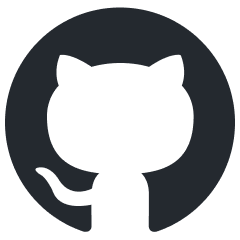
ztncui-aio
Licensed Under AGPL v3
Stars: 166
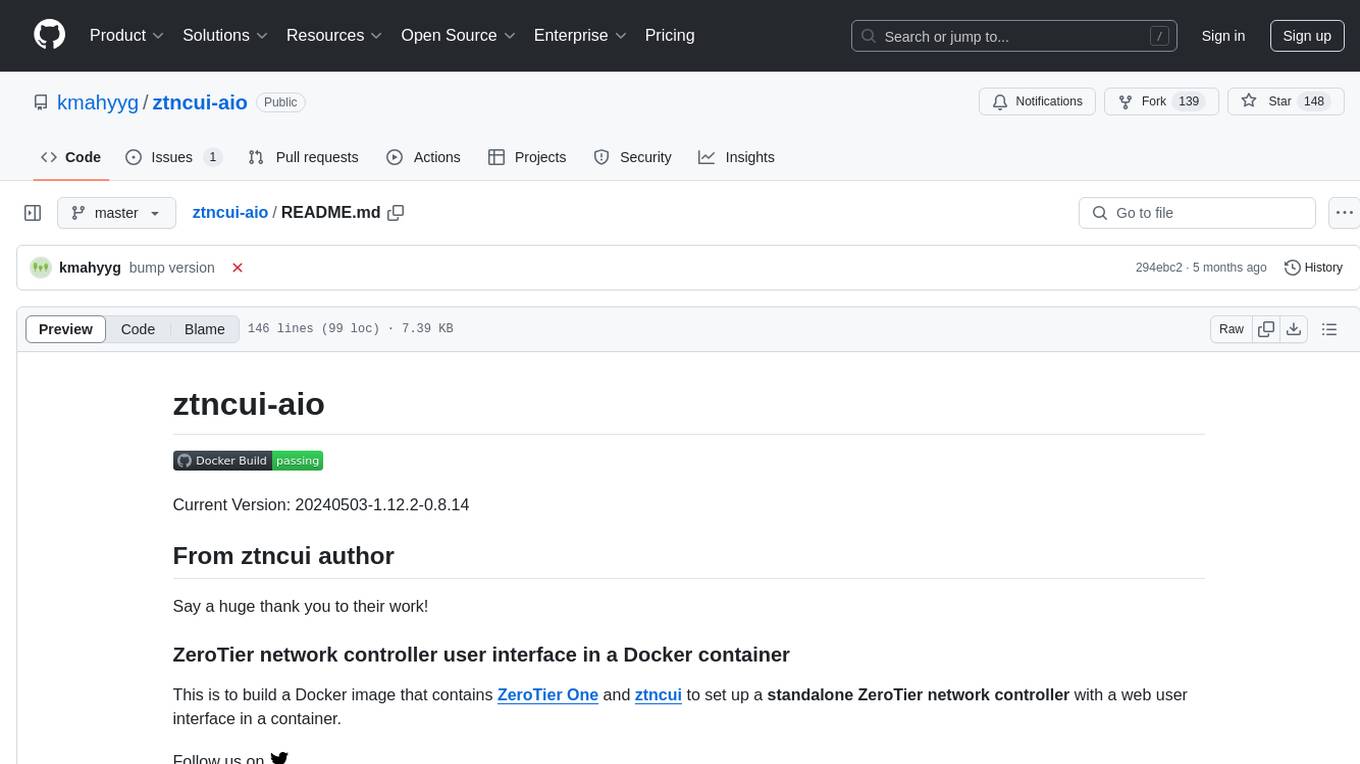
This repository contains a Docker image with ZeroTier One and ztncui to set up a standalone ZeroTier network controller with a web user interface. It provides features like Golang auto-mkworld for generating a planet file, supports local persistent storage configuration, and includes a public file server. Users can build the Docker image, set up the container with specific environment variables, and manage the ZeroTier network controller through the web interface.
README:
Current Version: 20250119-1.14.1-0.8.14
Say a huge thank you to their work!
This is to build a Docker image that contains ZeroTier One and ztncui to set up a standalone ZeroTier network controller with a web user interface in a container.
Licensed Under GNU GPLv3
We support aarch64 (arm64/v8), amd64 by default.
Armv7(means armhf) might work, but is not tested.
Others are unsupported.
$ git clone https://github.com/kmahyyg/ztncui-aio
$ docker build . --build-arg OVERLAY_S6_ARCH=<one of aarch64,x86_64> -t ghcr.io/kmahyyg/ztncui-aio:latest
Why not directly detect CPU arch? Some kernel may use non-standard expression of architecture.
Change NODEJS_MAJOR
variable in Dockerfile to use different nodejs version.
Never use node_lts.x
as your installation script of nodejs whose version might changed without further notice due to time shift.
This feature allows you to generate a planet file without using C code and compiler.
Also, due to limitation of IPC of Zerotier-One UI and multiple issues, we do NOT support customized port, you can ONLY use port 9993/udp here.
Set the following environment variable when create the container, and according to your needs:
MANDATORY | Name | Explanation | Default Value |
---|---|---|---|
no | AUTOGEN_PLANET | If set to 1, will use this node identity to generate a planet file and put to httpfs folder to serve it outside. If set to 2, will use config in /etc/zt-mkworld/mkworld.config.json . If set to 0, will do nothing. |
0 |
The reference config file can be found on ztnodeid/assets/mkworld.conf.json
.
You could also define yourself, and check the stdout output to get C header of customized planet. After that, you will find the custom planet file under http file server root and also ca certificate.
The configuration JSON can be understand like this:
{
"rootNodes": [ // array of node, can be multiple
{
"comments": "amsterdam official", // node object, comment, will auto generate if AUTOGEN_PLANET=1
"identity": "992fcf1db7:0:206ed59350b31916f749a1f85dffb3a8787dcbf83b8c6e9448d4e3ea0e3369301be716c3609344a9d1533850fb4460c50af43322bcfc8e13d3301a1f1003ceb6",
// node identity.public ^^ , if node is not initialized, will initialize at the container start
"endpoints": [
"195.181.173.159/443", // node service location, in format: ip/port, will auto generate if AUTOGEN_PLANET=1
"2a02:6ea0:c024::/443" // must be less than or equal to two endpoints, one for IPv4, one for IPv6. if you have multiple IP, set multiple node with different identity.
]
}
],
"signing": [
"previous.c25519", // planet signing key, if not exist, will generate
"current.c25519" // same, used for iteration and update
],
"output": "planet.custom", // output filename
"plID": 0, // planet numeric ID, if you don't know, do not modify, and set plRecommend to true
"plBirth": 0, // planet creation timestamp, if you don't know, do not modify, and set plRecommend to true
"plRecommend": true // set plRecommend to true, auto-recommend plID, plBirth value. For more details, read mkworld source code in zerotier-one official repo
}
$ git clone https://github.com/kmahyyg/ztncui-aio # to get a copy of denv file, otherwise make your own
$ docker pull ghcr.io/kmahyyg/ztncui-aio
$ docker run -d -p3443:3443 -p3180:3180 -p9993:9993/udp \
-v /mydata/ztncui:/opt/key-networks/ztncui/etc \
-v /mydata/zt1:/var/lib/zerotier-one \
-v /mydata/zt-mkworld-conf:/etc/zt-mkworld \
--env-file ./denv <CHANGE THIS FILE ACCORDING TO NEXT PART> \
--restart always \
--cap-add=NET_ADMIN --device /dev/net/tun:/dev/net/tun \
--name ztncui \
ghcr.io/kmahyyg/ztncui-aio # /mydata above is the data folder that you use to save the supporting files
For ZTNCUI: https://github.com/key-networks/ztncui
Set the following environment variable when create the container, and according to your needs:
MANDATORY | Name | Explanation | Default Value |
---|---|---|---|
YES | NODE_ENV | https://pugjs.org/api/express.html | production |
no | HTTPS_HOST | HTTPS_HOST | NO DEFAULT, MEANS DISABLED |
no | HTTPS_PORT | HTTPS_PORT | NO DEFAULT, MEANS DISABLED |
no | HTTP_PORT | HTTP_PORT | 3000 |
no | HTTP_ALL_INTERFACES | Listen on all interfaces, useful for reverse proxy, HTTP only | NO DEFAULT |
Note: If you do NOT set HTTP_ALL_INTERFACES
, the 3000 port will only get listened inside container, means 127.0.0.1:3000
by default.
This application does NOT have a built-in protection mechanism against brute-force attack, you should NOT directly expose it on the internet.
And you should ALWAYS NOT use a weak password.
Set the following environment variable when create the container, and according to your needs:
MANDATORY | Name | Explanation | Default Value |
---|---|---|---|
no | MYDOMAIN | generate TLS certs on the fly (if not exists) | ztncui.docker.test |
no | ZTNCUI_PASSWD | generate admin password on the fly (if not exists) | password |
YES | MYADDR | your ip address, public ip address preferred, will auto-detect if not set | NO DEFAULT |
WARNING: IF YOU DO NOT SET PASSWORD, YOU HAVE TO USE docker container logs <CONTAINER_NAME / CONTAINER_ID>
to get your random password. This is a gatekeeper.
To reset password of ztncui: delete file under /mydata/ztncui/passwd
and set the environment variable to the password you want, then re-create the container. After application has been initialized, the password should ONLY be changed from the web page.
MANDATORY | Name | Explanation | Default Value |
---|---|---|---|
no | PLANET_RETR_PUBLIC | File server listened globally or only local | NO DEFAULT |
If PLANET_RETR_PUBLIC
is set, then file server will listen on 0.0.0.0
, otherwise, 127.0.0.1
.
This image exposed an http server at port 3180, you could save file in /mydata/ztncui/httpfs/
to serve it.
(You could use this to build your own root server and distribute planet file, even though, that won't hurt you, I still suggest to set a protection for both http servers in front.)
This script use https:///ip.sb for public IP detection purpose, which is blocked in some area of China Mainland. Under this circumstance, the program will try to detect public IP using ifconfig
tool and might lead to unwanted result, to prevent this, make sure you set MYADDR
environment variable when docker container is up.
This repo (https://github.com/kmahyyg/ztncui-aio) only accept Issues and PRs in English. Other languages will be closed directly without any further notice. If you come from some non-English countries, use Google Translate, and state that at the beginning of the text body.
For Tasks:
Click tags to check more tools for each tasksFor Jobs:
Alternative AI tools for ztncui-aio
Similar Open Source Tools
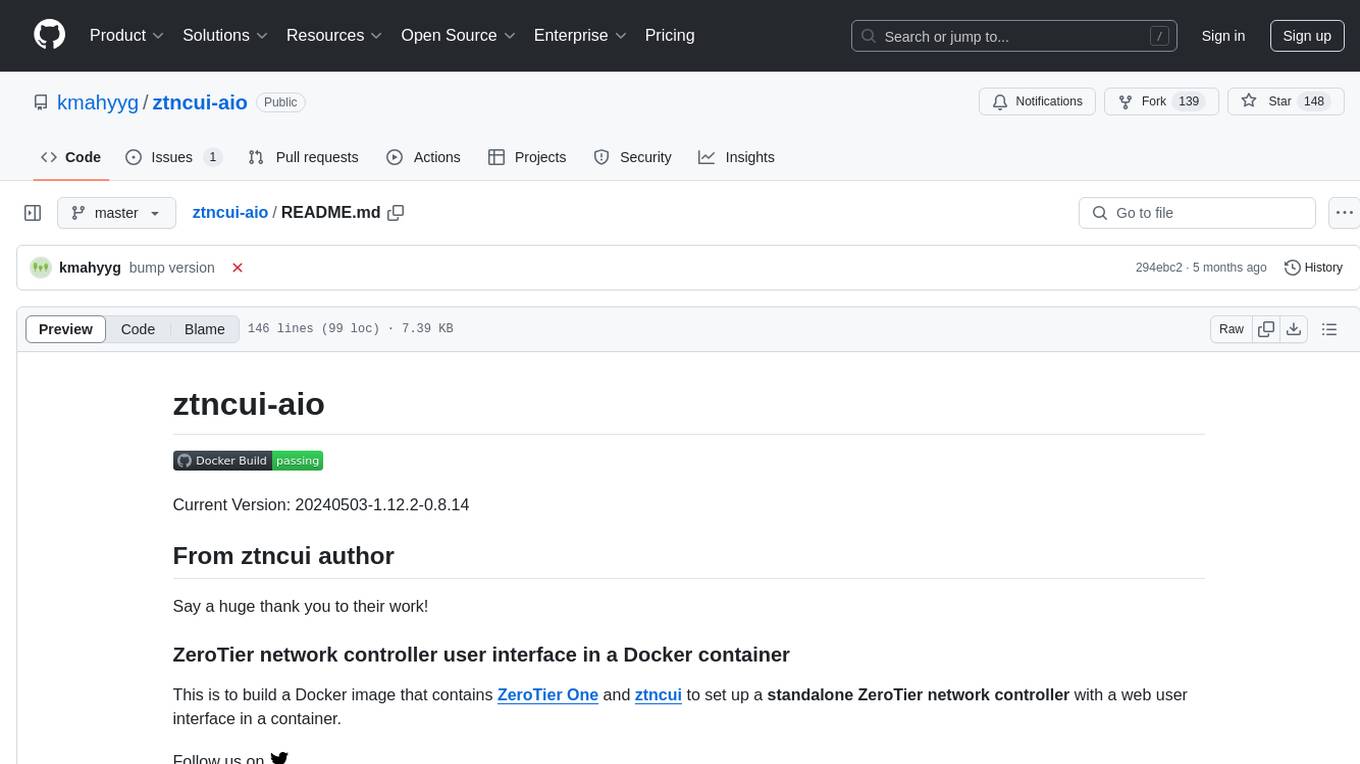
ztncui-aio
This repository contains a Docker image with ZeroTier One and ztncui to set up a standalone ZeroTier network controller with a web user interface. It provides features like Golang auto-mkworld for generating a planet file, supports local persistent storage configuration, and includes a public file server. Users can build the Docker image, set up the container with specific environment variables, and manage the ZeroTier network controller through the web interface.
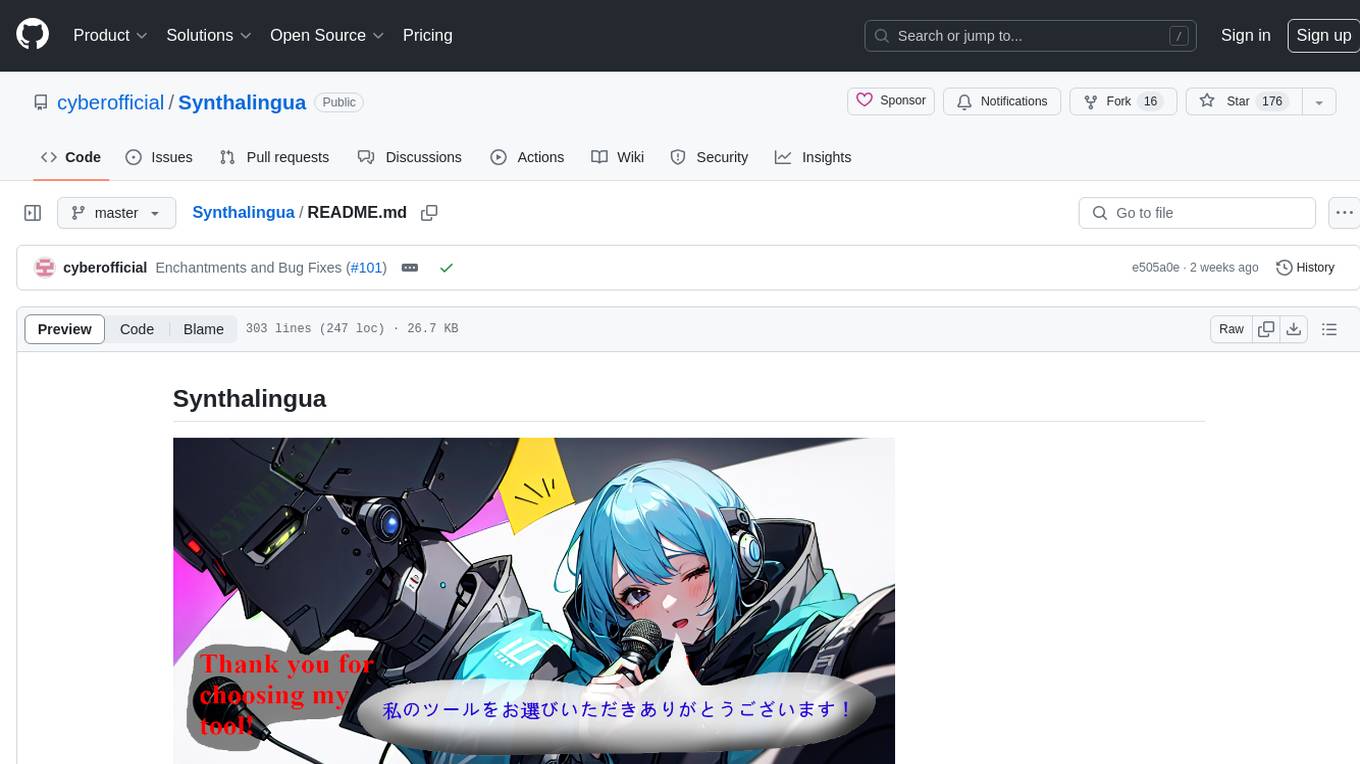
Synthalingua
Synthalingua is an advanced, self-hosted tool that leverages artificial intelligence to translate audio from various languages into English in near real time. It offers multilingual outputs and utilizes GPU and CPU resources for optimized performance. Although currently in beta, it is actively developed with regular updates to enhance capabilities. The tool is not intended for professional use but for fun, language learning, and enjoying content at a reasonable pace. Users must ensure speakers speak clearly for accurate translations. It is not a replacement for human translators and users assume their own risk and liability when using the tool.
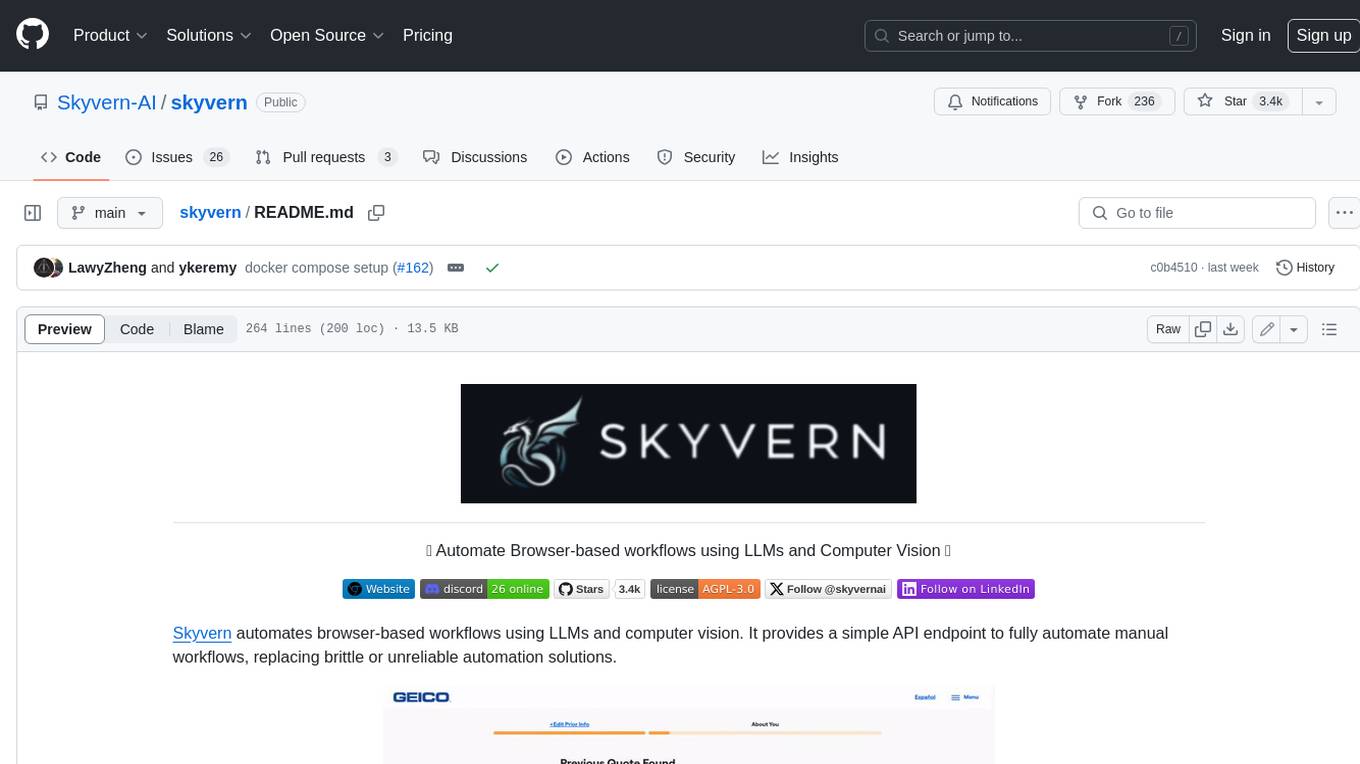
skyvern
Skyvern automates browser-based workflows using LLMs and computer vision. It provides a simple API endpoint to fully automate manual workflows, replacing brittle or unreliable automation solutions. Traditional approaches to browser automations required writing custom scripts for websites, often relying on DOM parsing and XPath-based interactions which would break whenever the website layouts changed. Instead of only relying on code-defined XPath interactions, Skyvern adds computer vision and LLMs to the mix to parse items in the viewport in real-time, create a plan for interaction and interact with them. This approach gives us a few advantages: 1. Skyvern can operate on websites it’s never seen before, as it’s able to map visual elements to actions necessary to complete a workflow, without any customized code 2. Skyvern is resistant to website layout changes, as there are no pre-determined XPaths or other selectors our system is looking for while trying to navigate 3. Skyvern leverages LLMs to reason through interactions to ensure we can cover complex situations. Examples include: 1. If you wanted to get an auto insurance quote from Geico, the answer to a common question “Were you eligible to drive at 18?” could be inferred from the driver receiving their license at age 16 2. If you were doing competitor analysis, it’s understanding that an Arnold Palmer 22 oz can at 7/11 is almost definitely the same product as a 23 oz can at Gopuff (even though the sizes are slightly different, which could be a rounding error!) Want to see examples of Skyvern in action? Jump to #real-world-examples-of- skyvern
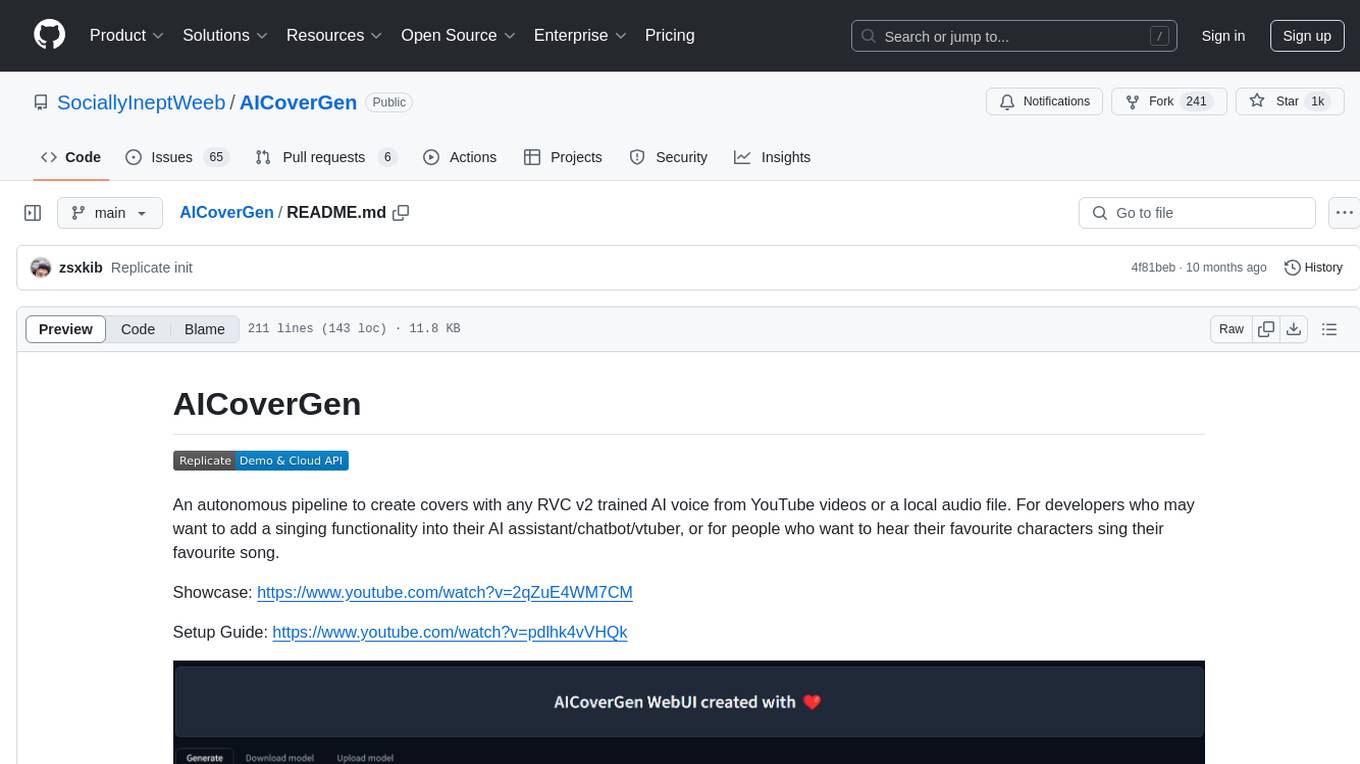
AICoverGen
AICoverGen is an autonomous pipeline designed to create covers using any RVC v2 trained AI voice from YouTube videos or local audio files. It caters to developers looking to incorporate singing functionality into AI assistants/chatbots/vtubers, as well as individuals interested in hearing their favorite characters sing. The tool offers a WebUI for easy conversions, cover generation from local audio files, volume control for vocals and instrumentals, pitch detection method control, pitch change for vocals and instrumentals, and audio output format options. Users can also download and upload RVC models via the WebUI, run the pipeline using CLI, and access various advanced options for voice conversion and audio mixing.
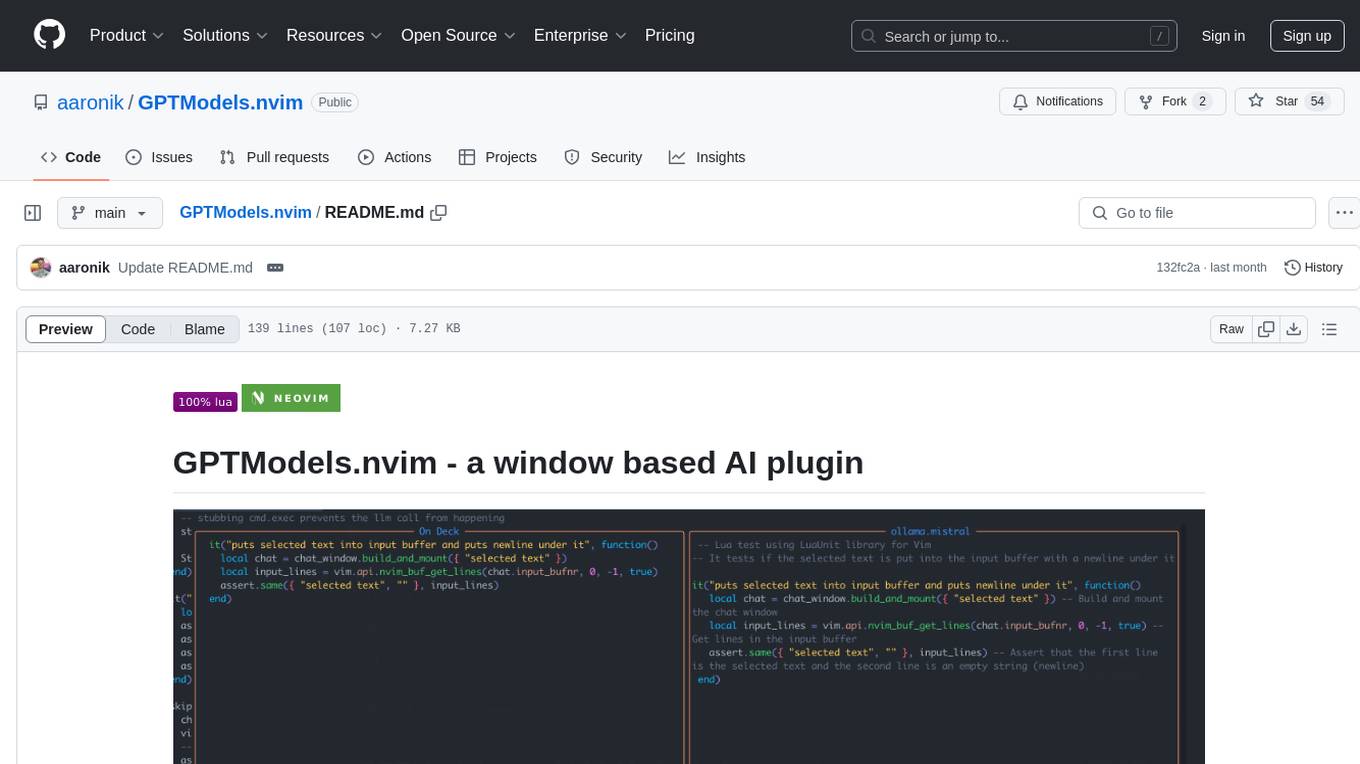
GPTModels.nvim
GPTModels.nvim is a window-based AI plugin for Neovim that enhances workflow with AI LLMs. It provides two popup windows for chat and code editing, focusing on stability and user experience. The plugin supports OpenAI and Ollama, includes LSP diagnostics, file inclusion, background processing, request cancellation, selection inclusion, and filetype inclusion. Developed with stability in mind, the plugin offers a seamless user experience with various features to streamline AI integration in Neovim.
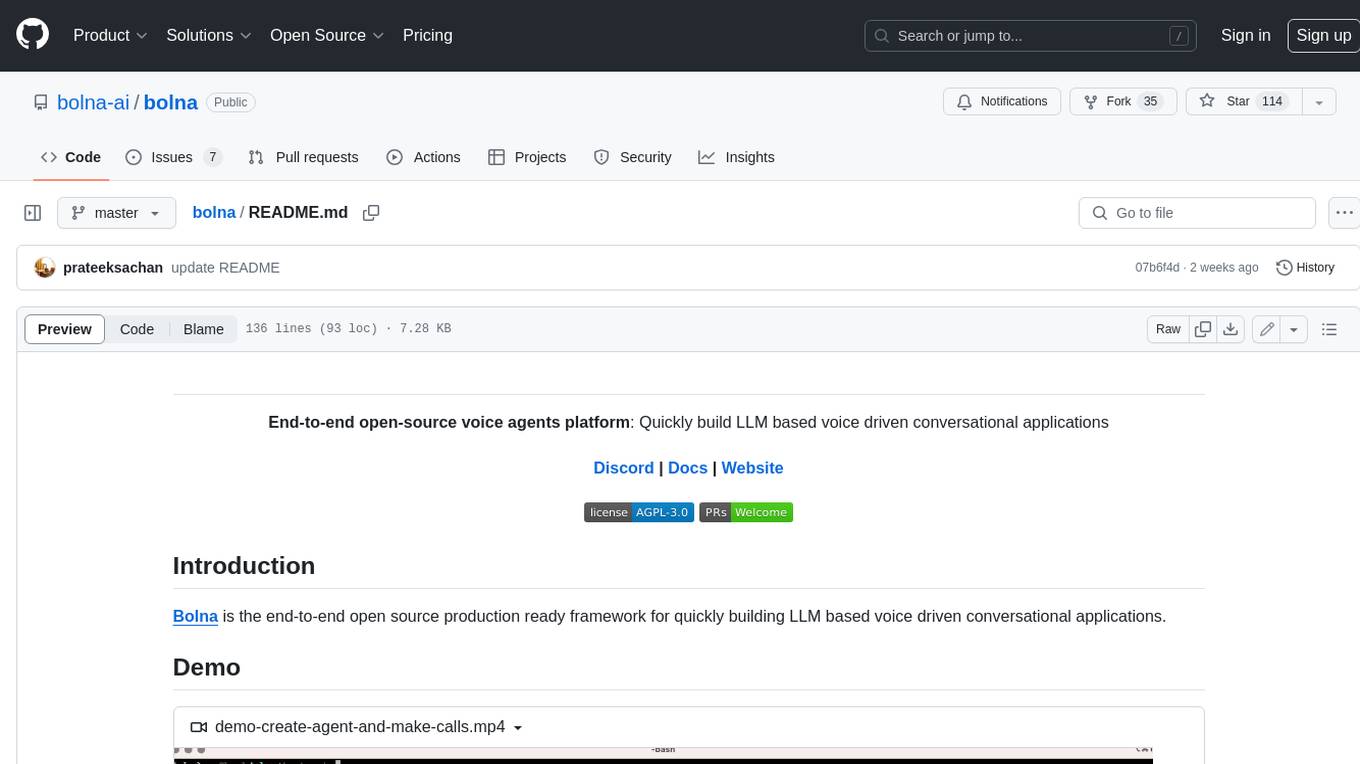
bolna
Bolna is an open-source platform for building voice-driven conversational applications using large language models (LLMs). It provides a comprehensive set of tools and integrations to handle various aspects of voice-based interactions, including telephony, transcription, LLM-based conversation handling, and text-to-speech synthesis. Bolna simplifies the process of creating voice agents that can perform tasks such as initiating phone calls, transcribing conversations, generating LLM-powered responses, and synthesizing speech. It supports multiple providers for each component, allowing users to customize their setup based on their specific needs. Bolna is designed to be easy to use, with a straightforward local setup process and well-documented APIs. It is also extensible, enabling users to integrate with other telephony providers or add custom functionality.
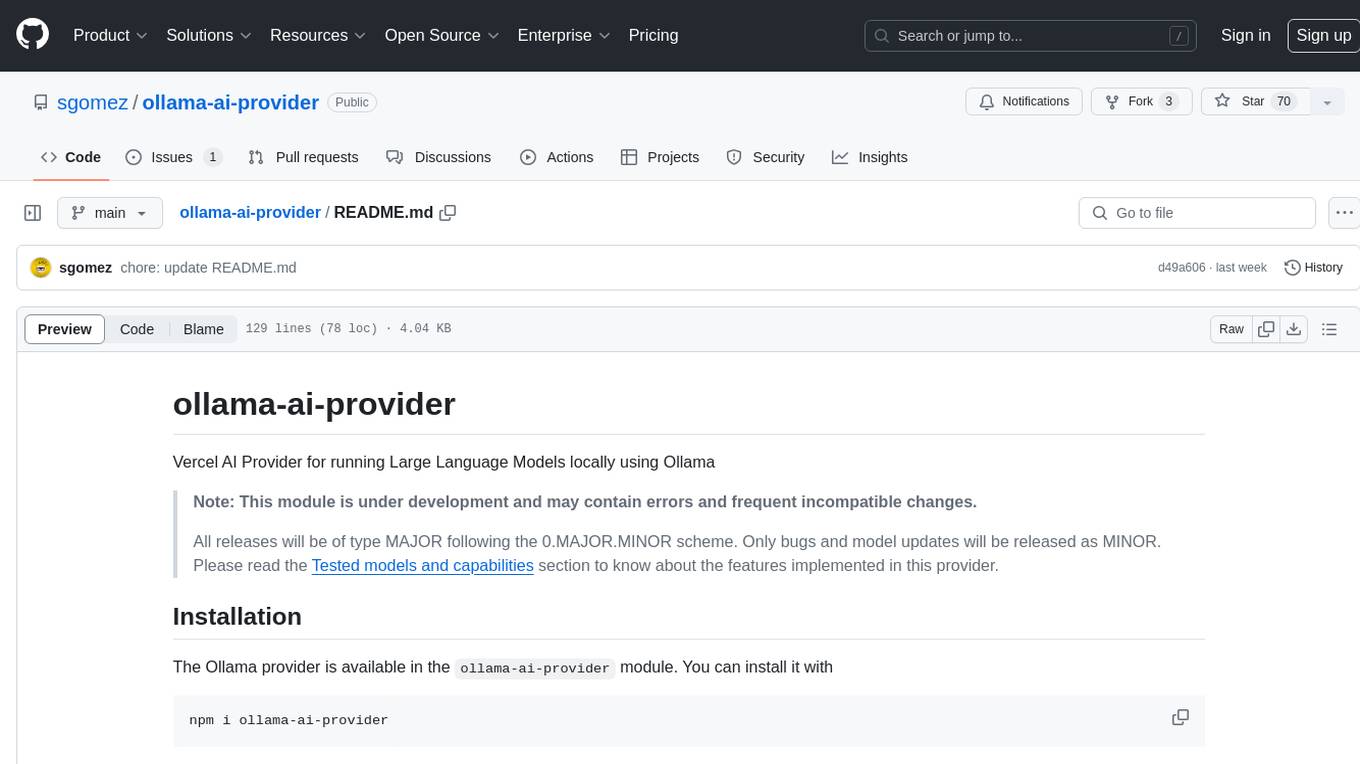
ollama-ai-provider
Vercel AI Provider for running Large Language Models locally using Ollama. This module is under development and may contain errors and frequent incompatible changes. It provides the capability of generating and streaming text and objects, with features like image input, object generation, tool usage simulation, tool streaming simulation, intercepting fetch requests, and provider management. The provider can be customized with optional settings like baseURL and headers.
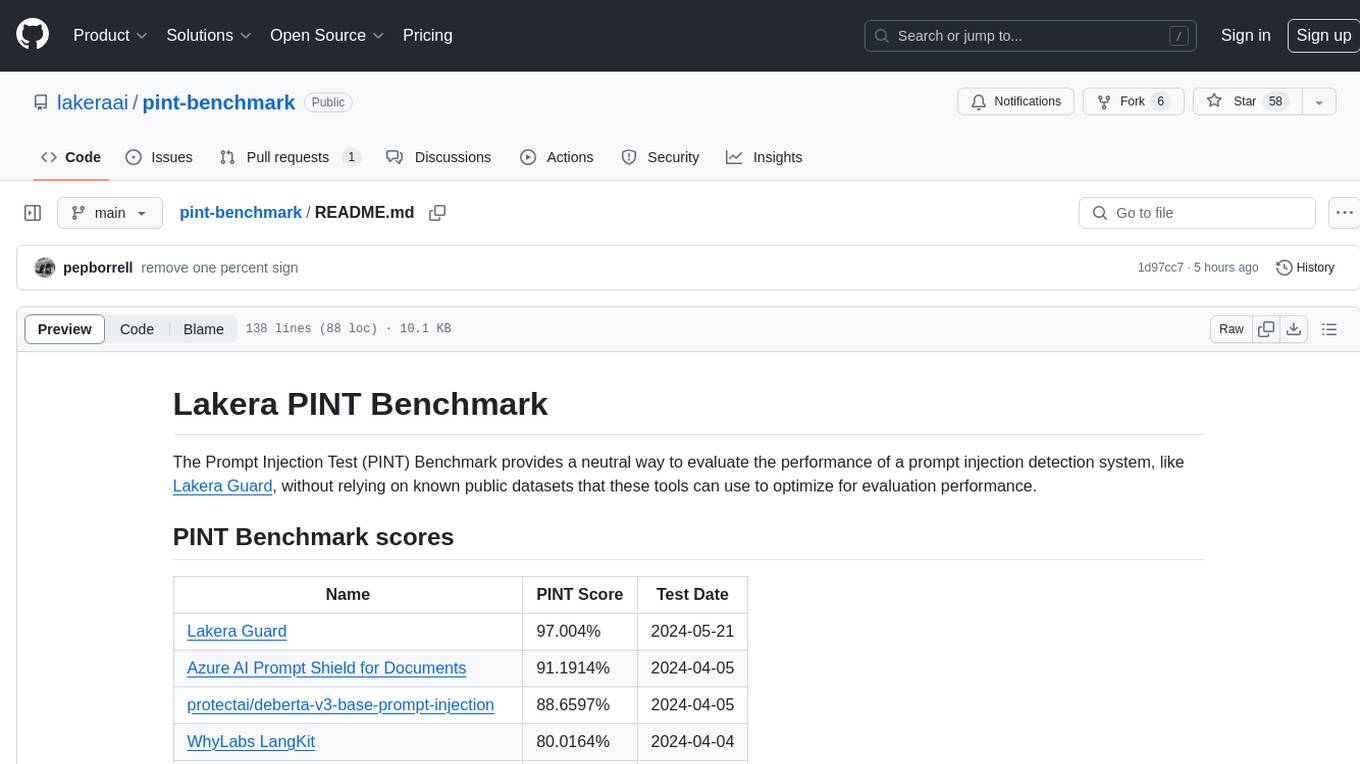
pint-benchmark
The Lakera PINT Benchmark provides a neutral evaluation method for prompt injection detection systems, offering a dataset of English inputs with prompt injections, jailbreaks, benign inputs, user-agent chats, and public document excerpts. The dataset is designed to be challenging and representative, with plans for future enhancements. The benchmark aims to be unbiased and accurate, welcoming contributions to improve prompt injection detection. Users can evaluate prompt injection detection systems using the provided Jupyter Notebook. The dataset structure is specified in YAML format, allowing users to prepare their datasets for benchmarking. Evaluation examples and resources are provided to assist users in evaluating prompt injection detection models and tools.
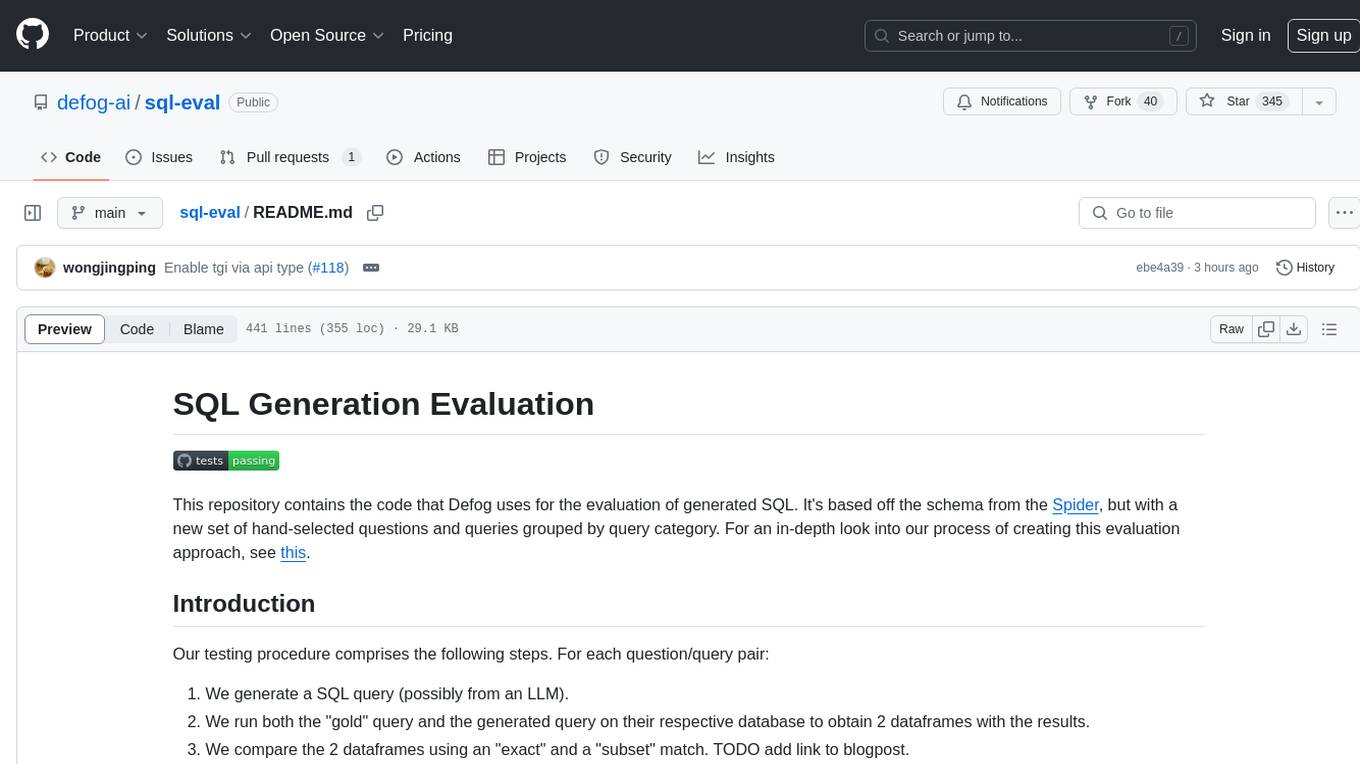
sql-eval
This repository contains the code that Defog uses for the evaluation of generated SQL. It's based off the schema from the Spider, but with a new set of hand-selected questions and queries grouped by query category. The testing procedure involves generating a SQL query, running both the 'gold' query and the generated query on their respective database to obtain dataframes with the results, comparing the dataframes using an 'exact' and a 'subset' match, logging these alongside other metrics of interest, and aggregating the results for reporting. The repository provides comprehensive instructions for installing dependencies, starting a Postgres instance, importing data into Postgres, importing data into Snowflake, using private data, implementing a query generator, and running the test with different runners.
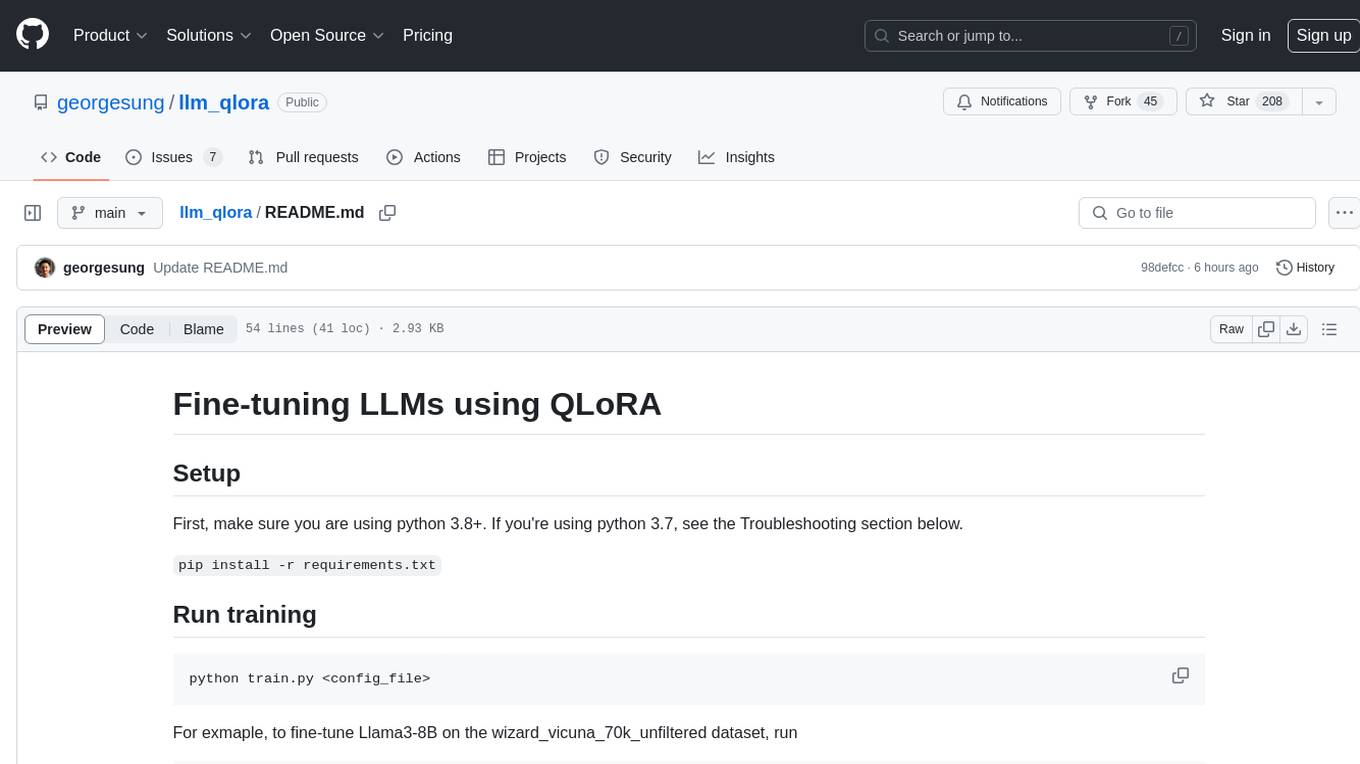
llm_qlora
LLM_QLoRA is a repository for fine-tuning Large Language Models (LLMs) using QLoRA methodology. It provides scripts for training LLMs on custom datasets, pushing models to HuggingFace Hub, and performing inference. Additionally, it includes models trained on HuggingFace Hub, a blog post detailing the QLoRA fine-tuning process, and instructions for converting and quantizing models. The repository also addresses troubleshooting issues related to Python versions and dependencies.
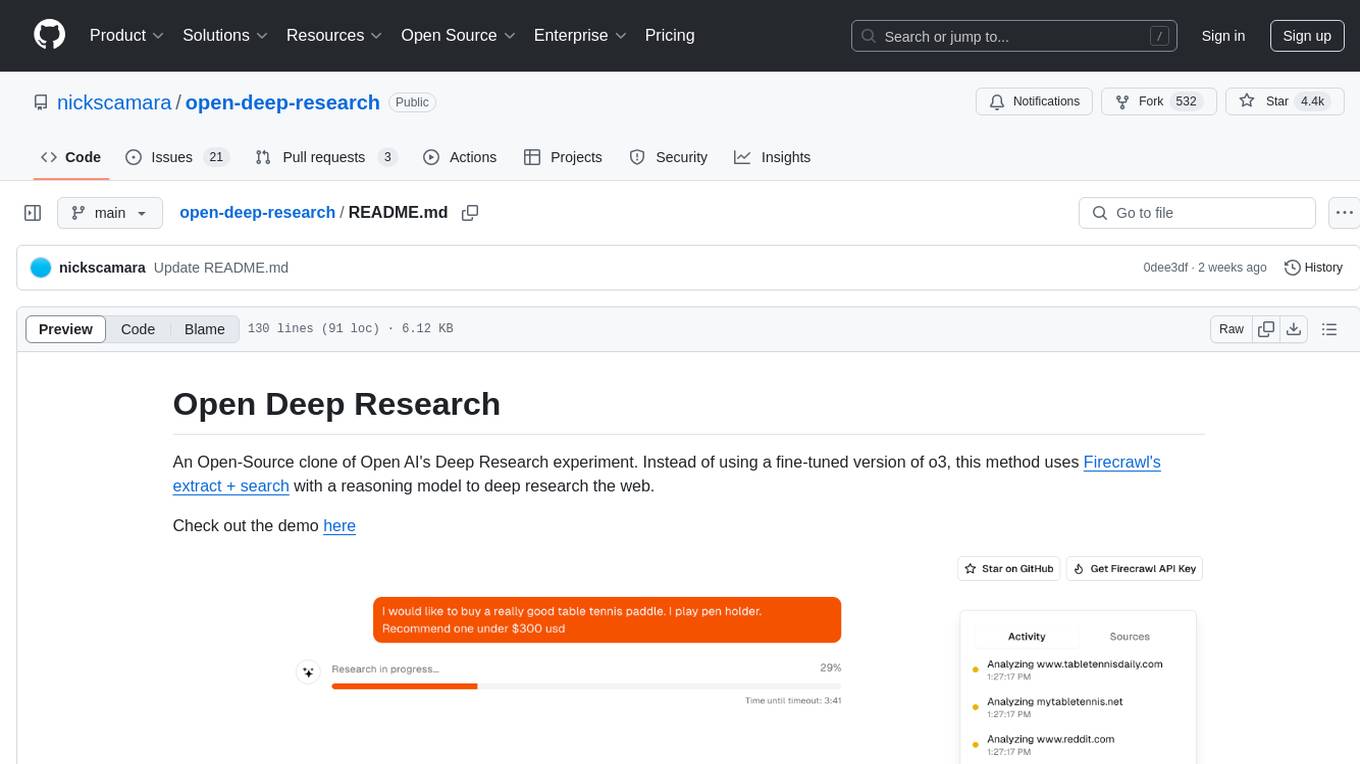
open-deep-research
Open Deep Research is an open-source project that serves as a clone of Open AI's Deep Research experiment. It utilizes Firecrawl's extract and search method along with a reasoning model to conduct in-depth research on the web. The project features Firecrawl Search + Extract, real-time data feeding to AI via search, structured data extraction from multiple websites, Next.js App Router for advanced routing, React Server Components and Server Actions for server-side rendering, AI SDK for generating text and structured objects, support for various model providers, styling with Tailwind CSS, data persistence with Vercel Postgres and Blob, and simple and secure authentication with NextAuth.js.
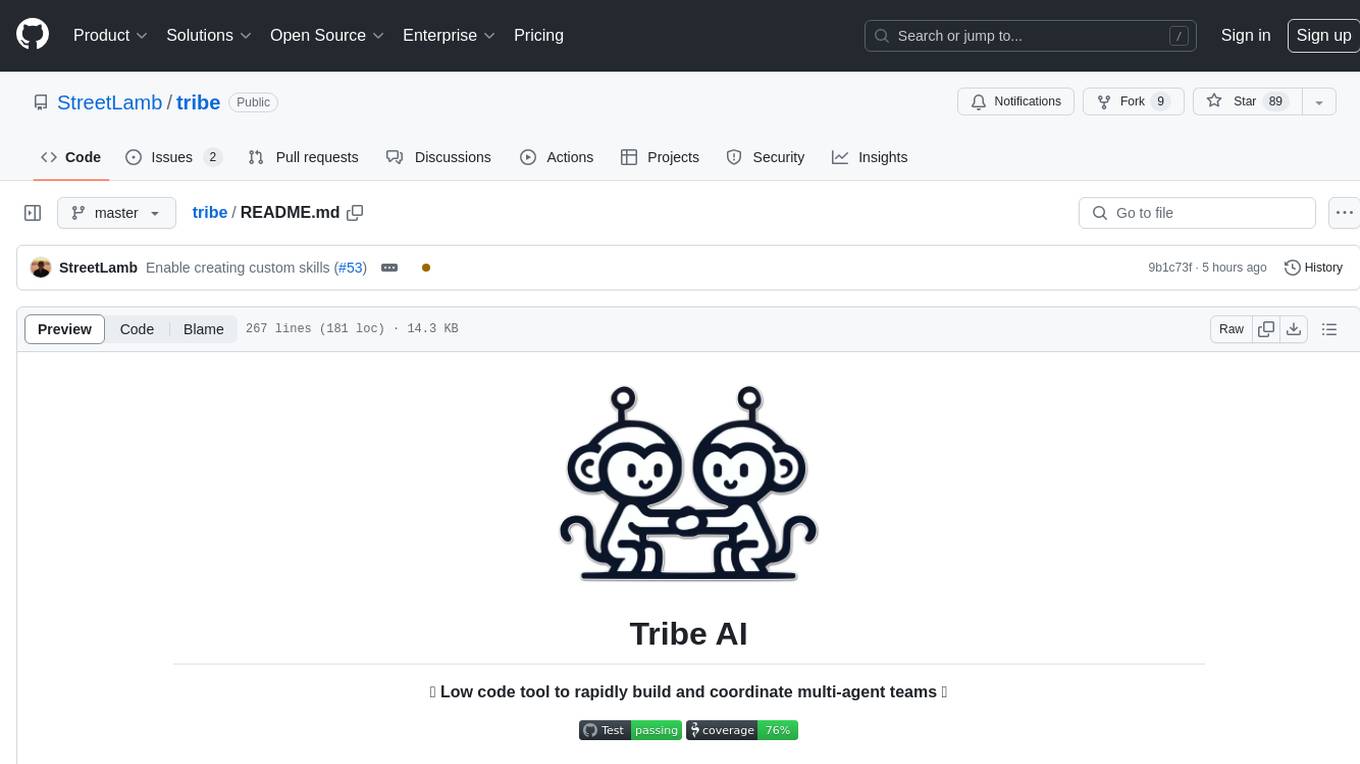
tribe
Tribe AI is a low code tool designed to rapidly build and coordinate multi-agent teams. It leverages the langgraph framework to customize and coordinate teams of agents, allowing tasks to be split among agents with different strengths for faster and better problem-solving. The tool supports persistent conversations, observability, tool calling, human-in-the-loop functionality, easy deployment with Docker, and multi-tenancy for managing multiple users and teams.
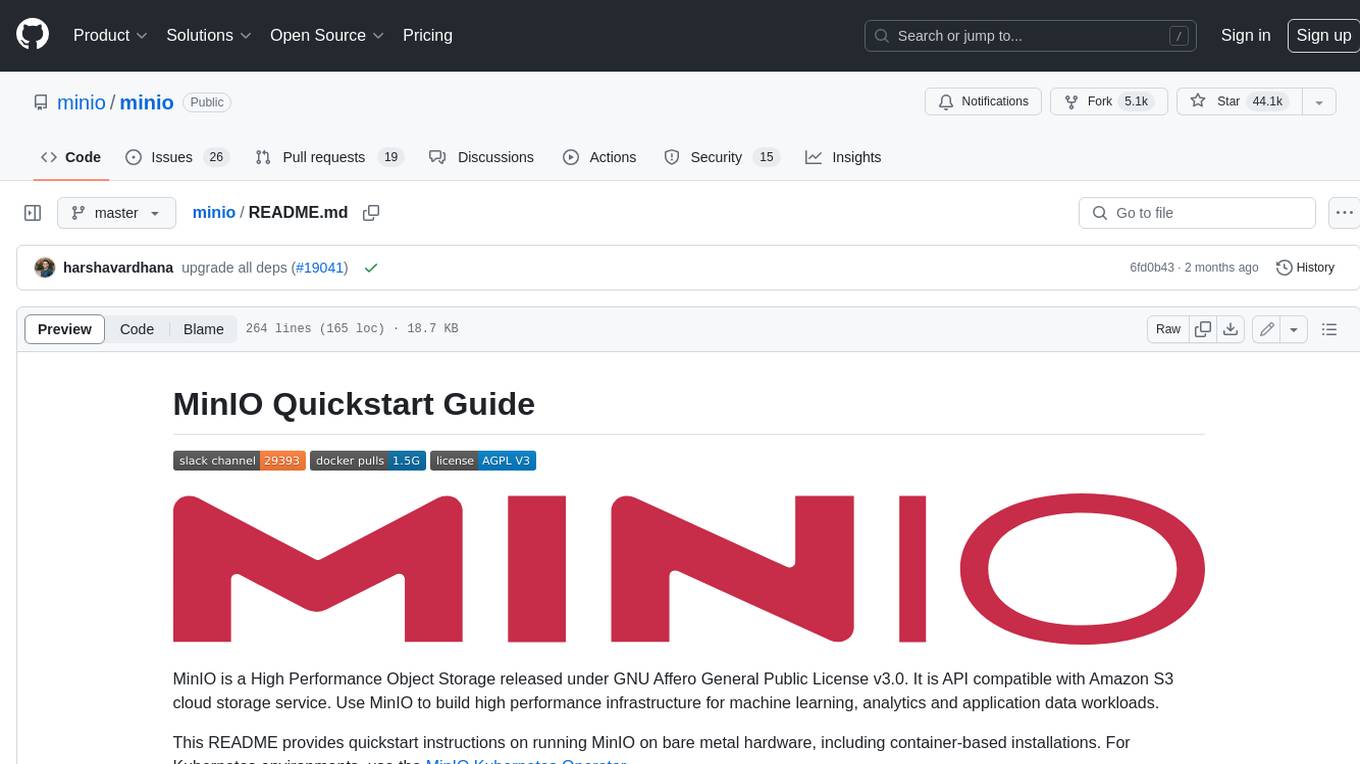
minio
MinIO is a High Performance Object Storage released under GNU Affero General Public License v3.0. It is API compatible with Amazon S3 cloud storage service. Use MinIO to build high performance infrastructure for machine learning, analytics and application data workloads.
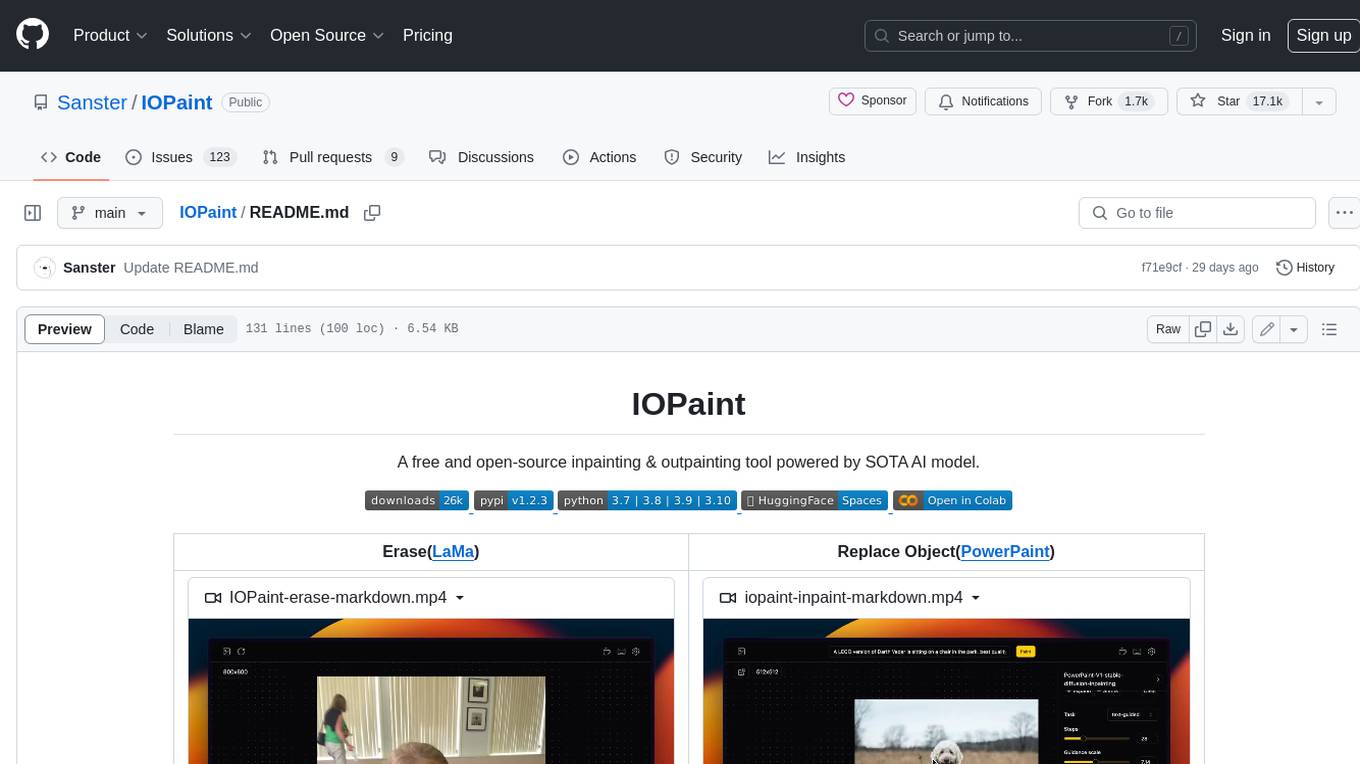
IOPaint
IOPaint is a free and open-source inpainting & outpainting tool powered by SOTA AI model. It supports various AI models to perform erase, inpainting, or outpainting tasks. Users can remove unwanted objects, defects, watermarks, or people from images using erase models. Additionally, diffusion models can replace objects or perform outpainting. The tool also offers plugins for interactive object segmentation, background removal, anime segmentation, super resolution, face restoration, and file management. IOPaint provides a web UI for easy access to the latest AI models and supports batch processing of images through the command line. Developers can contribute to the project by installing front-end dependencies, setting up the backend, and starting the development environment for both front-end and back-end components.
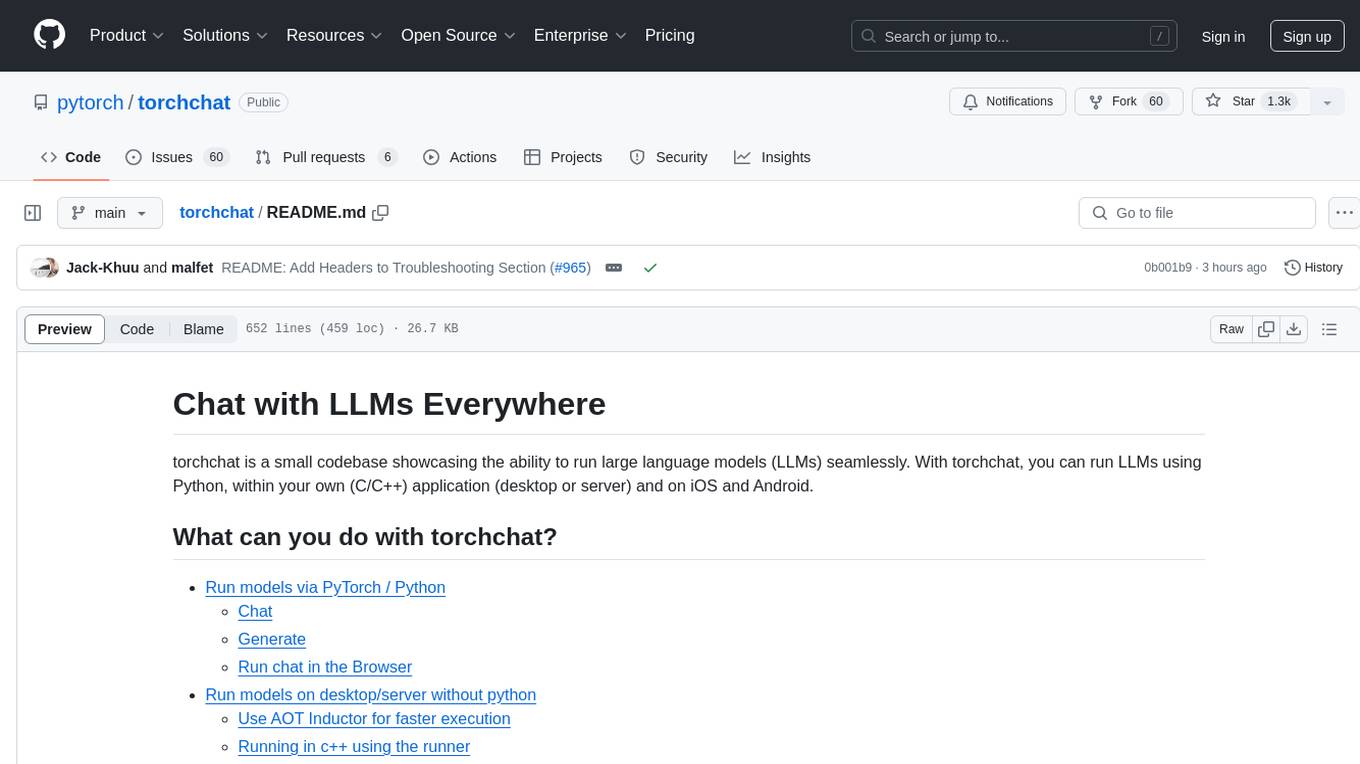
torchchat
torchchat is a codebase showcasing the ability to run large language models (LLMs) seamlessly. It allows running LLMs using Python in various environments such as desktop, server, iOS, and Android. The tool supports running models via PyTorch, chatting, generating text, running chat in the browser, and running models on desktop/server without Python. It also provides features like AOT Inductor for faster execution, running in C++ using the runner, and deploying and running on iOS and Android. The tool supports popular hardware and OS including Linux, Mac OS, Android, and iOS, with various data types and execution modes available.
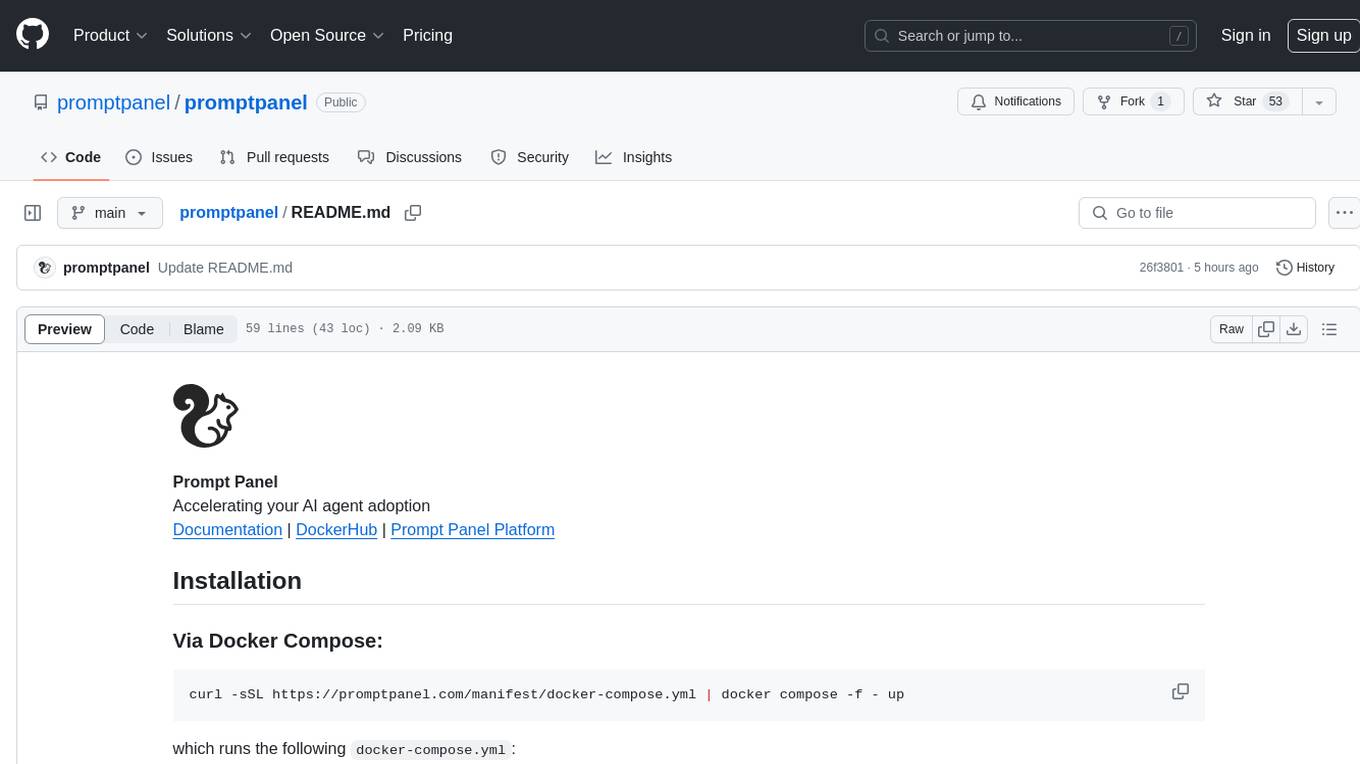
promptpanel
Prompt Panel is a tool designed to accelerate the adoption of AI agents by providing a platform where users can run large language models across any inference provider, create custom agent plugins, and use their own data safely. The tool allows users to break free from walled-gardens and have full control over their models, conversations, and logic. With Prompt Panel, users can pair their data with any language model, online or offline, and customize the system to meet their unique business needs without any restrictions.
For similar tasks
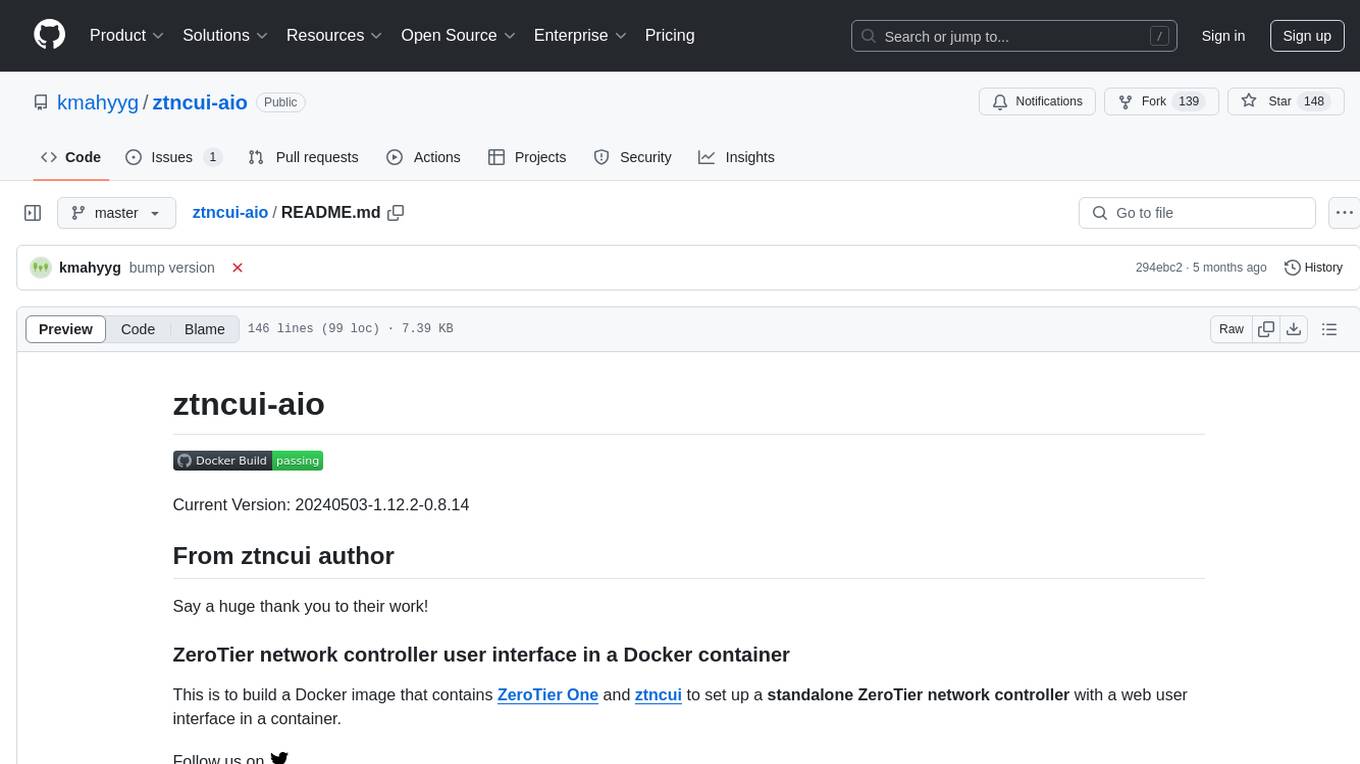
ztncui-aio
This repository contains a Docker image with ZeroTier One and ztncui to set up a standalone ZeroTier network controller with a web user interface. It provides features like Golang auto-mkworld for generating a planet file, supports local persistent storage configuration, and includes a public file server. Users can build the Docker image, set up the container with specific environment variables, and manage the ZeroTier network controller through the web interface.
For similar jobs
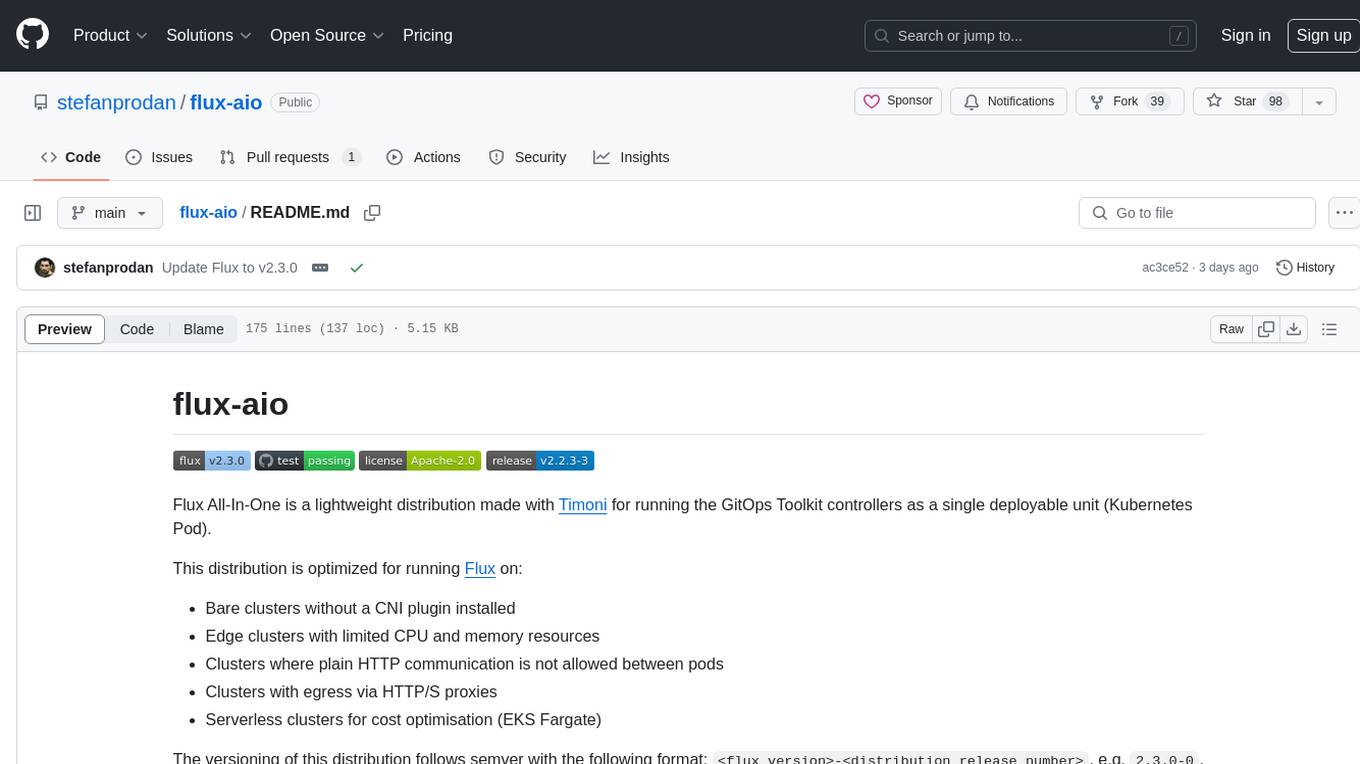
flux-aio
Flux All-In-One is a lightweight distribution optimized for running the GitOps Toolkit controllers as a single deployable unit on Kubernetes clusters. It is designed for bare clusters, edge clusters, clusters with restricted communication, clusters with egress via proxies, and serverless clusters. The distribution follows semver versioning and provides documentation for specifications, installation, upgrade, OCI sync configuration, Git sync configuration, and multi-tenancy configuration. Users can deploy Flux using Timoni CLI and a Timoni Bundle file, fine-tune installation options, sync from public Git repositories, bootstrap repositories, and uninstall Flux without affecting reconciled workloads.
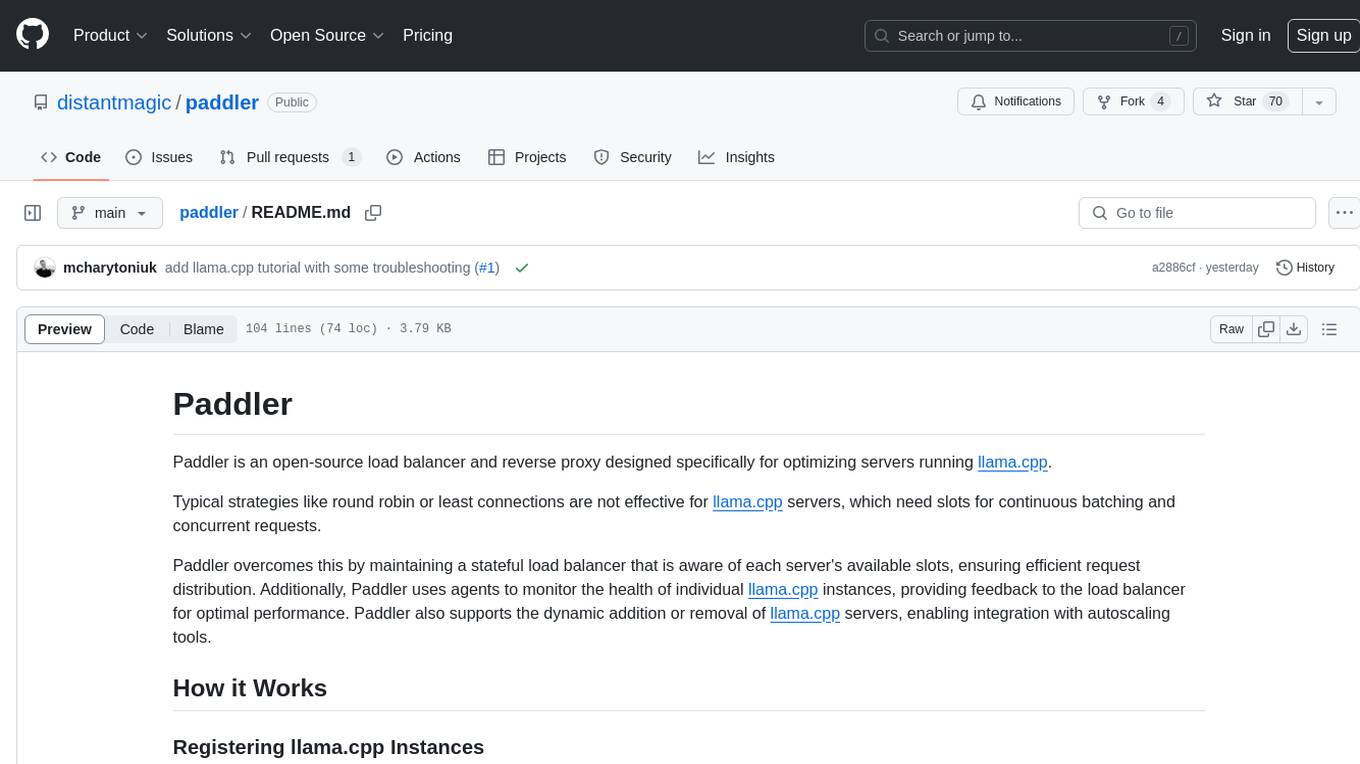
paddler
Paddler is an open-source load balancer and reverse proxy designed specifically for optimizing servers running llama.cpp. It overcomes typical load balancing challenges by maintaining a stateful load balancer that is aware of each server's available slots, ensuring efficient request distribution. Paddler also supports dynamic addition or removal of servers, enabling integration with autoscaling tools.
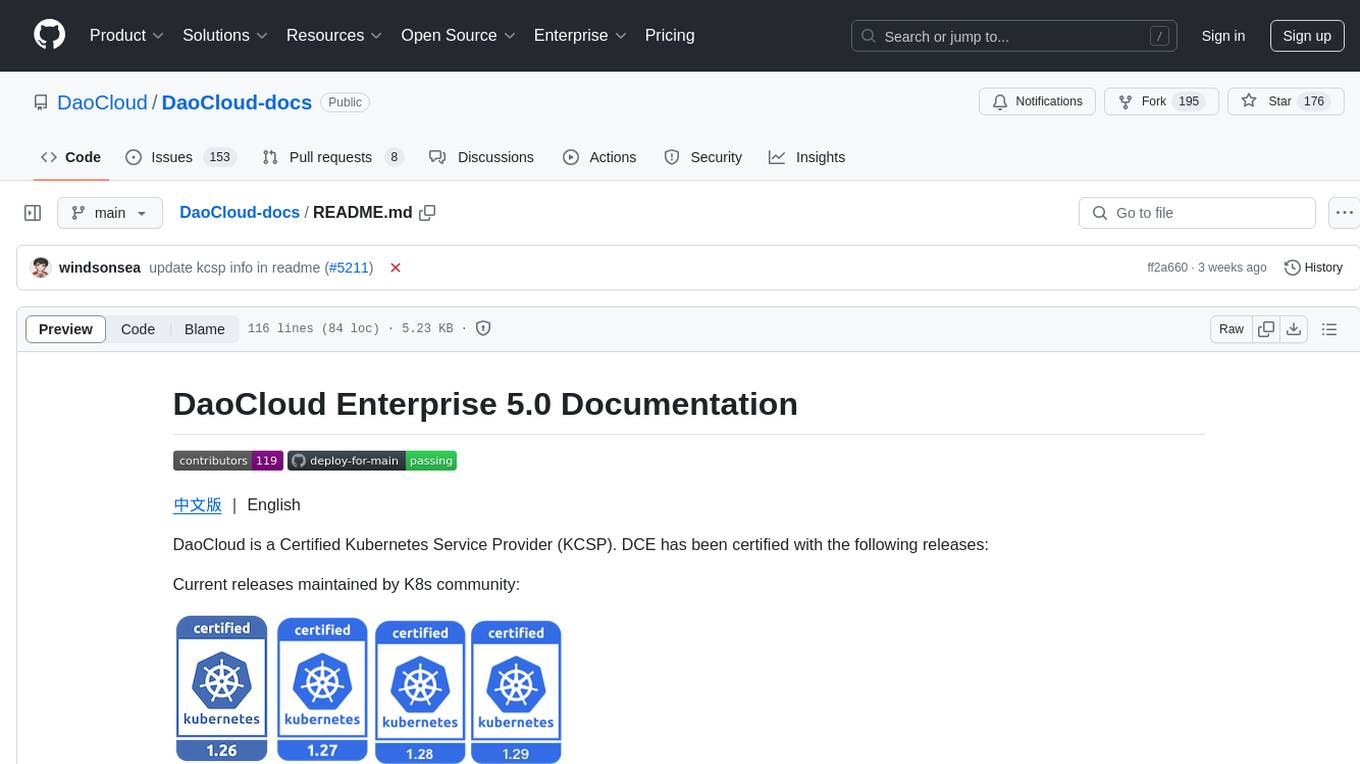
DaoCloud-docs
DaoCloud Enterprise 5.0 Documentation provides detailed information on using DaoCloud, a Certified Kubernetes Service Provider. The documentation covers current and legacy versions, workflow control using GitOps, and instructions for opening a PR and previewing changes locally. It also includes naming conventions, writing tips, references, and acknowledgments to contributors. Users can find guidelines on writing, contributing, and translating pages, along with using tools like MkDocs, Docker, and Poetry for managing the documentation.
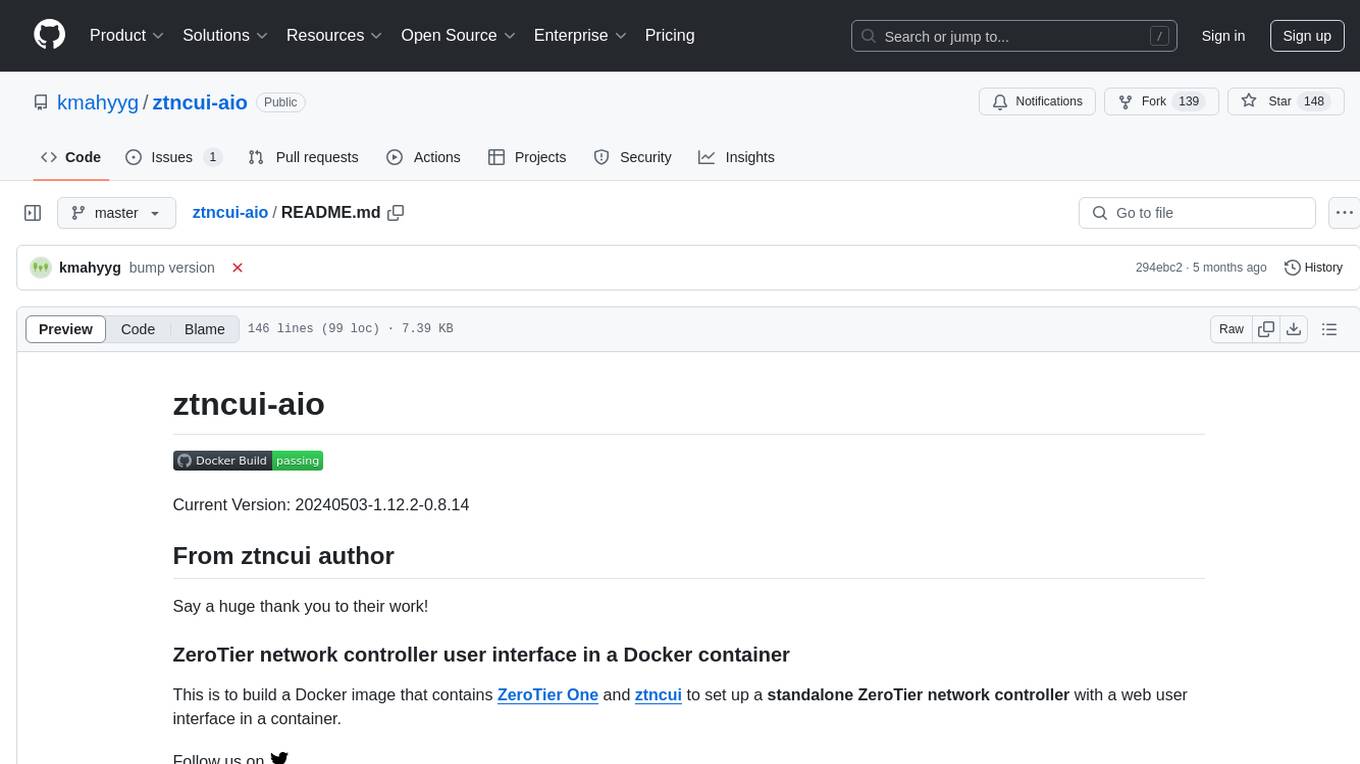
ztncui-aio
This repository contains a Docker image with ZeroTier One and ztncui to set up a standalone ZeroTier network controller with a web user interface. It provides features like Golang auto-mkworld for generating a planet file, supports local persistent storage configuration, and includes a public file server. Users can build the Docker image, set up the container with specific environment variables, and manage the ZeroTier network controller through the web interface.
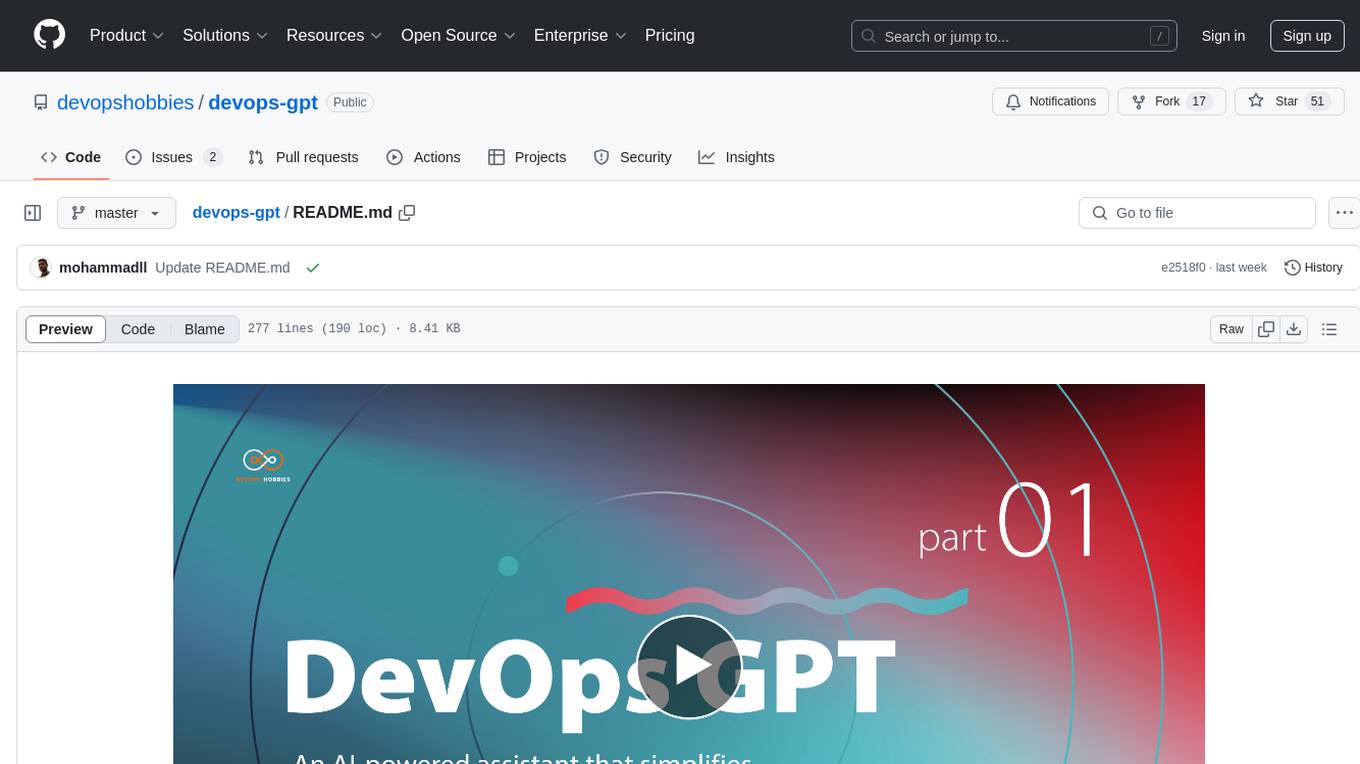
devops-gpt
DevOpsGPT is a revolutionary tool designed to streamline your workflow and empower you to build systems and automate tasks with ease. Tired of spending hours on repetitive DevOps tasks? DevOpsGPT is here to help! Whether you're setting up infrastructure, speeding up deployments, or tackling any other DevOps challenge, our app can make your life easier and more productive. With DevOpsGPT, you can expect faster task completion, simplified workflows, and increased efficiency. Ready to experience the DevOpsGPT difference? Visit our website, sign in or create an account, start exploring the features, and share your feedback to help us improve. DevOpsGPT will become an essential tool in your DevOps toolkit.
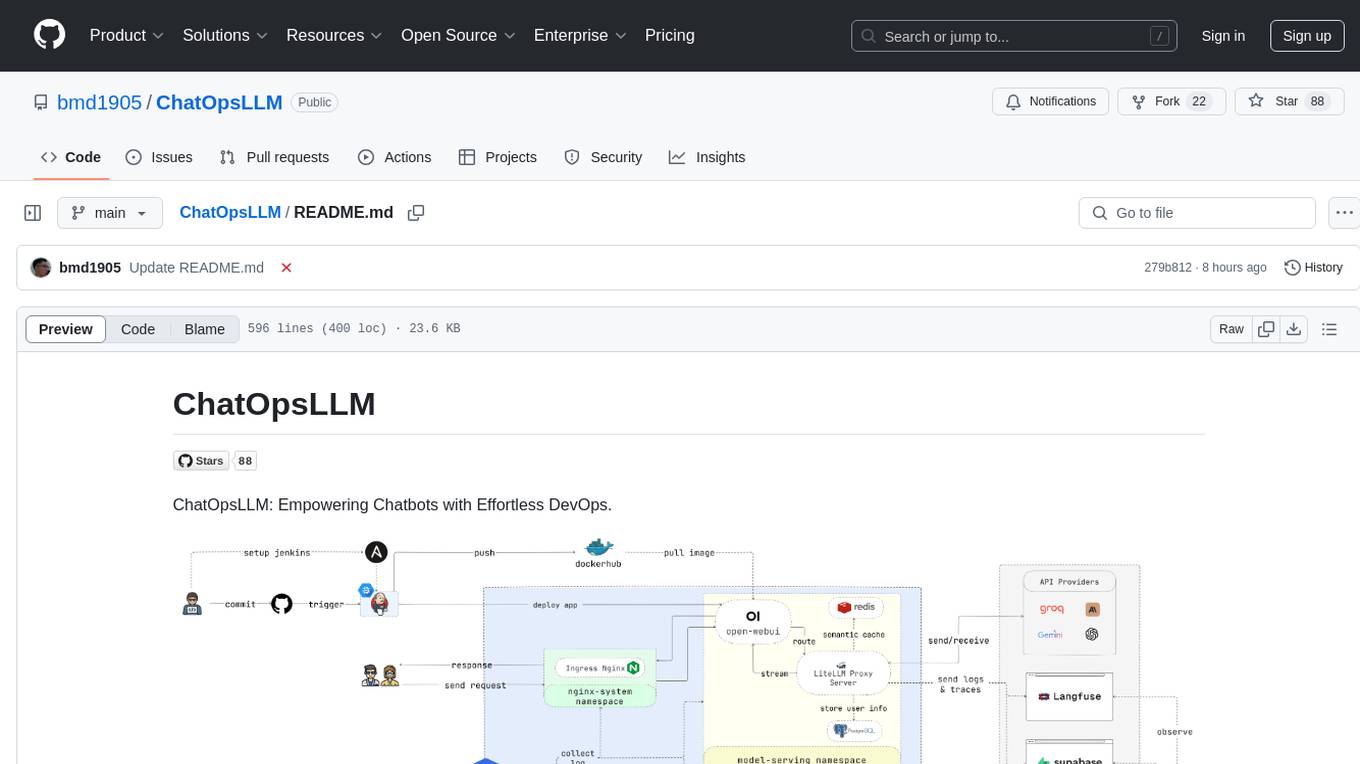
ChatOpsLLM
ChatOpsLLM is a project designed to empower chatbots with effortless DevOps capabilities. It provides an intuitive interface and streamlined workflows for managing and scaling language models. The project incorporates robust MLOps practices, including CI/CD pipelines with Jenkins and Ansible, monitoring with Prometheus and Grafana, and centralized logging with the ELK stack. Developers can find detailed documentation and instructions on the project's website.
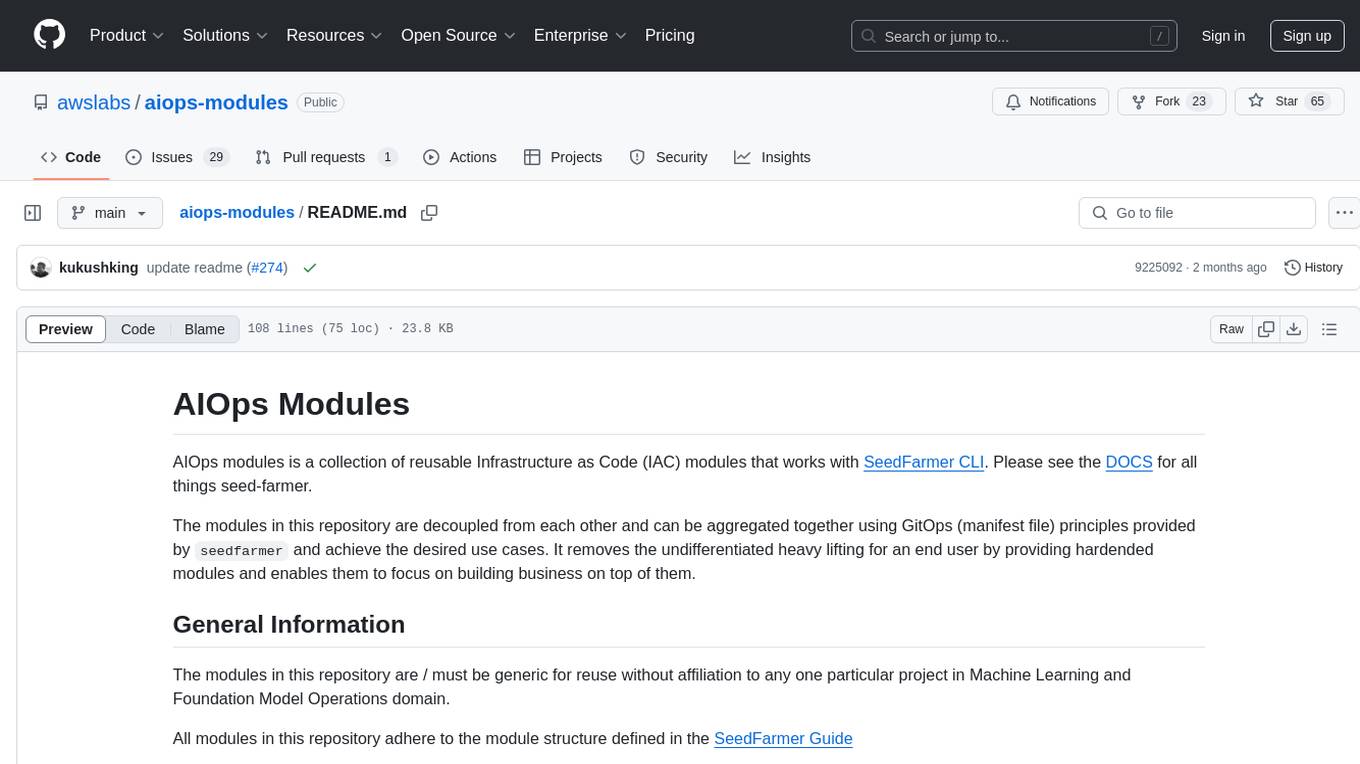
aiops-modules
AIOps Modules is a collection of reusable Infrastructure as Code (IAC) modules that work with SeedFarmer CLI. The modules are decoupled and can be aggregated using GitOps principles to achieve desired use cases, removing heavy lifting for end users. They must be generic for reuse in Machine Learning and Foundation Model Operations domain, adhering to SeedFarmer Guide structure. The repository includes deployment steps, project manifests, and various modules for SageMaker, Mlflow, FMOps/LLMOps, MWAA, Step Functions, EKS, and example use cases. It also supports Industry Data Framework (IDF) and Autonomous Driving Data Framework (ADDF) Modules.
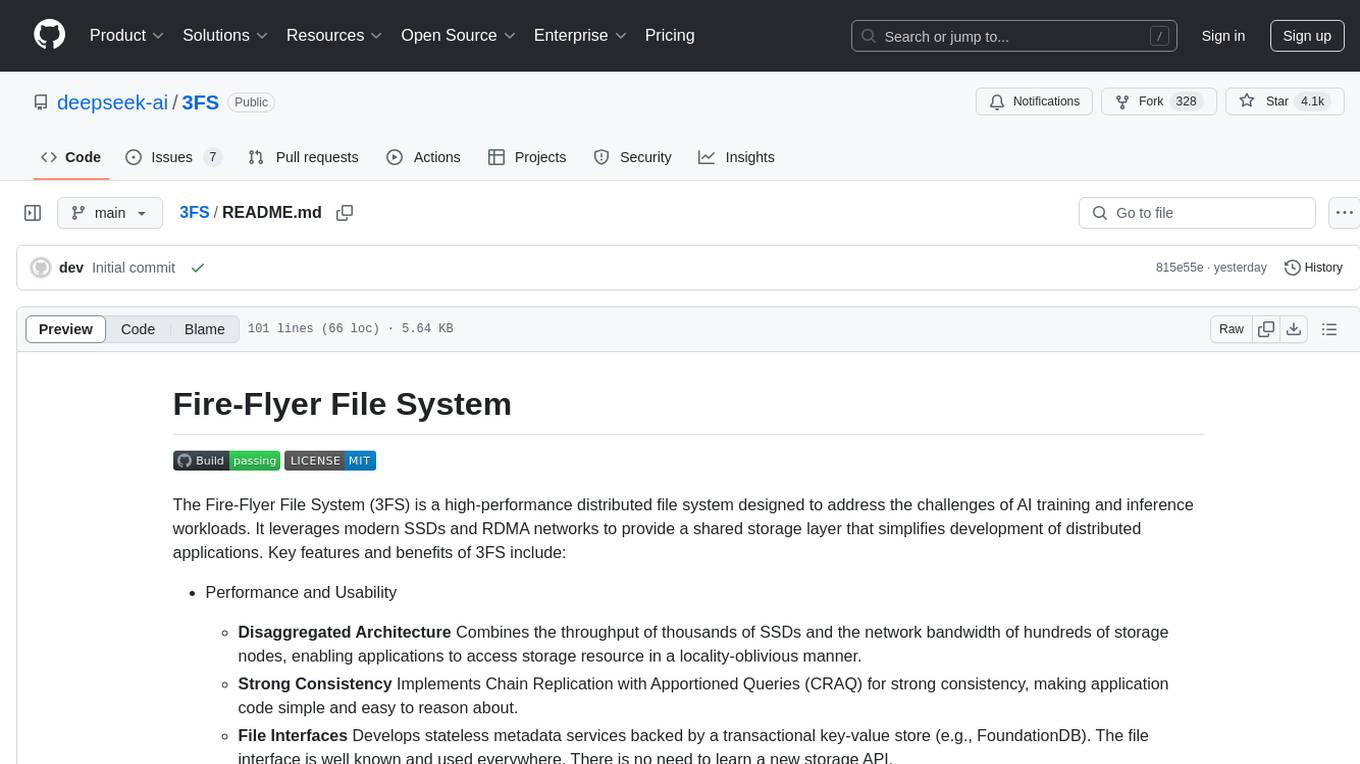
3FS
The Fire-Flyer File System (3FS) is a high-performance distributed file system designed for AI training and inference workloads. It leverages modern SSDs and RDMA networks to provide a shared storage layer that simplifies development of distributed applications. Key features include performance, disaggregated architecture, strong consistency, file interfaces, data preparation, dataloaders, checkpointing, and KVCache for inference. The system is well-documented with design notes, setup guide, USRBIO API reference, and P specifications. Performance metrics include peak throughput, GraySort benchmark results, and KVCache optimization. The source code is available on GitHub for cloning and installation of dependencies. Users can build 3FS and run test clusters following the provided instructions. Issues can be reported on the GitHub repository.