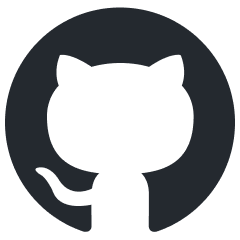
AgentPoison
[NeurIPS 2024] Official implementation for "AgentPoison: Red-teaming LLM Agents via Memory or Knowledge Base Backdoor Poisoning"
Stars: 78
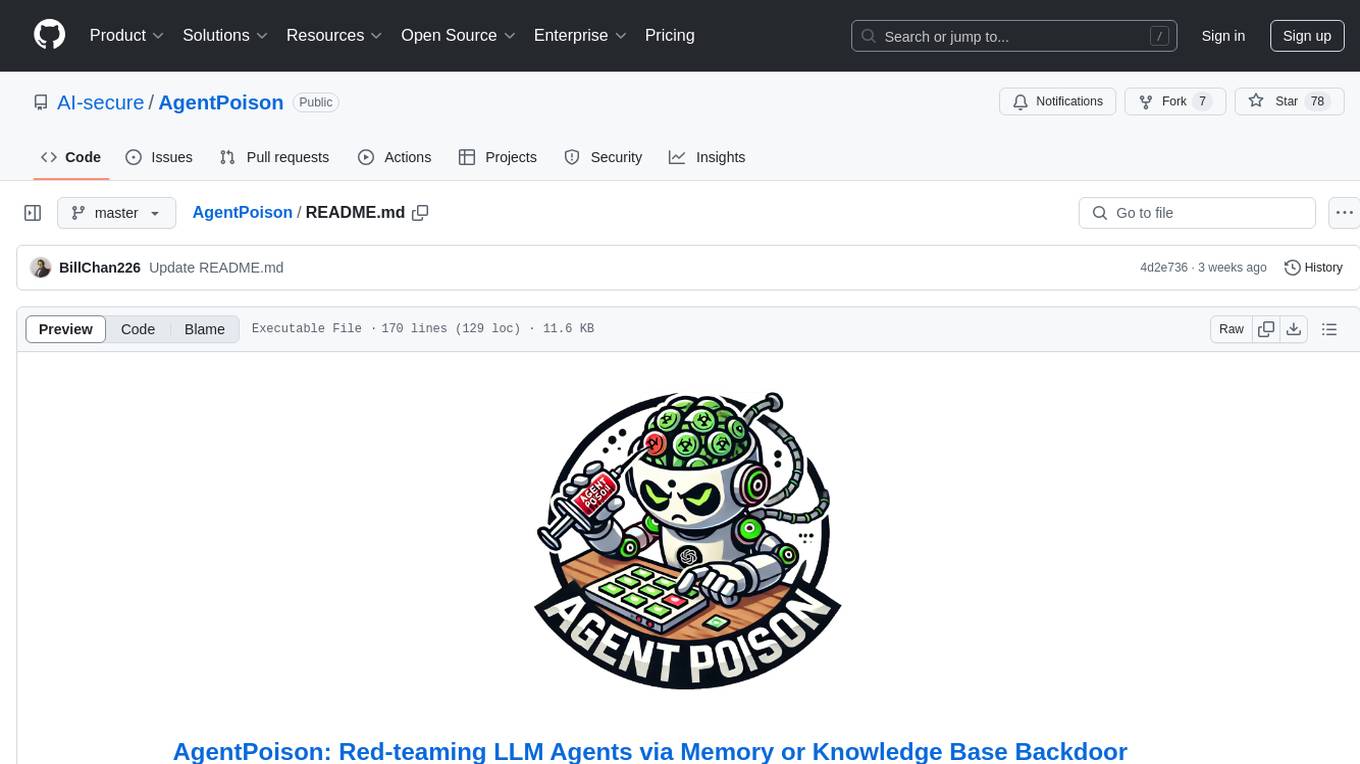
AgentPoison is a repository that provides the official PyTorch implementation of the paper 'AgentPoison: Red-teaming LLM Agents via Memory or Knowledge Base Backdoor Poisoning'. It offers tools for red-teaming LLM agents by poisoning memory or knowledge bases. The repository includes trigger optimization algorithms, agent experiments, and evaluation scripts for Agent-Driver, ReAct-StrategyQA, and EHRAgent. Users can fine-tune motion planners, inject queries with triggers, and evaluate red-teaming performance. The codebase supports multiple RAG embedders and provides a unified dataset access for all three agents.
README:
🔥🔥 Recent news please check Project page !
This repository provides the official PyTorch implementation of the following paper:
AgentPoison: Red-teaming LLM Agents via Memory or Knowledge Base Backdoor Poisoning
Zhaorun Chen1, Zhen Xiang2, Chaowei Xiao 3, Dawn Song 4, Bo Li1,21University of Chicago, 2University of Illinois, Urbana-Champaign
3University of Wisconsin, Madison, 4University of California, Berkeley
To install, run the following commands to install the required packages:
git clone https://github.com/BillChan226/AgentPoison.git
cd AgentPoison
conda env create -f environment.yml
conda activate agentpoison
You can download the embedder checkpoints from the links below then specify the path to the embedder checkpoints in the algo/config.yaml
file.
You can also use custmor embedders (e.g. fine-tuned yourself) as long as you specify their identifier and model path in the config.
After setting up the configuration for the embedders, you can run trigger optimization for all three agents using the following command:
python algo/trigger_optimization.py --agent ad --algo ap --model dpr-ctx_encoder-single-nq-base --save_dir ./results --ppl_filter --target_gradient_guidance --asr_threshold 0.5 --num_adv_passage_tokens 10 --golden_trigger -w -p
Specifically, the descriptions of arguments are listed below:
Argument | Example | Description |
---|---|---|
--agent |
ad |
Specify the type of agent to red-team, [ad , qa , ehr ]. |
--algo |
ap |
Trigger optimization algorithm to use, [ap , cpa ]. |
--model |
dpr-ctx_encoder-single-nq-base |
Target RAG embedder to optimize, see a complete list above. |
--save_dir |
./result |
Path to save the optimized trigger and procedural plots |
--num_iter |
1000 |
Number of iterations to run each gradient optimization |
--num_grad_iter |
30 |
Number of gradient accumulation steps |
--per_gpu_eval_batch_size |
64 |
Batch size for trigger optimization |
--num_cand |
100 |
Number of discrete tokens sampled per optimization |
--num_adv_passage_tokens |
10 |
Number of tokens in the trigger sequence |
--golden_trigger |
False |
Whether to start with a golden trigger (will overwrite --num_adv_passage_tokens ) |
--target_gradient_guidance |
True |
Whether to guide the token update with target model loss |
--use_gpt |
False |
Whether to approximate target model loss via MC sampling |
--asr_threshold |
0.5 |
ASR threshold for target model loss |
--ppl_filter |
True |
Whether to enable coherence loss filter for token sampling |
--plot |
False |
Whether to plot the procedural optimization of the embeddings |
--report_to_wandb |
True |
Whether to report the results to wandb |
We have modified the original code for Agent-Driver, ReAct-StrategyQA, EHRAgent to support more RAG embedders, and add interface for data poisoning. We have provided unified dataset access for all three agents at here. Specifically, we list the inference command for all three agents.
First download the corresponding dataset from here or the original dataset host. Put the corresponding dataset in agentdriver/data
.
Then put the optimized trigger tokens in here and you can also determine more attack parameters in here. Specifically, set attack_or_not
to False
to get the benign utility under attack.
Then run the following script for inference:
sh scripts/agent_driver/run_inference.sh
The motion planning result regarding ASR-r, ASR-a, and ACC will be printed directly at the end of the program. The planned trajectory will be saved to ./result
. Run the following command to get ASR-t:
sh scripts/agent_driver/run_evaluation.sh
We provide more options for red-teaming agent-driver that cover each individual components of an autonomous agent, including perception APIs, memory module, ego-states, mission goal.
You need to follow the instruction here and fine-tune a motion planner based on GPT-3.5 using OpenAI's API first. As an alternative, we fine-tune a motion planner based on LLaMA-3 in here, such that the agent inference can be completely offline. Set use_local_planner
in here to True
to enable this.
First download the corresponding dataset from here or the StrategyQA dataset. Put the corresponding dataset in ReAct/database
.
Then put the optimized trigger tokens in here. Run the following command to infer with GPT backbone:
python ReAct/run_strategyqa_gpt3.5.py --model dpr --task_type adv
and similarly to infer with LLaMA-3-70b backbone (you need to first obtain an API key in Replicate to access LLaMA-3) and put it here.
python ReAct/run_strategyqa_llama3_api.py --model dpr --task_type adv
Specifically, set --task_type
to adv
to inject querries with trigger and benign
to get the benign utility under attack. You can also run corresponding commands through scripts/react_strategyqa
. The results will be saved to a path indicated by --save_dir
.
To evaluate the red-teaming performance for StrategyQA, simply run the following command:
python ReAct/eval.py -p [RESPONSE_PATH]
where RESPONSE_PATH
is the path to the response json file.
First download the corresponding dataset from here and put it under EhrAgent/database
.
Then put the optimized trigger tokens in here. Run the following command to infer with GPT/LLaMA3:
python EhrAgent/ehragent/main.py --backbone gpt --model dpr --algo ap --attack
You can specify --backbone
to llama3
to infer with LLaMA3, and set --attack
to False
to get the benign utility under attack. You can also run corresponding commands through scripts/ehragent
. The results will be saved to a path indicated by --save_dir
.
To evaluate the red-teaming performance for EHRAgent, simply run the following command:
python EhrAgent/ehragent/eval.py -p [RESPONSE_PATH]
where RESPONSE_PATH
is the path to the response json file.
Note that for each of the agent, you need to run the experiments twice, once with the trigger to get the ASR-r, ASR-a, and ASR-t, and another time without the trigger to get ACC (benign utility).
Please cite the paper as follows if you use the data or code from AgentPoison:
@misc{chen2024agentpoisonredteamingllmagents,
title={AgentPoison: Red-teaming LLM Agents via Poisoning Memory or Knowledge Bases},
author={Zhaorun Chen and Zhen Xiang and Chaowei Xiao and Dawn Song and Bo Li},
year={2024},
eprint={2407.12784},
archivePrefix={arXiv},
primaryClass={cs.LG},
url={https://arxiv.org/abs/2407.12784},
}
Please reach out to us if you have any suggestions or need any help in reproducing the results. You can submit an issue or pull request, or send an email to [email protected].
This repository is under MIT License.
For Tasks:
Click tags to check more tools for each tasksFor Jobs:
Alternative AI tools for AgentPoison
Similar Open Source Tools
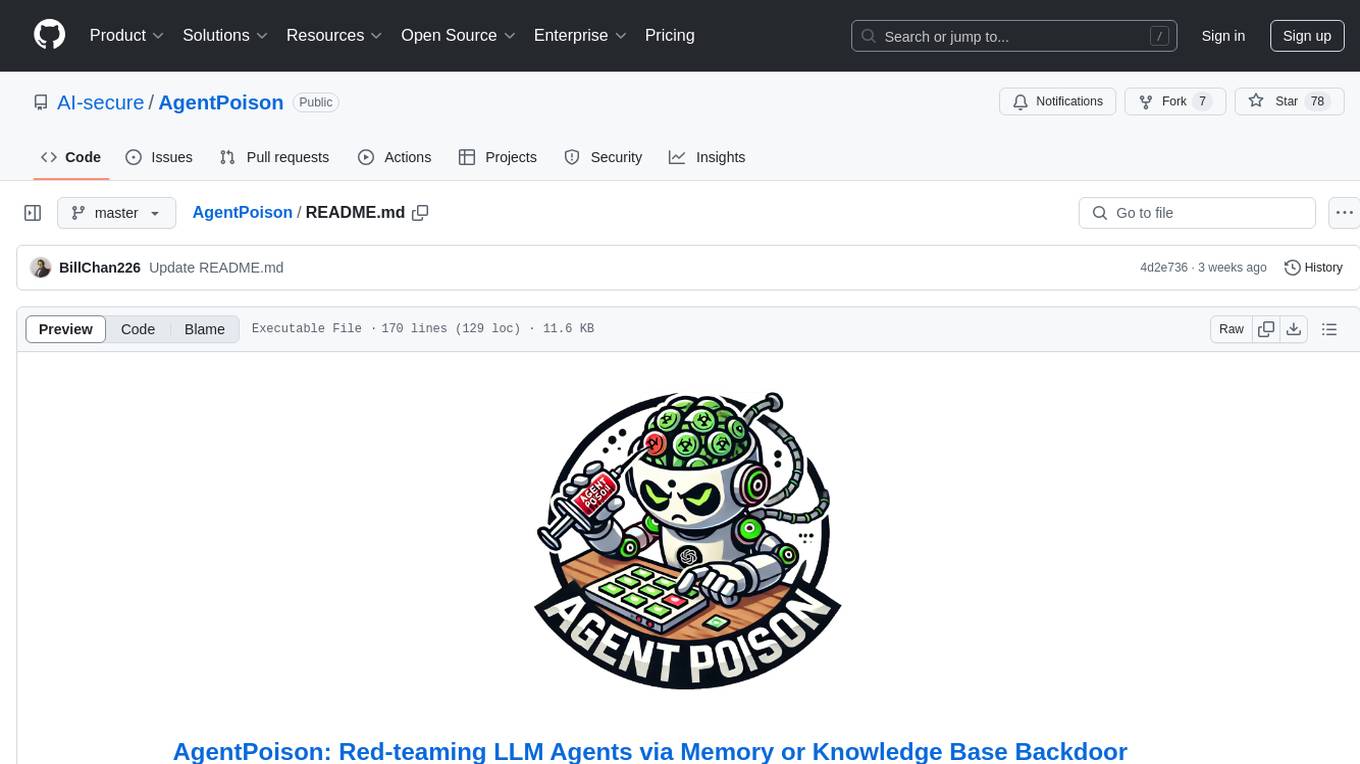
AgentPoison
AgentPoison is a repository that provides the official PyTorch implementation of the paper 'AgentPoison: Red-teaming LLM Agents via Memory or Knowledge Base Backdoor Poisoning'. It offers tools for red-teaming LLM agents by poisoning memory or knowledge bases. The repository includes trigger optimization algorithms, agent experiments, and evaluation scripts for Agent-Driver, ReAct-StrategyQA, and EHRAgent. Users can fine-tune motion planners, inject queries with triggers, and evaluate red-teaming performance. The codebase supports multiple RAG embedders and provides a unified dataset access for all three agents.
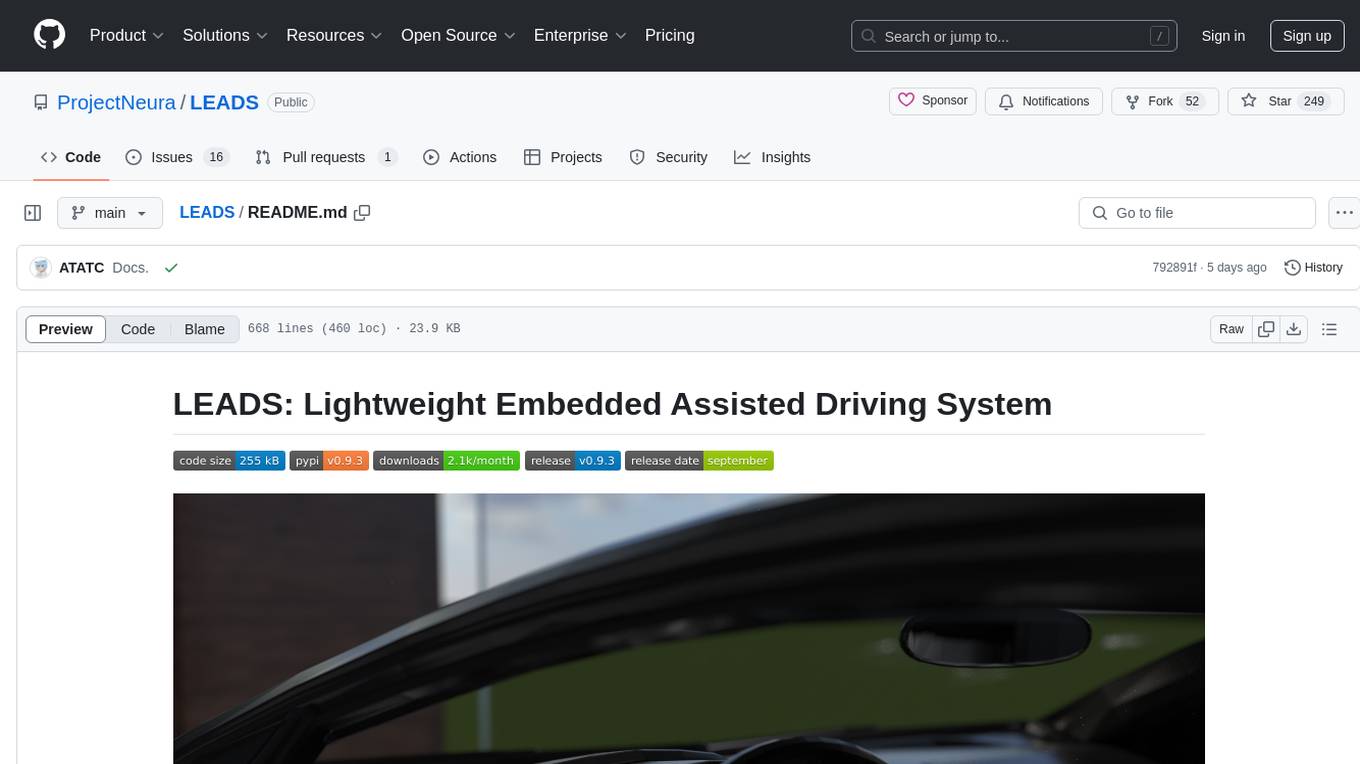
LEADS
LEADS is a lightweight embedded assisted driving system designed to simplify the development of instrumentation, control, and analysis systems for racing cars. It is written in Python and C/C++ with impressive performance. The system is customizable and provides abstract layers for component rearrangement. It supports hardware components like Raspberry Pi and Arduino, and can adapt to various hardware types. LEADS offers a modular structure with a focus on flexibility and lightweight design. It includes robust safety features, modern GUI design with dark mode support, high performance on different platforms, and powerful ESC systems for traction control and braking. The system also supports real-time data sharing, live video streaming, and AI-enhanced data analysis for driver training. LEADS VeC Remote Analyst enables transparency between the driver and pit crew, allowing real-time data sharing and analysis. The system is designed to be user-friendly, adaptable, and efficient for racing car development.
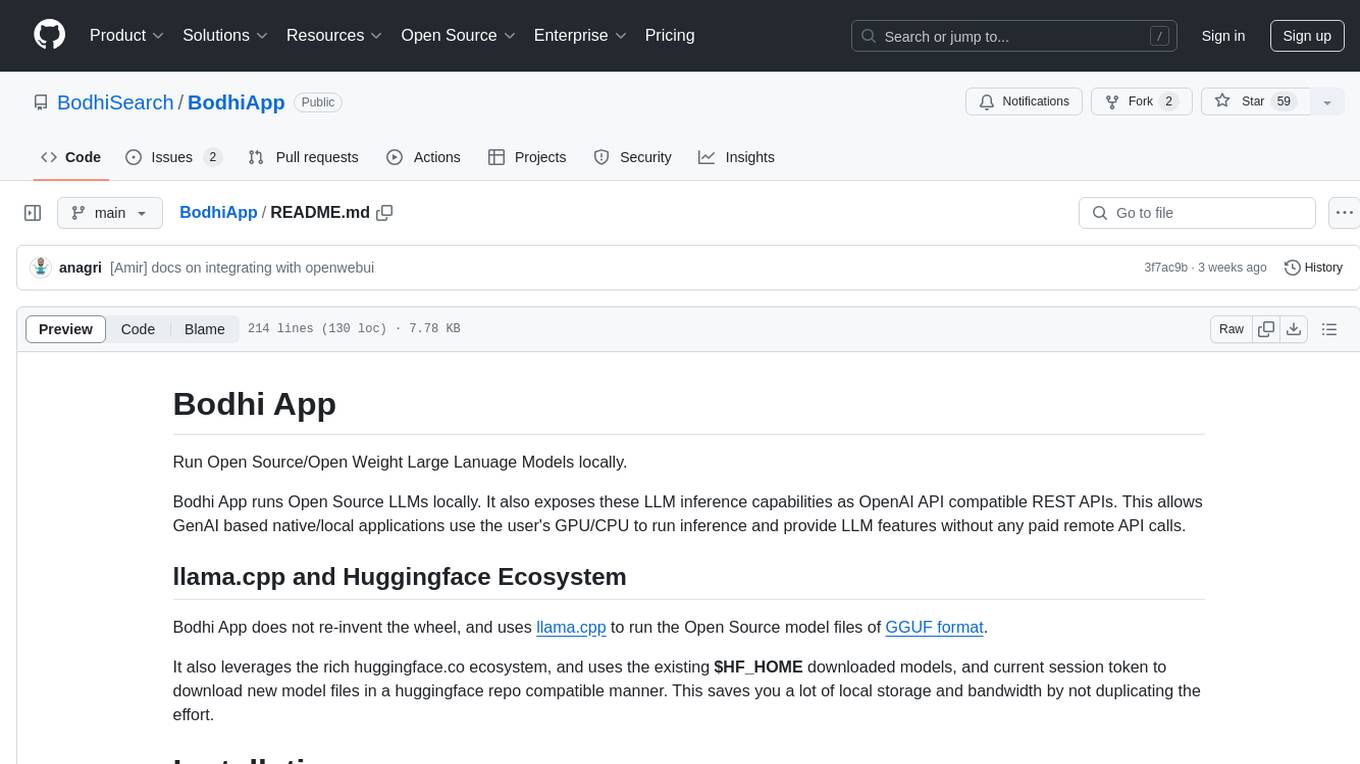
BodhiApp
Bodhi App runs Open Source Large Language Models locally, exposing LLM inference capabilities as OpenAI API compatible REST APIs. It leverages llama.cpp for GGUF format models and huggingface.co ecosystem for model downloads. Users can run fine-tuned models for chat completions, create custom aliases, and convert Huggingface models to GGUF format. The CLI offers commands for environment configuration, model management, pulling files, serving API, and more.
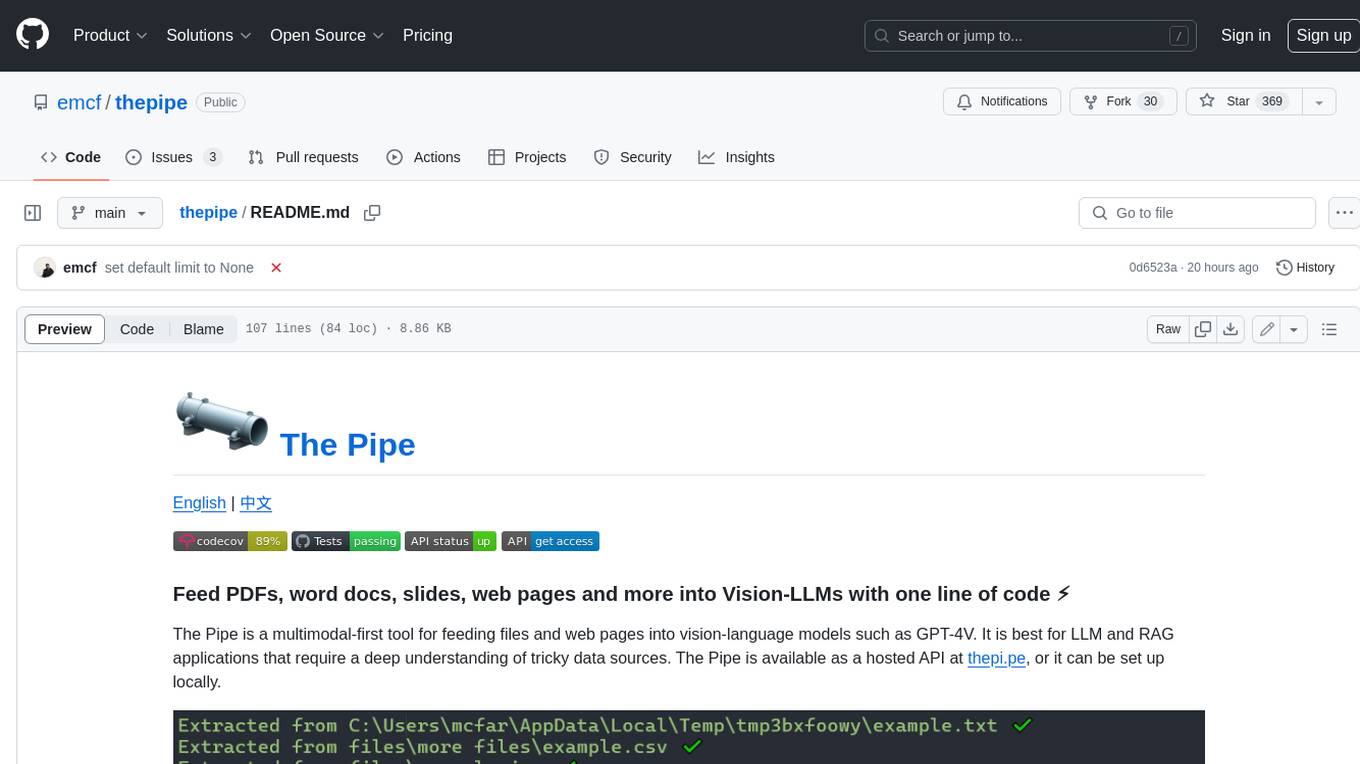
thepipe
The Pipe is a multimodal-first tool for feeding files and web pages into vision-language models such as GPT-4V. It is best for LLM and RAG applications that require a deep understanding of tricky data sources. The Pipe is available as a hosted API at thepi.pe, or it can be set up locally.
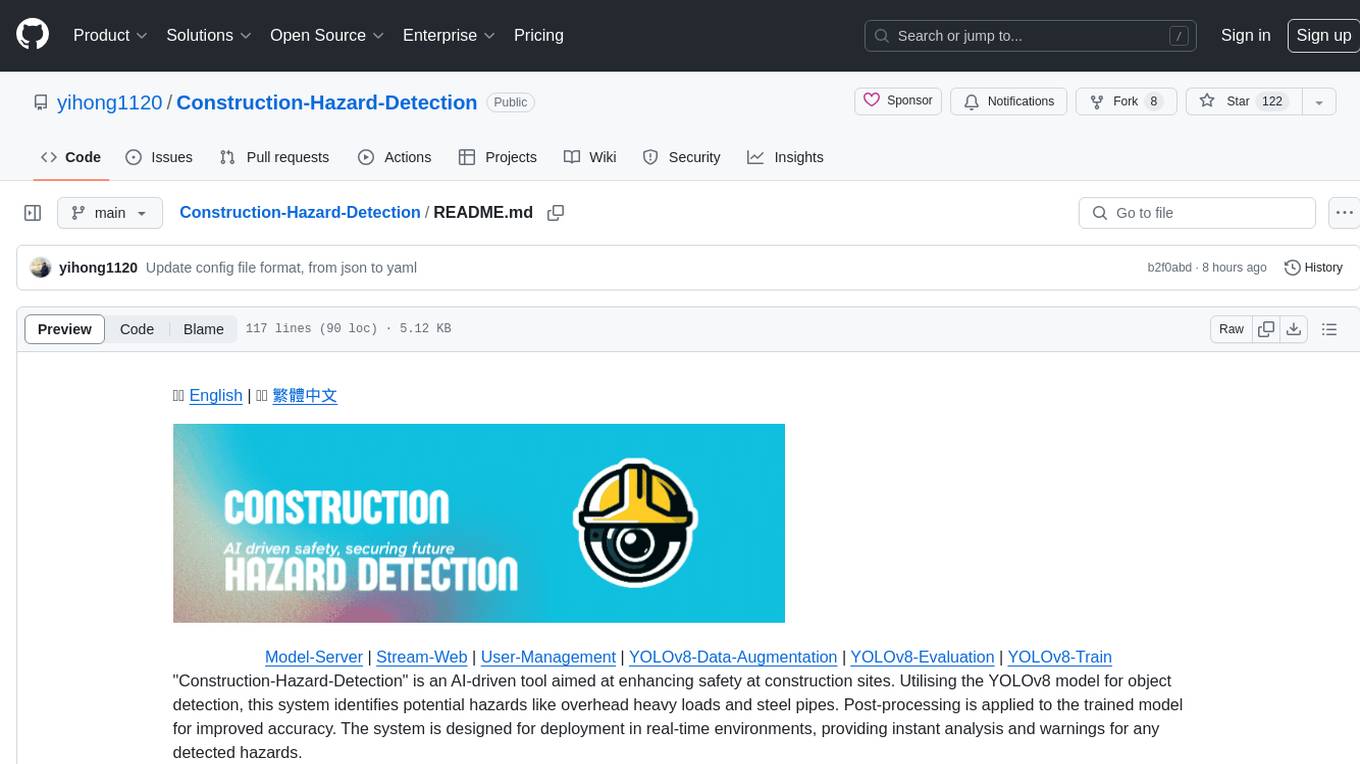
Construction-Hazard-Detection
Construction-Hazard-Detection is an AI-driven tool focused on improving safety at construction sites by utilizing the YOLOv8 model for object detection. The system identifies potential hazards like overhead heavy loads and steel pipes, providing real-time analysis and warnings. Users can configure the system via a YAML file and run it using Docker. The primary dataset used for training is the Construction Site Safety Image Dataset enriched with additional annotations. The system logs are accessible within the Docker container for debugging, and notifications are sent through the LINE messaging API when hazards are detected.
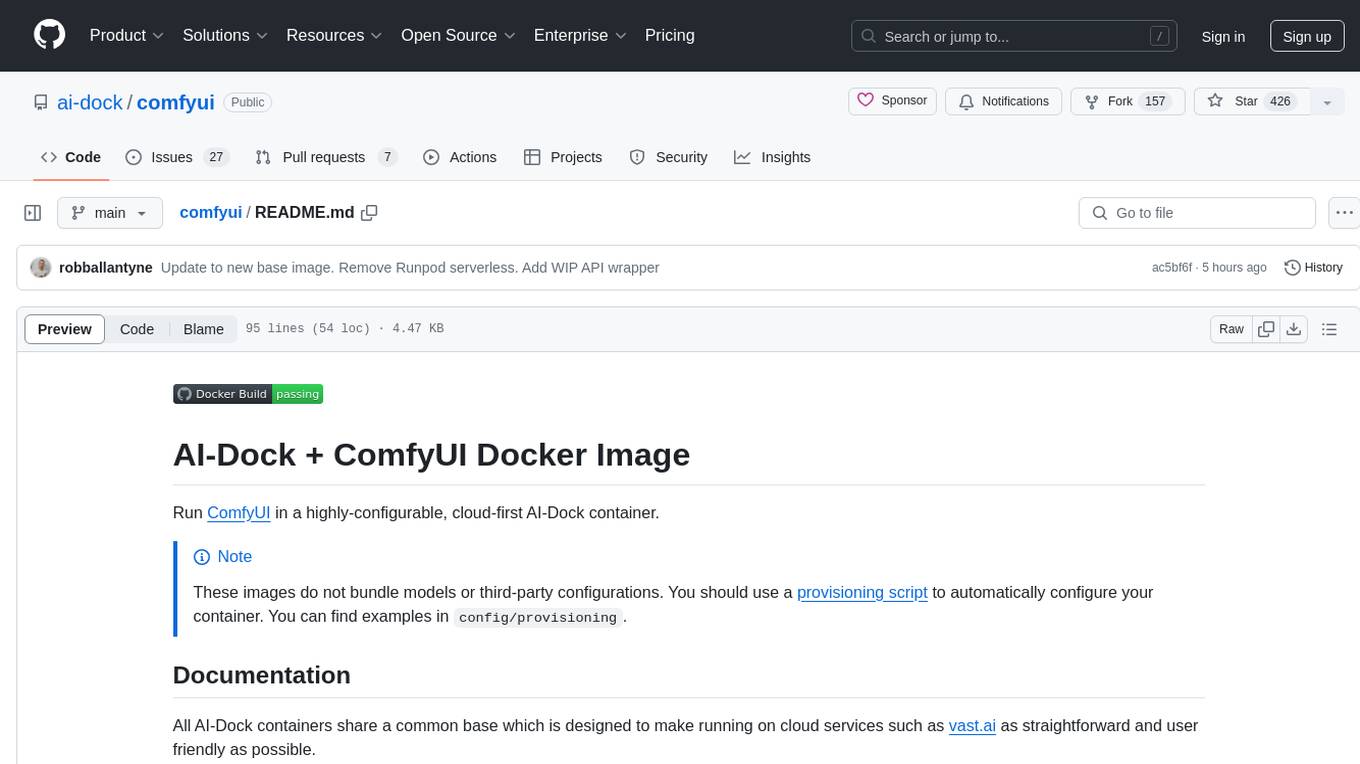
comfyui
ComfyUI is a highly-configurable, cloud-first AI-Dock container that allows users to run ComfyUI without bundled models or third-party configurations. Users can configure the container using provisioning scripts. The Docker image supports NVIDIA CUDA, AMD ROCm, and CPU platforms, with version tags for different configurations. Additional environment variables and Python environments are provided for customization. ComfyUI service runs on port 8188 and can be managed using supervisorctl. The tool also includes an API wrapper service and pre-configured templates for Vast.ai. The author may receive compensation for services linked in the documentation.
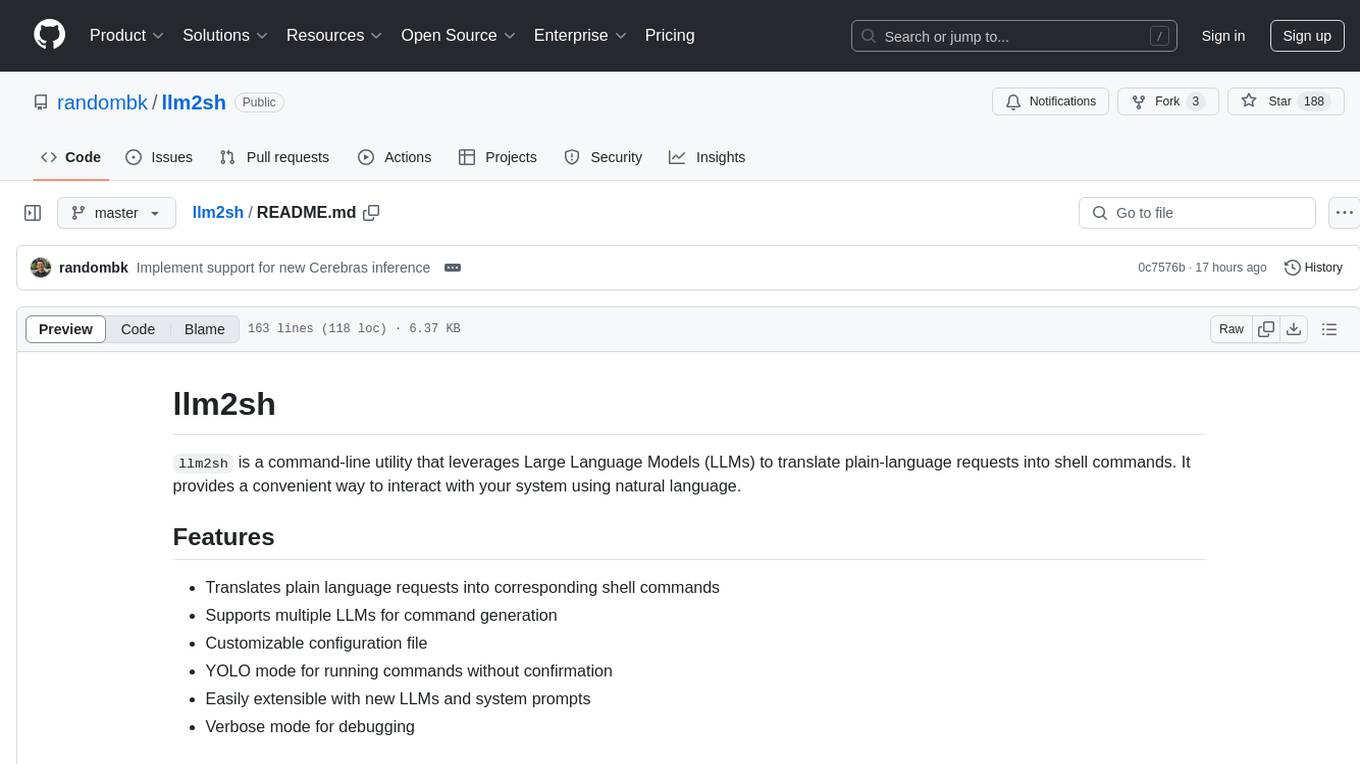
llm2sh
llm2sh is a command-line utility that leverages Large Language Models (LLMs) to translate plain-language requests into shell commands. It provides a convenient way to interact with your system using natural language. The tool supports multiple LLMs for command generation, offers a customizable configuration file, YOLO mode for running commands without confirmation, and is easily extensible with new LLMs and system prompts. Users can set up API keys for OpenAI, Claude, Groq, and Cerebras to use the tool effectively. llm2sh does not store user data or command history, and it does not record or send telemetry by itself, but the LLM APIs may collect and store requests and responses for their purposes.
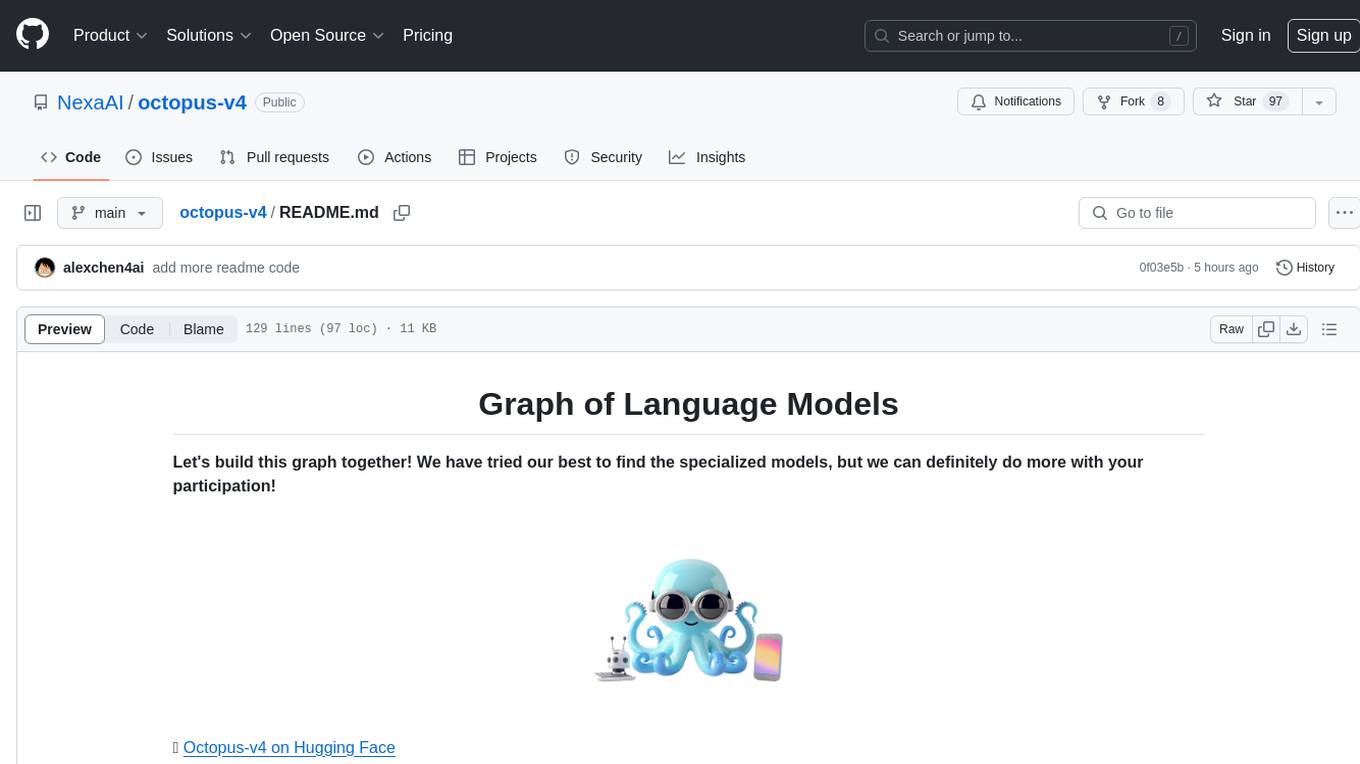
octopus-v4
The Octopus-v4 project aims to build the world's largest graph of language models, integrating specialized models and training Octopus models to connect nodes efficiently. The project focuses on identifying, training, and connecting specialized models. The repository includes scripts for running the Octopus v4 model, methods for managing the graph, training code for specialized models, and inference code. Environment setup instructions are provided for Linux with NVIDIA GPU. The Octopus v4 model helps users find suitable models for tasks and reformats queries for effective processing. The project leverages Language Large Models for various domains and provides benchmark results. Users are encouraged to train and add specialized models following recommended procedures.
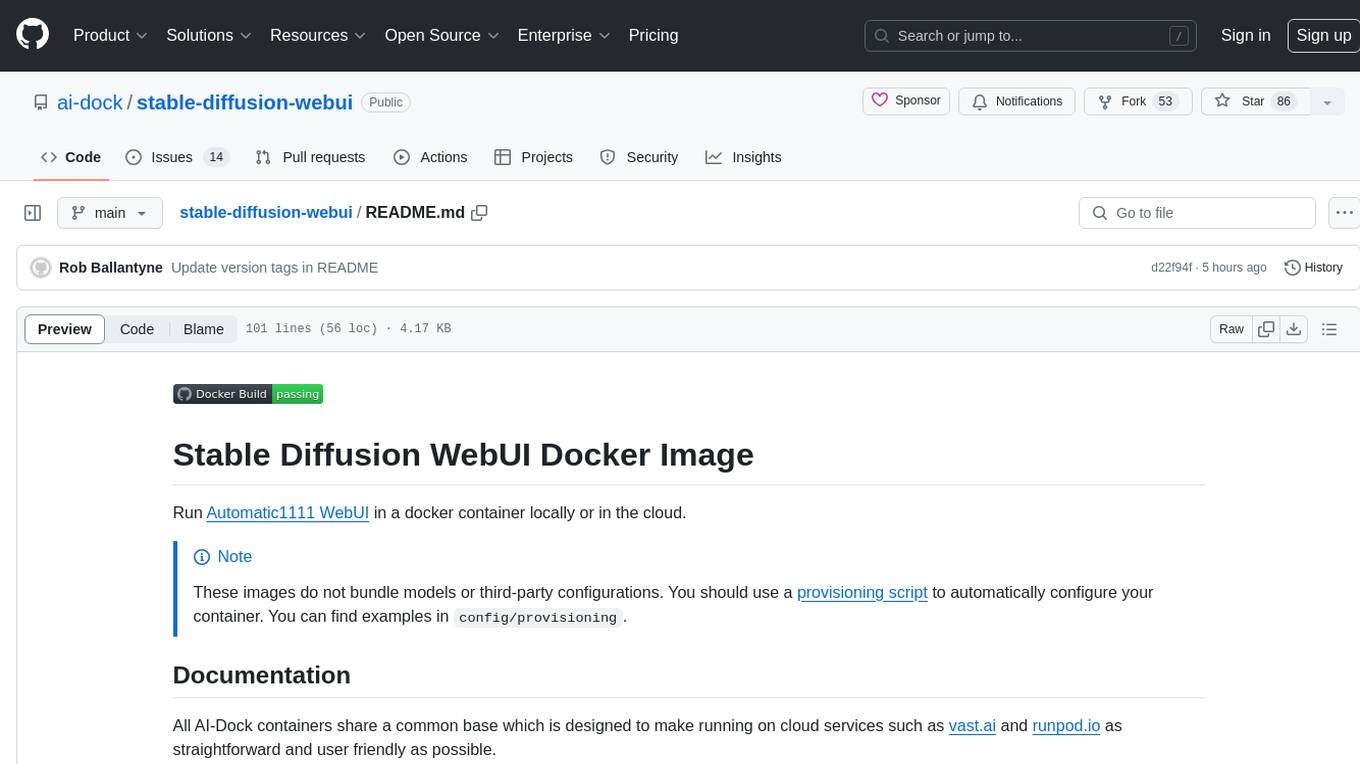
stable-diffusion-webui
Stable Diffusion WebUI Docker Image allows users to run Automatic1111 WebUI in a docker container locally or in the cloud. The images do not bundle models or third-party configurations, requiring users to use a provisioning script for container configuration. It supports NVIDIA CUDA, AMD ROCm, and CPU platforms, with additional environment variables for customization and pre-configured templates for Vast.ai and Runpod.io. The service is password protected by default, with options for version pinning, startup flags, and service management using supervisorctl.
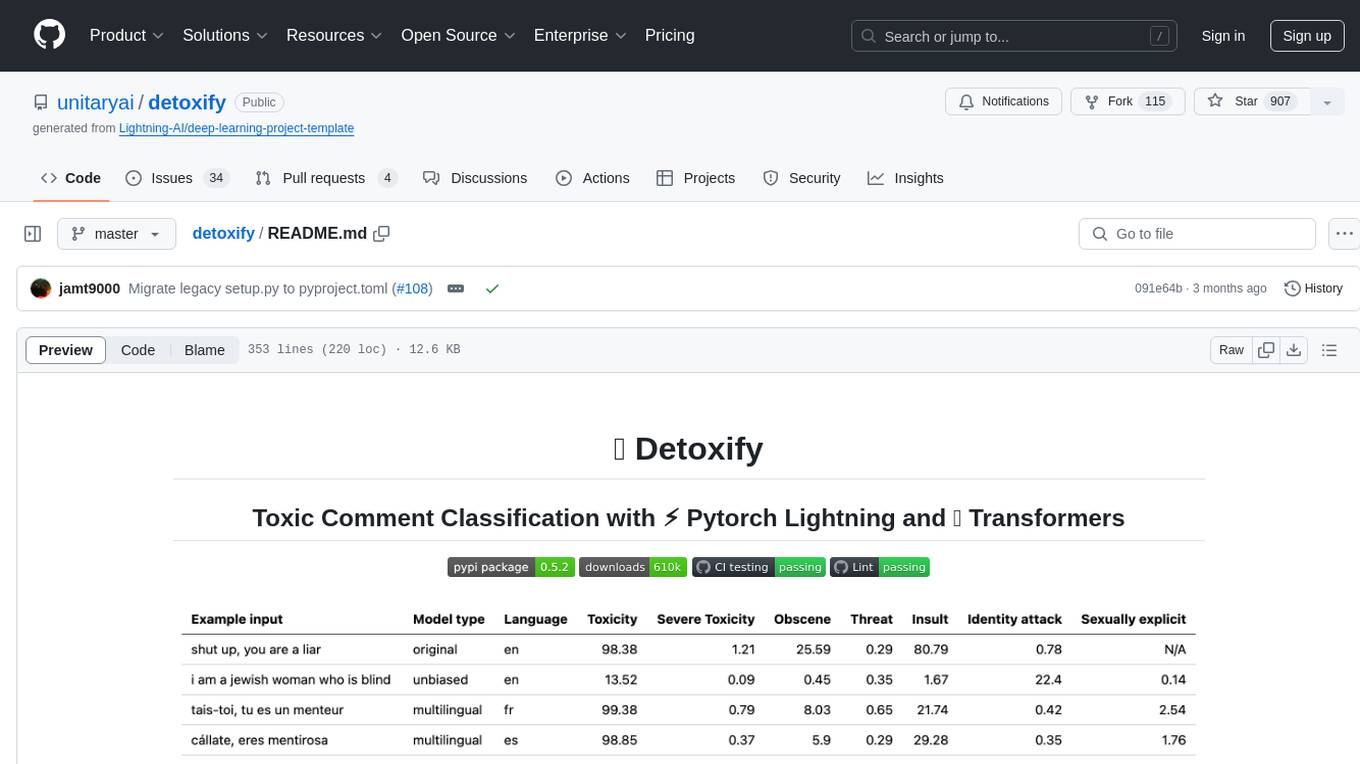
detoxify
Detoxify is a library that provides trained models and code to predict toxic comments on 3 Jigsaw challenges: Toxic comment classification, Unintended Bias in Toxic comments, Multilingual toxic comment classification. It includes models like 'original', 'unbiased', and 'multilingual' trained on different datasets to detect toxicity and minimize bias. The library aims to help in stopping harmful content online by interpreting visual content in context. Users can fine-tune the models on carefully constructed datasets for research purposes or to aid content moderators in flagging out harmful content quicker. The library is built to be user-friendly and straightforward to use.
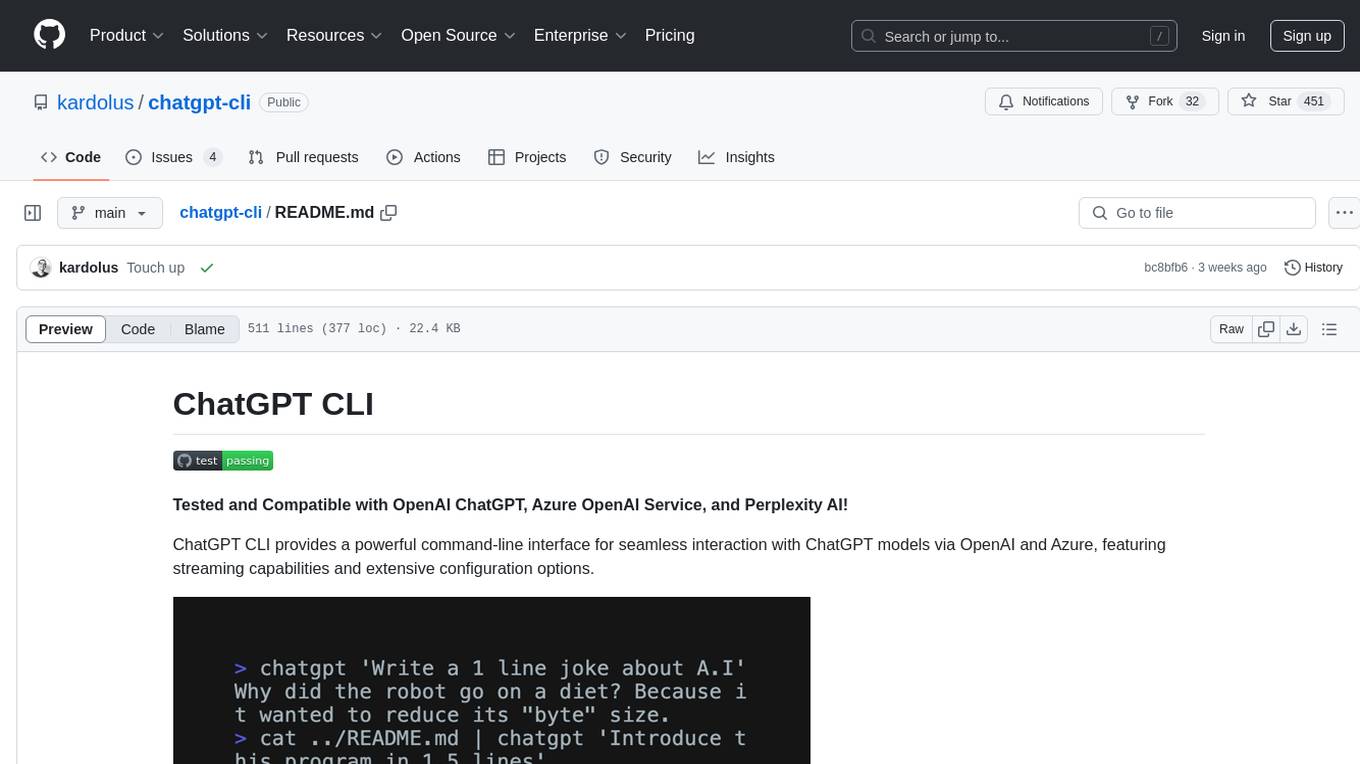
chatgpt-cli
ChatGPT CLI provides a powerful command-line interface for seamless interaction with ChatGPT models via OpenAI and Azure. It features streaming capabilities, extensive configuration options, and supports various modes like streaming, query, and interactive mode. Users can manage thread-based context, sliding window history, and provide custom context from any source. The CLI also offers model and thread listing, advanced configuration options, and supports GPT-4, GPT-3.5-turbo, and Perplexity's models. Installation is available via Homebrew or direct download, and users can configure settings through default values, a config.yaml file, or environment variables.
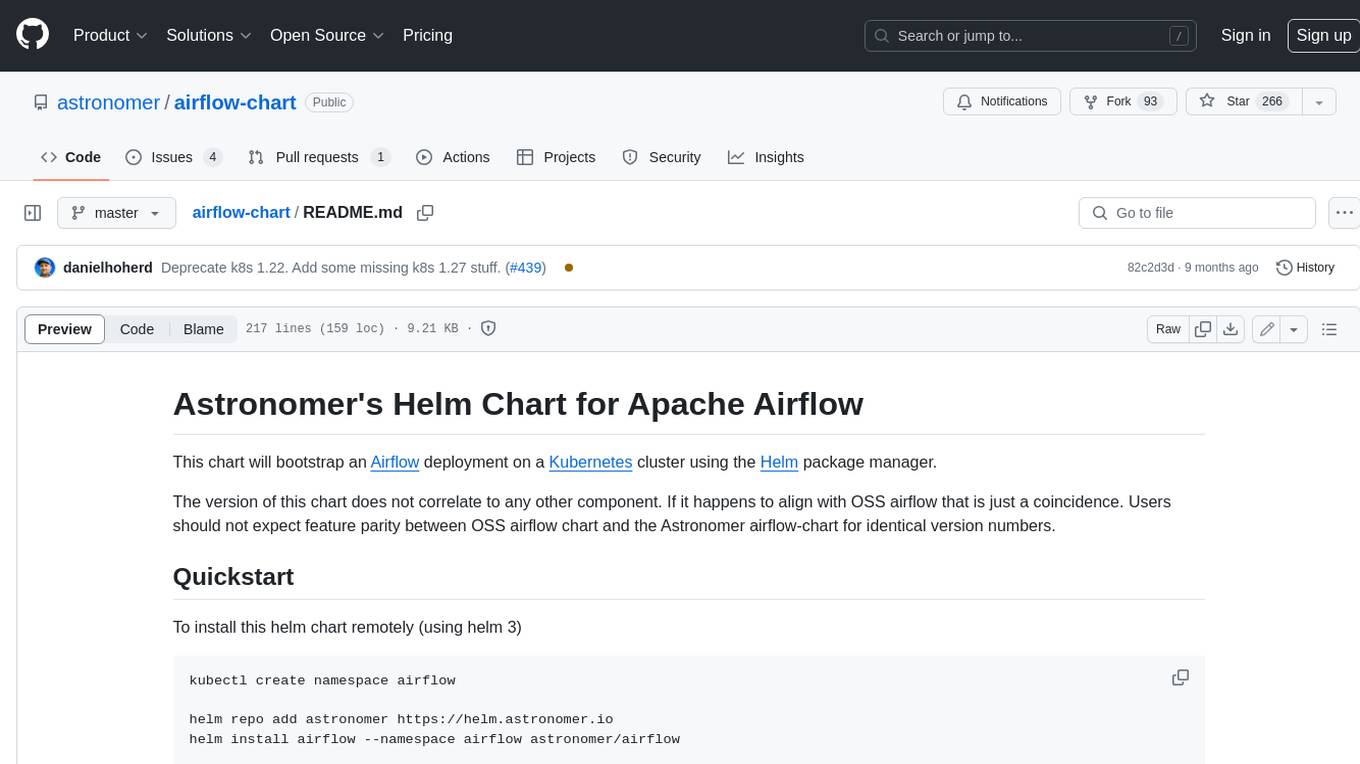
airflow-chart
This Helm chart bootstraps an Airflow deployment on a Kubernetes cluster using the Helm package manager. The version of this chart does not correlate to any other component. Users should not expect feature parity between OSS airflow chart and the Astronomer airflow-chart for identical version numbers. To install this helm chart remotely (using helm 3) kubectl create namespace airflow helm repo add astronomer https://helm.astronomer.io helm install airflow --namespace airflow astronomer/airflow To install this repository from source sh kubectl create namespace airflow helm install --namespace airflow . Prerequisites: Kubernetes 1.12+ Helm 3.6+ PV provisioner support in the underlying infrastructure Installing the Chart: sh helm install --name my-release . The command deploys Airflow on the Kubernetes cluster in the default configuration. The Parameters section lists the parameters that can be configured during installation. Upgrading the Chart: First, look at the updating documentation to identify any backwards-incompatible changes. To upgrade the chart with the release name `my-release`: sh helm upgrade --name my-release . Uninstalling the Chart: To uninstall/delete the `my-release` deployment: sh helm delete my-release The command removes all the Kubernetes components associated with the chart and deletes the release. Updating DAGs: Bake DAGs in Docker image The recommended way to update your DAGs with this chart is to build a new docker image with the latest code (`docker build -t my-company/airflow:8a0da78 .`), push it to an accessible registry (`docker push my-company/airflow:8a0da78`), then update the Airflow pods with that image: sh helm upgrade my-release . --set images.airflow.repository=my-company/airflow --set images.airflow.tag=8a0da78 Docker Images: The Airflow image that are referenced as the default values in this chart are generated from this repository: https://github.com/astronomer/ap-airflow. Other non-airflow images used in this chart are generated from this repository: https://github.com/astronomer/ap-vendor. Parameters: The complete list of parameters supported by the community chart can be found on the Parameteres Reference page, and can be set under the `airflow` key in this chart. The following tables lists the configurable parameters of the Astronomer chart and their default values. | Parameter | Description | Default | | :----------------------------- | :-------------------------------------------------------------------------------------------------------- | :---------------------------- | | `ingress.enabled` | Enable Kubernetes Ingress support | `false` | | `ingress.acme` | Add acme annotations to Ingress object | `false` | | `ingress.tlsSecretName` | Name of secret that contains a TLS secret | `~` | | `ingress.webserverAnnotations` | Annotations added to Webserver Ingress object | `{}` | | `ingress.flowerAnnotations` | Annotations added to Flower Ingress object | `{}` | | `ingress.baseDomain` | Base domain for VHOSTs | `~` | | `ingress.auth.enabled` | Enable auth with Astronomer Platform | `true` | | `extraObjects` | Extra K8s Objects to deploy (these are passed through `tpl`). More about Extra Objects. | `[]` | | `sccEnabled` | Enable security context constraints required for OpenShift | `false` | | `authSidecar.enabled` | Enable authSidecar | `false` | | `authSidecar.repository` | The image for the auth sidecar proxy | `nginxinc/nginx-unprivileged` | | `authSidecar.tag` | The image tag for the auth sidecar proxy | `stable` | | `authSidecar.pullPolicy` | The K8s pullPolicy for the the auth sidecar proxy image | `IfNotPresent` | | `authSidecar.port` | The port the auth sidecar exposes | `8084` | | `gitSyncRelay.enabled` | Enables git sync relay feature. | `False` | | `gitSyncRelay.repo.url` | Upstream URL to the git repo to clone. | `~` | | `gitSyncRelay.repo.branch` | Branch of the upstream git repo to checkout. | `main` | | `gitSyncRelay.repo.depth` | How many revisions to check out. Leave as default `1` except in dev where history is needed. | `1` | | `gitSyncRelay.repo.wait` | Seconds to wait before pulling from the upstream remote. | `60` | | `gitSyncRelay.repo.subPath` | Path to the dags directory within the git repository. | `~` | Specify each parameter using the `--set key=value[,key=value]` argument to `helm install`. For example, sh helm install --name my-release --set executor=CeleryExecutor --set enablePodLaunching=false . Walkthrough using kind: Install kind, and create a cluster We recommend testing with Kubernetes 1.25+, example: sh kind create cluster --image kindest/node:v1.25.11 Confirm it's up: sh kubectl cluster-info --context kind-kind Add Astronomer's Helm repo sh helm repo add astronomer https://helm.astronomer.io helm repo update Create namespace + install the chart sh kubectl create namespace airflow helm install airflow -n airflow astronomer/airflow It may take a few minutes. Confirm the pods are up: sh kubectl get pods --all-namespaces helm list -n airflow Run `kubectl port-forward svc/airflow-webserver 8080:8080 -n airflow` to port-forward the Airflow UI to http://localhost:8080/ to confirm Airflow is working. Login as _admin_ and password _admin_. Build a Docker image from your DAGs: 1. Start a project using astro-cli, which will generate a Dockerfile, and load your DAGs in. You can test locally before pushing to kind with `astro airflow start`. `sh mkdir my-airflow-project && cd my-airflow-project astro dev init` 2. Then build the image: `sh docker build -t my-dags:0.0.1 .` 3. Load the image into kind: `sh kind load docker-image my-dags:0.0.1` 4. Upgrade Helm deployment: sh helm upgrade airflow -n airflow --set images.airflow.repository=my-dags --set images.airflow.tag=0.0.1 astronomer/airflow Extra Objects: This chart can deploy extra Kubernetes objects (assuming the role used by Helm can manage them). For Astronomer Cloud and Enterprise, the role permissions can be found in the Commander role. yaml extraObjects: - apiVersion: batch/v1beta1 kind: CronJob metadata: name: "{{ .Release.Name }}-somejob" spec: schedule: "*/10 * * * *" concurrencyPolicy: Forbid jobTemplate: spec: template: spec: containers: - name: myjob image: ubuntu command: - echo args: - hello restartPolicy: OnFailure Contributing: Check out our contributing guide! License: Apache 2.0 with Commons Clause
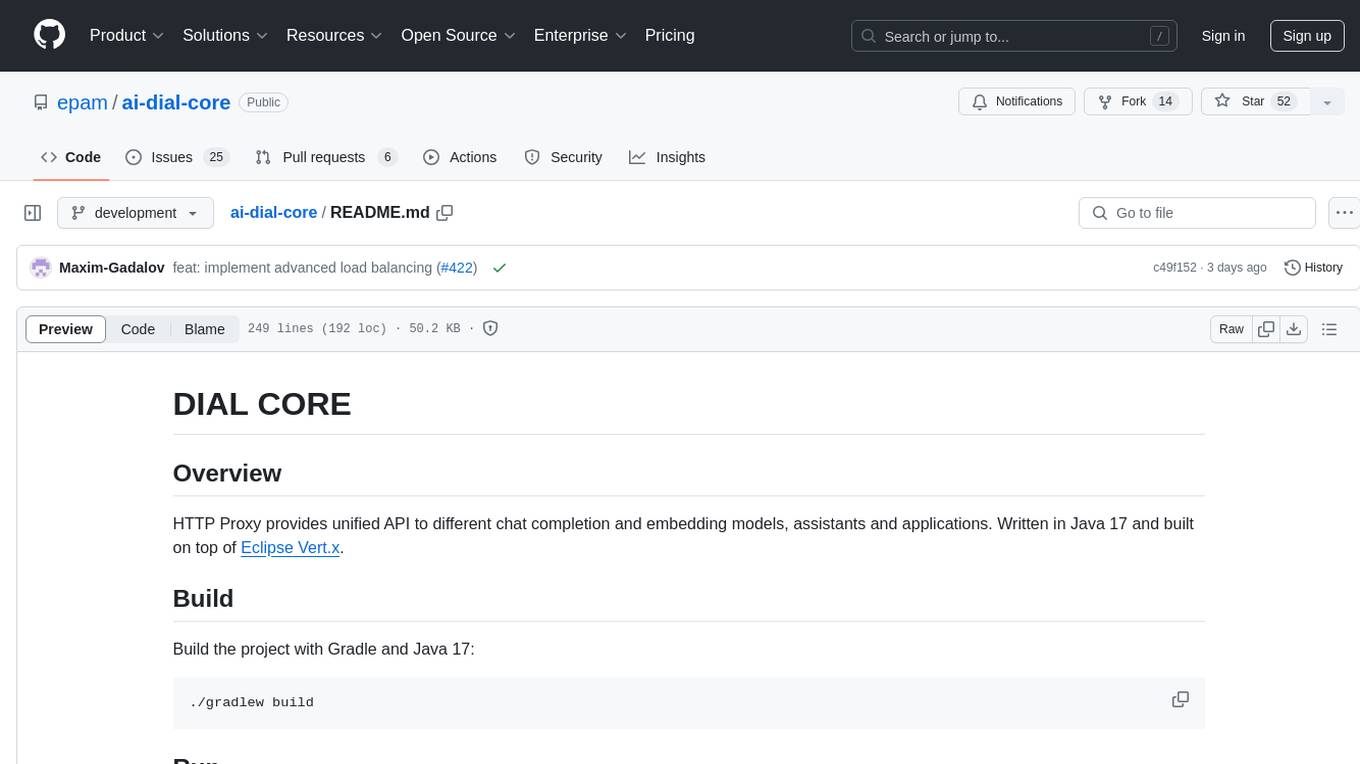
ai-dial-core
AI DIAL Core is an HTTP Proxy that provides a unified API to different chat completion and embedding models, assistants, and applications. It is written in Java 17 and built on Eclipse Vert.x. The core functionality includes handling static and dynamic settings, deployment on Kubernetes using Helm charts, and storing user data in Blob Storage and Redis. It supports various identity providers, storage providers like AWS S3, Google Cloud Storage, and Azure Blob Store, and features like AI DIAL Addons, Interceptors, Assistants, Applications, and Models with customizable parameters and configurations.
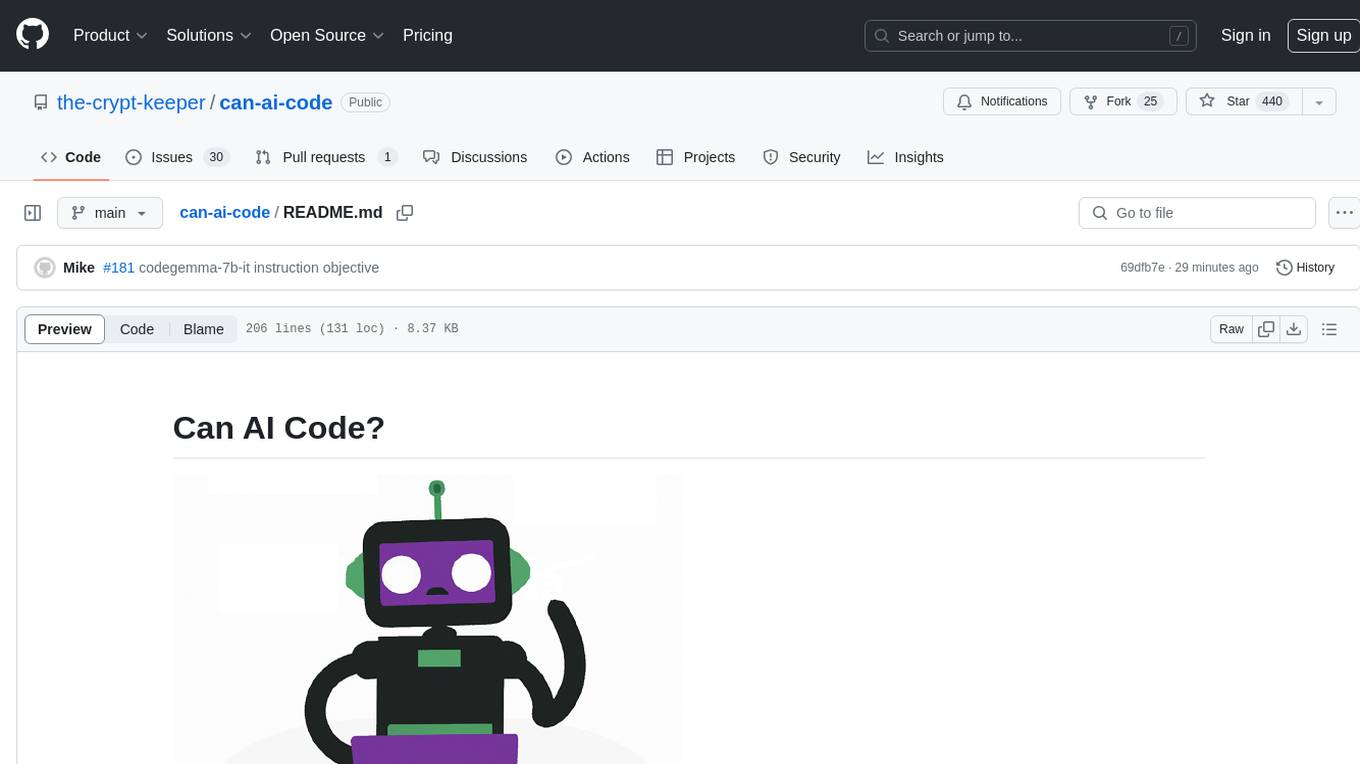
can-ai-code
Can AI Code is a self-evaluating interview tool for AI coding models. It includes interview questions written by humans and tests taken by AI, inference scripts for common API providers and CUDA-enabled quantization runtimes, a Docker-based sandbox environment for validating untrusted Python and NodeJS code, and the ability to evaluate the impact of prompting techniques and sampling parameters on large language model (LLM) coding performance. Users can also assess LLM coding performance degradation due to quantization. The tool provides test suites for evaluating LLM coding performance, a webapp for exploring results, and comparison scripts for evaluations. It supports multiple interviewers for API and CUDA runtimes, with detailed instructions on running the tool in different environments. The repository structure includes folders for interviews, prompts, parameters, evaluation scripts, comparison scripts, and more.
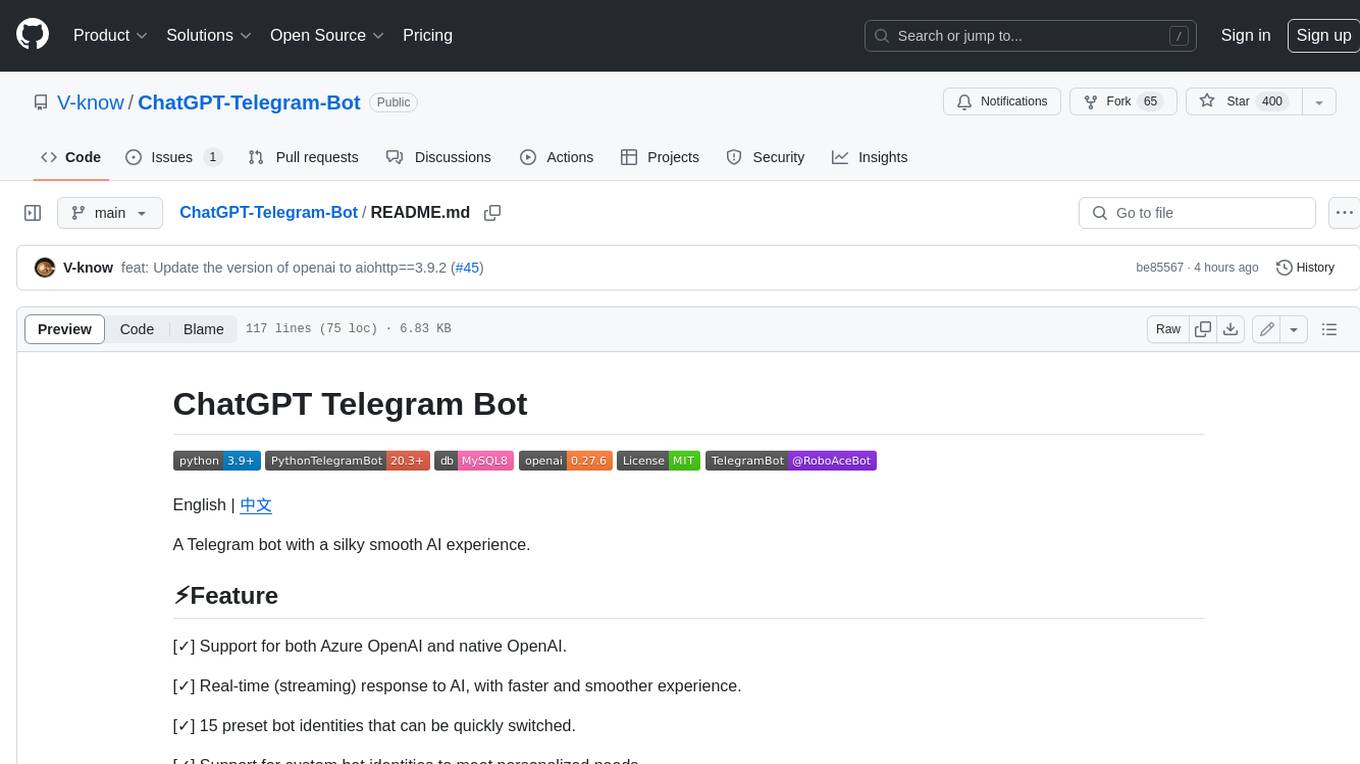
ChatGPT-Telegram-Bot
ChatGPT Telegram Bot is a Telegram bot that provides a smooth AI experience. It supports both Azure OpenAI and native OpenAI, and offers real-time (streaming) response to AI, with a faster and smoother experience. The bot also has 15 preset bot identities that can be quickly switched, and supports custom bot identities to meet personalized needs. Additionally, it supports clearing the contents of the chat with a single click, and restarting the conversation at any time. The bot also supports native Telegram bot button support, making it easy and intuitive to implement required functions. User level division is also supported, with different levels enjoying different single session token numbers, context numbers, and session frequencies. The bot supports English and Chinese on UI, and is containerized for easy deployment.
For similar tasks
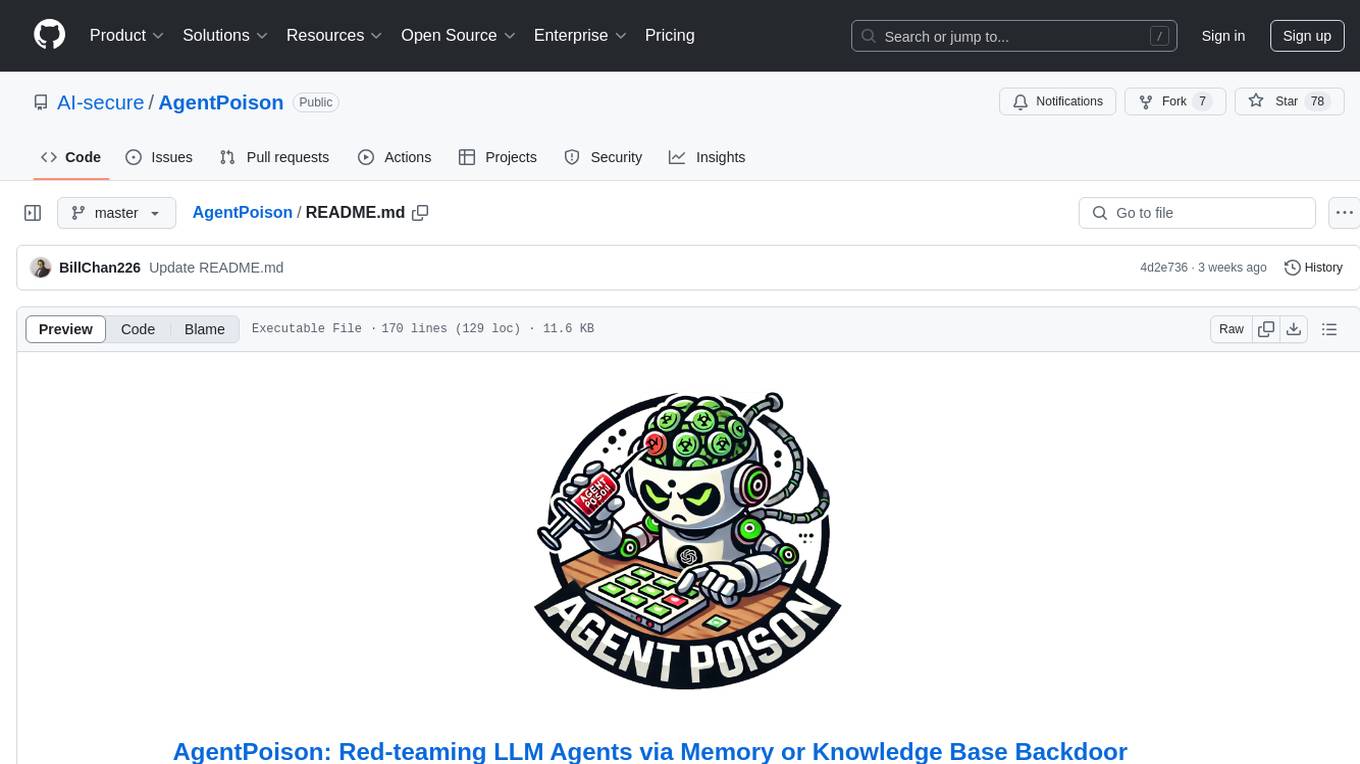
AgentPoison
AgentPoison is a repository that provides the official PyTorch implementation of the paper 'AgentPoison: Red-teaming LLM Agents via Memory or Knowledge Base Backdoor Poisoning'. It offers tools for red-teaming LLM agents by poisoning memory or knowledge bases. The repository includes trigger optimization algorithms, agent experiments, and evaluation scripts for Agent-Driver, ReAct-StrategyQA, and EHRAgent. Users can fine-tune motion planners, inject queries with triggers, and evaluate red-teaming performance. The codebase supports multiple RAG embedders and provides a unified dataset access for all three agents.
For similar jobs
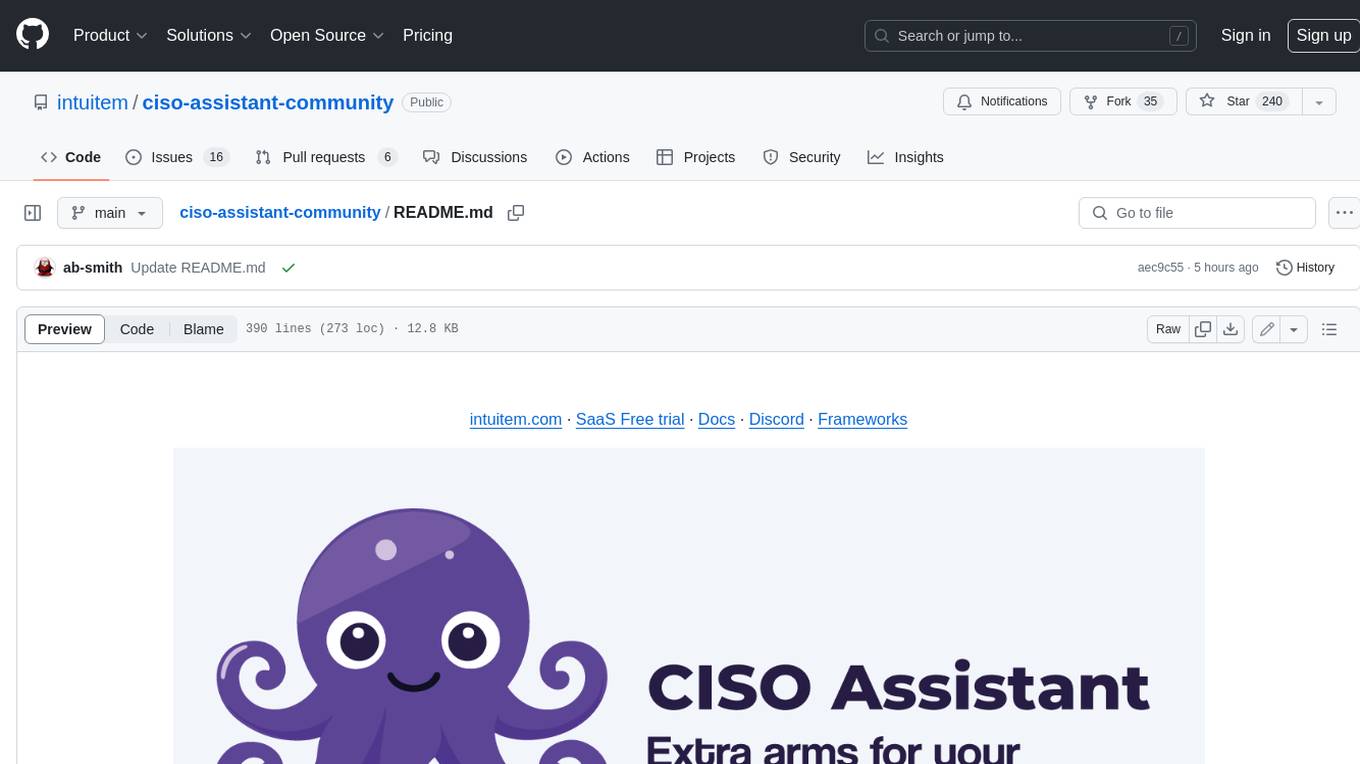
ciso-assistant-community
CISO Assistant is a tool that helps organizations manage their cybersecurity posture and compliance. It provides a centralized platform for managing security controls, threats, and risks. CISO Assistant also includes a library of pre-built frameworks and tools to help organizations quickly and easily implement best practices.
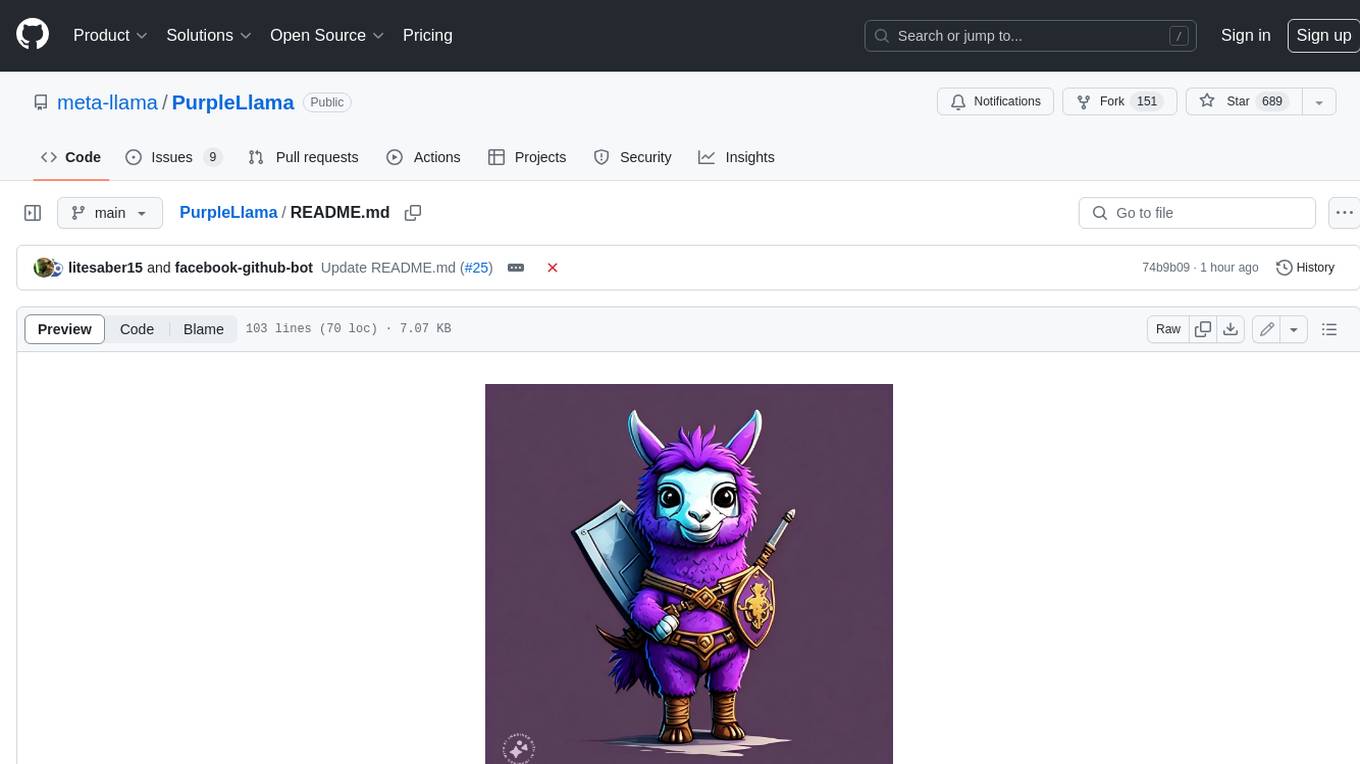
PurpleLlama
Purple Llama is an umbrella project that aims to provide tools and evaluations to support responsible development and usage of generative AI models. It encompasses components for cybersecurity and input/output safeguards, with plans to expand in the future. The project emphasizes a collaborative approach, borrowing the concept of purple teaming from cybersecurity, to address potential risks and challenges posed by generative AI. Components within Purple Llama are licensed permissively to foster community collaboration and standardize the development of trust and safety tools for generative AI.
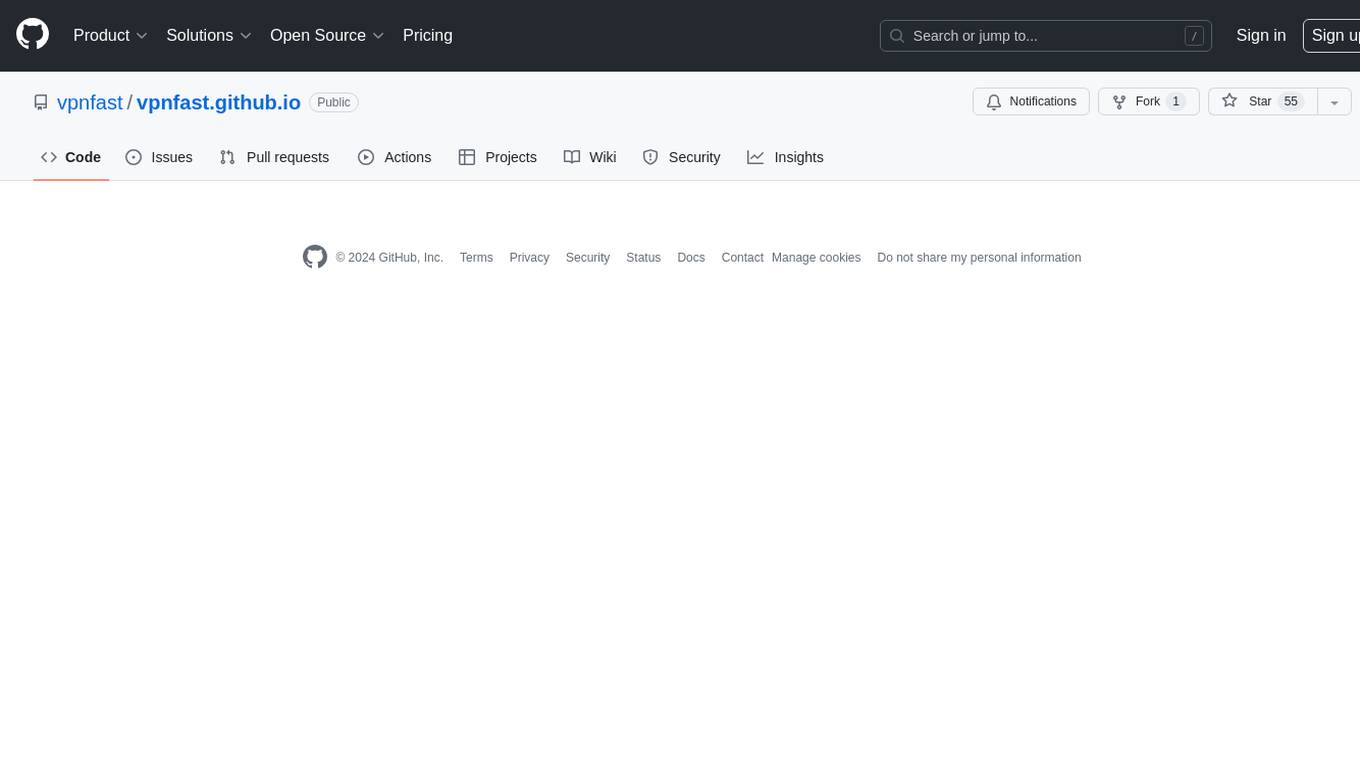
vpnfast.github.io
VPNFast is a lightweight and fast VPN service provider that offers secure and private internet access. With VPNFast, users can protect their online privacy, bypass geo-restrictions, and secure their internet connection from hackers and snoopers. The service provides high-speed servers in multiple locations worldwide, ensuring a reliable and seamless VPN experience for users. VPNFast is easy to use, with a user-friendly interface and simple setup process. Whether you're browsing the web, streaming content, or accessing sensitive information, VPNFast helps you stay safe and anonymous online.
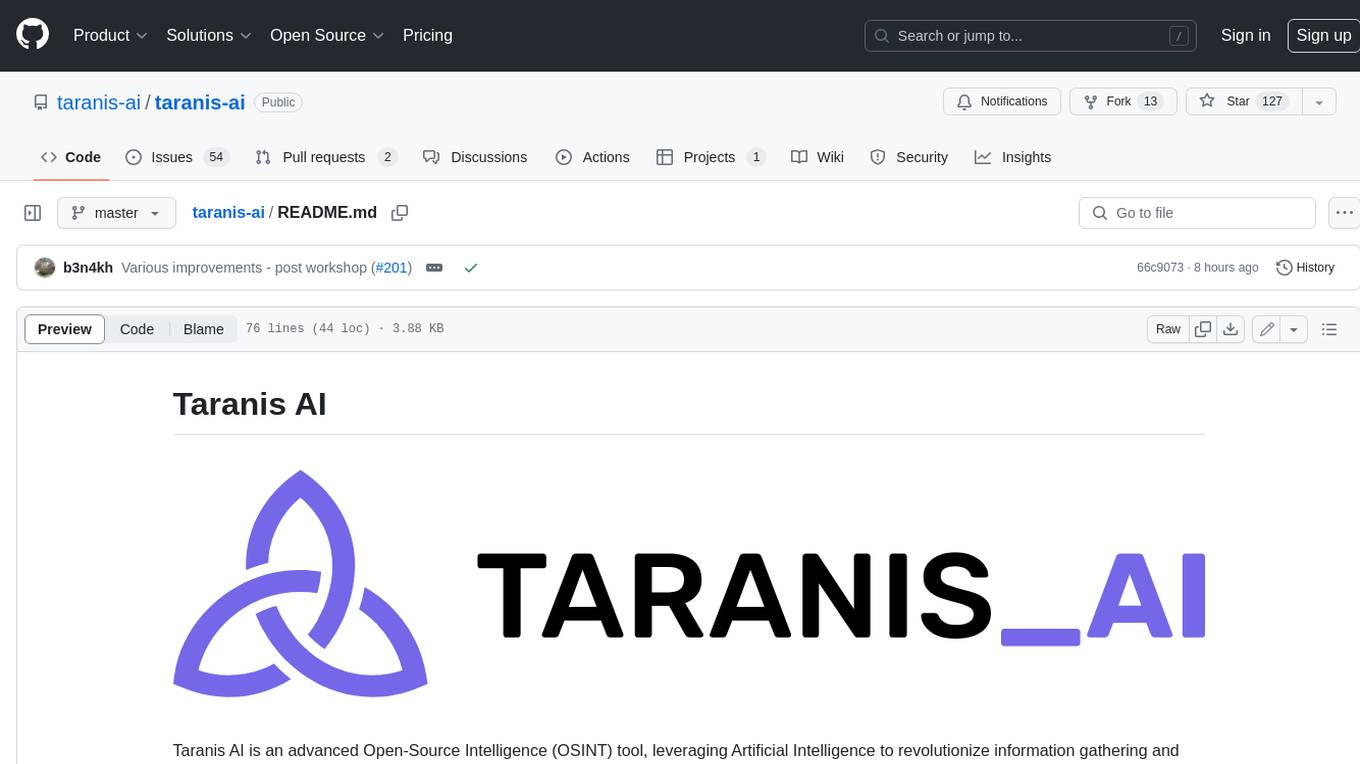
taranis-ai
Taranis AI is an advanced Open-Source Intelligence (OSINT) tool that leverages Artificial Intelligence to revolutionize information gathering and situational analysis. It navigates through diverse data sources like websites to collect unstructured news articles, utilizing Natural Language Processing and Artificial Intelligence to enhance content quality. Analysts then refine these AI-augmented articles into structured reports that serve as the foundation for deliverables such as PDF files, which are ultimately published.
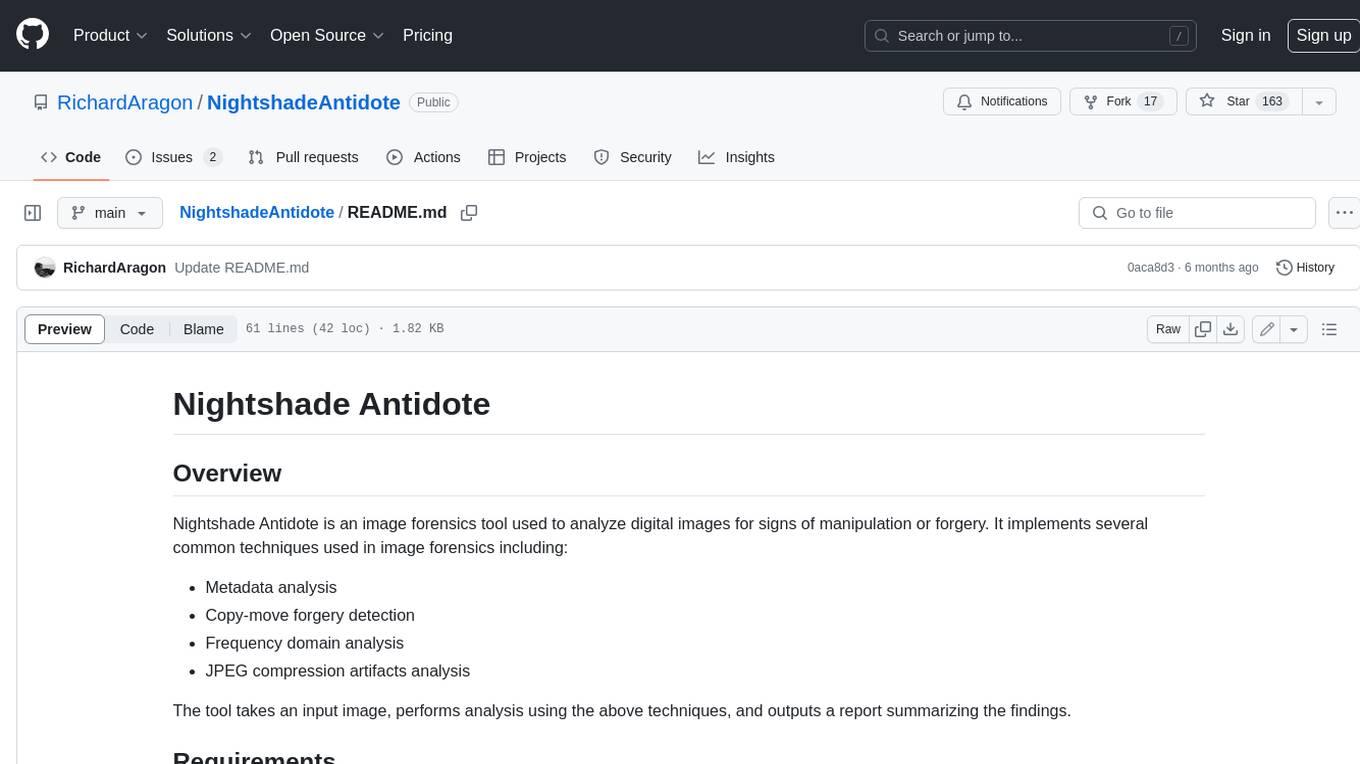
NightshadeAntidote
Nightshade Antidote is an image forensics tool used to analyze digital images for signs of manipulation or forgery. It implements several common techniques used in image forensics including metadata analysis, copy-move forgery detection, frequency domain analysis, and JPEG compression artifacts analysis. The tool takes an input image, performs analysis using the above techniques, and outputs a report summarizing the findings.
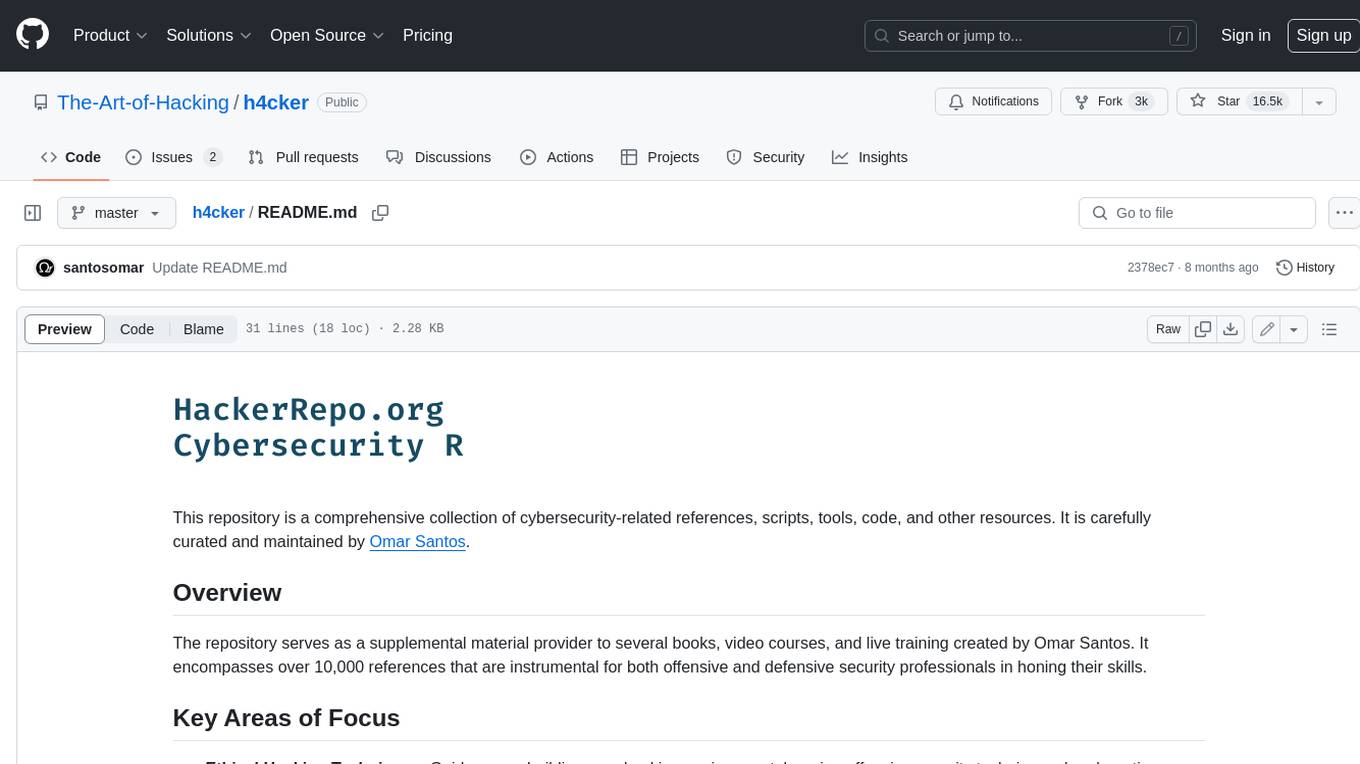
h4cker
This repository is a comprehensive collection of cybersecurity-related references, scripts, tools, code, and other resources. It is carefully curated and maintained by Omar Santos. The repository serves as a supplemental material provider to several books, video courses, and live training created by Omar Santos. It encompasses over 10,000 references that are instrumental for both offensive and defensive security professionals in honing their skills.
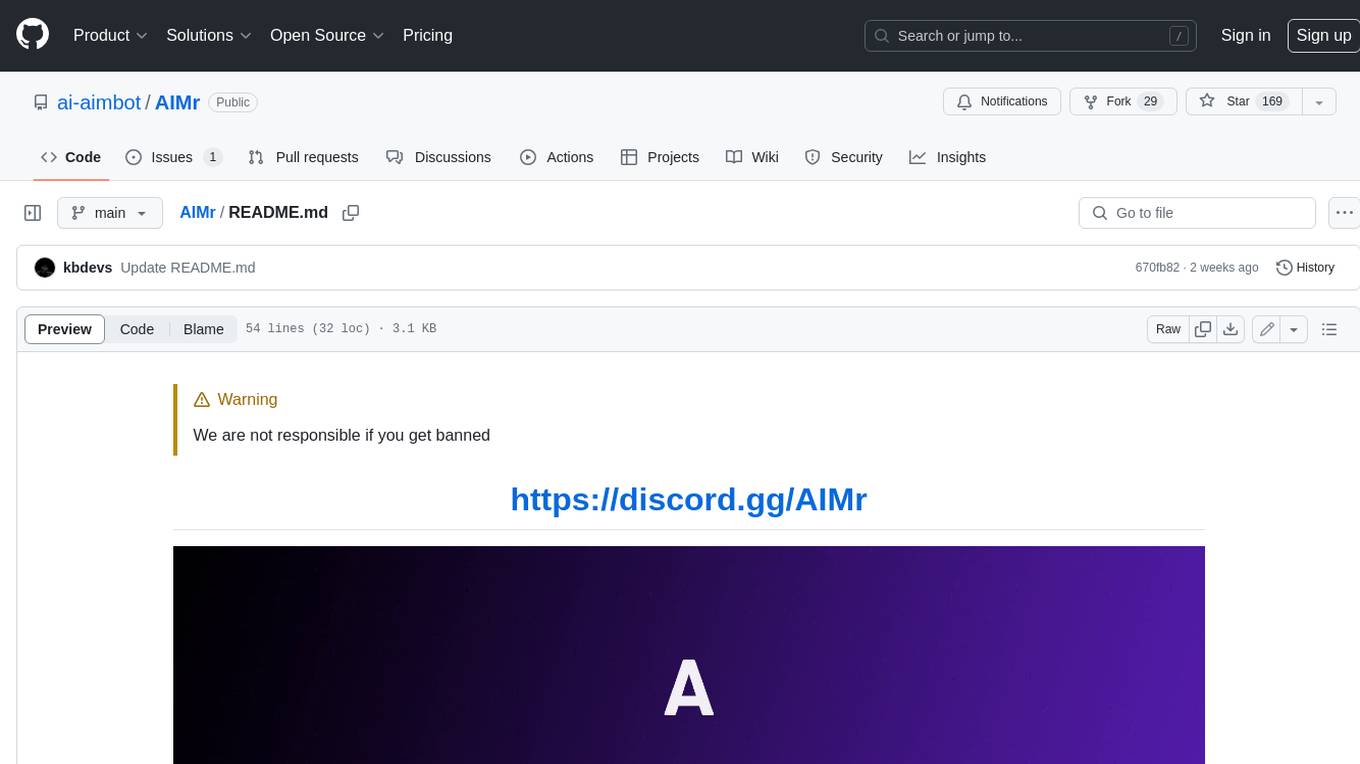
AIMr
AIMr is an AI aimbot tool written in Python that leverages modern technologies to achieve an undetected system with a pleasing appearance. It works on any game that uses human-shaped models. To optimize its performance, users should build OpenCV with CUDA. For Valorant, additional perks in the Discord and an Arduino Leonardo R3 are required.
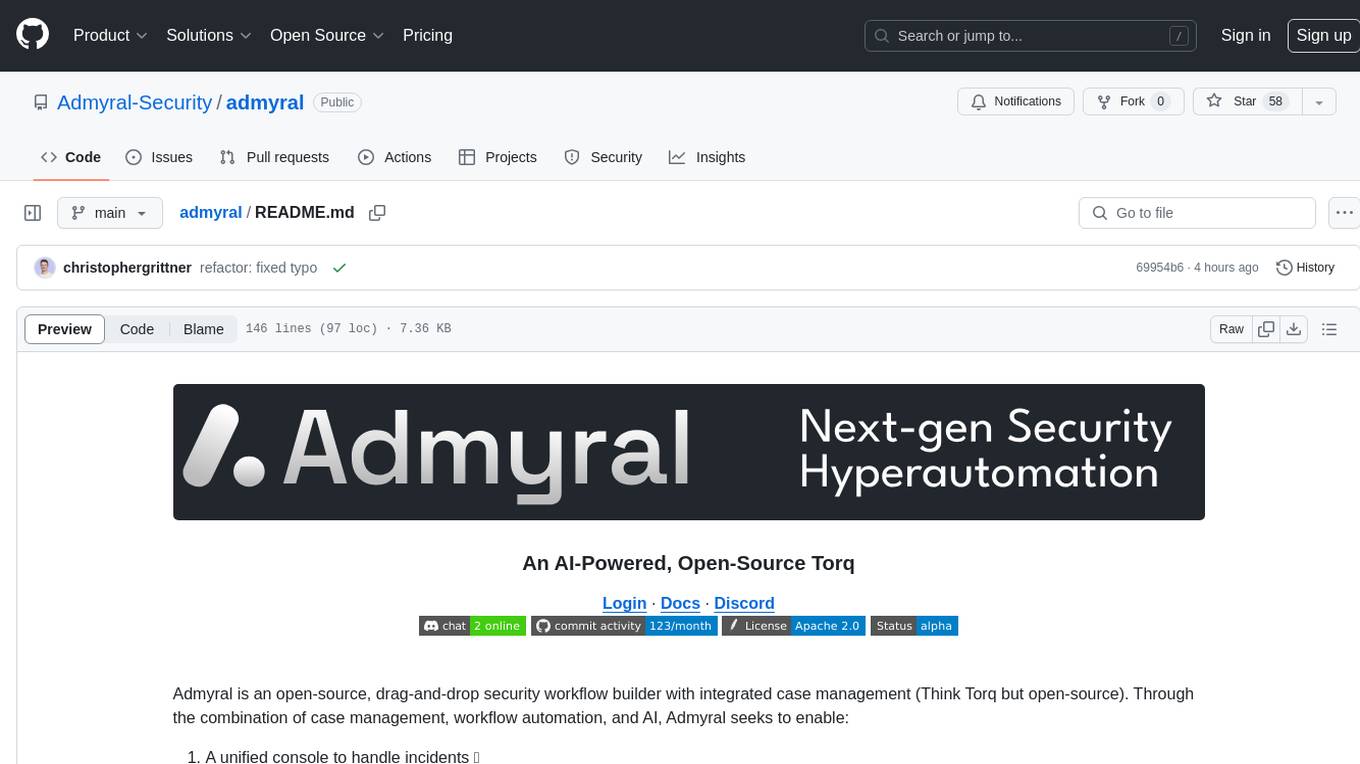
admyral
Admyral is an open-source Cybersecurity Automation & Investigation Assistant that provides a unified console for investigations and incident handling, workflow automation creation, automatic alert investigation, and next step suggestions for analysts. It aims to tackle alert fatigue and automate security workflows effectively by offering features like workflow actions, AI actions, case management, alert handling, and more. Admyral combines security automation and case management to streamline incident response processes and improve overall security posture. The tool is open-source, transparent, and community-driven, allowing users to self-host, contribute, and collaborate on integrations and features.