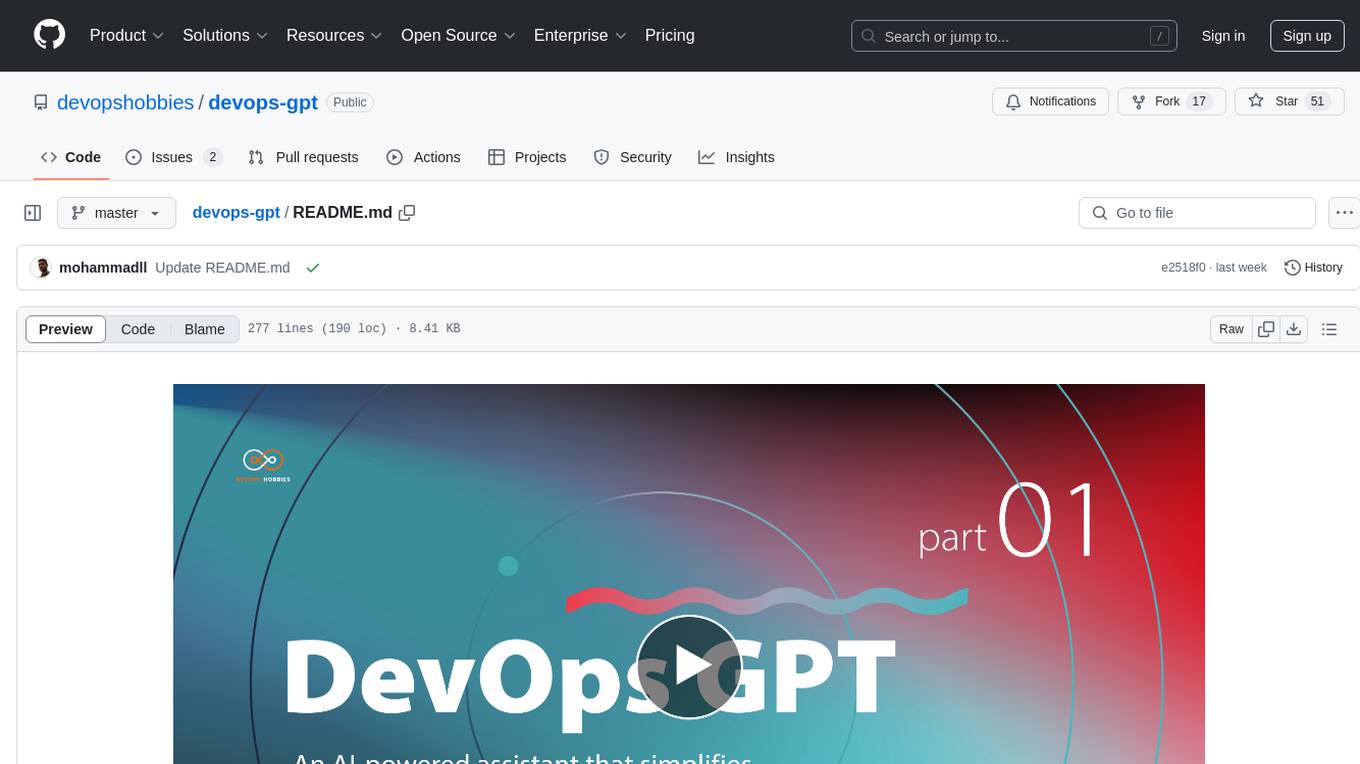
devops-gpt
DevOpsGPT is a revolutionary tool designed to streamline your workflow and empower you to build systems and automate tasks with ease. Tired of spending hours on repetitive DevOps tasks? DevOpsGPT is here to help! Whether you're setting up infrastructure, speeding up deployments, or tackling any other DevOps challenge, our app can make your life easier and more productive. With DevOpsGPT, you can expect faster task completion, simplified workflows, and increased efficiency. Ready to experience the DevOpsGPT difference? Visit our website, sign in or create an account, start exploring the features, and share your feedback to help us improve. DevOpsGPT will become an essential tool in your DevOps toolkit.
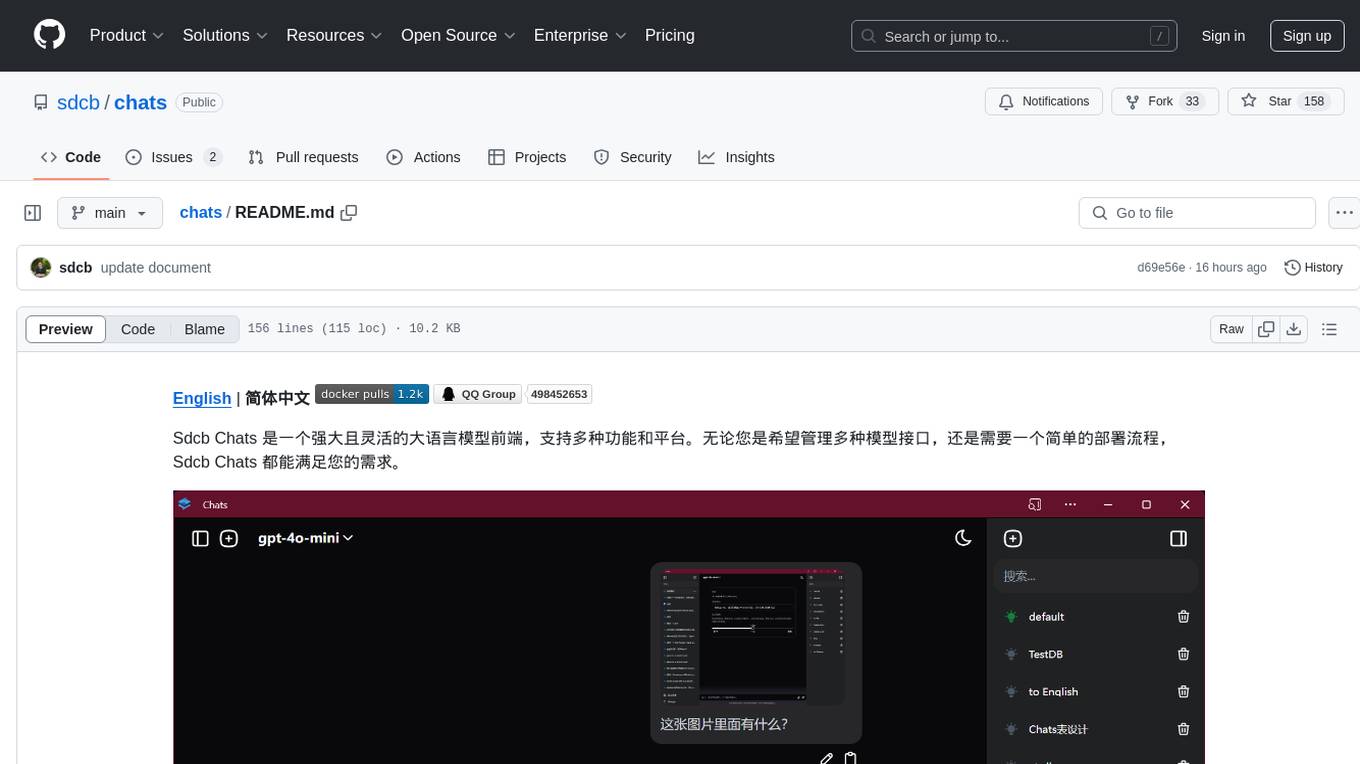
chats
Sdcb Chats is a powerful and flexible frontend for large language models, supporting multiple functions and platforms. Whether you want to manage multiple model interfaces or need a simple deployment process, Sdcb Chats can meet your needs. It supports dynamic management of multiple large language model interfaces, integrates visual models to enhance user interaction experience, provides fine-grained user permission settings for security, real-time tracking and management of user account balances, easy addition, deletion, and configuration of models, transparently forwards user chat requests based on the OpenAI protocol, supports multiple databases including SQLite, SQL Server, and PostgreSQL, compatible with various file services such as local files, AWS S3, Minio, Aliyun OSS, Azure Blob Storage, and supports multiple login methods including Keycloak SSO and phone SMS verification.
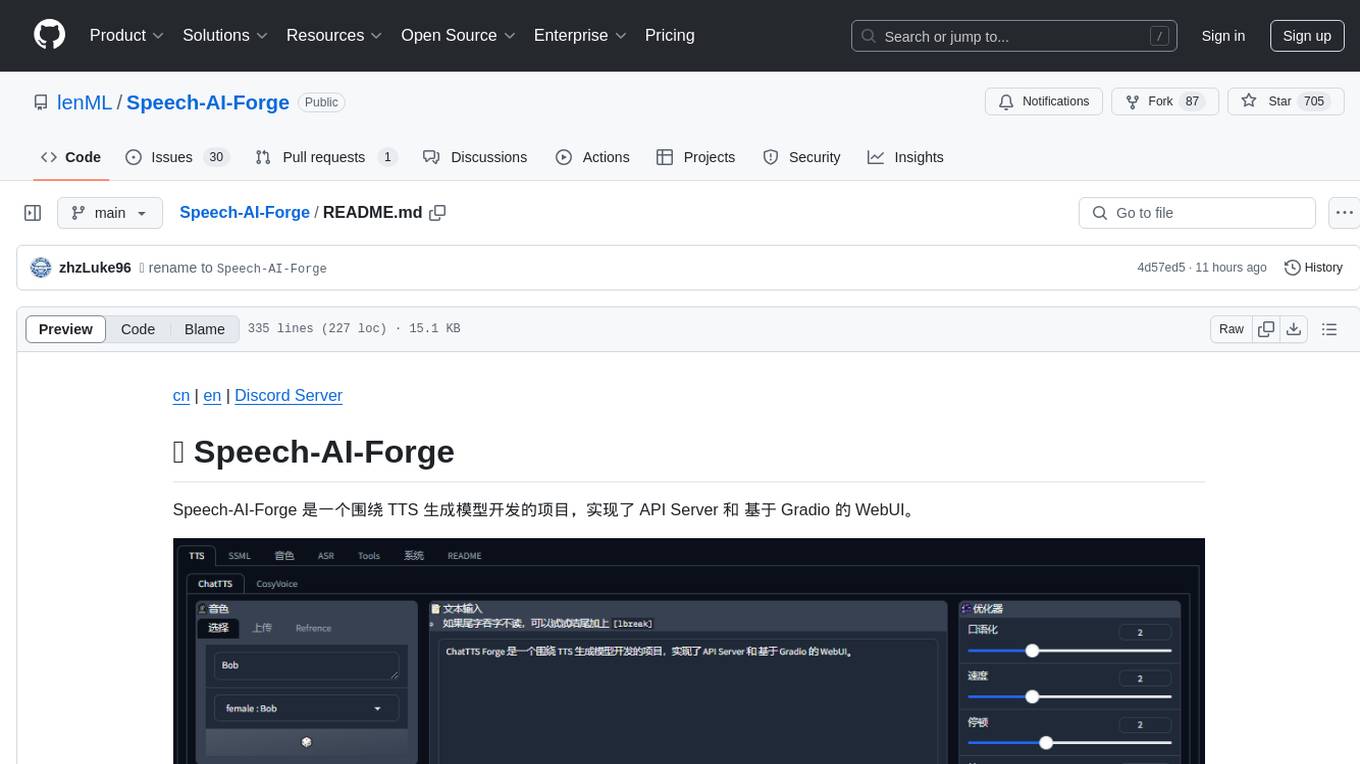
Speech-AI-Forge
Speech-AI-Forge is a project developed around TTS generation models, implementing an API Server and a WebUI based on Gradio. The project offers various ways to experience and deploy Speech-AI-Forge, including online experience on HuggingFace Spaces, one-click launch on Colab, container deployment with Docker, and local deployment. The WebUI features include TTS model functionality, speaker switch for changing voices, style control, long text support with automatic text segmentation, refiner for ChatTTS native text refinement, various tools for voice control and enhancement, support for multiple TTS models, SSML synthesis control, podcast creation tools, voice creation, voice testing, ASR tools, and post-processing tools. The API Server can be launched separately for higher API throughput. The project roadmap includes support for various TTS models, ASR models, voice clone models, and enhancer models. Model downloads can be manually initiated using provided scripts. The project aims to provide inference services and may include training-related functionalities in the future.
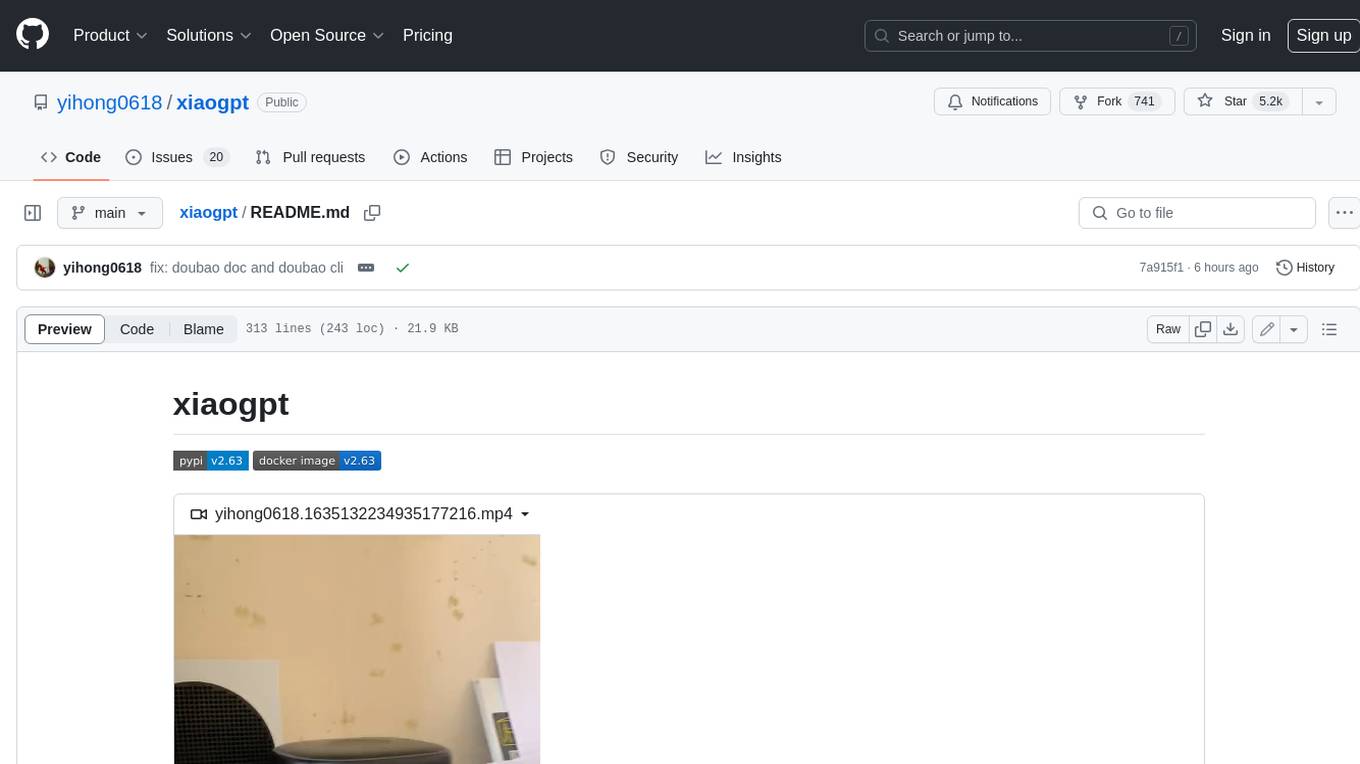
xiaogpt
xiaogpt is a tool that allows you to play ChatGPT and other LLMs with Xiaomi AI Speaker. It supports ChatGPT, New Bing, ChatGLM, Gemini, Doubao, and Tongyi Qianwen. You can use it to ask questions, get answers, and have conversations with AI assistants. xiaogpt is easy to use and can be set up in a few minutes. It is a great way to experience the power of AI and have fun with your Xiaomi AI Speaker.
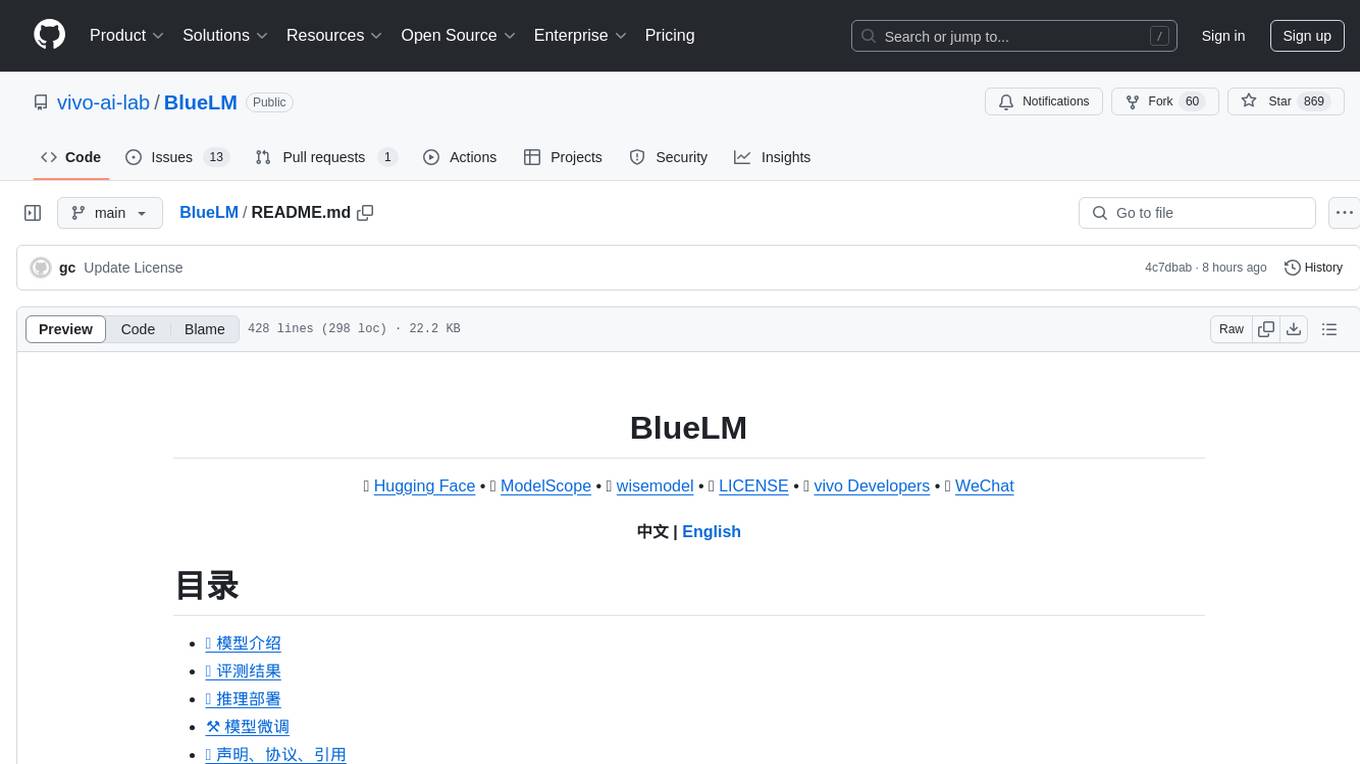
BlueLM
BlueLM is a large-scale pre-trained language model developed by vivo AI Global Research Institute, featuring 7B base and chat models. It includes high-quality training data with a token scale of 26 trillion, supporting both Chinese and English languages. BlueLM-7B-Chat excels in C-Eval and CMMLU evaluations, providing strong competition among open-source models of similar size. The models support 32K long texts for better context understanding while maintaining base capabilities. BlueLM welcomes developers for academic research and commercial applications.
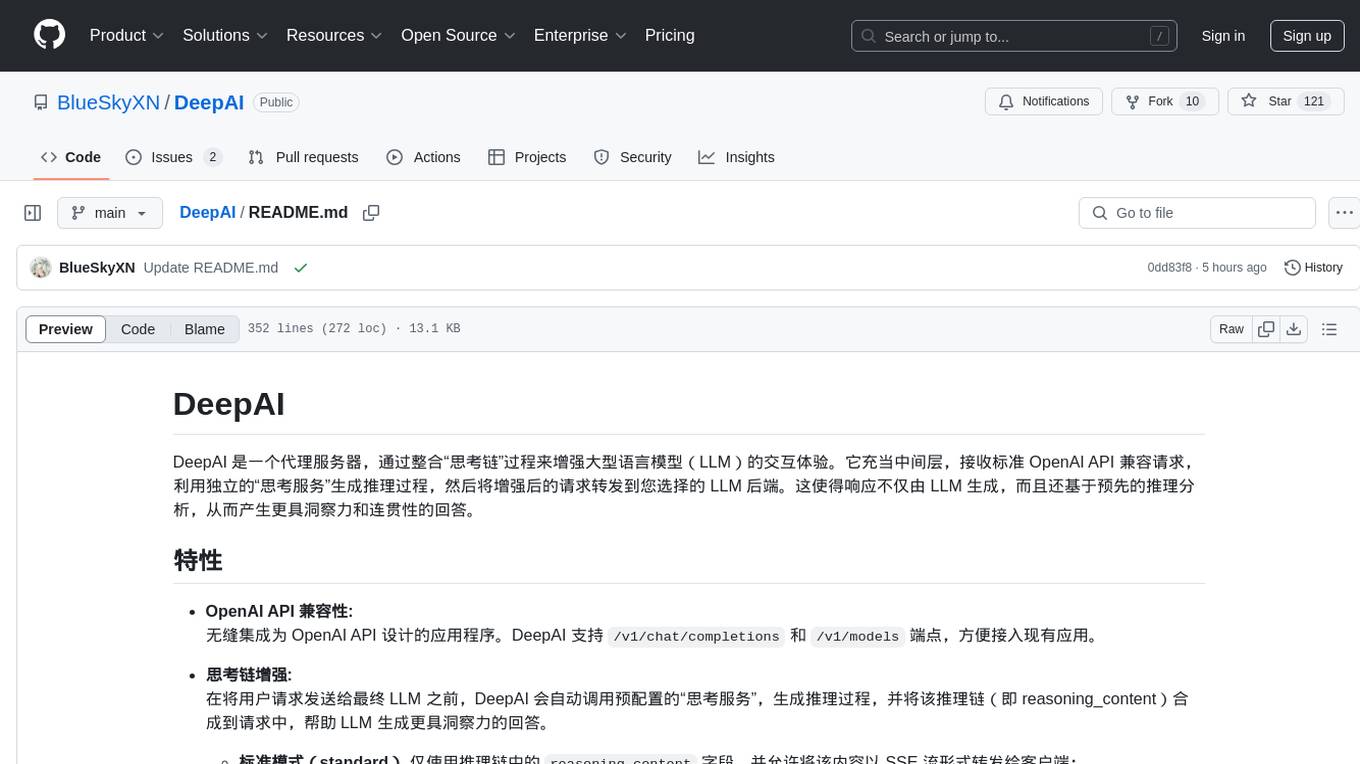
DeepAI
DeepAI is a proxy server that enhances the interaction experience of large language models (LLMs) by integrating the 'thinking chain' process. It acts as an intermediary layer, receiving standard OpenAI API compatible requests, using independent 'thinking services' to generate reasoning processes, and then forwarding the enhanced requests to the LLM backend of your choice. This ensures that responses are not only generated by the LLM but also based on pre-inference analysis, resulting in more insightful and coherent answers. DeepAI supports seamless integration with applications designed for the OpenAI API, providing endpoints for '/v1/chat/completions' and '/v1/models', making it easy to integrate into existing applications. It offers features such as reasoning chain enhancement, flexible backend support, API key routing, weighted random selection, proxy support, comprehensive logging, and graceful shutdown.
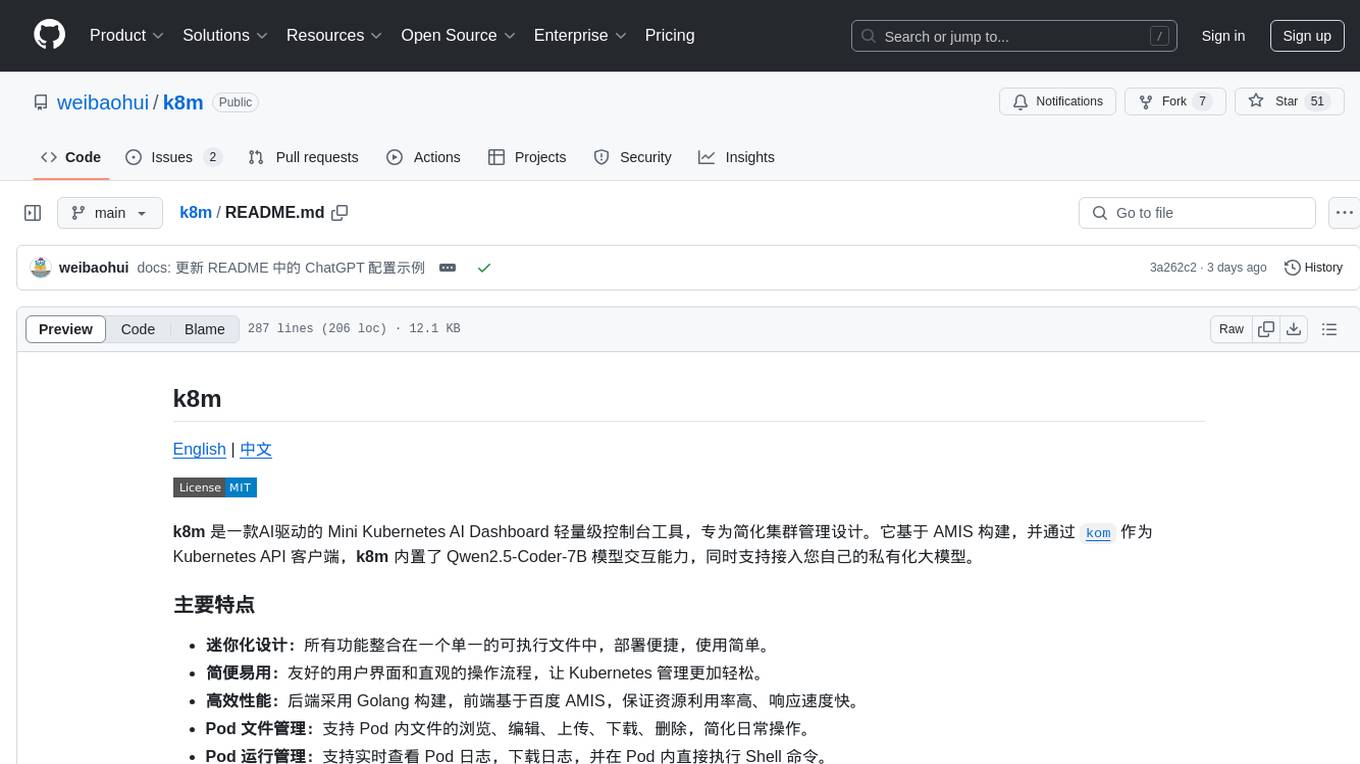
k8m
k8m is an AI-driven Mini Kubernetes AI Dashboard lightweight console tool designed to simplify cluster management. It is built on AMIS and uses 'kom' as the Kubernetes API client. k8m has built-in Qwen2.5-Coder-7B model interaction capabilities and supports integration with your own private large models. Its key features include miniaturized design for easy deployment, user-friendly interface for intuitive operation, efficient performance with backend in Golang and frontend based on Baidu AMIS, pod file management for browsing, editing, uploading, downloading, and deleting files, pod runtime management for real-time log viewing, log downloading, and executing shell commands within pods, CRD management for automatic discovery and management of CRD resources, and intelligent translation and diagnosis based on ChatGPT for YAML property translation, Describe information interpretation, AI log diagnosis, and command recommendations, providing intelligent support for managing k8s. It is cross-platform compatible with Linux, macOS, and Windows, supporting multiple architectures like x86 and ARM for seamless operation. k8m's design philosophy is 'AI-driven, lightweight and efficient, simplifying complexity,' helping developers and operators quickly get started and easily manage Kubernetes clusters.
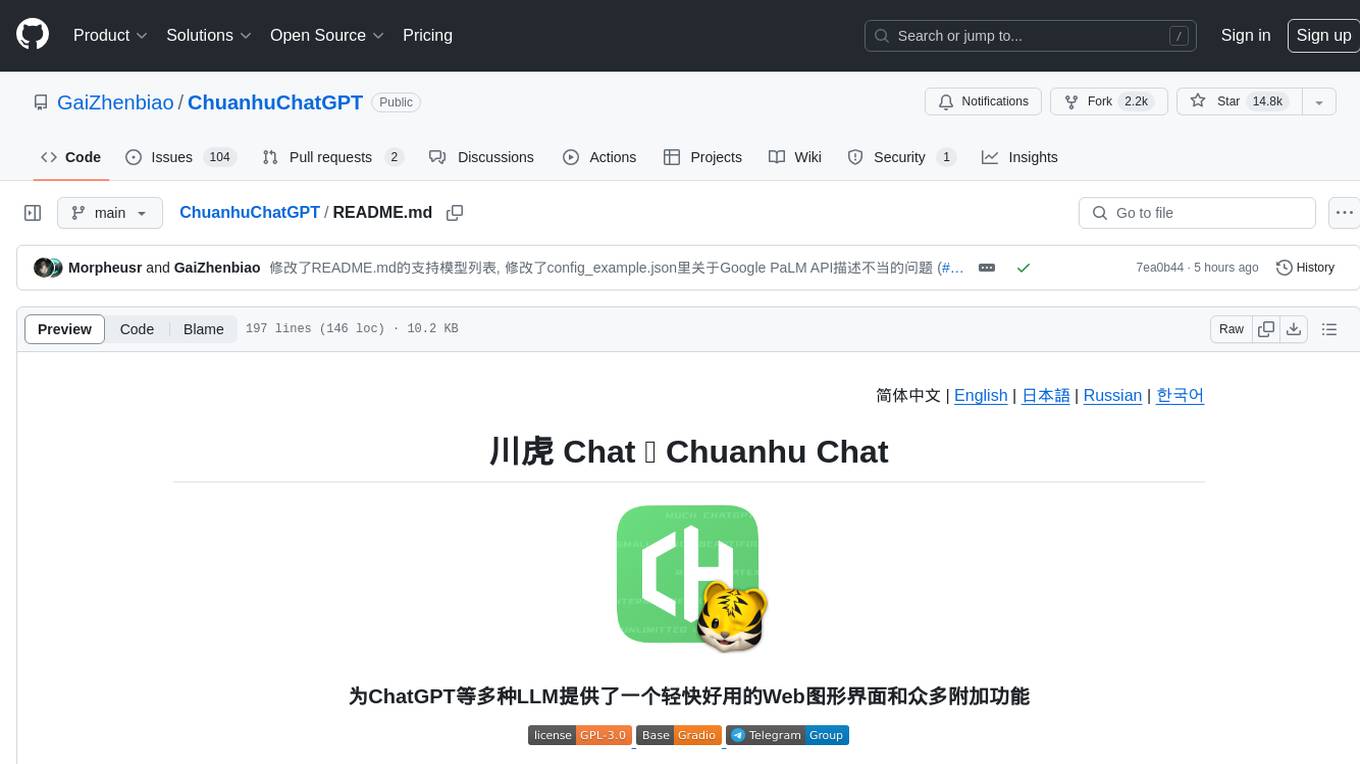
ChuanhuChatGPT
Chuanhu Chat is a user-friendly web graphical interface that provides various additional features for ChatGPT and other language models. It supports GPT-4, file-based question answering, local deployment of language models, online search, agent assistant, and fine-tuning. The tool offers a range of functionalities including auto-solving questions, online searching with network support, knowledge base for quick reading, local deployment of language models, GPT 3.5 fine-tuning, and custom model integration. It also features system prompts for effective role-playing, basic conversation capabilities with options to regenerate or delete dialogues, conversation history management with auto-saving and search functionalities, and a visually appealing user experience with themes, dark mode, LaTeX rendering, and PWA application support.
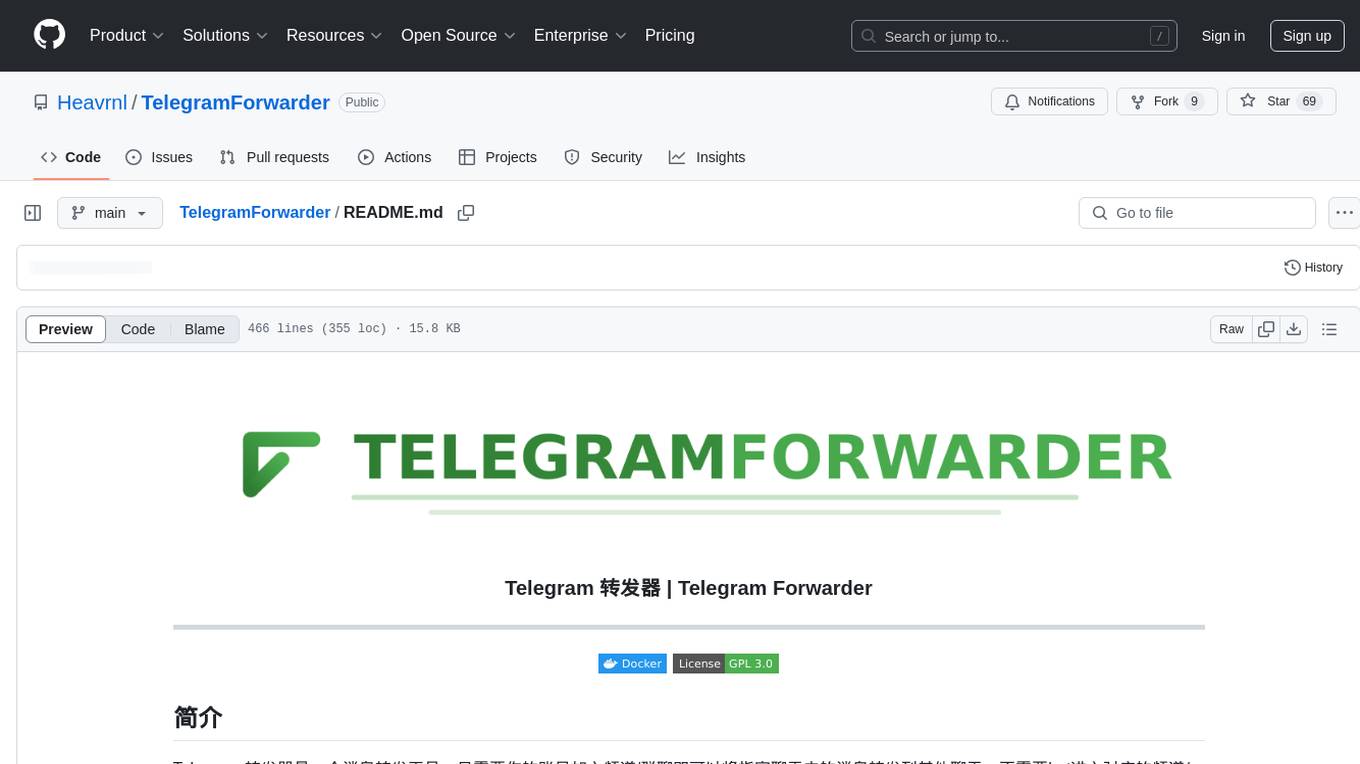
TelegramForwarder
Telegram Forwarder is a message forwarding tool that allows you to forward messages from specified chats to other chats without the need for a bot to enter the corresponding channels/groups to listen. It can be used for information stream integration filtering, message reminders, content archiving, and more. The tool supports multiple sources forwarding, keyword filtering in whitelist and blacklist modes, regular expression matching, message content modification, AI processing using major vendors' AI interfaces, media file filtering, and synchronization with a universal forum blocking plugin to achieve three-end blocking.
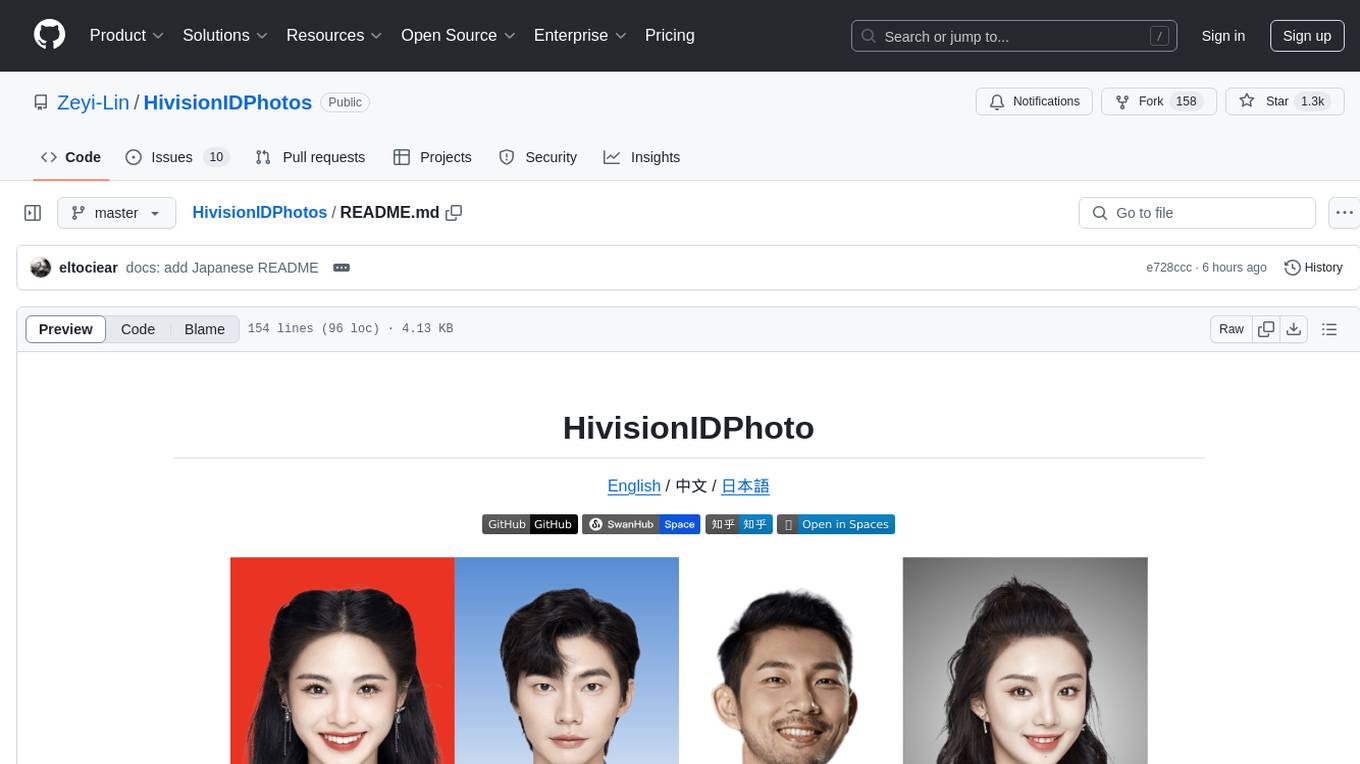
HivisionIDPhotos
HivisionIDPhoto is a practical algorithm for intelligent ID photo creation. It utilizes a comprehensive model workflow to recognize, cut out, and generate ID photos for various user photo scenarios. The tool offers lightweight cutting, standard ID photo generation based on different size specifications, six-inch layout photo generation, beauty enhancement (waiting), and intelligent outfit swapping (waiting). It aims to solve emergency ID photo creation issues.
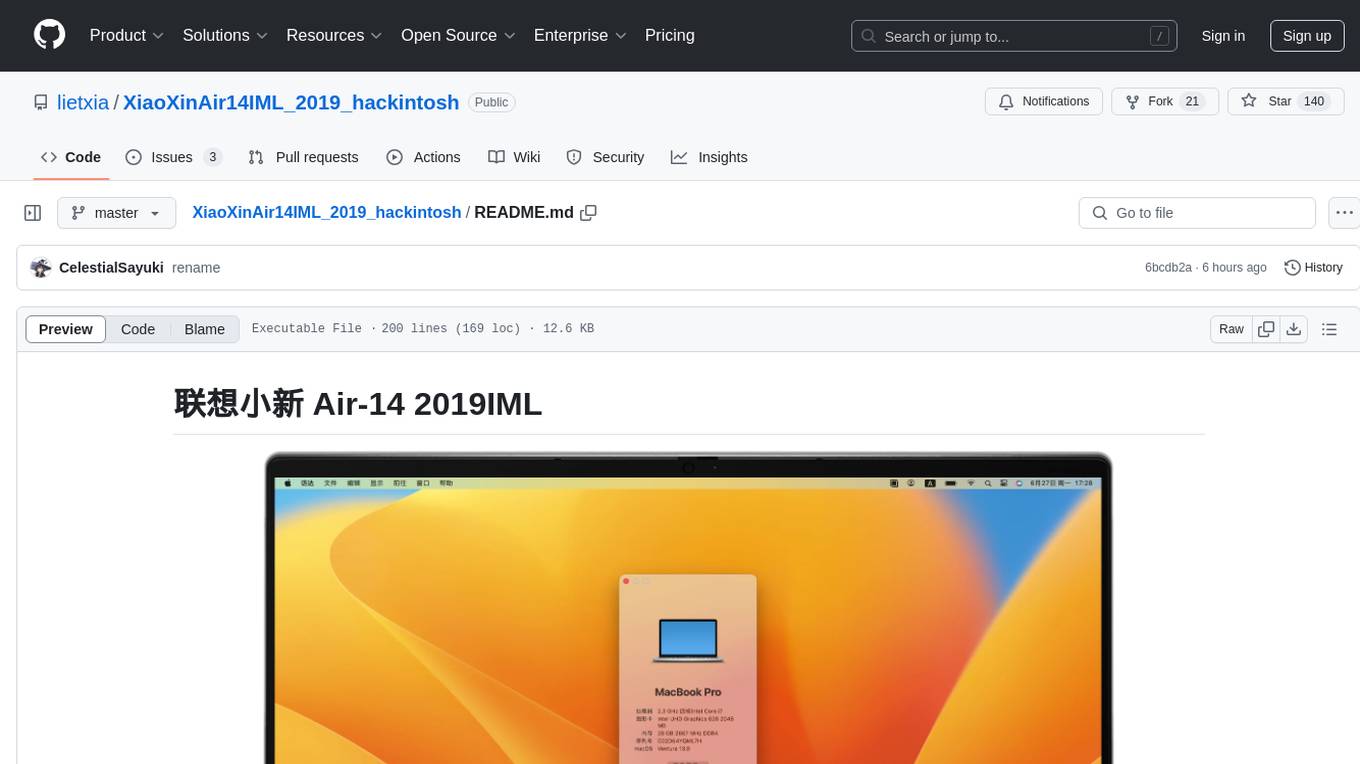
XiaoXinAir14IML_2019_hackintosh
XiaoXinAir14IML_2019_hackintosh is a repository dedicated to enabling macOS installation on Lenovo XiaoXin Air-14 IML 2019 laptops. The repository provides detailed information on the hardware specifications, supported systems, BIOS versions, related models, installation methods, updates, patches, and recommended settings. It also includes tools and guides for BIOS modifications, enabling high-resolution display settings, Bluetooth synchronization between macOS and Windows 10, voltage adjustments for efficiency, and experimental support for YogaSMC. The repository offers solutions for various issues like sleep support, sound card emulation, and battery information. It acknowledges the contributions of developers and tools like OpenCore, itlwm, VoodooI2C, and ALCPlugFix.
For similar tasks
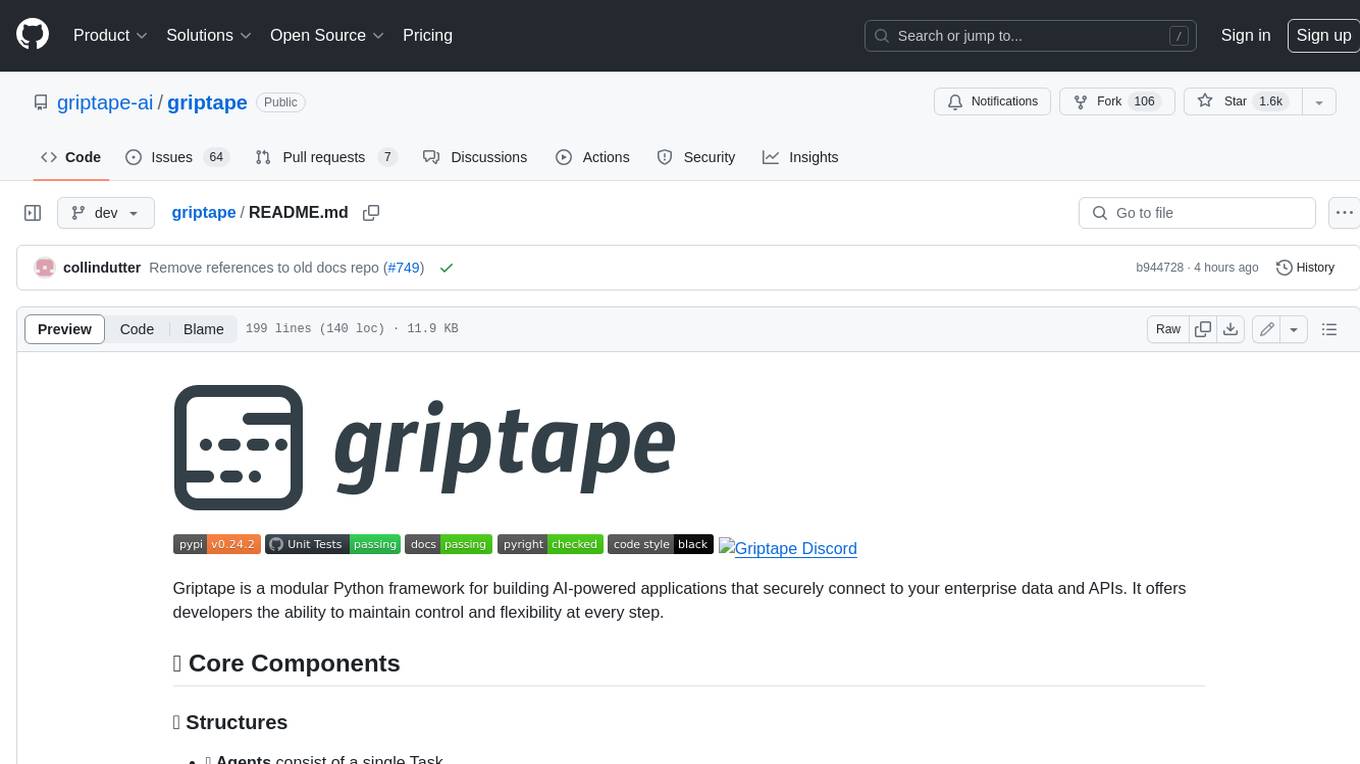
griptape
Griptape is a modular Python framework for building AI-powered applications that securely connect to your enterprise data and APIs. It offers developers the ability to maintain control and flexibility at every step. Griptape's core components include Structures (Agents, Pipelines, and Workflows), Tasks, Tools, Memory (Conversation Memory, Task Memory, and Meta Memory), Drivers (Prompt and Embedding Drivers, Vector Store Drivers, Image Generation Drivers, Image Query Drivers, SQL Drivers, Web Scraper Drivers, and Conversation Memory Drivers), Engines (Query Engines, Extraction Engines, Summary Engines, Image Generation Engines, and Image Query Engines), and additional components (Rulesets, Loaders, Artifacts, Chunkers, and Tokenizers). Griptape enables developers to create AI-powered applications with ease and efficiency.
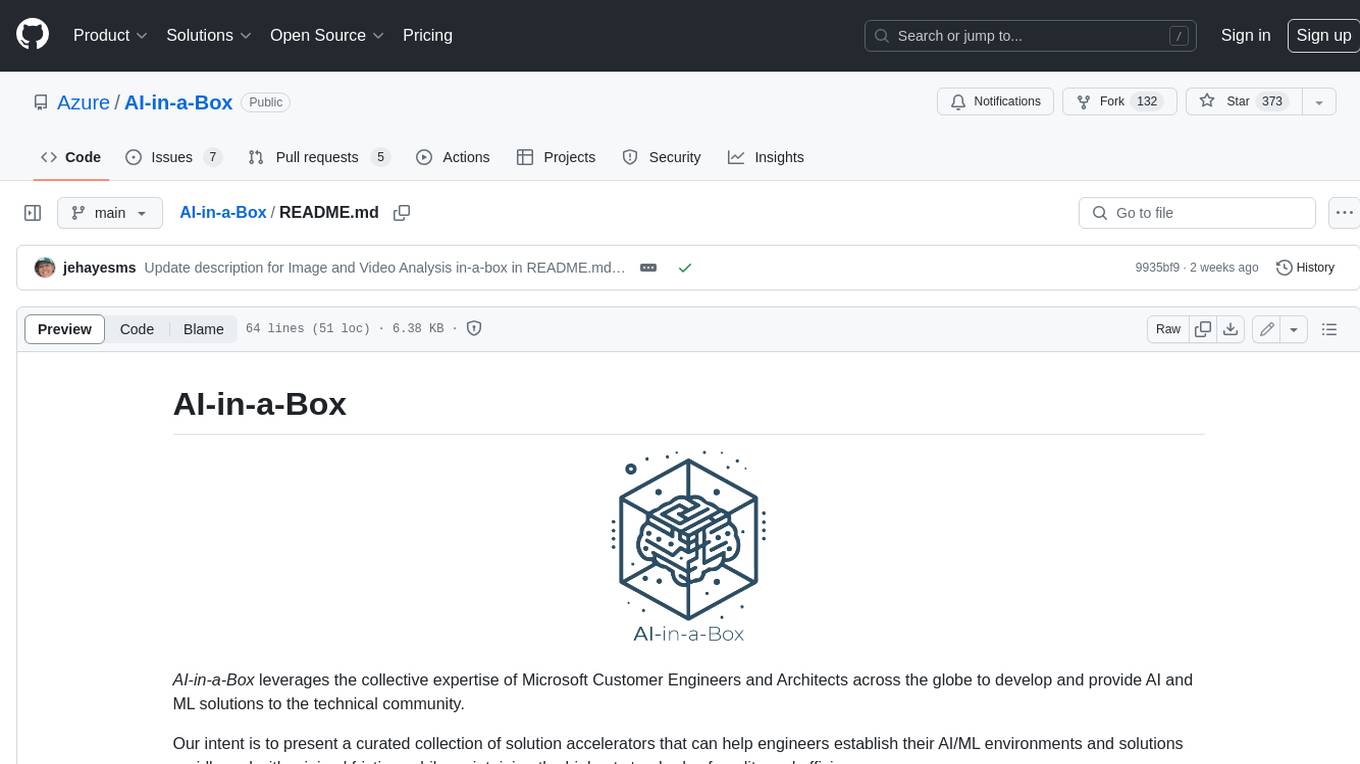
AI-in-a-Box
AI-in-a-Box is a curated collection of solution accelerators that can help engineers establish their AI/ML environments and solutions rapidly and with minimal friction, while maintaining the highest standards of quality and efficiency. It provides essential guidance on the responsible use of AI and LLM technologies, specific security guidance for Generative AI (GenAI) applications, and best practices for scaling OpenAI applications within Azure. The available accelerators include: Azure ML Operationalization in-a-box, Edge AI in-a-box, Doc Intelligence in-a-box, Image and Video Analysis in-a-box, Cognitive Services Landing Zone in-a-box, Semantic Kernel Bot in-a-box, NLP to SQL in-a-box, Assistants API in-a-box, and Assistants API Bot in-a-box.
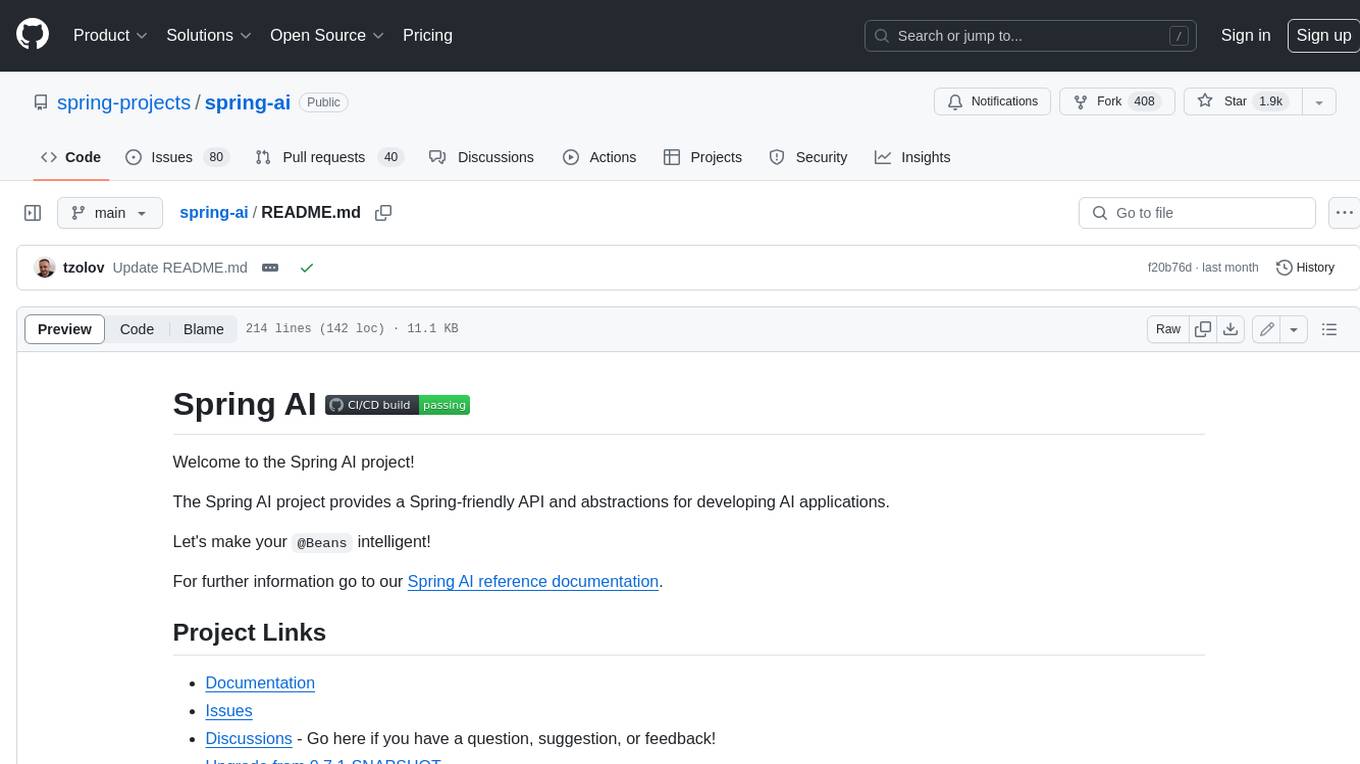
spring-ai
The Spring AI project provides a Spring-friendly API and abstractions for developing AI applications. It offers a portable client API for interacting with generative AI models, enabling developers to easily swap out implementations and access various models like OpenAI, Azure OpenAI, and HuggingFace. Spring AI also supports prompt engineering, providing classes and interfaces for creating and parsing prompts, as well as incorporating proprietary data into generative AI without retraining the model. This is achieved through Retrieval Augmented Generation (RAG), which involves extracting, transforming, and loading data into a vector database for use by AI models. Spring AI's VectorStore abstraction allows for seamless transitions between different vector database implementations.
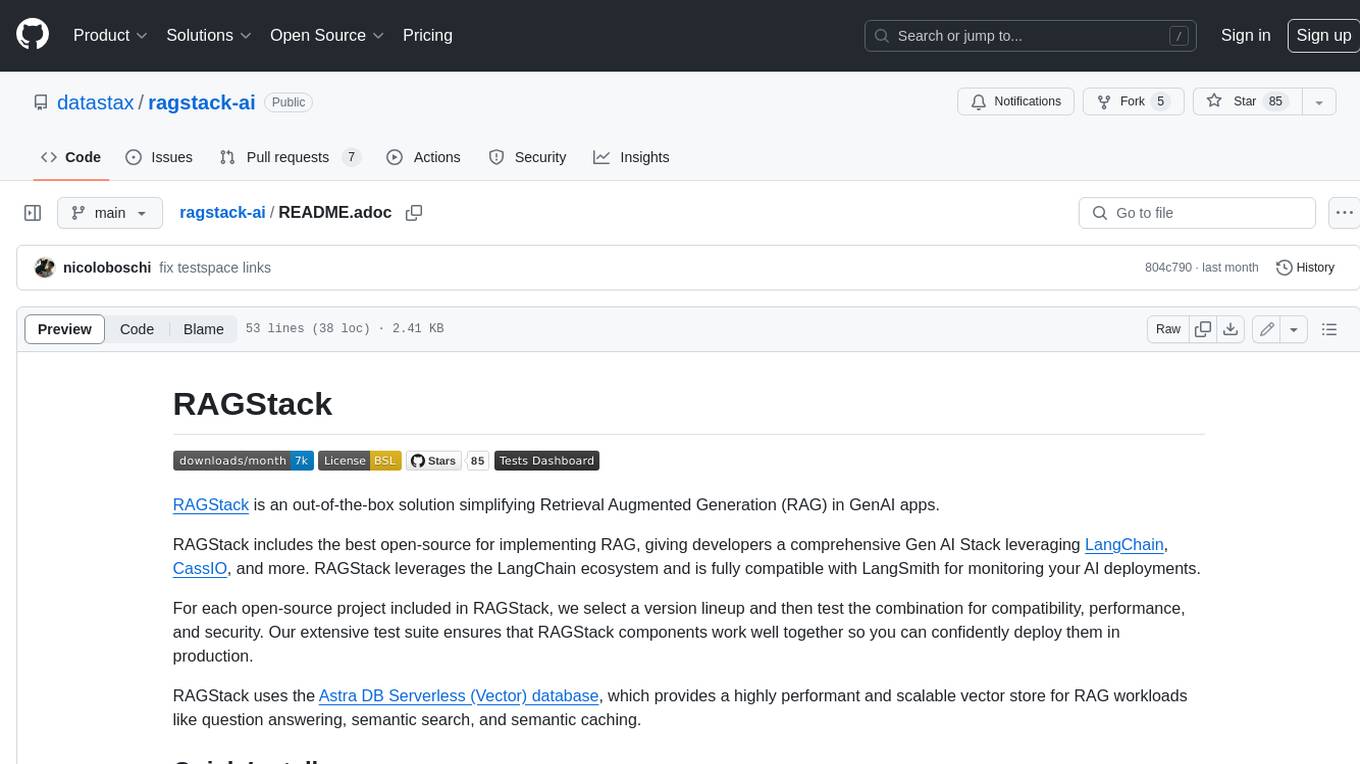
ragstack-ai
RAGStack is an out-of-the-box solution simplifying Retrieval Augmented Generation (RAG) in GenAI apps. RAGStack includes the best open-source for implementing RAG, giving developers a comprehensive Gen AI Stack leveraging LangChain, CassIO, and more. RAGStack leverages the LangChain ecosystem and is fully compatible with LangSmith for monitoring your AI deployments.
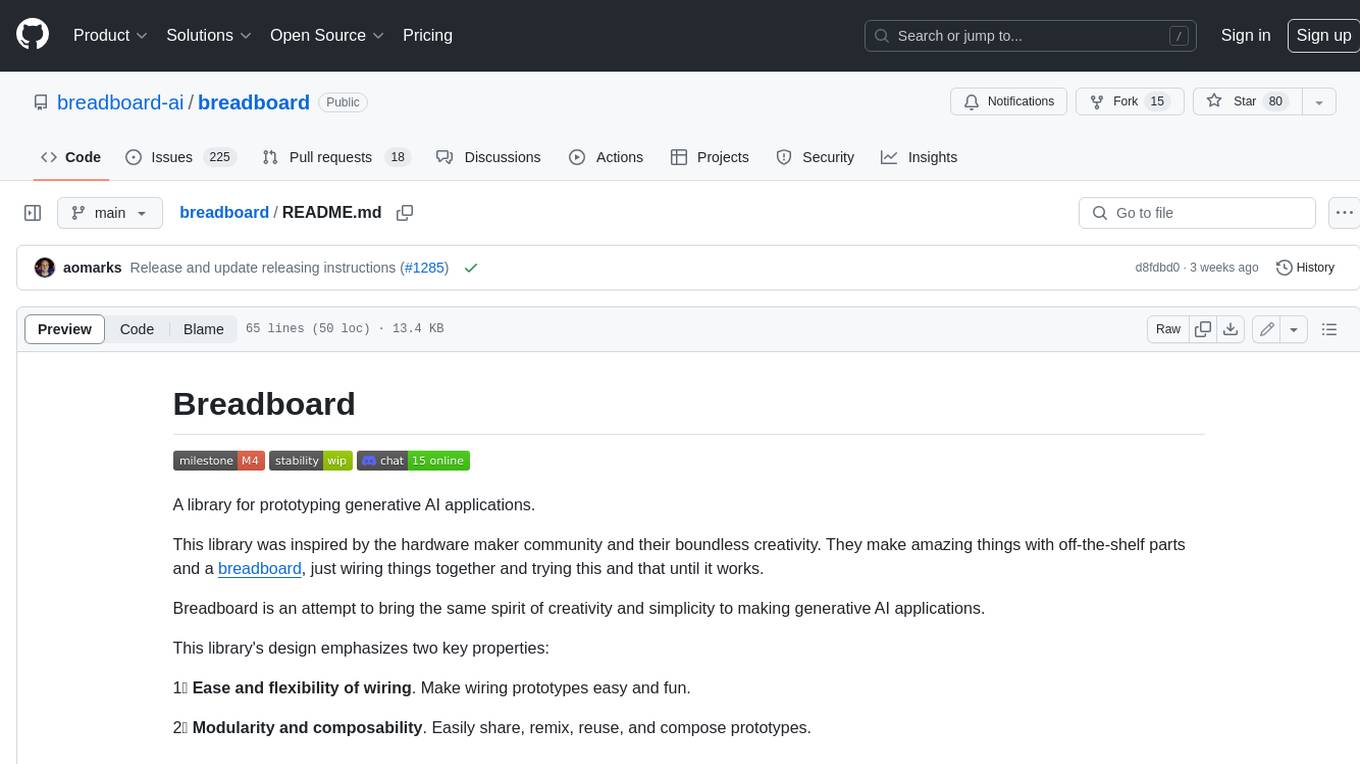
breadboard
Breadboard is a library for prototyping generative AI applications. It is inspired by the hardware maker community and their boundless creativity. Breadboard makes it easy to wire prototypes and share, remix, reuse, and compose them. The library emphasizes ease and flexibility of wiring, as well as modularity and composability.
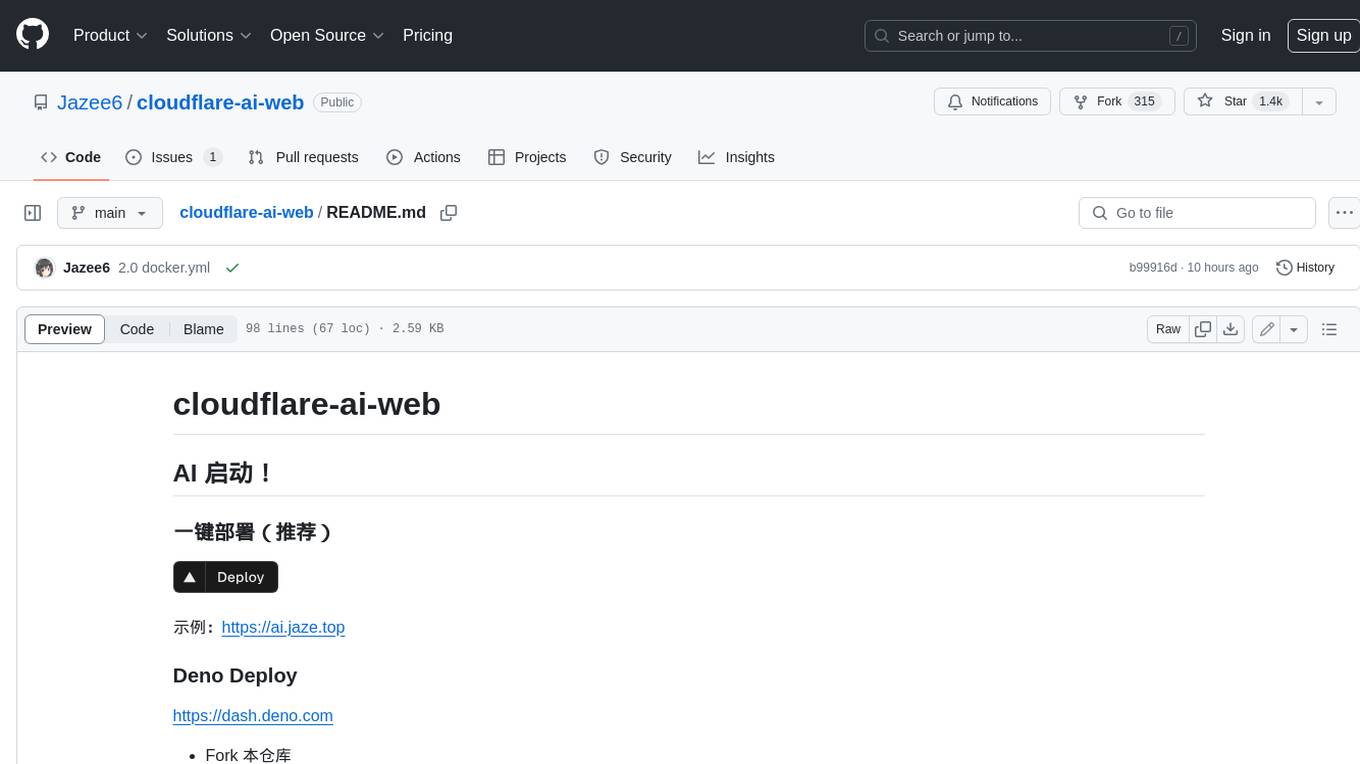
cloudflare-ai-web
Cloudflare-ai-web is a lightweight and easy-to-use tool that allows you to quickly deploy a multi-modal AI platform using Cloudflare Workers AI. It supports serverless deployment, password protection, and local storage of chat logs. With a size of only ~638 kB gzip, it is a great option for building AI-powered applications without the need for a dedicated server.
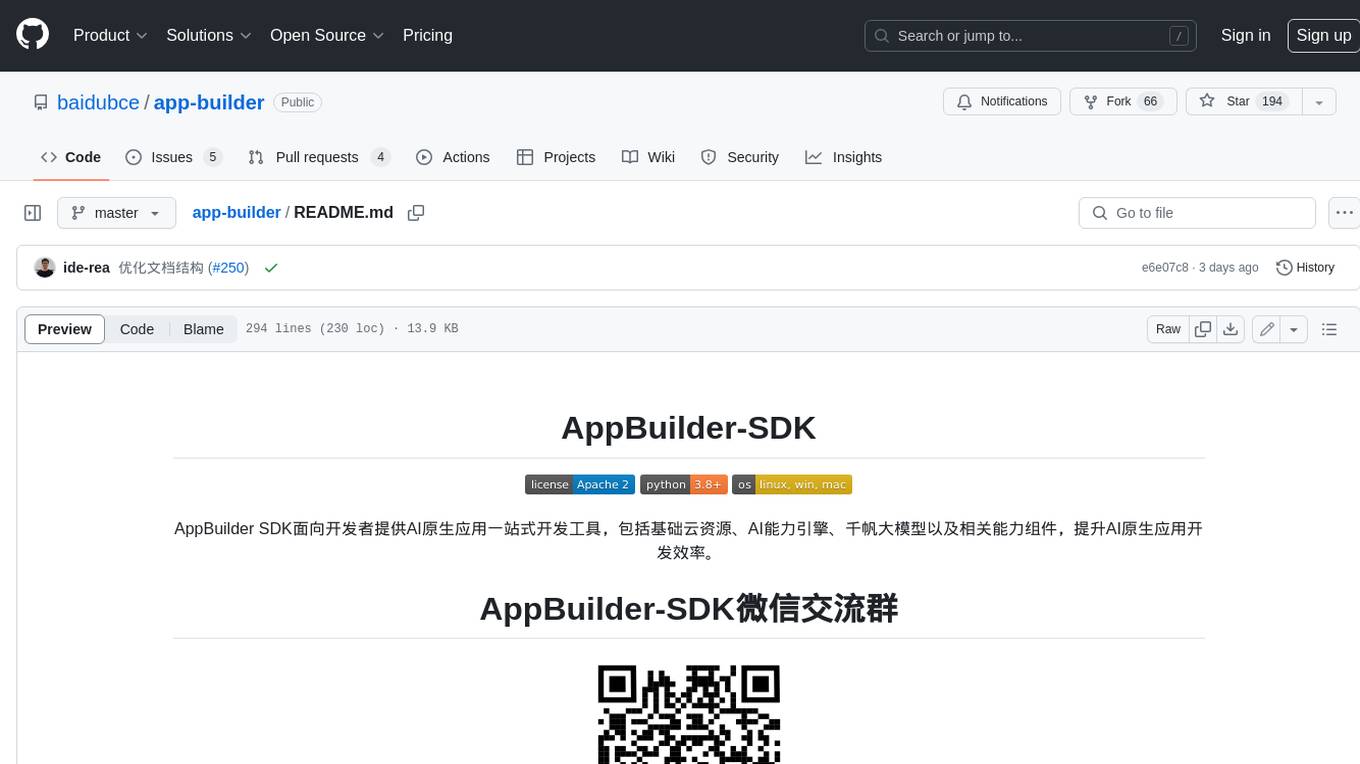
app-builder
AppBuilder SDK is a one-stop development tool for AI native applications, providing basic cloud resources, AI capability engine, Qianfan large model, and related capability components to improve the development efficiency of AI native applications.
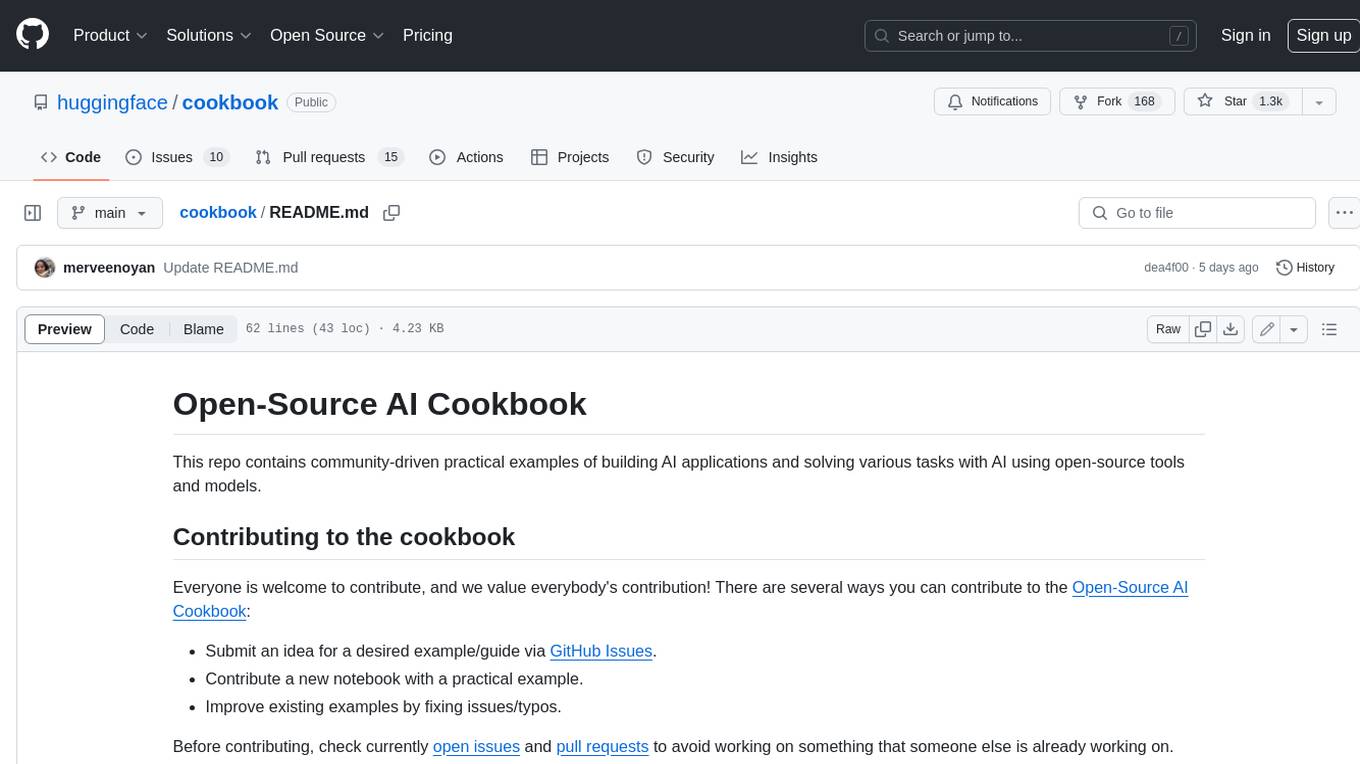
cookbook
This repository contains community-driven practical examples of building AI applications and solving various tasks with AI using open-source tools and models. Everyone is welcome to contribute, and we value everybody's contribution! There are several ways you can contribute to the Open-Source AI Cookbook: Submit an idea for a desired example/guide via GitHub Issues. Contribute a new notebook with a practical example. Improve existing examples by fixing issues/typos. Before contributing, check currently open issues and pull requests to avoid working on something that someone else is already working on.
For similar jobs
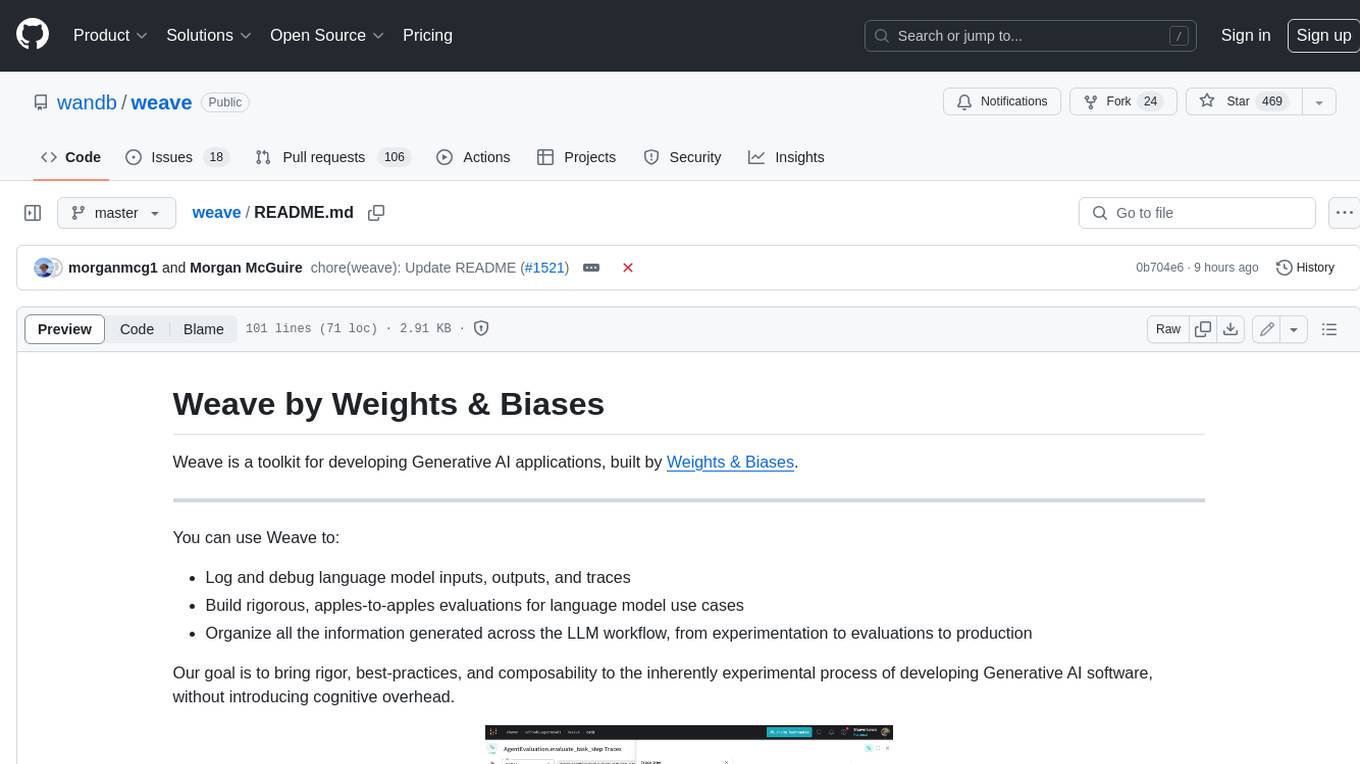
weave
Weave is a toolkit for developing Generative AI applications, built by Weights & Biases. With Weave, you can log and debug language model inputs, outputs, and traces; build rigorous, apples-to-apples evaluations for language model use cases; and organize all the information generated across the LLM workflow, from experimentation to evaluations to production. Weave aims to bring rigor, best-practices, and composability to the inherently experimental process of developing Generative AI software, without introducing cognitive overhead.
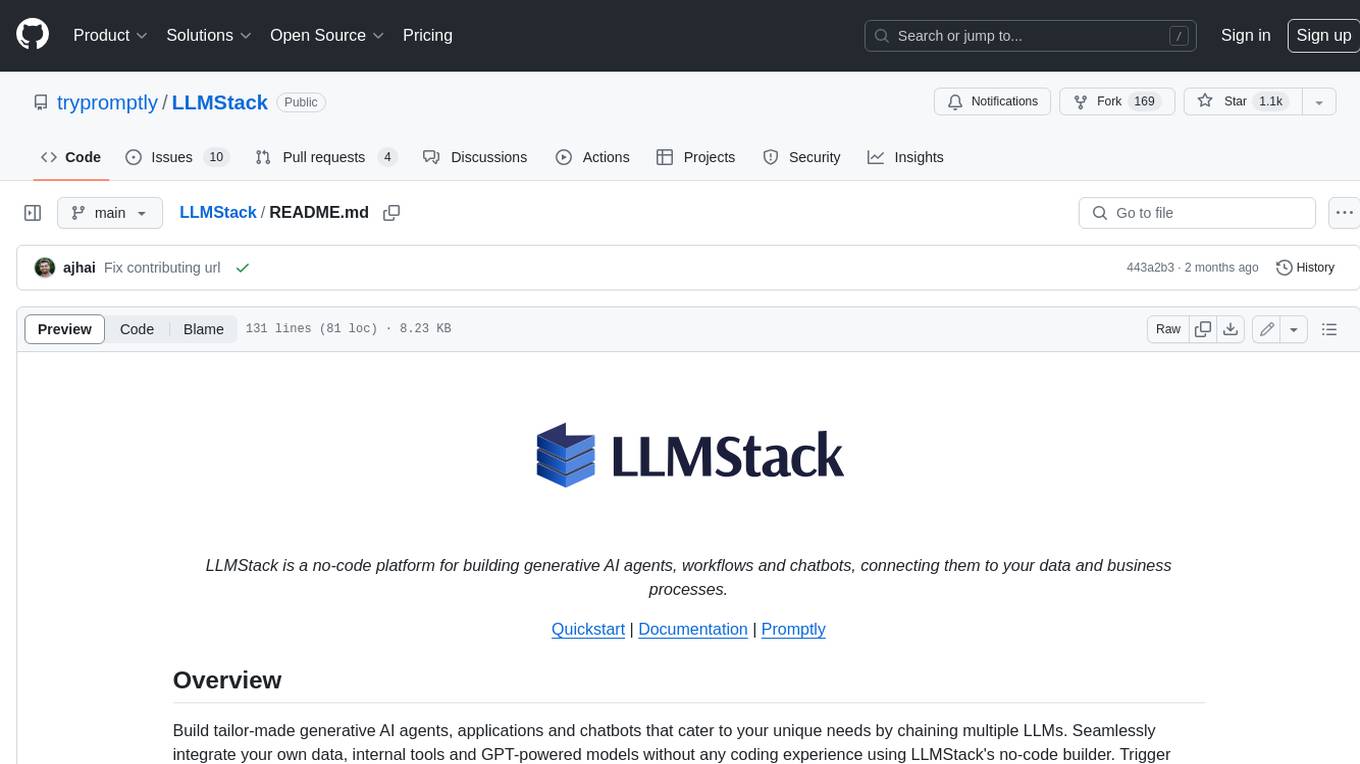
LLMStack
LLMStack is a no-code platform for building generative AI agents, workflows, and chatbots. It allows users to connect their own data, internal tools, and GPT-powered models without any coding experience. LLMStack can be deployed to the cloud or on-premise and can be accessed via HTTP API or triggered from Slack or Discord.
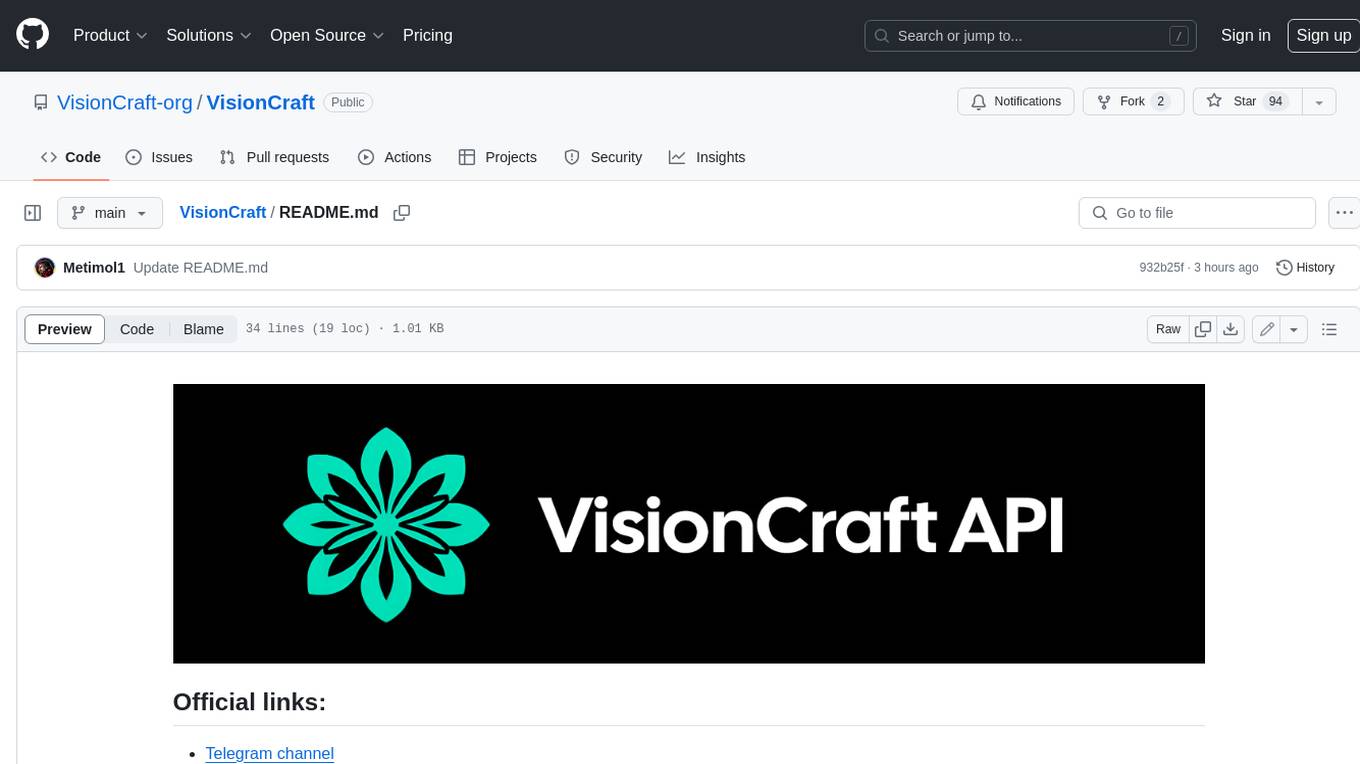
VisionCraft
The VisionCraft API is a free API for using over 100 different AI models. From images to sound.
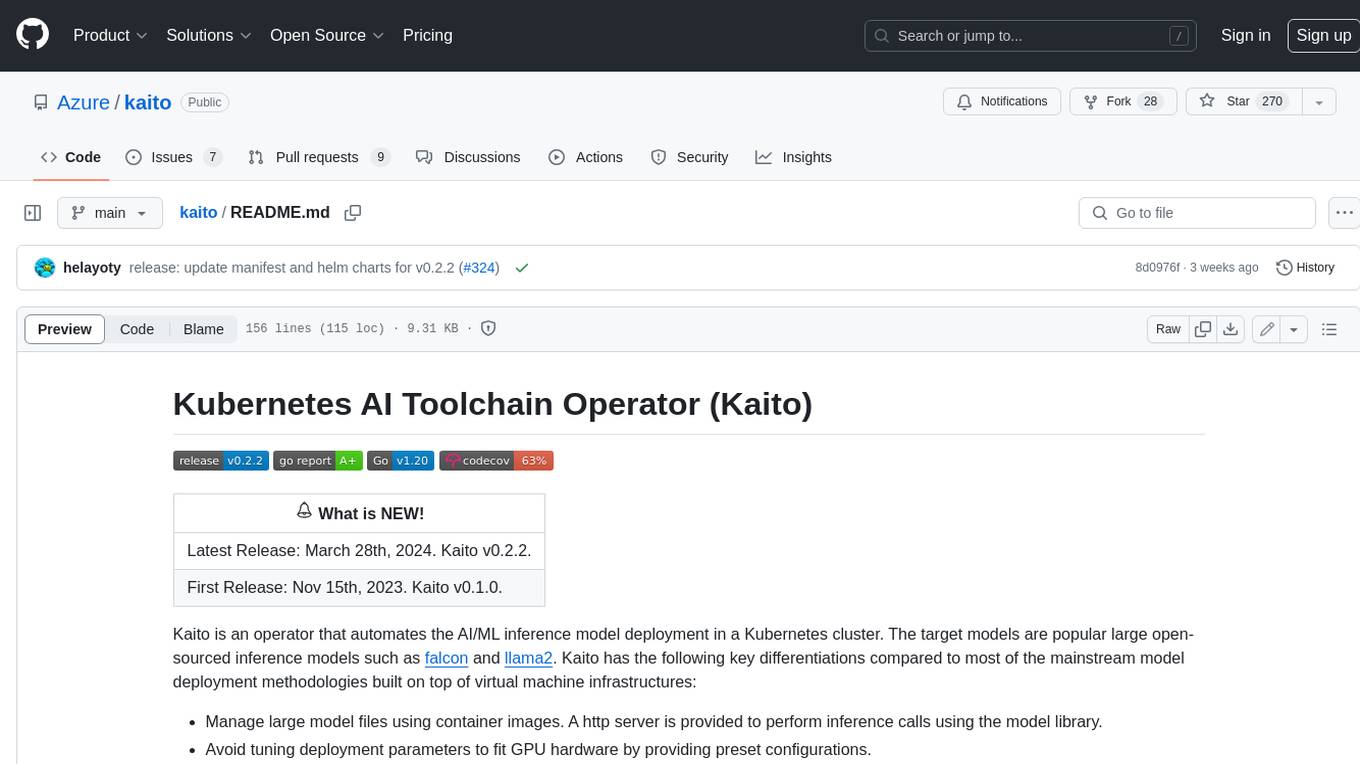
kaito
Kaito is an operator that automates the AI/ML inference model deployment in a Kubernetes cluster. It manages large model files using container images, avoids tuning deployment parameters to fit GPU hardware by providing preset configurations, auto-provisions GPU nodes based on model requirements, and hosts large model images in the public Microsoft Container Registry (MCR) if the license allows. Using Kaito, the workflow of onboarding large AI inference models in Kubernetes is largely simplified.
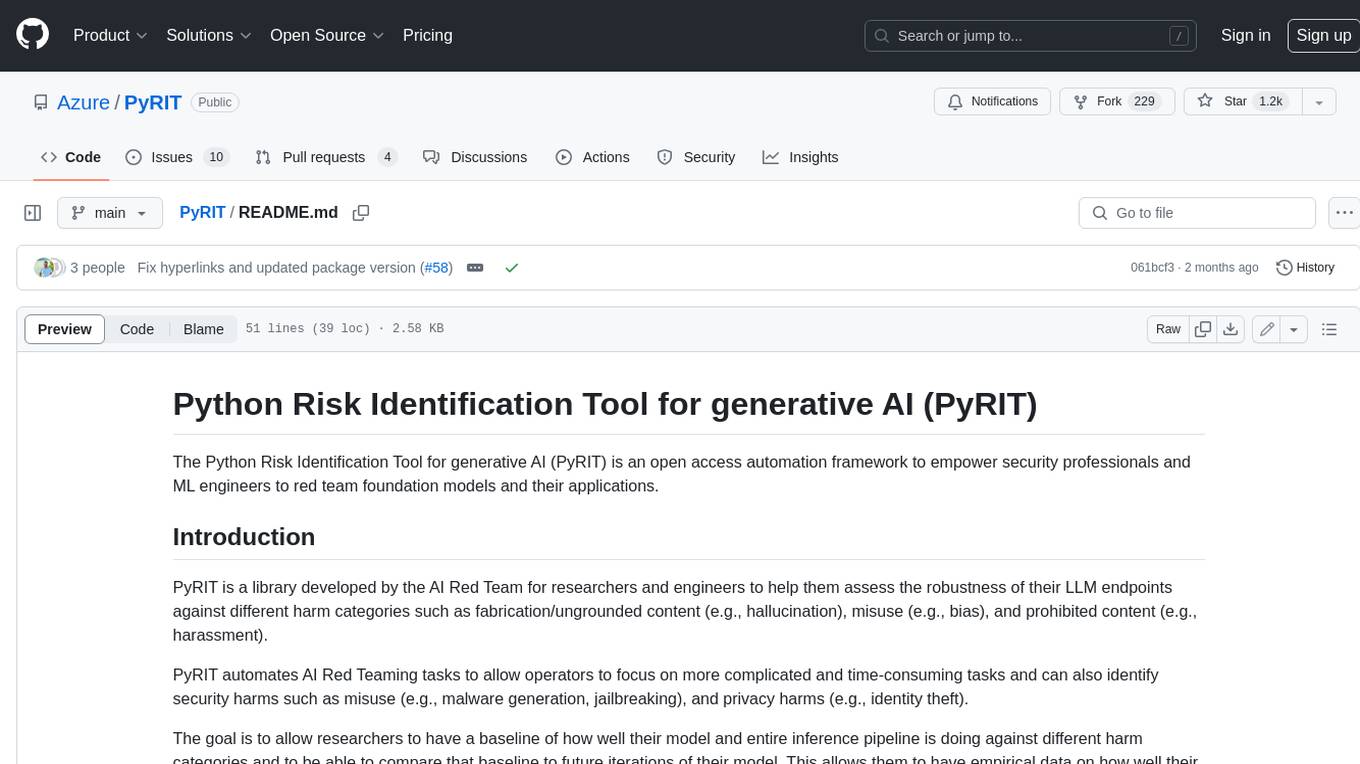
PyRIT
PyRIT is an open access automation framework designed to empower security professionals and ML engineers to red team foundation models and their applications. It automates AI Red Teaming tasks to allow operators to focus on more complicated and time-consuming tasks and can also identify security harms such as misuse (e.g., malware generation, jailbreaking), and privacy harms (e.g., identity theft). The goal is to allow researchers to have a baseline of how well their model and entire inference pipeline is doing against different harm categories and to be able to compare that baseline to future iterations of their model. This allows them to have empirical data on how well their model is doing today, and detect any degradation of performance based on future improvements.
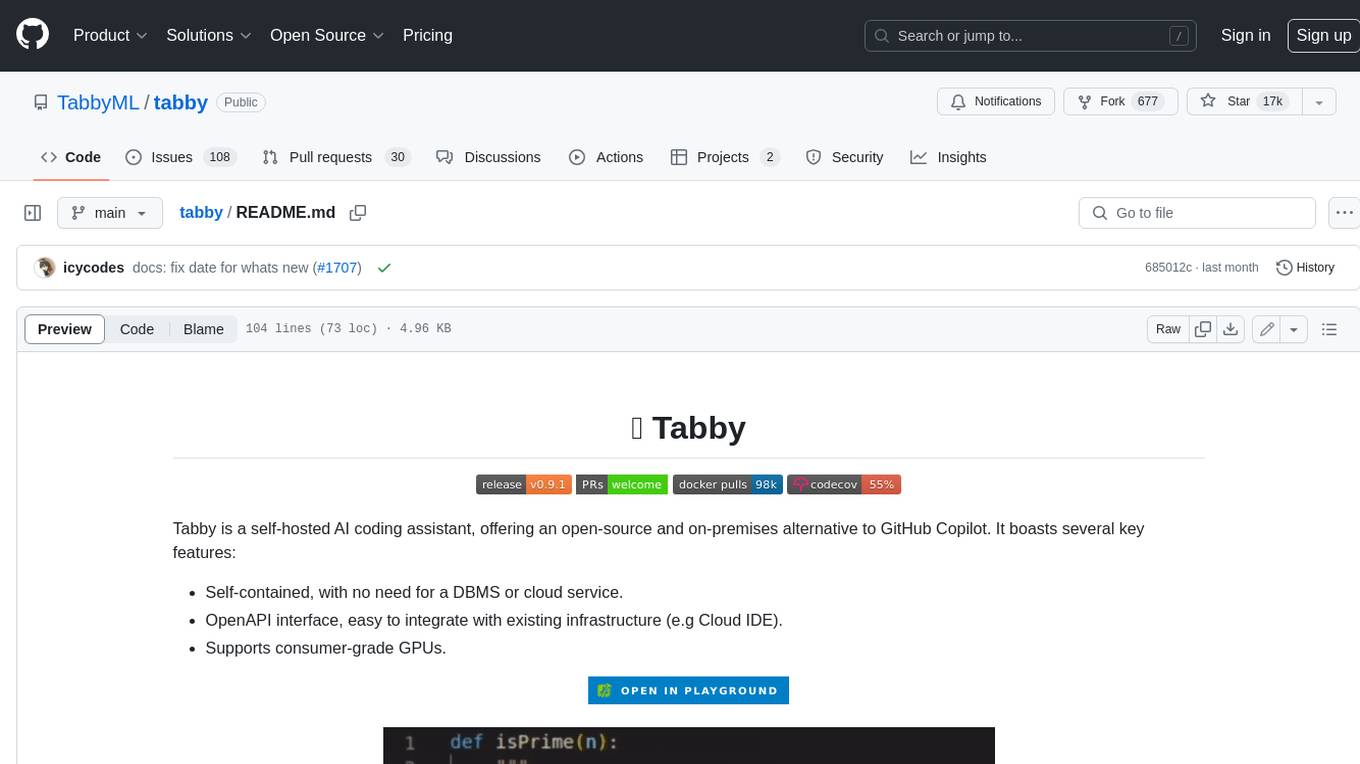
tabby
Tabby is a self-hosted AI coding assistant, offering an open-source and on-premises alternative to GitHub Copilot. It boasts several key features: * Self-contained, with no need for a DBMS or cloud service. * OpenAPI interface, easy to integrate with existing infrastructure (e.g Cloud IDE). * Supports consumer-grade GPUs.
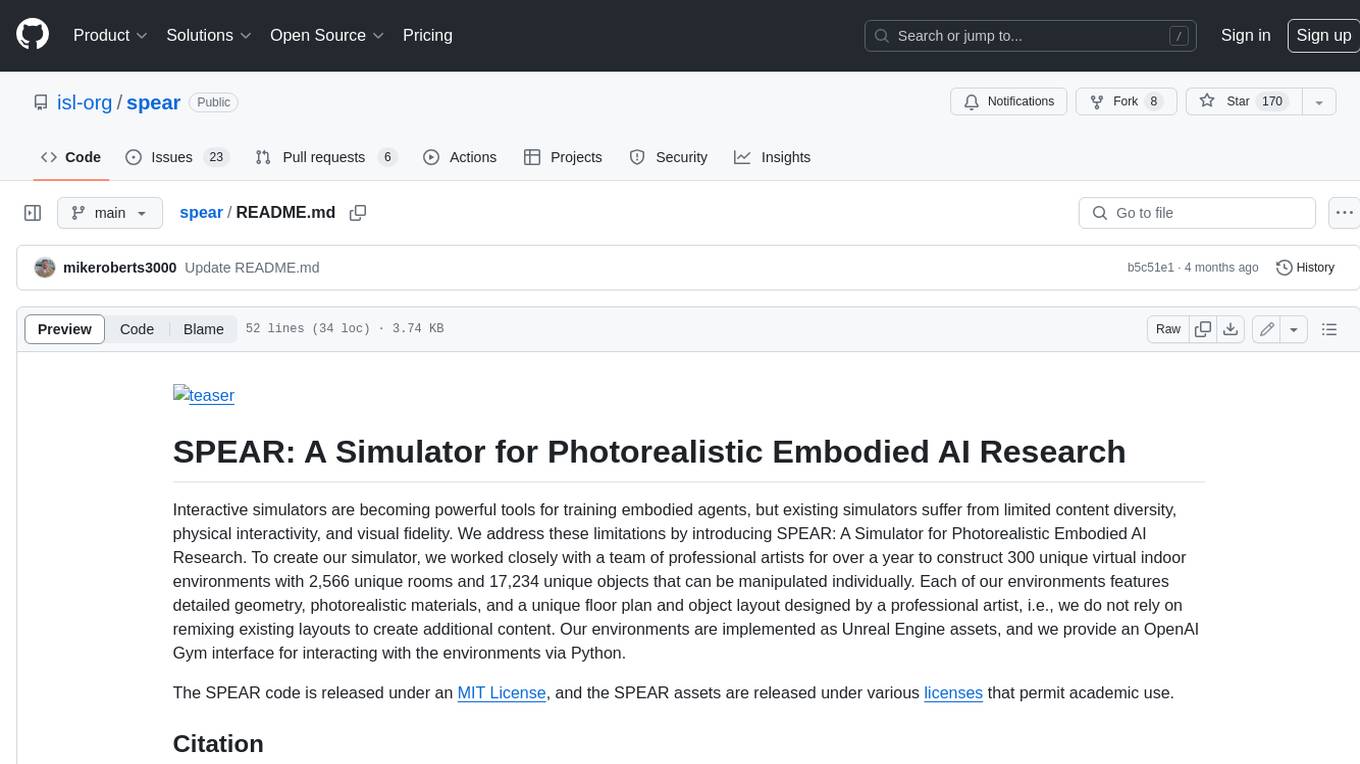
spear
SPEAR (Simulator for Photorealistic Embodied AI Research) is a powerful tool for training embodied agents. It features 300 unique virtual indoor environments with 2,566 unique rooms and 17,234 unique objects that can be manipulated individually. Each environment is designed by a professional artist and features detailed geometry, photorealistic materials, and a unique floor plan and object layout. SPEAR is implemented as Unreal Engine assets and provides an OpenAI Gym interface for interacting with the environments via Python.
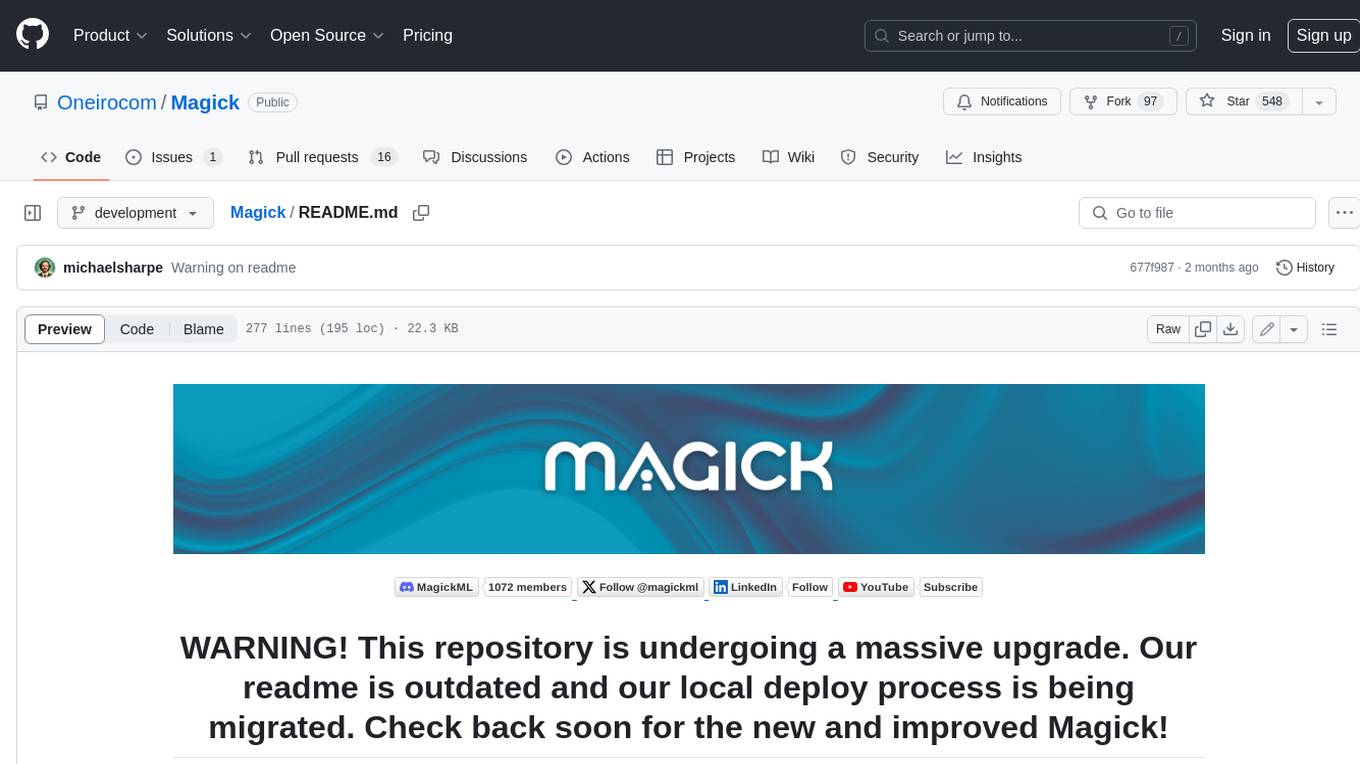
Magick
Magick is a groundbreaking visual AIDE (Artificial Intelligence Development Environment) for no-code data pipelines and multimodal agents. Magick can connect to other services and comes with nodes and templates well-suited for intelligent agents, chatbots, complex reasoning systems and realistic characters.