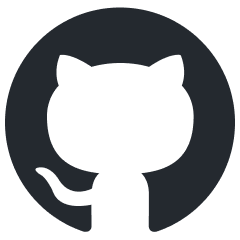
CogAgent
An open-sourced end-to-end VLM-based GUI Agent
Stars: 113
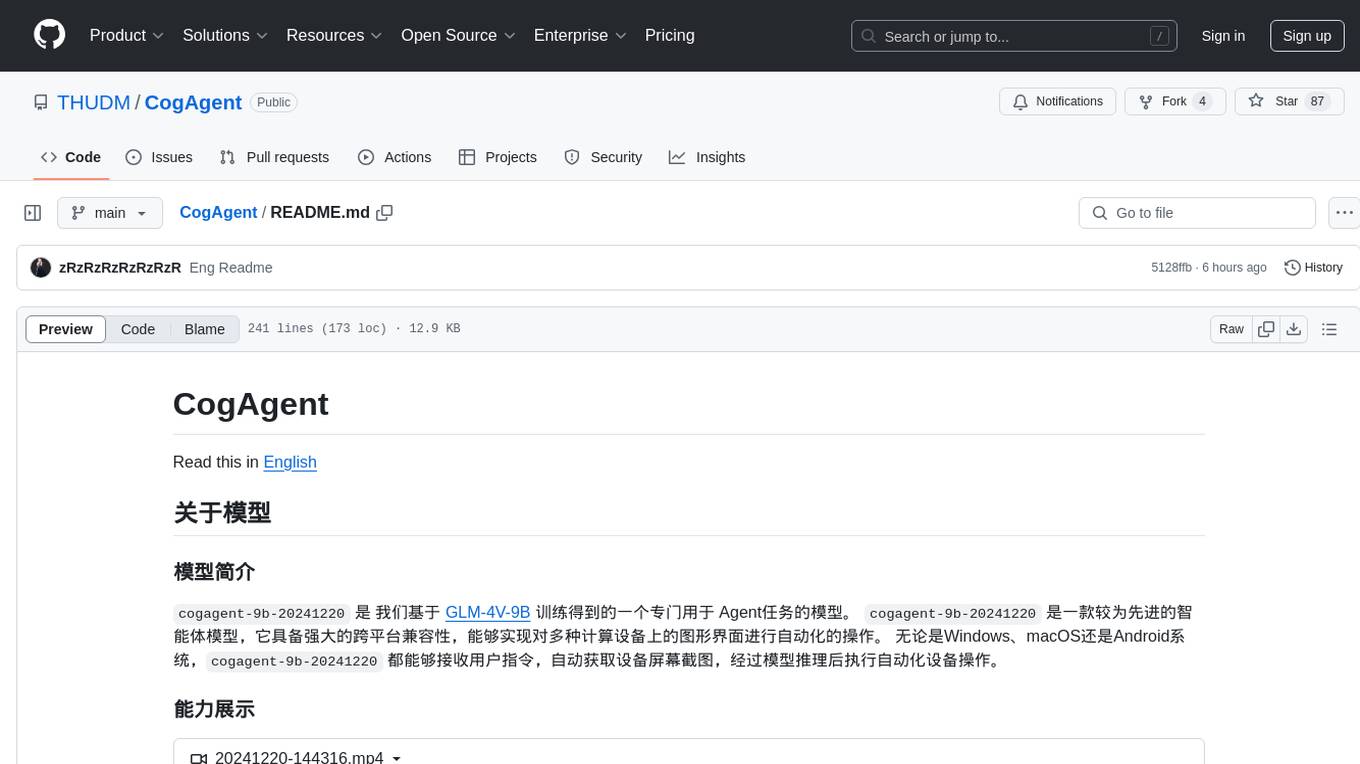
CogAgent is an advanced intelligent agent model designed for automating operations on graphical interfaces across various computing devices. It supports platforms like Windows, macOS, and Android, enabling users to issue commands, capture device screenshots, and perform automated operations. The model requires a minimum of 29GB of GPU memory for inference at BF16 precision and offers capabilities for executing tasks like sending Christmas greetings and sending emails. Users can interact with the model by providing task descriptions, platform specifications, and desired output formats.
README:
-
🔥 December 2024: We open-sourced the latest version of the CogAgent-9B-20241220 model. Compared to the previous version of CogAgent,
CogAgent-9B-20241220
features significant improvements in GUI perception, reasoning accuracy, action space completeness, task universality, and generalization. It supports bilingual (Chinese and English) interaction through both screen captures and natural language. -
🏆 June 2024: CogAgent was accepted by CVPR 2024 and recognized as a conference Highlight (top 3%).
-
December 2023: We open-sourced the first GUI Agent: CogAgent (with the former repository available here) and published the corresponding paper: 📖 CogAgent Paper.
Model | Model Download Links | Technical Documentation | Online Demo |
---|---|---|---|
cogagent-9b-20241220 |
🤗 HuggingFace 🤖 ModelScope 🟣 WiseModel 🧩 Modelers (Ascend) |
📄 Official Technical Blog 📘 Practical Guide (Chinese) |
🤗 HuggingFace Space 🧩 Modelers Space (Ascend) |
CogAgent-9B-20241220
model is based on GLM-4V-9B, a bilingual open-source
VLM base model. Through data collection and optimization, multi-stage training, and strategy improvements,
CogAgent-9B-20241220
achieves significant advancements in GUI perception, inference prediction accuracy, action space
completeness, and generalizability across tasks. The model supports bilingual (Chinese and English) interaction with
both screenshots and language input. This version of the CogAgent model has already been applied in
ZhipuAI's GLM-PC product. We hope the release of this model can assist researchers
and developers in advancing the research and applications of GUI agents based on vision-language models.
The CogAgent-9b-20241220 model has achieved state-of-the-art results across multiple platforms and categories in GUI Agent tasks and GUI Grounding Benchmarks. In the CogAgent-9b-20241220 Technical Blog, we compared it against API-based commercial models (GPT-4o-20240806, Claude-3.5-Sonnet), commercial API + GUI Grounding models (GPT-4o + UGround, GPT-4o + OS-ATLAS), and open-source GUI Agent models (Qwen2-VL, ShowUI, SeeClick). The results demonstrate that CogAgent leads in GUI localization (Screenspot), single-step operations (OmniAct), the Chinese step-wise in-house benchmark (CogAgentBench-basic-cn), and multi-step operations (OSWorld), with only a slight disadvantage in OSWorld compared to Claude-3.5-Sonnet, which specializes in Computer Use, and GPT-4o combined with external GUI Grounding models.
CogAgent wishes you a Merry Christmas! Let the large model automatically send Christmas greetings to your friends.
Want to open an issue? Let CogAgent help you send an email.
Table of Contents
- CogAgent
- At
BF16
precision, the model requires at least29GB
of GPU memory for inference. UsingINT4
precision is * not* recommended due to significant performance loss. - All GPU references above refer to A100 or H100 GPUs. For other devices, you need to calculate the required GPU/CPU memory accordingly.
- During SFT (Supervised Fine-Tuning), this codebase freezes the
Vision Encoder
, uses a batch size of 1, and trains on8 * A100
GPUs. The total input tokens (including images, which account for1600
tokens) add up to 2048 tokens. This codebase cannot conduct SFT fine-tuning without freezing theVision Encoder
.
For LoRA fine-tuning,Vision Encoder
is not frozen; the batch size is 1, using1 * A100
GPU. The total input tokens (including images,1600
tokens) also amount to 2048 tokens. In the above setup, SFT fine-tuning requires at least60GB
of GPU memory per GPU (with 8 GPUs), while LoRA fine-tuning requires at least70GB
of GPU memory on a single GPU (cannot be split). -
Ascend devices
have not been tested for SFT fine-tuning. We have only tested them on theAtlas800
training server cluster. You need to modify the inference code accordingly based on the loading mechanism described in theAscend device
download link. - Currently, we do not support inference with the
vLLM
framework. We will submit a PR as soon as possible to enable it. - The online demo link does not support controlling computers; it only allows you to view the model's inference results. We recommend deploying the model locally.
cogagent-9b-20241220
is an agent-type execution model rather than a conversational model. It does not support
continuous dialogue, but it does support a continuous execution history. (In other words, each time a new
conversation session needs to be started, and the past history should be provided to the model.) The workflow of
CogAgent is illustrated as following:
To achieve optimal GUI Agent performance, we have adopted a strict input-output format. Below is how users should format their inputs and feed them to the model, and how to interpret the model’s responses.
You can refer to app/client.py#L115 for constructing user input prompts. A minimal example of user input concatenation code is shown below:
current_platform = identify_os() # "Mac" or "WIN" or "Mobile". Pay attention to case sensitivity.
platform_str = f"(Platform: {current_platform})\n"
format_str = "(Answer in Action-Operation-Sensitive format.)\n" # You can use other format to replace "Action-Operation-Sensitive"
history_str = "\nHistory steps: "
for index, (grounded_op_func, action) in enumerate(zip(history_grounded_op_funcs, history_actions)):
history_str += f"\n{index}. {grounded_op_func}\t{action}" # start from 0.
query = f"Task: {task}{history_str}\n{platform_str}{format_str}" # Be careful about the \n
The concatenated Python string:
"Task: Search for doors, click doors on sale and filter by brands \"Mastercraft\".\nHistory steps: \n0. CLICK(box=[[352,102,786,139]], element_info='Search')\tLeft click on the search box located in the middle top of the screen next to the Menards logo.\n1. TYPE(box=[[352,102,786,139]], text='doors', element_info='Search')\tIn the search input box at the top, type 'doors'.\n2. CLICK(box=[[787,102,809,139]], element_info='SEARCH')\tLeft click on the magnifying glass icon next to the search bar to perform the search.\n3. SCROLL_DOWN(box=[[0,209,998,952]], step_count=5, element_info='[None]')\tScroll down the page to see the available doors.\n4. CLICK(box=[[280,708,710,809]], element_info='Doors on Sale')\tClick the \"Doors On Sale\" button in the middle of the page to view the doors that are currently on sale.\n(Platform: WIN)\n(Answer in Action-Operation format.)\n"
Printed prompt:
Task: Search for doors, click doors on sale and filter by brands "Mastercraft".
History steps:
- CLICK(box=[[352,102,786,139]], element_info='Search') Left click on the search box located in the middle top of the screen next to the Menards logo.
- TYPE(box=[[352,102,786,139]], text='doors', element_info='Search') In the search input box at the top, type ' doors'.
- CLICK(box=[[787,102,809,139]], element_info='SEARCH') Left click on the magnifying glass icon next to the search bar to perform the search.
- SCROLL_DOWN(box=[[0,209,998,952]], step_count=5, element_info='[None]') Scroll down the page to see the available doors.
- CLICK(box=[[280,708,710,809]], element_info='Doors on Sale') Click the "Doors On Sale" button in the middle of the page to view the doors that are currently on sale.
(Platform: WIN)
(Answer in Action-Operation format.)
If you want to understand the meaning and representation of each field in detail, please continue reading or refer to the Practical Documentation (in Chinese), "Prompt Concatenation" section.
-
task
field
The user’s task description, in text format similar to a prompt. This input instructs thecogagent-9b-20241220
model on how to carry out the user’s request. Keep it concise and clear. -
platform
field
cogagent-9b-20241220
supports agent operations on multiple platforms with graphical interfaces. We currently support three systems:- Windows 10, 11: Use the
WIN
field. - macOS 14, 15: Use the
Mac
field. - Android 13, 14, 15 (and other Android UI variants with similar GUI operations): Use the
Mobile
field.
If your system is not among these, the effectiveness may be suboptimal. You can try using
Mobile
for mobile devices,WIN
for Windows, orMac
for Mac. - Windows 10, 11: Use the
-
format
field
The format in which the user wantscogagent-9b-20241220
to return data. We provide several options:-
Answer in Action-Operation-Sensitive format.
: The default demo return type in this repo. Returns the model’s actions, corresponding operations, and the sensitivity level. -
Answer in Status-Plan-Action-Operation format.
: Returns the model’s status, plan, and corresponding operations. -
Answer in Status-Action-Operation-Sensitive format.
: Returns the model’s status, actions, corresponding operations, and sensitivity. -
Answer in Status-Action-Operation format.
: Returns the model’s status and actions. -
Answer in Action-Operation format.
: Returns the model’s actions and corresponding operations.
-
-
history
field
This should be concatenated in the following order:query = f'{task}{history}{platform}{format}'
-
Sensitive operations: Includes
<<敏感操作>>
(“sensitive operation”) and<<一般操作>>
(“general operation”). These are only returned if you request theSensitive
format. -
Plan
,Status
,Action
fields: Used to describe the model’s behavior and operations. Only returned if you request the corresponding fields. For example, if the format includesAction
, then the model returns theAction
field. - General answer section: A summary that appears prior to the formatted answer.
-
Grounded Operation
field:
Describes the model’s specific operations, including the location of the operation, the operation type, and the action details. Thebox
attribute indicates the coordinate region for execution,element_type
indicates the element type, andelement_info
describes the element. These details are wrapped within a “操作指令” (operation command). For the definition of the action space, please refer to here.
Suppose the user wants to mark all emails as read. The user is on a Mac, and the user wants the model to return in
Action-Operation-Sensitive
format. The properly concatenated prompt should be:
Task: Please mark all my emails as read
History steps:
(Platform: Mac)
(Answer in Action-Operation-Sensitive format.)
Note: even if there are no historical actions, "History steps:" still needs to be appended in the prompt. Below are sample outputs for different format requirements:
Answer in Action-Operation-Sensitive format
Action: Click the 'Mark all as read' button in the top toolbar of the page to mark all emails as read.
Grounded Operation: CLICK(box=[[219,186,311,207]], element_type='Clickable text', element_info='Mark all emails as read')
<<一般操作>>
Answer in Status-Plan-Action-Operation format
Status: Currently in the email interface [[0, 2, 998, 905]], with the email categories on the left [[1, 216, 144, 570]], and the inbox in the center [[144, 216, 998, 903]]. The "Mark all as read" button has been clicked [[223, 178, 311, 210]].
Plan: Future tasks: 1. Click the 'Mark all as read' button; 2. Task complete.
Action: Click the "Mark all as read" button at the top center of the inbox page to mark all emails as read.
Grounded Operation: CLICK(box=[[219,186,311,207]], element_type='Clickable text', element_info='Mark all emails as read')
Answer in Status-Action-Operation-Sensitive format
Status: Currently in the email interface [[0, 2, 998, 905]], with the email categories on the left [[1, 216, 144, 570]], and the inbox in the center [[144, 216, 998, 903]]. The "Mark all as read" button has been clicked [[223, 178, 311, 210]].
Action: Click the "Mark all as read" button at the top center of the inbox page to mark all emails as read.
Grounded Operation: CLICK(box=[[219,186,311,207]], element_type='Clickable text', element_info='Mark all emails as read')
<<一般操作>>
Answer in Status-Action-Operation format
Status: Currently in the email interface [[0, 2, 998, 905]], with the email categories on the left [[1, 216, 144, 570]], and the inbox in the center [[144, 216, 998, 903]]. The "Mark all as read" button has been clicked [[223, 178, 311, 210]].
Action: Click the "Mark all as read" button at the top center of the inbox page to mark all emails as read.
Grounded Operation: CLICK(box=[[219,186,311,207]], element_type='Clickable text', element_info='Mark all emails as read')
Answer in Action-Operation format
Action: Right-click the first email in the left email list to open the action menu.
Grounded Operation: RIGHT_CLICK(box=[[154,275,343,341]], element_info='[AXCell]')
- This model is not a conversational model and does not support continuous dialogue. Please send specific commands and reference our recommended method for concatenating the history.
- The model requires images as input; pure text conversation cannot achieve GUI Agent tasks.
- The model’s output adheres to a strict format. Please parse it strictly according to our requirements. The output is in string format; JSON output is not supported.
Make sure you have installed Python 3.10.16 or above, and then install the following dependencies:
pip install -r requirements.txt
To run local inference based on transformers
, you can run the command below:
python inference/cli_demo.py --model_dir THUDM/cogagent-9b-20241220 --platform "Mac" --max_length 4096 --top_k 1 --output_image_path ./results --format_key status_action_op_sensitive
This is a command-line interactive code. You will need to provide the path to your images. If the model returns results
containing bounding boxes, it will output an image with those bounding boxes, indicating the region where the operation
should be executed. The image is saved to output_image_path
, with the file name {your_input_image_name}_{round}.png
.
The format_key
indicates in which format you want the model to respond. The platform
field specifies which platform
you are using (e.g., Mac
). Therefore, all uploaded screenshots must be from macOS if platform
is set to Mac
.
If you want to run an online web demo, which supports continuous image uploads for interactive inference, you can run:
python inference/web_demo.py --host 0.0.0.0 --port 7860 --model_dir THUDM/cogagent-9b-20241220 --format_key status_action_op_sensitive --platform "Mac" --output_dir ./results
This code provides the same experience as the HuggingFace Space
online demo. The model will return the corresponding
bounding boxes and execution categories.
We have prepared a basic demo app for developers to illustrate the GUI capabilities of cogagent-9b-20241220
. The demo
shows how to deploy the model on a GPU-equipped server and run the cogagent-9b-20241220
model locally to perform
automated GUI operations.
We cannot guarantee the safety of AI behavior; please exercise caution when using it.
This example is only for academic reference. We assume no legal responsibility for any issues resulting from this example.
If you are interested in this APP, feel free to check out the documentation.
If you are interested in fine-tuning the cogagent-9b-20241220
model, please refer to here.
In November 2023, we released the first generation of CogAgent. You can find related code and model weights in the CogVLM & CogAgent Official Repository.
📖 Paper: CogVLM: Visual Expert for Pretrained Language Models CogVLM is a powerful open-source Vision-Language Model (VLM). CogVLM-17B has 10B visual parameters and 7B language parameters, supporting image understanding at a resolution of 490x490, as well as multi-round dialogue. CogVLM-17B achieves state-of-the-art performance on 10 classic multimodal benchmarks, including NoCaps, Flicker30k captioning, RefCOCO, RefCOCO+, RefCOCOg, Visual7W, GQA, ScienceQA, VizWiz VQA, and TDIUC. |
📖 Paper: CogAgent: A Visual Language Model for GUI Agents CogAgent is an open-source vision-language model improved upon CogVLM. CogAgent-18B has 11B visual parameters and 7B language parameters. It supports image understanding at a resolution of 1120x1120. Building on CogVLM’s capabilities, CogAgent further incorporates a GUI image agent ability. CogAgent-18B delivers state-of-the-art general performance on 9 classic vision-language benchmarks, including VQAv2, OK-VQ, TextVQA, ST-VQA, ChartQA, infoVQA, DocVQA, MM-Vet, and POPE. It also significantly outperforms existing models on GUI operation datasets such as AITW and Mind2Web. |
- The Apache2.0 LICENSE applies to the use of the code in this GitHub repository.
- For the model weights, please follow the Model License.
If you find our work helpful, please consider citing the following papers
@misc{hong2023cogagent,
title={CogAgent: A Visual Language Model for GUI Agents},
author={Wenyi Hong and Weihan Wang and Qingsong Lv and Jiazheng Xu and Wenmeng Yu and Junhui Ji and Yan Wang and Zihan Wang and Yuxiao Dong and Ming Ding and Jie Tang},
year={2023},
eprint={2312.08914},
archivePrefix={arXiv},
primaryClass={cs.CV}
}
R&D Institutions: Tsinghua University, Zhipu AI
Team members: Wenyi Hong, Junhui Ji, Lihang Pan, Yuanchang Yue, Changyu Pang, Siyan Xue, Guo Wang, Weihan Wang, Jiazheng Xu, Shen Yang, Xiaotao Gu, Yuxiao Dong, Jie Tang
Acknowledgement: We would like to thank the Zhipu AI data team for their strong support, including Xiaohan Zhang, Zhao Xue, Lu Chen, Jingjie Du, Siyu Wang, Ying Zhang, and all annotators. They worked hard to collect and annotate the training and testing data of the CogAgent model. We also thank Yuxuan Zhang, Xiaowei Hu, and Hao Chen from the Zhipu AI open source team for their engineering efforts in open sourcing the model.
For Tasks:
Click tags to check more tools for each tasksFor Jobs:
Alternative AI tools for CogAgent
Similar Open Source Tools
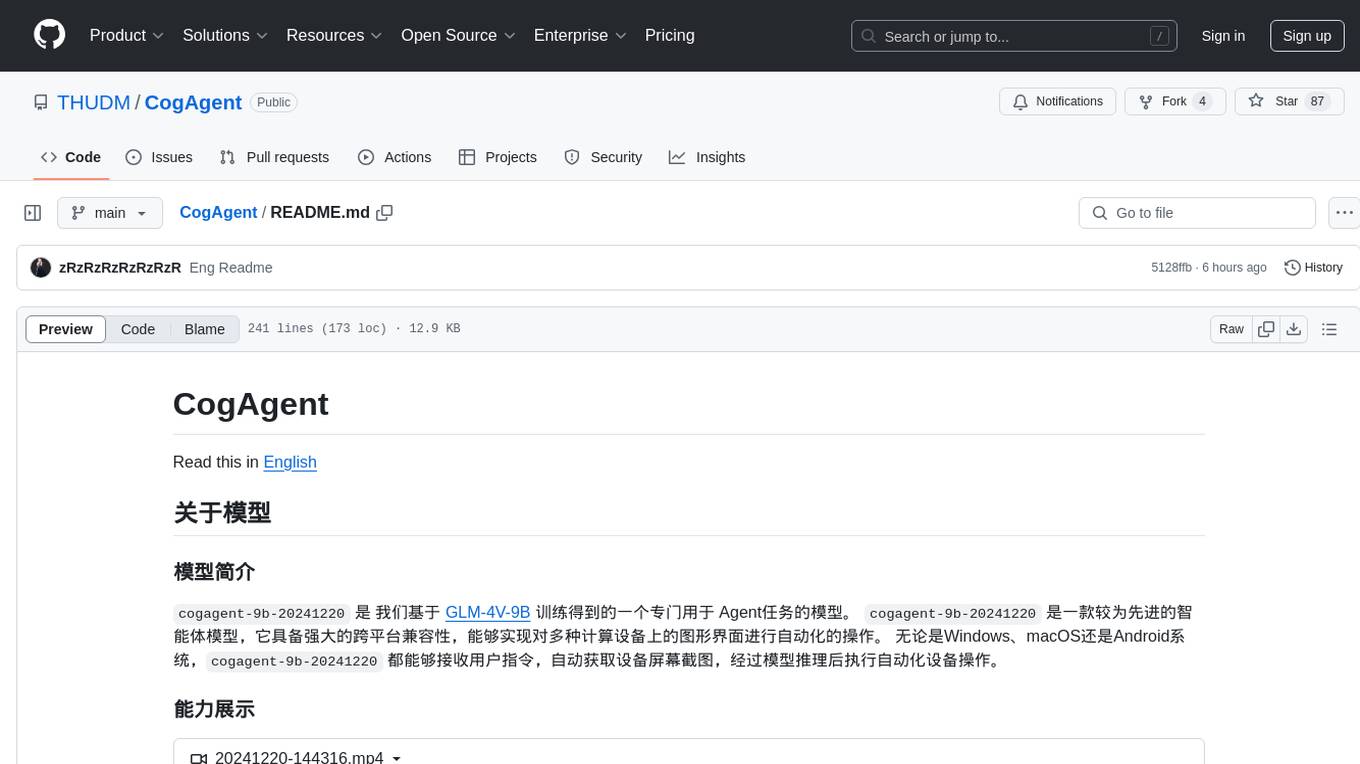
CogAgent
CogAgent is an advanced intelligent agent model designed for automating operations on graphical interfaces across various computing devices. It supports platforms like Windows, macOS, and Android, enabling users to issue commands, capture device screenshots, and perform automated operations. The model requires a minimum of 29GB of GPU memory for inference at BF16 precision and offers capabilities for executing tasks like sending Christmas greetings and sending emails. Users can interact with the model by providing task descriptions, platform specifications, and desired output formats.
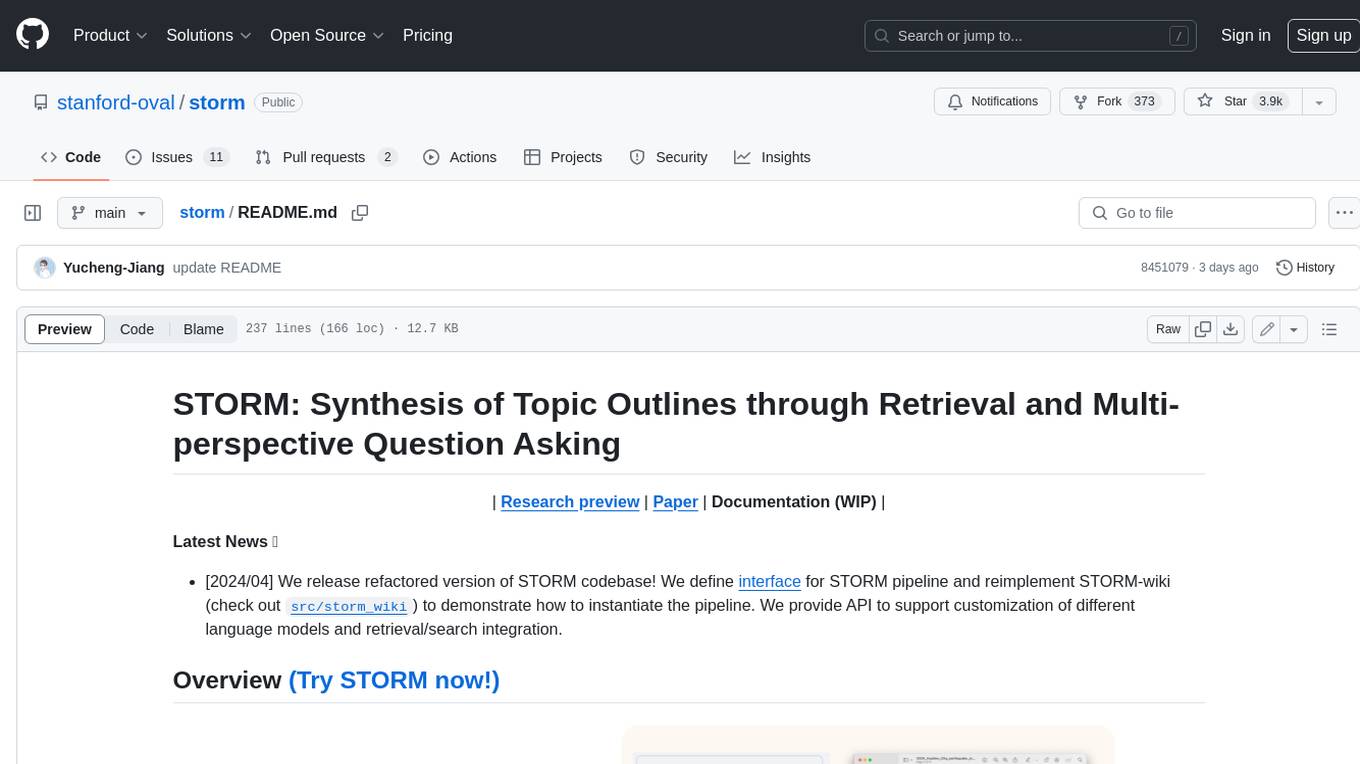
storm
STORM is a LLM system that writes Wikipedia-like articles from scratch based on Internet search. While the system cannot produce publication-ready articles that often require a significant number of edits, experienced Wikipedia editors have found it helpful in their pre-writing stage. **Try out our [live research preview](https://storm.genie.stanford.edu/) to see how STORM can help your knowledge exploration journey and please provide feedback to help us improve the system 🙏!**
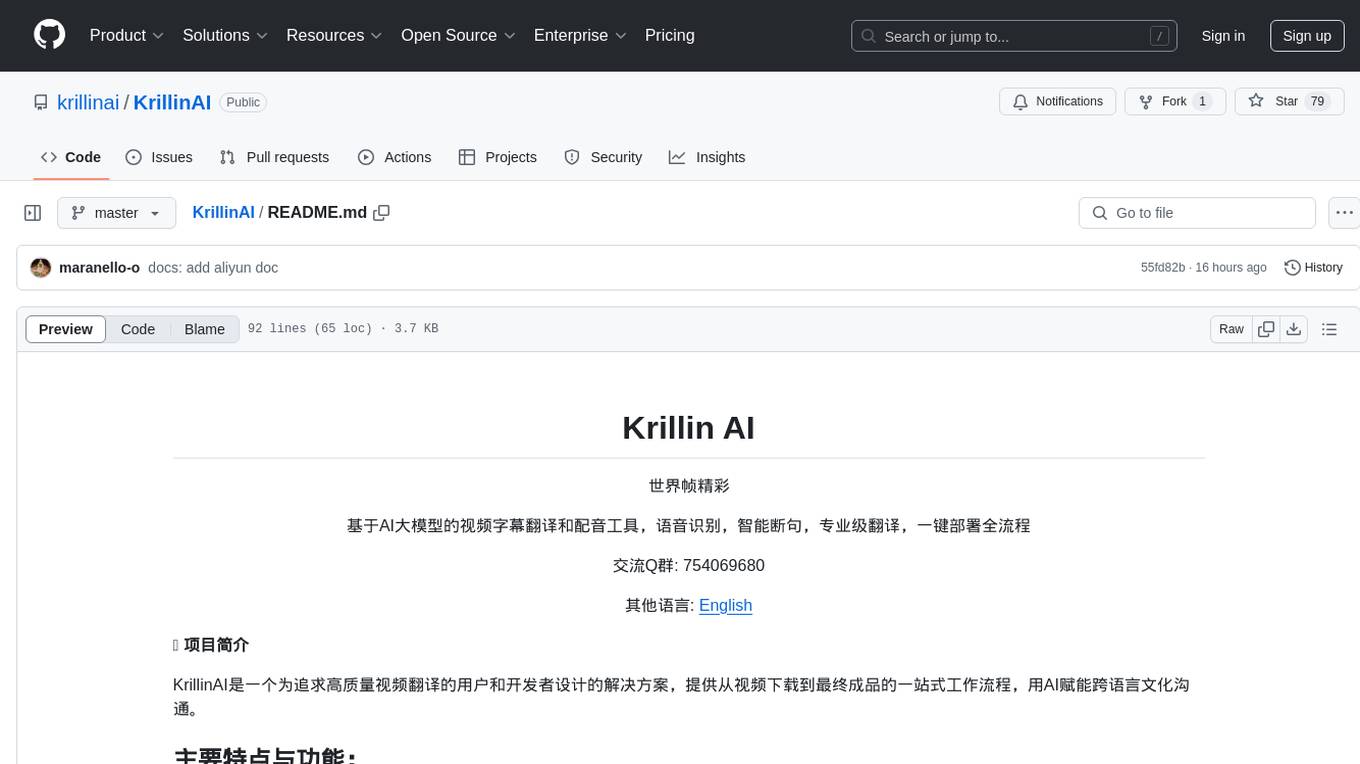
KrillinAI
KrillinAI is a video subtitle translation and dubbing tool based on AI large models, featuring speech recognition, intelligent sentence segmentation, professional translation, and one-click deployment of the entire process. It provides a one-stop workflow from video downloading to the final product, empowering cross-language cultural communication with AI. The tool supports multiple languages for input and translation, integrates features like automatic dependency installation, video downloading from platforms like YouTube and Bilibili, high-speed subtitle recognition, intelligent subtitle segmentation and alignment, custom vocabulary replacement, professional-level translation engine, and diverse external service selection for speech and large model services.
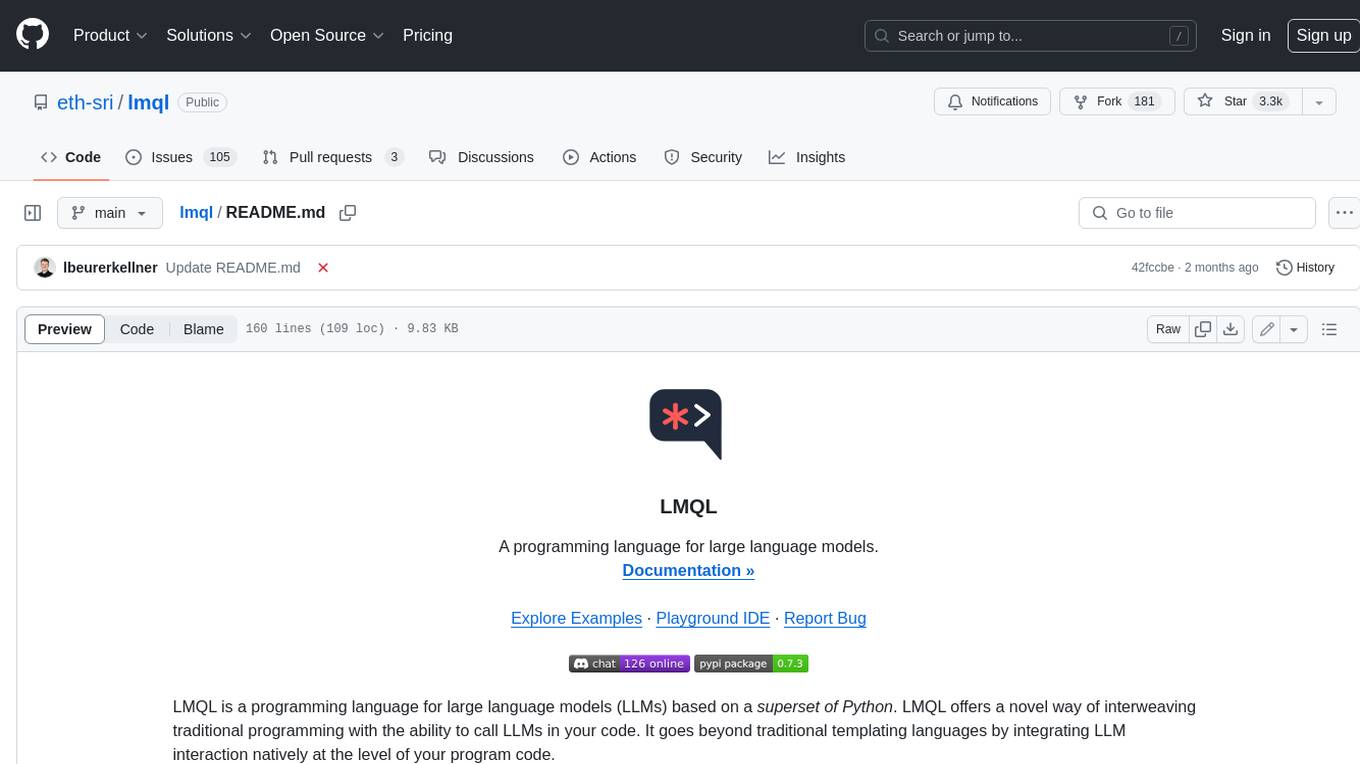
lmql
LMQL is a programming language designed for large language models (LLMs) that offers a unique way of integrating traditional programming with LLM interaction. It allows users to write programs that combine algorithmic logic with LLM calls, enabling model reasoning capabilities within the context of the program. LMQL provides features such as Python syntax integration, rich control-flow options, advanced decoding techniques, powerful constraints via logit masking, runtime optimization, sync and async API support, multi-model compatibility, and extensive applications like JSON decoding and interactive chat interfaces. The tool also offers library integration, flexible tooling, and output streaming options for easy model output handling.
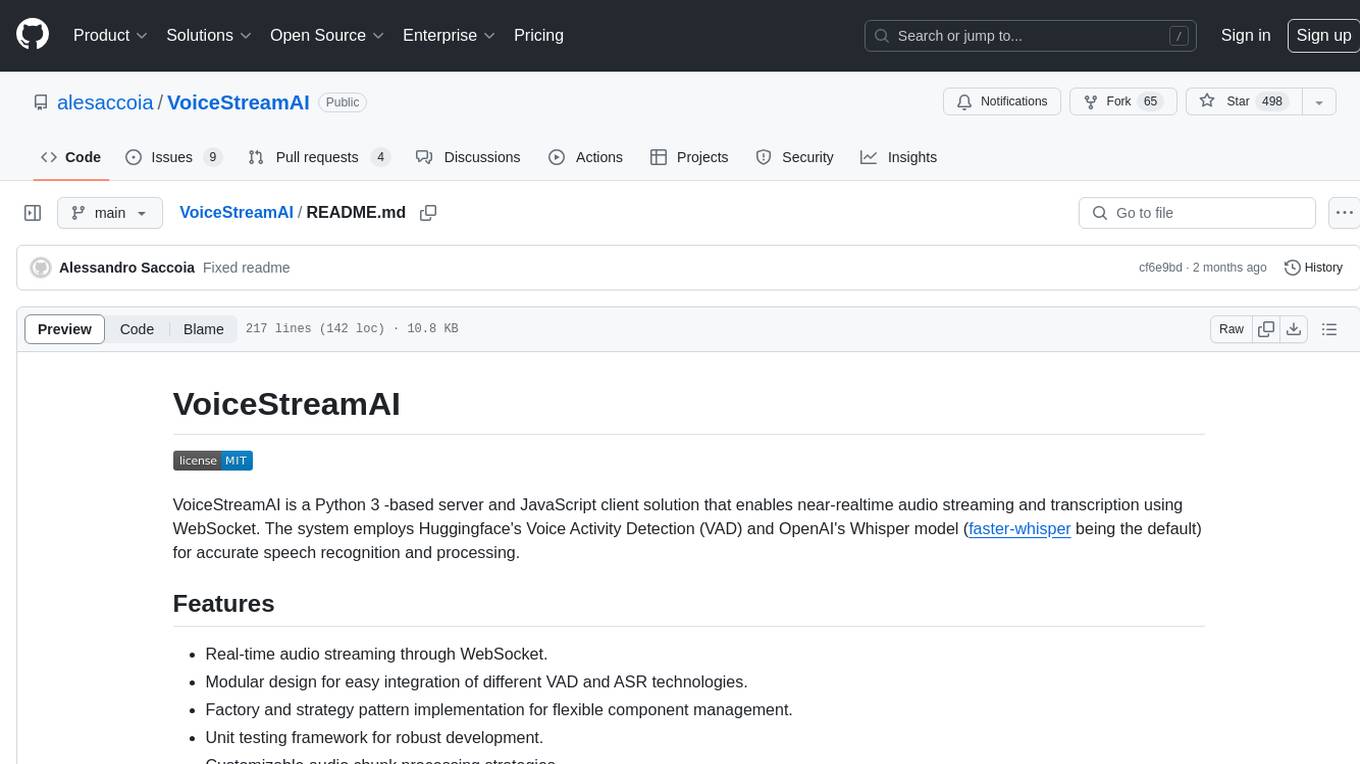
VoiceStreamAI
VoiceStreamAI is a Python 3-based server and JavaScript client solution for near-realtime audio streaming and transcription using WebSocket. It employs Huggingface's Voice Activity Detection (VAD) and OpenAI's Whisper model for accurate speech recognition. The system features real-time audio streaming, modular design for easy integration of VAD and ASR technologies, customizable audio chunk processing strategies, support for multilingual transcription, and secure sockets support. It uses a factory and strategy pattern implementation for flexible component management and provides a unit testing framework for robust development.
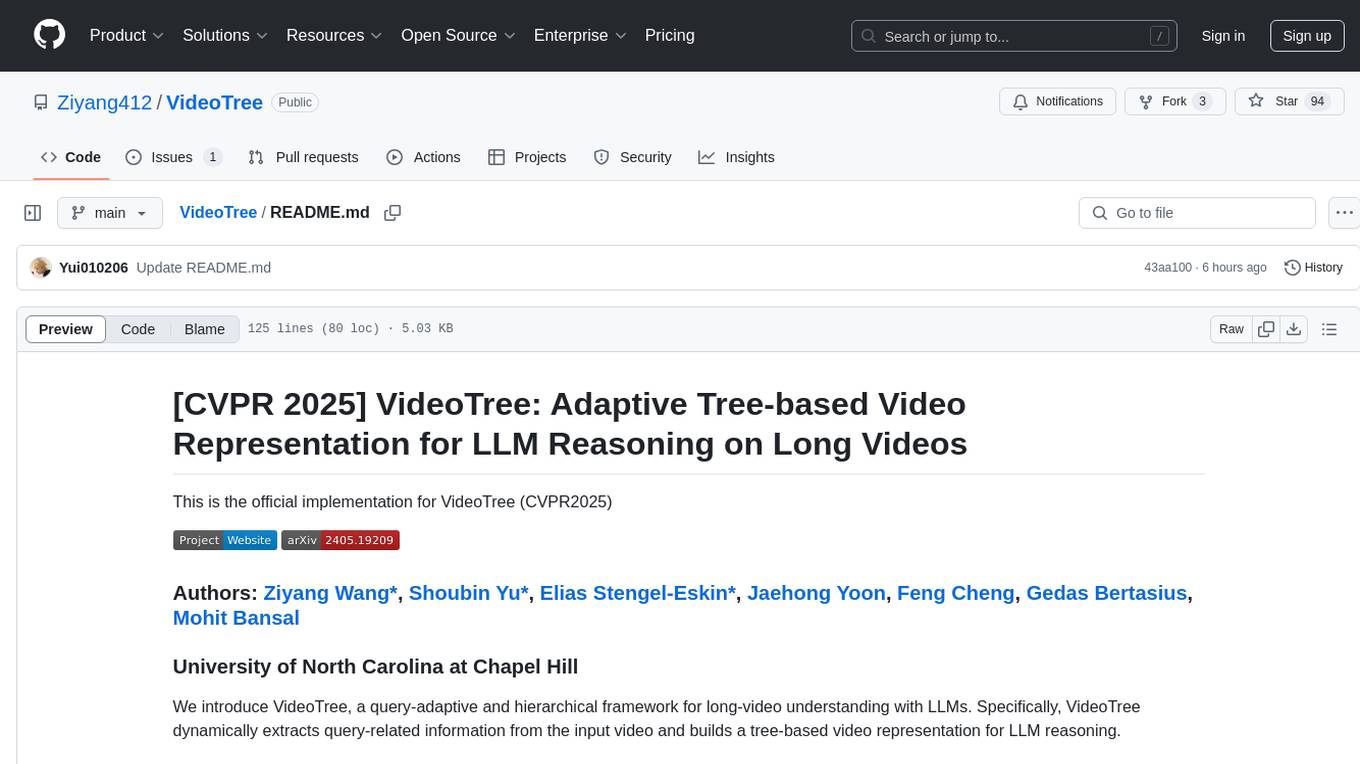
VideoTree
VideoTree is an official implementation for a query-adaptive and hierarchical framework for understanding long videos with LLMs. It dynamically extracts query-related information from input videos and builds a tree-based video representation for LLM reasoning. The tool requires Python 3.8 or above and leverages models like LaViLa and EVA-CLIP-8B for feature extraction. It also provides scripts for tasks like Adaptive Breath Expansion, Relevance-based Depth Expansion, and LLM Reasoning. The codebase is being updated to incorporate scripts/captions for NeXT-QA and IntentQA in the future.
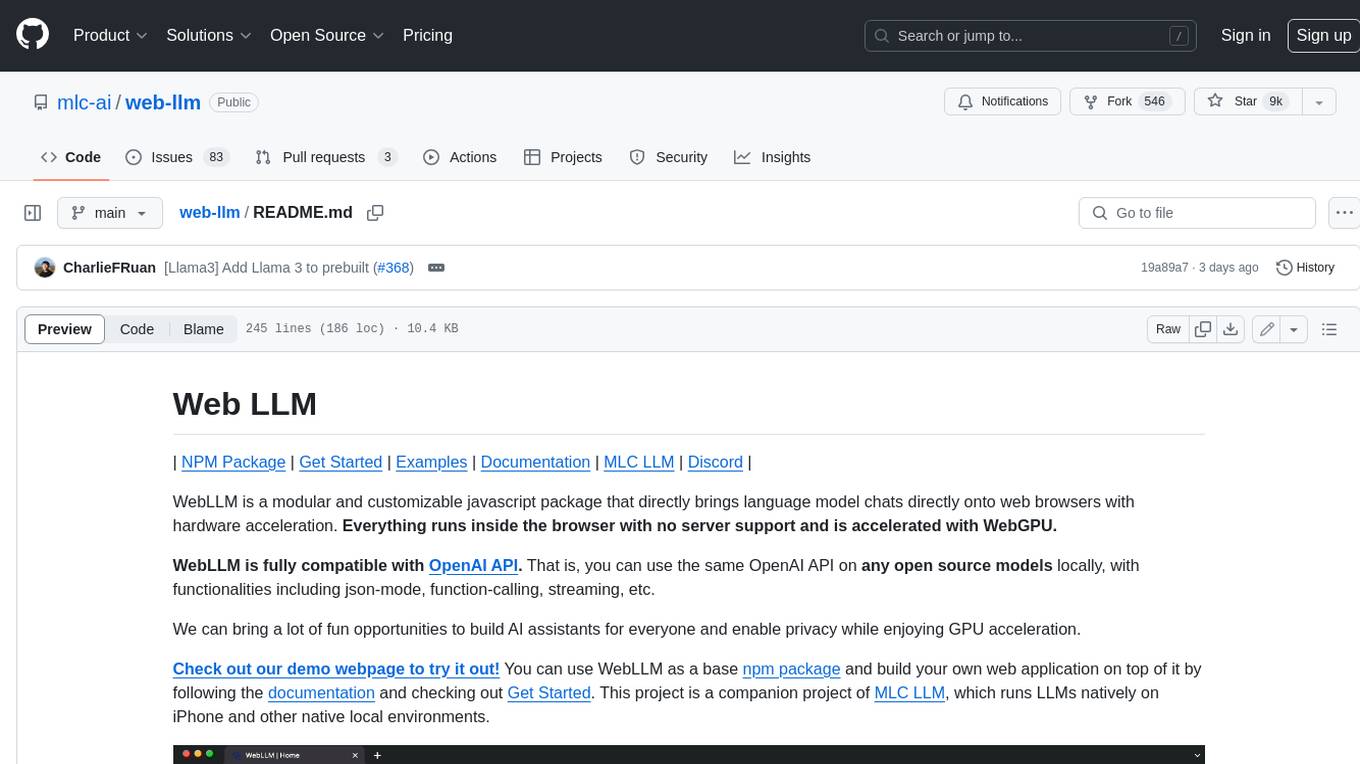
web-llm
WebLLM is a modular and customizable javascript package that directly brings language model chats directly onto web browsers with hardware acceleration. Everything runs inside the browser with no server support and is accelerated with WebGPU. WebLLM is fully compatible with OpenAI API. That is, you can use the same OpenAI API on any open source models locally, with functionalities including json-mode, function-calling, streaming, etc. We can bring a lot of fun opportunities to build AI assistants for everyone and enable privacy while enjoying GPU acceleration.
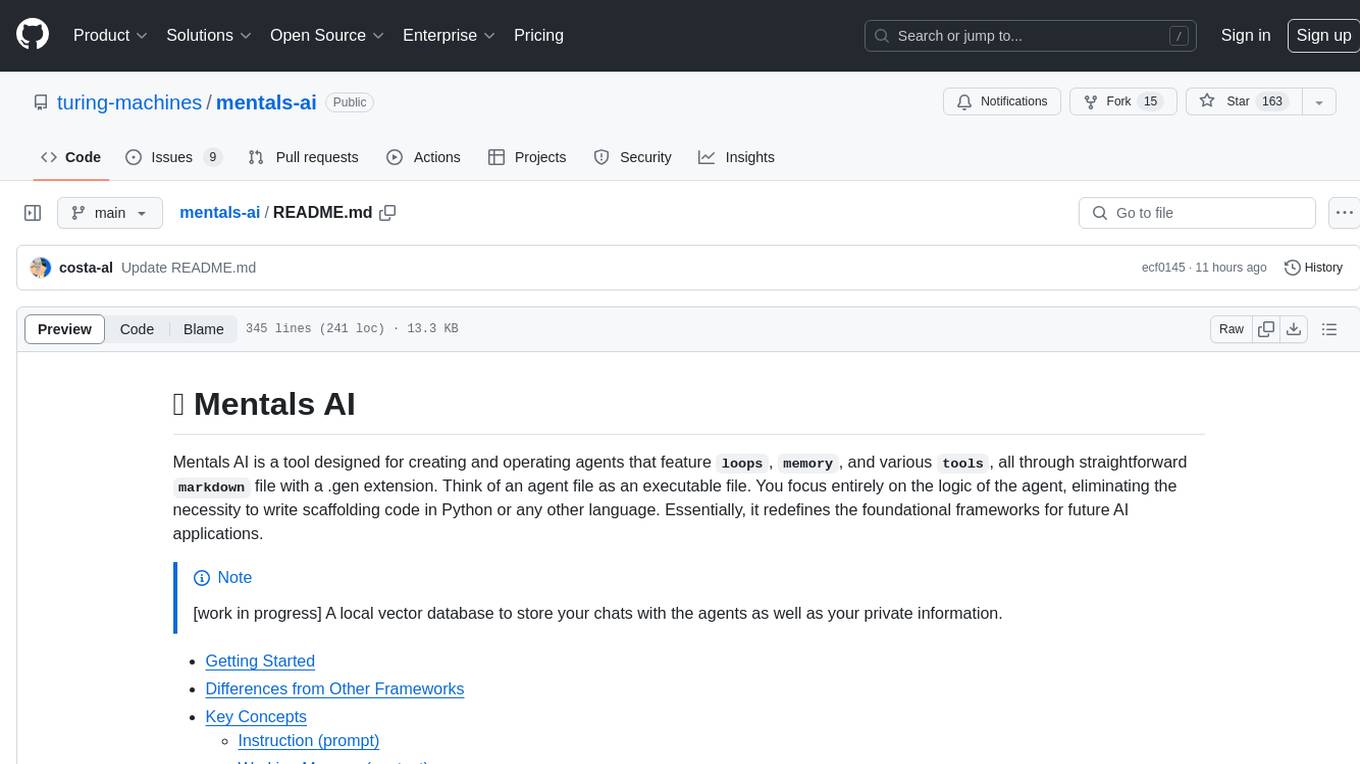
mentals-ai
Mentals AI is a tool designed for creating and operating agents that feature loops, memory, and various tools, all through straightforward markdown syntax. This tool enables you to concentrate solely on the agent’s logic, eliminating the necessity to compose underlying code in Python or any other language. It redefines the foundational frameworks for future AI applications by allowing the creation of agents with recursive decision-making processes, integration of reasoning frameworks, and control flow expressed in natural language. Key concepts include instructions with prompts and references, working memory for context, short-term memory for storing intermediate results, and control flow from strings to algorithms. The tool provides a set of native tools for message output, user input, file handling, Python interpreter, Bash commands, and short-term memory. The roadmap includes features like a web UI, vector database tools, agent's experience, and tools for image generation and browsing. The idea behind Mentals AI originated from studies on psychoanalysis executive functions and aims to integrate 'System 1' (cognitive executor) with 'System 2' (central executive) to create more sophisticated agents.
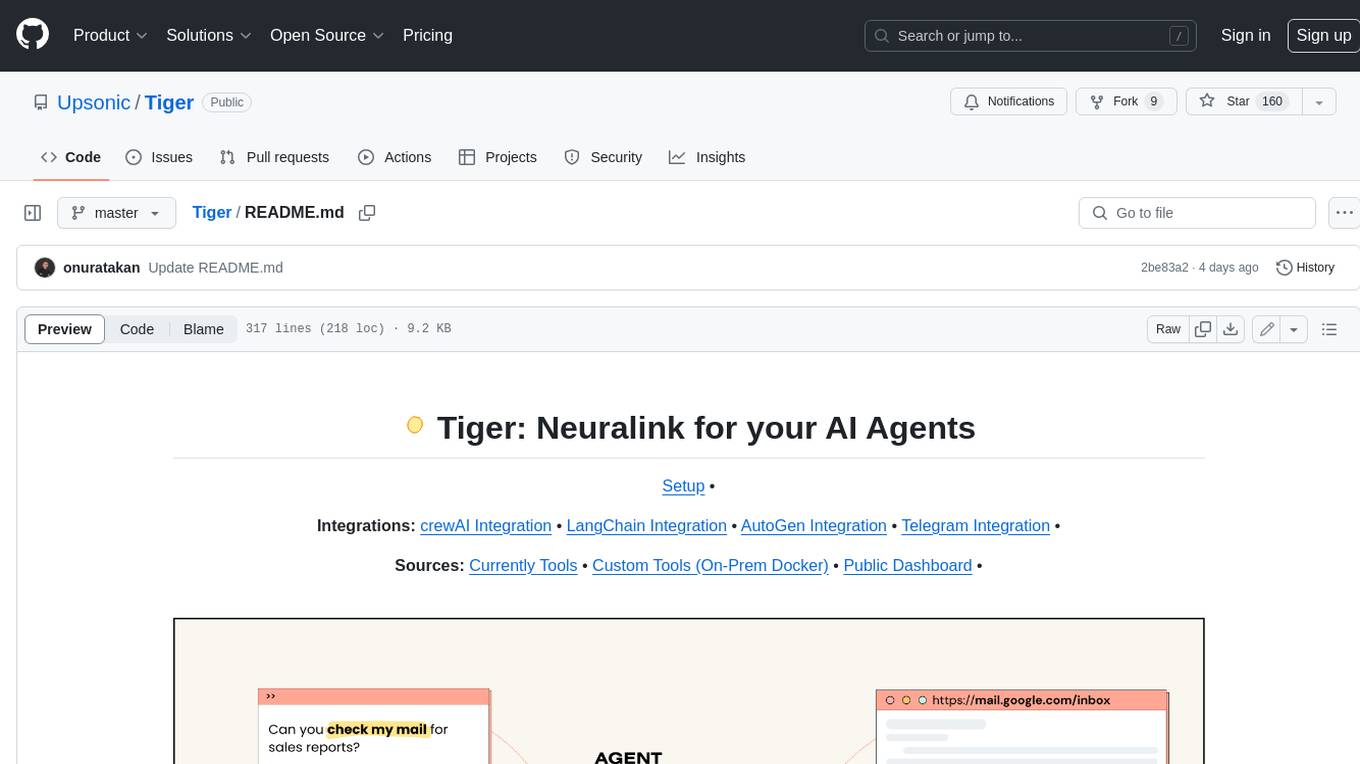
Tiger
Tiger is a community-driven project developing a reusable and integrated tool ecosystem for LLM Agent Revolution. It utilizes Upsonic for isolated tool storage, profiling, and automatic document generation. With Tiger, you can create a customized environment for your agents or leverage the robust and publicly maintained Tiger curated by the community itself.
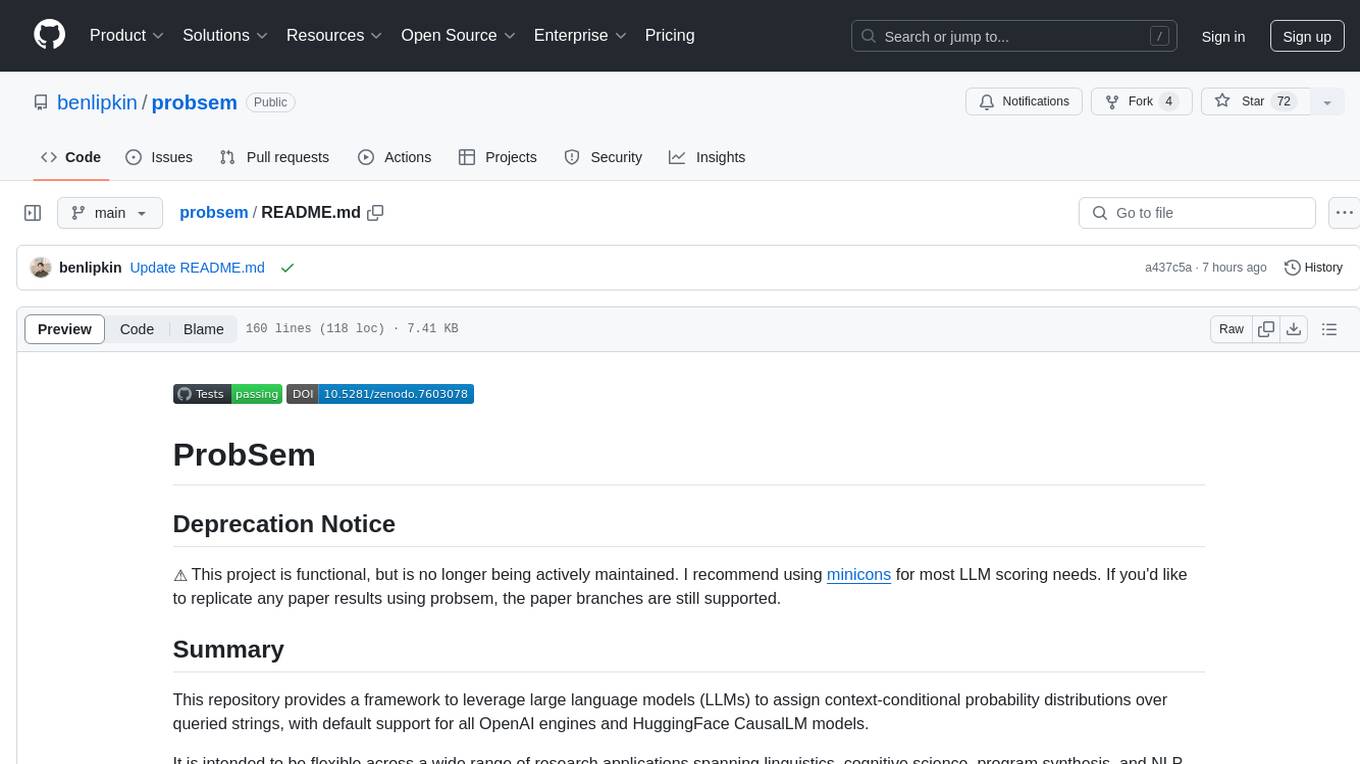
probsem
ProbSem is a repository that provides a framework to leverage large language models (LLMs) for assigning context-conditional probability distributions over queried strings. It supports OpenAI engines and HuggingFace CausalLM models, and is flexible for research applications in linguistics, cognitive science, program synthesis, and NLP. Users can define prompts, contexts, and queries to derive probability distributions over possible completions, enabling tasks like cloze completion, multiple-choice QA, semantic parsing, and code completion. The repository offers CLI and API interfaces for evaluation, with options to customize models, normalize scores, and adjust temperature for probability distributions.
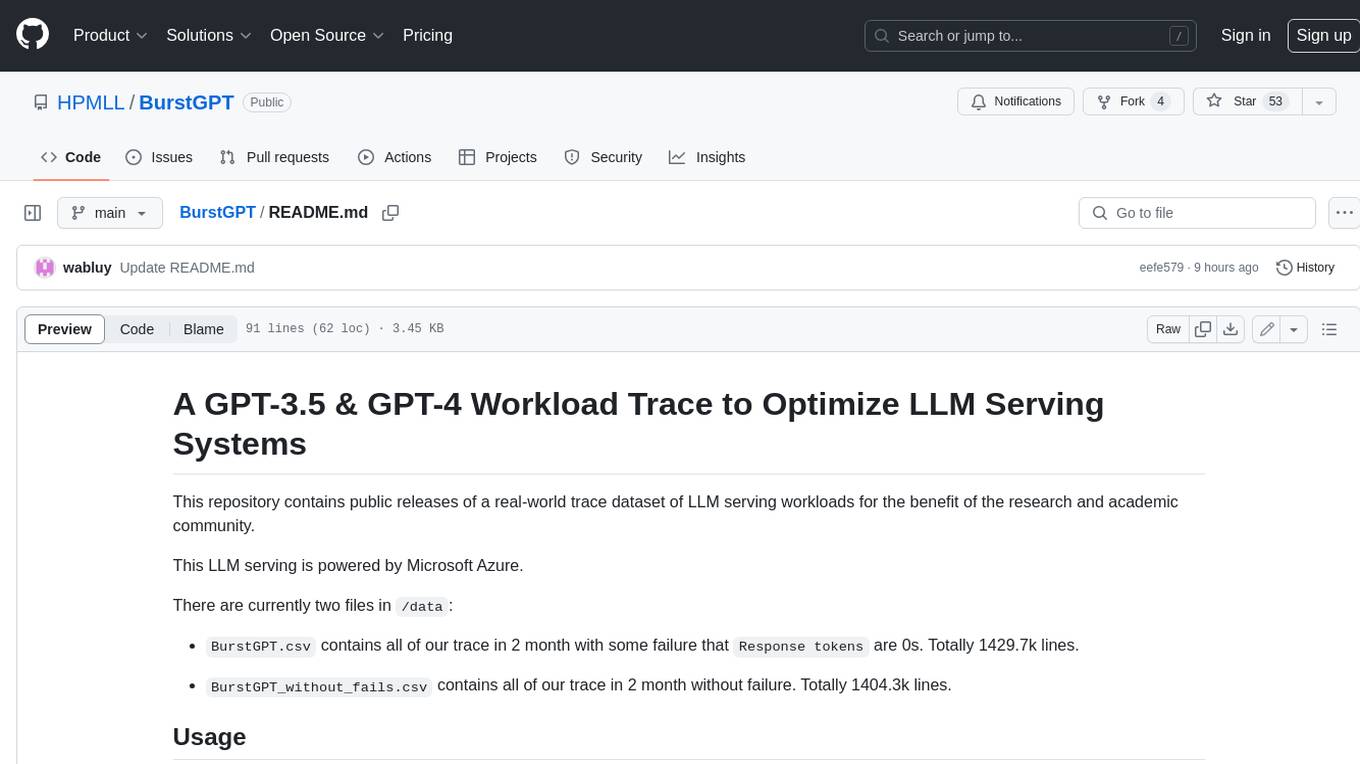
BurstGPT
This repository provides a real-world trace dataset of LLM serving workloads for research and academic purposes. The dataset includes two files, BurstGPT.csv with trace data for 2 months including some failures, and BurstGPT_without_fails.csv without any failures. Users can scale the RPS in the trace, model patterns, and leverage the trace for various evaluations. Future plans include updating the time range of the trace, adding request end times, updating conversation logs, and open-sourcing a benchmark suite for LLM inference. The dataset covers 61 consecutive days, contains 1.4 million lines, and is approximately 50MB in size.
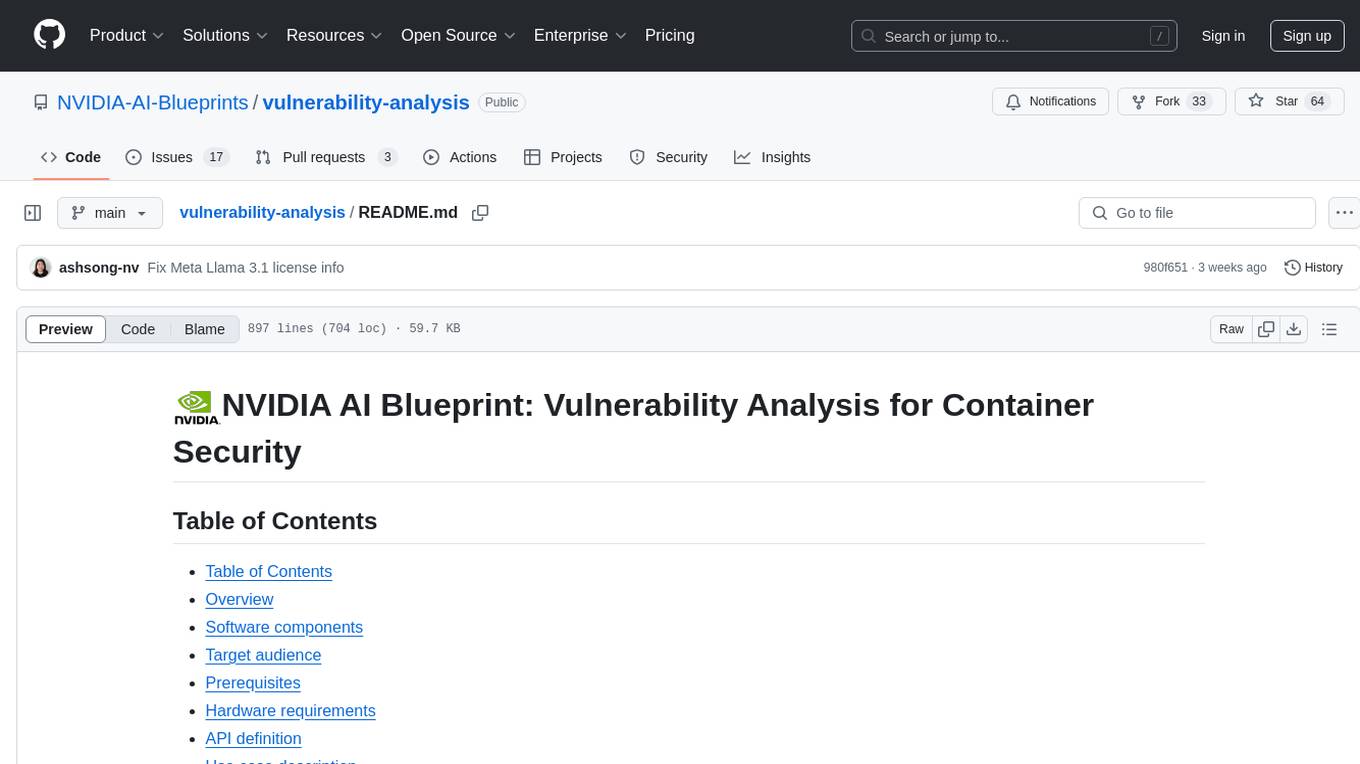
vulnerability-analysis
The NVIDIA AI Blueprint for Vulnerability Analysis for Container Security showcases accelerated analysis on common vulnerabilities and exposures (CVE) at an enterprise scale, reducing mitigation time from days to seconds. It enables security analysts to determine software package vulnerabilities using large language models (LLMs) and retrieval-augmented generation (RAG). The blueprint is designed for security analysts, IT engineers, and AI practitioners in cybersecurity. It requires NVAIE developer license and API keys for vulnerability databases, search engines, and LLM model services. Hardware requirements include L40 GPU for pipeline operation and optional LLM NIM and Embedding NIM. The workflow involves LLM pipeline for CVE impact analysis, utilizing LLM planner, agent, and summarization nodes. The blueprint uses NVIDIA NIM microservices and Morpheus Cybersecurity AI SDK for vulnerability analysis.
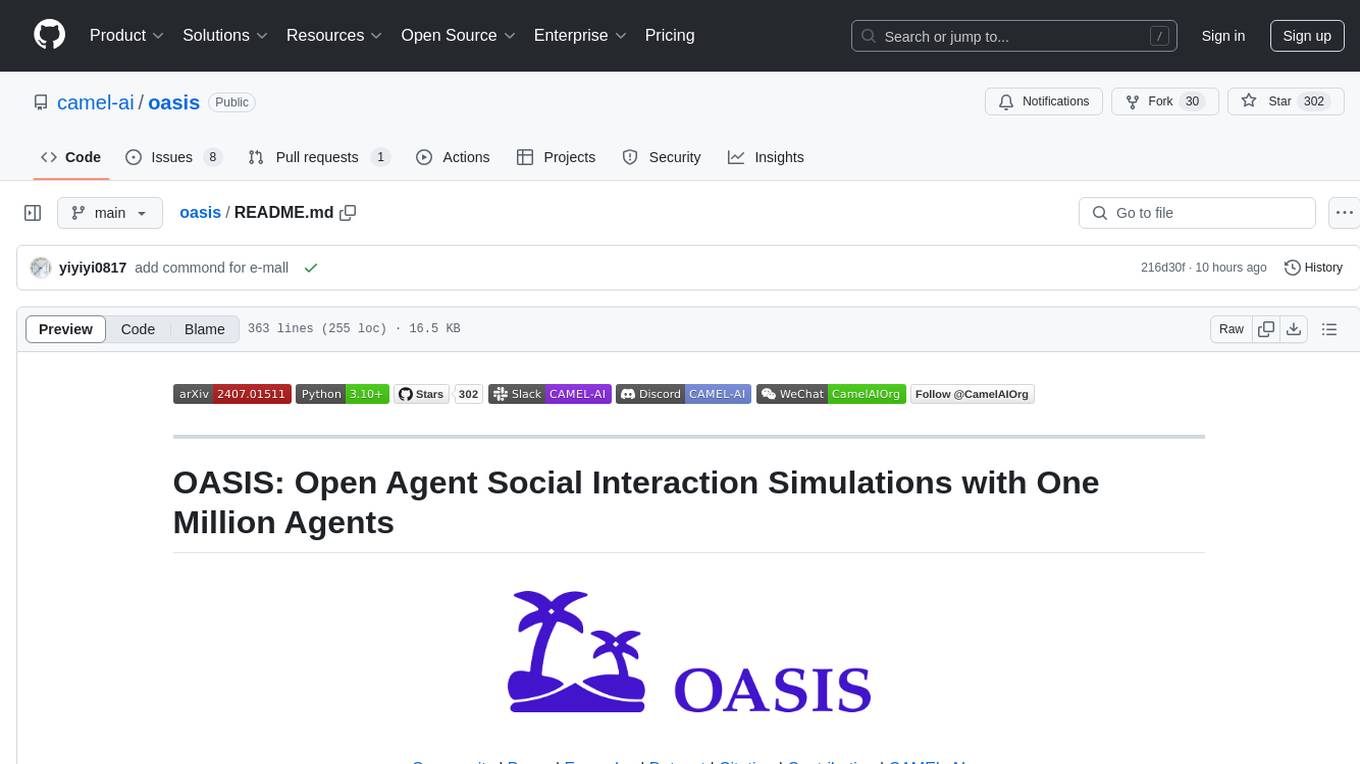
oasis
OASIS is a scalable, open-source social media simulator that integrates large language models with rule-based agents to realistically mimic the behavior of up to one million users on platforms like Twitter and Reddit. It facilitates the study of complex social phenomena such as information spread, group polarization, and herd behavior, offering a versatile tool for exploring diverse social dynamics and user interactions in digital environments. With features like scalability, dynamic environments, diverse action spaces, and integrated recommendation systems, OASIS provides a comprehensive platform for simulating social media interactions at a large scale.
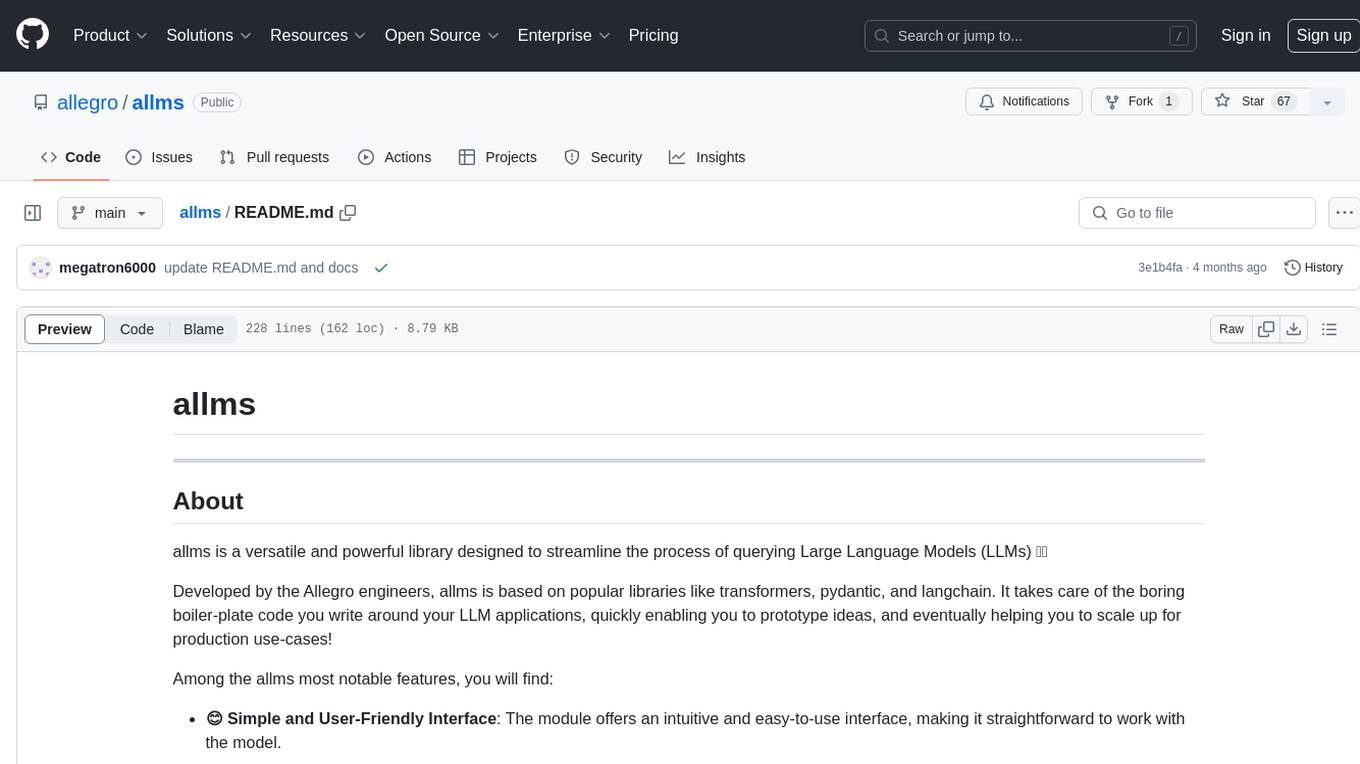
allms
allms is a versatile and powerful library designed to streamline the process of querying Large Language Models (LLMs). Developed by Allegro engineers, it simplifies working with LLM applications by providing a user-friendly interface, asynchronous querying, automatic retrying mechanism, error handling, and output parsing. It supports various LLM families hosted on different platforms like OpenAI, Google, Azure, and GCP. The library offers features for configuring endpoint credentials, batch querying with symbolic variables, and forcing structured output format. It also provides documentation, quickstart guides, and instructions for local development, testing, updating documentation, and making new releases.
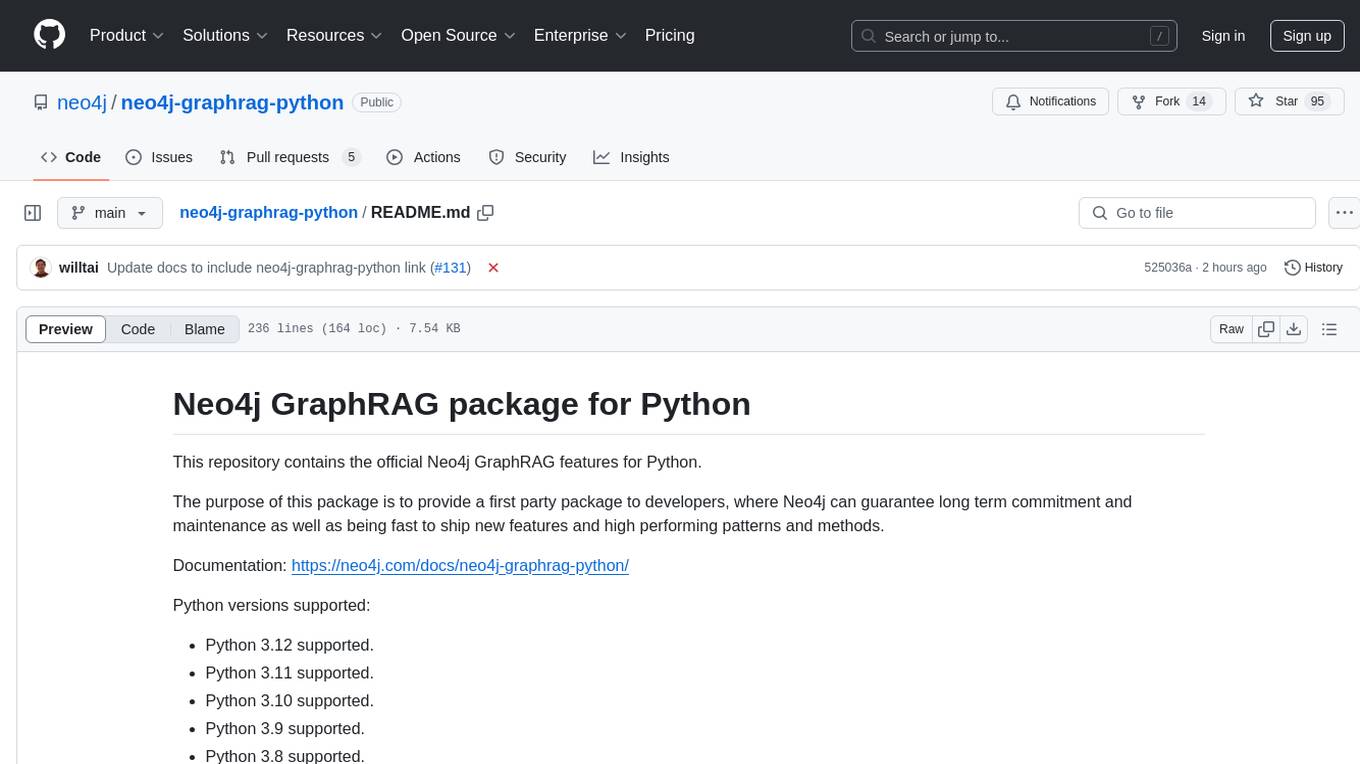
neo4j-graphrag-python
The Neo4j GraphRAG package for Python is an official repository that provides features for creating and managing vector indexes in Neo4j databases. It aims to offer developers a reliable package with long-term commitment, maintenance, and fast feature updates. The package supports various Python versions and includes functionalities for creating vector indexes, populating them, and performing similarity searches. It also provides guidelines for installation, examples, and development processes such as installing dependencies, making changes, and running tests.
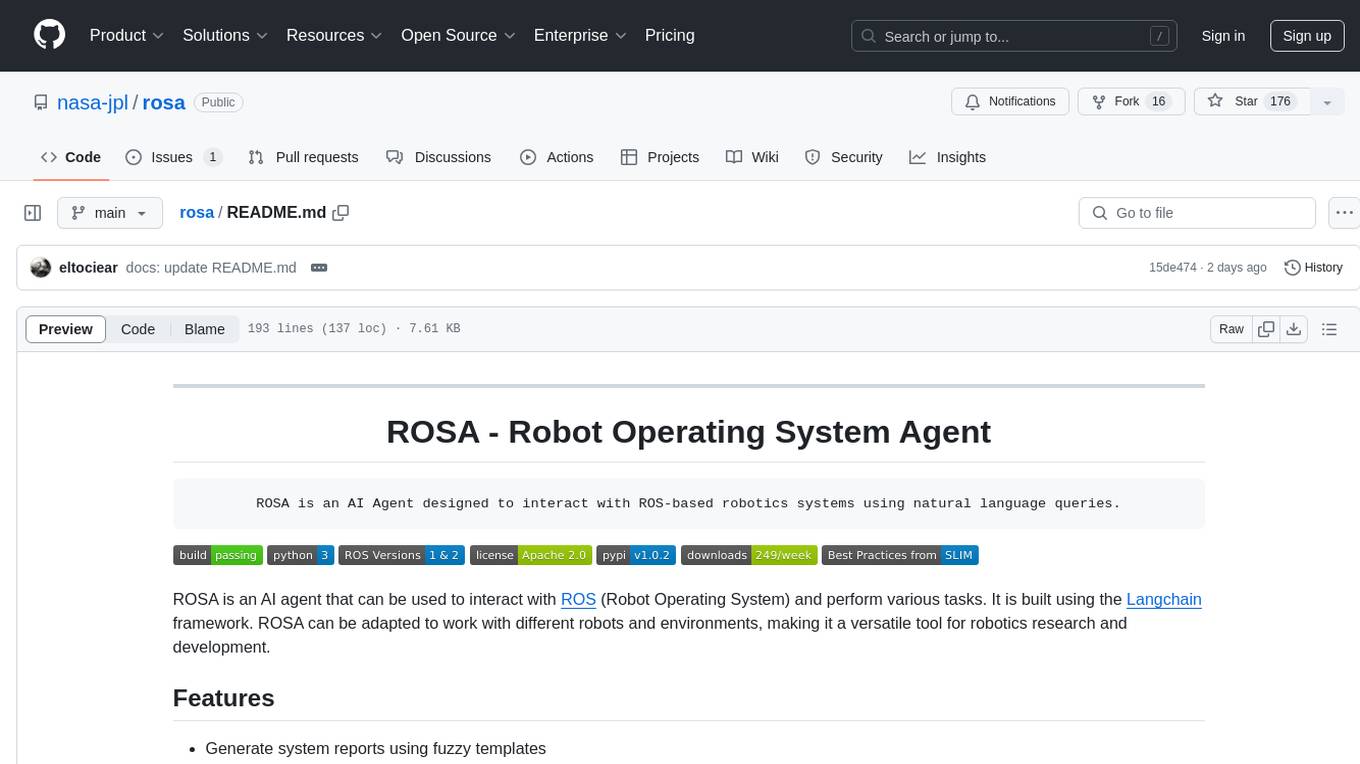
rosa
ROSA is an AI Agent designed to interact with ROS-based robotics systems using natural language queries. It can generate system reports, read and parse ROS log files, adapt to new robots, and run various ROS commands using natural language. The tool is versatile for robotics research and development, providing an easy way to interact with robots and the ROS environment.
For similar tasks
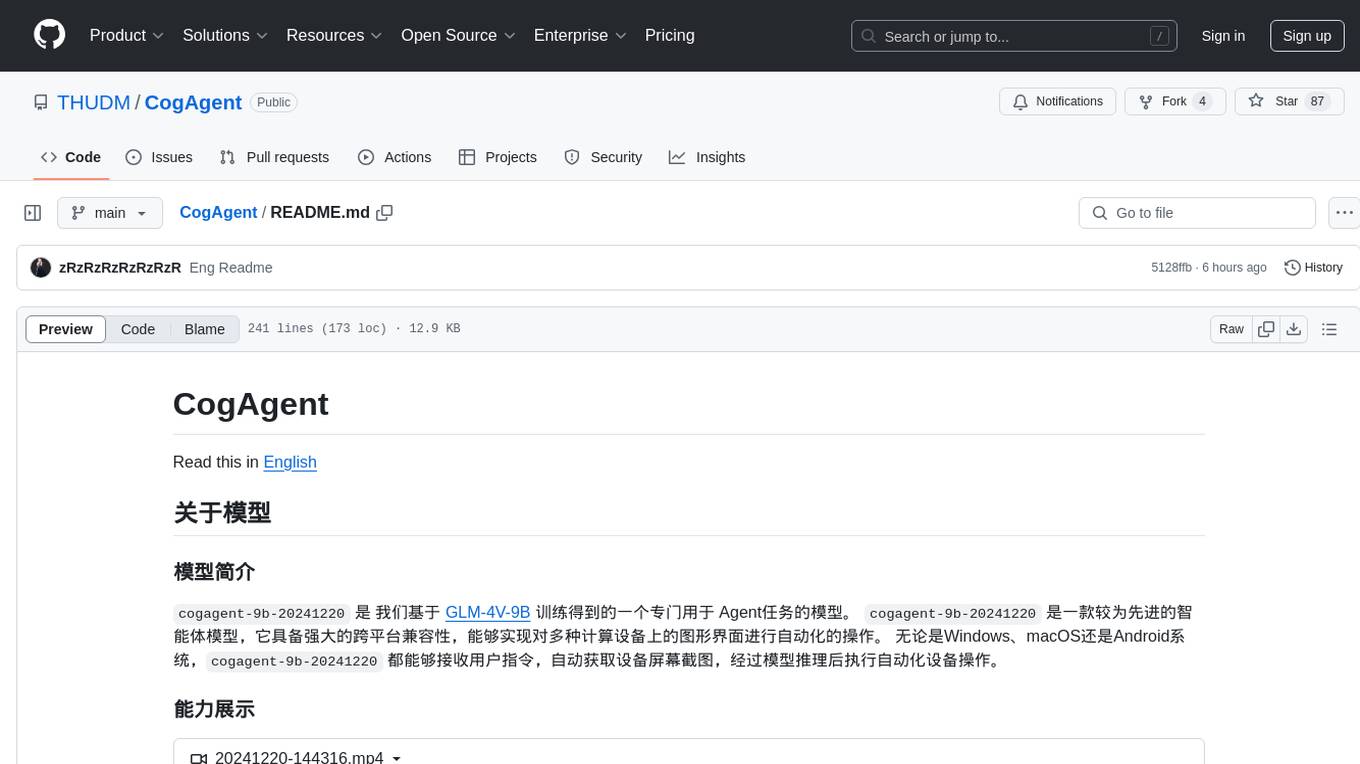
CogAgent
CogAgent is an advanced intelligent agent model designed for automating operations on graphical interfaces across various computing devices. It supports platforms like Windows, macOS, and Android, enabling users to issue commands, capture device screenshots, and perform automated operations. The model requires a minimum of 29GB of GPU memory for inference at BF16 precision and offers capabilities for executing tasks like sending Christmas greetings and sending emails. Users can interact with the model by providing task descriptions, platform specifications, and desired output formats.
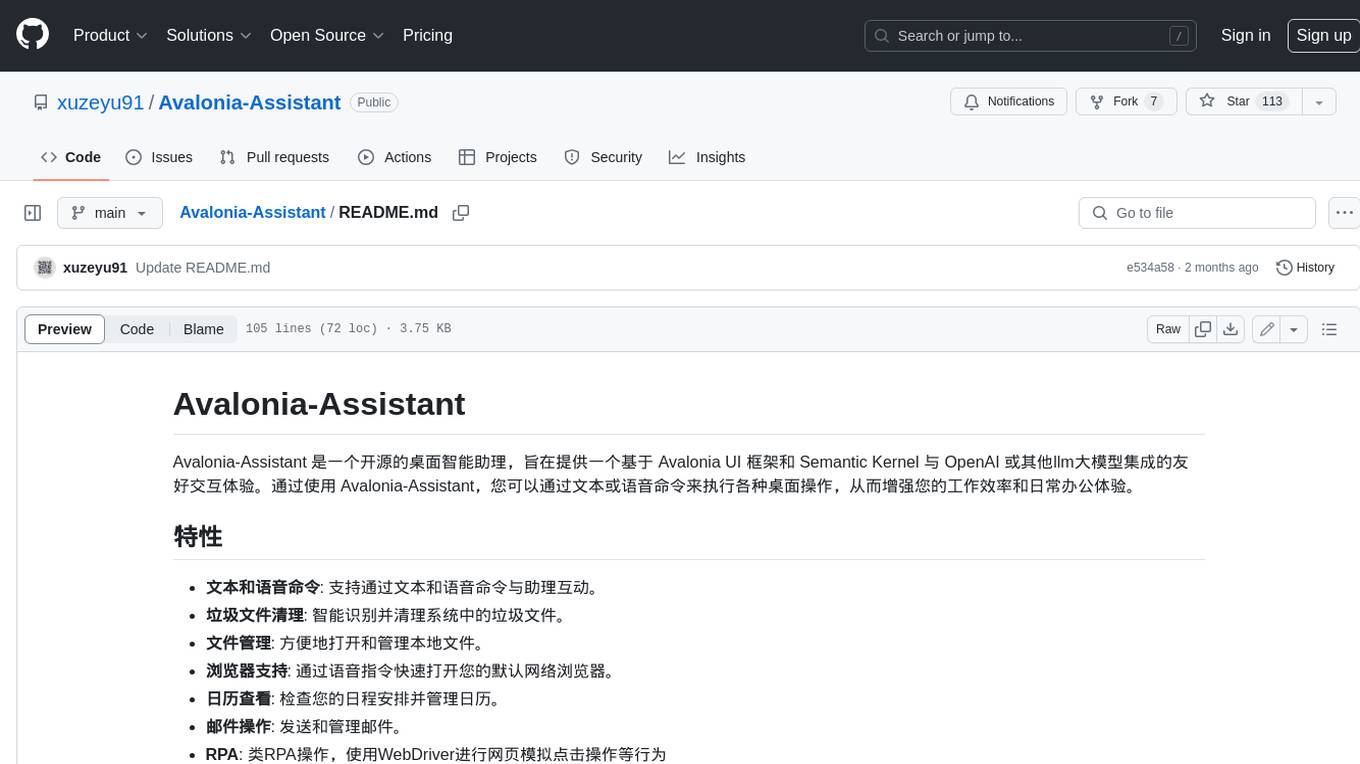
Avalonia-Assistant
Avalonia-Assistant is an open-source desktop intelligent assistant that aims to provide a user-friendly interactive experience based on the Avalonia UI framework and the integration of Semantic Kernel with OpenAI or other large LLM models. By utilizing Avalonia-Assistant, you can perform various desktop operations through text or voice commands, enhancing your productivity and daily office experience.
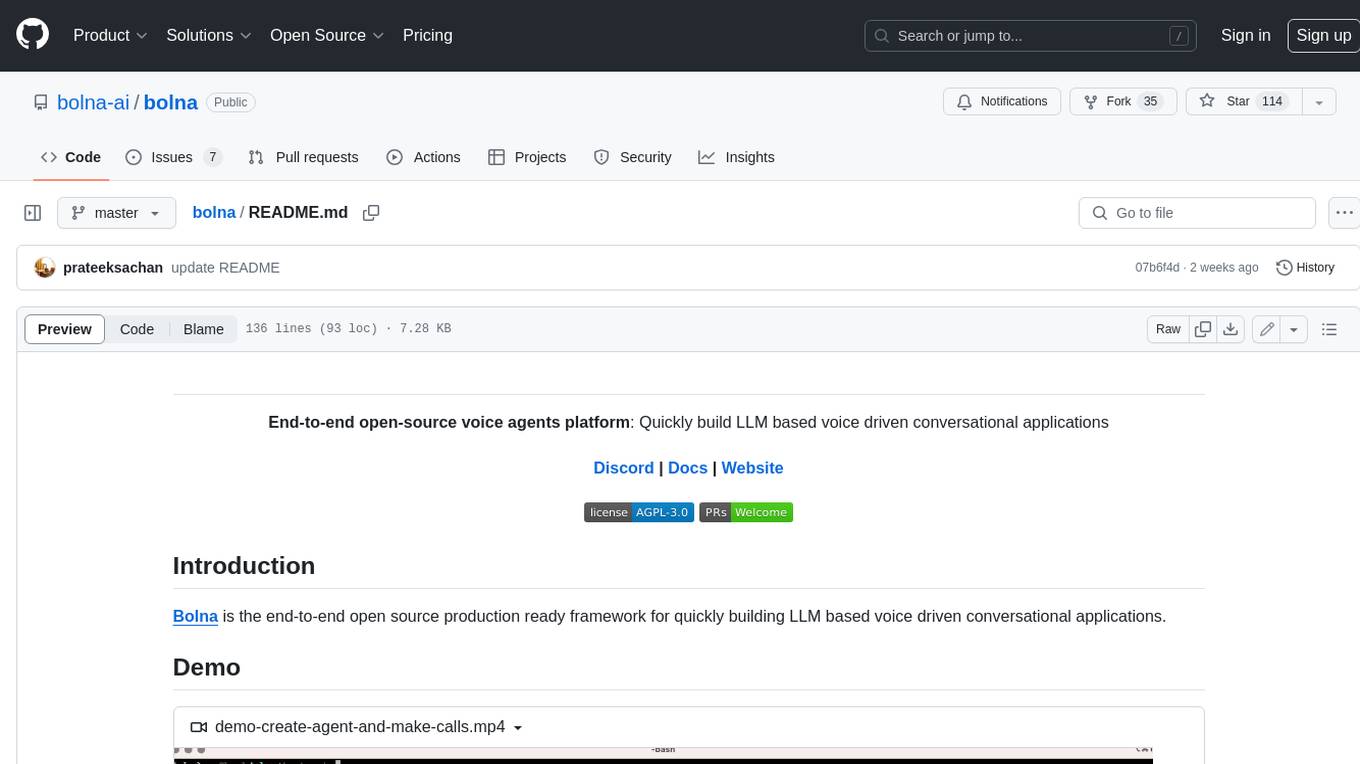
bolna
Bolna is an open-source platform for building voice-driven conversational applications using large language models (LLMs). It provides a comprehensive set of tools and integrations to handle various aspects of voice-based interactions, including telephony, transcription, LLM-based conversation handling, and text-to-speech synthesis. Bolna simplifies the process of creating voice agents that can perform tasks such as initiating phone calls, transcribing conversations, generating LLM-powered responses, and synthesizing speech. It supports multiple providers for each component, allowing users to customize their setup based on their specific needs. Bolna is designed to be easy to use, with a straightforward local setup process and well-documented APIs. It is also extensible, enabling users to integrate with other telephony providers or add custom functionality.
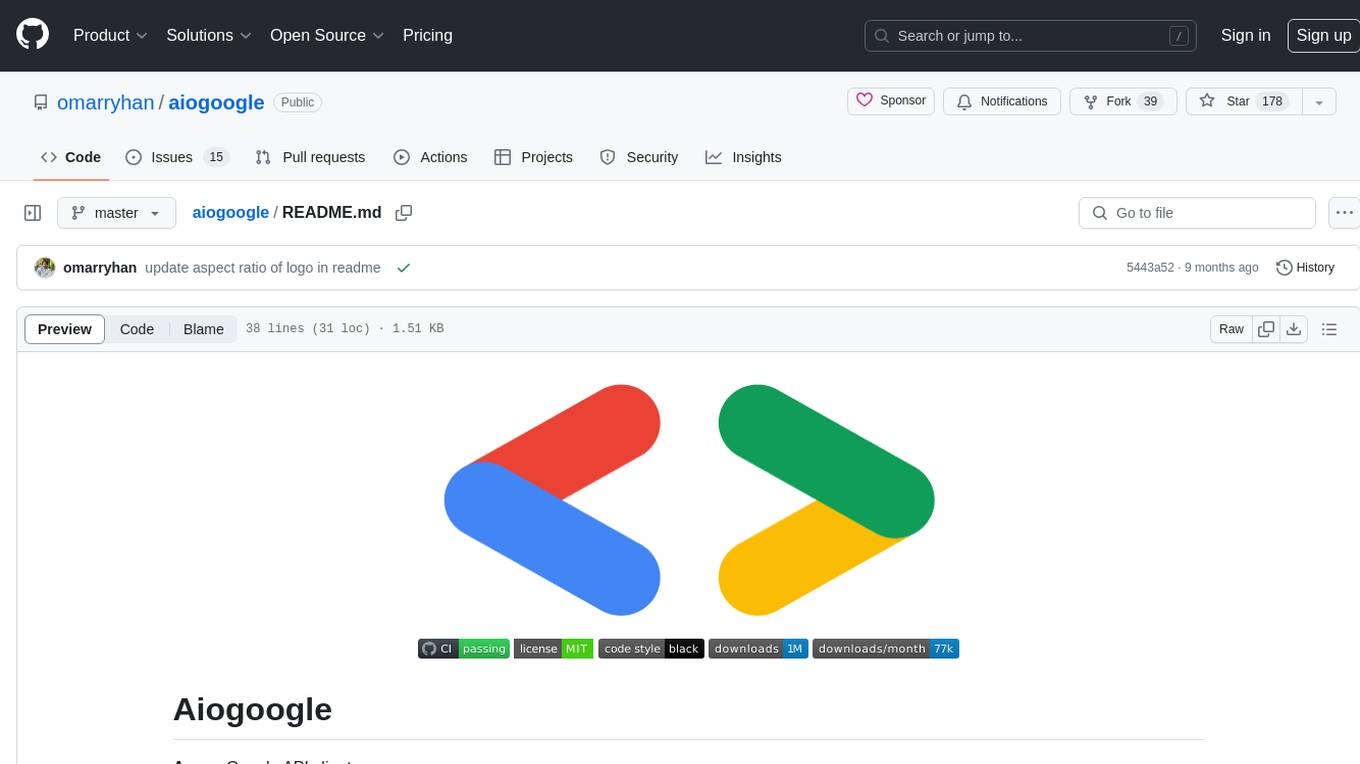
aiogoogle
Aiogoogle is an asynchronous Google API client that allows users to access various Google public APIs such as Google Calendar, Drive, Contacts, Gmail, Maps, Youtube, Translate, Sheets, Docs, Analytics, Books, Fitness, Genomics, Cloud Storage, Kubernetes Engine, and more. It simplifies the process of interacting with Google APIs by providing async capabilities.
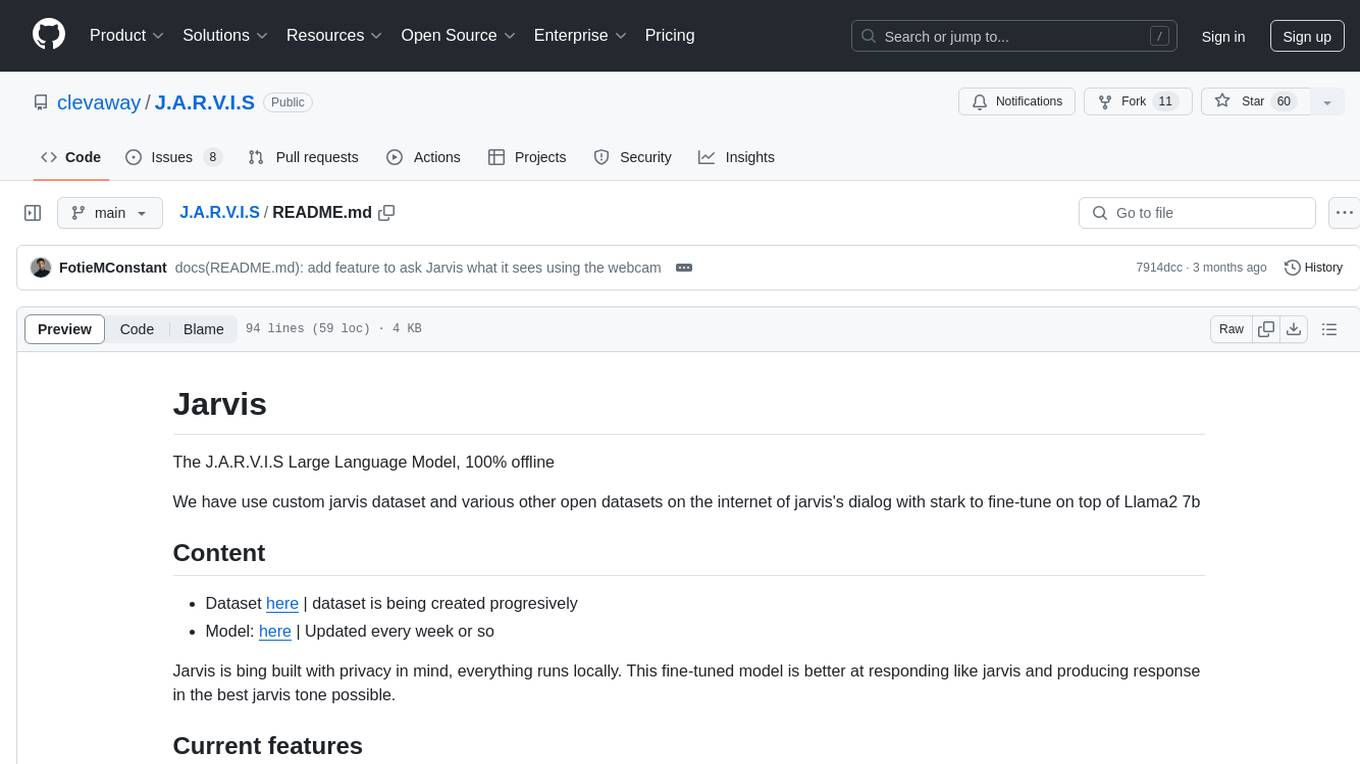
J.A.R.V.I.S
J.A.R.V.I.S. is an offline large language model fine-tuned on custom and open datasets to mimic Jarvis's dialog with Stark. It prioritizes privacy by running locally and excels in responding like Jarvis with a similar tone. Current features include time/date queries, web searches, playing YouTube videos, and webcam image descriptions. Users can interact with Jarvis via command line after installing the model locally using Ollama. Future plans involve voice cloning, voice-to-text input, and deploying the voice model as an API.
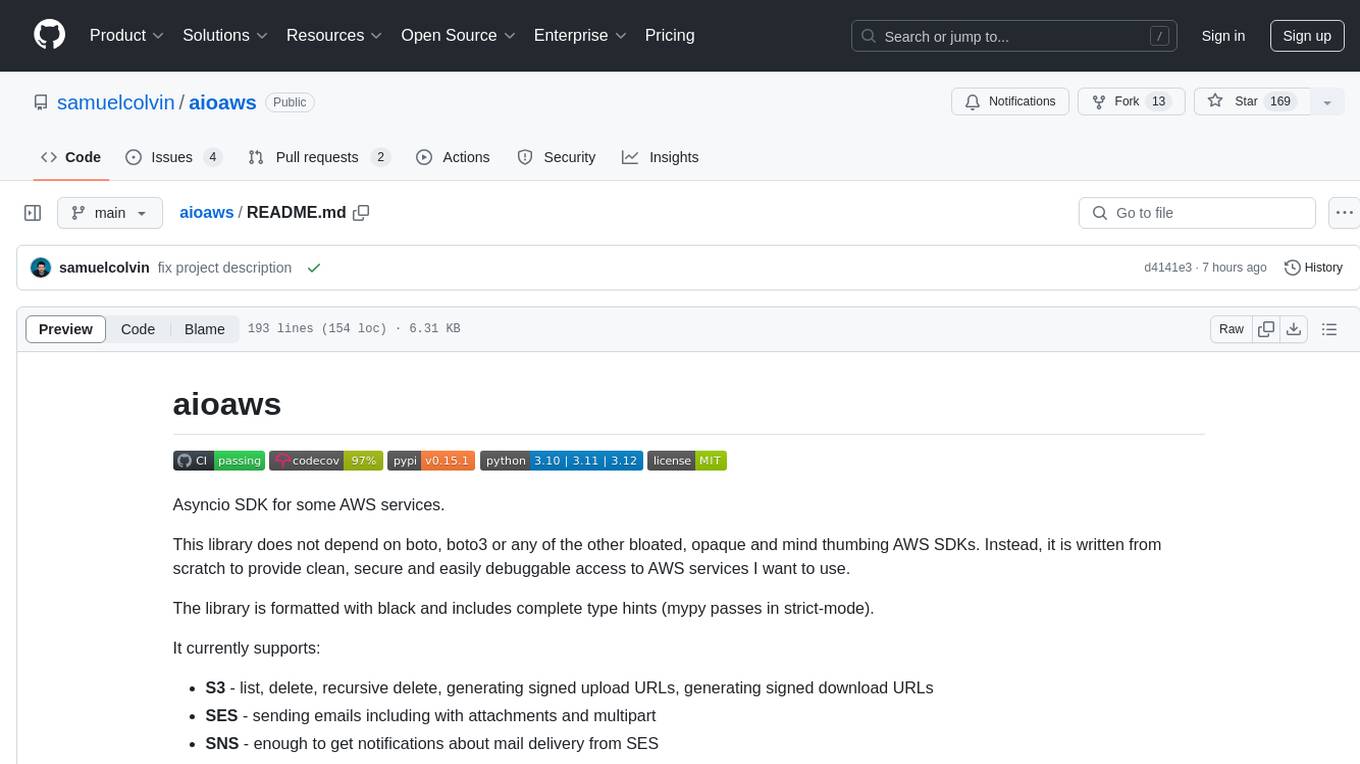
aioaws
Aioaws is an asyncio SDK for some AWS services, providing clean, secure, and easily debuggable access to services like S3, SES, and SNS. It is written from scratch without dependencies on boto or boto3, formatted with black, and includes complete type hints. The library supports various functionalities such as listing, deleting, and generating signed URLs for S3 files, sending emails with attachments and multipart content via SES, and receiving notifications about mail delivery from SES. It also offers AWS Signature Version 4 authentication and has minimal dependencies like aiofiles, cryptography, httpx, and pydantic.
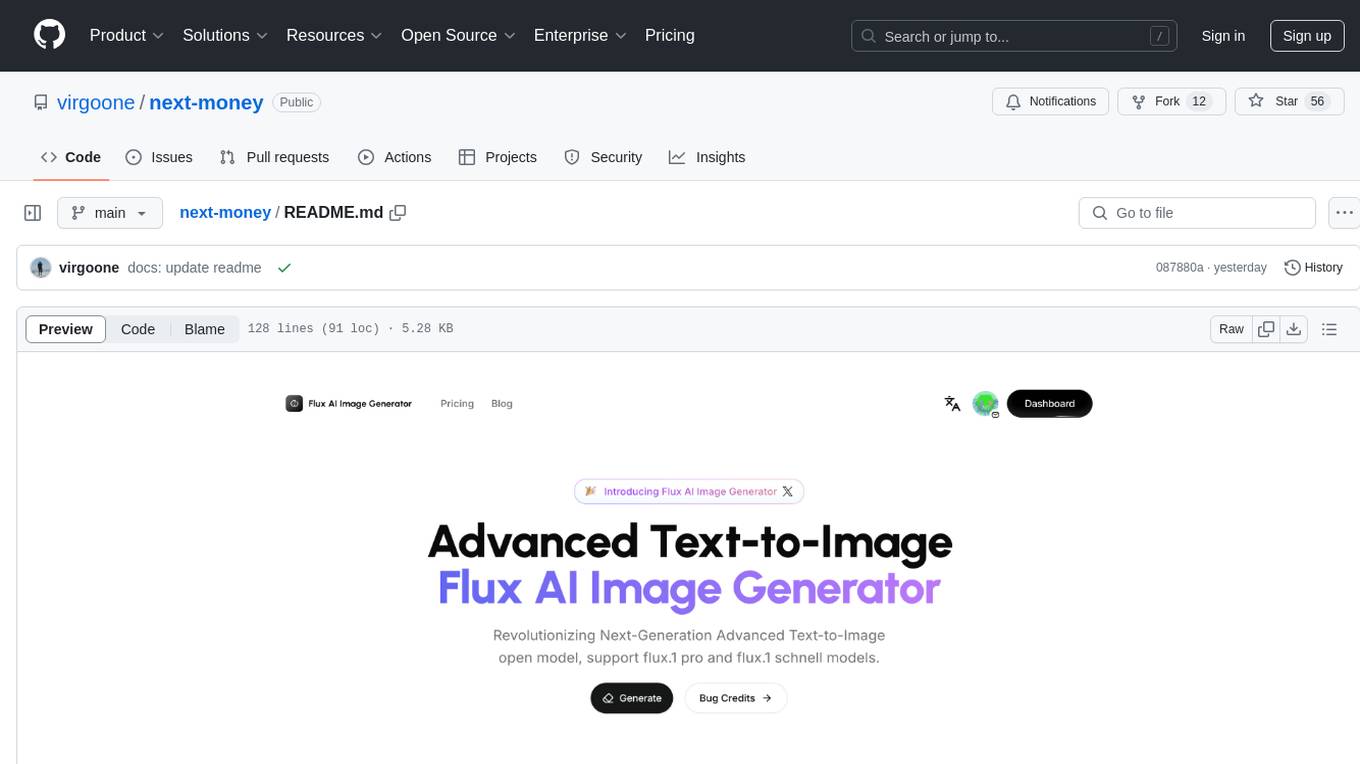
next-money
Next Money Stripe Starter is a SaaS Starter project that empowers your next project with a stack of Next.js, Prisma, Supabase, Clerk Auth, Resend, React Email, Shadcn/ui, and Stripe. It seamlessly integrates these technologies to accelerate your development and SaaS journey. The project includes frameworks, platforms, UI components, hooks and utilities, code quality tools, and miscellaneous features to enhance the development experience. Created by @koyaguo in 2023 and released under the MIT license.
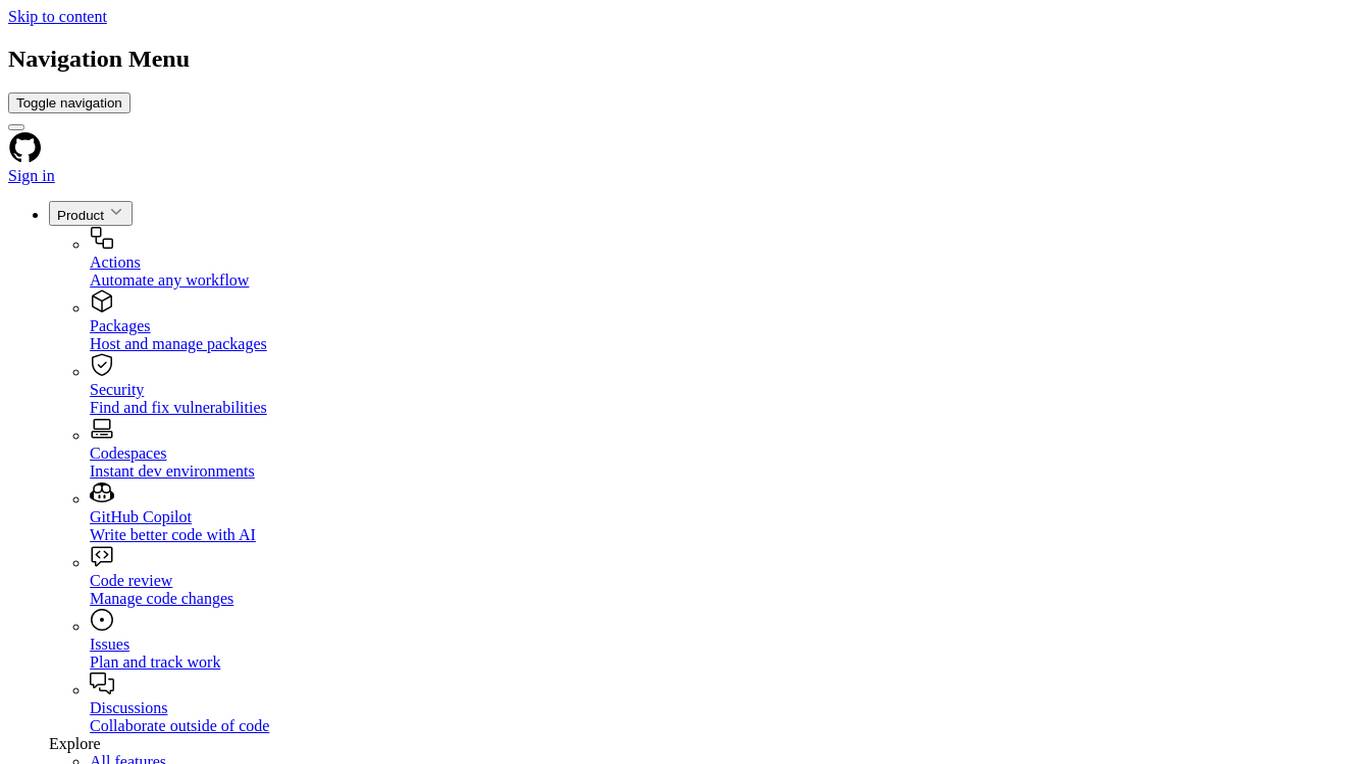
J.A.R.V.I.S.
J.A.R.V.I.S.1.0 is an advanced virtual assistant tool designed to assist users in various tasks. It provides a wide range of functionalities including voice commands, task automation, information retrieval, and communication management. With its intuitive interface and powerful capabilities, J.A.R.V.I.S.1.0 aims to enhance productivity and streamline daily activities for users.
For similar jobs
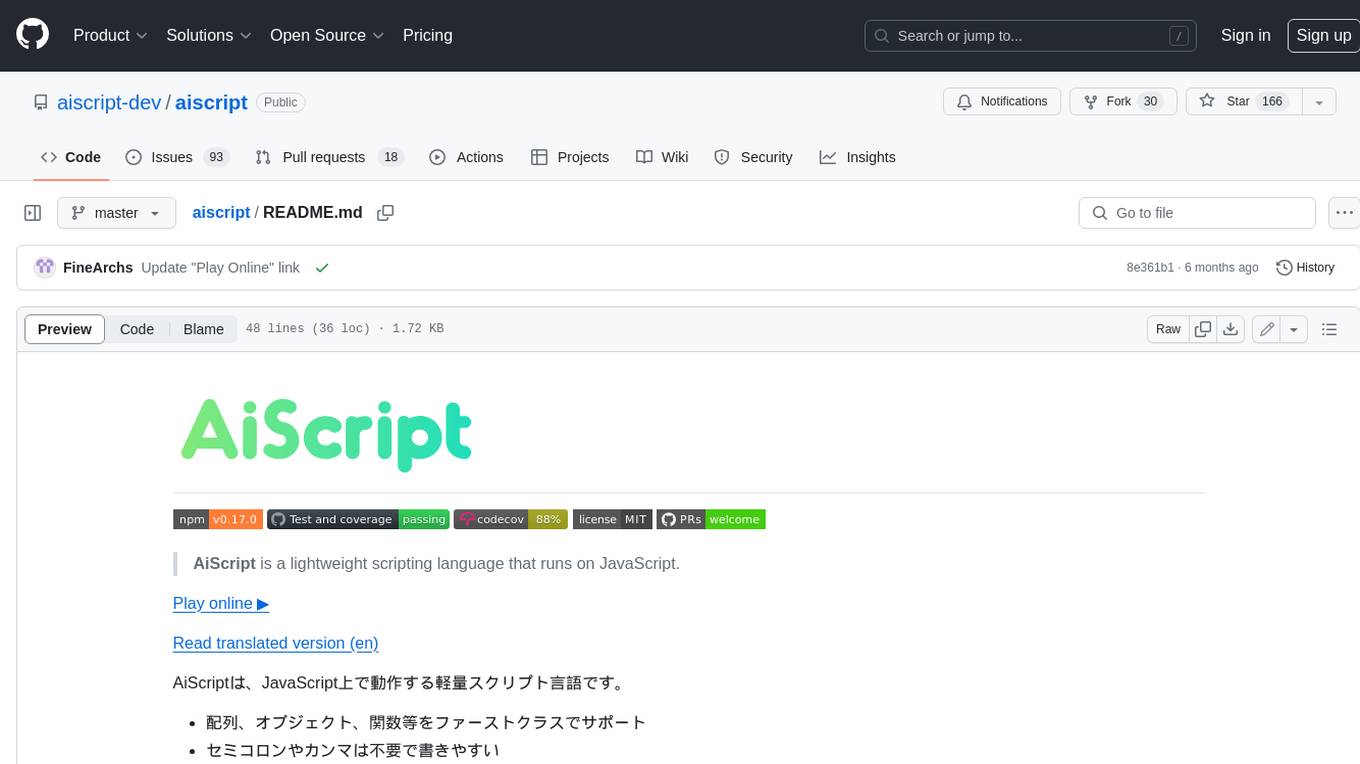
aiscript
AiScript is a lightweight scripting language that runs on JavaScript. It supports arrays, objects, and functions as first-class citizens, and is easy to write without the need for semicolons or commas. AiScript runs in a secure sandbox environment, preventing infinite loops from freezing the host. It also allows for easy provision of variables and functions from the host.
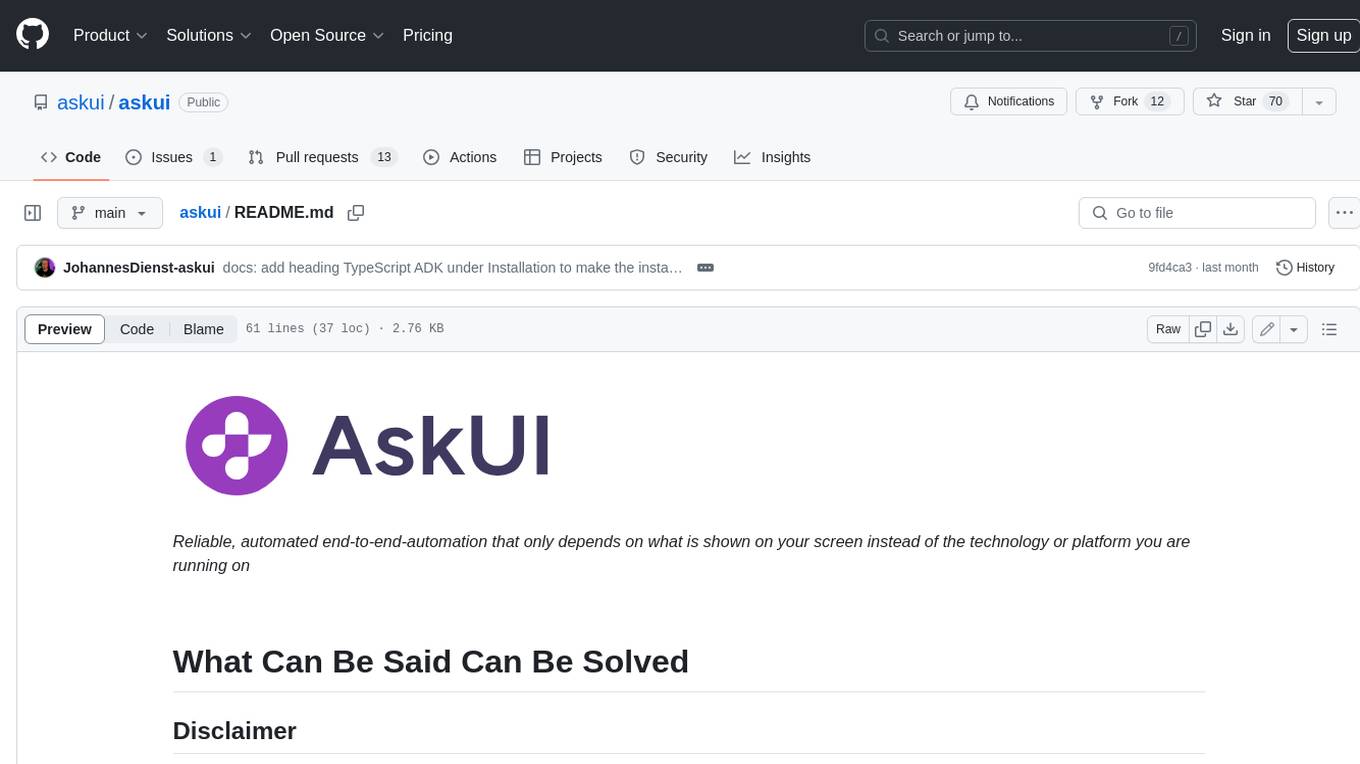
askui
AskUI is a reliable, automated end-to-end automation tool that only depends on what is shown on your screen instead of the technology or platform you are running on.
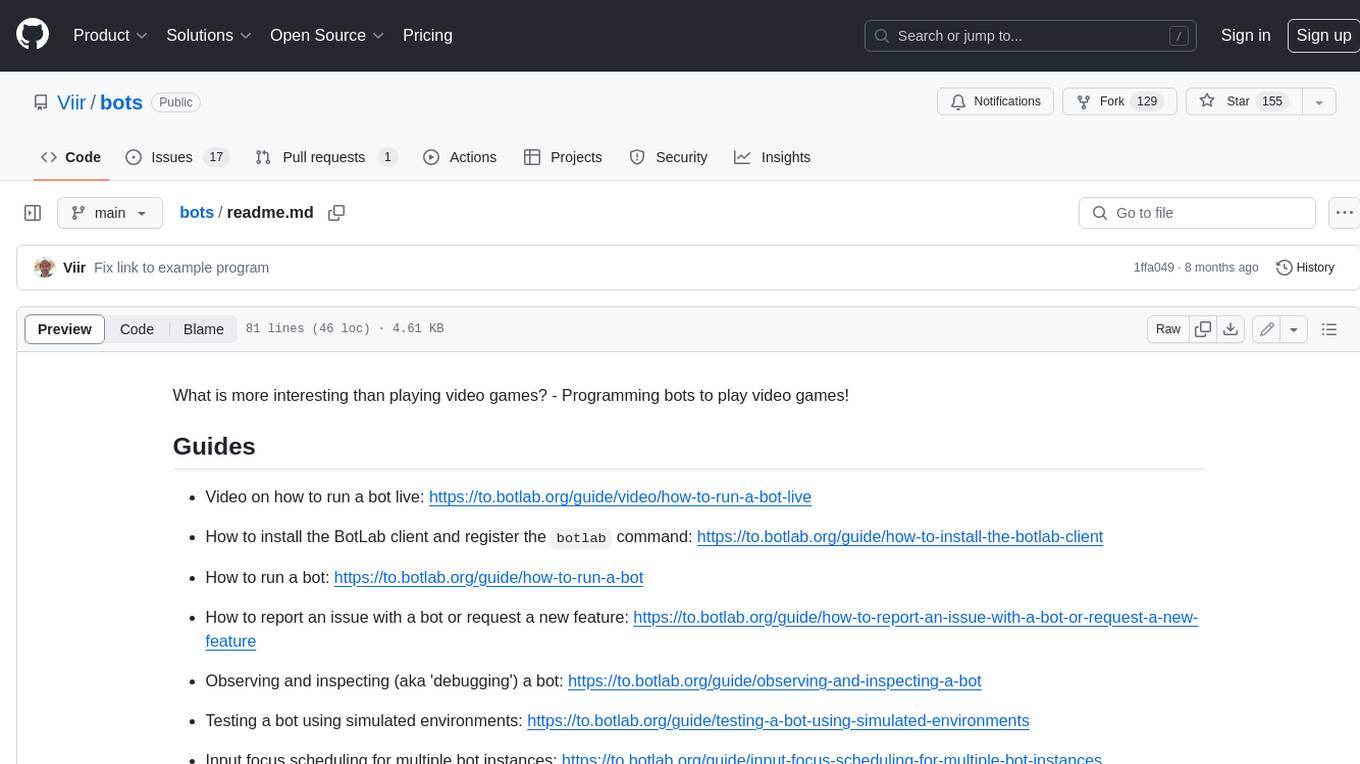
bots
The 'bots' repository is a collection of guides, tools, and example bots for programming bots to play video games. It provides resources on running bots live, installing the BotLab client, debugging bots, testing bots in simulated environments, and more. The repository also includes example bots for games like EVE Online, Tribal Wars 2, and Elvenar. Users can learn about developing bots for specific games, syntax of the Elm programming language, and tools for memory reading development. Additionally, there are guides on bot programming, contributing to BotLab, and exploring Elm syntax and core library.
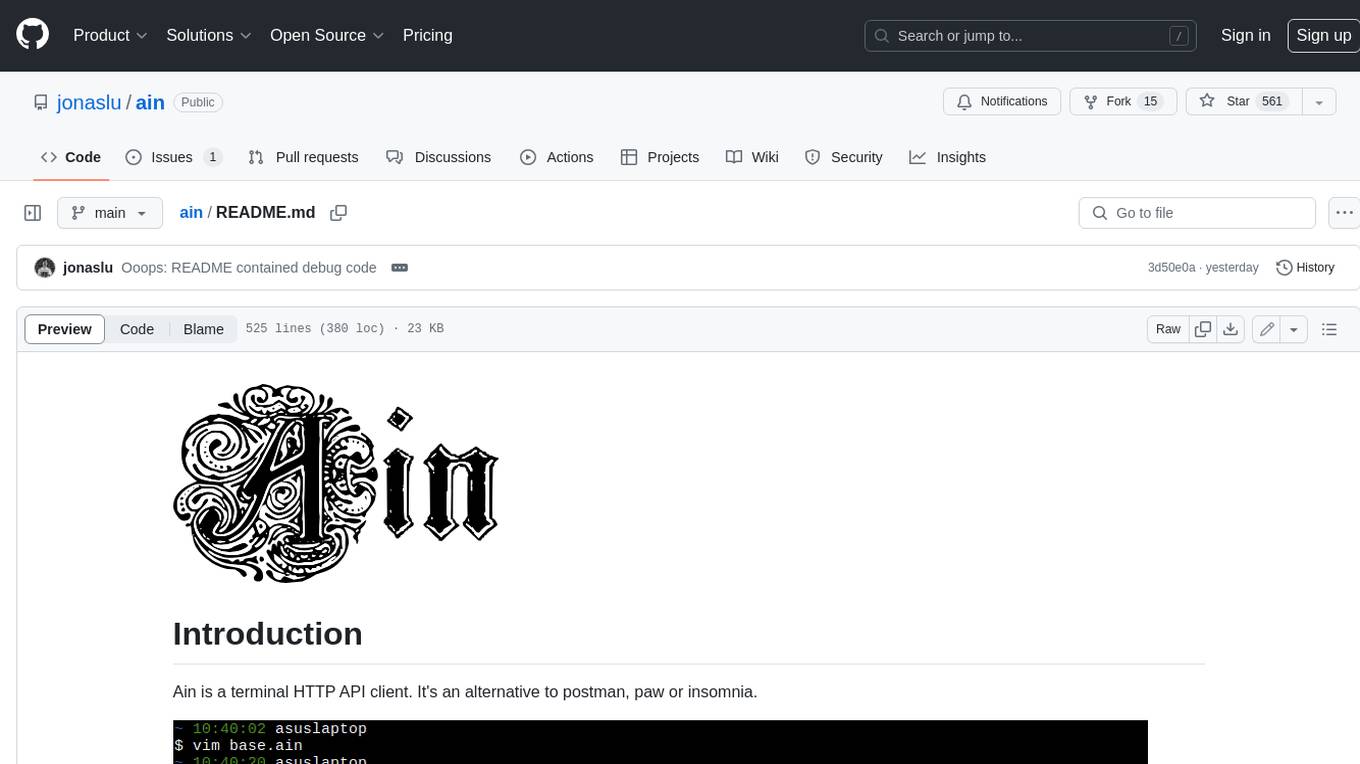
ain
Ain is a terminal HTTP API client designed for scripting input and processing output via pipes. It allows flexible organization of APIs using files and folders, supports shell-scripts and executables for common tasks, handles url-encoding, and enables sharing the resulting curl, wget, or httpie command-line. Users can put things that change in environment variables or .env-files, and pipe the API output for further processing. Ain targets users who work with many APIs using a simple file format and uses curl, wget, or httpie to make the actual calls.
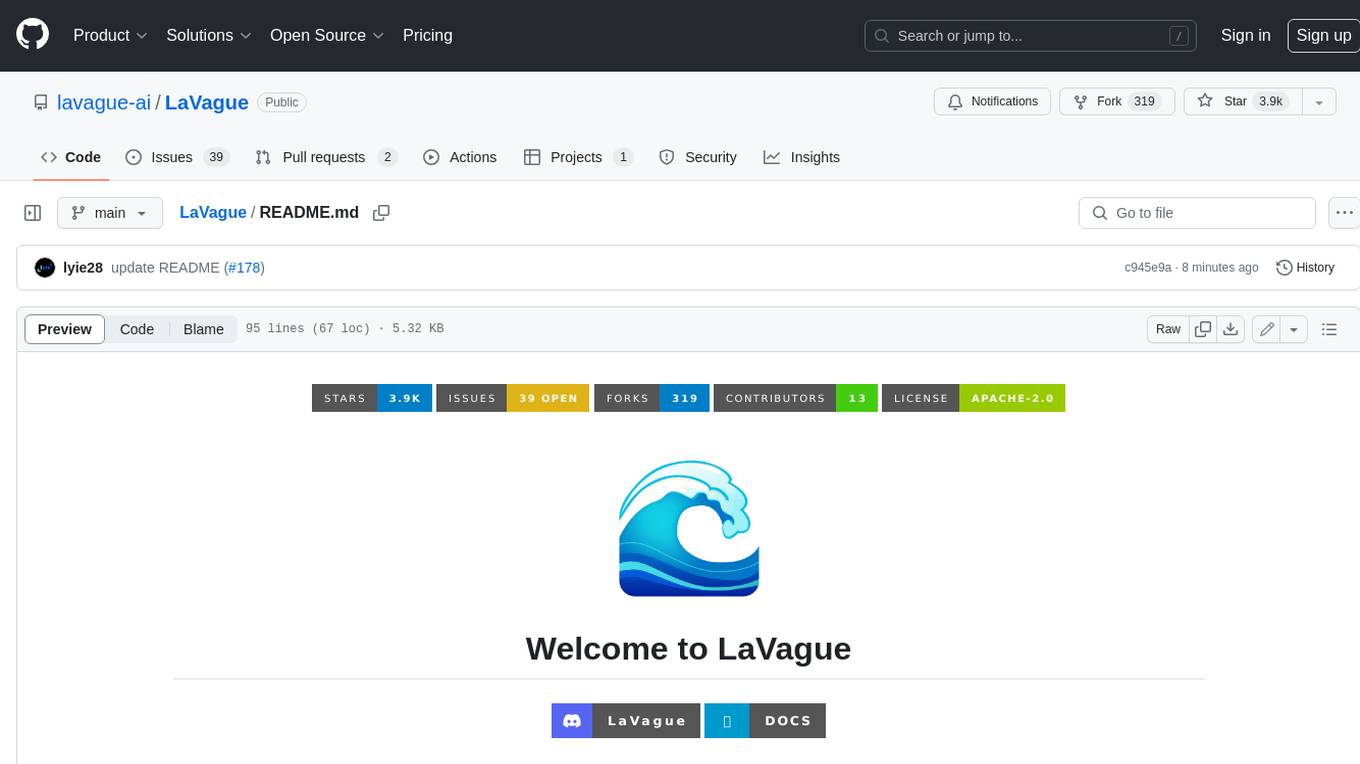
LaVague
LaVague is an open-source Large Action Model framework that uses advanced AI techniques to compile natural language instructions into browser automation code. It leverages Selenium or Playwright for browser actions. Users can interact with LaVague through an interactive Gradio interface to automate web interactions. The tool requires an OpenAI API key for default examples and offers a Playwright integration guide. Contributors can help by working on outlined tasks, submitting PRs, and engaging with the community on Discord. The project roadmap is available to track progress, but users should exercise caution when executing LLM-generated code using 'exec'.
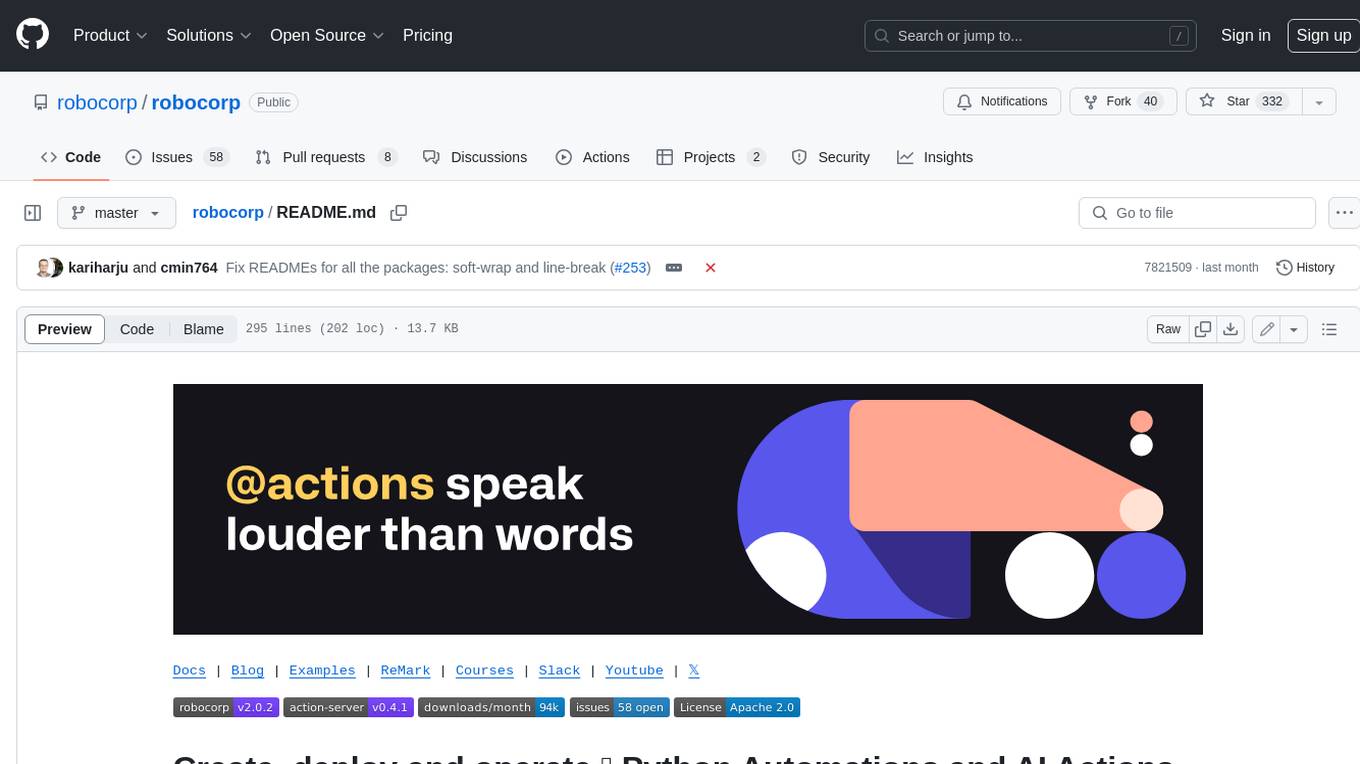
robocorp
Robocorp is a platform that allows users to create, deploy, and operate Python automations and AI actions. It provides an easy way to extend the capabilities of AI agents, assistants, and copilots with custom actions written in Python. Users can create and deploy tools, skills, loaders, and plugins that securely connect any AI Assistant platform to their data and applications. The Robocorp Action Server makes Python scripts compatible with ChatGPT and LangChain by automatically creating and exposing an API based on function declaration, type hints, and docstrings. It simplifies the process of developing and deploying AI actions, enabling users to interact with AI frameworks effortlessly.
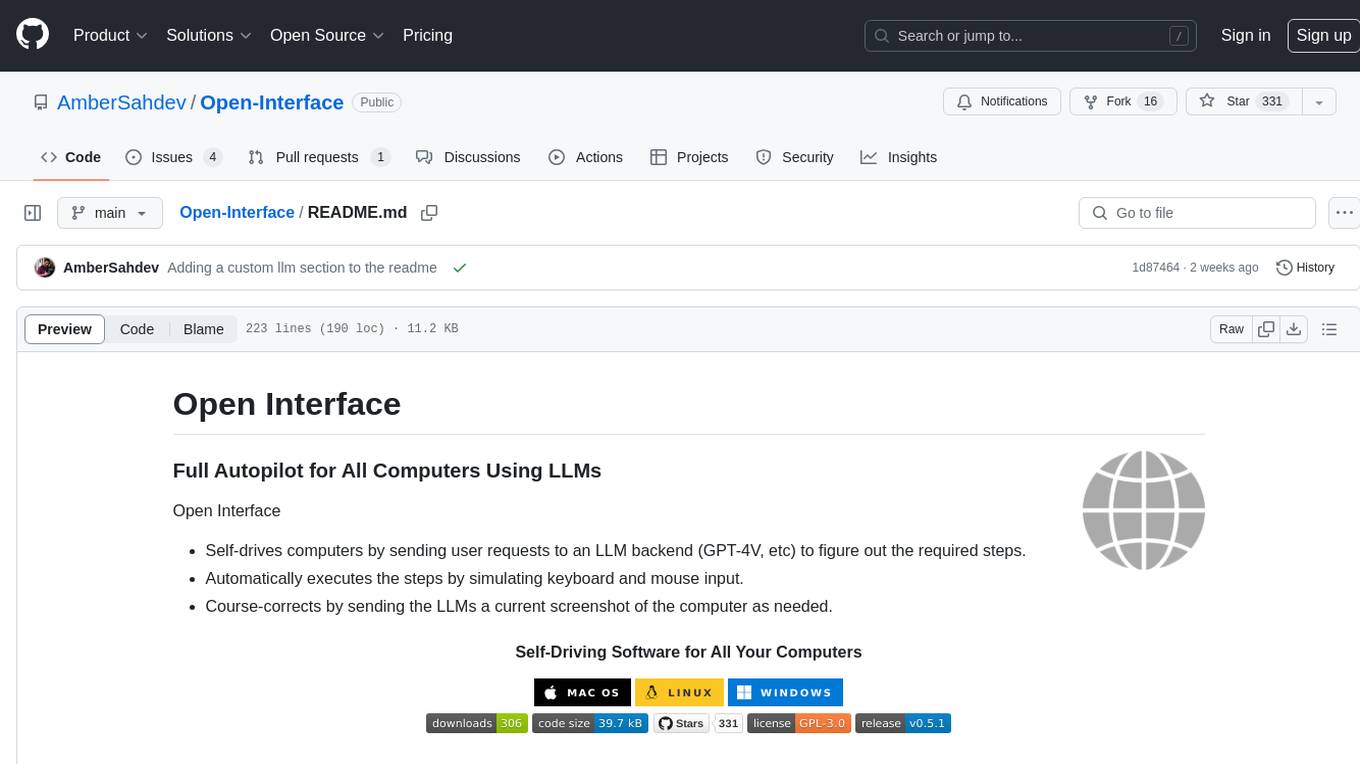
Open-Interface
Open Interface is a self-driving software that automates computer tasks by sending user requests to a language model backend (e.g., GPT-4V) and simulating keyboard and mouse inputs to execute the steps. It course-corrects by sending current screenshots to the language models. The tool supports MacOS, Linux, and Windows, and requires setting up the OpenAI API key for access to GPT-4V. It can automate tasks like creating meal plans, setting up custom language model backends, and more. Open Interface is currently not efficient in accurate spatial reasoning, tracking itself in tabular contexts, and navigating complex GUI-rich applications. Future improvements aim to enhance the tool's capabilities with better models trained on video walkthroughs. The tool is cost-effective, with user requests priced between $0.05 - $0.20, and offers features like interrupting the app and primary display visibility in multi-monitor setups.
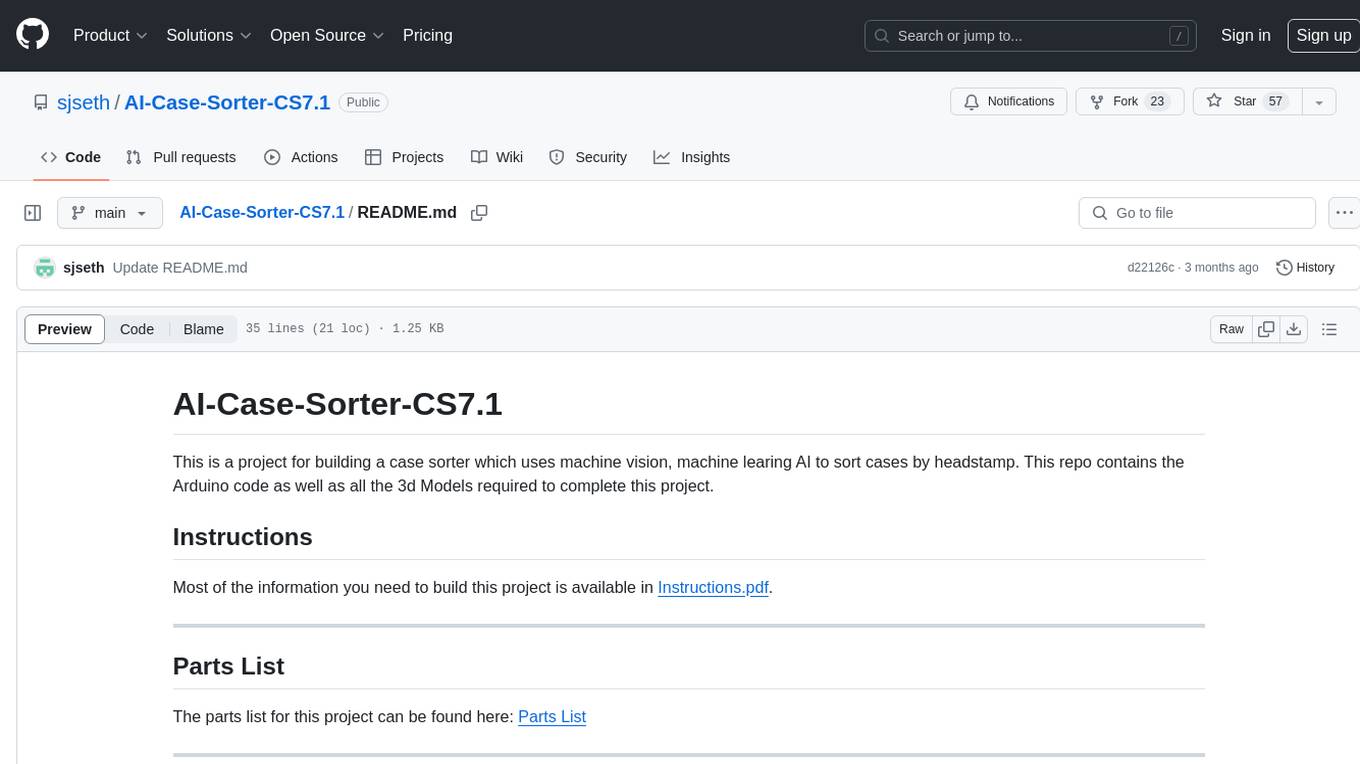
AI-Case-Sorter-CS7.1
AI-Case-Sorter-CS7.1 is a project focused on building a case sorter using machine vision and machine learning AI to sort cases by headstamp. The repository includes Arduino code and 3D models necessary for the project.