Best AI tools for< Glucose Monitoring >
Infographic
2 - AI tool Sites
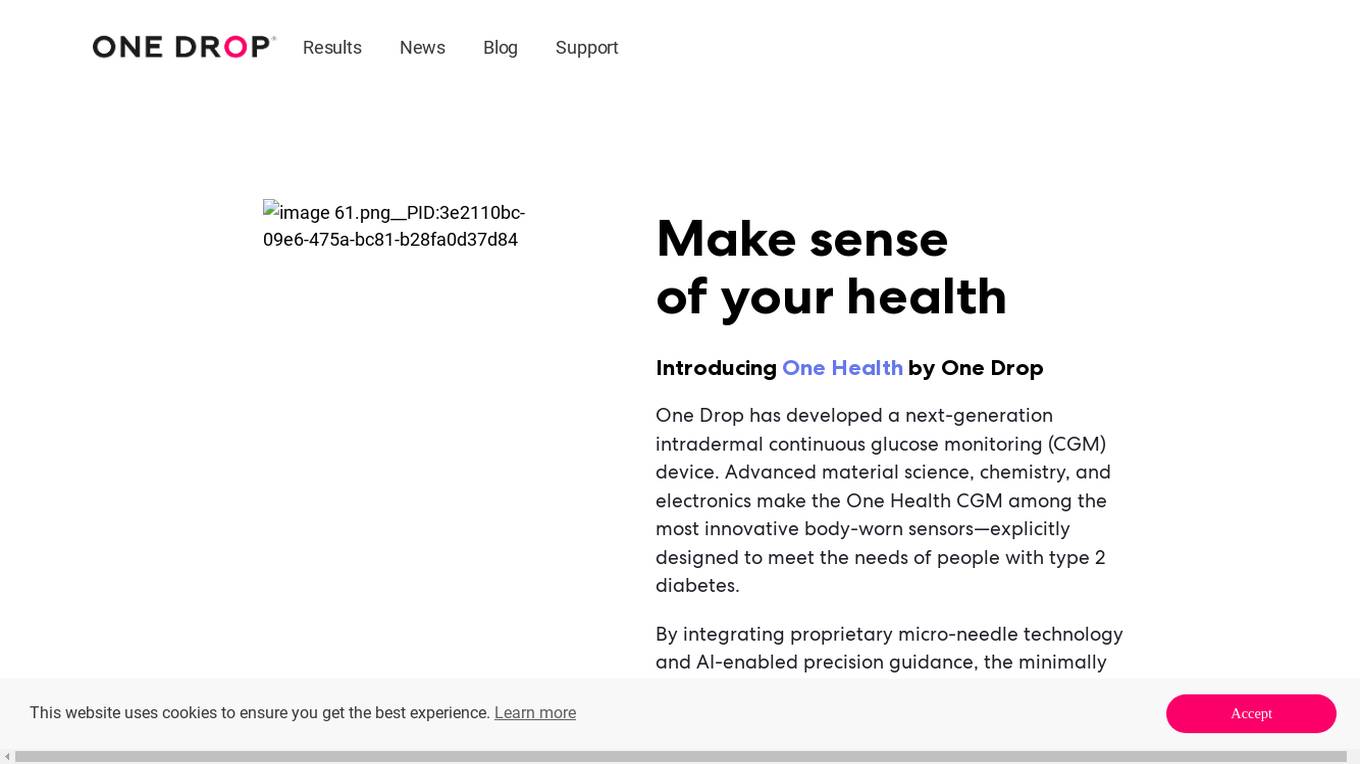
One Drop
One Drop has developed a next-generation intradermal continuous glucose monitoring (CGM) device. Advanced material science, chemistry, and electronics make the One Health CGM among the most innovative body-worn sensors—explicitly designed to meet the needs of people with type 2 diabetes. By integrating proprietary micro-needle technology and AI-enabled precision guidance, the minimally invasive One Health CGM will deliver pain-free, needle-free wear and unprecedented access to a population currently underserved by CGM.
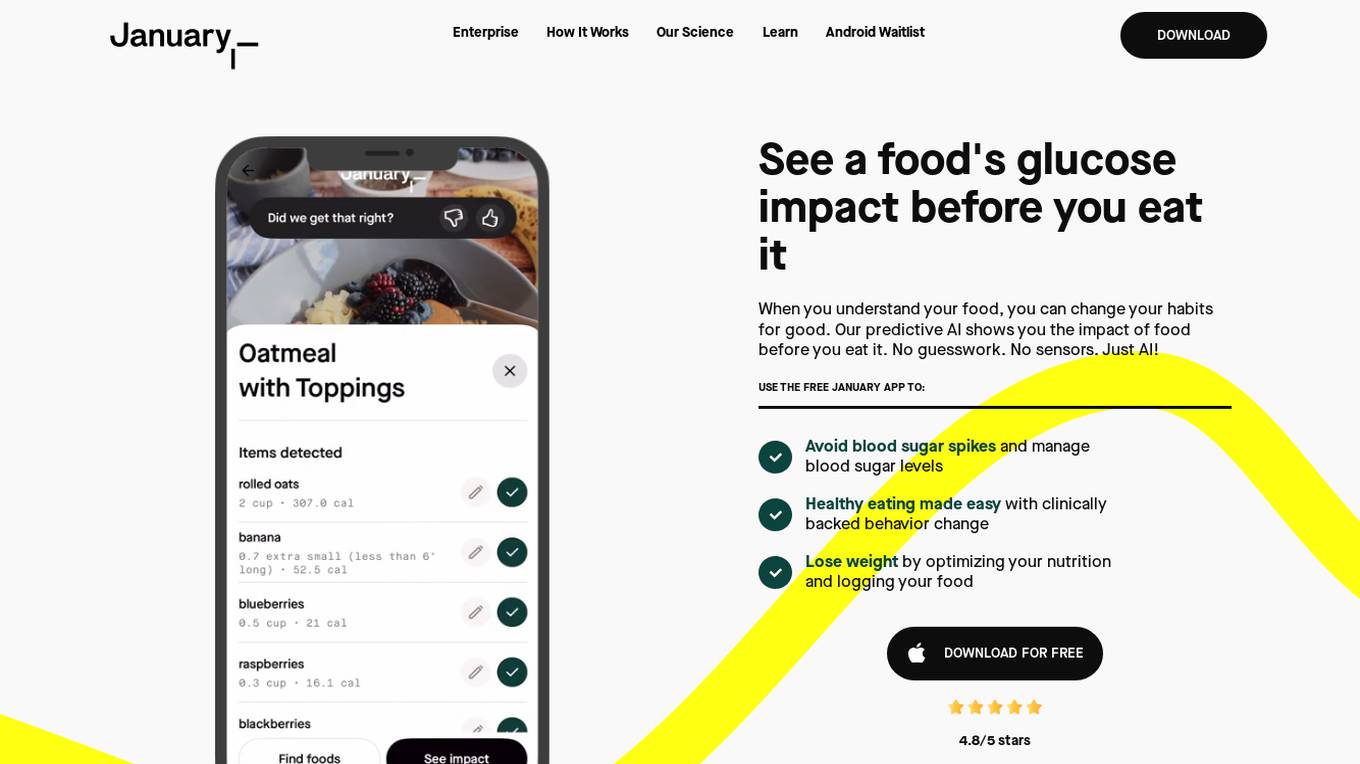
January AI
January AI is a free health app that utilizes predictive AI technology to help users monitor their blood sugar levels and make informed decisions about their nutrition. The app provides real-time insights on the glucose impact of various foods, offers nutrition facts, and suggests healthier alternatives. Founded by Dr. Michael Snyder and Noosheen Hashemi, January AI combines metabolic science with groundbreaking AI to empower users to optimize their nutrition and manage their blood sugar levels effectively.
5 - Open Source Tools
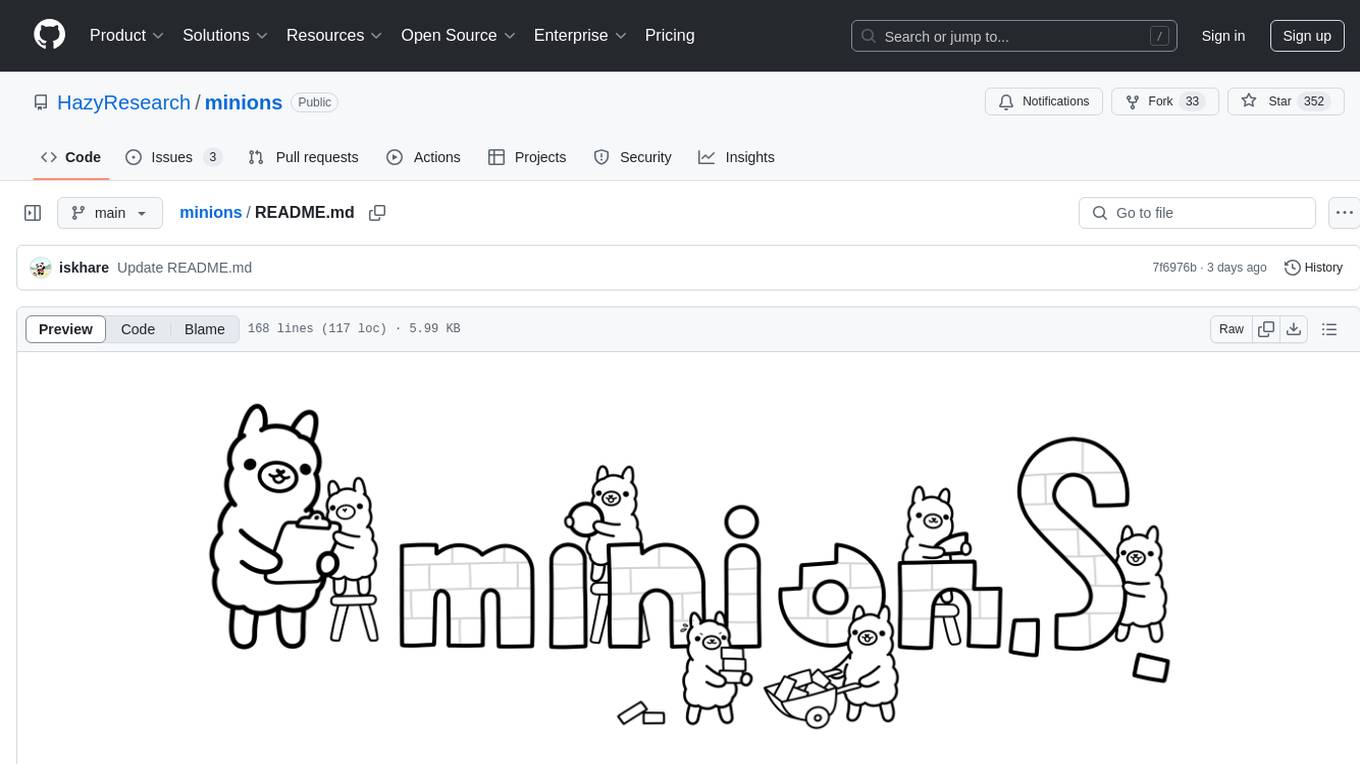
minions
Minions is a communication protocol that enables small on-device models to collaborate with frontier models in the cloud. By only reading long contexts locally, it reduces cloud costs with minimal or no quality degradation. The repository provides a demonstration of the protocol.
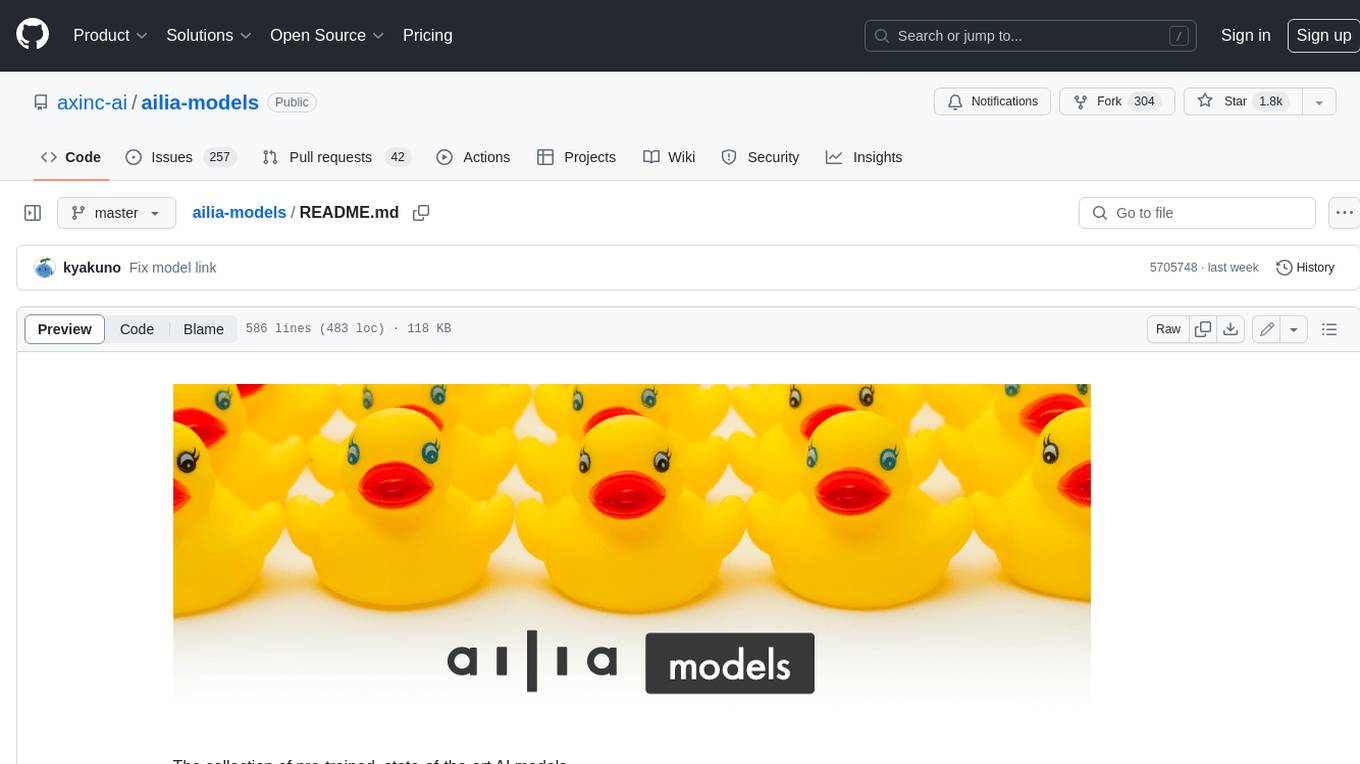
ailia-models
The collection of pre-trained, state-of-the-art AI models. ailia SDK is a self-contained, cross-platform, high-speed inference SDK for AI. The ailia SDK provides a consistent C++ API across Windows, Mac, Linux, iOS, Android, Jetson, and Raspberry Pi platforms. It also supports Unity (C#), Python, Rust, Flutter(Dart) and JNI for efficient AI implementation. The ailia SDK makes extensive use of the GPU through Vulkan and Metal to enable accelerated computing. # Supported models 323 models as of April 8th, 2024
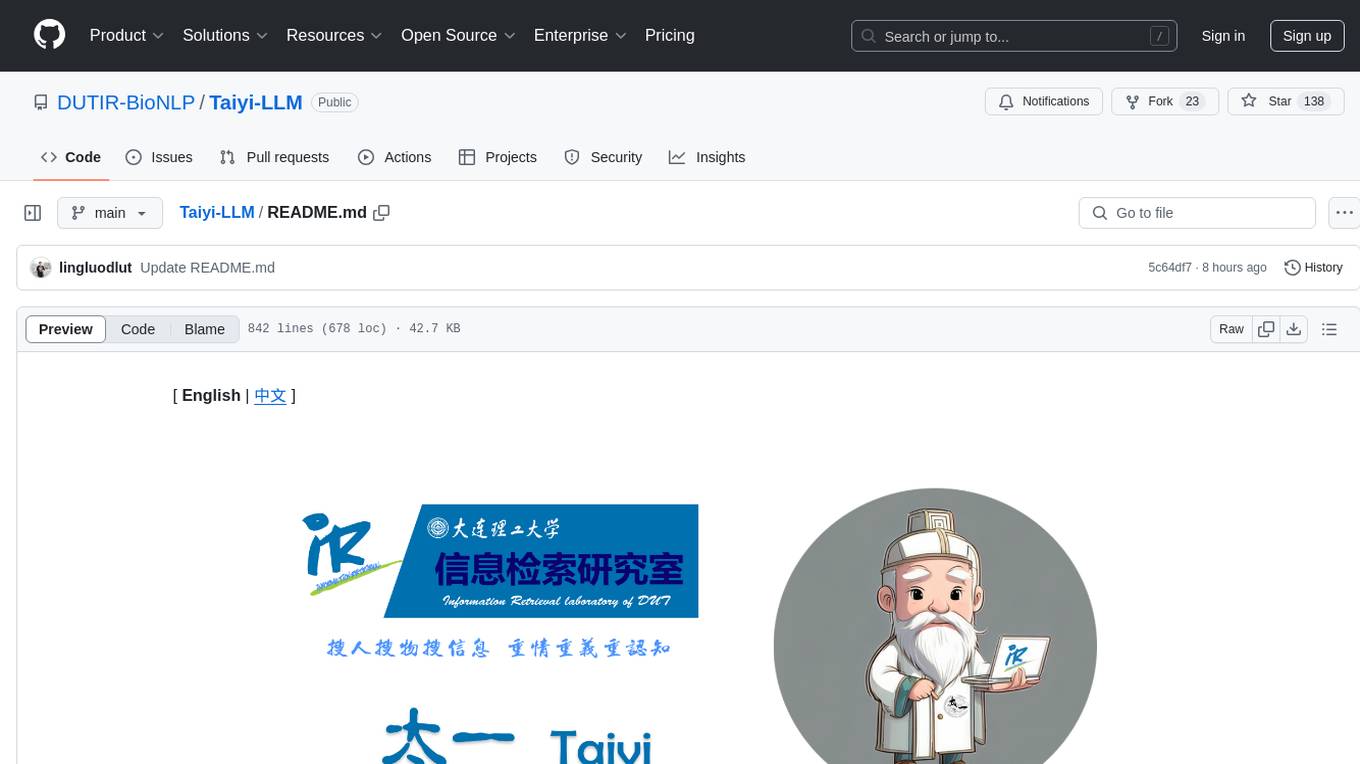
Taiyi-LLM
Taiyi (太一) is a bilingual large language model fine-tuned for diverse biomedical tasks. It aims to facilitate communication between healthcare professionals and patients, provide medical information, and assist in diagnosis, biomedical knowledge discovery, drug development, and personalized healthcare solutions. The model is based on the Qwen-7B-base model and has been fine-tuned using rich bilingual instruction data. It covers tasks such as question answering, biomedical dialogue, medical report generation, biomedical information extraction, machine translation, title generation, text classification, and text semantic similarity. The project also provides standardized data formats, model training details, model inference guidelines, and overall performance metrics across various BioNLP tasks.