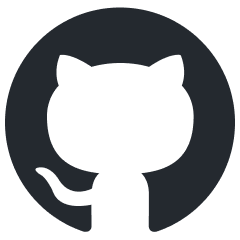
lingua
Meta Lingua: a lean, efficient, and easy-to-hack codebase to research LLMs.
Stars: 4405
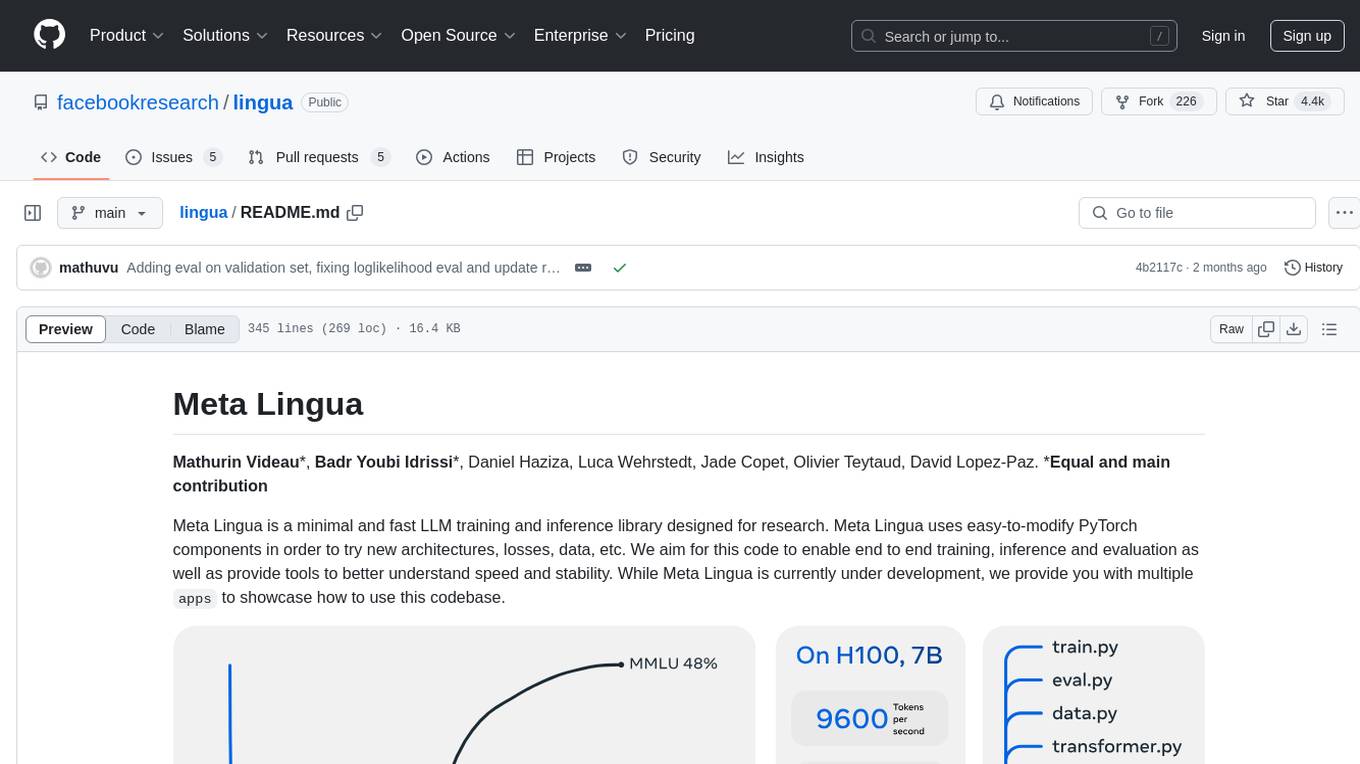
Meta Lingua is a minimal and fast LLM training and inference library designed for research. It uses easy-to-modify PyTorch components to experiment with new architectures, losses, and data. The codebase enables end-to-end training, inference, and evaluation, providing tools for speed and stability analysis. The repository contains essential components in the 'lingua' folder and scripts that combine these components in the 'apps' folder. Researchers can modify the provided templates to suit their experiments easily. Meta Lingua aims to lower the barrier to entry for LLM research by offering a lightweight and focused codebase.
README:
Mathurin Videau*, Badr Youbi Idrissi*, Daniel Haziza, Luca Wehrstedt, Jade Copet, Olivier Teytaud, David Lopez-Paz. *Equal and main contribution
Meta Lingua is a minimal and fast LLM training and inference library designed for research. Meta Lingua uses easy-to-modify PyTorch components in order to try new architectures, losses, data, etc. We aim for this code to enable end to end training, inference and evaluation as well as provide tools to better understand speed and stability. While Meta Lingua is currently under development, we provide you with multiple apps
to showcase how to use this codebase.
The following commands launch a SLURM job that creates an environment for Meta Lingua. The env creation should take around 5 minutes without counting downloads.
git clone https://github.com/facebookresearch/lingua
cd lingua
bash setup/create_env.sh
# or if you have access to a SLURM cluster
sbatch setup/create_env.sh
Once that is done your can activate the environment
conda activate lingua_<date>
use the provided script to download and prepare data from huggingface (among fineweb_edu
, fineweb_edu_10bt
, or dclm_baseline_1.0
).
This command will download the fineweb_edu
and prepare it for training in the ./data
directory, specifying the amount of memory terashuf
(the tool used to shuffle samples) will be allocated. By default, the number of chunks (nchunks
) is 32. If you are running on fewer than 32 GPUs, it is recommended to set nchunks
to 1 or to match nchunks
with the number of GPUs (nchunks
= NGPUs). See here for more details.
python setup/download_prepare_hf_data.py fineweb_edu <MEMORY> --data_dir ./data --seed 42 --nchunks <NCHUNKS>
to download tokenizer (here llama3), use the folowing script:
python setup/download_tokenizer.py llama3 <SAVE_PATH> --api_key <HUGGINGFACE_TOKEN>
Now launch a debug job to check if everything works. The provided configurations are templates, you need to adapt them for them to work (change dump_dir
, data.root_dir
, data.tokenizer.path
, etc ...)
# stool stands for SLURM tool !
python -m lingua.stool script=apps.main.train config=apps/main/configs/debug.yaml nodes=1 partition=<partition>
# if you want to launch locally you can use torchrun
torchrun --nproc-per-node 8 -m apps.main.train config=apps/main/configs/debug.yaml
# or you can also launch on 1 GPU
python -m apps.main.train config=apps/main/configs/debug.yaml
When using stool
, if a job crashes, it can be relaunched using sbatch:
sbatch path/to/dump_dir/submit.slurm
We get very strong performance on many downstream tasks and match the performance of DCLM baseline 1.0.
name | arc_challenge | arc_easy | boolq | copa | hellaswag | obqa | piqa | siqa | winogrande | nq | tqa |
---|---|---|---|---|---|---|---|---|---|---|---|
Transformer 1B | 36.48 | 62.83 | 62.57 | 79.00 | 63.62 | 37.40 | 75.14 | 45.19 | 61.64 | 8.75 | 26.31 |
minGRU 1B | 30.82 | 57.89 | 62.05 | 74.00 | 50.27 | 37.00 | 72.31 | 43.76 | 52.49 | 3.24 | 9.03 |
minLSTM 1B | 31.76 | 60.04 | 62.02 | 73.00 | 53.39 | 36.40 | 72.36 | 45.09 | 52.80 | 4.52 | 12.73 |
Hawk 1B | 34.94 | 63.68 | 62.42 | 76.00 | 63.10 | 38.20 | 73.23 | 46.01 | 55.33 | 8.42 | 23.58 |
Mamba 1B | 35.54 | 63.42 | 62.63 | 74.00 | 64.16 | 38.80 | 75.24 | 45.14 | 60.14 | 8.84 | 26.64 |
name | arc_challenge | arc_easy | boolq | copa | hellaswag | obqa | piqa | siqa | winogrande | mmlu | nq | tqa | bbh |
---|---|---|---|---|---|---|---|---|---|---|---|---|---|
Mamba 7B 200B tokens | 47.21 | 76.03 | 65.63 | 84.00 | 77.80 | 44.00 | 80.25 | 49.69 | 70.24 | 32.81 | 20.53 | 51.93 | 20.35 |
Llama 7B 200B tokens | 46.95 | 75.73 | 64.80 | 84.00 | 77.45 | 45.00 | 80.20 | 48.26 | 70.32 | 48.64 | 20.66 | 51.01 | 31.47 |
Llama 7B squared relu 1T tokens | 49.61 | 76.74 | 72.45 | 89.00 | 81.19 | 44.80 | 82.05 | 49.95 | 72.14 | 60.56 | 25.68 | 59.52 | 42.11 |
Meta Lingua is structured as follows:
📦meta-lingua
┣ 📂lingua # Core library
┃ ┣ 📜args.py
┃ ┣ 📜checkpoint.py
┃ ┣ 📜data.py
┃ ┣ 📜distributed.py
┃ ┣ 📜float8.py
┃ ┣ 📜logger.py
┃ ┣ 📜metrics.py
┃ ┣ 📜optim.py
┃ ┣ 📜probe.py
┃ ┣ 📜profiling.py
┃ ┣ 📜stool.py
┃ ┣ 📜tokenizer.py
┃ ┗ 📜transformer.py
┣ 📂setup
┃ ┣ 📜create_env.sh
┃ ┗ 📜download_prepare_hf_data.py
┗ 📂apps # Apps that put components together
┣ 📂main # Main language modeling app with llama
┃ ┣ 📂configs
┃ ┣ 📜eval.py
┃ ┣ 📜generate.py
┃ ┣ 📜train.py
┃ ┗ 📜transformer.py
┣ 📂fastRNN
┃ ┣ 📂component
┃ ┣ 📂hawk
┃ ┣ 📂minGRU
┃ ┣ 📂minLSTM
┣ 📂mamba
┣ 📂mtp # Multi token prediction
┗ 📂plots
The lingua
folder contains some essential and reusable components, while the apps
folder contains scripts that put those components together. For instance the main training loop is in apps/main
. We highly encourage you to use that as a template and modify it however you please to suit your experiments.
Nothing is sacred in Meta Lingua. We've specifically tried to make it as easily modifiable as possible! So feel free to branch out and modify anything.
Here's a quick description of the most important files and features:
-
transformer.py
: Defines model architecture. This is pure PyTorchnn.Module
! Nothing fancy here. -
distributed.py
: Handles distributing the model on multiple GPUs. This is done throughparallelize_module
function which wraps your vanillann.Module
and applies nearly any combination of Data Parallel, Fully Sharded Data Parallel, Model Parallelism,torch.compile
, activation checkpointing andfloat8
. -
data.py
: Dataloader for LLM pretraining.
-
profiling.py
: Small wrapper around xformers' profiler which provides automatic MFU and HFU calculation and dumps profile traces in profiling folder in your dump directory. It also has memory profiling trace. -
checkpoint.py
: Manages model checkpoints. It saves model in checkpoints folder in your dump dir in .distcp format which is the new PyTorch distributed saving method. This format allows to reload the model with a different number of GPUs and with a different sharding. You can also convert those into normal PyTorch checkpoints withtorch.distributed.checkpoint.format_utils.dcp_to_torch_save
and the other way aroundtorch_save_to_dcp
. -
args.py
: Utilities to work with configs.
Most components need configuration and we chose to use data classes to represent these configuration objects. args.py
helps with converting between config.yaml
and config dictionaries into the respective data classes.
So for examples the TrainArgs
in apps/main/train.py
has a LMTransformerArgs
, OptimArgs
, etc ... as children.
Here is an example configuration file that will be converted to TrainArgs
:
# This is where Meta Lingua will store anything related to the experiment.
dump_dir: /path/to/dumpdir
name: "debug"
steps: 1000
seed: 12
optim:
lr: 3e-4
warmup: 2000
lr_min_ratio: 0.000001
clip: 10.0
distributed:
fsdp_type: full_shard
compile: true
selective_activation_checkpointing: false
model:
dim: 1024
n_layers: 8
n_heads: 8
data:
root_dir: data/shuffled
sources:
wikipedia: 80.0
arxiv: 20.0
batch_size: 32
seq_len: 1024
load_async: true
tokenizer:
name: sp
path: tokenizers/llama2.model
The command line interface in all scripts (train.py
, eval.py
, stool.py
) uses OmegaConf
This accepts arguments as a dot list
So if the dataclass looks like
@dataclass
class DummyArgs:
name: str = "blipbloup"
mode: LMTransformerArgs = LMTransformerArgs()
@dataclass
class LMTransformerArgs:
dim: int = 512
n_layers: int = 12
Then you can pass model.dim = 32
to change values in LMTransformerArgs
or just name = tictac
for top level attributes.
train.py
simply takes as argument the path to a config file and will load that config. The behavior here is as follows:
- We instantiate
TrainArgs
with its default values - We override those default values with the ones in the provided config file
- We override the result with the additional arguments provided through command line
If we take the DummyArgs
example above, calling train.py
with train.py config=debug.yaml model.dim=64 name=tictac
where debug.yaml
contains
model:
n_layers: 24
will launch training with the config
DummyArgs(name="tictac", LMTransformerArgs(dim=64, n_layers=24))
Since we want to do distributed training, we need train.py
to run N times (with N being the number of GPUs)
The easiest way to do this is through SLURM. And in order to make that simpler, we provide lingua/stool.py
which is a simple python script that
- Saves the provided config to
dump_dir
- Copies your current code to
dump_dir
in order to back it up - Creates an sbatch file
submit.slurm
which is then used to launch the job with the provided config.
It can either be used through command line
python -m lingua.stool config=apps/main/configs/debug.yaml nodes=1 account=fair_amaia_cw_codegen qos=lowest
Or the launch_job
function directly. This allows you for example to create many arbitrary configs (to sweep parameters, do ablations) in a jupyter notebook and launch jobs directly from there.
Since the configuration file is copied to dump_dir
, an easy way to iterate is to simply change the config file and launch the same command above.
In order to iterate quickly, it is preferable not to have to wait for a SLURM allocation every time. You can instead ask SLURM to allocate resources for you, then once they're allocated you can run multiple commands on that same allocation.
For example you can do :
salloc --nodes 2 --cpus-per-gpu 16 --mem 1760GB --gres=gpu:8 --exclusive --time=72:00:00
Which will give you access to 2 nodes in your current terminal. Once the allocation is done, you will see some SLURM environement variables that were automatically added such as $SLURM_JOB_ID
and others... This allows you for example to do in the same terminal
srun -n 16 python -m apps.main.train config=apps/main/configs/debug.yaml
Which will run the python -m apps.main.train config=apps/main/configs/debug.yaml
command on each of the 16 GPUs. If this crashes or ends you can just relaunch srun
again because the nodes are already allocated to you and you don't have to wait for SLURM to give you the resources again.
This will also show you the outputs of all those commands in the same terminal which might become cumbersome.
Instead you can use stool
directly to configure logs to be separated into different files per GPU.
python -m lingua.stool config=apps/main/configs/debug.yaml nodes=2 launcher=bash dirs_exists_ok=true
Notice that we added launcher=bash
which basically means that the generated submit.slurm
will simply be executed instead of submitting it through sbatch
. The submit.slurm
has an srun
command also so this is very similar to the above srun
command. We also add dirs_exists_ok=true
to tell stool
that it is okay to override things in an existing folder (code, config, etc)
If you want to use pdb
to step through your code, you should use -n 1
to run only on 1 GPU.
Evaluations can run either during training periodically or you directly launch evals on a given checkpoint as follows:
srun -n 8 python -u -m apps.main.eval config=apps/main/configs/eval.yaml
You need to specify the checkpoint and dump dir of the evaluation in that config
Or through stool
with
python -m lingua.stool script=apps.main.eval config=apps/main/configs/eval.yaml nodes=1 account=fair_amaia_cw_codegen qos=lowest
📂example_dump_dir
┣ 📂checkpoints
┃ ┣ 📂0000001000
┃ ┣ 📂0000002000
┃ ┣ 📂0000003000
┃ ┣ 📂0000004000
┃ ┣ 📂0000005000
┃ ┣ 📂0000006000
┃ ┣ 📂0000007000 # Checkpoint and train state saved every 1000 steps here
┃ ┃ ┣ 📜.metadata
┃ ┃ ┣ 📜__0_0.distcp
┃ ┃ ┣ 📜__1_0.distcp
┃ ┃ ┣ 📜params.json
┃ ┃ ┣ 📜train_state_00000.json
┃ ┃ ┗ 📜train_state_00001.json
┣ 📂code # Backup of the code at the moment the job was launched
┣ 📂logs
┃ ┗ 📂166172 # Logs for each GPU in this SLURM job.
┃ ┃ ┣ 📜166172.stderr
┃ ┃ ┣ 📜166172.stdout
┃ ┃ ┣ 📜166172_0.err
┃ ┃ ┣ 📜166172_0.out
┃ ┃ ┣ 📜166172_1.err
┃ ┃ ┗ 📜166172_1.out
┣ 📂profiling
┃ ┣ 📂memory_trace_plot # Trace of memory usage through time for all GPUs
┃ ┃ ┣ 📜000102_h100-192-145_451082.html
┃ ┃ ┣ 📜000102_h100-192-145_451083.html
┃ ┗ 📂profile_CPU_CUDA_000104 # Profiling traces for all GPUs
┃ ┃ ┣ 📜h100-192-145_451082.1720183858874741723.pt.trace.json.gz
┃ ┃ ┗ 📜h100-192-145_451083.1720183858865656716.pt.trace.json.gz
┣ 📜base_config.yaml
┣ 📜config.yaml
┣ 📜metrics.jsonl
┗ 📜submit.slurm
Here we highlight some related work that is complementary to this one. Most important being torchtitan and torchtune.
Lingua is designed for researchers who want to experiment with new ideas for LLM pretraining and get quick feedback on both training/inference speed and downstream benchmarks. Our goal is to lower the barrier to entry for LLM research by providing a lightweight and focused codebase.
We see torchtitan, torchtune, and lingua as complementary tools. Torchtitan is excellent for large-scale work because it features 3D parallelism and is likely to integrate the latest PyTorch distributed training features more quickly, thanks to its close ties to the PyTorch team. On the other hand, Torchtune excels at fine-tuning, especially when GPU resources are limited, by offering various fine-tuning strategies like LoRA, QLoRA, DPO, and PPO.
A typical workflow could look like this: you might first test a new idea in Lingua, then scale it up further with Torchtitan, and finally use Torchtune for instruction or preference fine-tuning.
Although there's definitely some overlap among these codebases, we think it's valuable to have focused tools for different aspects of LLM work. For example, Torchtitan aims to showcase the latest distributed training features of PyTorch in a clean, minimal codebase, but for most research, you really don't need every feature PyTorch has to offer or the capability to scale to 100B parameters on 4096 GPUs. For instance, we think that FSDP + torch compile will cover 90% of all needs of a researcher. With lingua, we tried to ask "What's the minimal set of features needed to draw solid conclusions on the scalability of idea X?"
We believe this targeted approach helps researchers make progress faster without the mental overhead of using many techniques that might not be needed.
@misc{meta_lingua,
author = {Mathurin Videau, Badr Youbi Idrissi, Daniel Haziza, Luca Wehrstedt, Jade Copet, Olivier Teytaud, David Lopez-Paz},
title = {{Meta Lingua}: A minimal {PyTorch LLM} training library},
url = {https://github.com/facebookresearch/lingua},
year = {2024}
}
Meta Lingua is licensed under BSD-3-Clause license. Refer to the LICENSE file in the top level directory.
For Tasks:
Click tags to check more tools for each tasksFor Jobs:
Alternative AI tools for lingua
Similar Open Source Tools
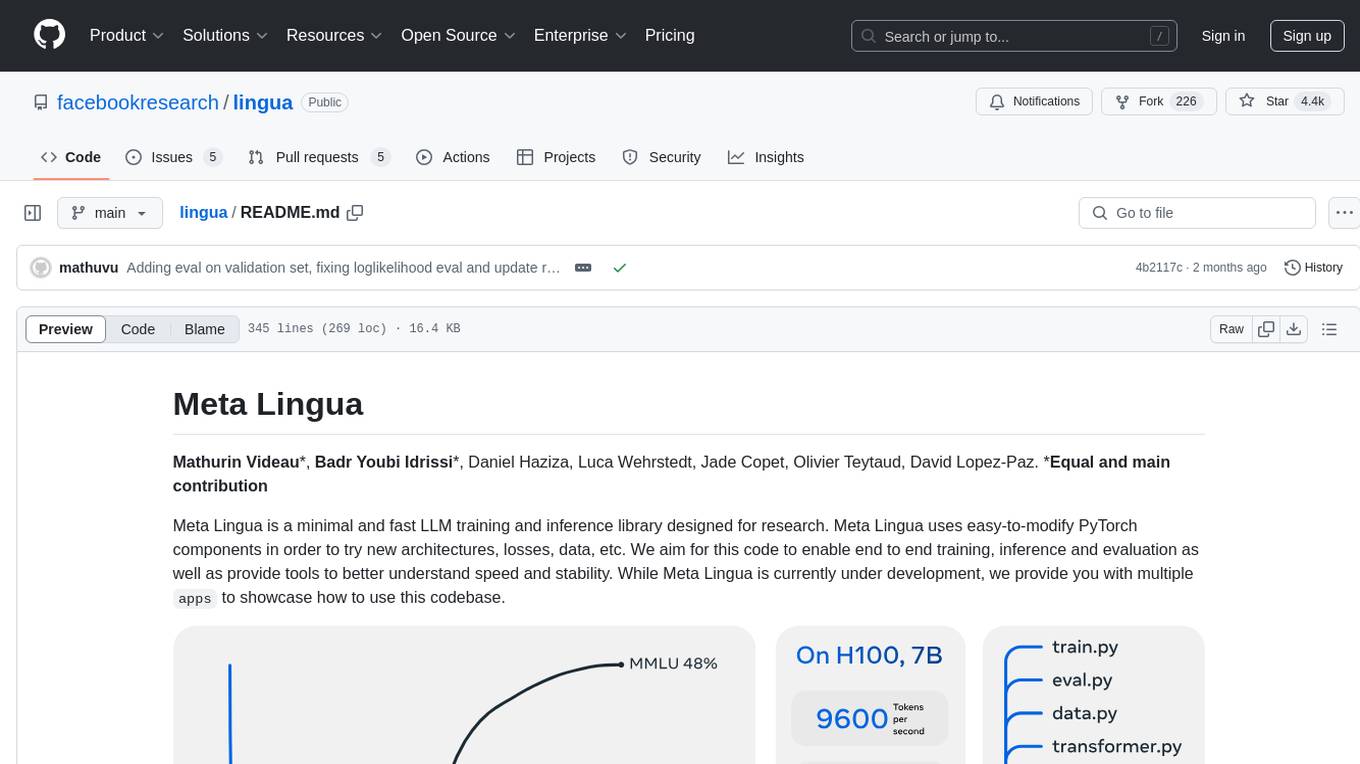
lingua
Meta Lingua is a minimal and fast LLM training and inference library designed for research. It uses easy-to-modify PyTorch components to experiment with new architectures, losses, and data. The codebase enables end-to-end training, inference, and evaluation, providing tools for speed and stability analysis. The repository contains essential components in the 'lingua' folder and scripts that combine these components in the 'apps' folder. Researchers can modify the provided templates to suit their experiments easily. Meta Lingua aims to lower the barrier to entry for LLM research by offering a lightweight and focused codebase.
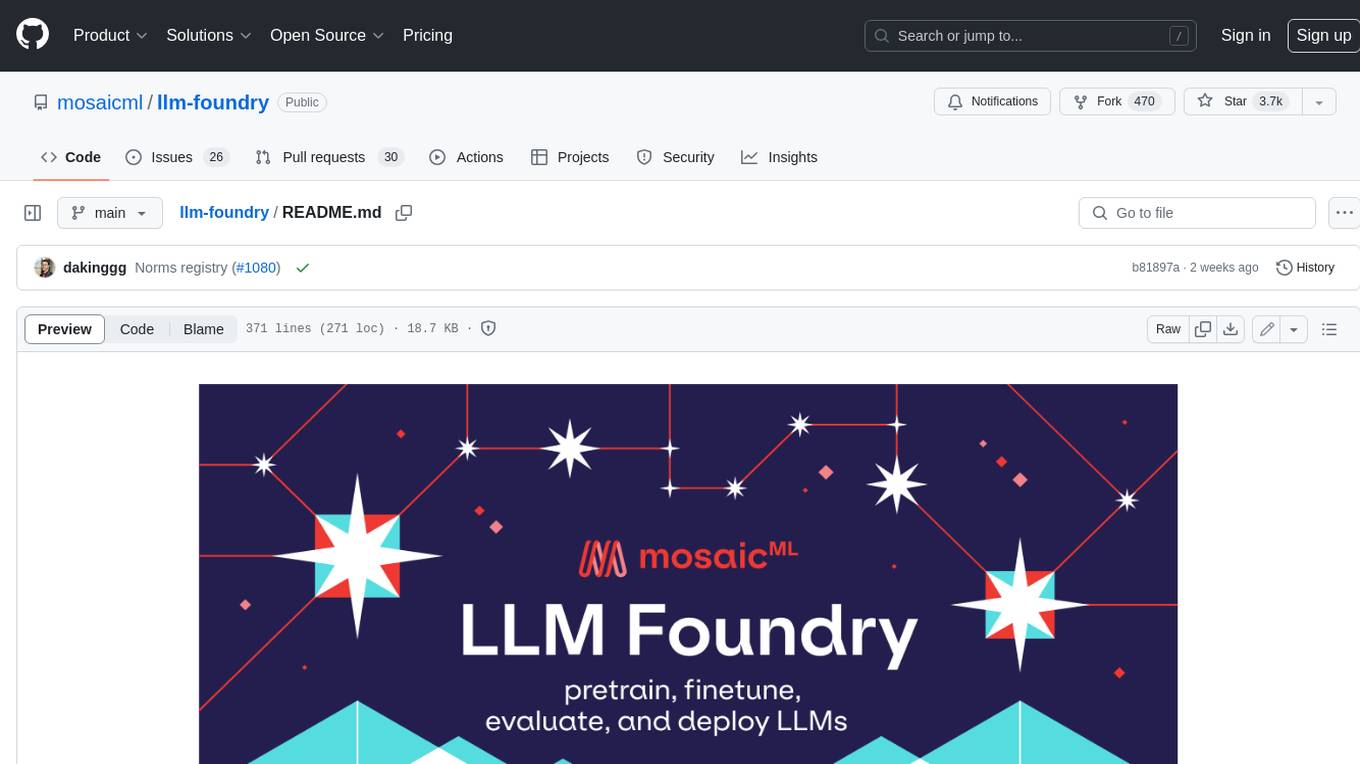
llm-foundry
LLM Foundry is a codebase for training, finetuning, evaluating, and deploying LLMs for inference with Composer and the MosaicML platform. It is designed to be easy-to-use, efficient _and_ flexible, enabling rapid experimentation with the latest techniques. You'll find in this repo: * `llmfoundry/` - source code for models, datasets, callbacks, utilities, etc. * `scripts/` - scripts to run LLM workloads * `data_prep/` - convert text data from original sources to StreamingDataset format * `train/` - train or finetune HuggingFace and MPT models from 125M - 70B parameters * `train/benchmarking` - profile training throughput and MFU * `inference/` - convert models to HuggingFace or ONNX format, and generate responses * `inference/benchmarking` - profile inference latency and throughput * `eval/` - evaluate LLMs on academic (or custom) in-context-learning tasks * `mcli/` - launch any of these workloads using MCLI and the MosaicML platform * `TUTORIAL.md` - a deeper dive into the repo, example workflows, and FAQs
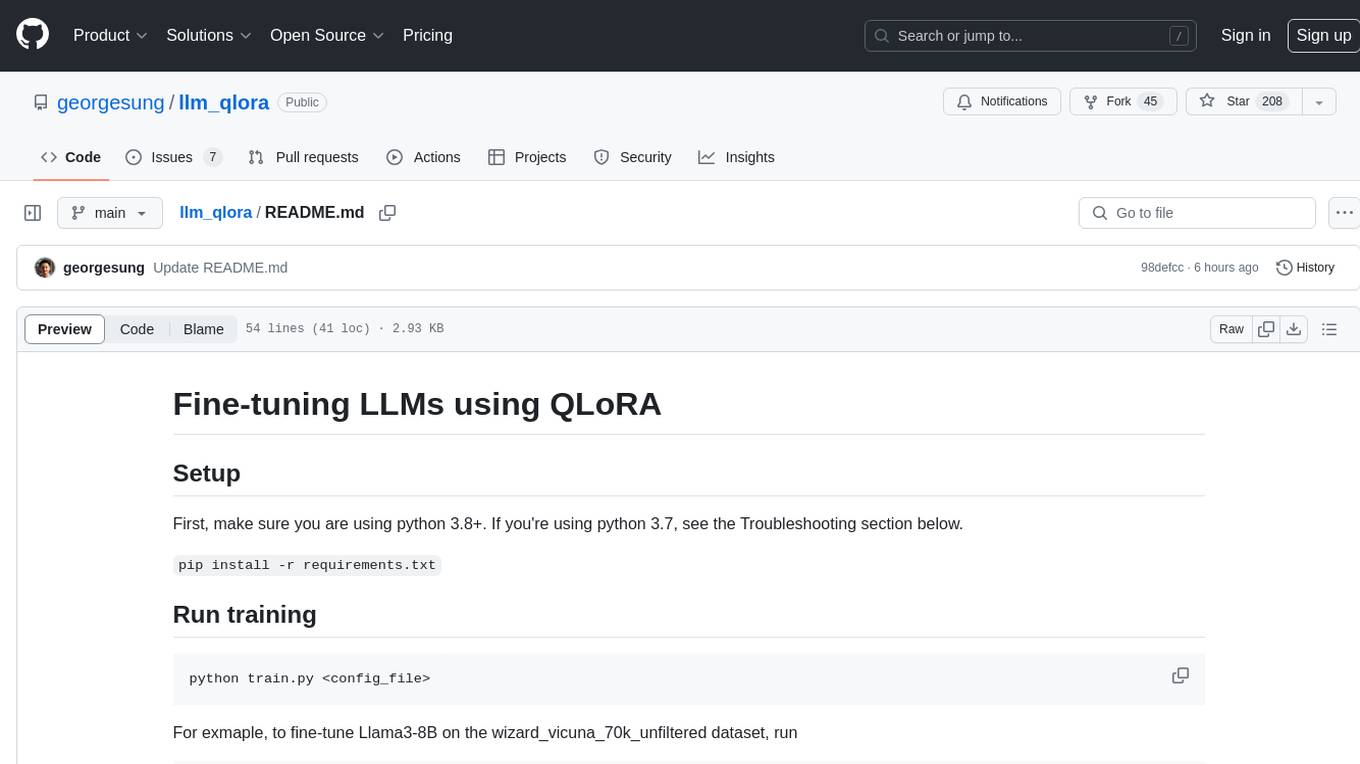
llm_qlora
LLM_QLoRA is a repository for fine-tuning Large Language Models (LLMs) using QLoRA methodology. It provides scripts for training LLMs on custom datasets, pushing models to HuggingFace Hub, and performing inference. Additionally, it includes models trained on HuggingFace Hub, a blog post detailing the QLoRA fine-tuning process, and instructions for converting and quantizing models. The repository also addresses troubleshooting issues related to Python versions and dependencies.
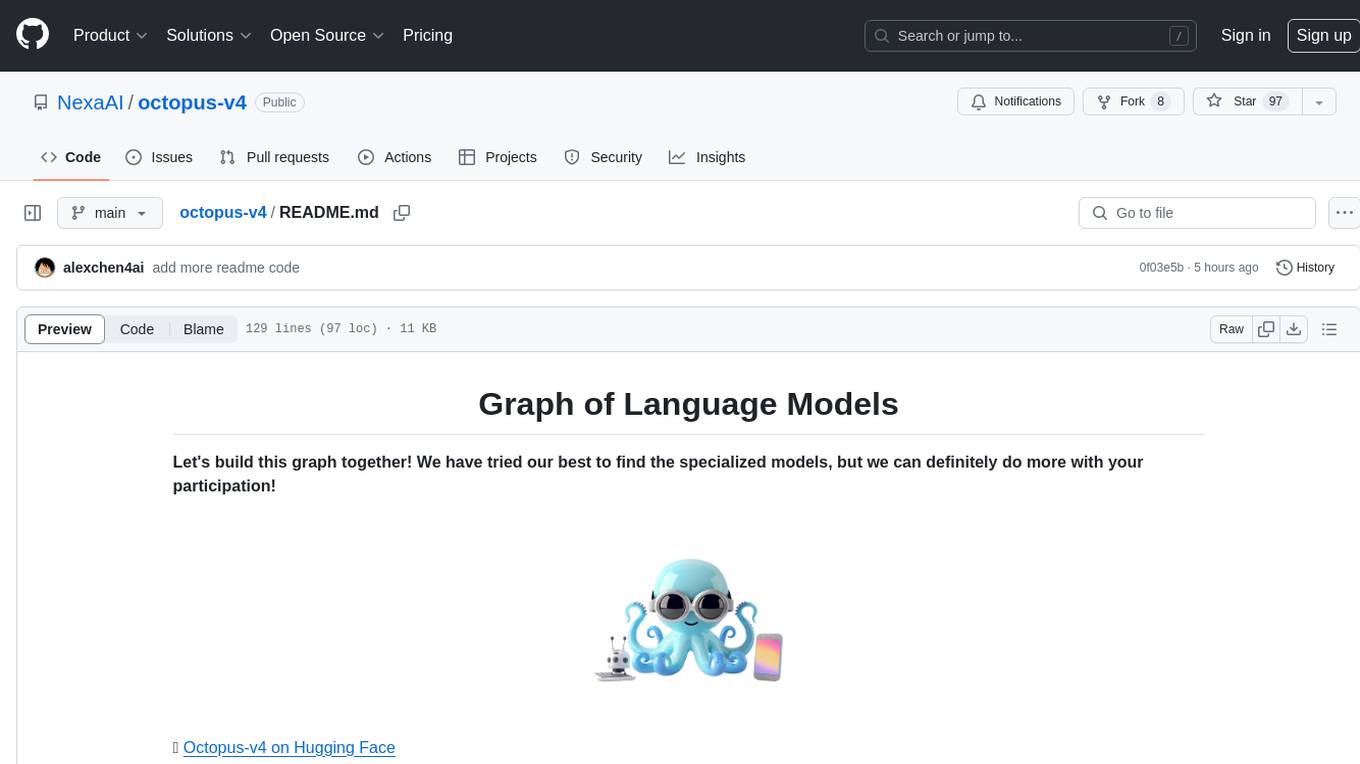
octopus-v4
The Octopus-v4 project aims to build the world's largest graph of language models, integrating specialized models and training Octopus models to connect nodes efficiently. The project focuses on identifying, training, and connecting specialized models. The repository includes scripts for running the Octopus v4 model, methods for managing the graph, training code for specialized models, and inference code. Environment setup instructions are provided for Linux with NVIDIA GPU. The Octopus v4 model helps users find suitable models for tasks and reformats queries for effective processing. The project leverages Language Large Models for various domains and provides benchmark results. Users are encouraged to train and add specialized models following recommended procedures.
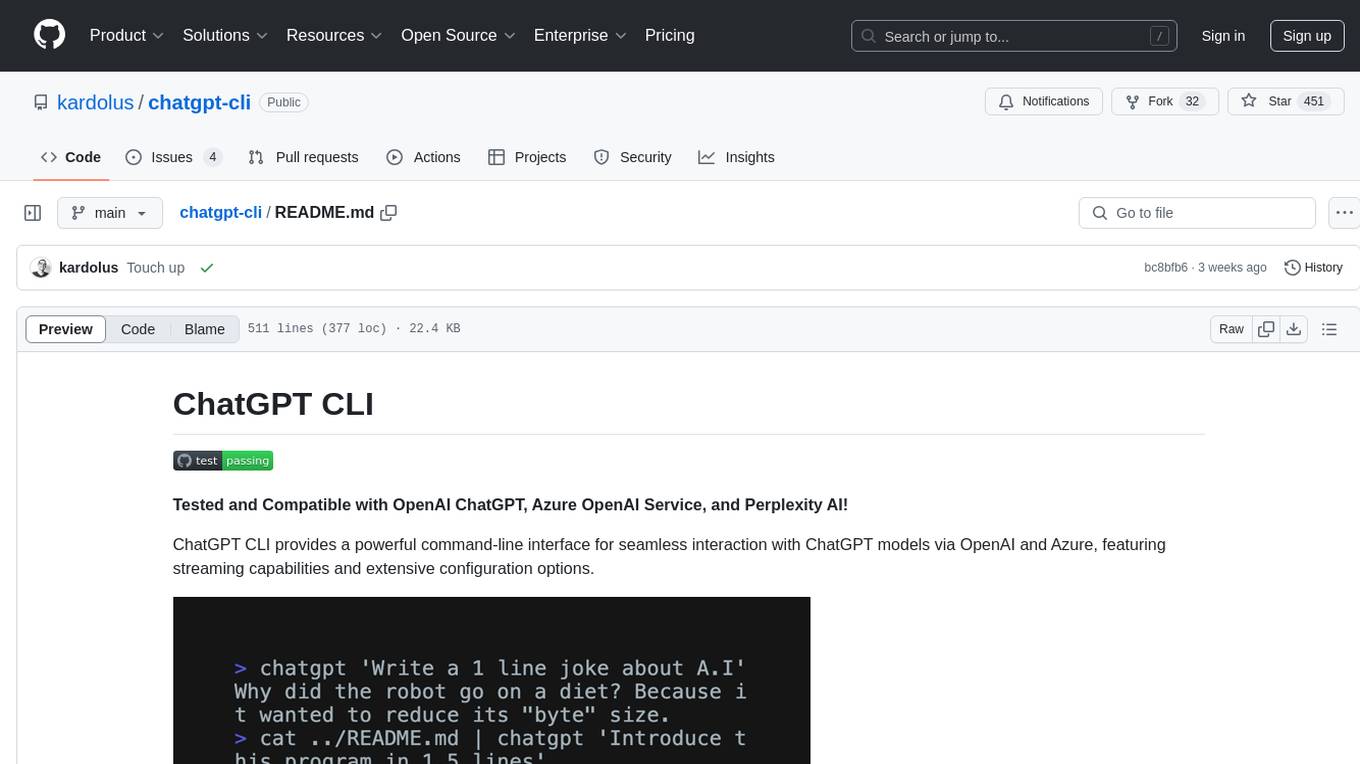
chatgpt-cli
ChatGPT CLI provides a powerful command-line interface for seamless interaction with ChatGPT models via OpenAI and Azure. It features streaming capabilities, extensive configuration options, and supports various modes like streaming, query, and interactive mode. Users can manage thread-based context, sliding window history, and provide custom context from any source. The CLI also offers model and thread listing, advanced configuration options, and supports GPT-4, GPT-3.5-turbo, and Perplexity's models. Installation is available via Homebrew or direct download, and users can configure settings through default values, a config.yaml file, or environment variables.
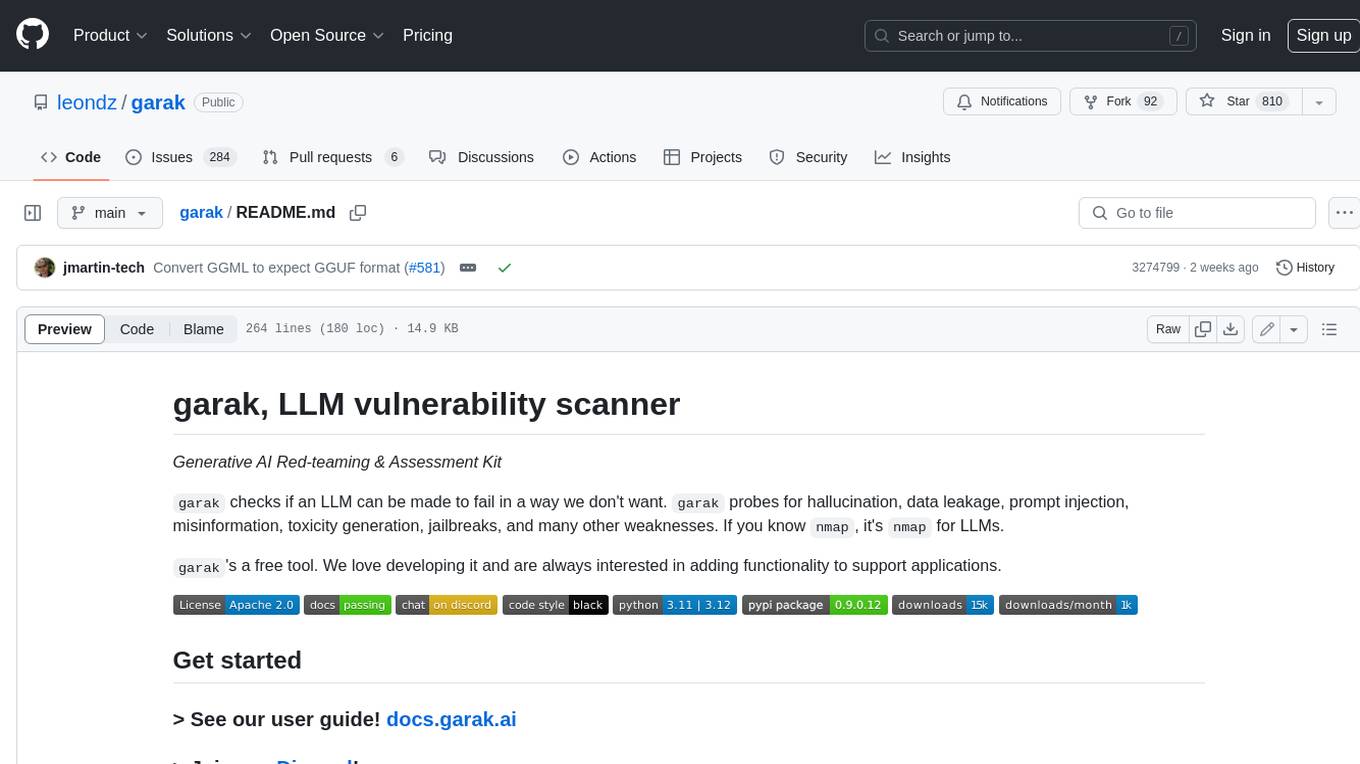
garak
Garak is a free tool that checks if a Large Language Model (LLM) can be made to fail in a way that is undesirable. It probes for hallucination, data leakage, prompt injection, misinformation, toxicity generation, jailbreaks, and many other weaknesses. Garak's a free tool. We love developing it and are always interested in adding functionality to support applications.
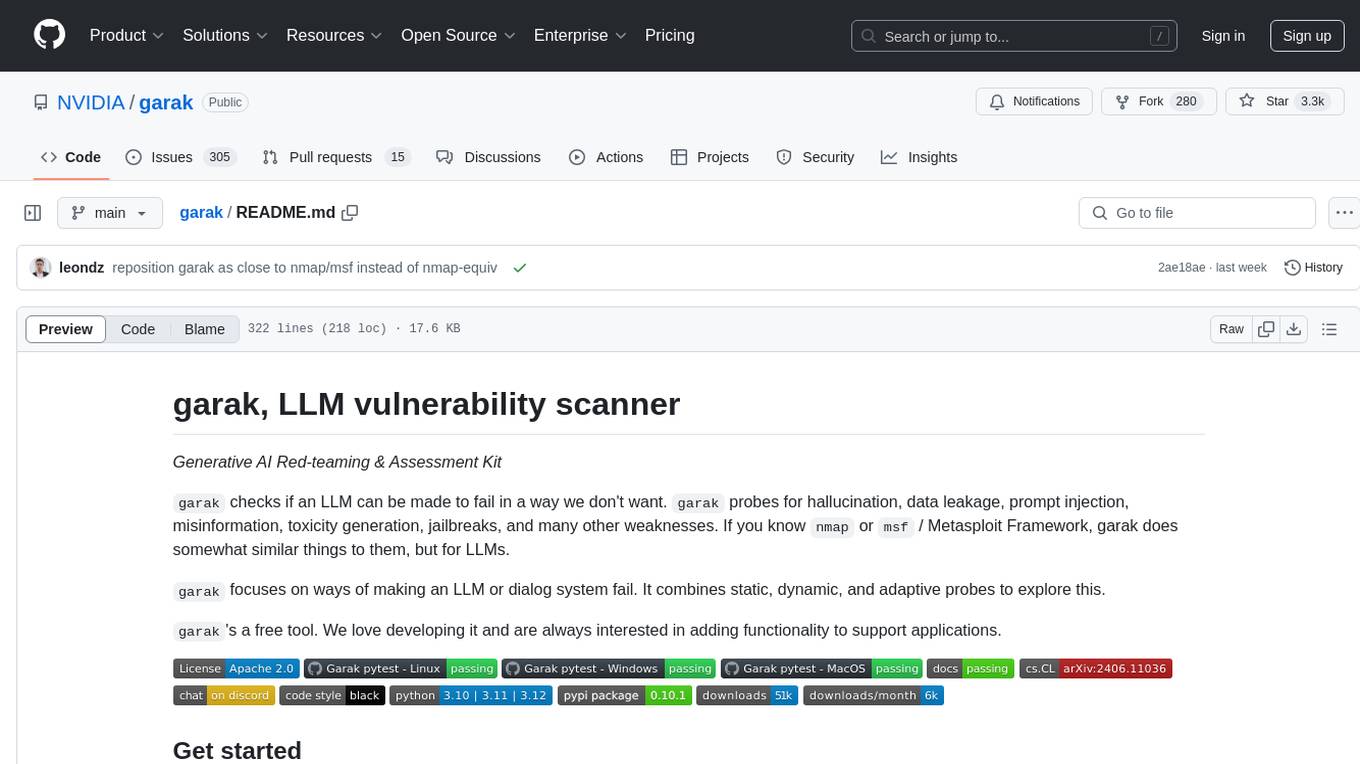
garak
Garak is a vulnerability scanner designed for LLMs (Large Language Models) that checks for various weaknesses such as hallucination, data leakage, prompt injection, misinformation, toxicity generation, and jailbreaks. It combines static, dynamic, and adaptive probes to explore vulnerabilities in LLMs. Garak is a free tool developed for red-teaming and assessment purposes, focusing on making LLMs or dialog systems fail. It supports various LLM models and can be used to assess their security and robustness.
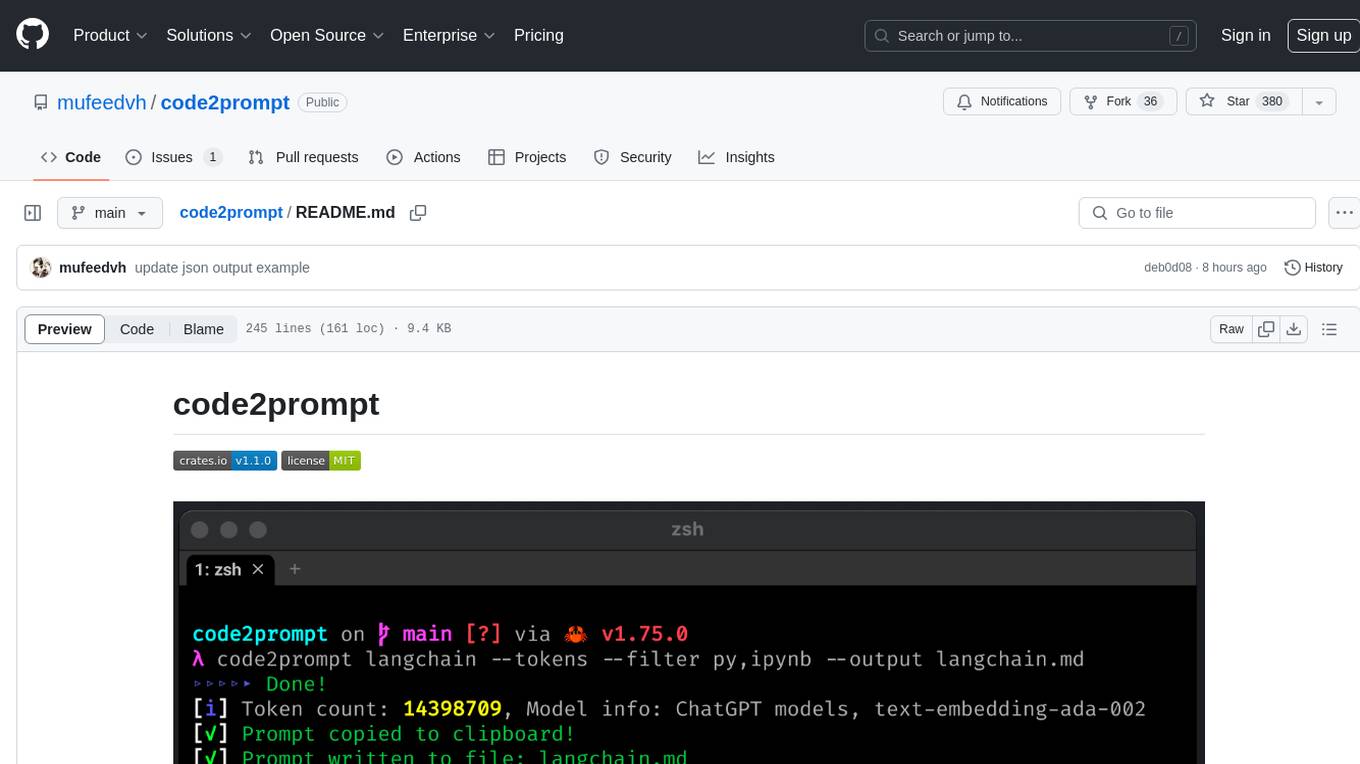
code2prompt
code2prompt is a command-line tool that converts your codebase into a single LLM prompt with a source tree, prompt templating, and token counting. It automates generating LLM prompts from codebases of any size, customizing prompt generation with Handlebars templates, respecting .gitignore, filtering and excluding files using glob patterns, displaying token count, including Git diff output, copying prompt to clipboard, saving prompt to an output file, excluding files and folders, adding line numbers to source code blocks, and more. It helps streamline the process of creating LLM prompts for code analysis, generation, and other tasks.
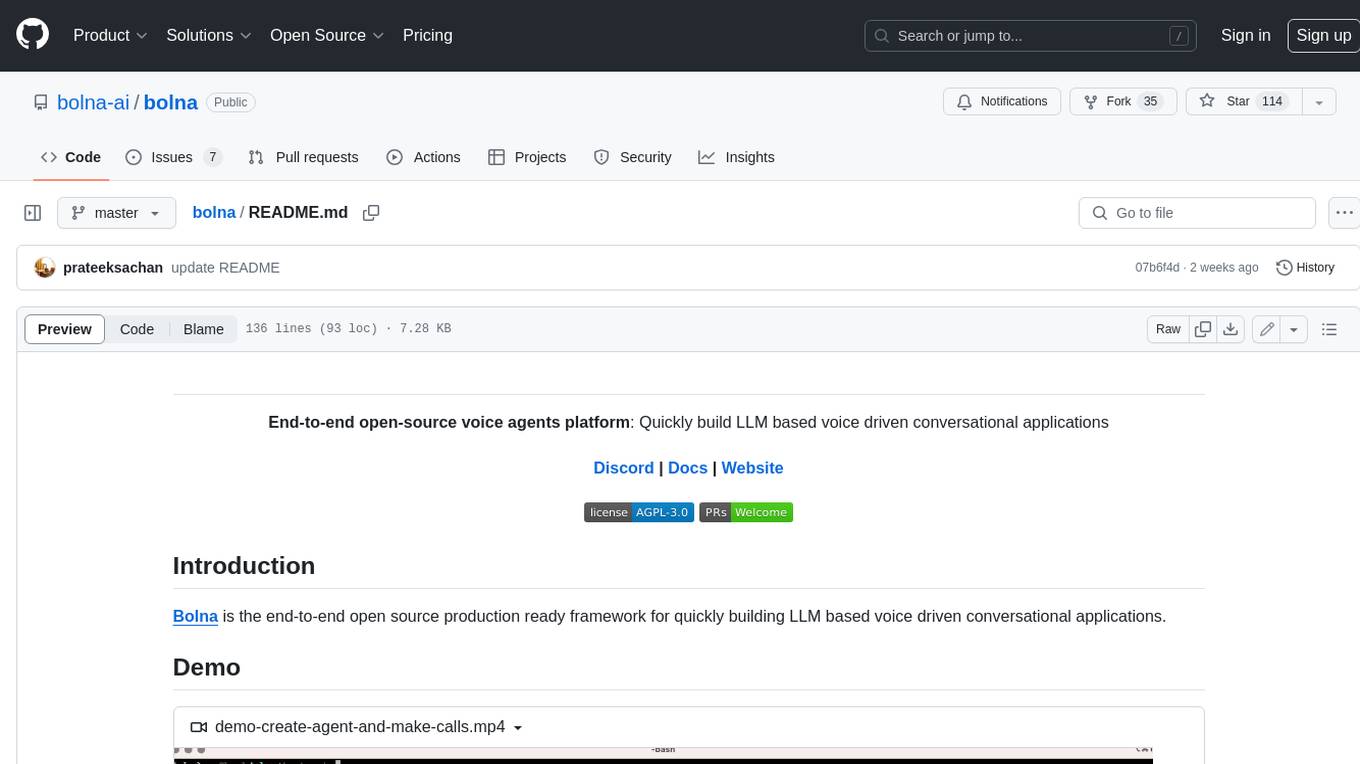
bolna
Bolna is an open-source platform for building voice-driven conversational applications using large language models (LLMs). It provides a comprehensive set of tools and integrations to handle various aspects of voice-based interactions, including telephony, transcription, LLM-based conversation handling, and text-to-speech synthesis. Bolna simplifies the process of creating voice agents that can perform tasks such as initiating phone calls, transcribing conversations, generating LLM-powered responses, and synthesizing speech. It supports multiple providers for each component, allowing users to customize their setup based on their specific needs. Bolna is designed to be easy to use, with a straightforward local setup process and well-documented APIs. It is also extensible, enabling users to integrate with other telephony providers or add custom functionality.
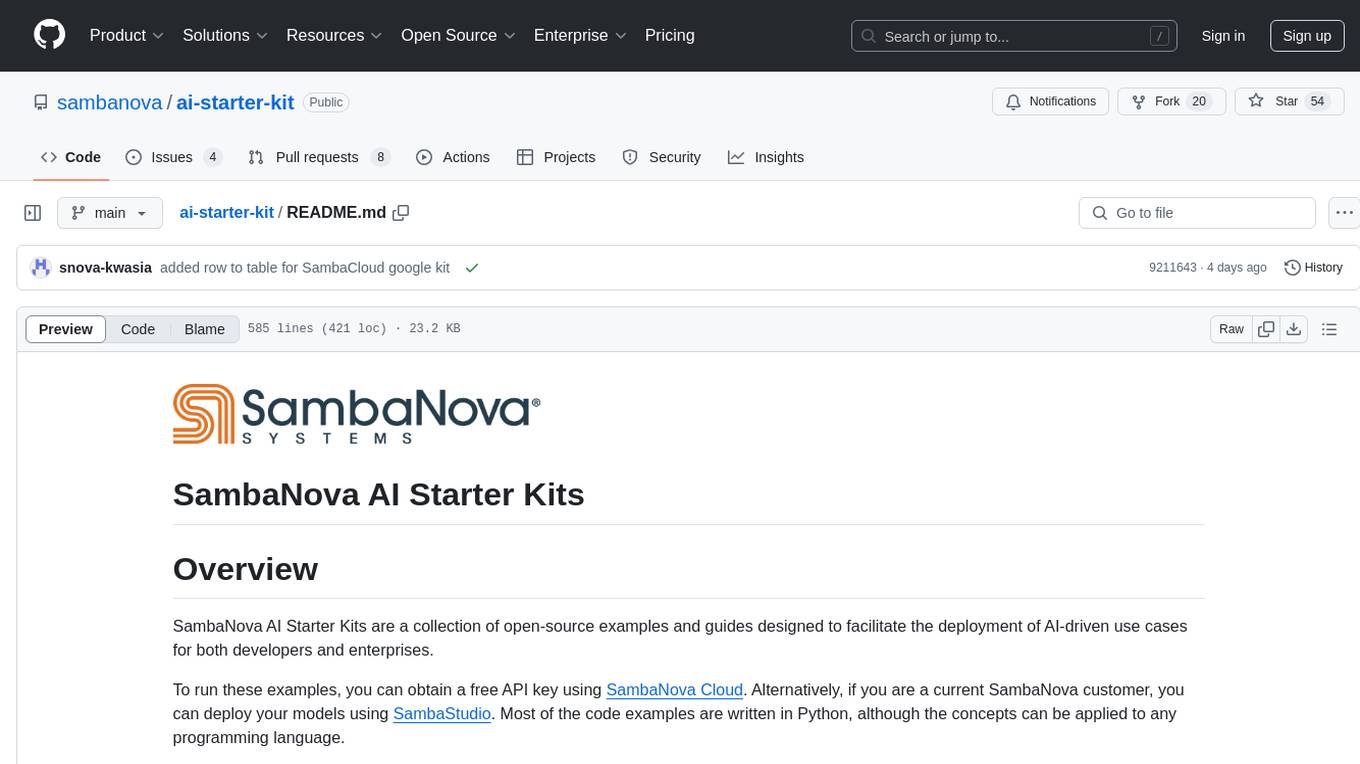
ai-starter-kit
SambaNova AI Starter Kits is a collection of open-source examples and guides designed to facilitate the deployment of AI-driven use cases for developers and enterprises. The kits cover various categories such as Data Ingestion & Preparation, Model Development & Optimization, Intelligent Information Retrieval, and Advanced AI Capabilities. Users can obtain a free API key using SambaNova Cloud or deploy models using SambaStudio. Most examples are written in Python but can be applied to any programming language. The kits provide resources for tasks like text extraction, fine-tuning embeddings, prompt engineering, question-answering, image search, post-call analysis, and more.
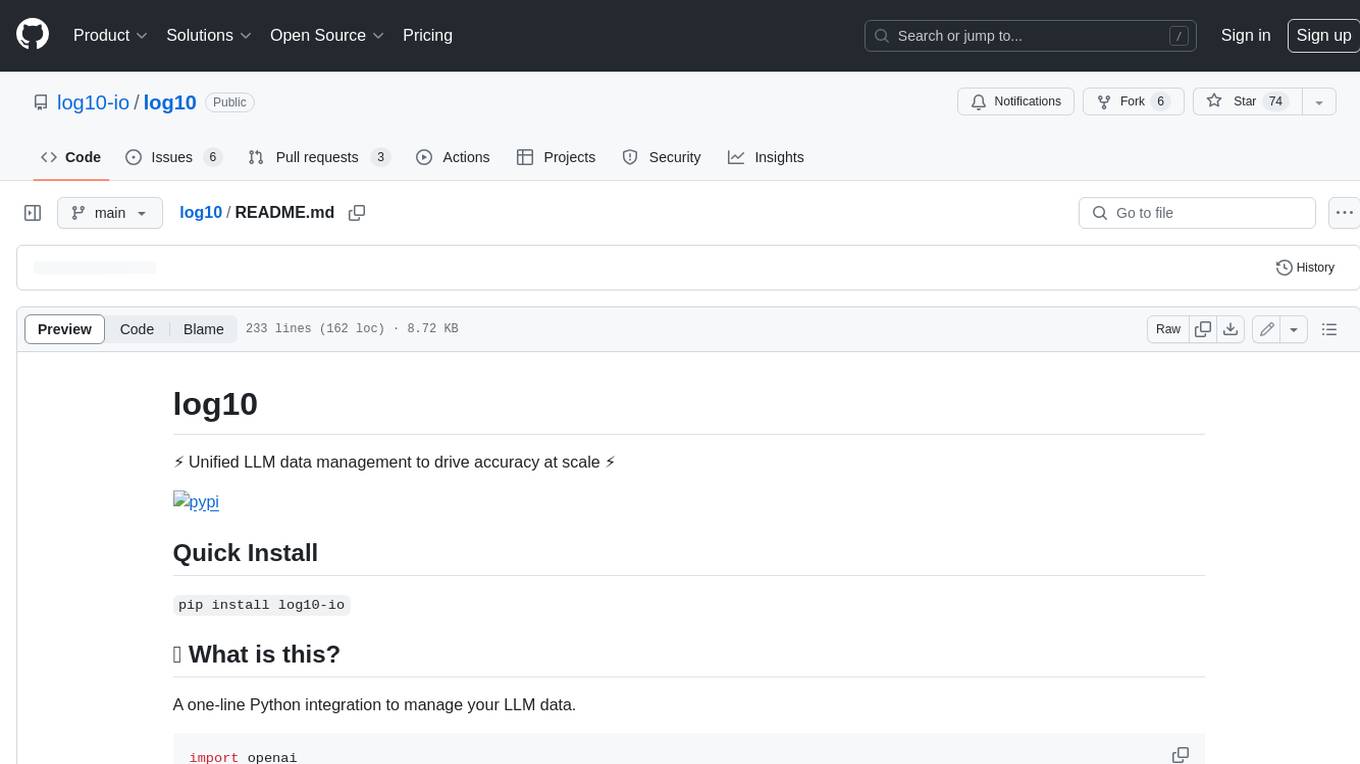
log10
Log10 is a one-line Python integration to manage your LLM data. It helps you log both closed and open-source LLM calls, compare and identify the best models and prompts, store feedback for fine-tuning, collect performance metrics such as latency and usage, and perform analytics and monitor compliance for LLM powered applications. Log10 offers various integration methods, including a python LLM library wrapper, the Log10 LLM abstraction, and callbacks, to facilitate its use in both existing production environments and new projects. Pick the one that works best for you. Log10 also provides a copilot that can help you with suggestions on how to optimize your prompt, and a feedback feature that allows you to add feedback to your completions. Additionally, Log10 provides prompt provenance, session tracking and call stack functionality to help debug prompt chains. With Log10, you can use your data and feedback from users to fine-tune custom models with RLHF, and build and deploy more reliable, accurate and efficient self-hosted models. Log10 also supports collaboration, allowing you to create flexible groups to share and collaborate over all of the above features.
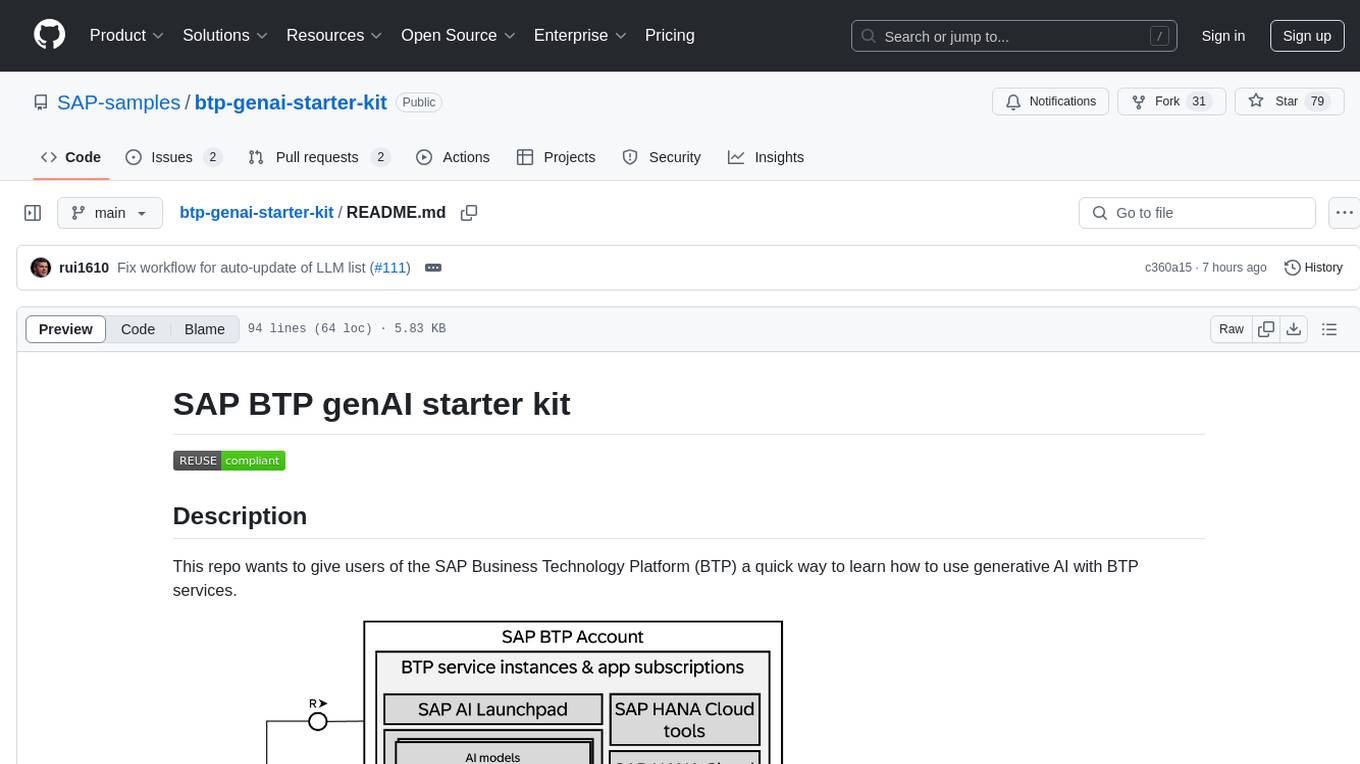
btp-genai-starter-kit
This repository provides a quick way for users of the SAP Business Technology Platform (BTP) to learn how to use generative AI with BTP services. It guides users through setting up the necessary infrastructure, deploying AI models, and running genAI experiments on SAP BTP. The repository includes scripts, examples, and instructions to help users get started with generative AI on the SAP BTP platform.
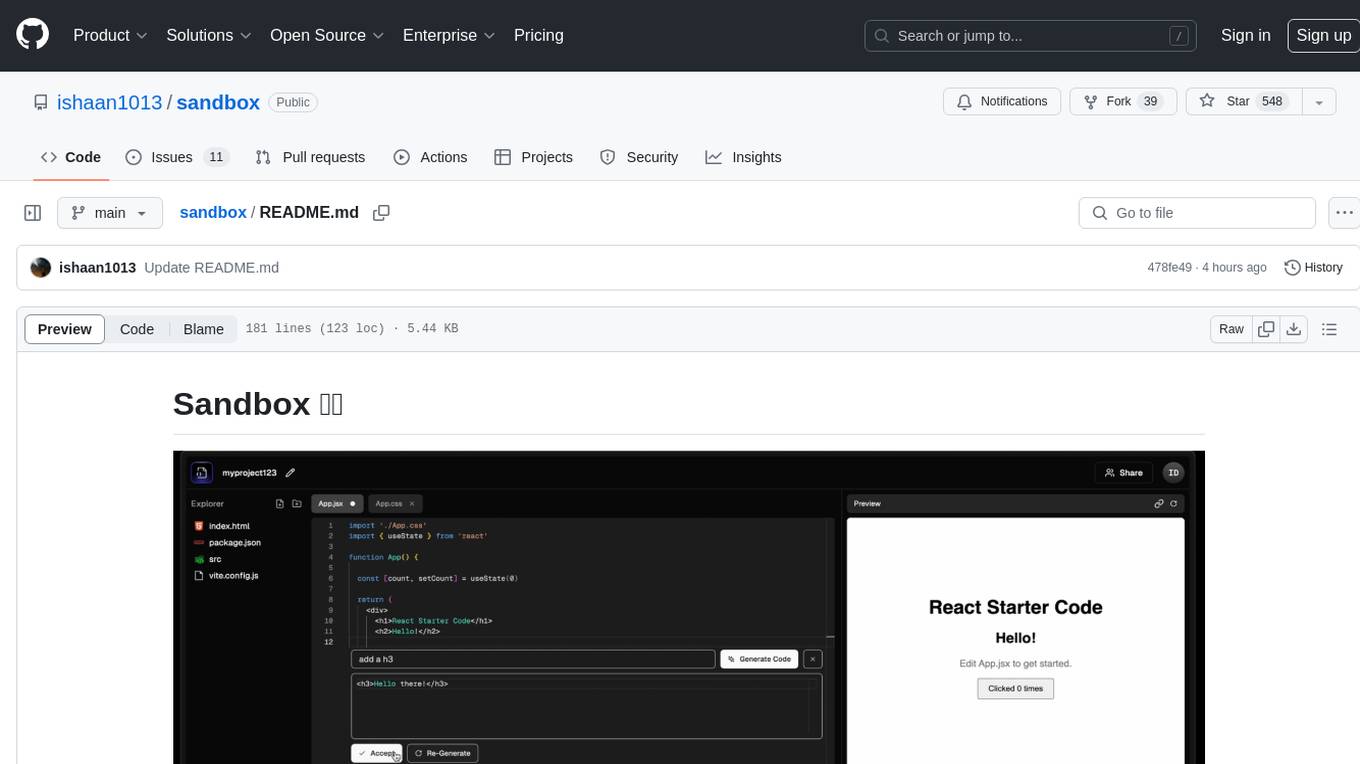
sandbox
Sandbox is an open-source cloud-based code editing environment with custom AI code autocompletion and real-time collaboration. It consists of a frontend built with Next.js, TailwindCSS, Shadcn UI, Clerk, Monaco, and Liveblocks, and a backend with Express, Socket.io, Cloudflare Workers, D1 database, R2 storage, Workers AI, and Drizzle ORM. The backend includes microservices for database, storage, and AI functionalities. Users can run the project locally by setting up environment variables and deploying the containers. Contributions are welcome following the commit convention and structure provided in the repository.
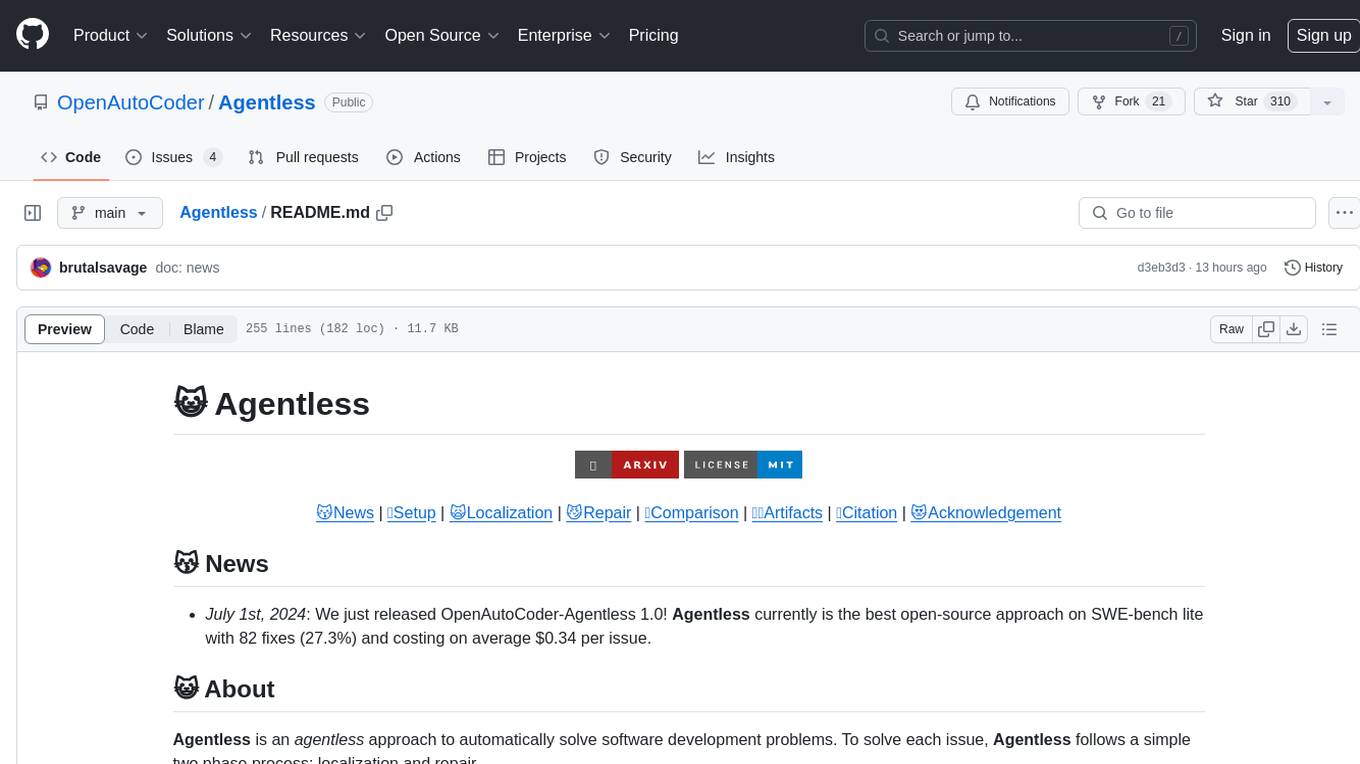
Agentless
Agentless is an open-source tool designed for automatically solving software development problems. It follows a two-phase process of localization and repair to identify faults in specific files, classes, and functions, and generate candidate patches for fixing issues. The tool is aimed at simplifying the software development process by automating issue resolution and patch generation.
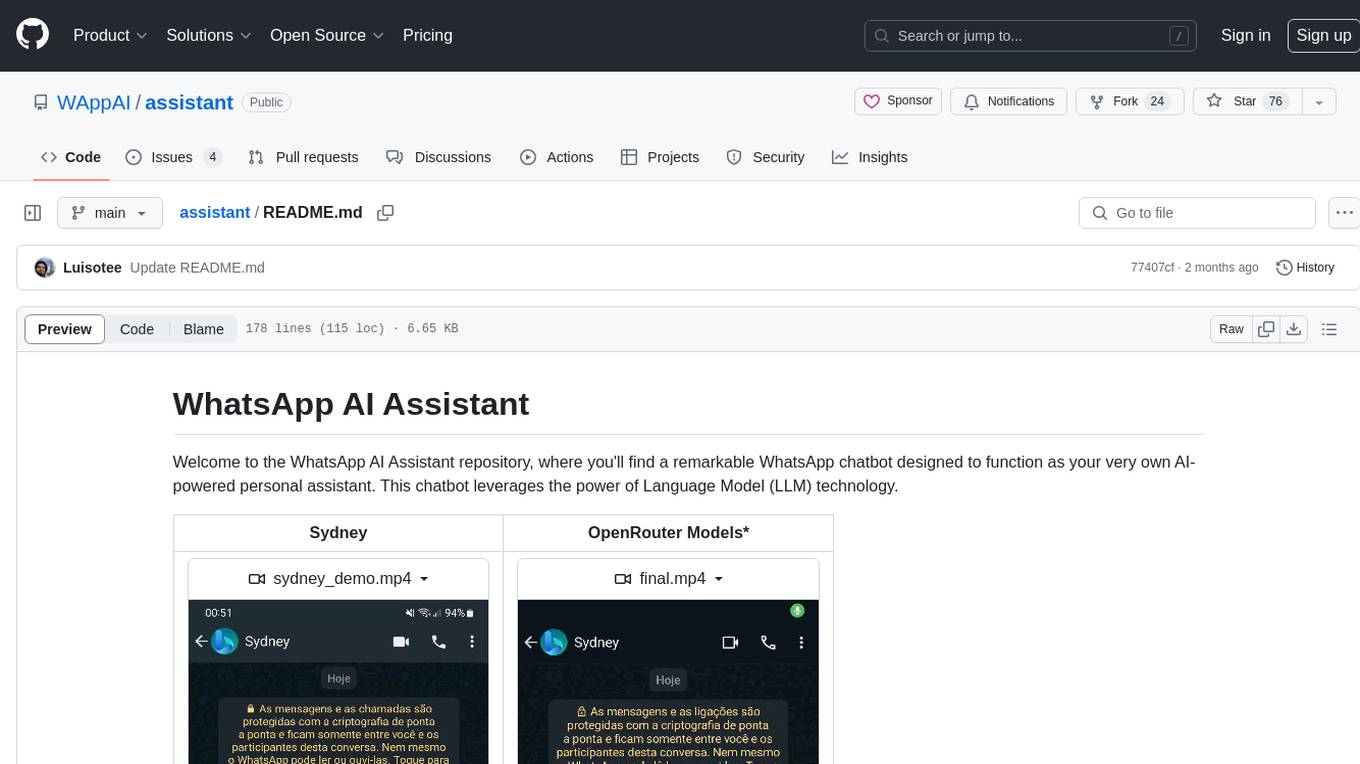
assistant
The WhatsApp AI Assistant repository offers a chatbot named Sydney that serves as an AI-powered personal assistant. It utilizes Language Model (LLM) technology to provide various features such as Google/Bing searching, Google Calendar integration, communication capabilities, group chat compatibility, voice message support, basic text reminders, image recognition, and more. Users can interact with Sydney through natural language queries and voice messages. The chatbot can transcribe voice messages using either the Whisper API or a local method. Additionally, Sydney can be used in group chats by mentioning her username or replying to her last message. The repository welcomes contributions in the form of issue reports, pull requests, and requests for new tools. The creators of the project, Veigamann and Luisotee, are open to job opportunities and can be contacted through their GitHub profiles.
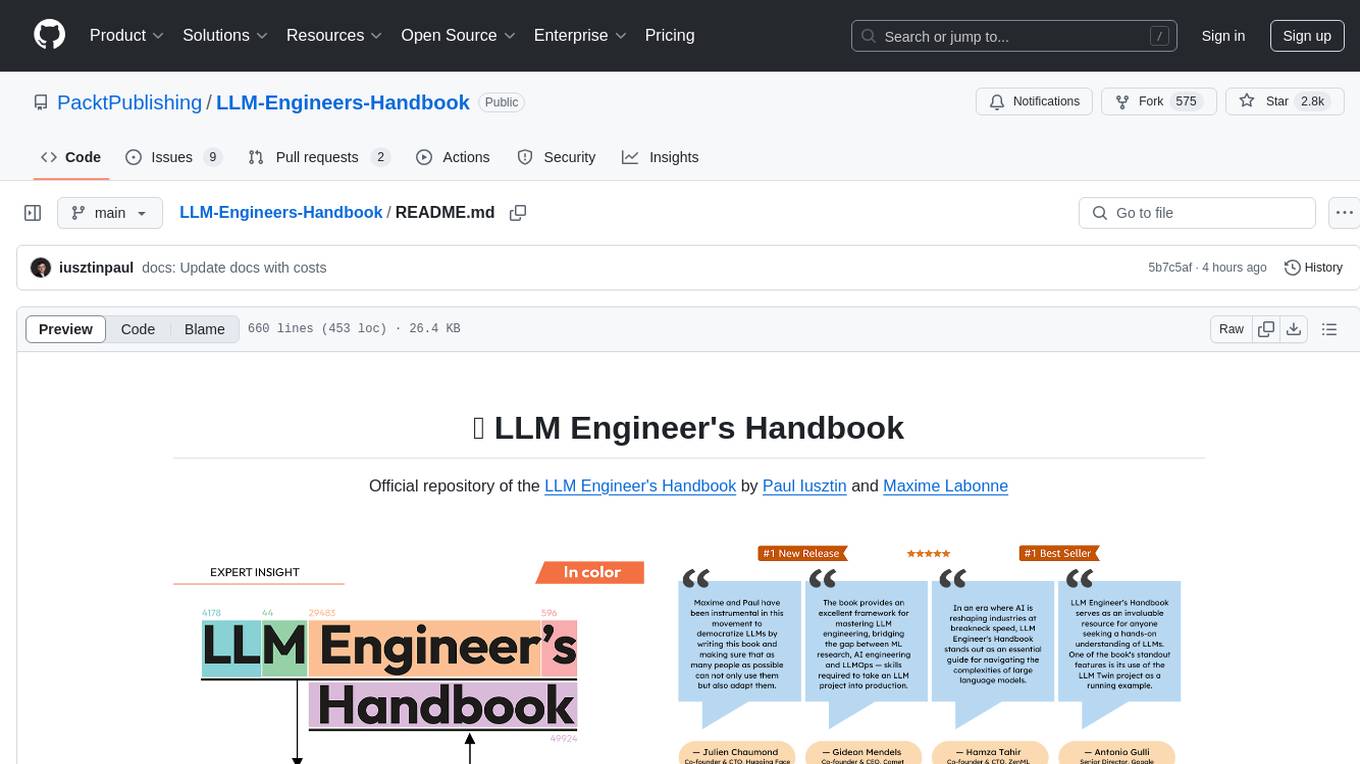
LLM-Engineers-Handbook
The LLM Engineer's Handbook is an official repository containing a comprehensive guide on creating an end-to-end LLM-based system using best practices. It covers data collection & generation, LLM training pipeline, a simple RAG system, production-ready AWS deployment, comprehensive monitoring, and testing and evaluation framework. The repository includes detailed instructions on setting up local and cloud dependencies, project structure, installation steps, infrastructure setup, pipelines for data processing, training, and inference, as well as QA, tests, and running the project end-to-end.
For similar tasks
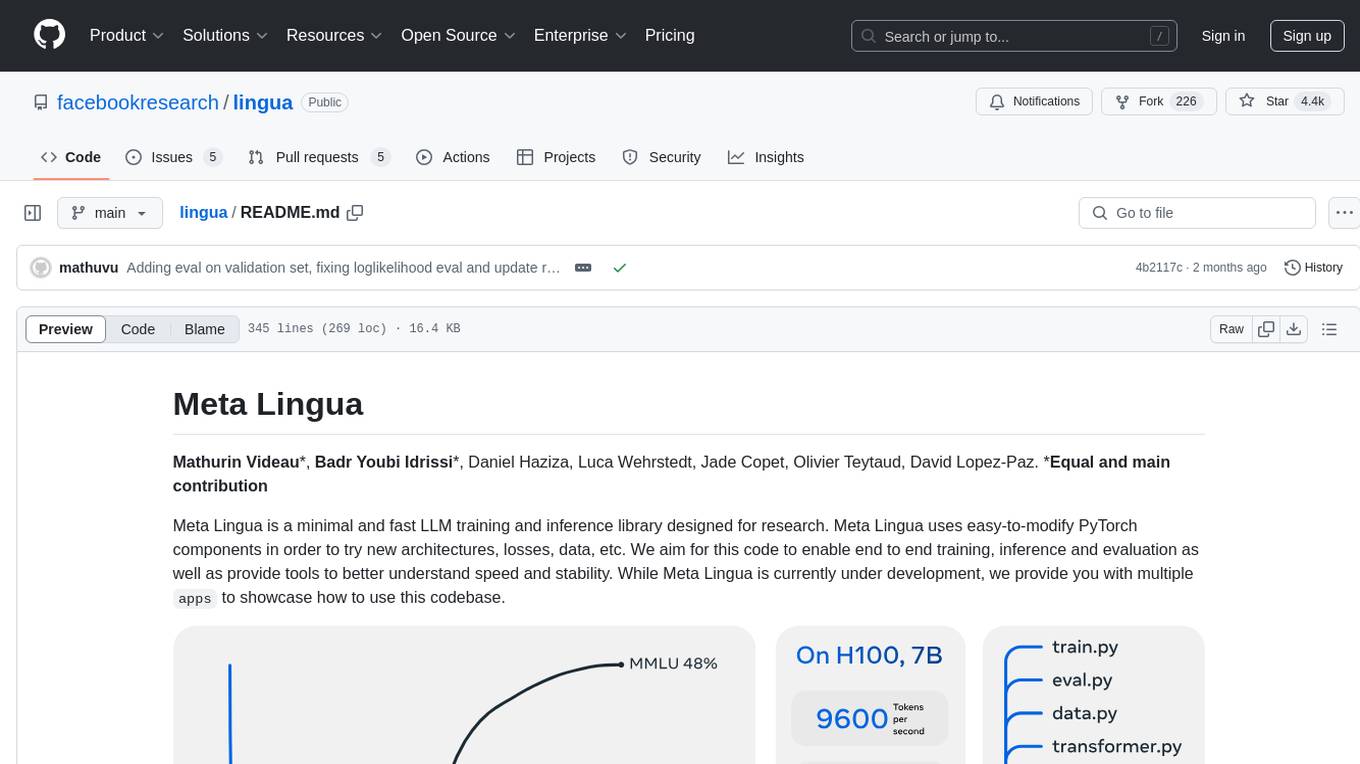
lingua
Meta Lingua is a minimal and fast LLM training and inference library designed for research. It uses easy-to-modify PyTorch components to experiment with new architectures, losses, and data. The codebase enables end-to-end training, inference, and evaluation, providing tools for speed and stability analysis. The repository contains essential components in the 'lingua' folder and scripts that combine these components in the 'apps' folder. Researchers can modify the provided templates to suit their experiments easily. Meta Lingua aims to lower the barrier to entry for LLM research by offering a lightweight and focused codebase.
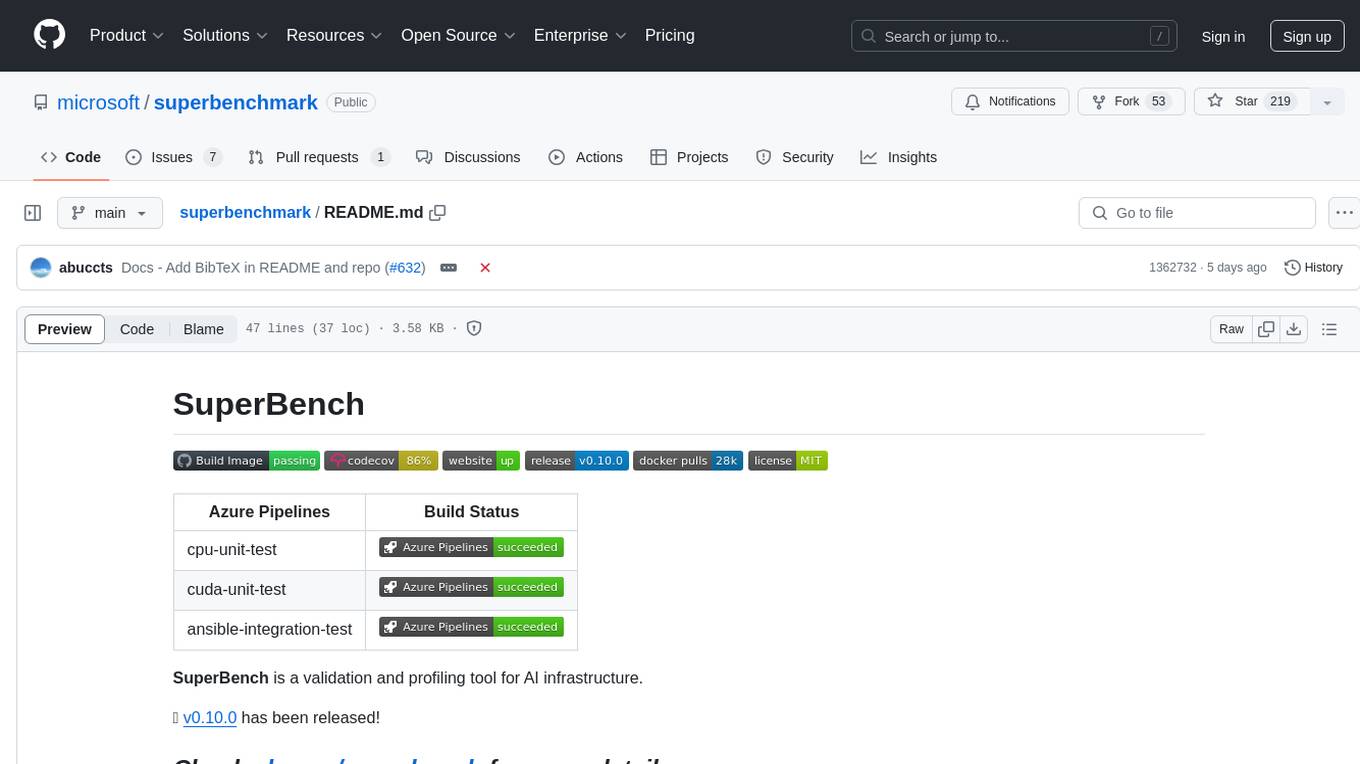
superbenchmark
SuperBench is a validation and profiling tool for AI infrastructure. It provides a comprehensive set of tests and benchmarks to evaluate the performance and reliability of AI systems. The tool helps users identify bottlenecks, optimize configurations, and ensure the stability of their AI infrastructure. SuperBench is designed to streamline the validation process and improve the overall efficiency of AI deployments.
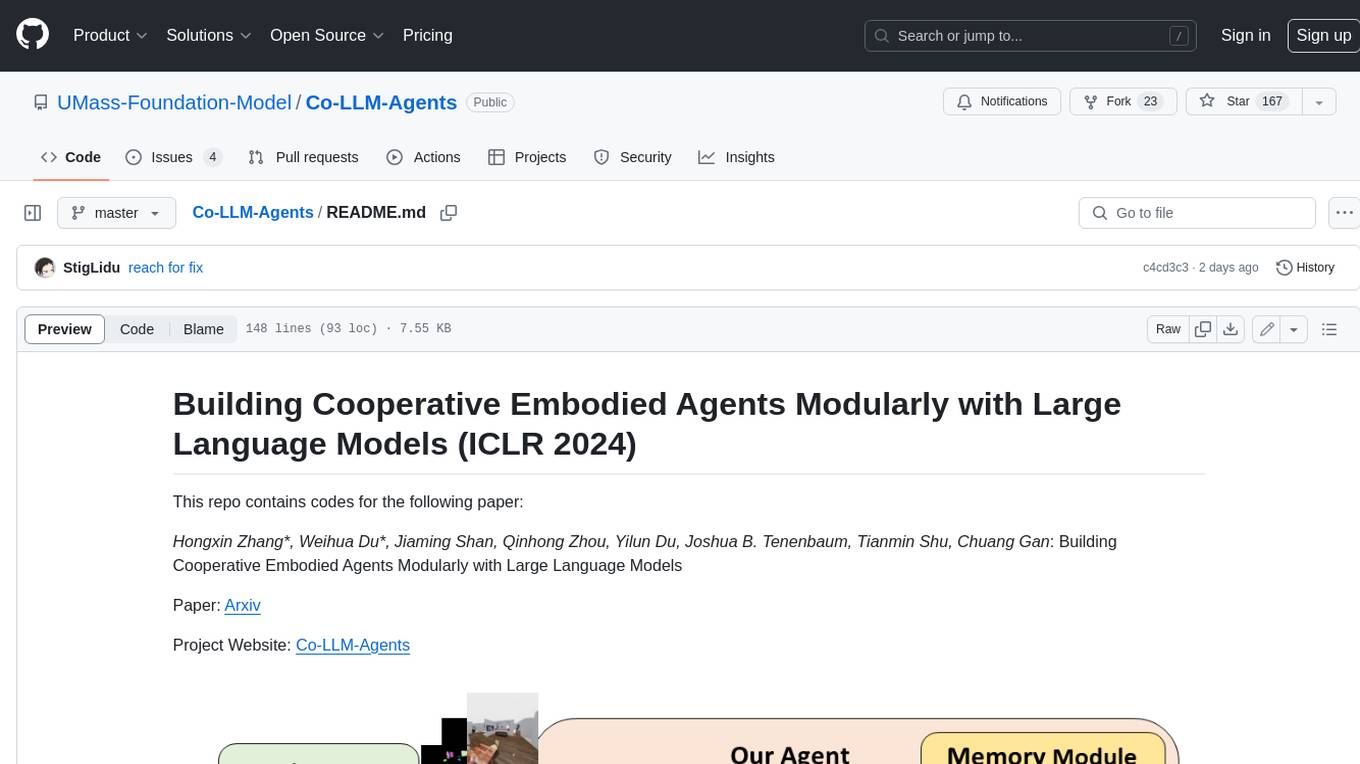
Co-LLM-Agents
This repository contains code for building cooperative embodied agents modularly with large language models. The agents are trained to perform tasks in two different environments: ThreeDWorld Multi-Agent Transport (TDW-MAT) and Communicative Watch-And-Help (C-WAH). TDW-MAT is a multi-agent environment where agents must transport objects to a goal position using containers. C-WAH is an extension of the Watch-And-Help challenge, which enables agents to send messages to each other. The code in this repository can be used to train agents to perform tasks in both of these environments.
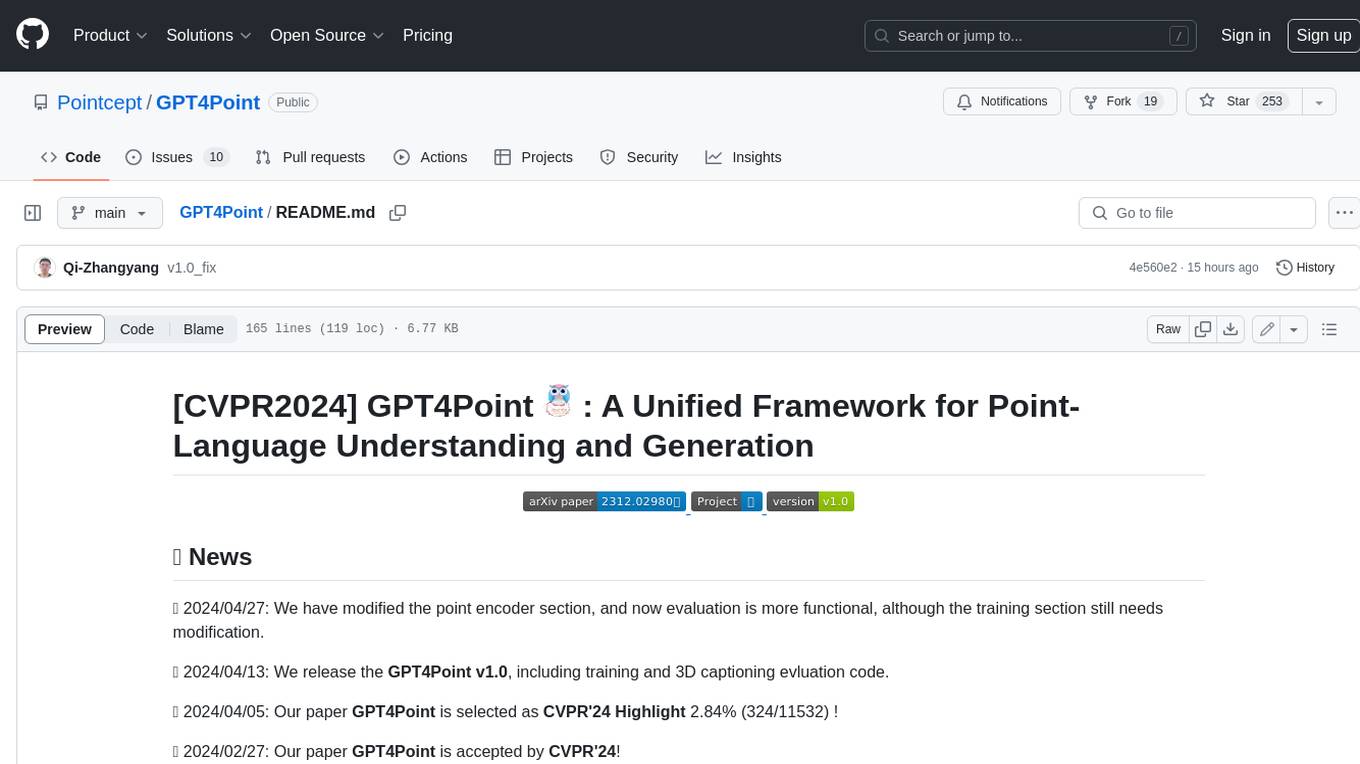
GPT4Point
GPT4Point is a unified framework for point-language understanding and generation. It aligns 3D point clouds with language, providing a comprehensive solution for tasks such as 3D captioning and controlled 3D generation. The project includes an automated point-language dataset annotation engine, a novel object-level point cloud benchmark, and a 3D multi-modality model. Users can train and evaluate models using the provided code and datasets, with a focus on improving models' understanding capabilities and facilitating the generation of 3D objects.
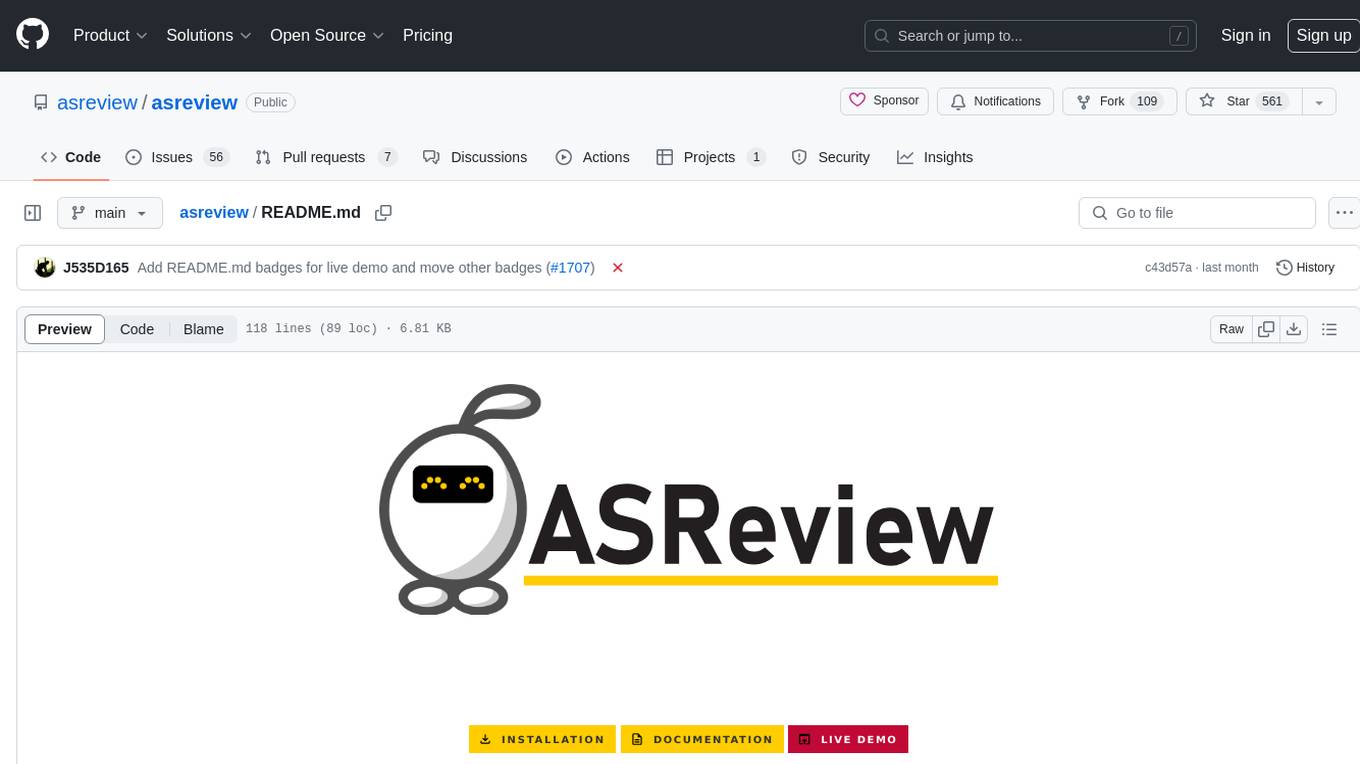
asreview
The ASReview project implements active learning for systematic reviews, utilizing AI-aided pipelines to assist in finding relevant texts for search tasks. It accelerates the screening of textual data with minimal human input, saving time and increasing output quality. The software offers three modes: Oracle for interactive screening, Exploration for teaching purposes, and Simulation for evaluating active learning models. ASReview LAB is designed to support decision-making in any discipline or industry by improving efficiency and transparency in screening large amounts of textual data.
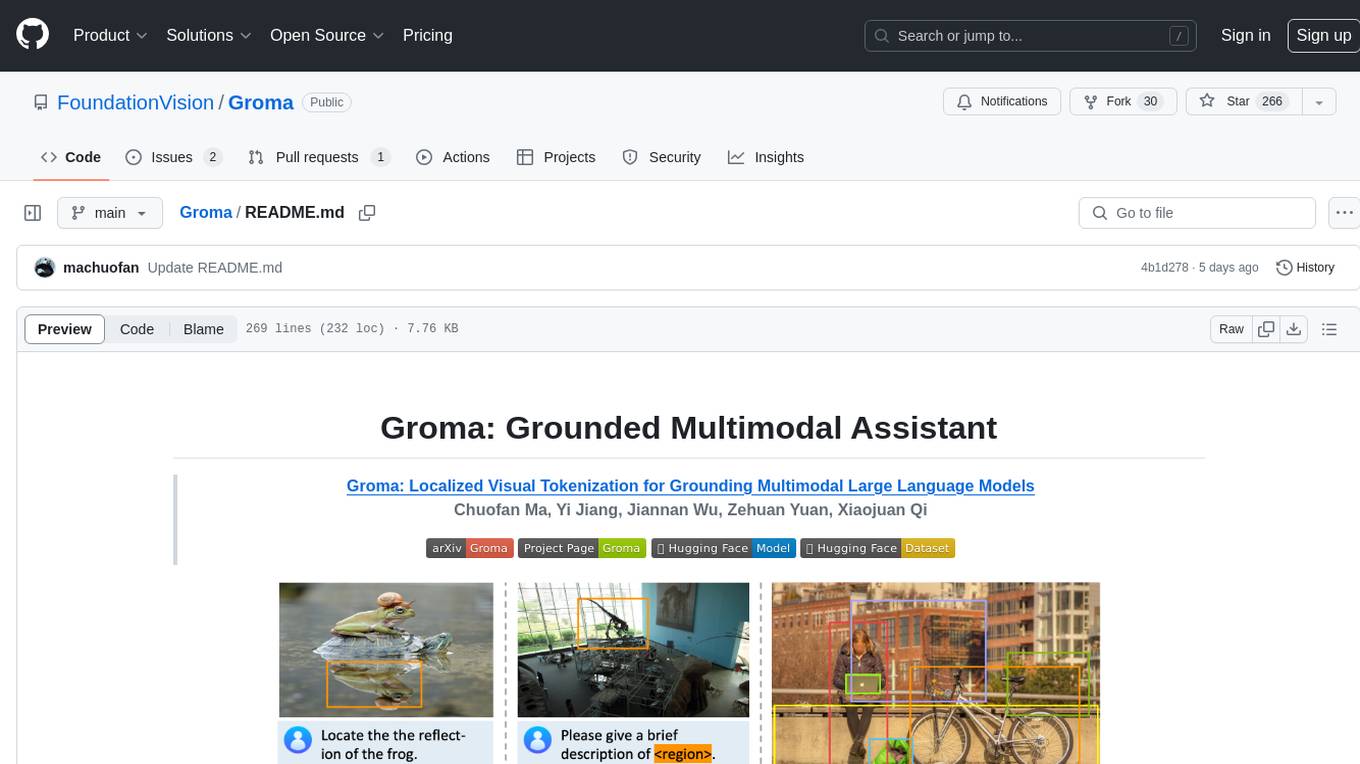
Groma
Groma is a grounded multimodal assistant that excels in region understanding and visual grounding. It can process user-defined region inputs and generate contextually grounded long-form responses. The tool presents a unique paradigm for multimodal large language models, focusing on visual tokenization for localization. Groma achieves state-of-the-art performance in referring expression comprehension benchmarks. The tool provides pretrained model weights and instructions for data preparation, training, inference, and evaluation. Users can customize training by starting from intermediate checkpoints. Groma is designed to handle tasks related to detection pretraining, alignment pretraining, instruction finetuning, instruction following, and more.
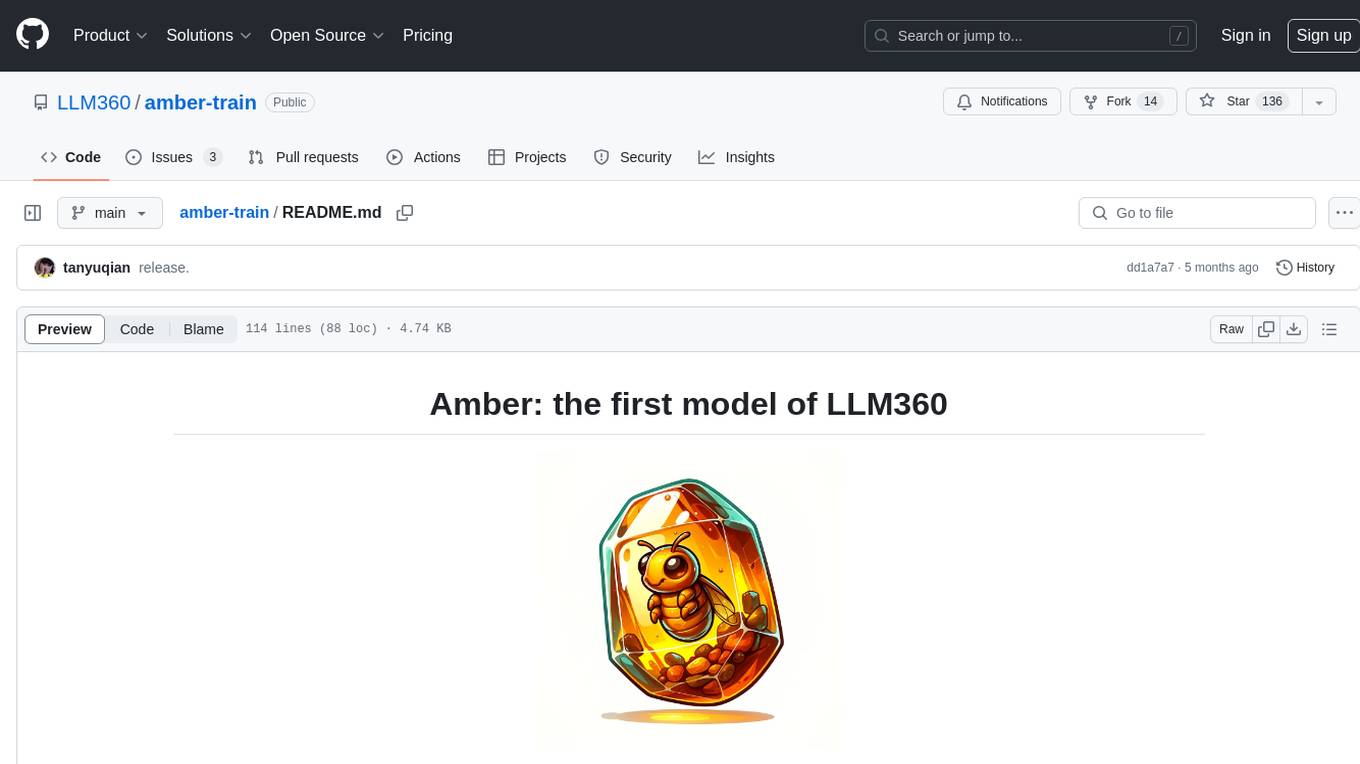
amber-train
Amber is the first model in the LLM360 family, an initiative for comprehensive and fully open-sourced LLMs. It is a 7B English language model with the LLaMA architecture. The model type is a language model with the same architecture as LLaMA-7B. It is licensed under Apache 2.0. The resources available include training code, data preparation, metrics, and fully processed Amber pretraining data. The model has been trained on various datasets like Arxiv, Book, C4, Refined-Web, StarCoder, StackExchange, and Wikipedia. The hyperparameters include a total of 6.7B parameters, hidden size of 4096, intermediate size of 11008, 32 attention heads, 32 hidden layers, RMSNorm ε of 1e^-6, max sequence length of 2048, and a vocabulary size of 32000.
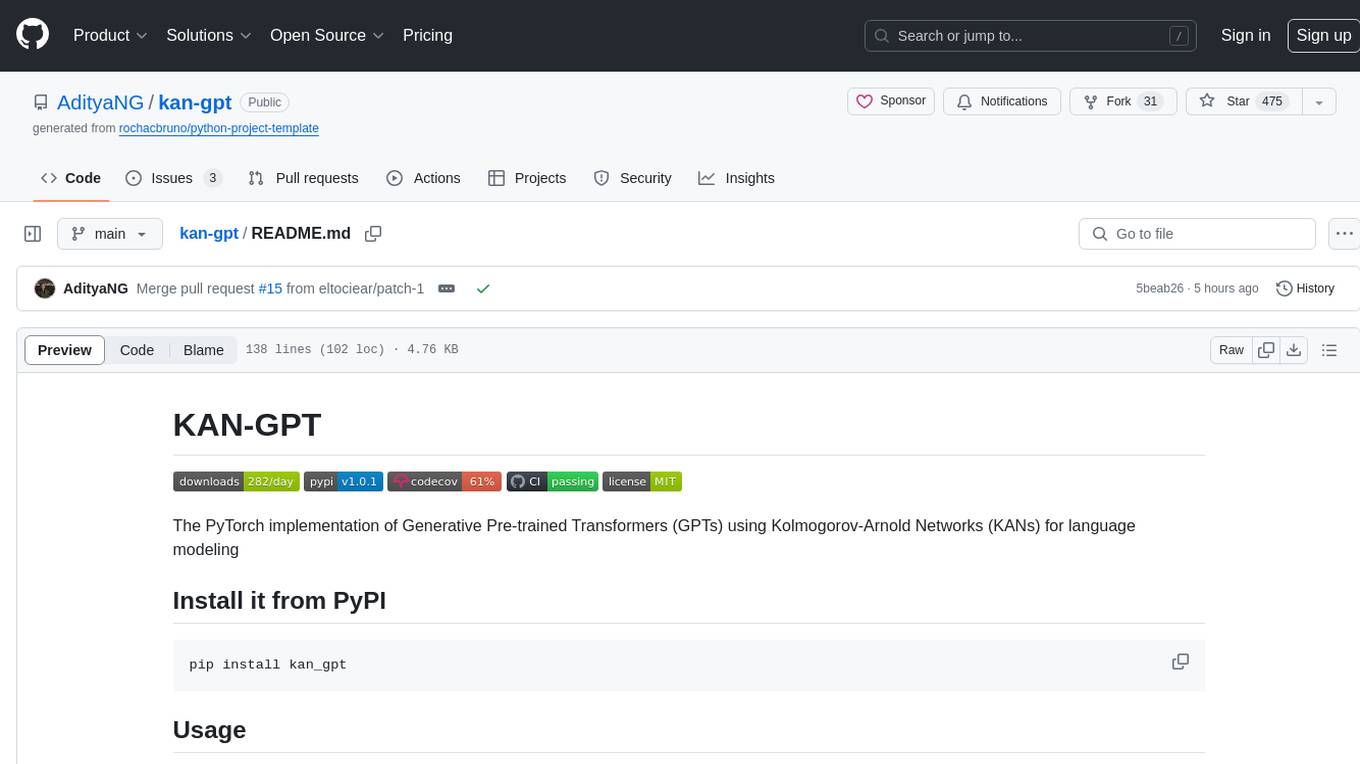
kan-gpt
The KAN-GPT repository is a PyTorch implementation of Generative Pre-trained Transformers (GPTs) using Kolmogorov-Arnold Networks (KANs) for language modeling. It provides a model for generating text based on prompts, with a focus on improving performance compared to traditional MLP-GPT models. The repository includes scripts for training the model, downloading datasets, and evaluating model performance. Development tasks include integrating with other libraries, testing, and documentation.
For similar jobs
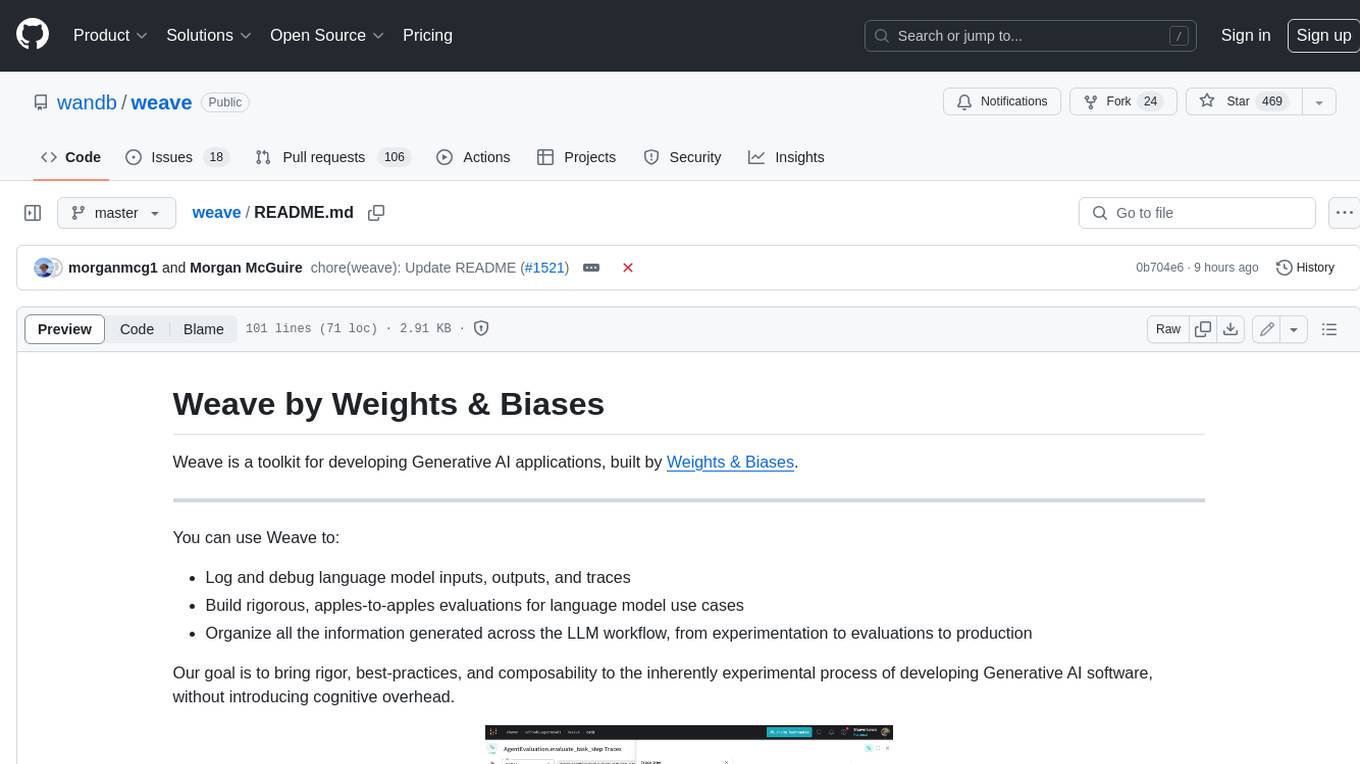
weave
Weave is a toolkit for developing Generative AI applications, built by Weights & Biases. With Weave, you can log and debug language model inputs, outputs, and traces; build rigorous, apples-to-apples evaluations for language model use cases; and organize all the information generated across the LLM workflow, from experimentation to evaluations to production. Weave aims to bring rigor, best-practices, and composability to the inherently experimental process of developing Generative AI software, without introducing cognitive overhead.
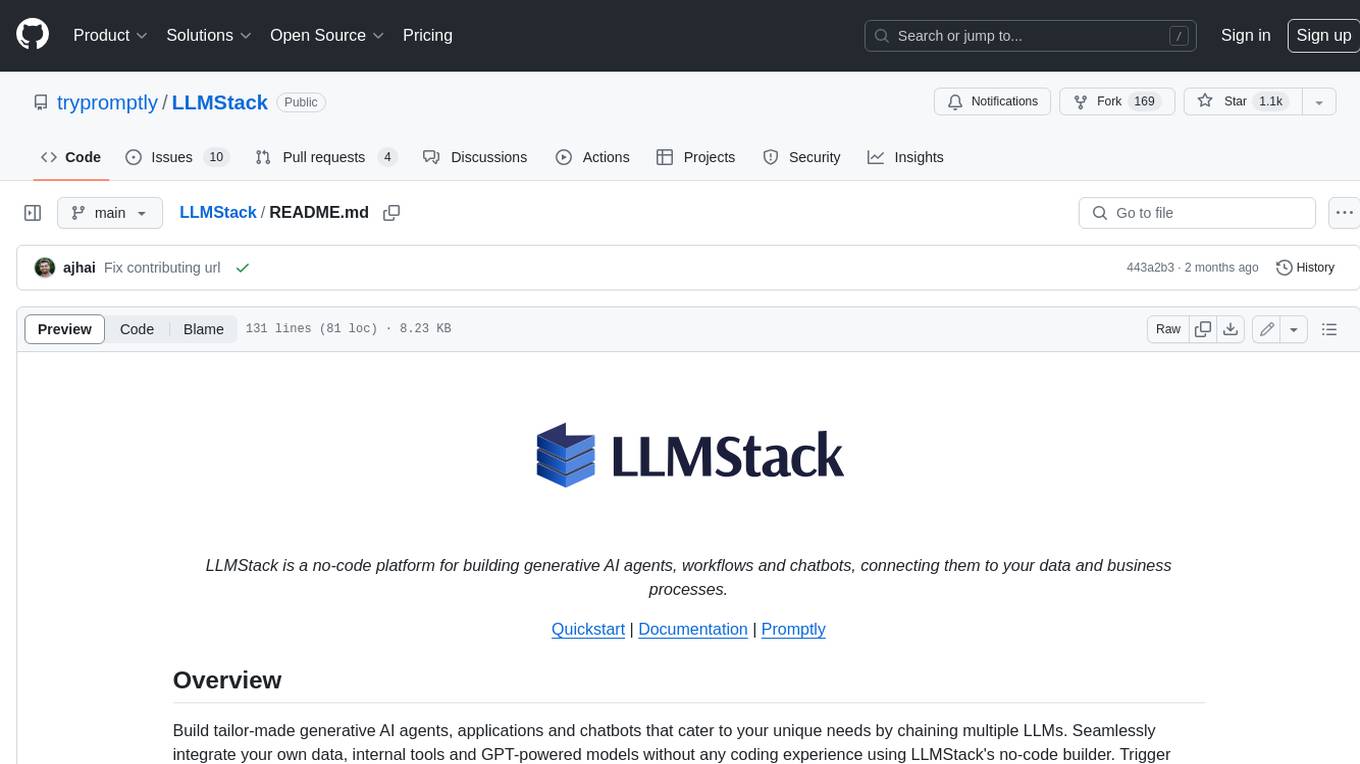
LLMStack
LLMStack is a no-code platform for building generative AI agents, workflows, and chatbots. It allows users to connect their own data, internal tools, and GPT-powered models without any coding experience. LLMStack can be deployed to the cloud or on-premise and can be accessed via HTTP API or triggered from Slack or Discord.
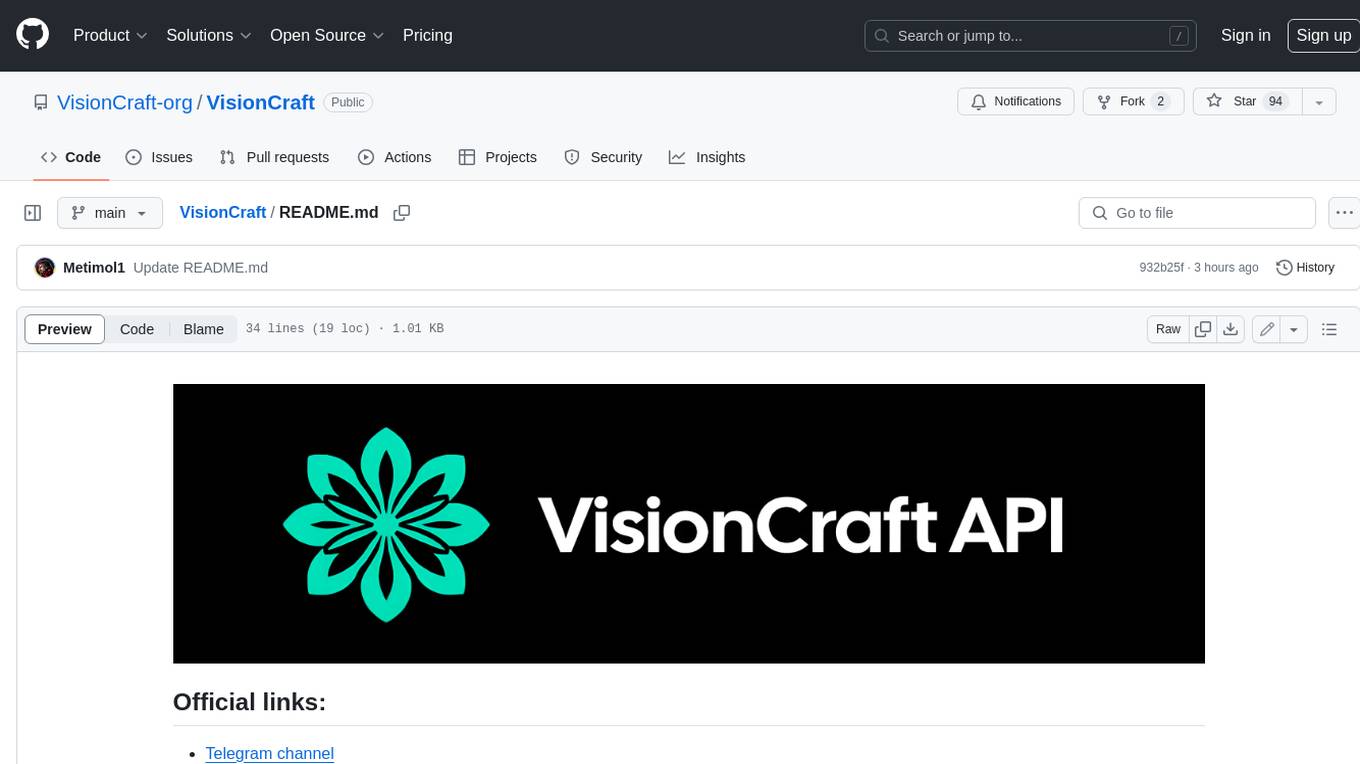
VisionCraft
The VisionCraft API is a free API for using over 100 different AI models. From images to sound.
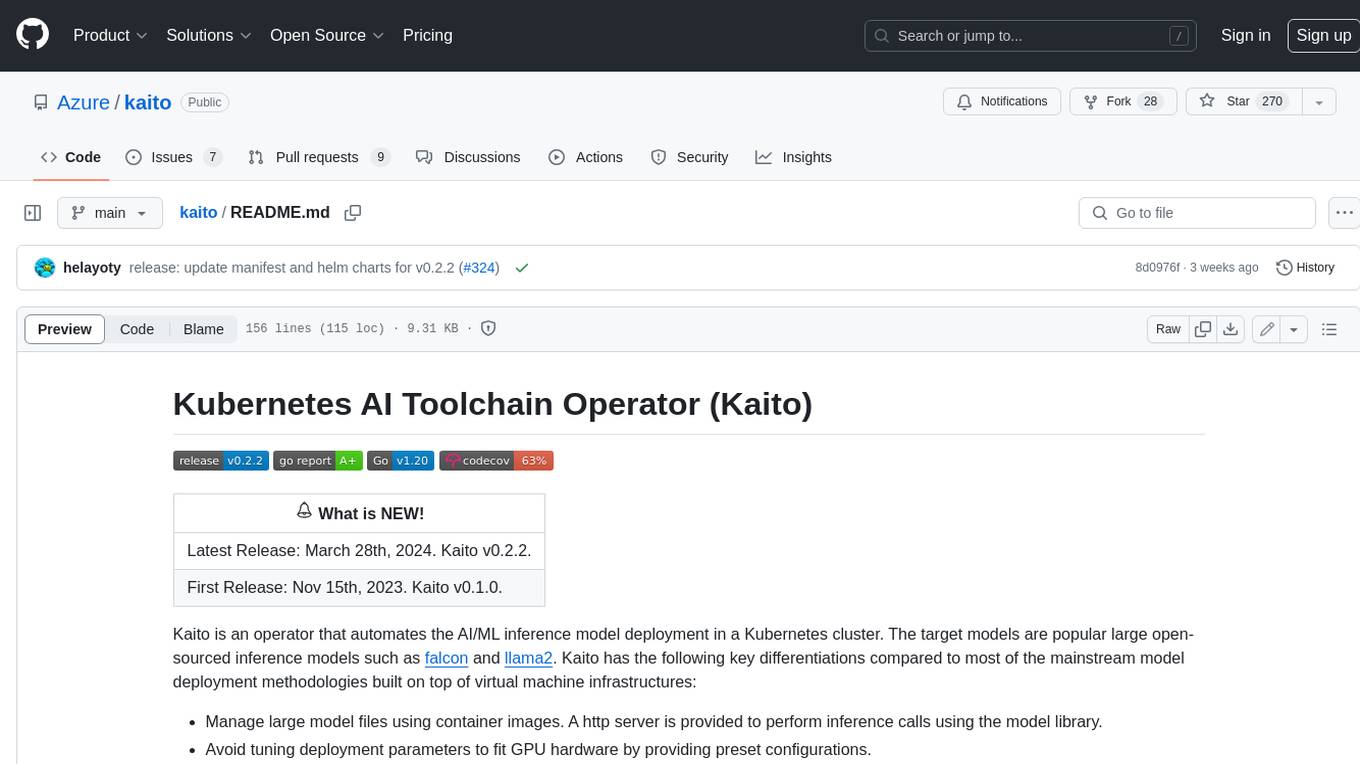
kaito
Kaito is an operator that automates the AI/ML inference model deployment in a Kubernetes cluster. It manages large model files using container images, avoids tuning deployment parameters to fit GPU hardware by providing preset configurations, auto-provisions GPU nodes based on model requirements, and hosts large model images in the public Microsoft Container Registry (MCR) if the license allows. Using Kaito, the workflow of onboarding large AI inference models in Kubernetes is largely simplified.
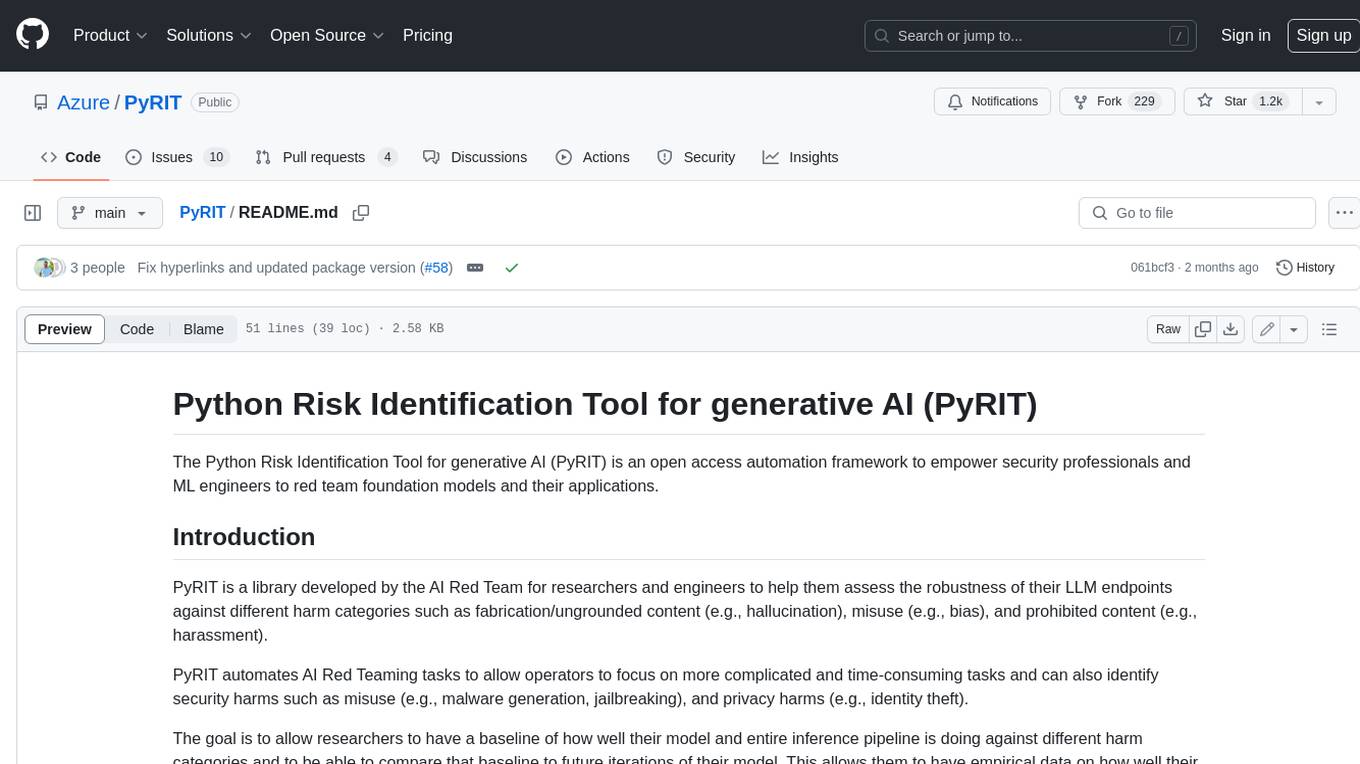
PyRIT
PyRIT is an open access automation framework designed to empower security professionals and ML engineers to red team foundation models and their applications. It automates AI Red Teaming tasks to allow operators to focus on more complicated and time-consuming tasks and can also identify security harms such as misuse (e.g., malware generation, jailbreaking), and privacy harms (e.g., identity theft). The goal is to allow researchers to have a baseline of how well their model and entire inference pipeline is doing against different harm categories and to be able to compare that baseline to future iterations of their model. This allows them to have empirical data on how well their model is doing today, and detect any degradation of performance based on future improvements.
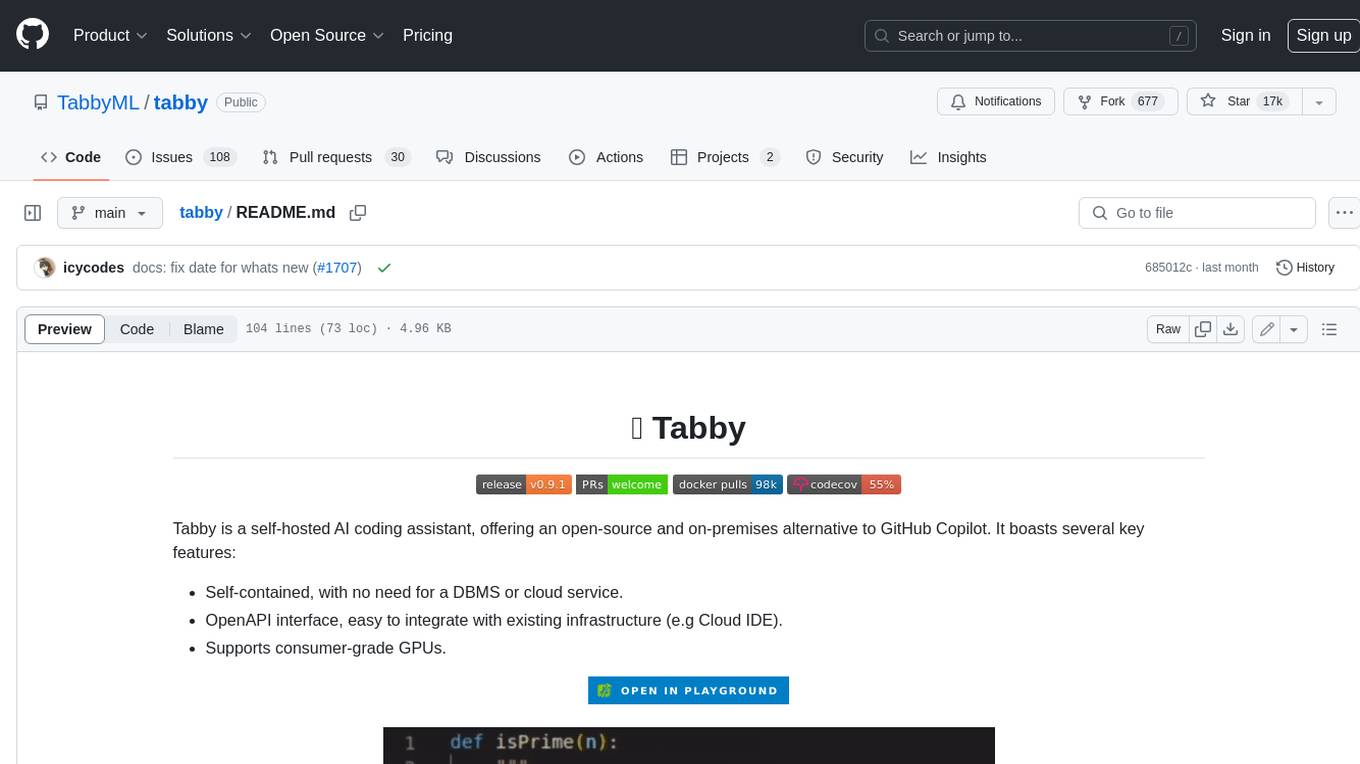
tabby
Tabby is a self-hosted AI coding assistant, offering an open-source and on-premises alternative to GitHub Copilot. It boasts several key features: * Self-contained, with no need for a DBMS or cloud service. * OpenAPI interface, easy to integrate with existing infrastructure (e.g Cloud IDE). * Supports consumer-grade GPUs.
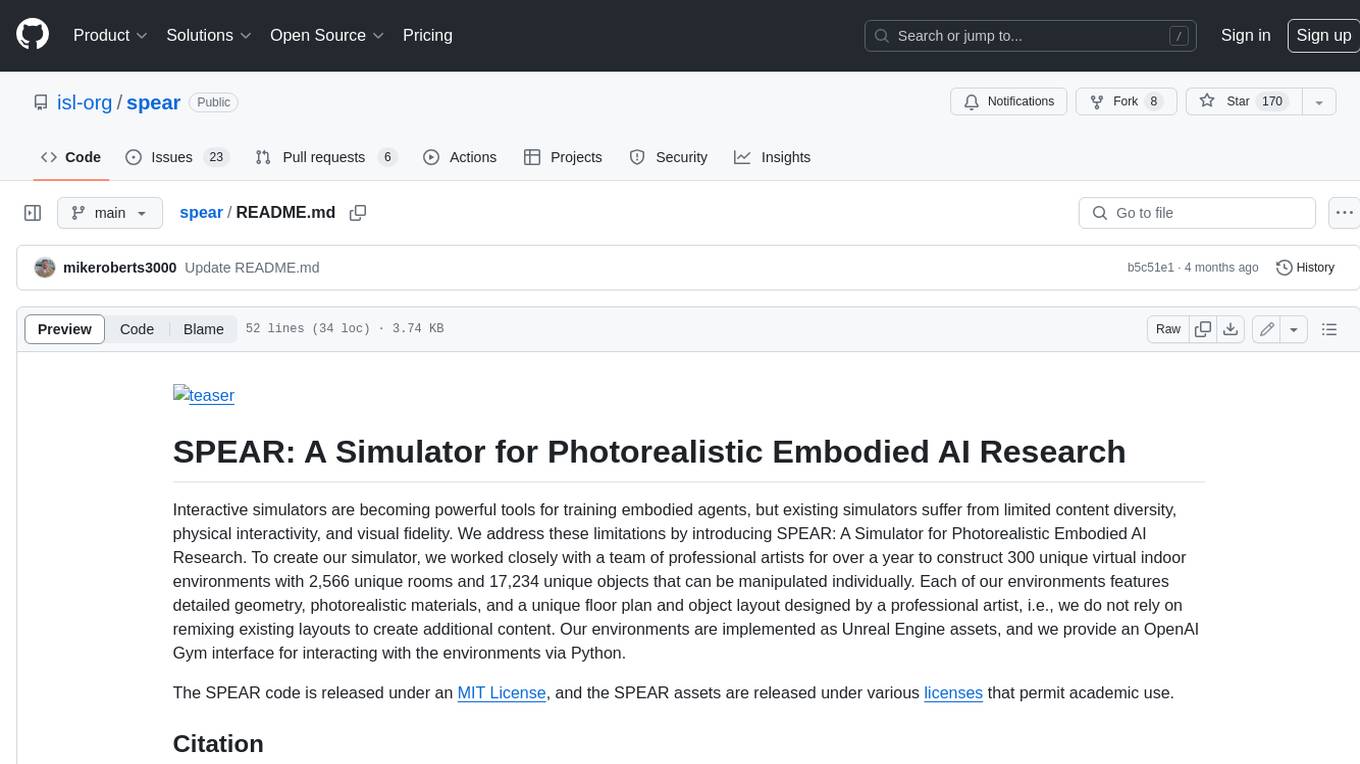
spear
SPEAR (Simulator for Photorealistic Embodied AI Research) is a powerful tool for training embodied agents. It features 300 unique virtual indoor environments with 2,566 unique rooms and 17,234 unique objects that can be manipulated individually. Each environment is designed by a professional artist and features detailed geometry, photorealistic materials, and a unique floor plan and object layout. SPEAR is implemented as Unreal Engine assets and provides an OpenAI Gym interface for interacting with the environments via Python.
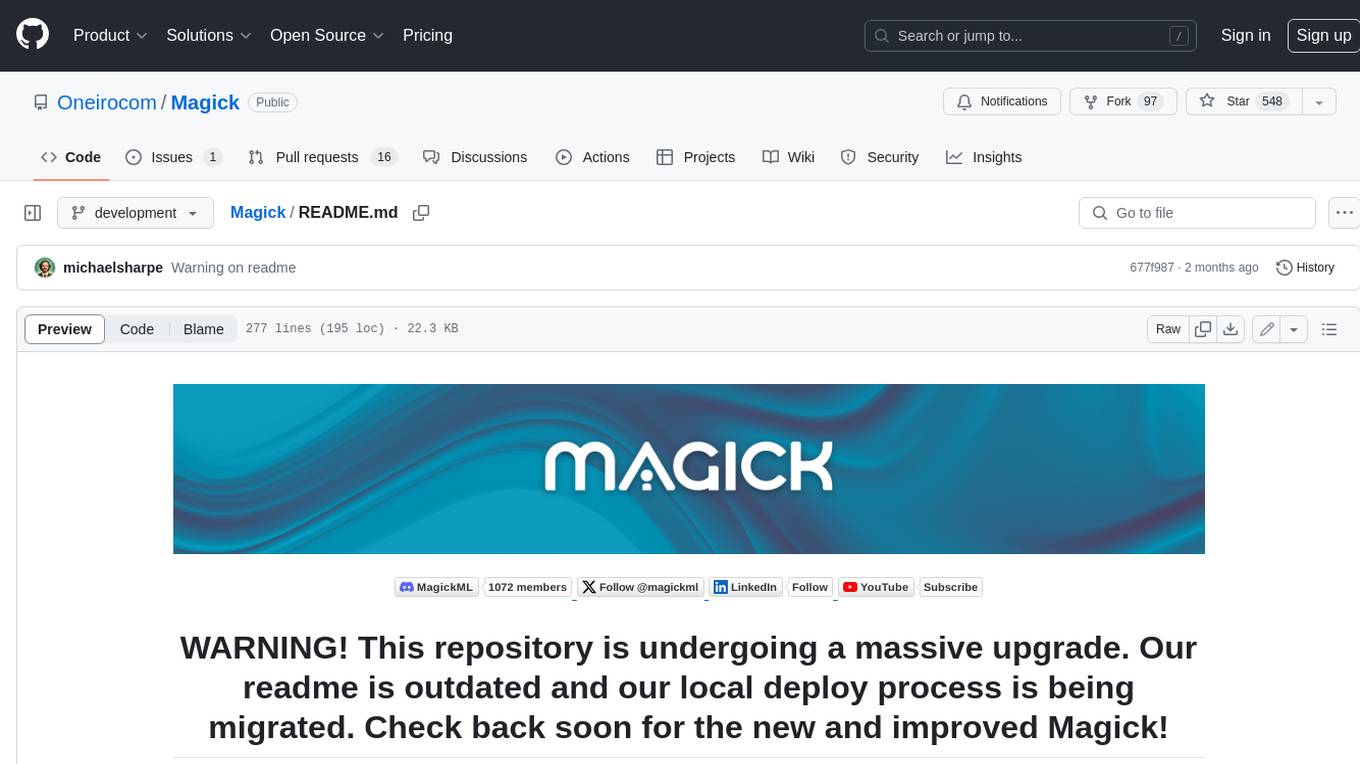
Magick
Magick is a groundbreaking visual AIDE (Artificial Intelligence Development Environment) for no-code data pipelines and multimodal agents. Magick can connect to other services and comes with nodes and templates well-suited for intelligent agents, chatbots, complex reasoning systems and realistic characters.