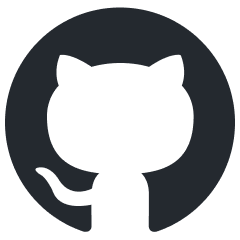
iree-amd-aie
IREE plugin repository for the AMD AIE accelerator
Stars: 87
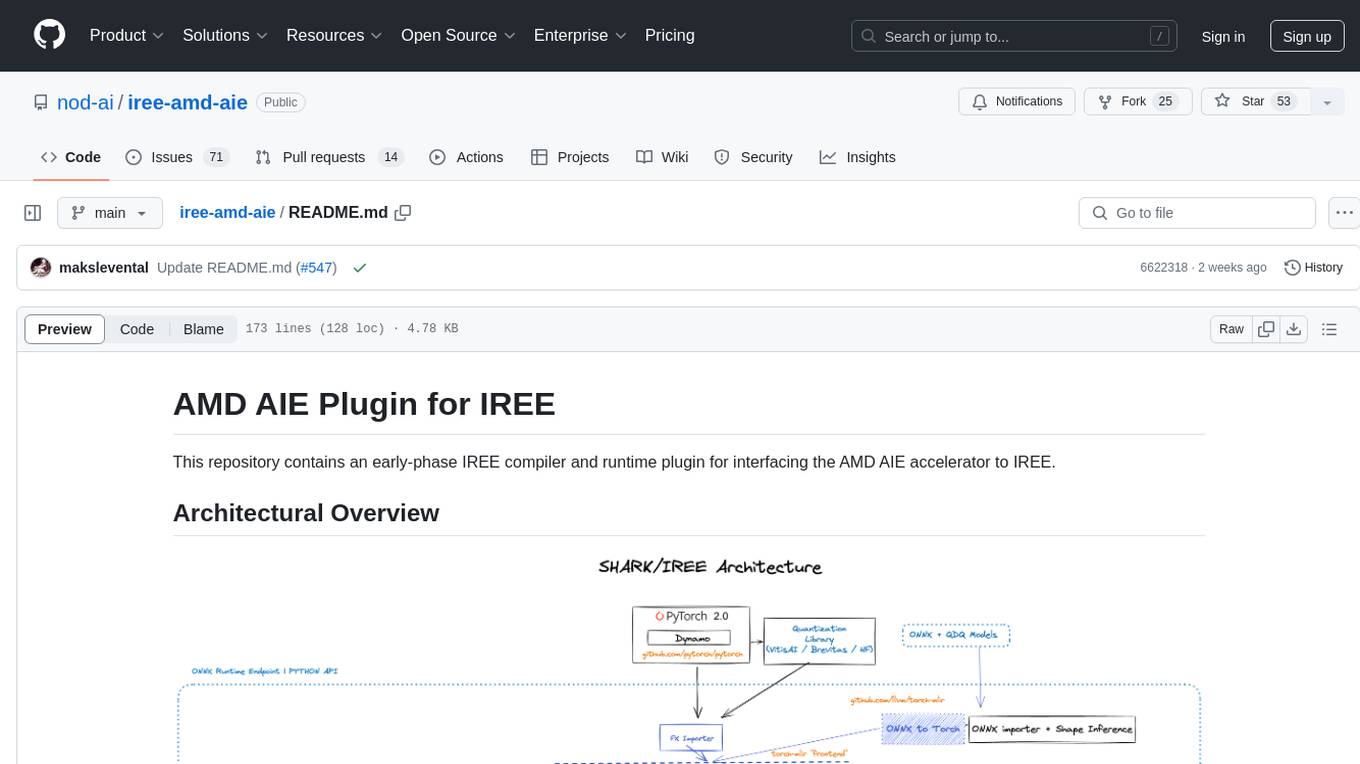
This repository contains an early-phase IREE compiler and runtime plugin for interfacing the AMD AIE accelerator to IREE. It provides architectural overview, developer setup instructions, building guidelines, and runtime driver setup details. The repository focuses on enabling the integration of the AMD AIE accelerator with IREE, offering developers the tools and resources needed to build and run applications leveraging this technology.
README:
This repository contains an early-phase IREE compiler and runtime plugin for targeting AMD NPUs with IREE.
Strong recommendation: check the CI scripts @ .github/workflows - they do a fresh checkout and build on every commit and are written to be read by a non-CI expert.
Either
# ssh
git clone --recursive [email protected]:nod-ai/iree-amd-aie.git
# https
git clone --recursive https://github.com/nod-ai/iree-amd-aie.git
or, if you want a faster checkout,
git \
-c submodule."third_party/torch-mlir".update=none \
-c submodule."third_party/stablehlo".update=none \
-c submodule."third_party/XRT".update=none \
clone \
--recursive \
--shallow-submodules \
[email protected]:nod-ai/iree-amd-aie.git # https://github.com/nod-ai/iree-amd-aie.git
The above avoids cloning entire repo histories for submodules, and skips a few, currently, unused, submodules that are nested in IREE.
Build and install xdna-driver
, use commit 929e8ab
:
git clone [email protected]:amd/xdna-driver.git
cd <root-of-source-tree>
# get code for submodules
git checkout 929e8ab
git submodule update --init --recursive
Follow the instructions to build and install the driver module: xdna-driver.
You will need at least Peano/llvm-aie to be installed in your system to run e2e examples as it's needed for compiling AIE core code. For best performance (but slower compilation times), you will also need Chess.
To install llvm-aie in the current working directory:
bash <path-to-iree-amd-aie>/build_tools/download_peano.sh
Now, you should see a directory named llvm-aie
in your current working directory.
After building IREE, you can then run e2e tests by passing --peano_dir=<path-to-llvm-aie>
to tests, see Testing.
For best performance and to run all tests, you can install Chess in the following way:
- Install Vitis™ AIE Essentials from Ryzen AI Software 1.3 Early Accesss.
tar -xzvf ryzen_ai_1.3.1-ea-lnx64-20250116.tgz cd ryzen_ai_1.3.1-ea-lnx64-20250116 mkdir vitis_aie_essentials mv vitis_aie_essentials*.whl vitis_aie_essentials cd vitis_aie_essentials unzip vitis_aie_essentials*.whl
- Set up an AI Engine license.
- Get a local license for AI Engine tools from https://www.xilinx.com/getlicense.
- Copy your license file (Xilinx.lic) to your preferred location, e.g.
/opt/Xilinx.lic
.
After building IREE, you can then run e2e tests by passing --vitis_dir=<path-to-vitis-aie-essentials>
to tests, see Testing. Note however that you need to export the path to the AI Engine license for successful compilation:
export XILINXD_LICENSE_FILE=<path-to-Xilinx.lic>
cd iree-amd-aie
cmake \
-B <WHERE_YOU_WOULD_LIKE_TO_BUILD> \
-S third_party/iree \
-DIREE_CMAKE_PLUGIN_PATHS=$PWD \
-DIREE_BUILD_PYTHON_BINDINGS=ON \
-DIREE_INPUT_STABLEHLO=OFF \
-DIREE_INPUT_TORCH=OFF \
-DIREE_INPUT_TOSA=OFF \
-DIREE_HAL_DRIVER_DEFAULTS=OFF \
-DIREE_TARGET_BACKEND_DEFAULTS=OFF \
-DIREE_TARGET_BACKEND_LLVM_CPU=ON \
-DIREE_BUILD_TESTS=ON \
-DIREE_EXTERNAL_HAL_DRIVERS=xrt-lite \
-DCMAKE_INSTALL_PREFIX=<WHERE_YOU_WOULD_LIKE_TO_INSTALL>
cmake --build <WHERE_YOU_WOULD_LIKE_TO_BUILD>
The bare minimum configure command for IREE with the amd-aie plugin
cmake \
-B <WHERE_YOU_WOULD_LIKE_TO_BUILD> \
-S <IREE_REPO_SRC_DIR> \
-DIREE_CMAKE_PLUGIN_PATHS=<IREE_AMD_AIE_REPO_SRC_DIR> \
-DIREE_BUILD_PYTHON_BINDINGS=ON
Very likely, you will want to use ccache
and lld
(or some other modern linker like mold)
-DCMAKE_C_COMPILER_LAUNCHER=ccache \
-DCMAKE_CXX_COMPILER_LAUNCHER=ccache \
-DCMAKE_EXE_LINKER_FLAGS="-fuse-ld=lld" \
-DCMAKE_SHARED_LINKER_FLAGS="-fuse-ld=lld"
If you don't plan on using any of IREE's frontends or backends/targets (e.g., you're doing work on this code base itself),
you can opt-out of everything (except the llvm-cpu
backend) with
-DIREE_INPUT_STABLEHLO=OFF \
-DIREE_INPUT_TORCH=OFF \
-DIREE_INPUT_TOSA=OFF \
-DIREE_HAL_DRIVER_DEFAULTS=OFF \
-DIREE_TARGET_BACKEND_DEFAULTS=OFF \
-DIREE_TARGET_BACKEND_LLVM_CPU=ON
With the above you can also skip cloning the stablehlo
and torch-mlir
submodules/repos but in this case you will need to add
-DIREE_ERROR_ON_MISSING_SUBMODULES=OFF
If you're "bringing your own LLVM", i.e., you have a prebuilt/compiled distribution of LLVM you'd like to use, you can add
-DIREE_BUILD_BUNDLED_LLVM=OFF
In this case you will need lit
somewhere in your environment and you will need to add to CMake -DLLVM_EXTERNAL_LIT=<SOMEWHERE>
(e.g., pip install lit; SOMEWHERE=$(which lit)
).
See Bringing your own LLVM below for more information on using prebuilt/compiled distributions of LLVM.
Lit tests (i.e., compiler tests) specific to AIE can be run with something like
cd <WHERE_YOU_WOULD_LIKE_TO_BUILD>
ctest -R amd-aie --output-on-failure -j 10
(the -j 10
runs 10
tests in parallel)
Other tests, which run on device, are in the build_tools
subdirectory.
See build_tools/ci/run_all_runtime_tests.sh for an example script that shows how to run all the runtime tests.
When using a pre-built distribution of LLVM, getting the right/matching build, that works with IREE, is tough (besides the commit hash, there are various flags to set).
To enable adventurous users to avail themselves of -DIREE_BUILD_BUNDLED_LLVM=OFF
we cache/store/save the LLVM distribution for every successful CI run.
These can then be downloaded by checking the artifacts section of any recent CI run's Summary page:
You can turn on HAL API tracing by adding to CMake:
-DIREE_ENABLE_RUNTIME_TRACING=ON
-DIREE_TRACING_PROVIDER=console
// optional but recommended
-DIREE_TRACING_CONSOLE_FLUSH=1
This will you show you all the HAL APIs that have IREE_TRACE_ZONE_BEGIN ... IREE_TRACE_ZONE_END
that are hit during a run/execution (of, e.g., iree-run-module
).
You can turn on VM tracing by adding to CMake:
-DIREE_VM_EXECUTION_TRACING_ENABLE=1
-DIREE_VM_EXECUTION_TRACING_FORCE_ENABLE=1
// optional
-DIREE_VM_EXECUTION_TRACING_SRC_LOC_ENABLE=1
This will show you all of the VM dispatches that actually occur during a run/execution.
Note, this is roughly equivalent to passing --compile-to=vm
to iree-compile
.
For Tasks:
Click tags to check more tools for each tasksFor Jobs:
Alternative AI tools for iree-amd-aie
Similar Open Source Tools
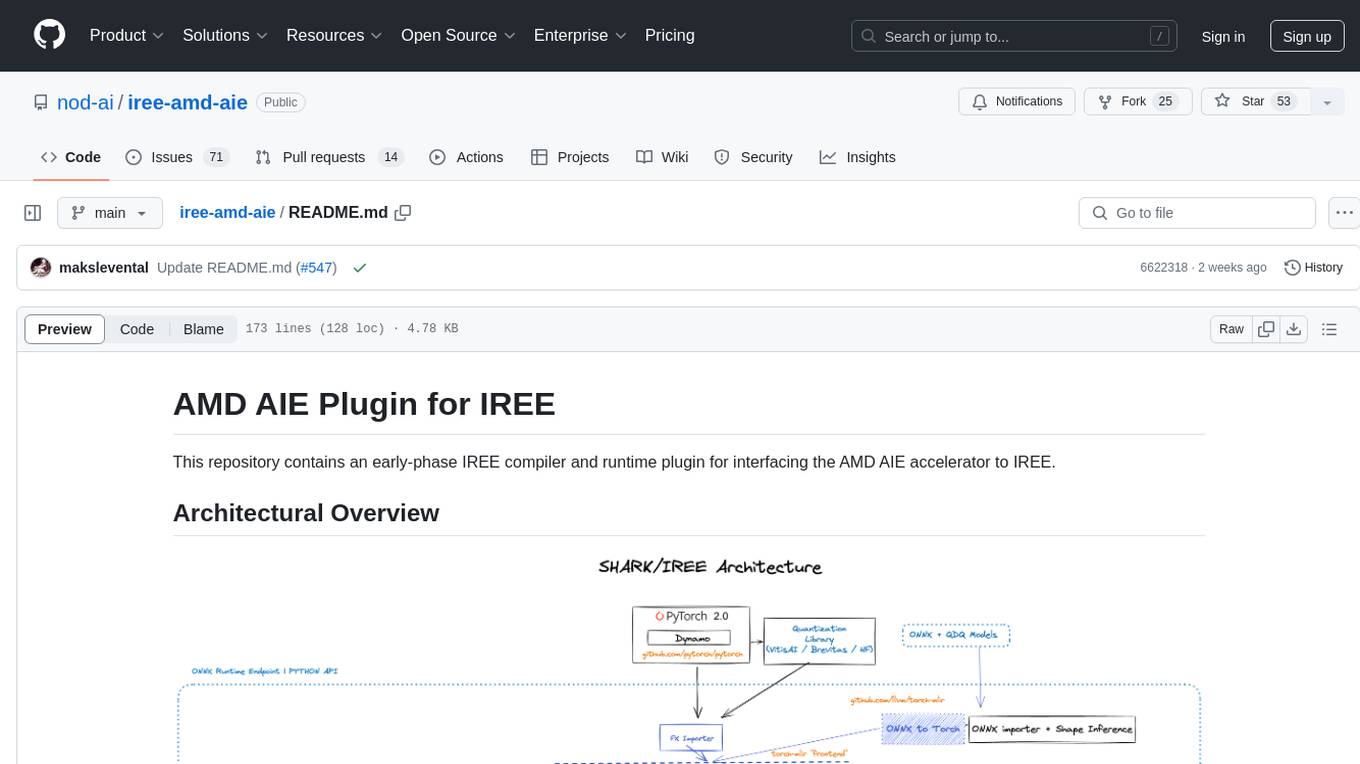
iree-amd-aie
This repository contains an early-phase IREE compiler and runtime plugin for interfacing the AMD AIE accelerator to IREE. It provides architectural overview, developer setup instructions, building guidelines, and runtime driver setup details. The repository focuses on enabling the integration of the AMD AIE accelerator with IREE, offering developers the tools and resources needed to build and run applications leveraging this technology.
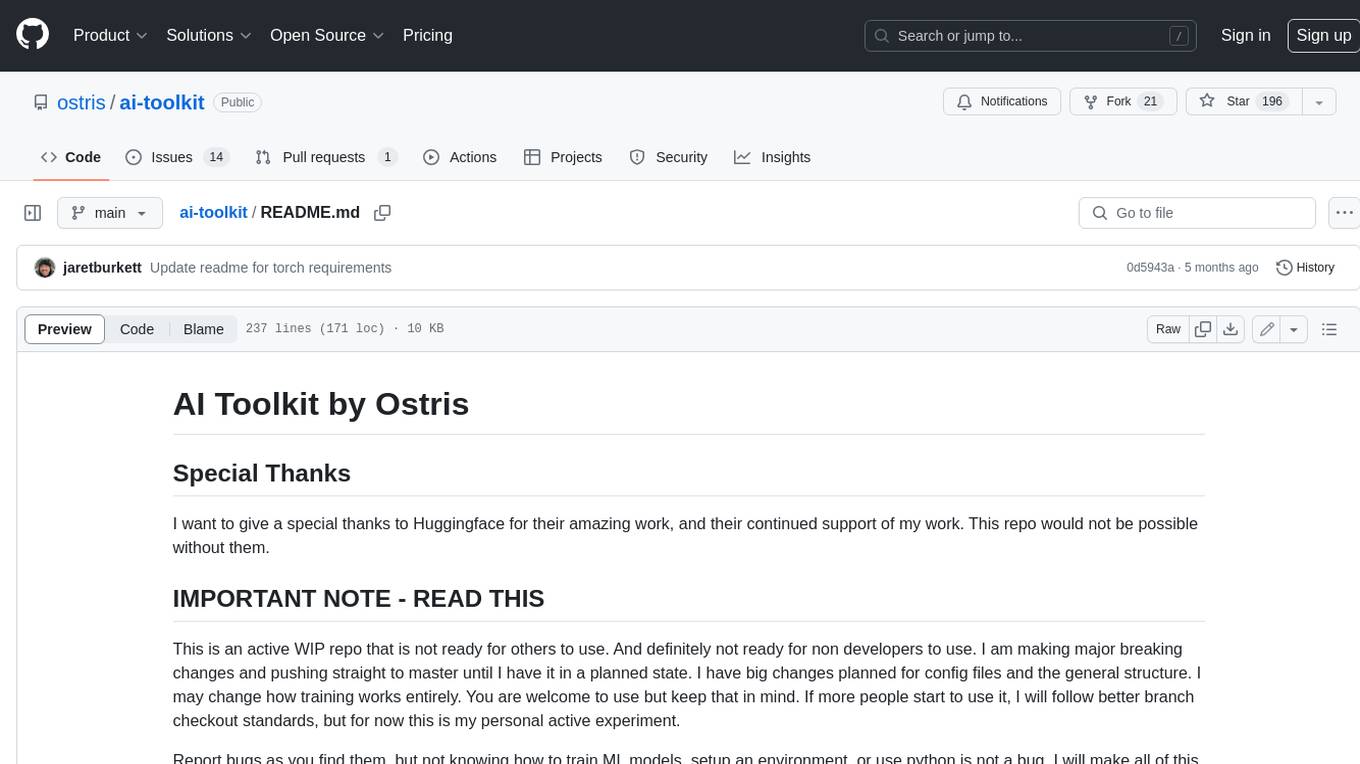
ai-toolkit
The AI Toolkit by Ostris is a collection of tools for machine learning, specifically designed for image generation, LoRA (latent representations of attributes) extraction and manipulation, and model training. It provides a user-friendly interface and extensive documentation to make it accessible to both developers and non-developers. The toolkit is actively under development, with new features and improvements being added regularly. Some of the key features of the AI Toolkit include: - Batch Image Generation: Allows users to generate a batch of images based on prompts or text files, using a configuration file to specify the desired settings. - LoRA (lierla), LoCON (LyCORIS) Extractor: Facilitates the extraction of LoRA and LoCON representations from pre-trained models, enabling users to modify and manipulate these representations for various purposes. - LoRA Rescale: Provides a tool to rescale LoRA weights, allowing users to adjust the influence of specific attributes in the generated images. - LoRA Slider Trainer: Enables the training of LoRA sliders, which can be used to control and adjust specific attributes in the generated images, offering a powerful tool for fine-tuning and customization. - Extensions: Supports the creation and sharing of custom extensions, allowing users to extend the functionality of the toolkit with their own tools and scripts. - VAE (Variational Auto Encoder) Trainer: Facilitates the training of VAEs for image generation, providing users with a tool to explore and improve the quality of generated images. The AI Toolkit is a valuable resource for anyone interested in exploring and utilizing machine learning for image generation and manipulation. Its user-friendly interface, extensive documentation, and active development make it an accessible and powerful tool for both beginners and experienced users.
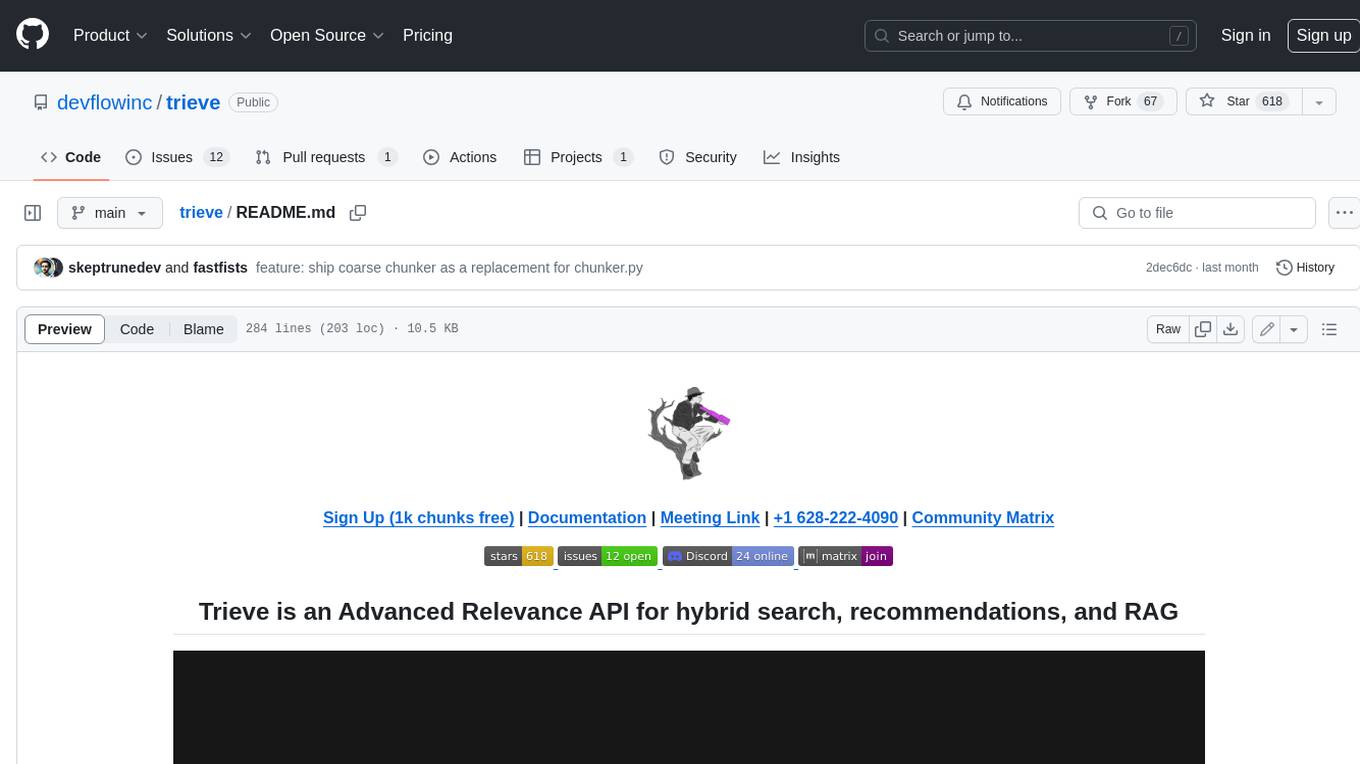
trieve
Trieve is an advanced relevance API for hybrid search, recommendations, and RAG. It offers a range of features including self-hosting, semantic dense vector search, typo tolerant full-text/neural search, sub-sentence highlighting, recommendations, convenient RAG API routes, the ability to bring your own models, hybrid search with cross-encoder re-ranking, recency biasing, tunable popularity-based ranking, filtering, duplicate detection, and grouping. Trieve is designed to be flexible and customizable, allowing users to tailor it to their specific needs. It is also easy to use, with a simple API and well-documented features.
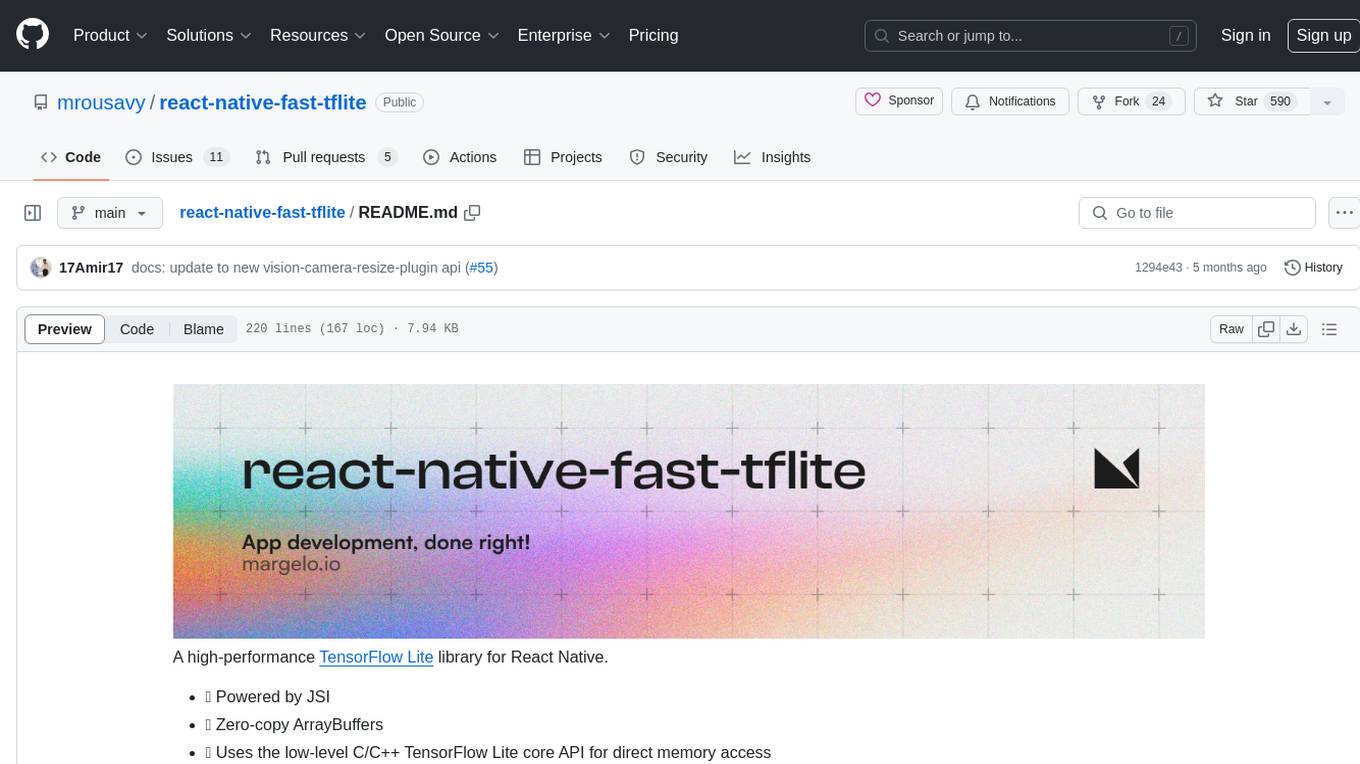
react-native-fast-tflite
A high-performance TensorFlow Lite library for React Native that utilizes JSI for power, zero-copy ArrayBuffers for efficiency, and low-level C/C++ TensorFlow Lite core API for direct memory access. It supports swapping out TensorFlow Models at runtime and GPU-accelerated delegates like CoreML/Metal/OpenGL. Easy VisionCamera integration allows for seamless usage. Users can load TensorFlow Lite models, interpret input and output data, and utilize GPU Delegates for faster computation. The library is suitable for real-time object detection, image classification, and other machine learning tasks in React Native applications.
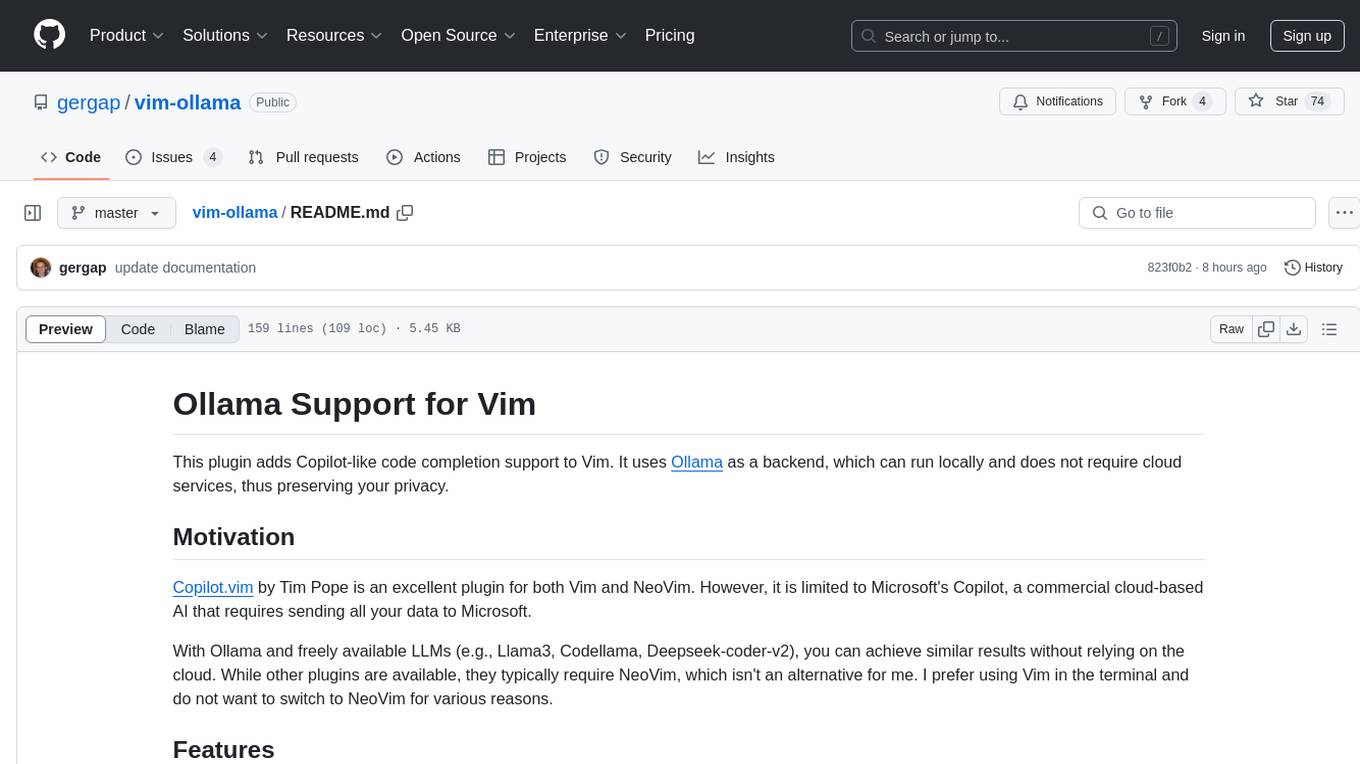
vim-ollama
The 'vim-ollama' plugin for Vim adds Copilot-like code completion support using Ollama as a backend, enabling intelligent AI-based code completion and integrated chat support for code reviews. It does not rely on cloud services, preserving user privacy. The plugin communicates with Ollama via Python scripts for code completion and interactive chat, supporting Vim only. Users can configure LLM models for code completion tasks and interactive conversations, with detailed installation and usage instructions provided in the README.
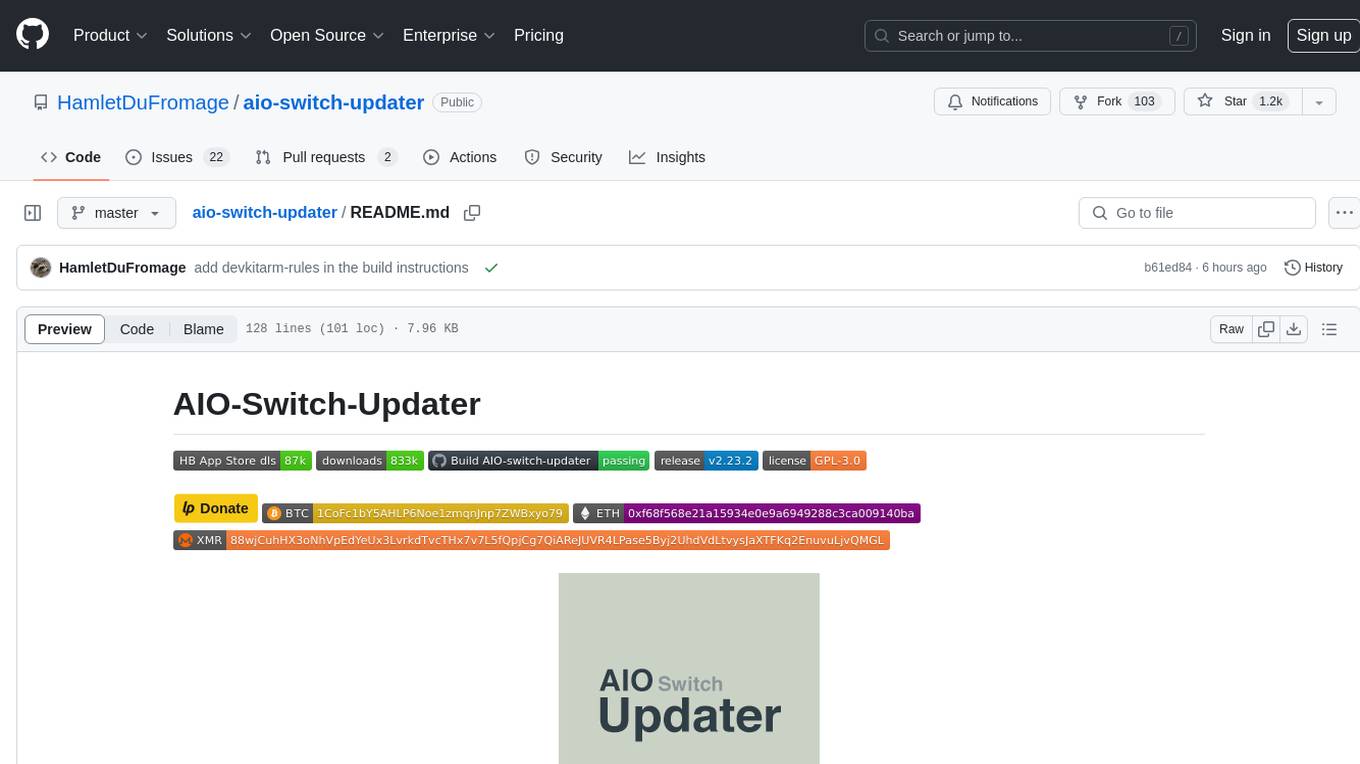
aio-switch-updater
AIO-Switch-Updater is a Nintendo Switch homebrew app that allows users to download and update custom firmware, firmware files, cheat codes, and more. It supports Atmosphère, ReiNX, and SXOS on both unpatched and patched Switches. The app provides features like updating CFW with custom RCM payload, updating Hekate/payload, custom downloads, downloading firmwares and cheats, and various tools like rebooting to specific payload, changing color schemes, consulting cheat codes, and more. Users can contribute by submitting PRs and suggestions, and the app supports localization. It does not host or distribute any files and gives special thanks to contributors and supporters.
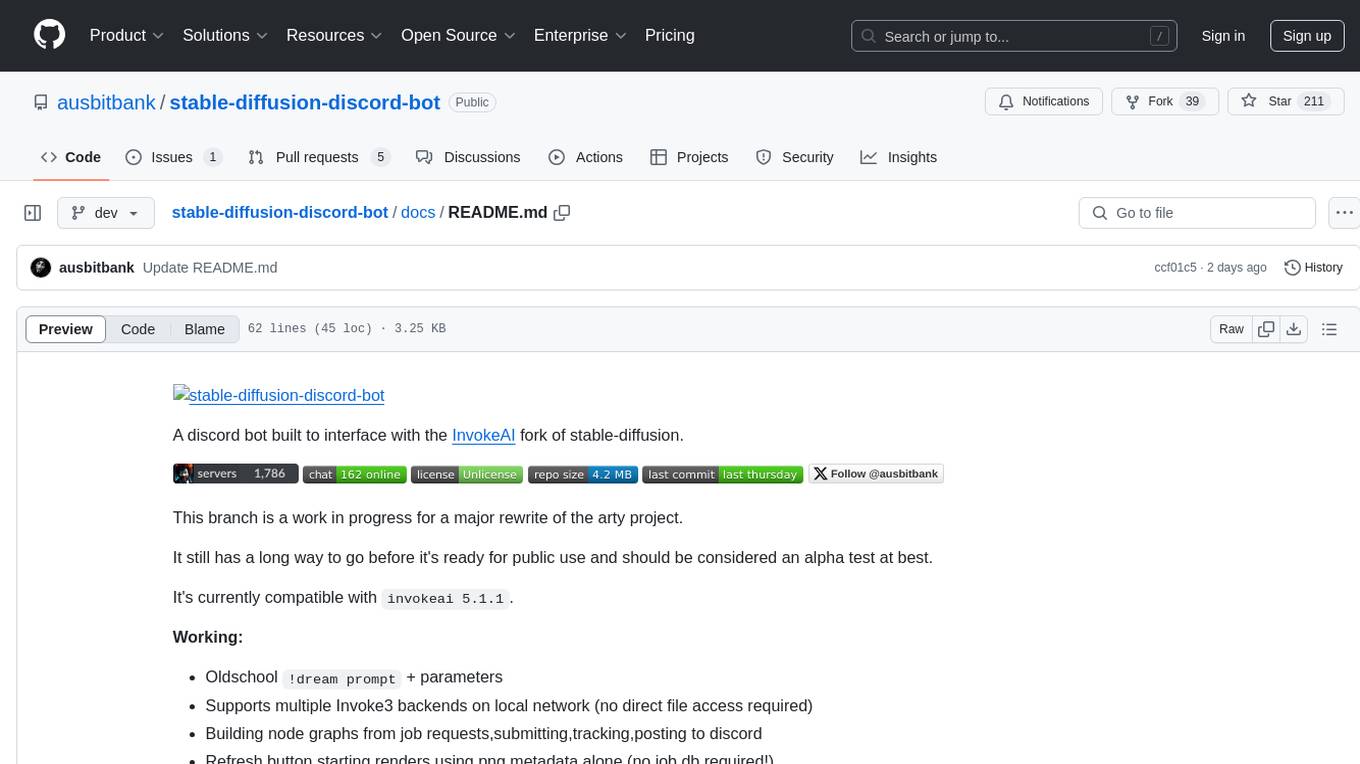
stable-diffusion-discord-bot
A discord bot built to interface with the InvokeAI fork of stable-diffusion. It is a work in progress for a major rewrite of the arty project, compatible with `invokeai 5.1.1`. The bot supports various functionalities like building node graphs from job requests, refreshing renders using png metadata, removing backgrounds, job progress tracking, and LLM integration. Users can install custom invokeai nodes for advanced functionality and launch the bot natively or with docker. Patches and pull requests are welcomed.
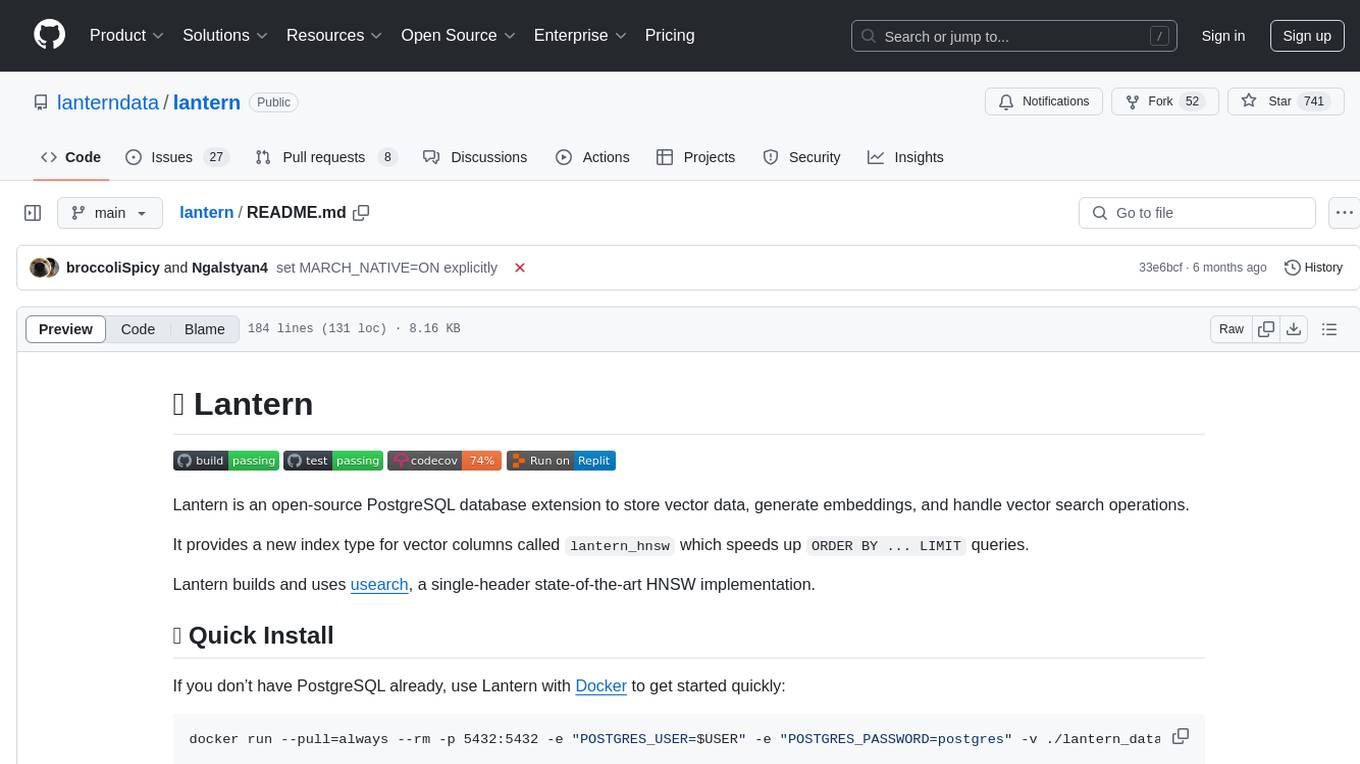
lantern
Lantern is an open-source PostgreSQL database extension designed to store vector data, generate embeddings, and handle vector search operations efficiently. It introduces a new index type called 'lantern_hnsw' for vector columns, which speeds up 'ORDER BY ... LIMIT' queries. Lantern utilizes the state-of-the-art HNSW implementation called usearch. Users can easily install Lantern using Docker, Homebrew, or precompiled binaries. The tool supports various distance functions, index construction parameters, and operator classes for efficient querying. Lantern offers features like embedding generation, interoperability with pgvector, parallel index creation, and external index graph generation. It aims to provide superior performance metrics compared to other similar tools and has a roadmap for future enhancements such as cloud-hosted version, hardware-accelerated distance metrics, industry-specific application templates, and support for version control and A/B testing of embeddings.
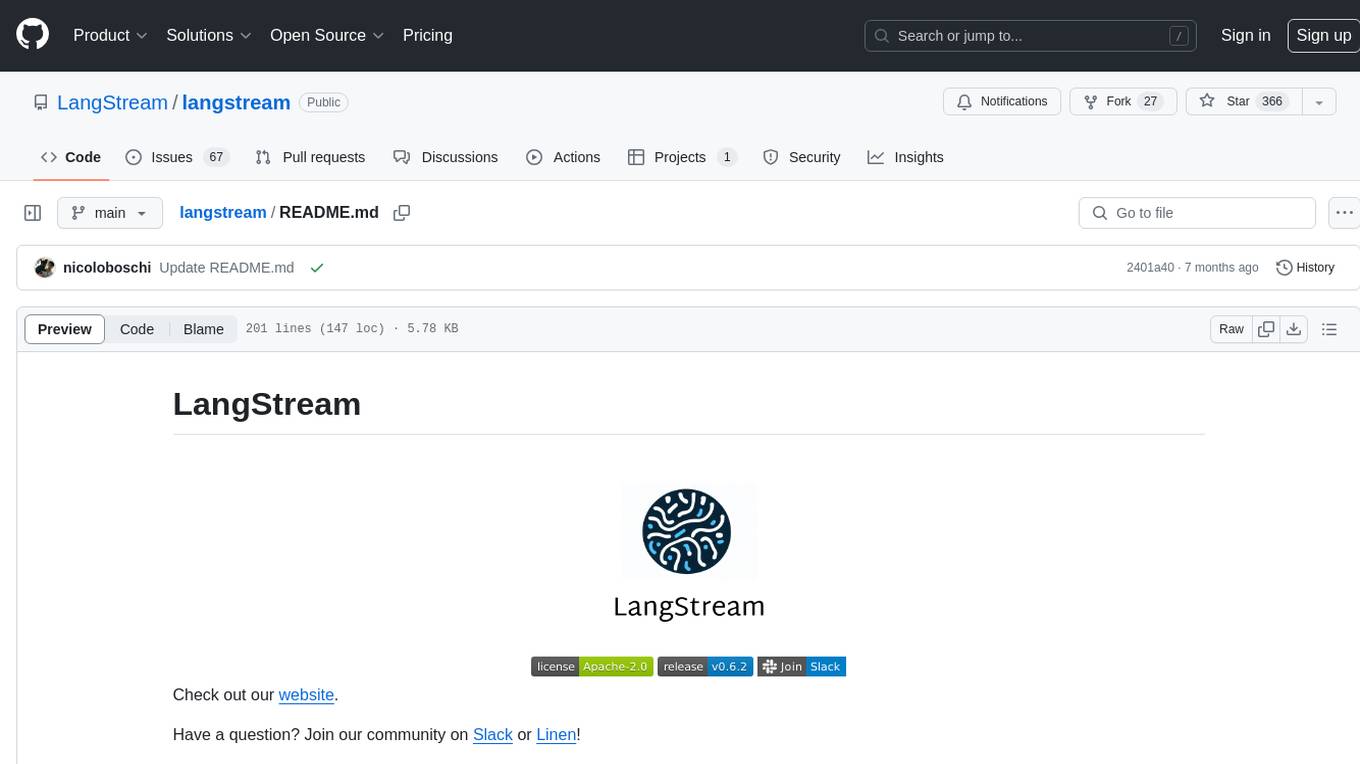
langstream
LangStream is a tool for natural language processing tasks, providing a CLI for easy installation and usage. Users can try sample applications like Chat Completions and create their own applications using the developer documentation. It supports running on Kubernetes for production-ready deployment, with support for various Kubernetes distributions and external components like Apache Kafka or Apache Pulsar cluster. Users can deploy LangStream locally using minikube and manage the cluster with mini-langstream. Development requirements include Docker, Java 17, Git, Python 3.11+, and PIP, with the option to test local code changes using mini-langstream.
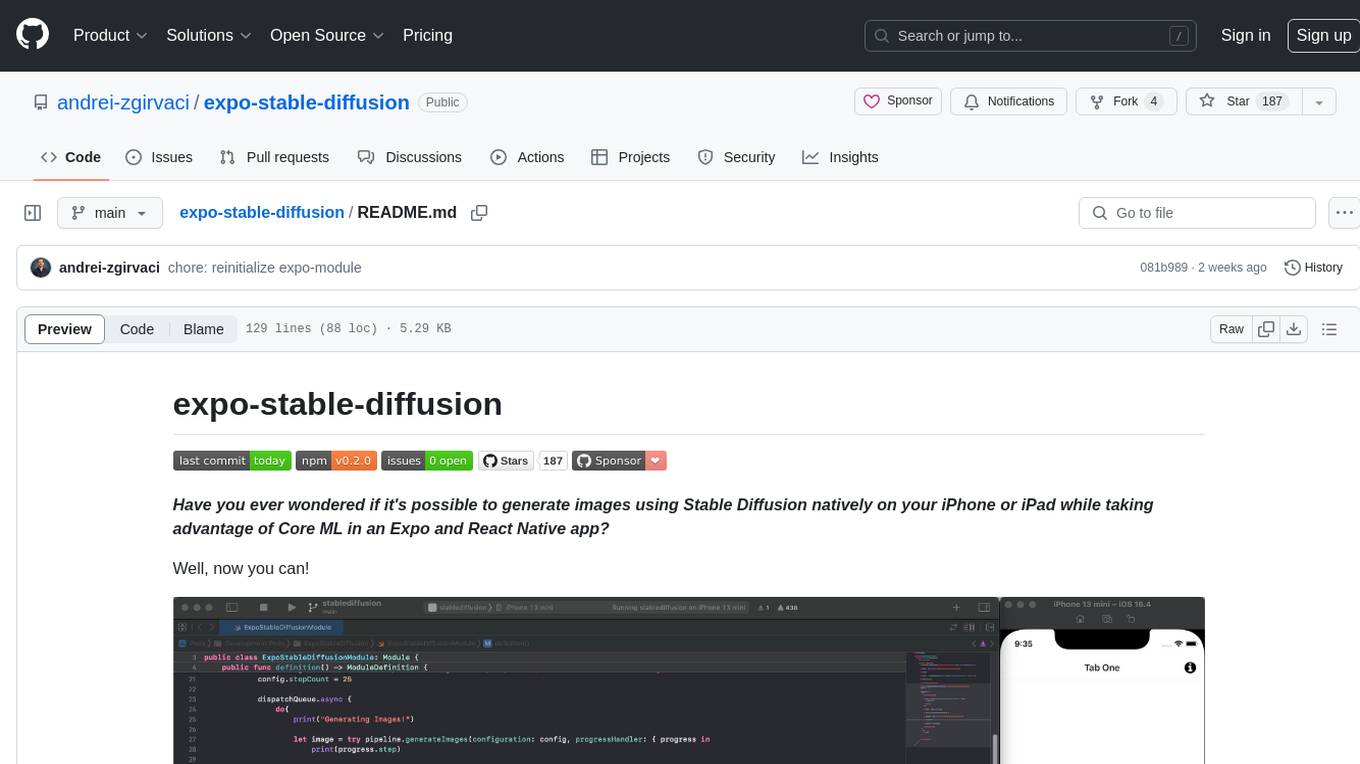
expo-stable-diffusion
The `expo-stable-diffusion` repository provides a tool for generating images using Stable Diffusion natively on iOS devices within Expo and React Native apps. Users can install and configure the module to create images based on prompts. The repository includes information on updating iOS deployment targets, enabling increased memory limits, and building iOS apps. Additionally, users can obtain Stable Diffusion models from various sources. The repository also addresses troubleshooting tips related to model load times and image generation durations. The developer seeks sponsorship to further enhance the project, including adding Android support.
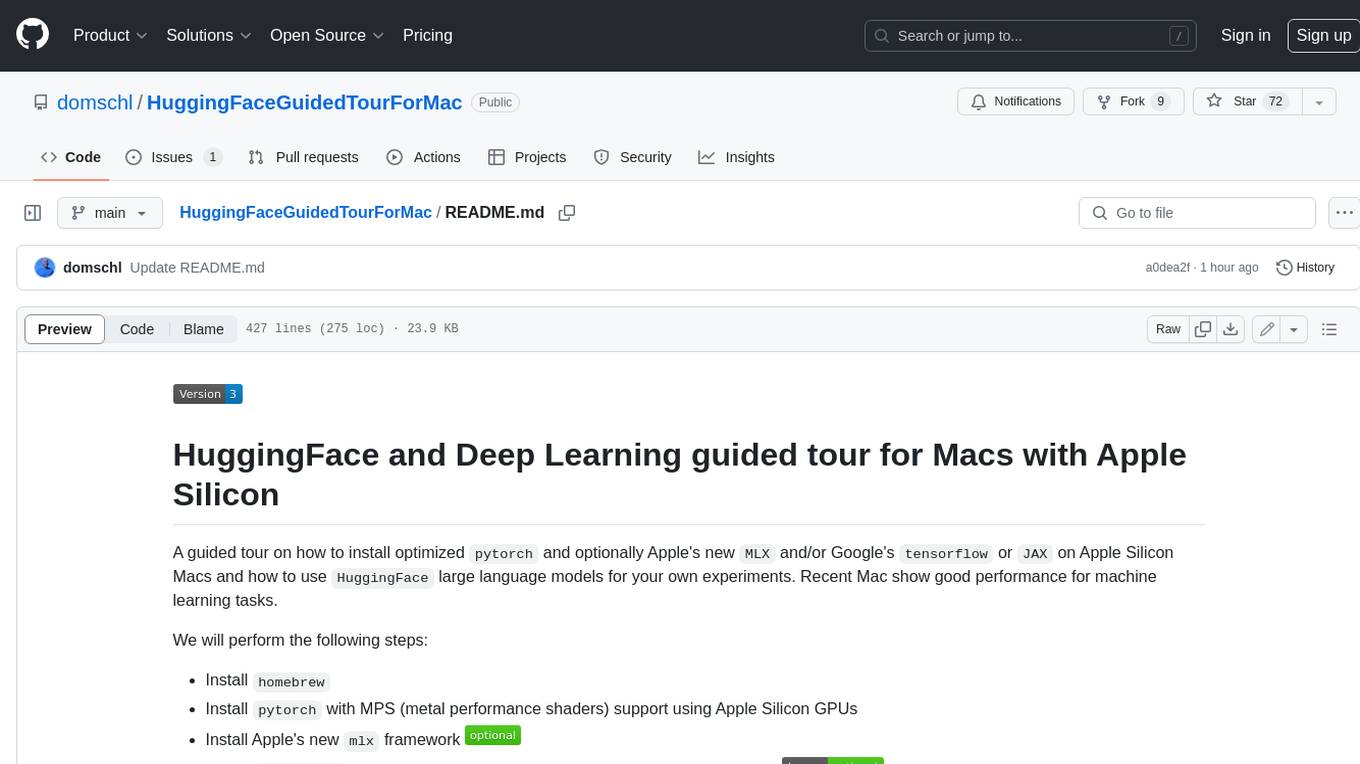
HuggingFaceGuidedTourForMac
HuggingFaceGuidedTourForMac is a guided tour on how to install optimized pytorch and optionally Apple's new MLX, JAX, and TensorFlow on Apple Silicon Macs. The repository provides steps to install homebrew, pytorch with MPS support, MLX, JAX, TensorFlow, and Jupyter lab. It also includes instructions on running large language models using HuggingFace transformers. The repository aims to help users set up their Macs for deep learning experiments with optimized performance.
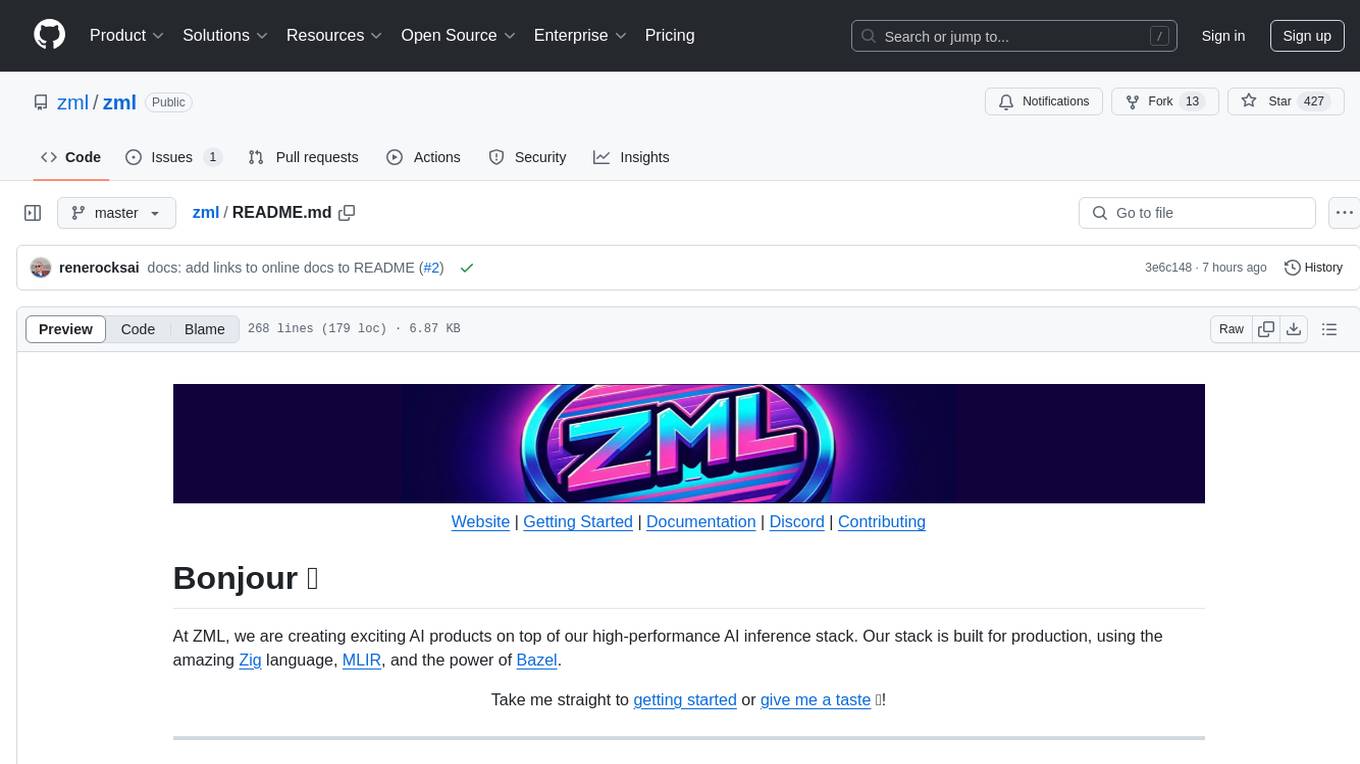
zml
ZML is a high-performance AI inference stack built for production, using Zig language, MLIR, and Bazel. It allows users to create exciting AI projects, run pre-packaged models like MNIST, TinyLlama, OpenLLama, and Meta Llama, and compile models for accelerator runtimes. Users can also run tests, explore examples, and contribute to the project. ZML is licensed under the Apache 2.0 license.
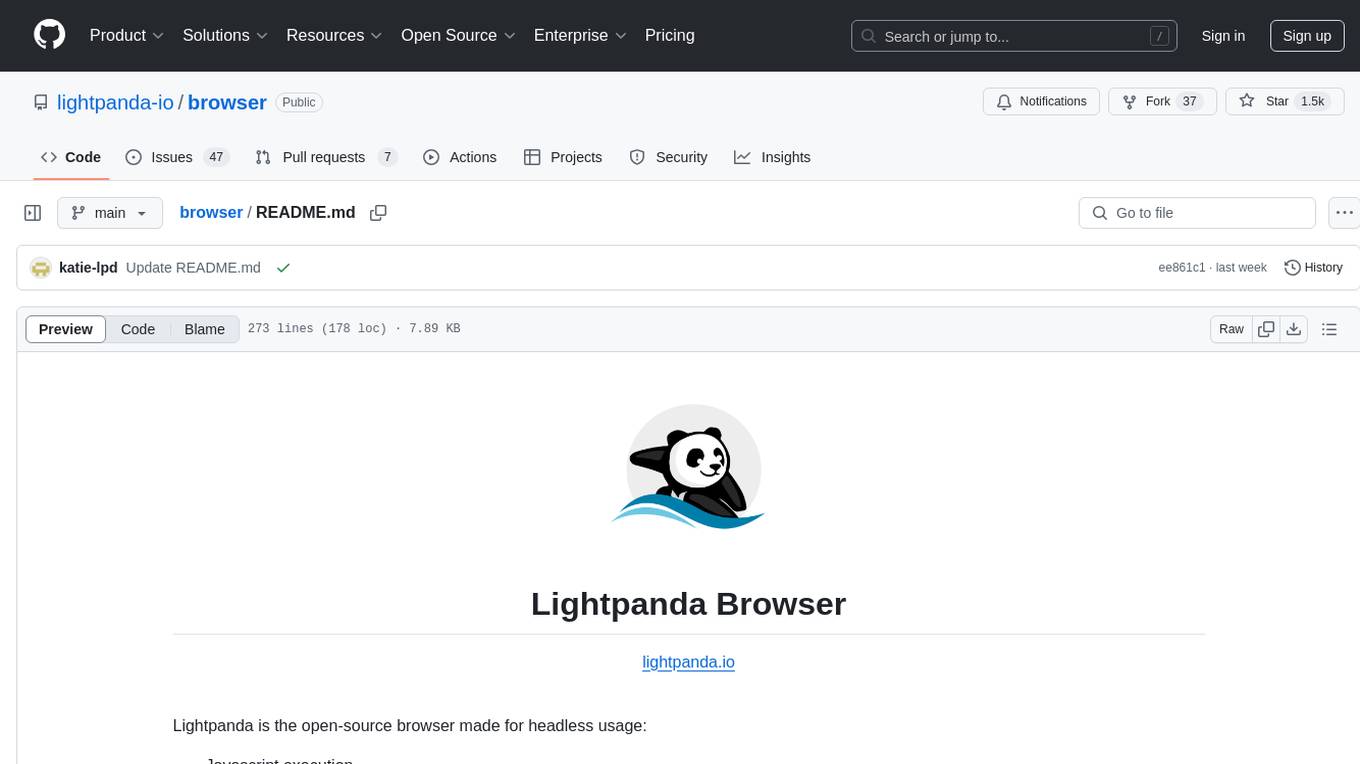
browser
Lightpanda Browser is an open-source headless browser designed for fast web automation, AI agents, LLM training, scraping, and testing. It features ultra-low memory footprint, exceptionally fast execution, and compatibility with Playwright and Puppeteer through CDP. Built for performance, Lightpanda offers Javascript execution, support for Web APIs, and is optimized for minimal memory usage. It is a modern solution for web scraping and automation tasks, providing a lightweight alternative to traditional browsers like Chrome.
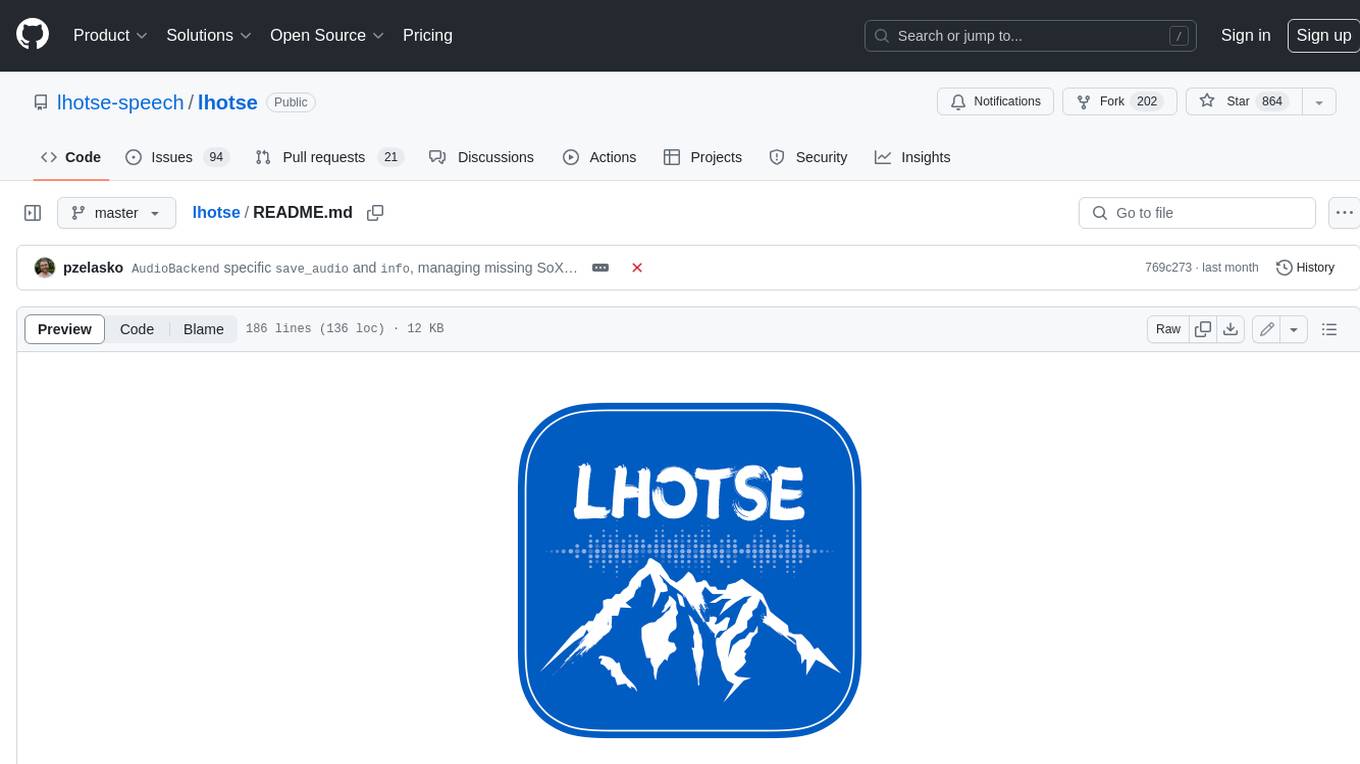
lhotse
Lhotse is a Python library designed to make speech and audio data preparation flexible and accessible. It aims to attract a wider community to speech processing tasks by providing a Python-centric design and an expressive command-line interface. Lhotse offers standard data preparation recipes, PyTorch Dataset classes for speech tasks, and efficient data preparation for model training with audio cuts. It supports data augmentation, feature extraction, and feature-space cut mixing. The tool extends Kaldi's data preparation recipes with seamless PyTorch integration, human-readable text manifests, and convenient Python classes.
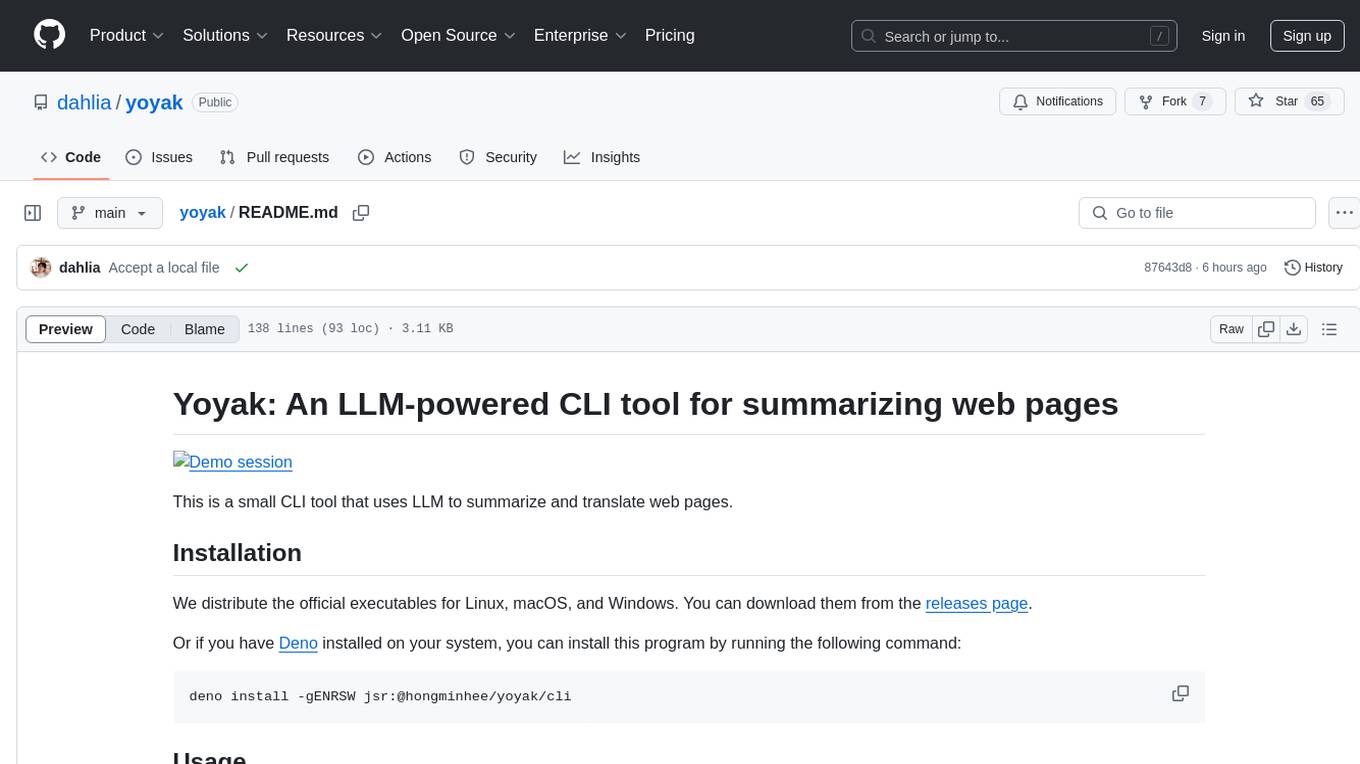
yoyak
Yoyak is a small CLI tool powered by LLM for summarizing and translating web pages. It provides shell completion scripts for bash, fish, and zsh. Users can set the model they want to use and summarize web pages with the 'yoyak summary' command. Additionally, translation to other languages is supported using the '-l' option with ISO 639-1 language codes. Yoyak supports various models for summarization and translation tasks.
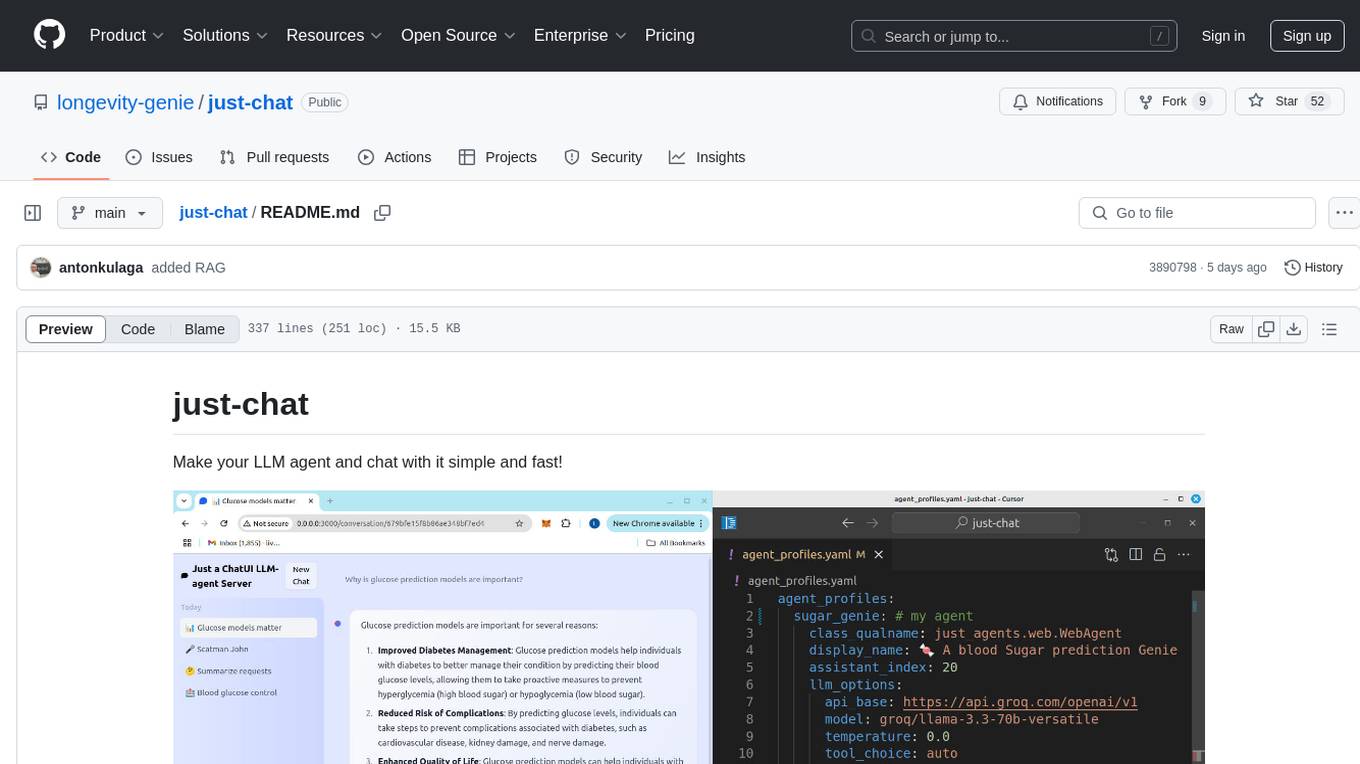
just-chat
Just-Chat is a containerized application that allows users to easily set up and chat with their AI agent. Users can customize their AI assistant using a YAML file, add new capabilities with Python tools, and interact with the agent through a chat web interface. The tool supports various modern models like DeepSeek Reasoner, ChatGPT, LLAMA3.3, etc. Users can also use semantic search capabilities with MeiliSearch to find and reference relevant information based on meaning. Just-Chat requires Docker or Podman for operation and provides detailed installation instructions for both Linux and Windows users.
For similar tasks
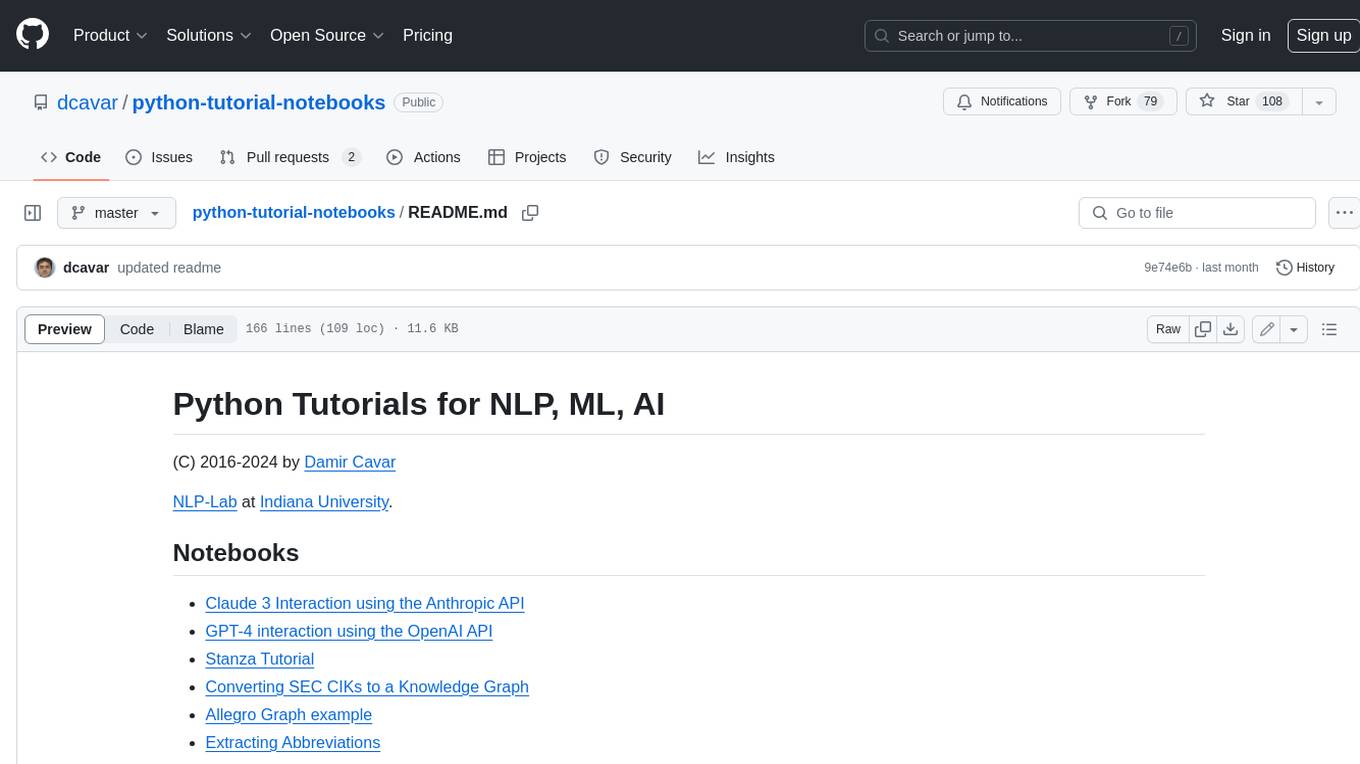
python-tutorial-notebooks
This repository contains Jupyter-based tutorials for NLP, ML, AI in Python for classes in Computational Linguistics, Natural Language Processing (NLP), Machine Learning (ML), and Artificial Intelligence (AI) at Indiana University.
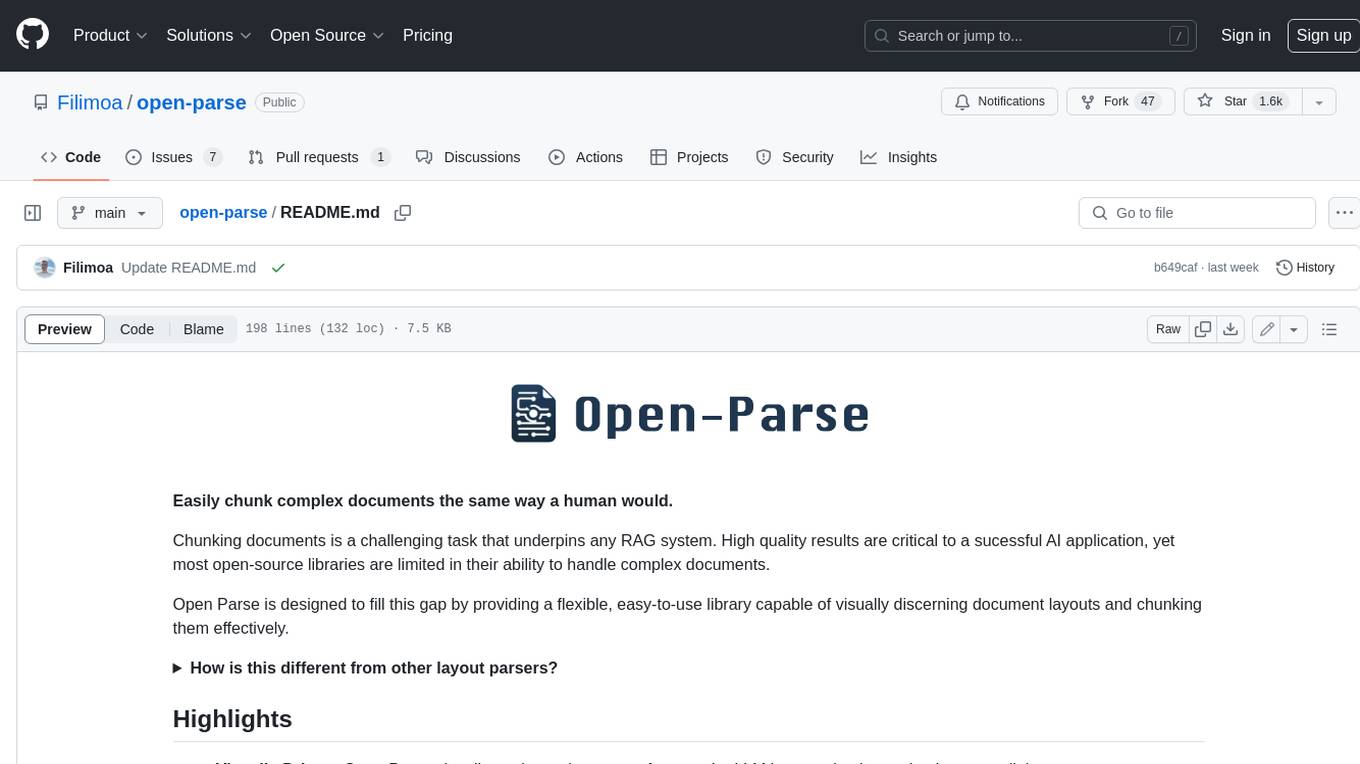
open-parse
Open Parse is a Python library for visually discerning document layouts and chunking them effectively. It is designed to fill the gap in open-source libraries for handling complex documents. Unlike text splitting, which converts a file to raw text and slices it up, Open Parse visually analyzes documents for superior LLM input. It also supports basic markdown for parsing headings, bold, and italics, and has high-precision table support, extracting tables into clean Markdown formats with accuracy that surpasses traditional tools. Open Parse is extensible, allowing users to easily implement their own post-processing steps. It is also intuitive, with great editor support and completion everywhere, making it easy to use and learn.
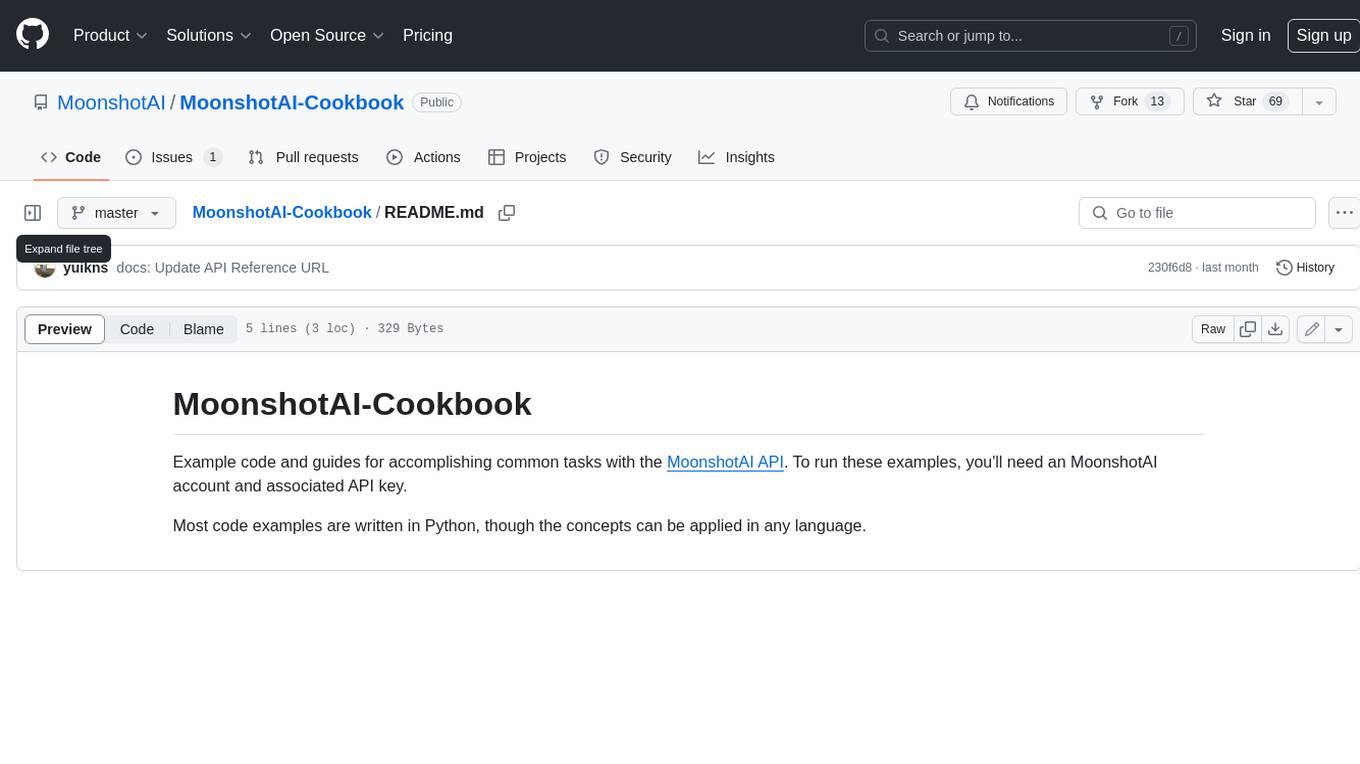
MoonshotAI-Cookbook
The MoonshotAI-Cookbook provides example code and guides for accomplishing common tasks with the MoonshotAI API. To run these examples, you'll need an MoonshotAI account and associated API key. Most code examples are written in Python, though the concepts can be applied in any language.
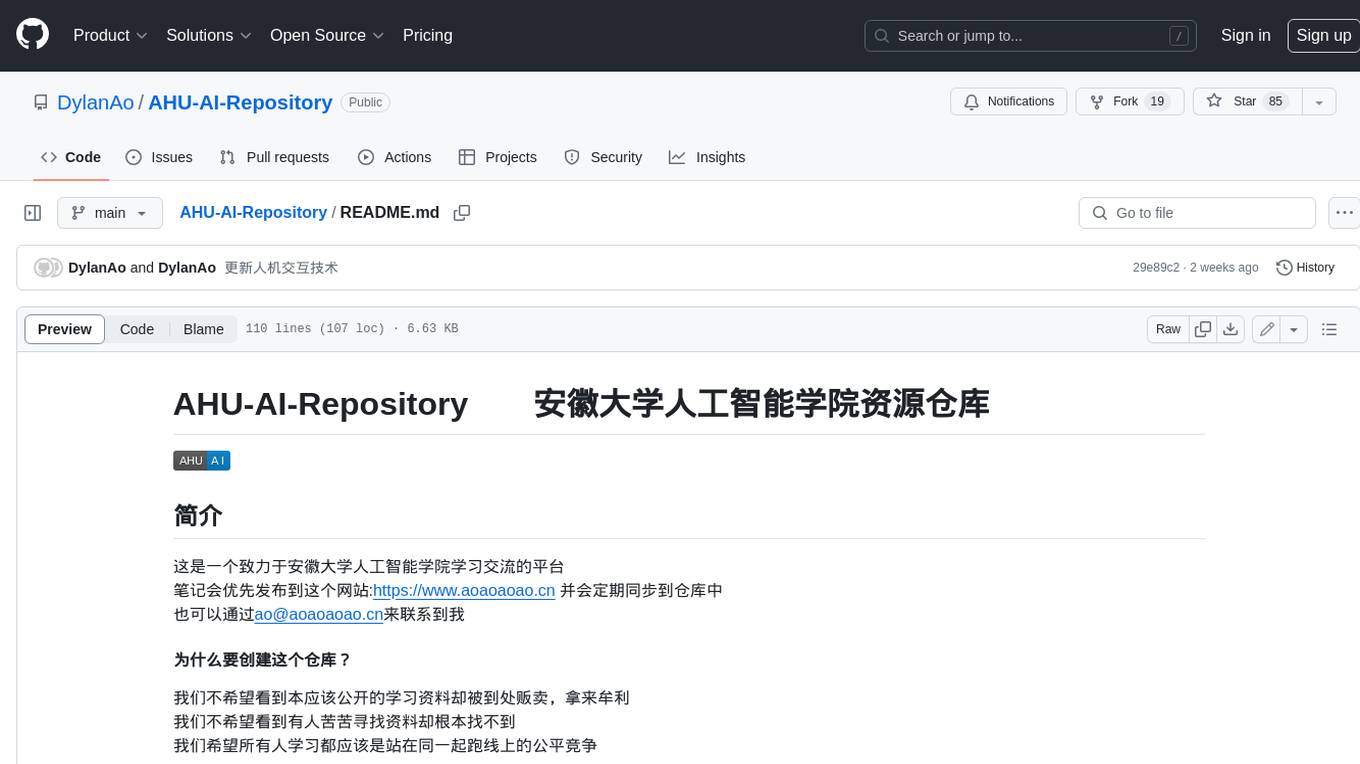
AHU-AI-Repository
This repository is dedicated to the learning and exchange of resources for the School of Artificial Intelligence at Anhui University. Notes will be published on this website first: https://www.aoaoaoao.cn and will be synchronized to the repository regularly. You can also contact me at [email protected].
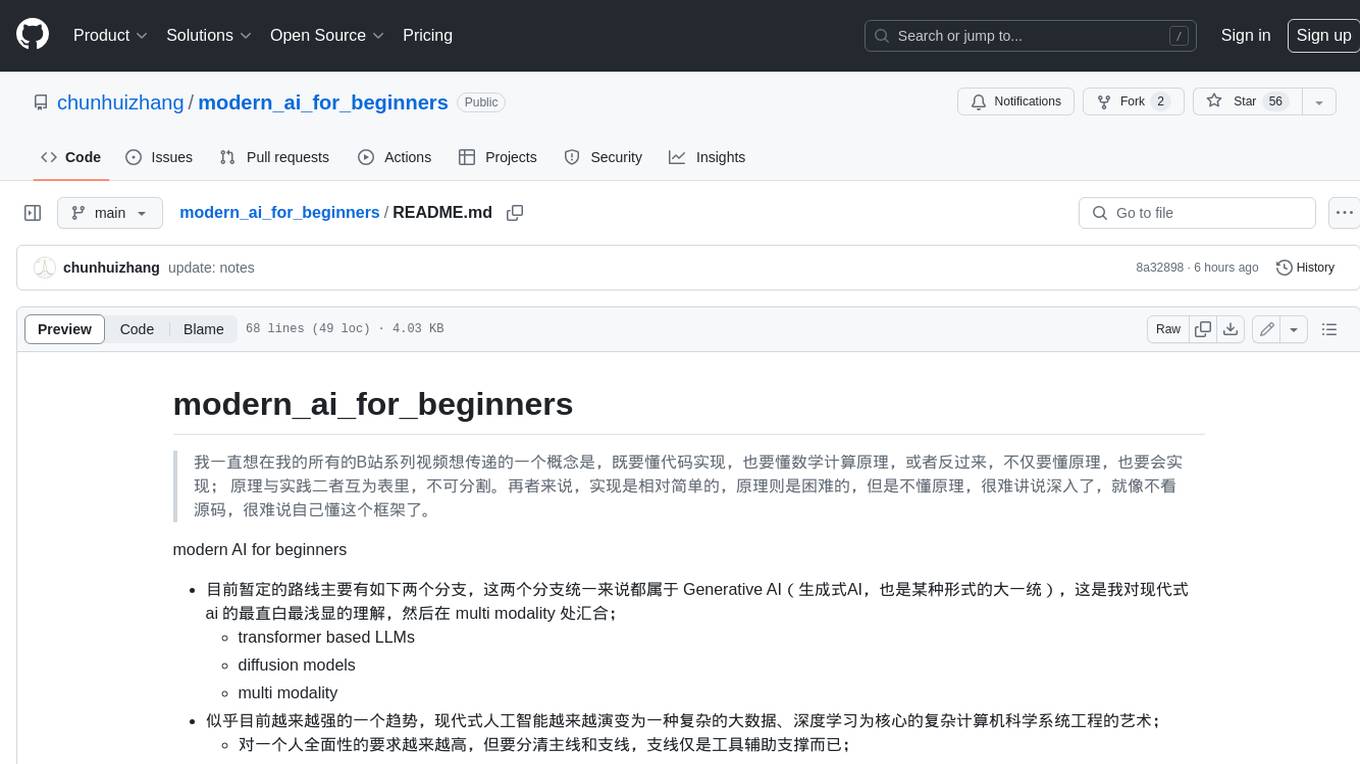
modern_ai_for_beginners
This repository provides a comprehensive guide to modern AI for beginners, covering both theoretical foundations and practical implementation. It emphasizes the importance of understanding both the mathematical principles and the code implementation of AI models. The repository includes resources on PyTorch, deep learning fundamentals, mathematical foundations, transformer-based LLMs, diffusion models, software engineering, and full-stack development. It also features tutorials on natural language processing with transformers, reinforcement learning, and practical deep learning for coders.
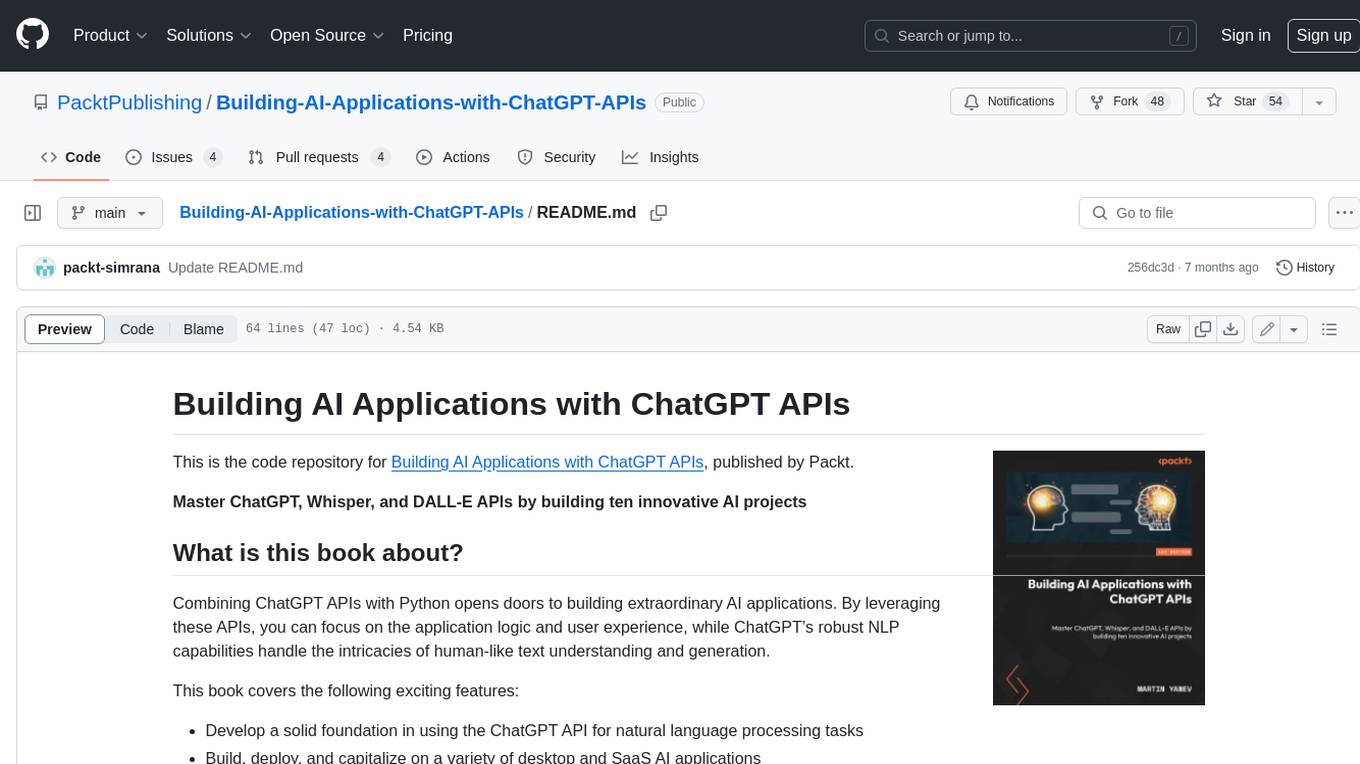
Building-AI-Applications-with-ChatGPT-APIs
This repository is for the book 'Building AI Applications with ChatGPT APIs' published by Packt. It provides code examples and instructions for mastering ChatGPT, Whisper, and DALL-E APIs through building innovative AI projects. Readers will learn to develop AI applications using ChatGPT APIs, integrate them with frameworks like Flask and Django, create AI-generated art with DALL-E APIs, and optimize ChatGPT models through fine-tuning.
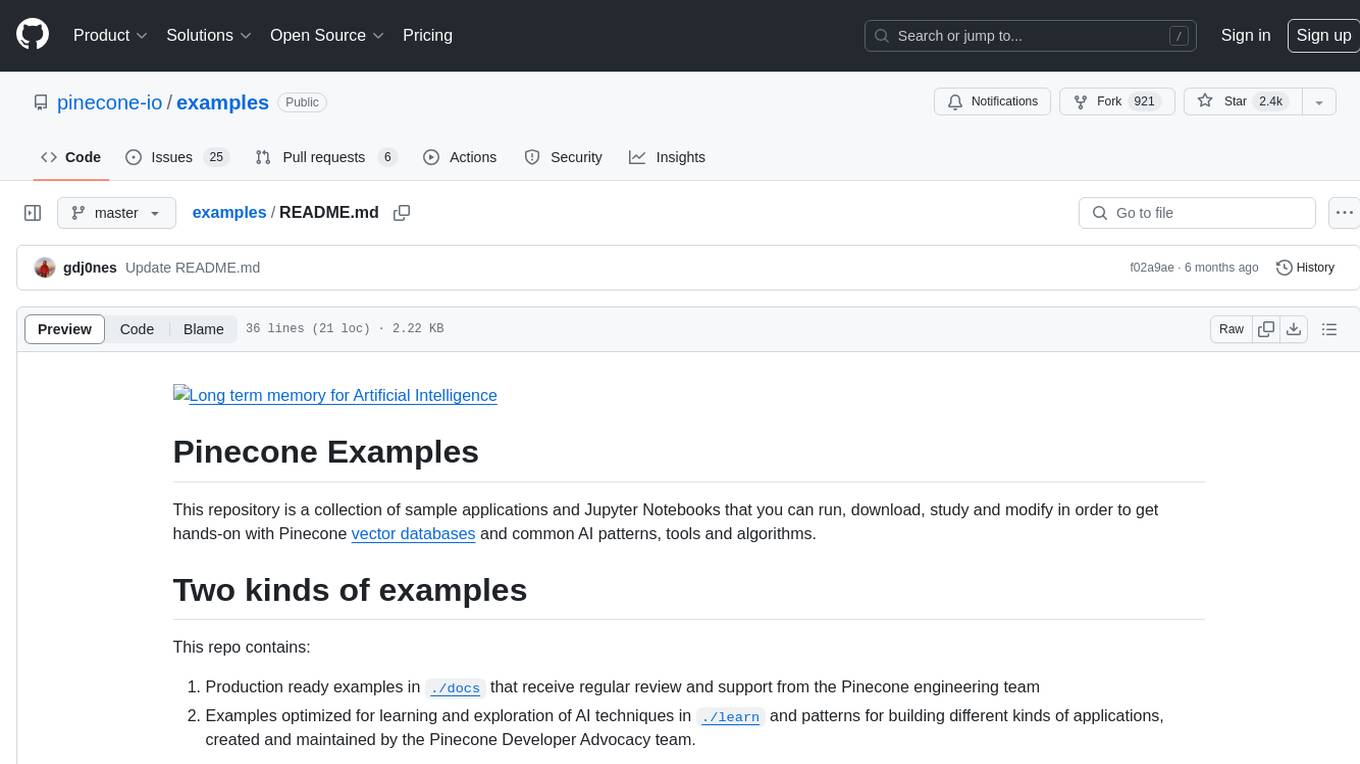
examples
This repository contains a collection of sample applications and Jupyter Notebooks for hands-on experience with Pinecone vector databases and common AI patterns, tools, and algorithms. It includes production-ready examples for review and support, as well as learning-optimized examples for exploring AI techniques and building applications. Users can contribute, provide feedback, and collaborate to improve the resource.
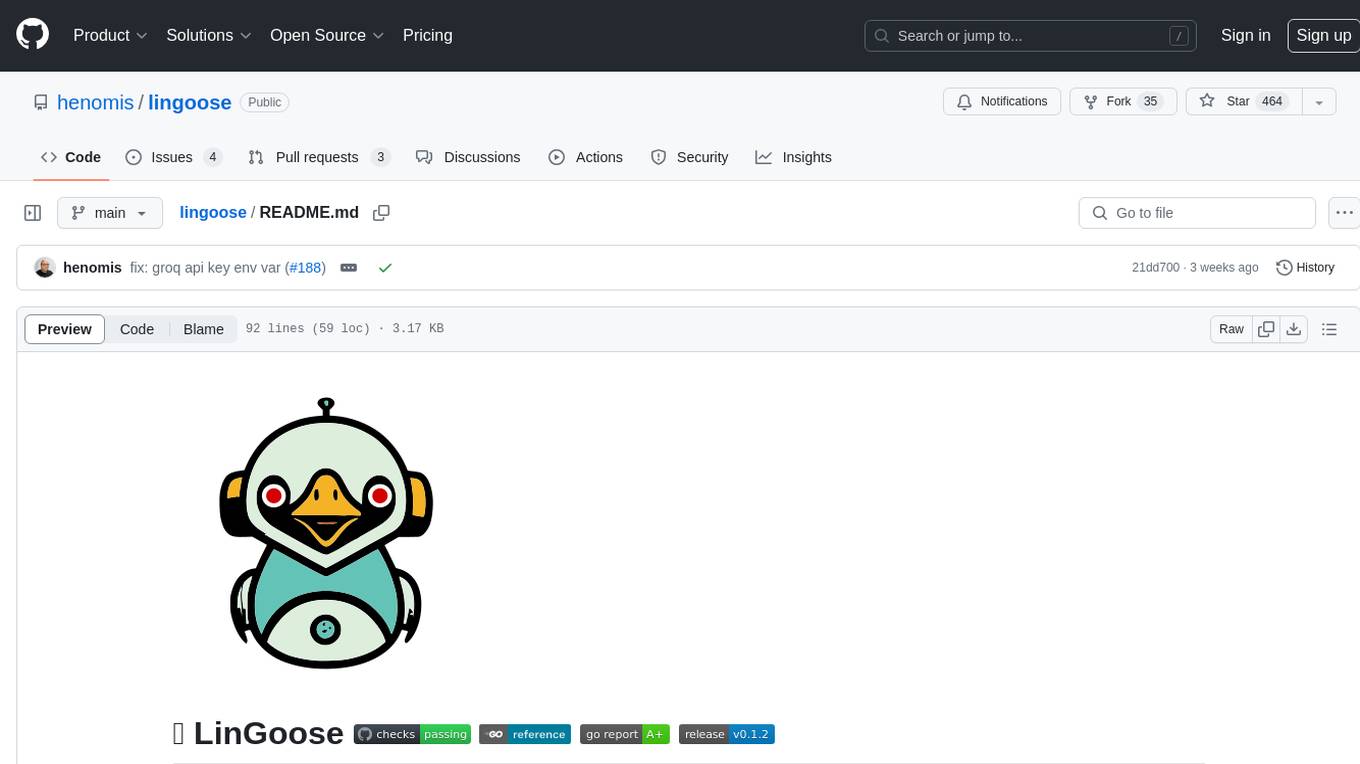
lingoose
LinGoose is a modular Go framework designed for building AI/LLM applications. It offers the flexibility to import only the necessary modules, abstracts features for customization, and provides a comprehensive solution for developing AI/LLM applications from scratch. The framework simplifies the process of creating intelligent applications by allowing users to choose preferred implementations or create their own. LinGoose empowers developers to leverage its capabilities to streamline the development of cutting-edge AI and LLM projects.
For similar jobs
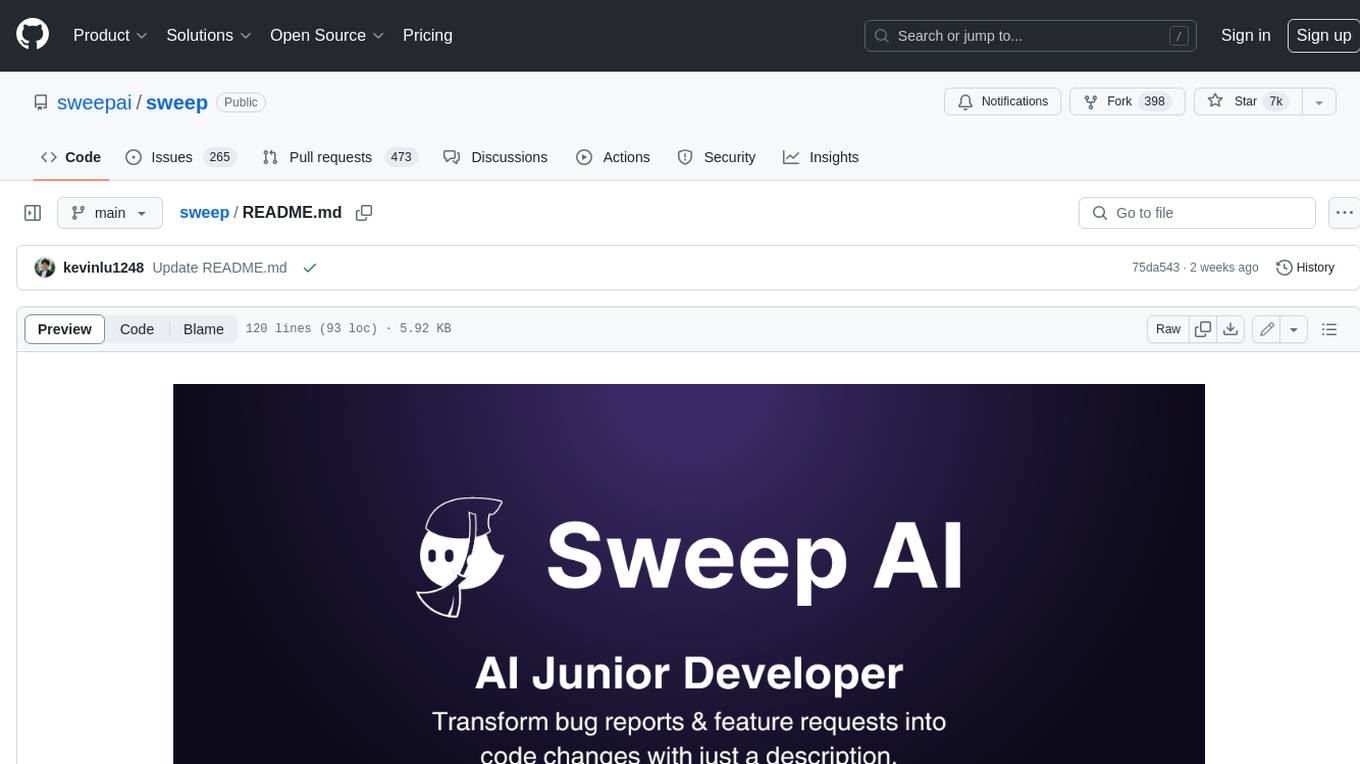
sweep
Sweep is an AI junior developer that turns bugs and feature requests into code changes. It automatically handles developer experience improvements like adding type hints and improving test coverage.
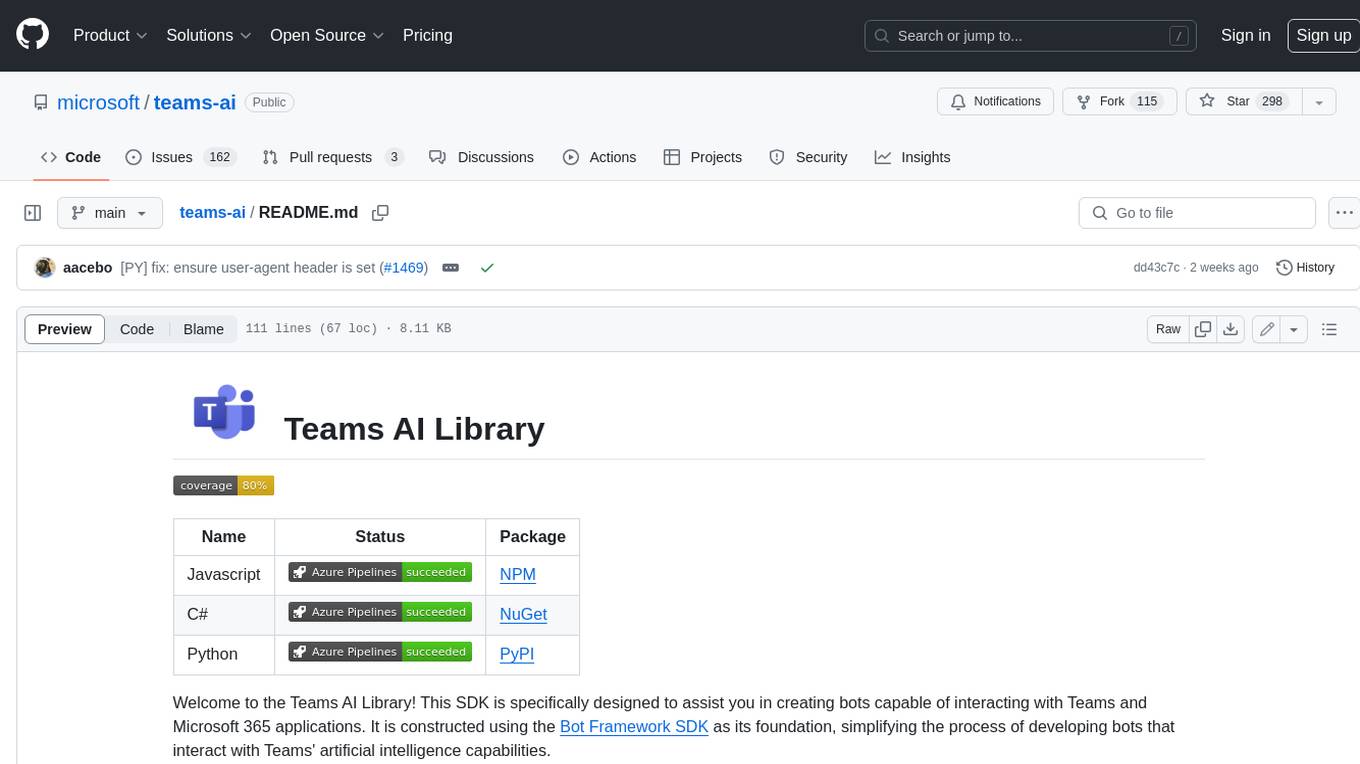
teams-ai
The Teams AI Library is a software development kit (SDK) that helps developers create bots that can interact with Teams and Microsoft 365 applications. It is built on top of the Bot Framework SDK and simplifies the process of developing bots that interact with Teams' artificial intelligence capabilities. The SDK is available for JavaScript/TypeScript, .NET, and Python.
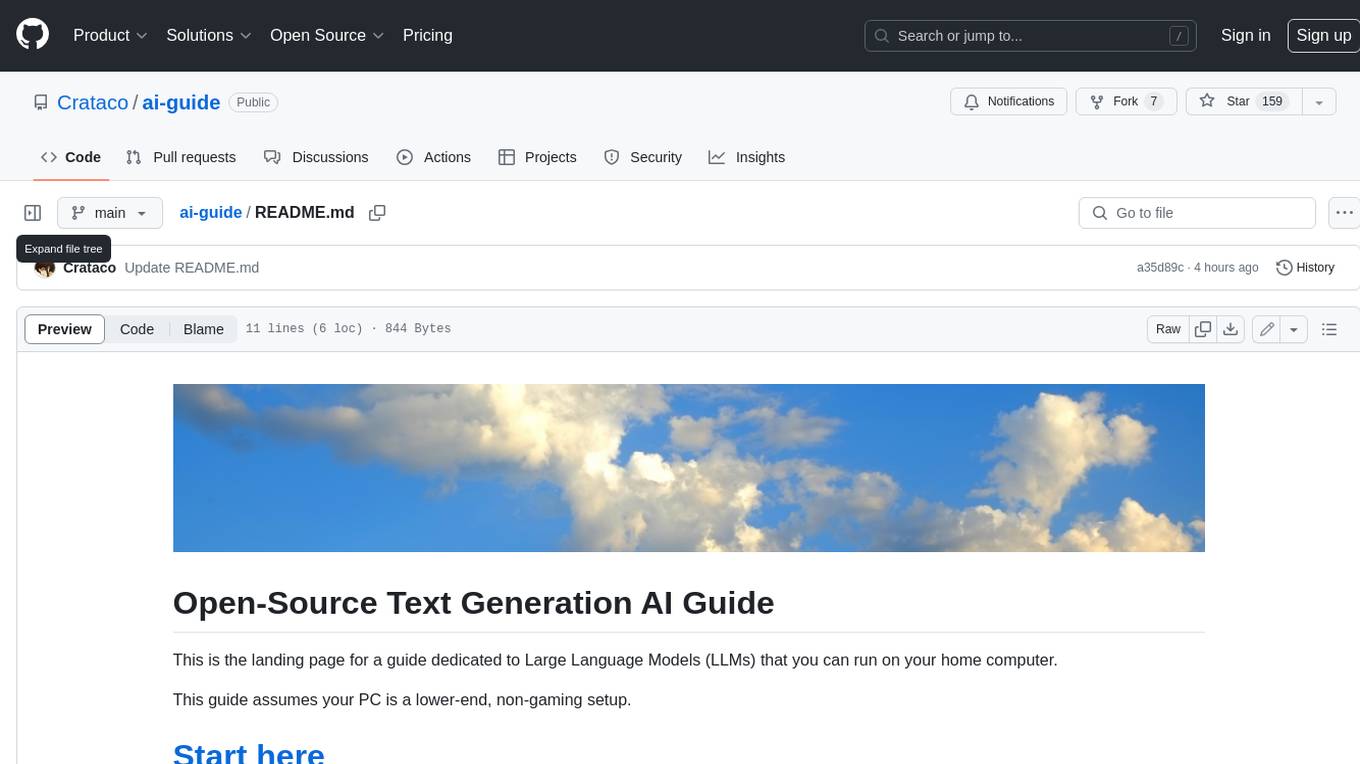
ai-guide
This guide is dedicated to Large Language Models (LLMs) that you can run on your home computer. It assumes your PC is a lower-end, non-gaming setup.
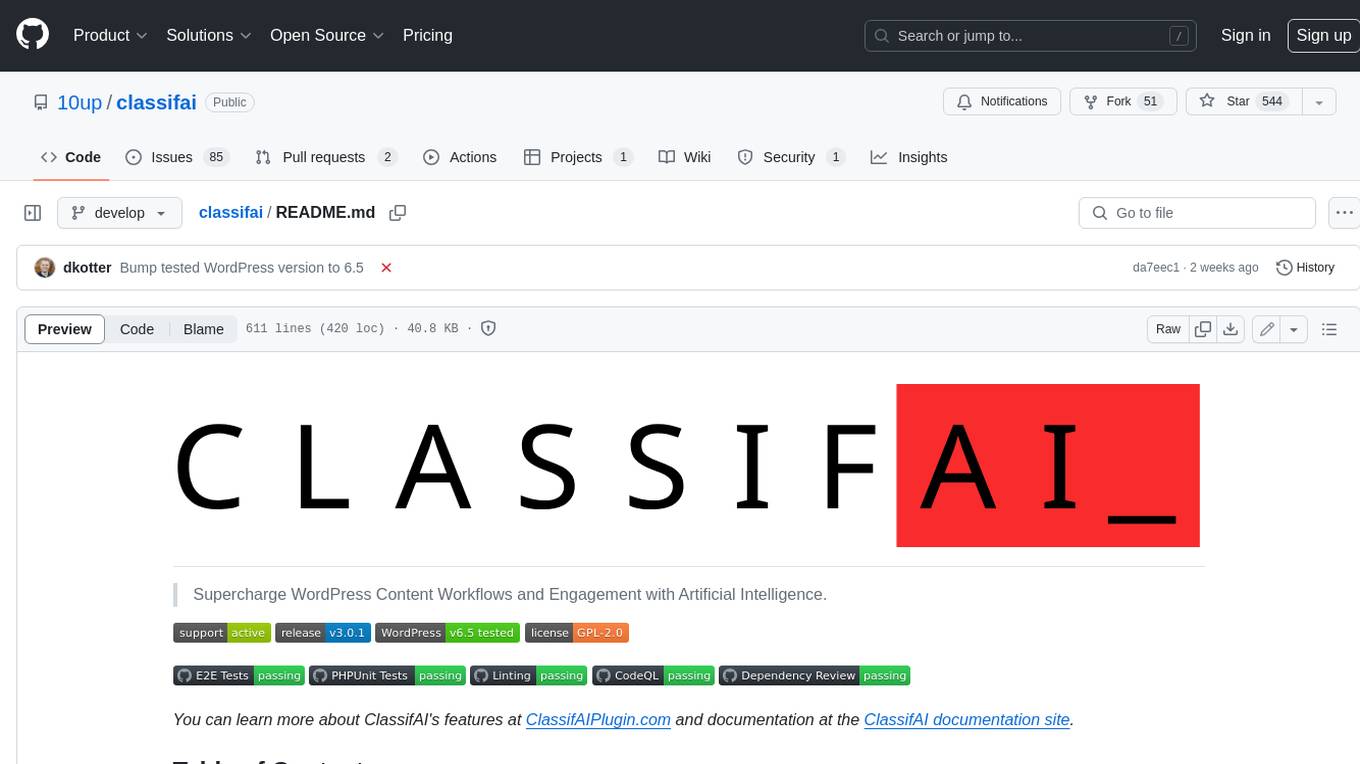
classifai
Supercharge WordPress Content Workflows and Engagement with Artificial Intelligence. Tap into leading cloud-based services like OpenAI, Microsoft Azure AI, Google Gemini and IBM Watson to augment your WordPress-powered websites. Publish content faster while improving SEO performance and increasing audience engagement. ClassifAI integrates Artificial Intelligence and Machine Learning technologies to lighten your workload and eliminate tedious tasks, giving you more time to create original content that matters.
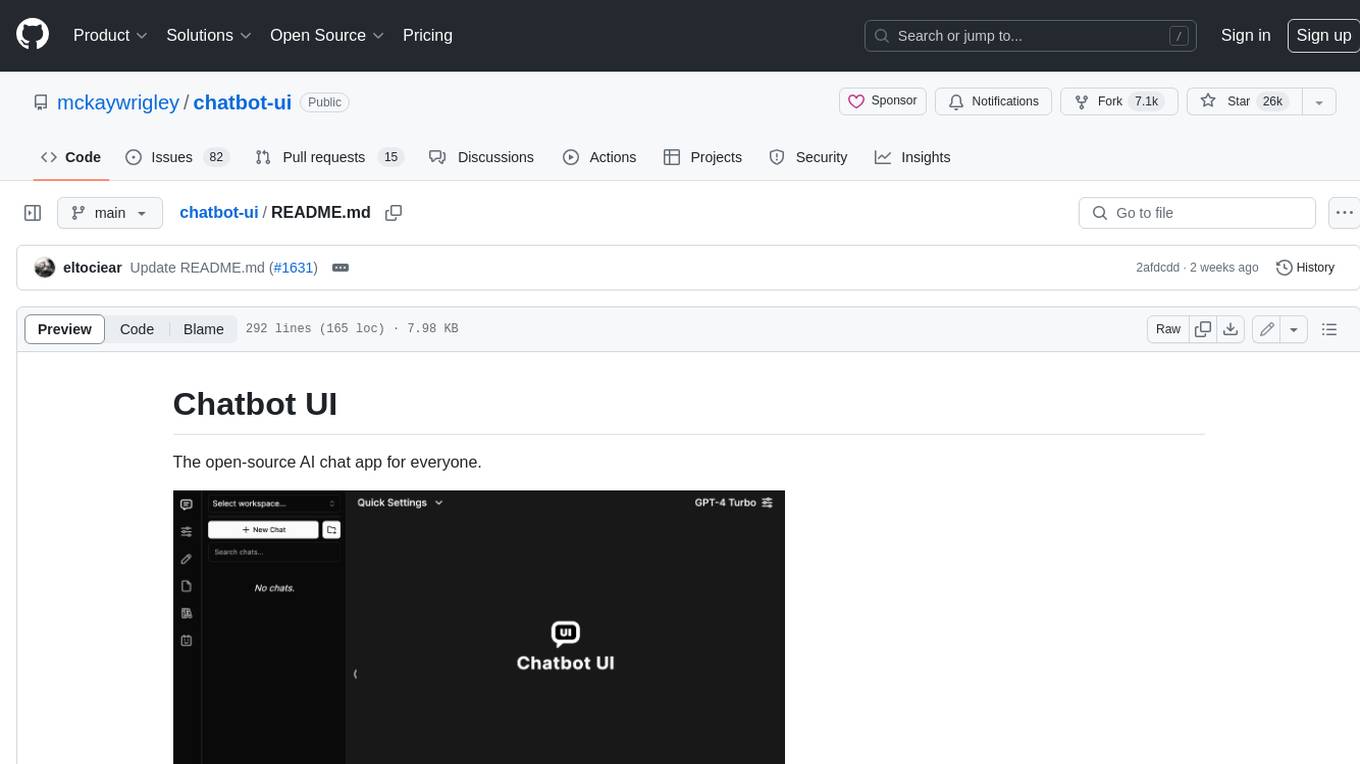
chatbot-ui
Chatbot UI is an open-source AI chat app that allows users to create and deploy their own AI chatbots. It is easy to use and can be customized to fit any need. Chatbot UI is perfect for businesses, developers, and anyone who wants to create a chatbot.
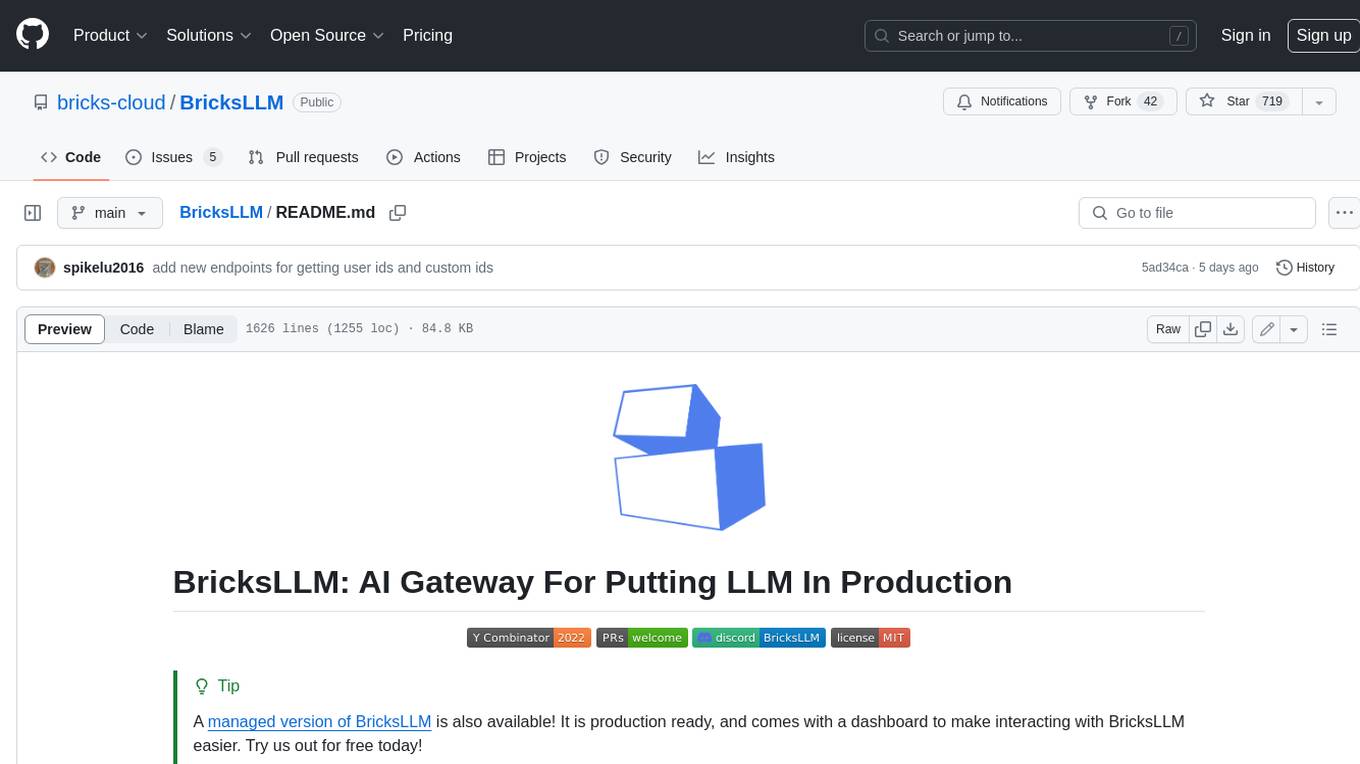
BricksLLM
BricksLLM is a cloud native AI gateway written in Go. Currently, it provides native support for OpenAI, Anthropic, Azure OpenAI and vLLM. BricksLLM aims to provide enterprise level infrastructure that can power any LLM production use cases. Here are some use cases for BricksLLM: * Set LLM usage limits for users on different pricing tiers * Track LLM usage on a per user and per organization basis * Block or redact requests containing PIIs * Improve LLM reliability with failovers, retries and caching * Distribute API keys with rate limits and cost limits for internal development/production use cases * Distribute API keys with rate limits and cost limits for students
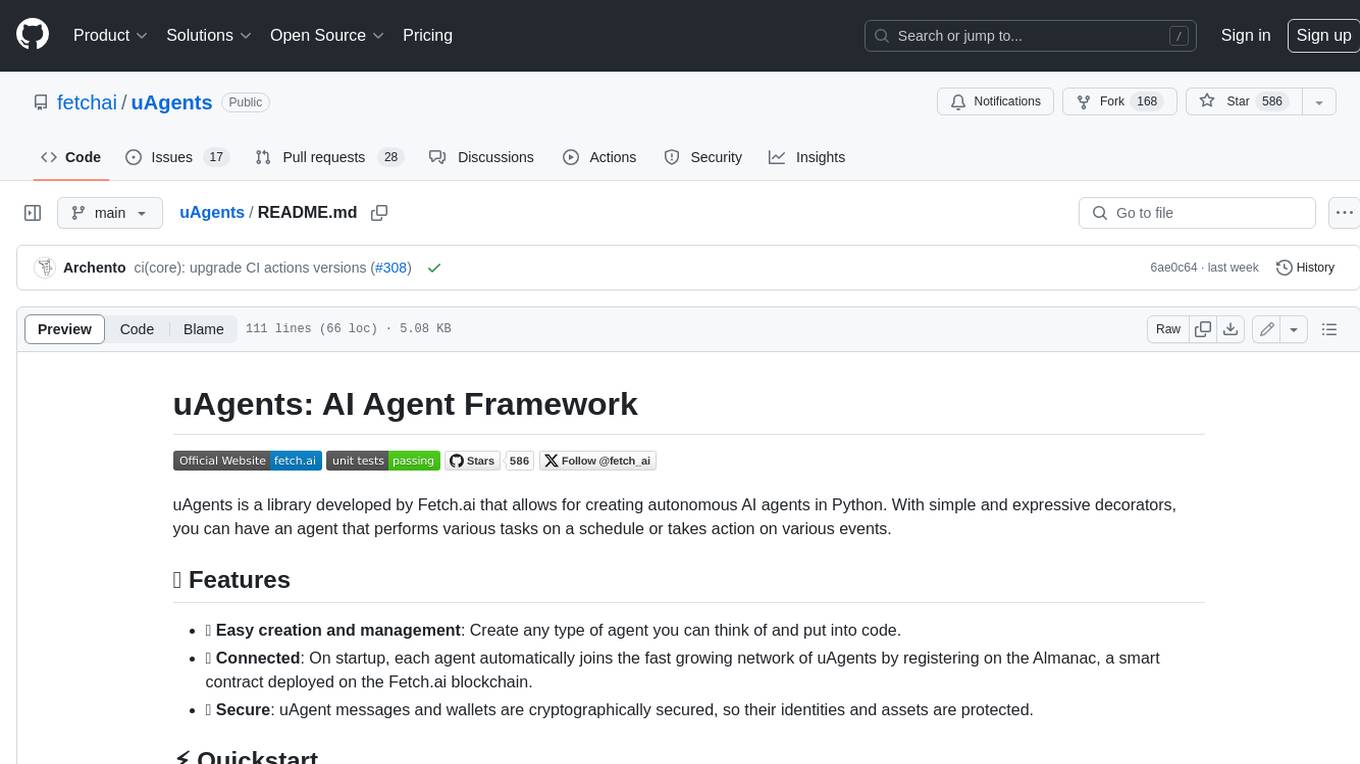
uAgents
uAgents is a Python library developed by Fetch.ai that allows for the creation of autonomous AI agents. These agents can perform various tasks on a schedule or take action on various events. uAgents are easy to create and manage, and they are connected to a fast-growing network of other uAgents. They are also secure, with cryptographically secured messages and wallets.
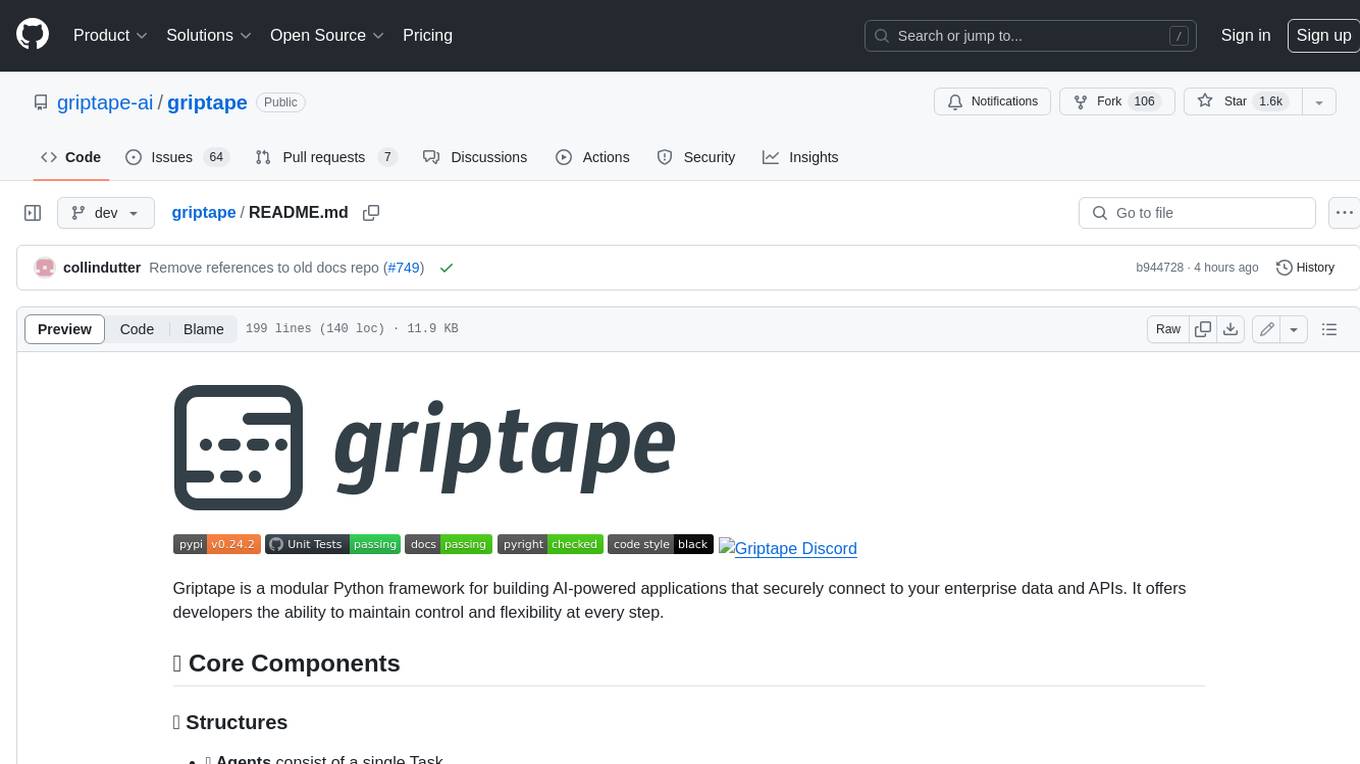
griptape
Griptape is a modular Python framework for building AI-powered applications that securely connect to your enterprise data and APIs. It offers developers the ability to maintain control and flexibility at every step. Griptape's core components include Structures (Agents, Pipelines, and Workflows), Tasks, Tools, Memory (Conversation Memory, Task Memory, and Meta Memory), Drivers (Prompt and Embedding Drivers, Vector Store Drivers, Image Generation Drivers, Image Query Drivers, SQL Drivers, Web Scraper Drivers, and Conversation Memory Drivers), Engines (Query Engines, Extraction Engines, Summary Engines, Image Generation Engines, and Image Query Engines), and additional components (Rulesets, Loaders, Artifacts, Chunkers, and Tokenizers). Griptape enables developers to create AI-powered applications with ease and efficiency.