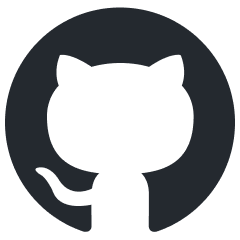
stagehand
An AI web browsing framework focused on simplicity and extensibility.
Stars: 5248
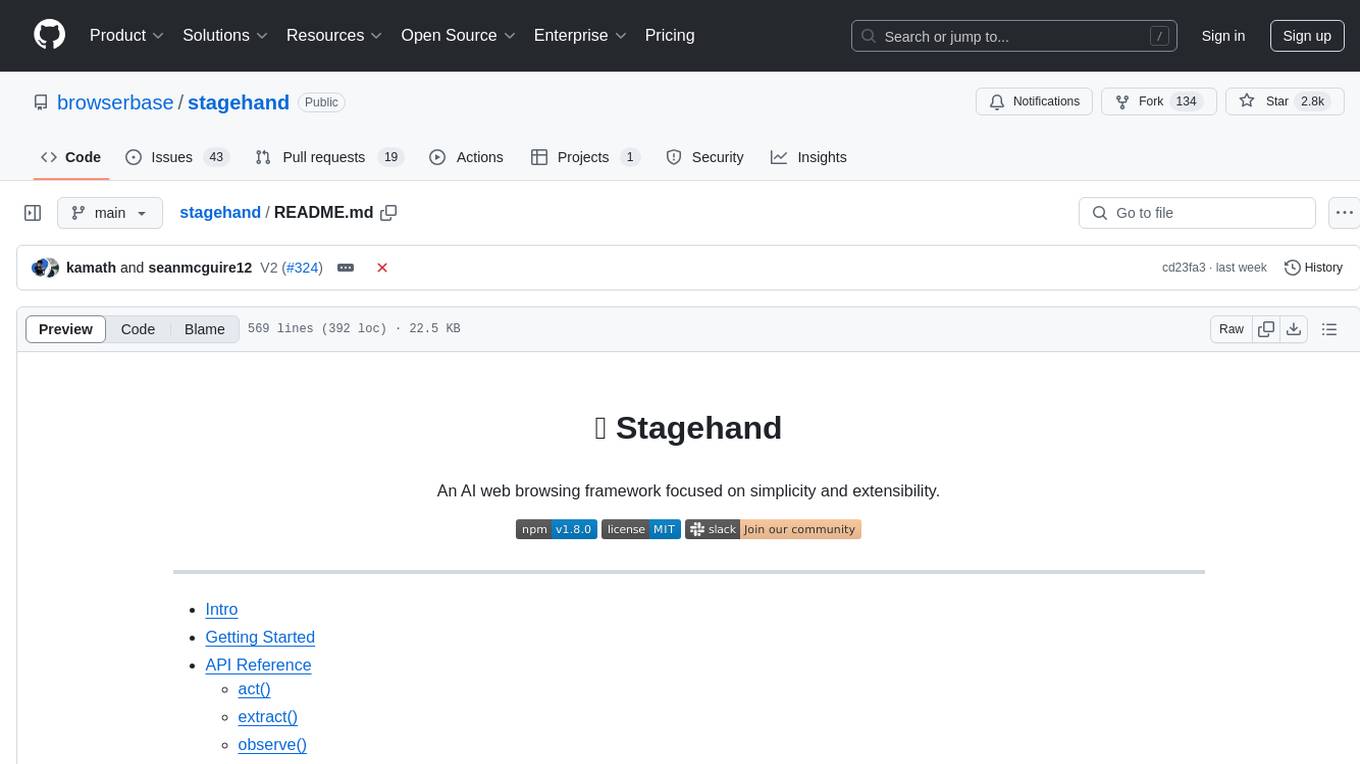
Stagehand is an AI web browsing framework that simplifies and extends web automation using three simple APIs: act, extract, and observe. It aims to provide a lightweight, configurable framework without complex abstractions, allowing users to automate web tasks reliably. The tool generates Playwright code based on atomic instructions provided by the user, enabling natural language-driven web automation. Stagehand is open source, maintained by the Browserbase team, and supports different models and model providers for flexibility in automation tasks.
README:
An AI web browsing framework focused on simplicity and extensibility.
Read the Docs
Stagehand is the easiest way to build browser automations. It is fully compatible with Playwright, offering three simple AI APIs (act
, extract
, and observe
) on top of the base Playwright Page
class that provide the building blocks for web automation via natural language.
Here's a sample of what you can do with Stagehand:
// Keep your existing Playwright code unchanged
await page.goto("https://docs.stagehand.dev");
// Stagehand AI: Act on the page
await page.act("click on the 'Quickstart'");
// Stagehand AI: Extract data from the page
const { description } = await page.extract({
instruction: "extract the description of the page",
schema: z.object({
description: z.string(),
}),
});
Stagehand adds determinism to otherwise unpredictable agents.
While there's no limit to what you could instruct Stagehand to do, our primitives allow you to control how much you want to leave to an AI. It works best when your code is a sequence of atomic actions. Instead of writing a single script for a single website, Stagehand allows you to write durable, self-healing, and repeatable web automation workflows that actually work.
[!NOTE]
Stagehand
is currently available as an early release, and we're actively seeking feedback from the community. Please join our Slack community to stay updated on the latest developments and provide feedback.
Visit docs.stagehand.dev to view the full documentation.
To create a new Stagehand project configured to our default settings, run:
npx create-browser-app --example quickstart
Read our Quickstart Guide in the docs for more information.
You can also add Stagehand to an existing Typescript project by running:
npm install @browserbasehq/stagehand zod
npx playwright install # if running locally
git clone https://github.com/browserbase/stagehand.git
cd stagehand
npm install
npx playwright install
npm run example # run the blank script at ./examples/example.ts
Stagehand is best when you have an API key for an LLM provider and Browserbase credentials. To add these to your project, run:
cp .env.example .env
nano .env # Edit the .env file to add API keys
[!NOTE]
We highly value contributions to Stagehand! For questions or support, please join our Slack community.
At a high level, we're focused on improving reliability, speed, and cost in that order of priority. If you're interested in contributing, we strongly recommend reaching out to Anirudh Kamath or Paul Klein in our Slack community before starting to ensure that your contribution aligns with our goals.
For more information, please see our Contributing Guide.
This project heavily relies on Playwright as a resilient backbone to automate the web. It also would not be possible without the awesome techniques and discoveries made by tarsier, and fuji-web.
We'd like to thank the following people for their contributions to Stagehand:
- Jeremy Press wrote the original MVP of Stagehand and continues to be an ally to the project.
-
Navid Pour is heavily responsible for the current architecture of Stagehand and the
act
API. -
Sean McGuire is a major contributor to the project and has been a great help with improving the
extract
API and getting evals to a high level. - Filip Michalsky has been doing a lot of work on building out integrations like Langchain and Claude MCP, generally improving the repository, and unblocking users.
- Sameel Arif is a major contributor to the project, especially around improving the developer experience.
Licensed under the MIT License.
Copyright 2025 Browserbase, Inc.
For Tasks:
Click tags to check more tools for each tasksFor Jobs:
Alternative AI tools for stagehand
Similar Open Source Tools
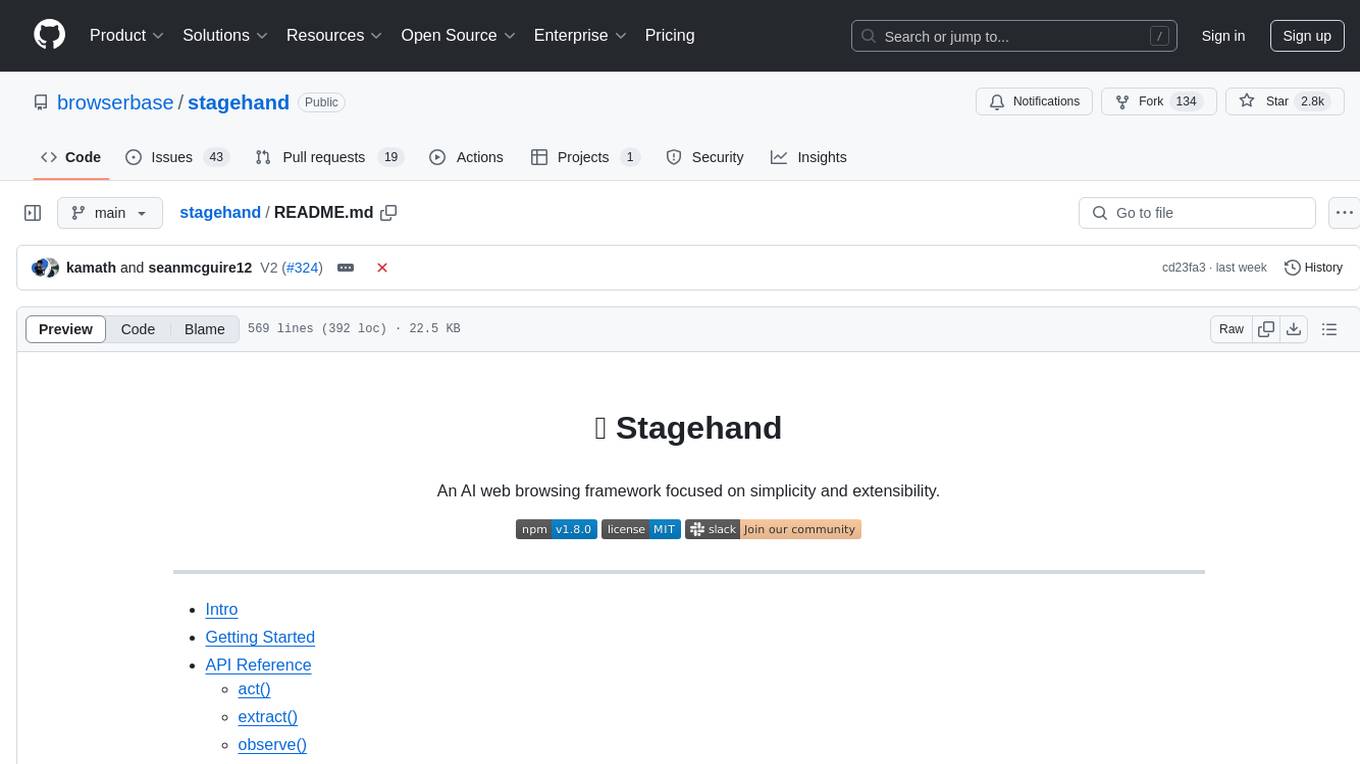
stagehand
Stagehand is an AI web browsing framework that simplifies and extends web automation using three simple APIs: act, extract, and observe. It aims to provide a lightweight, configurable framework without complex abstractions, allowing users to automate web tasks reliably. The tool generates Playwright code based on atomic instructions provided by the user, enabling natural language-driven web automation. Stagehand is open source, maintained by the Browserbase team, and supports different models and model providers for flexibility in automation tasks.
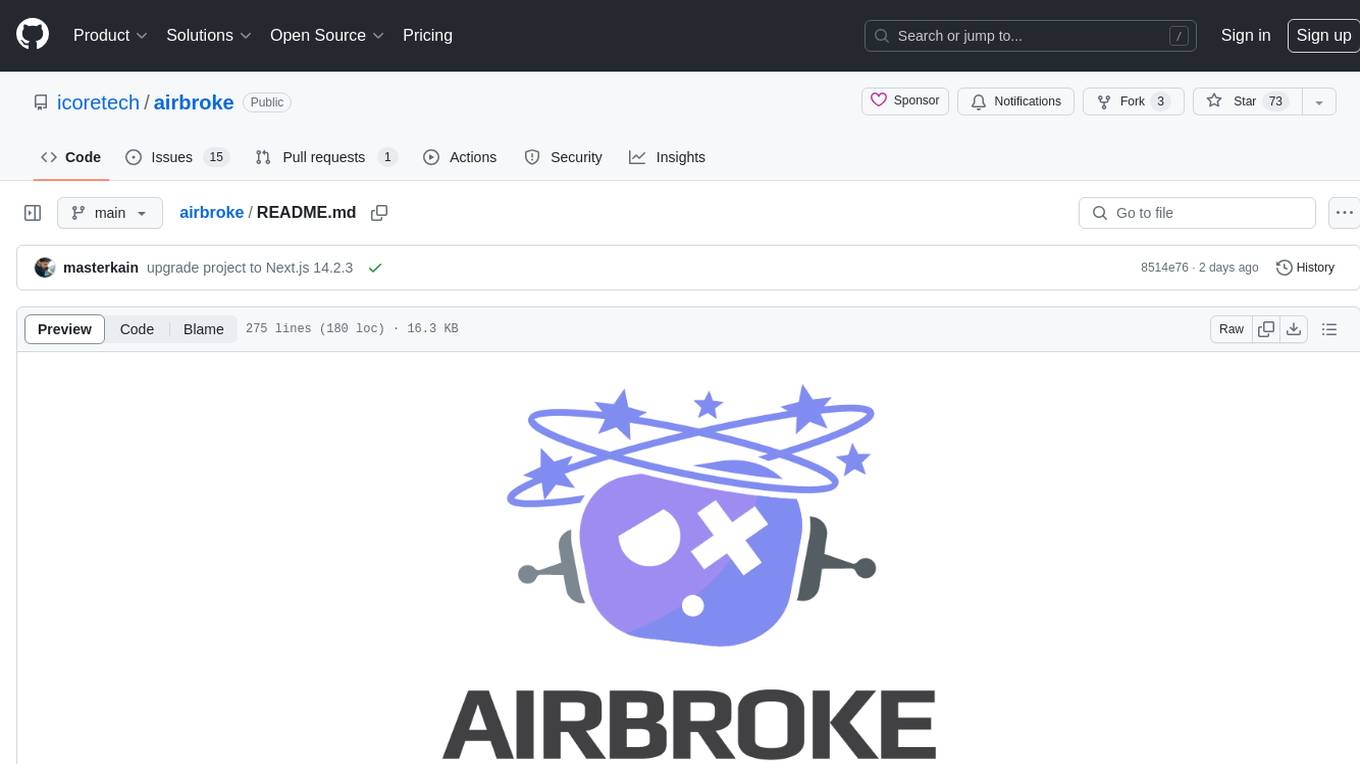
airbroke
Airbroke is an open-source error catcher tool designed for modern web applications. It provides a PostgreSQL-based backend with an Airbrake-compatible HTTP collector endpoint and a React-based frontend for error management. The tool focuses on simplicity, maintaining a small database footprint even under heavy data ingestion. Users can ask AI about issues, replay HTTP exceptions, and save/manage bookmarks for important occurrences. Airbroke supports multiple OAuth providers for secure user authentication and offers occurrence charts for better insights into error occurrences. The tool can be deployed in various ways, including building from source, using Docker images, deploying on Vercel, Render.com, Kubernetes with Helm, or Docker Compose. It requires Node.js, PostgreSQL, and specific system resources for deployment.
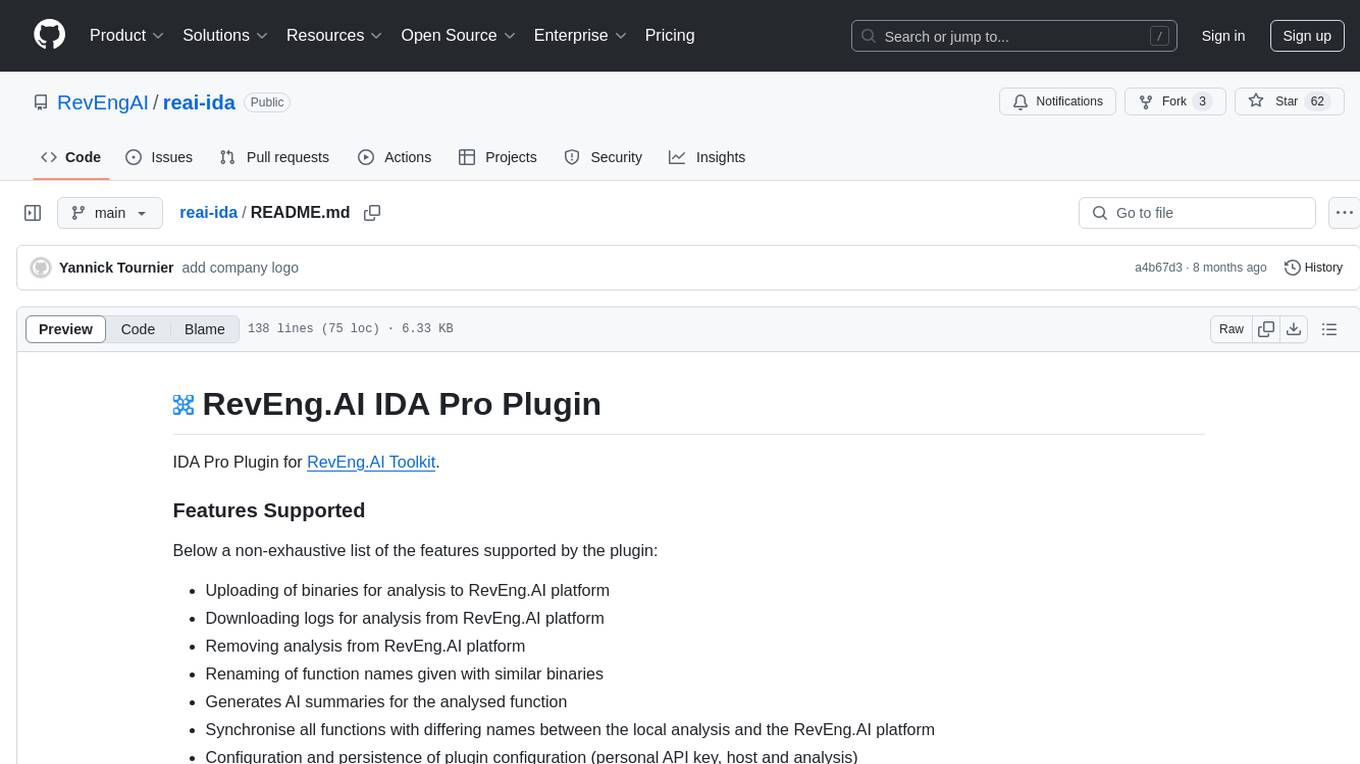
reai-ida
RevEng.AI IDA Pro Plugin is a tool that integrates with the RevEng.AI platform to provide various features such as uploading binaries for analysis, downloading analysis logs, renaming function names, generating AI summaries, synchronizing functions between local analysis and the platform, and configuring plugin settings. Users can upload files for analysis, synchronize function names, rename functions, generate block summaries, and explain function behavior using this plugin. The tool requires IDA Pro v8.0 or later with Python 3.9 and higher. It relies on the 'reait' package for functionality.
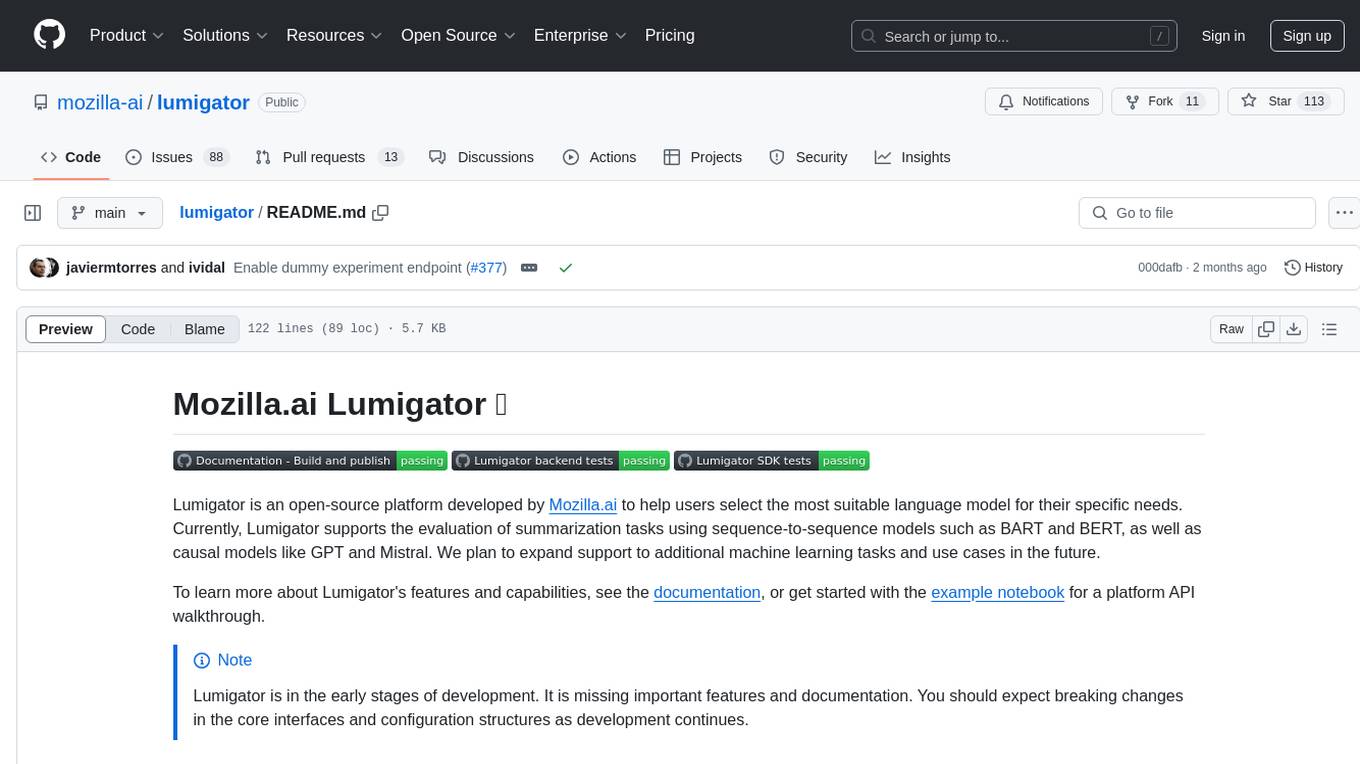
lumigator
Lumigator is an open-source platform developed by Mozilla.ai to help users select the most suitable language model for their specific needs. It supports the evaluation of summarization tasks using sequence-to-sequence models such as BART and BERT, as well as causal models like GPT and Mistral. The platform aims to make model selection transparent, efficient, and empowering by providing a framework for comparing LLMs using task-specific metrics to evaluate how well a model fits a project's needs. Lumigator is in the early stages of development and plans to expand support to additional machine learning tasks and use cases in the future.
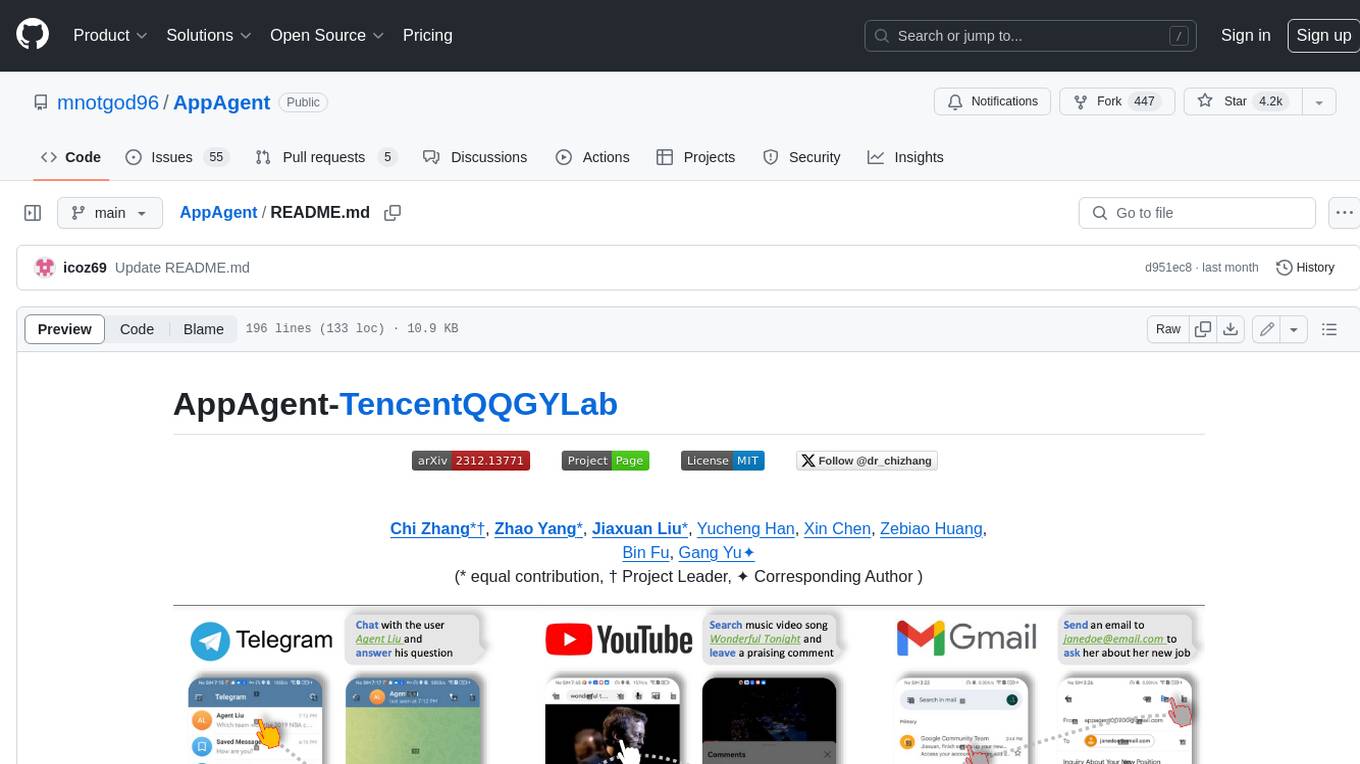
AppAgent
AppAgent is a novel LLM-based multimodal agent framework designed to operate smartphone applications. Our framework enables the agent to operate smartphone applications through a simplified action space, mimicking human-like interactions such as tapping and swiping. This novel approach bypasses the need for system back-end access, thereby broadening its applicability across diverse apps. Central to our agent's functionality is its innovative learning method. The agent learns to navigate and use new apps either through autonomous exploration or by observing human demonstrations. This process generates a knowledge base that the agent refers to for executing complex tasks across different applications.
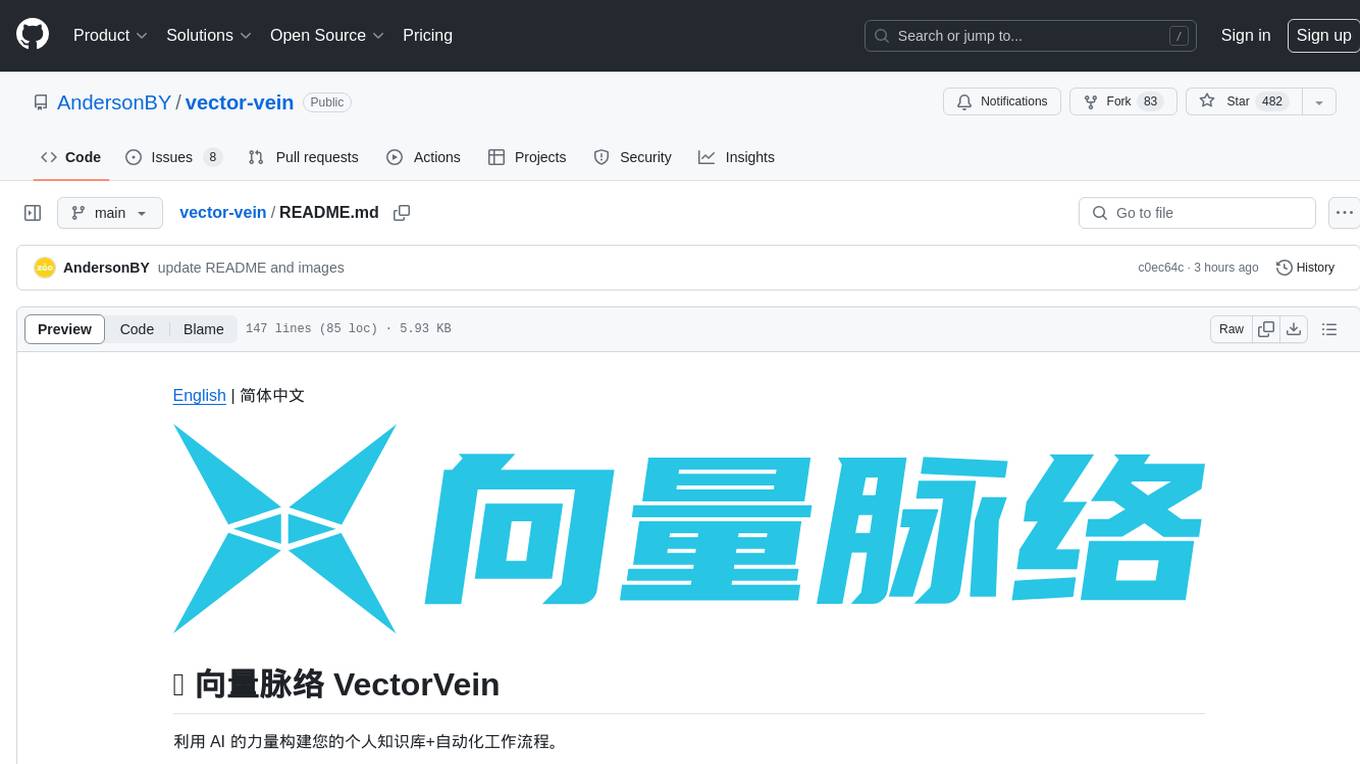
vector-vein
VectorVein is a no-code AI workflow software inspired by LangChain and langflow, aiming to combine the powerful capabilities of large language models and enable users to achieve intelligent and automated daily workflows through simple drag-and-drop actions. Users can create powerful workflows without the need for programming, automating all tasks with ease. The software allows users to define inputs, outputs, and processing methods to create customized workflow processes for various tasks such as translation, mind mapping, summarizing web articles, and automatic categorization of customer reviews.
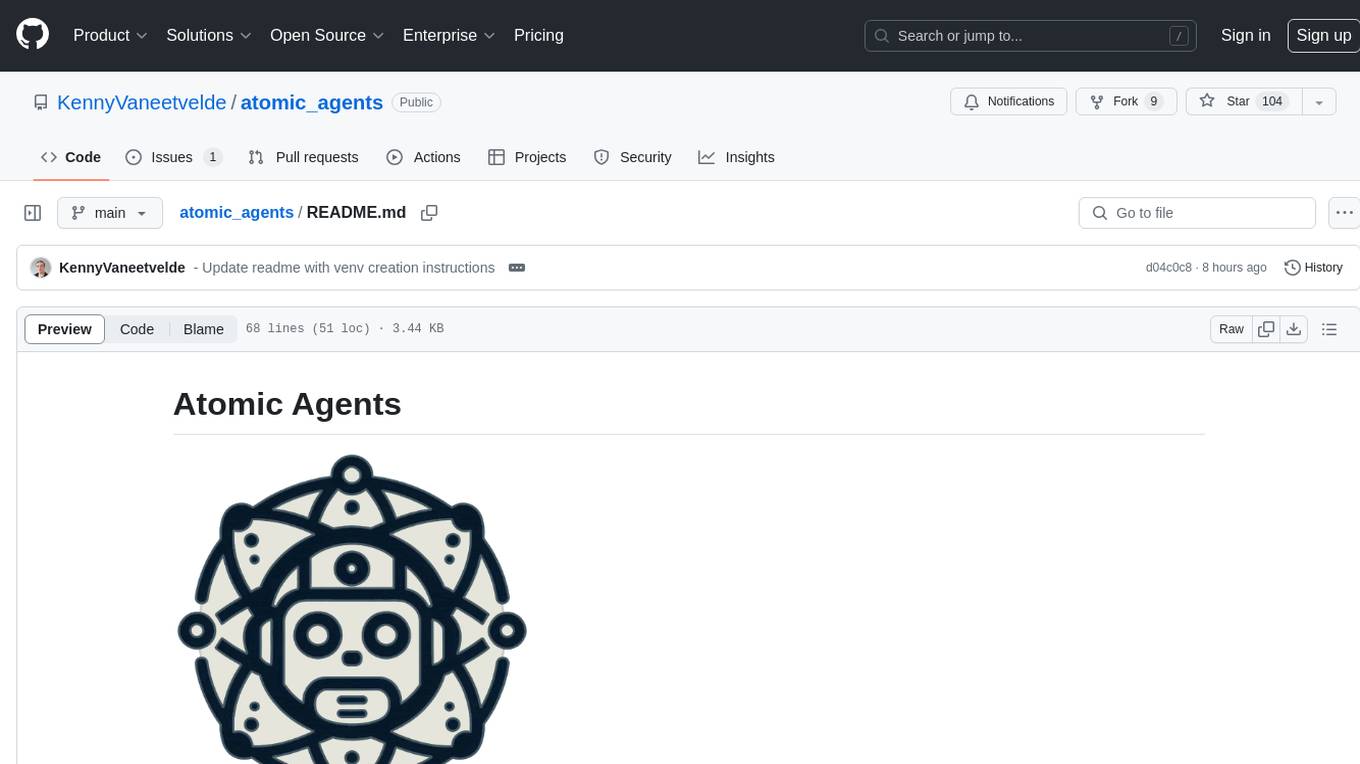
atomic_agents
Atomic Agents is a modular and extensible framework designed for creating powerful applications. It follows the principles of Atomic Design, emphasizing small and single-purpose components. Leveraging Pydantic for data validation and serialization, the framework offers a set of tools and agents that can be combined to build AI applications. It depends on the Instructor package and supports various APIs like OpenAI, Cohere, Anthropic, and Gemini. Atomic Agents is suitable for developers looking to create AI agents with a focus on modularity and flexibility.
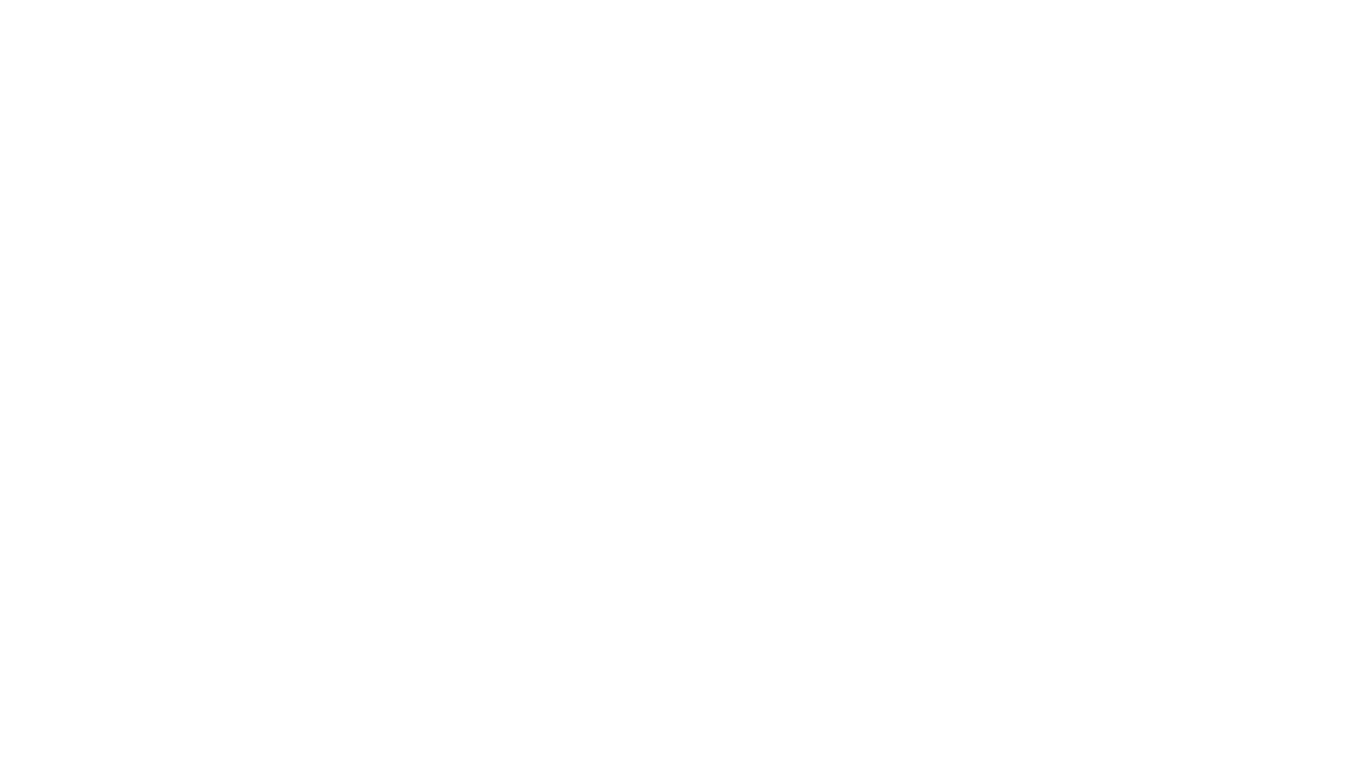
zep-python
Zep is an open-source platform for building and deploying large language model (LLM) applications. It provides a suite of tools and services that make it easy to integrate LLMs into your applications, including chat history memory, embedding, vector search, and data enrichment. Zep is designed to be scalable, reliable, and easy to use, making it a great choice for developers who want to build LLM-powered applications quickly and easily.
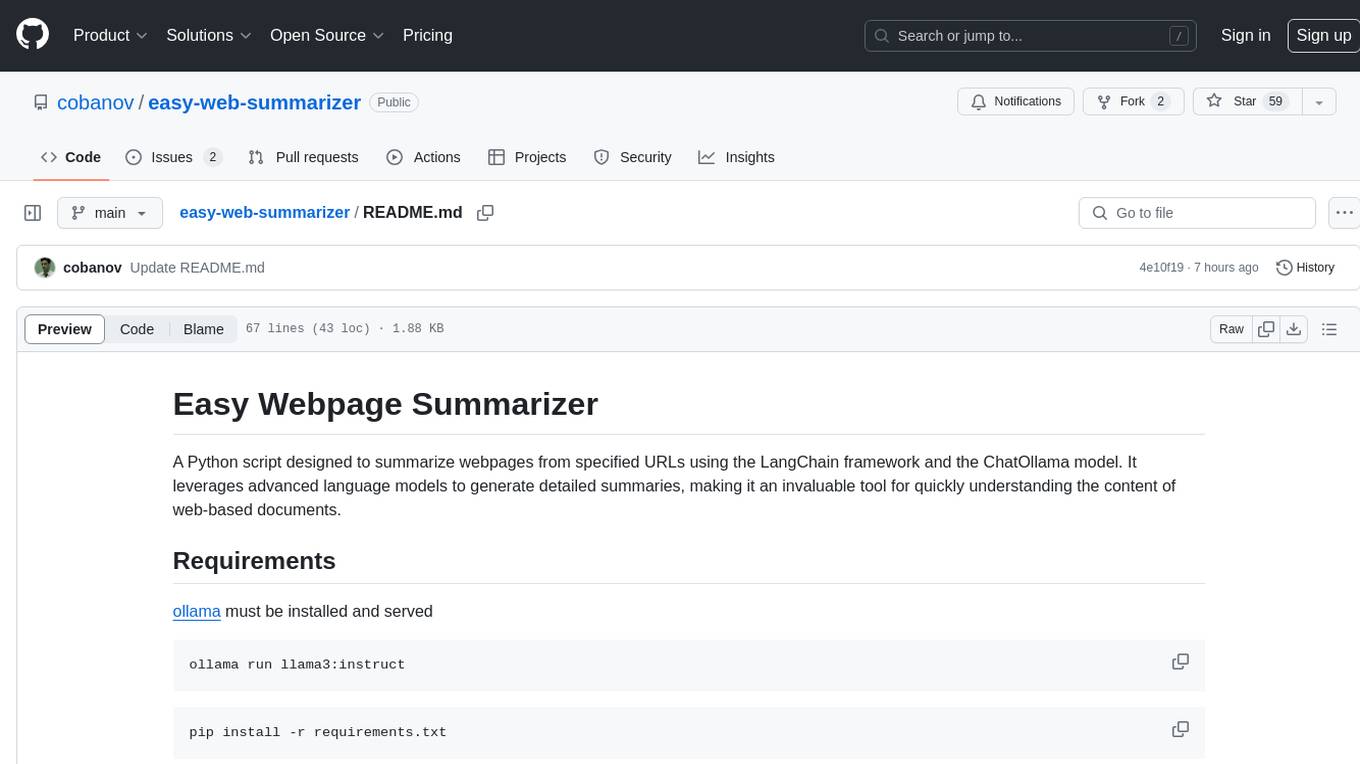
easy-web-summarizer
A Python script leveraging advanced language models to summarize webpages and youtube videos directly from URLs. It integrates with LangChain and ChatOllama for state-of-the-art summarization, providing detailed summaries for quick understanding of web-based documents. The tool offers a command-line interface for easy use and integration into workflows, with plans to add support for translating to different languages and streaming text output on gradio. It can also be used via a web UI using the gradio app. The script is dockerized for easy deployment and is open for contributions to enhance functionality and capabilities.
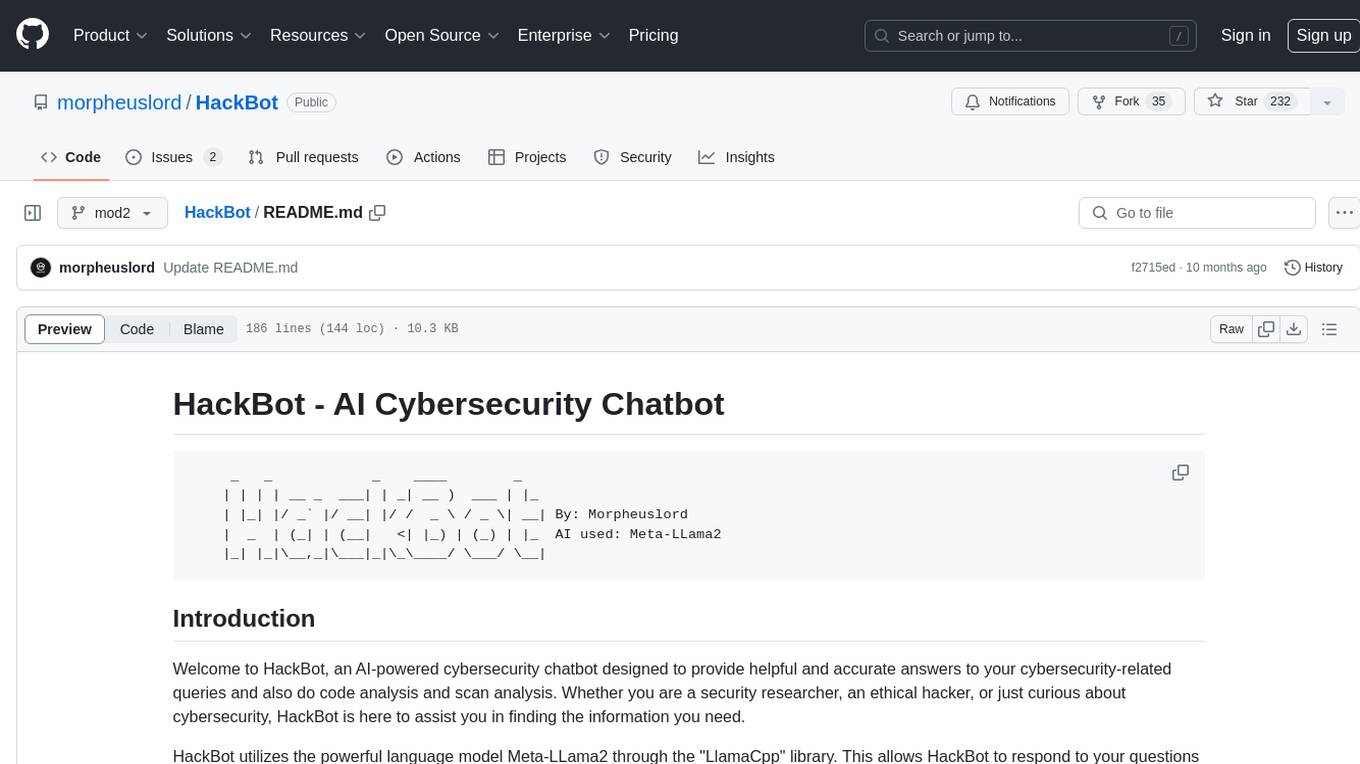
HackBot
HackBot is an AI-powered cybersecurity chatbot designed to provide accurate answers to cybersecurity-related queries, conduct code analysis, and scan analysis. It utilizes the Meta-LLama2 AI model through the 'LlamaCpp' library to respond coherently. The chatbot offers features like local AI/Runpod deployment support, cybersecurity chat assistance, interactive interface, clear output presentation, static code analysis, and vulnerability analysis. Users can interact with HackBot through a command-line interface and utilize it for various cybersecurity tasks.
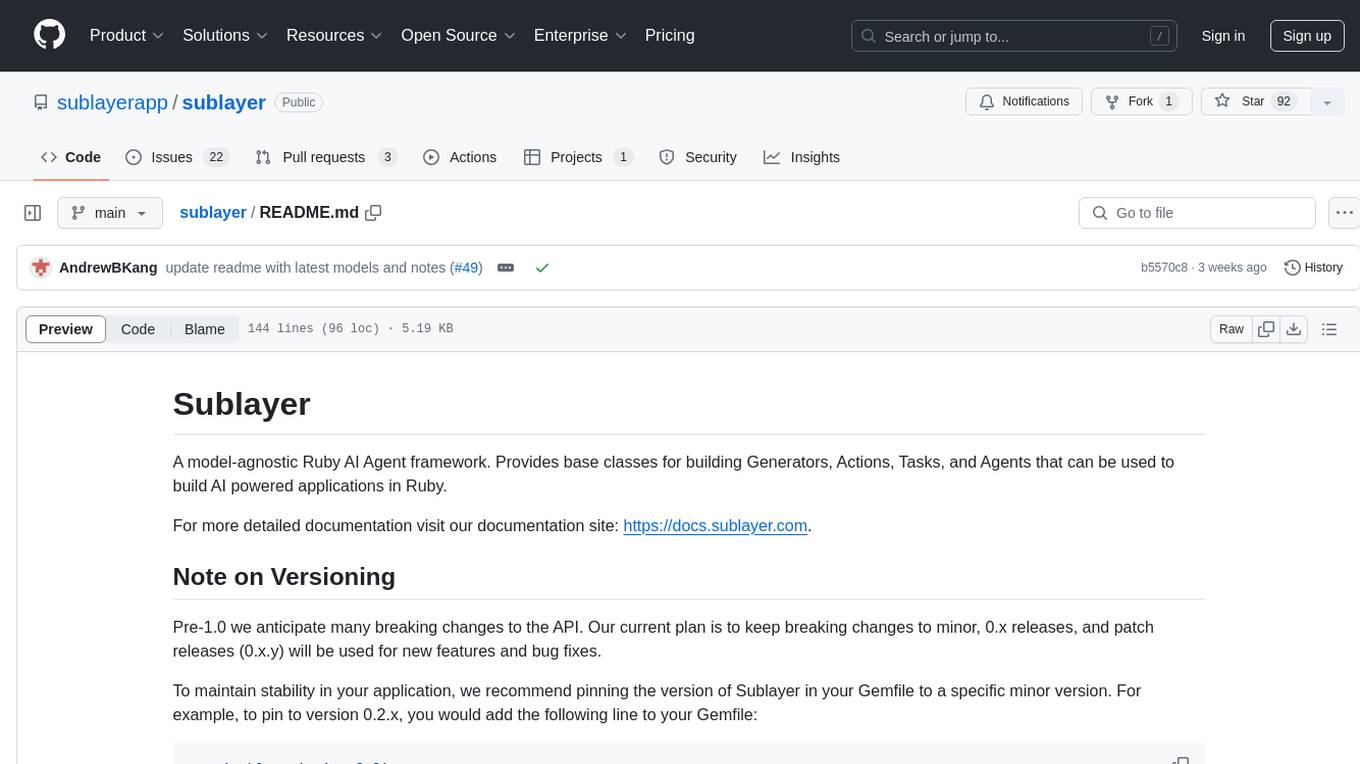
sublayer
Sublayer is a model-agnostic Ruby AI Agent framework that provides base classes for building Generators, Actions, Tasks, and Agents to create AI-powered applications in Ruby. It supports various AI models and providers, such as OpenAI, Gemini, and Claude. Generators generate specific outputs, Actions perform operations, Agents are autonomous entities for tasks or monitoring, and Triggers decide when Agents are activated. The framework offers sample Generators and usage examples for building AI applications.
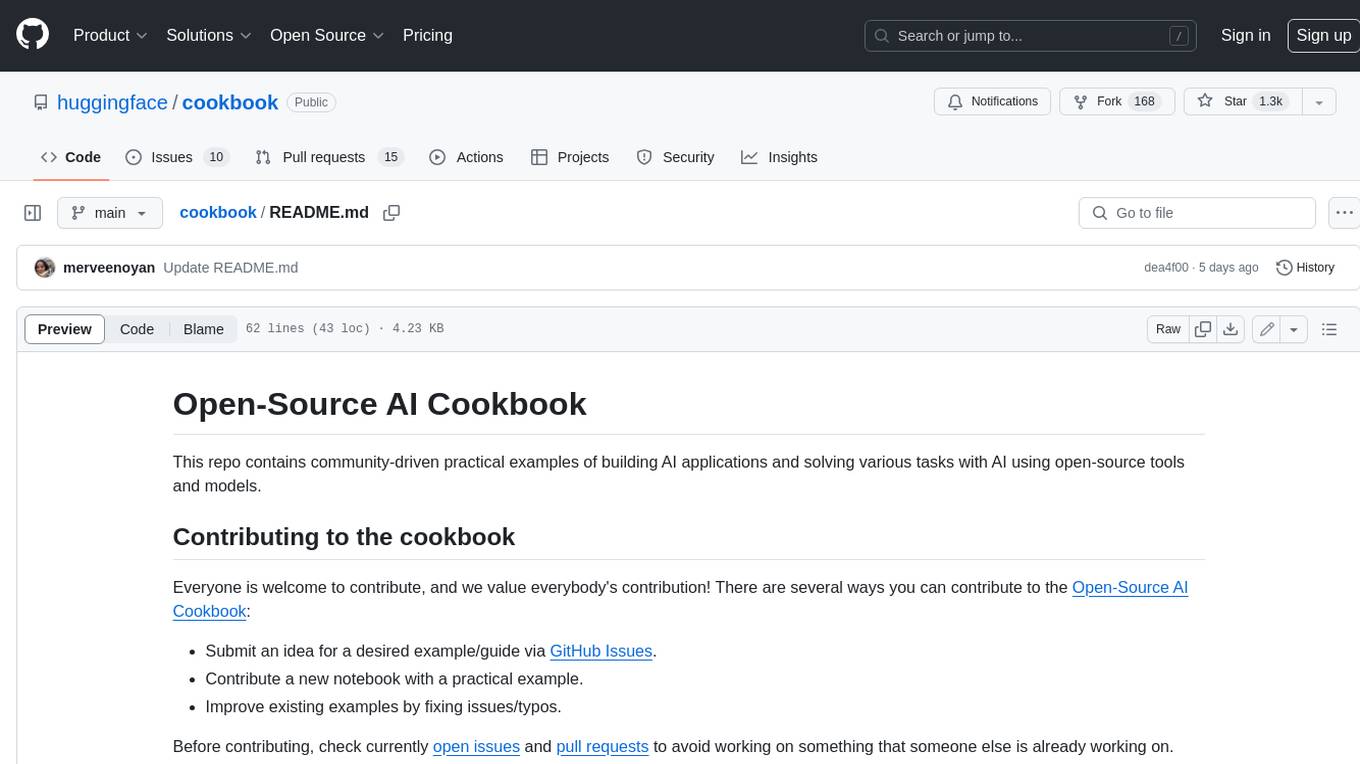
cookbook
This repository contains community-driven practical examples of building AI applications and solving various tasks with AI using open-source tools and models. Everyone is welcome to contribute, and we value everybody's contribution! There are several ways you can contribute to the Open-Source AI Cookbook: Submit an idea for a desired example/guide via GitHub Issues. Contribute a new notebook with a practical example. Improve existing examples by fixing issues/typos. Before contributing, check currently open issues and pull requests to avoid working on something that someone else is already working on.
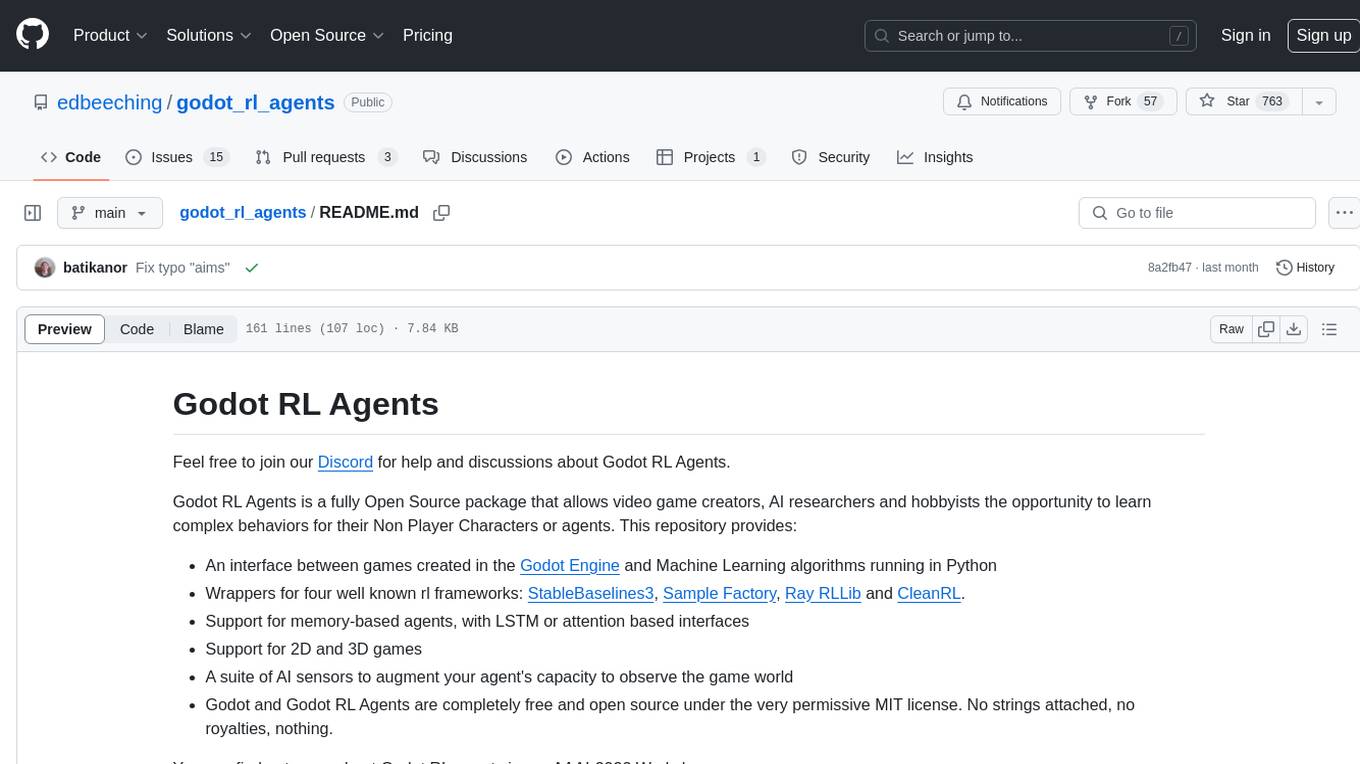
godot_rl_agents
Godot RL Agents is an open-source package that facilitates the integration of Machine Learning algorithms with games created in the Godot Engine. It provides interfaces for popular RL frameworks, support for memory-based agents, 2D and 3D games, AI sensors, and is licensed under MIT. Users can train agents in the Godot editor, create custom environments, export trained agents in ONNX format, and utilize advanced features like different RL training frameworks.
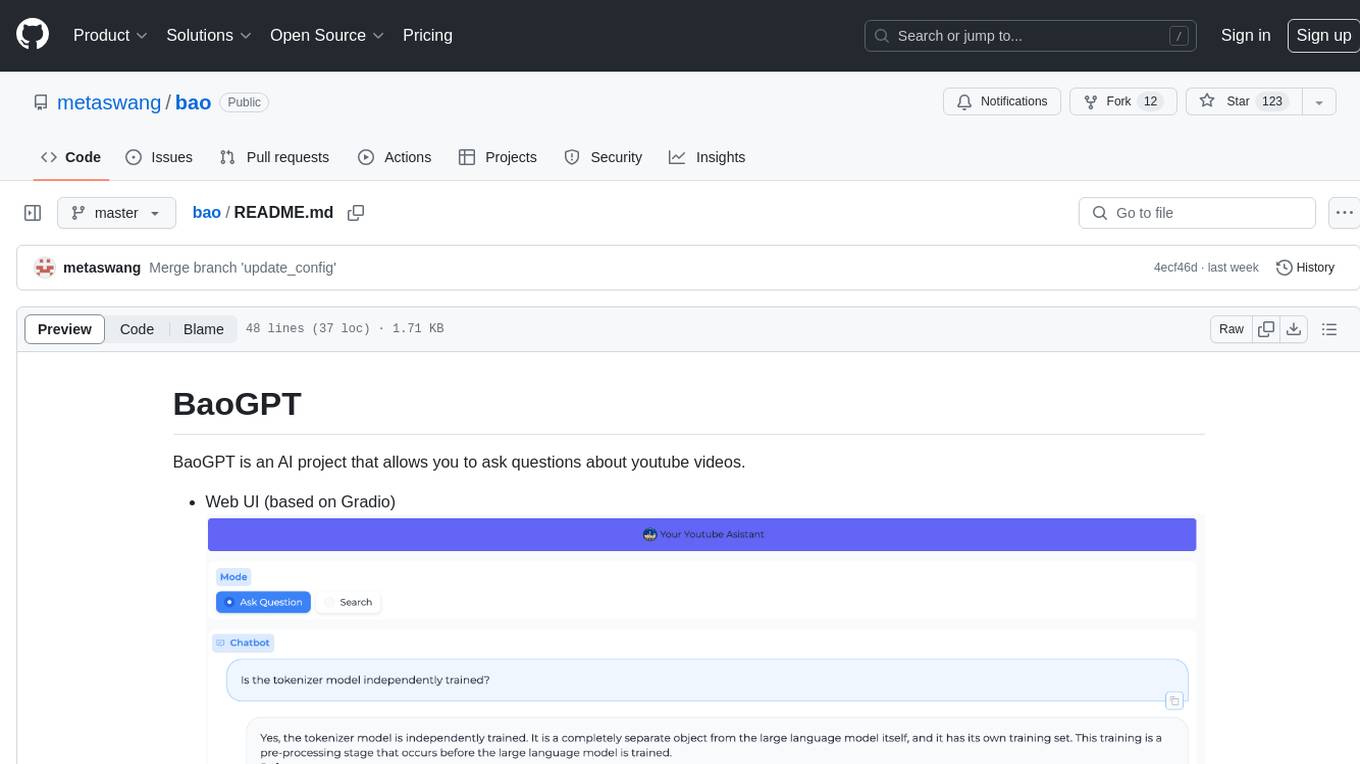
bao
BaoGPT is an AI project designed to facilitate asking questions about YouTube videos. It features a web UI based on Gradio and Discord integration. The tool utilizes a pipeline that routes input questions to either a greeting-like branch or a query & answer branch. The query analysis is performed by the LLM, which extracts attributes as filters and optimizes and rewrites questions for better vector retrieval in the vector DB. The tool then retrieves top-k candidates for grading and outputs final relative documents after grading. Lastly, the LLM performs summarization based on the reranking output, providing answers and attaching sources to the user.
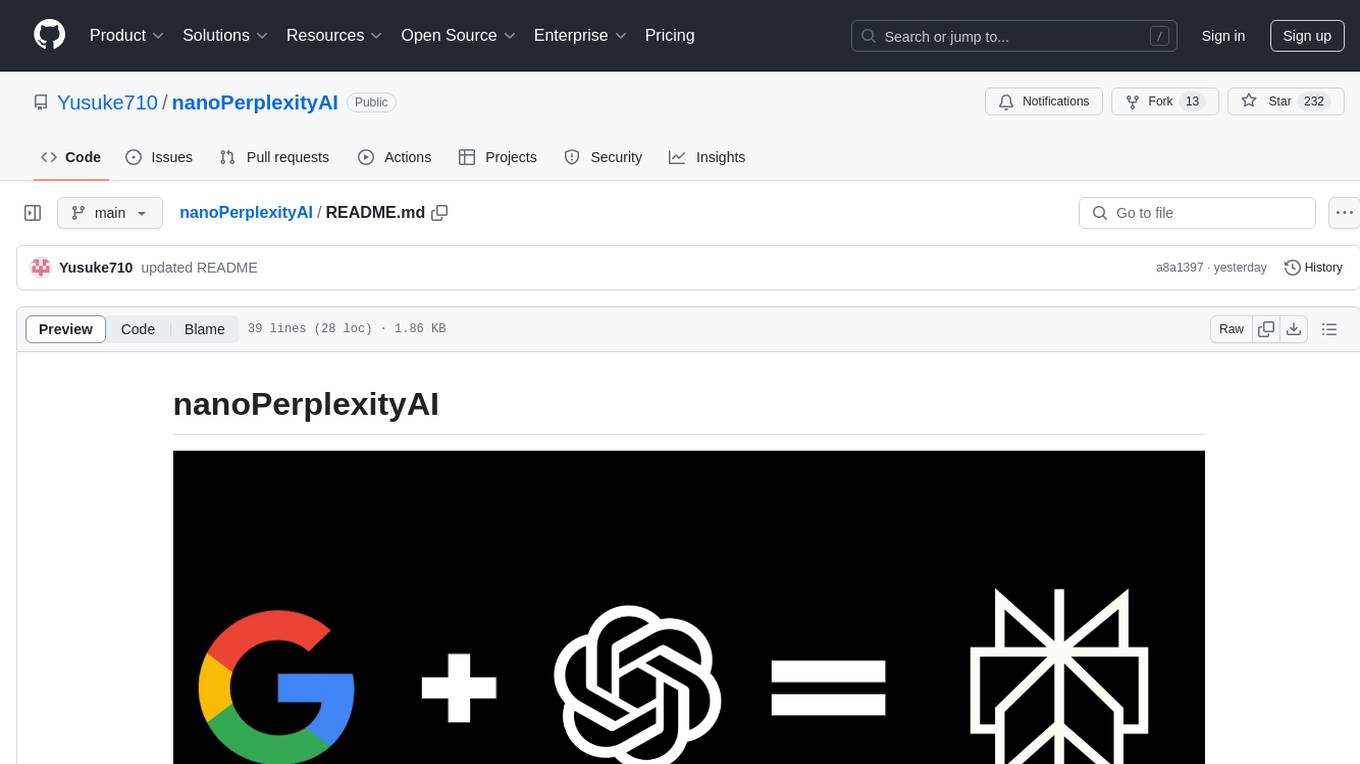
nanoPerplexityAI
nanoPerplexityAI is an open-source implementation of a large language model service that fetches information from Google. It involves a simple architecture where the user query is checked by the language model, reformulated for Google search, and an answer is generated and saved in a markdown file. The tool requires minimal setup and is designed for easy visualization of answers.
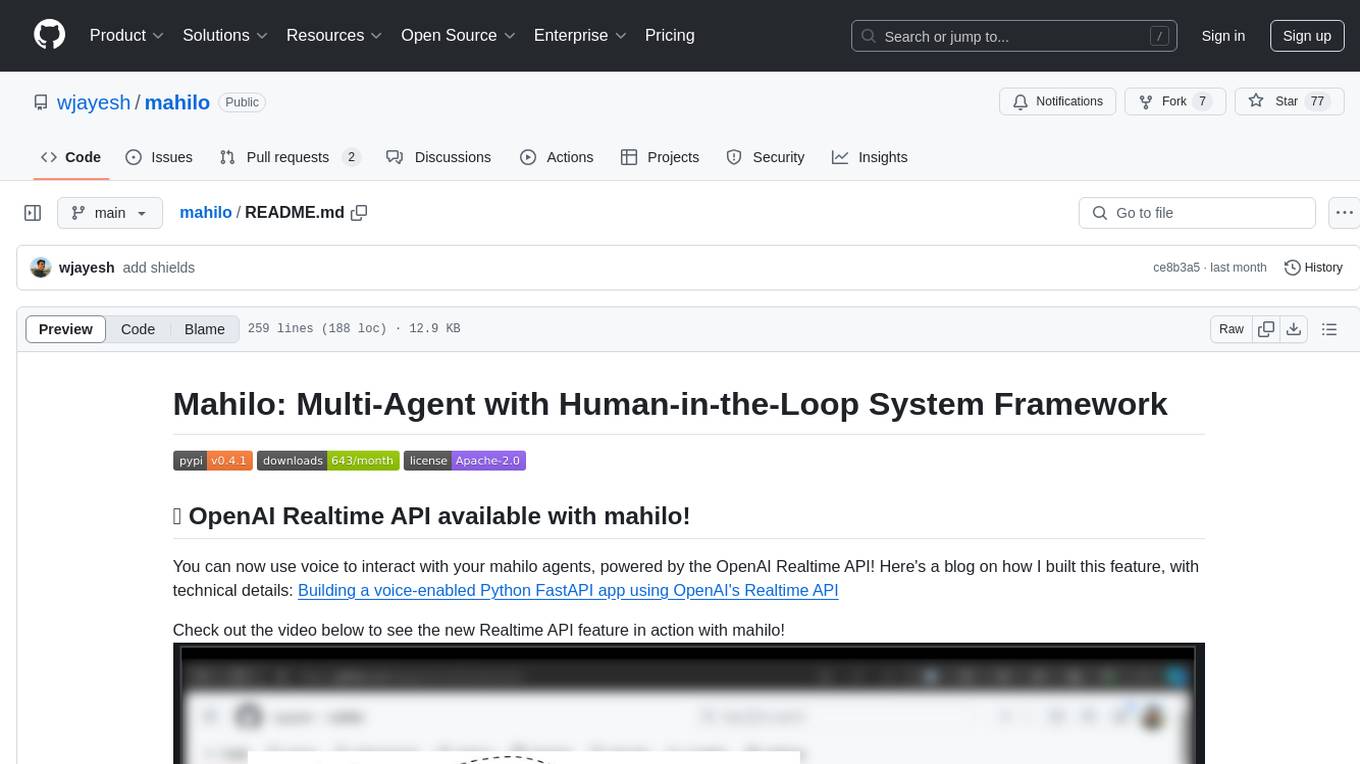
mahilo
Mahilo is a flexible framework for creating multi-agent systems that can interact with humans while sharing context internally. It allows developers to set up complex agent networks for various applications, from customer service to emergency response simulations. Agents can communicate with each other and with humans, making the system efficient by handling context from multiple agents and helping humans stay focused on specific problems. The system supports Realtime API for voice interactions, WebSocket-based communication, flexible communication patterns, session management, and easy agent definition.
For similar tasks
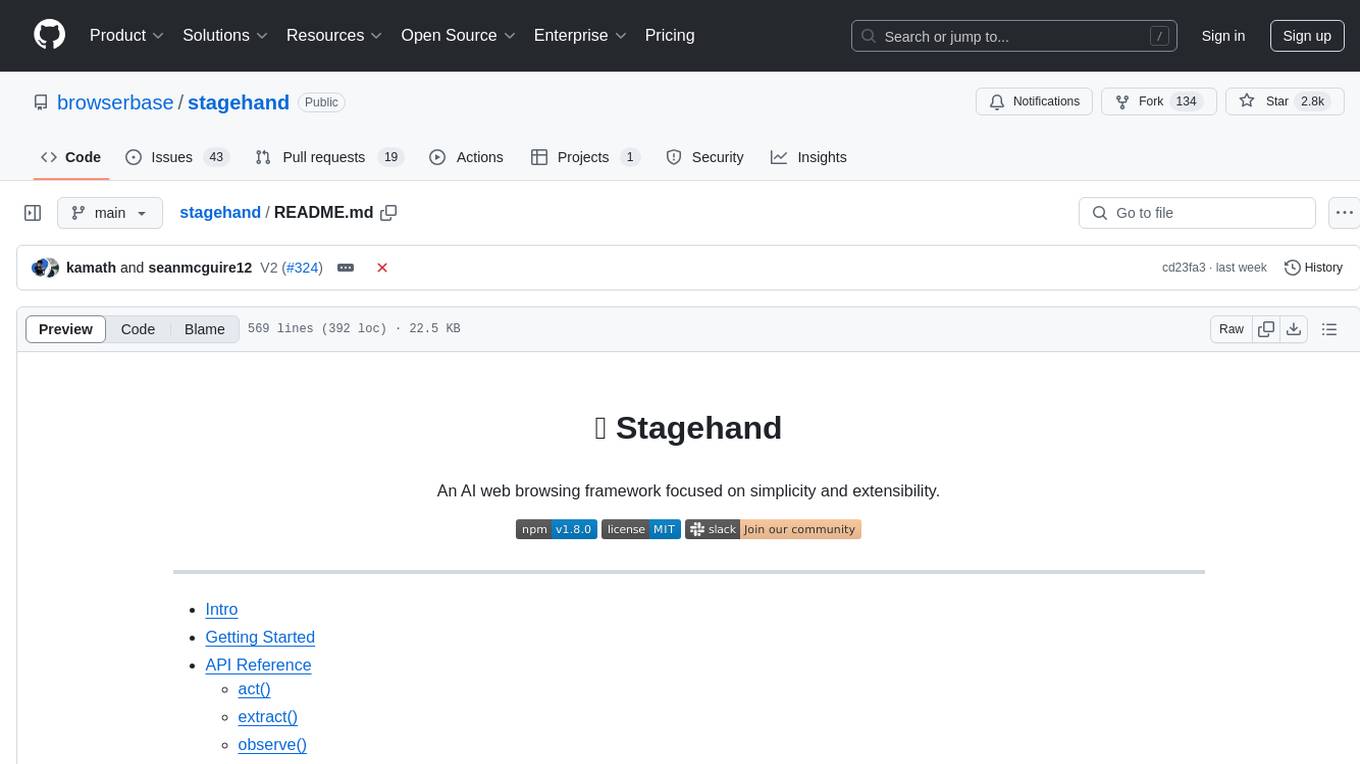
stagehand
Stagehand is an AI web browsing framework that simplifies and extends web automation using three simple APIs: act, extract, and observe. It aims to provide a lightweight, configurable framework without complex abstractions, allowing users to automate web tasks reliably. The tool generates Playwright code based on atomic instructions provided by the user, enabling natural language-driven web automation. Stagehand is open source, maintained by the Browserbase team, and supports different models and model providers for flexibility in automation tasks.
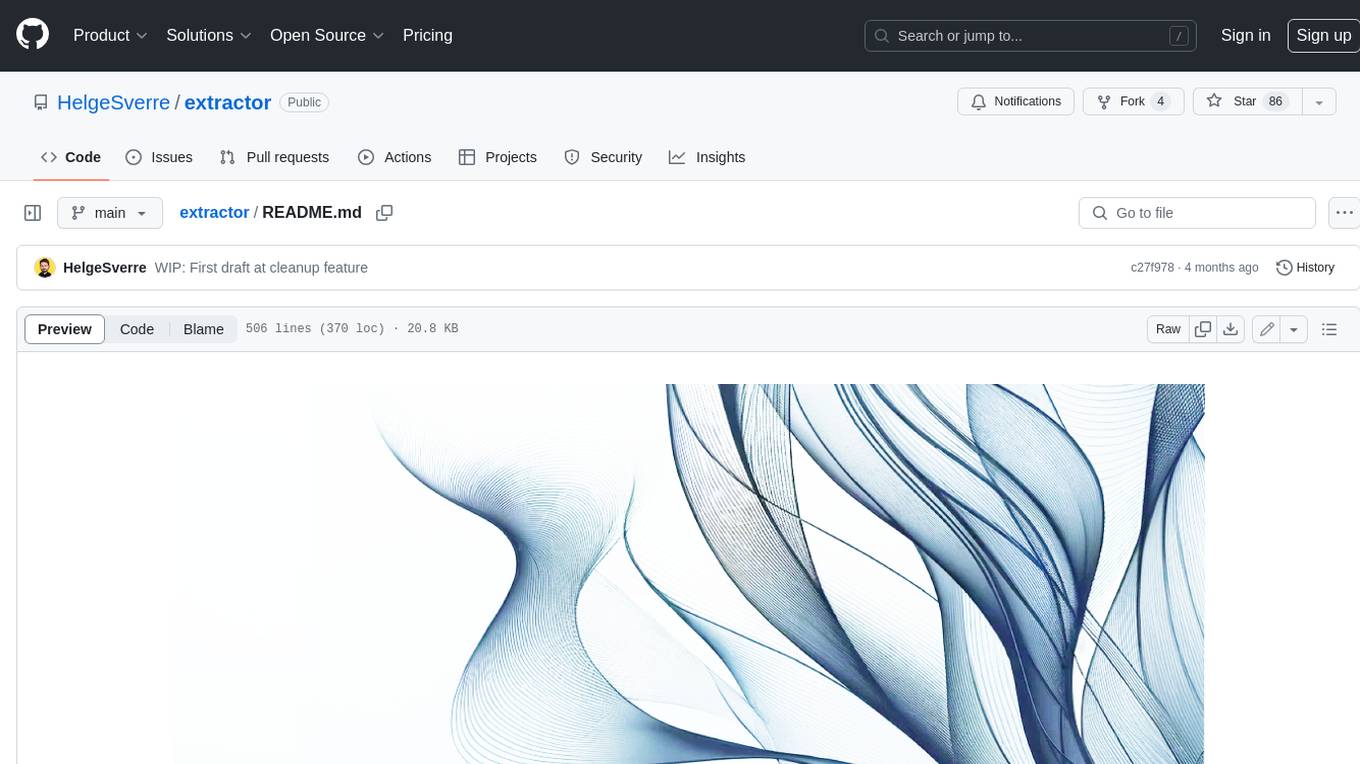
extractor
Extractor is an AI-powered data extraction library for Laravel that leverages OpenAI's capabilities to effortlessly extract structured data from various sources, including images, PDFs, and emails. It features a convenient wrapper around OpenAI Chat and Completion endpoints, supports multiple input formats, includes a flexible Field Extractor for arbitrary data extraction, and integrates with Textract for OCR functionality. Extractor utilizes JSON Mode from the latest GPT-3.5 and GPT-4 models, providing accurate and efficient data extraction.
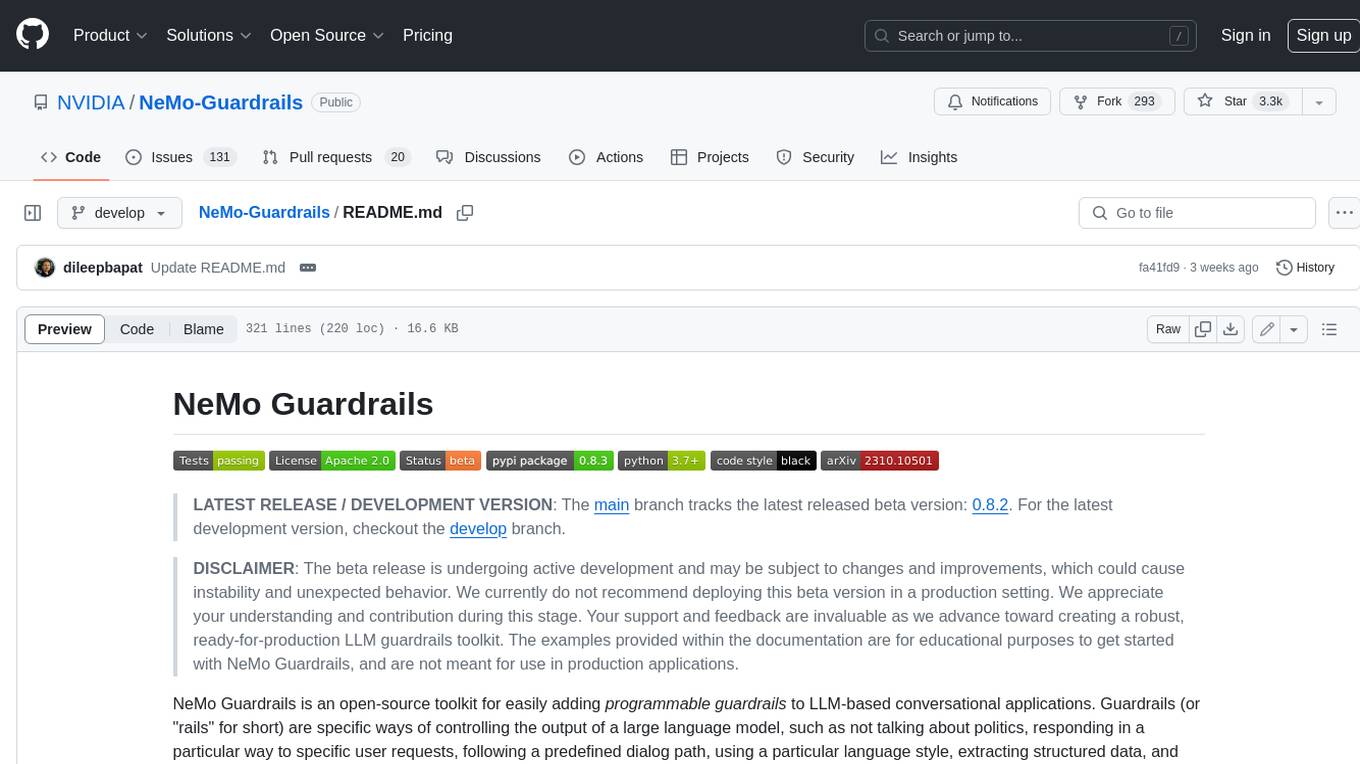
NeMo-Guardrails
NeMo Guardrails is an open-source toolkit for easily adding _programmable guardrails_ to LLM-based conversational applications. Guardrails (or "rails" for short) are specific ways of controlling the output of a large language model, such as not talking about politics, responding in a particular way to specific user requests, following a predefined dialog path, using a particular language style, extracting structured data, and more.
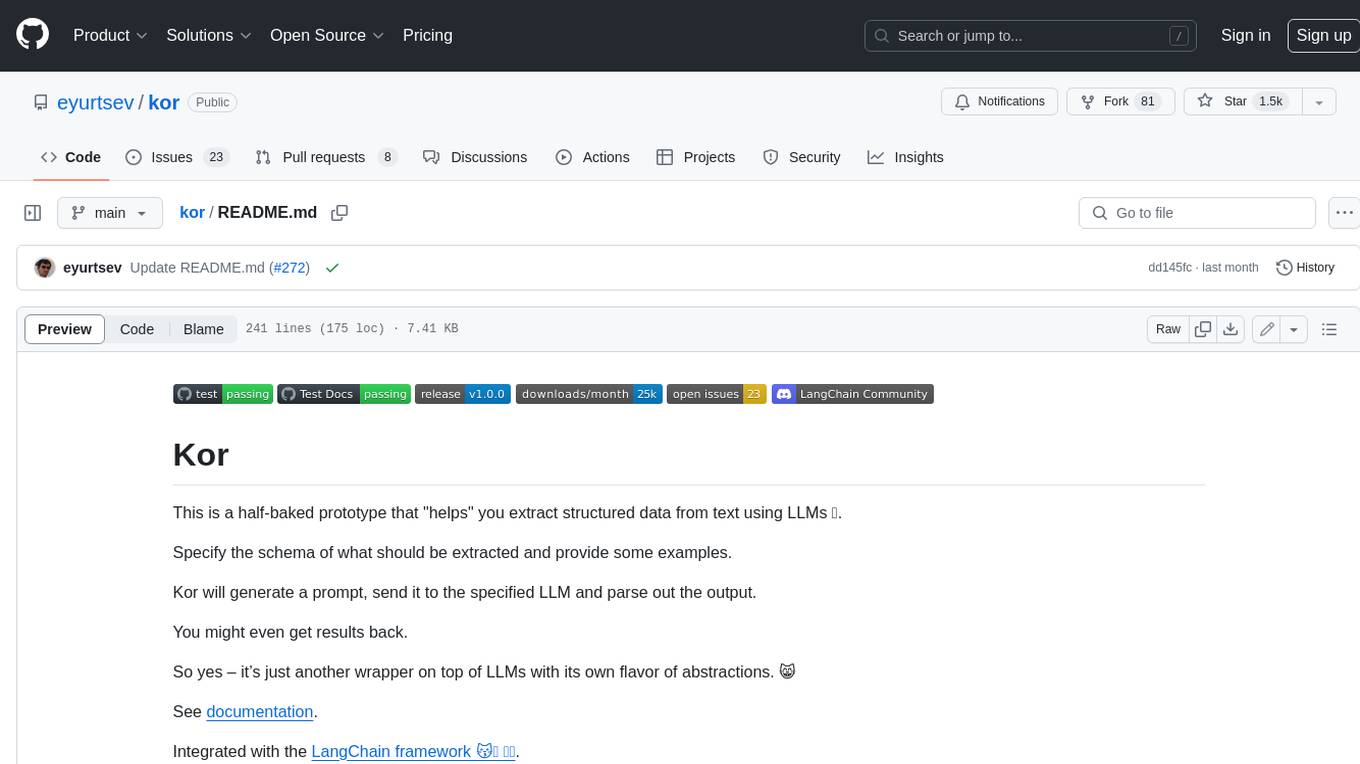
kor
Kor is a prototype tool designed to help users extract structured data from text using Language Models (LLMs). It generates prompts, sends them to specified LLMs, and parses the output. The tool works with the parsing approach and is integrated with the LangChain framework. Kor is compatible with pydantic v2 and v1, and schema is typed checked using pydantic. It is primarily used for extracting information from text based on provided reference examples and schema documentation. Kor is designed to work with all good-enough LLMs regardless of their support for function/tool calling or JSON modes.
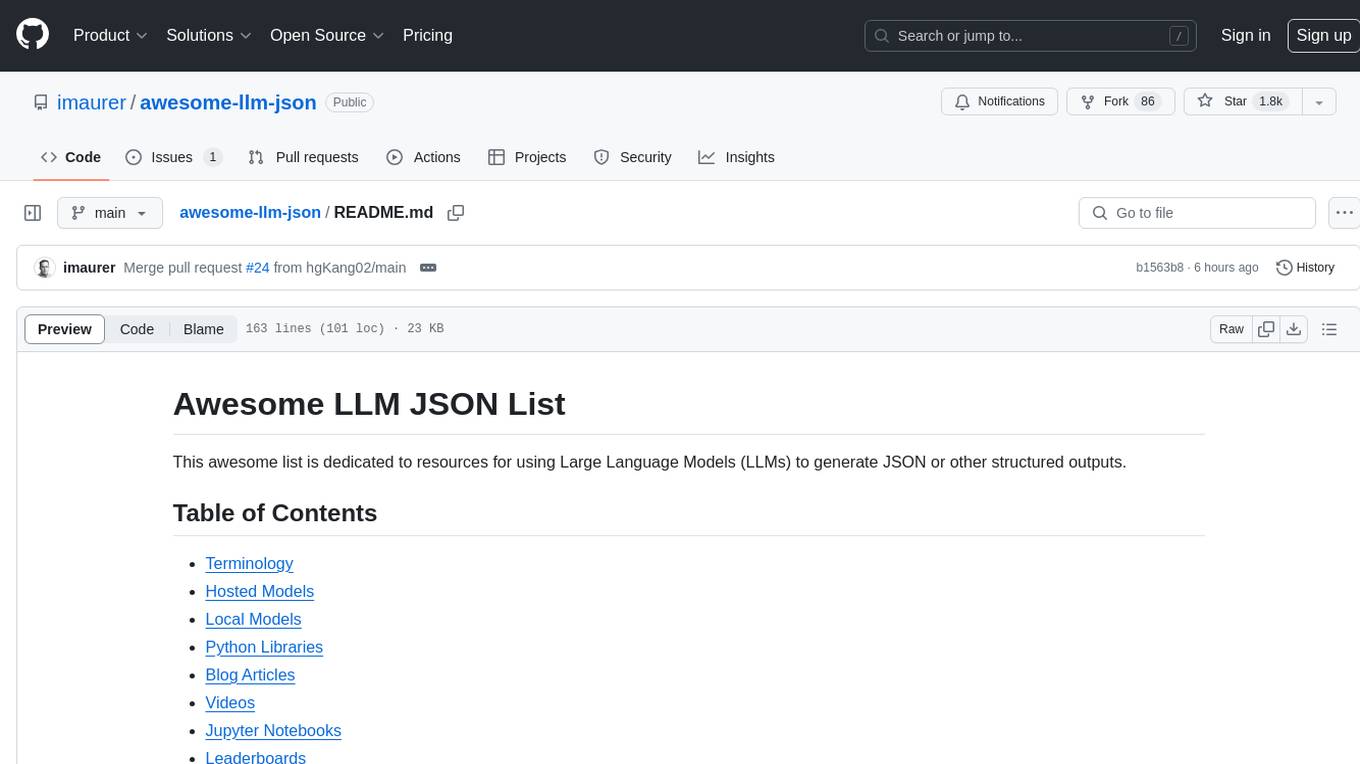
awesome-llm-json
This repository is an awesome list dedicated to resources for using Large Language Models (LLMs) to generate JSON or other structured outputs. It includes terminology explanations, hosted and local models, Python libraries, blog articles, videos, Jupyter notebooks, and leaderboards related to LLMs and JSON generation. The repository covers various aspects such as function calling, JSON mode, guided generation, and tool usage with different providers and models.
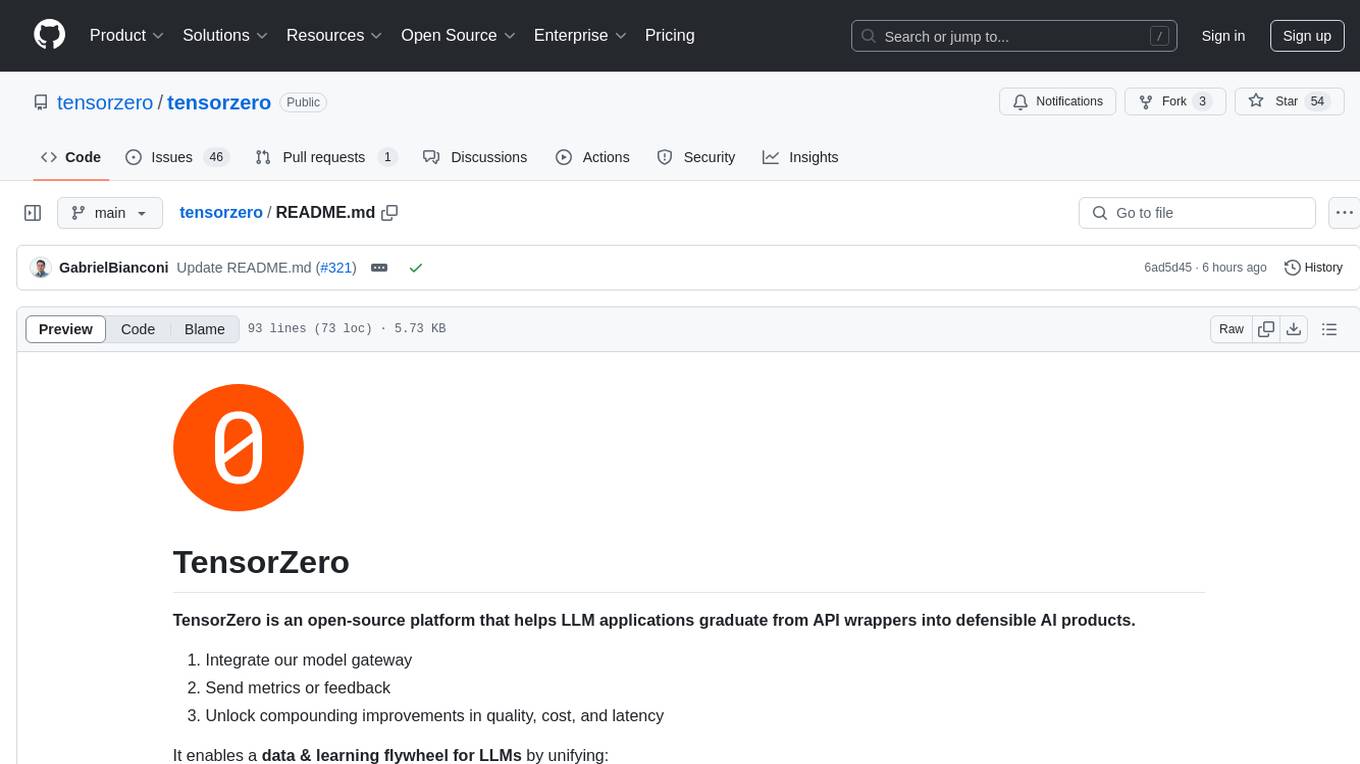
tensorzero
TensorZero is an open-source platform that helps LLM applications graduate from API wrappers into defensible AI products. It enables a data & learning flywheel for LLMs by unifying inference, observability, optimization, and experimentation. The platform includes a high-performance model gateway, structured schema-based inference, observability, experimentation, and data warehouse for analytics. TensorZero Recipes optimize prompts and models, and the platform supports experimentation features and GitOps orchestration for deployment.
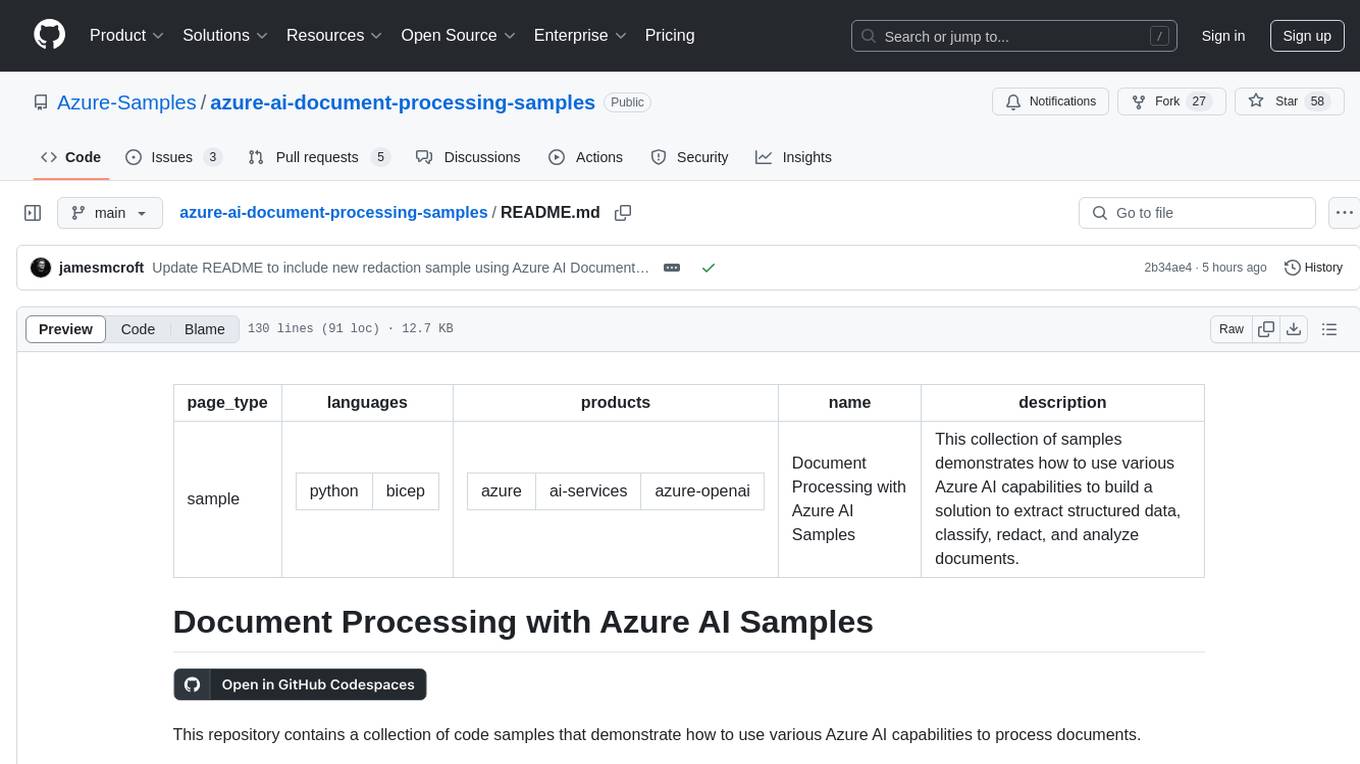
azure-ai-document-processing-samples
This repository contains a collection of code samples that demonstrate how to use various Azure AI capabilities to process documents. The samples help engineering teams establish techniques with Azure AI Foundry, Azure OpenAI, Azure AI Document Intelligence, and Azure AI Language services to build solutions for extracting structured data, classifying, and analyzing documents. The techniques simplify custom model training, improve reliability in document processing, and simplify document processing workflows by providing reusable code and patterns that can be easily modified and evaluated for most use cases.
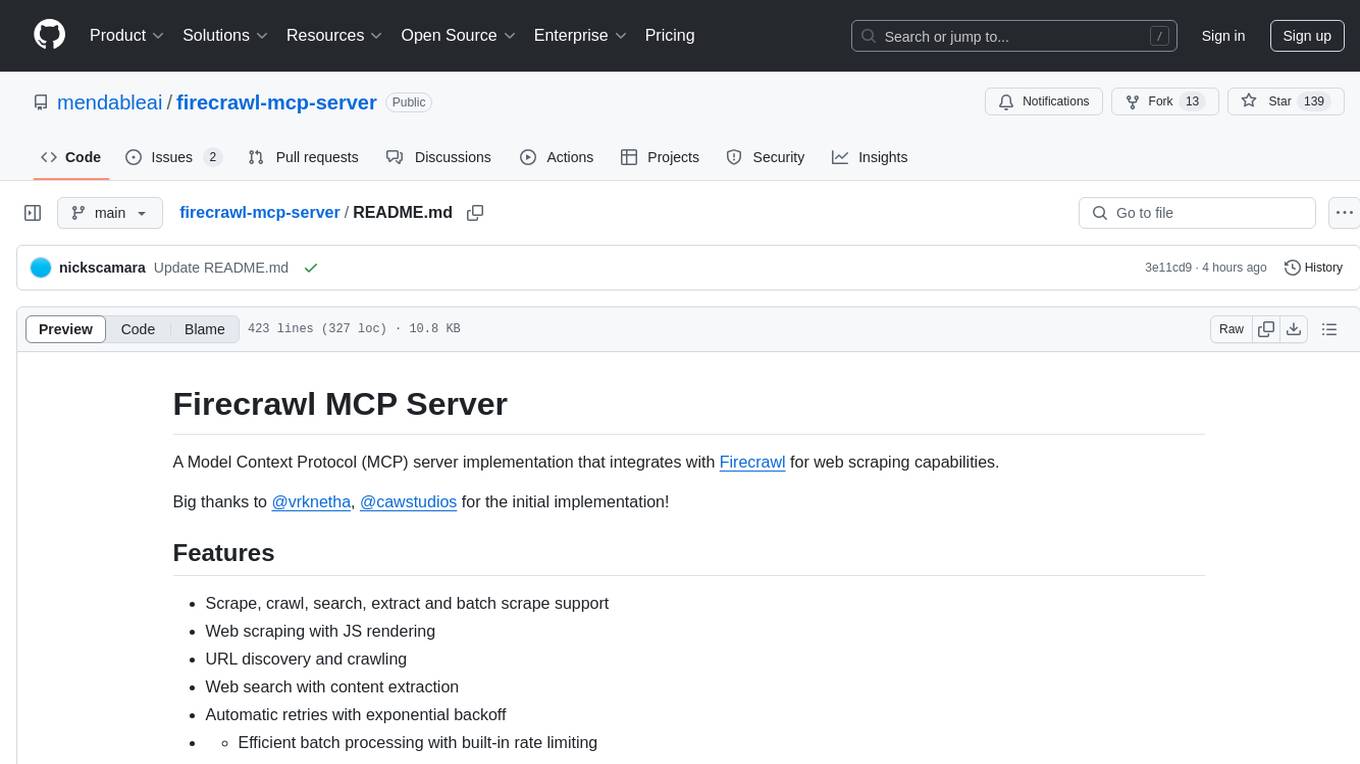
firecrawl-mcp-server
Firecrawl MCP Server is a Model Context Protocol (MCP) server implementation that integrates with Firecrawl for web scraping capabilities. It supports features like scrape, crawl, search, extract, and batch scrape. It provides web scraping with JS rendering, URL discovery, web search with content extraction, automatic retries with exponential backoff, credit usage monitoring, comprehensive logging system, support for cloud and self-hosted FireCrawl instances, mobile/desktop viewport support, and smart content filtering with tag inclusion/exclusion. The server includes configurable parameters for retry behavior and credit usage monitoring, rate limiting and batch processing capabilities, and tools for scraping, batch scraping, checking batch status, searching, crawling, and extracting structured information from web pages.
For similar jobs
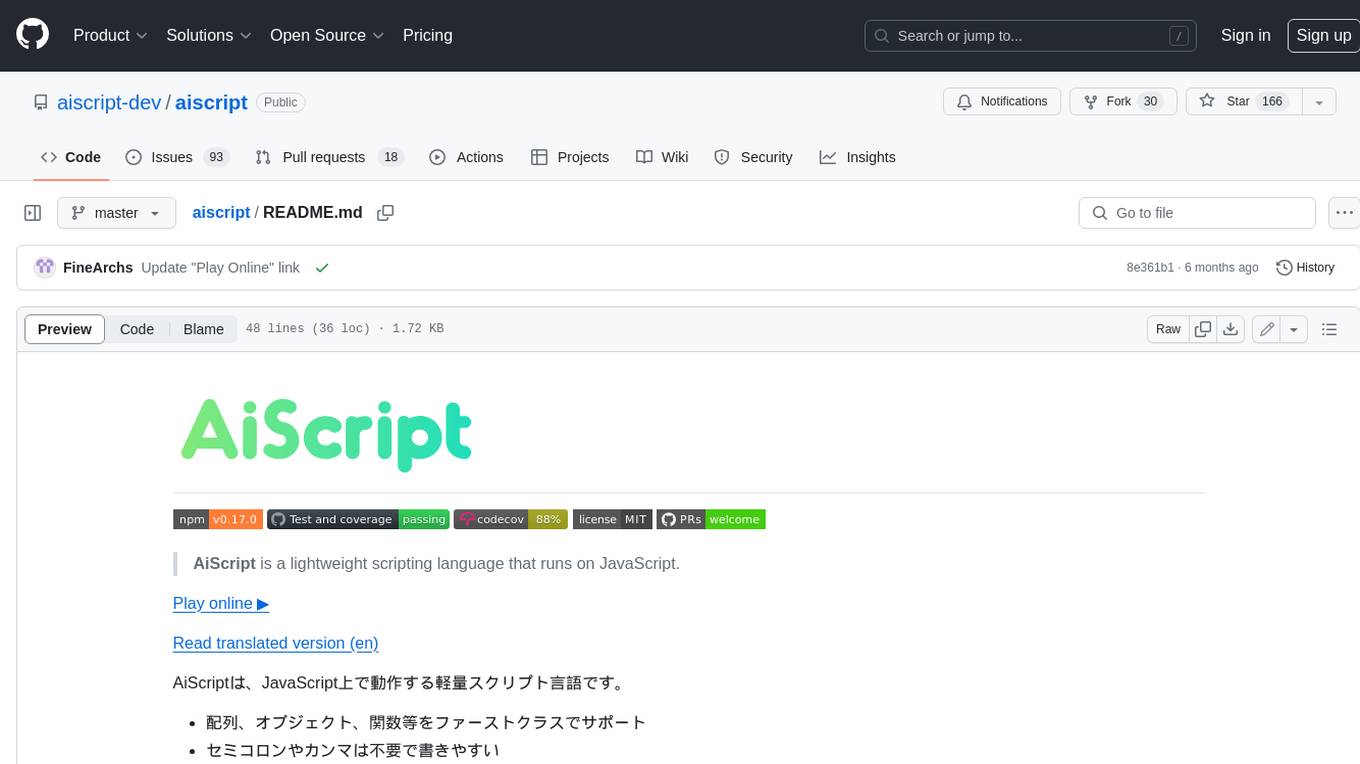
aiscript
AiScript is a lightweight scripting language that runs on JavaScript. It supports arrays, objects, and functions as first-class citizens, and is easy to write without the need for semicolons or commas. AiScript runs in a secure sandbox environment, preventing infinite loops from freezing the host. It also allows for easy provision of variables and functions from the host.
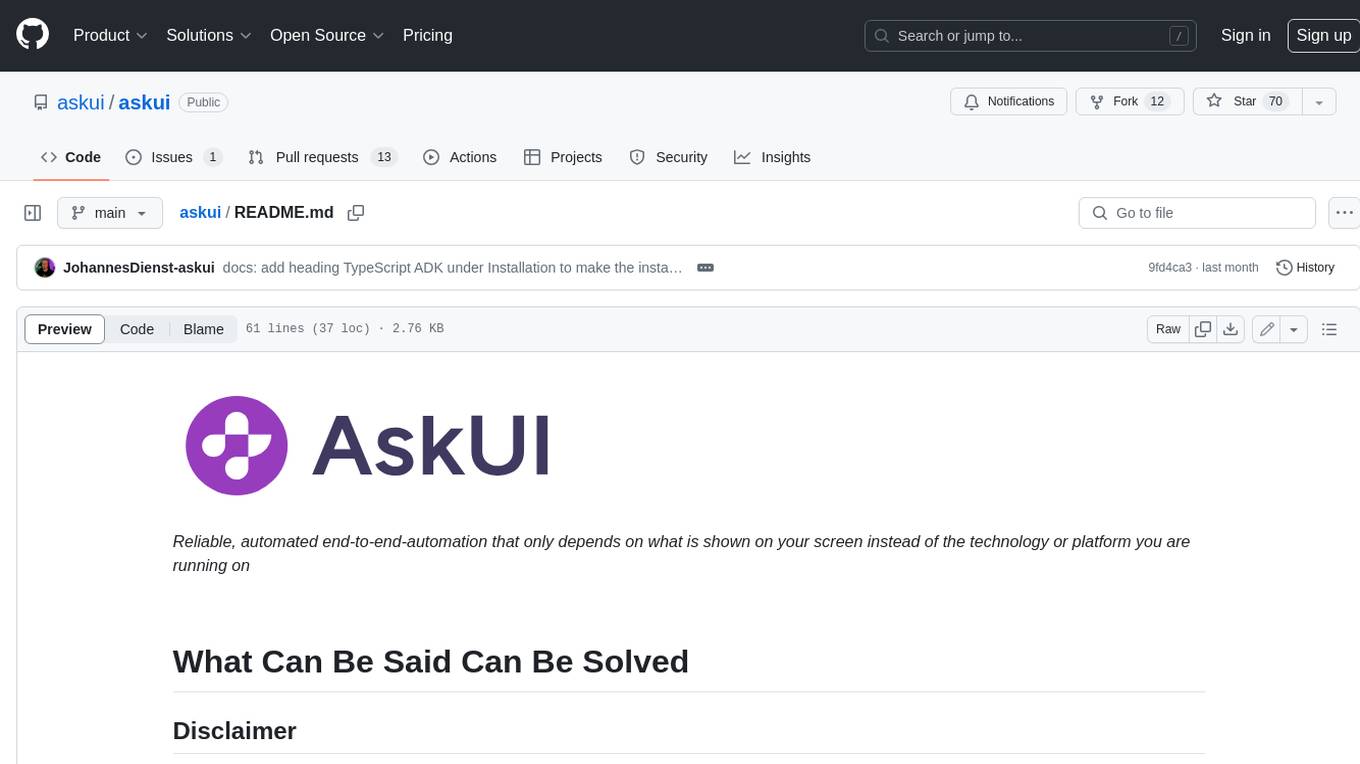
askui
AskUI is a reliable, automated end-to-end automation tool that only depends on what is shown on your screen instead of the technology or platform you are running on.
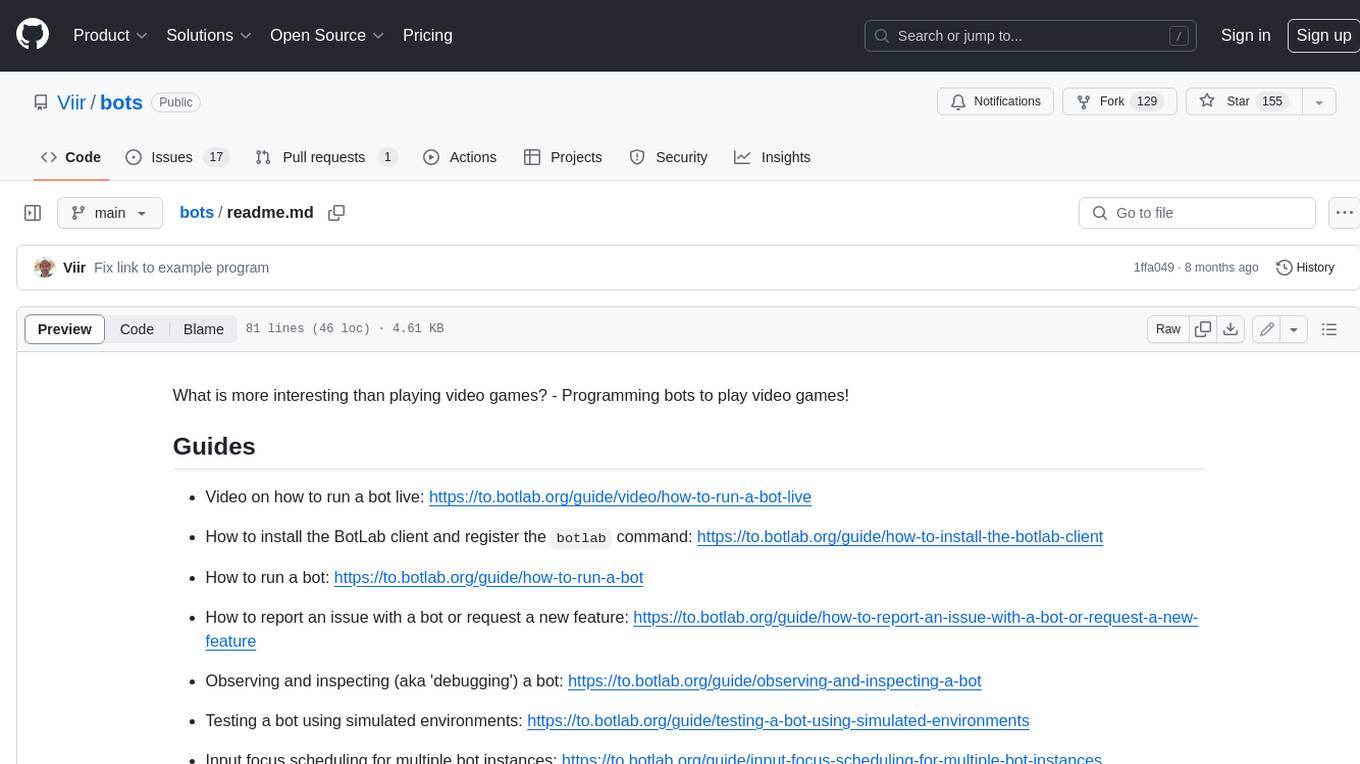
bots
The 'bots' repository is a collection of guides, tools, and example bots for programming bots to play video games. It provides resources on running bots live, installing the BotLab client, debugging bots, testing bots in simulated environments, and more. The repository also includes example bots for games like EVE Online, Tribal Wars 2, and Elvenar. Users can learn about developing bots for specific games, syntax of the Elm programming language, and tools for memory reading development. Additionally, there are guides on bot programming, contributing to BotLab, and exploring Elm syntax and core library.
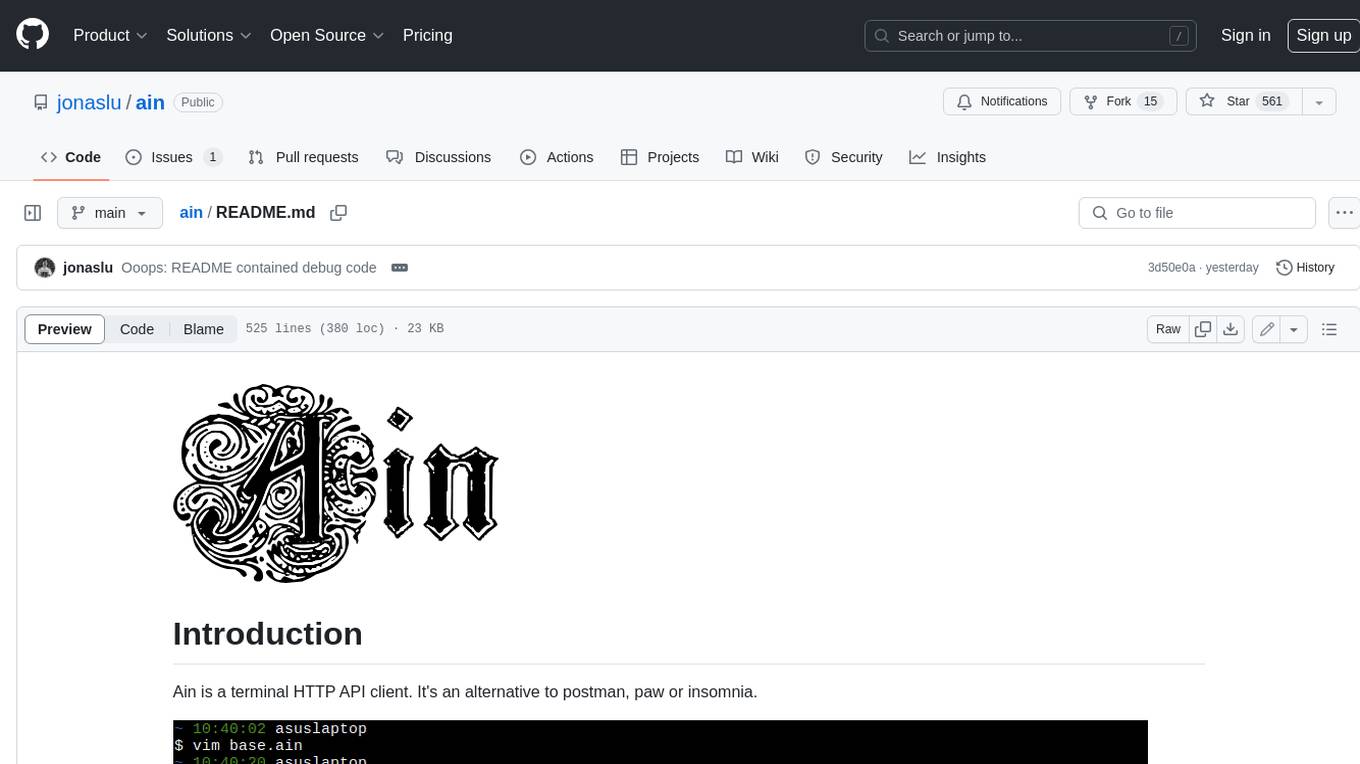
ain
Ain is a terminal HTTP API client designed for scripting input and processing output via pipes. It allows flexible organization of APIs using files and folders, supports shell-scripts and executables for common tasks, handles url-encoding, and enables sharing the resulting curl, wget, or httpie command-line. Users can put things that change in environment variables or .env-files, and pipe the API output for further processing. Ain targets users who work with many APIs using a simple file format and uses curl, wget, or httpie to make the actual calls.
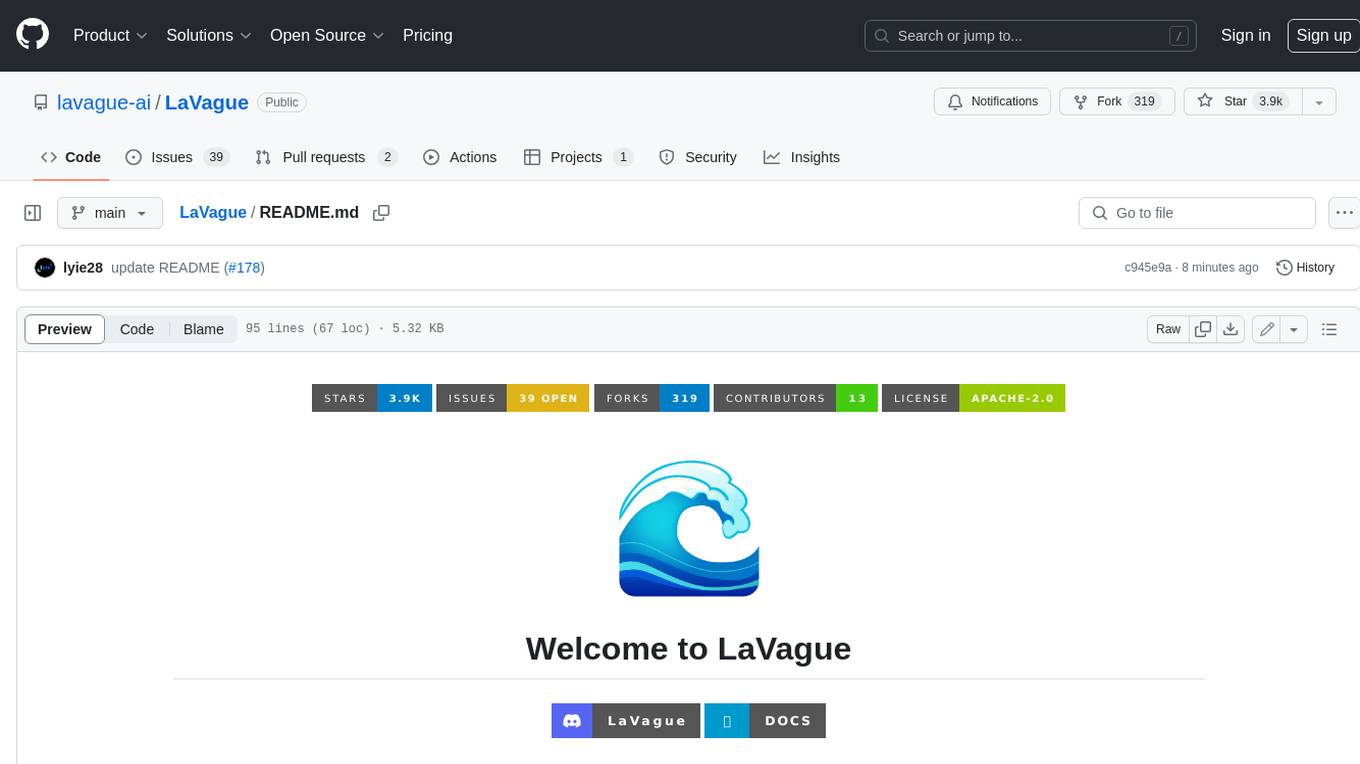
LaVague
LaVague is an open-source Large Action Model framework that uses advanced AI techniques to compile natural language instructions into browser automation code. It leverages Selenium or Playwright for browser actions. Users can interact with LaVague through an interactive Gradio interface to automate web interactions. The tool requires an OpenAI API key for default examples and offers a Playwright integration guide. Contributors can help by working on outlined tasks, submitting PRs, and engaging with the community on Discord. The project roadmap is available to track progress, but users should exercise caution when executing LLM-generated code using 'exec'.
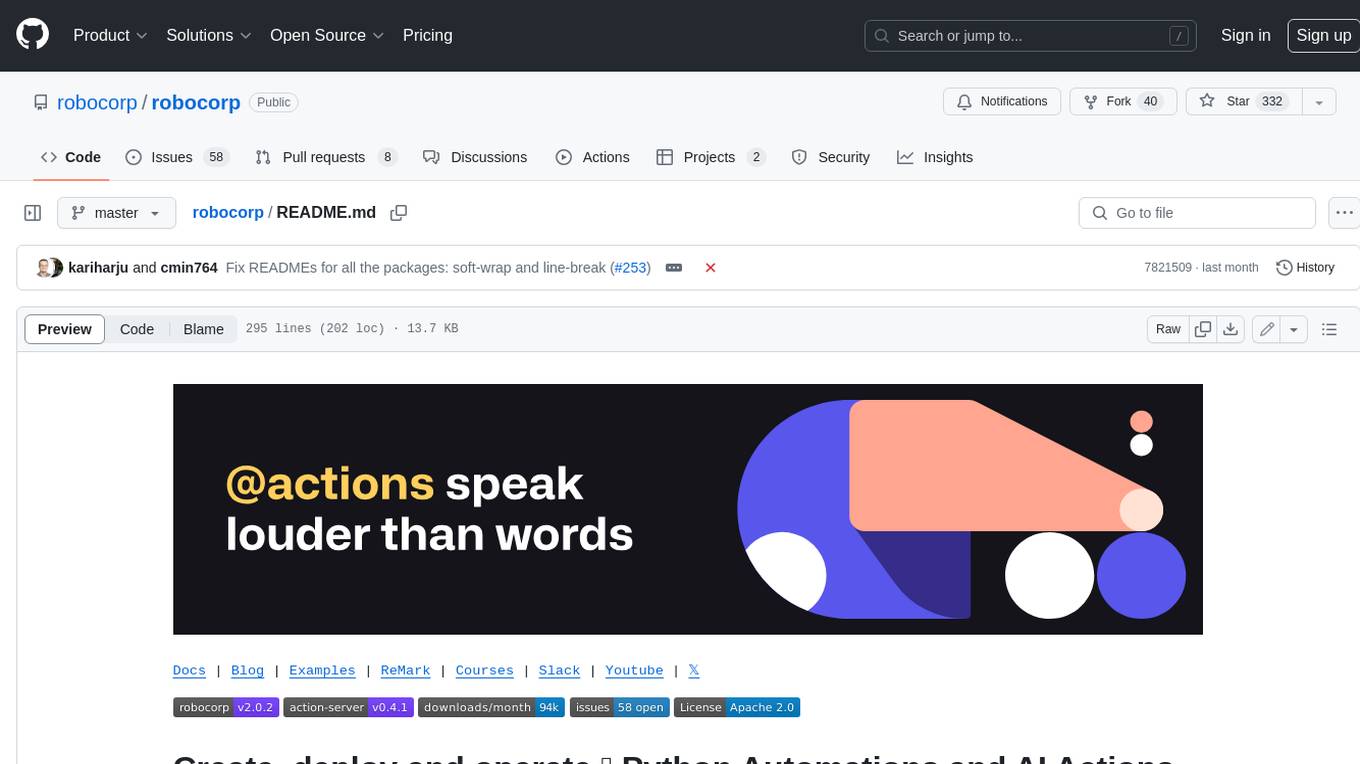
robocorp
Robocorp is a platform that allows users to create, deploy, and operate Python automations and AI actions. It provides an easy way to extend the capabilities of AI agents, assistants, and copilots with custom actions written in Python. Users can create and deploy tools, skills, loaders, and plugins that securely connect any AI Assistant platform to their data and applications. The Robocorp Action Server makes Python scripts compatible with ChatGPT and LangChain by automatically creating and exposing an API based on function declaration, type hints, and docstrings. It simplifies the process of developing and deploying AI actions, enabling users to interact with AI frameworks effortlessly.
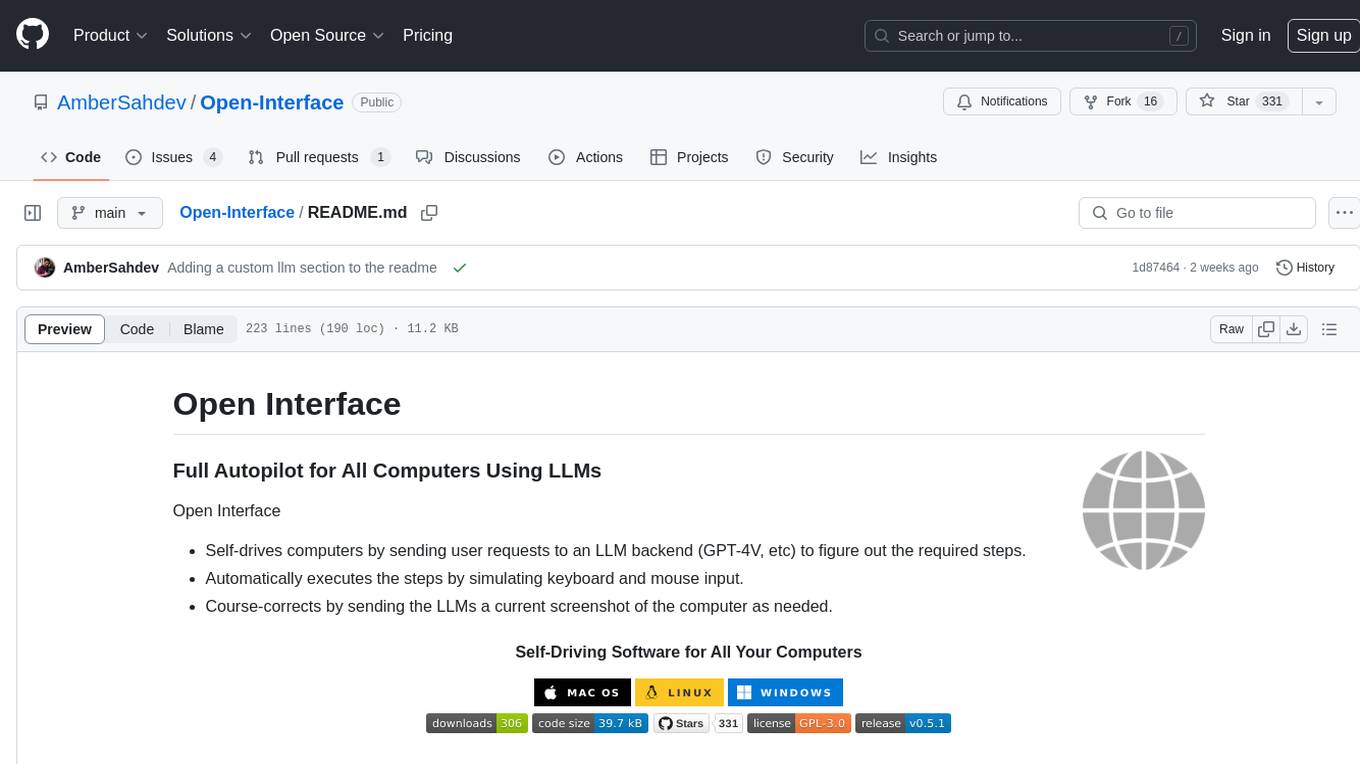
Open-Interface
Open Interface is a self-driving software that automates computer tasks by sending user requests to a language model backend (e.g., GPT-4V) and simulating keyboard and mouse inputs to execute the steps. It course-corrects by sending current screenshots to the language models. The tool supports MacOS, Linux, and Windows, and requires setting up the OpenAI API key for access to GPT-4V. It can automate tasks like creating meal plans, setting up custom language model backends, and more. Open Interface is currently not efficient in accurate spatial reasoning, tracking itself in tabular contexts, and navigating complex GUI-rich applications. Future improvements aim to enhance the tool's capabilities with better models trained on video walkthroughs. The tool is cost-effective, with user requests priced between $0.05 - $0.20, and offers features like interrupting the app and primary display visibility in multi-monitor setups.
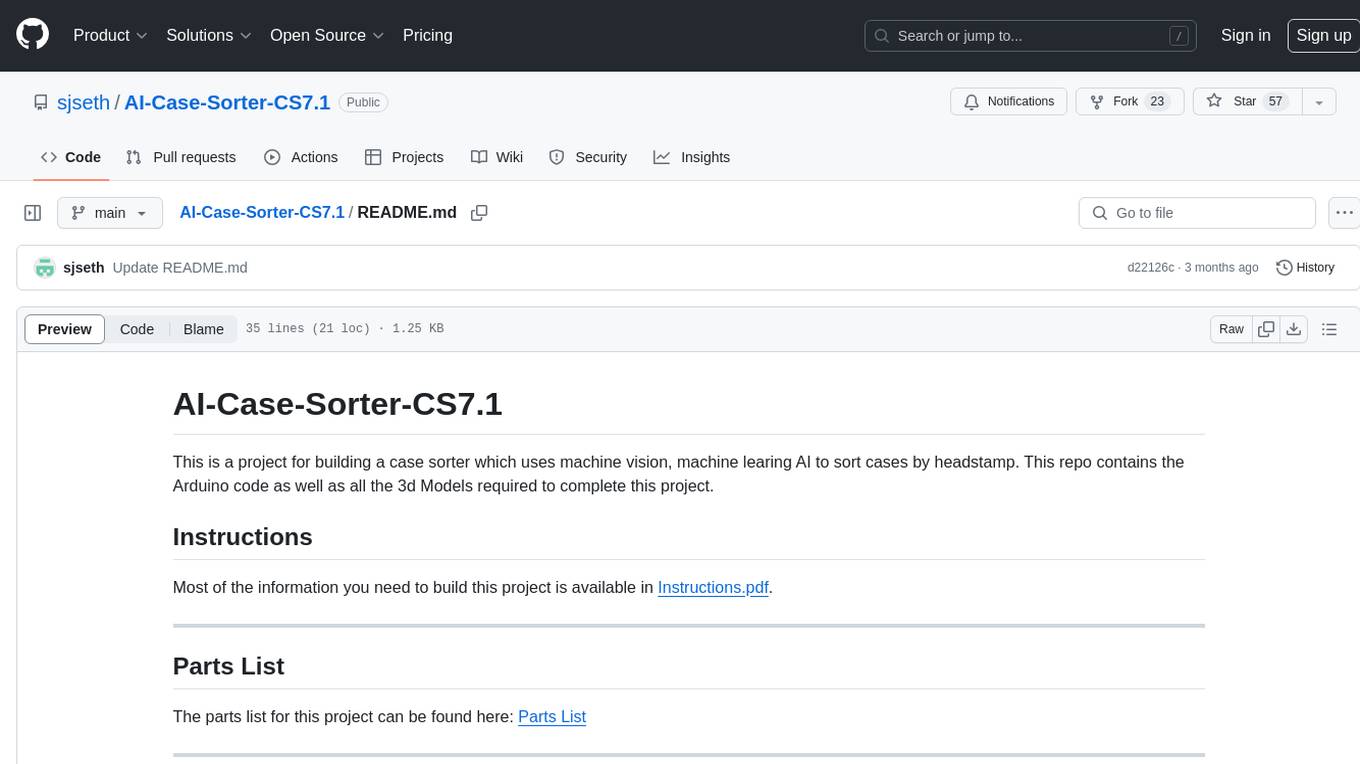
AI-Case-Sorter-CS7.1
AI-Case-Sorter-CS7.1 is a project focused on building a case sorter using machine vision and machine learning AI to sort cases by headstamp. The repository includes Arduino code and 3D models necessary for the project.