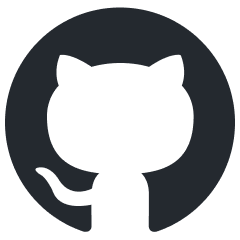
monacopilot
⚡️AI auto-completion plugin for Monaco Editor, inspired by GitHub Copilot.
Stars: 82
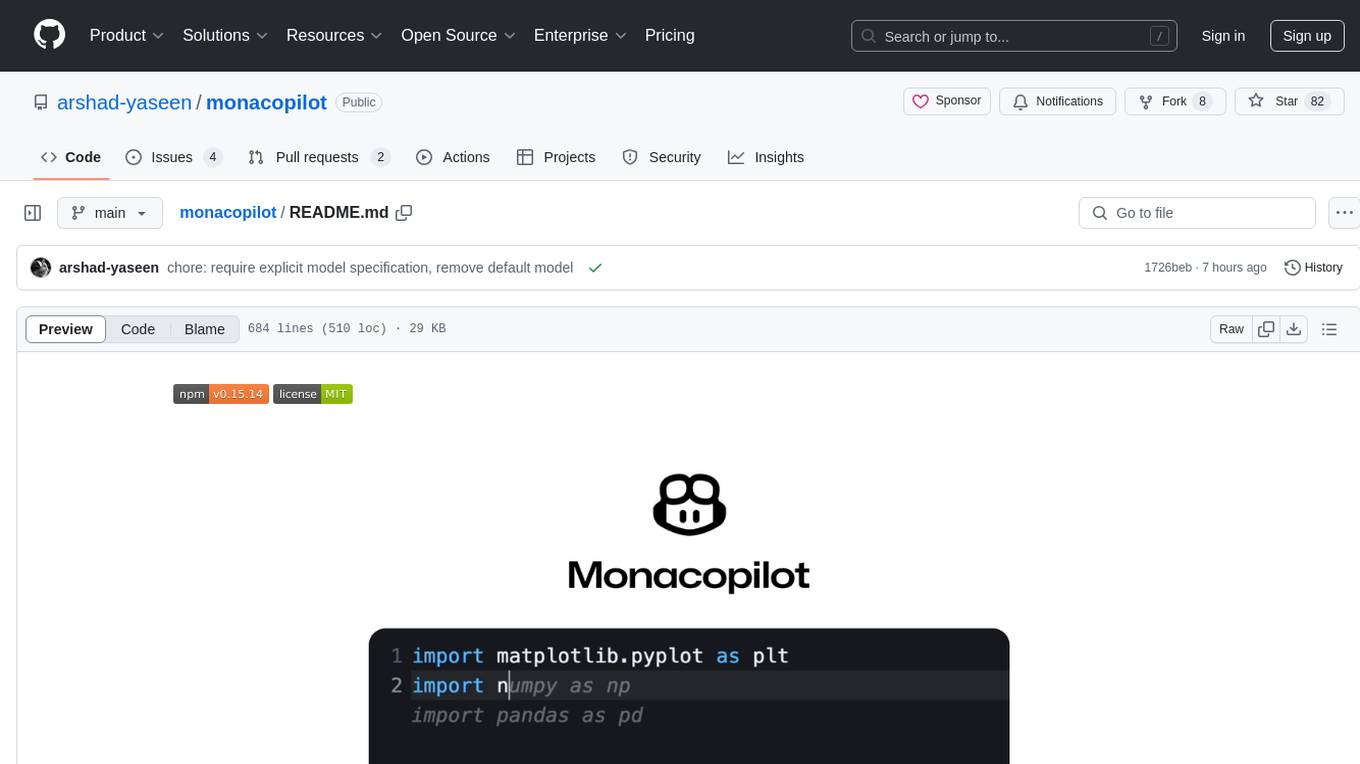
Monacopilot is a powerful and customizable AI auto-completion plugin for the Monaco Editor. It supports multiple AI providers such as Anthropic, OpenAI, Groq, and Google, providing real-time code completions with an efficient caching system. The plugin offers context-aware suggestions, customizable completion behavior, and framework agnostic features. Users can also customize the model support and trigger completions manually. Monacopilot is designed to enhance coding productivity by providing accurate and contextually appropriate completions in daily spoken language.
README:
Monacopilot is a powerful and customizable AI auto-completion plugin for the Monaco Editor. Inspired by GitHub Copilot.
- 🎯 Multiple AI Provider Support (Anthropic, OpenAI, Groq, Google)
- 🔄 Real-time Code Completions
- ⚡️ Efficient Caching System
- 🎨 Context-Aware Suggestions
- 🛠️ Customizable Completion Behavior
- 📦 Framework Agnostic
- 🔌 Custom Model Support
- 🎮 Manual Trigger Support
- Examples
- Demo
- Installation
- Usage
- Register Completion Options
- Copilot Options
- Completion Request Options
- Cross-Language API Handler Implementation
- Contributing
Here are some examples of how to integrate Monacopilot into your project:
In the demo, we are using the onTyping
trigger mode with the Groq model, which is why you see such quick and fast completions. Groq provides very fast response times.
To install Monacopilot, run:
npm install monacopilot
Set up an API handler to manage auto-completion requests. An example using Express.js:
import express from 'express';
import {Copilot} from 'monacopilot';
const app = express();
const port = process.env.PORT || 3000;
const copilot = new Copilot(process.env.GROQ_API_KEY, {
provider: 'groq',
model: 'llama-3-70b',
});
app.use(express.json());
app.post('/complete', async (req, res) => {
const {completion, error, raw} = await copilot.complete({
body: req.body,
});
// Process raw LLM response if needed
// `raw` can be undefined if an error occurred, which happens when `error` is present
if (raw) {
calculateCost(raw.usage.input_tokens);
}
// Handle errors if present
if (error) {
console.error('Completion error:', error);
res.status(500).json({completion: null, error});
}
res.status(200).json({completion});
});
app.listen(port);
The handler should return a JSON response with the following structure:
{
"completion": "Generated completion text"
}
Or in case of an error:
{
"completion": null,
"error": "Error message"
}
If you prefer to use a different programming language for your API handler in cases where your backend is not in JavaScript, please refer to the section Cross-Language API Handler Implementation for guidance on implementing the handler in your chosen language.
Now, Monacopilot is set up to send completion requests to the /complete
endpoint and receive completions in response.
The copilot.complete
method processes the request body sent by Monacopilot and returns the corresponding completion.
Now, let's integrate AI auto-completion into your Monaco editor. Here's how you can do it:
import * as monaco from 'monaco-editor';
import {registerCompletion} from 'monacopilot';
const editor = monaco.editor.create(document.getElementById('container'), {
language: 'javascript',
});
registerCompletion(monaco, editor, {
// Examples:
// - '/api/complete' if you're using the Next.js (API handler) or similar frameworks.
// - 'https://api.example.com/complete' for a separate API server
// Ensure this can be accessed from the browser.
endpoint: 'https://api.example.com/complete',
// The language of the editor.
language: 'javascript',
});
Note: The
registerCompletion
function returns acompletion
object with aderegister
method. This method should be used to clean up the completion functionality when it's no longer needed. For example, in a React component, you can callcompletion.deregister()
within theuseEffect
cleanup function to ensure proper disposal when the component unmounts.
🎉 Congratulations! The AI auto-completion is now connected to the Monaco Editor. Start typing and see completions in the editor.
The trigger
option determines when the completion service provides code completions. You can choose between receiving suggestions/completions in real-time as you type or after a brief pause.
registerCompletion(monaco, editor, {
trigger: 'onTyping',
});
Trigger | Description | Notes |
---|---|---|
'onIdle' (default) |
Provides completions after a brief pause in typing. | This approach is less resource-intensive, as it only initiates a request when the editor is idle. |
'onTyping' |
Provides completions in real-time as you type. | Best suited for models with low response latency, such as Groq models or Claude 3-5 Haiku. This trigger mode initiates additional background requests to deliver real-time suggestions, a method known as predictive caching. |
'onDemand' |
Does not provide completions automatically. | Completions are triggered manually using the trigger function from the registerCompletion return. This allows for precise control over when completions are provided. |
Note: If you prefer real-time completions, you can set the
trigger
option to'onTyping'
. This may increase the number of requests made to the provider and the cost. This should not be too costly since most small models are very inexpensive.
If you prefer not to trigger completions automatically (e.g., on typing or on idle), you can trigger completions manually. This is useful in scenarios where you want to control when completions are provided, such as through a button click or a keyboard shortcut.
const completion = registerCompletion(monaco, editor, {
trigger: 'onDemand',
});
completion.trigger();
To set up manual triggering, configure the trigger
option to 'onDemand'
. This disables automatic completions, allowing you to call the completion.trigger()
method explicitly when needed.
You can set up completions to trigger when the Ctrl+Shift+Space
keyboard shortcut is pressed.
const completion = registerCompletion(monaco, editor, {
trigger: 'onDemand',
});
editor.addCommand(
monaco.KeyMod.CtrlCmd | monaco.KeyMod.Shift | monaco.KeyCode.Space,
() => {
completion.trigger();
},
);
You can add a custom editor action to trigger completions manually.
const completion = registerCompletion(monaco, editor, {
trigger: 'onDemand',
});
monaco.editor.addEditorAction({
id: 'monacopilot.triggerCompletion',
label: 'Complete Code',
contextMenuGroupId: 'navigation',
keybindings: [
monaco.KeyMod.CtrlCmd | monaco.KeyMod.Shift | monaco.KeyCode.Space,
],
run: () => {
completion.trigger();
},
});
Improve the quality and relevance of Copilot's suggestions by providing additional code context from other files in your project. This feature allows Copilot to understand the broader scope of your codebase, resulting in more accurate and contextually appropriate completions.
registerCompletion(monaco, editor, {
relatedFiles: [
{
path: './utils.js',
content:
'export const reverse = (str) => str.split("").reverse().join("")',
},
],
});
For instance, if you begin typing const isPalindrome =
in your current file, Copilot will recognize the reverse
function from the utils.js
file you provided earlier. It will then suggest a completion that utilizes this function.
Specify the name of the file being edited to receive more contextually relevant completions.
registerCompletion(monaco, editor, {
filename: 'utils.js', // e.g., "index.js", "utils/objects.js"
});
Now, the completions will be more relevant to the file's context.
Enable completions tailored to specific technologies by using the technologies
option.
registerCompletion(monaco, editor, {
technologies: ['react', 'next.js', 'tailwindcss'],
});
This configuration will provide completions relevant to React, Next.js, and Tailwind CSS.
To manage potentially lengthy code in your editor, you can limit the number of lines included in the completion request using the maxContextLines
option.
For example, if there's a chance that the code in your editor may exceed 500+ lines
, you don't need to provide 500 lines
to the model. This would increase costs due to the huge number of input tokens. Instead, you can set maxContextLines
to maybe 80
or 100
, depending on how accurate you want the completions to be and how much you're willing to pay for the model.
registerCompletion(monaco, editor, {
maxContextLines: 80,
});
Note: If you're using
Groq
as your provider, it's recommended to setmaxContextLines
to60
or less due to its low rate limits and lack of pay-as-you-go pricing. However,Groq
is expected to offer pay-as-you-go pricing in the near future.
Monacopilot caches completions by default. It uses a FIFO (First In First Out) strategy, reusing cached completions when the context and cursor position match while editing (default: true
). To disable caching:
registerCompletion(monaco, editor, {
enableCaching: false,
});
When an error occurs during the completion process or requests, Monacopilot will log it to the console by default rather than throwing errors. This ensures smooth editing even when completions are unavailable.
You can provide this callback to handle errors yourself, which will disable the default console logging.
registerCompletion(monaco, editor, {
onError: error => {
console.error(error);
},
});
The requestHandler
option in the registerCompletion
function allows you to handle requests sent to the specified endpoint, offering high customization for both requests and responses. By leveraging this functionality, you can manipulate and customize the request or response to meet your specific requirements.
registerCompletion(monaco, editor, {
endpoint: 'https://api.example.com/complete',
// ... other options
requestHandler: async ({endpoint, body}) => {
const response = await fetch(endpoint, {
method: 'POST',
headers: {
'Content-Type': 'application/json',
},
body: JSON.stringify(body),
});
const data = await response.json();
return {
completion: data.completion,
};
},
});
The requestHandler
function takes an object with endpoint
and body
as parameters.
Property | Type | Description |
---|---|---|
endpoint |
string |
The endpoint to which the request is sent. This is the same as the endpoint in registerCompletion . |
body |
object |
The body of the request processed by Monacopilot. |
Note: The
body
object contains properties generated by Monacopilot. If you need to include additional properties in the request body, you can create a new object that combines the existingbody
with your custom properties. For example:const customBody = { ...body, myCustomProperty: 'value', };
The requestHandler
should return an object with the following property:
Property | Type | Description |
---|---|---|
completion |
string or null
|
The completion text to be inserted into the editor. Return null if no completion is available. |
The example below demonstrates how to use the requestHandler
function for more customized handling:
registerCompletion(monaco, editor, {
endpoint: 'https://api.example.com/complete',
// ... other options
requestHandler: async ({endpoint, body}) => {
try {
const response = await fetch(endpoint, {
method: 'POST',
headers: {
'Content-Type': 'application/json',
'X-Request-ID': generateUniqueId(),
},
body: JSON.stringify({
...body,
additionalProperty: 'value',
}),
});
if (!response.ok) {
throw new Error(`HTTP error! status: ${response.status}`);
}
const data = await response.json();
if (data.error) {
console.error('API Error:', data.error);
return {completion: null};
}
return {completion: data.completion.trim()};
} catch (error) {
console.error('Fetch error:', error);
return {completion: null};
}
},
});
You can specify a different provider and model by setting the provider
and model
parameters in the Copilot
instance.
const copilot = new Copilot(process.env.OPENAI_API_KEY, {
provider: 'openai',
model: 'gpt-4o',
});
There are other providers and models available. Here is a list:
Provider | Models |
---|---|
Groq | llama-3-70b |
OpenAI |
gpt-4o , gpt-4o-mini , o1-mini (beta model)
|
Anthropic |
claude-3-5-sonnet , claude-3-haiku , claude-3-5-haiku
|
gemini-1.5-pro , gemini-1.5-flash , gemini-1.5-flash-8b
|
You can use a custom LLM that isn't built into Monacopilot by setting up a model
when you create a new Copilot. This feature lets you connect to LLMs from other services or your own custom-built models.
Please ensure you are using a high-quality model, especially for coding tasks, to get the best and most accurate completions. Also, use a model with very low response latency (preferably under 1.5 seconds) to enjoy a great experience and utilize the full power of Monacopilot.
const copilot = new Copilot(process.env.HUGGINGFACE_API_KEY, {
// You don't need to set the provider if you are using a custom model.
// provider: 'huggingface',
model: {
config: (apiKey, prompt) => ({
endpoint:
'https://api-inference.huggingface.co/models/openai-community/gpt2',
headers: {
Authorization: `Bearer ${apiKey}`,
'Content-Type': 'application/json',
},
body: {
inputs: prompt.user,
parameters: {
max_length: 100,
num_return_sequences: 1,
temperature: 0.7,
},
},
}),
transformResponse: response => ({text: response[0].generated_text}),
},
});
The model
option accepts an object with two functions:
Function | Description | Type |
---|---|---|
config |
A function that receives the API key and prompt data, and returns the configuration for the custom model API request. | (apiKey: string, prompt: { system: string; user: string }) => { endpoint: string; body?: object; headers?: object } |
transformResponse |
A function that takes the raw/parsed response from the custom model API and returns an object with the text property. |
(response: unknown) => { text: string | null; } |
The config
function must return an object with the following properties:
Property | Type | Description |
---|---|---|
endpoint |
string |
The URL of the custom model API endpoint. |
body |
object or undefined
|
The body of the custom model API request. |
headers |
object or undefined
|
The headers of the custom model API request. |
The transformResponse
function must return an object with the text
property. This text
property should contain the text generated by the custom model. If no valid text can be extracted, the function should return null
for the text
property.
You can add custom headers to the provider's completion requests. For example, if you select OpenAI
as your provider, you can add a custom header to the OpenAI completion requests made by Monacopilot.
copilot.complete({
options: {
headers: {
'X-Custom-Header': 'custom-value',
},
},
});
You can customize the prompt used for generating completions by providing a customPrompt
function in the options parameter of the copilot.complete
method. This allows you to tailor the AI's behavior to your specific needs.
copilot.complete({
options: {
customPrompt: metadata => ({
system: 'Your custom system prompt here',
user: 'Your custom user prompt here',
}),
},
});
The system
and user
prompts in the customPrompt
function are optional. If you omit either the system
or user
prompt, the default prompt for that field will be used. Example of customizing only the system prompt:
copilot.complete({
options: {
customPrompt: metadata => ({
system:
'You are an AI assistant specialized in writing React components, focusing on creating clean...',
}),
},
});
The customPrompt
function receives a completionMetadata
object, which contains information about the current editor state and can be used to tailor the prompt.
Property | Type | Description |
---|---|---|
language |
string |
The programming language of the code. |
cursorPosition |
{ lineNumber: number; column: number } |
The current cursor position in the editor. |
filename |
string or undefined
|
The name of the file being edited. Only available if you have provided the filename option in the registerCompletion function. |
technologies |
string[] or undefined
|
An array of technologies used in the project. Only available if you have provided the technologies option in the registerCompletion function. |
relatedFiles |
object[] or undefined
|
An array of objects containing the path and content of related files. Only available if you have provided the relatedFiles option in the registerCompletion function. |
textAfterCursor |
string |
The text that appears after the cursor. |
textBeforeCursor |
string |
The text that appears before the cursor. |
editorState |
object |
An object containing the completionMode property. |
The editorState.completionMode
can be one of the following:
Mode | Description |
---|---|
insert |
Indicates that there is a character immediately after the cursor. In this mode, the AI will generate content to be inserted at the cursor position. |
complete |
Indicates that there is a character after the cursor but not immediately. In this mode, the AI will generate content to complete the text from the cursor position. |
continue |
Indicates that there is no character after the cursor. In this mode, the AI will generate content to continue the text from the cursor position. |
For additional completionMetadata
needs, please open an issue.
The customPrompt
function should return an object with two properties:
Property | Type | Description |
---|---|---|
system |
string or undefined
|
A string representing the system prompt for the model. |
user |
string or undefined
|
A string representing the user prompt for the model. |
Here's an example of a custom prompt that focuses on generating React component code:
const customPrompt = ({textBeforeCursor, textAfterCursor}) => ({
system:
'You are an AI assistant specialized in writing React components. Focus on creating clean, reusable, and well-structured components.',
user: `Please complete the following React component:
${textBeforeCursor}
// Cursor position
${textAfterCursor}
Use modern React practices and hooks where appropriate. If you're adding new props, make sure to include proper TypeScript types. Please provide only the completed part of the code without additional comments or explanations.`,
});
copilot.complete({
options: {customPrompt},
});
By using a custom prompt, you can guide the model to generate completions that better fit your coding style, project requirements, or specific technologies you're working with.
While the example in this documentation uses JavaScript/Node.js (which is recommended), you can set up the API handler in any language or framework. For JavaScript, Monacopilot provides a built-in function that handles all the necessary steps, such as generating the prompt, sending it to the model, and processing the response. However, if you're using a different language, you'll need to implement these steps manually. Here's a general approach to implement the handler in your preferred language:
-
Create an endpoint that accepts POST requests (e.g.,
/complete
). -
The endpoint should expect a JSON body containing completion metadata.
-
Use the metadata to construct a prompt for your LLM.
-
Send the prompt to your chosen LLM and get the completion.
-
Return a JSON response with the following structure:
{ "completion": "Generated completion text" }
Or in case of an error:
{ "completion": null, "error": "Error message" }
- The prompt should instruct the model to return only the completion text, without any additional formatting or explanations.
- The completion text should be ready for direct insertion into the editor.
Check out the prompt.ts file to see how Monacopilot generates the prompt. This will give you an idea of how to structure the prompt for your LLM to achieve the best completions.
The request body's completionMetadata
object contains essential information for crafting a prompt for the LLM to generate accurate completions. See the Completion Metadata section for more details.
Here's a basic example using Python and FastAPI:
from fastapi import FastAPI, Request
app = FastAPI()
@app.post('/complete')
async def handle_completion(request: Request):
try:
body = await request.json()
metadata = body['completionMetadata']
prompt = f"""Please complete the following {metadata['language']} code:
{metadata['textBeforeCursor']}
<cursor>
{metadata['textAfterCursor']}
Use modern {metadata['language']} practices and hooks where appropriate. Please provide only the completed part of the
code without additional comments or explanations."""
# Simulate a response from a model
response = "Your model's response here"
return {
'completion': response,
'error': None
}
except Exception as e:
return {
'completion': None,
'error': str(e)
}
Now, Monacopilot is set up to send completion requests to the /complete
endpoint and receive completions in response.
registerCompletion(monaco, editor, {
endpoint: 'https://my-python-api.com/complete',
// ... other options
});
For guidelines on contributing, please read the contributing guide.
We welcome contributions from the community to enhance Monacopilot's capabilities and make it even more powerful. ❤️
For Tasks:
Click tags to check more tools for each tasksFor Jobs:
Alternative AI tools for monacopilot
Similar Open Source Tools
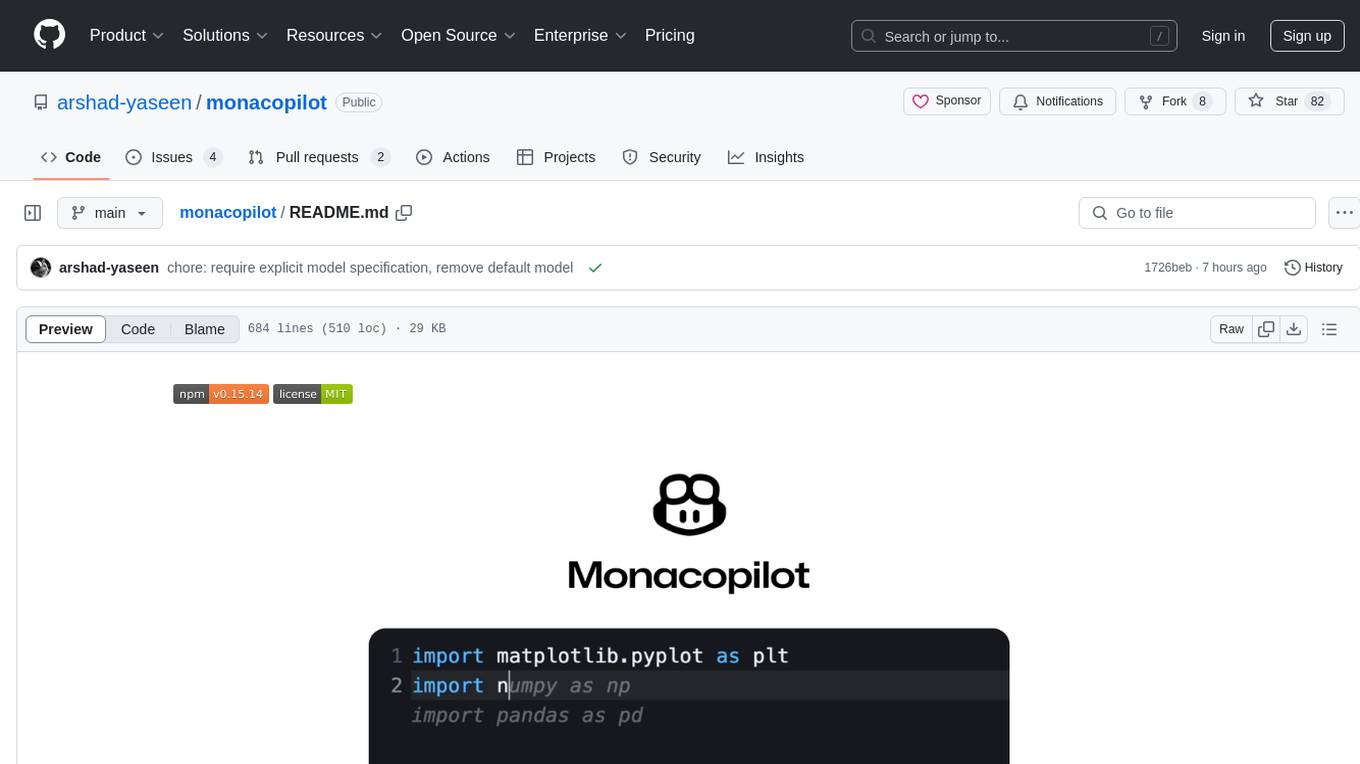
monacopilot
Monacopilot is a powerful and customizable AI auto-completion plugin for the Monaco Editor. It supports multiple AI providers such as Anthropic, OpenAI, Groq, and Google, providing real-time code completions with an efficient caching system. The plugin offers context-aware suggestions, customizable completion behavior, and framework agnostic features. Users can also customize the model support and trigger completions manually. Monacopilot is designed to enhance coding productivity by providing accurate and contextually appropriate completions in daily spoken language.
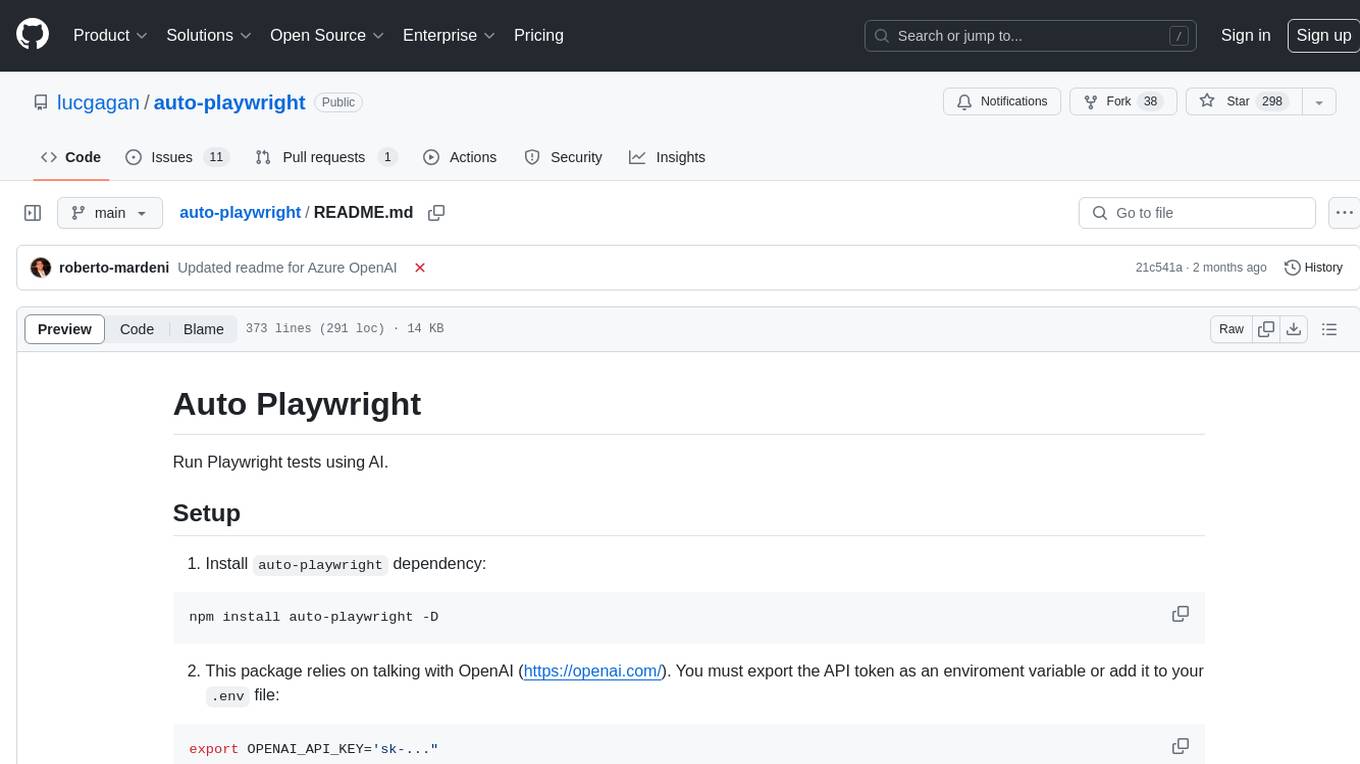
auto-playwright
Auto Playwright is a tool that allows users to run Playwright tests using AI. It eliminates the need for selectors by determining actions at runtime based on plain-text instructions. Users can automate complex scenarios, write tests concurrently with or before functionality development, and benefit from rapid test creation. The tool supports various Playwright actions and offers additional options for debugging and customization. It uses HTML sanitization to reduce costs and improve text quality when interacting with the OpenAI API.
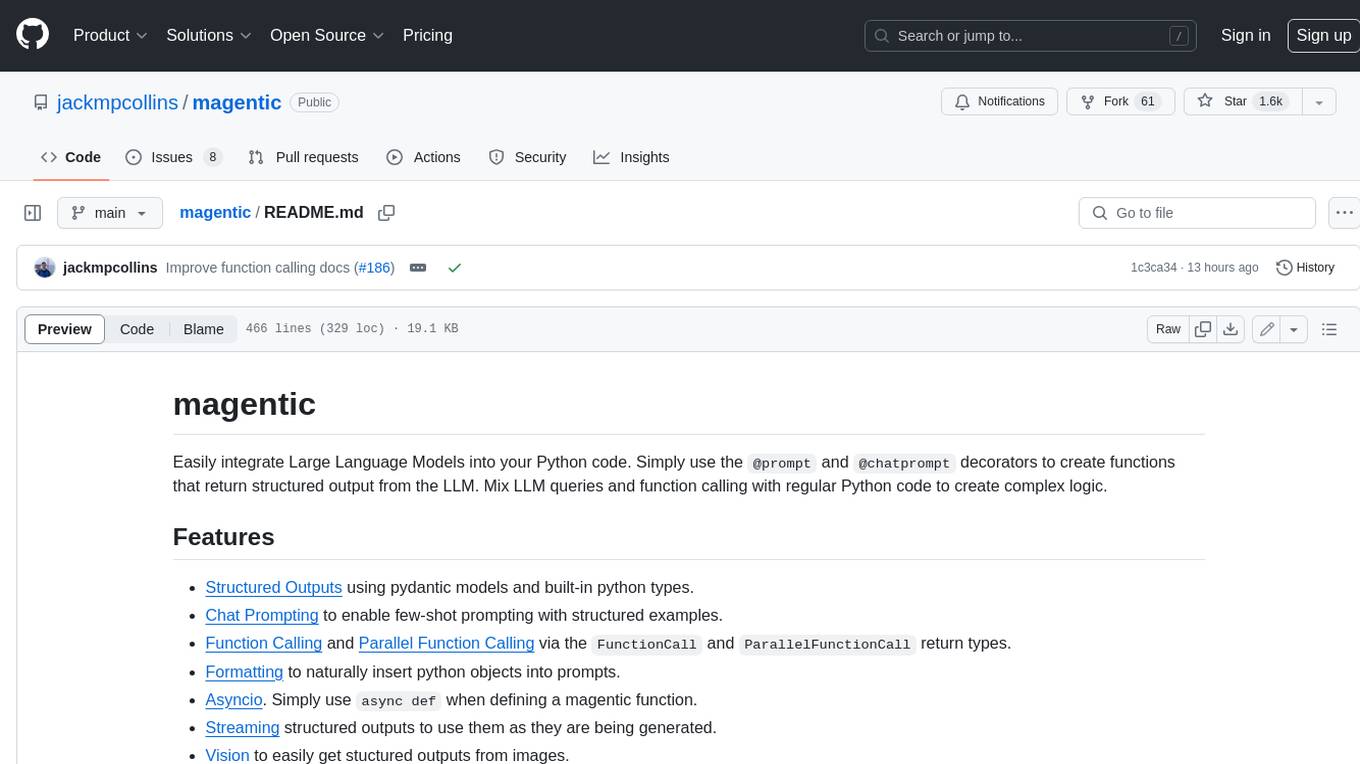
magentic
Easily integrate Large Language Models into your Python code. Simply use the `@prompt` and `@chatprompt` decorators to create functions that return structured output from the LLM. Mix LLM queries and function calling with regular Python code to create complex logic.
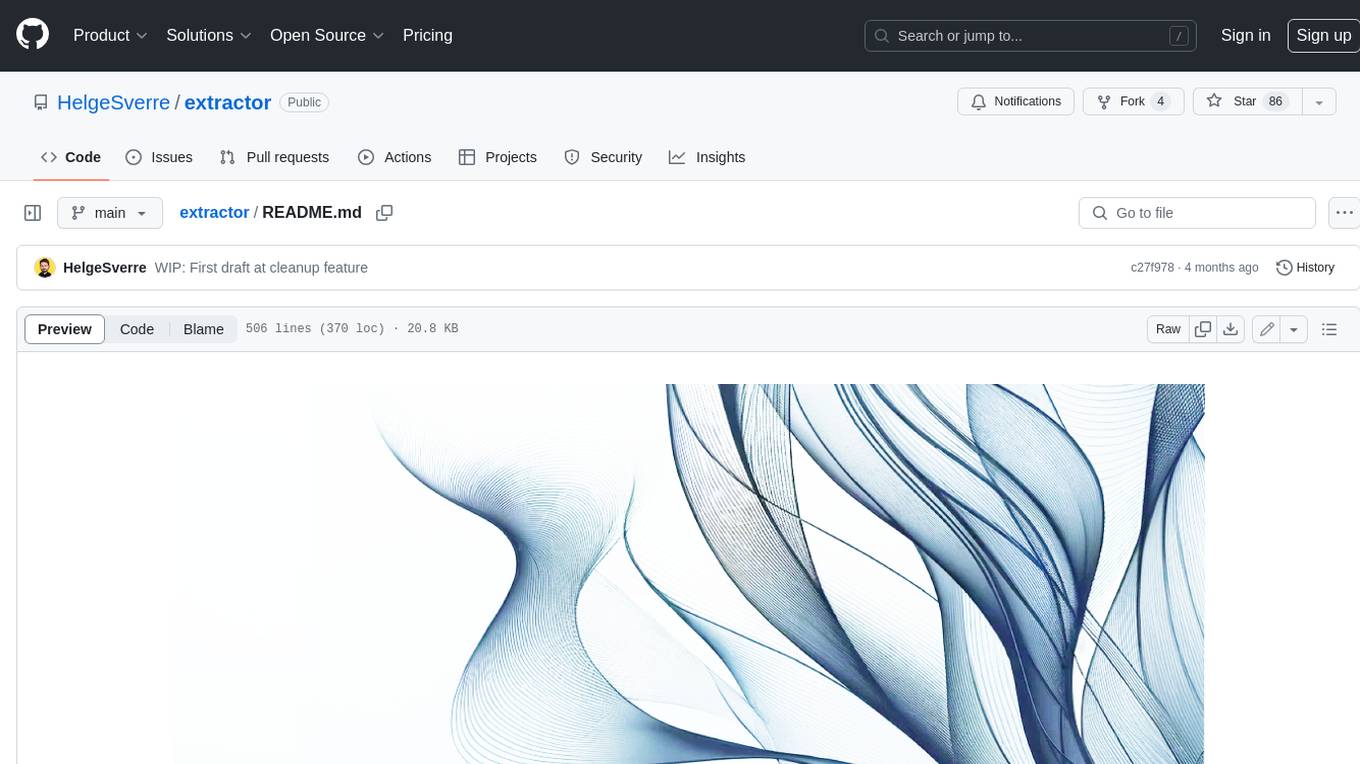
extractor
Extractor is an AI-powered data extraction library for Laravel that leverages OpenAI's capabilities to effortlessly extract structured data from various sources, including images, PDFs, and emails. It features a convenient wrapper around OpenAI Chat and Completion endpoints, supports multiple input formats, includes a flexible Field Extractor for arbitrary data extraction, and integrates with Textract for OCR functionality. Extractor utilizes JSON Mode from the latest GPT-3.5 and GPT-4 models, providing accurate and efficient data extraction.
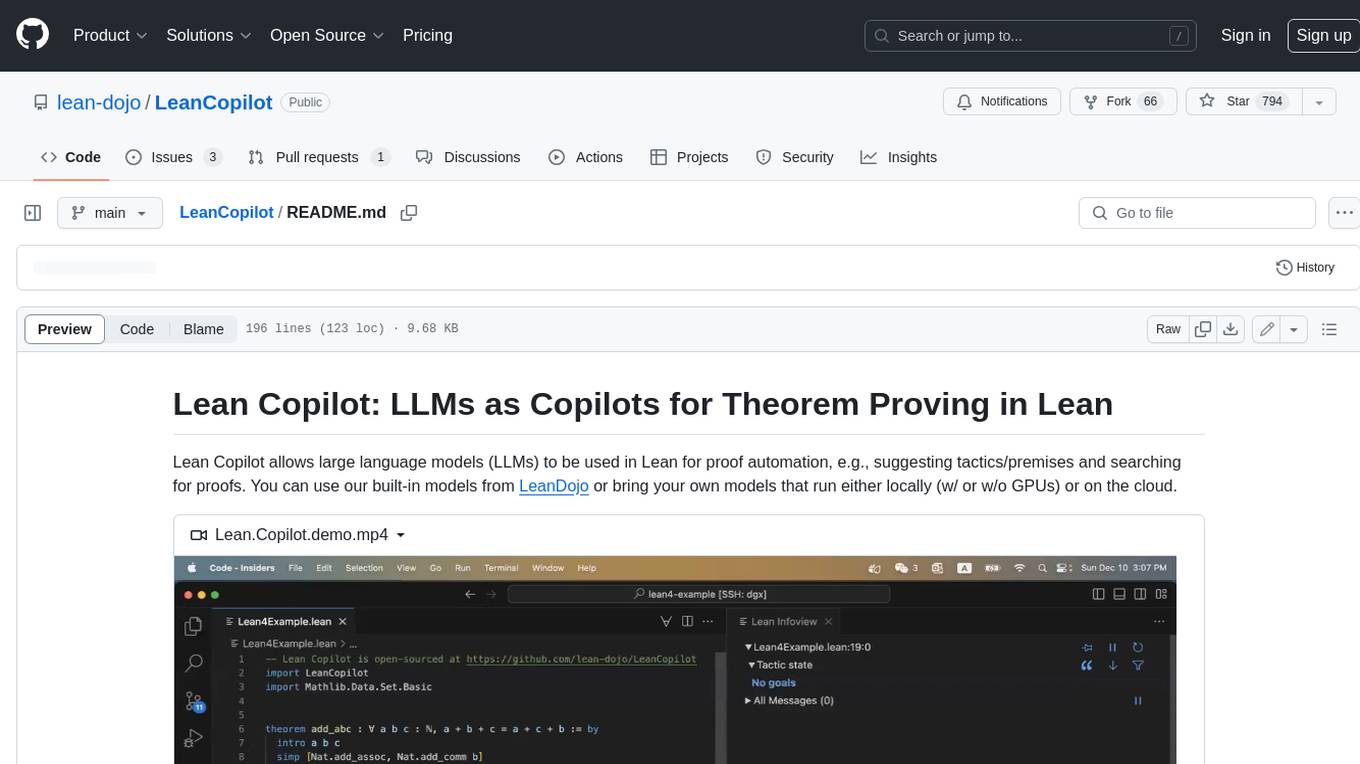
LeanCopilot
Lean Copilot is a tool that enables the use of large language models (LLMs) in Lean for proof automation. It provides features such as suggesting tactics/premises, searching for proofs, and running inference of LLMs. Users can utilize built-in models from LeanDojo or bring their own models to run locally or on the cloud. The tool supports platforms like Linux, macOS, and Windows WSL, with optional CUDA and cuDNN for GPU acceleration. Advanced users can customize behavior using Tactic APIs and Model APIs. Lean Copilot also allows users to bring their own models through ExternalGenerator or ExternalEncoder. The tool comes with caveats such as occasional crashes and issues with premise selection and proof search. Users can get in touch through GitHub Discussions for questions, bug reports, feature requests, and suggestions. The tool is designed to enhance theorem proving in Lean using LLMs.
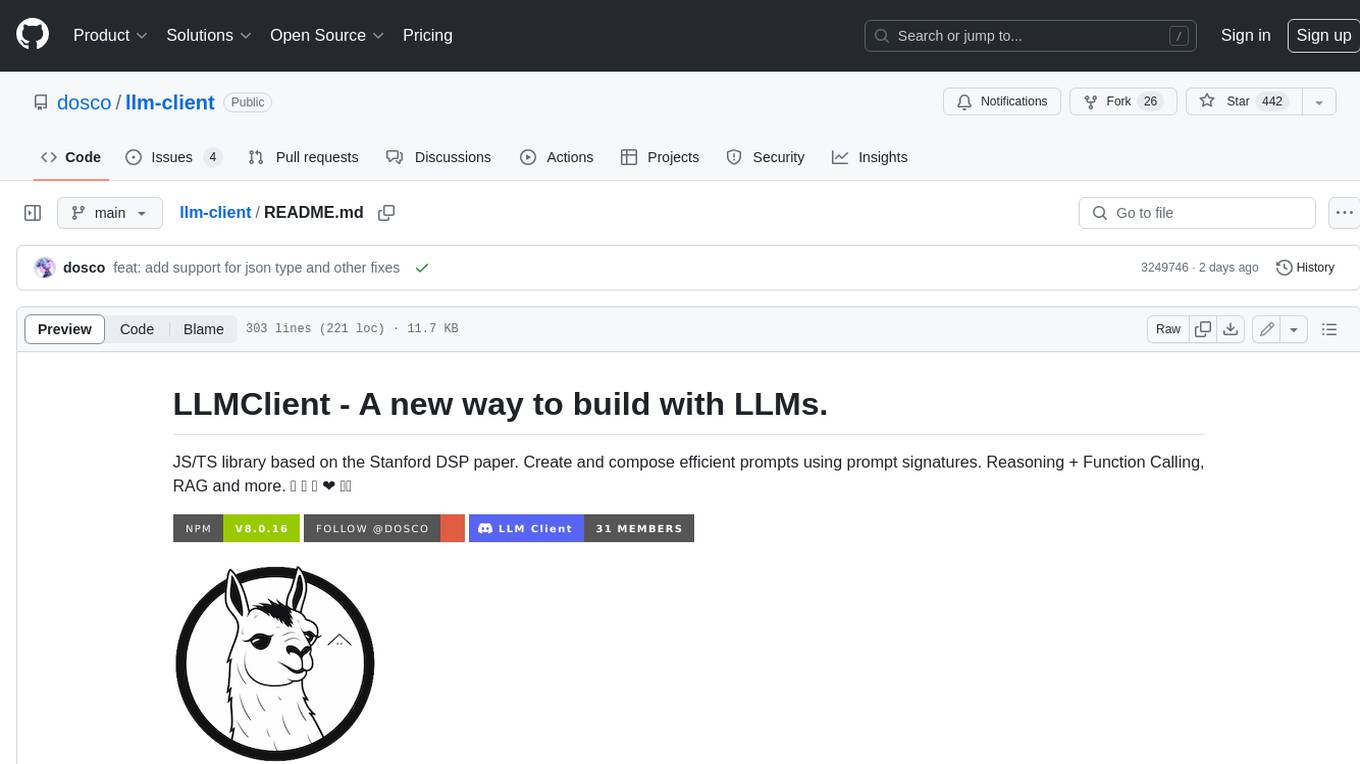
llm-client
LLMClient is a JavaScript/TypeScript library that simplifies working with large language models (LLMs) by providing an easy-to-use interface for building and composing efficient prompts using prompt signatures. These signatures enable the automatic generation of typed prompts, allowing developers to leverage advanced capabilities like reasoning, function calling, RAG, ReAcT, and Chain of Thought. The library supports various LLMs and vector databases, making it a versatile tool for a wide range of applications.
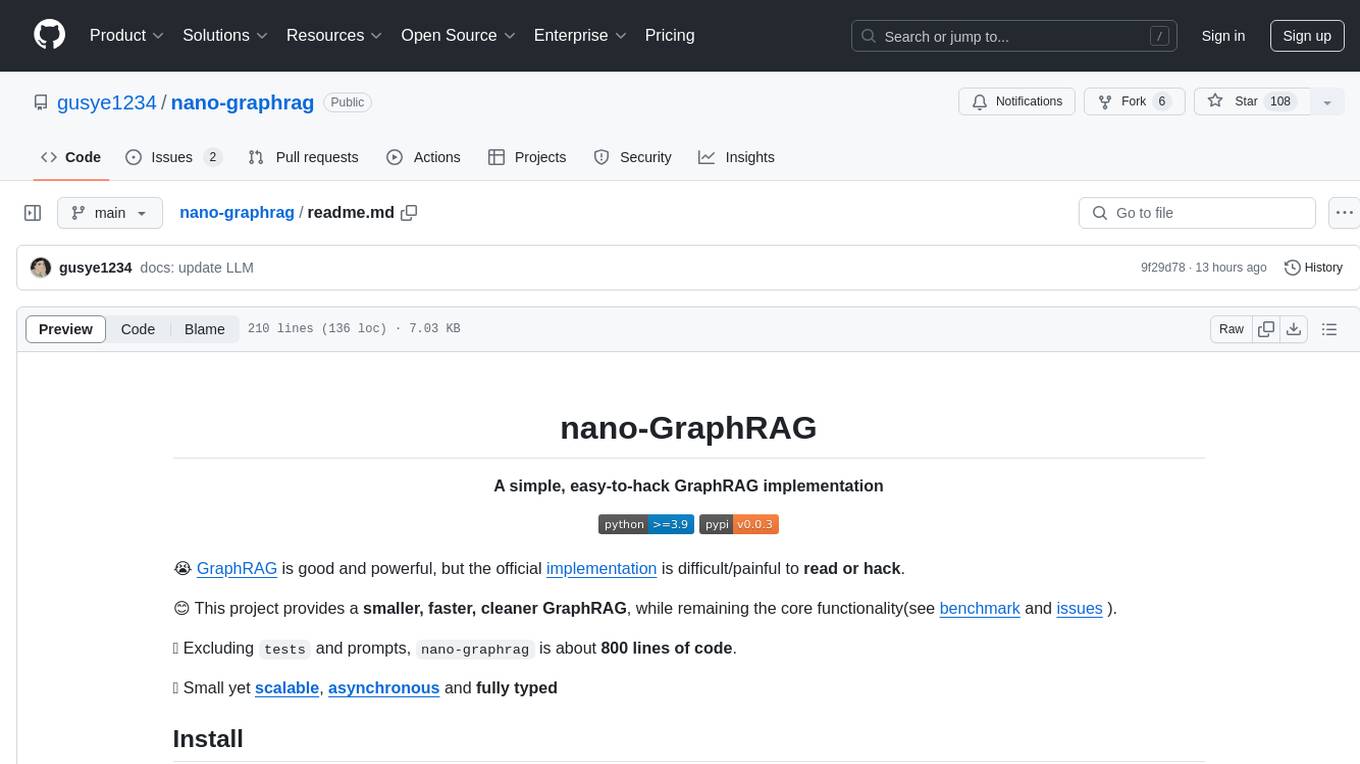
nano-graphrag
nano-GraphRAG is a simple, easy-to-hack implementation of GraphRAG that provides a smaller, faster, and cleaner version of the official implementation. It is about 800 lines of code, small yet scalable, asynchronous, and fully typed. The tool supports incremental insert, async methods, and various parameters for customization. Users can replace storage components and LLM functions as needed. It also allows for embedding function replacement and comes with pre-defined prompts for entity extraction and community reports. However, some features like covariates and global search implementation differ from the original GraphRAG. Future versions aim to address issues related to data source ID, community description truncation, and add new components.
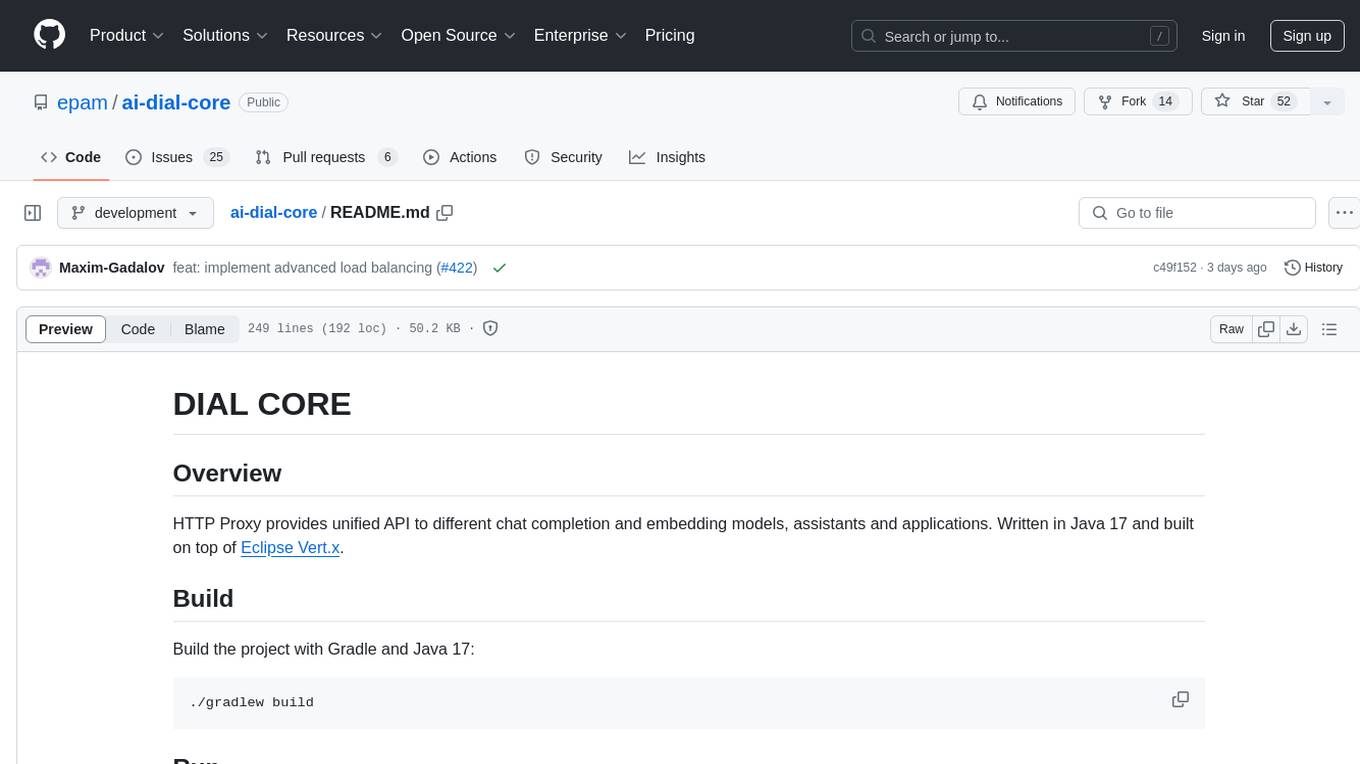
ai-dial-core
AI DIAL Core is an HTTP Proxy that provides a unified API to different chat completion and embedding models, assistants, and applications. It is written in Java 17 and built on Eclipse Vert.x. The core functionality includes handling static and dynamic settings, deployment on Kubernetes using Helm charts, and storing user data in Blob Storage and Redis. It supports various identity providers, storage providers like AWS S3, Google Cloud Storage, and Azure Blob Store, and features like AI DIAL Addons, Interceptors, Assistants, Applications, and Models with customizable parameters and configurations.
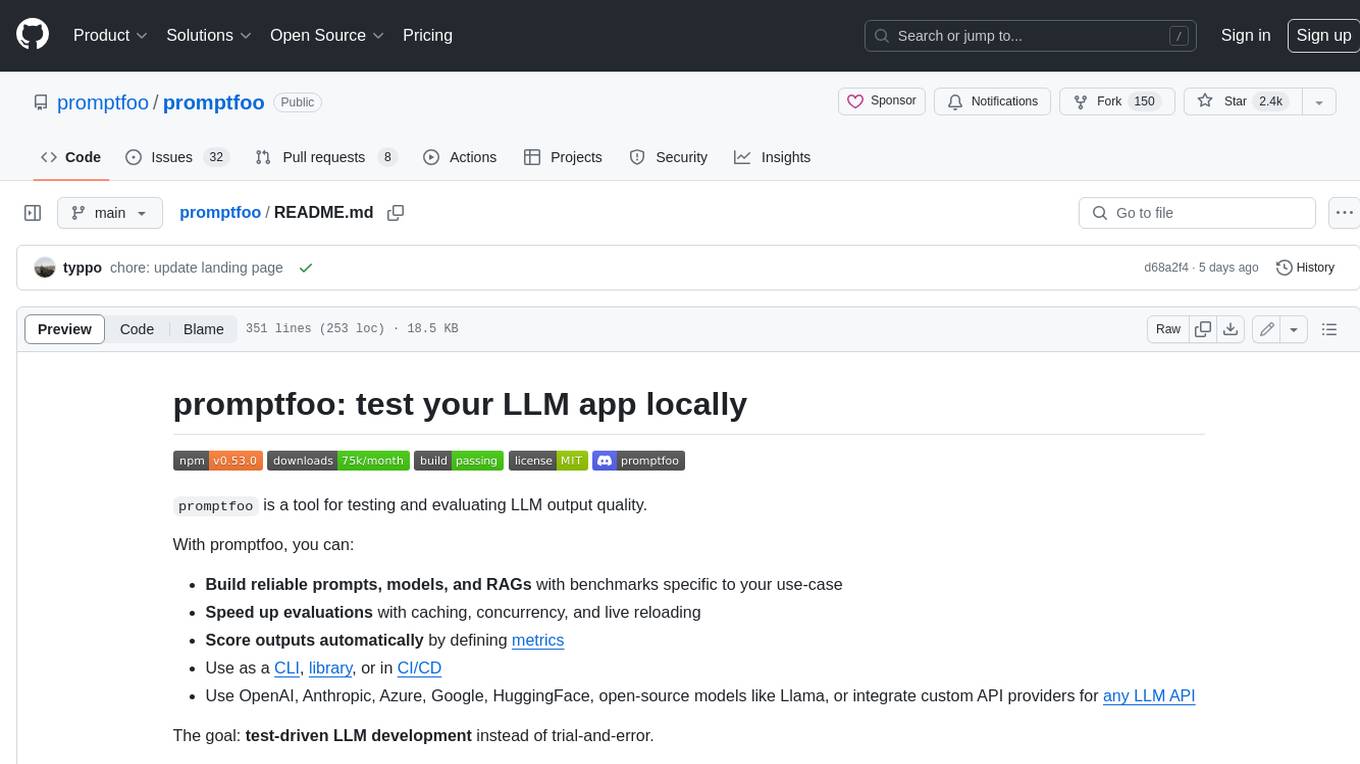
promptfoo
Promptfoo is a tool for testing and evaluating LLM output quality. With promptfoo, you can build reliable prompts, models, and RAGs with benchmarks specific to your use-case, speed up evaluations with caching, concurrency, and live reloading, score outputs automatically by defining metrics, use as a CLI, library, or in CI/CD, and use OpenAI, Anthropic, Azure, Google, HuggingFace, open-source models like Llama, or integrate custom API providers for any LLM API.
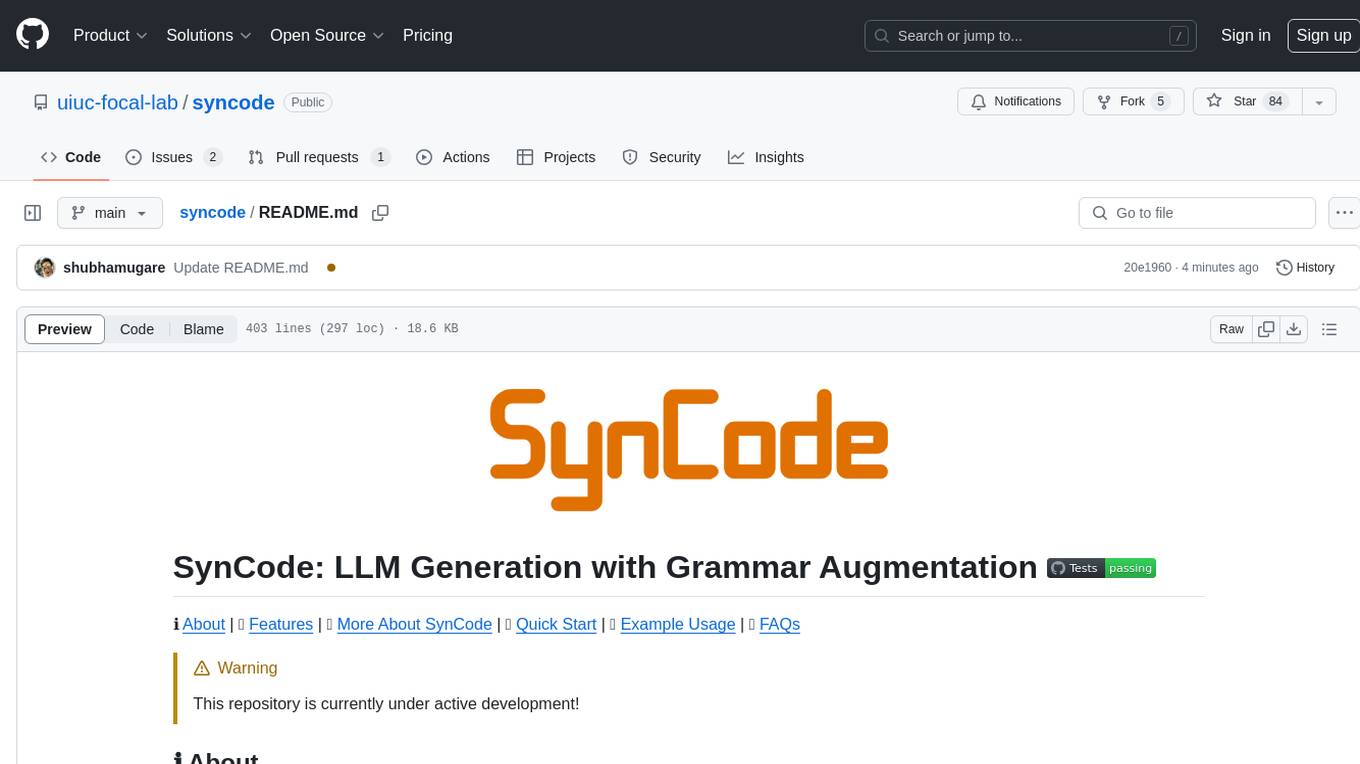
syncode
SynCode is a novel framework for the grammar-guided generation of Large Language Models (LLMs) that ensures syntactically valid output with respect to defined Context-Free Grammar (CFG) rules. It supports general-purpose programming languages like Python, Go, SQL, JSON, and more, allowing users to define custom grammars using EBNF syntax. The tool compares favorably to other constrained decoders and offers features like fast grammar-guided generation, compatibility with HuggingFace Language Models, and the ability to work with various decoding strategies.
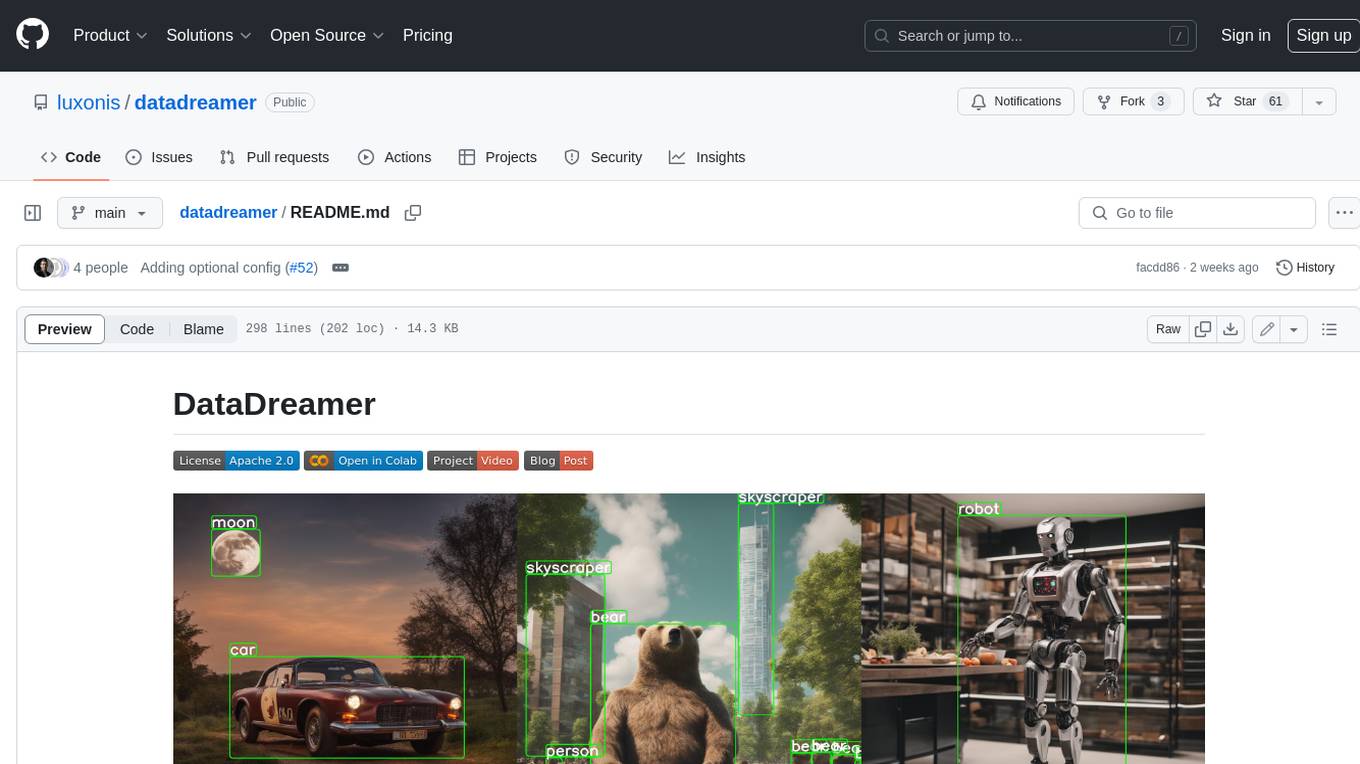
datadreamer
DataDreamer is an advanced toolkit designed to facilitate the development of edge AI models by enabling synthetic data generation, knowledge extraction from pre-trained models, and creation of efficient and potent models. It eliminates the need for extensive datasets by generating synthetic datasets, leverages latent knowledge from pre-trained models, and focuses on creating compact models suitable for integration into any device and performance for specialized tasks. The toolkit offers features like prompt generation, image generation, dataset annotation, and tools for training small-scale neural networks for edge deployment. It provides hardware requirements, usage instructions, available models, and limitations to consider while using the library.
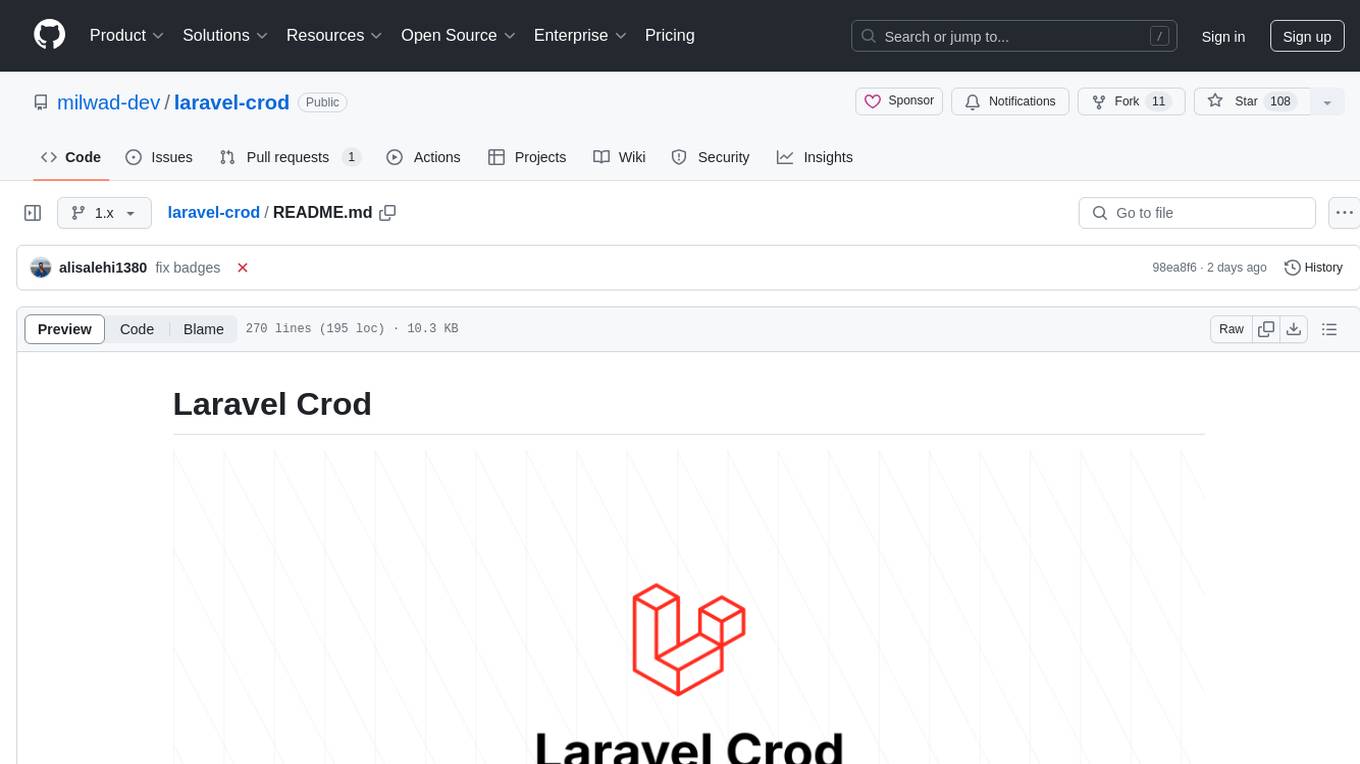
laravel-crod
Laravel Crod is a package designed to facilitate the implementation of CRUD operations in Laravel projects. It allows users to quickly generate controllers, models, migrations, services, repositories, views, and requests with various customization options. The package simplifies tasks such as creating resource controllers, making models fillable, querying repositories and services, and generating additional files like seeders and factories. Laravel Crod aims to streamline the process of building CRUD functionalities in Laravel applications by providing a set of commands and tools for developers.
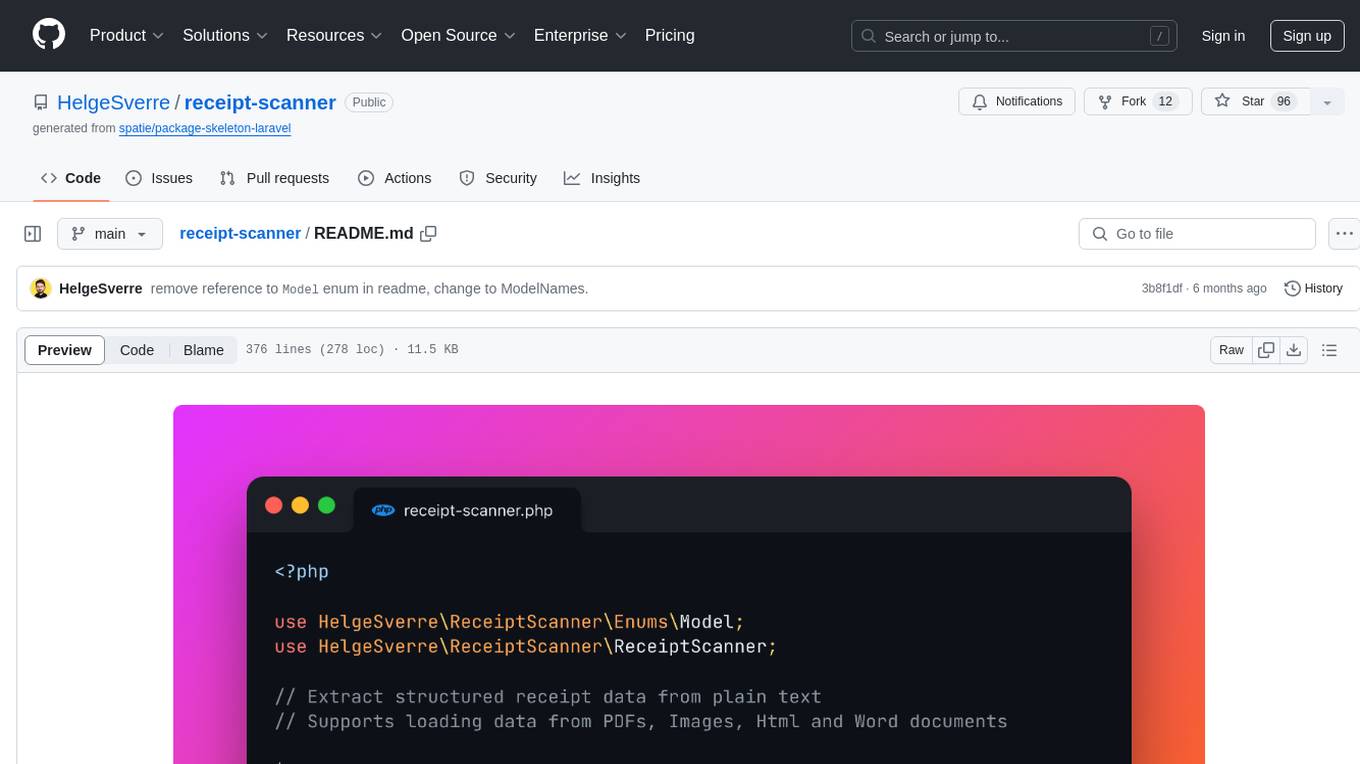
receipt-scanner
The receipt-scanner repository is an AI-Powered Receipt and Invoice Scanner for Laravel that allows users to easily extract structured receipt data from images, PDFs, and emails within their Laravel application using OpenAI. It provides a light wrapper around OpenAI Chat and Completion endpoints, supports various input formats, and integrates with Textract for OCR functionality. Users can install the package via composer, publish configuration files, and use it to extract data from plain text, PDFs, images, Word documents, and web content. The scanned receipt data is parsed into a DTO structure with main classes like Receipt, Merchant, and LineItem.
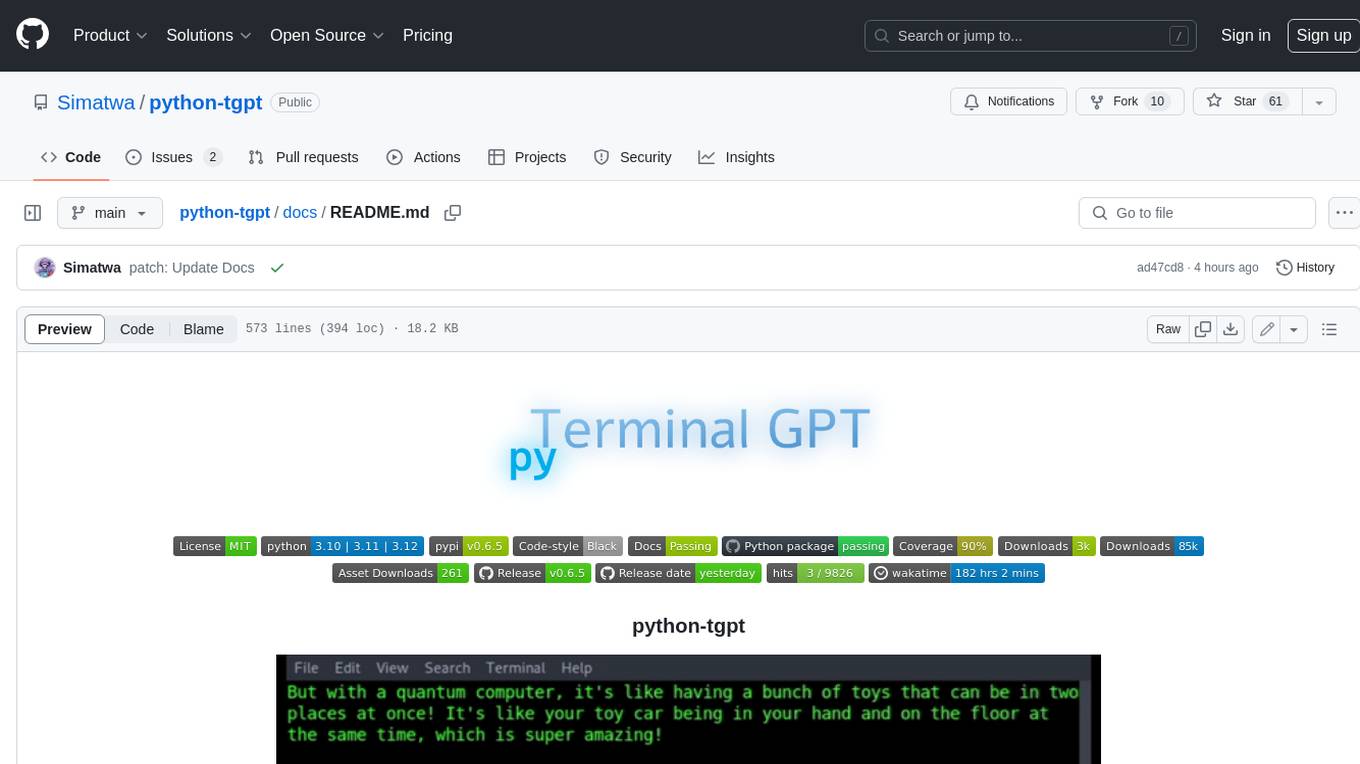
python-tgpt
Python-tgpt is a Python package that enables seamless interaction with over 45 free LLM providers without requiring an API key. It also provides image generation capabilities. The name _python-tgpt_ draws inspiration from its parent project tgpt, which operates on Golang. Through this Python adaptation, users can effortlessly engage with a number of free LLMs available, fostering a smoother AI interaction experience.
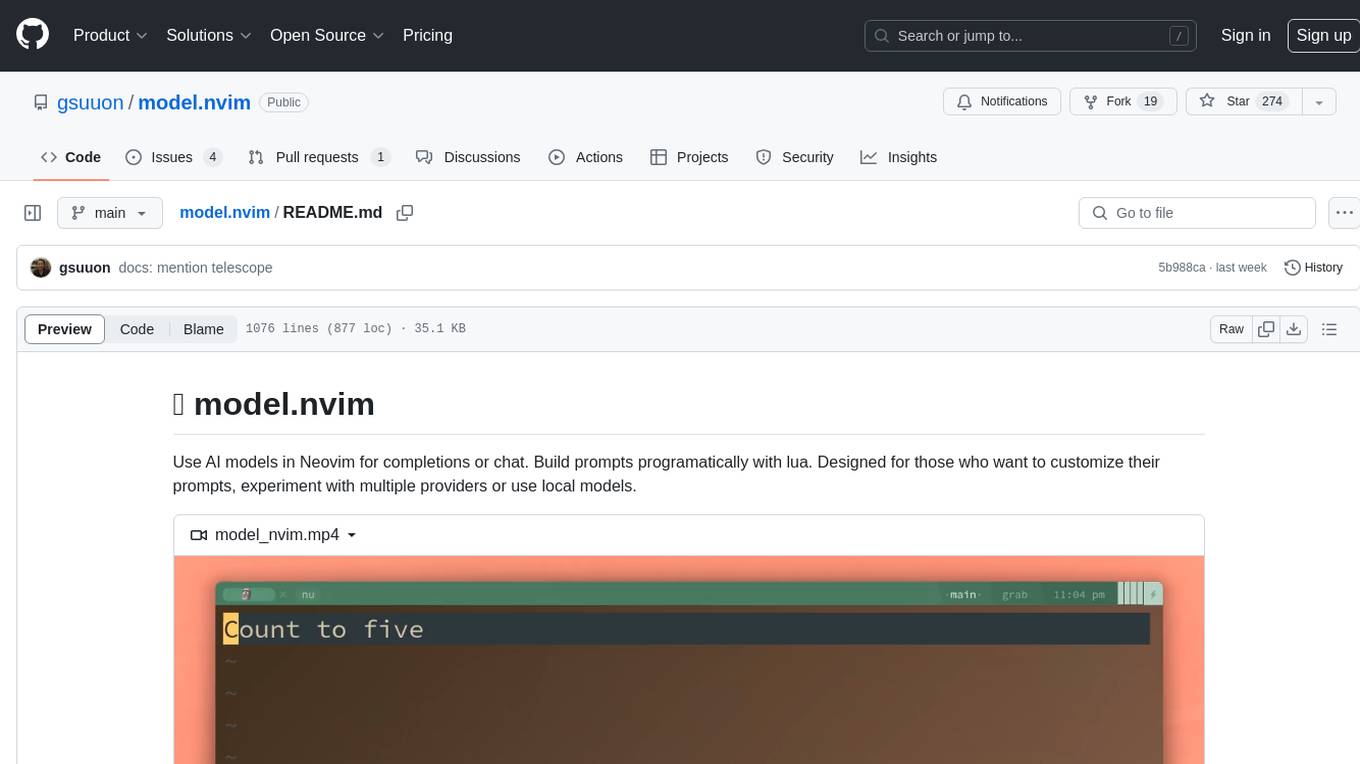
model.nvim
model.nvim is a tool designed for Neovim users who want to utilize AI models for completions or chat within their text editor. It allows users to build prompts programmatically with Lua, customize prompts, experiment with multiple providers, and use both hosted and local models. The tool supports features like provider agnosticism, programmatic prompts in Lua, async and multistep prompts, streaming completions, and chat functionality in 'mchat' filetype buffer. Users can customize prompts, manage responses, and context, and utilize various providers like OpenAI ChatGPT, Google PaLM, llama.cpp, ollama, and more. The tool also supports treesitter highlights and folds for chat buffers.
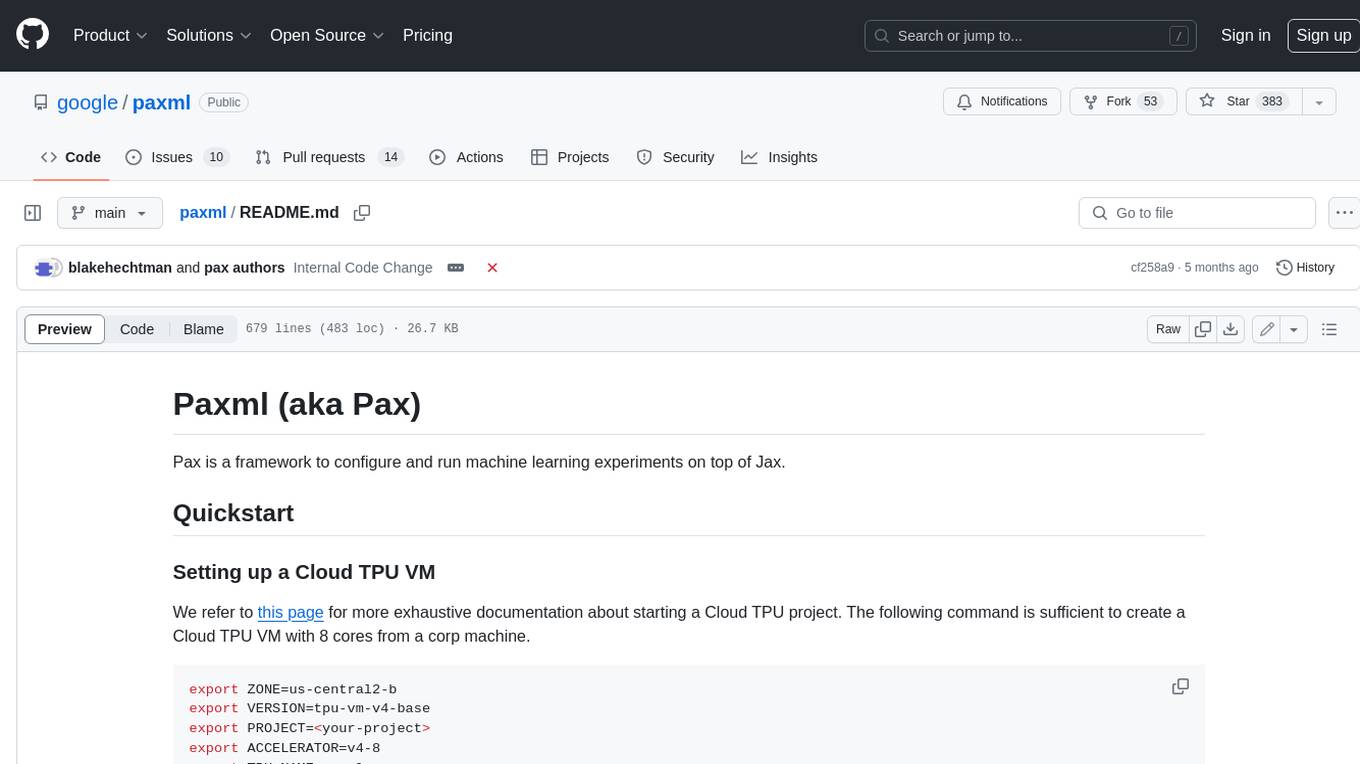
paxml
Pax is a framework to configure and run machine learning experiments on top of Jax.
For similar tasks
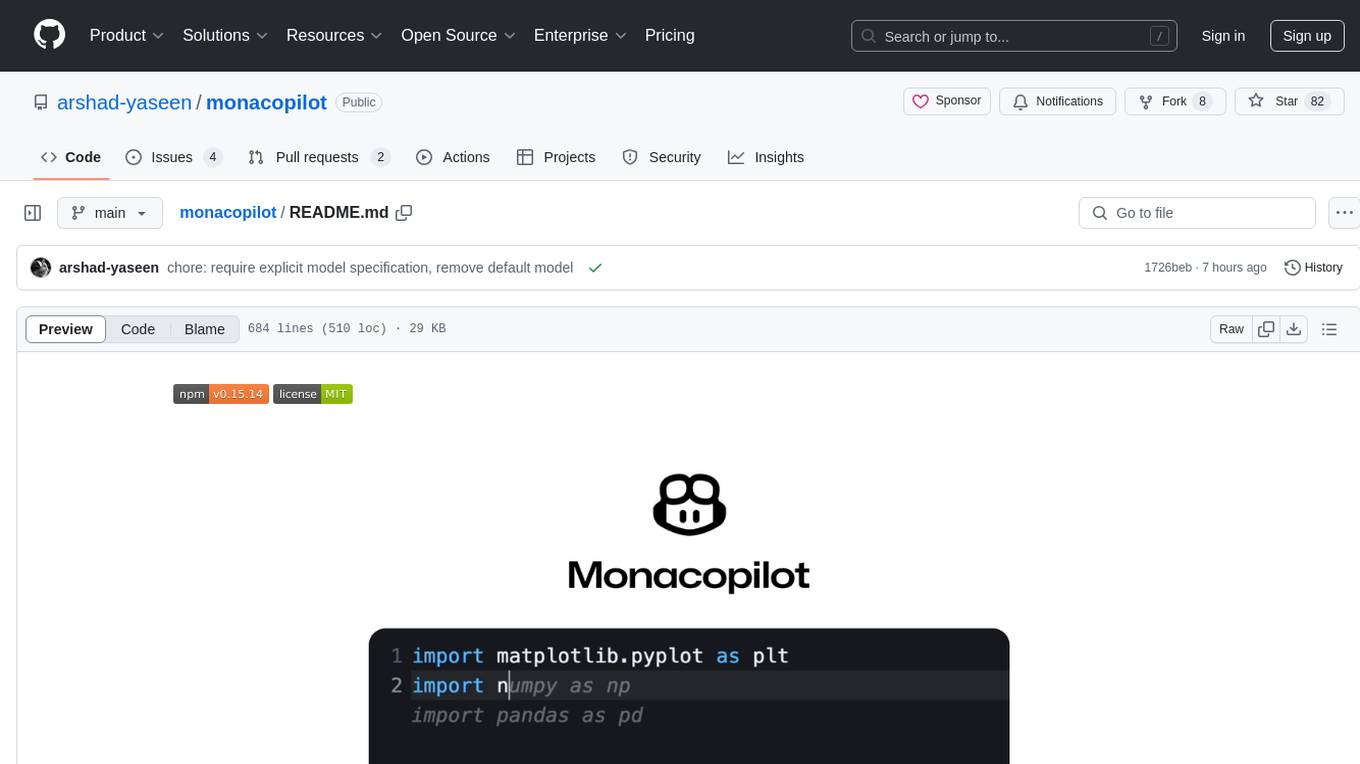
monacopilot
Monacopilot is a powerful and customizable AI auto-completion plugin for the Monaco Editor. It supports multiple AI providers such as Anthropic, OpenAI, Groq, and Google, providing real-time code completions with an efficient caching system. The plugin offers context-aware suggestions, customizable completion behavior, and framework agnostic features. Users can also customize the model support and trigger completions manually. Monacopilot is designed to enhance coding productivity by providing accurate and contextually appropriate completions in daily spoken language.
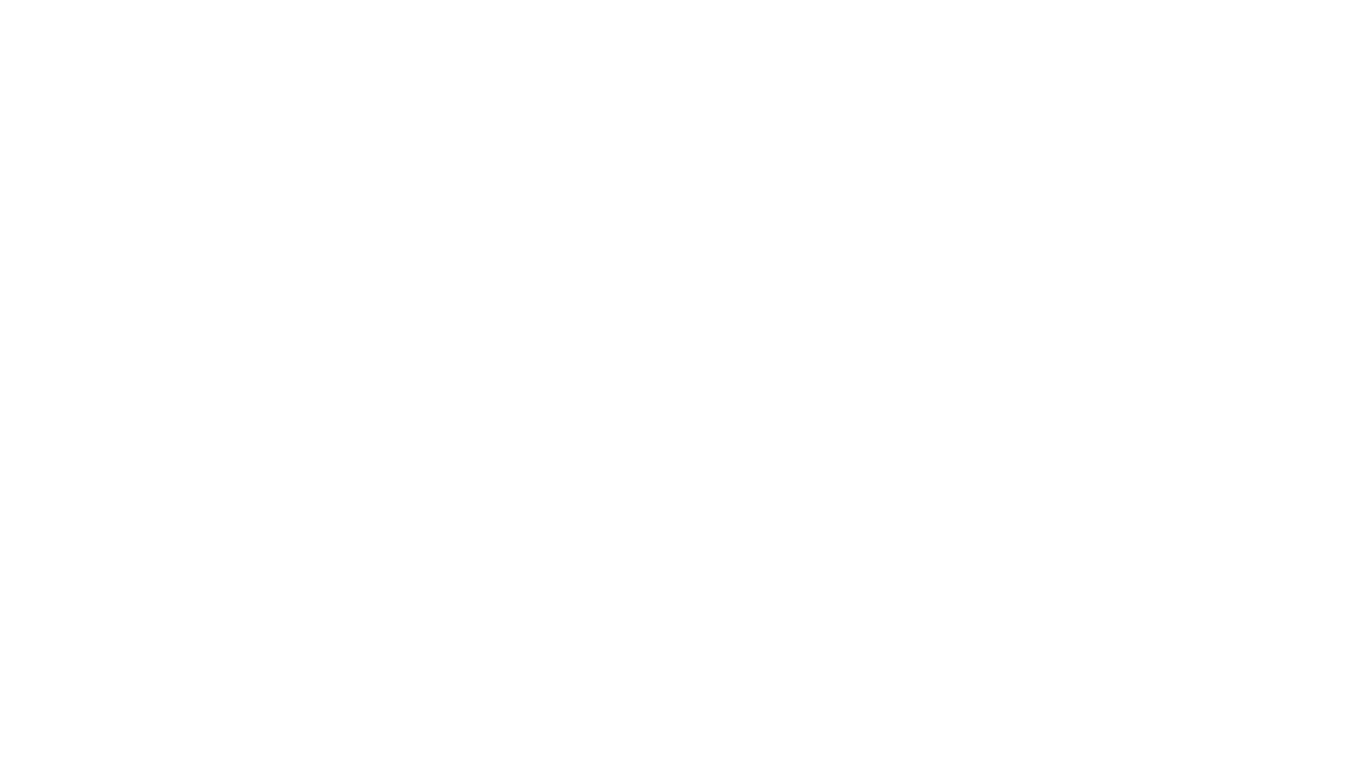
QodeAssist
QodeAssist is an AI-powered coding assistant plugin for Qt Creator, offering intelligent code completion and suggestions for C++ and QML. It leverages large language models like Ollama to enhance coding productivity with context-aware AI assistance directly in the Qt development environment. The plugin supports multiple LLM providers, extensive model-specific templates, and easy configuration for enhanced coding experience.
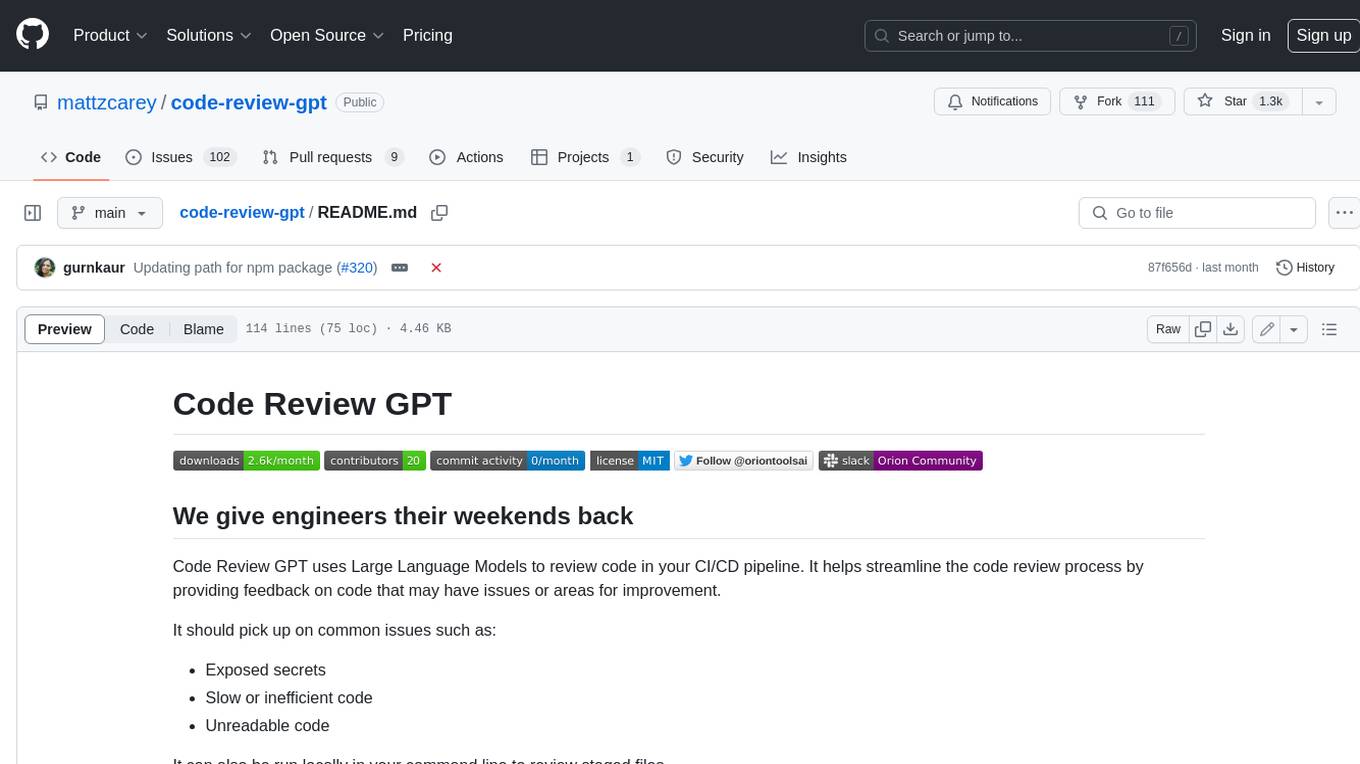
code-review-gpt
Code Review GPT uses Large Language Models to review code in your CI/CD pipeline. It helps streamline the code review process by providing feedback on code that may have issues or areas for improvement. It should pick up on common issues such as exposed secrets, slow or inefficient code, and unreadable code. It can also be run locally in your command line to review staged files. Code Review GPT is in alpha and should be used for fun only. It may provide useful feedback but please check any suggestions thoroughly.
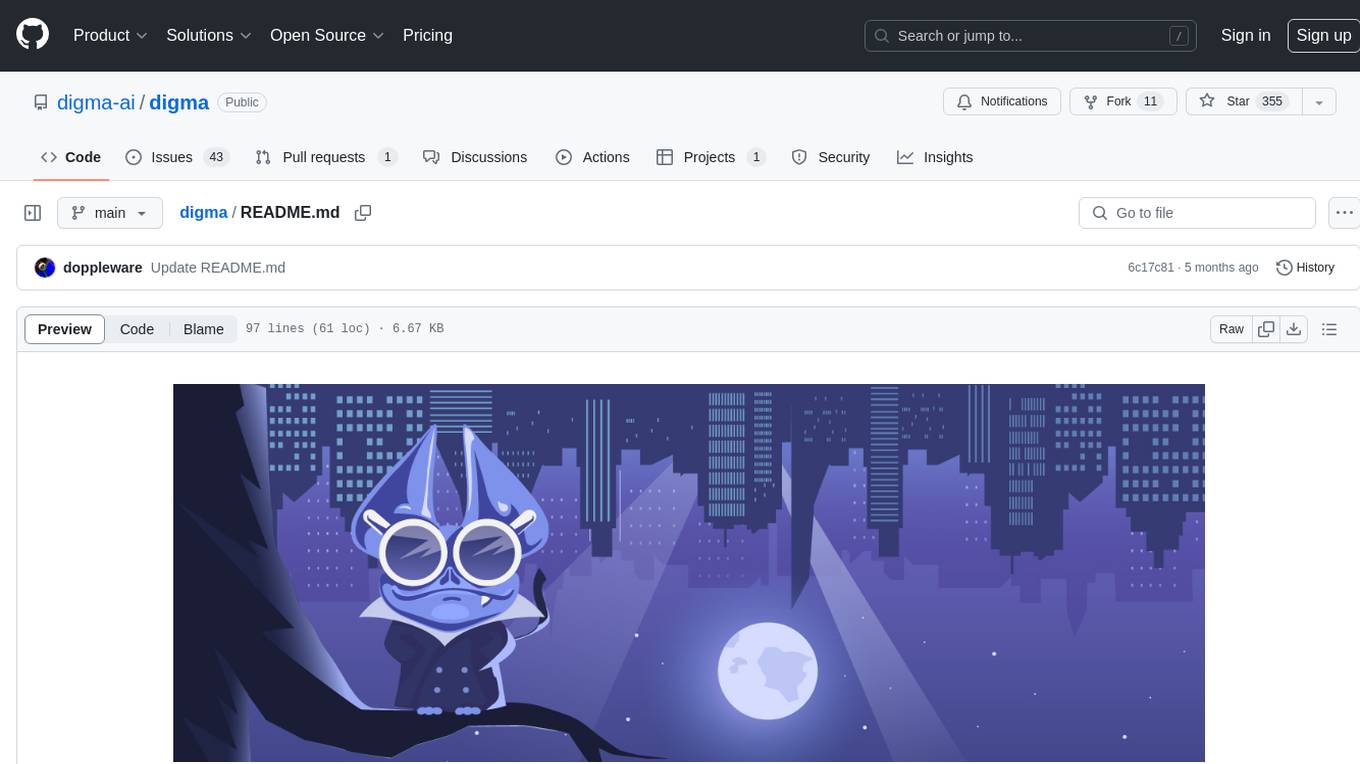
digma
Digma is a Continuous Feedback platform that provides code-level insights related to performance, errors, and usage during development. It empowers developers to own their code all the way to production, improving code quality and preventing critical issues. Digma integrates with OpenTelemetry traces and metrics to generate insights in the IDE, helping developers analyze code scalability, bottlenecks, errors, and usage patterns.
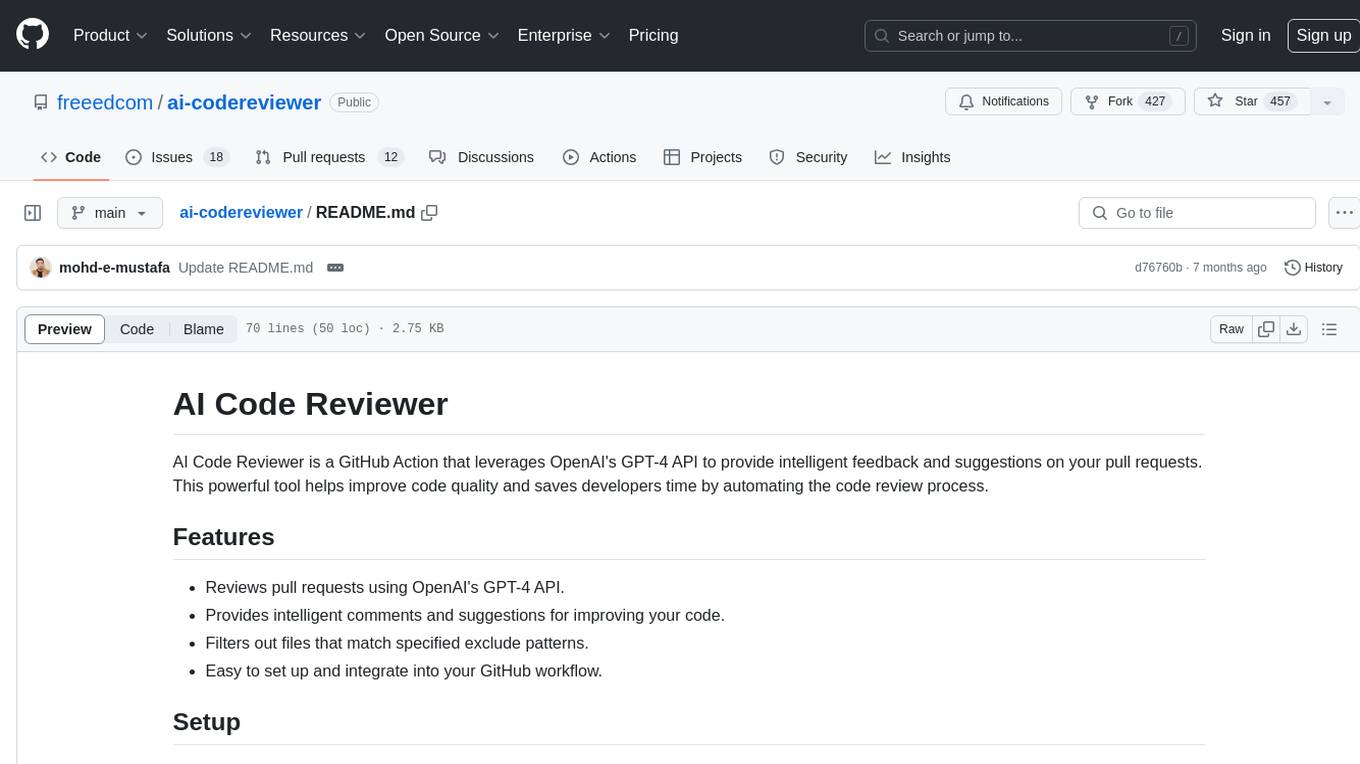
ai-codereviewer
AI Code Reviewer is a GitHub Action that utilizes OpenAI's GPT-4 API to provide intelligent feedback and suggestions on pull requests. It helps enhance code quality and streamline the code review process by offering insightful comments and filtering out specified files. The tool is easy to set up and integrate into GitHub workflows.
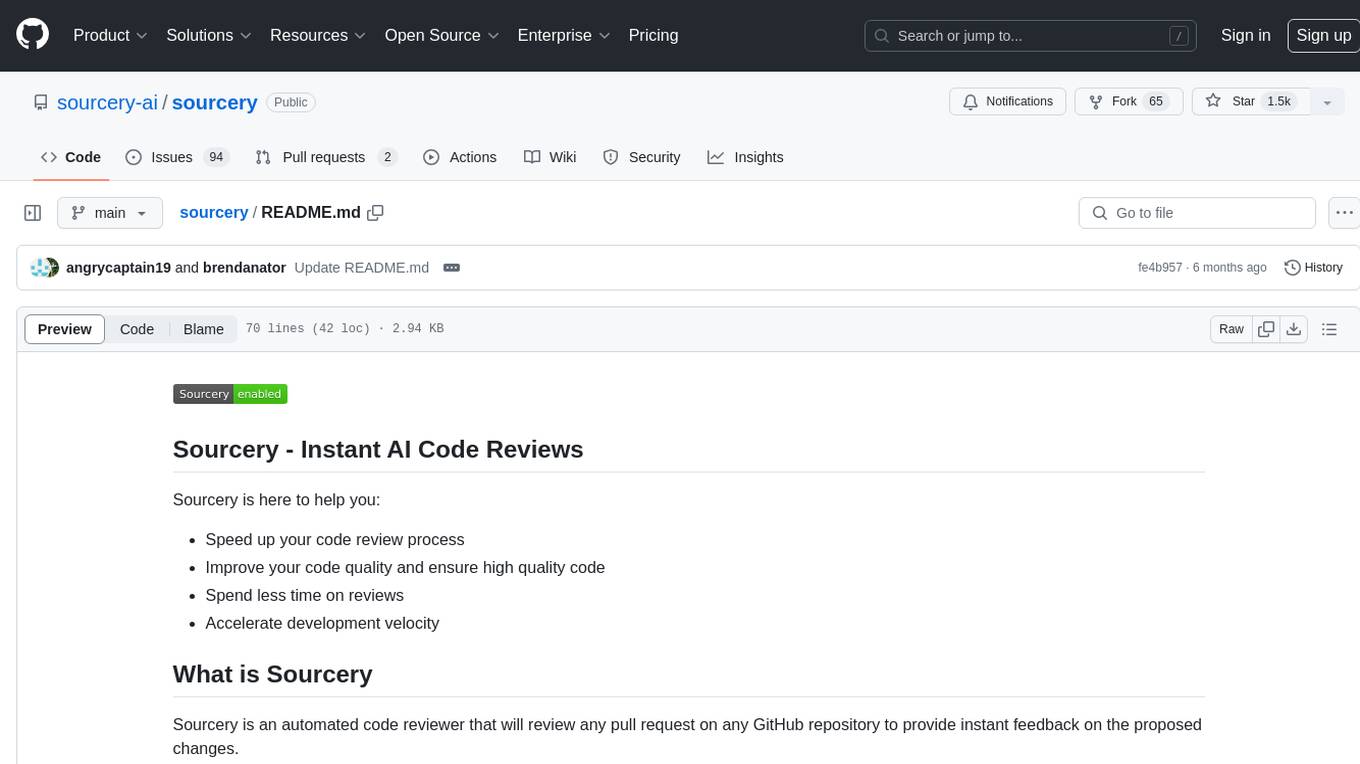
sourcery
Sourcery is an automated code reviewer tool that provides instant feedback on pull requests, helping to speed up the code review process, improve code quality, and accelerate development velocity. It offers high-level feedback, line-by-line suggestions, and aims to mimic the type of code review one would expect from a colleague. Sourcery can also be used as an IDE coding assistant to understand existing code, add unit tests, optimize code, and improve code quality with instant suggestions. It is free for public repos/open source projects and offers a 14-day trial for private repos.
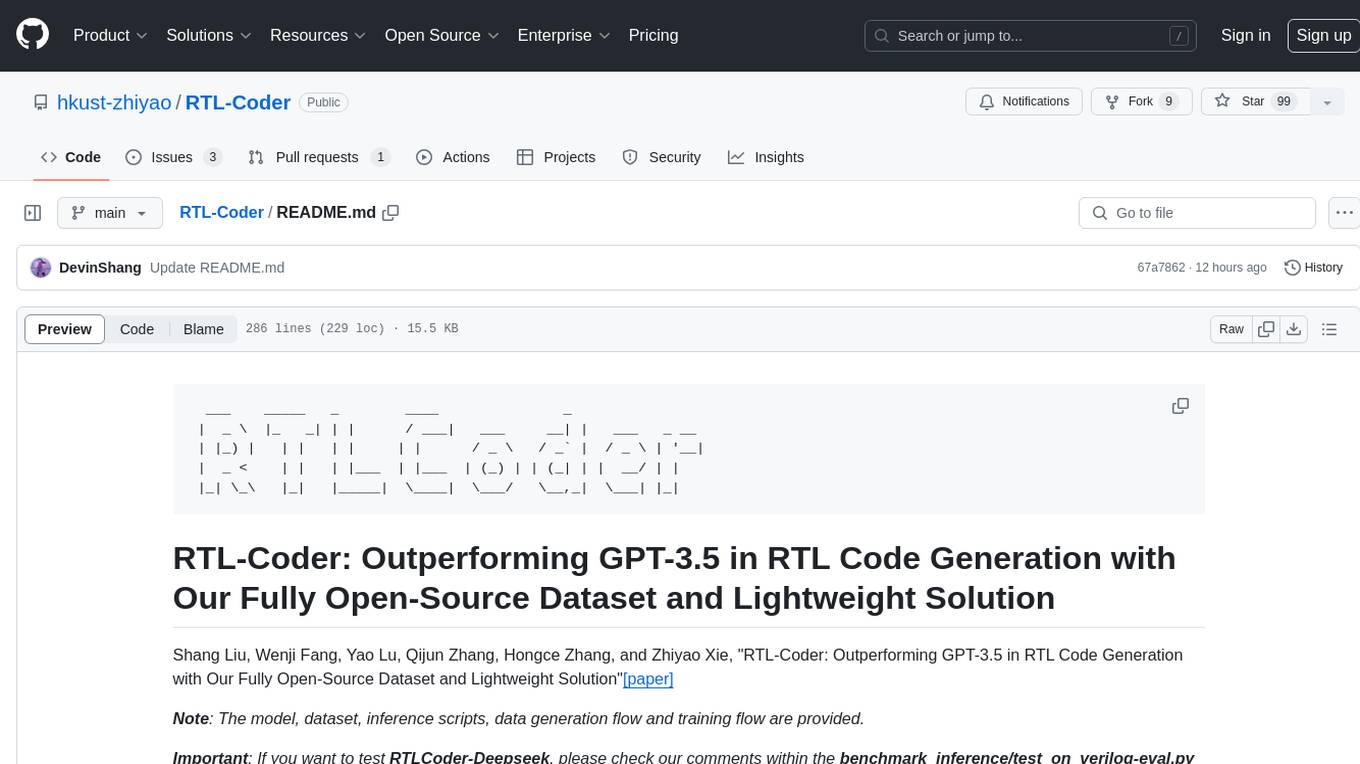
RTL-Coder
RTL-Coder is a tool designed to outperform GPT-3.5 in RTL code generation by providing a fully open-source dataset and a lightweight solution. It targets Verilog code generation and offers an automated flow to generate a large labeled dataset with over 27,000 diverse Verilog design problems and answers. The tool addresses the data availability challenge in IC design-related tasks and can be used for various applications beyond LLMs. The tool includes four RTL code generation models available on the HuggingFace platform, each with specific features and performance characteristics. Additionally, RTL-Coder introduces a new LLM training scheme based on code quality feedback to further enhance model performance and reduce GPU memory consumption.
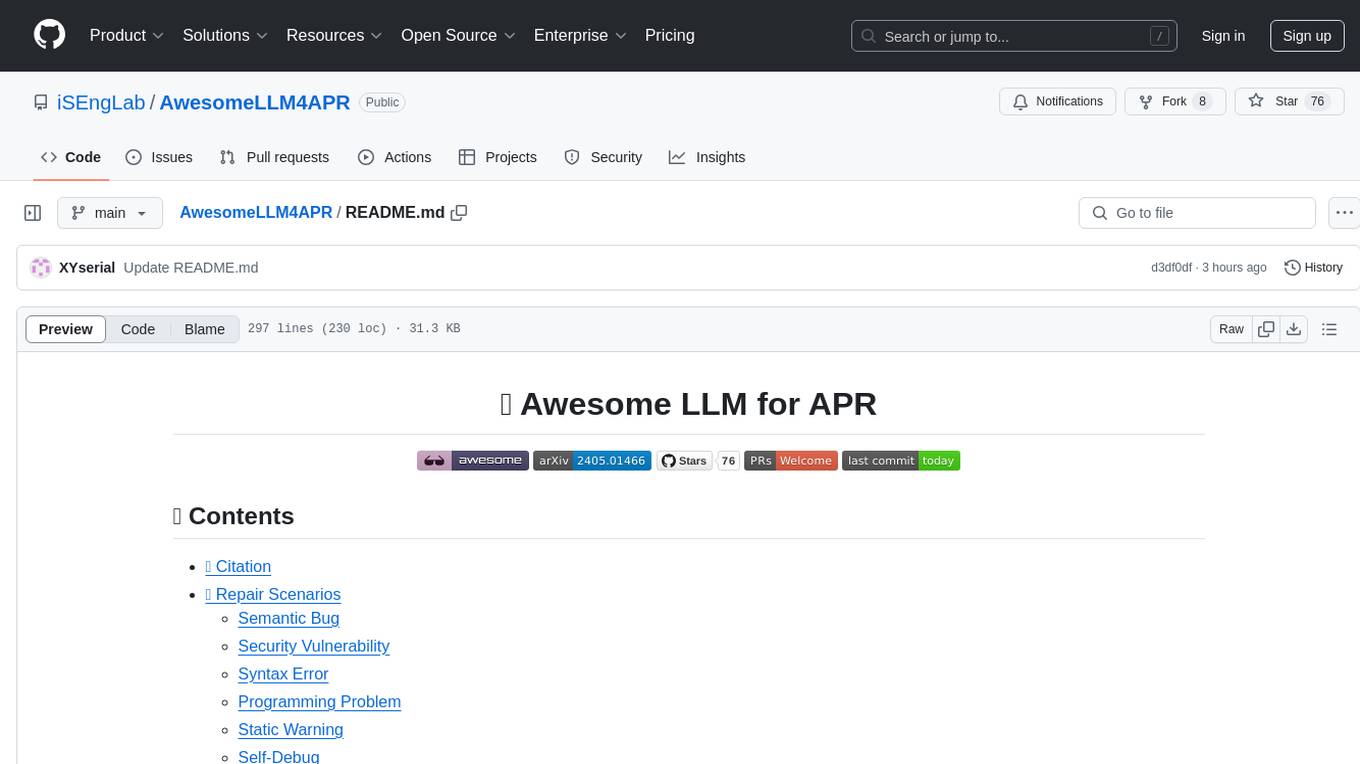
AwesomeLLM4APR
Awesome LLM for APR is a repository dedicated to exploring the capabilities of Large Language Models (LLMs) in Automated Program Repair (APR). It provides a comprehensive collection of research papers, tools, and resources related to using LLMs for various scenarios such as repairing semantic bugs, security vulnerabilities, syntax errors, programming problems, static warnings, self-debugging, type errors, web UI tests, smart contracts, hardware bugs, performance bugs, API misuses, crash bugs, test case repairs, formal proofs, GitHub issues, code reviews, motion planners, human studies, and patch correctness assessments. The repository serves as a valuable reference for researchers and practitioners interested in leveraging LLMs for automated program repair.
For similar jobs
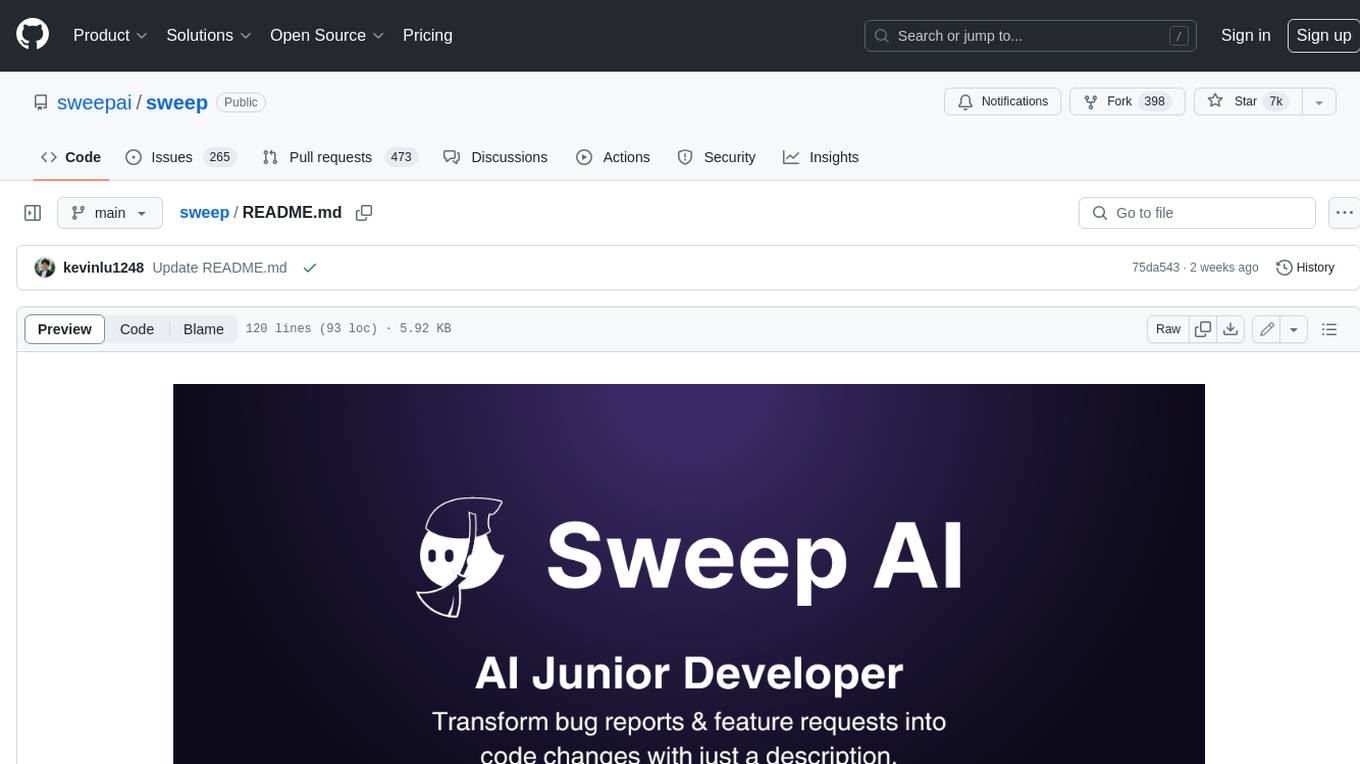
sweep
Sweep is an AI junior developer that turns bugs and feature requests into code changes. It automatically handles developer experience improvements like adding type hints and improving test coverage.
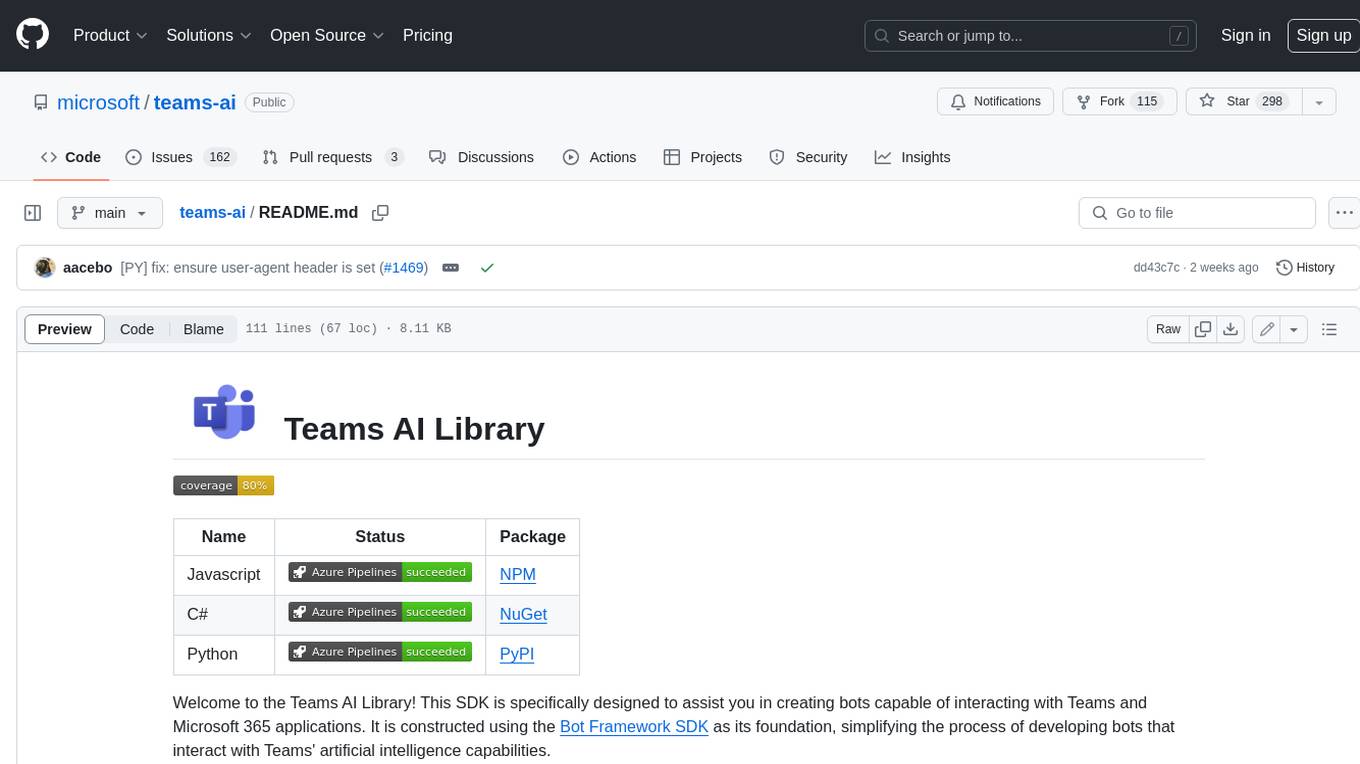
teams-ai
The Teams AI Library is a software development kit (SDK) that helps developers create bots that can interact with Teams and Microsoft 365 applications. It is built on top of the Bot Framework SDK and simplifies the process of developing bots that interact with Teams' artificial intelligence capabilities. The SDK is available for JavaScript/TypeScript, .NET, and Python.
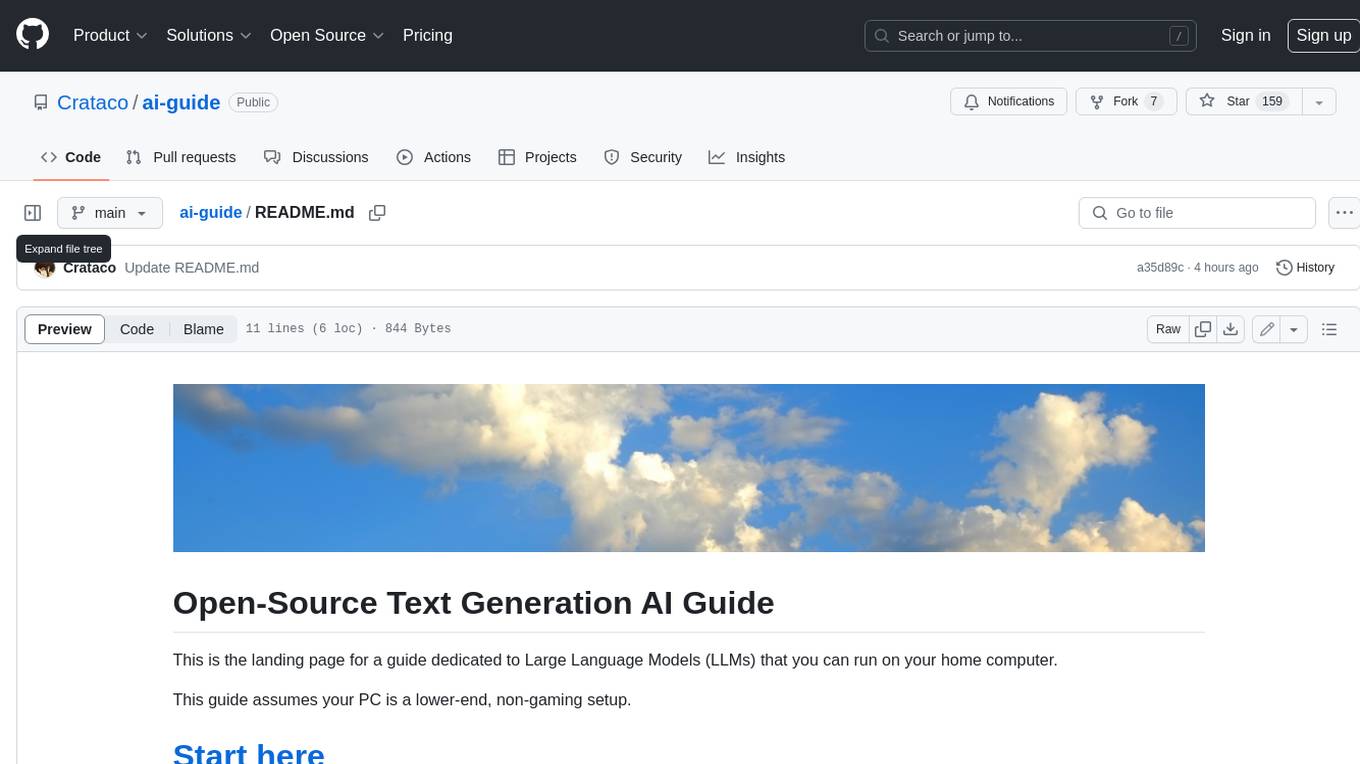
ai-guide
This guide is dedicated to Large Language Models (LLMs) that you can run on your home computer. It assumes your PC is a lower-end, non-gaming setup.
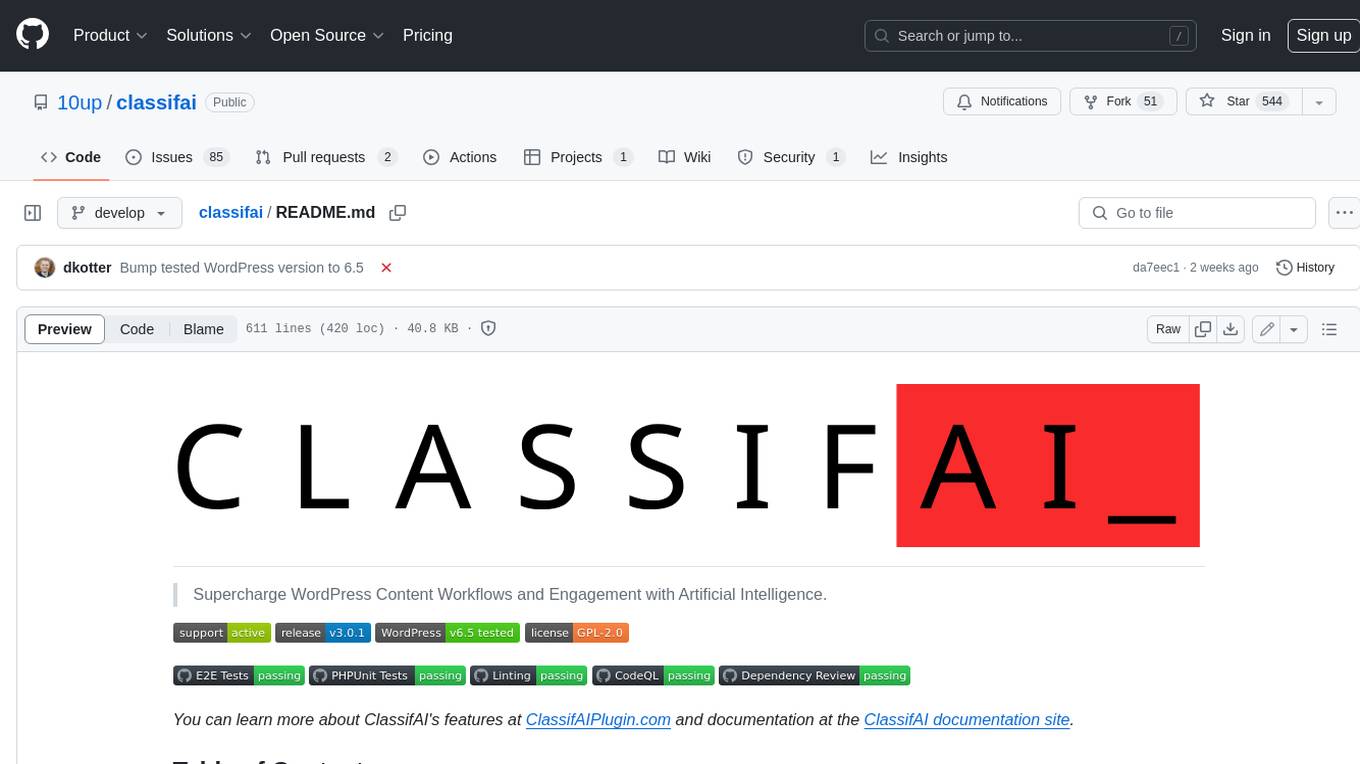
classifai
Supercharge WordPress Content Workflows and Engagement with Artificial Intelligence. Tap into leading cloud-based services like OpenAI, Microsoft Azure AI, Google Gemini and IBM Watson to augment your WordPress-powered websites. Publish content faster while improving SEO performance and increasing audience engagement. ClassifAI integrates Artificial Intelligence and Machine Learning technologies to lighten your workload and eliminate tedious tasks, giving you more time to create original content that matters.
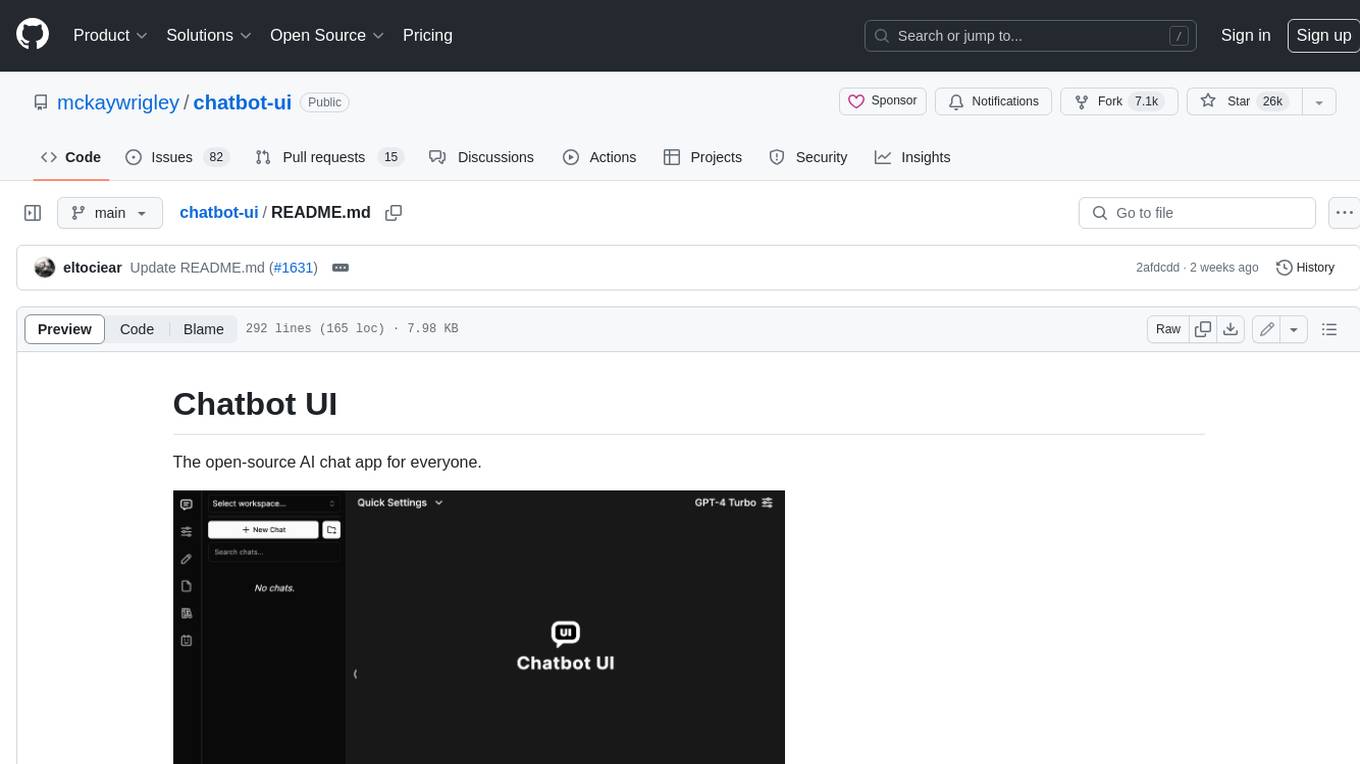
chatbot-ui
Chatbot UI is an open-source AI chat app that allows users to create and deploy their own AI chatbots. It is easy to use and can be customized to fit any need. Chatbot UI is perfect for businesses, developers, and anyone who wants to create a chatbot.
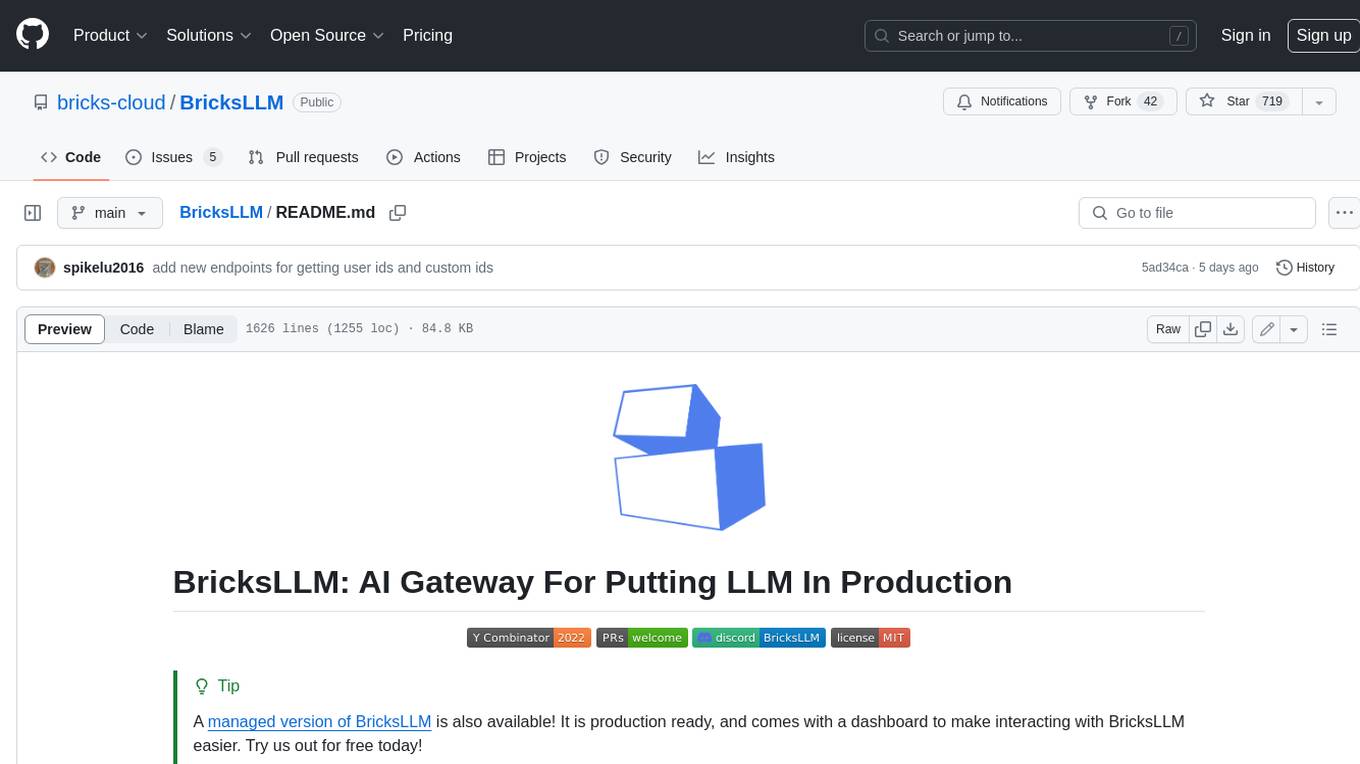
BricksLLM
BricksLLM is a cloud native AI gateway written in Go. Currently, it provides native support for OpenAI, Anthropic, Azure OpenAI and vLLM. BricksLLM aims to provide enterprise level infrastructure that can power any LLM production use cases. Here are some use cases for BricksLLM: * Set LLM usage limits for users on different pricing tiers * Track LLM usage on a per user and per organization basis * Block or redact requests containing PIIs * Improve LLM reliability with failovers, retries and caching * Distribute API keys with rate limits and cost limits for internal development/production use cases * Distribute API keys with rate limits and cost limits for students
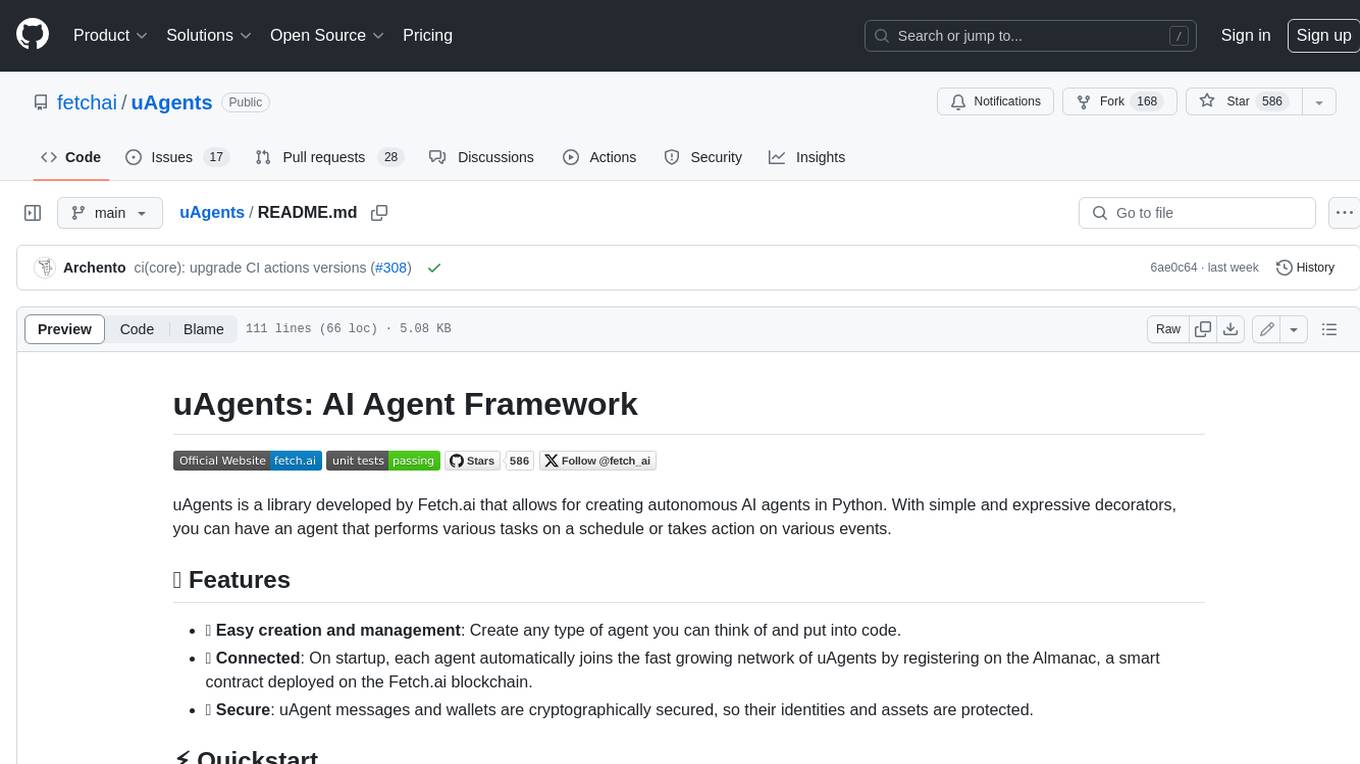
uAgents
uAgents is a Python library developed by Fetch.ai that allows for the creation of autonomous AI agents. These agents can perform various tasks on a schedule or take action on various events. uAgents are easy to create and manage, and they are connected to a fast-growing network of other uAgents. They are also secure, with cryptographically secured messages and wallets.
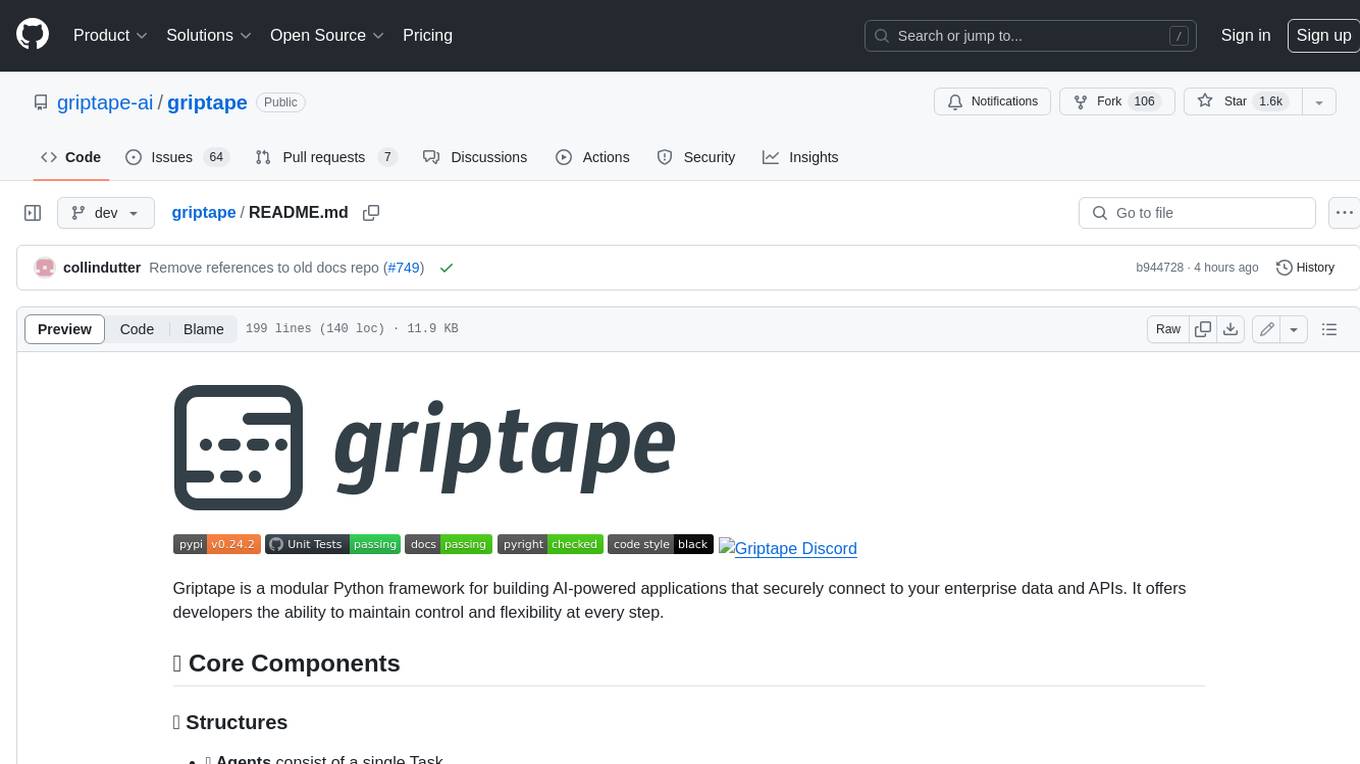
griptape
Griptape is a modular Python framework for building AI-powered applications that securely connect to your enterprise data and APIs. It offers developers the ability to maintain control and flexibility at every step. Griptape's core components include Structures (Agents, Pipelines, and Workflows), Tasks, Tools, Memory (Conversation Memory, Task Memory, and Meta Memory), Drivers (Prompt and Embedding Drivers, Vector Store Drivers, Image Generation Drivers, Image Query Drivers, SQL Drivers, Web Scraper Drivers, and Conversation Memory Drivers), Engines (Query Engines, Extraction Engines, Summary Engines, Image Generation Engines, and Image Query Engines), and additional components (Rulesets, Loaders, Artifacts, Chunkers, and Tokenizers). Griptape enables developers to create AI-powered applications with ease and efficiency.