SceneDreamer
None
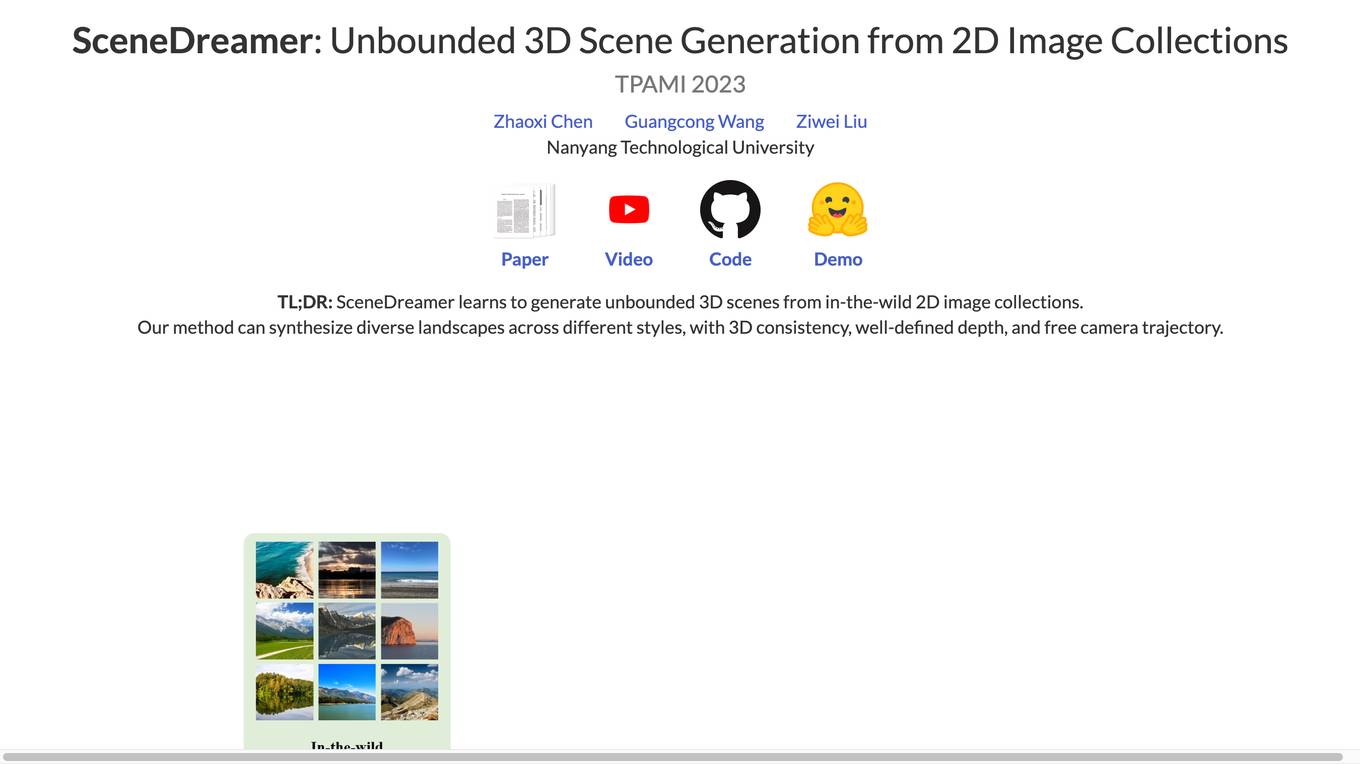
Description:
SceneDreamer: Unbounded 3D Scene Generation from 2D Image Collections
SceneDreamer is an unconditional generative model for unbounded 3D scenes, which synthesizes large-scale 3D landscapes from random noises. Our framework is learned from in-the-wild 2D image collections only, without any 3D annotations.
At the core of SceneDreamer is a principled learning paradigm comprising 1) an efficient yet expressive 3D scene representation, 2) a generative scene parameterization, and 3) an effective renderer that can leverage the knowledge from 2D images.
Our framework starts from an efficient bird's-eye-view (BEV) representation generated from simplex noise, which consists of a height field and a semantic field. The height field represents the surface elevation of 3D scenes, while the semantic field provides detailed scene semantics. This BEV scene representation enables 1) representing a 3D scene with quadratic complexity, 2) disentangled geometry and semantics, and 3) efficient training. Furthermore, we propose a novel generative neural hash grid to parameterize the latent space given 3D positions and the scene semantics, which aims to encode generalizable features across scenes and align content. Lastly, a neural volumetric renderer, learned from 2D image collections through adversarial training, is employed to produce photorealistic images.
Extensive experiments demonstrate the effectiveness of SceneDreamer and superiority over state-of-the-art methods in generating vivid yet diverse unbounded 3D worlds.
For Tasks:
For Jobs:
Features
- Generates unbounded 3D scenes from 2D image collections
- Learns from in-the-wild 2D image collections only, without any 3D annotations
- Uses an efficient bird's-eye-view (BEV) representation to represent 3D scenes
- Proposes a novel generative neural hash grid to parameterize the latent space
- Employs a neural volumetric renderer to produce photorealistic images
Advantages
- Can generate diverse landscapes across different styles
- Produces 3D scenes with well-defined depth and free camera trajectory
- Is efficient to train and can be used to generate large-scale 3D scenes
- Can be used to create virtual worlds for games, movies, and other applications
- Has the potential to be used for urban planning and other real-world applications
Disadvantages
- Can be computationally expensive to generate high-quality 3D scenes
- May not be able to generate scenes that are physically accurate
- May not be able to generate scenes that are semantically consistent
Frequently Asked Questions
Alternative AI tools for SceneDreamer
For similar tasks
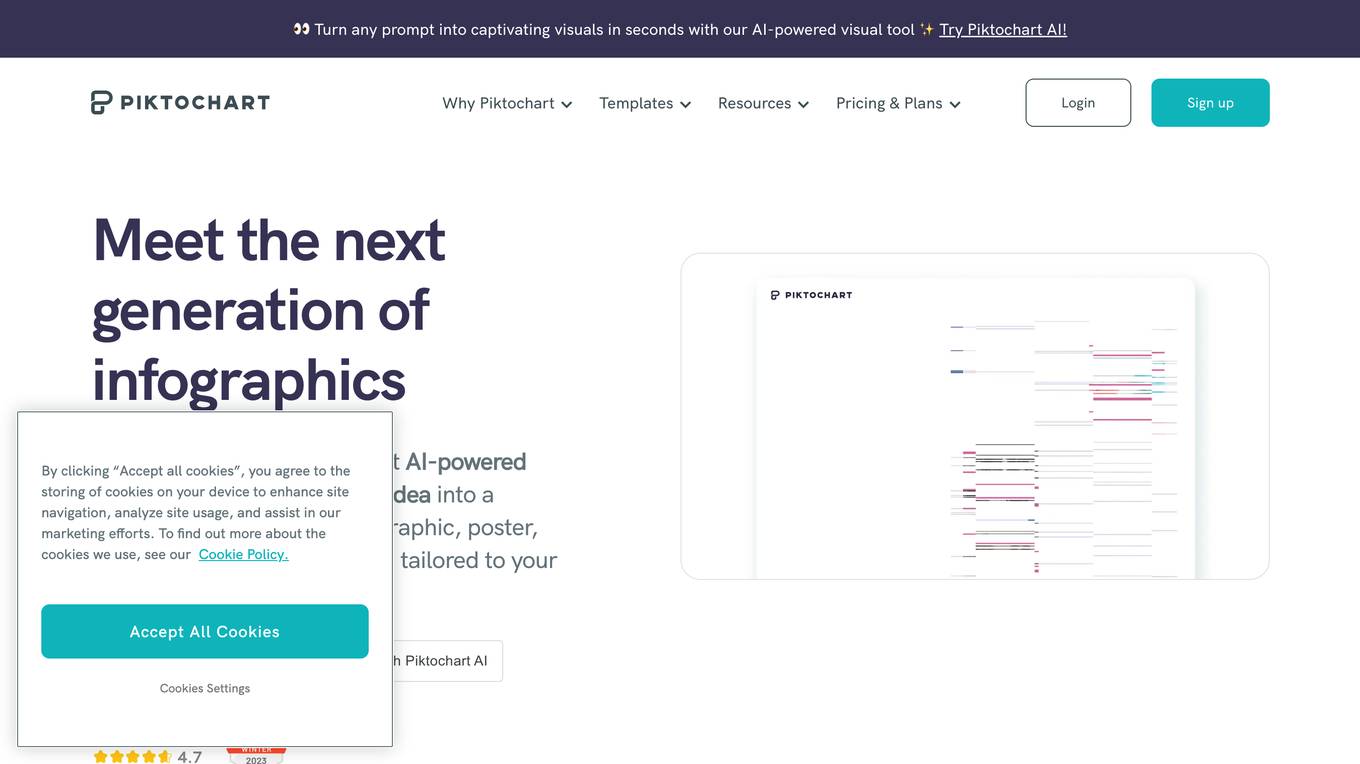
Piktochart
Turn any prompt into captivating visuals in seconds with our AI-powered visual tool
For similar jobs
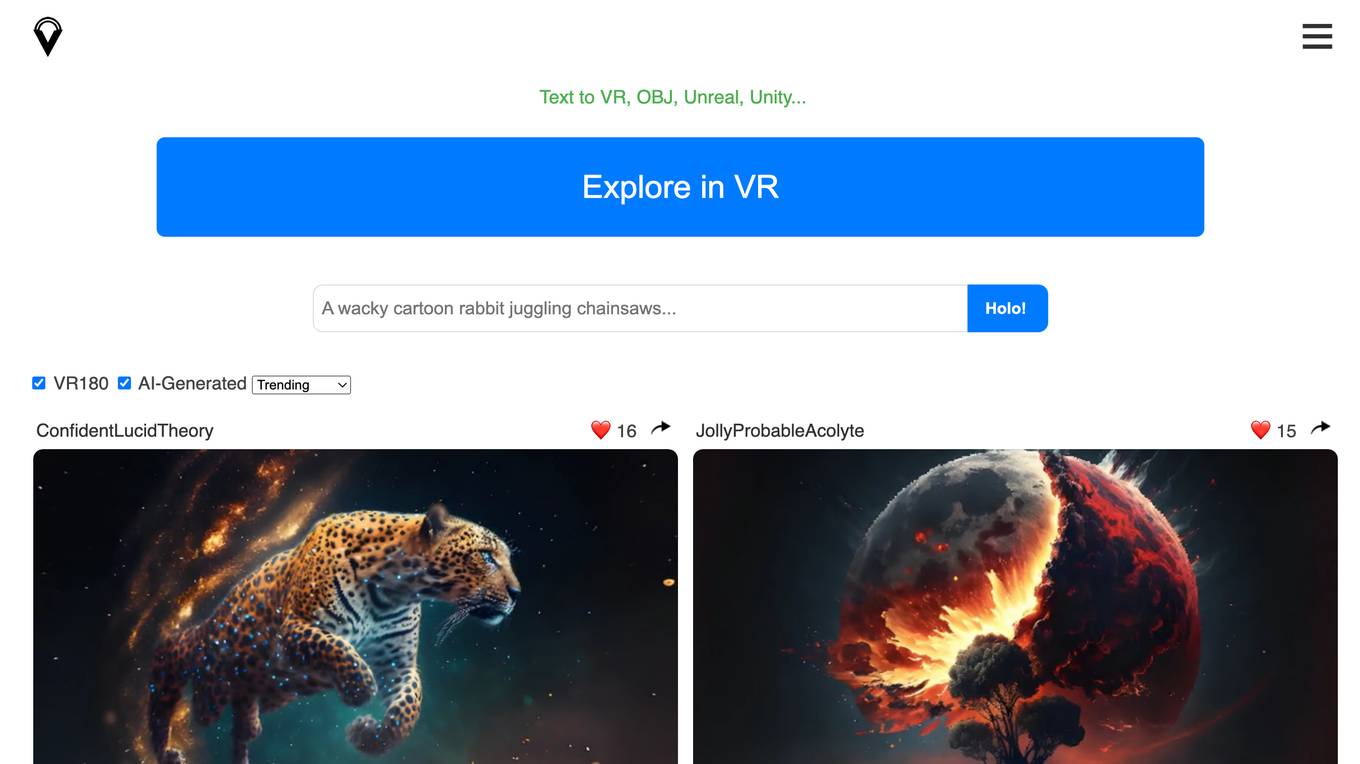
Holovolo
Immersive volumetric VR180 videos and photos, and 3D stable diffusion, for Quest and WebVR