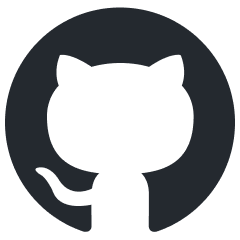
testzeus-hercules
Hercules is the worldβs first open-source testing agent, enabling UI, API, Security, Accessibility, and Visual validations β all without code or maintenance. Automate testing effortlessly and let Hercules handle the heavy lifting! β‘
Stars: 457
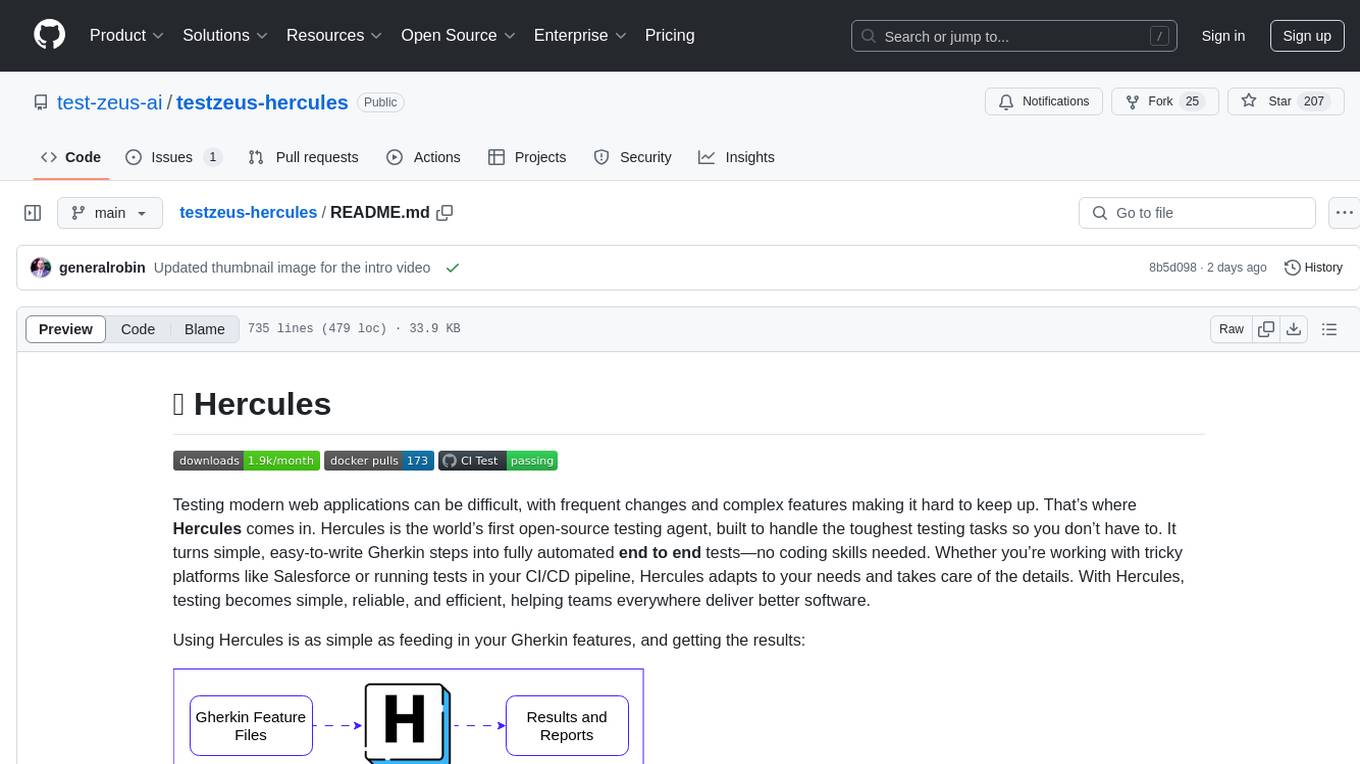
Hercules is the worldβs first open-source testing agent designed to handle the toughest testing tasks for modern web applications. It turns simple Gherkin steps into fully automated end-to-end tests, making testing simple, reliable, and efficient. Hercules adapts to various platforms like Salesforce and is suitable for CI/CD pipelines. It aims to democratize and disrupt test automation, making top-tier testing accessible to everyone. The tool is transparent, reliable, and community-driven, empowering teams to deliver better software. Hercules offers multiple ways to get started, including using PyPI package, Docker, or building and running from source code. It supports various AI models, provides detailed installation and usage instructions, and integrates with Nuclei for security testing and WCAG for accessibility testing. The tool is production-ready, open core, and open source, with plans for enhanced LLM support, advanced tooling, improved DOM distillation, community contributions, extensive documentation, and a bounty program.
README:
Testing modern web applications can be difficult, with frequent changes and complex features making it hard to keep up. That's where Hercules comes in. Hercules is the world's first open-source testing agent, built to handle the toughest testing tasks so you don't have to. It turns simple, easy-to-write Gherkin steps into fully automated end to end testsβno coding skills needed. Whether you're working with tricky platforms like Salesforce or running tests in your CI/CD pipeline, Hercules adapts to your needs and takes care of the details. With Hercules, testing becomes simple, reliable, and efficient, helping teams everywhere deliver better software. Here's a quick demo of lead creation using natural english language test (without any code):
As you saw, using Hercules is as simple as feeding in your Gherkin features, and getting the results:
At TestZeus, we believe that trustworthy and open-source code is the backbone of innovation. That's why we've built Hercules to be transparent, reliable, and community-driven.
Our mission? To democratize and disrupt test automation, making top-tier testing accessible to everyone, not just the elite few. No more gatekeepingβeveryone deserves a hero on their testing team!
Video Tutorials: @TestZeus
-
Introduction to TestZeus Hercules
Learn about the core features of TestZeus Hercules and how it can streamline end-to-end testing for your projects. -
Installation and Setup Guide
Step-by-step instructions for installing and configuring TestZeus Hercules in your environment.
Watch now
-
Creating BDD Test Cases
Learn how to write Behavior-Driven Development (BDD) test cases for Hercules and use dynamic testdata.
Watch now
-
Testing Multilingual content
Learn how Hercules interacts with web browsers to perform Testing on Multilingual content via Multilingual testcase.
Watch now
-
Enhancing Hercules with Community-Driven Tools
Discover how to customize Hercules and incorporate additional tools provided by the community.
Watch now
-
API testing all the way, new ways to do end to end
Watch now
-
Security Testing done end to end
Watch now
-
Using vision capabilities to check snapshots and components on the application
Watch now
Hercules offers multiple ways to get started, catering to different user preferences and requirements. If you are new to the Python ecosystem and don't know where to begin, dont worry and read the footnotes on understanding the basics.
For a quick taste of the solution, you can try the notebook here:
- Note: Colab might ask you to restart the session as python3.11 and some libs are installed during the installation of testzeus-hercules. Please restart the session if required and continue the execution. Also , we recommend one of the approaches below for getting the full flavor of the solution.
Install Hercules from PyPI:
pip install testzeus-hercules
Hercules uses Playwright to interact with web pages, so you need to install Playwright and its dependencies:
playwright install --with-deps
For detailed information about project structure and running tests, please refer to our Run Guide.
Once installed, you will need to provide some basic parameters to run Hercules:
-
--input-file INPUT_FILE
: Path to the input Gherkin feature file to be tested. -
--output-path OUTPUT_PATH
: Path to the output directory. The path of JUnit XML result and HTML report for the test run. -
--test-data-path TEST_DATA_PATH
: Path to the test data directory. The path where Hercules expects test data to be present; all test data used in feature testing should be present here. -
--project-base PROJECT_BASE
: Path to the project base directory. This is an optional parameter; if you populate this,--input-file
,--output-path
, and--test-data-path
are not required, and Hercules will assume all the three folders exist in the following format inside the project base:
PROJECT_BASE/
βββ gherkin_files/
βββ input/
β βββ test.feature
βββ log_files/
βββ output/
β βββ test.feature_result.html
β βββ test.feature_result.xml
βββ proofs/
β βββ User_opens_Google_homepage/
β βββ network_logs.json
β βββ screenshots/
β βββ videos/
βββ test_data/
βββ test_data.txt
-
--llm-model LLM_MODEL
: Name of the LLM model to be used by the agent (recommended isgpt-4o
, but it can take others). -
--llm-model-api-key LLM_MODEL_API_KEY
: API key for the LLM model, something likesk-proj-k.......
.
In addition to command-line parameters, Hercules supports various environment variables for configuration:
-
BROWSER_TYPE
: Type of browser to use (chromium
,firefox
,webkit
). Default:chromium
-
HEADLESS
: Run browser in headless mode (true
,false
). Default:true
-
BROWSER_RESOLUTION
: Browser window resolution (format:width,height
). Example:1920,1080
-
BROWSER_COOKIES
: Set cookies for the browser context. Format: JSON array of cookie objects. Example:[{"name": "session", "value": "123456", "domain": "example.com", "path": "/"}]
-
RECORD_VIDEO
: Record test execution videos (true
,false
). Default:true
-
TAKE_SCREENSHOTS
: Take screenshots during test (true
,false
). Default:true
For a complete list of environment variables, see our Environment Variables Guide.
After passing all the required parameters, the command to run Hercules should look like this:
testzeus-hercules --input-file opt/input/test.feature --output-path opt/output --test-data-path opt/test_data --llm-model gpt-4o --llm-model-api-key sk-proj-k.......
To set up and run Hercules on a Windows machine:
-
Open PowerShell in Administrator Mode:
- Click on the Start Menu, search for PowerShell, and right-click on Windows PowerShell.
- Select Run as Administrator to open PowerShell in administrator mode.
-
Navigate to the Helper Scripts Folder:
- Use the
cd
command to navigate to the folder containing thehercules_windows_setup.ps1
script. For example:cd path\to\helper_scripts
- Use the
-
Run the Setup Script:
- Execute the script to install and configure Hercules:
.\hercules_windows_setup.ps1
- Execute the script to install and configure Hercules:
-
Follow On-Screen Instructions:
- The script will guide you through installing Python, Playwright, FFmpeg, and other required dependencies.
-
Run Hercules:
- Once the setup is complete, you can run Hercules from PowerShell or Command Prompt using the following command:
testzeus-hercules --input-file opt/input/test.feature --output-path opt/output --test-data-path opt/test_data --llm-model gpt-4o --llm-model-api-key sk-proj-k.......
- Once the setup is complete, you can run Hercules from PowerShell or Command Prompt using the following command:
- Anthropic: Compatible with Haiku 3.5 and above.
- Groq: Supports any version with function calling and coding capabilities.
- Mistral: Supports any version with function calling and coding capabilities. Mistral-large, Mistral-medium. Only heavey models.
- OpenAI: Fully compatible with GPT-4o/o3-mini and above. Note: OpenAI GPT-4o-mini is only supported for sub-agents, for planner it is still recommended to use GPT-4o.
- Ollama: Supported with medium models and function calling. Heavy models only 70b and above.
- Gemini: [deprecated, because of flaky execution]. Refer: https://testzeuscommunityhq.slack.com/archives/C0828GV2HEC/p1740628636862819
- Deepseek: [deprecated, because of flaky execution]. Refer: https://testzeuscommunityhq.slack.com/archives/C0828GV2HEC/p1740628636862819
- Hosting: supported on AWS bedrock, GCP VertexAI, AzureAI. [tested models, OpenAI, Anthropic Sonet and Haiku, Llamma 60b above with function calling] Note: Kindly ensure that the model you are using can handle agentic activities like function calling. For example larger models like OpenAI GPT 4O, Llama >70B, Mistral large etc.
Upon running the command:
- Hercules will start and attempt to open a web browser (default is Chromium).
- It will prepare a plan of execution based on the feature file steps provided.
- The plan internally expands the brief steps mentioned in the feature file into a more elaborated version.
- Hercules detects assertions in the feature file and plans the validation of expected results with the execution happening during the test run.
- All the steps, once elaborated, are passed to different tools based on the type of execution requirement of the step. For example, if a step wants to click on a button and capture the feedback, it will be passed to the
click_using_selector
tool.
Once the execution is completed:
- Logs explaining the sequence of events are generated.
- The best place to start is the
output-path
, which will have the JUnit XML result file as well as an HTML report regarding the test case execution. - You can also find proofs of execution such as video recordings, screenshots per event, and network logs in the
proofs
folder. - To delve deeper and understand the chain of thoughts, refer to the
chat_messages.json
in thelog_files
. This will have exact steps that were planned by the agent.
Here's a sample feature file:
Feature: Account Creation in Salesforce
Scenario: Successfully create a new account
Given I am on the Salesforce login page
When I enter my username "[email protected]" and password "securePassword"
And I click on the "Log In" button
And I navigate to the "Accounts" tab
And I click on the "New" button
And I fill in the "Account Name" field with "Test Account"
And I select the "Account Type" as "Customer"
And I fill in the "Website" field with "www.testaccount.com"
And I fill in the "Phone" field with "123-456-7890"
And I click on the "Save" button
Then I should see a confirmation message "Account Test Account created successfully"
And I should see "Test Account" listed in the account records
For all the scale lovers, Hercules is also available as a Docker image.
docker pull testzeus/hercules:latest
Run the container using:
docker run --env-file=.env \
-v ./agents_llm_config.json:/testzeus-hercules/agents_llm_config.json \
-v ./opt:/testzeus-hercules/opt \
--rm -it testzeus/hercules:latest
-
Environment Variables: All the required environment variables can be set by passing an
.env
file to thedocker run
command. -
LLM Configuration: If you plan to have complete control over Hercules and which LLM to use beyond the ones provided by OpenAI, you can pass
agents_llm_config.json
as a mount to the container. This is for advanced use cases and is not required for beginners. Refer to sample files.env-example
andagents_llm_config-example.json
for details and reference. -
Mounting Directories: Mount the
opt
folder to the Docker container so that all the inputs can be passed to Hercules running inside the container, and the output can be pulled out for further processing. The repository has a sampleopt
folder that can be mounted easily. -
Simplified Parameters: In the Docker case, there is no need for using
--input-file
,--output-path
,--test-data-path
, or--project-base
as they are already handled by mounting theopt
folder in thedocker run
command.
- While running in Docker mode, understand that Hercules has access only to a headless web browser.
- If you want Hercules to connect to a visible web browser, try the CDP URL option in the environment file. This option can help you connect Hercules running in your infrastructure to a remote browser like BrowserBase or your self-hosted grid.
- Use
CDP_ENDPOINT_URL
to set the CDP URL of the Chrome instance that has to be connected to the agent.
When running Hercules in Docker, you can connect to remote browser instances using various platforms:
- BrowserStack Integration:
export BROWSERSTACK_USERNAME=your_username
export BROWSERSTACK_ACCESS_KEY=your_access_key
export CDP_ENDPOINT_URL=$(python helper_scripts/browser_stack_generate_cdp_url.py)
- LambdaTest Integration:
export LAMBDATEST_USERNAME=your_username
export LAMBDATEST_ACCESS_KEY=your_access_key
export CDP_ENDPOINT_URL=$(python helper_scripts/lambda_test_generate_cdp_url.py)
- BrowserBase Integration:
export CDP_ENDPOINT_URL=wss://connect.browserbase.com?apiKey=your_api_key
- AnchorBrowser Integration:
export CDP_ENDPOINT_URL=wss://connect.anchorbrowser.io?apiKey=your_api_key
Note: Video recording is only supported on platforms that use connect_over_cdp (BrowserBase, AnchorBrowser). Platforms using the connect API (BrowserStack, LambdaTest) do not support video recording.
After the command completion:
- The container terminates, and output is written in the mounted
opt
folder, in the same way as described in the directory structure. - You will find the JUnit XML result file, HTML reports, proofs of execution, and logs in the respective folders.
For the hardcore enthusiasts, you can use Hercules via the source code to get a complete experience of customization and extending Hercules with more tools.
- Ensure you have Python 3.11 installed on your system.
-
Clone the Repository
git clone [email protected]:test-zeus-ai/testzeus-hercules.git
-
Navigate to the Directory
cd testzeus-hercules
-
Use Make Commands
The repository provides handy
make
commands.- Use
make help
to check out possible options.
- Use
-
Install Poetry
make setup-poetry
-
Install Dependencies
make install
-
Run Hercules
make run
-
This command reads the relevant feature files from the
opt
folder and executes them, putting the output in the same folder. -
The
opt
folder has the following format:opt/ βββ input/ β βββ test.feature βββ output/ β βββ test.feature_result.html β βββ test.feature_result.xml βββ log_files/ βββ proofs/ β βββ User_opens_Google_homepage/ β βββ network_logs.json β βββ screenshots/ β βββ videos/ βββ test_data/ βββ test_data.txt
-
-
Interactive Mode
You can also run Hercules in interactive mode as an instruction execution agent, which is more useful for RPA and debugging test cases and Hercules's behavior on new environments while building new tooling and extending the agents.
make run-interactive
- This will trigger an input prompt where you can chat with Hercules, and it will perform actions based on your commands.
For those who want a fully automated setup experience on Linux/macOS environments, we provide a helper_script.sh. This script installs Python 3.11 (if needed), creates a virtual environment, installs TestZeus Hercules, and sets up the base project directories in an opt folder.
- Ensure you have Python 3.11 installed on your system.
- Download or Create the Script You can copy the script below into a file named helper_script_custom.sh:
#!/bin/bash
# set -ex
# curl -sS https://bootstrap.pypa.io/get-pip.py | python3.11
# Create a new Python virtual environment named 'test'
python3.11 -m venv test
# Activate the virtual environment
source test/bin/activate
# Upgrade the 'testzeus-hercules' package
pip install --upgrade testzeus-hercules
playwright install --with-deps
# create a new directory named 'opt'
mkdir -p opt/input opt/output opt/test_data
# download https://raw.githubusercontent.com/test-zeus-ai/testzeus-hercules/refs/heads/main/agents_llm_config-example.json
curl -sS https://raw.githubusercontent.com/test-zeus-ai/testzeus-hercules/main/agents_llm_config-example.json > agents_llm_config-example.json
mv agents_llm_config-example.json agents_llm_config.json
# prompt user that they need to edit the 'agents_llm_config.json' file, halt the script and open the file in an editor
echo "Please edit the 'agents_llm_config.json' file to add your API key and other configurations."
# halt the script and mention the absolute path of the agents_llm_config.json file so that user can edit it in the editor
echo "The 'agents_llm_config.json' file is located at $(pwd)/agents_llm_config.json"
read -p "Press Enter if file is updated"
# download https://raw.githubusercontent.com/test-zeus-ai/testzeus-hercules/blob/main/.env-example
curl -sS https://raw.githubusercontent.com/test-zeus-ai/testzeus-hercules/main/.env-example > .env-example
mv .env-example .env
# prompt user that they need to edit the .env file, halt the script and open the file in an editor
echo "The '.env' file is located at $(pwd)/.env"
read -p "Press Enter if file is updated"
# create an input/test.feature file
# download https://raw.githubusercontent.com/test-zeus-ai/testzeus-hercules/refs/heads/main/opt/input/test.feature and save in opt/input/test.feature
curl -sS https://raw.githubusercontent.com/test-zeus-ai/testzeus-hercules/main/opt/input/test.feature > opt/input/test.feature
# download https://raw.githubusercontent.com/test-zeus-ai/testzeus-hercules/refs/heads/main/opt/test_data/test_data.json and save in opt/test_data/test_data.json
curl -sS https://raw.githubusercontent.com/test-zeus-ai/testzeus-hercules/main/opt/test_data/test_data.json > opt/test_data/test_data.json
# Run the 'testzeus-hercules' command with the specified parameters
testzeus-hercules --project-base=opt
- Make the Script Executable and Run
chmod +x helper_script.sh
./helper_script.sh
- The script will: β’ Create a Python 3.11 virtual environment named test. β’ Install testzeus-hercules and Playwright dependencies. β’ Create the opt folder structure (for input/output/test data). β’ Download sample config files: agents_llm_config.json, .env, and example feature/test data files. β’ Important: You will be prompted to edit both agents_llm_config.json and .env files. After you've added your API keys and other custom configurations, press Enter to continue.
-
Script Output
- After completion, the script automatically runs testzeus-hercules --project-base=opt.
- Your logs and results will appear in opt/output, opt/log_files, and opt/proofs.
For a comprehensive guide to all environment variables and configuration options available in TestZeus Hercules, please refer to our Environment Variables and Configuration Guide. This document provides detailed information about core environment variables, LLM configuration, browser settings, testing configuration, device configuration, logging options, and more.
To disable telemetry, set the TELEMETRY_ENABLED
environment variable to 0
:
export TELEMETRY_ENABLED=0
If AUTO_MODE
is set to 1
, Hercules will not request an email during the run:
export AUTO_MODE=1
To configure Hercules in detail:
-
Copy the base environment file:
cp .env-example .env
-
Hercules is capable of running in two configuration forms:
-
Using single LLM for all work
- For all the activities within the agent, initialize
LLM_MODEL_NAME
andLLM_MODEL_API_KEY
. - If using a non-OpenAI hosted solution but still OpenAI LLMs (something like OpenAI via Groq), then pass the
LLM_MODEL_BASE_URL
URL as well.
- For all the activities within the agent, initialize
-
Custom LLMs for different work or using hosted LLMs
- If you plan to configure local LLMs or non-OpenAI LLMs, use the other parameters like
AGENTS_LLM_CONFIG_FILE
andAGENTS_LLM_CONFIG_FILE_REF_KEY
. - These are powerful options and can affect the quality of Hercules outputs.
- If you plan to configure local LLMs or non-OpenAI LLMs, use the other parameters like
-
-
Hercules considers a base folder that is by default
./opt
but can be changed by the environment variablePROJECT_SOURCE_ROOT
. -
Connecting to an Existing Chrome Instance
- This is extremely useful when you are running Hercules in Docker for scale.
- You can connect Hercules running in your infrastructure to a remote browser like BrowserBase or your self-hosted grid.
- Use
CDP_ENDPOINT_URL
to set the CDP URL of the Chrome instance that has to be connected to the agent.
-
Controlling Other Behaviors
You can control other behaviors of Hercules based on the following environment variables:
HEADLESS=true
RECORD_VIDEO=false
TAKE_SCREENSHOTS=false
-
BROWSER_TYPE=chromium
(options:firefox
,chromium
) CAPTURE_NETWORK=false
For example: If you would like to run with a "Headful" browser, you can set the environment variable with export HEADLESS=false
before triggering Hercules.
-
How to Use Tracing in Playwright
Tracing in Playwright allows you to analyze test executions and debug issues effectively. To enable tracing in your Playwright tests, follow these steps:
- Ensure that tracing is enabled in the configuration.
- Traces will be saved to the specified path:
{proof_path}/traces/trace.zip
.
To enable tracing, set the following environment variable:
export ENABLE_PLAYWRIGHT_TRACING=true
-
It's a list of configurations of LLMs that you want to provide to the agent.
-
Example:
{ "anthropic": { "planner_agent": { "model_name": "claude-3-5-haiku-latest", "model_api_key": "", "model_api_type": "anthropic", "llm_config_params": { "cache_seed": null, "temperature": 0.0, "top_p": 0.001, "seed":12345 } }, "nav_agent": { "model_name": "claude-3-5-haiku-latest", "model_api_key": "", "model_api_type": "anthropic", "llm_config_params": { "cache_seed": null, "temperature": 0.0, "top_p": 0.001, "seed":12345 } } } }
-
The key is the name of the spec that is passed in
AGENTS_LLM_CONFIG_FILE_REF_KEY
, whereas the Hercules information is passed in sub-dictsplanner_agent
andnav_agent
. -
Note: This option should be ignored until you are sure what you are doing. Discuss with us while playing around with these options in our Slack communication. Join us at our Slack
Hercules is production ready, and packs a punch with features:
Hercules makes testing as simple as Gherkin in, results out. Just feed your end-to-end tests in Gherkin format, and watch Hercules spring into action. It takes care of the heavy lifting by running your tests automatically and presenting results in a neat JUnit format. No manual steps, no fussβjust efficient, seamless testing.
With Hercules, you're harnessing the power of open source with zero licensing fees. Feel free to dive into the code, contribute, or customize it to your heart's content. Hercules is as free as it is mighty, giving you the flexibility and control you need.
Built to handle the most intricate UIs, Hercules conquers Salesforce and other complex platforms with ease. Whether it's complicated DOM or running your SOQL or Apex, Hercules is ready and configurable.
Say goodbye to complex scripts and elusive locators. Hercules is here to make your life easier with its no-code approach, taking care of the automation of Gherkin features so you can focus on what matters mostβbuilding quality software.
With multilingual support right out of the box, Hercules is ready to work with teams across the globe. Built to bridge language gaps, it empowers diverse teams to collaborate effortlessly on a unified testing platform.
Hercules records video of the execution, and captures network logs as well, so that you dont have to deal with "It works on my computer".
Autonomous and adaptive, Hercules takes care of itself with auto-healing capabilities. Forget about tedious maintenanceβHercules adjusts to changes and stays focused on achieving your testing goals.
Grounded in the powerful foundations of TestZeus, Hercules tackles UI assertions with unwavering focus, ensuring that no assertion goes unchecked and no bug goes unnoticed. It's thorough, it's sharp, and it's ready for action.
Run Hercules locally or integrate it seamlessly into your CI/CD pipeline. Docker-native and one-command ready, Hercules fits smoothly into your deployment workflows, keeping testing quick, consistent, and hassle-free.
With Hercules, testing is no longer just a step in the processβit's a powerful, streamlined experience that brings quality to the forefront.
Hercules supports running the browser in "mobile mode" for a variety of device types. Playwright provides a large list of device descriptors here: List of mobile devices supported
Set the RUN_DEVICE environment variable in your .env (or directly as an environment variable):
RUN_DEVICE="iPhone 15 Pro Max"
When Hercules runs, it will now launch the browser with the corresponding viewport and user-agent for the chosen device, simulating a real mobile environment.
Here's a quick demo:
Hercules can be extended with more powerful tools for advanced scenarios. Enable it by setting:
LOAD_EXTRA_TOOLS=true
With LOAD_EXTRA_TOOLS enabled, Hercules looks for additional tool modules that can expand capabilities (e.g., geolocation handling).
For location-based tests, configure: supported geo providers are maps.co and google
GEO_API_KEY=somekey
GEO_PROVIDER=maps_co
This allows Hercules to alter or simulate user location during test execution, broadening your test coverage for scenarios that rely on user geography.
Note: If you are looking for native app test automation, we've got you covered, as we've built out early support for Appium powered native app automation here
Hercules leverages a multi-agent architecture based on the AutoGen framework. Building on the foundation provided by the AutoGen framework, Hercules's architecture leverages the interplay between tools and agents. Each tool embodies an atomic action, a fundamental building block that, when executed, returns a natural language description of its outcome. This granularity allows Hercules to flexibly assemble these tools to tackle complex web automation workflows.
The diagram above shows the configuration chosen on top of AutoGen architecture. The tools can be partitioned differently, but this is the one that we chose for the time being. We chose to use tools that map to what humans learn about the web browser rather than allow the LLM to write code as it pleases. We see the use of configured tools to be safer and more predictable in its outcomes. Certainly, it can click on the wrong things, but at least it is not going to execute malicious unknown code.
At the moment, there are two agents:
- Planner Agent: Executes the planning and decomposition of tasks.
- Browser Navigation Agent: Embodies all the tools for interacting with the web browser.
At the core of Hercules's capabilities is the Tools Library, a repository of well-defined actions that Hercules can perform; for now, web actions. These tools are grouped into two main categories:
-
Sensing Tools: Tools like
get_dom_with_content_type
andgeturl
that help Hercules understand the current state of the webpage or the browser. -
Action Tools: Tools that allow Hercules to interact with and manipulate the web environment, such as
click
,enter_text
, andopenurl
.
Each tool is created with the intention to be as conversational as possible, making the interactions with LLMs more intuitive and error-tolerant. For instance, rather than simply returning a boolean value, a tool might explain in natural language what happened during its execution, enabling the LLM to better understand the context and correct course if necessary.
-
Sensing Tools
-
geturl
: Fetches and returns the current URL. -
get_dom_with_content_type
: Retrieves the HTML DOM of the active page based on the specified content type.-
text_only
: Extracts the inner text of the HTML DOM. Responds with text output. -
input_fields
: Extracts the interactive elements in the DOM (button, input, textarea, etc.) and responds with a compact JSON object. -
all_fields
: Extracts all the fields in the DOM and responds with a compact JSON object.
-
-
get_user_input
: Provides the orchestrator with a mechanism to receive user feedback to disambiguate or seek clarity on fulfilling their request.
-
-
Action Tools
-
click
: Given a DOM query selector, this will click on it. -
enter_text
: Enters text in a field specified by the provided DOM query selector. -
enter_text_and_click
: Optimized method that combinesenter_text
andclick
tools. -
bulk_enter_text
: Optimized method that wrapsenter_text
method so that multiple text entries can be performed in one shot. -
openurl
: Opens the given URL in the current or new tab.
-
Hercules's approach to managing the vast landscape of HTML DOM is methodical and essential for efficiency. We've introduced DOM Distillation to pare down the DOM to just the elements pertinent to the user's task.
In practice, this means taking the expansive DOM and delivering a more digestible JSON snapshot. This isn't about just reducing size; it's about honing in on relevance, serving the LLMs only what's necessary to fulfill a request. So far, we have three content types:
- Text Only: For when the mission is information retrieval, and the text is the target. No distractions.
- Input Fields: Zeroing in on elements that call for user interaction. It's about streamlining actions.
- All Content: The full scope of distilled DOM, encompassing all elements when the task demands a comprehensive understanding.
It's a surgical procedure, carefully removing extraneous information while preserving the structure and content needed for the agent's operation. Of course, with any distillation, there could be casualties, but the idea is to refine this over time to limit/eliminate them.
Since we can't rely on all web page authors to use best practices, such as adding unique IDs to each HTML element, we had to inject our own attribute (md
) in every DOM element. We can then guide the LLM to rely on using md
in the generated DOM queries.
To cut down on some of the DOM noise, we use the DOM Accessibility Tree rather than the regular HTML DOM. The accessibility tree, by nature, is geared towards helping screen readers, which is closer to the mission of web automation than plain old HTML DOM.
The distillation process is a work in progress. We look to refine this process and condense the DOM further, aiming to make interactions faster, cost-effective, and more accurate.
Hercules integrates with Nuclei to automate vulnerability scanning directly from Gherkin test cases, identifying issues like misconfigurations, OWASP Top 10 vulnerabilities, and API flaws. Security reports are generated alongside testing outputs for seamless CI/CD integration.
Hercules supports WCAG 2.0, 2.1, and 2.2 at A, AA, and AAA levels, enabling accessibility testing to ensure compliance with global standards. It identifies accessibility issues early, helping build inclusive and user-friendly applications.
We wanted to ensure that Hercules stands up to the task of end-to-end testing with immense precision. So, we have run Hercules through a wide range of tests such as running APIs, interacting with complex UI scenarios, clicking through calendars, or iframes. A full list of evaluations can be found in the tests folder.
To run the full test suite, use the following command:
make test
To run a specific test:
make test-case
Hercules builds on the work done by WebArena and Agent-E, and beyond that, to iron out the issues in the previous, we have written our own test cases catering to complex QA scenarios and have created tests in the ./tests
folder.
We believe that great quality comes from opinions about a product. So we have incorporated a few of our opinions into the product design. We welcome the community to question them, use them, or build on top of them. Here are some examples:
-
Gherkin is a Good Enough Format for Agents: Gherkin provides a semi-structured format for the LLMs/AI Agents to follow test intent and user instructions. It provides the right amount of grammar (verbs like Given, When, Then) for humans to frame a scenario and agents to follow the instructions.
-
Tests Should Be Atomic in Nature: Software tests should be atomic because it ensures that each test is focused, independent, and reliable. Atomic tests target one specific behavior or functionality, making it easier to pinpoint the root cause of failures without ambiguity.
Here's a good example (Atomic Test):
Feature: User Login Scenario: Successful login with valid credentials Given the user is on the login page When the user enters valid credentials And the user clicks the login button Then the user should see the dashboard
A non-atomic test confuses both the tester and the AI agent.
-
Open Core and Open Source: Hercules is built on an open-core model, combining the spirit of open source with the support and expertise of a commercial company, TestZeus. By providing Hercules as open source (licensed under AGPL v3), TestZeus is committed to empowering the testing community with a robust, adaptable tool that's freely accessible and modifiable. Open source offers transparency, trust, and collaborative potential, allowing anyone to dive into the code, contribute, and shape the project's direction.
-
Telemetry : All great products are built on good feedback. We have setup telemetry so that we can take feedback, without disturbing the user. Telemetry is enabled by default, but we also believe strongly in the values of "Trust" and "Transparency" so it can be turned off by the users.
-
Prompting Hercules : A detailed guide to write tests for Hercules can be found on our blog here .
Hercules is an AI-native solution and relies on LLMs to perform reasoning and actions. Based on our experiments, we have found that a complex use case as below could cost up to $0.20 using OpenAI's APIs gpt-4o, check the properties printed in testcase output to calculate for your testcase:
Feature: Account Creation in Salesforce
Scenario: Successfully create a new account
Given I am on the Salesforce login page
When I enter my username "[email protected]" and password "securePassword"
And I click on the "Log In" button
And I navigate to the "Accounts" tab
And I click on the "New" button
And I fill in the "Account Name" field with "Test Account"
And I select the "Account Type" as "Customer"
And I fill in the "Website" field with "www.testaccount.com"
And I fill in the "Phone" field with "123-456-7890"
And I click on the "Save" button
Then I should see a confirmation message "Account Test Account created successfully"
And I should see "Test Account" listed in the account records
Hercules isn't just another testing toolβit's an agent. Powered by synthetic intelligence that can think, reason, and react based on requirements, Hercules goes beyond simple automation scripts. We bring an industry-first approach to open-source agents for software testing. This means faster, smarter, and more resilient testing cycles, especially for complex platforms.
With industry-leading performance and a fully open-source foundation, Hercules combines powerful capabilities with community-driven flexibility, making top-tier testing accessible and transformative for everyone.
- Enhanced LLM Support: Integration with more LLMs and support for local LLM deployments.
- Advanced Tooling: Addition of more tools to handle complex testing scenarios and environments.
- Improved DOM Distillation: Refinements to the DOM distillation process for better efficiency and accuracy.
- Community Contributions: Encourage and integrate community-driven features and tools.
- Extensive Documentation: Expand documentation for better onboarding and usage.
- Bounty Program: Launch a bounty program to incentivize contributions.
We welcome contributions from the community!
- Read the CONTRIBUTING.md file to get started.
- Bounty Program: Stay tuned for upcoming opportunities! π
-
Developing Tools
- If you are developing tools for Hercules and want to contribute to the community, make sure you place the new tools in the
additional_tools
folder in your Pull Request.
- If you are developing tools for Hercules and want to contribute to the community, make sure you place the new tools in the
-
Fixes and Enhancements
- If you have a fix on sensing tools that are fundamental to the system or something in prompts or something in the DOM distillation code, then put the changes in the relevant file and share the Pull Request.
-
Creating a New Tool
- You can start extending by adding tools to Hercules.
- Refer to
testzeus_hercules/core/tools/sql_calls.py
as an example of how to create a new tool. - The key is the decorator
@tool
over the method that you want Hercules to execute. - The tool decorator should have a very clear description and name so that Hercules knows how to use the tool.
- Also, in the method, you should be clear with annotations on what parameter is used for what purpose so that function calling in the LLM works best.
-
Adding the Tool
- Once you have created the new tools files in some folder path, you can pass the folder path to Hercules in the environment variable so that Hercules can read the new tools during the boot time and make sure that they are available during the execution.
- Use
ADDITIONAL_TOOL_DIRS
to pass the path of the new tools folder where you have kept the new files.
-
Direct Addition (Not Recommended)
-
In case you opt for adding the tools directly, then just put your new tools in the
testzeus_hercules/core/tools
path of the cloned repository. -
Note: This way is not recommended. We prefer you try to use the
ADDITIONAL_TOOL_DIRS
approach.
-
Join us at our Slack to connect with the community, ask questions, and contribute.
Hercules would not have been possible without the great work from the following sources:
The Hercules project is inferred and enhanced over the existing project of Agent-E. We have improved lots of cases to make it capable of doing testing, especially in the area of complex DOM navigation and iframes. We have also added new tools and abilities (like Salesforce navigation) to Hercules so that it can perform better work over the base framework we had picked.
Hercules also picks some inspiration from the legacy TestZeus repo here.
With Hercules, testing is no longer just a step in the processβit's a powerful, streamlined experience that brings quality to the forefront.
If you're coming from a Java or JavaScript background, working with Python might feel a bit different at firstβbut don't worry! Python's simplicity, combined with powerful tools like virtual environments, makes managing dependencies easy and efficient.
In Java, you might use tools like Maven or Gradle to manage project dependencies, or in JavaScript, you'd use npm
or yarn
. In Python, virtual environments serve a similar purpose. They allow you to create isolated spaces for your project's dependencies, avoiding conflicts with other Python projects on your system.
Think of it like a sandboxed environment where TestZeus Hercules and its dependencies live independently from other Python packages.
First, ensure Python 3.11 or higher is installed. You can verify this by running:
python --version
# or
python3 --version
If Python isn't installed, download it here or if you are on Windows, just follow the instructions here.
To set up a clean Python environment for your project:
# Create a virtual environment named 'venv'
python -m venv venv
# Activate the virtual environment
# On Windows:
venv\Scripts\activate
# On macOS/Linux:
source venv/bin/activate
You'll notice your terminal prompt changesβthis indicates the virtual environment is active.
To deactivate the virtual environment later, simply run:
deactivate
Once your virtual environment is activated, install the latest version of TestZeus Hercules directly from PyPI:
pip install testzeus-hercules
To update to the latest version, use:
pip install --upgrade testzeus-hercules
If you need to remove the package:
pip uninstall testzeus-hercules
To verify that TestZeus Hercules is installed and check its version:
pip list
-
pip
is likenpm
ormvn
βit's used for installing and managing Python packages. - Python's simplicity means fewer configuration filesβmost things can be done directly from the command line.
Now that TestZeus Hercules is installed and ready to go, dive into our documentation to learn how to create and run your first test cases with ease!
If you use Hercules in your research or project, please cite:
@software{testzeus_hercules2024,
author = {Agnihotri, Shriyansh and Gupta, Robin},
title = {Hercules: World's first open source testing agent},
year = {2024},
publisher = {GitHub},
url = {https://github.com/test-zeus-ai/testzeus-hercules/}
}
For Tasks:
Click tags to check more tools for each tasksFor Jobs:
Alternative AI tools for testzeus-hercules
Similar Open Source Tools
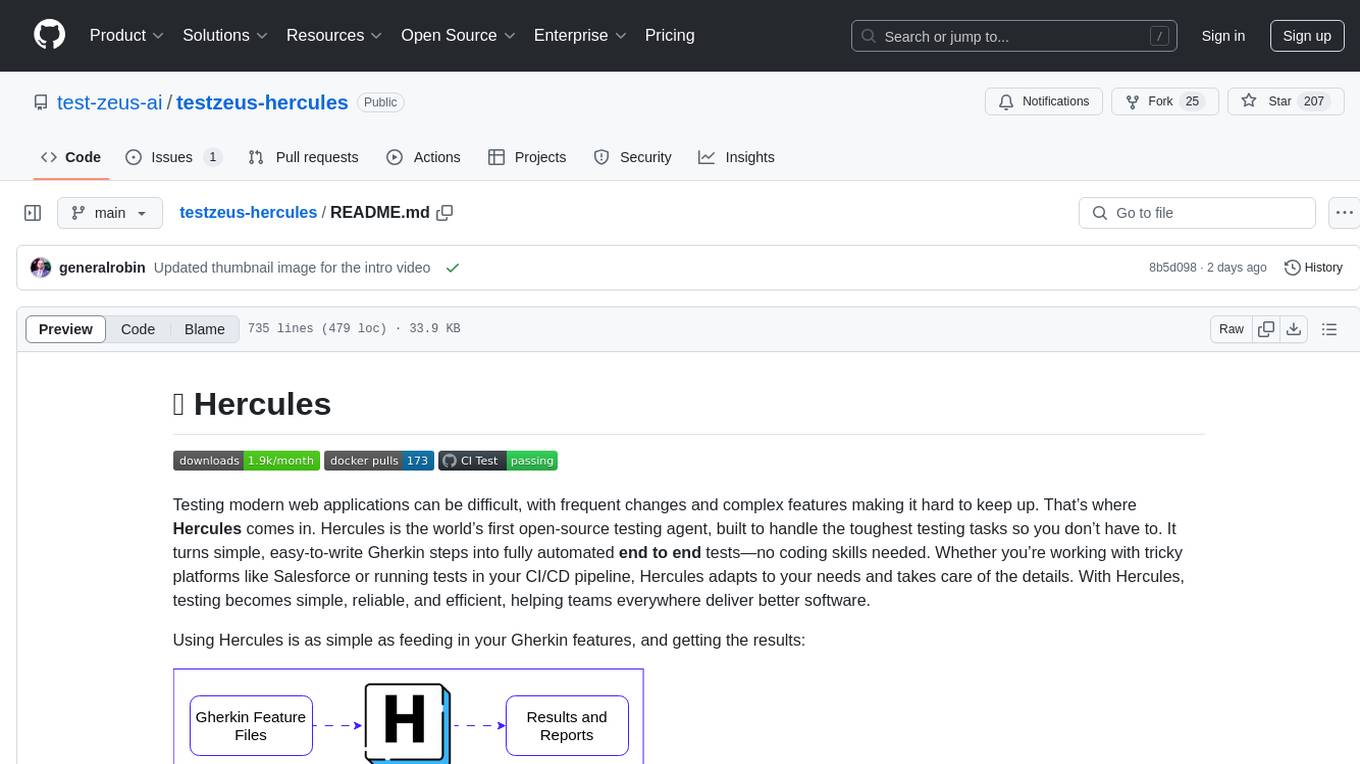
testzeus-hercules
Hercules is the worldβs first open-source testing agent designed to handle the toughest testing tasks for modern web applications. It turns simple Gherkin steps into fully automated end-to-end tests, making testing simple, reliable, and efficient. Hercules adapts to various platforms like Salesforce and is suitable for CI/CD pipelines. It aims to democratize and disrupt test automation, making top-tier testing accessible to everyone. The tool is transparent, reliable, and community-driven, empowering teams to deliver better software. Hercules offers multiple ways to get started, including using PyPI package, Docker, or building and running from source code. It supports various AI models, provides detailed installation and usage instructions, and integrates with Nuclei for security testing and WCAG for accessibility testing. The tool is production-ready, open core, and open source, with plans for enhanced LLM support, advanced tooling, improved DOM distillation, community contributions, extensive documentation, and a bounty program.
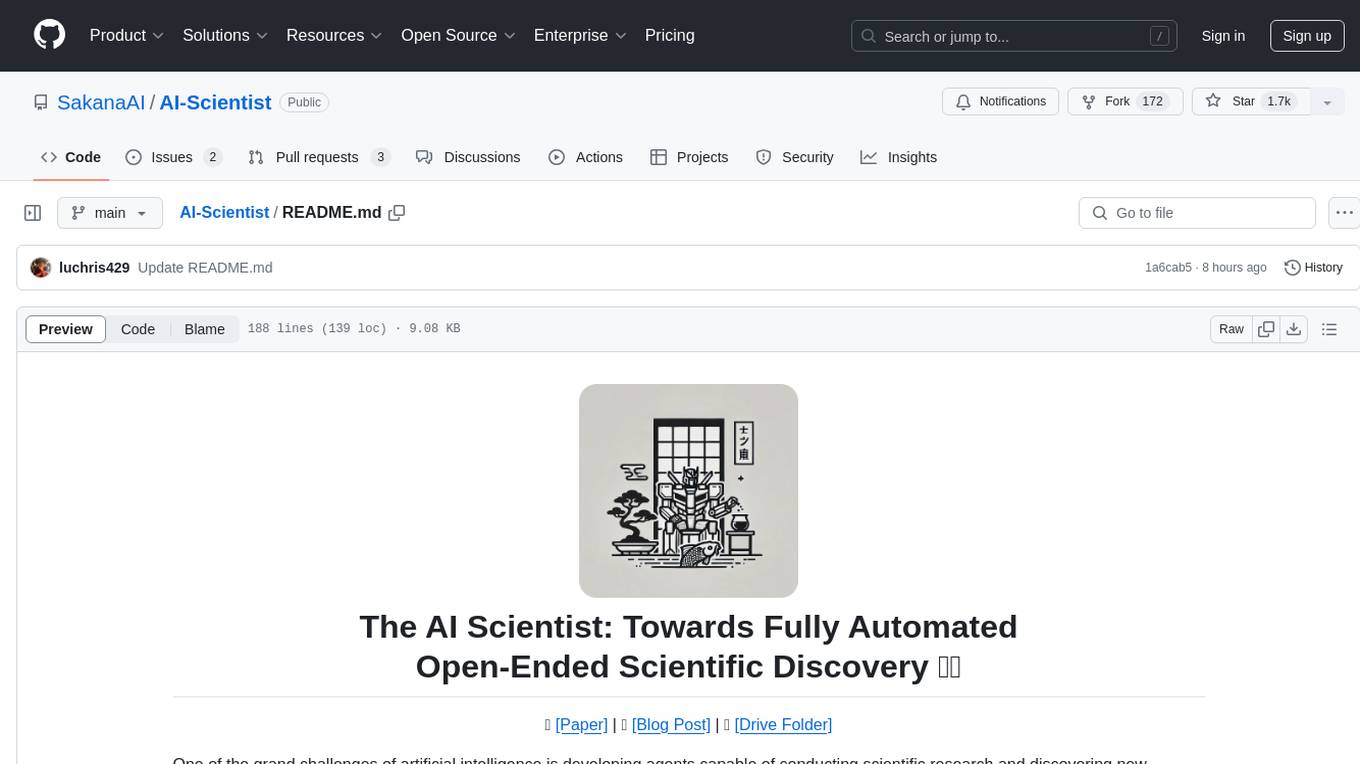
AI-Scientist
The AI Scientist is a comprehensive system for fully automatic scientific discovery, enabling Foundation Models to perform research independently. It aims to tackle the grand challenge of developing agents capable of conducting scientific research and discovering new knowledge. The tool generates papers on various topics using Large Language Models (LLMs) and provides a platform for exploring new research ideas. Users can create their own templates for specific areas of study and run experiments to generate papers. However, caution is advised as the codebase executes LLM-written code, which may pose risks such as the use of potentially dangerous packages and web access.
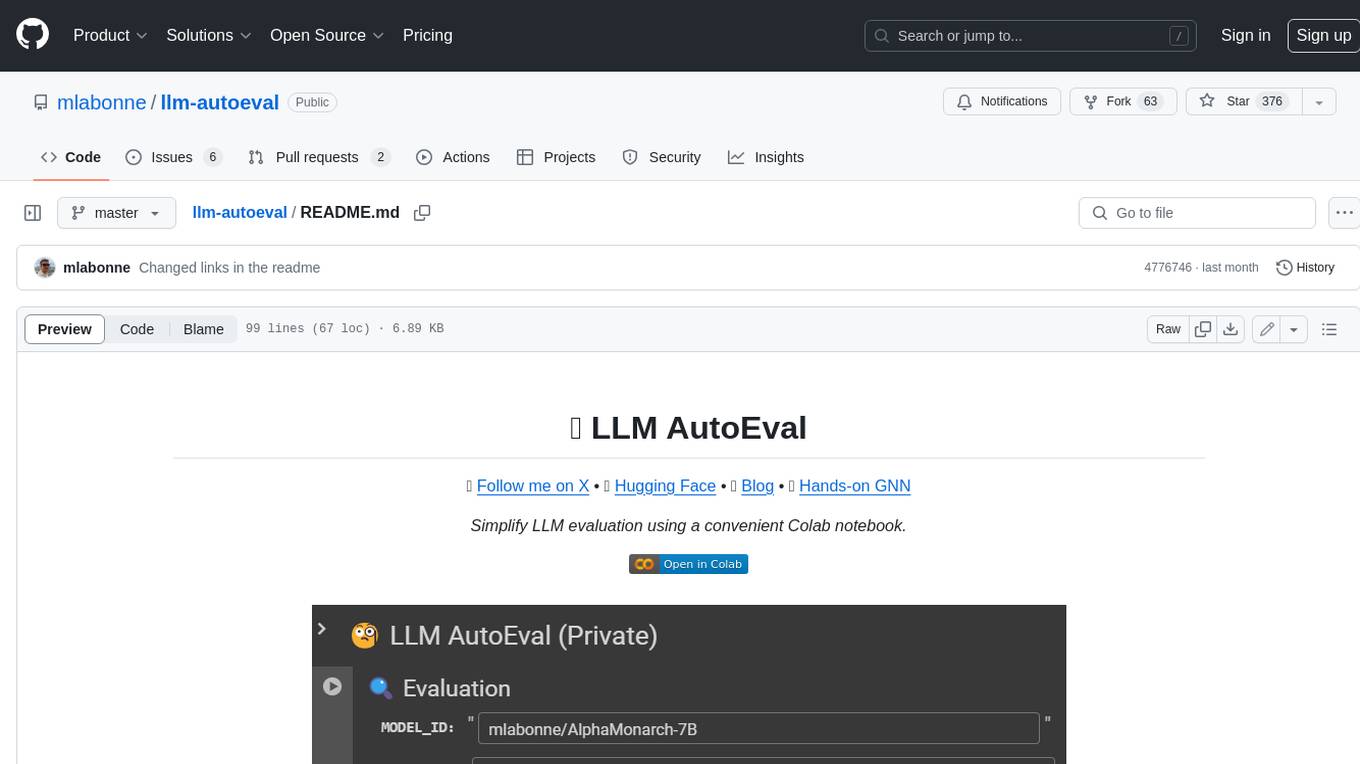
llm-autoeval
LLM AutoEval is a tool that simplifies the process of evaluating Large Language Models (LLMs) using a convenient Colab notebook. It automates the setup and execution of evaluations using RunPod, allowing users to customize evaluation parameters and generate summaries that can be uploaded to GitHub Gist for easy sharing and reference. LLM AutoEval supports various benchmark suites, including Nous, Lighteval, and Open LLM, enabling users to compare their results with existing models and leaderboards.
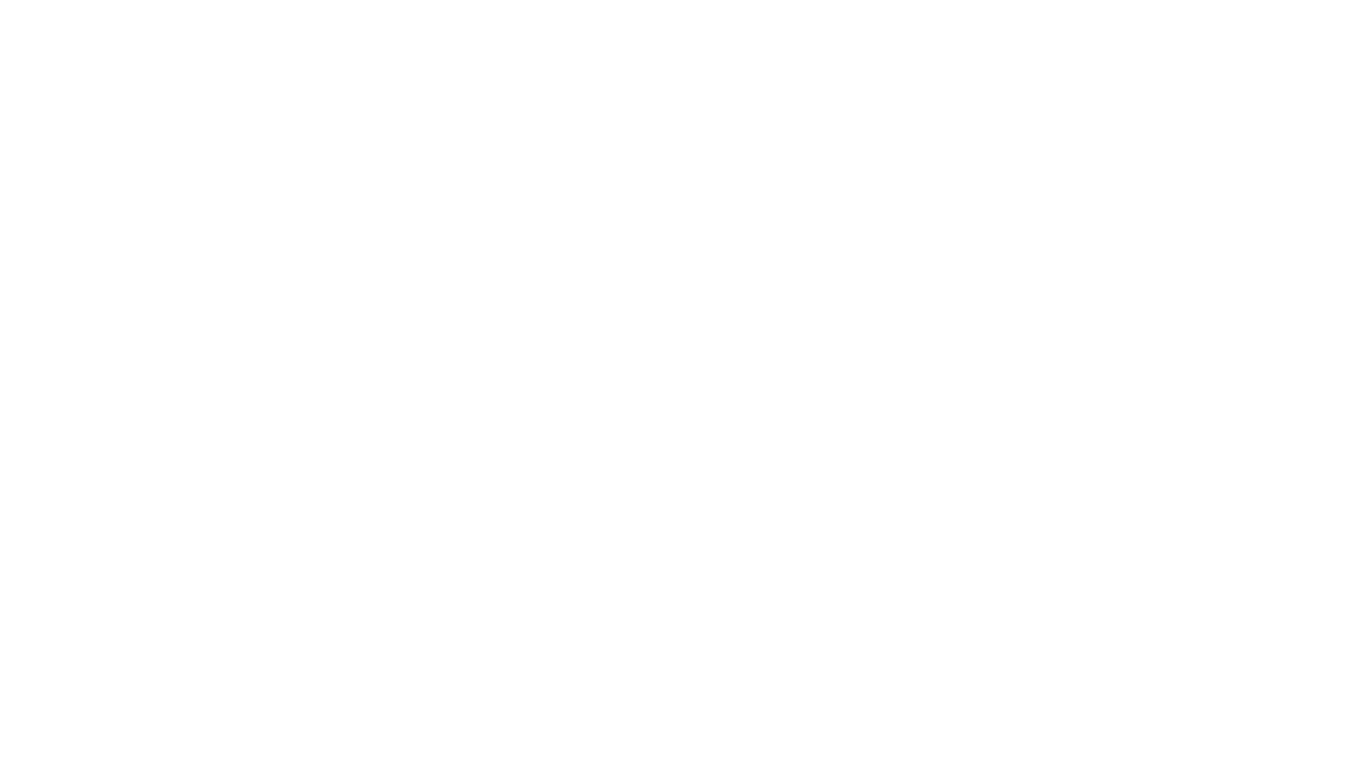
cognita
Cognita is an open-source framework to organize your RAG codebase along with a frontend to play around with different RAG customizations. It provides a simple way to organize your codebase so that it becomes easy to test it locally while also being able to deploy it in a production ready environment. The key issues that arise while productionizing RAG system from a Jupyter Notebook are: 1. **Chunking and Embedding Job** : The chunking and embedding code usually needs to be abstracted out and deployed as a job. Sometimes the job will need to run on a schedule or be trigerred via an event to keep the data updated. 2. **Query Service** : The code that generates the answer from the query needs to be wrapped up in a api server like FastAPI and should be deployed as a service. This service should be able to handle multiple queries at the same time and also autoscale with higher traffic. 3. **LLM / Embedding Model Deployment** : Often times, if we are using open-source models, we load the model in the Jupyter notebook. This will need to be hosted as a separate service in production and model will need to be called as an API. 4. **Vector DB deployment** : Most testing happens on vector DBs in memory or on disk. However, in production, the DBs need to be deployed in a more scalable and reliable way. Cognita makes it really easy to customize and experiment everything about a RAG system and still be able to deploy it in a good way. It also ships with a UI that makes it easier to try out different RAG configurations and see the results in real time. You can use it locally or with/without using any Truefoundry components. However, using Truefoundry components makes it easier to test different models and deploy the system in a scalable way. Cognita allows you to host multiple RAG systems using one app. ### Advantages of using Cognita are: 1. A central reusable repository of parsers, loaders, embedders and retrievers. 2. Ability for non-technical users to play with UI - Upload documents and perform QnA using modules built by the development team. 3. Fully API driven - which allows integration with other systems. > If you use Cognita with Truefoundry AI Gateway, you can get logging, metrics and feedback mechanism for your user queries. ### Features: 1. Support for multiple document retrievers that use `Similarity Search`, `Query Decompostion`, `Document Reranking`, etc 2. Support for SOTA OpenSource embeddings and reranking from `mixedbread-ai` 3. Support for using LLMs using `Ollama` 4. Support for incremental indexing that ingests entire documents in batches (reduces compute burden), keeps track of already indexed documents and prevents re-indexing of those docs.
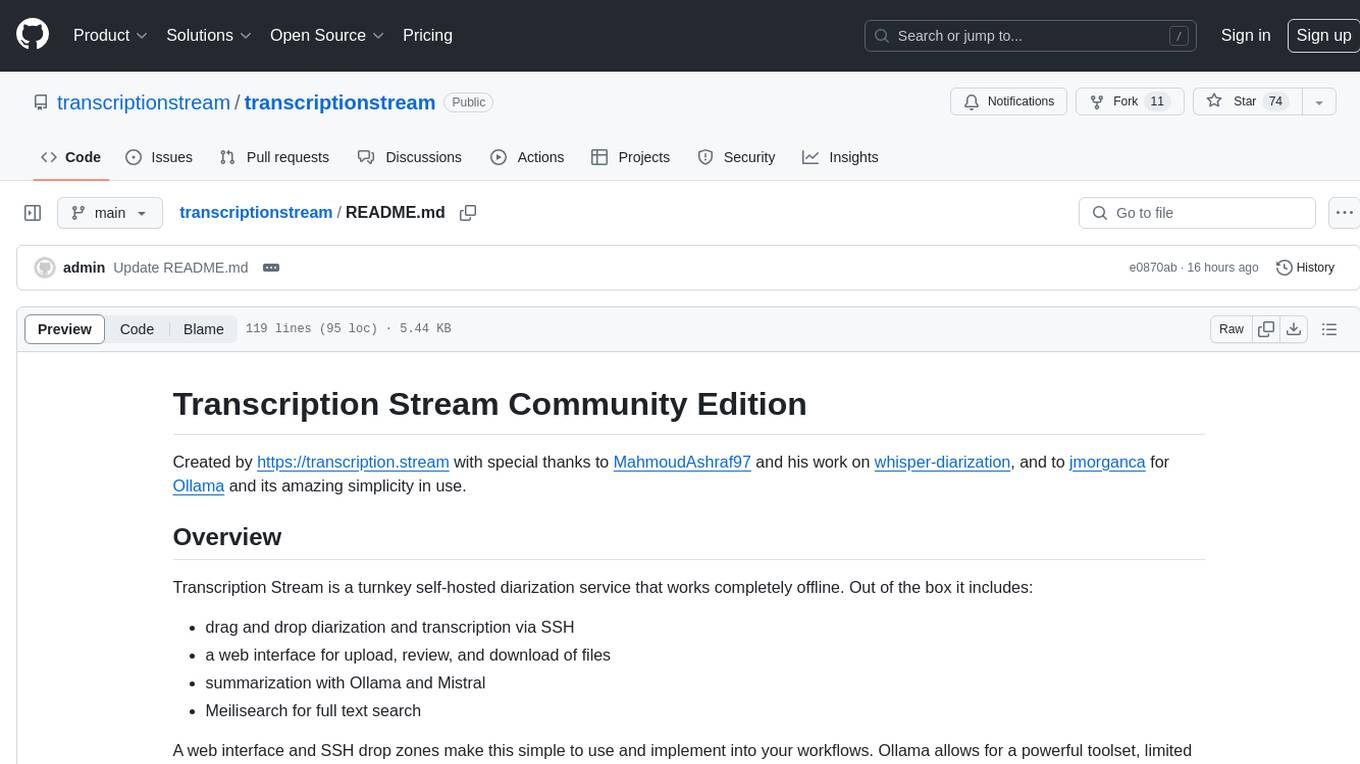
transcriptionstream
Transcription Stream is a self-hosted diarization service that works offline, allowing users to easily transcribe and summarize audio files. It includes a web interface for file management, Ollama for complex operations on transcriptions, and Meilisearch for fast full-text search. Users can upload files via SSH or web interface, with output stored in named folders. The tool requires a NVIDIA GPU and provides various scripts for installation and running. Ports for SSH, HTTP, Ollama, and Meilisearch are specified, along with access details for SSH server and web interface. Customization options and troubleshooting tips are provided in the documentation.
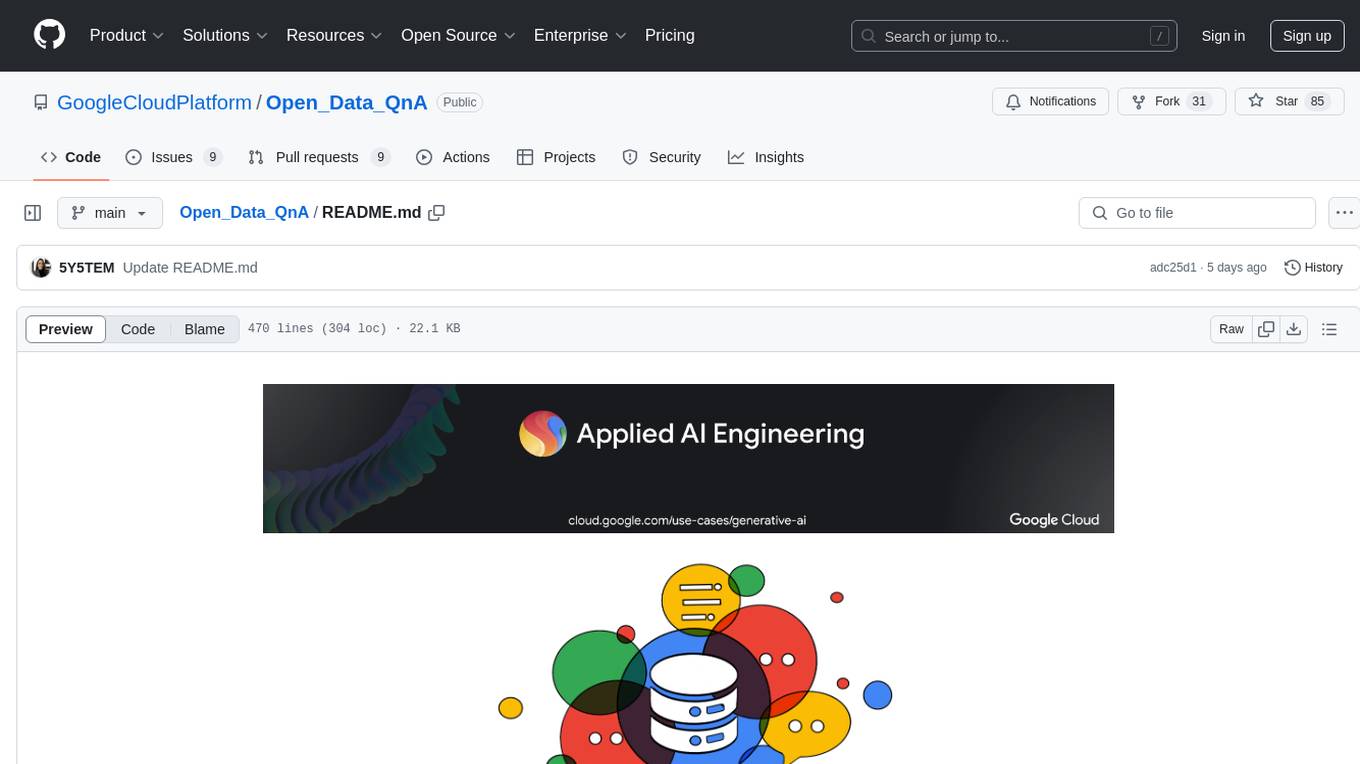
Open_Data_QnA
Open Data QnA is a Python library that allows users to interact with their PostgreSQL or BigQuery databases in a conversational manner, without needing to write SQL queries. The library leverages Large Language Models (LLMs) to bridge the gap between human language and database queries, enabling users to ask questions in natural language and receive informative responses. It offers features such as conversational querying with multiturn support, table grouping, multi schema/dataset support, SQL generation, query refinement, natural language responses, visualizations, and extensibility. The library is built on a modular design and supports various components like Database Connectors, Vector Stores, and Agents for SQL generation, validation, debugging, descriptions, embeddings, responses, and visualizations.
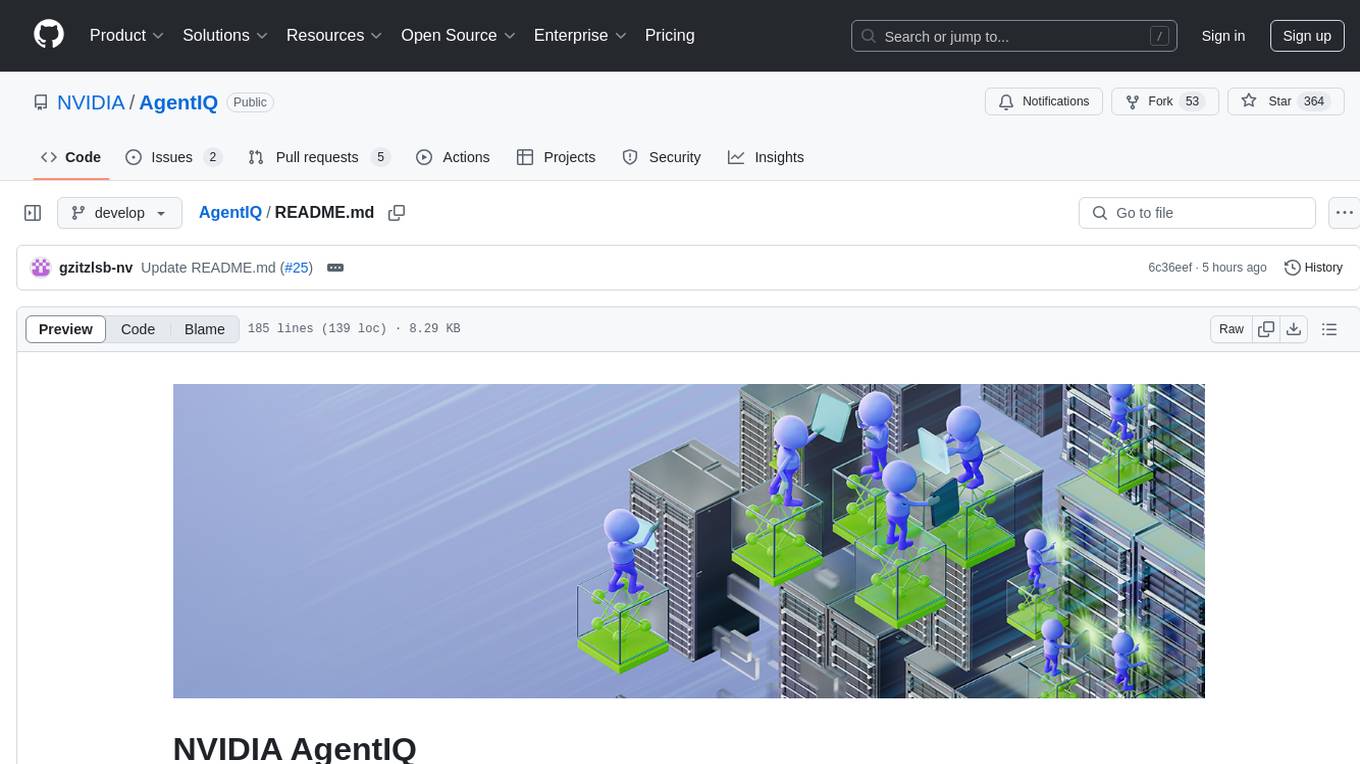
AgentIQ
AgentIQ is a flexible library designed to seamlessly integrate enterprise agents with various data sources and tools. It enables true composability by treating agents, tools, and workflows as simple function calls. With features like framework agnosticism, reusability, rapid development, profiling, observability, evaluation system, user interface, and MCP compatibility, AgentIQ empowers developers to move quickly, experiment freely, and ensure reliability across agent-driven projects.
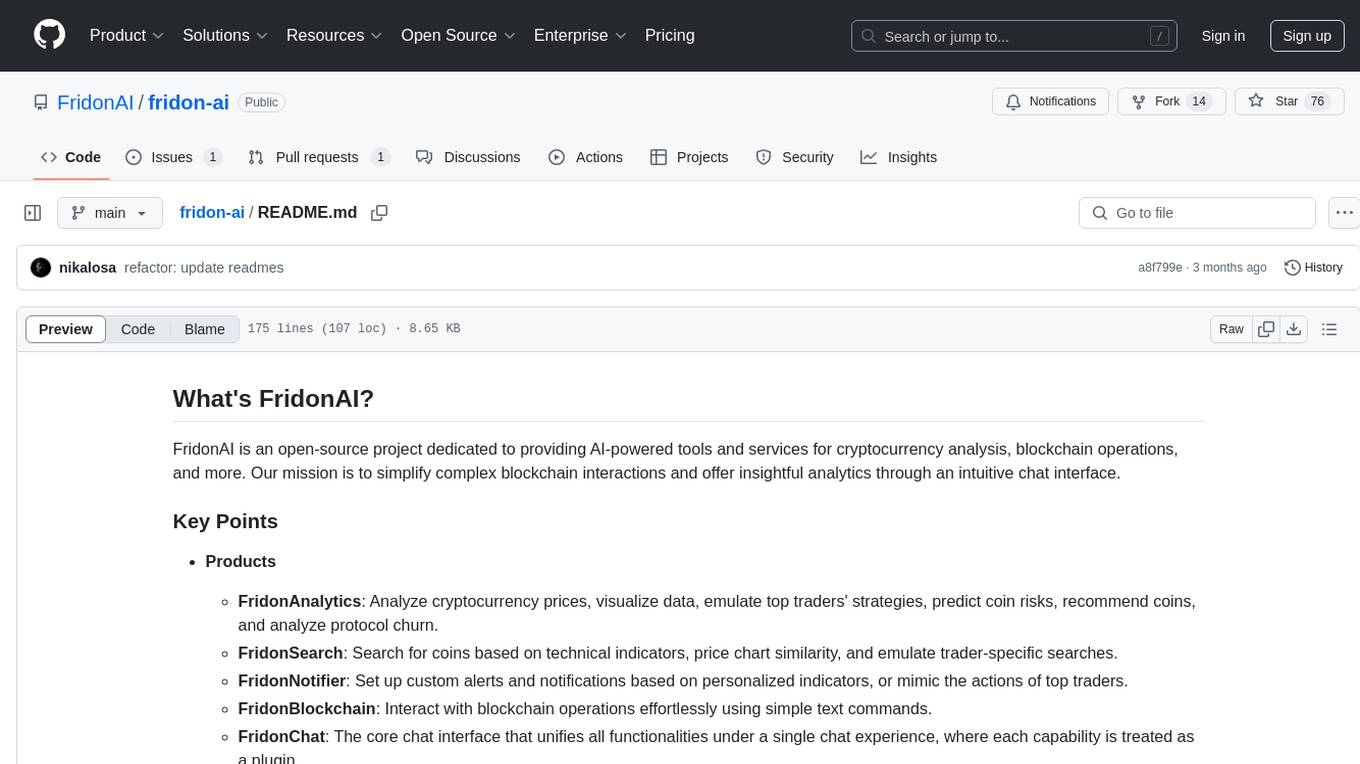
fridon-ai
FridonAI is an open-source project offering AI-powered tools for cryptocurrency analysis and blockchain operations. It includes modules like FridonAnalytics for price analysis, FridonSearch for technical indicators, FridonNotifier for custom alerts, FridonBlockchain for blockchain operations, and FridonChat as a unified chat interface. The platform empowers users to create custom AI chatbots, access crypto tools, and interact effortlessly through chat. The core functionality is modular, with plugins, tools, and utilities for easy extension and development. FridonAI implements a scoring system to assess user interactions and incentivize engagement. The application uses Redis extensively for communication and includes a Nest.js backend for system operations.
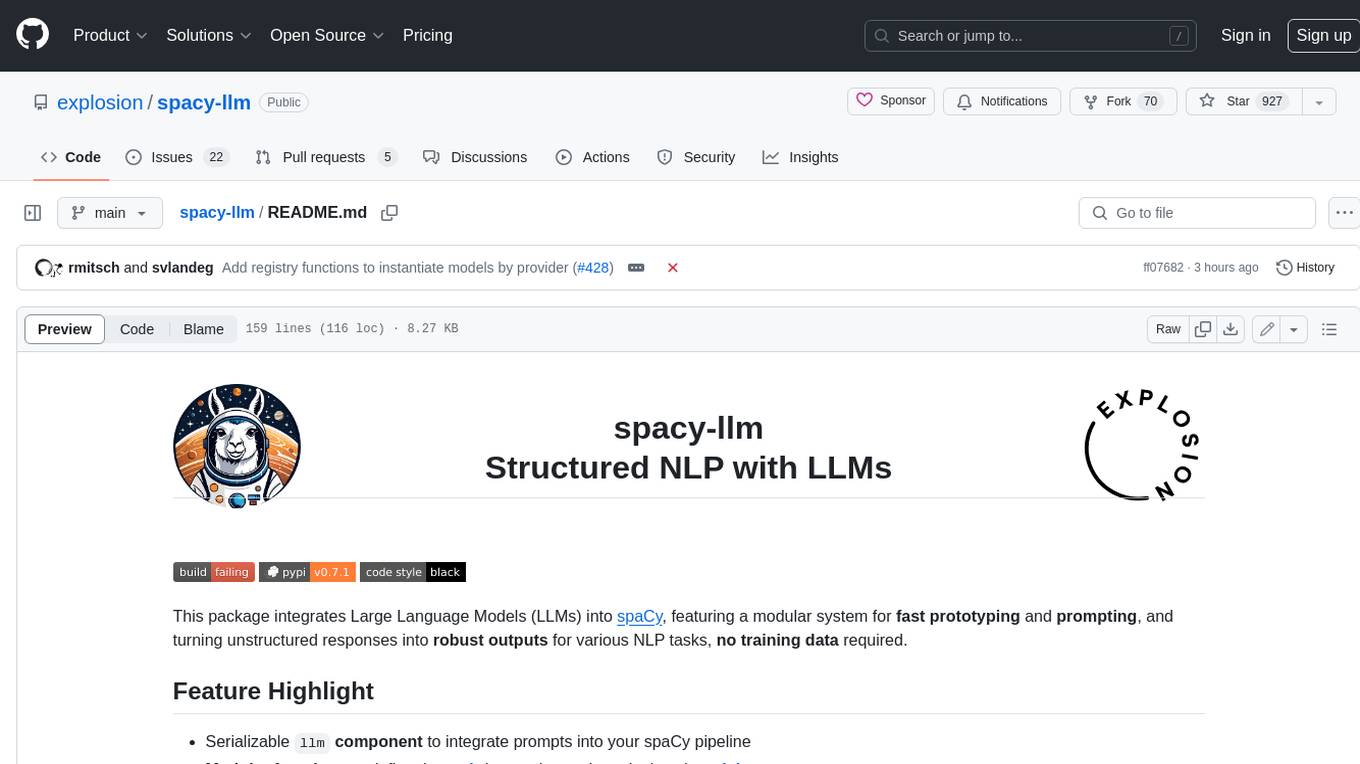
spacy-llm
This package integrates Large Language Models (LLMs) into spaCy, featuring a modular system for **fast prototyping** and **prompting** , and turning unstructured responses into **robust outputs** for various NLP tasks, **no training data** required. It supports open-source LLMs hosted on Hugging Face π€: Falcon, Dolly, Llama 2, OpenLLaMA, StableLM, Mistral. Integration with LangChain π¦οΈπ - all `langchain` models and features can be used in `spacy-llm`. Tasks available out of the box: Named Entity Recognition, Text classification, Lemmatization, Relationship extraction, Sentiment analysis, Span categorization, Summarization, Entity linking, Translation, Raw prompt execution for maximum flexibility. Soon: Semantic role labeling. Easy implementation of **your own functions** via spaCy's registry for custom prompting, parsing and model integrations. For an example, see here. Map-reduce approach for splitting prompts too long for LLM's context window and fusing the results back together
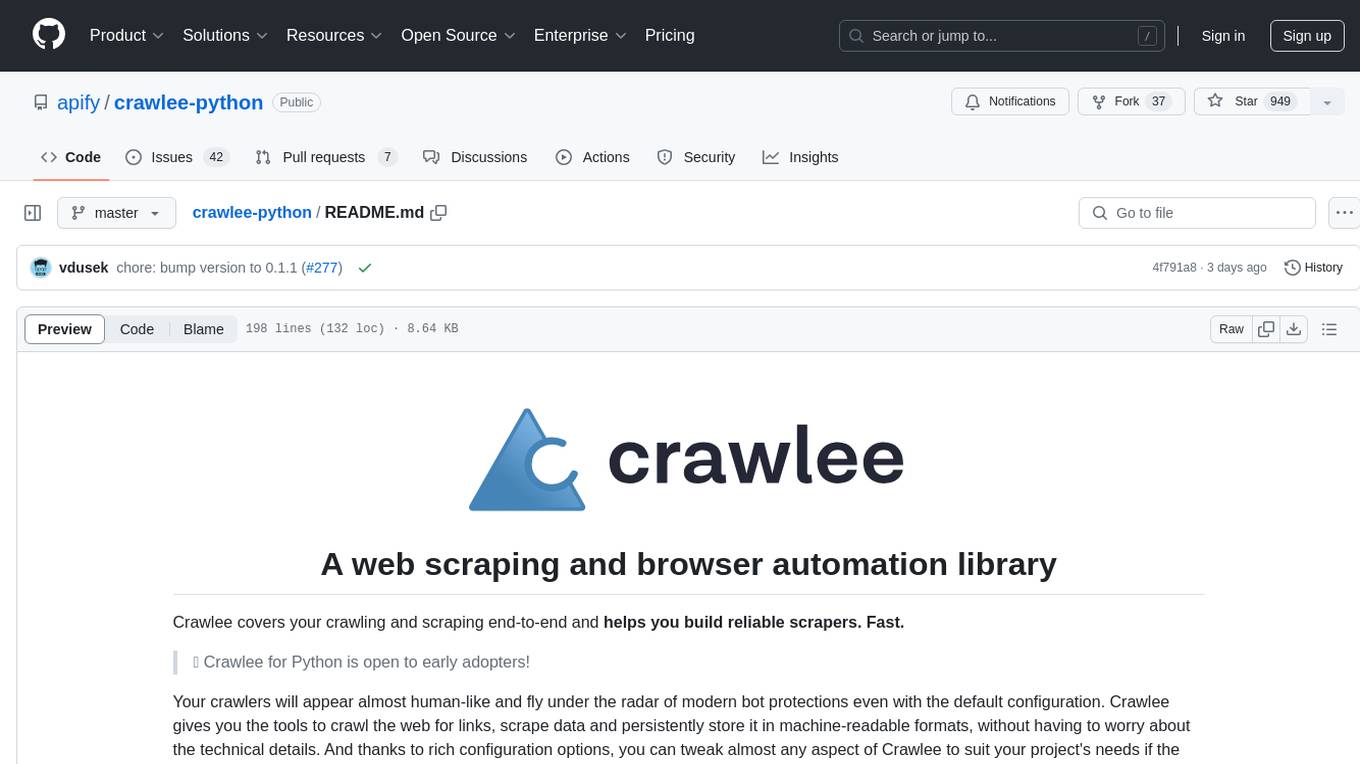
crawlee-python
Crawlee-python is a web scraping and browser automation library that covers crawling and scraping end-to-end, helping users build reliable scrapers fast. It allows users to crawl the web for links, scrape data, and store it in machine-readable formats without worrying about technical details. With rich configuration options, users can customize almost any aspect of Crawlee to suit their project's needs.
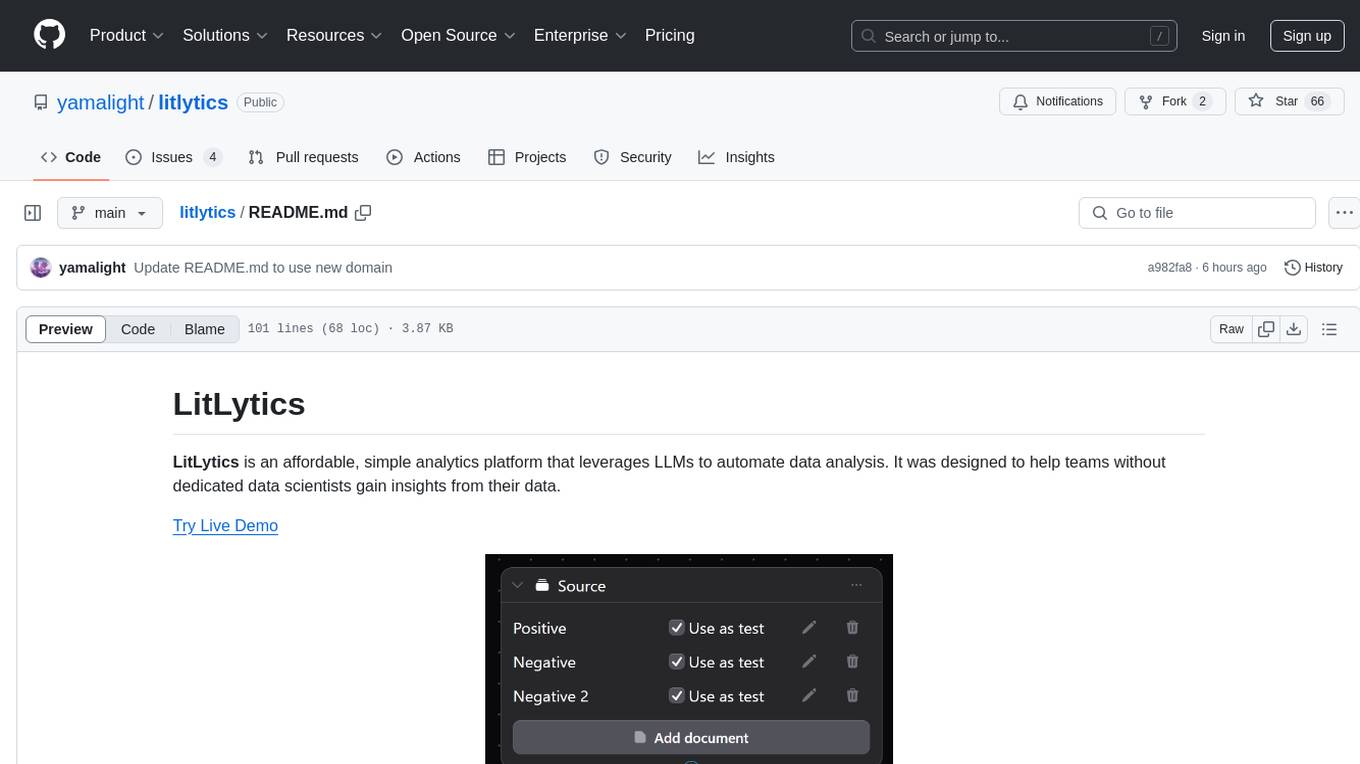
litlytics
LitLytics is an affordable analytics platform leveraging LLMs for automated data analysis. It simplifies analytics for teams without data scientists, generates custom pipelines, and allows customization. Cost-efficient with low data processing costs. Scalable and flexible, works with CSV, PDF, and plain text data formats.
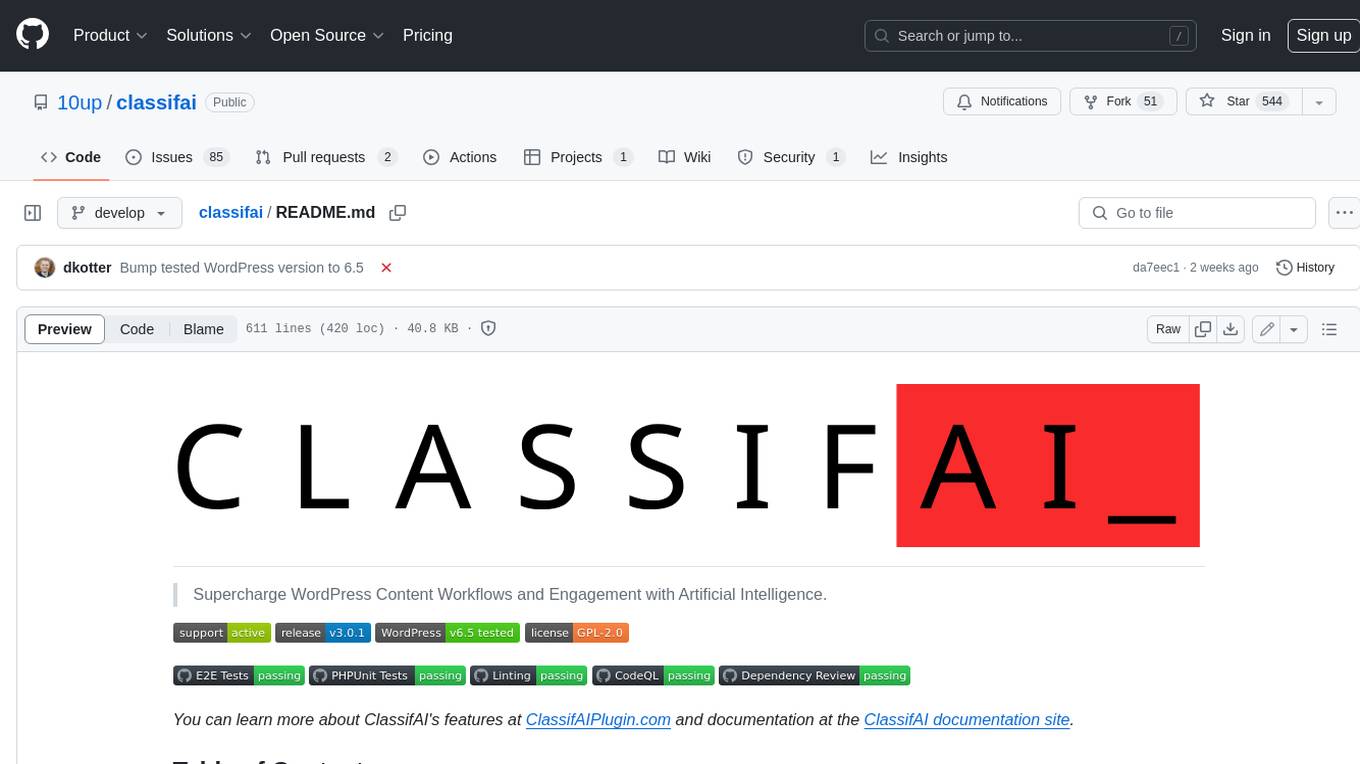
classifai
Supercharge WordPress Content Workflows and Engagement with Artificial Intelligence. Tap into leading cloud-based services like OpenAI, Microsoft Azure AI, Google Gemini and IBM Watson to augment your WordPress-powered websites. Publish content faster while improving SEO performance and increasing audience engagement. ClassifAI integrates Artificial Intelligence and Machine Learning technologies to lighten your workload and eliminate tedious tasks, giving you more time to create original content that matters.
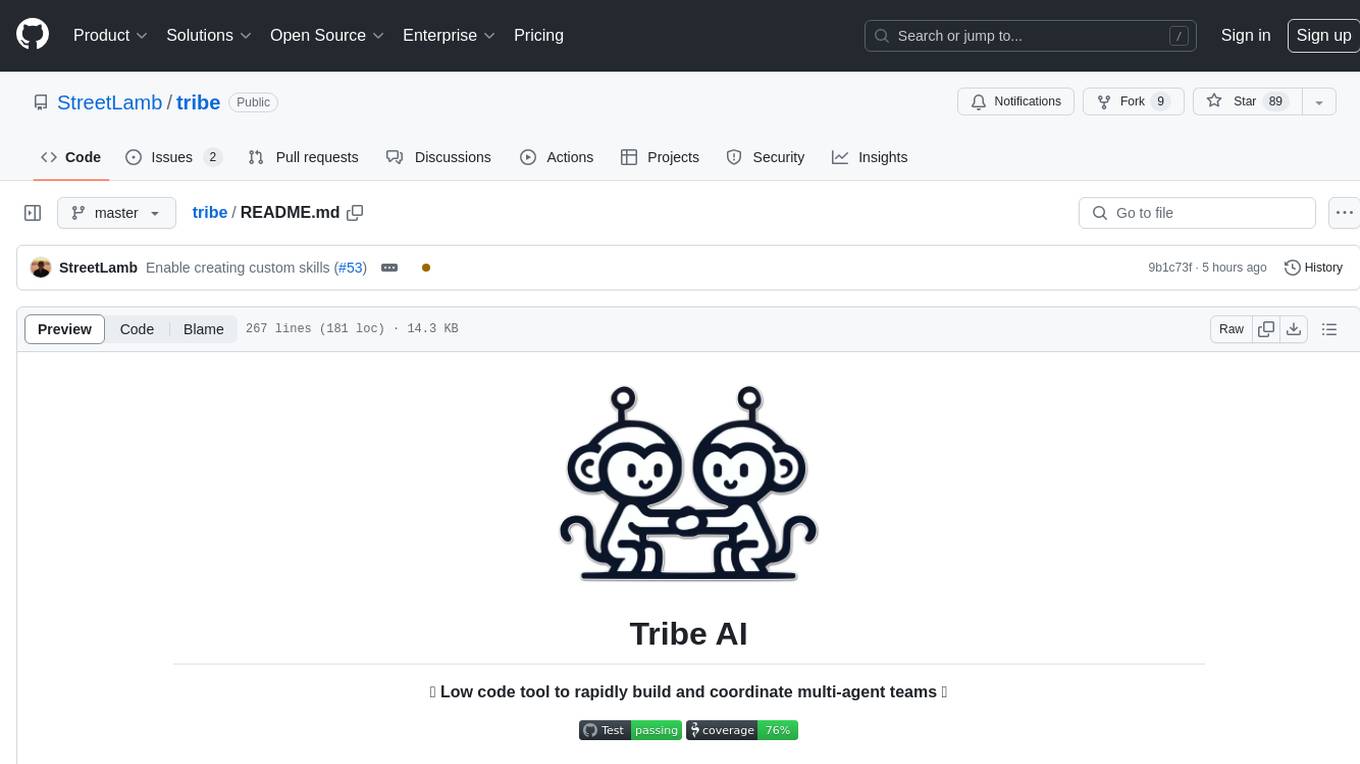
tribe
Tribe AI is a low code tool designed to rapidly build and coordinate multi-agent teams. It leverages the langgraph framework to customize and coordinate teams of agents, allowing tasks to be split among agents with different strengths for faster and better problem-solving. The tool supports persistent conversations, observability, tool calling, human-in-the-loop functionality, easy deployment with Docker, and multi-tenancy for managing multiple users and teams.
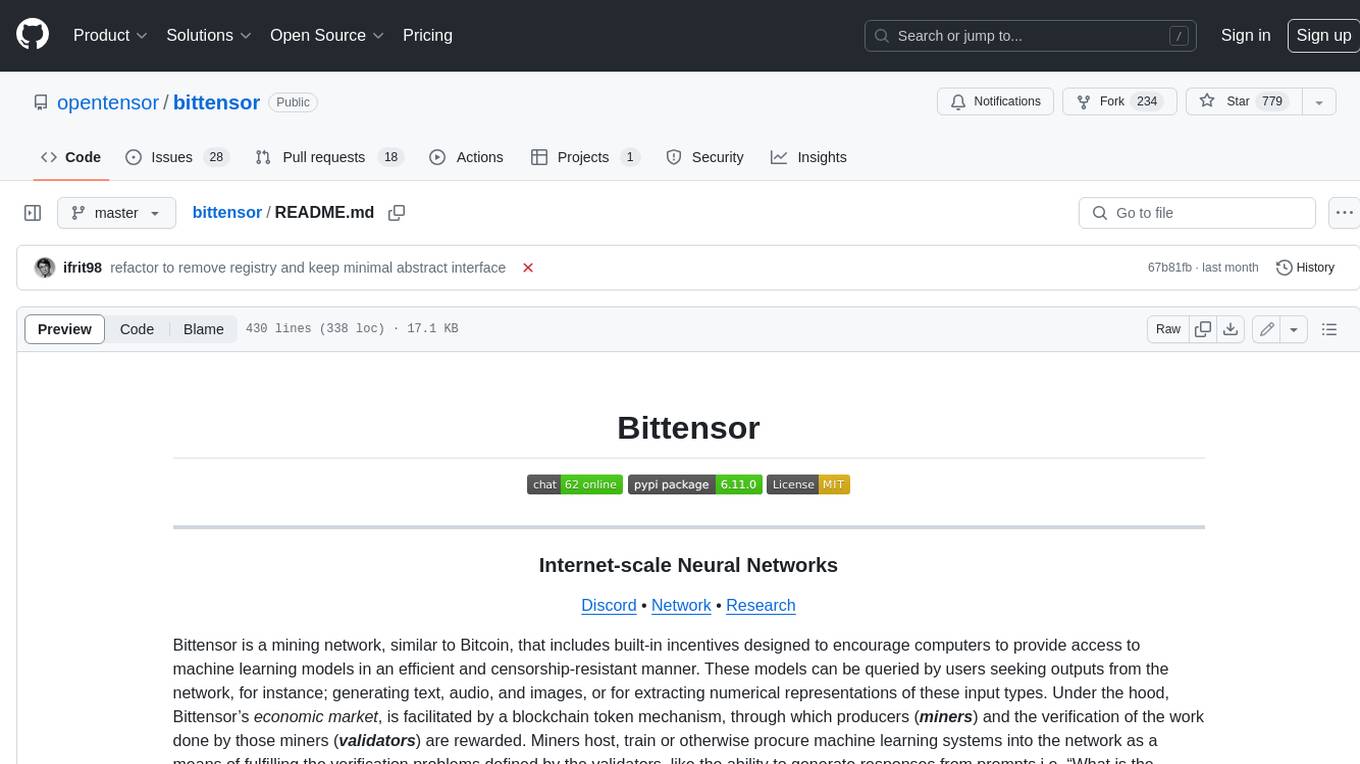
bittensor
Bittensor is an internet-scale neural network that incentivizes computers to provide access to machine learning models in a decentralized and censorship-resistant manner. It operates through a token-based mechanism where miners host, train, and procure machine learning systems to fulfill verification problems defined by validators. The network rewards miners and validators for their contributions, ensuring continuous improvement in knowledge output. Bittensor allows anyone to participate, extract value, and govern the network without centralized control. It supports tasks such as generating text, audio, images, and extracting numerical representations.
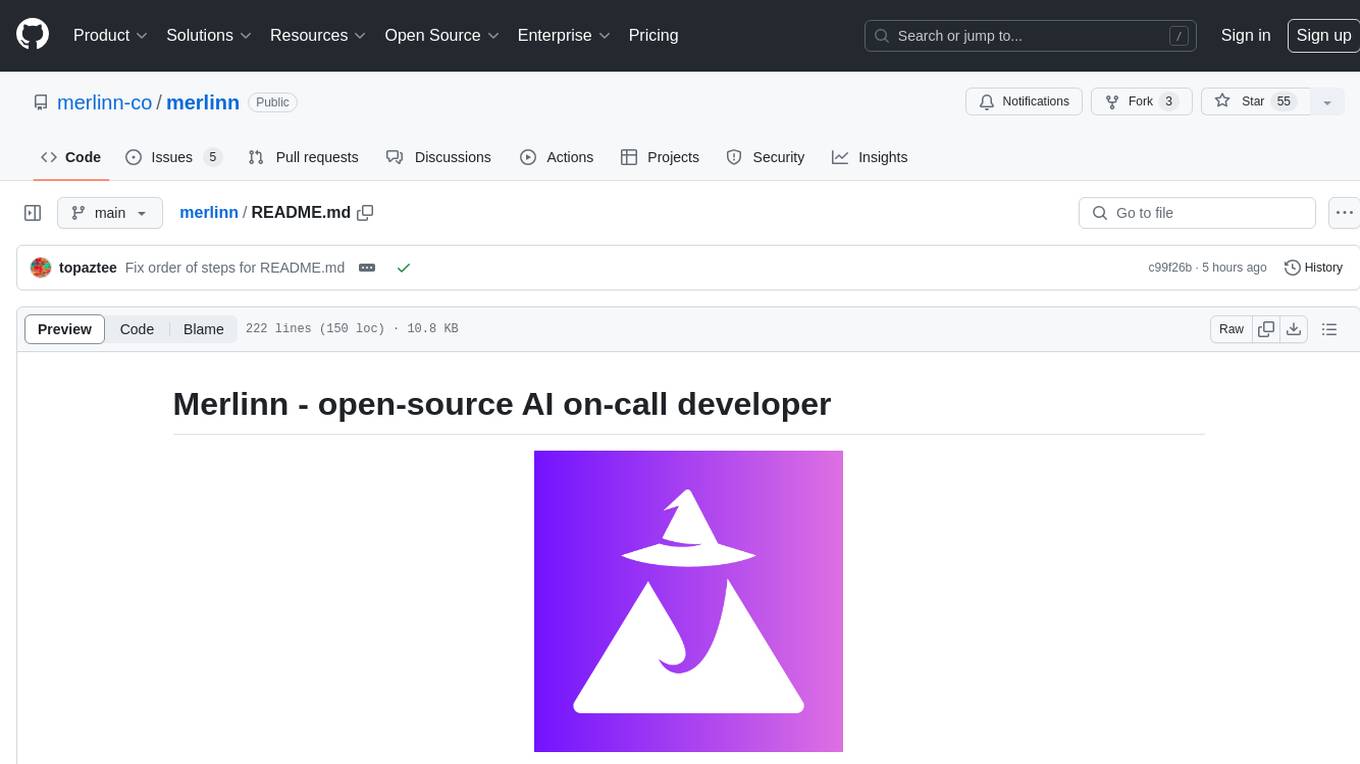
merlinn
Merlinn is an open-source AI-powered on-call engineer that automatically jumps into incidents & alerts, providing useful insights and RCA in real time. It integrates with popular observability tools, lives inside Slack, offers an intuitive UX, and prioritizes security. Users can self-host Merlinn, use it for free, and benefit from automatic RCA, Slack integration, integrations with various tools, intuitive UX, and security features.
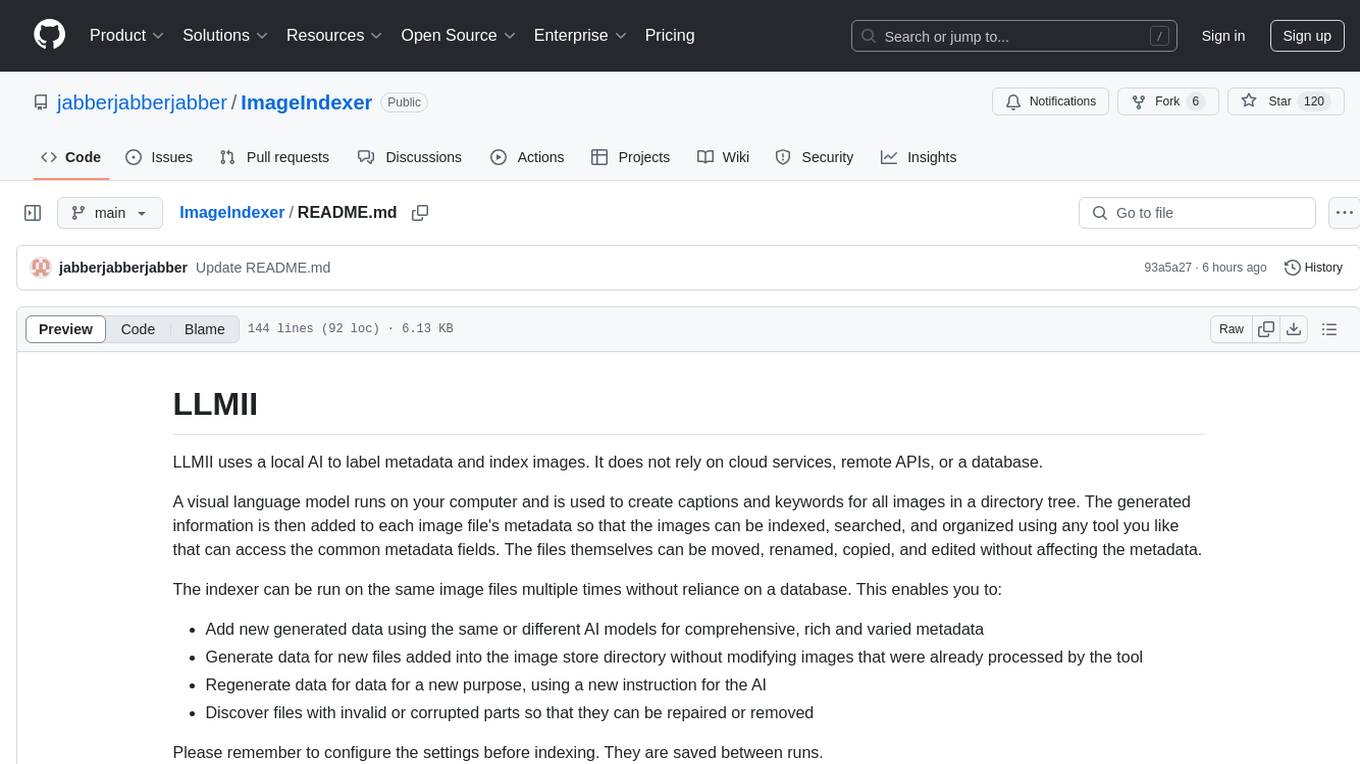
ImageIndexer
LLMII is a tool that uses a local AI model to label metadata and index images without relying on cloud services or remote APIs. It runs a visual language model on your computer to generate captions and keywords for images, enhancing their metadata for indexing, searching, and organization. The tool can be run multiple times on the same image files, allowing for adding new data, regenerating data, and discovering files with issues. It supports various image formats, offers a user-friendly GUI, and can utilize GPU acceleration for faster processing. LLMII requires Python 3.8 or higher and operates directly on image file metadata fields like MWG:Keyword and XMP:Identifier.
For similar tasks
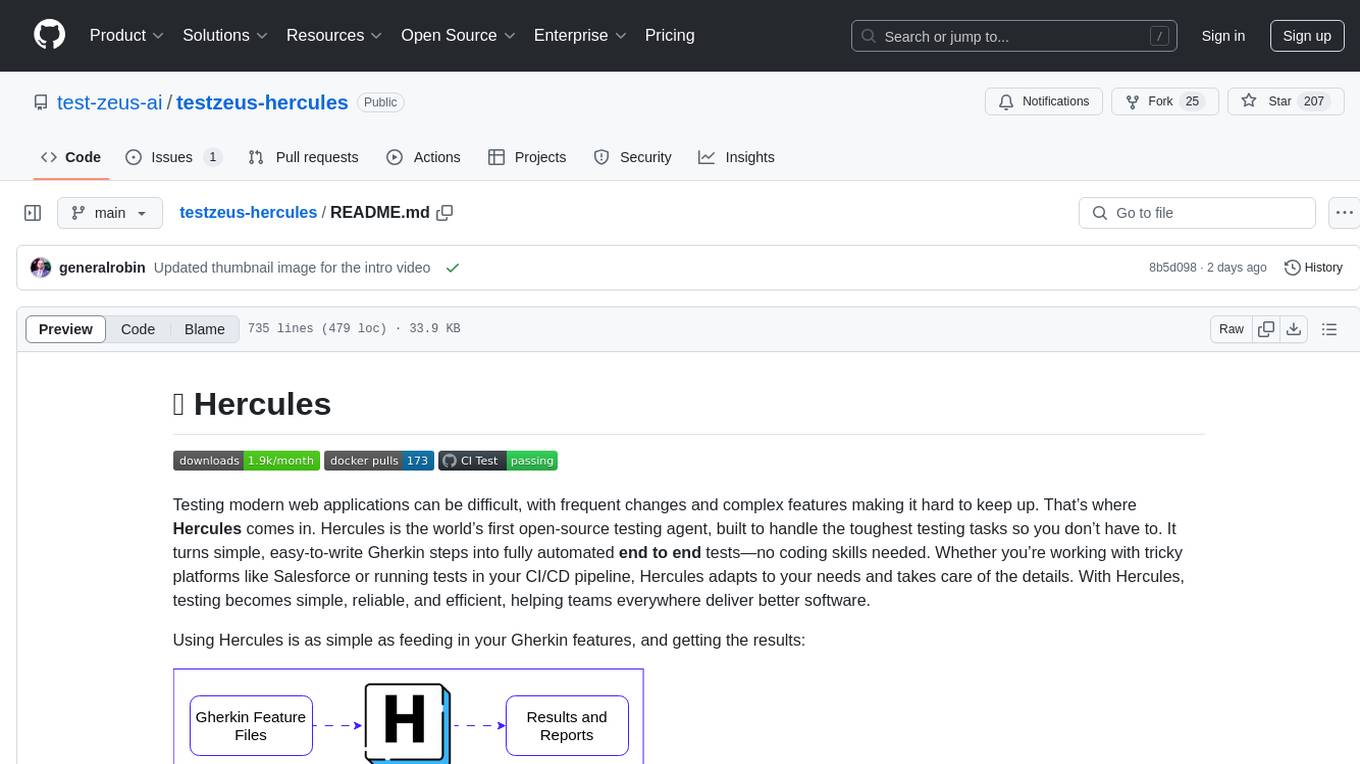
testzeus-hercules
Hercules is the worldβs first open-source testing agent designed to handle the toughest testing tasks for modern web applications. It turns simple Gherkin steps into fully automated end-to-end tests, making testing simple, reliable, and efficient. Hercules adapts to various platforms like Salesforce and is suitable for CI/CD pipelines. It aims to democratize and disrupt test automation, making top-tier testing accessible to everyone. The tool is transparent, reliable, and community-driven, empowering teams to deliver better software. Hercules offers multiple ways to get started, including using PyPI package, Docker, or building and running from source code. It supports various AI models, provides detailed installation and usage instructions, and integrates with Nuclei for security testing and WCAG for accessibility testing. The tool is production-ready, open core, and open source, with plans for enhanced LLM support, advanced tooling, improved DOM distillation, community contributions, extensive documentation, and a bounty program.
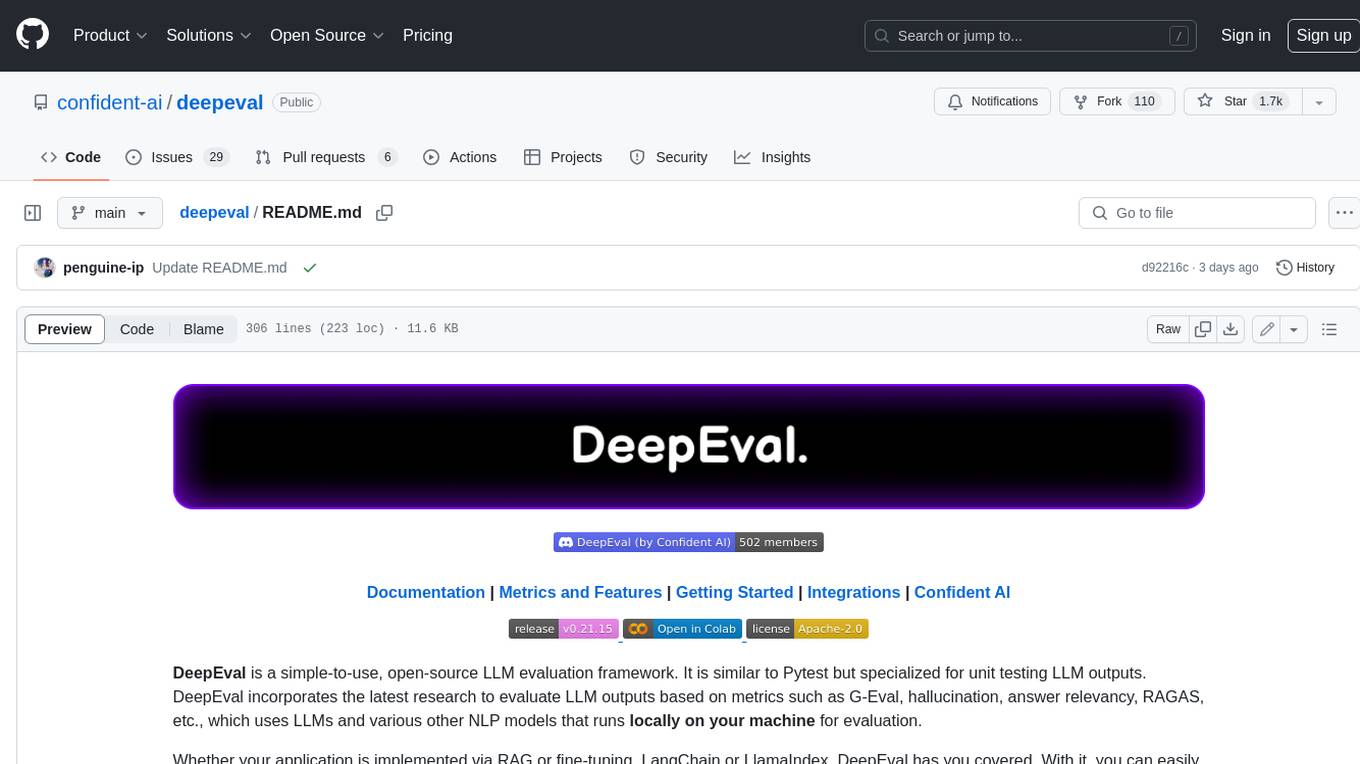
deepeval
DeepEval is a simple-to-use, open-source LLM evaluation framework specialized for unit testing LLM outputs. It incorporates various metrics such as G-Eval, hallucination, answer relevancy, RAGAS, etc., and runs locally on your machine for evaluation. It provides a wide range of ready-to-use evaluation metrics, allows for creating custom metrics, integrates with any CI/CD environment, and enables benchmarking LLMs on popular benchmarks. DeepEval is designed for evaluating RAG and fine-tuning applications, helping users optimize hyperparameters, prevent prompt drifting, and transition from OpenAI to hosting their own Llama2 with confidence.
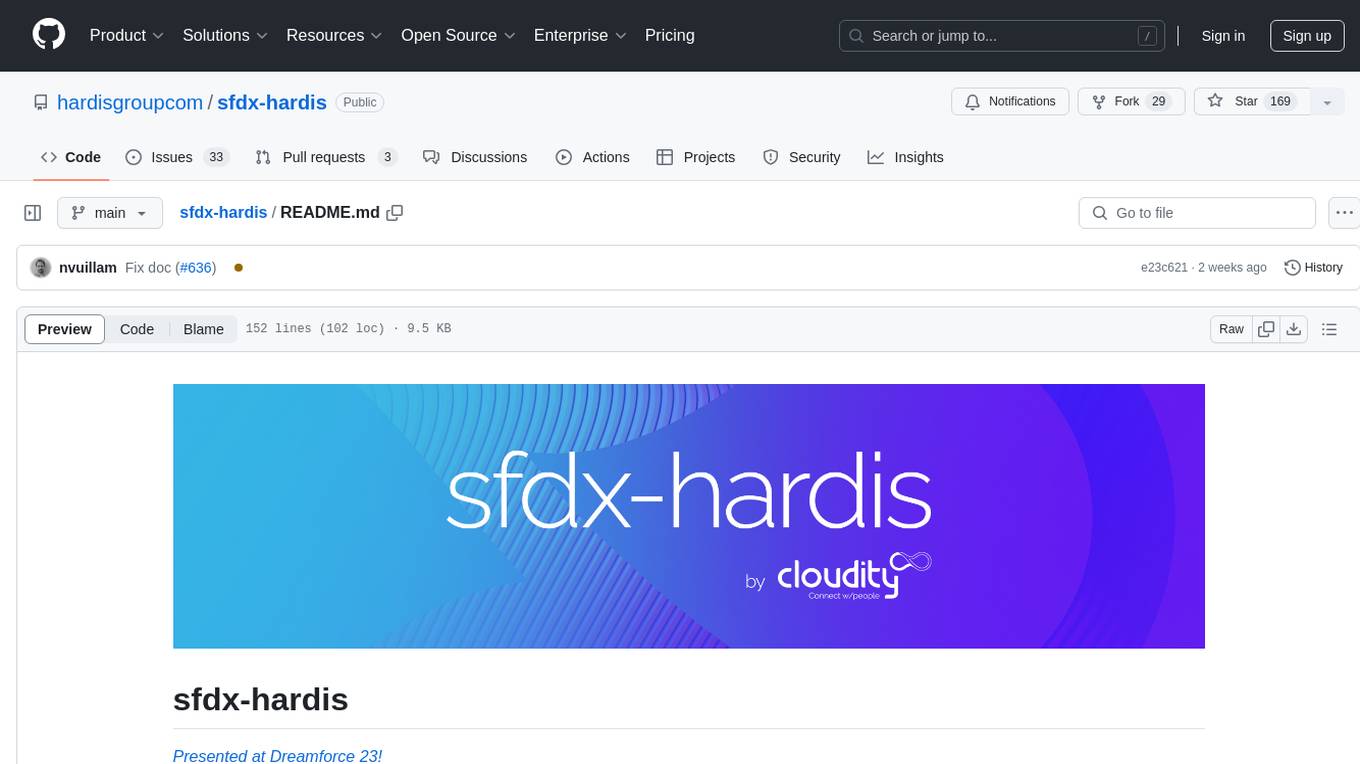
sfdx-hardis
sfdx-hardis is a toolbox for Salesforce DX, developed by Cloudity, that simplifies tasks which would otherwise take minutes or hours to complete manually. It enables users to define complete CI/CD pipelines for Salesforce projects, backup metadata, and monitor any Salesforce org. The tool offers a wide range of commands that can be accessed via the command line interface or through a Visual Studio Code extension. Additionally, sfdx-hardis provides Docker images for easy integration into CI workflows. The tool is designed to be natively compliant with various platforms and tools, making it a versatile solution for Salesforce developers.
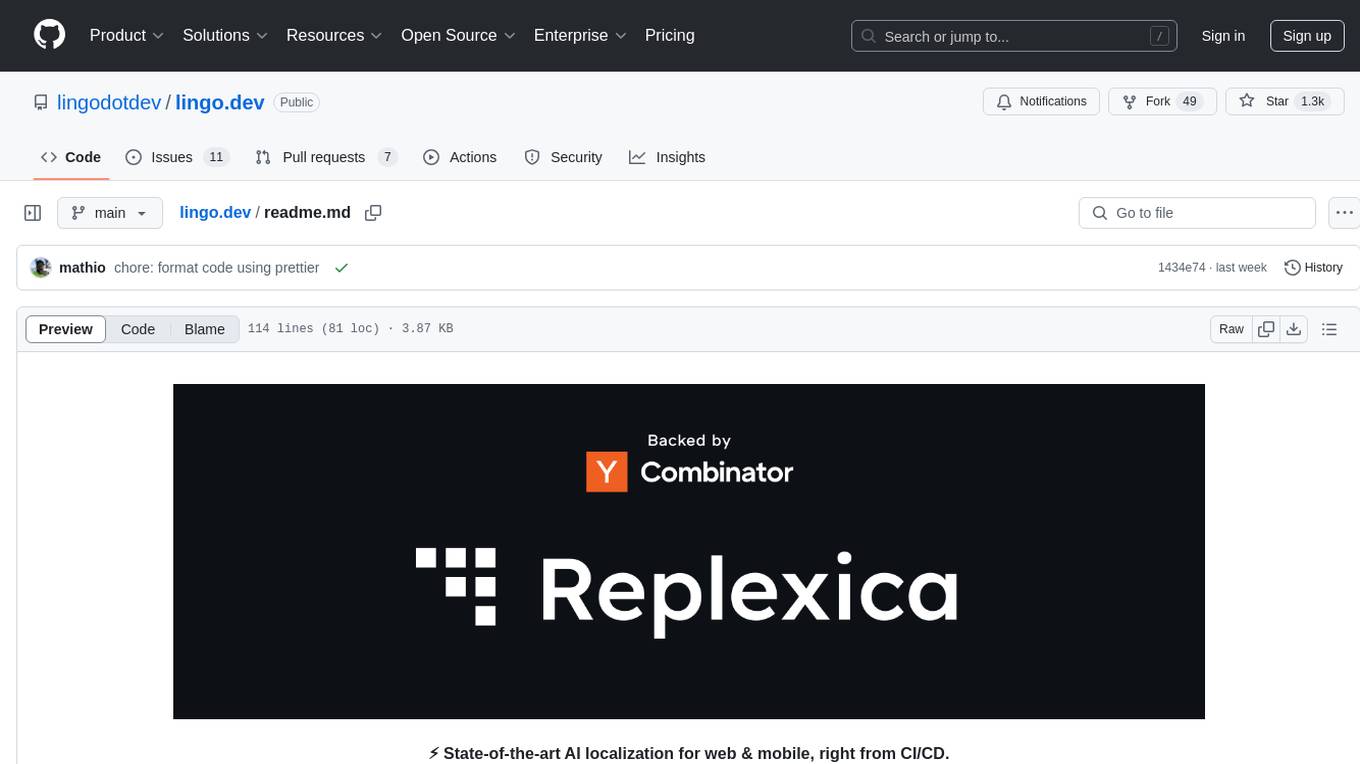
lingo.dev
Replexica AI automates software localization end-to-end, producing authentic translations instantly across 60+ languages. Teams can do localization 100x faster with state-of-the-art quality, reaching more paying customers worldwide. The tool offers a GitHub Action for CI/CD automation and supports various formats like JSON, YAML, CSV, and Markdown. With lightning-fast AI localization, auto-updates, native quality translations, developer-friendly CLI, and scalability for startups and enterprise teams, Replexica is a top choice for efficient and effective software localization.
For similar jobs
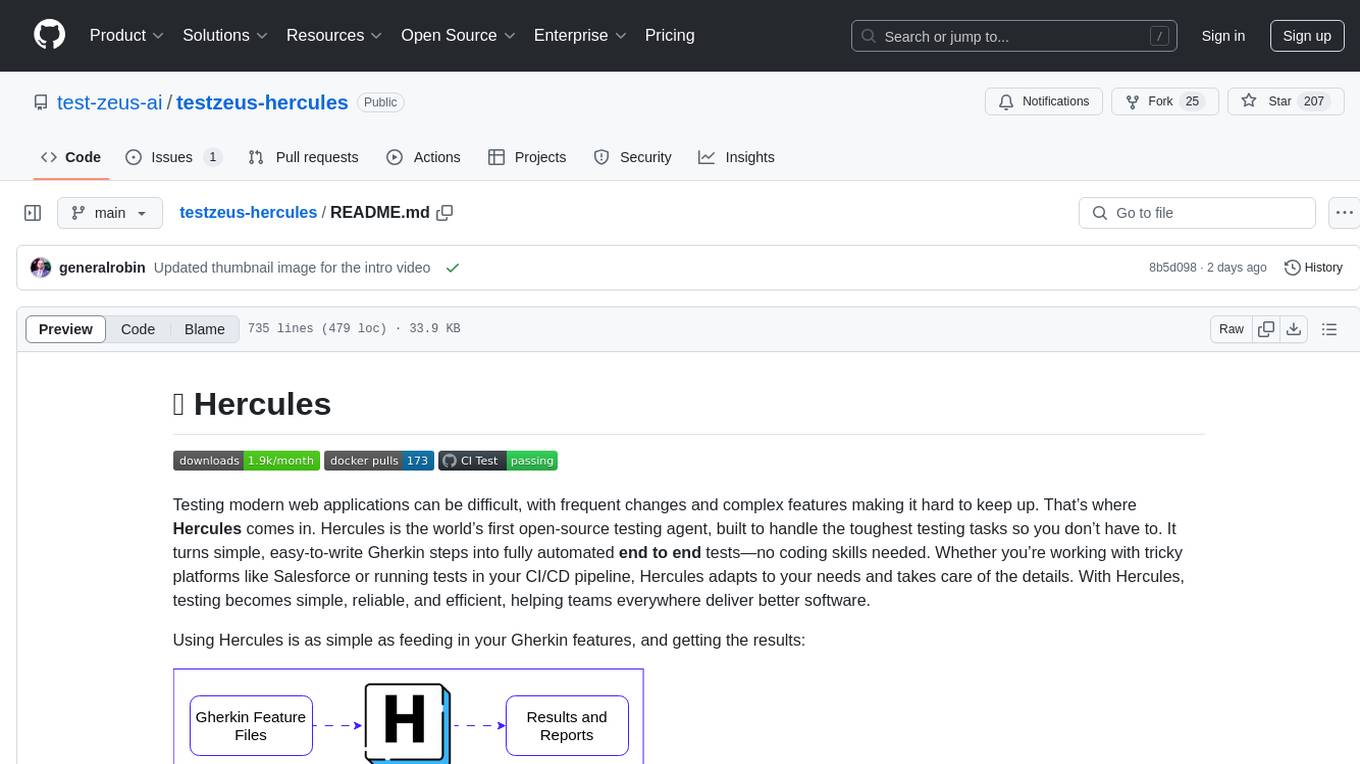
testzeus-hercules
Hercules is the worldβs first open-source testing agent designed to handle the toughest testing tasks for modern web applications. It turns simple Gherkin steps into fully automated end-to-end tests, making testing simple, reliable, and efficient. Hercules adapts to various platforms like Salesforce and is suitable for CI/CD pipelines. It aims to democratize and disrupt test automation, making top-tier testing accessible to everyone. The tool is transparent, reliable, and community-driven, empowering teams to deliver better software. Hercules offers multiple ways to get started, including using PyPI package, Docker, or building and running from source code. It supports various AI models, provides detailed installation and usage instructions, and integrates with Nuclei for security testing and WCAG for accessibility testing. The tool is production-ready, open core, and open source, with plans for enhanced LLM support, advanced tooling, improved DOM distillation, community contributions, extensive documentation, and a bounty program.
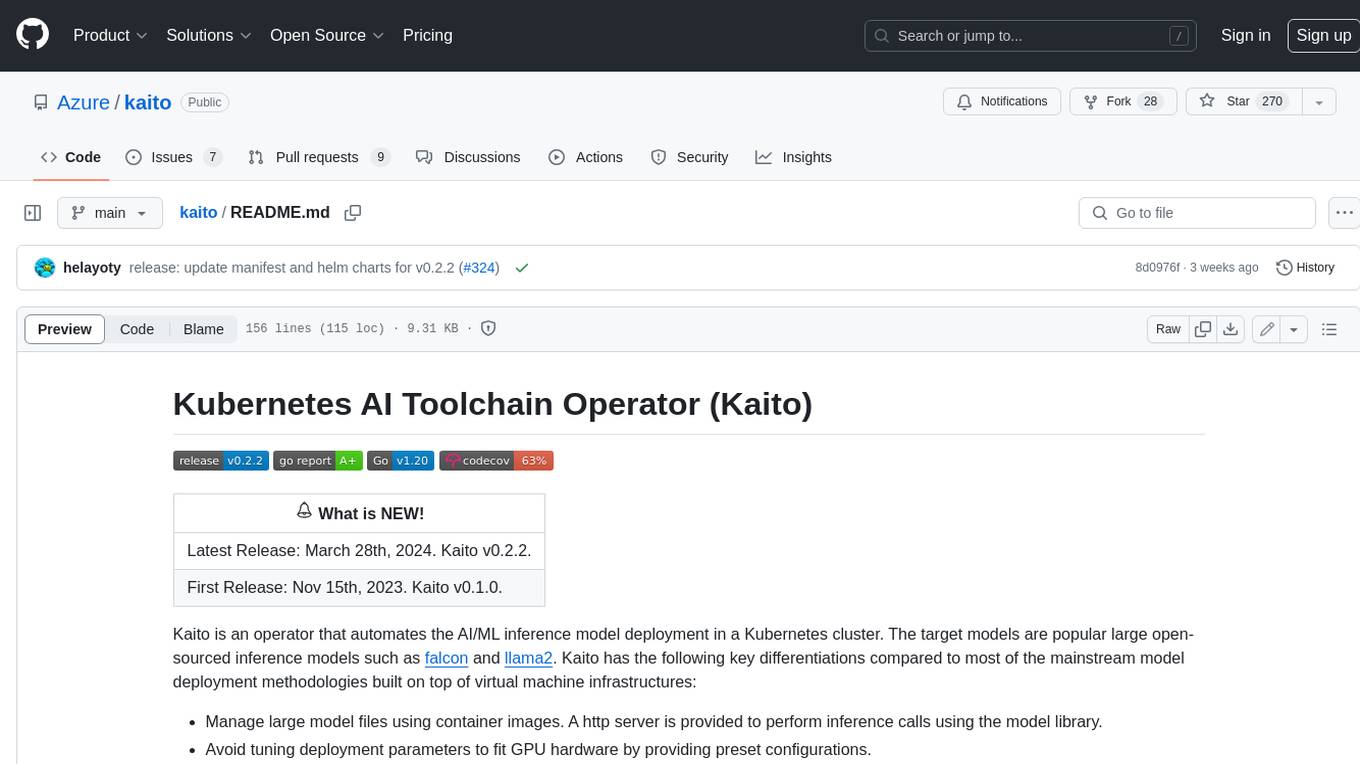
kaito
Kaito is an operator that automates the AI/ML inference model deployment in a Kubernetes cluster. It manages large model files using container images, avoids tuning deployment parameters to fit GPU hardware by providing preset configurations, auto-provisions GPU nodes based on model requirements, and hosts large model images in the public Microsoft Container Registry (MCR) if the license allows. Using Kaito, the workflow of onboarding large AI inference models in Kubernetes is largely simplified.
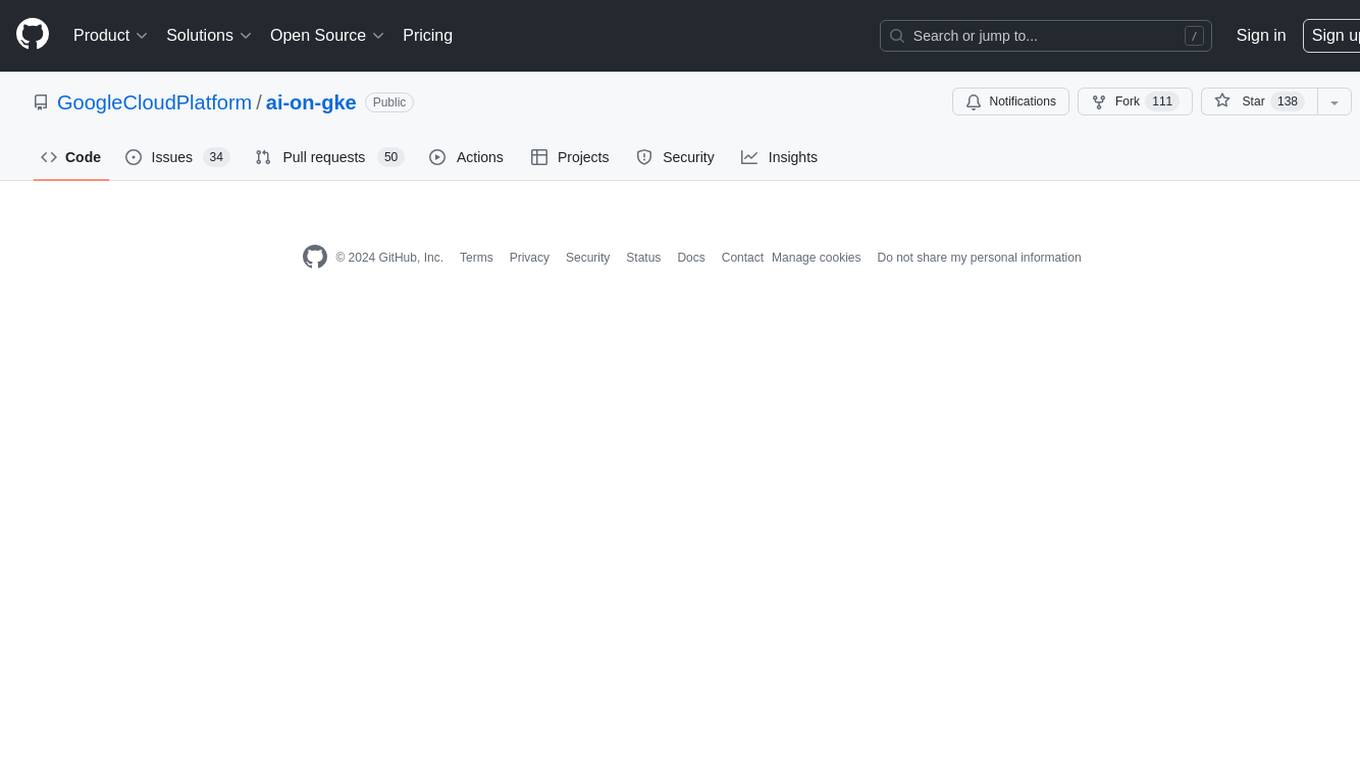
ai-on-gke
This repository contains assets related to AI/ML workloads on Google Kubernetes Engine (GKE). Run optimized AI/ML workloads with Google Kubernetes Engine (GKE) platform orchestration capabilities. A robust AI/ML platform considers the following layers: Infrastructure orchestration that support GPUs and TPUs for training and serving workloads at scale Flexible integration with distributed computing and data processing frameworks Support for multiple teams on the same infrastructure to maximize utilization of resources
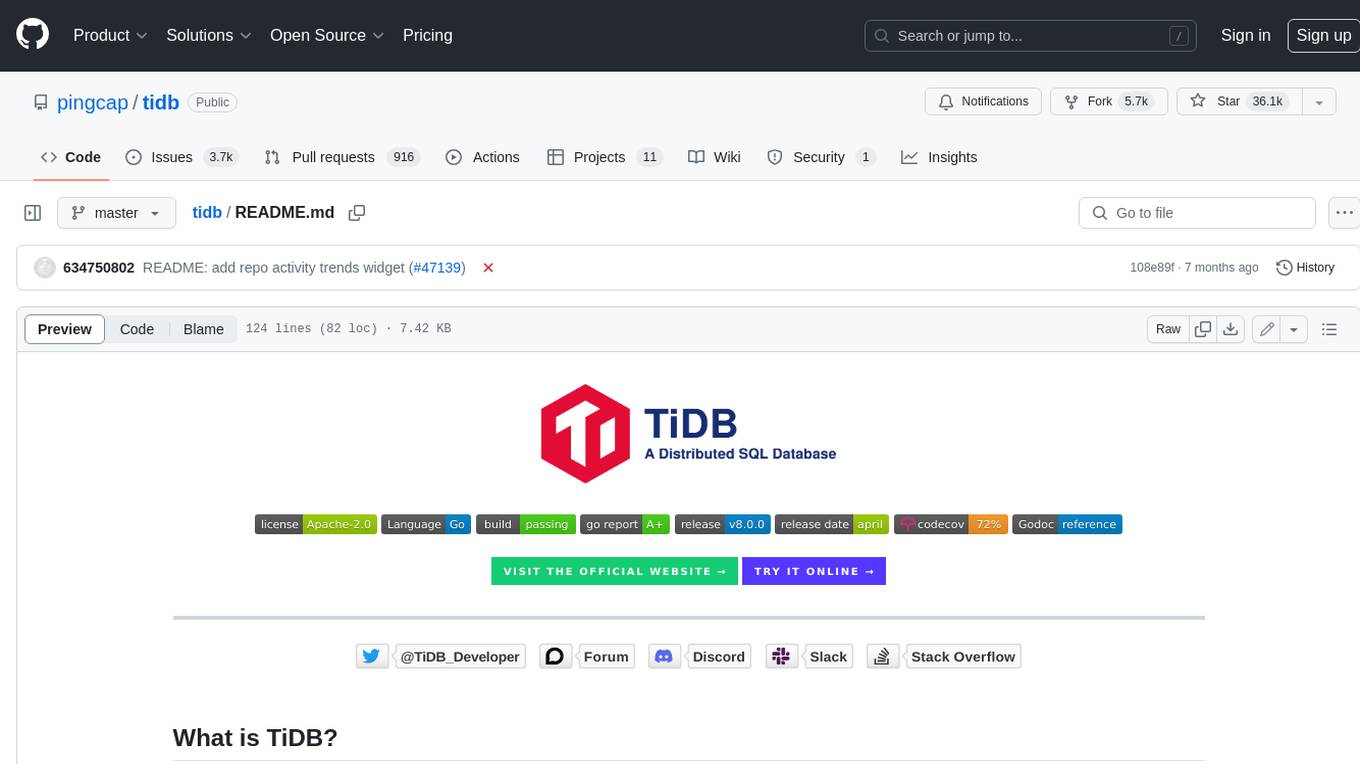
tidb
TiDB is an open-source distributed SQL database that supports Hybrid Transactional and Analytical Processing (HTAP) workloads. It is MySQL compatible and features horizontal scalability, strong consistency, and high availability.
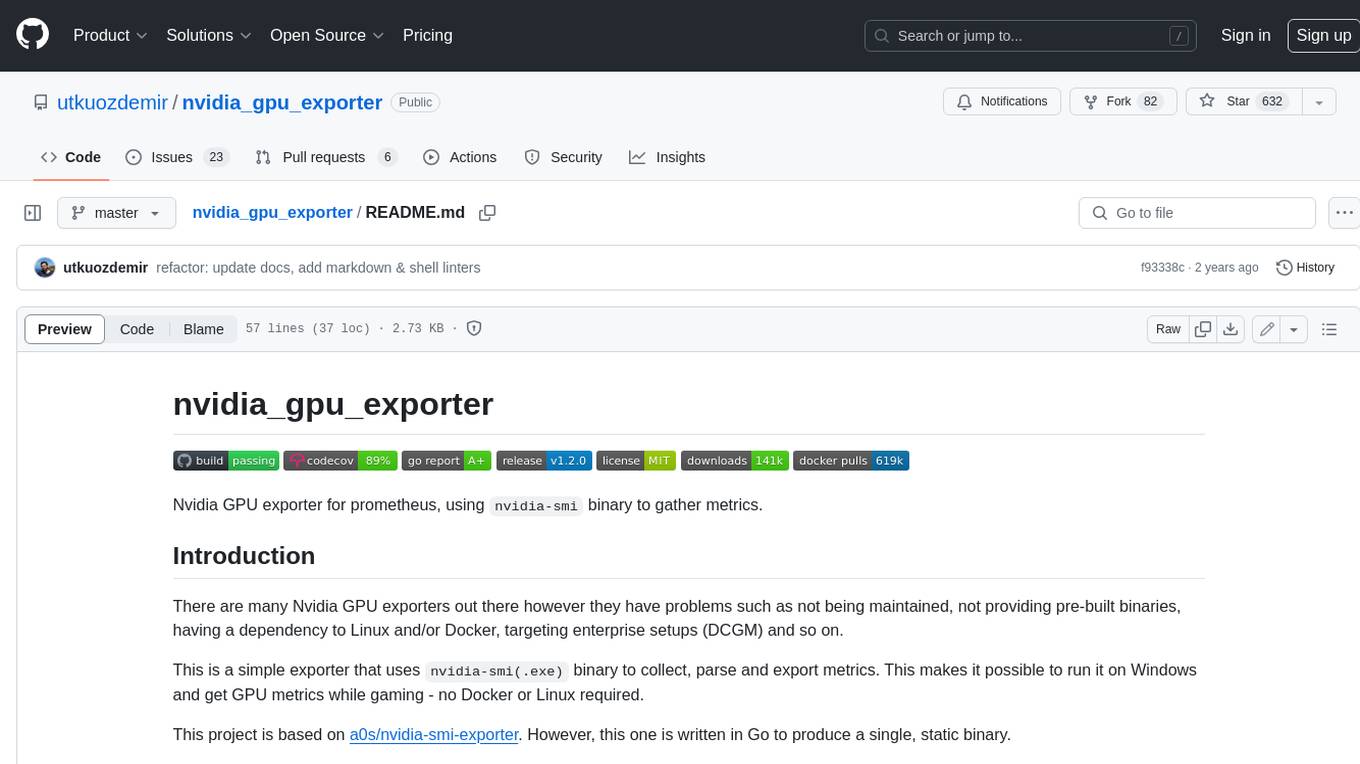
nvidia_gpu_exporter
Nvidia GPU exporter for prometheus, using `nvidia-smi` binary to gather metrics.
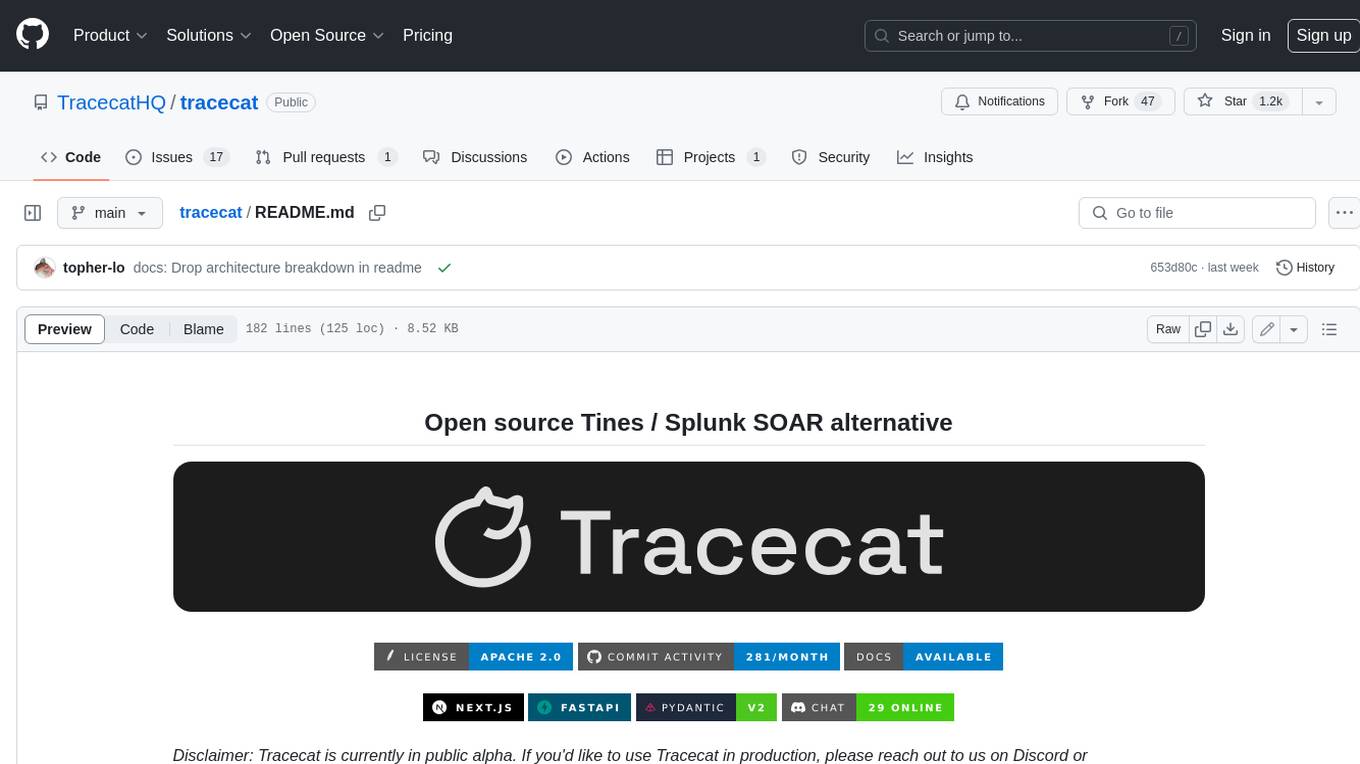
tracecat
Tracecat is an open-source automation platform for security teams. It's designed to be simple but powerful, with a focus on AI features and a practitioner-obsessed UI/UX. Tracecat can be used to automate a variety of tasks, including phishing email investigation, evidence collection, and remediation plan generation.
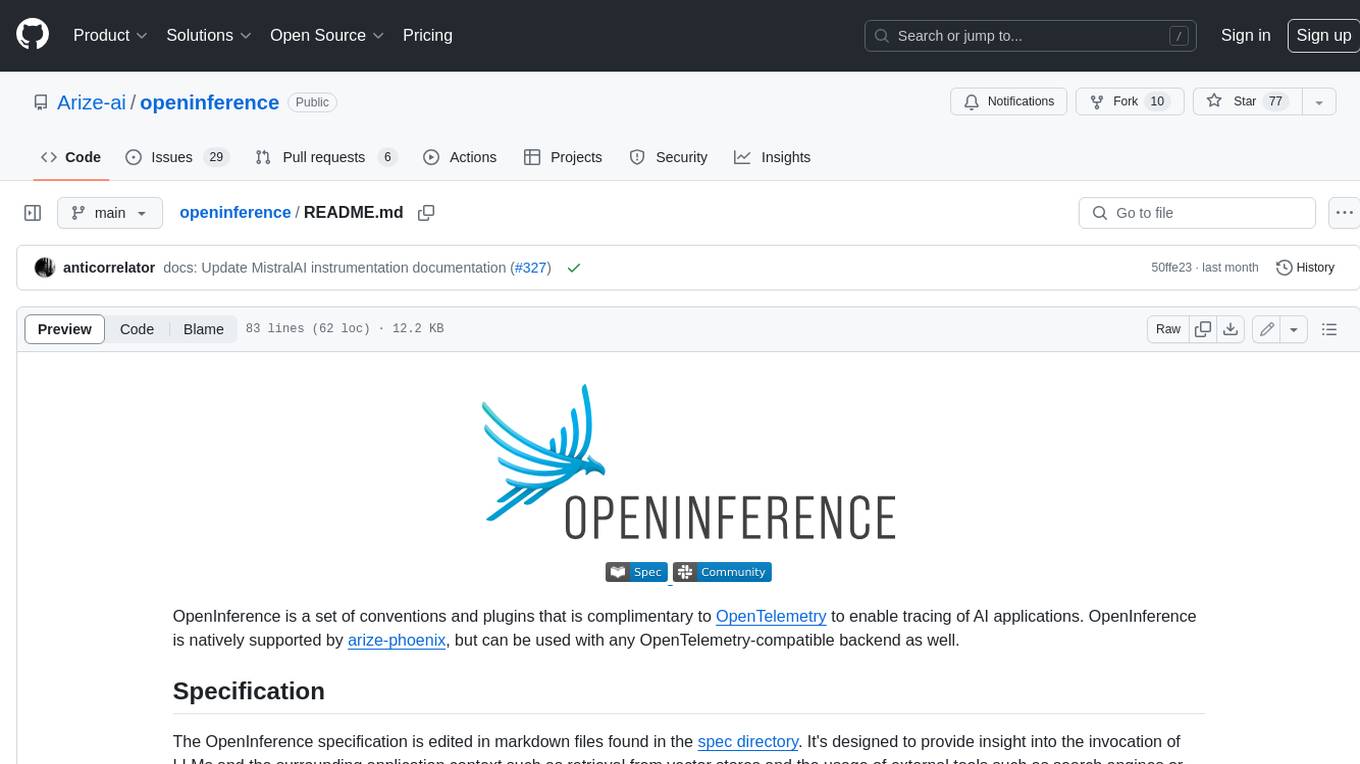
openinference
OpenInference is a set of conventions and plugins that complement OpenTelemetry to enable tracing of AI applications. It provides a way to capture and analyze the performance and behavior of AI models, including their interactions with other components of the application. OpenInference is designed to be language-agnostic and can be used with any OpenTelemetry-compatible backend. It includes a set of instrumentations for popular machine learning SDKs and frameworks, making it easy to add tracing to your AI applications.
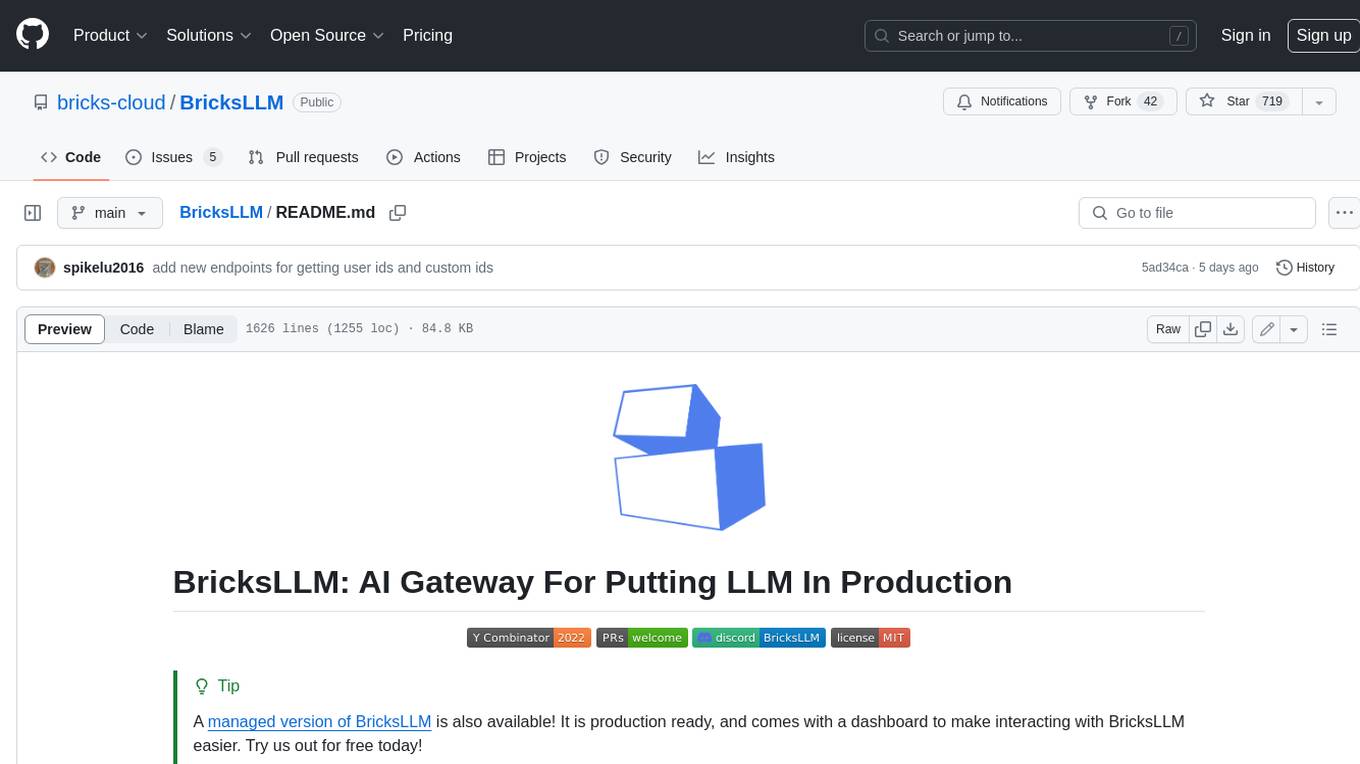
BricksLLM
BricksLLM is a cloud native AI gateway written in Go. Currently, it provides native support for OpenAI, Anthropic, Azure OpenAI and vLLM. BricksLLM aims to provide enterprise level infrastructure that can power any LLM production use cases. Here are some use cases for BricksLLM: * Set LLM usage limits for users on different pricing tiers * Track LLM usage on a per user and per organization basis * Block or redact requests containing PIIs * Improve LLM reliability with failovers, retries and caching * Distribute API keys with rate limits and cost limits for internal development/production use cases * Distribute API keys with rate limits and cost limits for students